Diagnosis and Management of Dementia: Review
Affiliations.
- 1 Rush Alzheimer's Disease Center, Rush University Medical Center, Chicago, Illinois.
- 2 Department of Neurological Sciences, Rush University Medical Center, Chicago, Illinois.
- 3 Department of Family Medicine, Rush University Medical Center, Chicago, Illinois.
- PMID: 31638686
- PMCID: PMC7462122
- DOI: 10.1001/jama.2019.4782
Importance: Worldwide, 47 million people live with dementia and, by 2050, the number is expected to increase to 131 million.
Observations: Dementia is an acquired loss of cognition in multiple cognitive domains sufficiently severe to affect social or occupational function. In the United States, Alzheimer disease, one cause of dementia, affects 5.8 million people. Dementia is commonly associated with more than 1 neuropathology, usually Alzheimer disease with cerebrovascular pathology. Diagnosing dementia requires a history evaluating for cognitive decline and impairment in daily activities, with corroboration from a close friend or family member, in addition to a thorough mental status examination by a clinician to delineate impairments in memory, language, attention, visuospatial cognition such as spatial orientation, executive function, and mood. Brief cognitive impairment screening questionnaires can assist in initiating and organizing the cognitive assessment. However, if the assessment is inconclusive (eg, symptoms present, but normal examination findings), neuropsychological testing can help determine whether dementia is present. Physical examination may help identify the etiology of dementia. For example, focal neurologic abnormalities suggest stroke. Brain neuroimaging may demonstrate structural changes including, but not limited to, focal atrophy, infarcts, and tumor, that may not be identified on physical examination. Additional evaluation with cerebrospinal fluid assays or genetic testing may be considered in atypical dementia cases, such as age of onset younger than 65 years, rapid symptom onset, and/or impairment in multiple cognitive domains but not episodic memory. For treatment, patients may benefit from nonpharmacologic approaches, including cognitively engaging activities such as reading, physical exercise such as walking, and socialization such as family gatherings. Pharmacologic approaches can provide modest symptomatic relief. For Alzheimer disease, this includes an acetylcholinesterase inhibitor such as donepezil for mild to severe dementia, and memantine (used alone or as an add-on therapy) for moderate to severe dementia. Rivastigmine can be used to treat symptomatic Parkinson disease dementia.
Conclusions and relevance: Alzheimer disease currently affects 5.8 million persons in the United States and is a common cause of dementia, which is usually accompanied by other neuropathology, often cerebrovascular disease such as brain infarcts. Causes of dementia can be diagnosed by medical history, cognitive and physical examination, laboratory testing, and brain imaging. Management should include both nonpharmacologic and pharmacologic approaches, although efficacy of available treatments remains limited.
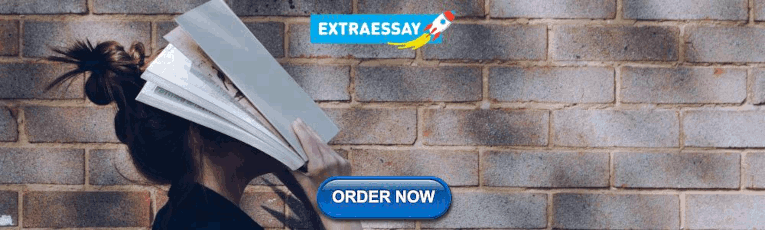
Publication types
- Research Support, N.I.H., Extramural
- Research Support, Non-U.S. Gov't
- Alzheimer Disease / diagnosis
- Alzheimer Disease / therapy
- Cholinesterase Inhibitors / adverse effects
- Cholinesterase Inhibitors / therapeutic use
- Dementia / diagnosis*
- Dementia / therapy*
- Excitatory Amino Acid Antagonists / adverse effects
- Excitatory Amino Acid Antagonists / therapeutic use
- Memantine / adverse effects
- Memantine / therapeutic use
- Neuroimaging
- Neuropsychological Tests
- Cholinesterase Inhibitors
- Excitatory Amino Acid Antagonists
Grants and funding
- P30 AG010161/AG/NIA NIH HHS/United States
- R01 AG040039/AG/NIA NIH HHS/United States
- R01 NS084965/NS/NINDS NIH HHS/United States
- RF1 AG059621/AG/NIA NIH HHS/United States
Featured Clinical Reviews
- Screening for Atrial Fibrillation: US Preventive Services Task Force Recommendation Statement JAMA Recommendation Statement January 25, 2022
- Evaluating the Patient With a Pulmonary Nodule: A Review JAMA Review January 18, 2022
- Download PDF
- Share X Facebook Email LinkedIn
- Permissions
Diagnosis and Management of Dementia: Review
- 1 Rush Alzheimer’s Disease Center, Rush University Medical Center, Chicago, Illinois
- 2 Department of Neurological Sciences, Rush University Medical Center, Chicago, Illinois
- 3 Department of Family Medicine, Rush University Medical Center, Chicago, Illinois
- JAMA Patient Page Dementia Zoe Arvanitakis, MD, MS; David A. Bennett, MD JAMA
- Viewpoint Caring for Persons Living With Dementia and Their Care Partners and Caregivers Eric B. Larson, MD, MPH; Clare Stroud, PhD JAMA
Importance Worldwide, 47 million people live with dementia and, by 2050, the number is expected to increase to 131 million.
Observations Dementia is an acquired loss of cognition in multiple cognitive domains sufficiently severe to affect social or occupational function. In the United States, Alzheimer disease, one cause of dementia, affects 5.8 million people. Dementia is commonly associated with more than 1 neuropathology, usually Alzheimer disease with cerebrovascular pathology. Diagnosing dementia requires a history evaluating for cognitive decline and impairment in daily activities, with corroboration from a close friend or family member, in addition to a thorough mental status examination by a clinician to delineate impairments in memory, language, attention, visuospatial cognition such as spatial orientation, executive function, and mood. Brief cognitive impairment screening questionnaires can assist in initiating and organizing the cognitive assessment. However, if the assessment is inconclusive (eg, symptoms present, but normal examination findings), neuropsychological testing can help determine whether dementia is present. Physical examination may help identify the etiology of dementia. For example, focal neurologic abnormalities suggest stroke. Brain neuroimaging may demonstrate structural changes including, but not limited to, focal atrophy, infarcts, and tumor, that may not be identified on physical examination. Additional evaluation with cerebrospinal fluid assays or genetic testing may be considered in atypical dementia cases, such as age of onset younger than 65 years, rapid symptom onset, and/or impairment in multiple cognitive domains but not episodic memory. For treatment, patients may benefit from nonpharmacologic approaches, including cognitively engaging activities such as reading, physical exercise such as walking, and socialization such as family gatherings. Pharmacologic approaches can provide modest symptomatic relief. For Alzheimer disease, this includes an acetylcholinesterase inhibitor such as donepezil for mild to severe dementia, and memantine (used alone or as an add-on therapy) for moderate to severe dementia. Rivastigmine can be used to treat symptomatic Parkinson disease dementia.
Conclusions and Relevance Alzheimer disease currently affects 5.8 million persons in the United States and is a common cause of dementia, which is usually accompanied by other neuropathology, often cerebrovascular disease such as brain infarcts. Causes of dementia can be diagnosed by medical history, cognitive and physical examination, laboratory testing, and brain imaging. Management should include both nonpharmacologic and pharmacologic approaches, although efficacy of available treatments remains limited.
Read More About
Arvanitakis Z , Shah RC , Bennett DA. Diagnosis and Management of Dementia: Review. JAMA. 2019;322(16):1589–1599. doi:10.1001/jama.2019.4782
Manage citations:
© 2024
Artificial Intelligence Resource Center
Cardiology in JAMA : Read the Latest
Browse and subscribe to JAMA Network podcasts!
Others Also Liked
Select your interests.
Customize your JAMA Network experience by selecting one or more topics from the list below.
- Academic Medicine
- Acid Base, Electrolytes, Fluids
- Allergy and Clinical Immunology
- American Indian or Alaska Natives
- Anesthesiology
- Anticoagulation
- Art and Images in Psychiatry
- Artificial Intelligence
- Assisted Reproduction
- Bleeding and Transfusion
- Caring for the Critically Ill Patient
- Challenges in Clinical Electrocardiography
- Climate and Health
- Climate Change
- Clinical Challenge
- Clinical Decision Support
- Clinical Implications of Basic Neuroscience
- Clinical Pharmacy and Pharmacology
- Complementary and Alternative Medicine
- Consensus Statements
- Coronavirus (COVID-19)
- Critical Care Medicine
- Cultural Competency
- Dental Medicine
- Dermatology
- Diabetes and Endocrinology
- Diagnostic Test Interpretation
- Drug Development
- Electronic Health Records
- Emergency Medicine
- End of Life, Hospice, Palliative Care
- Environmental Health
- Equity, Diversity, and Inclusion
- Facial Plastic Surgery
- Gastroenterology and Hepatology
- Genetics and Genomics
- Genomics and Precision Health
- Global Health
- Guide to Statistics and Methods
- Hair Disorders
- Health Care Delivery Models
- Health Care Economics, Insurance, Payment
- Health Care Quality
- Health Care Reform
- Health Care Safety
- Health Care Workforce
- Health Disparities
- Health Inequities
- Health Policy
- Health Systems Science
- History of Medicine
- Hypertension
- Images in Neurology
- Implementation Science
- Infectious Diseases
- Innovations in Health Care Delivery
- JAMA Infographic
- Law and Medicine
- Leading Change
- Less is More
- LGBTQIA Medicine
- Lifestyle Behaviors
- Medical Coding
- Medical Devices and Equipment
- Medical Education
- Medical Education and Training
- Medical Journals and Publishing
- Mobile Health and Telemedicine
- Narrative Medicine
- Neuroscience and Psychiatry
- Notable Notes
- Nutrition, Obesity, Exercise
- Obstetrics and Gynecology
- Occupational Health
- Ophthalmology
- Orthopedics
- Otolaryngology
- Pain Medicine
- Palliative Care
- Pathology and Laboratory Medicine
- Patient Care
- Patient Information
- Performance Improvement
- Performance Measures
- Perioperative Care and Consultation
- Pharmacoeconomics
- Pharmacoepidemiology
- Pharmacogenetics
- Pharmacy and Clinical Pharmacology
- Physical Medicine and Rehabilitation
- Physical Therapy
- Physician Leadership
- Population Health
- Primary Care
- Professional Well-being
- Professionalism
- Psychiatry and Behavioral Health
- Public Health
- Pulmonary Medicine
- Regulatory Agencies
- Reproductive Health
- Research, Methods, Statistics
- Resuscitation
- Rheumatology
- Risk Management
- Scientific Discovery and the Future of Medicine
- Shared Decision Making and Communication
- Sleep Medicine
- Sports Medicine
- Stem Cell Transplantation
- Substance Use and Addiction Medicine
- Surgical Innovation
- Surgical Pearls
- Teachable Moment
- Technology and Finance
- The Art of JAMA
- The Arts and Medicine
- The Rational Clinical Examination
- Tobacco and e-Cigarettes
- Translational Medicine
- Trauma and Injury
- Treatment Adherence
- Ultrasonography
- Users' Guide to the Medical Literature
- Vaccination
- Venous Thromboembolism
- Veterans Health
- Women's Health
- Workflow and Process
- Wound Care, Infection, Healing
- Register for email alerts with links to free full-text articles
- Access PDFs of free articles
- Manage your interests
- Save searches and receive search alerts
Advertisement
Machine Learning for Dementia Prediction: A Systematic Review and Future Research Directions
- Original Paper
- Open access
- Published: 01 February 2023
- Volume 47 , article number 17 , ( 2023 )
Cite this article
You have full access to this open access article
- Ashir Javeed 1 , 2 na1 ,
- Ana Luiza Dallora 2 na1 ,
- Johan Sanmartin Berglund 2 ,
- Arif Ali 3 ,
- Liaqata Ali 4 &
- Peter Anderberg 2 , 5
15k Accesses
25 Citations
46 Altmetric
Explore all metrics
Nowadays, Artificial Intelligence (AI) and machine learning (ML) have successfully provided automated solutions to numerous real-world problems. Healthcare is one of the most important research areas for ML researchers, with the aim of developing automated disease prediction systems. One of the disease detection problems that AI and ML researchers have focused on is dementia detection using ML methods. Numerous automated diagnostic systems based on ML techniques for early prediction of dementia have been proposed in the literature. Few systematic literature reviews (SLR) have been conducted for dementia prediction based on ML techniques in the past. However, these SLR focused on a single type of data modality for the detection of dementia. Hence, the purpose of this study is to conduct a comprehensive evaluation of ML-based automated diagnostic systems considering different types of data modalities such as images, clinical-features, and voice data. We collected the research articles from 2011 to 2022 using the keywords dementia, machine learning, feature selection, data modalities, and automated diagnostic systems. The selected articles were critically analyzed and discussed. It was observed that image data driven ML models yields promising results in terms of dementia prediction compared to other data modalities, i.e., clinical feature-based data and voice data. Furthermore, this SLR highlighted the limitations of the previously proposed automated methods for dementia and presented future directions to overcome these limitations.
Similar content being viewed by others
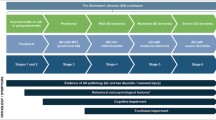
Diagnosis of Early Alzheimer’s Disease: Clinical Practice in 2021
A. P. Porsteinsson, R. S. Isaacson, … I. Rubino
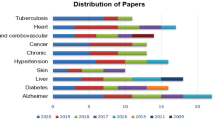
Artificial intelligence in disease diagnosis: a systematic literature review, synthesizing framework and future research agenda
Yogesh Kumar, Apeksha Koul, … Muhammad Fazal Ijaz
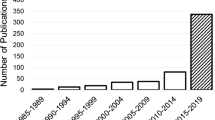
Artificial Intelligence for Mental Health and Mental Illnesses: an Overview
Sarah Graham, Colin Depp, … Dilip V. Jeste
Avoid common mistakes on your manuscript.
Introduction
Over a period of time, the advancements made in the field of medical science helped to increase the lifespan in the modern world [ 1 ]. This increased life expectancy raised the prevalence of neurocognitive disorders, affecting a significant part of the older population as well as global economies. In 2010, it was estimated that $604 billion have been spent on dementia patients in the USA alone[ 2 ]. The number of dementia patients is rapidly increasing worldwide, and statistical projections suggest that 135 million people might be affected by dementia by 2050 [ 3 ]. There are several risk factors that contribute to the development of dementia, including aging, head injury, and lifestyle. While age is the most prominent risk factor for dementia; figures suggest that a person at the age of 65 years old has 1–2% risk of developing dementia disease. By the age of 85 years old, this risk can reach to 30% [ 4 ].
Dementia is a mental disorder that is characterized by a progressive deterioration of cognitive functions that can affect daily life activities such as memory, problem solving, visual perception, and the ability to focus on a particular task. Usually, older adults are most vulnerable to dementia, and people take it as an inevitable consequence of aging, which is perhaps the wrong perception. Dementia is not a part of the normal ageing process; however, it should be considered a serious form of cognitive decline that affects your daily life. Actually, the primary cause for the development of dementia is the several diseases and injuries that affect the human brain [ 5 ]. Dementia is ranked on the seventh place in the leading causes of deaths in the world [ 6 ]. Furthermore, it is the major cause of disability and dependency among older people globally [ 6 ]. A change in the person’s ordinary mental functioning and obvious signs of high cognitive deterioration are required for a diagnosis of dementia [ 7 ]. Figure 1 presents the progression of dementia with age.
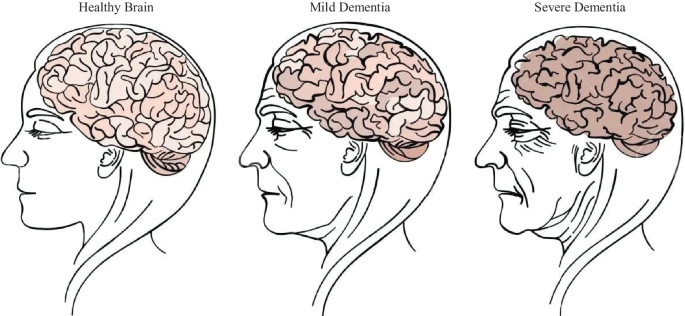
Progression of dementia disease with ageing
Types of dementia
Dementia is not a single disease, but, it is used as a generic term for several different cognitive disorders. Figure 2 provides the overview of different types of dementia along with the percentage of particular dementia type occurrence in the patients [ 8 ]. To have a better idea about dementia, we have studied common types of dementia for better problem awareness.
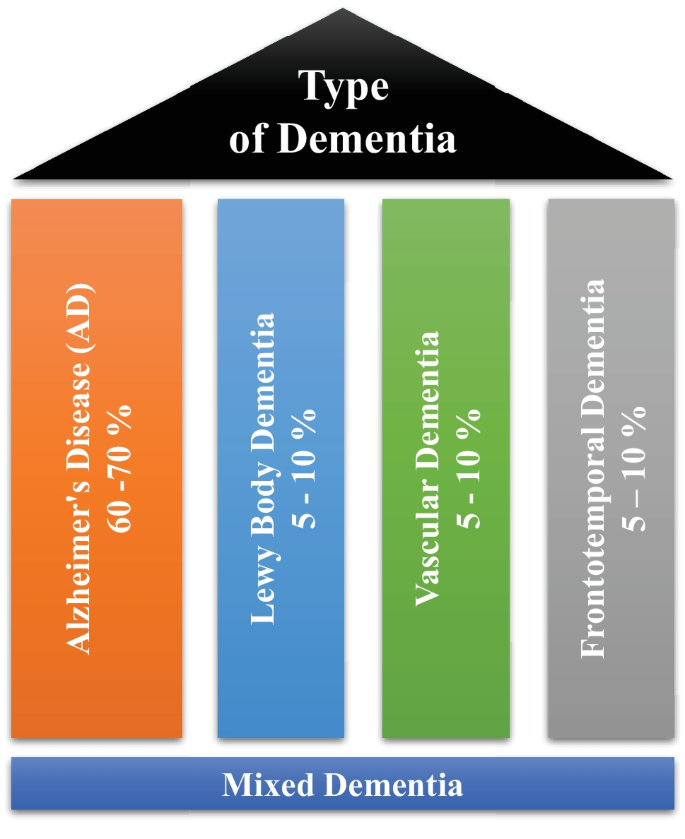
Types of dementia disease
Alzheimer’s disease
Alzheimer’s disease (AD) is thought to develop when abnormal amounts of amyloid beta (A \(\beta\) ) build up in the brain, either extracellularly as amyloid plaques, tau proteins or intracellularly as neurofibrillary tangles, affecting neuronal function, connectivity and leading to progressive brain function loss [ 9 ]. This diminished ability to eliminate proteins with ageing is regulated by brain cholesterol [ 10 ] and is linked to other neurodegenerative illnesses [ 11 ]. Except for 1–2% of cases where deterministic genetic anomalies have been discovered, the aetiology of the majority of Alzheimer’s patients remains unexplained [ 12 ]. The amyloid beta (A \(\beta\) ) hypothesis and the cholinergic hypothesis are two competing theories presented to explain the underlying cause of AD [ 13 ].
