Comprehensive survey on data warehousing research
- Original Research
- Published: 15 December 2017
- Volume 10 , pages 217–224, ( 2018 )
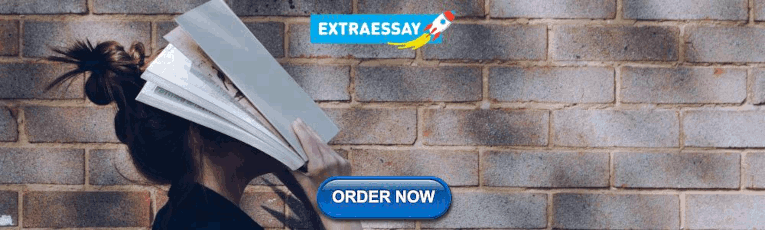
Cite this article
- Pravin Chandra 1 &
- Manoj K. Gupta 2
1399 Accesses
13 Citations
Explore all metrics
Data, information and knowledge have important role in various human activities because by processing data, information is extracted and by analyzing data and information the knowledge is extracted. The problem of storing, managing and analyzing the huge volumes of data, which is generated regularly by the various sources has been arisen which leads to the need of large data repositories, e.g. data warehouses. In view of the above, a considerable amount attention of research and industry has been attracted by the data warehousing (DW). Various issues and challenges in the field of data warehousing are presented in many studies during the recent years. In this paper, a comprehensive survey is presented to take a holistic view of the research trends in the fields of data warehousing. This paper presents a systematic division of work of researchers in the fields of data warehousing. Finally, current research issues and challenges in the area of data warehousing are summarized for future directions.
This is a preview of subscription content, log in via an institution to check access.
Access this article
Price includes VAT (Russian Federation)
Instant access to the full article PDF.
Rent this article via DeepDyve
Institutional subscriptions
Similar content being viewed by others
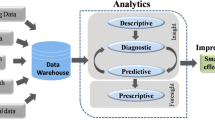
Big data in healthcare: management, analysis and future prospects
Sabyasachi Dash, Sushil Kumar Shakyawar, … Sandeep Kaushik
Trends and Future Perspective Challenges in Big Data
Big Data Analytics: Applications, Prospects and Challenges
Akal F, Böhm K, Schek HJ (2002) OLAP query evaluation in a database cluster: a performance study on intra-query parallelism. In: East-European conf. on advances in databases and information systems (ADBIS), Bratislava, Slovakia
Aleem S, Capretz LF, Ahmed F (2014) Security issues in data warehouse. In: Mastorakis NE, Musić J (eds) Recent advances in information technology. WSEAS Press, pp 15–20
Arora M, Gosain A (2011) Schema evolution for data warehouse: a survey. Int J Comput Appl 22(6):6–14
Google Scholar
Arora RK, Gupta MK (2017) e-Governance using data warehousing and data mining. Int J Comput Appl 169(8):28–31
Astriani W, Trisminingsih R (2015) Extraction, transformation, and loading (ETL) module for hotspot spatial data warehouse using Geokettle. In: Procedia, environmental science, Elsevier, the 2nd international symposium on LAPAN-IPB satellite for food security and environmental monitoring 2015, LISAT-FSEM 2015
Chaudhary S, Murala DP, Srivastav VK (2011) A critical review of data warehouse. Glob J Bus Manag Inf Technol 1(2):95–103
Chaudhuri S, Dayal U (1997) An overview of data warehousing and OLAP technology. ACM SIGMOD Rec 26:517–526
Article Google Scholar
Codd EF, Codd SB, Salley CT (1993) Providing OLAP (On-line Analytical Processing) to user-analysts: an IT mandate (white paper)
Dehne F, Robillard D, Rau-Chaplin A, Burke N (2016) VOLAP: a scalable distributed system for real-time OLAP with high velocity data. In: 2016 IEEE international conference on cluster computing (CLUSTER). IEEE, pp 354–363
ElGamal N, El-Bastawissy A, Galal-Edeen GH (2016) An architecture-oriented data warehouse testing approach. In: COMAD, pp 24–34
Furtado P (2009) A survey on parallel and distributed data warehouses. Int J Data Warehouse Min 5(2):57–77
Geary N, Jarvis B, Mew C, Gore H, Precisionpoint Software Limited (2017) Method and apparatus for automatically creating a data warehouse and OLAP cube. US Patent 9,684,703
Golfarelli M, Rizzi S (2009) A comprehensive approach to data warehouse testing. In: ACM, DOLAP’09, Hong Kong, China, November 6, 2009
Golfarelli M, Rizzi S (2018) From star schemas to big data: 20+ years of data warehouse research. In: A comprehensive guide through the Italian database research over the last 25 years. Springer International Publishing, pp 93–107
Gosain A, Heena (2015) Literature review of data model quality metrics of data warehouse. In: Procedia, computer science, Elsevier, international conference on intelligent computing, communication and convergence (ICCC-2014)
