Qualitative Methods in Health Care Research
Affiliations.
- 1 School of Nursing and Midwifery, Royal College of Surgeons Ireland - Bahrain (RCSI Bahrain), Al Sayh Muharraq Governorate, Bahrain.
- 2 Department of Mental Health Nursing, Manipal College of Nursing Manipal, Manipal Academy of Higher Education, Manipal, Karnataka, India.
- 3 Department of OBG Nursing, Manipal College of Nursing Manipal, Manipal Academy of Higher Education, Manipal, Karnataka, India.
- 4 School of Nursing, MGH Institute of Health Professions, Boston, USA.
- 5 Department of Child Health Nursing, Manipal College of Nursing Manipal, Manipal Academy of Higher Education, Manipal, Karnataka, India.
- PMID: 34084317
- PMCID: PMC8106287
- DOI: 10.4103/ijpvm.IJPVM_321_19
Healthcare research is a systematic inquiry intended to generate robust evidence about important issues in the fields of medicine and healthcare. Qualitative research has ample possibilities within the arena of healthcare research. This article aims to inform healthcare professionals regarding qualitative research, its significance, and applicability in the field of healthcare. A wide variety of phenomena that cannot be explained using the quantitative approach can be explored and conveyed using a qualitative method. The major types of qualitative research designs are narrative research, phenomenological research, grounded theory research, ethnographic research, historical research, and case study research. The greatest strength of the qualitative research approach lies in the richness and depth of the healthcare exploration and description it makes. In health research, these methods are considered as the most humanistic and person-centered way of discovering and uncovering thoughts and actions of human beings.
Keywords: Ethnography; grounded theory; qualitative research; research design.
Copyright: © 2021 International Journal of Preventive Medicine.
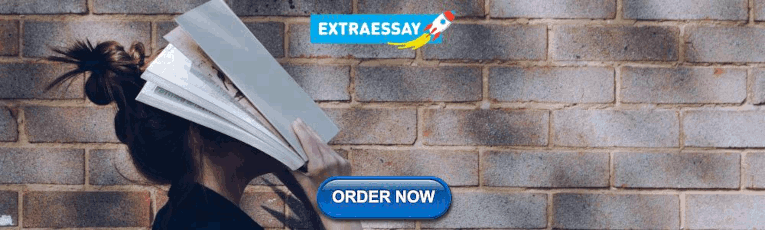
Publication types
- Open access
- Published: 13 December 2018
Using qualitative Health Research methods to improve patient and public involvement and engagement in research
- Danielle E. Rolfe 1 ,
- Vivian R. Ramsden 2 ,
- Davina Banner 3 &
- Ian D. Graham 1
Research Involvement and Engagement volume 4 , Article number: 49 ( 2018 ) Cite this article
43k Accesses
58 Citations
23 Altmetric
Metrics details
Plain English summary
Patient engagement (or patient and public involvement) in health research is becoming a requirement for many health research funders, yet many researchers have little or no experience in engaging patients as partners as opposed to research subjects. Additionally, many patients have no experience providing input on the research design or acting as a decision-making partner on a research team. Several potential risks exist when patient engagement is done poorly, despite best intentions. Some of these risks are that: (1) patients’ involvement is merely tokenism (patients are involved but their suggestions have little influence on how research is conducted); (2) engaged patients do not represent the diversity of people affected by the research; and, (3) research outcomes lack relevance to patients’ lives and experiences.
Qualitative health research (the collection and systematic analysis of non-quantitative data about peoples’ experiences of health or illness and the healthcare system) offers several approaches that can help to mitigate these risks. Several qualitative health research methods, when done well, can help research teams to: (1) accurately incorporate patients’ perspectives and experiences into the design and conduct of research; (2) engage diverse patient perspectives; and, (3) treat patients as equal and ongoing partners on the research team.
This commentary presents several established qualitative health research methods that are relevant to patient engagement in research. The hope is that this paper will inspire readers to seek more information about qualitative health research, and consider how its established methods may help improve the quality and ethical conduct of patient engagement for health research.
Research funders in several countries have posited a new vision for research that involves patients and the public as co-applicants for the funding, and as collaborative partners in decision-making at various stages and/or throughout the research process. Patient engagement (or patient and public involvement) in health research is presented as a more democratic approach that leads to research that is relevant to the lives of the people affected by its outcomes. What is missing from the recent proliferation of resources and publications detailing the practical aspects of patient engagement is a recognition of how existing research methods can inform patient engagement initiatives. Qualitative health research, for example, has established methods of collecting and analyzing non-quantitative data about individuals’ and communities’ lived experiences with health, illness and/or the healthcare system. Included in the paradigm of qualitative health research is participatory health research, which offers approaches to partnering with individuals and communities to design and conduct research that addresses their needs and priorities.
The purpose of this commentary is to explore how qualitative health research methods can inform and support meaningful engagement with patients as partners. Specifically, this paper addresses issues of: rigour (how can patient engagement in research be done well?); representation (are the right patients being engaged?); and, reflexivity (is engagement being done in ways that are meaningful, ethical and equitable?). Various qualitative research methods are presented to increase the rigour found within patient engagement. Approaches to engage more diverse patient perspectives are presented to improve representation beyond the common practice of engaging only one or two patients. Reflexivity, or the practice of identifying and articulating how research processes and outcomes are constructed by the respective personal and professional experiences of researchers and patients, is presented to support the development of authentic, sustainable, equitable and meaningful engagement of patients as partners in health research.
Conclusions
Researchers will need to engage patients as stakeholders in order to satisfy the overlapping mandate in health policy, care and research for engaging patients as partners in decision-making. This paper presents several suggestions to ground patient engagement approaches in established research designs and methods.
Peer Review reports
Patient engagement (or patient and public involvement) in research involves partnering with ‘patients’ (a term more often used in Canada and the US, that is inclusive of individuals, caregivers, and/or members of the public) to facilitate research related to health or healthcare services. Rather than research subjects or participants, patients are engaged as partners in the research process. This partnership is intended to be meaningful and ongoing, from the outset of planning a research project, and/or at various stages throughout the research process. Engagement can include the involvement of patients in defining a research question, identifying appropriate outcomes and methods, collecting and interpreting data, and developing and delivering a knowledge translation strategy [ 1 ].
The concept of engaging non-researchers throughout the research process is not new to participatory health researchers, or integrated knowledge translation researchers, as the latter involves ongoing collaboration with clinicians, health planners and policy makers throughout the research process in order to generate new knowledge [ 2 , 3 ]. Patients, however, are less frequently included as partners on health research teams, or as knowledge users in integrated knowledge translation research teams compared to clinicians, healthcare managers and policy-makers, as these individuals are perceived as having “the authority to invoke change in the practice or policy setting.” (p.2) [ 2 ] Recent requirements for patient engagement by health research funders [ 4 , 5 , 6 ], ,and mandates by most healthcare planners and organizations to engage patients in healthcare improvement initiatives, suggest that it would be prudent for integrated knowledge translation (and indeed all) health researchers to begin engaging patients as knowledge users in many, if not all, of their research projects.
Training and tools for patient engagement are being developed and implemented in Canada via the Canadian Institutes for Health Research (CIHR) Strategy for Patient Oriented Research (SPOR) initiative, in the US via Patient Centered Outcomes Research Institute (PCORI), and very practical resources are already available from the UK’s more established INVOLVE Advisory Group [ 5 , 6 , 7 ]. What is seldom provided by these ‘get started’ guides, however, are rigorous methods and evidence-based approaches to engaging diverse patient perspectives, and ensuring that their experiences, values and advice are appropriately incorporated into the research process.
The purpose of this commentary is to stimulate readers’ further discussion and inquiry into qualitative health research methods as a means of fostering the more meaningfully engagement of patients as partners for research. Specifically, this paper will address issues of: rigour (how do we know that the interpretation of patients’ perspectives has been done well and is applicable to other patients?); representation (are multiple and diverse patient perspectives being sought?); and, reflexivity (is engagement being done ethically and equitably?). This commentary alone is insufficient to guide researchers and patient partners to use the methods presented as part of their patient engagement efforts. However, with increased understanding of these approaches and perhaps guidance from experienced qualitative health researchers, integrated knowledge translation and health researchers alike may be better prepared to engage patients in a meaningful way in research that has the potential to improve health and healthcare experiences and outcomes.
What can be learned from methods utilized in qualitative health research?
There is wide variation in researchers’ and healthcare providers’ openness to engaging patients [ 8 ]. Often, the patients that are engaged are a select group of individuals known to the research team, sometimes do not reflect the target population of the research, are involved at a consultative rather than a partnership level, and are more likely to be involved in the planning rather than the dissemination of research [ 9 , 10 , 11 ]. As a result, patient engagement can be seen as tokenistic and the antithesis of the intention of most patient engagement initiatives, which is to have patients’ diverse experiences and perspectives help to shape what and how research is done. The principles, values, and practices of qualitative health research (e.g., relativism, social equity, inductive reasoning) have rich epistemological traditions that align with the conceptual and practical spirit of patient engagement. It is beyond the scope of this commentary, however, to describe in detail the qualitative research paradigm, and readers are encouraged to gain greater knowledge of this topic via relevant courses and texts. Nevertheless, several qualitative research considerations and methods can be applied to the practice of patient engagement, and the following sections describe three of these: rigour, representation and reflexivity.
Rigour: Interpreting and incorporating patients’ experiences into the design and conduct of research
When patient engagement strategies go beyond the inclusion of a few patient partners on the research team, for example, by using focus groups, interviews, community forums, or other methods of seeking input from a broad range of patient perspectives, the diversity of patients’ experiences or perspectives may be a challenge to quickly draw conclusions from in order to make decisions about the study design. To make these decisions, members of the research team (which should include patient partners) may discuss what they heard about patients’ perspectives and suggestions, and then unsystematically incorporate these suggestions, or they may take a vote, try to achieve consensus, implement a Delphi technique [ 12 ], or use another approach designed specifically for patient engagement like the James Lind Alliance technique for priority setting [ 13 ]. Although the information gathered from patients is not data (and indeed would require ethical review to be used as such), a number of qualitative research practices designed to increase rigour can be employed to help ensure that the interpretation and incorporation of patients’ experiences and perspectives has been done systematically and could be reproduced [ 14 ]. These practices include member checking , dense description , and constant comparative analysis . To borrow key descriptors of rigour from qualitative research, these techniques improve “credibility” (i.e., accurate representations of patients’ experiences and preferences that are likely to be understood or recognized by other patients in similar situations – known in quantitative research as internal validity), and “transferability” (or the ability to apply what was found among a group of engaged patients to other patients in similar contexts – known in quantitative research as external validity) [ 15 ].
Member checking
Member checking in qualitative research involves “taking ideas back to the research participants for their confirmation” (p. 111) [ 16 ]. The objective of member checking is to ensure that a researcher’s interpretation of the data (whether a single interview with a participant, or after analyzing several interviews with participants) accurately reflects the participants’ intended meaning (in the case of a member check with a single participant about their interview), or their lived experience (in the case of sharing an overall finding about several individuals with one or more participants) [ 16 ]. For research involving patient engagement, member checking can be utilized to follow-up with patients who may have been engaged at one or only a few time points, or on an on-going basis with patient partners. A summary of what was understood and what decisions were made based on patients’ recommendations could be used to initiate this discussion and followed up with questions such as, “have I understood correctly what you intended to communicate to me?” or “do you see yourself or your experience(s) reflected in these findings or suggestions for the design of the study?”
Dense description
As with quantitative research, detailed information about qualitative research methods and study participants is needed to enable other researchers to understand the context and focus of the research and to establish how these findings relate more broadly. This helps researchers to not only potentially repeat the study, but to extend its findings to similar participants in similar contexts. Dense description provides details of the social, demographic and health profile of participants (e.g., gender, education, health conditions, etc.), as well as the setting and context of their experiences (i.e., where they live, what access to healthcare they have). In this way, dense description improves the transferability of study findings to similar individuals in similar situations [ 15 ]. To date, most studies involving patient engagement provide limited details about their engagement processes and who was engaged [ 17 ]. This omission may be done intentionally (e.g., to protect the privacy of engaged patients, particularly those with stigmatizing health conditions), or as a practical constraint such as publication word limits. Nonetheless, reporting of patient engagement using some aspects of dense description of participants (as appropriate), the ways that they were engaged, and recommendations that emanated from engaged patients can also contribute to greater transferability and understanding of how patient engagement influenced the design of a research study.
Constant comparative analysis
Constant comparative analysis is a method commonly used in grounded theory qualitative research [ 18 ]. Put simply, the understanding of a phenomenon or experience that a researcher acquires through engaging with participants is constantly redeveloped and refined based on subsequent participant interactions. This process of adapting to new information in order to make it more relevant is similar to processes used in rapid cycle evaluation during implementation research [ 19 ]. This method can be usefully adapted and applied to research involving ongoing collaboration and partnership with several engaged patient partners, and/or engagement strategies that seek the perspectives of many patients at various points in the research process. For example, if, in addition to having ongoing patient partners, a larger group of patients provides input and advice (e.g., a steering or advisory committee) at different stages in the research process, their input may result in multiple course corrections during the design and conduct of the research processes to incorporate their suggestions. These suggestions may result in refinement of earlier decisions made about study design or conduct, and as such, the research process becomes more iterative rather than linear. In this way, engaged patients and patient partners are able to provide their input and experience to improve each step of the research process from formulating an appropriate research question or objective, determining best approaches to conducting the research and sharing it with those most affected by the outcomes.
Representation: Gathering diverse perspectives to design relevant and appropriate research studies
The intention of engaging patients is to have their lived experience of health care or a health condition contribute to the optimization of a research project design [ 20 ]. Development of a meaningful and sustainable relationship with patient partners requires considerable time, a demonstrated commitment to partnership by both the patient partners and the researcher(s), resources to facilitate patient partners’ engagement, and often, an individual designated to support the development of this relationship [ 17 , 21 ]. This may lead some research teams to sustain this relationship with only one or two patients who are often previously known to the research team [ 17 ]. The limitation of this approach is that the experiences of these one or two individuals may not adequately reflect the diverse perspectives of patients that may be affected by the research or its outcomes. The notion of gaining ‘ the patient perspective’ from a single or only a few individuals has already been problematized [ 22 , 23 ]. To be sure, the engagement of a single patient is better than none at all, but the engagement of a broader and diverse population of patients should be considered to better inform the research design, and to help prevent further perpetuation of health disparities. Key issues to be considered include (1) how engagement can be made accessible to patients from diverse backgrounds, and (2) which engagement strategies (e.g., ranging from a community information forum to full partnership on the research team) are most appropriate to reach the target population [ 24 ].
Making engagement accessible
Expecting patient partner(s) to attend regular research team meetings held during working hours in a boardroom setting in a hospital, research institute or university limits the participation of many individuals. To support the participation and diversity of engaged patients, effort should be made to increase the accessibility and emotional safety of engagement initiatives [ 25 ]. A budget must be allocated for patient partners’ transportation, childcare or caregiving support, remuneration for time or time taken off work and, at the very least, covering expenses related to their engagement. Another consideration that is often made by qualitative health researchers is whether brief counselling support can be provided to patients should the sharing of their experiences result in emotional distress. There are some resources that can help with planning for costs [ 26 ], including an online cost calculator [ 27 ].
Engagement strategies
Patient partners can be coached to consider the needs and experiences of people unlike them, but there are other methods of engagement that can help to gain a more fulsome perspective of what is likely a diverse patient population that is the focus of the research study. In qualitative health research, this is known as purposeful or purposive sampling: finding people who can provide information-rich descriptions of the phenomenon under study [ 28 ]. Engagement may require different approaches (e.g., deliberative group processes, community forums, focus groups, and patient partners on the research team), at different times in the research process to reach different individuals or populations (e.g., marginalized patients, or patients or caregivers experiencing illnesses that inhibit their ability to maintain an ongoing relationship with the research team). Engagement strategies of different forms at different times may be required. For example, ongoing engagement may occur with patient partners who are members of the research team (e.g., co-applicants on a research grant), and intermittent engagement may be sought from other patients through other methods that may be more time-limited or accessible to a diverse population of patients (e.g., a one-time focus group, community forum, or ongoing online discussion) to address issues that may arise during various stages of the research or dissemination processes. The result of this approach is that patients are not only consulted or involved (one-time or low commitment methods), but are also members of the research team and have the ability to help make decisions about the research being undertaken.
Engagement can generate a wealth of information from very diverse perspectives. Each iteration of engagement may yield new information. Knowing when enough information has been gathered to make decisions with the research team (that includes patient partners) about how the research may be designed or conducted can be challenging. One approach from qualitative research that can be adapted for patient engagement initiatives is theoretical saturation [ 29 ], or “the point in analysis when…further data gathering and analysis add little new to the conceptualization, though variations can always be discovered.” (p. 263) [ 18 ]. That is, a one-time engagement strategy (e.g., a discussion with a single patient partner) may be insufficient to acquire the diverse perspectives of the individuals that will be affected by the research or its outcomes. Additional strategies (e.g., focus groups or interviews with several individuals) may be initiated until many patients identify similar issues or recommendations.
Engagement approaches should also consider: how patients are initially engaged (e.g., through known or new networks, posted notices, telephone or in-person recruitment) and whether involvement has been offered widely enough to garner multiple perspectives; how patients’ experiences are shared (e.g., community forums, formal meetings, individual or group discussions) and whether facilitation enables broad participation; and finally, how patients’ participation and experiences are incorporated into the research planning and design, with patients having equal decision-making capacity to other research team members. Several publications and tools are available that can help guide researchers who are new to processes of engaging patients in research [ 24 , 30 , 31 , 32 , 33 , 34 ], but unfortunately few address how to evaluate the effectiveness of engagement [ 35 ].
Reflexivity: Ensuring meaningful and authentic engagement
In qualitative research, reflexivity is an ongoing process of “the researcher’s scrutiny of his or her research experience, decisions, and interpretations in ways that bring the researcher into the process and allow the reader to assess how and to what extent the researcher’s interests, positions, and assumptions influenced inquiry. A reflexive stance informs how the researcher conducts his or her research, relates to the research participants, and represents them in written reports,” (p.188–189) [ 16 ]. The concept of reflexivity can be applied to research involving patient engagement by continually and explicitly considering how decisions about the research study were made. All members of the research team must consider (and perhaps discuss): (1) how patient partners are invited to participate in research planning and decision-making; (2) how their input is received relative to other team members (i.e., do their suggestions garner the same respect as researchers’ or providers’?); and, (3) whether engaged patients or patient partners feel sufficiently safe, able and respected to share their experiences, preferences and recommendations with the research team.
Ideally, reflexivity becomes a practice within the research team and may be operationalized through regular check-ins with patients and researchers about their comfort in sharing their views, and whether they feel that their views have been considered and taken onboard. Power dynamics should also be considered during patient engagement initiatives. For example, reflecting on how community forums, focus groups or interviews are to be facilitated, including a consideration of who is at the table/who is not, who speaks/who does not, whose suggestions are implemented/whose are not? Reflexivity can be practiced through informal discussions, or using methods that may allow more candid responses by engaged patients (e.g., anonymous online survey or feedback forms). At the very least, if these practices were not conducted throughout the research process, the research team (including patient partners) should endeavor to reflect upon team dynamics and consider how these may have contributed to the research design or outcomes. For example, were physicians and researchers seen as experts and patients felt less welcome or able to share their personal experiences? Were patients only engaged by telephone rather than in-person and did this influence their ability to easily engage in decision-making? Reflexive practices may be usefully supplemented by formal evaluation of the process of patient engagement from the perspective of patients and other research team members [ 36 , 37 ], and some tools are available to do this [ 35 ].
A note about language
One way to address the team dynamic between researchers, professional knowledge users (such as clinicians or health policy planners) and patients is to consider the language used to engage with patients in the planning of patient engagement strategies. That is, the term ‘patient engagement’ is a construction of an individual’s identity that exists only within the healthcare setting, and in the context of a patient-provider dynamic. This term does not consider how people make decisions about their health and healthcare within a broader context of their family, community, and culture [ 22 , 38 ]. This may be why research communities in some countries (e.g., the United Kingdom) use the term ‘patient and public involvement’. Additionally, research that involves communities defined by geography, shared experiences, cultural or ethnic identity, as is the case with participatory health research, may refer to ‘community engagement.’ Regardless of the term used, partnerships with patients, the public, or with communities need to be conceived instead as person-to-person interactions between researchers and individuals who are most affected by the research. Discussions with engaged patients should be conducted early on to determine how to best describe their role on the team or during engagement initiatives (e.g., as patient partners, community members, or people with lived experience).
Tokenism is the “difference between…the empty ritual of participation and having the real power needed to affect the outcome,” (p.2) [ 39 ]. Ongoing reflection on the power dynamic between researchers and engaged patients, a central tenet of critical qualitative health research [ 40 , 41 ], can increase the likelihood that engagement involves equitable processes and will result in meaningful engagement experiences by patients rather than tokenism [ 36 , 42 ]. Patient engagement initiatives should strive for “partnership” amongst all team members, and not just reflect a patient-clinician or researcher-subject dynamic [ 43 ]. To develop meaningful, authentic and sustainable relationships with engaged patients, methods used for participatory, action or community-based research (approaches that fall under the paradigm of qualitative inquiry) provide detailed experiential guidance [ 44 ]. For example, a realist review of community-based participatory research projects reported that gaining and maintaining trust with patient or community partners, although time-intensive, is foundational to equitable and sustainable partnerships that benefit communities and individuals [ 45 , 46 ]. Additionally, Chapter Nine of the Canadian Tri-Council Policy Statement on Research involving Humans, which has to date been applied to research involving First Nations, Inuit and, Métis Peoples in Canada [ 47 ], provides useful information and direction that can be applied to working with patient partners on research [ 48 ].
Authentic patient engagement should include their involvement at all stages of the research process [ 49 , 50 ], but this is often not the case [ 10 ]. .Since patient partners are not research subjects or participants, their engagement does not (usually) require ethics approval, and they can be engaged as partners as early as during the submission of grant applications [ 49 ]. This early engagement helps to incorporate patients’ perspectives into the proposed research before the project is wedded to particular objectives, outcomes and methods, and can also serve to allocate needed resources to support patient engagement (including remuneration for patient partners’ time). Training in research for patient partners can also support their meaningful engagement by increasing their ability to fully engage in decision-making with other members of the research team [ 51 , 52 ]. Patient partners may also thrive in co-leading the dissemination of findings to healthcare providers, researchers, patients or communities most affected by the research [ 53 ].
Patient engagement has gained increasing popularity, but many research organizations are still at the early stages of developing approaches and methods, many of which are based on experience rather than evidence. As health researchers and members of the public will increasingly need to partner for research to satisfy the overlapping mandate of patient engagement in health policy, healthcare and research, the qualitative research methods highlighted in this commentary provide some suggestions to foster rigorous, meaningful and sustained engagement initiatives while addressing broader issues of power and representation. By incorporating evidence-based methods of gathering and learning from multiple and diverse patient perspectives, we will hopefully conduct better patient engaged research, live out the democratic ideals of patient engagement, and ultimately contribute to research that is more relevant to the lives of patients; as well as, contribute to the improved delivery of healthcare services. In addition to the references provided in this paper, readers are encouraged to learn more about the meaningful engagement of patients in research from several key texts [ 54 , 55 , 56 ].
Abbreviations
Canadian Institutes for Health Research
Patient Centered Outcomes Research Institute
Strategy for Patient Oriented Research
Canadian Institutes of Health Research. Strategy for Patient-Oriented Research. 2014. http://www.cihr-irsc.gc.ca/e/documents/spor_framework-en.pdf . Accessed 27 Sep 2017.
Google Scholar
Kothari A, Wathen CN. Integrated knowledge translation: digging deeper, moving forward. J Epidemiol Community Health. 2017;71:619–23. https://doi.org/10.1136/jech-2016-208490 .
Article PubMed Google Scholar
Gagliardi AR, Berta W, Kothari A, Boyko J, Urquhart R. Integrated knowledge translation (IKT) in health care: a scoping review. Implement Sci. 2016;11:38. https://doi.org/10.1186/s13012-016-0399-1 .
Article PubMed PubMed Central Google Scholar
Canadian Institutes of Health Research. Strategy for Patient-Oriented Research - CIHR. http://www.cihr-irsc.gc.ca/e/41204.html . Accessed 20 Sep 2017.
Patient-Centered Outcomes Research Institute. Patient-Centered Outcomes Research Institute website. https://www.pcori.org/ . Accessed 20 Sep 2017.
National Institute for Health Research. INVOLVE | INVOLVE Supporting public involvement in NHS, public health and social care research. http://www.invo.org.uk/ . Accessed 27 Sep 2017.
Canadian Institutes of Health Research. SPOR SUPPORT Units - CIHR. http://www.cihr-irsc.gc.ca/e/45859.html . Accessed 27 Sep 2017.
Ocloo J, Matthews R. From tokenism to empowerment: progressing patient and public involvement in healthcare improvement. BMJ Qual Saf. 2016;25:626–32.
Hearld KR, Hearld LR, Hall AG. Engaging patients as partners in research: factors associated with awareness, interest, and engagement as research partners. SAGE open Med. 2017;5:2050312116686709. https://doi.org/10.1177/2050312116686709.
Domecq JP, Prutsky G, Elraiyah T, Wang Z, Nabhan M, Shippee N, et al. Patient engagement in research: a systematic review. BMC Health Serv Res. 2014;14:89. https://doi.org/10.1186/1472-6963-14-89 .
Concannon TW, Fuster M, Saunders T, Patel K, Wong JB, Leslie LK, et al. A systematic review of stakeholder engagement in comparative effectiveness and patient-centered outcomes research. J Gen Intern Med. 2014;29:1692–701.
Oostendorp LJM, Durand M-A, Lloyd A, Elwyn G. Measuring organisational readiness for patient engagement (MORE): an international online Delphi consensus study. BMC Health Serv Res. 2015;15:61. https://doi.org/10.1186/s12913-015-0717-3 .
Boddy K, Cowan K, Gibson A, Britten N. Does funded research reflect the priorities of people living with type 1 diabetes? A secondary analysis of research questions. BMJ Open. 2017;7:e016540. https://doi.org/10.1136/bmjopen-2017-016540 .
Mays N, Pope C. Rigour and qualitative research. BMJ Br Med J. 1995;311:109–12.
Article CAS Google Scholar
Krefting L. Rigor in qualitative research: the assessment of trustworthiness. Am J Occup Ther. 1991;45:214–22.
Article CAS PubMed Google Scholar
Charmaz K. Constructing grounded theory : a practical guide through qualitative analysis. London: Sage Publications; 2006.
Wilson P, Mathie E, Keenan J, McNeilly E, Goodman C, Howe A, et al. ReseArch with patient and public invOlvement: a RealisT evaluation – the RAPPORT study. Heal Serv Deliv Res. 2015;3:1–176. https://doi.org/10.3310/hsdr03380 .
Corbin JM, Strauss AL, Strauss AL. Basics of qualitative research : techniques and procedures for developing grounded theory. Thousand Oaks: Sage Publications; 2008.
Keith RE, Crosson JC, O’Malley AS, Cromp D, Taylor EF. Using the consolidated framework for implementation research (CFIR) to produce actionable findings: a rapid-cycle evaluation approach to improving implementation. Implement Sci. 2017;12:15. https://doi.org/10.1186/s13012-017-0550-7 .
Esmail L, Moore E. Evaluating patient and stakeholder engagement in research: moving from theory to practice. J Comp Eff Res. 2015;4:133–45. https://doi.org/10.2217/cer.14.79 .
Brett J, Staniszewska S, Mockford C, Herron-Marx S, Hughes J, Tysall C, et al. A systematic review of the impact of patient and public involvement on service users, researchers and communities. Patient. 2014;7:387–95.
Rowland P, McMillan S, McGillicuddy P, Richards J. What is “the patient perspective” in patient engagement programs? Implicit logics and parallels to feminist theories. Heal An Interdiscip J Soc Study Heal Illn Med. 2016. https://doi.org/10.1177/1363459316644494 .
Article Google Scholar
Martin GP. “Ordinary people only”: knowledge, representativeness, and the publics of public participation in healthcare. Sociol Health Illn. 2008;30:35–54. https://doi.org/10.1111/j.1467-9566.2007.01027.x .
Snow B, Tweedie K, Pederson A, Shrestha H, Bachmann L. Patient Engagement: Heard and valued. 2013. http://www.cfhi-fcass.ca/sf-docs/default-source/patient-engagement/awesome_workbook-fraserhealth.pdf . Accessed 24 Jan 2018.
Montesanti SR, Abelson J, Lavis JN, Dunn JR. Enabling the participation of marginalized populations: case studies from a health service organization in Ontario. Canada Health Promot Int. 2017;32(4):636–49. https://doi.org/10.1093/heapro/dav118.
The Change Foundation. Should Money Come into It? A Tool for Deciding Whether to Pay Patient- Engagement Participants. Toronto; 2015. http://www.changefoundation.ca/site/wp-content/uploads/2016/05/Should-money-come-into-it.pdf . Accessed 18 June 2018
INVOLVE. Involvement Cost Calculator | INVOLVE. http://www.invo.org.uk/resource-centre/payment-and-recognition-for-public-involvement/involvement-cost-calculator/ . Accessed 11 Oct 2017.
Patton MQ. Qualitative research and evaluation methods. 3rd ed. Thousand Oaks: Sage Publications; 2002.
Kerr C, Nixon A, Wild D. Assessing and demonstrating data saturation in qualitative inquiry supporting patient-reported outcomes research. Expert Rev Pharmacoecon Outcomes Res. 2010;10(3):269–81. https://doi.org/10.1586/erp.10.30 .
Canadian Institutes of Health Research. CIHR’S Citizen Engagement Handbook. http://www.cihr-irsc.gc.ca/e/documents/ce_handbook_e.pdf . Accessed 18 June 2018.
Elliott J, Heesterbeek S, Lukensmeyer CJ, Slocum N. Participatory and deliberative methods toolkit how to connect with citizens: a practitioner’s manual. King Baudouin Foundation; 2005. https://www.kbs-frb.be/en/Virtual-Library/2006/294864 . Accessed 28 Sep 2017.
Robbins M, Tufte J, Hsu C. Learning to “swim” with the experts: experiences of two patient co-investigators for a project funded by the Patient-Centered Outcomes Research Institute. Perm J. 2016;20:85–8.
PubMed PubMed Central Google Scholar
Gauvin F-P, Abelson J, Lavis JN. Strengthening public and patient engagement in health technology assessment in Ontario. 2014. https://www.mcmasterforum.org/docs/default-source/product-documents/evidence-briefs/public-engagement-in-health-technology-assessement-in-ontario-eb.pdf?sfvrsn=2 . Accessed 18 June 2018.
Abelson J, Montesanti S, Li K, Gauvin F-P, Martin E. Effective strategies for interactive public engagement in the development of healthcare policies and Programs. 2010; https://www.cfhi-fcass.ca/sf-docs/default-source/commissionedresearch-reports/Abelson_EN_FINAL.pdf?sfvrsn=0. . Accessed 16 Nov 2018.
Abelson J. Public and patient engagement evaluation tool (version 1.0), vol. 0; 2015. https://www.fhs.mcmaster.ca/publicandpatientengagement/ppeet_request_form.html ! Accessed 18 June 2018
Johnson DS, Bush MT, Brandzel S, Wernli KJ. The patient voice in research—evolution of a role. Res Involv Engagem. 2016;2:6. https://doi.org/10.1186/s40900-016-0020-4 .
Crocker JC, Boylan AM, Bostock J, Locock L. Is it worth it? Patient and public views on the impact of their involvement in health research and its assessment: a UK-based qualitative interview study. Health Expect. 2017;20(3):519–28. https://doi.org/10.1111/hex.12479 .
Renedo A, Marston C. Healthcare professionals’ representations of “patient and public involvement” and creation of “public participant” identities: implications for the development of inclusive and bottom-up community participation initiatives. J Community Appl Soc Psychol. 2011;21:268–80. https://doi.org/10.1002/casp.1092 .
Hahn DL, Hoffmann AE, Felzien M, LeMaster JW, Xu J, Fagnan LJ. Tokenism in patient engagement. Fam Pract. 2017;34(3):290–5. https://doi.org/10.1093/fampra/cmw097.
Hart C, Poole JM, Facey ME, Parsons JA. Holding firm: power, push-Back, and opportunities in navigating the liminal space of critical qualitative Health Research. Qual Health Res. 2017;27:1765–74. https://doi.org/10.1177/1049732317715631 .
Eakin JM. Educating critical qualitative health researchers in the land of the randomized controlled trial. Qual Inq. 2016;22:107–18. https://doi.org/10.1177/1077800415617207 .
Safo S, Cunningham C, Beckman A, Haughton L, Starrels JL. “A place at the table:” a qualitative analysis of community board members’ experiences with academic HIV/AIDS research. BMC Med Res Methodol. 2016;16:80. https://doi.org/10.1186/s12874-016-0181-8 .
Thompson J, Bissell P, Cooper C, Armitage CJ, Barber R, Walker S, et al. Credibility and the “professionalized” lay expert: reflections on the dilemmas and opportunities of public involvement in health research. Heal Interdiscip J Soc Study Heal Illn Med. 2012;16:602–18. https://doi.org/10.1177/1363459312441008 .
Burke JG, Jones J, Yonas M, Guizzetti L, Virata MC, Costlow M, et al. PCOR, CER, and CBPR: alphabet soup or complementary fields of Health Research? Clin Transl Sci. 2013;6:493–6. https://doi.org/10.1111/cts.12064 .
Jagosh J, Bush PL, Salsberg J, Macaulay AC, Greenhalgh T, Wong G, et al. A realist evaluation of community-based participatory research: partnership synergy, trust building and related ripple effects. BMC Public Health. 2015;15:725. https://doi.org/10.1186/s12889-015-1949-1 .
Jagosh J, Macaulay AC, Pluye P, Salsberg J, Bush PL, Henderson J, et al. Uncovering the benefits of participatory research: implications of a realist review for health research and practice. Milbank Q. 2012;90:311–46. https://doi.org/10.1111/j.1468-0009.2012.00665.x .
Government of Canada IAP on RE. TCPS 2 - Chapter 9 Research Involving the First Nations, Inuit and Métis Peoples of Canada. http://www.pre.ethics.gc.ca/eng/policy-politique/initiatives/tcps2-eptc2/chapter9-chapitre9/ . Accessed 16 Oct 2017.
Ramsden VR, Crowe J, Rabbitskin N, Rolfe D, Macaulay A. Authentic engagement, co-creation and action research. In: Goodyear-smith F, Mash B, editors. How to do primary care research. Boca Raton: CRC press, medicine, Taylor & Francis Group; 2019.
Ramsden VR, Salsberg J, Herbert CP, Westfall JM, LeMaster J, Macaulay AC. Patient- and community-oriented research: how is authentic engagement identified in grant applications? Can Fam Physician. 2017;63:74–6.
Woolf SH, Zimmerman E, Haley A, Krist AH. Authentic engagement of patients and communities can transform research, practice, and policy. Health Aff. 2016;35:590–4.
Parkes JH, Pyer M, Wray P, Taylor J. Partners in projects: preparing for public involvement in health and social care research. Health Policy. 2014;117:399–408. https://doi.org/10.1016/j.healthpol.2014.04.014 .
Norman N, Bennett C, Cowart S, Felzien M, Flores M, Flores R, et al. Boot camp translation: a method for building a community of solution. J Am Board Fam Med. 2013;26:254–63. https://doi.org/10.3122/jabfm.2013.03.120253 .
Ramsden VR, Rabbitskin N, Westfall JM, Felzien M, Braden J, Sand J. Is knowledge translation without patient or community engagement flawed. Fam Pract. 2016:1–3.
Denzin NK, Lincoln YS. SAGE Handbook of qualitative research. 3rd ed. Thousand Oaks: Sage Publications; 2005.
Pearce V, Baraitser P, Smith G, Greenhalgh T. Experience-based co-design. In: User involvement in health care. Oxford: Wiley-Blackwell; 2010. p. 28–51. https://doi.org/10.1002/9781444325164.ch3 .
Chapter Google Scholar
Hulatt I, Lowes L. Involving service users in health and social care research: Routledge; 2005. https://doi.org/10.4324/9780203865651.
Book Google Scholar
Download references
Acknowledgements
This paper was drafted in response to a call for concept papers related to integrated knowledge translation issued by the Integrated Knowledge Translation Research Network (CIHR FDN #143237).
This paper was commissioned by the Integrated Knowledge Translation Network (IKTRN). The IKTRN brings together knowledge users and researchers to advance the science and practice of integrated knowledge translation and train the next generation of integrated knowledge translation researchers. Honorariums were provided for completed papers. The IKTRN is funded by a Canadian Institutes of Health Research Foundation Grant (FDN #143247).
Availability of data and materials
Not applicable.
Author information
Authors and affiliations.
School of Epidemiology and Public Health, University of Ottawa, 307D- 600 Peter Morand Crescent, Ottawa, ON, K1G 5Z3, Canada
Danielle E. Rolfe & Ian D. Graham
Department of Academic Family Medicine, University of Saskatchewan, West Winds Primary Health Centre, 3311 Fairlight Drive, Saskatoon, SK, S7M 3Y5, Canada
Vivian R. Ramsden
School of Nursing, University of Northern British Columbia, 3333 University Way, Prince George, BC, V2K4C6, Canada
Davina Banner
You can also search for this author in PubMed Google Scholar
Contributions
DR conceived and drafted this paper. All authors were involved in critiquing and revising the manuscript. All authors read and approved the final manuscript.
Corresponding author
Correspondence to Danielle E. Rolfe .
Ethics declarations
Ethics approval and consent to participate, consent for publication, competing interests.
The authors declare that they have no competing interests.
Publisher’s Note
Springer Nature remains neutral with regard to jurisdictional claims in published maps and institutional affiliations.
Rights and permissions
Open Access This article is distributed under the terms of the Creative Commons Attribution 4.0 International License ( http://creativecommons.org/licenses/by/4.0/ ), which permits unrestricted use, distribution, and reproduction in any medium, provided you give appropriate credit to the original author(s) and the source, provide a link to the Creative Commons license, and indicate if changes were made. The Creative Commons Public Domain Dedication waiver ( http://creativecommons.org/publicdomain/zero/1.0/ ) applies to the data made available in this article, unless otherwise stated.
Reprints and permissions
About this article
Cite this article.
Rolfe, D.E., Ramsden, V.R., Banner, D. et al. Using qualitative Health Research methods to improve patient and public involvement and engagement in research. Res Involv Engagem 4 , 49 (2018). https://doi.org/10.1186/s40900-018-0129-8
Download citation
Received : 18 June 2018
Accepted : 09 November 2018
Published : 13 December 2018
DOI : https://doi.org/10.1186/s40900-018-0129-8
Share this article
Anyone you share the following link with will be able to read this content:
Sorry, a shareable link is not currently available for this article.
Provided by the Springer Nature SharedIt content-sharing initiative
- Qualitative methods
- Qualitative health research
- Patient-oriented research
- Integrated knowledge translation
- Patient engagement
- Patient partners
- Patient and public involvement
Research Involvement and Engagement
ISSN: 2056-7529
- General enquiries: [email protected]
- - Google Chrome
Intended for healthcare professionals
- Access provided by Google Indexer
- My email alerts
- BMA member login
- Username * Password * Forgot your log in details? Need to activate BMA Member Log In Log in via OpenAthens Log in via your institution