Vascular dementia
Vascular dementia (VaD) is a subtype of dementia caused by problems with the brain’s blood flow, generally in the form of a series of minor strokes, which results in a slow decline of cognitive capacity [ 14 ]. The VaD refers to a disorder characterized by a complicated mix of cerebrovascular illnesses that result in structural changes in the brain, as a result of strokes and lesions, which lead to cognitive impairment. A chronological relationship between stroke and cognitive impairments is necessary to make the diagnosis [ 15 ]. Ischemic or hemorrhagic infarctions in several brain areas, such as the anterior cerebral artery region, the parietal lobes, or the cingulate gyrus, are associated with VaD. In rare cases, infarcts in the hippocampus or thalamus might cause dementia [ 16 ]. A stroke increases the risk of dementia by 70%, whereas a recent stroke increases the risk by almost 120% [ 17 ]. Brain vascular lesions can also be caused by diffuse cerebrovascular disease, such as small vessel disease [ 18 ]. Risk factors for VaD include age, hypertension, smoking, hypercholesterolemia, diabetes mellitus, cardiovascular disease, and cerebrovascular sickness; geographic origin, genetic proclivity, and past strokes are also risk factors [ 19 ]. Cerebral amyloid angiopathy, which develops when beta amyloid accumulates in the brain, can occasionally lead to vascular dementia.
Lewy body dementia
Lewy body dementia (LBD) is a subtype of dementia characterized by abnormal deposits of the protein alpha-synuclein in the brain. These deposits, known as Lewy bodies, affect brain chemistry, causing problems with thinking, movement, behavior, and mood. Lewy body dementia is one of the most common causes of dementia [ 20 ]. Progressive loss of mental functions, visual hallucinations, as well as changes in alertness and concentration are prevalent in persons with LBD. Other adverse effects include tight muscles, delayed movement, difficulty walking, and tremors, all of which are also signs and symptoms of Parkinson’s disease [ 21 ]. LBD might be difficult to identify. Early LBD symptoms are commonly confused with those of other brain diseases or mental problems. Lewy body dementia can occur alone or in conjunction with other brain disorders [ 22 ]. It is a progressive disorder, which means that symptoms emerge gradually and worsen with time. A timespan of five to eight years is averaged, although it can last anywhere from two to twenty years for certain people [ 23 ]. The rate at which symptoms arise varies greatly from person to person, depending on overall health, age, and the severity of symptoms.
Frontotemporal dementia
Frontotemporal Dementia (FTD) is a subtype of dementia characterized by nerve cell loss in the frontal and temporal lobes of the brain [ 24 ]. As a result, the lobes contract. FTD can have an impact on behavior, attitude, language, and movement. This is one of the most common dementias in people under the age of 65. FTD most commonly affects persons between the ages of 40 and 65; however, it may also afflict young adults and older individuals [ 25 ]. The lobes decrease, and behavior, attitude, language, and mobility can all be affected by FTD. FTD affects both men and women equally. Dissociation from family, extreme oniomania, obscene speech, screaming, and the inability to regulate emotions, behavior, personality, and temperament are examples of social display patterns caused by FTD [ 26 ]. The symptoms of FTD appeared several years prior to visiting a neurologist [ 27 ].
Mixed Dementia (MD)
Mixed dementia occurs, when more than one kind of dementia coexists in a patient, and it is estimated to happen in around 10% of all dementia cases [ 6 ]. AD and VaD dementia are the two subtypes that are most common in MD [ 28 ]. This case is usually associated with factors such as old age, high blood pressure, and brain blood vessel damage [ 29 ]. Because one dementia subtype often predominates, MD is difficult to identify. As a result, the individuals affected by MD are rarely treated and miss out on potentially life-changing medicines. MD can cause symptoms to begin earlier than the actual diagnosis of the disease and spread swiftly to affect the most areas of the brain [ 30 ].
Recently, numerous automated methods have been developed based on machine learning for early the prediction of different diseases [ 31 , 32 , 33 , 34 , 35 , 36 , 37 , 38 , 39 , 40 , 41 , 42 , 43 , 44 , 45 , 46 , 47 , 48 ]. This systematic literature review (SLR) presented hereby, investigates machine learning-based automated diagnostic systems that are designed and developed by scientists to predict dementia and its subtypes, such as AD, VaD, LBD, FTD and MD. We used the Preferred Reporting Items for Systematic Reviews and Meta-Analysis (PRISMA) criteria to conduct this SLR [ 49 , 50 ]. A comprehensive search was conducted to retrieve the research articles that contain ML approaches to predict the development of dementia and its subtypes using three different types of data modalities (images, clinical-variables, voice).
Aim of the study
SLRs are done to synthesize current evidence, to identify gaps in the literature, and to provide the groundwork for future studies [ 51 ]. Previous, SLRs studies have been done on automated diagnostic systems for dementia prediction based on ML approaches, which focused on a single sort of data modality. These SLR investigations did not emphasize the limits of previously published automated approaches for dementia prediction. The SLR presented herein assesses the previously proposed automated diagnostic systems based on deep learning (DL) and ML algorithms for the prediction of dementia and its common subtypes (e.g. AD, VaD, FTD, MD). The aim of this SLR is to analyse and evaluate the performance of automated diagnostic systems for dementia prediction using different data modalities. The main question is decomposed in the following sub-research questions:
What types of ML and DL techniques have been used by researchers to diagnose dementia?
Examine the methods of feature extraction or selection used by the researchers.
Analyze the different performance evaluation measures that are adopted by the researcher to validate the effectiveness of the proposed diagnostic system for demetnia.
Analyze the performance of ML models on various data types.
Identification of weaknesses in previously proposed ML models for dementia prediction.
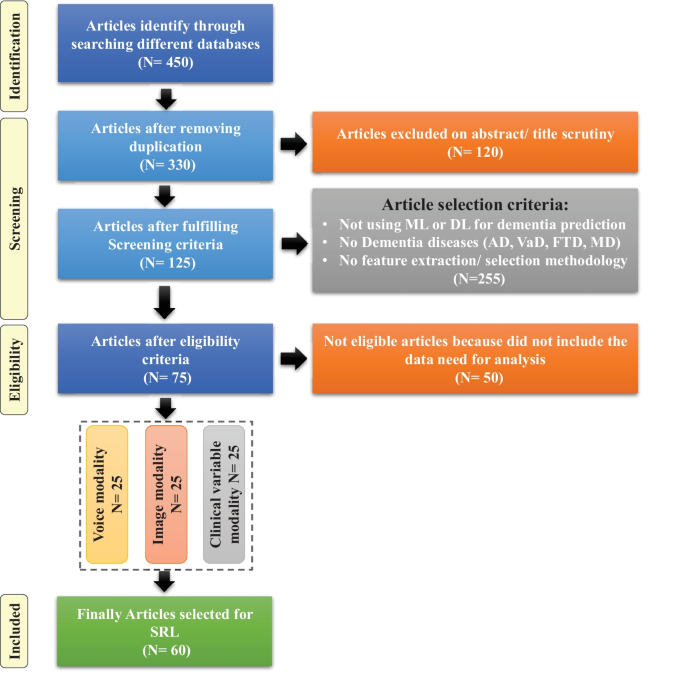
Flow diagram of PRISMA (Preferred Reporting Items for Systematic Reviews and Meta-analyses)
Article selection
For this SLR study, the research articles were selected based on keywords such as ML, DL, dementia and its subtypes (AD, VaD, FTD, and MD). For the collection of research articles, we conducted an electronic search from different online databases such as ScienceDirect, PubMed, IEEE Xplore Digital Library, Springer, Hindawi, and PLOs, which helped to gather 450 research studies on the specific topic. After reviewing the title and abstract in each study, 120 publications were found to be ineligible for processing, while 330 articles were selected for further processing. Following the deduplication of data, 125 full-text publications were retrieved for further processing after the screening phase of the article selection, with 205 of them being eliminated due to not satisfying the article selection criteria of the screening phase. Finally, 50 research articles were eliminated due to not fulfilling the eligibility criteria for article selection. The final set of selected papers consisted of 75 research papers, among these final selected articles, each of the data modalities (image, clinical-variables, voice) contained 25 papers. After rerunning the database searches in May 2022, no further suitable research article was found for the selection. Figure 3 presents the workflow for article selection, which includes the four PRISMA guidelines-recommended steps such as identification, screening, eligibility, and inclusion [ 49 , 50 ]. In recent years, ML scientists have shown a strong interest in designing and developing ML-based automated diagnostic systems for dementia prediction. Therefore, the number of research articles in this research area has been increased and it can be depicted from Fig. 4 where research articles are published years wise with regarding data modality. The publications utilized in this study were selected based on the following criteria:
Studies that present automated diagnostic systems for dementia and its common subtypes (AD,VaD, FTD, MD).
Studies published between 2011 and 2022.
Studies employing ML approaches for dementia diagnosis.
Studies which have utilized several data modalities.
Studies published in the English language.
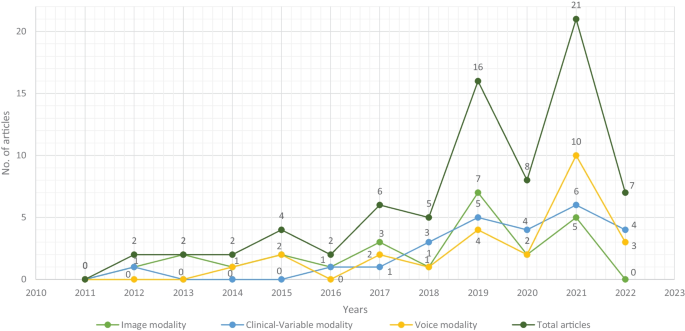
Selected research articles which are published from 2011 to 2022 regarding data modality
Machine learning for dementia
Over the years, the increasing use and availability of medical equipment has resulted in a massive collection of electronic health records (EHR) that might be utilized to identify dementia using developing technologies such as ML and DL [ 52 ]. These EHRs are one of the most widely available and used clinical datasets. They are a crucial component of contemporary healthcare delivery, providing rapid access to accurate, up-to-date, comprehensive patient information while also assisting with precise diagnosis and coordinated, efficient care [ 53 ]. Laboratory tests, vital signs, drugs, and other therapies, as well as comorbidities, can be used to identify the people at risk of dementia using the EHRs’ data [ 54 ]. In some situations, patients may also be subjected to costly and invasive treatments such as neuroimaging scans i.e., magnetic resonance imaging (MRI) and position emission tomography (PET)) and cerebrospinal fluid (CSF) collection for biomarker testing [ 55 , 56 , 57 ]. These tests’ findings may also be found in the EHR. According to researchers, such longitudinal clinical EHR data can be used to track the advancement of AD dementia over time [ 58 ]. Recently, several automated diagnostic systems for different diseases, such as Parkinson’s disease [ 59 ], hepatitis [ 47 ], carcinoma [ 41 ], and heart failure [ 60 , 61 , 62 ] prediction have been designed by employing ML and DL techniques. Inspired by this fact, the unmet demand for dementia knowledge, along with the availability of relevant huge datasets, has motivated scientists to investigate the utility of artificial intelligence (AI), which is gaining a prominent role in the area of healthcare innovation [ 63 ]. ML, a subset of AI, can model the relationship between input quantities and clinical outcomes, identify hidden patterns in enormous volumes of data, and draw conclusions or make decisions that help with more accurate clinical decision-making [ 51 ]. However, computational hypotheses generated by ML models must still be confirmed by subject matter experts in order to achieve enough precision for clinical decision-making [ 64 ].
In this SLR, we have included studies that have used ML predictive models (supervised and unsupervised) for dementia prediction and excluded studies that have used statistical methods for cohort summarization and hypothesis testing (e.g., odds ratio, chi-square distribution, Kruskal-Wallis test, and Kappa-Cohen test). Furthermore, we have referenced the data modality-based study [ 65 ] for this literature review, where we have categorized the three data modality types such as image, clinical-variable and voice. Thus, we have studied each modality-based automated diagnostic system for dementia prediction that has been proposed in the past using ML and DL.
This section explains the datasets that were used in the selected research papers for experiments and performance evaluation of the proposed automated diagnostic systems designed by the researchers using ML algorithms for dementia and its subtypes. A total of 61 datasets were studied from the selected research articles. These datasets are compiled from a wide range of organizations and hospitals throughout the world. Only a few datasets are openly available to the public, while others are compiled by researchers from various hospitals and healthcare institutes. We have only included datasets that have been used to diagnose AD, VaD, FTD, MD, and LBD using ML and DL techniques. On the basis of data modality, we have categorised the dataset into three types: images, clinical_variables and voice datasets. The datasets differ in terms of the number of variables (features) and samples. As a result, we examined each modality of the dataset one by one.
Image modality based datasets
There are several image datasets based on brain imaging, such as magnetic resonance imaging (MRI), collected by the researchers for the diagnosis of dementia. From the Table 1 , it can be depicted that Open Access Series of Imaging Studies (OASIS) and Alzheimer’s Disease Neuroimaging Initiative (ADNI) datasets are mostly used by the researchers for the experimental purpose. OASIS aims to make neuroimaging datasets available to the scientific community for free. By gathering and openly disseminating this multimodal dataset produced by the Knight ADRC and its related researchers, they had used different samples and variables of the datasets in their research work. ADNI researchers acquire, validate, and use data such as MRI, PET imaging, genetics, cognitive assessments, CSF, and blood biomarkers as disease predictors. The ADNI website contains research information and data from the North American ADNI project, which includes Alzheimer’s disease patients, people with mild cognitive impairment, and older controls. Table 1 provides us with the following information: dataset_id, dataset name, number of samples in the particular dataset, variables in the dataset, and finally, the type of dementia.
Clinical-variables modality based datasets
Throughout the course of time, the growing usage and availability of medical devices have resulted in an overwhelming collection of clinical EHR data. Furthermore, the patient’s medical history consists of medical tests and clinical records that can be used for the prediction of diseases. Thus, the importance of clinical data emerges as a vital tool for proactive management of disease. The dataset based on clinical variables for dementia consists of medical tests that are used by doctors to check the dementia status in patients, such as the Mini Mental Status Exam (MMSE), the Montreal Cognitive Assessment (MoCA), the Telephone Interview for Cognitive Status (TICS), and the Brief Interview for Mental Status (BIMS). Clinical-variables based datasets consist of information about these medical tests along with patient personal information, i.e., age, sex, and marital status. Hereby, Table 2 provides the information regarding clinical-variables modality-based datasets that are used by the researchers for the design and development of automated diagnostic systems for dementia patients based on ML. Table 2 presents the dataset_id, dataset name, number of samples in the particular dataset, variables in the dataset, and finally the type of dementia.
Voice modality based datasets
Speech analysis is a useful technique for clinical linguists in detecting various types of neurodegenerative disorders affecting the language processing areas. Individuals suffering from Parkinson’s disease (PD, deterioration of voice quality, unstable pitch), Alzheimer’s disease (AD, monotonous pitch), and the non-fluent form of Primary Progressive Aphasia (PPA-NF, hesitant, non-fluent speech) may experience difficulties with prosody, fluency, and voice quality. Besides imaging and clinical-variables data, the researchers employed voice recording data to identify dementia using ML and DL algorithms. The data collection process for voice data varies from dataset to dataset, for example, in a few datasets, patients were requested to answer a prepared set of questions (interview) in a specific time interval. In a few datasets, selected neuropsychological tests were carried out, the description of each neuropsychological test was played and was followed by an answering window. Table 3 presents the dataset_id, dataset name, number of samples in the particular dataset, variables in the dataset, and finally the subtype of dementia.
Data sharing challenges
In this digital era, public health decision-making has grown progressively complicated, and the utilization of data has become critical [ 66 ]. Data are employed at the local level to observe public health and target interventions; at the national scale for resource allocation, prioritization, and planning; and at the global scale for disease burden estimates, progress in health and development measurement, and the containment of evolving global health threats [ 67 , 68 ]. Van Panhuis et al. have adequately described the challenges to exchanging health data [ 69 ]. Based on our initial analysis, we built on this taxonomy to identify the hurdles related to data sharing in global public health, and we have highlighted how they may apply to each typology as given below.
Lack of complete data, lost data, restrictive as well as conflicting data formats, a lack of metadata and standards, a lack of interoperability of datasets (e.g., structure or “language”), and a lack of appropriate analytic solutions are examples of technical barriers encountered by health information management systems.
Individuals and organizations face motivational challenges when it comes to sharing data. These impediments include a lack of incentives, opportunity costs, apprehension about criticism, and disagreements over data usage and access.
The potential and present costs of sharing data are both economic hurdles.
Political obstacles are those that are built into the norms of local health governance and often emerge as regulations and guidelines. They can also entail trust and ownership difficulties.
Legal issues that arise as a result of data collection, analysis, and usage include questions regarding who owns or controls the data, transparency, informed permission, security, privacy, copyright, human rights, damage, and stigma.
Ethical constraints include a lack of perceived reciprocity (i.e., the other side will not disclose data) and proportionality (i.e., deciding not to share data based on an assessment of the risks and benefits). An overall concern is that frameworks, rules, and regulations have not kept up with technological changes that are transforming how data is collected, analyzed, shared, and used.
ML based diagnostic models for dementia: Image modality
In recent years, researchers have designed many ML and DL algorithms for the detection of dementia and its subtypes using MRI images of the brain. For example, Dashtipour et al. [ 70 ] proposed a ML based method for the prediction of Alzheimer’s disease. In their proposed model, they used DL techniques to extract the features from brain images, and for classification purposes, they deployed SVM and bidirectional long short-term memory (BiLSTM). Through their proposed model, they had reported the classification accuracy of 91.28%. Moreover, for early detection of the AD, a DL based approach was proposed by Helaly et al. In their proposed work, they employed convolutional neural networks (CNN). The Alzheimer’s disease spectrum is divided into four phases. Furthermore, different binary medical image classifications were used for each two-pair class of Alzheimer’s disease stages. Two approaches were used to categorize medical images and diagnose Alzheimer’s disease. The first technique employs basic CNN architectures based on 2D and 3D convolution to cope with 2D and 3D structural brain images from the ADNI dataset. They had achieved highly promising accuracies for 2D and 3D multi-class AD stage classification of 93.61% and 95.17%, respectively. The VGG19 pre-trained model had been fine-tuned and obtained an accuracy of 97% for multi-class AD stage classification [ 71 ]. Vandenberghe et al. had proposed a method for binary classification of 18F-flutemetramol PET using ML techniques for AD and mild cognitive impairment (MCI). They had tested whether support vector machines (SVM), a supervised ML technique, can duplicate the assignments made by blindfolded visual readers, as well as which image components had the highest diagnostic value according to SVM and how 18F-fluoromethylamol-based SVM classification compares to structural MRI-based SVM classification in the same cases. Their F-flutemetamol based classifier was able to replicate the assignments obtained by visual read with 100% accuracy [ 72 ]. Odusami et al. proposed a novel method for the detection of early-stage dementia from functional brain changes in MRI using a fine-tuned ResNet-18 network. Their research work presents a DL based technique for predicting MCI, early MCI, late MCI, and Alzheimer’s disease (AD). The ADNI fMRI dataset was used for analysis and consisted of 138 participants. On EMCI vs. AD, LMCI vs. AD, and MCI vs. AD, the fine-tuned ResNet18 network obtained classification accuracy of 99.99%, 99.95%, and 99.95%, respectively [ 73 ]. Zheng et al. had presented a ML based framework for differential diagnosis between VaD and AD using structural MRI features. The least absolute shrinkage and selection operator (LASSO) was then used to build a feature set that was fed into SVM for classification. To ensure unbiased evaluation of model performance, a comparative analysis of classification models was conducted using different ML algorithms to discover which one had better performance in the differential diagnosis between VaD and AD. The diagnostic performance of the classification models was evaluated using quantitative parameters derived from the receiver operating characteristic curve (ROC). The experimental finding had shown that the SVM with RBF performed well for the differential diagnosis of VaD and AD, with sensitivity (SEN), specificity (SPE), and accuracy (ACC) values of 82.65%, 87.17%, and 84.35%, respectively (AUC = 86.10–95%, CI = 0.820–0.902) [ 74 ]. Basheer et al. [ 75 ] had presented an innovative technique by making improvements in capsule network design for the best prediction outcomes. The study used the OASIS dataset with dimensions (373 X 15) to categorize the labels as demented or non-demented. To make the model swifter and more accurate, several optimization functions were performed on the variables, as well as the feature selection procedure. The claims were confirmed by demonstrating the correlation accuracy at various iterations and layers with an allowable accuracy of 92.39%. L. K. Leong and A. A. Abdullah had proposed a method for the prediction of AD based on ML techniques with the Boruta algorithm as a feature selection method. According to the Boruta algorithm, Random Forest Grid Search Cross Validation (RF GSCV) outperformed other 12 ML models, including conventional and fine-tuned models, with 94.39% accuracy, 88.24% sensitivity, 100.00% specificity, and 94.44% AUC even for the small OASIS-2 longitudinal MRI dataset [ 76 ]. Battineni et al. had presented a SVM based ML model for the prediction of dementia. Their proposed model had achieved an accuracy and precision of 68.75% and 64.18% using the OASIS-2 dataset [ 77 ]. Mathotaarachchi et al. had analyzed the amyloid imaging using ML approaches for the detection of dementia. To overcome the inherent unfavorable and imbalance proportions between persons with stable and progressing moderate cognitive impairment in a short observation period. The innovative method had achieved 84.00% accuracy and an AUC of 91.00% for the ROC [ 78 ]. Aruna and Chitra had presented a ML approach for the identification of dementia from MRI images, where they had deployed Independent Component Analysis (ICA) to extract the features from the images, and for classification purposes, SVM with different kernels is used. Through their proposed method, they had obtained an accuracy of 90.24% [ 79 ] (Fig. 5 ).