Gupta A, Harinarayan V, Quass D (1995) Aggregate-query processing in data warehousing environment. In: Proc. 21st int. conf. very large data bases, pp 358–369, Zurich, Switzerland, Sept. 1995
Gupta SL, Mathur S, Schema P (2012) Data warehouse vulnerability and security. Int J Sci Eng Res 3(5):1–5
Haertzen D (2009) Testing the data warehouse. http://www.infogoal.com
Han J, Kamber M, Pei J (2012) Data mining concepts and techniques, 3rd edn. Elsevier
Hurtado CA, Gutierrez C, Mendelzon AO (2005) Capturing summarizability with integrity constraints in OLAP. ACM Trans Database Syst 30(3):854–886
Inmon WH (2005) Building the data warehouse, 5th edn. Wiley, New York
Jaiswal A (2014) Security measures for data warehouse. Int J Sci Eng Technol Res 3(6):1729–1733
Jindal R, Taneja S (2012) Comparative study of data warehouse design approaches: a survey. Int J Database Manag Syst (IJDMS) 4(1):33–45
Kuijpers B, Gomez L, Vaisman A (2017) Performing OLAP over graph data: query language, implementation, and a case study. In: BIRTE '17 proceedings of the international workshop on real-time business intelligence and analytics, no 6. ACM, New York
Kumar S, Singh B, Kaur G (2016) Data warehouse security issue. Int J Adv Res Comput Sci 7(6):177–179
Mathen MP (2010) Data warehouse testing. Infosys White Paper, Mar 2010
Mookerjea A, Malisetty P (2008) Best practices in data warehouse testing. In: Proc. test, New Delhi, 2008
O’Neil P, Graefe G (1995) Multi-table joins through bitmapped join indices. SIGMOD Rec 24(3):8–11
Oliveira B, Belo O (2015) A domain-specific language for ETL patterns specification in data warehousing systems. In: Chapter in progress in artificial intelligence, Springer, Volume 9273 of the series lecture notes in computer science, pp 597–602
Oracle Corporation (2005) Oracle advanced security transparent data encryption best practices. Oracle White Paper, July 2010
Oueslati W, Akaichi J (2010) A survey on data warehouse evolution. Int J Database Manag Syst (IJDMS) 2(4):11–24
Ponniah P (2001) Data warehousing fundamentals. Wiley, New York
Book Google Scholar
Rizzi S, Golfarelli M (1999) A methodological framework for data warehouse design. DOLAP 98 Washington DC USA, Copyright ACM, l-581 13-120-8/98/l 1
Rousopoulos R (1998) Materialized views and data warehouses. SIGMOD Rec 27(1):21–26
Santos RJ, Bernardino J, Vieira M (2011) A survey on data security in data warehousing: issues, challenges and opportunities. In: EUROCON-International Conference on Computer as a Tool (EUROCON), 2011 IEEE, Print ISBN: 978-1-4244-7486-8
Taktak S, Alshomrani S, Feki J, Zurfluh G (2017) The power of a model-driven approach to handle evolving data warehouse requirements. In: MODELSWARD, pp 169–181
Tang B, Han S, Yiu ML, Ding R, Zhang D (2017) Extracting top-k insights from multi-dimensional data. In: Proceedings of the 2017 ACM international conference on management of data. ACM, pp 1509–1524
Trujillo J, Palomar M, Gómez J, Song IY (2001) Designing data warehouses with OO conceptual models. IEEE Comput 34(12):66–75
Vassiliadis P, Sellis T (1999) A survey of logical models for OLAP databases. SIGMOD Rec 28(4):64–69
Venkatadri M, Reddy LC (2011) A review on data mining from Past to the Future. Int J Comput Appl 15(7):19–22
Vishnu B, Manjunath TN, Hamsa C (2014) An effective data warehouse security framework. Int J Comput Appl Recent Adv Inf Technol 33–37
Wang Z, Chu Y, Tan KL, Agrawal D, Abbadi AE (2016) HaCube: extending MapReduce for efficient OLAP cube materialization and view maintenance. In: International conference on database systems for advanced applications. Springer, Cham, pp 113–129
Yangui R, Nabli A, Gargouri F (2016) Automatic transformation of data warehouse schema to NoSQL data base: comparative study. In: Procedia, computer science, Elsevier, 20th international conference on knowledge based and intelligent information and engineering systems, KES2016, 5–7 September 2016, York, UK
Zeng K, Agarwal S, Stoica I (2016) IOLAP: managing uncertainty for efficient incremental OLAP. In: Proceedings of the 2016 international conference on management of data. ACM, pp 1347–1361
Download references
Author information
Authors and affiliations.