Search form
- Advanced search
- Search responses
- Search blogs
- Methodological...
Methodological standards for qualitative and mixed methods patient centered outcomes research
- Related content
- Peer review
- Bridget Gaglio , senior program officer 1 ,
- Michelle Henton , program manager 1 ,
- Amanda Barbeau , program associate 1 ,
- Emily Evans , research health science specialist 2 ,
- David Hickam , director of clinical effectiveness and decision sciences 1 ,
- Robin Newhouse , dean 3 ,
- Susan Zickmund , research health scientist and professor 4 5
- 1 Patient-Centered Outcomes Research Institute, 1828 L Street, Suite 900, Washington, DC, 20036, USA
- 2 Veterans Health Administration, United States Department of Veterans Affairs, Washington, DC, USA
- 3 Indiana University School of Nursing, Indianapolis, IN, USA
- 4 United States Department of Veterans Affairs, Salt Lake City, UT, USA
- 5 University of Utah School of Medicine, Salt Lake City, UT, USA
- Correspondence to: B Gaglio bgaglio{at}pcori.org
- Accepted 20 October 2020
The Patient-Centered Outcomes Research Institute’s (PCORI) methodology standards for qualitative methods and mixed methods research help ensure that research studies are designed and conducted to generate the evidence needed to answer patients’ and clinicians’ questions about which methods work best, for whom, and under what circumstances. This set of standards focuses on factors pertinent to patient centered outcomes research, but it is also useful for providing guidance for other types of clinical research. The standards can be used to develop and evaluate proposals, conduct the research, and interpret findings. The standards were developed following a systematic process: survey the range of key methodological issues and potential standards, narrow inclusion to standards deemed most important, draft preliminary standards, solicit feedback from a content expert panel and the broader public, and use this feedback to develop final standards for review and adoption by PCORI’s board of governors. This article provides an example on how to apply the standards in the preparation of a research proposal.
Rigorous methodologies are critical for ensuring the trustworthiness of research results. This paper will describe the process for synthesizing the current literature providing guidance on the use of qualitative and mixed methods in health research; and the process for development of methodology standards for qualitative and mixed methods used in patient centered outcomes research. Patient centered outcomes research is comparative clinical effectiveness research that aims to evaluate the clinical outcomes resulting from alternative clinical or care delivery approaches for fulfilling specific health and healthcare needs. By focusing on outcomes that are meaningful to patients, studies on patient centered outcomes research strengthen the evidence base and inform the health and healthcare decisions made by patients, clinicians, and other stakeholders.
The methods used in patient centered outcomes research are diverse and often include qualitative methodologies. Broadly, qualitative research is a method of inquiry used to generate and analyze open ended textual data to enhance the understanding of a phenomenon by identifying underlying reasons, opinions, and motivations for behavior. Many different methodologies can be used in qualitative research, each with its own set of frameworks and procedures. 1 This multitude of qualitative approaches allows investigators to select and synergize methods with the specific needs associated with the aims of the study.
Qualitative methods can also be used to supplement and understand quantitative results; the integration of these approaches for scientific inquiry and evaluation is known as mixed methods. 2 This type of approach is determined a priori, because the research question drives the choice of methods, and draws on the strengths of both quantitative and qualitative approaches to resolve complex and contemporary issues in health services. This strategy is achieved by integrating qualitative and quantitative approaches at the design, methods, interpretation, and reporting levels of research. 3 Table 1 lists definitions of qualitative methods, mixed methods, and patient centered outcomes research. The methodology standards described here are intended to improve the rigor and transparency of investigations that include qualitative and mixed methods. The standards apply to designing projects, conducting the studies, and reporting the results. Owing to its focus on patient centered outcomes research, this article is not intended to be a comprehensive summary of the difficulties encountered in the conduct of qualitative and mixed methods research.
Terms and definitions used in the development of the Patient-Centered Outcomes Research Institute’s (PCORI) qualitative and mixed methods research methodology standards
- View inline
Summary points
Many publications provide guidance on how to use qualitative and mixed methods in health research
The methodological standards reported here and adopted by Patient-Centered Outcomes Research Institute (PCORI) synthesize and refine various recommendations to improve the design, conduct, and reporting of patient centered, comparative, clinical effectiveness research
PCORI has developed and adopted standards that provide guidance on key areas where research applications and research reports have been deficient in the plans for and use of qualitative and mixed methods in conducting patient centered outcomes research
The standards provide guidance to health researchers to ensure that studies of this research are designed and conducted to generate valid evidence needed to analyze patients’ and clinicians’ questions about what works best, for whom, and under what circumstances
Established by the United States Congress in 2010 13 and reauthorized in 2019, 14 the Patient-Centered Outcomes Research Institute (PCORI) funds scientifically rigorous comparative effectiveness research, previously defined as patient centered outcomes research, to improve the quality and relevance of evidence that patients, care givers, clinicians, payers, and policy makers need to make informed healthcare decisions. Such decisions might include choices about which prevention strategies, diagnostic methods, and treatment options are most appropriate based on personal preferences and unique patient characteristics.
PCORI’s focus on patient centeredness and stakeholder engagement in research has generated increased interest in and use of methodologies of qualitative and mixed methods research within comparative effectiveness research studies. Qualitative data have a central role in understanding the human experience. As with any research, the potential for these studies to generate high integrity, evidence based information depends on the quality of the methods and approaches that were used. PCORI’s authorizing legislation places a unique emphasis on ensuring scientific rigor, including the creation of a methodology committee that develops and approves methodology standards to guide PCORI funded research. 13 The methodology committee consists of 15 individuals who were appointed by the Comptroller General of the US and the directors of the Agency for Healthcare Research and Quality and the National Institutes of Health. The members of the committee are medical and public health professionals with expertise in study design and methodology for comparative effectiveness research or patient centered outcomes research ( https://www.pcori.org/about-us/governance/methodology-committee ).
The methodology committee began developing its initial group of methodology standards in 2012 (with adoption by the PCORI’s board of governors that year). Since then, the committee has revised and expanded the standards based on identified methodological issues and input from stakeholders. Before the adoption of the qualitative and mixed methods research standards, the PCORI methodology standards consisted of 56 individual standards in 13 categories. 15 The first five categories of the standards are crosscutting and relevant to most studies on patient centered outcomes research, while the other eight categories are applicable depending on a study’s purpose and design. 15
Departures from good research practices are partially responsible for weaknesses in the quality and subsequent relevance of research. The PCORI methodology standards provide guidance that helps to ensure that studies on patient centered outcomes research are designed and conducted to generate the evidence needed to answer patients’ and clinicians’ questions about what works best, for whom, and under what circumstances. These standards do not represent a complete, comprehensive set of all requirements for high quality patient centered outcomes research; rather, they cover topics that are likely to contribute to improvements in quality and value. Specifically, the standards focus on selected methodological issues that have substantial deficiencies or inconsistencies regarding how available methods are applied in practice. These methodological issues might include a lack of rigor or inappropriate use of approaches for conducting patient centered outcomes research. As a research funder, PCORI uses the standards in the scientific review of applications, monitoring of funded research projects, and evaluation of final reports of research findings.
Use of qualitative methods has become more prevalent over time. Based on a PubMed search in June 2020 (search terms “qualitative methods” and “mixed methods”), the publication of qualitative and mixed methods studies has grown steadily from 1980 to 2019. From 1980 to 1989, 63 qualitative and 110 mixed methods papers were identified. Between 1990 to 1999, the number of qualitative and mixed methods papers was 420 and 58, respectively; by 2010 to 2019, these numbers increased to 5481 and 17 031, respectively. The prominent increase in publications in recent years could be associated with more sophisticated indexing methods in PubMed as well as the recognition that both qualitative and mixed methods research are important approaches to scientific inquiry within the health sciences. These approaches allow investigators to obtain a more detailed perspective and to incorporate patients’ motivations, beliefs, and values.
Although the use of qualitative and mixed methods research has increased, consensus regarding definitions and application of the methods remain elusive, reflecting wide disciplinary variation. 16 17 Many investigators and organizations have attempted to resolve these differences by proposing guidelines and checklists that help define essential components. 12 16 18 19 20 21 22 23 24 25 26 27 28 29 For example, Treloar et al 20 offer direction for qualitative researchers in designing and publishing research by providing a 10 point checklist for assessing the quality of qualitative research in clinical epidemiological studies. Tong et al 22 provide a 32 item checklist to help investigators report important aspects of the research process for interviews and focus groups such as the study team, study methods, context of the study, findings, analysis, and interpretations.
The goal of the PCORI Methodology Standards on Qualitative and Mixed Methods is to provide authoritative guidance on the use of these methodologies in comparative effectiveness research and patient centered outcomes research. The purpose of these types of research is to improve the clinical evidence base and, particularly, to help end users understand how the evidence provided by individual research studies can be applied to particular clinical circumstances. Use of qualitative and mixed methods can achieve this goal but can also introduce specific issues that need to be captured in PCORI’s methodological guidance. The previously published guidelines generally have a broader focus and different points of emphasis.
This article describes the process for synthesizing the current literature providing guidance on the use of qualitative and mixed methods in health research; and developing methodology standards for qualitative and mixed methods used in patient centered outcomes research. We then provide an example showing how to apply the standards in the design of a patient centered outcomes research application.
Methodology standards development process
Literature review and synthesis.
The purpose of the literature review was to identify published journal articles that defined criteria for rigorous qualitative and mixed methods research in health research. With the guidance of PCORI’s medical librarian, we designed and executed searches in PubMed, and did four different keyword searches for both qualitative and mixed methods (eight searches in total; supplemental table 1). We aimed to identify articles that provided methodological guidance rather than studies that simply used the methods.
We encountered two major challenges. First, qualitative and mixed methods research has a broad set of perspectives. 30 31 Second, some medical subject headings (MeSH terms) in our queries were not introduced until recently (eg, “qualitative methods” introduced in 2003, “comparative effectiveness” introduced in 2010), which required us to search for articles by identifying a specific qualitative method (eg, interviews, focus groups) to capture the literature before 2003 ( table 1 ). These challenges could have led to missed publications. To refine and narrow our search results, we applied the following inclusion criteria:
Articles on health services or clinical research, published in English, and published between 1 January 1990 and 14 April 2017
Articles that proposed or discussed a guideline, standard, framework, or set of principles for conducting rigorous qualitative and mixed methods research
Articles that described or discussed the design, methods for, or reporting of qualitative and mixed methods research.
The search queries identified 1933 articles (1070 on qualitative methods and 863 on mixed methods). The initial citation lists were reviewed, and 204 duplicates were removed. Three authors (BG, MH, and AB) manually reviewed the 1729 remaining article abstracts. Titles and abstracts were independently evaluated by each of the three reviewers using the inclusion criteria. Disagreements were adjudicated by an in-person meeting to determine which articles to include. This initial round of review yielded 212 references, for which the full articles were obtained. The full articles were reviewed using the same inclusion and exclusion criteria as the abstracts. Most of these articles were studies that had used a qualitative or mixed methods approach but were only reporting on the results of the completed research. Therefore, these articles were not able to inform the development of standards for conducting qualitative and mixed methods research and they were excluded, resulting in the final inclusion of 56 articles (supplemental table 2). Following the original search, the literature was scanned for new articles providing guidance on qualitative and mixed methods, resulting in four articles being added to the final set of literature. These articles come from psychology and health psychology specialties and seek to provide not only minimal standards in relation to qualitative and mixed methods research but also standards for best practice that apply across a wide range of fields. 32 33 34 35
Initial set of methodology standards
Using an abstraction form that outlined criteria for qualitative and mixed methods manuscripts and research proposals, we abstracted the articles to identify key themes, recommendations, and guidance under each criterion. Additional information was noted when considered relevant. A comprehensive document was created to include the abstractions and notes for all articles. This document outlined the themes in the literature related to methodological guidance. We began with the broadest set of themes organized into 11 major domains: the theoretical approach, research topics, participants, data collection, analysis and interpretation, data management, validity and reliability, presentation of results, context of research, impact of the researchers (that is, reflexivity), and mixed methods. As our goal was to distill the themes into broad standards that did not overlap with pre-existing PCORI methodology standards, we initially condensed the themes into six qualitative and three mixed methods standards. Following discussion among members of the working group, some standards were combined and two were dropped because of substantial overlap with each other or with previously developed PCORI methodology standards.
The key themes identified from the abstracted information were used as the foundation for the first draft of the new methodology standards. We then further discussed the themes as a team and removed redundancies, refined the labeling of themes, and removed themes deemed extraneous through a team based adjudication process. The draft standards were presented to PCORI’s methodology committee to solicit feedback. Revisions were made on the basis of this feedback.
Expert panel one day workshop
A one day expert panel workshop was held in Washington, DC, on 18 January 2018. Ten individuals regarded as international leaders in qualitative and mixed methods were invited to attend—including those who had created standards previously or had a substantial number of peer reviewed publications reporting qualitative and mixed methods in health research; had many years’ experience as primary researchers; and had served as editors of major textbooks and journals. The panel was selected on the basis of their influence and experience in these methodologies as well as their broad representation from various fields of study. The representation of expertise spanned the fields of healthcare, anthropology, and the social sciences (supplemental table 3).
Before the meeting, we emailed the panel members the draft set of qualitative and mixed methods standards, PCORI’s methodology standards document, and the background document describing how the draft standards had been developed. At the meeting, the experts provided extensive feedback, including their recommendations regarding what needs to be done well when using these methodological approaches. The panel emphasized that when conducting mixed methods research, this approach should be selected a priori, based on the research question, and that integration of the mixed approaches is critical at all levels of the research process (from inception to data analysis). The panel emphasized that when conducting qualitative research, flexibility and reflexive iteration should be maintained throughout the process—that is, the sampling, data collection, and data analysis. The main theme from the meeting was that the draft standards were not comprehensive enough to provide guidance for studies on patient centered outcomes research or comparative effectiveness research that involved qualitative and mixed methods. After the conclusion of the workshop, feedback and recommendations were synthesized, and the draft standards were reworked in the spring of 2018 ( fig 1 ). This work resulted in a new set of four qualitative methods standards and three mixed methods standards representing the unique features of each methodology that were not already included in the methodology standards previously adopted by PCORI.

Process of development and adoption of the Patient-Centered Outcomes Research Institute’s (PCORI) methodology standards on qualitative and mixed methods research
- Download figure
- Open in new tab
- Download powerpoint
Continued refinement and approval of methodology standards
In late spring 2018, the revised draft methodology standards were presented to PCORI’s methodology committee first by sharing a draft of the standards and then via oral presentation. Feedback from the methodology committee centered around eliminating redundancy in the standards proposed (both across the draft standards and in relation to the previously adopted categories of standards) and making the standards more actionable. The areas where the draft standards overlapped with the current standards were those for formulating research questions, for patient centeredness, and for data integrity and rigorous analyses. Each draft standard was reviewed and assessed by the methodology committee members and the staff workgroup to confirm its unique contribution to PCORI’s methodology standards. After this exercise, each remaining standard was reworded to be primarily action guiding (rather than explanatory). This version of proposed standards was approved by the methodology committee to be sent to PCORI’s board of governors for a vote to approve for public comment. The board of governors approved the standards to be posted for public comment.
The public comment period hosted on PCORI’s website ( https://www.pcori.org/engagement/engage-us/provide-input/past-opportunities-provide-input ) was held from 24 July 2018 to 21 September 2018. Thirty nine comments were received from nine different stakeholders—seven health researchers, one training institution, and one professional organization. Based on the public comments, minor wording changes were made to most of the draft standards. The final version of the standards underwent review by both the methodology committee and PCORI’s board of governors. The board voted to adopt the final version of the standards on 26 February 2019 ( table 2 ).
Patient-Centered Outcomes Research Institute’s (PCORI) methodology standards for qualitative methods and mixed methods
Application of methodology standards in research design
The standards can be used across the research continuum, from research design and application development, conduct of the research, and reporting of research findings. We provide an example for researchers on how these standards can be used in the preparation of a research application ( table 3 ).
Guidance for researchers on how to use Patient-Centered Outcomes Research Institute’s (PCORI) methodology standards for qualitative and mixed methods research in application preparation
QM-1: State the qualitative approach to research inquiry, design, and conduct
Many research proposals on patient centered outcomes research or comparative effectiveness research propose the use of qualitative methods but lack adequate description of and justification for the qualitative approach that will be used. Often the rationale for using qualitative methods is not tied back to the applicable literature and the identified evidence gap, missing the opportunity to link the importance of the approach in capturing the human experience or patient voice in the research aims. The approach to inquiry should be explicitly stated along with the rationale and a description of how it ties to the research question(s). The research proposal should clearly define how the qualitative approach will be operationalized and supports the choice of methods for participant recruitment, data collection, and analysis. Moreover, procedures for data collection should be stated, as well as the types of data to be collected, when data will be collected (that is, one point in time v longitudinal), data management, codebook development, intercoder reliability process, data analysis, and procedures for ensuring full confidentiality.
QM-2: Select and justify appropriate qualitative methods and sampling strategy
While the number of participants who will be recruited for focus groups or in-depth interviews is usually described, the actual sampling strategy is often not stated. The description of the sampling strategy should state how it aligns with the qualitative approach, how it relates to the research question(s), and the variation in sampling that might occur over the course of the study. Furthermore, most research proposals state that data will be collected until thematic saturation is reached, but how this will be determined is omitted. As such, this standard outlines the information essential for understanding who is participating in the study and aims to reduce the likelihood of making unsupported statements, emphasizing transparency in the criteria used to determine the stopping point for recruitment and data collection.
QM-3: Link the qualitative data analysis, interpretations, and conclusions to the study question
Qualitative analysis transforms data into information that can be used by the relevant stakeholder. It is a process of reviewing, synthesizing, and interpreting data to describe and explain the phenomena being studied. The interpretive process occurs at many points in the research process. It begins with making sense of what is heard and observed during data gathering, and then builds understanding of the meaning of the data through data analysis. This is followed by development of a description of the findings that makes sense of the data, in which the researcher’s interpretation of the findings is embedded. Many research proposals state that the data will be coded, but it is unclear by whom, their qualifications, or the process. Very little, if any, description is provided as to how conclusions will be drawn and how they will be related to the original data, and this standard highlights the need for detailed information on the analytical and interpretive processes for qualitative data and its relationship to the overall study.
QM-4: Establish trustworthiness and credibility of qualitative research
The qualitative research design should incorporate elements demonstrating validity and reliability, which are also known by terms such as trustworthiness and credibility. Studies with qualitative components can use several approaches to help ensure the validity and reliability of their findings, including audit trail, reflexivity, negative or deviant case analysis, triangulation, or member checking (see table 1 for definitions).
MM-1: Specify how mixed methods are integrated across design, data sources, and/or data collection phases
This standard requires investigators to declare and support their intent to conduct a mixed methods approach a priori in order to avoid a haphazard approach to the design and resulting data. Use of mixed methods can enhance the study design, by using the strengths of both quantitative and qualitative research as investigators are afforded the use of multiple data collection tools rather than being restricted to one approach. Mixed methods research designs have three key factors: integration of data, relative timing, and implications of linkages for methods in each component. Additionally, the standards for mixed methods, quantitative, and qualitative methodologies must be met in the design, implementation, and reporting stages. This is different from a multimethod research design in which two or more forms of data (qualitative, quantitative, or both) are used to resolve different aspects of the research question independently and are not integrated.
MM-2: Select and justify appropriate mixed methods sampling strategy
Mixed methods research aims to contribute insights and knowledge beyond that obtained from quantitative or qualitative methods only, which should be reflected in the sampling strategies as well as in the design of the study and the research plan. Qualitative and quantitative components can occur simultaneously or sequentially, and researchers must select and justify the most appropriate mixed method sampling strategy and demonstrate that the desired number and type of participants can be achieved with respect to the available time, cost, research team skillset, and resources. Those sampling strategies that are unique to mixed methods (eg, interdependent, independent, and combined) should focus on the depth and breadth of information across research components.
MM-3: Integrate data analysis, data interpretations, and conclusions
Qualitative and quantitative data often are analyzed in isolation, with little thought given to when these analyses should occur or how the analysis, interpretation, and conclusions integrate with one another. There are multiple approaches to integration in the analysis of qualitative and quantitative data (eg, merging, embedding, and connecting). As such, the approach to integration should determine the priority of the qualitative and quantitative components, as well as the temporality with which analysis will take place (eg, sequentially, or concurrently; iterative or otherwise). Either a priori or emergently, where appropriate, researchers should define these characteristics, identify the points of integration, and explain how integrated analyses will proceed with respect to the two components and the selected approach.
The choice between multiple options for prevention, diagnosis, and treatment of health conditions presents a considerable challenge to patients, clinicians, and policy makers as they seek to make informed decisions. Patient centered outcomes research focuses on the pragmatic comparison of two or more health interventions to determine what works best for which patients and populations in which settings. 5 The use of qualitative and mixed methods research can enable more robust capture and understanding of information from patients, caregivers, clinicians, and other stakeholders in research, thereby improving the strength, quality, and relevance of findings. 4
Despite extensive literature on qualitative and mixed methods research in general, the use of these methodologies in the context of patient centered outcomes research or comparative effectiveness research continues to grow and requires additional guidance. This guidance could facilitate the appropriate design, conduct, analysis, and reporting of these approaches. For example, the need for including multiple stakeholder perspectives, understanding how an intervention was implemented across multiple settings, or documenting the clinical context so decision makers can evaluate whether findings would be transferable to their respective settings pose unique challenges to the rigor and agility of qualitative and mixed methods approaches.
PCORI’s methodology standards for qualitative and mixed methods research represent an opportunity for further strengthening the design, conduct, and reporting of patient centered outcomes research or comparative effectiveness research by providing guidance that encompasses the broad range of methods that stem from various philosophical assumptions, disciplines, and procedures. These standards directly affect factors related to methodological integrity, accuracy, and clarity as identified by PCORI staff, methodology committee members, and merit reviewers in studies on patient centered outcomes research or comparative effectiveness research. The standards are presented at a level accessible to researchers new to qualitative and mixed methods research; however, they are not a substitute for appropriate expertise.
The challenges of ensuring rigorous methodology in the design and conduct of research are not unique to qualitative and mixed methods research, because the imperative to increase value and reduce waste in research design, conduct, and analysis is widely recognized. 36 Consistent with such efforts, PCORI recognizes the importance of continued methodological development and evaluation and is committed to listening to the research community and providing updated guidance based on methodological advances and research needs. 37
Acknowledgments
We thank the Patient-Centered Outcomes Research Institute’s (PCORI) methodology committee during this work (Naomi Aronson, Ethan Bach, Stephanie Chang, David Flum, Cynthia Girman, Steven Goodman (chairperson), Mark Helfand, Michael S Lauer, David O Meltzer, Brian S Mittman, Sally C Morton, Robin Newhouse (vice chairperson), Neil R Powe, and Adam Wilcox); and Frances K Barg, Benjamin F Crabtree, Deborah Cohen, Michael Fetters, Suzanne Heurtin-Roberts, Deborah K Padgett, Janice Morse, Lawrence A Palinkas, Vicki L Plano Clark, and Catherine Pope, for participating in the expert panel meeting and consultation.
Contributors: BG led the development of the methodology standards and wrote the first draft of the paper. MH, AB, SZ, EE, DH, and RN made a substantial contribution to all stages of developing the methodology standards. BG, SZ, MH, and AB drafted the methodology standards. DH, EE, and RN gave critical insights into PCORI’s methodology standards development processes and guidance. SZ served as qualitative methods consultant to the workgroup. BG provided project leadership and guidance. MH and AB facilitated the expert panel meeting. SZ is senior author. BG and SZ are the guarantors of this work and accept full responsibility for the finished article and controlled the decision to publish. The corresponding author attests that all listed authors meet authorship criteria and that no others meeting the criteria have been omitted.
Funding: No funding was used to support this work. All statements in this report, including its findings and conclusions, are solely those of the authors and do not necessarily represent the views of PCORI, its board of governors or methodology committee. The views expressed in this article are those of the authors and do not necessarily represent the views of the Department of Veterans Affairs.
Competing interests: All authors have completed the ICMJE uniform disclosure form at http://www.icmje.org/conflicts-of-interest/ and declare: no support from any organization for the submitted work; no financial relationships with any organizations that might have an interest in the submitted work in the previous three years; no other relationships or activities that could appear to have influenced the submitted work.
The lead author affirms that the manuscript is an honest, accurate, and transparent account of the work being reported; that no important aspects of the study have been omitted; and that any discrepancies from the work as planned have been explained.
Provenance and peer review: Not commissioned; externally peer reviewed.
Patient and public involvement: Patients and stakeholders were invited to comment on the draft standards during the public comment period held from 24 July 2018 to 21 September 2018. Comments were reviewed and revisions made accordingly. Development of the standards, including the methods, were presented at two PCORI board of governors’ meetings, which are open to the public, recorded, and posted on the PCORI website.
This is an Open Access article distributed in accordance with the Creative Commons Attribution Non Commercial (CC BY-NC 4.0) license, which permits others to distribute, remix, adapt, build upon this work non-commercially, and license their derivative works on different terms, provided the original work is properly cited and the use is non-commercial. See: http://creativecommons.org/licenses/by-nc/4.0/ .
- Collins CS ,
- Stockton CM
- Creswell JW ,
- Plano Clark VL
- Fetters MD ,
- Creswell JW
- ↵ Patient-Centered Outcomes Research Institute. Patient-centered outcomes research. 2010-19. https://www.pcori.org/research-results/about-our-research/research-we-support .
- Institute of Medicine
- Crabtree BF ,
- Klassen AC ,
- Plano Clark VL ,
- Clegg Smith KC ,
- Office of Behavioral and Social Sciences Research
- ↵ Patient Protection and Affordable Care Act, Pub. L. No. 111-148 Stat. 119 (March 23, 2010).
- ↵ Further Consolidated Appropriations Act, 2020, Pub. L. No. 116-94 (20 December 2019).
- ↵ Patient-Centered Outcomes Research Institute (PCORI). PCORI methodology standards. 2011-19. https://www.pcori.org/research-results/about-our-research/research-methodology/pcori-methodology-standards .
- Molina-Azorin JF
- Chapple A ,
- Treloar C ,
- Champness S ,
- Simpson PL ,
- Higginbotham N
- Cesario S ,
- Santa-Donato A
- Sainsbury P ,
- Flemming K ,
- McInnes E ,
- Davidoff F ,
- Batalden P ,
- Stevens D ,
- Mooney SE ,
- SQUIRE development group
- Gagnon MP ,
- Griffiths F ,
- Johnson-Lafleur J
- ↵ National Cancer Institute. Qualitative methods in implementation science. 2018. https://cancercontrol.cancer.gov/sites/default/files/2020-09/nci-dccps-implementationscience-whitepaper.pdf
- O’Brien BC ,
- Harris IB ,
- Beckman TJ ,
- ↵ National Institute for Health and Clinical Excellence. The guidelines manual. Appendix H: Methodology checklist: qualitative studies. https://www.nice.org.uk/process/pmg6/resources/the-guidelines-manual-appendices-bi-2549703709/chapter/appendix-h-methodology-checklist-qualitative-studies .
- Crabtree BF
- Johnson RB ,
- Onwuegbuzie AJ ,
- American Psychological Association
- Levitt HM ,
- Bamberg M ,
- Josselson R ,
- Suárez-Orozco C
- Motulsky SL ,
- Morrow SL ,
- Ponterotto JG
- Bishop FL ,
- Horwood J ,
- Chilcot J ,
- Ioannidis JPA ,
- Greenland S ,
- Hlatky MA ,
- ↵ Patient-Centered Outcomes Research Institute. The PCORI methodology report. 2019. https://www.pcori.org/sites/default/files/PCORI-Methodology-Report.pdf .
- Find My Rep
You are here
Research Methods for Health Care Practice
- Frances Griffiths - University of Warwick, UK
- Description
Research Methods for Health Care Practice guides the researcher through their journey, giving detailed, step-by-step advice on planning and carrying out each stage of the research. Useful examples from health care research are included throughout to illustrate the application of the techniques and methods discussed.
The book provides discussion of all the key issues and stages of research, including:
- user involvement in research
- research ethics
- deciding on a research approach
- data collection and analysis methods
'In addition to the expected content of a book on research methods, this text offers detailed discussion of subjects that are often peripheral in research, and brings them to a wider audience' - Nursing Standard
The book as a whole is simple and clear , analysing data section is a must read for novice researchers
I felt the first part of this book was clear and easy for students to follow; however I felt the second part of the book tried to achieve to much in each chapter.
A really useful book for students who are considering undertaking a piece of research. It provides detailed information on developing research question, aims and objectives as well as provoking thought regarding whether research is needed, ethical issues and various methodological approaches which could be used to undertake the research.
I think this book is useful and supplementary for the students that have a biostatistics basis
Liked the lay out of the book and that it was linked closely with how to complete a research project.
A ggod introduction to research methods with practical examples
A very informative book, the content of which would be appropriate for learners just starting out in the field of 'research'.
Used supplement selected sessions focusing on utility of research in every day clinical practice
very good introductory text for clinicians who are new to research
Preview this book
Sample materials & chapters.
Introduction
Chapter One
Chapter Two
For instructors
Please select a format:
Select a Purchasing Option
- Electronic Order Options VitalSource Amazon Kindle Google Play eBooks.com Kobo
Related Products