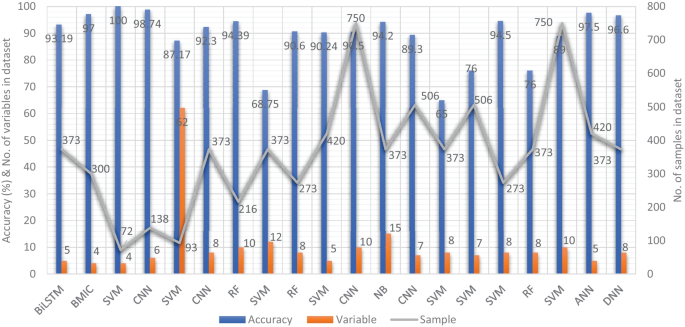
Accuracy comparison of different ML models based on image modality
Supervised ML techniques and CNNs were examined by Herzog and Magoulas. They had achieved the accuracy of 92.5% and 75.0% for NC vs EMCI, 93.0% and 90.5% for NC vs. AD, respectively [ 80 ]. Battineni et al. had comprehensive applied ML model on MRI to predict Alzheimer’s disease (AD) in older subjects, and they had proposed two ML models for AD detection. In the first trial, manual feature selection was utilized for model training, and ANN produced the highest AUC of 81.20% by ROC. The NB had earned the greatest AUC of 94.20% by ROC in the second trial, which included wrapping approaches for the automated feature selection procedure [ 81 ]. Ma et al. had conducted a study where they compared feature-engineered and non-feature-engineered ML methods for blinded clinical evaluation for dementia of Alzheimer’s type classification using FDG-PET. The highest accuracy of 84.20% was obtained through CNN’s [ 82 ]. Bidani et al. had presented a novel approach in the field of DL that combines both the deep convolutional neural network (DCNN) model and the transfer learning model to detect and classify dementia. When the features were retrieved, the dementia detection and classification strategy from brain MRI images using the DCNN model provided an improved classification accuracy of 81.94%. The transfer learning model, on the other hand, had achieved an accuracy of 68.13% [ 83 ].
Moscoso et al. had designed a predictive model for the prediction of Alzheimer’s disease using MRI images. Their proposed model had obtained the highest accuracy of 84.00% [ 84 ]. Khan and Zubair had presented an improved multi-modal based ML approach for the prognosis of AD. Their proposed model had a five-stage ML pipeline, where each stage was further categorized into different sub-levels. Their proposed model had reported the highest accuracy of 86.84% using RF [ 85 ]. Mohammed et al. had evaluated the two CNN models (AlexNet and ResNet-50) and hybrid DL/ML approaches (AlexNet+SVM and ResNet-50+SVM) for AD diagnosis using the OASIS dataset. They had found that RF algorithm had attained an overall accuracy of 94%, as well as precision, recall, and F1 scores of 93%, 98%, and 96%, respectively [ 86 ]. Salvatore et al. had developed a ML method for early AD diagnosis using magnetic resonance imaging indicators. In their proposed ML model, they used PCA for extracting features from the images and SVM for the classification of dementia. They had achieved a classification accuracy of 76% using a 20-fold cross validation scheme [ 87 ]. Katako et al. had identified the AD related FDGPET pattern that is also found in LBD and Parkinson’s disease dementia using ML approaches. They studied different ML algorithms, but SVM with an iterative single data algorithm produced the best performance, i.e., sensitivity 84.00%, specificity 95.00% through 10-fold cross-validation [ 88 ]. Gray et al. had presented a system in which RF proximities were utilized to learn a low-dimensional manifold from labelled training data and then infer the clinical labels of test data that translated to this space. Their proposed model, voxel-based (FDG-PET), obtained an accuracy of 87.9% using ten-fold cross-validation [ 89 ]. Table 4 provides the overall performance evaluation of the ML models that were presented by the researchers for the prediction of dementia and its subtypes by using image data as a modality.
ML based diagnostic models for dementia: Clinical-variable modality
Aside from image-based ML techniques for dementia prediction, several research studies have utilized clinical-variable data with ML algorithms to predict dementia and its subtypes. For instance, Chiu et al. had designed a screening instrument to detect MCI and dementia using ML techniques. They had developed a questionnaire to assist neurologists and neuropsychologists in the screening of MCI and dementia. The contribution of 45 items that matched the patient’s replies to questions was ranked using feature selection through information gain (IG). Among the 45 items, 12 were ranked the highest in feature selection. The ROC analysis showed that AUC in test group was 94.00% [ 96 ]. Stamate et al. had developed a framework for the prediction of MCI and dementia. Their proposed framework was based on the ReliefF approach paired with statistical permutation tests for feature selection, model training, tweaking, and testing using ML algorithms such as RF, SVM, Gaussian Processes, Stochastic Gradient Boosting, and eXtreme Gradient Boosting. The stability of model performances was studied using computationally expensive Monte Carlo simulations, and the results of their proposed framework were given as for dementia detection, the accuracy was 88.00%, sensitivity was 93.00%, and the specificity was 94.00%, whereas moderate cognitive impairment had a sensitivity of 86.00% and a specificity of 90% [ 97 ]. Stamate et al. developed a system for detecting dementia subtypes (AD) in blood utilizing DL and other supervised ML approaches such as RF and extreme gradient boosting. The AUC for the proposed DL method was 85% (0.80–0.89), for XGBoost it was 88% (0.86–0.89), and for RF it was 85% (0.83–0.87). In comparison, CSF measurements of amyloid, p-tau, and t-tau (together with age and gender) gave AUC values of 78%, 83%, and 87%, respectively, by using the XGBoost [ 98 ]. Bansal1 et al. had performed the comparative analysis of the different ML methods for the detection of dementia using clinical-variables. In their experiments, they exploited the performance of four ML models, such as J48, NB, RF, and multilayer perceptrons. From the results of experiments, they had concluded that j48 outperformed the rest of the ML models for the detection of dementia [ 99 ]. Nori et al. had experimented the lasso algorithm on a big dataset of patient and identify the 50 variables by ML model with an AUC of 69.30% [ 100 ]. Alam et al. [ 101 ]used signal processing on wearable sensor data streams (e.g., electrodermal activity (EDA), photoplethysmogram (PPG), and accelerometer (ACC)) and machine learning techniques to measure cognitive deficits and their relationship with functional health deterioration.
Gurevich et al. had used SVM and neuropsychological test for the classification of AD from other causes of cognitive impairment. The highest classification accuracy they had achieved through their proposed method was 89.00% [ 102 ]. Karaglani et al. had proposed a ML based automated diagnosis system for AD by using blood-based biosignatures. In their proposed method, they used mRNA-based statistically equivalent signatures for feature ranking and a RF model for classification. Their proposed automated diagnosis system had reported the accuracy of 84.60% using RF [ 103 ]. Ryzhikova et al. had analyzed cerebrospinal fluid for the diagnosis of AD by using ML algorithms. For classification purposes, artificial neural networks (ANN) and SVM discriminant analysis (SVM-DA) statistical methods were applied, with the best findings allowing for the distinguishing of AD and HC participants with 84.00% sensitivity and specificity. The proposed classification models have a high discriminative power, implying that the technique has a lot of potential for AD diagnosis [ 104 ]. Cho and Chen had designed a double layer dementia diagnosis system based on ML where fuzzy cognitive maps (FCMs) and probability neural networks (PNNs) were used to provide initial diagnoses at the base layer, and Bayesian networks (BNs) were used to provide final diagnoses at the top layer. Diagnosis results, “proposed treatment,” and “no treatment required” might be used to provide medical institutions with self-testing or secondary dementia diagnosis. The highest accuracy reported by their proposed system was 83.00% [ 105 ]. Facal et al. had studied the role of cognitive reserve in the conversion from MCI to dementia using ML. Nine ML classification algorithms were tried in their study, and seven relevant performance parameters were generated to assess the prediction accuracy for converted and non-converted individuals. The use of ML algorithms on socio-demographic, basic health, and CR proxy data allowed for the prediction of dementia conversion. The Gradient Boosting Classifier (ACC = 0.93; F1 = 0.86 and Cohen’s kappa = 0.82) and RF Classifier (ACC = 92%; F1 = 0.79 and Cohen’s kappa = 0.71) performed the best [ 106 ]. Jin et al. had proposed automatic classification of dementia from learning of clinical consensus diagnosis in India using ML techniques. All viable ML models exhibited remarkable discriminative skills (AUC >90%) as well as comparable accuracy and specificity (both around 95%). The SVM model beat other ML models by obtaining the highest sensitivity (0.81), F1 score (0.72), kappa (.70, showing strong agreement), and accuracy (second highest) (0.65). As a consequence, the SVM was chosen as the best model in their research work [ 107 ]. James et al. had evaluated the performance of ML algorithms for predicting the progression of dementia in memory clinic patients. According to their findings, ML algorithms outperformed humans in predicting incident all-cause dementia within two years. Using all 258 variables, the gradient-boosted trees approach had an overall accuracy of 92% , sensitivity of 0.45, specificity of 0.97, and an AUC of 0.92. Analysis of variable significance had indicated that just 6 variables were necessary for ML algorithms to attain an accuracy of 91% and an AUC of at least 89.00% [ 108 ]. Bougea et al. had investigated the effectiveness of logistic regression (LR), K-nearest neighbours (K-NNs), SVM, the Naive Bayes classifier, and the Ensemble Model to correctly predict PDD or DLB. The K-NN classification model exhibited an overall accuracy of 91.2% based on 15 top clinical and cognitive scores, with 96.42% sensitivity and 81% specificity in distinguishing between DLB and PDD. Based on the 15 best characteristics, the binomial logistic regression classification model had attained an accuracy of 87.5%, with 93.93% sensitivity and 87% specificity. Based on the 15 best characteristics, the SVM classification model had achieved an accuracy of 84.6% of overall instances, 90.62% sensitivity, and 78.58% specificity. A model based on NB classification obtained an accuracy of 82.05%, sensitivity of 93.10%, and a specificity of 74.41%. Finally, an ensemble model, which was constructed by combining the separate ones, attained 89.74% accuracy, 93.75% sensitivity, and 85.73% specificity [ 109 ] (Fig. 6 ).
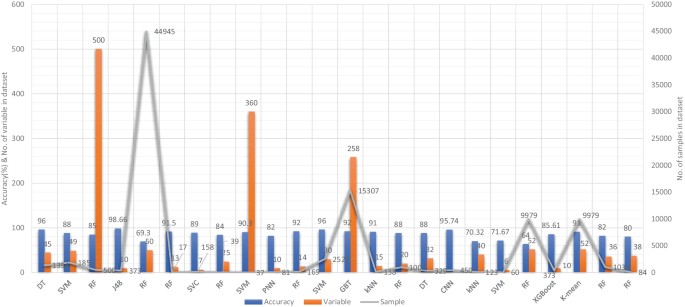
Accuracy comparison of different ML models based on clinical-variable modality
Salem et al. had presented a regression-based ML model for the prediction of dementia. In their proposed method, they had investigated ML approaches for unbalanced learning. In their suggested supervised ML approach, they started by intentionally oversampling the minority class and undersampling the majority class, in order to reduce the bias of the ML model to be trained on the dataset. Furthermore, they had deployed cost-sensitive strategies to penalize the ML models when an instance was misclassified in the minority class. According to their findings, the balanced RF was the most resilient probabilistic model (with just 20 features/variables) with an F1 score of 0.82, a G-Mean of 0.88, and an AUC of 0.88 using ROC. With a F1-score of 0.74 and an AUC of 0.80 by ROC, the calibrated-weighted SVM was their top classification model for the same number of features [ 110 ]. Gutierrez et al. had designed an automated diagnosis system for the detection of AD and FTD by using feature engineering and genetic algorithms. Their proposed system had obtained the accuracy of 84% [ 111 ]. Mirzaei and Adeli had analyzed the state-of-the-art ML techniques used for the detection and classification of AD [ 112 ]. Hsiu et al. had studied ML algorithms for early identification of cognitive impairment. Their proposed model had obtained the accuracy of 70.32% by threefold cross-validation scheme [ 113 ]. Several classification models were constructed using various ML and feature selection methodologies to automate MCI detection using gait biomarkers. They had demonstrated, however, that dual-task walking differentiated between MCI and CN individuals. The ML model used for MCI pre-screening based on inertial sensor-derived gait biomarkers achieved 71.67% accuracy and 83.33% sensitivity, respectively, as reported by Shahzad et al. [ 114 ]. Hane et al. investigated the use of deidentified clinical notes acquired from multiple hospital systems over a 10-year period to enhance retrospective ML models predicting the risk of developing AD. The AUC improved from 85.00% to 94.00% by utilizing clinical notes, and the positive predictive value (PPV) rose from 45.07% (25,245/56,018) to 68.32% (14,153/20,717) in the model at the beginning of disease [ 115 ]. Table 5 provides the overall performance evaluation of the ML models that were presented by the researchers for the prediction of dementia and its subtypes by using clinical-variable data as a modality.
ML based diagnostic models for dementia: Voice modality
Similar to the image and clinical-variable modalities, researchers had also developed automated diagnostic systems based on voice data for the prediction of dementia. Hereby, we have reviewed the research work done by the scientists in detail. For example, Chlasta and Wolk had worked on the computer-based automated screening of dementia patients by spontaneous speech analysis using DL and ML techniques. In their work, they used neural networks to extract the features from the voice data; the extracted features were then fed into a linear SVM for classification purposes. Their SVM model had obtained the accuracy of 59.1% while CNN based ML model had reported the accuracy of 63.6% [ 121 ]. Chien et al. had presented an ML model for the assessment of AD using speech data. Their suggested model included a feature sequence that was used to extract the features from the raw audio data, as well as a recurrent neural network (RNN) for classification. Their proposed ML model had reported an accuracy of 83.80% based on the ROC curve [ 122 ]. Shimoda et al. had designed an ML model that identified the risk of dementia based on the voice feature in telephone conversations. Extreme gradient boosting (XGBoost), RF, and LR based ML models were used, with each audio file serving as one observation. The predictive performance of the constructed ML models was tested by characterizing the ROC curve and determining the AUC, sensitivity, and specificity [ 123 ]. Nishikawa et al. had developed an ensemble discriminating system based on a classifier with statistical acoustic characteristics and a neural network of transformer models, with an F1-score of 90.70% [ 124 ]. Liu et al. had introduced a new technique for recognizing Alzheimer’s disease that used spectrogram features derived from speech data, which aided families in comprehending the illness development of patients at an earlier stage, allowing them to take preventive measures. They used ML techniques to diagnose AD using speech data collected from older adults who displayed the attributes described in the speech. Their proposed method had obtained the maximum accuracy of 84.40% based on LogisticRegressionCV [ 125 ]. Searle et al. had created a ML model to assess spontaneous speech, which might potentially give an efficient diagnostic tool for earlier AD detection. Their suggested model was a fundamental Term Frequency-Inverse Document Frequency (TF-IDF) vectorizer as input into an SVM model, and the top performing models were a pre-trained transformer-based model ’DistilBERT’ when used as an embedding layer into simple linear models. The proposed model had obtained the highest accuracy of 82.00% [ 126 ]. Zhu et al. had suggested an ML model that employed the speech pause as an effective biomarker in dementia detection, with the purpose of reducing the detection, model’s confidence levels by adding perturbation to the speech pauses of the testing samples. They next investigated the impact of the perturbation in training data on the detection model using an adversarial training technique. The proposed model had achieved an accuracy of 84.00% [ 127 ]. Ossewaarde et al. had proposed ML model based on SVM for the classification of spontaneous speech of individuals with dementia based on automatic prosody analysis. Their findings suggest that the classifier can distinguish some dementia types (PPA-NF, AD), but not others (PD) [ 128 ]. Xue et al. had developed an ML model based on DL for the detection of dementia by using voice recordings. In their ML model, long short-term memory (LSTM) network and the convolutional neural network (CNN) utilized audio recordings to categorize whether the recording contained a participant with either NC or only DE and to discriminate between recordings belonging to those with DE and those without DE (i.e., NDE (NC+MCI)) [ 129 ]. Weiner et al. had presented two pipelines of feature extraction for dementia detection: the manual pipeline used manual transcriptions, while the fully automatic pipeline used transcriptions created by automatic speech recognition (ASR). The acoustic and linguistic features that they had extracted need no language specific tools other than the ASR system. Using these two different feature extraction pipelines, they had automatically detect dementia [ 130 ] (Fig. 7 ).
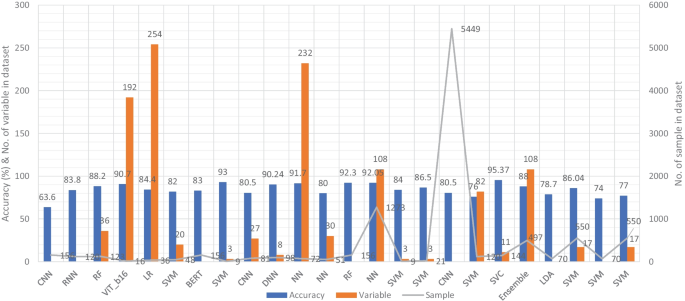
Accuracy comparison of different ML models based on voice modality
Furthermore, Sadeghian et al. had presented the empirical evidence that a combination of acoustic features from speech, linguistic features were extracted from an automatically determined transcription of the speech including punctuation, and results of a mini mental state exam (MMSE) had achieved strong discrimination between subjects with a probable AD versus matched normal controls [ 131 ]. Khodabakhsh et al. had evaluated the linguistic and prosodic characteristics in Turkish conversational language for the identification of AD. Their research suggested that prosodic characteristics outperformed linguistic features by a wide margin. Three of the prosodic features had helped to achieve a classification accuracy of more than 80%, However, their feature fusion experiments did not improve classification performance any more [ 132 ]. Edwards et al. had analyzed the text data at both the word level and phoneme level, which leads to the best-performing system in combination with audio features. Thus, the proposed system was both multi-modal (audio and text) and multi-scale (word and phoneme levels). Experiments with larger neural language models had not resulted in improvement, given the small amount of text data available [ 133 ]. Kumar et al. had identified speech features relevant in predicting AD based on ML. They had deployed neural network for the classification and obtained the accuracy of 92.05% [ 134 ]. Ossewaarde et al. had built ML model based on SVM for the classification from spontaneous speech of individuals with dementia by using automatic prosody [ 128 ]. Luz et al. had developed an ML approach for analyzing patient speech in dialogue for dementia identification. They had designed a prediction model, and the suggested strategy leveraged additive logistic regression (ML boosting method) on content-free data gathered through dialogical interaction. Their proposed model obtained the accuracy of 86.50% [ 135 ]. Sysed et al. had designed a multimodal system that identified linguistic and paralinguistic traits of dementia using an automated screening tool. Their proposed system had used bag-of-deep-feature for feature selection and ensemble model for classification [ 136 ]. Moreover, Sarawgi et al. had used multimodal inductive transfer learning for AD detection and severity. Their proposed system further achieved state-of-the-art AD classification accuracy of 88.0% when evaluated on the full benchmark DementiaBank Pitt database. Table 6 provides the overall performance evaluation of the ML models that were presented by the researchers for the prediction of dementia and its subtypes by using voice-modality data.