University School of Information, Communication & Technology, Guru Gobind Singh Indraprastha University, Delhi, India
Pravin Chandra
Rukmini Devi Institute of Advanced Studies, Delhi, India
Manoj K. Gupta
You can also search for this author in PubMed Google Scholar
Corresponding author
Correspondence to Manoj K. Gupta .
Rights and permissions
Reprints and permissions
About this article
Chandra, P., Gupta, M.K. Comprehensive survey on data warehousing research. Int. j. inf. tecnol. 10 , 217–224 (2018). https://doi.org/10.1007/s41870-017-0067-y
Download citation
Received : 11 August 2017
Accepted : 05 December 2017
Published : 15 December 2017
Issue Date : June 2018
DOI : https://doi.org/10.1007/s41870-017-0067-y
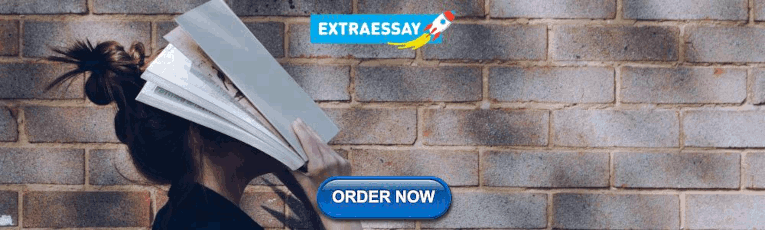
Share this article
Anyone you share the following link with will be able to read this content:
Sorry, a shareable link is not currently available for this article.
Provided by the Springer Nature SharedIt content-sharing initiative
- Data warehousing
- Data warehouse design
- Data warehouse testing
- Research trends
- Find a journal
- Publish with us
- Track your research
A Data Warehouse Approach for Business Intelligence
Ieee account.
- Change Username/Password
- Update Address
Purchase Details
- Payment Options
- Order History
- View Purchased Documents
Profile Information
- Communications Preferences
- Profession and Education
- Technical Interests
- US & Canada: +1 800 678 4333
- Worldwide: +1 732 981 0060
- Contact & Support
- About IEEE Xplore
- Accessibility
- Terms of Use
- Nondiscrimination Policy
- Privacy & Opting Out of Cookies
A not-for-profit organization, IEEE is the world's largest technical professional organization dedicated to advancing technology for the benefit of humanity. © Copyright 2024 IEEE - All rights reserved. Use of this web site signifies your agreement to the terms and conditions.
Help | Advanced Search
Computer Science > Machine Learning
Title: megalodon: efficient llm pretraining and inference with unlimited context length.