SAGE Research Methods is a research methods tool created to help researchers, faculty and students with their research projects. SAGE Research Methods links over 175,000 pages of SAGE’s renowned book, journal and reference content with truly advanced search and discovery tools. Researchers can explore methods concepts to help them design research projects, understand particular methods or identify a new method, conduct their research, and write up their findings. Since SAGE Research Methods focuses on methodology rather than disciplines, it can be used across the social sciences, health sciences, and more.
With SAGE Research Methods, researchers can explore their chosen method across the depth and breadth of content, expanding or refining their search as needed; read online, print, or email full-text content; utilize suggested related methods and links to related authors from SAGE Research Methods' robust library and unique features; and even share their own collections of content through Methods Lists. SAGE Research Methods contains content from over 720 books, dictionaries, encyclopedias, and handbooks, the entire “Little Green Book,” and "Little Blue Book” series, two Major Works collating a selection of journal articles, and specially commissioned videos.
Research Methods for Health Services Planning
- First Online: 04 November 2020
Cite this chapter
- Richard K. Thomas 2
759 Accesses
Much of the effort involved in planning can be attributed to the research activities that support the planning function. The type and amount of research undertaken are dictated by a number of factors, including, among other things, the intended use of the findings. Even the most basic type of planning research is likely to require certain skills, substantive knowledge, and familiarity with multiple methodologies and perspectives. A major decision, thus, involves the assignment of responsibility for the research function. Planning research involves a number of distinct components that generally follow a certain sequence. With each component the intent is to collect data that can be converted into information as the basis for identifying solutions. The core activities involved in data analysis include describing, identifying, comparing, evaluating, monitoring, interpreting, and recommending. The research approach can be exploratory, descriptive, or causal. A distinction is made between primary research and secondary research, although the steps in the research process are similar regardless of the type of research being conducted.
This is a preview of subscription content, log in via an institution to check access.
Access this chapter
- Available as PDF
- Read on any device
- Instant download
- Own it forever
- Available as EPUB and PDF
- Compact, lightweight edition
- Dispatched in 3 to 5 business days
- Free shipping worldwide - see info
- Durable hardcover edition
Tax calculation will be finalised at checkout
Purchases are for personal use only
Institutional subscriptions
Creswell, J. W. (1994). Research design: Qualitative and quantitative approaches . Thousand Oaks, CA: Sage.
Google Scholar
Dever, G. E. A. (1991). Community health assessment . Gaithersburg, MD: Aspen.
Dillman, D. A. (1978). Mail and telephone survey: The total design . New York: Wiley.
Plane, D. A., & Rogerson, P. A. (1994). The geographical analysis of population . New York: Wiley.
Further Reading
Aday, L. A. (1989). Designing and conducting health surveys . San Francisco, CA: Jossey-Bass.
Berkowitz, E. N., Pol, L. G., & Thomas, R. K. (1997). Healthcare market research . Burr Ridge, IL: Irwin Professional Publishing.
Curry, L., & Nunez-Smith, M. (2014). Mixed methods in health sciences research: A primer . Thousand Oaks, CA: Sage.
Forister, J. G., & Blessing, J. D. (2019). Introduction to research and medical literature for health professionals . Burlington, MA: Jones & Bartlett.
Mackridge, R., & Rowe, P. (2018). A practical approach to using statistics in health research: From planning to reporting . Hoboken, NJ: Wiley.
Book Google Scholar
Ozdenerol, E. (2017). Spatial health inequalities . Boca Raton, FL: CRC Press.
Download references
Author information
Authors and affiliations.
Public Health Research Institute, University of Tennessee Health Science Center, Health and Performance Resources, LLC, Mississippi State University (Social Science Research Center), Memphis, TN, USA
Richard K. Thomas
You can also search for this author in PubMed Google Scholar
Rights and permissions
Reprints and permissions
Copyright information
© 2021 Springer Science+Business Media, LLC, part of Springer Nature
About this chapter
Thomas, R.K. (2021). Research Methods for Health Services Planning. In: Health Services Planning. Springer, New York, NY. https://doi.org/10.1007/978-1-0716-1076-3_12
Download citation
DOI : https://doi.org/10.1007/978-1-0716-1076-3_12
Published : 04 November 2020
Publisher Name : Springer, New York, NY
Print ISBN : 978-1-0716-1075-6
Online ISBN : 978-1-0716-1076-3
eBook Packages : Medicine Medicine (R0)
Share this chapter
Anyone you share the following link with will be able to read this content:
Sorry, a shareable link is not currently available for this article.
Provided by the Springer Nature SharedIt content-sharing initiative
- Publish with us
Policies and ethics
- Find a journal
- Track your research