In this SLR, we examined the research work that employed ML and DL algorithms to analyze clinical data in order to identify variables that might help predict dementia. We studied 75 research articles that were published in the last 10 years that used image, clinical-variable, and voice data to predict dementia and its subtypes. Nowadays, the healthcare industry creates a vast quantity of data on patients’ health; this data is used by researchers to enhance individual health by utilizing developing technologies such as ML and DL. As a result, researchers can not only distinguish dementia patients from healthy people with high accuracy, but also forecast the disease progression of MCI patients.
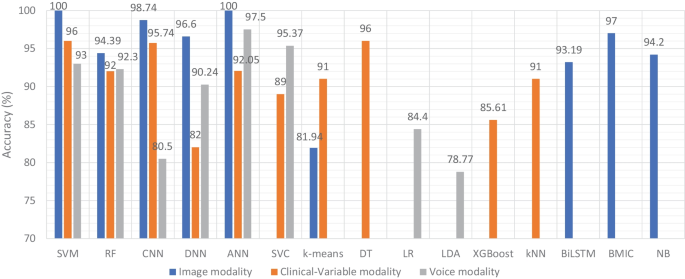
Accuracy comparison of ML models based on data modality
Therefore, researchers have expressed a strong interest in designing and developing automated diagnostic systems based on ML and DL techniques. As seen in Fig. 4 ., there has been an exponential increase in the number of such research publications that use ML algorithms for dementia prediction and detection in the previous four years. We investigated the selected papers using significant performance assessment criteria for ML and DL approaches such as data attributes, computational methodologies, and study emphasis. In this SLR, we have uncovered research gaps in the present literature as well as anticipated future research opportunities. Additionally, in Fig. 8 model comparison, we examined the performance of multiple ML algorithms for dementia prediction based on three types of data modalities: image, clinical-variable, and voice. The accuracy gained by image-based ML algorithms is higher when compared to clinical-variable and voice modalities, as shown in Fig. 8 model comparison. Moreover, the researchers’ suggested SVM, RF, and ANN-based ML techniques outperformed the rest of the ML algorithms in terms of performance. According to Fig. 8 model comparison, voice modality-based ML models show worse accuracy when compared to image and clinical-variable modality data. As a result, there is still a performance gap for researchers to close in order to improve the performance of ML algorithms for the prediction of dementia using voice data. Hence, researchers have shown a strong interest in the creation of automated diagnosis systems for dementia prediction utilizing speech data and ML algorithms, as illustrated in Fig. 4 .
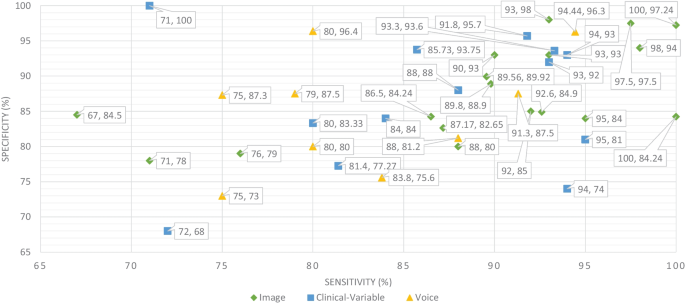
Sensitivity and specificity comparison of ML based on modality
The ML and DL models are likely prone to problems such as poor quality of data, poor selection of ML model, Bias Variance tradeoff and training too complex models. Thus, scientists have developed various evaluation metrics (i.e., ROC, AUC, MCC, F1-score, K-fold) and methods to avoid these problems. The data is a crucial element in ML because ML models work only with numeric data; therefore, poor data quality results in lower performance of ML models. Moreover, imbalance classes in the dataset also cause the bias results from the ML models. Thus, this problem can be overcome by oversampling or undersampling the training data. There are different techniques that are used by the AI engineers for oversampling, such as random oversampling and the synthetic minority oversampling technique (SMOTE). To evaluate the bias researchers’ work, use sensitivity and specificity as an evaluation metric to measure the bias of the ML model. Higher values of sensitivity and specificity means model is free from the biasness while having either one parameter value higher and other one is lower means there is biasness exist. Thus, we have also studied the sensitivity and specificity, along with the accuracy, of the previously proposed ML models for dementia prediction. Figure 9 Comparison provides a brief description of the sensitivity and specificity of the ML models for the detection of dementia based on different data modalities. From Fig. 9 , we can observe that ML models have higher values for sensitivity and specificity when using image data as compared to clinical-variable and voice modality data. In comparison to accuracy from Fig. 8 to sensitivity and specificity from Fig. 9 , we have noted that the results obtained from image based modality are more reliable and precise using ML and DL algorithms in spite of clinical and voice modality.
Furthermore, the correlation between sensitivity and specificity would help us understand the efficacy of the ML models, which are designed for automated disease prediction. The mathematical terms “sensitivity” and “specificity” indicate the accuracy of a test that reports the presence or absence of a disease. Individuals who meet the requirement are labelled “positive,” while those who do not are considered “negative”. The chance of a positive test, conditioned on being actually positive, is referred to as sensitivity (the true positive rate), while specificity (true negative rate) is the likelihood of a negative test if it is actually negative. Sensitivity and specificity are inversely proportional, which means that as sensitivity rises, specificity falls, and vice versa. Mathematically, sensitivity and specificity are given as:
On the other hand, accuracy is a ratio of number of correct assessments / number of all assessments. The proportion of genuine positive outcomes (both true positive and true negative) in the selected population is represented by the numerical value of accuracy. The test result is accurate 99% of the time, whether positive or negative. For the most part, this is right. However, it is worth noting that the equation of accuracy means that even if both sensitivity and specificity are high, say 99%, this does not imply that the test’s accuracy is also high. In addition to sensitivity and specificity, accuracy is determined by the prevalence of the illness in the target population. A diagnosis for a rare ailment in the target group may have high sensitivity and specificity but low accuracy. However, for a balanced dataset, ML models with higher sensitivity and specificity result in higher accuracy. Hence, accuracy must be interpreted carefully. The mathematical formula for accuracy is given as:
where, TP stands for the number of true positives, FP stands for the number of false positives, TN stands for the true negative, and FN stands for the false negative.
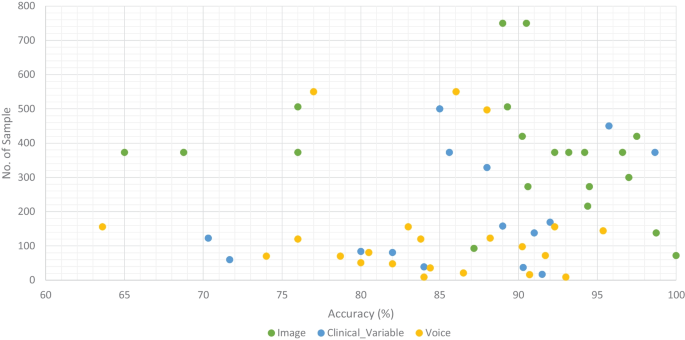
Accuracy comparison of ML models along with number of sample in the dataset based on data modality
We classified all datasets that were used by researchers to test the performance of their proposed ML models for the prediction of dementia (AD, VaD, MCI, and FTD) into three types: image, clinical-variable, and voice. A total of 61 datasets were examined in terms of the number of samples and variables in the datasets. In image modality datasets from the Table 1 , it can be observed that the ADNI dataset has a significant number of samples, which is 750, while the NINDS-AIREN dataset has more variables as compared to the rest of the datasets in the image modality data. Moreover, from the Table 2 of clinical-variable modality datasets, it can be noticed that the ADRD dataset has the highest number of samples (44945) as compared to the rest of the dataset, while the Raman spectral dataset has the highest number of variables (366). In the last, Table 3 of voice data modality elaborated the dataset of voice modality where FHS dataset has highest number of samples of 5449 while VBSD dataset had highest variables of 254 as compared to rest of the datasets in voice modality. The type of data and the size of the dataset are two important factors that have a significant influence on the performance of ML models. Thus, we have also studied this factor by comparing the accuracy along with the number of samples in the dataset with respect to data modalities. From Fig. 10 , it can be observed that the majority of the ML models that used image data have higher accuracy along with a higher number of samples in the dataset. There are few ML models that show poor performance when the number of samples in the dataset is large. While, clinical-variable and voice modalities show prominent performance when the number of samples in the dataset is small.
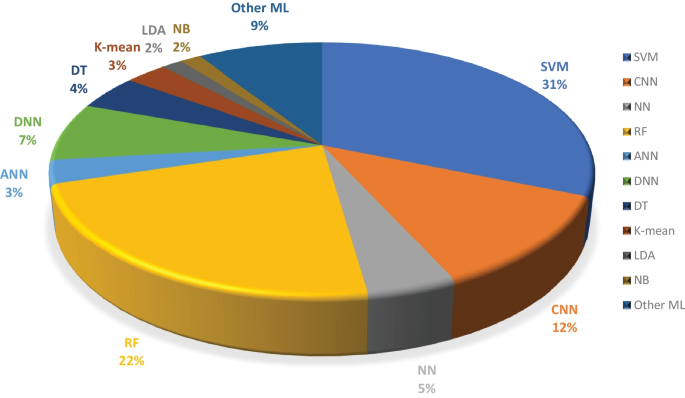
Overall percentage of ML models used in the selected research articles regardless of data modality
Moreover, we examined the effectiveness of ML classifiers utilized by the researchers in their proposed automated diagnostic systems for dementia prediction and classification. According to the selected studies of this SLR, SVM is the most commonly used ML classifier by researchers for the classification of patients and normal subjects using three data modalities (i.e., image, clinical-variable, voice), RF is the second most commonly used ML classifier by researchers, and CNN is the third most commonly used ML classifier by researchers. It can be observed from the Fig. 11 . SVMs are the most powerful tools for the binary classification task, along with RF. From Fig. 8 , we can see that SVM also obtained the highest average accuracy based on three types of data modalities. Hence, this factor also encourages the scientists to employ SVM as a binary classifier for dementia prediction or other disease prediction systems. From Fig. 11 , we can observe the percentage of other ML classifiers that were used by the researchers in selected research articles for the automated diagnosis of dementia.
There are several evaluation metrics that are used for the performance assessment of ML models, such as F1score, AUC, ROC, Matthew’s correlation coefficient (MCC), cross-validation, K-fold, specificity, sensitivity, and accuracy. Each evaluation metric has its own pros and cons. Thus, the selection of appropriate evaluation metrics for the assessment of the ML model is essential to understanding its efficiency and performance. For instance, when data plays a vital role in ML models for decision-making and a dataset has unbalanced classes, it may be possible that results from the ML predictive model might be biassed due to the unbalanced nature of the data in the dataset. Thus, here evaluation metrics help to eliminate the factor of biasness in the results, i.e., the k-fold. The F1-score evolution metric is suitable for the classification of multiple classes in the dataset. while ROC tells us how well the ML model can differentiate binary classes. As a result, AUC and ROC reveal how effectively the probabilities from the positive classes are separated from the probabilities from the negative classes. From Fig. 12 , it can be depicted that cross validation is mostly used in the studies that were selected for this SLR to evaluate the performance of proposed ML models. MCC is the second most used evaluation metric, while ROC is in third place. The proposition of other evaluation metrics used by the researchers to validate the efficiency of their proposed ML models can be observed from Fig. 12 .
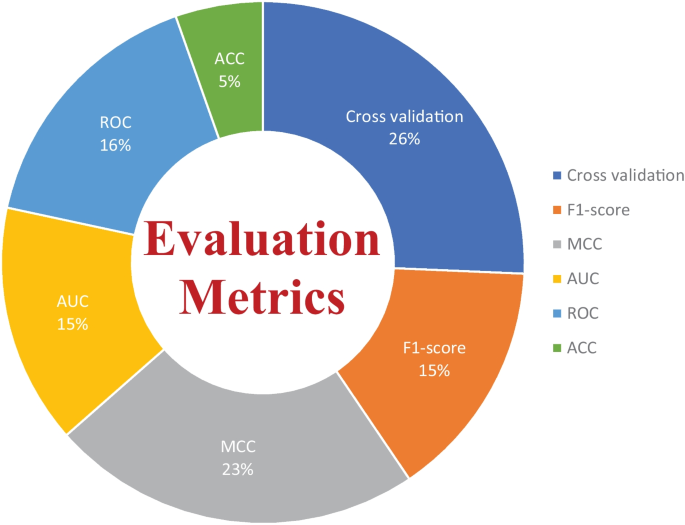
Overall percentage of evaluation metrics of ML models used by the researchers in the selected research articles
Limitations in the previously proposed ML models
ML algorithms have been effectively applied to a broad range of real-world challenges, including banking, cybersecurity, transportation, and robots. They do, however, have fundamental limitations that make them inappropriate for every problem. In the clinical domain, researchers have concentrated on the supervised learning approach, developing various automated diagnostics for AD, MCI, and dementia prediction using supervised machine algorithms. From the Figs. 8 and 11 , It can be noticed that supervised ML classifiers are mostly used by the researchers in the selected past research articles. Because supervised machine learning approaches have various limitations, automated diagnostic methods for dementia prediction based on supervised techniques suffer from some, if not all, of these constraints. In this part, we have examined the drawbacks of supervised ML-based techniques for dementia prediction, which are as follows:
The model overfitting problem affects the performance of ML models. As previously indicated, several researchers have used the k-fold cross-validation approach to evaluate the efficacy of their constructed diagnostic system. However, because of data leaks, it may result in highly biassed findings.
To deal with problem of imbalance classes in the dataset, Researchers and scientists had devised several techniques to eliminate the problem of imbalance classes such as random oversampling example (ROSE), synthetic minority over-sampling technique (SMOTE) and random over sampling (ROS) etc. Unfortunately, in the selected study, the researchers had not considered this factor to deal with the problem of imbalanced classes in the dataset that cause problems of bias.
Supervised ML models require training on a dataset; nevertheless, training on a large quantity of significant data is a hard and time-consuming job, especially for slow learning algorithms like kNN.
For training and testing of the ML models, researchers had used different data partitioning methods, which resulted in inconsistent comparisons of accuracy and other evaluation metrics among the proposed ML models for dementia prediction. Thus, standard data partition schemes should be adopted (holdout) for the comparison of ML models developed by the researcher for dementia prediction.
Another challenge with ML-based automated diagnostic systems for dementia is the time complexity of the proposed ML algorithms. The time complexity means the overall time require to complete all the computational tasks by the ML model for making a prediction. The ML model can forecast results only after it has been trained on the training data, which takes time to analyze. Furthermore, ML models include a large number of parameters that must be manually modified in the case of supervised learning. As a result, it takes a significant amount of effort and time to fine-tune the hyperparameters of the ML model in order to get higher performance.
DL technology has demonstrated cutting-edge performances for the prediction of various diseases in the recent years. However, DL technology needs a massive quantity of data for model training, which is a time-consuming and tough task. Due to the complexity of data models, training is quite costly. Furthermore, DL necessitates the use of pricey GPUs and hundreds of workstations, which are not effective in terms of economics.
Future research directions
In recent years, several ML models have been presented for the prediction of AD and MCI; nevertheless, there are still certain areas that need to be explored by academics and experts. In this section, we have discussed different research areas and the future prospects of ML algorithms for dementia detection. We infer from this study that the following major parameters have a role in the efficient identification of dementia and its forms.
Data is extremely important in the case of ML-based automated detection of dementia, especially when DL models are considered. Many of the publicly available datasets, however, are modest in size. But future research should concentrate on gathering a huge number of samples for the datasets. In this SLR, we studied ML-based automated diagnostic systems for dementia prediction using three different kinds of data modalities (image, clinical_variable, voice). From Fig. 10 , it can be observed that only the image modality based ML model obtained the higher accuracy along with the large size of the dataset, while the voice modality based ML model obtained the higher accuracy on a small dataset. Thus, for the researchers, there is still room available for designing and developing the automated prediction of dementia and its sub-types by using voice data. Therefore, the interest of researchers have been tremendously raised for the development of automated diagnostic systems for dementia prediction using voice data modality and this trend can be confirmed from the Fig. 4 . There is still a lot room available for the improvement in design and construction of automated diagnostic systems for the dementia using clinical-variable data modality for the researchers. Because, the ML model was developed in the past using clinical-variable data, it displays mix performance by using clinical_variable modality, i.e., when the number of samples is lower in the dataset, the ML shows lower accuracy. Thus. In the future, we need to increase the number of samples in the dataset so that we have larger datasets for experimental purposes and the designed ML model can be effectively evaluated.
In selected studies of this SLR, the majority of ML algorithms belong to the supervised category of learning. While few researchers used an unsupervised ML approach for the prediction of dementia and its subtypes, Altough, unspervsied learning approaches suffer from the limitation such as less accuracy, more expensive in term of computational etc. Therefore, it will encourage scientists and researchers to design and construct new techniques and methods using supervised ML algorithms that are more precise and accurate for the prediction of dementia and its subtypes. Moreover, in this SLR, we have analysed the various ML models based on three data modalities (image, clinical-variable, and voice), and we have comprehensively compared previously proposed ML-based systems in terms of various evaluation metrics, but with different data modalities, it would be suggested that multimodal processing techniques based on ML would provide more reliable and efficient results. Hence, in the future, researchers should exploit multimodal approaches based on ML for a better prediction of dementia and its subtypes.
In contrast to earlier SLR studies that examined numerous ML techniques proposed for the automated diagnosis of dementia and its subtypes (AD, VaD, FTD, and MCI) using one type of data modality, this study reviewed ML methods for dementia considering different types of data modalities such as image data, clinical variables, and voice data. The research articles published from 2011 to 2022 were gathered using different databases. It was pointed out that ML approaches based on image data modality has shown better performance compared with ML methods trained on clinical variables based data and voice data modality. Furthermore, this study critically evaluated the previously proposed methods and highlighted limitations in these methods. To overcome these limitations, this study presented future research directions in the domain of automated dementia prediction using ML approaches. We hope that this SLR will be helpful for AI and ML researchers and medical practitioners who are working in the domain of automated diagnostic systems for dementia prediction.
Data Availability
Not applicable.