Abstract: The quadratic complexity and weak length extrapolation of Transformers limits their ability to scale to long sequences, and while sub-quadratic solutions like linear attention and state space models exist, they empirically underperform Transformers in pretraining efficiency and downstream task accuracy. We introduce Megalodon, a neural architecture for efficient sequence modeling with unlimited context length. Megalodon inherits the architecture of Mega (exponential moving average with gated attention), and further introduces multiple technical components to improve its capability and stability, including complex exponential moving average (CEMA), timestep normalization layer, normalized attention mechanism and pre-norm with two-hop residual configuration. In a controlled head-to-head comparison with Llama2, Megalodon achieves better efficiency than Transformer in the scale of 7 billion parameters and 2 trillion training tokens. Megalodon reaches a training loss of 1.70, landing mid-way between Llama2-7B (1.75) and 13B (1.67). Code: this https URL
Submission history
Access paper:.
- Other Formats
References & Citations
- Google Scholar
- Semantic Scholar
BibTeX formatted citation

Bibliographic and Citation Tools
Code, data and media associated with this article, recommenders and search tools.
- Institution
arXivLabs: experimental projects with community collaborators
arXivLabs is a framework that allows collaborators to develop and share new arXiv features directly on our website.
Both individuals and organizations that work with arXivLabs have embraced and accepted our values of openness, community, excellence, and user data privacy. arXiv is committed to these values and only works with partners that adhere to them.
Have an idea for a project that will add value for arXiv's community? Learn more about arXivLabs .
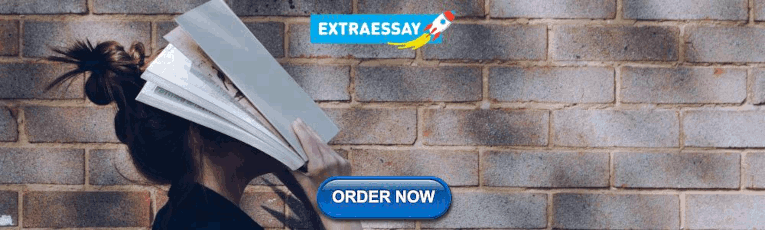
IMAGES
VIDEO
COMMENTS
Abstract. A data warehouse is a r epository for all data which is collected by an organization in various operational systems; it can. be either physical or l ogical. It is a subject oriented ...
Data Systems Research (CIDR '21), January 11-15, 2021, Online. data at low cost, but on the other hand, punted the problem of data quality and governance downstream. In this architecture, a small subset of data in the lake would later be ETLed to a downstream data warehouse (such as Teradata) for the most important decision
Data extraction from "foreign" sources is usually implemented via gateways and standard interfaces (such as Information Builders EDA/SQL, ODBC, Oracle Open Connect, Sybase Enterprise Connect, Informix Enterprise Gateway). Data Cleaning Since a data warehouse is used for decision making, it is important that the data in the warehouse be correct.
The paper will show the whole process of a data warehouse along with 3 case studies to show the business intelligence capabilities of data warehouse. The most popular definition of the data ...
This paper discusses how a data lakehouse, a new architectural approach, achieves the same benefits of an RDBMS-OLAP and cloud data lake combined, while also providing additional advan-tages. We take today's data warehousing and break it down into implementation-independent components, capabilities, and prac-tices.
In this paper, we. intr oduce the basic concepts and mechanisms of data war ehousing. The aim of data warehousing. Data warehousing technology comprises a set of ne w. concepts and tools which ...
Although data warehouses and data lakes are used as two interchangeable terms, they are not the same [21]. One of the major differences between them is the different structures (i.e., processed vs. raw data). A data warehouse stores data in processed and filtered form, whereas data lakes store raw or unprocessed data.
A data warehouse (DW) is an integrated repository of data for supporting decision-making applications of an enterprise. The most widely cited definition of a DW is from Inmon [3] who states that "a data warehouse is a subject-oriented, integrated, nonvolatile, and time-variant collection of data in support of management's decisions.".
Chapter Objectives 1 1. Escalating Need for Strategic Information 2. The Information Crisis 3. Technology Trends 4. Opportunities and Risks 5. Failures of Past Decision-Support Systems 7. History of Decision-Support Systems 8. Inability to Provide Information 9. Operational Versus Decision-Support Systems.
Data is the lifeblood of any organization. In today's world, organizations recognize the vital role of data in modern business intelligence systems for making meaningful decisions and staying competitive in the field. Efficient and optimal data analytics provides a competitive edge to its performance and services. Major organizations generate, collect and process vast amounts of data ...