An official website of the United States government
The .gov means it’s official. Federal government websites often end in .gov or .mil. Before sharing sensitive information, make sure you’re on a federal government site.
The site is secure. The https:// ensures that you are connecting to the official website and that any information you provide is encrypted and transmitted securely.
- Publications
- Account settings
Preview improvements coming to the PMC website in October 2024. Learn More or Try it out now .
- Advanced Search
- Journal List
- Sultan Qaboos Univ Med J
- v.8(1); 2008 Mar
Qualitative Research and its Uses in Health Care
Although relatively uncommon in health care research, qualitative research is now receiving recognition and is increasingly used in health care research with social and cultural dimensions. Unlike quantitative research, which is deductive and tends to analyze phenomena in terms of trends and frequencies, qualitative research seeks to determine the meaning of a phenomenon through description. It aims to develop concepts that aid in the understanding of natural phenomena with emphasis on the meaning, experiences and views of the participants. Differences among qualitative researchers exist on matters of ontology, epistemology, data collection methods and methods of evaluation. The aim of this article is not to act as a practical guide on how to conduct qualitative research, but is an attempt to give an introduction to qualitative research methods and their use in health-related research.
Q ualitative research is defined as an umbrella term covering an array of interpretative techniques which seek to describe, decode, translate and otherwise come to terms with the meaning, not the frequency, of certain more or less naturally occurring phenomena in the social world. 1 , 2
As a method of inquiry, it was first used by sociologists and anthropologists in the early twentieth century, although it existed much earlier than that in its non-structural form. Researchers studied cultures and groups in their own and foreign settings and told stories of their experience long before then. In the 1920s and 1930s, social anthropologists and sociologists implemented a more focused approach compared to the old unsystematic and journalistic style used in those days. Since the 1960s, qualitative research has experienced a steady growth starting with the development of grounded theory and new publications in ethnography. 3 , 4 The number of books, articles and papers related to qualitative research has increased tremendously during the past 20 years and more researchers, including health-related professionals, have moved to a more qualitative paradigm adapting and modifying these approaches to the study needs of their own areas. 4
Since qualitative research does not aim to enumerate, it is sometimes viewed as the exact opposite to quantitative methods and the two methods are frequently presented as antagonists. Quantitative research is based on structure and uses experiments and surveys as methods. In addition, it is deductive in nature and uses statistical sampling methods. In contrast, qualitative research is described as an action research using observation and interview methods. It is inductive in nature and depends on the purposeful selection of participants. Whereas quantitative researchers use reliability as a way of verification, qualitative researchers use validity. Recently, there has been a growing recognition that the quantitative-qualitative debate and distinction is unnecessary and that it would be more fruitful for the relation between the two methods to be complementary and overlapping rather than exclusive. 6 In fact, neither qualitative nor quantitative research is superior to the other; there are weaknesses and strengths in each method. Qualitative and quantitative research methods can indeed be seen as complementary and both are necessary to provide an understanding of a phenomenon.
Several researchers have argued that the research questions and the phenomenon under investigation should determine the methodology to used. 7 , 8 , 9 The crucial question, therefore, is not “what is the best research method?” but “what is the best research method for answering this question most effectively and efficiently?” 10 So, while qualitative and quantitative research may well investigate similar topics, each will address a different type of question. Holman sums up this position: “true understanding in medicine cannot be achieved without adding qualitative methods to the research arsenal”. 11
Traditional quantitative methods, such as randomised controlled trials, are the appropriate means of testing, for example, the effect of an intervention or a treatment, while a qualitative exploration of beliefs and understandings is needed to find out why the results of research are often not implemented in clinical practice. The aim of qualitative research is to develop concepts that can help us understand social phenomena in natural settings, giving emphasis on the meanings, experiences and views of the participants. 10
Qualitative research methods are the most suitable for this approach because of their emphasis on people’s lived experience. They are considered to be well suited for locating the meanings that people place on the events, processes, and structures of their lives and their perceptions, presuppositions and assumptions. 5 In his advice to graduate students, Patton lists a number of conditions that are suitable for a qualitative study. These include: questions about people’s experiences; inquiry into the meanings people make of their experiences; studying a person in the context of her or his social/interpersonal environment and research where it is difficult to develop a standardised instrument due to the lack of knowledge on the phenomenon. 12
ARGUMENTS FOR AND AGAINST QUALITATIVE METHODS
There are major differences of opinions among qualitative researchers on matters of ontology and epistemology as well as the methods to be used and criteria of evaluation. There are also disagreements about the nature, purpose, status and practice of its methods. A large number of authors take a predominantly method-based approach; authors such as Miles and Huberman 5 and Patton 12 put emphasis on data collection techniques. Another approach is to classify qualitative research according to research traditions, i.e. whether phenomenological, grounded theory or ethnography, amongst others. Authors such as Creswel 9 and Denzin and Lincolin 3 prefer this approach, which has the advantage of being based on systematisation of knowledge providing a sense of order and orientation. On the other hand, it has the disadvantage of oversimplification, ignoring the issues of the research question and conceptual frameworks used and the way these issues can shape the research process and the findings. 13 In addition, some researchers have decided to classify qualitative research according to the research question or the method of analysis.
Although the majority of qualitative researchers stress that qualitative research is inductive in nature, in contrast to quantitative research which is deductive, there are qualitative researchers who argue that both can be used for different purposes and at different times, and that qualitative research can be done in a deductive way where prior assumptions are tested on new cases. 14 Retroduction, which is defined as the movement backward and forward between theory and data or the combination of deduction and induction, is said to be a characteristic of qualitative research. 11 The degree of deduction or induction and which one follows the other depends on the research question. 12 .
Guba and Lincoln 15 used the term “emergent design” to describe a qualitative study design that emerges as the study progresses in response to the researcher’s early observations. There are also qualitative studies in health care that base their research questions on the results of prior literature of quantitative studies on the subject. 16 , 17 While the research questions in these studies were relatively precise, the method used was flexible.
Miles and Huberman 5 state that no study conforms exactly to a standard methodology and that the researcher bends the methodology to the peculiarities of the study. According to Creswell, 9 traditions of inquiry used “need not be pure, and one might mix procedures from several”. Mixing approaches and procedures is encouraged by some authors and regarded as a creative approach to qualitative research. 12
Studies using qualitative research in health care have been criticised for the misguided separation of method from theory and of technique from the conceptual underpinnings. 18 However, qualitative health researchers respond by stating that the choice of method and how it is used can perfectly well be matched to what is being studied rather than to the methodological leanings of the researcher. 10 It has been suggested that incorporating qualitative research method experts into health research teams enriches research and ensures that the right methodology is used for answering the right questions. Finally, using qualitative methods in health-related research has resulted in more insight into health professionals’ perceptions of lay participation in care and identification of barriers to changing healthcare practice.
QUALITATIVE RESEARCH APPROACHES
Grounded theory, phenomenology and ethnography are three approaches used in qualitative research. Grounded theory approach is a commonly used qualitative method in the social sciences to inductively generate or discover a theory out of the data. 9 Phenomenology and ethnography are more commonly used qualitative approaches in health care and will be highlighted below.
PHENOMENOLOGY
One of the major strengths of qualitative research is its emphasis upon understanding the phenomenon of interest holistically and in its context. The term phenomenology is popular and widely embraced, but its meaning has become confusing and faint. Different researchers refer to phenomenology differently. It can refer to an inquiry paradigm, an interpretive theory, a philosophy, an analytical perspective, a major qualitative research tradition or a research method framework. 12
In spite of the differences, all of these approaches share the focus of phenomenology, which is exploring how human beings make sense of experience and the meaning they give to these experiences. Phenomenology is being used in the social and human sciences including sociology, education, psychology, nursing and health sciences. 9
Phenomenologists are interested in how people put together the phenomena they experience in such a way as to make sense of the world and develop a worldview. They assume commonality in human experience and focus on meaning-making as the essence of human experience. The essence is the core meaning mutually understood through a phenomenon commonly experienced. 12 The phenomenon under study may be emotions, relationships, a programme, an organisation or a culture. 12 Bracketing is one of the central ideas in phenomenology. It means that the researcher has to set aside all of his prejudgments and his previous experience about the phenomena and approach the field with an open mind, imagination and intuition. Although important, bracketing is often said to be a difficult task. 9
Patton 12 describes the difference between conducting a phenomenological study and using a phenomenological perspective to a study. He argues that, “one can employ a general phenomenological perspective to elucidate the importance of using methods that capture people’s experience of the world without conducting a phenomenological study that focuses on the essence of shared experience”.
ETHNOGRAPHY
In ethnography, the researcher studies the structure and function of a group of people. An example of a structure or configuration is the kinship, while the function refers to patterns of relationships affecting and regulating behaviour. 9 The aim of ethnographic studies is to give a holistic picture of the social group studied, attempting to describe aspects of the cultural and social system of that particular group. These aspects could be the group history, religion, economy, politics or environment.
Data collection methods vary in ethnographic studies with observation and interviews being the most popular methods. Although some authors expressed concern that anthropological methods may be misused or applied superficially by the medical profession, 19 others, however, expressed the need for acknowledging and incorporating ethnographic methods in health care research. 20 Savage 19 explains that today the term ethnography can be applied to any small-scale social research carried out in everyday settings and uses several methods evolving in design and focusing on an individual’s meanings and explanations. In health care, ethnography has been used in topics related to health beliefs and practices, allowing these issues to be viewed in the context in which they occur and therefore helping broaden the understanding of behaviours related to health and illness. 20
In addition, there has been an increase in the amount of cross-cultural research and a change in the form of this research. Recently, more short-term ethnographic and cross-cultural studies have been undertaken, for example, studies sponsored by international development agencies or student exchange projects. 12
QUALITATIVE RESEARCH INSTRUMENTS
Qualitative research instruments used for data collection include interviews, observations, and analysis of documents. Interviews are the most common techniques used to gather research information. There are three types of interviews: structured, semi-structured and unstructured, described in some books as structured, informed and guided, respectively. 13
The semi-structured interview is more commonly used in health care-related qualitative research. Such an interview is characteristically based on a flexible topic guide that provides a loose structure of open-ended questions to explore experiences and attitudes. It has the advantage of great flexibility, enabling the researcher to enter new areas and produce richer data. In addition, it helps the researcher to develop a rapport with the informants. Semi-structured interviews elicit people’s own views and descriptions and have the benefit of uncovering issues or concerns that have not been anticipated by the researcher. 6 They are commonly used when the aim is to gain information on the perspectives, understandings and meanings constructed by people regarding the events and experiences of their lives. However, this type of interviewing is claimed to reduce the researcher’s control over the interview situation and take a longer time to conduct and analyse, in addition to the difficulties of the analysis process. 21
In health care, interviews are the appropriate tool to be used if the research is concerned with interpersonal aspects of care or if the available evidence is limited. 6
Another qualitative research instrument that can be used in health care research is the focus group. Focus group interviews have the advantage of being more time efficient as more people can be interviewed for the same amount of time. They also provide a richer source of data. On the other hand, focus group interviews tend to document the ‘public’ rather than the ‘private’ views of the individuals. In addition some people do not interview well in-group situations. 13
THE USE OF THE INTERVIEW GUIDE
Some qualitative researchers are reluctant to plan a design of their study in advance of the data collection. They argue that the phenomenon studied must first be discovered and they describe their design as emergent. However, increasingly more qualitative researchers appear ready to define a research question and develop an interview guide prior to starting the data collection. 11 Patton defines an interview guide as a series of topics or broad interview questions which the researcher is free to explore and probe with the interviewee. 12 The advantage of an interview guide is that it helps the interviewer pursue the same basic lines of inquiry with each person interviewed and manage the interviews in a more systematic and comprehensive way. The findings of earlier work are increasingly being used as a facilitator for further research. However, concepts drawn from earlier work are supposed to be held lightly and to be subject to reformulation or rejection by the researcher especially as the study goes on and the research progresses. 11 The extent to which such a flexibility of design is important will vary depending on the topic and the aim of the study.
Sampling strategies in qualitative research are largely determined by the purpose of the study. Statistical representativeness is not considered as a prime requirement in qualitative research and is not normally sought. Furthermore, qualitative data collection is more time consuming and expensive, which makes the use of a probability sample impractical. 6 The aim of sampling in qualitative research is to identify specific groups of people who hold characteristics or live in circumstances relevant to the phenomena being studied. In this way, identified informants are expected to enable enriched exploration of attitudes and aspects of behaviour relevant to the research. 10 Two types of samples used in qualitative research, maximum variation and homogenous samples, are explained below.
According to Patton, 12 maximum variation sampling is where the researcher attempts to study a phenomenon by seeking out settings or persons that represent the greatest differences in that phenomenon. A maximum variation sample documents diverse variations and identifies important common patterns by representing diverse cases to develop fully multiple perspectives about the cases. 9 This means that the participants are sampled based on particular predetermined criteria in order to cover a range of constituencies, such as different age, cultural background or class. 6
Similarly, the researcher can select the setting of the data collection on the basis that it is sufficiently similar to other settings in which generalisation is sought. This way the researcher is demonstrating the possibility that the setting studied is representative of the population studied. 2
In homogeneous sampling, the researcher chooses a small homogenous sample with the purpose of describing some particular subgroups in depth. 12
The sample size in qualitative research is not determined by fixed rules, but by factors such as the depth and duration of the interview and what is feasible for a single interviewer. 22 Although it is theoretically possible to carry out qualitative research on large samples, qualitative researchers find themselves obliged by time and resource limits to trade breadth for depth. 11 The sample size for interview studies is usually much smaller than those of a quantitative research, usually not exceeding 50 participants, although this can vary with the research question asked. 6 Patton 12 states, “there are no rules for sample size in qualitative inquiry”. In other words, sample size depends on the aim of the study and what is possible, given the time and resources available.
ANALYSING QUALITATIVE RESEARCH
Although there are plenty of guidelines for analysing qualitative research, applying these guidelines requires judgment and creativity because each qualitative study is unique. 12 A researcher might also be confused by the different terms used by qualitative researchers when describing analysis. Analysis might be described as interpretation, making sense of data, or transforming data. Analysis is sometimes presented to indicate different procedures based on language, theory or what is described as interpretive/descriptive analysis. 11 However, overlap can take place between these different methods and a researcher might decide to use a method of analysis that is based on language, such as symbolic interactionist, while using grounded theory to develop a theory at the same time. Most of the analytical approaches to qualitative research in health care are ‘generic’ and are not labelled within one of the specific traditions of qualitative research. A common approach in most of these studies is general and inductive in nature, but does not comply with the very systematic and rigorous inductive approach of grounded theory. In addition, it has been described that many researchers use a simple two-level analysis scheme followed by a more specific level. This means that the researcher can initiate the analysis based on the conceptual framework used in order to produce more inductive data, and the coding moves from the descriptive to the more interpretative and inferential codes. 5
COMPUTER USE IN QUALITATIVE DATA ANALYSIS
Qualitative research studies typically produce very large amount of data that needs to be managed efficiently. Computer packages can improve the efficiency of data management. 11 Computer programs provide a way of storing and retrieving material. They are therefore useful in locating cases, statements, phrases or even words, thereby replacing the tedious and time-consuming process of “cutting and pasting” and “colour coding”. The use of computer packages, however, is claimed to distance the analyst from the data, 11 and may take the place of a close and careful analysis. Using a computer programme can lead to quantitative analysis instead of qualitative, for example, counting occurrences, giving more weight to more frequent events, and ignoring isolated incidences. 23 In addition, computer programmes are said to fix and label categories during the analysis process and the researcher may be reluctant to change these categories. 9 Furthermore, the researcher is required to learn the computer programme, which may add to the time and effort he or she will need to spend on the research project. The researcher also has to be aware of the limitations of computer programmes. While computer packages can help with the intensive process of analysis and the management of large data sets, they are not a substitute for “immersion” in the data, and thorough knowledge that can enable the researcher to make comparisons, identify patterns and develop interpretations. 6 There are different packages available: Ethnograph, Atlas and NUD.IST are the mostly used. QSR NVivo is a new product developed by the makers of NUD.IST and is user-friendlier, more suitable for individual research projects and more visually attractive than previous packages.
THE ROLE OF THE RESEARCHER IN QUALITATIVE RESEARCH
Patton 12 states that, “the human element in qualitative research is both its strength and weakness”. It is considered a point of strength because it allows human insight and experience to develop new understandings of the world, and a point of weakness because it depends heavily on the researcher’s skills, creativity, training and intellect. Qualitative methods depend on both critical and creative thinking and the balance between the two in conducting the study and interpreting its results. The role of the researcher is approached differently according to the type of research tradition used. A phenomenologist researcher is required to bracket his/her own assumptions when collecting data. On the other hand, in an ethnographic study, the effect of the researcher on the interview and the interaction is seen as inevitable and important in shaping the results of the study.
Although practising clinicians routinely interview patients during their clinical work, interviewing is a well-established technique in sociology and related disciplines. One of the differences between clinical and research-aimed interviews lies in their different purposes. The usual aim of the clinical interview is to fit the patient’s problem into the appropriate medical category for diagnosis and management. On the other hand, the aim of a qualitative research interview is to discover the interviewee’s own meaning and avoid prior assumptions and preset categories. 22 Having said that, there are general skills in clinical interviewing that can be useful, such as listening and observation. A good level of self-awareness is necessary in the researcher in order to reduce possible biases.
Researchers in qualitative research need to consider how they are perceived by interviewees and the effect of features related to the researcher, such as class, race, and sex on the interview. This question is more important if the interviewee knows that the interviewer is a doctor. It has been described that a patient, or someone who is likely to be become one, may give what he or she thinks is a desirable response, thinking that the doctor will be pleased.
RIGOUR IN QUALITATIVE RESEARCH
Health field research is generally quantitative and based on biomedical traditions and experimental methods. In this field, qualitative research is criticized for being subject to researcher bias and for lacking reproducibility and generalisability. 10 Researchers presenting their qualitative work in health-related research are partly responsible for this view. Many qualitative researchers neglect the importance of giving an adequate description of their theoretical concepts and methods used in their research. A systematic research method is also essential when conducting qualitative research. Rigour in qualitative research includes procedures taken at different stages of the research process including during data collection and analysis. Several procedures have been described to increase rigour in qualitative research. For example, triangulation is commonly used as a way of validating of data.
TRIANGULATION
In triangulation, the researcher uses multiple methods, sources, researchers or theories to provide evidence that strengthens his or her study. Triangulation provides different ways of looking at the same phenomenon and adds credibility and confidence in the conclusions drawn from the study. There are two main types of triangulation, triangulation of sources and analyst triangulation. Patton 12 defines triangulation of sources as “checking out the consistency of different data sources within the same method”. When using this type of triangulation, the researcher compares the perspectives of people from different points of view. For example, studies in programme evaluation might compare the views of staff, clients or funding bodies. 12 Studies in health care have used this method of verification to study the accounts of doctors, patients, and managers in order to identify similarities and differences in views. An example is the study of patients’ versus doctors’ agendas in general practice. 23 Both similarities and differences from different sources, when given reasonable explanation, could contribute significantly to the credibility of the findings. Triangulation with multiple analysts can also be used as a method of verification. It is defined as “having two or more persons independently analyse the same qualitative data and compare their findings”. 12
QUALITATIVE RESEARCH IN HEALTH CARE MANAGEMENT
Recently, there has been a greater acceptance of the qualitative approach, even as a stand-alone method, in health care research. Institutions that control funding for medical research have developed ethical guidelines for assessing qualitative studies which indicates formal acceptance of this form of research within an area previously dominated by quantitative methods. 13 More qualitative research articles are published in health-related journals, in addition to a new qualitative research journal (Qualitative Health Research).
Quality of health care is one of the areas where qualitative methods can be used. The concept of quality in health care is multidimensional and multifaceted and some of the questions asked related to the quality of care or services may not be acquiescent to quantitative methods. 6 Qualitative research offers a variety of methods to be used for identifying what is really important to both patients and carers. It can also be used to identify and detect obstacles to change and the reasons why improvement does not occur. 6 It is therefore an essential component of health services research because it enables us to reach areas not amenable to quantitative research, for example, lay and professional health beliefs. In addition, qualitative description can be a prerequisite of quantitative research, particularly in areas that have received little previous investigation.
Qualitative research is widely used to study issues related to doctor-patient interaction especially in general practice. Studies concerning patients’ versus doctors’ agendas in general practice and general practitioners perceptions of effective health care are examples. 23 , 24 Recently there have been more studies concentrating on patients’ own perceptions and views regarding their health and health care services, for example a study on women’s views on the impact of operative delivery in the second stage of labour. 25 Another example is the study on middle-aged person’s experience of living with severe heart failure. 26
Another area where qualitative research is being used in health care is to identify obstacles and barriers to practice change by exploring the reasons behind certain behaviours. A good example of this is the study of patients’ decisions about whether or not to take anti-hypertensive drugs. 27 There are similar studies on issues such as the use of antibiotics in general practice and patient compliance.
In addition to issues related to the patients’ perception, some qualitative studies concentrated on factors fostering the doctor’s motivation and the effect of doctors’ social life and culture, in addition to issues related to the doctor’s own health. Examples are the study by Dumelow et al. 28 on the relation between a career and family life for English hospital consultants. Another example is a study aimed at exploring general practitioners’ perceptions of the effects of their profession and training on their attitudes to illness in themselves and colleagues. 29
Qualitative work can help in identifying cultural and social factors that affect health care positively or negatively. Such information can be helpful in improving service delivery. 6 Studies on patients from ethnic minorities have identified administrative and language barriers that affected health care and shed light on some of the beliefs and behaviours of these patients that might have affected help seeking and compliance. Good examples are the studies by Bush et al. 30 exploring the influences on smoking in Bangladeshi and Pakistani adults in the UK, and the multi-centre cross-cultural postnatal depression study. 31
There has been an increasing interest and use of qualitative research methods in primary health care and general practice articles. Britten 32 states that “the nature of general practice is such that a variety of research methods are needed to explore all its intricacies” He adds that qualitative methods can enrich research in general practice by opening up areas not amenable to quantitative methods, topics such as patient satisfaction, doctor-patient interaction, in addition to identifying and explaining attitudes, beliefs and behaviour. In addition, qualitative research has been used in the assessment of new technology methods used in health care. For example, the studies on the implementation of the National Health System information technology programme in the UK. 11 , 33
Qualitative research methods are receiving an increasing recognition in health care related research. The use of qualitative research in health care enables researchers to answer questions that may not be easily answered by quantitative methods. Moreover, it seeks to understand the phenomenon under study in the context of the culture or the setting in which it has been studied, therefore, aiding in the development of new research instruments, such as questionnaires that are more culturally acceptable. However, because health care related research has, for decades, been based on quantitative methods, the introduction of a new method requires researchers in health care who attempt to use it, to have a thorough understanding of its theoretical basis, methodology and evaluation techniques.
Acknowledgments
I would like to thank Sultan Qaboos University, Oman, for granting me a scholarship to do my PhD at the University of Nottingham, UK. I am also grateful to my PhD supervisor, Dr Margaret Oates, for her guidance and support.
- Policy and Social Care Move Fast: How…
Policy and Social Care Move Fast: How Rapid Qualitative Methods Can Help Researchers Match Their Pace
Date posted:.
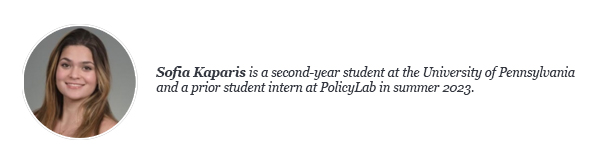
The field of social care integration, which refers to the study and implementation of clinically based programs to address the social needs of patients and families, is advancing at an increasingly rapid pace. This acceleration, driven by heightened need post-pandemic as well as mandates at the state and federal levels for health systems to implement screening and referral programs, has increased the urgency for high-quality evidence to support policy decisions about the delivery of social care—in other words, how health systems identify and address social needs, like access to healthy food and safe housing.
Qualitative research is particularly useful in guiding social care integration as it can shed light on the patient or caregiver experience of participating in social care interventions, barriers to getting help that should be addressed, and appropriate next steps from the perspective of those directly impacted.
However, traditional qualitative data analysis can be time consuming, and evidence-based solutions for addressing families’ social needs from the clinical setting are needed in the short term. In this post, I’ll share how we adapted and applied rapid qualitative methods to a social care-focused study as an example of how this approach can be used to inform social care integration in real time.
Integrating a Rapid Research Approach
The Socially Equitable Care by Understanding Resource Engagement ( SECURE ) study is a mixed method pragmatic trial aimed at understanding how best to increase family-level engagement with social resources from the pediatric health care setting. Caregivers in the study were randomized to complete one of three different social assessments (surveys asking about their social circumstances and/or desire for social resources) before receiving a resource map on their personal smartphone where, if interested, they could search for community resources in their neighborhood. Caregivers also had the option of talking to our study-specific resource navigator to receive additional support finding resources.
The overall goal of the qualitative component of the study is to capture caregivers’ preferences and experiences receiving social care through SECURE. Our traditional qualitative protocol involved transcribing caregiver interviews verbatim, coding transcripts and conducting thematic analysis. Recognizing the need for implementation-oriented results on a fast timeline, our team explored rapid qualitative methodologies to supplement the traditional approach. The rapid methods we chose were derived from existing literature on rapid qualitative approaches, which were then adapted to suit our study’s protocol and the social care field in general.
In our rapid approach, interviewers took notes using a structured template during or immediately after each caregiver interview. The template was designed to capture the data most salient to social care integration efforts such as caregiver’s likes, dislikes and preferences about receiving social care at their child’s doctor’s office. Then, content from the templates was transposed onto an analytic matrix, where we compared data across participants to identify themes. While we explored the full range of themes that emerged from our caregiver interviews in traditional qualitative analysis, we wanted to be sure that rapid analysis focused on findings that would be most applicable to social care integration efforts so the results could inform social care policy at Children’s Hospital of Philadelphia (CHOP) and elsewhere in real time. For example, what parts of participating in SECURE were helpful for caregivers? Did anything make them uncomfortable?
To ensure that our rapid approach produced results in line with those generated through traditional methods, we analyzed ten of our interviews using both traditional and rapid methods and compared the results. This analysis yielded a 92.8% theme match—meaning the two qualitative methods yielded largely the same themes. This builds upon previous literature, indicating that rapid analysis can be an effective tool in capturing implementation-oriented themes from qualitative data.
How the SECURE Study Can Inform Future Research Efforts
Our rapid qualitative methods allowed us to effectively adapt and respond to the quickly evolving landscape of social care integration, even before we had the full study results. I personally saw this first-hand while working with the SECURE team in 2023 conducting caregiver interviews. For example, we were able to inform hospital efforts in response to a recent insurance requirement of health systems to share caregivers' responses to social screening questions. We successfully gathered patients’ feedback on this new requirement and shared this information and suggestions for what CHOP could do to make caregivers feel more comfortable answering social assessment questions.
While not intended to replace traditional qualitative analysis, being able to produce actionable qualitative findings in a timely manner through rapid methods has allowed SECURE findings to help shape social care interventions at CHOP and beyond in real time.
Our hope is that other researchers in social care who face time pressures may find similar rapid qualitative methods as a useful and effective approach to adapt to the dynamic nature of the field and generate family-centered solutions faster than would otherwise be possible.
Latest Blog Posts
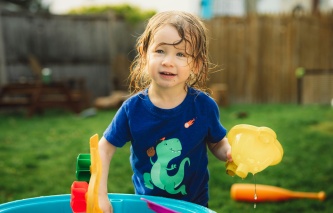
The Heat Is Turned Up: Focus on Climate Solutions for Kids
2023 was the hottest year on record and 2024 is predicted to rank among the top five warmest years. Extreme heat at this scale can be a danger to not…
A Sense of Purpose Can Support Teen Mental Health
Adolescence–the years between about 10 to about 25–is an essential time to help young people proactively build positive mental health and well-being…
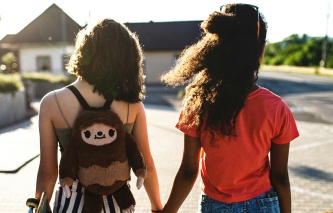
Q&A: Engaging Communities to Alleviate Period Poverty with Lynette Medley
There are 16.9 million women, girls and all other people who experience a menstrual cycle in the United States living in poverty, many of whom are…
Check out our new Publications View Publications
Adolescent Health & Well-Being
Behavioral health, population health sciences, health equity, family & community health.
Robert D. and Patricia E. Kern Center for the Science of Health Care Delivery
Applied machine intelligence.
The Applied Machine Intelligence Program in the Mayo Clinic Kern Center for the Science of Health Care Delivery develops innovative practice solutions using advanced computational and machine learning methods. The team optimizes the capacity of Mayo Clinic's hospital and clinical service and increases Mayo's ability to care for patients with serious conditions who need complex care. At the same time, the program team prioritizes workforce well-being in every project across diverse practice areas.
In the Applied Machine Intelligence Program, experts use visualization methods and computational data from biological and clinical knowledge domains to develop new theories and algorithms for machine intelligence. Team members engage in research across all facets of machine learning, including:
- Classical machine learning algorithms.
- Deep graph learning.
- Deep learning.
- Immersive intelligence.
- Large language models.
- Natural language learning.
- Probabilistic inference.
Program experts explore the feasibility, efficiency and effectiveness of translating these technologies into clinical practice.
Solutions developed within the Applied Machine Intelligence Program support Mayo Clinic's multidisciplinary medical care by facilitating point-of-care solutions personalized for each patient — no matter how complex the individual's care needs. The program creates these solutions by drawing on knowledge and data from hundreds of millions of patient characteristics and care episodes.
The program develops intelligent machines that produce solutions — and then improve on those solutions — without direct human intervention. Team members aim to integrate their advanced artificial intelligence solutions into clinical practice, thereby transforming healthcare for today and tomorrow.
Focus areas
The Applied Machine Intelligence Program is focused on:
- Safety. Identifying and characterizing patient safety hazards and providing point-of-care recommendations for alternative care pathways that could lead to better outcomes and patient experiences.
Affiliations
The Applied Machine Intelligence Program works with partners across Mayo's clinical practice. Recent collaborators include:
- Department of Obstetrics and Gynecology
- Department of Surgery
- Division of Endocrinology, Diabetes, Metabolism, and Nutrition
- Division of Medical Oncology
The program has multiple collaborative projects in development. Research aimed at advancing "intelligent" technology is designated with an "i" at the beginning of the project name. Projects include:
- iMidWife. An end-to-end artificial intelligence system for labor and delivery that supports obstetrics staff and patients for a safer and more comfortable birth experience.
- iPCa. Use of extended reality — which can include virtual, augmented or mixed reality technologies — for risk assessment and psychosocial support among patients with prostate cancer.
- iRareGene. Large language models for identifying patients with rare genetic disorders, which could shorten their diagnostic journeys and lead to more informed decision-making.
- iRemoteCare. Artificial intelligence-based trajectory models for clinical decompensation in intensive care units and among patients receiving remote monitoring care.
- LLaMPS. A new large language model for patient safety along the entire continuum of care.
Che G. Ngufor, Ph.D.
- Scientific Director
- Email: [email protected]
More about research at Mayo Clinic
- Research Faculty
- Laboratories
- Core Facilities
- Centers & Programs
- Departments & Divisions
- Clinical Trials
- Institutional Review Board
- Postdoctoral Fellowships
- Training Grant Programs
- Publications
Mayo Clinic Footer
- Request Appointment
- About Mayo Clinic
- About This Site
Legal Conditions and Terms
- Terms and Conditions
- Privacy Policy
- Notice of Privacy Practices
- Notice of Nondiscrimination
- Manage Cookies
Advertising
Mayo Clinic is a nonprofit organization and proceeds from Web advertising help support our mission. Mayo Clinic does not endorse any of the third party products and services advertised.
- Advertising and sponsorship policy
- Advertising and sponsorship opportunities
Reprint Permissions
A single copy of these materials may be reprinted for noncommercial personal use only. "Mayo," "Mayo Clinic," "MayoClinic.org," "Mayo Clinic Healthy Living," and the triple-shield Mayo Clinic logo are trademarks of Mayo Foundation for Medical Education and Research.
- Open access
- Published: 24 April 2024
Feasibility of a quality-improvement program based on routinely collected health outcomes in Dutch primary care physical therapist practice: a mixed-methods study
- LSF Smeekens 1 ,
- AC Verburg 1 ,
- MJM Maas 1 , 2 ,
- R van Heerde 1 ,
- A van Kerkhof 3 &
- PJ van der Wees 1
BMC Health Services Research volume 24 , Article number: 509 ( 2024 ) Cite this article
12 Accesses
Metrics details
This study evaluates the feasibility of a nine-month advanced quality-improvement program aimed at enhancing the quality of care provided by primary care physical therapists in the Netherlands. The evaluation is based on routinely collected health outcomes of patients with nonspecific low back pain, assessing three feasibility domains: (1) appropriateness, feasibility, and acceptability for quality-improvement purposes; (2) impact on clinical performance; and (3) impact on learning and behavioral change.
A mixed-methods quality-improvement study using a concurrent triangulation design was conducted in primary care physical therapist practice. Feedback reports on the processes and outcomes of care, peer assessment, and self-assessment were used in a Plan-Do-Study-Act cycle based on self-selected goals. The program’s appropriateness, feasibility, and acceptability, as well as the impact on clinical performance, were evaluated using the Intervention Appropriate Measure, Feasibility Intervention Measure, Acceptability Intervention Measure (for these three measure, possible scores range from 4 to 20), and with a self-assessment of clinical performance (scored 0–10), respectively. The impact on learning and behavioral change was evaluated qualitatively with a directed content analysis.
Ten physical therapists from two practices participated in this study. They rated the program with a mean of 16.5 (SD 1.9) for appropriateness, 17.1 (SD 2.2) for feasibility, and 16.4 (SD 1.5) for acceptability. Participants gave their development in clinical performance a mean score of 6.7 (SD 1.8). Participants became aware of the potential value of using outcome data and gained insight into their own routines and motivations. They changed their data collection routines, implemented data in their routine practice, and explored the impact on their clinical behavior.
Conclusions
This explorative study demonstrated that a quality-improvement program, using health outcomes from a national registry, is judged to be feasible.
Impact statement
This study provides preliminary evidence on how physical therapists may use health outcomes to improve their quality, which can be further used in initiatives to improve outcome-based care in primary physical therapy.
Peer Review reports
High-quality health care is defined as care that is safe, timely, equitable, effective, efficient, and patient centered [ 1 ]. Against a background of rapidly increasing healthcare costs, service restrictions, and differences in quality, there is an increasing need for initiatives to improve quality of care [ 2 ]. This has led the Royal Dutch Society for Physical Therapy (KNGF) to initiate the ‘Quality in Motion’ program, which aims to improve the effectiveness and patient centeredness of care in physical therapist practice by providing therapists with feedback on health outcomes [ 3 ]. Outcome measures include patient-reported outcomes (PROs), which are used to assess aspects of a patient’s health status coming directly from the patient. Patient-reported outcome measures (PROMs) are questionnaires or single-item scales used to assess PROs [ 4 ], and can be used to support quality improvement [ 3 ]; however, there is a clear lack of understanding about how physical therapists can best utilize feedback about PROs to improve quality of care [ 5 , 6 , 7 ].
Nonspecific low back pain (NSLBP) is one of the most common health conditions in primary physical therapist practice [ 8 , 9 ]. Based on health outcomes from a clinical registry and consensus among stakeholders (i.e., physical therapists, researchers, patients, and health insurers), Verburg et al. [ 4 ] developed a core set of PRO-based quality indicators for patients with NSLBP in primary physical therapist practice. The set was found to be useful for quality-improvement initiatives, and stakeholders reported that it added value for routine practice [ 3 , 4 ]. These outcomes can be aggregated across patients in clinical registries, providing data for managing clinical quality, benchmarking and public reporting across organizations, and in clinical research; however, their aggregated use for quality improvement was found to be suboptimal [ 10 , 11 , 12 ]. An earlier study found that electronic health record (EHR) compatibility and therapist knowledge of the PROMs are the two key barriers to wider PROM use [ 13 ], with similar issues reported in other professions [ 14 , 15 ].
Feedback interventions, particularly when provided by a colleague both verbally and in writing [ 16 ], have shown promise in improving physical therapist practice [ 17 , 18 ]. Correspondingly, feedback reporting on processes and outcomes of care has been identified as an effective intervention that can support the exchange of best practices and mutual learning [ 16 , 18 , 19 ]. Additionally, involving peers as feedback providers in peer assessment creates meaningful learning experiences and is associated with behavioral change and measurable performance improvement in healthcare professionals [ 20 , 21 , 22 ]. Maas et al. [ 23 ] showed that peer assessment using video recordings of client communication and clinical records is an effective feedback intervention method in enhancing commitment to change and improving the clinical performance of physical therapists. Furthermore, feedback interventions seem to be more effective in changing clinical behavior when including clear targets and an action plan [ 16 ]. Accordingly, the Plan-Do-Study-Act facilitates systematic testing of changes in real-world settings, allowing for rapid learning and adaptation. This approach has been effectively utilized in various healthcare studies to enhance clinical outcomes and process efficiencies [ 24 ]; however, most physical therapists are not familiar with such quality-improvement interventions based on health outcomes [ 25 ].
The aim of this study is therefore to evaluate the feasibility of an advanced quality-improvement program for physical therapists in primary care. The evaluation involves feedback, peer assessment, and self-assessment in a rapid improvement Plan-Do-Study-Act cycle, using the routinely collected health outcome data of patients with NSLBP.
Study design and setting
The program feasibility was evaluated through an explorative quality-improvement study using a mixed-methods approach in a concurrent triangulation design [ 26 ]. The following program feasibility domains were addressed [ 27 ]: (1) appropriateness, feasibility, and acceptability for quality-improvement; (2) impact on clinical performance; and (3) impact on learning and behavioral change. We used the Standards for QUality Improvement Reporting Excellence (SQUIRE) Guidelines [ 28 ]. The evaluation was conducted between January and October 2022. We tested our program in a convenience sample of Dutch primary care physical therapists organized in a regional network of communities of practice (the Cooperation of Physical Therapists Nijmegen; CFN).
Participants
All physical therapy practices within the CFN network ( n = 30) were approached to recruit therapists for the study. Invitations were extended via a digital newsletter, which included the goals of the study and contact details of the first author (LS). Physical therapists willing to participate received detailed study information by email and were screened for eligibility using the inclusion criteria below. Participation was voluntary. All participants provided written informed consent.
Inclusion criteria
Licensed Dutch physical therapists were eligible to participate in this study if they provided primary care to patients with NSLBP aged 18 years or older [ 3 , 4 ]. They also had to evaluate selected outcomes as part of a standard clinical routine in patients with NSLBP using the following PROMs (associated domain): Numeric Pain Rating Scale (NPRS) (pain intensity), Patient Specific Functioning Scale (PSFS) (physical activity), Quebec Pain Disability Scale (QBPDS) (physical functioning), Global Perceived Effect (GPE-DV) (perceived treatment effect), and STarT Back Screening Tool (SBST) (profile grouping based on risk of poor outcome) [ 3 , 4 ]. Physical therapists collected outcomes using these PROMs, which were directly recorded into their EHRs. These data were transferred to the national data registry of the Royal Dutch Society for Physical Therapy (KNGF). Additionally, to facilitate meaningful participation in the quality-improvement program, particularly during peer assessment sessions and outcome discussions, it was essential for participants to have contributed sufficient data to the national clinical registry from January 2021 to November 2021 (a minimum requirement of five patients with a closed treatment episode). An episode was considered closed when the physical therapist closed the episode in the EHR, or if six weeks had passed after the last visit. Informed consent for delivering data to the national clinical registry was obtained from every patient. This approach ensured that participants could engage with actual data reflective of their clinical practices rather than hypothetical scenarios, fostering deeper learning and reflection on professional conduct and patient care. The requirement for therapists to have already been actively collecting and submitting data as part of their clinical routine underlines the study’s aim to engage therapists who were not only familiar with the use of PROMs, but who also had sufficient data to enable a meaningful analysis and discussion within the context of the quality-improvement program.
The quality-improvement program content
The nine-month program consisted of a rapid improvement cycle comprising multiple consecutive steps and quality-improvement interventions. In step 1, participants were offered the opportunity to complete an e-learning module on using data in clinical practice [ 29 ]. In step 2, personal data exports were extracted from the national clinical registry. Participants received feedback reports on the processes and outcomes of their care in step 3 [ 30 , 31 , 32 ], then attended peer assessment meetings in step 4 [ 18 , 23 , 33 ], In step 4, the therapists drafted a rapid improvement Plan-Do-Study-Act cycle and individual quality-improvement goals [ 6 , 34 , 35 ], and in step 5, they performed a self-assessment of their clinical performance [ 36 ]. See Additional File 1 for further details of the program. The process and outcome indicators of the PROMs for patients with NSLBP were used in the program (see Additional File 2 ) [ 3 , 4 ].
Evaluation of program feasibility and outcome measures
The program’s perceived appropriateness, acceptability, and feasibility for quality-improvement purposes were evaluated using the Dutch versions of the Intervention Appropriate Measure (IAM), the Feasibility Intervention Measure (FIM), and the Acceptability Intervention Measure (AIM), respectively [ 37 ], which have been demonstrated to be valid and reliable tools [ 37 ]. Each measure consists of four items scored on a five-point Likert scale, with higher scores indicating better appropriateness, acceptability, and feasibility, respectively (scoring range: 4–20 for each tool). The impact on clinical performance was evaluated using self-assessment checklists [ 36 ] (steps 5 and 7 of the quality-improvement program), while the impact on learning and behavioral change was qualitatively determined during the peer assessment (steps 4 and 6). We used a parallel approach in collecting the quantitative and qualitative data, giving equal weight to both methods.
Data collection
Participants were invited by email to attend the peer assessment meetings. A script (see Additional Files 5 and 6 ) for each meeting was designed by the research team, addressing different quality-improvement interventions. A participatory evaluation strategy was used, allowing an assessment of the impact of the program on learning and behavioral change during the actual implementation [ 38 ]. The peer assessment meetings lasted 100–120 min and were conducted face-to-face by an external coach (RvH) using open-ended questions, which facilitated group discussion and knowledge development. A safe environment was encouraged within each peer group [ 20 , 22 ]. The peer assessment meetings were audio-taped, video-recorded, and subsequently transcribed verbatim. Written informed consent was obtained from all participants. The identities of the participants were considered confidential; therefore, the transcripts of the meetings were processed anonymously. Participants were asked to complete a self-assessment checklist halfway through the program, at the end, and six months after via email. Likewise, participants completed the IAM, FIM, and AIM at the end of the study, following the second peer assessment meeting.
Data analysis
Quantitative analysis.
The mean scores and standard deviations (SDs) of the IAM, FIM, and IAM were calculated. For the quality-improvement program to be considered appropriate, feasible, and acceptable [ 37 ], a minimum mean score of 15 out of 20, averaged over all participants, was required for each measure. The mean scores and SDs were calculated separately for the self-assessment checklists at three timepoints. For the quality-improvement program to be considered to impact the development of clinical performance, a minimum mean score of 5 out of 10 was required [ 36 ], averaged over all competed self-assessment checklists. Our comparative analysis focused on the mean scores and differences in process and outcome indicators between two periods: the pre-improvement period (the 12 months before the start of the study) and the quality-improvement period (the nine months after the study began). The latter period integrates data from both the initial and subsequent phases of the quality-improvement program, reflecting insights consolidated from the two feedback reports received by the participants during the program (Fig. 1 ). Our analysis focused exclusively on complete case episodes with both baseline and endpoint measurements to ensure the integrity and applicability of the data for participation in the quality-improvement program. All quantitative data were analyzed using SPSS Statistics, version 26 (IBM, Armonk, New York, USA).
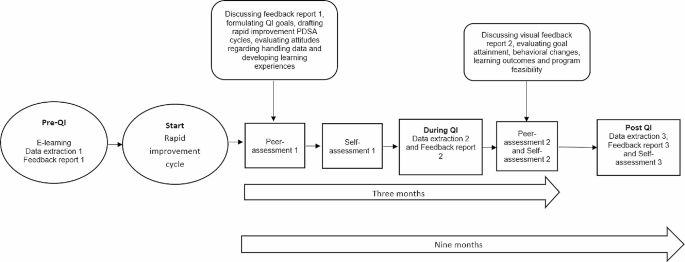
The structure of the quality-improvement (QI) program QI = quality improvement; PDSA = Plan-Do-Study-Act
Qualitative analysis
Transcripts of the peer assessment meetings were read in detail, and a directed content analysis was used to study them [ 39 , 40 ]. A codebook was developed in advance, informed by the research questions. Text fragments were labeled according to these a priori codes, which were further refined during the coding process. Meaningful text fragments that could not be labeled were coded inductively. The transcript analysis was supported by ATLAS.ti version 8.4 [ 41 ]. Two researchers (LS and AvK) independently coded the transcripts, discussed the codes to reach consensus, and created the codebook, allocating codes into categories based on their similarities [ 42 ]. A researcher (MM) with ample experience in peer assessment and qualitative research guided this process. Kirkpatrick’s model, which was designed to evaluate the impact of an educational program, was used to allocate the identified categories to four domains: reaction, learning, behavior, and results (see Additional File 7 ) [ 43 ]. Preliminary findings after both peer assessment meetings and the final codes, categories, and the allocation of categories to the domains were discussed by the research team (LS, MM, RvH, AV, and PvdW) in several meetings. A member checking procedure was conducted by sending a summary with preliminary results to all participants after the first meeting to increase the credibility of the results. To optimize the transferability of the results, we aimed to saturate the information by recruiting at least three peer groups.
In total, 10 physical therapists from two different practices participated in the program. Two mixed-practice peer groups were formed, each consisting of five participants. The participants’ characteristics are outlined in Table 1 .
Quantitative results
Table 2 provides an overview of the appropriateness, feasibility, and acceptability of the program, as well as the perceived development in clinical performance. All predefined criteria regarding the minimum score on the IAM, FIM, AIM, and the self-assessment checklists were met.
The mean process and outcome indicator scores for the three data periods are compared in Table 3 . All process indicators improved substantially during and after the quality-improvement cycle, with mean improvements ranging from 9 to 26%.
Qualitative results
We conducted four peer assessment meetings, two for each peer group. After analyzing the qualitative data, the codes were classified into eight major categories. These categories were allocated to the four domains of Kirkpatrick’s model of evaluation (see Table 4 ). Quotes are numbered and labeled by peer group (see Table 5 ).
Domsain: reaction
Program appreciation; suggestions for program improvement.
Participating in a quality-improvement program based on routinely collected health outcomes was novel for most participants. In general, the therapists considered the program’s content meaningful, pleasant, acceptable, and accessible (Q1-G2), and proposed several advancements to increase future program experiences and satisfaction (Q2-G1)(Q3-G1).
Domain: learning
Awareness and insight.
Most participants became more aware of the existing data and the possibilities for analyzing and comparing them. They developed an understanding of the clinical relevance of the data presented, and identified possible explanatory factors by interpreting and clarifying the data (Q4-G1). Participants also gained insight into how to appropriately design data collection, the importance of proper data collection methods (Q5-G1), and potential areas for implementing data in routine practice.
Participants became more aware of data collection throughout the quality-improvement cycle, but acknowledged the lack of a standardized, valid, and reliable data collection method (Q6-G1). Before the quality-improvement program, most participants did not routinely use data to guide and improve their practice, despite dedicating considerable effort to its collection (Q7-G1).
The evolving knowledge gained from the quality-improvement cycle led participants to realize that routinely implementing data can enhance their clinical practice, and more importantly can significantly benefit patients (Q8-G2). Some participants openly argued that using data will not improve the quality of their physical therapy. They challenged the perceived value of the data in comparison with their own expertise and discussed the required time investment in relation to the perceived returns.
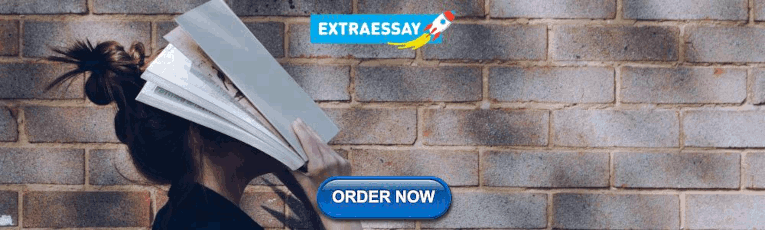
Motivational change
Collecting and using data with the objective of improving quality of care for the patient was not a common mindset among participants. Instead, data collection was performed to meet obligatory external requirements and was not considered a priority. However, as the quality-improvement cycle continued, most participants reported a shift to more intrinsically motivated efforts for collecting data (Q9-G2).
Domain: Behavior
Intentions for behavioral change.
Participants were encouraged to reflect on their own clinical behavior and reported feeling motivated to change their routine practice. All participants planned to improve their process indicators and data collection routines, particularly by allowing patients to complete their own questionnaires. Some participants proposed integrating the data into their practice and investigating its impact on their clinical behavior (Q10-G2).
Demonstrated behavioral change
All participants revised the extent and approach of their data collection. Most participants successfully applied some form of data use in routine practice, such as to evaluate treatment progress, to guide treatment and decision-making processes, as input for taking patient histories, for patient empowerment, for goal setting with the patient, and to complement or contradict their own assumptions (Q11-G2). Although they changed their data collection routines, two participants admitted they still rarely used data to support their clinical behavior (Q12-G1).
Barriers to and facilitators of behavioral change
Participants identified several barriers and facilitators that hindered or helped them to achieve their intended behavioral changes (Q13-G2)(Q14-G2)(Q15-G1) (see Table 6 ). These factors impacted the quantity of data collected, influenced the data collection protocols used, and shaped efforts to integrate data into routine practice.
Domain: results
Goal attainment.
The majority of participants set goals related to processes and collection routines. Seven of the 10 participants accomplished their personal targets regarding improving process indicators (Q16-G2). All participants achieved their objectives around changing data collection routines. One participant openly debated the benefit of goal attainment on the added value and quality of care for the patient (Q17-G1).
This study explored the feasibility of a quality-improvement program designed to enhance the quality of primary care physical therapists. The program uses health outcomes from a national registry and incorporates feedback, peer assessment, and self-assessment in a Plan-Do-Study-Act cycle. We found that the participants considered the program an appropriate, feasible, and acceptable intervention for quality-improvement purposes, and found it beneficial for improving their clinical performance. All participants improved the completeness of the data they collected. They also gained insights into the potential value of using outcome data in clinical practice, as well as in examining their routines and motivation. Participants recognized the importance of handling data, revised their data collection methods, began to implement data use into their routine practice, and observed the impact on their clinical behavior. They acknowledged the added value of using data when formulating clear treatment targets, monitoring treatment processes, motivating patients, and, on an aggregated level, improving the quality of care. While most participants reacted positively to the program and acknowledged its added value, they faced significant challenges, such as the complexity of integrating systematic data collection into daily practice, external pressures to meet specific outcome benchmarks, and the need for more knowledge and skills in data interpretation and application. These factors sometimes hindered the full realization of the program’s benefits and highlighted areas for improvement that should be addressed to improve the program before wider implementation.
Comparison to similar studies
This study builds upon previous research that highlighted the potential value of outcome data in quality-improvement initiatives [ 4 , 24 ]. When evaluating the potential value of feedback, peer assessment, self-assessment, and Plan-Do-Study-Act cycles in physical therapist care, most previous studies did not use aggregated real-world data from clinical registries. Maas et al. [ 23 ] and Steenbruggen et al. [ 36 ] incorporated feedback, peer assessment, and self-assessment in comprehensive quality-improvement programs aimed at the professional development of physical therapists, using client records, video recordings of client communication, and the tracer methodology, respectively. Both programs were found to be feasible and led to improvements in clinical performance [ 23 , 36 ]. The results of the present study support and extend previous findings of these quality-improvement strategies in physical therapist practice.
During the initial peer assessment meeting, the participants gained new knowledge and became more conscious of their own behavior. These findings are consistent with previous research indicating that peer assessment promotes learning, increases self-awareness [ 22 , 44 ], and builds self-concept [ 45 , 46 ]. Additionally, participants developed a critical perspective regarding their daily routines and expressed a desire to change their behavior. A similar enhanced commitment to change was reported by physical therapists who underwent cycles of peer assessment and self-assessment [ 23 ]. These findings are in line with theories of health behavior, which suggest that all behavioral change begins with recognizing one’s own behavior [ 47 ], and with the intention to change [ 48 ].
Another important finding was the observed shift in motivation for collecting data. Prior to the quality-improvement program, data were often collected in a non-validated manner, driven by external factors such as health insurers, and were not used to improve patient care. This is consistent with previous findings that the use of feedback in quality improvement is hindered by a perceived political motive for public reporting rather than improved patient care [ 7 ], by financial incentives from health insurers [ 49 ], and by a lack of experience and skills [ 7 , 50 ]. Instead of collecting data to meet an external goal, most participants moving along the quality-improvement cycle reported a shift to a more intrinsic motivation. This could be attributed to participants giving new meaning to collecting and handling data in their daily practice, and establishing their own personal values. These findings are consistent with Ryan and Deci’s self-determination theory, which states that the basis for intrinsic motivation and behavior is formed by people finding a rationale within themselves [ 51 ]. Indeed, participants in the current study emphasized the importance of having clear self-directed motives for data collection as a key driver of behavioral change. Consistent with this, healthcare providers previously reported being more likely to take steps for quality improvement in response to the feedback of aggregated PROMs if they perceived these data to be credible and beneficial for improving patient care [ 19 ]. Throughout the quality-improvement cycle, learning and understanding of data management continued to be developed through experience and reflection, in line with Dewey’s experiential learning theory [ 52 ].
All participants made self-initiated behavioral changes during the program, which was believed to be supported by the application of knowledge gained by following the Plan-Do-Study-Act cycle [ 24 ]. Setting specific targets and making an action plan may increase the effectiveness of feedback and facilitate behavioral changes [ 18 ]. In the present study, feedback was provided by a colleague, more than once, both verbally and in writing to further increase its effectiveness. The participants were largely successful in changing their data-collection procedures; however, there is still room for improvement in the use of data in routine daily practice. Previous studies have shown that clinicians find PROMs useful for supporting the therapeutic process [ 19 ]; however, it took more time or effort to develop these application skills than was available within the timespan of the program. This assumption is supported by the feedback intervention theory, which assumes that the effectiveness of feedback is lower when the ‘task novelty’ and ‘task complexity’ are higher [ 53 ]. Indeed, participants mentioned a lack of knowledge and skills regarding data application as important barriers to its use. Feeling competent is very important for accomplishing behavioral change, according to the self-determination theory [ 51 ]. Correspondingly, previous research indicated that healthcare providers need more support and guidance on how to structurally implement data into their daily practice [ 19 ].
Strengths and limitations
In this explorative study, an innovative theory- and evidence-based quality-improvement program was developed and implemented in daily physical therapy practice. Integrating multiple proven quality-improvement interventions, combined and informed by outcome data, clearly contributed to the inventive character of this program. Using a participatory strategy for the evaluation of program feasibility during the implementation enhanced the evaluation relevance, as well as providing valuable information regarding the program’s beneficial features and suggestions for improvements from the direct perspectives of the intended end-users. Using both qualitative and quantitative data in a concurrent triangulation design also contributed to the rigor of this study.
This study has several limitations. First, although we intended to include three peer groups for data saturation, only two were ultimately recruited. This could have impacted the validity and transferability of the results. Despite this, the two peer groups provided us with rich data that were deemed sufficient for program evaluation and feasibility study purposes [ 54 ]. Second, the peer groups were comprised of physical therapists selected based on the amount of data they collected. As all participants needed to meet external requirements regarding data collection, they could be seen as early adopters. The voluntary participation and external motivation of the participants may have influenced the results and may limit generalizability to other physical therapists. Third, indicative of its exploratory nature, the study’s sample size was limited, but was deemed sufficient to address our research questions. Additionally, the gender distribution among participants, with nine out of 10 being male, does not reflect the typical gender distribution in primary care physiotherapy in the Netherlands. This discrepancy was unintentional, emerging from the recruitment process, but could nevertheless constitute a selection bias, and underscores the need for caution when generalizing findings across diverse physiotherapy contexts. Lastly, although the coach promoted a safe environment during the group meetings, they were not anonymous, and participants may have felt unable to talk openly. Alongside the fact that the assessments could not be blinded, this may have introduced social desirability bias.
Implications for research and practice
Our findings can be used by national physical therapist bodies and other stakeholders in the field to develop initiatives for improving outcome-based care. This program is well suited for use in primary physical therapy care as it integrates with the peer assessment methodology commonly used in many practices. Such integration minimizes the opportunity costs usually associated with new initiatives by leveraging existing peer-learning and feedback structures, making it a feasible and cost-effective strategy for quality improvement [ 55 ]. Additionally, recommendations for advancing the national clinical data registry may further improve the usability for end-users and future researchers, who may wish to study whether the findings are also generalizable to other primary care physical therapist practices. In this study, feedback reporting appeared to support the establishment of quality-improvement goals, and future research could investigate the value of these strategies in evaluating results and changing clinical practices. The sustainability of the observed participant’s behavioral changes and their translation of their revised data-collection routines into quality improvements in care require further consideration. Future studies could improve the program’s feasibility by directly addressing the identified facilitators. Additionally, the program’s impact on patient outcomes should be explored in a full-scale study with long-term follow up.
This explorative study demonstrated that a quality-improvement program incorporating feedback, peer assessment, and self-assessment in a Plan-Do-Study-Act cycle, and using health outcomes from a national registry, was deemed feasible for quality improvement. The implementation of the program led to knowledge development, perceived improvements in clinical performance, and a change in the behavior of the physical therapists regarding data handling in their routine practice.
Data availability
The datasets used and/or analyzed during the current study are available from the corresponding author upon reasonable request.
Abbreviations
Acceptability Intervention Measure
Cooperation of Physical Therapists Nijmegen
Electronic health system
Feasibility Intervention Measure
Global Perceived Effect
Intervention Appropriate Measure
Royal Dutch Society for Physical Therapy
- Nonspecific low back pain
Numeric Pain Rating Scale
Patient-reported outcome measures
Patient-reported outcomes
Patient Specific Functioning Scale
Quebec Pain Disability Scale
STarT Back Screening Tool
Standard deviation
QUality Improvement Reporting Excellence
Spath P, Kelly DL. Applying quality management in healthcare: a systems approach. Health Administration Press Chicago; 2017.
Porter ME. Value-based Health care Delivery. 2008;248:503–9.
Google Scholar
Meerhoff GA, Verburg AC, Schapendonk RM, Cruijsberg J, Nijhuis-van der Sanden MW, van Dulmen SA et al. Reliability, validity and discriminability of patient reported outcomes for non-specific low back pain in a nationwide physical therapy registry: a retrospective observational cohort study 2021;16:e0251892.
Verburg AC, van Dulmen SA, Kiers H, Nijhuis-van der Sanden MW, van der Wees PJ. Patient-reported outcome-based quality indicators in Dutch primary care physical therapy for patients with nonspecific low back Pain. A Cohort Study; 2021.
Swinkels RA, Meerhoff GM, Custers JW, van Peppen RP, Beurskens AJ, Wittink H. Using outcome measures in daily practice: development and evaluation of an implementation strategy for physiotherapists in. Neth. 2015;67:357–64.
van Dulmen S, van der Wees P. Eindrapport toepassing van minimale dataset en kwaliteitsindicatoren voor lage rugklachten in de fysiotherapie n.d.
Greenhalgh J, Dalkin S, Gibbons E, Wright J, Valderas JM, Meads D et al. How do aggregated patient-reported outcome measures data stimulate health care improvement? A realist synthesis. 2018;23(1):57–65.
Koes BW, Van Tulder M, Lin C-WC, Macedo LG, McAuley J, Maher C. An updated overview of clinical guidelines for the management of non-specific low back pain in primary care. 2010;19(12):2075–94.
Koninklijk Nederlands Genootschap voor Fysiotherapie (KNGF). / vereniging van Oefentherapeuten Cesar en Mensendieck (VvOCM). KNGF-richtlijn Lage rugpijn en lumbosacraal radiculair syndroom. Amersfoort/Utrecht: KNGF/VvoCM; 2021. n.d.
Copeland JM, Taylor WJ, Dean SG. Factors influencing the use of outcome measures for patients with low back pain: a survey of New. Z Phys Therapists. 2008;88(12):1492–505.
Jette DU, Halbert J, Iverson C, Miceli E, Shah P. Use of standardized outcome measures. Phys Therapist Practice: Perceptions Appl. 2009;89(2):125–35.
van Dulmen SA, van der Wees PJ, Staal JB, Braspenning J, Nijhuis-van der Sanden MW. Patient reported outcome measures (PROMs) for goalsetting and outcome measurement in primary care physiotherapy. Explorative Field Study. 2017;103:66–72.
Meerhoff GA, van Dulmen SA, Cruijsberg JK, Nijhuis-van der Sanden MW, Van der Wees PJ. Which factors influence the use of patient-reported outcome measures in Dutch physiotherapy practice? A cross-sectional study. 2020;72(1):63–70.
Irizarry T, DeVito Dabbs A, Curran CR. Patient portals and patient engagement: a state of the science review. 2015;17(6):e148.
Sorondo B, Allen A, Bayleran J, Doore S, Fathima S, Sabbagh I et al. Using a patient portal to transmit patient reported health information into the electronic record: workflow implications and user experience. 2016;4(3).
Ivers N, Jamtvedt G, Flottorp S, Young JM, Odgaard-Jensen J, French SD et al. Audit and feedback: effects on professional practice and healthcare outcomes. 2012.
van der Wees P, van der Vleuten C. Marjo Maas Ria Nijhuis–van der Sanden Geert Rutten Yvonne Heerkens n.d.:187.
Gibbons C, Porter I, Gonçalves-Bradley DC, Stoilov S, Ricci-Cabello I, Tsangaris E et al. Routine provision of feedback from patient-reported outcome measurements to healthcare providers and patients in clinical practice 2021.
Greenhalgh J, Dalkin S, Gooding K, Gibbons E, Wright J, Meads D et al. Functionality and feedback: a realist synthesis of the collation, interpretation and utilisation of patient-reported outcome measures data to improve patient care. 2017;5:1–280.
Maas MJ, Nijhuis-van der Sanden MW, Driehuis F, Heerkens YF, van der Vleuten CP, van der Wees PJ. Feasibility of peer assessment and clinical audit to self-regulate the quality of physiotherapy services: a mixed methods study 2017;7:e013726.
Maas MJ, van der Wees PJ, Braam C, Koetsenruijter J, Heerkens YF, van der Vleuten CP et al. An innovative peer assessment approach to enhance guideline adherence in physical therapy: single-masked, cluster-randomized controlled trial. 2015;95(4):600–12.
Phillips Fox D. Peer review of health care professionals: a systematic review of the literature. Melbourne. 2009. https://www.safetyandquality.gov.au/wp-content/uploads/2012/01/25738-LitReview.pdf (accessed June 2022).
Maas MJ, Driehuis F, Meerhoff GA, Heerkens YF, van der Vleuten CP, Nijhuis-van der Sanden MW et al. Impact of self-and peer assessment on the clinical performance of physiotherapists in primary care: a cohort study 2018;70:393–401.
Kampstra NA, Zipfel N, van der Nat PB, Westert GP, van der Wees PJ, Groenewoud AS. Health outcomes measurement and organizational readiness support quality improvement: a. Syst Rev. 2018;18:1–14.
McDonald PL, Foley TJ, Verheij R, Braithwaite J, Rubin J, Harwood K et al. Data to knowledge to improvement: creating the learning health system. 2024;384.
Creswell JW, Plano Clark VL, Gutmann ML, Hanson WE. An expanded typology for classifying mixed methods research into designs 2003:209–40.
Bowen DJ, Kreuter M, Spring B, Cofta-Woerpel L, Linnan L, Weiner D, et al. How we Des Feasibility Stud. 2009;36:452–7.
Ogrinc G, Armstrong GE, Dolansky MA, Singh MK, Davies L. SQUIRE-EDU (standards for QUality Improvement Reporting Excellence in Education): publication guidelines for educational improvement. 2019;94(10):1461.
https://www.npi.nl/cursussen-en-opleidingen/e-learning-en-toetsen/13025-e-learning-hoe-gebruik-ik-data-in-de-praktijk . n.d.
Krägeloh CU, Czuba KJ, Billington DR, Kersten P, Siegert RJ. Using feedback from patient-reported outcome measures in mental health services: a scoping study and typology. 2015;66:224–41.
Hildon Z, Neuburger J, Allwood D, van der Meulen J, Black N. Clinicians’ and patients’ views of metrics of change derived from patient reported outcome measures (PROMs) for comparing providers’. Perform Surg. 2012;12:1–13.
Hildon Z, Allwood D, Black N. Making data more meaningful: patients’ views of the format and content of quality indicators comparing health. care Providers. 2012;88:298–304.
Pronovost PJ, Hudson DW. Improving healthcare quality through organisational peer-to-peer assessment: lessons from the nuclear power industry. 2012;21:872–5.
Wakefield J, Herbert CP, Maclure M, Dormuth C, Wright JM, Legare J, et al. Commit Change Statements can Predict Actual Change Pract. 2003;23:81–92.
Rehring SMA, Steiner JF, Reifler LM, Glenn KA, Daley MF. Commitment to change statements and actual practice change after a Continuing Medical. Educ Intervention. 2021;41:145–52.
Steenbruggen RA, van Heusden-Scholtalbers LA, Hoogeboom TJ, Maas M, Brand P, van der Wees P. Impact and feasibility of a tailor-made patient communication quality improvement programme for hospital-based physiotherapists: a mixed-methods study. 2021;10:e001286.
Weiner BJ, Lewis CC, Stanick C, Powell BJ, Dorsey CN, Clary AS, et al. Psychometric Assess Three New Developed Implement Outcome Measures. 2017;12:1–12.
Cousins JB, Whitmore E. Framing participatory evaluation 1998;1998:5–23.
van Staa A, de Vries K. Directed content analysis: een meer deductieve dan inductieve aanpak bij kwalitatieve analyse 2014;19.
Kiger ME, Varpio L. Thematic analysis of qualitative data. AMEE Guide 131. 2020;42:846–54.
Friese S. Qualitative data analysis with ATLAS. Ti. Sage; 2019.
O’Connor C, Joffe H. Intercoder reliability in qualitative research: debates and practical guidelines 2020;19:1609406919899220.
Kirkpatrick D. Great Ideas Revisit. 1996;50:54–60.
van Dulmen SA, Maas M, Staal JB, Rutten G, Kiers H, Nijhuis-van der Sanden M, et al. Effectiveness of peer assessment for implementing a Dutch physical therapy low back pain guideline. Cluster Randomized Controlled Trial. 2014;94:1396–409.
Epstein RM, Siegel DJ, Silberman J. Self-monitoring in clinical practice: a challenge for medical educators 2008;28:5–13.
Daves DA, Mazmanian PE, Fordis M, Van Harrision R, Thorpe KE, Perrier L. Accuracy of physician self-assessment compared with observed measures of competence 2006;296:1094–102.
Prochaska JO, Velicer WF. Transtheoretical Model Health Behav Change. 1997;12:38–48.
CAS Google Scholar
Ajzen I. The theory of planned behavior 1991;50:179–211.
Scholte M, Neeleman-van der Steen CW, van der Wees PJ, Nijhuis-van der Sanden MW, Braspenning J. The reasons behind the (non) use of feedback reports for quality improvement in physical therapy: a mixed-method study. 2016;11(8):e0161056.
Alvarado N, McVey L, Elshehaly M, Greenhalgh J, Dowding D, Ruddle R, et al. Analysis of a web-based dashboard to support the Use of National Audit Data in Quality Improvement. Realist Evaluation. 2021;23(11):e28854.
Ryan RM, Deci EL. Self-determination theory 2017.
Dewey J, Authentic IEL. Experiential learning 1938.
Kluger AN, DeNisi A. The effects of feedback interventions on performance: a historical review, a meta-analysis, and a preliminary feedback intervention theory. 1996;119:254.
Anderson R. New MRC guidance on evaluating complex interventions. 2008;337.
Maas MJ, van Poelgeest E, Weymans C, PJvd W. Module 3 feedback op proces en uitkomsten. KNGF; 201.
Download references
Acknowledgements
The authors express their gratitude to the participating physical therapists from the regional network of the Cooperation of Physical Therapists Nijmegen (CFN).
This research received no specific grant from any funding agency in the public, commercial, or not-for-profit sectors.
Author information
Authors and affiliations.
Scientific Institute for Quality of Healthcare, Radboud university medical center, Kapittelweg 54, 6525 EP, Nijmegen, The Netherlands
LSF Smeekens, AC Verburg, MJM Maas, R van Heerde & PJ van der Wees
HAN University of Applied Sciences, Nijmegen, The Netherlands
Leidsche Rijn Julius Gezondheidscentra, Utrecht, The Netherlands
A van Kerkhof
You can also search for this author in PubMed Google Scholar
Contributions
Concept/idea/research design: LS, AV and PvdW Data analysis: LS, AvK and MM Project management: LS and AV Writing of the manuscript: LS and AV Peer assessment coach: RvH Approval of final manuscript: LS, MM, RvH, AV and PvdW.
Corresponding author
Correspondence to LSF Smeekens .
Ethics declarations
Ethics approval and consent to participate.
The study protocol was approved by the Medical Ethical Committee of Radboud university medical center (registration #2021–13329). This study complies with the Declaration of Helsinki. All participants provided written informed consent.
Consent for publication
Not applicable.
Competing interests
The authors declare that they have no competing interests.
Additional information
Publisher’s note.
Springer Nature remains neutral with regard to jurisdictional claims in published maps and institutional affiliations.
Electronic supplementary material
Below is the link to the electronic supplementary material.
Supplementary Material 1
Supplementary material 2, supplementary material 3, supplementary material 4, supplementary material 5, supplementary material 6, rights and permissions.
Open Access This article is licensed under a Creative Commons Attribution 4.0 International License, which permits use, sharing, adaptation, distribution and reproduction in any medium or format, as long as you give appropriate credit to the original author(s) and the source, provide a link to the Creative Commons licence, and indicate if changes were made. The images or other third party material in this article are included in the article’s Creative Commons licence, unless indicated otherwise in a credit line to the material. If material is not included in the article’s Creative Commons licence and your intended use is not permitted by statutory regulation or exceeds the permitted use, you will need to obtain permission directly from the copyright holder. To view a copy of this licence, visit http://creativecommons.org/licenses/by/4.0/ . The Creative Commons Public Domain Dedication waiver ( http://creativecommons.org/publicdomain/zero/1.0/ ) applies to the data made available in this article, unless otherwise stated in a credit line to the data.
Reprints and permissions
About this article
Cite this article.
Smeekens, L., Verburg, A., Maas, M. et al. Feasibility of a quality-improvement program based on routinely collected health outcomes in Dutch primary care physical therapist practice: a mixed-methods study. BMC Health Serv Res 24 , 509 (2024). https://doi.org/10.1186/s12913-024-10958-5
Download citation
Received : 27 July 2023
Accepted : 05 April 2024
Published : 24 April 2024
DOI : https://doi.org/10.1186/s12913-024-10958-5
Share this article
Anyone you share the following link with will be able to read this content:
Sorry, a shareable link is not currently available for this article.
Provided by the Springer Nature SharedIt content-sharing initiative
- Health outcomes
- Physical therapy
- Quality improvement
BMC Health Services Research
ISSN: 1472-6963
- General enquiries: [email protected]
- Open access
- Published: 22 April 2024
Training nurses in an international emergency medical team using a serious role-playing game: a retrospective comparative analysis
- Hai Hu 1 , 2 , 3 na1 ,
- Xiaoqin Lai 2 , 4 , 5 na1 &
- Longping Yan 6 , 7 , 8
BMC Medical Education volume 24 , Article number: 432 ( 2024 ) Cite this article
103 Accesses
Metrics details
Although game-based applications have been used in disaster medicine education, no serious computer games have been designed specifically for training these nurses in an IEMT setting. To address this need, we developed a serious computer game called the IEMTtraining game. In this game, players assume the roles of IEMT nurses, assess patient injuries in a virtual environment, and provide suitable treatment options.
The design of this study is a retrospective comparative analysis. The research was conducted with 209 nurses in a hospital. The data collection process of this study was conducted at the 2019-2020 academic year. A retrospective comparative analysis was conducted on the pre-, post-, and final test scores of nurses in the IEMT. Additionally, a survey questionnaire was distributed to trainees to gather insights into teaching methods that were subsequently analyzed.
There was a significant difference in the overall test scores between the two groups, with the game group demonstrating superior performance compared to the control group (odds ratio = 1.363, p value = 0.010). The survey results indicated that the game group exhibited higher learning motivation scores and lower cognitive load compared with the lecture group.
Conclusions
The IEMT training game developed by the instructor team is a promising and effective method for training nurses in disaster rescue within IEMTs. The game equips the trainees with the necessary skills and knowledge to respond effectively to emergencies. It is easily comprehended, enhances knowledge retention and motivation to learn, and reduces cognitive load.
Peer Review reports
Since the beginning of the twenty-first century, the deployment of international emergency medical teams in disaster-stricken regions has increased world wide [ 1 ]. To enhance the efficiency of these teams, the World Health Organization (WHO) has introduced the International Emergency Medical Team (IEMT) initiative to guarantee their competence. Adequate education and training play a vital role in achieving this objective [ 2 ].
Nurses play a vital role as IEMTs by providing essential medical care and support to populations affected by disasters and emergencies. Training newly joined nurses is an integral part of IEMT training.
Typical training methods include lectures, field-simulation exercises, and tabletop exercises [ 3 , 4 , 5 ]. However, lectures, despite requiring fewer teaching resources, are often perceived as boring and abstract. This may not be the most ideal method for training newly joined nurses in the complexities of international medical responses. However, simulation field exercises can be effective in mastering the knowledge and skills of disaster medicine responsiveness. However, they come with significant costs and requirements, such as extended instructional periods, additional teachers or instructors, and thorough preparation. These high costs make it challenging to organize simulation exercises repeatedly, making them less ideal for training newly joined nurses [ 6 ].
Moreover, classic tabletop exercises that use simple props, such as cards in a classroom setting, have limitations. The rules of these exercises are typically simple, which makes it challenging to simulate complex disaster scenarios. In addition, these exercises cannot replicate real-life situations, making them too abstract for newly joined nurses to fully grasp [ 7 , 8 ].
Recently, game-based learning has gained increasing attention as an interactive teaching method [ 9 , 10 ]. Previous studies have validated the efficacy of game-based mobile applications [ 11 , 12 ]. Serious games that align with curricular objectives have shown potential to facilitate more effective learner-centered educational experiences for trainees [ 13 , 14 ]. Although game-based applications have been used in disaster medicine education, no serious computer games have been designed specifically for training newly joined nurses in an international IEMT setting.
Our team is an internationally certified IEMT organization verified by the WHO, underscoring the importance of providing training for newly joined nurses in international medical responses. To address this need, we organized training courses for them. As part of the training, we incorporated a serious computer game called the IEMTtraining game. In this game, players assume the roles of IEMT nurses, assess patient injuries in a virtual environment, and provide suitable treatment options. This study aims to investigate the effectiveness of the IEMTtraining game. To the best of our knowledge, this is the first serious game specifically designed to train newly joined nurses in an IEMT setting.
The IEMTtraining game was subsequently applied to the training course for newly joined nurses, and this study aimed to investigate its effectiveness. To the best of our knowledge, this is the first serious game specifically designedto train newly joined nurses in an IEMT setting.
Study design
This study was conducted using data from the training records database of participants who had completed the training. The database includes comprehensive demographic information, exam scores, and detailed information from post-training questionnaires for all trainees. We reviewed the training scores and questionnaires of participants who took part in the training from Autumn 2019 to Spring 2020.
The local Institutional Review Committee approved the study and waived the requirement for informed consent due to the study design. The study complied with the international ethical guidelines for human research, such as the Declaration of Helsinki. The accessed data were anonymized.
Participants
A total of 209 newly joined nurses needed to participate in the training. Due to limitations in the size of the training venue, the trainees had to be divided into two groups for the training. All trainees were required to choose a group and register online. The training team provided the schedule and training topic for the two training sessions to all trainees before the training commenced. Each trainee had the opportunity to sign up based on their individual circumstances. Furthermore, the training team set a maximum limit of 110 trainees for each group, considering the dimensions of the training venue. Trainees were assigned on a first-come-first-served basis. In the event that a group reached its capacity, any unregistered trainees would be automatically assigned to another group.
In the fall of 2019, 103 newly joined nurses opted for the lecture training course (lecture group). In this group, instructors solely used the traditional teaching methods of lectures and demonstrations. The remaining 106 newly joined nurses underwent game-based training (game group). In addition to the traditional lectures and demonstrations, the instructor incorporated an IEMTtraining game to enhance the training experience in the game group.
The IEMTTraining game
The IEMTtraining game, a role-playing game, was implemented using the RPG Maker MV Version1.6.1 (Kadokawa Corporation, Tokyo, Tokyo Metropolis, Japan). Players assumed the roles of rescuers in a fictional setting of an earthquake (Part1 of Supplemental Digital Content ).
The storyline revolves around an earthquake scenario, with the main character being an IEMT nurse. Within the game simulation, there were 1000 patients in the scenario. The objective for each player was to treat as many patients as possible to earn higher experience points compared to other players. In addition, within the game scene, multiple nonplayer characters played the role of injured patients. The players navigate the movements of the main character using a computer mouse. Upon encountering injured persons, the player can view their injury information by clicking on them and selecting the triage tags. The player can then select the necessary medical supplies from the kit to provide treatment. Additionally, the player is required to act according to the minimum standards for IEMTs, such as registration in the IEMT coordination cell and reporting of injury information following the minimum data set (MDS) designed by the WHO [ 15 , 16 ]. This portion of the training content imposes uniform requirements for all IEMT members, hence it is necessary for IEMT nurses to learn it. All correct choices result in the accumulation of experience points. Game duration can be set by the instructor and the player with the highest experience points at the end of the game.
Measurement
We have collected the test scores of the trainees in our training database to explore their knowledge mastery. Additionally, we have collected post-training questionnaire data from the trainees to investigate their learning motivation, cognitive load, and technology acceptance.
Pre-test, post-test, and final test
All trainees were tested on three separate occasions: (1) a “pre-test”before the educational intervention, (2) a “post-test”following the intervention, and (3) a “final test”at the end of the term (sixweeks after the intervention). Each test comprised 20 multiple-choice questions (0.5 points per item) assessing the trainees’ mastery of crucial points in their knowledge and decision-making. The higher the score, the better the grade will be.
Questionnaires
The questionnaires used in this study can be found in Part 2 of the Supplemental Digital Content .
The learning motivation questionnaire used in this study was based on the measure developed by Hwang and Chang [ 17 ]. It comprises seven items rated on a six-point scale. The reliability of the questionnaire, as indicated by Cronbach’s alpha, was 0.79.
The cognitive load questionnaire was adapted from the questionnaire developed by Hwang et al [ 18 ]. It consisted of five items for assessing “mental load” and three items for evaluating “mental effort.” The items were rated using a six-point Likert scale. The Cronbach’s alpha values for the two parts of the questionnaire were 0.86 and 0.85, respectively.
The technology acceptance questionnaire, which was only administered to the game group, as it specifically focused on novel teaching techniques and lacked relevance tothe lecture group, was derived from the measurement instrument developed by Chu et al [ 19 ]. It comprised seven items for measuring “perceived ease of use” and six items for assessing “perceived usefulness.” The items were rated on a six-point Likert scale. The Cronbach’s alpha values for the two parts of the questionnaire were 0.94 and 0.95, respectively.
The lecture group received 4 hours of traditional lectures. Additionally, 1 week before the lecture, the trainees were provided with a series of references related to the topic and were required to preview the content before the class. A pre-test was conducted before the lecture to assess the trainees’ prior knowledge, followed by a post-test immediately after the lecture, and a final test 6 weeks after training.
In the game group, the delivery and requirements for references were the same as those in the lecture group. However, the training format differed. The game group received a half-hour lecture introducinggeneral principles, followed by 3 hours of gameplay. The last halfhour was dedicated to summarizing the course and addressing questions or concerns. Similar to the lecture group, the trainees in this group also completed pre-, post-, and final tests. Additionally, a brief survey ofthe teaching methods was conducted at the end of the final test (see Fig. 1 ).
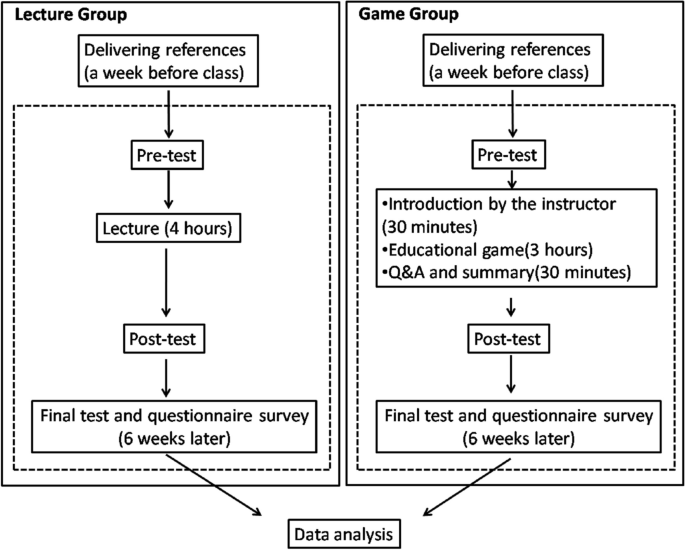
General overview of the teaching procedure. Figure Legend: The diagram shows the teaching and testing processes for the two groups of trainees. Q&A: questions and answers
Data analysis
All data were analyzed using IBM SPSS Statistics (version 20.0;IBM Inc., Armonk, NY, USA). Only the trainees who participated in all three tests were included in the analysis. In total, there were 209 trainees, but 11 individuals (6 from the lecture group and 5 from the game group) were excluded due to incomplete data. Therefore, the data of 198 trainees were ultimately included in the analysis.
In addition, measurement data with a normal distribution were described as mean (standard deviation, SD). In contrast, measurement data with non-normal distributions were expressed as median [first quartile, third quartile]. Furthermore, enumeration data were constructed using composition ratios.
Moreover, a generalized estimating equation (GEE) was employed to compare the groups’ pre-, post-, and final test scores. The Mann–Whitney U test was used to compare the questionnaire scores between the two groups. The statistical significance was set at a level of 0.05.
Among the data included in the analysis, 97 (48.99%) participants were in the lecture group, and 101 (51.01%)were in the game group.
The number of male trainees in the lecture and game groups was 30 (30.93%) and 33 (32.67%), respectively. The mean age of participants in the lecture group was 27.44 ± 4.31 years, whereas that of the game group was 28.05 ± 4.29 years. There were no significant differences in sex or age (Table 1 ). Regarding the test scores, no significant differences were found between the two groups in the pre- and post-tests. However, a significant difference was observed in the final test scores conducted 6 weeks later (Table 1 ).
According to the GEE analysis, the overall scores for the post-test and final test were higher compared to the pre-test scores. Additionally, there was a significant difference in the overall test scores between the two groups, with the game group demonstrating superior performance compared to the control group (odds ratio = 1.363, p value = 0.010). Further details of the GEE results can be found in Part 3 of the supplementary materials .
Table 2 presents the results of the questionnaire ratings for the two groups. The median [first quartile, third quartile] of the learning motivation questionnaire ratings were 4 [3, 4] for the lecture group and 5 [4, 5] for the game group. There were significant differences between the questionnaire ratings of the two groups ( p < 0.001), indicating that the game group had higher learning motivation for the learning activity.
The median [first quartile, third quartile] of the overall cognitive load ratings were 3 [3, 4] and 4 [4, 5] for the game and lecture groups, respectively. There was a significant difference between the cognitive load ratings of the two groups ( p < 0.001).
This study further compared two aspects of cognitive load: mental load and mental effort. The median [first quartile, third quartile] for the mental effort dimension were 3 [2, 3] and 4 [4, 5] for the game and lecture groups, respectively (p < 0.001). For mental load, the median [first quartile, third quartile] were 4 [3, 4] and 4 [3, 4] for the game and lecture groups, respectively. There was no significant difference in the mental load ratings between the two groups ( p = 0.539).
To better understand the trainees’ perceptions of the use of the serious game, this study collected the feedback of the trainees in the game group regarding “perceived usefulness” and “perceived ease of use,” as shown in Table 2 . Most trainees provided positive feedback on the two dimensions of the serious game.
To the best of our knowledge, this IEMT training game is the first serious game intended for newly joined nurses of IEMTs. Therefore, this study presents an initial investigation into the applicability of serious games.
Both lectures and serious games improved post-class test scores to the same level, consistent with previous studies. Krishnan et al. found that an educational game on hepatitis significantly improved knowledge scores [ 20 ]. Additionally, our study showed higher knowledge retention in the game group after 6 weeks, in line with previous studies on serious games. In a study on sexually transmitted diseases, game-based instruction was found to improve knowledge retention for resident physicians compared to traditional teaching methods [ 21 ]. The IEMTtraining game, designed as a role-playing game, is more likely to enhance knowledge retention in newly joined nurses in the long term. Therefore, serious games should be included in the teaching of IEMT training.
This study demonstrated improved learning motivation in the game group, consistent with previous research indicating that game-based learning enhances motivation due to the enjoyable and challenging nature of the games [ 22 , 23 ]. A systematic review by Allan et al. further supports the positive impact of game-based learning tools on the motivation, attitudes, and engagement of healthcare trainees [ 24 ].
As serious games are a novel learning experience for trainees, it is worth investigating the cognitive load they experience. Our study found that serious games effectively reduce trainees’ overall cognitive load, particularly in terms of lower mental effort. Mental effort refers to the cognitive capacity used to handle task demands, reflecting the cognitive load associated with organizing and presenting learning content, as well as guiding student learning strategies [ 25 , 26 ]. This reduction in cognitive load is a significant advantage of serious gaming, as it helps learners better understand and organize their knowledge. However, our study did not find a significant difference in mental load between the two groups. Mental load considers the interaction between task and subject characteristics, based on students’ understanding of tasks and subject characteristics [ 18 ]. This finding is intriguing as it aligns with similar observations in game-based education for elementary and secondary school students [ 27 ], but is the first mention of game-based education in academic papers related to nursing training.
In our survey of the game group participants, we found that their feedback regarding the perceived ease of use and usefulness of the game was overwhelmingly positive. This indicates that the designed game was helpful to learners during the learning process. Moreover, the game’s mechanics were easily understood by the trainees without requiring them to investsignificant time and effort to understand the game rules and controls.
This study had some limitations. First, this retrospective observational study may have been susceptible to sampling bias due to the non-random grouping of trainees. It only reviewed existing data from the training database, and future research should be conducted to validate our findings through prospective studies. Therefore, randomized controlled trials are required. Second, the serious game is currently available only in China. We are currently developing an English version to better align with the training requirements of international IEMT nurses. Third, the development of such serious gamescan be time-consuming. To address this problem, we propose a meta-model to help researchers and instructors select appropriate game development models to implement effective serious games.
An IEMT training game for newly joined nurses is a highly promising training method. Its potential lies in its ability to offer engaging and interactive learning experiences, thereby effectively enhancing the training process. Furthermore, the game improved knowledge retention, increased motivation to learn, and reduced cognitive load. In addition, the game’s mechanics are easily understood by trainees, which further enhances its effectiveness as a training instrument.
Availability of data and materials
Availability of data and materials can be ensured through direct contact with the author. If you require access to specific data or materials mentioned in a study or research article, reaching out to the author is the best way to obtain them. By contacting the author directly, you can inquire about the availability of the desired data and materials, as well as any necessary procedures or restrictions for accessing them.
Authors are willing to provide data and materials to interested parties. They understand the importance of transparency and the positive impact of data sharing on scientific progress. Whether it is raw data, experimental protocols, or unique materials used in the study, authors can provide valuable insights and resources to support further investigations or replications.
To contact the author, one can refer to the email address provided in the article.
Abbreviations
World Health Organization
International Emergency Medical Team
Minimum Data Set
Generalized estimating eq.
Standard deviation
World Health Organization.Classification and minimum standards for emergency medical teams. https://apps.who.int/iris/rest/bitstreams/1351888/retrieve . Published 2021. Accessed May 6, 2023.
World Health Organization. Classification and Minimum Standards for Foreign Medical Teams in Sudden Onset Disasters. https://cdn.who.int/media/docs/default-source/documents/publications/classification-and-minimum-standards-for-foreign-medical-teams-in-suddent-onset-disasters65829584-c349-4f98-b828-f2ffff4fe089.pdf?sfvrsn=43a8b2f1_1&download=true . Published 2013. Accessed May 6, 2023.
Brunero S, Dunn S, Lamont S. Development and effectiveness of tabletop exercises in preparing health practitioners in violence prevention management: a sequential explanatory mixed methods study. Nurse Educ Today. 2021;103:104976. https://doi.org/10.1016/j.nedt.2021.104976 .
Article Google Scholar
Sena A, Forde F, Yu C, Sule H, Masters MM. Disaster preparedness training for emergency medicine residents using a tabletop exercise. Med Ed PORTAL. 2021;17:11119. https://doi.org/10.15766/mep_2374-8265.11119 .
Moss R, Gaarder C. Exercising for mass casualty preparedness. Br J Anaesth. 2022;128(2):e67–70. https://doi.org/10.1016/j.bja.2021.10.016 .
Hu H, Liu Z, Li H. Teaching disaster medicine with a novel game-based computer application: a case study at Sichuan University. Disaster Med Public Health Prep. 2022;16(2):548–54. https://doi.org/10.1017/dmp.2020.309 .
Chi CH, Chao WH, Chuang CC, Tsai MC, Tsai LM. Emergency medical technicians' disaster training by tabletop exercise. Am J Emerg Med. 2001;19(5):433–6. https://doi.org/10.1053/ajem.2001.24467 .
Hu H, Lai X, Li H, et al. Teaching disaster evacuation management education to nursing students using virtual reality Mobile game-based learning. Comput Inform Nurs. 2022;40(10):705–10. https://doi.org/10.1097/CIN.0000000000000856 .
van Gaalen AEJ, Brouwer J, Schönrock-Adema J, et al. Gamification of health professions education: a systematic review. Adv Health Sci Educ Theory Pract. 2021;26(2):683–711. https://doi.org/10.1007/s10459-020-10000-3 .
Adjedj J, Ducrocq G, Bouleti C, et al. Medical student evaluation with a serious game compared to multiple choice questions assessment. JMIR Serious Games. 2017;5(2):e11. https://doi.org/10.2196/games.7033 .
Hu H, Xiao Y, Li H. The effectiveness of a serious game versus online lectures for improving medical Students' coronavirus disease 2019 knowledge. Games Health J. 2021;10(2):139–44. https://doi.org/10.1089/g4h.2020.0140.E .
Pimentel J, Arias A, Ramírez D, et al. Game-based learning interventions to Foster cross-cultural care training: a scoping review. Games Health J. 2020;9(3):164–81. https://doi.org/10.1089/g4h.2019.0078 .
Hu H, Lai X, Yan L. Improving nursing Students' COVID-19 knowledge using a serious game. Comput Inform Nurs. 2021;40(4):285–9. https://doi.org/10.1097/CIN.0000000000000857 .
Menin A, Torchelsen R, Nedel L. An analysis of VR technology used in immersive simulations with a serious game perspective. IEEE Comput Graph Appl. 2018;38(2):57–73. https://doi.org/10.1109/MCG.2018.021951633 .
Kubo T, Chimed-Ochir O, Cossa M, et al. First activation of the WHO emergency medical team minimum data set in the 2019 response to tropical cyclone Idai in Mozambique. Prehosp Disaster Med. 2022;37(6):727–34.
Jafar AJN, Sergeant JC, Lecky F. What is the inter-rater agreement of injury classification using the WHO minimum data set for emergency medical teams? Emerg Med J. 2020;37(2):58–64. https://doi.org/10.1136/emermed-2019-209012 .
Hwang GJ, Chang HF. A formative assessment-based mobile learning approach to improving the learning attitudes and achievements of students. Comput Educ. 2011;56(4):1023–31. https://doi.org/10.1016/j.compedu.2010.12.002 .
Hwang G-J, Yang L-H. Sheng-yuan Wang.Concept map-embedded educational computer game for improving students’ learning performance in natural science courses. Comput Educ. 2013;69:121–30.
Chu HC, Hwang GJ, Tsai CC, et al. A two-tier test approach to developing location-aware mobile learning system for natural science course. Comput Educ. 2010;55(4):1618–27. https://doi.org/10.1016/j.compedu.2010.07.004 .
Krishnan S, Blebil AQ, Dujaili JA, Chuang S, Lim A. Implementation of a hepatitis-themed virtual escape room in pharmacy education: A pilot study. Educ Inf Technol (Dordr). 2023;5:1–13. https://doi.org/10.1007/s10639-023-11745-1 . Epub ahead of print. PMID: 37361790; PMCID: PMC10073791
Butler SK, Runge MA, Milad MP. A game show-based curriculum for teaching principles of reproductive infectious disease (GBS PRIDE trial). South Med J. 2020;113(11):531–7. https://doi.org/10.14423/SMJ.0000000000001165 . PMID: 33140104
Haruna H, Hu X, Chu SKW, et al. Improving sexual health education programs for adolescent students through game-based learning and gamification. Int J Environ Res Public Health. 2018;15(9):2027. https://doi.org/10.3390/ijerph15092027 .
Rewolinski JA, Kelemen A, Liang Y. Type I diabetes self-management with game-based interventions for pediatric and adolescent patients. Comput Inform Nurs. 2020;39(2):78–88. https://doi.org/10.1097/CIN.0000000000000646 .
Allan R, McCann L, Johnson L, Dyson M, Ford J. A systematic review of 'equity-focused' game-based learning in the teaching of health staff. Public Health Pract (Oxf). 2023;27(7):100462. https://doi.org/10.1016/j.puhip.2023.100462 . PMID: 38283754; PMCID: PMC10820634
Zumbach J, Rammerstorfer L, Deibl I. Cognitive and metacognitive support in learning with a serious game about demographic change. Comput Hum Behav. 2020;103:120–9. https://doi.org/10.1016/j.chb.2019.09.026 .
Chang C-C, Liang C, Chou P-N, et al. Is game-based learning better in flow experience and various types of cognitive load than non-game-based learning? Perspective from multimedia and media richness. Comput Hum Behav. 2017;71:218–27. https://doi.org/10.1016/j.chb.2017.01.031 .
Kalmpourtzis G, Romero M. Constructive alignment of learning mechanics and game mechanics in serious game design in higher education. Int J Serious Games. 2020;7(4):75–88. https://doi.org/10.17083/ijsg.v7i4.361 .
Download references
Acknowledgements
We would like to thank all the staffs who contribute to the database. We would like to thank Editage ( www.editage.cn ) for English language editing. We also would like to thank Dr. Yong Yang for statistics help. We would like to thank The 10th Sichuan University Higher Education Teaching Reform Research Project (No. SCU10170) and West China School of Medicine (2023-2024) Teaching Reform Research Project (No. HXBK-B2023016) for the support.
Author information
Both Hai Hu and Xiaoqin Lai contributed equally to this work and should be regarded as co-first authors.
Authors and Affiliations
Emergency Management Office of West China Hospital, Sichuan University, The street address: No. 37. Guoxue Road, Chengdu City, Sichuan Province, China
China International Emergency Medical Team (Sichuan), Chengdu City, Sichuan Province, China
Hai Hu & Xiaoqin Lai
Emergency Medical Rescue Base, Sichuan University, Chengdu City, Sichuan Province, China
Day Surgery Center, West China Hospital, Sichuan University, Chengdu City, Sichuan Province, China
Xiaoqin Lai
Department of Thoracic Surgery, West China Tianfu Hospital, Sichuan University, Chengdu City, Sichuan Province, China
West China School of Nursing, Sichuan University, Chengdu City, Sichuan Province, China
Longping Yan
West China School of Public Health, Sichuan University, Chengdu, Sichuan, China
West China Fourth Hospital, Sichuan University, Chengdu, Sichuan, China
You can also search for this author in PubMed Google Scholar
Contributions
HH conceived the study, designed the trial, and obtained research funding. XL supervised the conduct of the data collection from the database, and managed the data, including quality control. HH and LY provided statistical advice on study design and analyzed the data. All the authors drafted the manuscript, and contributed substantially to its revision. HH takes responsibility for the paper as a whole.
Corresponding author
Correspondence to Hai Hu .
Ethics declarations
Ethics approval and consent to participate.
The local institutional review committee approved the study and waived the need for informed consent from the participants owing to the study design.
Consent for publication
Not applicable.
Competing interests
The authors declare that they have no competing interests.
Additional information
Publisher’s note.
Springer Nature remains neutral with regard to jurisdictional claims in published maps and institutional affiliations.
Supplementary Information
Supplementary material 1., rights and permissions.
Open Access This article is licensed under a Creative Commons Attribution 4.0 International License, which permits use, sharing, adaptation, distribution and reproduction in any medium or format, as long as you give appropriate credit to the original author(s) and the source, provide a link to the Creative Commons licence, and indicate if changes were made. The images or other third party material in this article are included in the article's Creative Commons licence, unless indicated otherwise in a credit line to the material. If material is not included in the article's Creative Commons licence and your intended use is not permitted by statutory regulation or exceeds the permitted use, you will need to obtain permission directly from the copyright holder. To view a copy of this licence, visit http://creativecommons.org/licenses/by/4.0/ . The Creative Commons Public Domain Dedication waiver ( http://creativecommons.org/publicdomain/zero/1.0/ ) applies to the data made available in this article, unless otherwise stated in a credit line to the data.
Reprints and permissions
About this article
Cite this article.
Hu, H., Lai, X. & Yan, L. Training nurses in an international emergency medical team using a serious role-playing game: a retrospective comparative analysis. BMC Med Educ 24 , 432 (2024). https://doi.org/10.1186/s12909-024-05442-x
Download citation
Received : 05 November 2023
Accepted : 17 April 2024
Published : 22 April 2024
DOI : https://doi.org/10.1186/s12909-024-05442-x
Share this article
Anyone you share the following link with will be able to read this content:
Sorry, a shareable link is not currently available for this article.
Provided by the Springer Nature SharedIt content-sharing initiative
- Rescue work
- Gamification
- Simulation training
BMC Medical Education
ISSN: 1472-6920
- Submission enquiries: [email protected]
- General enquiries: [email protected]