Code Availability
Menéndez, G.: La revolución de la longevidad: cambio tecnológico, envejecimiento poblacional y transformación cultural. Revista de Ciencias Sociales 30 (41), 159–178 (2017)
Prince, M.J., Wimo, A., Guerchet, M.M., Ali, G.C., Wu, Y.-T., Prina, M.: World alzheimer report 2015-the global impact of dementia: An analysis of prevalence, incidence, cost and trends (2015)
Vrijsen, J., Matulessij, T., Joxhorst, T., de Rooij, S.E., Smidt, N.: Knowledge, health beliefs and attitudes towards dementia and dementia risk reduction among the dutch general population: a cross-sectional study. BMC public health 21 (1), 1–11 (2021)
Widiger, T.A., Costa, P.T., Association, A.P., et al : Personality Disorders and the Five-factor Model of Personality. JSTOR, (2013)
Lo, R.Y.: The borderland between normal aging and dementia. Tzu-Chi Medical Journal 29 (2), 65 (2017)
WHO: Dementia. World Health Organization. https://www.who.int/news-room/fact-sheets/detail/dementia
Budson, A.E., Solomon, P.R.: Memory Loss E-book: A Practical Guide for Clinicians. Elsevier Health Sciences, (2011)
friendly wyoming, D.: Types of dementia. Dementia friendly wyoming. https://www.dfwsheridan.org/types-dementia
Tackenberg, C., Kulic, L., Nitsch, R.M.: Familial alzheimer’s disease mutations at position 22 of the amyloid \(\beta\) -peptide sequence differentially affect synaptic loss, tau phosphorylation and neuronal cell death in an ex vivo system. PloS one 15 (9), 0239584 (2020)
Wang, H., Kulas, J.A., Wang, C., Holtzman, D.M., Ferris, H.A., Hansen, S.B.: Regulation of beta-amyloid production in neurons by astrocyte-derived cholesterol. Proceedings of the National Academy of Sciences 118 (33) (2021)
Vilchez, D., Saez, I., Dillin, A.: The role of protein clearance mechanisms in organismal ageing and age-related diseases. Nature communications 5 (1), 1–13 (2014)
Breijyeh, Z., Karaman, R.: Comprehensive review on alzheimer’s disease: Causes and treatment. Molecules 25 (24), 5789 (2020)
Long, J.M., Holtzman, D.M.: Alzheimer disease: an update on pathobiology and treatment strategies. Cell 179 (2), 312–339 (2019)
Román, G.C.: Vascular dementia may be the most common form of dementia in the elderly. Journal of the neurological sciences 203 , 7–10 (2002)
Gold, G., Bouras, C., Canuto, A., Bergallo, M.F., Herrmann, F.R., Hof, P.R., Mayor, P.-A., Michel, J.-P., Giannakopoulos, P.: Clinicopathological validation study of four sets of clinical criteria for vascular dementia. American Journal of Psychiatry 159 (1), 82–87 (2002)
Lina, R.: Atrial fibrillation in aging; methodological aspects and the relation to dementia and cerebral vascular disease (2022)
Kuźma, E., Lourida, I., Moore, S.F., Levine, D.A., Ukoumunne, O.C., Llewellyn, D.J.: Stroke and dementia risk: a systematic review and meta-analysis. Alzheimer’s & Dementia 14 (11), 1416–1426 (2018)
Tay, J., Morris, R.G., Tuladhar, A.M., Husain, M., de Leeuw, F.-E., Markus, H.S.: Apathy, but not depression, predicts all-cause dementia in cerebral small vessel disease. Journal of Neurology, Neurosurgery & Psychiatry 91 (9), 953–959 (2020)
Morton, R.E., St. John, P.D., Tyas, S.L.: Migraine and the risk of all-cause dementia, alzheimer’s disease, and vascular dementia: A prospective cohort study in community-dwelling older adults. International journal of geriatric psychiatry 34 (11), 1667–1676 (2019)
Sanford, A.M.: Lewy body dementia. Clinics in geriatric medicine 34 (4), 603–615 (2018)
Taylor, J.-P., McKeith, I.G., Burn, D.J., Boeve, B.F., Weintraub, D., Bamford, C., Allan, L.M., Thomas, A.J., T O’Brien, J.: New evidence on the management of lewy body dementia. The Lancet Neurology 19 (2), 157–169 (2020)
McKEITH, I.G., Galasko, D., Wilcock, G.K., Byrne, E.J.: Lewy body dementia–diagnosis and treatment. The British Journal of Psychiatry 167 (6), 709–717 (1995)
Surendranathan, A., Kane, J.P., Bentley, A., Barker, S.A., Taylor, J.-P., Thomas, A.J., Allan, L.M., McNally, R.J., James, P.W., McKeith, I.G., et al.: Clinical diagnosis of lewy body dementia. BJPsych open 6 (4) (2020)
Finger, E.C.: Frontotemporal dementias. Continuum: Lifelong Learning in Neurology 22 (2 Dementia), 464 (2016)
Rabinovici, G.D., Miller, B.L.: Frontotemporal lobar degeneration. CNS drugs 24 (5), 375–398 (2010)
Organization, W.H., et al.: The ICD-10 classification of mental and behavioural disorders. Clinical descriptions and diagnostic guidelines; 2004. Geneva: WHO (2021)
Ghetti, B., Buratti, E., Boeve, B., Rademakers, R.: Frontotemporal Dementias vol. 320. Springer, (2021)
Custodio, N., Montesinos, R., Lira, D., Herrera-Pérez, E., Bardales, Y., Valeriano-Lorenzo, L.: Mixed dementia: A review of the evidence. Dementia & neuropsychologia 11 , 364–370 (2017)
Garcia-Ptacek, S., Kåreholt, I., Cermakova, P., Rizzuto, D., Religa, D., Eriksdotter, M.: Causes of death according to death certificates in individuals with dementia: a cohort from the swedish dementia registry. Journal of the American Geriatrics Society 64 (11), 137–142 (2016)
Arvanitakis, Z., Shah, R.C., Bennett, D.A.: Diagnosis and management of dementia. Jama 322 (16), 1589–1599 (2019)
Ullah, H., Bin Heyat, M.B., AlSalman, H., Khan, H.M., Akhtar, F., Gumaei, A., Mehdi, A., Muaad, A.Y., Islam, M.S., Ali, A., et al.: An effective and lightweight deep electrocardiography arrhythmia recognition model using novel special and native structural regularization techniques on cardiac signal. Journal of Healthcare Engineering 2022 (2022)
Javeed, A., Ali, L., Mohammed Seid, A., Ali, A., Khan, D., Imrana, Y.: A clinical decision support system (cdss) for unbiased prediction of caesarean section based on features extraction and optimized classification. Computational Intelligence and Neuroscience 2022 (2022)
Imrana, Y., Xiang, Y., Ali, L., Abdul-Rauf, Z., Hu, Y.-C., Kadry, S., Lim, S.: \(\chi\) 2-bidlstm: A feature driven intrusion detection system based on \(\chi\) 2 statistical model and bidirectional lstm. Sensors 22 (5), 2018 (2022)
Ali, L., Zhu, C., Zhao, H., Zhang, Z., Liu, Y.: An integrated system for unbiased parkinson’s disease detection from handwritten drawings. In: Advances in Intelligent Systems and Computing, pp. 3–13. Springer, (2022)
Ali, L., Niamat, A., Khan, J.A., Golilarz, N.A., Xingzhong, X., Noor, A., Nour, R., Bukhari, S.A.C.: An optimized stacked support vector machines based expert system for the effective prediction of heart failure. IEEE Access 7 , 54007–54014 (2019)
Ali, L., Zhu, C., Zhou, M., Liu, Y.: Early diagnosis of parkinson’s disease from multiple voice recordings by simultaneous sample and feature selection. Expert Systems with Applications 137 , 22–28 (2019)
Ali, L., Khan, S.U., Golilarz, N.A., Yakubu, I., Qasim, I., Noor, A., Nour, R.: A feature-driven decision support system for heart failure prediction based on statistical model and gaussian naive bayes. Computational and Mathematical Methods in Medicine 2019 (2019)
Mehbodniya, A., Khan, I.R., Chakraborty, S., Karthik, M., Mehta, K., Ali, L., Nuagah, S.J.: Data mining in employee healthcare detection using intelligence techniques for industry development. Journal of Healthcare Engineering 2022 (2022)
Ali, L., Khan, S.U., Arshad, M., Ali, S., Anwar, M.: A multi-model framework for evaluating type of speech samples having complementary information about parkinson’s disease. In: 2019 International Conference on Electrical, Communication, and Computer Engineering (ICECCE), pp. 1–5 (2019). IEEE
Ahmad, F.S., Ali, L., Khattak, H.A., Hameed, T., Wajahat, I., Kadry, S., Bukhari, S.A.C., et al : A hybrid machine learning framework to predict mortality in paralytic ileus patients using electronic health records (ehrs). Journal of Ambient Intelligence and Humanized Computing 12 (3), 3283–3293 (2021)
Ali, L., Wajahat, I., Golilarz, N.A., Keshtkar, F., Bukhari, S.A.C.: Lda–ga–svm: improved hepatocellular carcinoma prediction through dimensionality reduction and genetically optimized support vector machine. Neural Computing and Applications 33 (7), 2783–2792 (2021)
Ali, L., Zhu, C., Zhang, Z., Liu, Y.: Automated detection of parkinson’s disease based on multiple types of sustained phonations using linear discriminant analysis and genetically optimized neural network. IEEE journal of translational engineering in health and medicine 7 , 1–10 (2019)
Ali, L., He, Z., Cao, W., Rauf, H.T., Imrana, Y., Heyat, M.B.B.: Mmdd-ensemble: A multimodal data–driven ensemble approach for parkinson’s disease detection. Frontiers in Neuroscience 15 (2021)
Rehman, A., Khan, A., Ali, M.A., Khan, M.U., Khan, S.U., Ali, L.: Performance analysis of pca, sparse pca, kernel pca and incremental pca algorithms for heart failure prediction. In: 2020 International Conference on Electrical, Communication, and Computer Engineering (ICECCE), pp. 1–5 (2020). IEEE
Ahmed, F.S., Ali, L., Joseph, B.A., Ikram, A., Mustafa, R.U., Bukhari, S.A.C.: A statistically rigorous deep neural network approach to predict mortality in trauma patients admitted to the intensive care unit. Journal of Trauma and Acute Care Surgery 89 (4), 736–742 (2020)
Imrana, Y., Xiang, Y., Ali, L., Abdul-Rauf, Z.: A bidirectional lstm deep learning approach for intrusion detection. Expert Systems with Applications 185 , 115524 (2021)
Akbar, W., Wu, W.-p., Saleem, S., Farhan, M., Saleem, M.A., Javeed, A., Ali, L.: Development of hepatitis disease detection system by exploiting sparsity in linear support vector machine to improve strength of adaboost ensemble model. Mobile Information Systems 2020 (2020)
Ali, L., Bukhari, S.: An approach based on mutually informed neural networks to optimize the generalization capabilities of decision support systems developed for heart failure prediction. Irbm 42 (5), 345–352 (2021)
McInnes, M.D., Moher, D., Thombs, B.D., McGrath, T.A., Bossuyt, P.M., Clifford, T., Cohen, J.F., Deeks, J.J., Gatsonis, C., Hooft, L., et al : Preferred reporting items for a systematic review and meta-analysis of diagnostic test accuracy studies: the prisma-dta statement. Jama 319 (4), 388–396 (2018)
Liberati, A., Altman, D.G., Tetzlaff, J., Mulrow, C., Gøtzsche, P.C., Ioannidis, J.P., Clarke, M., Devereaux, P.J., Kleijnen, J., Moher, D.: The prisma statement for reporting systematic reviews and meta-analyses of studies that evaluate health care interventions: explanation and elaboration. Journal of clinical epidemiology 62 (10), 1–34 (2009)
Martí-Juan, G., Sanroma-Guell, G., Piella, G.: A survey on machine and statistical learning for longitudinal analysis of neuroimaging data in alzheimer’s disease. Computer methods and programs in biomedicine 189 , 105348 (2020)
McGinnis, J.M., Olsen, L., Goolsby, W.A., Grossmann, C., et al : Clinical Data as the Basic Staple of Health Learning: Creating and Protecting a Public Good: Workshop Summary. National Academies Press, (2011)
Vaughn, V.M., Linder, J.A.: Thoughtless design of the electronic health record drives overuse, but purposeful design can nudge improved patient care. BMJ Publishing Group Ltd (2018)
Doody, R., Stevens, J., Beck, C., Dubinsky, R., Kaye, J., Gwyther, L., Mohs, R., Thal, L., Whitehouse, P., DeKosky, S., et al : Practice parameter: Management of dementia (an evidence-based review): Report of the quality standards subcommittee of the american academy of neurology. Neurology 56 (9), 1154–1166 (2001)
Chi, C.-L., Zeng, W., Oh, W., Borson, S., Lenskaia, T., Shen, X., Tonellato, P.J.: Personalized long-term prediction of cognitive function: Using sequential assessments to improve model performance. Journal of biomedical informatics 76 , 78–86 (2017)
Johnson, K.A., Minoshima, S., Bohnen, N.I., Donohoe, K.J., Foster, N.L., Herscovitch, P., Karlawish, J.H., Rowe, C.C., Carrillo, M.C., Hartley, D.M., et al : Appropriate use criteria for amyloid pet: a report of the amyloid imaging task force, the society of nuclear medicine and molecular imaging, and the alzheimer’s association. Alzheimer’s & Dementia 9 (1), 1–16 (2013)
Shaw, L.M., Arias, J., Blennow, K., Galasko, D., Molinuevo, J.L., Salloway, S., Schindler, S., Carrillo, M.C., Hendrix, J.A., Ross, A., et al : Appropriate use criteria for lumbar puncture and cerebrospinal fluid testing in the diagnosis of alzheimer’s disease. Alzheimer’s & Dementia 14 (11), 1505–1521 (2018)
Mills, K.L., Tamnes, C.K.: Methods and considerations for longitudinal structural brain imaging analysis across development. Developmental cognitive neuroscience 9 , 172–190 (2014)
Ali, L., Zhu, C., Golilarz, N.A., Javeed, A., Zhou, M., Liu, Y.: Reliable parkinson’s disease detection by analyzing handwritten drawings: construction of an unbiased cascaded learning system based on feature selection and adaptive boosting model. Ieee Access 7 , 116480–116489 (2019)
Javeed, A., Rizvi, S.S., Zhou, S., Riaz, R., Khan, S.U., Kwon, S.J.: Heart risk failure prediction using a novel feature selection method for feature refinement and neural network for classification. Mobile Information Systems 2020 (2020)
Javeed, A., Zhou, S., Yongjian, L., Qasim, I., Noor, A., Nour, R.: An intelligent learning system based on random search algorithm and optimized random forest model for improved heart disease detection. IEEE Access 7 , 180235–180243 (2019)
Ali, L., Rahman, A., Khan, A., Zhou, M., Javeed, A., Khan, J.A.: An automated diagnostic system for heart disease prediction based on chi2 statistical model and optimally configured deep neural network. IEEE Access 7 , 34938–34945 (2019)
Maddox, T.M., Rumsfeld, J.S., Payne, P.R.: Questions for artificial intelligence in health care. Jama 321 (1), 31–32 (2019)
Chen, P.-H.C., Liu, Y., Peng, L.: How to develop machine learning models for healthcare. Nature materials 18 (5), 410–414 (2019)
Javeed, A., Khan, S.U., Ali, L., Ali, S., Imrana, Y., Rahman, A.: Machine learning-based automated diagnostic systems developed for heart failure prediction using different types of data modalities: A systematic review and future directions. Computational and Mathematical Methods in Medicine 2022 (2022)
Thacker, S.B., Qualters, J.R., Lee, L.M., for Disease Control, C., Prevention, et al : Public health surveillance in the united states: evolution and challenges. MMWR Suppl 61 (3), 3–9 (2012)
Boerma, J.T., Stansfield, S.K.: Health statistics now: are we making the right investments? The Lancet 369 (9563), 779–786 (2007)
Walport, M., Brest, P.: Sharing research data to improve public health. The Lancet 377 (9765), 537–539 (2011)
Van Panhuis, W.G., Paul, P., Emerson, C., Grefenstette, J., Wilder, R., Herbst, A.J., Heymann, D., Burke, D.S.: A systematic review of barriers to data sharing in public health. BMC public health 14 (1), 1–9 (2014)
Dashtipour, K., Taylor, W., Ansari, S., Zahid, A., Gogate, M., Ahmad, J., Assaleh, K., Arshad, K., Imran, M.A., Abbai, Q.: Detecting alzheimer’s disease using machine learning methods. In: EAI (2021)
Helaly, H.A., Badawy, M., Haikal, A.Y.: Deep learning approach for early detection of alzheimer’s disease. Cognitive Computation, 1–17 (2021)
Vandenberghe, R., Nelissen, N., Salmon, E., Ivanoiu, A., Hasselbalch, S., Andersen, A., Korner, A., Minthon, L., Brooks, D.J., Van Laere, K., et al : Binary classification of 18f-flutemetamol pet using machine learning: comparison with visual reads and structural mri. Neuroimage 64 , 517–525 (2013)
Odusami, M., Maskeliūnas, R., Damaševičius, R., Krilavičius, T.: Analysis of features of alzheimer’s disease: Detection of early stage from functional brain changes in magnetic resonance images using a finetuned resnet18 network. Diagnostics 11 (6), 1071 (2021)
Zheng, Y., Guo, H., Zhang, L., Wu, J., Li, Q., Lv, F.: Machine learning-based framework for differential diagnosis between vascular dementia and alzheimer’s disease using structural mri features. Frontiers in Neurology, 1097 (2019)
Basheer, S., Bhatia, S., Sakri, S.B.: Computational modeling of dementia prediction using deep neural network: Analysis on oasis dataset. IEEE Access 9 , 42449–42462 (2021)
Leong, L.K., Abdullah, A.A.: Prediction of alzheimer’s disease (ad) using machine learning techniques with boruta algorithm as feature selection method. In: Journal of Physics: Conference Series, vol. 1372, p. 012065 (2019). IOP Publishing
Battineni, G., Chintalapudi, N., Amenta, F.: Machine learning in medicine: Performance calculation of dementia prediction by support vector machines (svm). Informatics in Medicine Unlocked 16 , 100200 (2019)
Mathotaarachchi, S., Pascoal, T.A., Shin, M., Benedet, A.L., Kang, M.S., Beaudry, T., Fonov, V.S., Gauthier, S., Rosa-Neto, P., Initiative, A.D.N., et al : Identifying incipient dementia individuals using machine learning and amyloid imaging. Neurobiology of aging 59 , 80–90 (2017)
Aruna, S., Chitra, S.: Machine learning approach for identifying dementia from mri images. International Journal of Computer and Information Engineering 9 (3), 881–888 (2016)
Herzog, N.J., Magoulas, G.D.: Brain asymmetry detection and machine learning classification for diagnosis of early dementia. Sensors 21 (3), 778 (2021)
Battineni, G., Chintalapudi, N., Amenta, F., Traini, E.: A comprehensive machine-learning model applied to magnetic resonance imaging (mri) to predict alzheimer’s disease (ad) in older subjects. Journal of Clinical Medicine 9 (7), 2146 (2020)
Ma, D., Yee, E., Stocks, J.K., Jenkins, L.M., Popuri, K., Chausse, G., Wang, L., Probst, S., Beg, M.F.: Blinded clinical evaluation for dementia of alzheimer’s type classification using fdg-pet: A comparison between feature-engineered and non-feature-engineered machine learning methods. Journal of Alzheimer’s Disease 80 (2), 715–726 (2021)
Bidani, A., Gouider, M.S., Travieso-González, C.M.: Dementia detection and classification from mri images using deep neural networks and transfer learning. In: International Work-Conference on Artificial Neural Networks, pp. 925–933 (2019). Springer
Moscoso, A., Silva-Rodríguez, J., Aldrey, J.M., Cortés, J., Fernández-Ferreiro, A., Gómez-Lado, N., Ruibal, Á., Aguiar, P., Initiative, A.D.N., et al : Prediction of alzheimer’s disease dementia with mri beyond the short-term: Implications for the design of predictive models. NeuroImage: Clinical 23 , 101837 (2019)
Khan, A., Zubair, S.: An improved multi-modal based machine learning approach for the prognosis of alzheimer’s disease. Journal of King Saud University-Computer and Information Sciences (2020)
Mohammed, B.A., Senan, E.M., Rassem, T.H., Makbol, N.M., Alanazi, A.A., Al-Mekhlafi, Z.G., Almurayziq, T.S., Ghaleb, F.A.: Multi-method analysis of medical records and mri images for early diagnosis of dementia and alzheimer’s disease based on deep learning and hybrid methods. Electronics 10 (22), 2860 (2021)
Salvatore, C., Cerasa, A., Battista, P., Gilardi, M.C., Quattrone, A., Castiglioni, I.: Magnetic resonance imaging biomarkers for the early diagnosis of alzheimer’s disease: a machine learning approach. Frontiers in neuroscience 9 , 307 (2015)
Katako, A., Shelton, P., Goertzen, A.L., Levin, D., Bybel, B., Aljuaid, M., Yoon, H.J., Kang, D.Y., Kim, S.M., Lee, C.S., et al : Machine learning identified an alzheimer’s disease-related fdg-pet pattern which is also expressed in lewy body dementia and parkinson’s disease dementia. Scientific reports 8 (1), 1–13 (2018)