Our mission was to build an enterprise-ready data warehousing solution for the cloud. The result is the Snowflake Elastic Data Warehouse, or "Snowflake" for short. Snowflake is a multi-tenant, transactional, secure, highly scalable and elastic system with full SQL support and built-in extensions for semi-structured and schema-less data.
Various issues and challenges in the field of data warehousing are presented in many studies during the recent years. In this paper, a comprehensive survey is presented to take a holistic view of the research trends in the fields of data warehousing. This paper presents a systematic division of work of researchers in the fields of data warehousing.
A data warehouse is attractive as the main repository of an organization's historical data and is optimized for reporting and analysis. In this paper, we present a data warehouse the process of data warehouse architecture development and design. We highlight the different aspects to be considered in building a data warehouse. These range from data store characteristics to data modeling and ...
It is possible to implement data warehouse for typical university information system [8]. Academic data warehouse supports the decisional and analytical activities regarding the three major components in the university context: didactics, research, and management [9]. Data warehouse has important role in educational data analysis [10]. Table 1.
The idea of data warehousing is deceptively very simple. It is very much important to prepare data warehouse by using the proper design methodology and process. This is because data warehousing provides users with large amounts of clean, organized, and summarized data. Which greatly facilitates data mining.
This paper motivates the concept of a data warehouse, outlines a general data warehousing architecture, and proposes a number of technical issues arising from the architecture that are suitable topics for exploratory research. The topic of data warehousing encompasses architectures, algorithms, and tools for bringing together selected data from multiple databases or other information sources ...
This paper analyzes the performance of the data warehouse architectures, through studding and comparing many research works in this filed. the study involves the extract, transform and load the ...
over multiple petabytes of data. In this paper, we describe the design of Snow ake and its novel multi-cluster, shared-data architecture. The paper highlights some of the key features of Snow ake: extreme elasticity and availability, semi-structured and schema-less data, time travel, and end-to-end security. It concludes with
Abstract: In a cloud based data warehouse (DW), business users can access and query data from multiple sources and geographically distributed places. Business analysts and decision makers are counting on DWs especially for data analysis and reporting. Temporal and spatial data are two factors that affect seriously decision-making and marketing strategies and many applications require modelling ...
There are some efforts in the area of data warehouse for building data warehouse for education domain. The paper by Carlo DELL'AQUILA [10] summarizes the experience in designing and modeling an academic data warehouse. Existing facilities and databases affect the chosen data warehouse that brings them together to support decisional
However, the 3D data world has no such consistency. 3D data can be represented as multi-view image sets, voxels, point clouds, meshes, etc. So, choosing a suitable data representation is half the battle if it will be used in the 3D-DL research field. Consequently, in the following subsections, based on what is mentioned above, this
View PDF HTML (experimental) Abstract: Document Question Answering (QA) presents a challenge in understanding visually-rich documents (VRD), particularly those dominated by lengthy textual content like research journal articles. Existing studies primarily focus on real-world documents with sparse text, while challenges persist in comprehending the hierarchical semantic relations among multiple ...
newly e merged types of data, which a re usually characterized by. 4Vs, but also lately by 7Vs [4]: volume - the amounts of data are vast. variety - there is a great number of data format and ...
The paper will also use a specific examples to demonstrate how the quality of data stored in a data warehousing will impact the result of data mining Discover the world's research 25+ million members
View PDF Abstract: The quadratic complexity and weak length extrapolation of Transformers limits their ability to scale to long sequences, and while sub-quadratic solutions like linear attention and state space models exist, they empirically underperform Transformers in pretraining efficiency and downstream task accuracy. We introduce Megalodon, a neural architecture for efficient sequence ...
Conclusions. We provided a national-scale, systematic evaluation of China's city subsidence. Of the urban lands in China's major cities, 45% are subsiding with a velocity faster than 3 mm/year, and 16% are subsiding faster than 10 mm/year; these urban lands contain 29 and 7% of urban population, respectively.
Though a lot has been written about how a data warehouse should be designed, there is no consensus on a design method yet. This paper follows from a wide discus- sion that took place in Dagstuhl ...