- MEMBERS IN THE NEWS
- SCHOOL & PROGRAM UPDATES
- GLOBAL ACTION
- FACULTY & STAFF HONORS
- MEMBER RESEARCH & REPORTS
- STUDENT & ALUMNI ACHIEVEMENTS
- PARTNER NEWS
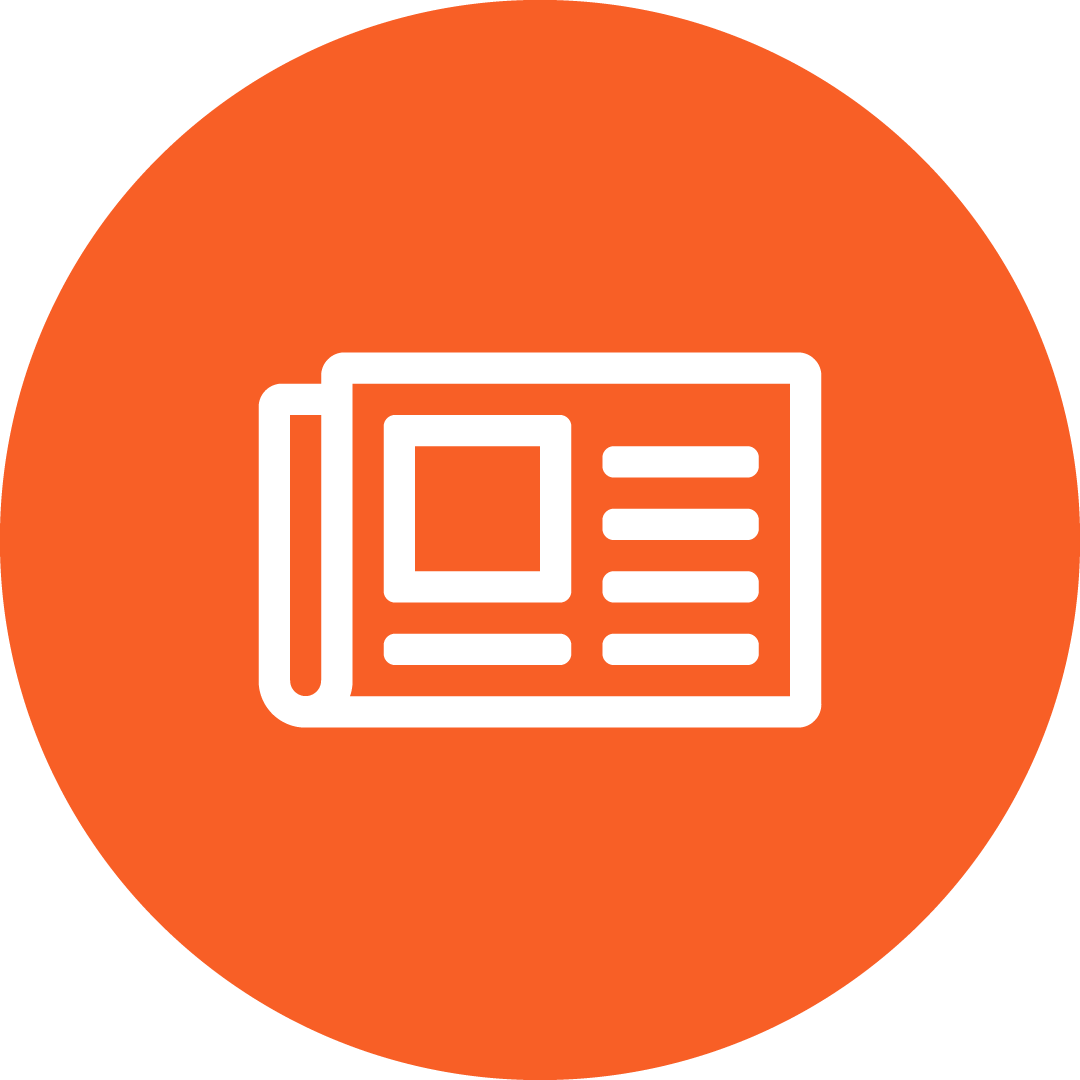
EXPLORE THE FRIDAY LETTER