Gray, K.R., Aljabar, P., Heckemann, R.A., Hammers, A., Rueckert, D.: Random forest-based manifold learning for classification of imaging data in dementia. In: International Workshop on Machine Learning in Medical Imaging, pp. 159–166 (2011). Springer
De Bruijne, M.: Machine learning approaches in medical image analysis: From detection to diagnosis. Elsevier (2016)
Tong, T., Wolz, R., Gao, Q., Hajnal, J.V., Rueckert, D.: Multiple instance learning for classification of dementia in brain mri. In: International Conference on Medical Image Computing and Computer-Assisted Intervention, pp. 599–606 (2013). Springer
Akhila, J., Markose, C., Aneesh, R.: Feature extraction and classification of dementia with neural network. In: 2017 International Conference on Intelligent Computing, Instrumentation and Control Technologies (ICICICT), pp. 1446–1450 (2017). IEEE
Chen, Y., Pham, T.D.: Development of a brain mri-based hidden markov model for dementia recognition. Biomedical engineering online 12 (1), 1–16 (2013)
Patil, M., Yardi, A.: Ann based dementia diagnosis using dct for brain mr image compression. In: 2013 International Conference on Communication and Signal Processing, pp. 451–454 (2013). IEEE
Gulhare, K.K., Shukla, S., Sharma, L.: Deep neural network classification method to alzheimer’s disease detection. International Journals of Advanced Research in Computer Science and Software Engineering 7 (6), 1–4 (2017)
Chiu, P.-Y., Tang, H., Wei, C.-Y., Zhang, C., Hung, G.-U., Zhou, W.: Nmd-12: A new machine-learning derived screening instrument to detect mild cognitive impairment and dementia. PloS one 14 (3), 0213430 (2019)
Stamate, D., Alghamdi, W., Ogg, J., Hoile, R., Murtagh, F.: A machine learning framework for predicting dementia and mild cognitive impairment. In: 2018 17th IEEE International Conference on Machine Learning and Applications (ICMLA), pp. 671–678 (2018). IEEE
Visser, P.J., Lovestone, S., Legido-Quigley, C.: A metabolite-based machine learning approach to diagnose alzheimer-type dementia in blood: Results from the european medical information framework for alzheimer disease biomarker discovery cohort (2019)
Bansal, D., Chhikara, R., Khanna, K., Gupta, P.: Comparative analysis of various machine learning algorithms for detecting dementia. Procedia computer science 132 , 1497–1502 (2018)
Nori, V.S., Hane, C.A., Martin, D.C., Kravetz, A.D., Sanghavi, D.M.: Identifying incident dementia by applying machine learning to a very large administrative claims dataset. PLoS One 14 (7), 0203246 (2019)
Alam, M.A.U., Roy, N., Holmes, S., Gangopadhyay, A., Galik, E.: Automated functional and behavioral health assessment of older adults with dementia. In: 2016 IEEE First International Conference on Connected Health: Applications, Systems and Engineering Technologies (CHASE), pp. 140–149 (2016). IEEE
Gurevich, P., Stuke, H., Kastrup, A., Stuke, H., Hildebrandt, H.: Neuropsychological testing and machine learning distinguish alzheimer’s disease from other causes for cognitive impairment. Frontiers in aging neuroscience 9 , 114 (2017)
Karaglani, M., Gourlia, K., Tsamardinos, I., Chatzaki, E.: Accurate blood-based diagnostic biosignatures for alzheimer’s disease via automated machine learning. Journal of clinical medicine 9 (9), 3016 (2020)
Ryzhikova, E., Ralbovsky, N.M., Sikirzhytski, V., Kazakov, O., Halamkova, L., Quinn, J., Zimmerman, E.A., Lednev, I.K.: Raman spectroscopy and machine learning for biomedical applications: Alzheimer’s disease diagnosis based on the analysis of cerebrospinal fluid. Spectrochimica Acta Part A: Molecular and Biomolecular Spectroscopy 248 , 119188 (2021)
Cho, P.-C., Chen, W.-H.: A double layer dementia diagnosis system using machine learning techniques. In: International Conference on Engineering Applications of Neural Networks, pp. 402–412 (2012). Springer
Facal, D., Valladares-Rodriguez, S., Lojo-Seoane, C., Pereiro, A.X., Anido-Rifon, L., Juncos-Rabadán, O.: Machine learning approaches to studying the role of cognitive reserve in conversion from mild cognitive impairment to dementia. International journal of geriatric psychiatry 34 (7), 941–949 (2019)
Jin, H., Chien, S., Meijer, E., Khobragade, P., Lee, J., et al : Learning from clinical consensus diagnosis in india to facilitate automatic classification of dementia: Machine learning study. JMIR Mental Health 8 (5), 27113 (2021)
James, C., Ranson, J.M., Everson, R., Llewellyn, D.J.: Performance of machine learning algorithms for predicting progression to dementia in memory clinic patients. JAMA network open 4 (12), 2136553–2136553 (2021)
Bougea, A., Efthymiopoulou, E., Spanou, I., Zikos, P.: A novel machine learning algorithm predicts dementia with lewy bodies versus parkinson’s disease dementia based on clinical and neuropsychological scores. Journal of geriatric psychiatry and neurology, 0891988721993556 (2021)
Salem, F.A., Chaaya, M., Ghannam, H., Al Feel, R.E., El Asmar, K.: Regression based machine learning model for dementia diagnosis in a community setting. Alzheimer’s & Dementia 17 , 053839 (2021)
Garcia-Gutierrez, F., Delgado-Alvarez, A., Delgado-Alonso, C., Díaz-Álvarez, J., Pytel, V., Valles-Salgado, M., Gil, M.J., Hernández-Lorenzo, L., Matías-Guiu, J., Ayala, J.L., et al.: Diagnosis of alzheimer’s disease and behavioural variant frontotemporal dementia with machine learning-aided neuropsychological assessment using feature engineering and genetic algorithms. International journal of geriatric psychiatry 37 (2) (2022)
Mirzaei, G., Adeli, H.: Machine learning techniques for diagnosis of alzheimer disease, mild cognitive disorder, and other types of dementia. Biomedical Signal Processing and Control 72 , 103293 (2022)
Hsiu, H., Lin, S.-K., Weng, W.-L., Hung, C.-M., Chang, C.-K., Lee, C.-C., Chen, C.-T.: Discrimination of the cognitive function of community subjects using the arterial pulse spectrum and machine-learning analysis. Sensors 22 (3), 806 (2022)
Shahzad, A., Dadlani, A., Lee, H., Kim, K.: Automated prescreening of mild cognitive impairment using shank-mounted inertial sensors based gait biomarkers. IEEE Access (2022)
Hane, C.A., Nori, V.S., Crown, W.H., Sanghavi, D.M., Bleicher, P.: Predicting onset of dementia using clinical notes and machine learning: case-control study. JMIR medical informatics 8 (6), 17819 (2020)
Aschwanden, D., Aichele, S., Ghisletta, P., Terracciano, A., Kliegel, M., Sutin, A.R., Brown, J., Allemand, M.: Predicting cognitive impairment and dementia: A machine learning approach. Journal of Alzheimer’s Disease 75 (3), 717–728 (2020)
Ryu, S.-E., Shin, D.-H., Chung, K.: Prediction model of dementia risk based on xgboost using derived variable extraction and hyper parameter optimization. IEEE Access 8 , 177708–177720 (2020)
de Langavant, L.C., Bayen, E., Yaffe, K., et al : Unsupervised machine learning to identify high likelihood of dementia in population-based surveys: development and validation study. Journal of medical Internet research 20 (7), 10493 (2018)
Fouladvand, S., Mielke, M.M., Vassilaki, M., Sauver, J.S., Petersen, R.C., Sohn, S.: Deep learning prediction of mild cognitive impairment using electronic health records. In: 2019 IEEE International Conference on Bioinformatics and Biomedicine (BIBM), pp. 799–806 (2019). IEEE
Balea-Fernandez, F.J., Martinez-Vega, B., Ortega, S., Fabelo, H., Leon, R., Callico, G.M., Bibao-Sieyro, C.: Analysis of risk factors in dementia through machine learning. Journal of Alzheimer’s Disease 79 (2), 845–861 (2021)
Chlasta, K., Wołk, K.: Towards computer-based automated screening of dementia through spontaneous speech. Frontiers in Psychology, 4091 (2021)
Chien, Y.-W., Hong, S.-Y., Cheah, W.-T., Yao, L.-H., Chang, Y.-L., Fu, L.-C.: An automatic assessment system for alzheimer’s disease based on speech using feature sequence generator and recurrent neural network. Scientific Reports 9 (1), 1–10 (2019)
Shimoda, A., Li, Y., Hayashi, H., Kondo, N.: Dementia risks identified by vocal features via telephone conversations: A novel machine learning prediction model. PloS one 16 (7), 0253988 (2021)
Nishikawa, K., Akihiro, K., Hirakawa, R., Kawano, H., Nakatoh, Y.: Machine learning model for discrimination of mild dementia patients using acoustic features. Cognitive Robotics (2021)
Liu, L., Zhao, S., Chen, H., Wang, A.: A new machine learning method for identifying alzheimer’s disease. Simulation Modelling Practice and Theory 99 , 102023 (2020)
Searle, T., Ibrahim, Z., Dobson, R.: Comparing natural language processing techniques for alzheimer’s dementia prediction in spontaneous speech. arXiv preprint arXiv:2006.07358 (2020)
Zhu, Y., Tran, B., Liang, X., Batsis, J.A., Roth, R.M.: Towards interpretability of speech pause in dementia detection using adversarial learning. arXiv preprint arXiv:2111.07454 (2021)
Ossewaarde, R., Jonkers, R., Jalvingh, F., Bastiaanse, R.: Classification of spontaneous speech of individuals with dementia based on automatic prosody analysis using support vector machines (svm). In: The Thirty-Second International Flairs Conference (2019)
Xue, C., Karjadi, C., Paschalidis, I.C., Au, R., Kolachalama, V.B.: Detection of dementia on voice recordings using deep learning: a framingham heart study. Alzheimer’s research & therapy 13 (1), 1–15 (2021)
Weiner, J., Engelbart, M., Schultz, T.: Manual and automatic transcriptions in dementia detection from speech. In: INTERSPEECH, pp. 3117–3121 (2017)
Sadeghian, R., Schaffer, J.D., Zahorian, S.A.: Speech processing approach for diagnosing dementia in an early stage (2017)
Khodabakhsh, A., Yesil, F., Guner, E., Demiroglu, C.: Evaluation of linguistic and prosodic features for detection of alzheimer’s disease in turkish conversational speech. EURASIP Journal on Audio, Speech, and Music Processing 2015 (1), 1–15 (2015)
Edwards, E., Dognin, C., Bollepalli, B., Singh, M.K., Analytics, V.: Multiscale system for alzheimer’s dementia recognition through spontaneous speech. In: INTERSPEECH, pp. 2197–2201 (2020)
Kumar, Y., Maheshwari, P., Joshi, S., Baths, V.: Ml-based analysis to identify speech features relevant in predicting alzheimer’s disease. arXiv preprint arXiv:2110.13023 (2021)
Luz, S., de la Fuente, S., Albert, P.: A method for analysis of patient speech in dialogue for dementia detection. arXiv preprint arXiv:1811.09919 (2018)
Syed, Z.S., Syed, M.S.S., Lech, M., Pirogova, E.: Automated recognition of alzheimer’s dementia using bag-of-deep-features and model ensembling. IEEE Access 9 , 88377–88390 (2021)
Orsulic-Jeras, S., Sanders, B., Powers, S., Ejaz, F., Cordell, A., Wilk, C.: Developing a reminiscence therapy platform-lifebio memorytm-with a novel machine-learning-based application that transfers speech to text and generates life stories for nursing home residents with dementia. Alzheimer’s & Dementia 17 , 052281 (2021)
Sarawgi, U., Zulfikar, W., Soliman, N., Maes, P.: Multimodal inductive transfer learning for detection of alzheimer’s dementia and its severity. arXiv preprint arXiv:2009.00700 (2020)
Calzà, L., Gagliardi, G., Favretti, R.R., Tamburini, F.: Linguistic features and automatic classifiers for identifying mild cognitive impairment and dementia. Computer Speech & Language 65 , 101113 (2021)
Haider, F., De La Fuente, S., Luz, S.: An assessment of paralinguistic acoustic features for detection of alzheimer’s dementia in spontaneous speech. IEEE Journal of Selected Topics in Signal Processing 14 (2), 272–281 (2019)
Lopez-de-Ipiña, K., Alonso, J.B., Solé-Casals, J., Barroso, N., Henriquez, P., Faundez-Zanuy, M., Travieso, C.M., Ecay-Torres, M., Martinez-Lage, P., Eguiraun, H.: On automatic diagnosis of alzheimer’s disease based on spontaneous speech analysis and emotional temperature. Cognitive Computation 7 (1), 44–55 (2015)
Orimaye, S.O., Wong, J.S.-M., Golden, K.J.: Learning predictive linguistic features for alzheimer’s disease and related dementias using verbal utterances. In: Proceedings of the Workshop on Computational Linguistics and Clinical Psychology: From Linguistic Signal to Clinical Reality, pp. 78–87 (2014)
Santander-Cruz, Y., Salazar-Colores, S., Paredes-García, W.J., Guendulain-Arenas, H., Tovar-Arriaga, S.: Semantic feature extraction using sbert for dementia detection. Brain Sciences 12 (2), 270 (2022)
Download references
Acknowledgements
The first author’s learning process was supported by the National E-Infrastructure for Aging Research (NEAR), Sweden. NEAR is working on improving the health condition of older adults in Sweden.
Open access funding provided by Blekinge Institute of Technology. This research received no external funding.
Author information
Ashir Javeed and Ana Luiza Dallora contributed equally to this work.
Authors and Affiliations
Aging Research Center, Karolinska Institutet, Tomtebodavagen, Stockholm, 17165, Solna, Sweden
Ashir Javeed
Department of Health, Blekinge Institute of Technology, Valhallavägen 1, Karlskrona, 37141, Blekinge, Sweden
Ashir Javeed, Ana Luiza Dallora, Johan Sanmartin Berglund & Peter Anderberg
Department of Computer Science, University of Science and Technology Bannu, Township, Bannu, 28100, Khyber-Pakhtunkhwa, Pakistan
Department of Electrical Engineering, University of Science and Technology Bannu, Township, Bannu, 28100, Khyber-Pakhtunkhwa, Pakistan
Liaqata Ali
School of Health Sciences, University of Skovde, Högskolevägen 1, Skövde, SE-541 28, Skövde, Sweden
Peter Anderberg
You can also search for this author in PubMed Google Scholar
Contributions
Conceptualization by Peter Anderber, Data curation by Liaqat ALi, Formal analysis by Ana Luiza Dallora, Write up and Methodology by Ashir Javeed, Proofread by Arif Ali, Supervised by Johan Sanmartin Berglund. If any of the sections are not relevant to your manuscript.
Corresponding author
Correspondence to Johan Sanmartin Berglund .
Ethics declarations
Ethics approval.
This study was carried out in accordance with the Declaration of Helsinki and was approved by the Research Ethics Committee at Blekinge Institute of Technology (BTH).
Consent to Participate
Consent for publication, conflict of interest.
The authors declare no conflict of interest.
Additional information
Publisher’s note.
Springer Nature remains neutral with regard to jurisdictional claims in published maps and institutional affiliations.
Rights and permissions
Open Access This article is licensed under a Creative Commons Attribution 4.0 International License, which permits use, sharing, adaptation, distribution and reproduction in any medium or format, as long as you give appropriate credit to the original author(s) and the source, provide a link to the Creative Commons licence, and indicate if changes were made. The images or other third party material in this article are included in the article's Creative Commons licence, unless indicated otherwise in a credit line to the material. If material is not included in the article's Creative Commons licence and your intended use is not permitted by statutory regulation or exceeds the permitted use, you will need to obtain permission directly from the copyright holder. To view a copy of this licence, visit http://creativecommons.org/licenses/by/4.0/ .
Reprints and permissions
About this article
Javeed, A., Dallora, A.L., Berglund, J.S. et al. Machine Learning for Dementia Prediction: A Systematic Review and Future Research Directions. J Med Syst 47 , 17 (2023). https://doi.org/10.1007/s10916-023-01906-7
Download citation
Received : 16 July 2022
Accepted : 03 January 2023
Published : 01 February 2023
DOI : https://doi.org/10.1007/s10916-023-01906-7
Share this article
Anyone you share the following link with will be able to read this content:
Sorry, a shareable link is not currently available for this article.
Provided by the Springer Nature SharedIt content-sharing initiative
- Dementia prediction
- Feature selection
- Machine learning
- Deep learning
- Find a journal
- Publish with us
- Track your research
Thank you for visiting nature.com. You are using a browser version with limited support for CSS. To obtain the best experience, we recommend you use a more up to date browser (or turn off compatibility mode in Internet Explorer). In the meantime, to ensure continued support, we are displaying the site without styles and JavaScript.
- View all journals
- My Account Login
- Explore content
- About the journal
- Publish with us
- Sign up for alerts
- Open access
- Published: 20 June 2022
Multimodal deep learning for Alzheimer’s disease dementia assessment
- Shangran Qiu 1 , 2 na1 ,
- Matthew I. Miller 1 na1 ,
- Prajakta S. Joshi 3 , 4 , 5 ,
- Joyce C. Lee 1 ,
- Chonghua Xue 1 , 3 ,
- Yunruo Ni 1 ,
- Yuwei Wang 1 ,
- Ileana De Anda-Duran ORCID: orcid.org/0000-0003-3629-9465 6 ,
- Phillip H. Hwang ORCID: orcid.org/0000-0001-6780-6808 3 ,
- Justin A. Cramer 7 ,
- Brigid C. Dwyer 8 ,
- Honglin Hao 9 ,
- Michelle C. Kaku 8 ,
- Sachin Kedar 10 , 11 , 12 ,
- Peter H. Lee 13 ,
- Asim Z. Mian 14 ,
- Daniel L. Murman 10 ,
- Sarah O’Shea 8 ,
- Aaron B. Paul 13 ,
- Marie-Helene Saint-Hilaire 8 ,
- E. Alton Sartor 8 ,
- Aneeta R. Saxena 8 ,
- Ludy C. Shih ORCID: orcid.org/0000-0002-6590-8365 8 ,
- Juan E. Small ORCID: orcid.org/0000-0002-4931-3564 13 ,
- Maximilian J. Smith 13 ,
- Arun Swaminathan 10 ,
- Courtney E. Takahashi 8 ,
- Olga Taraschenko 10 ,
- Hui You 15 ,
- Jing Yuan ORCID: orcid.org/0000-0002-3923-925X 9 ,
- Yan Zhou 9 ,
- Shuhan Zhu 8 ,
- Michael L. Alosco 8 , 16 ,
- Jesse Mez 5 , 8 , 16 ,
- Thor D. Stein 16 , 17 , 18 , 19 ,
- Kathleen L. Poston ORCID: orcid.org/0000-0003-3424-7143 20 ,
- Rhoda Au 3 , 5 , 8 , 16 , 21 &
- Vijaya B. Kolachalama ORCID: orcid.org/0000-0002-5312-8644 1 , 16 , 22 , 23
Nature Communications volume 13 , Article number: 3404 ( 2022 ) Cite this article
37k Accesses
65 Citations
103 Altmetric
Metrics details
- Alzheimer's disease
- Translational research
Worldwide, there are nearly 10 million new cases of dementia annually, of which Alzheimer’s disease (AD) is the most common. New measures are needed to improve the diagnosis of individuals with cognitive impairment due to various etiologies. Here, we report a deep learning framework that accomplishes multiple diagnostic steps in successive fashion to identify persons with normal cognition (NC), mild cognitive impairment (MCI), AD, and non-AD dementias (nADD). We demonstrate a range of models capable of accepting flexible combinations of routinely collected clinical information, including demographics, medical history, neuropsychological testing, neuroimaging, and functional assessments. We then show that these frameworks compare favorably with the diagnostic accuracy of practicing neurologists and neuroradiologists. Lastly, we apply interpretability methods in computer vision to show that disease-specific patterns detected by our models track distinct patterns of degenerative changes throughout the brain and correspond closely with the presence of neuropathological lesions on autopsy. Our work demonstrates methodologies for validating computational predictions with established standards of medical diagnosis.