The Health Survey Research Methods Conference Call for Papers
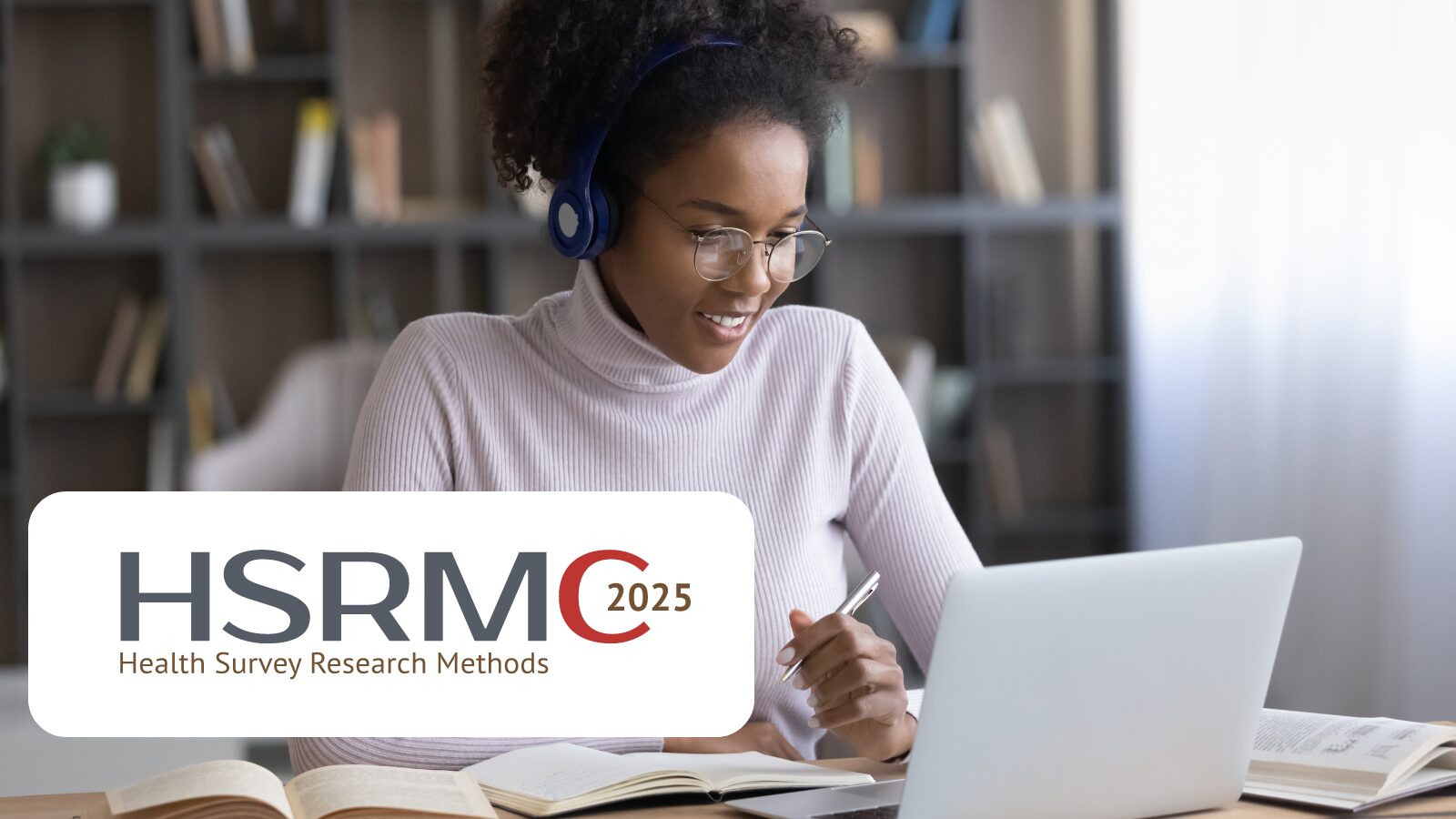
The twelfth Health Survey Research Methods Conference (HSRMC) will continue the series that began 50 years ago to discuss innovative survey research methods that improve the quality of health survey data. The next conference will be held in Williamsburg, VA, from March 4-7, 2025, and HSRMC is seeking abstracts by June 30, 2024 for papers to be presented at the conference.
Abstracts can include general overview papers that summarize and integrate current knowledge, papers that identify and address future research challenges, innovative theoretical essays, and other papers that describe new empirical research that advances the field of survey methods and their application to health-related issues. All abstracts must be submitted online between April 1 and June 30, 2024. Submitted abstracts will be reviewed by the HSRMC steering committee. Read more about the 2025 call and learn more about the history of the HSRMC .
- Alzheimer's disease & dementia
- Arthritis & Rheumatism
- Attention deficit disorders
- Autism spectrum disorders
- Biomedical technology
- Diseases, Conditions, Syndromes
- Endocrinology & Metabolism
- Gastroenterology
- Gerontology & Geriatrics
- Health informatics
- Inflammatory disorders
- Medical economics
- Medical research
- Medications
- Neuroscience
- Obstetrics & gynaecology
- Oncology & Cancer
- Ophthalmology
- Overweight & Obesity
- Parkinson's & Movement disorders
- Psychology & Psychiatry
- Radiology & Imaging
- Sleep disorders
- Sports medicine & Kinesiology
- Vaccination
- Breast cancer
- Cardiovascular disease
- Chronic obstructive pulmonary disease
- Colon cancer
- Coronary artery disease
- Heart attack
- Heart disease
- High blood pressure
- Kidney disease
- Lung cancer
- Multiple sclerosis
- Myocardial infarction
- Ovarian cancer
- Post traumatic stress disorder
- Rheumatoid arthritis
- Schizophrenia
- Skin cancer
- Type 2 diabetes
- Full List »
share this!
April 19, 2024
This article has been reviewed according to Science X's editorial process and policies . Editors have highlighted the following attributes while ensuring the content's credibility:
fact-checked
peer-reviewed publication
trusted source
Researchers explore causal machine learning, a new advancement for AI in health care
by Ludwig Maximilian University of Munich
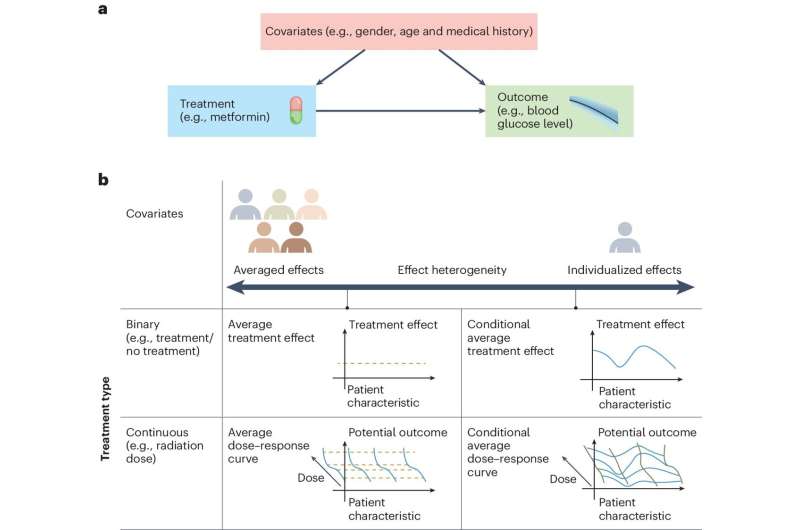
Artificial intelligence is making progress in the medical arena. When it comes to imaging techniques and the calculation of health risks, there is a plethora of AI methods in development and testing phases. Wherever it is a matter of recognizing patterns in large data volumes, it is expected that machines will bring great benefit to humanity. Following the classical model, the AI compares information against learned examples, draws conclusions, and makes extrapolations.
Now an international team led by Professor Stefan Feuerriegel, Head of the Institute of Artificial Intelligence (AI) in Management at LMU, is exploring the potential of a comparatively new branch of AI for diagnostics and therapy. Can causal machine learning (ML) estimate treatment outcomes—and do so better than the ML methods generally used to date? Yes, says a study by the group, which has been published in Nature Medicine and is titled "Causal ML can improve the effectiveness and safety of treatments."
In particular, the new ML variant offers "an abundance of opportunities for personalizing treatment strategies and thus individually improving the health of patients," write the researchers, who hail from Munich, Cambridge (United Kingdom), and Boston (United States) and include Stefan Bauer and Niki Kilbertus, professors of computer science at the Technical University of Munich (TUM) and group leaders at Helmholtz AI.
As regards machine assistance in therapy decisions, the authors anticipate a decisive leap forward in quality. Classical ML recognizes patterns and discovers correlations, they argue. However, the causal principle of cause and effect remains closed to machines as a rule; they cannot address the question of why. And yet many questions that arise when making therapy decisions contain causal problems within them.
The authors illustrate this with the example of diabetes: Classical ML would aim to predict how probable a disease is for a given patient with a range of risk factors. With causal ML, it would ideally be possible to answer how the risk changes if the patient gets an anti-diabetes drug; that is, gauge the effect of a cause (prescription of medication). It would also be possible to estimate whether another treatment plan would be better, for example, than the commonly prescribed medication, metformin.
To be able to estimate the effect of a—hypothetical—treatment, however, "the AI models must learn to answer questions of a 'What if?' nature," says Jonas Schweisthal, doctoral candidate in Feuerriegel's team.
"We give the machine rules for recognizing the causal structure and correctly formalizing the problem," says Feuerriegel. Then the machine has to learn to recognize the effects of interventions and understand, so to speak, how real-life consequences are mirrored in the data that has been fed into the computers.
"The software we need for causal ML methods in medicine doesn't exist out of the box," says Feuerriegel. Rather, "complex modeling" of the respective problem is required, involving "close collaboration between AI experts and doctors."
Like his TUM colleagues Stefan Bauer and Niki Kilbertus, Feuerriegel also researches questions relating to AI in medicine, decision-making, and other topics at the Munich Center for Machine Learning (MCML) and the Konrad Zuse School of Excellence in Reliable AI.
In other fields of application, such as marketing, explains Feuerriegel, the work with causal ML has already been in the testing phase for some years now. "Our goal is to bring the methods a step closer to practice. The paper describes the direction in which things could move over the coming years."
Explore further
Feedback to editors

Illusion demystifies the way vision works: Experiments imply brightness perception occurs deeper in brain than thought
15 minutes ago

How buildings influence the microbiome and human health
16 minutes ago

Biomarkers identified for successful treatment of bone marrow tumors
20 minutes ago

Neuroscientists investigate how the target of an arm movement is spatially encoded in the primate brain
28 minutes ago

Homelessness found to be a major issue for many patients in the emergency department
29 minutes ago

Undocumented Latinx patients got COVID-19 vaccine at same rate as US citizens, study finds

Experimental malaria monoclonal antibody protective in Malian children

People with rare longevity mutation may also be protected from cardiovascular disease

Study identifies driver of liver cancer that could be target for treatment
35 minutes ago

International study fills data gap on adolescent mental health
38 minutes ago
Related Stories

Artificial intelligence facilitates better control of global development aid
Apr 13, 2022

AI model provides a hypoglycemia early warning system when driving
Feb 8, 2024

Model uses AI to create better outcomes and save costs for prediabetic patients
Feb 28, 2024

Advancing causal inference in clinical neuroscience research
Jul 13, 2023

Researcher: The quantum computer doesn't exist yet, but we are better understanding what problems it can solve
Apr 10, 2024

Causal reasoning meets visual representation learning: A prospective study
Nov 22, 2023
Recommended for you