Similar content being viewed by others
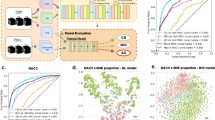
Generalizable deep learning model for early Alzheimer’s disease detection from structural MRIs
Sheng Liu, Arjun V. Masurkar, … Narges Razavian
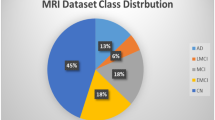
A novel CNN architecture for accurate early detection and classification of Alzheimer’s disease using MRI data
A. M. El-Assy, Hanan M. Amer, … M. A. Mohamed
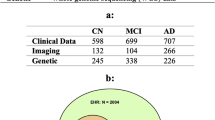
Multimodal deep learning models for early detection of Alzheimer’s disease stage
Janani Venugopalan, Li Tong, … May D. Wang
Introduction
Alzheimer’s disease (AD) is the most common cause of dementia worldwide 1 , and future expansions in caseload due to an aging population are likely to accentuate existing needs for health services 2 . This increase in clinical demand will likely contribute to an already considerable burden of morbidity and mortality among the elderly 3 , thus requiring improvements in the treatment and timely identification of AD. Significant efforts have been made in recent years towards the development of cerebrospinal fluid (CSF) biomarkers 4 , as well as advanced imaging modalities such as amyloid and tau positron emission tomography (PET) 5 , 6 , 7 , 8 . Furthermore, novel generations of disease-modifying therapies for AD are now coming into clinical purview 9 , though their efficacy remains controversial. Despite this progress, many emerging diagnostic and treatment modalities remain limited to research contexts, and the backbone of antemortem diagnosis remains traditional clinical assessment, neuropsychological testing 10 , and magnetic resonance imaging (MRI) 11 . Mild cognitive impairment (MCI), a prodromal stage of dementia, may also be a subtle early presentation of AD whose diagnosis similarly requires significant clinical acumen from qualified specialists. Complicating matters is the presence of a multitude of other non-Alzheimer’s disease dementia (nADD) syndromes whose clinical presentations often overlap with AD. Thus, common causes of dementias outside of AD such as vascular dementia (VD), Lewy body dementia (LBD), and frontotemporal dementia (FTD) widen the differential diagnosis of neurodegenerative conditions and contribute to variability in diagnostic sensitivity and specificity 12 .
Reliably differentiating between normal cognitive aging, MCI, AD, and other dementia etiologies requires significant clinical acumen from qualified specialists treating memory disorders, yet timely access to memory clinics is often limited for patients and families. This is a major problem in remote, rural regions within developed countries and in still economically developing nations, where there is a dearth of specialized practitioners. Furthermore, the need for skilled clinicians is rising, yet the United States is facing a projected shortage of qualified clinicians, such as neurologists, in coming decades 13 , 14 . As increasing clinical demand intersects with a diminishing supply of medical expertise, machine learning methods for aiding neurologic diagnoses have begun to attract interest 15 . Complementing the high diagnostic accuracy reported by other groups 16 , we have previously reported interpretable deep learning approaches capable of distinguishing participants with age-appropriate normal cognition (NC) from those with AD using magnetic resonance imaging (MRI) scans, age, sex, and mini-mental state examination (MMSE) 17 . Others have also demonstrated the efficacy of deep learning in discriminating AD from specific types of nADD 18 , 19 , 20 . However, clinical evaluation of persons presenting in a memory clinic involves consideration of multiple etiologies of cognitive impairment. Therefore, the ability to successfully differentiate between NC, MCI, AD, and nADD across diverse study cohorts in a unified framework remains to be developed.
In this study, we report the development and validation of a deep learning framework capable of accurately classifying subjects with NC, MCI, AD, and nADD in multiple cohorts of participants with different etiologies of dementia and varying levels of cognitive function (Table 1 , Fig. 1 ). Using data from the National Alzheimer’s Coordinating Center (NACC) 21 , 22 , we developed and externally validated models capable of classifying cognitive status using MRI, non-imaging variables, and combinations thereof. To validate our approach, we demonstrated comparability of our model’s accuracy to the diagnostic performance of a team of practicing neurologists and neuroradiologists. We then leveraged SHapley Additive exPlanations (SHAP) 23 , to link computational predictions with well-known anatomical and pathological markers of neurodegeneration. Our strategy provides evidence that automated methods driven by deep learning may approach clinical standards of accurate diagnosis even amidst heterogeneous datasets.
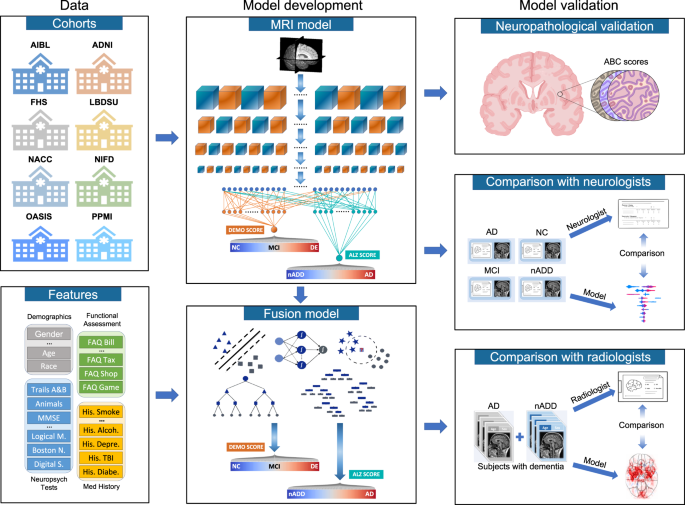
Multimodal data including MRI scans, demographics, medical history, functional assessments, and neuropsychological test results were used to develop deep learning models on various classification tasks. Eight independent datasets were used for this study, including NACC, ADNI, AIBL, FHS, LBDSU, NIFD, OASIS, and PPMI. We selected the NACC dataset to develop three separate models: (i) an MRI-only CNN model (ii) non-imaging models in the form of traditional machine learning classifiers, which did not use any MRI data (iii) a fusion model combining imaging and non-imaging data within a hybrid architecture joining a CNN to a CatBoost model. The MRI-only model was validated across all eight cohorts, whereas external validation of non-imaging and fusion models was performed only on OASIS. First, T1-weighted MRI scans were input to a CNN to calculate a continuous DEmentia MOdel (DEMO) score to assess cognitive status on a 0 to 2 scale, where “0” indicated NC “1” indicated MCI and “2” indicated DE. DEMO scores were converted to class labels using an optimal thresholding algorithm, with these assignments constituting the COG task. For individuals with DE diagnosis, the multi-task CNN model simultaneously discriminated their risk of having AD versus nADD, a classification that we refer to as the ADD task. We denoted the probability of AD diagnosis as the ALZheimer (ALZ) score. Both MRI-derived DEMO scores and ALZ scores were then input alongside non-imaging variables to various machine learning classifiers to form fusion models, which then predicted outcomes on the COG and ADD tasks, respectively. A portion of cases with confirmed dementia ( n = 50) from the NACC testing cohort was randomly selected for direct comparison of the fusion model with an international team of practicing neuroradiologists. Both the model and neuroradiologists completed the ADD task using available MRI scans, age, and gender. Additionally, a portion of NACC cases ( n = 100) was randomly selected to compare the fusion model performance to practicing neurologists, with both the model and clinicians having access to a common set of multimodal data. Lastly, model predictions were compared with neuropathology grades from NACC, ADNI and FHS cohorts ( n = 110).
We divided the process of differential diagnosis into staged tasks. The first, which we refer to as the COG task, labeling persons as having either NC, MCI, or dementia (DE) due to any cause. Of note, the COG task may be seen as comprising three separate binary classification subtasks: (i) COG NC task: Separation of NC and MCI/DE cases (ii) COG MCI task: Separation of MCI from NC/DE cases, and (iii) COG DE task: Separation of DE from NC/MCI cases. After completion of the overall COG task, we next formulated the ADD task, in which we assigned all persons labeled as DE to a diagnosis of either AD or nADD. Successive completion of the COG and the ADD tasks allowed execution of an overarching 4-way classification that fully delineated NC, MCI, AD, and nADD cases (See Supplementary Information : Glossary of Tasks, Models, and Metrics).
We also created three separate models: (i) MRI-only model: A convolutional neural network (CNN) that internally computed a continuous DEmentia MOdel (DEMO) score to complete the COG task, as well as an ALZheimer’s (ALZ) score to complete the ADD task. (ii) Non-imaging model: A traditional machine learning classifier that took as input only scalar-valued clinical variables from demographics, past medical history, neuropsychological testing, and functional assessments. As in the MRI-only model, the non-imaging model also computed the DEMO and the ALZ scores from which the COG and the ADD tasks could be completed. We tested multiple machine learning architectures for these purposes and ultimately selected a CatBoost model as our final non-imaging model architecture. (iii) Fusion model: This framework linked a CNN to a CatBoost model. With this approach, the DEMO and the ALZ scores computed by the CNN were recycled and used alongside available clinical variables. The CatBoost model then recalculated these scores in the context of the additional non-imaging information. We provide definitions of our various prediction tasks, cognitive metrics, and model types within the Supplementary Information . Further details of model design may be found within the Methods.
Assessment for confounding
We used two-dimensional t-distributed stochastic neighbor embedding (tSNE) to assess for the presence of confounding relationships between disease status and certain forms of metadata. Using this approach, we observed no obvious clustering of post-processed MRI embeddings among the eight cohorts used for testing of MRI-only models (Fig. 2a, b ). Within the NACC cohort, we also observed no appreciable clustering based on individual Alzheimer’s Disease Research Centers (ADRCs, Fig. 2c, d ) or scanner manufacturer (Fig. 2e, f ). Relatedly, although tSNE analysis of CNN hidden layer activations did yield clustering of NACC data points (Fig. 2b ), this was an expected phenomenon given the selection of NACC as our cohort for model training. Otherwise, we appreciated no obvious conglomeration of embeddings from hidden layer activations due to specific ADRCs (Fig. 2d ) or scanner manufacturers (Fig. 2f ). Lastly, Mutual Information Scores (MIS) computed from the NACC cohort indicated negligible correlation of diagnostic labels (NC, MCI, AD, and nADD) between specific scanner manufacturers (MIS = 0.010, Fig. 2g ) and ADRCs (MIS = 0.065, Fig. 2h ).
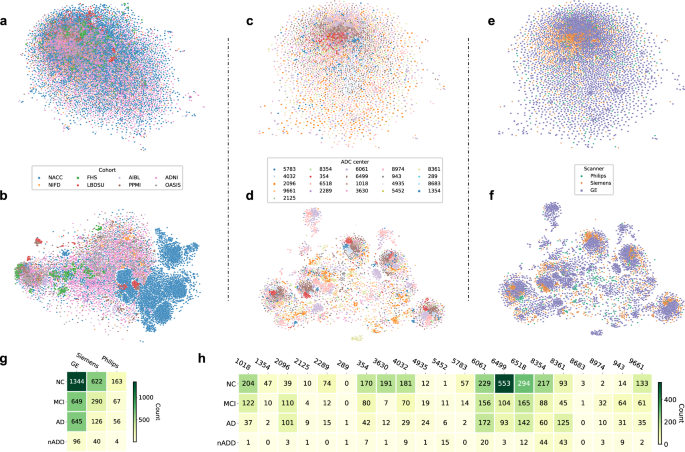
Unsupervised clustering of post-processed MRIs and hidden layer activations assessed for systematic biases in input data and model predictions, respectively. a Two-dimensional (2D) t-distributed stochastic neighbor embedding (tSNE) embeddings of downsampled MRI scans are shown. The downsampling was performed on the post-processed MRI scans using spline interpolation with a downsampling factor of 8 on each axis. Individual points represent MRIs from a single subject and are colored according to their original cohort (either NACC, ADNI, AIBL, FHS, LBDSU, NIFD, OASIS, or PPMI). b We demonstrate 2D tSNEs of hidden-layer activations from the penultimate CNN hidden layer. Individual points correspond to internal representations of MRI scans during testing and are colored by cohort label. c Plot of 2D tSNE embeddings of downsampled MRI scans from the NACC dataset is shown. Individual points representing MRI scans are colored by the unique identifier of one of twenty-one Alzheimer Disease Research Centers (ADRCs) that participate in the NACC collaboration. d tSNE embeddings for penultimate layer activations colored by ADRC ID are shown. e Plot of 2D tSNE embeddings of downsampled MRI scans from the NACC dataset is shown. Embeddings in this plot are the same as those in c but colored according to the manufacturer of the scanner used to acquire each MRI, either General Electric (GE), Siemens, or Philips. f Plot of 2D tSNE of penultimate layer activations is shown for cases in the NACC dataset. Embeddings are equivalent to those visualized in d but are now colored by the manufacturer of the scanner used for image acquisition. g A tabular representation of disease category counts by manufacturer is presented. Only cases from the NACC dataset are included. We provide the Mutual Information Score (MIS) to quantify the correlation between disease type and scanner manufacturer. h We also provided a tabular representation of disease category counts stratified by ADRC ID in the NACC dataset. MIS is once again shown to quantify the degree of correlation between diagnostic labels and individual centers participating in the NACC study. Source data are provided as a Source Data file.
Deep learning model performance
We observed that our fusion model provided the most accurate classification of cognitive status for NC, MCI, AD and nADD across a range of clinical diagnosis tasks (Table 2 ). We found strong model performance on the COG NC task between both the NACC test set (Fig. 3a , Row 1) and an external validation set (OASIS; Fig. 3b , Row 1) as indicated by area under the receiver operating characteristic (AUC) curve values of 0.945 [95% confidence interval (CI): 0.939, 0.951] and 0.959 [CI: 0.955, 0.963], respectively. Similar values for area under precision-recall (AP) curves were also observed, yielding 0.946 [CI: 0.940, 0.952] and 0.969 [CI: 0.964, 0.974], respectively. Such correspondence between AUC and AP performance supports robustness to class imbalance across datasets. In the COG DE task, comparable results were also seen, as the fusion model yielded respective AUC and AP scores of 0.971 [CI: 0.966, 0.976]/0.917 [CI: 0.906, 0.928] (Fig. 3a , Row 2) in the NACC dataset and 0.971 [CI: 0.969, 0.973]/0.959 [CI: 0.957, 0.961] in the OASIS dataset (Fig. 3b , Row 2). Conversely, classification performance dropped slightly for the ADD task, with respective AUC/AP values of 0.773 [CI: 0.712, 0.834]/0.938 [CI: 0.918, 0.958] in the NACC dataset (Fig. 3a , Row 3) and 0.773 [CI: 0.732, 0.814]/0.965 [CI: 0.956, 0.974] in the OASIS dataset (Fig. 3b , Row 3).
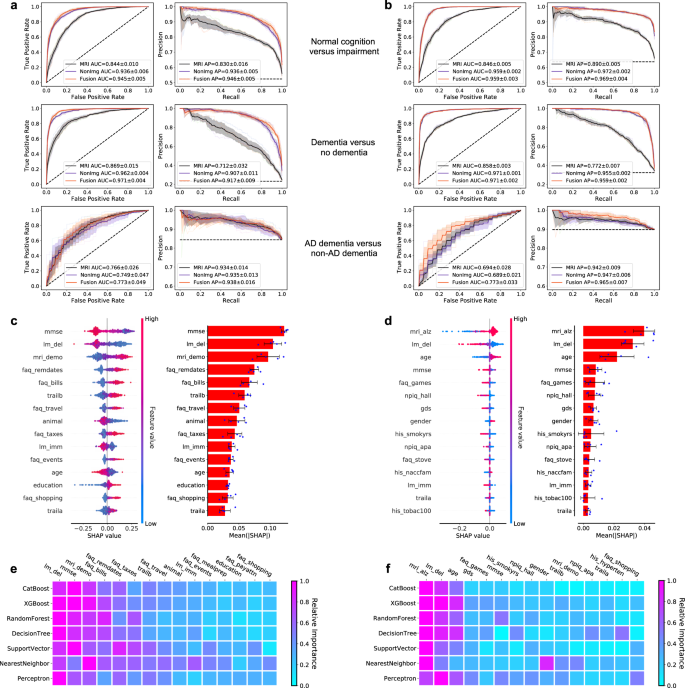
a , b ROC curves showing true positive rate versus false positive rate and PR curves showing the positive predictive value versus sensitivity on the a NACC test set and b OASIS dataset. The first row in a and b denotes the performance of the MRI-only model, the non-imaging model, and the fusion model (CNN + CatBoost) trained to classify cases with NC from those without NC (COG NC task). The second row shows ROC and PR curves of the MRI-only model, the non-imaging model, and the fusion model for the COG DE task aimed at distinguishing cases with DE from those who do not have DE. The third row illustrates performance of the MRI-only model, the non-imaging model, and the fusion model focused on discriminating AD from nADD. For each curve, mean AUC was computed. In each plot, the mean ROC/PR curve and standard deviation are shown as bolded lines and shaded regions, respectively. The dotted lines in each plot indicate the classifier with the random performance level. c , d Fifteen features with highest mean absolute SHAP values from the fusion model are shown for the COG and ADD tasks, respectively across cross-validation rounds ( n = 5). Error bars overlaid on bar plots are centered at the mean of the data and extend + /− one standard deviation. For each task, the MRI scans, demographic information, medical history, functional assessments, and neuropsychological test results were used as inputs to the deep learning model. The left plots in c and d illustrate the distribution of SHAP values and the right plots show the mean absolute SHAP values. All the plots in c and d are organized in decreasing order of mean absolute SHAP values. e , f For comparison, we also constructed traditional machine learning models to predict cognitive status and AD status using the same set of features used for the deep learning model, and the results are presented in e and f , respectively. The heat maps show fifteen features with the highest mean absolute SHAP values obtained for each model. Source data are provided as a Source Data file.