Using AI to improve diagnosis of rare genetic disorders
Apr 25, 2024

Researchers create an AI-powered digital imaging system to speed up cancer biopsy results

Research identifies pitfalls and opportunities for generative AI in patient messaging systems
Apr 24, 2024

New algorithm could provide early warning for asthma attacks

Study finds AI can develop treatments to prevent 'superbugs'

New study uses AI to predict malaria outbreaks in South Asia
Let us know if there is a problem with our content.
Use this form if you have come across a typo, inaccuracy or would like to send an edit request for the content on this page. For general inquiries, please use our contact form . For general feedback, use the public comments section below (please adhere to guidelines ).
Please select the most appropriate category to facilitate processing of your request
Thank you for taking time to provide your feedback to the editors.
Your feedback is important to us. However, we do not guarantee individual replies due to the high volume of messages.
E-mail the story
Your email address is used only to let the recipient know who sent the email. Neither your address nor the recipient's address will be used for any other purpose. The information you enter will appear in your e-mail message and is not retained by Medical Xpress in any form.
Newsletter sign up
Get weekly and/or daily updates delivered to your inbox. You can unsubscribe at any time and we'll never share your details to third parties.
More information Privacy policy
Donate and enjoy an ad-free experience
We keep our content available to everyone. Consider supporting Science X's mission by getting a premium account.
E-mail newsletter
This paper is in the following e-collection/theme issue:
Published on 25.4.2024 in Vol 26 (2024)
Effect of Prosocial Behaviors on e-Consultations in a Web-Based Health Care Community: Panel Data Analysis
Authors of this article:

Original Paper
- Xiaoxiao Liu 1, 2 , PhD ;
- Huijing Guo 3 , PhD ;
- Le Wang 4 , PhD ;
- Mingye Hu 5 , PhD ;
- Yichan Wei 1 , BBM ;
- Fei Liu 6 , PhD ;
- Xifu Wang 7 , MCM
1 School of Management, Xi’an Jiaotong University, Xi'an, China
2 China Institute of Hospital Development and Reform, Xi'an Jiaotong University, Xi'an, China
3 School of Economics and Management, China University of Mining and Technology, Xuzhou, China
4 College of Business, City University of Hong Kong, Hong Kong, China (Hong Kong)
5 School of Economics and Management, Xi’an University of Technology, Xi'an, China
6 School of Management, Harbin Engineering University, Harbin, China
7 Healthcare Simulation Center, Guangzhou First People’s Hospital, Guangzhou, China
Corresponding Author:
Xifu Wang, MCM
Healthcare Simulation Center
Guangzhou First People’s Hospital
1 Pan Fu Road
Yuexiu District
Guangzhou, 510180
Phone: 86 13560055951
Email: [email protected]
Background: Patients using web-based health care communities for e-consultation services have the option to choose their service providers from an extensive digital market. To stand out in this crowded field, doctors in web-based health care communities often engage in prosocial behaviors, such as proactive and reactive actions, to attract more users. However, the effect of these behaviors on the volume of e-consultations remains unclear and warrants further exploration.
Objective: This study investigates the impact of various prosocial behaviors on doctors’ e-consultation volume in web-based health care communities and the moderating effects of doctors’ digital and offline reputations.
Methods: A panel data set containing information on 2880 doctors over a 22-month period was obtained from one of the largest web-based health care communities in China. Data analysis was conducted using a 2-way fixed effects model with robust clustered SEs. A series of robustness checks were also performed, including alternative measurements of independent variables and estimation methods.
Results: Results indicated that both types of doctors’ prosocial behaviors, namely, proactive and reactive actions, positively impacted their e-consultation volume. In terms of the moderating effects of external reputation, doctors’ offline professional titles were found to negatively moderate the relationship between their proactive behaviors and their e-consultation volume. However, these titles did not significantly affect the relationship between doctors’ reactive behaviors and their e-consultation volume ( P =.45). Additionally, doctors’ digital recommendations from patients negatively moderated both the relationship between doctors’ proactive behaviors and e-consultation volume and the relationship between doctors’ reactive behaviors and e-consultation volume.
Conclusions: Drawing upon functional motives theory and social exchange theory, this study categorizes doctors’ prosocial behaviors into proactive and reactive actions. It provides empirical evidence that prosocial behaviors can lead to an increase in e-consultation volume. This study also illuminates the moderating roles doctors’ digital and offline reputations play in the relationships between prosocial behaviors and e-consultation volume.
Introduction
e-Consultations, offered through web-based health care communities [ 1 ], are increasingly becoming vital complements to traditional hospital services [ 2 - 4 ]. In hospital consultations, patients can only passively accept treatment [ 5 ] from a limited pool of medical resources within a geographical radius. However, when engaging with web-based health care communities, patients can search for primary care solutions [ 6 ] from an extensive digital market in a relatively short time [ 7 ]. Given that the diagnostic accuracy of e-consultations matches that of hospital consultations [ 8 - 10 ], e-consultations are becoming increasingly attractive to patients [ 3 , 11 ].
Doctors are also showing a growing interest in e-consultations, motivated by economic and social benefits. First, doctors can achieve economic gains by participating in e-consultations [ 7 , 12 ]. Web-based consultation platforms facilitate an efficient reputation system, enabling patients to easily provide feedback about doctors. Consequently, doctors can use e-consultation to strengthen their relationship with patients [ 13 , 14 ] and foster positive word-of-mouth [ 15 ]. More e-consultations can benefit doctors by retaining current patients, attracting new ones, and boosting in-person hospital visits [ 16 , 17 ]. Second, doctors could also receive social returns from engaging in e-consultation [ 7 ]. Active participation in e-consultations allows doctors to demonstrate their skills, attitude, and experience, aiding in accumulating professional capital [ 7 ], building their reputation [ 18 ], and increasing their social influence [ 19 ]. Given these tangible and intangible benefits, it is essential for doctors to diligently provide the desired e-consultations and make additional efforts to highlight their service attributes to stand out [ 6 , 20 , 21 ]. This involves engaging in prosocial behaviors in web-based health care communities, which is the primary research focus of this study.
Prior studies have examined the effects of prosocial behaviors on financial outcomes, such as actions reflecting social responsibility in the workplace [ 22 ]. In the health care sector, previous research has explored doctors’ prosocial behaviors within traditional, offline medical services. Doctors, working in established medical institutes and serving patients with limited choices of clinical service providers, often aim for self-satisfaction and patient satisfaction with their offline prosocial behaviors. For example, research indicates that doctors may act prosocially to regulate their self-oriented feelings [ 23 ] and foster a caring and understanding attitude toward patients [ 24 , 25 ]. Additionally, doctors who demonstrate more empathy and care can elicit positive emotions in patients and improve the doctor-patient relationship [ 26 , 27 ].
Compared to the offline context, doctors’ prosocial behaviors in a digital context may differ in 2 aspects. First, the internet allows patients to choose from a broader, more diverse range of doctors without the constraints of time and space [ 7 ]. However, the uncertainty inherent in the digital environment creates a more pronounced information asymmetry between patients and doctors [ 28 ], consequently making it more challenging for patients to establish trust. Therefore, doctors’ prosocial behaviors are crucial in building their self-image, establishing patients’ trust, and assisting patients in identifying suitable doctors [ 29 , 30 ]. Second, unlike offline environments, web-based medical platforms offer a range of functions, including asynchronous activities such as publishing articles, as well as real-time interactional actions such as answering questions during live streams. This array of functions facilitates the adoption of more diverse prosocial behaviors by doctors.
Although these differences underscore the importance of studying doctors’ prosocial behavior, there has been limited research focusing on the impact of such behaviors in the digital context. One previous study has scrutinized the impact of prosocial behaviors, such as answering patients’ questions freely, on patient engagement within web-based health care communities [ 31 ]. An aspect that requires further exploration is how doctors’ motivations and patients’ involvement vary in doctors’ helping behaviors. Consequently, studies on web-based health care communities should differentiate between diverse prosocial actions to understand their effects on doctors’ web-based service outcomes. This study aims to contribute new knowledge regarding the full breadth of doctors’ prosocial behaviors.
Unlike the previous study that exclusively investigated doctors’ asynchronous behaviors in web-based health care communities [ 31 ], this study also explores the role of synchronous reactive actions in achieving optimal doctors’ e-consultation volume. Recently, web-based health care communities have developed and released live-streaming functions to assist doctors in providing voluntary interactions with patients. The effect of doctors’ engagement in medical live streaming on e-consultation services remains unexplored. While these behaviors could demonstrate doctors’ ethical traits and ability to fulfill an e-consultation workflow, a potential trade-off with e-consultations may exist when doctors engage in prosocial behaviors.
In summary, this study examines the effects of doctors’ proactive and reactive prosocial behaviors, considering their digital and offline reputations as potential moderating factors. First, drawing from functional motives theory (FMT), we explore the impact of doctors’ web-based proactive actions on their e-consultation volume. Proactive behaviors are actions in which individuals exceed their assigned work, focusing on long-term goals to prevent future problems [ 32 , 33 ]. According to FMT, these behaviors reflect helping actions that satisfy personal needs [ 34 ], driven by self-focused motivations [ 35 ], such as impression management and the realization of self-worth goals. For example, knowledge-based proactive behaviors, such as disseminating expertise to preempt future issues, are self-initiated and not reactions to immediate requests [ 36 ]. This study categorizes doctors’ sharing of professional articles as a form of proactive behavior that creates a professional image for their patient audience. This is because these actions aim to assist patients with future health concerns rather than directly responding to patients’ immediate needs.
Second, this study explores the role of doctors’ reactive prosocial behaviors in increasing e-consultations, guided by social exchange theory (SET). Unlike proactive behaviors, reactive behaviors are characterized by instances of individuals engaging in helping activities [ 35 ], typically in response to others’ needs [ 34 ]. SET posits that individuals incurring additional social costs in relationships may anticipate reciprocal value [ 37 , 38 ]. Reactive prosocial behaviors, per SET, are initiated by the motivation to satisfy others’ desires, leading to the development of cooperative social values. In our context, medical live streams facilitate real-time, synchronized interactions, enabling patients to ask questions and doctors to provide immediate responses. Patients’ health questions during these streams indicate their immediate needs. Thus, a higher frequency of live streams within a certain period suggests doctors are increasingly responding to patients’ needs during that time. Therefore, this study uses the number of medical live-streaming sessions conducted by doctors as a measure for their synchronous reactive behaviors.
Finally, considering that doctors’ reputations play a crucial role in their workflow on web-based health care communities [ 39 , 40 ], we test the moderating roles of digital and offline reputation—measured by doctors’ offline professional titles and patients’ recommendations in the digital context, respectively—on the main effects.
Based on previous studies and practices within web-based health care communities, we aim to extend the literature by testing the impact of 2 types of web-based prosocial behaviors by doctors: proactive and synchronous reactive actions on e-consultation volume. We then explore the moderating roles of doctors’ offline and digital reputations on these main effects.
Research Framework and Hypothesis Development
We have developed a research framework, shown in Figure 1 , to identify effective prosocial strategies used by doctors within web-based health care communities to achieve a preferred e-consultation volume from the supply side.

Primarily, we explore the relationships between doctors’ prosocial behaviors and e-consultation volume, drawing on FMT and SET. These theories are widely adopted for measuring and classifying the outcomes of prosocial behaviors from 2 fundamental perspectives based on human nature [ 34 ]. While doctors’ offline prosocial behaviors may help satisfy patients [ 24 , 25 ], who are already service acceptors, the outcomes of doctors’ web-based prosocial behaviors still need careful distinction. It is essential to clearly differentiate between various types of doctors’ prosocial behaviors to identify their nature. In this study, following the leads of FMT and SET, we test 2 kinds of prosocial behavior: proactive (posting professional articles to achieve self-worth) and reactive (conducting medical live streaming to create cooperative social values).
Subsequently, we examine how doctors’ external reputation moderates the impacts of doctors’ proactive and reactive prosocial behaviors. This examination is conducted from the perspectives of reducing uncertainty and building trust, respectively.
Doctors’ Proactive Behaviors and e-Consultation Volume
FMT places emphasis on the primary motivations behind individuals’ behaviors, adopting an atheoretical stance [ 41 ]. Through the exploratory process, previous studies have provided examples to identify the functional motivations behind prosocial behaviors [ 42 ], such as expressing important personal values. In web-based health care communities, doctors have the opportunity to demonstrate personal traits through proactive behaviors. According to FMT, these proactive behaviors stem from the actors’ active efforts to satisfy their own needs and achieve self-worth [ 34 , 35 ].
Doctors might post professional articles, such as clinical notes and scientific papers, on web-based health care communities to help patient readers handle future health problems. These proactive prosocial behaviors are primarily driven by a desire to showcase personal medical competence, a crucial characteristic of a professional image [ 43 ], in medical consultations. By posting professional articles, doctors can display their medical knowledge, care delivery capability, and service quality, thereby enhancing their professional image. We hypothesize that this effort will lead to an increase in the e-consultation volume. Therefore, we propose the following hypothesis:
- Hypothesis 1: The posting of professional articles by doctors positively impacts their e-consultation volume on web-based health care communities.
Doctors’ Reactive Behaviors and e-Consultation Volume
Considering the social environment in the working context, SET suggests that reactive prosocial behaviors stem from responding to others’ needs [ 34 ]. Engaging in such behaviors can foster positive perceptions among the audience and build cooperative social values [ 44 ] through reactive social exchange. People with a high orientation toward cooperative social values act to maximize mutual interests [ 45 ], a trait highly valued in the medical field.
We use medical live streaming as a measure of doctors’ reactive behaviors on web-based health care communities. Volunteering to provide interactional live streaming, a typical reactive behavior that may generate cooperative social value, gives the patient audience the impression that the doctors will prioritize demand-side interests during e-consultation services. Additionally, engaging in medical live streaming allows doctors to present themselves as authentic and recognized experts. This enhances their social presence [ 46 ], potentially leading to increased service use [ 47 ] and greater popularity [ 48 ]. Consequently, patients are more likely to perceive doctors who participate in medical live streaming as trustworthy for consultations. Given that e-consultations are closely related to the health conditions of the demand side, a credible doctor is likely to attract more e-consultations. Therefore, we propose the following hypothesis:
- Hypothesis 2: The conduct of medical live streaming by doctors positively impacts their e-consultation volume on web-based health care communities.
Moderating Roles of Offline and Digital Reputation
As doctors’ proactive and reactive behaviors potentially affect their consultation performance, based on 2 distinct theoretical foundations of human nature, there exists a discrepancy in how doctors’ reputations influence the relationship between various prosocial behaviors and e-consultation.
We formulate hypotheses regarding the moderating effects within the context of digital health care, by taking into account the inherent information asymmetry and the significance of establishing patient trust. Specifically, our hypotheses explore the influence of reputation on the relationship between doctors’ proactive behaviors and e-consultation volume, with a focus on reducing uncertainty. Additionally, we examine how reputation moderates the impact of doctors’ reactive behaviors, emphasizing the perspective of trust building.
First, in the marketing literature, service providers’ reputations, which can reduce information asymmetry and purchase uncertainty [ 49 ], are key factors influencing purchasing behavior and sales performance in the digital context [ 50 - 52 ]. Similarly, for doctors, reputations are related to the experiences and beliefs of other stakeholders [ 53 ]. As health care services are credence goods [ 54 ]—whose quality patients cannot discern even after experiencing the services—and given the nature of web-based platforms (eg, the absence of face-to-face meetings), there is a significant information asymmetry [ 51 ]. This increases patients’ uncertainty regarding the quality of doctors. Consequently, doctors’ reputations play crucial roles in patients’ decision-making processes [ 18 , 39 ]. We use doctors’ professional titles and patients’ recommendations on web-based health care communities to measure doctors’ offline and digital reputations.
Proactive behaviors by low-reputation doctors can create deeper professional impressions [ 34 , 35 ] to reduce uncertainty in e-consultations than high-reputation doctors, who are less uncertain in medical services. Then, doctors’ reputations—measured by offline professional titles and digital patients’ recommendations on web-based health care communities—will negatively moderate the relationship between proactive behavior and e-consultation volume. Thus, we propose the following hypotheses:
- Hypothesis 3a: Doctors’ offline professional titles negatively moderate the relationship between the posting of professional articles and e-consultation volume on web-based health care communities.
- Hypothesis 3b: Doctors’ digital recommendations from patients negatively moderate the relationship between the posting of professional articles and e-consultation volume on web-based health care communities.
Second, one of the central elements of SET is the concept of trust between actors in the exchange process [ 55 - 58 ]. In the context of digital health, patient’s trust in doctors is important to establish in order to refine the doctor-patient relationship. Doctors’ reputations can reflect their personality traits [ 39 ] and promote trust from patients [ 53 ]. Conducting medical live streaming, a form of reactive prosocial behavior, includes doctors’ cooperative social value orientations that are preferred in e-consultations. For low-reputation doctors, such as those with relatively junior professional titles and few digital patient recommendations, conducting medical live streaming will build patients’ confidence in e-consultations to a greater extent than doctors with high reputations, who are usually already highly trusted. Then, offline and digital reputation may negatively moderate the relationship between engaging in medical live streaming and e-consultation volume. Thus, we propose the final hypotheses:
- Hypothesis 4a: Doctors’ offline professional titles will negatively moderate the relationship between conducting medical live streaming and e-consultation volume on web-based health care communities.
- Hypothesis 4b: Doctors’ digital recommendations from patients will negatively moderate the relationship between conducting medical live streaming and e-consultation volume on web-based health care communities.
Research Context and Data Collection
Our research context is one of the largest web-based health care communities in China. This platform, established in 2006, offers e-consultation services to patients. As of July 2023, it boasts over 260,000 active doctors from 10,000 hospitals nationwide and has provided web-based medical services to 79 million patients.
The platform allows doctors to create home pages where they can display relevant information such as offline professional titles, experiences shared by other patients, and personal introductions. Patients can select doctors for e-consultation by browsing this information. Besides e-consultation, doctors can engage in prosocial behavior primarily focused on knowledge sharing. This includes posting professional articles in various formats (text, voice, and short videos) and conducting medical live streams for real-time interaction with patients.
We collected data over a 22-month period, from January 2021 to October 2022, focusing on common diseases such as diabetes, depression, infertility, skin diseases, and gynecological diseases. To ensure that our findings are generalizable to a typical and active doctor on the platform, we included doctors who had posted at least 1 article and conducted at least 1 live stream before the end of the study period in our analysis [ 59 - 61 ]. Our sample consists of 2880 doctors and includes the following information for each doctor: professional title, patient recommendations, records of experiences shared by the doctor’s patients, records of professional articles posted, records of live streams conducted, and records of the doctor’s e-consultations.
Variable Operationalization
Our unit of analysis is each doctor. We investigate how doctors’ prosocial behaviors, including proactive behaviors (posting professional articles) and reactive behaviors (conducting medical live streams), influence their e-consultation volume.
Dependent Variable
Our dependent variable is the doctors’ e-consultation volume, denoted as Consultation it , which is measured by the number of e-consultations of doctor i in month t .
Independent Variables
Our independent variables are doctors’ proactive behaviors and reactive behaviors. Doctors’ proactive behavior is operationalized as the posting of professional articles. Specifically, we denote proactive behavior as Articles it , which is measured by the number of professional articles posted by doctor i in month t . Doctors’ reactive behavior is operationalized as medical live streaming. This variable is denoted as LiveStreaming it , which is calculated as the number of medical live streams conducted by doctor i in month t .
Moderating Variables
We are also interested in how doctors’ external reputation, including their offline professional titles and digital recommendations from patients, influences the relationship between prosocial behaviors and e-consultation volume. A doctor’s offline professional title is denoted as Title i , which is a dummy variable indicating whether doctor i is a chief doctor ( Title i =1 indicates the doctor is a chief doctor, and Title i =0 indicates the doctor has a lower-ranked title). Digital recommendations are captured by Recommendations i , which is the digital recommendation level of doctor i as calculated by the platform based on the recommendations provided by their past patients.
Control Variables
We incorporated several control variables to account for factors that may influence patient’s choices of doctors in the digital context. The shared experiences of patients regarding a doctor’s treatment [ 39 ], as well as the number of patients who have previously consulted with the doctors [ 17 , 62 ], can indicate the doctor’s overall popularity. This, in turn, may affect patient choice. Therefore, we controlled for (1) the total number of patients who consulted with doctor i in the digital context before month t ( TotalPatients it ) and (2) the total number of patient-shared experiences about offline treatment by doctor i before month t ( TotalExperiences it ). Furthermore, doctors’ past behaviors, including article publishing and live streaming, can influence their current practices in posting articles and conducting live streams. Simultaneously, these factors may also act as signals affecting patients’ judgments and selection of doctors [ 12 ]. To account for these influences, we also controlled for (1) the total number of articles posted by doctor i before month t ( TotalArticles it ) and (2) the total number of medical live streams conducted by doctor i before month t ( TotalLiveStreaming it ).
To control for both observed and unobserved doctor-specific factors that do not change over time, individual-fixed effects were added. Additionally, time-fixed effects were introduced into our analysis to account for both observed and unobserved factors that vary over time but remain constant across doctors. Table 1 shows the variables and their definitions.
Estimation Model
To estimate the direct impact of doctors’ proactive behaviors and reactive behaviors on their e-consultation volume, the following 2-way fixed effects regression model was used:
Consultation it = β 0 + β 1 Articles it + β 2 LiveStreaming it + β 3 TotalPatients it + β 4 TotalExperiences it + β 5 TotalArticles it + β 6 TotalLiveStreaming it + α i + δ t + μ it (1)
where i denotes doctor, t denotes month, α i is doctor-fixed effects, δ t is month-fixed effects, Consultation it is the number of e-consultations of doctor i in month t , Articles it is the number of professional articles posted by doctor i in month t , LiveStreaming it is the number of medical live streams conducted by doctor i in month t , TotalPatients it is the total number of patients who consulted doctor i in the digital context before month t , TotalExperiences it is the total number of patient-shared experiences about offline treatment by doctor i before month t , TotalArticles it is the total number of articles posted by doctor i before month t , TotalLiveStreaming it is the total number of medical live streams doctor i conducted before month t , β is the coefficient, and μ it is the error term. We took the log transformation for our continuous variables in the model to reduce the skewness of the variables [ 63 ].
Next, the moderating effects of doctors’ offline professional titles and digital recommendations by patients were investigated based on the following specification:
Consultation it = β 0 + β 1 Articles it + β 2 LiveStreaming it + β 3 Articles it × Title i + β 4 LiveStreaming it × Title i + β 5 Articles it × Recommendation i + β 6 LiveStreaming it × Recommendation i + β 7 TotalPatients it + β 8 TotalExperiences it + β 9 TotalArticles it + β 10 TotalLiveStreaming it + α i + δ t + μ it (2)
where Title i indicates whether doctor i is a chief doctor ( Title i =1 indicates the doctor is a chief doctor, and Title i =0 indicates the doctor has a lower-ranked title). Recommendations i is the digital recommendation level of doctor i by other patients.
Ethical Considerations
This study used secondary publicly available data obtained from a website and did not involve the collection of original data pertaining to human participants. As such, there is no evidence of unethical behavior in the study. Consequently, ethics approval by an ethics committee or institutional review board was not deemed necessary.
In this section, we present our empirical results. The descriptive statistics are shown in Table 2 , and the correlation matrix is shown in Table 3 .
Empirical Results
Results for direct effects.
The analysis was conducted progressively. We first estimated the equation without control variables (model 1) and then added control variables in model 2. The estimated results are shown in Table 4 . From the results, we can see that the coefficient of Articles is significant and positive in model 2 (β=.093; P <.001), indicating that doctors’ proactive behaviors (ie, posting professional articles) can help them obtain more e-consultations. Thus, hypothesis 1 is supported. Regarding doctors’ engagement in medical live streaming, the results show that the coefficient of LiveStreaming is significantly positive (β=.214; P <.001), which suggests that doctors’ reactive behaviors (ie, conducting medical live streaming) can increase their e-consultation volume. This supports hypothesis 2.
a All models include doctor-fixed effects and month-fixed effects; robust SEs clustered by doctors are reported; the number of doctors is 2880, and the number of observations is 63,360.
b R 2 =0.843; F 2,2879 =175.98; P <.001.
c R 2 =0.851; F 6,2879 =119.72; P <.001.
d N/A: not applicable.
Results for Moderating Effects
The results for moderating effects are shown in Table 5 . In model 1, interaction terms were initially introduced between Title and Articles , as well as between Title and LiveStreaming , to estimate the moderating effect of doctors’ offline professional titles. The interaction terms were then added between Recommendations and Articles , as well as between Recommendations and LiveStreaming , to estimate the moderating effect of doctors’ digital recommendations in model 2. Finally, a full model was estimated by incorporating all interaction terms. We find that the results are consistent across all models. Wald tests and likelihood ratio were used to compare the fit among nested models [ 64 , 65 ], and the results show that the inclusion of moderating variables significantly enhances the model’s fit.
Regarding the moderating effect of doctors’ offline professional titles, we find that the coefficient of Articles × Title in model 1 of Table 5 is significantly negative (β=–.058; P <.001), which supports hypothesis 3a that doctors’ offline professional titles have a negative moderating effect on the relationship between doctors’ proactive behaviors and e-consultation volume. However, the coefficient of LiveStreaming × Title is insignificant (β=–.024; P =.45), which suggests that doctors’ offline professional titles have no moderating effect on the relationship between doctors’ reactive behaviors and e-consultation volume. Thus, hypothesis 4a is not supported.
b R 2 =0.851; F 8,2879 =89.98; P <.001; Wald test: P <.001; likelihood ratio: P <.001.
c R 2 =0.851; F 8,2879 =89.13; P <.001; Wald test: P <.001; likelihood ratio: P <.001.
d R 2 =0.852; F 10,2879 =71.44; P <.001; Wald test: P <.001; likelihood ratio: P <.001.
e N/A: not applicable.
For the moderating effect of digital patient recommendations, we find that both of the coefficients of Articles × Recommendations and LiveStreaming × Recommendations are negative and significant (β=–.055; P <.001 and β=–.100; P <.001, respectively, in model 2 of Table 5 ). This indicates that digital recommendations from patients have negative moderating effects on the relationship between doctors’ proactive behaviors and e-consultation volume as well as on the relationship between doctors’ reactive behaviors and e-consultation volume; this finding supports hypotheses 3b and 4b.
Robustness Check
First, additional analysis was performed to check whether our findings are robust to different measures of doctors’ reactive behaviors. In the main analysis, we used the number of medical live streams to construct doctors’ reactive behaviors. In the robustness check, doctors’ reactive behaviors were measured using the following measures: (1) the length of time spent in medical live streaming ( LSDuration it ), which is calculated as the total duration of all medical live streams conducted by doctor i in month t ; and (2) the number of doctor-patient interactions in the medical live streams ( LSInteractions it ), which is calculated as the total number of interactions between doctor i and patients in medical live streams in month t . This measure is likely to more effectively capture the reactive element of the behavior. The estimated results are shown in Table 6 , and we can see that the results are consistent with the main results.
Second, in the above analysis, the total number of articles posted by the doctors was used to measure doctors’ proactive behaviors. As doctors can post articles that are either their own original work or reposts from others, we further used the number of original articles ( OriArticles it ) to measure doctors’ proactive prosocial behaviors. Specifically, the number of articles was replaced with the number of original articles posted by doctor i in month t ( OriArticles it ). Models 1 and 2 in Table 7 show the results. We can see that using this alternative measure of proactive behavior does not materially change the results.
Third, as our dependent variable takes nonnegative values, negative binomial regression was further used to re-estimate our models. We find that the results (models 3 and 4 in Table 7 ) are similar to the main results.
Fourth, to further enhance the robustness and validity of our findings, article quality was used as a measure of doctors’ proactive behaviors. This approach is based on the premise that article quality more accurately reflects the effort and time invested by doctors in content creation. Specifically, we assessed article quality based on either the length of each article or the number of likes it received and then re-estimated our model. As indicated in Table 8 , the results remain consistent with our main findings, thereby further reinforcing the validity of our conclusions.
b R 2 =0.851; F 6,2879 =131.71; P <.001.
c R 2 =0.851; F 10,2879 =79.29; P <.001; Wald test: P <.001; likelihood ratio: P <.001.
d R 2 =0.850; F 6,2879 =112.52; P <.001.
e R 2 =0.851; F 10,2879 =68.43; P <.001; Wald test: P <.001; likelihood ratio: P <.001.
f N/A: not applicable.
a All models include doctor-fixed effects and month-fixed effects; robust SEs clustered by doctors are reported in models 1 and 2; bootstrap SEs in models 3 and 4.
b R 2 =0.851; F 6,2879 =118.99; P <.001.
c R 2 =0.852; F 10,2879 =71.04; P <.001; Wald test: P <.001; likelihood ratio: P <.001.
d Log likelihood=–150,015.36.
e Log likelihood=–149,888.24.
b R 2 =0.851; F 6,2879 =127.75; P <.001.
c R 2 =0.851; F 10,2879 =89.97; P <.001; Wald test: P <.001; likelihood ratio: P <.001.
d R 2 =0.851; F 6 , 2879 =133.39; P <.001.
e R 2 =0.852; F 10 , 2879 =84.94; P <.001; Wald test: P <.001; likelihood ratio: P <.001.
Analysis of Results
Web-based medical platforms offer a variety of functions to support doctors’ engagement in different types of prosocial behaviors. However, few studies have investigated the effects of these behaviors. Drawing on FMT and SET, this study categorized doctors’ prosocial practices in web-based health care communities into proactive and reactive actions and examined their effects on e-consultation volume. Briefly, prosocial behaviors positively impact on e-consultation, and a doctor’s digital and offline reputation moderates the relationship between prosocial behavior and e-consultation, albeit with some nuances.
First, we expanded upon existing literature on proactive prosocial behaviors, concluding that these actions can help doctors create professional images [ 43 ] in the medical consultation context. Our panel data analysis reveals that doctors’ posting of professional articles, which contribute to their professional image in the digital context, attracts more e-consultations. This finding aligns with the prior study [ 31 ], which observed that a health professional’s previous asynchronous prosocial behavior positively influences their future economic performance.
Second, drawing from SET, we analyzed the impact of synchronous reactive prosocial behaviors, a less explored area in prior literature. Our findings confirm that engaging in medical live streaming, a form of reactive prosocial behavior, leads to higher e-consultation volumes. Interestingly, we found that the positive impact of conducting a live stream exceeds that of posting an article.
Third, we expanded our research by testing the moderating roles of digital and offline reputations, measured by doctors’ offline professional titles and patients’ recommendations on web-based health care communities. We found that digital reputations significantly moderate the relationships between both types of prosocial behaviors and e-consultation volume. Specifically, doctors who post professional articles or conduct medical live streams attract more e-consultations when they have fewer patient recommendations compared to those with higher recommendations. Regarding offline professional titles, our results indicate a significant moderating effect on the relationship between proactive prosocial behaviors and e-consultation volume. Notably, junior doctors should focus more on posting articles in web-based health care communities to compensate for limitations associated with their titles [ 66 ]. However, the moderating effect of offline titles on the impact of reactive prosocial behaviors was found to be insignificant. We attribute this to the unique dynamics of trust conversion in Chinese health care settings. As doctors’ offline titles are granted by medical institutions, these titles could enhance patients’ trust in doctors only if there is a conversion of trust from the organization to the individual doctor, which represents different types of trust [ 67 ]. Consequently, doctors with the same offline titles from different hospitals may be perceived differently. For example, a senior doctor from a 3-A hospital is usually seen as highly professional in their clinical field, while a doctor with the same title in a 1-A hospital might typically handle primary diseases. Due to this trust conversion phenomenon, patients may not uniformly trust doctors from different hospitals with the same offline titles, leading to the insignificant moderating effect of offline titles on the impact of reactive prosocial behaviors.
In summary, this study underscores the importance of prosocial behaviors and reputation in shaping doctors’ e-consultation volumes on web-based health care communities, offering valuable insights for health care professionals aiming to increase their consultation outreach.
Implications
This study makes several theoretical implications. First, this study contributes to web-based health care community literature by offering a nuanced understanding of how doctors’ prosocial behaviors enhance e-consultation volume. While a limited number of studies have examined the effects of doctors’ freely provided behaviors in the digital context [ 31 ], the specific impact of different types of prosocial behaviors on e-consultation volume remains largely unexplored. This study addresses this knowledge gap by theoretically categorizing doctors’ prosocial behaviors in web-based health care communities into proactive and reactive types and exploring their impacts on e-consultations.
Second, this study enriches web-based health care communities and live streaming literature by validating the role of medical live streaming in web-based health services. Prior research on live streaming has mainly concentrated on e-commerce [ 68 ], web-based gaming [ 69 ], and web-based learning [ 70 ]. Our study extends this research to the health care context, highlighting the importance of live streaming on web-based health care platforms. Specifically, this study delves into how doctors’ synchronous, reactive volunteer interactions via live streaming influence patient decision-making.
Finally, this study advances FMT and SET by highlighting the importance of context in theory development and providing guidance for context-specific theorizing on web-based health platforms. It also sheds light on how the impact of different prosocial behaviors on e-consultation volume varies depending on a doctor’s offline and digital reputations. Notably, this study validates that proactive behaviors work more effectively in promoting e-consultations for doctors with lower titles or fewer digital recommendations, while reactive behaviors are more effective for doctors with fewer digital recommendations.
This study offers several practical implications for doctors and platform managers. First, the beneficial effects of prosocial behaviors suggest that doctors should adapt their engagement activities when participating in web-based health care platforms. Nowadays, an increasing number of doctors are joining web-based health care communities and focusing on e-consultations, attracted by the economic and social benefits. Based on our results, posting professional articles can help doctors establish a professional image, potentially leading to more e-consultations. Additionally, conducting medical live streams can bolster e-consultations by fostering cooperative social value for doctors and enhancing their credibility among patient audiences. Therefore, doctors may prefer engaging in both proactive and reactive prosocial activities in web-based health care communities to attract more patients to their e-consultation services.
Second, the boundary conditions of the effects of prosocial behaviors imply that doctors should strategically leverage the beneficial effect of proactive and reactive behaviors according to their offline and digital reputations. Doctors with fewer digital recommendations should focus more on prosocial behavior to attract patients to e-consultations. Meanwhile, doctors with lower titles should devote their efforts to proactive behaviors to demonstrate their capability in fulfilling the e-consultations, thereby reducing information asymmetry between patients and themselves.
Third, our findings offer implications for web-based health care platform managers in designing effective functions. An increasing number of platforms are launching various features to better serve doctors and patients, meeting the needs of both groups more effectively. Our empirical findings suggest that doctors’ proactive and reactive prosocial behaviors, such as posting professional articles and conducting medical live streams, can help them establish professional image and enhance patient trust, leading to improved performance. Importantly, these behaviors also benefit patients by enhancing their health knowledge and literacy. Thus, platform managers could introduce functions (eg, article posting, live streaming, and doctor-driven communities) to encourage more prosocial behaviors by doctors. Additionally, platform managers might consider incorporating guidelines or incentive mechanisms for prosocial behaviors into their platforms. For example, it is recommended that platforms collect and analyze doctors’ proactive and reactive prosocial behaviors and guide them on how to effectively use these functions and engage in different types of activities.
Limitations
Despite its contributions, this study also presents several limitations that future research should consider. First, various classifications of prosocial behavior are available; for instance, Richaud et al [ 71 ] classified such behavior as altruistic, compliant, emotional, public, anonymous, or dire actions. Given the intricacy of web-based medical services, future studies would benefit from further exploring the roles of these other types of prosocial behavior exhibited by doctors on web-based health care communities. Second, our research model was constructed primarily from the doctor’s perspective and thus did not investigate the influence of doctors’ prosocial behaviors on patients’ satisfaction and well-being. Future research should delve into these relationships to obtain a more comprehensive understanding of the impacts of doctors’ prosocial behaviors. Finally, this study focused only on the quantity of medical live-streaming sessions, overlooking the quality aspect, which could be a crucial factor influencing e-consultation volume. Future research will concentrate on exploring this aspect.
Conclusions
Building upon prior studies on doctors’ prosocial behaviors on web-based health care communities, this study further delineates doctors’ beneficial actions into proactive and synchronous reactive behaviors. This distinction is based on the divergence in doctors’ motives for engaging and patients’ levels of involvement. Drawing from FMT and SET, this study offers insights that could aid doctors in increasing their e-consultation volume by adopting these beneficial behaviors. Concurrently, this research augments our understanding of the roles a doctor’s reputation plays in the relationships between various prosocial behaviors—specifically, proactive and reactive actions—and their e-consultation volume. This study may inspire doctors with comparatively lower offline professional titles and digital popularity to achieve their desired e-consultation volume.
Acknowledgments
This work was supported by the National Natural Science Foundation of China (72001170 and 72102179), the Fundamental Research Funds for the Central Universities (SK2024028), the Ministry of Education in China Project of Humanities and Social Sciences (21XJC630003), the China Postdoctoral Science Foundation (2022T150515, 2023M742818, and 2020M673432), the National Natural Science Foundation of China (72004042), and the Heilongjiang Natural Science Foundation (YQ2023G003), and the grants from City University of Hong Kong (projects 7005959, 7006152, and 7200725).
Conflicts of Interest
None declared.
- Qi M, Cui J, Li X, Han Y. Influence of e-consultation on the intention of first-visit patients to select medical services: results of a scenario survey. J Med Internet Res. 2023;25:e40993. [ FREE Full text ] [ CrossRef ] [ Medline ]
- Cao B, Huang W, Chao N, Yang G, Luo N. Patient activeness during online medical consultation in China: multilevel analysis. J Med Internet Res. 2022;24(5):e35557. [ FREE Full text ] [ CrossRef ] [ Medline ]
- Jiang S. Talk to your doctors online: an internet-based intervention in China. Health Commun. 2021;36(4):405-411. [ FREE Full text ] [ CrossRef ] [ Medline ]
- Jiang J, Cameron AF, Yang M. Analysis of massive online medical consultation service data to understand physicians' economic return: observational data mining study. JMIR Med Inform. 2020;8(2):e16765. [ FREE Full text ] [ CrossRef ] [ Medline ]
- Street RL, Millay B. Analyzing patient participation in medical encounters. Health Commun. 2001;13(1):61-73. [ FREE Full text ] [ CrossRef ] [ Medline ]
- Ren D, Ma B. Effectiveness of interactive tools in online health care communities: social exchange theory perspective. J Med Internet Res. 2021;23(3):e21892. [ FREE Full text ] [ CrossRef ] [ Medline ]
- Guo S, Guo X, Fang Y, Vogel D. How doctors gain social and economic returns in online health-care communities: a professional capital perspective. J Manag Inf Syst. 2017;34(2):487-519. [ FREE Full text ] [ CrossRef ]
- Tande AJ, Berbari EF, Ramar P, Ponamgi SP, Sharma U, Philpot L, et al. Association of a remotely offered infectious diseases eConsult service with improved clinical outcomes. Open Forum Infect Dis. 2020;7(1):ofaa003. [ FREE Full text ] [ CrossRef ] [ Medline ]
- Richardson BR, Truter P, Blumke R, Russell TG. Physiotherapy assessment and diagnosis of musculoskeletal disorders of the knee via telerehabilitation. J Telemed Telecare. 2017;23(1):88-95. [ CrossRef ] [ Medline ]
- Castaneda PR, Duffy B, Andraska EA, Stevens J, Reschke K, Osborne N, et al. Outcomes and safety of electronic consult use in vascular surgery. J Vasc Surg. 2020;71(5):1726-1732. [ FREE Full text ] [ CrossRef ] [ Medline ]
- Jiang S. The relationship between face-to-face and online patient-provider communication: examining the moderating roles of patient trust and patient satisfaction. Health Commun. 2020;35(3):341-349. [ FREE Full text ] [ CrossRef ] [ Medline ]
- Li J, Tang J, Jiang L, Yen DC, Liu X. Economic success of physicians in the online consultation market: a signaling theory perspective. Int J Electron Commer. 2019;23(2):244-271. [ FREE Full text ] [ CrossRef ]
- Guo S, Guo X, Zhang X, Vogel D. Doctor–patient relationship strength’s impact in an online healthcare community. Inf Technol Dev. 2017;24(2):279-300. [ FREE Full text ] [ CrossRef ]
- Audrain-Pontevia AF, Menvielle L. Do online health communities enhance patient-physician relationship? an assessment of the impact of social support and patient empowerment. Health Serv Manage Res. 2018;31(3):154-162. [ FREE Full text ] [ CrossRef ] [ Medline ]
- Yang Y, Zhang X, Lee PK. Improving the effectiveness of online healthcare platforms: an empirical study with multi-period patient-doctor consultation data. Int J Prod Econ. 2019;207:70-80. [ FREE Full text ] [ CrossRef ]
- Fan W, Zhou Q, Qiu L, Kumar S. Should doctors open online consultation services? An empirical investigation of their impact on offline appointments. Inf Syst Res. 2023;34(2):629-651. [ FREE Full text ] [ CrossRef ]
- Liu QB, Liu X, Guo X. The effects of participating in a physician-driven online health community in managing chronic disease: evidence from two natural experiments. MIS Q. 2020;44(1):391-419. [ FREE Full text ] [ CrossRef ]
- Yan Z, Wang T, Chen Y, Zhang H. Knowledge sharing in online health communities: a social exchange theory perspective. Inf Manag. 2016;53(5):643-653. [ FREE Full text ] [ CrossRef ]
- Luo P, Chen K, Wu C, Li Y. Exploring the social influence of multichannel access in an online health community. J Assoc Inf Sci Technol. 2018;69(1):98-109. [ FREE Full text ] [ CrossRef ]
- Chai S, Bagchi-Sen S, Morrell C, Rao HR, Upadhyaya SJ. Internet and online information privacy: an exploratory study of preteens and early teens. IEEE Trans Profess Commun. 2009;52(2):167-182. [ FREE Full text ] [ CrossRef ]
- Ahearne M, Rapp A, Hughes DE, Jindal R. Managing sales force product perceptions and control systems in the success of new product introductions. J Mark Res. 2010;47(4):764-776. [ FREE Full text ] [ CrossRef ]
- Awaysheh A, Heron RA, Perry T, Wilson JI. On the relation between corporate social responsibility and financial performance. Strateg Manag J. 2020;41(6):965-987. [ FREE Full text ] [ CrossRef ]
- Coll MP, Grégoire M, Eugène F, Jackson PL. Neural correlates of prosocial behavior towards persons in pain in healthcare providers. Biol Psychol. 2017;128:1-10. [ FREE Full text ] [ CrossRef ] [ Medline ]
- Kapıkıran NA. Sources of ethnocultural empathy: personality, intergroup relations, affects. Curr Psychol. 2021;42(14):11510-11528. [ FREE Full text ] [ CrossRef ]
- Yin Y, Wang Y. Is empathy associated with more prosocial behaviour? a meta‐analysis. Asian J Soc Psychol. 2023;26(1):3-22. [ FREE Full text ] [ CrossRef ]
- Finset A. "I am worried, Doctor!" emotions in the doctor-patient relationship. Patient Educ Couns. 2012;88(3):359-363. [ FREE Full text ] [ CrossRef ] [ Medline ]
- Larson EB, Yao X. Clinical empathy as emotional labor in the patient-physician relationship. JAMA. 2005;293(9):1100-1106. [ FREE Full text ] [ CrossRef ] [ Medline ]
- Bloom G, Standing H, Lloyd R. Markets, information asymmetry and health care: towards new social contracts. Soc Sci Med. 2008;66(10):2076-2087. [ FREE Full text ] [ CrossRef ] [ Medline ]
- Guo F, Zou B, Guo J, Shi Y, Bo Q, Shi L. What determines academic entrepreneurship success? A social identity perspective. Int Entrep Manag J. 2019;15(3):929-952. [ FREE Full text ] [ CrossRef ]
- Yang H, Zhang X. Investigating the effect of paid and free feedback about physicians' telemedicine services on patients' and physicians' behaviors: panel data analysis. J Med Internet Res. 2019;21(3):e12156. [ FREE Full text ] [ CrossRef ] [ Medline ]
- Yan Z, Kuang L, Qiu L. Prosocial behaviors and economic performance: evidence from an online mental healthcare platform. Prod Oper Manag. 2022;31(10):3859-3876. [ FREE Full text ] [ CrossRef ]
- Frese M, Fay D. 4. Personal initiative: an active performance concept for work in the 21st century. Res Organ Behav. 2001;23:133-187. [ FREE Full text ] [ CrossRef ]
- Frese M, Kring W, Soose A, Zempel J. Personal initiative at work: differences between East and West Germany. Acad Manage J. 1996;39(1):37-63. [ FREE Full text ] [ CrossRef ]
- Spitzmuller M, Van Dyne L. Proactive and reactive helping: contrasting the positive consequences of different forms of helping. J Organ Behavior. 2013;34(4):560-580. [ FREE Full text ] [ CrossRef ]
- Bandura A. Social cognitive theory: an agentic perspective. Annu Rev Psychol. 2001;52(1):1-26. [ FREE Full text ] [ CrossRef ] [ Medline ]
- Mittal S, Sengupta A, Agrawal NM, Gupta S. How prosocial is proactive: developing and validating a scale and process model of knowledge-based proactive helping. J Manag Organ. 2018;26(4):625-650. [ FREE Full text ] [ CrossRef ]
- Walter A, Ritter T, Gemünden HG. Value creation in buyer–seller relationships: theoretical considerations and empirical results from a supplier's perspective. Ind Mark Manag. 2001;30(4):365-377. [ FREE Full text ] [ CrossRef ]
- Eggert A, Ulaga W, Schultz F. Value creation in the relationship life cycle: a quasi-longitudinal analysis. Ind Mark Manag. 2006;35(1):20-27. [ FREE Full text ] [ CrossRef ]
- Deng Z, Hong Z, Zhang W, Evans R, Chen Y. The effect of online effort and reputation of physicians on patients' choice: 3-wave data analysis of China's good doctor website. J Med Internet Res. 2019;21(3):e10170. [ FREE Full text ] [ CrossRef ] [ Medline ]
- Kurihara H, Maeno T, Maeno T. Importance of physicians' attire: factors influencing the impression it makes on patients, a cross-sectional study. Asia Pac Fam Med. 2014;13(1):2. [ FREE Full text ] [ CrossRef ] [ Medline ]
- Cooper ML, Shapiro CM, Powers AM. Motivations for sex and risky sexual behavior among adolescents and young adults: a functional perspective. J Pers Soc Psychol. 1998;75(6):1528-1558. [ CrossRef ] [ Medline ]
- Clary EG, Snyder M, Ridge RD, Copeland J, Stukas AA, Haugen J, et al. Understanding and assessing the motivations of volunteers: a functional approach. J Pers Soc Psychol. 1998;74(6):1516-1530. [ CrossRef ] [ Medline ]
- Epstein RM, Hundert EM. Defining and assessing professional competence. JAMA. 2002;287(2):226-235. [ FREE Full text ] [ CrossRef ] [ Medline ]
- Zhang L, Qiu Y, Zhang N, Li S. How difficult doctor‒patient relationships impair physicians' work engagement: the roles of prosocial motivation and problem-solving pondering. Psychol Rep. 2020;123(3):885-902. [ FREE Full text ] [ CrossRef ] [ Medline ]
- Dabholkar PA, Johnston WJ, Cathey AS. The dynamics of long-term business-to-business exchange relationships. J Acad Mark Sci. 1994;22(2):130-145. [ FREE Full text ] [ CrossRef ]
- Ochs M, Mestre D, de Montcheuil G, Pergandi JM, Saubesty J, Lombardo E, et al. Training doctors’ social skills to break bad news: evaluation of the impact of virtual environment displays on the sense of presence. J Multimodal User Interfaces. 2019;13(1):41-51. [ FREE Full text ] [ CrossRef ]
- Schroeder T, Seaman K, Nguyen A, Gewald H, Georgiou A. Enablers and inhibitors to the adoption of mHealth apps by patients—a qualitative analysis of German doctors' perspectives. Patient Educ Couns. 2023;114:107865. [ FREE Full text ] [ CrossRef ] [ Medline ]
- Liu X, Hu M, Xiao BS, Shao J. Is my doctor around me? investigating the impact of doctors’ presence on patients’ review behaviors on an online health platform. J Assoc Inf Sci Technol. 2022;73(9):1279-1296. [ FREE Full text ] [ CrossRef ]
- Chen Y, Xie J. Online consumer review: word-of-mouth as a new element of marketing communication mix. Manage Sci. 2008;54(3):477-491. [ FREE Full text ] [ CrossRef ]
- Ye Q, Li Y, Kiang M, Wu W. The impact of seller reputation on the performance of online sales: evidence from TaoBao Buy-It-Now (BIN) data. SIGMIS Database. 2009;40(1):12-19. [ FREE Full text ] [ CrossRef ]
- Liu X, Guo X, Wu H, Wu T. The impact of individual and organizational reputation on physicians’ appointments online. Int J Electron Commer. 2016;20(4):551-577. [ FREE Full text ] [ CrossRef ]
- Dewan S, Hsu V. Adverse selection in electronic markets: evidence from online stamp auctions. J Ind Econ. 2004;52(4):497-516. [ CrossRef ]
- Torres E, Vasquez-Parraga AZ, Barra C. The path of patient loyalty and the role of doctor reputation. Health Mark Q. 2009;26(3):183-197. [ FREE Full text ] [ CrossRef ] [ Medline ]
- Gottschalk F, Mimra W, Waibel C. Health services as credence goods: a field experiment. Econ J. 2020;130(629):1346-1383. [ FREE Full text ] [ CrossRef ]
- Nunkoo R, Ramkissoon H. Power, trust, social exchange and community support. Ann Tour Res. 2012;39(2):997-1023. [ FREE Full text ] [ CrossRef ]
- Blau PM. Exchange and Power in Social Life. New Brunswick. Transaction Publishers; 1964.
- Molm LD, Takahashi N, Peterson G. Risk and trust in social exchange: an experimental test of a classical proposition. Am J Sociol. 2000;105(5):1396-1427. [ FREE Full text ] [ CrossRef ]
- Lioukas CS, Reuer JJ. Isolating trust outcomes from exchange relationships: social exchange and learning benefits of prior ties in alliances. Acad Manage J. 2015;58(6):1826-1847. [ FREE Full text ] [ CrossRef ]
- Malgonde OS, Saldanha TJ, Mithas S. Resilience in the open source software community: how pandemic and unemployment shocks influence contributions to others’ and one’s own projects. MIS Q. 2023;47(1):361-390. [ FREE Full text ] [ CrossRef ]
- Mayya R, Ye S, Viswanathan S, Agarwal R. Who forgoes screening in online markets and why? Evidence from Airbnb. MIS Q. 2021;45(4):1745-1776. [ FREE Full text ] [ CrossRef ]
- Moqri M, Mei X, Qiu L, Bandyopadhyay S. Effect of “following” on contributions to open source communities. J Manag Inf Syst. 2018;35(4):1188-1217. [ FREE Full text ] [ CrossRef ]
- Chen Q, Jin J, Zhang T, Yan X. The effects of log-in behaviors and web reviews on patient consultation in online health communities: longitudinal study. J Med Internet Res. 2021;23(6):e25367. [ FREE Full text ] [ CrossRef ] [ Medline ]
- Mo J, Sarkar S, Menon S. Competing tasks and task quality: an empirical study of crowdsourcing contests. MIS Q. 2021;45(4):1921-1948. [ FREE Full text ] [ CrossRef ]
- Gourieroux C, Holly A, Monfort A. Likelihood ratio test, Wald test, and Kuhn-Tucker test in linear models with inequality constraints on the regression parameters. Econometrica. 1982;50(1):63. [ CrossRef ]
- Peng CH, Yin D, Zhang H. More than words in medical question-and-answer sites: a content-context congruence perspective. Inf Syst Res. 2020;31(3):913-928. [ FREE Full text ] [ CrossRef ]
- Guo L, Jin B, Yao C, Yang H, Huang D, Wang F. Which doctor to trust: a recommender system for identifying the right doctors. J Med Internet Res. 2016;18(7):e186. [ FREE Full text ] [ CrossRef ] [ Medline ]
- Zheng S, Hui SF, Yang Z. Hospital trust or doctor trust? A fuzzy analysis of trust in the health care setting. J Bus Res. 2017;78:217-225. [ FREE Full text ] [ CrossRef ]
- Mao Z, Du Z, Yuan R, Miao Q. Short-term or long-term cooperation between retailer and MCN? new launched products sales strategies in live streaming e-commerce. J Retail Consum Serv. 2022;67:102996. [ FREE Full text ] [ CrossRef ]
- Hilvert-Bruce Z, Neill JT, Sjöblom M, Hamari J. Social motivations of live-streaming viewer engagement on Twitch. Comput Hum Behav. 2018;84:58-67. [ FREE Full text ] [ CrossRef ]
- Tang YM, Chen PC, Law KMY, Wu CH, Lau YY, Guan J, et al. Comparative analysis of student's live online learning readiness during the coronavirus (COVID-19) pandemic in the higher education sector. Comput Educ. 2021;168:104211. [ FREE Full text ] [ CrossRef ] [ Medline ]
- Richaud MC, Mesurado B, Cortada AK. Analysis of dimensions of prosocial behavior in an Argentinean sample of children. Psychol Rep. 2012;111(3):687-696. [ FREE Full text ] [ CrossRef ] [ Medline ]
Abbreviations
Edited by G Eysenbach; submitted 11.09.23; peer-reviewed by P Luo, Y Zhu, C Fu; comments to author 05.10.23; revised version received 30.12.23; accepted 09.03.24; published 25.04.24.
©Xiaoxiao Liu, Huijing Guo, Le Wang, Mingye Hu, Yichan Wei, Fei Liu, Xifu Wang. Originally published in the Journal of Medical Internet Research (https://www.jmir.org), 25.04.2024.
This is an open-access article distributed under the terms of the Creative Commons Attribution License (https://creativecommons.org/licenses/by/4.0/), which permits unrestricted use, distribution, and reproduction in any medium, provided the original work, first published in the Journal of Medical Internet Research, is properly cited. The complete bibliographic information, a link to the original publication on https://www.jmir.org/, as well as this copyright and license information must be included.
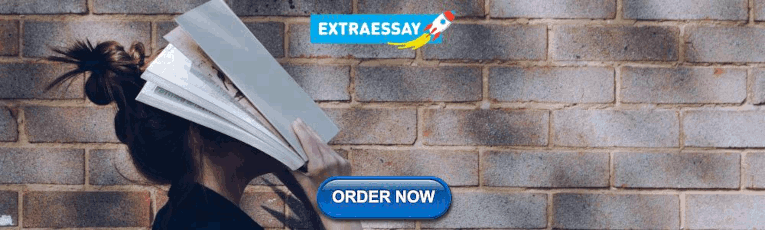
IMAGES
VIDEO
COMMENTS
The greatest strength of the qualitative research approach lies in the richness and depth of the healthcare exploration and description it makes. In health research, these methods are considered as the most humanistic and person-centered way of discovering and uncovering thoughts and actions of human beings. Table 1.
Health research methodology: A guide for training in research methods INTRODUCTION This is a revised version of an earlier manual on Health Research Methodology and deals with the basic concepts and principles of scientific research methods with particular attention to research in the health field. The research process is the cornerstone for ...
Quantitative and qualitative research explore various social phenomena using different methods. However, there has been a tendency to treat quantitative studies using complicated statistical techniques as more scientific and superior, whereas relatively few qualitative studies have been conducted in the medical and healthcare fields.
JOURNAL HOMEPAGE. Research Methods in Medicine & Health Sciences is a peer reviewed journal, publishing rigorous research on established "gold standard" methods and new cutting edge research methods in the health sciences and clinical medicine. View full journal description. This journal is a member of the Committee on Publication Ethics ...
It is just as applicable to clinical trials as it is to health services research, enabling an in depth understanding of the problem that quantitative research alone cannot deliver. 1 It is imperative, however, that qualitative studies are well-designed with pre-determined methods to ensure reduction in research bias. While quantitative research ...
The purpose classification of mixed research methods was first introduced in 1989 by Greene, Caracelli, and Graham, based on an analysis of published studies of mixed methods. This classification is still used and we have a total of five "purposes" for why a mixed methodology is used in research (4). Classification of the purpose of using ...
Healthcare research is a systematic inquiry intended to generate robust evidence about important issues in the fields of medicine and healthcare. Qualitative research has ample possibilities within the arena of healthcare research. ... In health research, these methods are considered as the most humanistic and person-centered way of discovering ...
Content last reviewed April 2022. Effective Health Care Program, Agency for Healthcare Research and Quality, Rockville, MD. The Evidence-based Practice Center (EPC) Program has developed evidence synthesis guidance, tools, and education resources for those conducting and learning about research synthesis. Select a topic below to learn more.
In health services research (HSR), a broad spectrum of qualitative research methods is used to explore the perspectives of patients, healthcare professionals and other actors to gain insights into healthcare practice, health policy and health interventions. Research design, methods for data collection and data analysis depend primarily on the ...
Several qualitative health research methods, when done well, can help research teams to: (1) accurately incorporate patients' perspectives and experiences into the design and conduct of research; (2) engage diverse patient perspectives; and, (3) treat patients as equal and ongoing partners on the research team. This commentary presents ...
The Patient-Centered Outcomes Research Institute's (PCORI) methodology standards for qualitative methods and mixed methods research help ensure that research studies are designed and conducted to generate the evidence needed to answer patients' and clinicians' questions about which methods work best, for whom, and under what circumstances. This set of standards focuses on factors ...
Reference numbers. ISBN: 92 9021 363 9. This comprehensive guide to health research reaches out to a wide spectrum of people: students who wish to learn the basic principles of health research and how to conduct it, field researchers, and those involved in teaching and training of health research methodologies.
A practical introduction to epidemiology, biostatistics, and research methodology for the whole health care community This comprehensive text, which has been extensively revised with new material and additional topics, utilizes a practical slant to introduce health professionals and students to epidemiology, biostatistics, and research methodology. It draws examples from a wide range of topics ...
This is the methods section to a report that describes three decades (1990-2020) of AHRQ's primary care research and highlights how that research has impacted delivery of primary care. It details AHRQ's steady investment in research to improve primary care organization, workforce, quality and safety, digital healthcare, finance and cost, and prevention.
Research Methods for Health Care Practice guides the researcher through their journey, giving detailed, step-by-step advice on planning and carrying out each stage of the research. Useful examples from health care research are included throughout to illustrate the application of the techniques and methods discussed.
The use of research questionnaires or surveys in nursing is a long standing tradition, dating back to the 1960s (Logan, 1966) and 1970s (Oberst, 1978), when the scientific discipline emerged.This type of tool enables nursing researchers to gather primary data from a specific population, whether it is patients, carers, nurses, or other stakeholders to address gaps in the existing evidence base ...
Observational methods are typically used in planning research when data cannot be obtained through interviews or from secondary sources. This approach is particularly useful when a process is being analyzed. For example, a hospital might place a trained observer in its waiting area to observe the admitting process.
Supporting transformative primary care research, tools and methods to improve the delivery of primary care. ... The Agency for Healthcare Research and Quality's (AHRQ) mission is to produce evidence to make health care safer, higher quality, more accessible, equitable, and affordable, and to work within the U.S. Department of Health and Human ...
Healthcare Research Methods Bernadette Howlett, PhD INTRODUCTION Research methods and statistics are foundational concepts for evidence-based practice (EBP). This chapter was written with the expectation that you have taken courses at the undergraduate level that address both research methods and statistics. We recommend that you have books
Grounded theory, phenomenology and ethnography are three approaches used in qualitative research. Grounded theory approach is a commonly used qualitative method in the social sciences to inductively generate or discover a theory out of the data. 9 Phenomenology and ethnography are more commonly used qualitative approaches in health care and ...
The growing adoption of continuous quality improvement (CQI) initiatives in healthcare has generated a surge in research interest to gain a deeper understanding of CQI. However, comprehensive evidence regarding the diverse facets of CQI in healthcare has been limited. Our review sought to comprehensively grasp the conceptualization and principles of CQI, explore existing models and tools ...
Integrating a Rapid Research Approach. The Socially Equitable Care by Understanding Resource Engagement (SECURE) study is a mixed method pragmatic trial aimed at understanding how best to increase family-level engagement with social resources from the pediatric health care setting. Caregivers in the study were randomized to complete one of ...
In the Applied Machine Intelligence Program, experts use visualization methods and computational data from biological and clinical knowledge domains to develop new theories and algorithms for machine intelligence. Team members engage in research across all facets of machine learning, including: Classical machine learning algorithms.
Study design and setting. The program feasibility was evaluated through an explorative quality-improvement study using a mixed-methods approach in a concurrent triangulation design [].The following program feasibility domains were addressed []: (1) appropriateness, feasibility, and acceptability for quality-improvement; (2) impact on clinical performance; and (3) impact on learning and ...
Patient and Family Engagement in Primary Care. Teach-back is a technique for health care providers to ensure that they have explained medical information clearly so that patients and their families understand what is communicated to them. This intervention includes several materials to support adoption. Note: Resources on this page were used in ...
Methods: We conducted semistructured interviews with 36 participants—15 (42%) obstetric health professionals, 10 (28%) mental health professionals, and 11 (31%) postpartum Black patients. ... Journal of Medical Internet Research 8325 articles ... (200) Systemic Racism and Racial Bias in Health Care and Society (66) Focus Groups and ...
The research was conducted with 209 nurses in a hospital. The data collection process of this study was conducted at the 2019-2020 academic year. ... The IEMT training game developed by the instructor team is a promising and effective method for training nurses in disaster rescue within IEMTs. ... World Health Organization.Classification and ...
The twelfth Health Survey Research Methods Conference will continue the series that began 50 years ago to discuss innovative survey research methods that improve the quality of health survey data. The next conference will be held in Williamsburg, VA, from March 4-7, 2025, and HSRMC is seeking abstracts by June 30, 2024 for papers to be presented at the conference.
Researchers explore causal machine learning, a new advancement for AI in health care. by Ludwig Maximilian University of Munich. Formalizing tasks for causal ML. Credit: Nature Medicine (2024 ...
This paper is in the following e-collection/theme issue: Peer-to-Peer Support and Online Communities (557) Virtual Communities and Communities of Practice for Healthcare Providers (41) Physician and Health Services Rating by Consumers (124) Quality/Credibility of eHealth Information and Trust Issues (471) Health Care Quality and Health Services Research (221) Clinical Communication, Electronic ...