Relative to the fusion model, we observed moderate performance reductions across classifications in our MRI-only model. For the COG NC task, the MRI-only framework yielded AUC and AP scores of 0.844 [CI: 0.832, 0.856]/0.830 [CI: 0.810, 0.850] (NACC) and 0.846 [CI: 0.840, 0.852]/0.890 [CI: 0.884, 0.896] (OASIS). Model results were comparable on the COG DE task, in which the MRI-only model achieved respective AUC and AP scores of 0.869 [CI: 0.850, 0.888]/0.712 [CI: 0.672, 0.752] (NACC) and 0.858 [CI: 0.854, 0.862]/0.772 [CI: 0.763, 0.781] (OASIS). For the ADD task as well, the results of the MRI-only model were approximately on par with those of the fusion model, giving respective AUC and AP scores of 0.766 [CI: 0.734, 0.798]/0.934 [CI: 0.917, 0.951] (NACC) and 0.694 [CI: 0.659,0.729]/0.942 [CI: 0.931, 0.953] (OASIS). For both fusion and MRI-only models, we also reported ROC and PR curves for the ADD task stratified by nADD subtypes in the Supplementary Information (Figs. S1 and S2 ).
Interestingly, we note that a non-imaging model generally yielded similar results to those of both the fusion and MRI-only models. Specifically, a CatBoost model trained for the COG NC task gave AUC and AP values 0.936 [CI: 0.929, 0.943] /0.936 [CI: 0.930, 0.942] (NACC), as well as 0.959 [CI: 0.957, 0.961]/0.972 [CI: 0.970, 0.974] (OASIS). Results remained strong for the COG DE task, with AUC/PR pairs of 0.962 [CI: 0.957, 0.967]/0.907 [0.893, 0.921] (NACC) and 0.971 [CI: 0.970, 0.972]/0.955 [CI: 0.953, 0.957] (OASIS). For the ADD task, the non-imaging model resulted in respective AUC/PR scores of 0.749 [CI: 0.691, 0.807]/0.935 [CI: 0.919, 0.951] (NACC) and 0.689 [CI: 0.663, 0.715]/0.947 [CI: 0.940, 0.954] (OASIS). A full survey of model performance metrics across all classification tasks may be found in the Supplementary Information (Tables S1 – S4 ). Performance of the MRI-only model across all external datasets is demonstrated via ROC and PR curves (Fig. S3 ).
To assess the contribution of various imaging and non-imaging features to classification outcomes, we calculated fifteen features with highest mean absolute SHAP values for the COG (Fig. 3c ) and the ADD prediction tasks using the fusion model (Fig. 3d ). Though MMSE score was the primary discriminative feature for the COG task, the DEMO score derived from the CNN portion of the model ranked third in predicting cognitive status. Analogously, the ALZ score derived from the CNN was the most salient feature in solving the ADD task. Interestingly, the relative importance of features remained largely unchanged when a variety of other machine learning classifiers were substituted to the fusion model in lieu of the CatBoost model (Fig. 3e, f ). This consistency indicated that our prediction framework was robust to the specific choice of model architecture, and instead relied on a consistent set of clinical features to achieve discrimination between NC, MCI, AD, and nADD classes. Relatedly, we also observed that non-imaging and fusion models retained predictive performance across a variety of input feature combinations, showing flexibility to operate across differences in information availability. Importantly, however, the addition of MRI-derived DEMO and ALZ scores improved 4-way classification performance across all combinations of non-imaging variables (Figs. S4 and S5 ).
Neuroimaging signatures of AD and non-AD dementia
The provenance of model predictions was visualized by pixel-wise SHAP mapping of hidden layers within the CNN model. The SHAP matrices were then correlated to physical locations within each subject’s MRI to visualize conspicuous brain regions implicated in each stage of cognitive decline from NC to dementia (Fig. 4a ). This approach allowed neuroanatomical risk mapping to distinguish regions associated with AD from those with nADD (Fig. 4b ). Indeed, the direct overlay of color maps representing disease risk on an anatomical atlas derived from traditional MRI scans facilitates interpretability of the deep learning model. Also, the uniqueness of the SHAP-derived representation allows us to observe disease suggestive regions that are specific to each outcome of interest (Table S5 and Fig. S6 ).
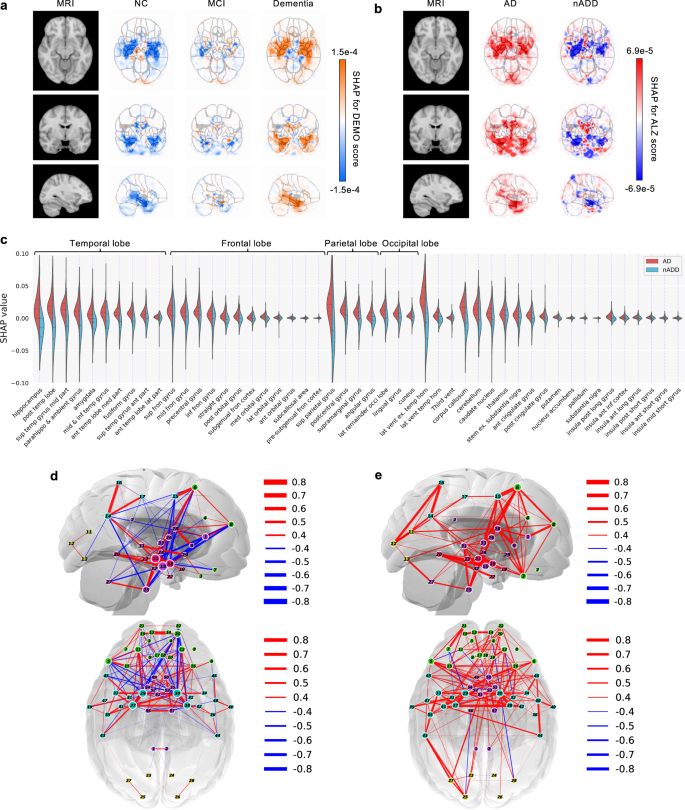
a , b SHAP value-based illustration of brain regions that are most associated with the outcomes. The first columns in both a and b show a template MRI oriented in axial, coronal, and sagittal planes. In a , the second, third and fourth columns show SHAP values from the input features of the second convolutional block of the CNN averaged across all NACC test subjects with NC, MCI, and dementia, respectively. In b , the second and third columns show SHAP values averaged across all NACC test subjects with AD and nADD, respectively. c Brain region-specific SHAP values for both AD and nADD cases obtained from the NACC testing data are shown. The violin plots are organized per lobe and in decreasing order of mean absolute SHAP values. d , e Network of brain regions implicated in the classification of AD and nADD, respectively. We selected 33 representative brain regions for graph analysis and visualization of sagittal regions, as well as 57 regions for axial analyses. Nodes representing brain regions are overlaid on a two-dimensional brain template and sized according to weighted degree. The color of the segments connecting different nodes indicates the sign of correlation and the thickness of the segments indicates the magnitude of the correlation. It must be noted that not all nodes can be seen either from the sagittal or the axial planes. Source data are provided as a Source Data file.
A key feature of SHAP is that a single voxel or a sub-region within the brain can contribute to accurate prediction of one or more class labels. For example, the SHAP values were negative in the hippocampal region in NC participants, but they were positive in participants with dementia, underscoring the well-recognized role of the hippocampus in memory function. Furthermore, positive SHAP values were observed within the hippocampal region for AD and negative SHAP values for the nADD cases, indicating that hippocampal atrophy has direct proportionality with AD-related etiology. The SHAP values sorted according to their importance on the parcellated brain regions also further confirm the role of hippocampus and its relationship with dementia prediction, particularly in the setting of AD (Fig. 4c ), as well as nADD cases (Fig. S7 ). In the case of nADD, the role of other brain regions such as the lateral ventricles and frontal lobes was also evident. Evidently, SHAP-based network analysis revealed pairwise relationships between brain regions that simultaneously contribute to patterns indicative of AD (Fig. 4d ). The set of brain networks evinced by this analysis also demonstrate marked differences in structural changes between AD and nADD (Fig. 4e ).
Neuropathologic validation
In addition to mapping hidden layer SHAP values to original neuroimaging, correlation of deep learning predictions with neuropathology data provided further validation of our modeling approach. Qualitatively, we observed that areas of high SHAP scores for the COG task correlated with region-specific neuropathological scores obtained from autopsy (Fig. 5a ). Similarly, the severity of regional neuropathologic changes in these persons demonstrated a moderate to high degree of concordance with the regional cognitive risk scores derived from our CNN using the Spearman’s rank correlation test. Of note, the strongest correlations appeared to occur within areas affected by AD pathology such as the temporal lobe, amygdala, hippocampus, and parahippocampal gyrus (Fig. 5b ). Using the one-way ANOVA test, we also rejected a null hypothesis of there being no significant differences in DEMO scores between semi-quantitative neuropathological score groups (0–3) with a confidence level of 0.95, including for the global ABC severity scores of Thal phase for Aβ (A score F-test: F(3, 51) = 3.665, p = 1.813e-2), Braak & Braak for neurofibrillary tangles (NFTs) (B score F-test: F(3, 102) = 11.528, p = 1.432e-6), and CERAD neuritic plaque scores (C score F-test: F(3, 103) = 4.924, p = 3.088e-3) (Fig. 5c ). We further performed post hoc testing using Tukey’s procedure to compare pairwise group means of DEMO scores, observing consistently significant differences between individuals with the highest and lowest burdens of neurodegenerative findings, respectively (Fig. S8 ). Of note, we also observed an increasing trend of ALZ score with the semi-quantitative neuropathological scores (Fig. 5d ).
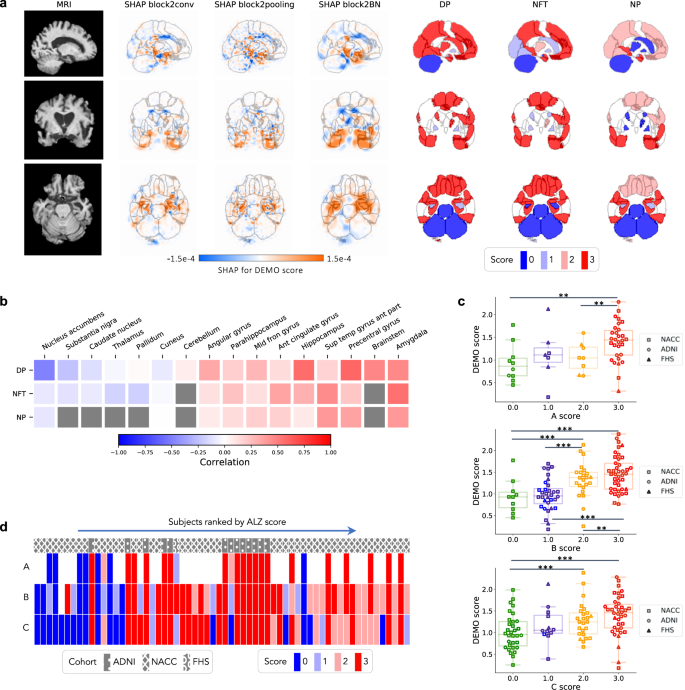
We correlated model findings with regional ABC scores of neuropathologic severity obtained autopsied participants in NACC, ADNI, and FHS cohorts ( n = 110). a An example case from the Alzheimer’s Disease Neuroimaging Initiative (ADNI) dataset is displayed in sagittal, axial, and coronal views. The SHAP values derived from the second convolutional block and neuropathologic ABC scores are mapped to brain regions where they were measured at the time of autopsy. Visually, high concordance is observed between anatomically mapped SHAP values regardless of the hidden layer from which they are derived. Concordance is observed between the SHAP values and neurofibrillary tangles (NFT) scores within the temporal lobe. b A heatmap is shown demonstrating Spearman correlations between population-averaged SHAP values from the input features of the second convolutional layer and stain-specific ABC scores at various regions of the brain. A strong positive correlation is observed between the SHAP values and neuropathologic changes within several areas well-known to be affected in AD such as the hippocampus/parahippocampus, amygdala and temporal gyrus. c Beeswarm plots with overlying box-and-whisker diagrams are shown to denote the distribution of ABC system sub-scores (horizontal axis) versus model-predicted cognitive scores (vertical axis). The displayed data points represent a pooled set of participants from ADNI, NACC, and FHS for whom neuropathology reports were available from autopsy. Each symbol represents a study participant, boxes are centered at the median and extend over the interquartile range (IQR), while bottom and top whiskers represent 1st and 3rd quartiles −/+ 1.5 x IQR, respectively. We denote p < 0.05 as *; p < 0.001 as **, and p < 0.0001 as *** based on post-hoc Tukey testing. d A heatmap demonstrating the distribution of neuropathology scores versus model predicted AD probabilities. Herein, each column within the map represents a unique individual whose position along the horizontal axis is a descending function of AD risk according to the deep learning model. The overlying hatching pattern represents the dataset (ADNI, NACC, and FHS), from which everyone is drawn. Source data are provided as a Source Data file.
Expert-level validation
Lastly, to provide clinical benchmarking of our modeling approach, both neurologists and neuroradiologists were recruited to perform diagnostic tasks on a subset of NACC cases. The approach and performance of the neurologists and the neuroradiologists indicated variability across different clinical practices (See Supplementary Information : Neurologist and Neuroradiologist Accounts), with a moderate inter-rater agreement as evaluated using pairwise kappa (κ) scoring for all the tasks. Among neurologists specifically, we observed average κ = 0.600 for the COG NC task (Fig. 6a , Row 1) and average κ = 0.601 for the COG DE task (Fig. 6a , Row 2). Among neuroradiologists performing the ADD task, we found average κ = 0.292 (Fig. 6b ). In the overall 4-way classification of NC, MCI, AD, and nADD, we observed that the accuracy of our fusion model (mean: 0.558, 95% CI: [0.482,0.634]) reached that of neurologists (mean: 0.565, 95% CI: [0.529,0.601]). Interestingly, a similar level of 4-way accuracy was achieved by a non-imaging CatBoost model (mean: 0.544, 95% CI: [0.517,0.571]), though not on an MRI-only model (mean: 0.412, 95% CI: [0.380,0.444]). However, an MRI-only model did yield a moderate improvement in diagnostic accuracy (mean: 0.692, 95% CI: [0.649,0.735]) over neuroradiologists (mean: 0.566, 95% CI: [0.516,0.616]) in the ADD task (Fig. 6b ). Full performance metrics (including accuracy, sensitivity, specificity, F-1 score, and Matthews Correlation Coefficient) may be found in Tables S6 and S7 for respective comparison of machine learning models to neurologists and neuroradiologists in diagnostic simulations. Performance metrics for simple thresholding of various neuropsychologic test scores can be found in Table S8 . We also sought to correlate region-specific SHAP values with structural changes observed by the neuroradiologists throughout the brain, with particular attention towards limbic and temporal lobe structures. Statistically significant correlations between regional SHAP averages and clinically graded atrophy severity suggested a connection between CNN features and widely known markers of dementia (Fig. 6c ).
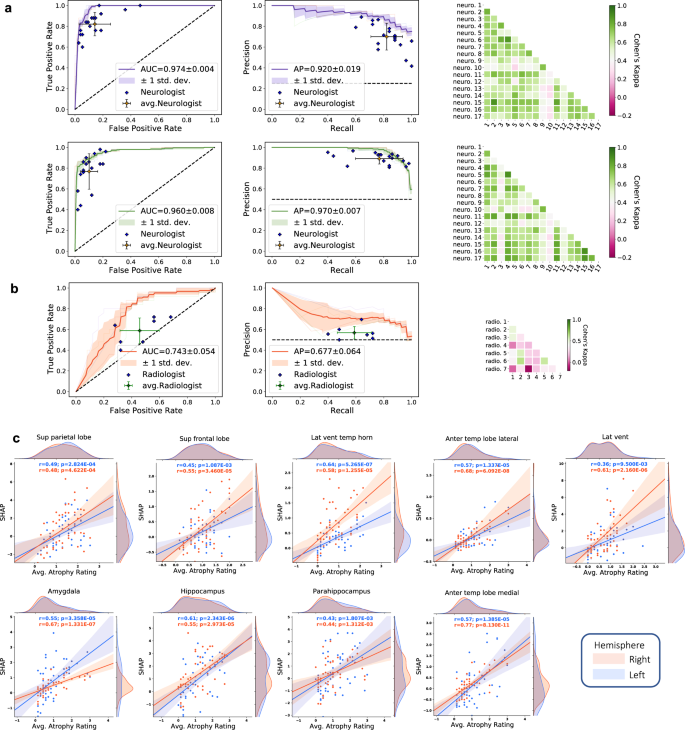
a For the COG NC task (Row 1), the diagnostic accuracy of board-certified neurologists ( n = 17) is compared to the performance of our deep learning model using a random subset of cases from the NACC dataset ( n = 100). Metrics from individual clinicians are plotted in relation to the ROC and PR curves from the trained model. Individual clinician performance is indicated by the blue plus symbol and averaged clinician performance along with error bars is indicated by the green plus symbol on both the ROC and PR curves. The mean ROC/PR curve and the standard deviation are shown as the bold line and shaded region, respectively. A heatmap of pairwise Cohen’s kappa statistic is also displayed to demonstrate inter-rater agreement across the clinician cohort. For the COG DE task (Row 2), ROC, PR, and interrater agreement graphics are illustrated with comparison to board-certified neurologists in identical fashion. For these tasks, all neurologists were granted access to multimodal patient data, including MRIs, demographics, medical history, functional assessments, and neuropsychological testing. The same data was used as input to train the deep learning model. b For validation of our ADD task, a random subset ( n = 50) of cases with dementia from the NACC cohort was provided to the team of neuroradiologists ( n = 7), who classified AD versus those with dementia due to other etiologies (nADD). As above, the diagnostic accuracy of the physician cohort is compared to model performance using ROC and PR curves. Graphical conventions for visualizing model and clinician performance are as described above in a and, once more, pairwise Cohen’s kappa values are shown to demonstrate inter-rater agreement. c SHAP values from the second convolutional layer averaged from selected brain regions are shown plotted against atrophy scores assigned by neuroradiologists. Orange and blue points (and along with regression lines and 95% confidence intervals) represent left and right hemispheres, respectively. Spearman correlation coefficients and corresponding two-tailed p values are also shown and demonstrate a statistically significant proportionality between SHAP scores, and the severity of regional atrophy assigned by clinicians. Source data are provided as a Source Data file.
In this work, we presented a range of machine learning models that can process multimodal clinical data to accurately perform a differential diagnosis of AD. These frameworks can achieve multiple diagnostic steps in succession, first delineating persons based on overall cognitive status (NC, MCI, and DE) and then separating likely cases of AD from those with nADD. Importantly, our models are capable of functioning with flexible combinations of imaging and non-imaging data, and their performance generalized well across multiple datasets featuring a diverse range of cognitive statuses and dementia subtypes.
Our fusion model demonstrated the highest overall classification accuracy across diagnostic tasks, achieving results on par with neurologists recruited from multiple institutions to complete clinical simulations. Notably, similar levels of performance were observed both in the NACC testing set, and in the OASIS external validation set. Our MRI-only model also surpassed the average diagnostic accuracy of practicing neuroradiologists and maintained a similar level of performance in 6 additional external cohorts (ADNI, AIBL, FHS, NIFD, PPMI, and LBDSU), thereby suggesting that diagnostic capability was not biased to any single data source. It is also worth noting that the DEMO and the ALZ scores bore strong analytic importance like that of traditional information used for dementia diagnosis. For instance, in the ADD task, the ALZ score was shown by SHAP analysis to have a greater impact in accurately predicting disease status than key demographic and neuropsychological test variables used in standard clinical practice such as age, sex, and MMSE score. These CNN-derived scores maintained equal levels of importance when used in other machine learning classifiers, suggesting wide utility for digital health workflows.
Furthermore, post-hoc analyses demonstrated that the performance of our machine learning models was grounded in well-established patterns of dementia-related neurodegeneration. Network analyses evinced differing regional distributions of SHAP values between AD and nADD populations, which were most pronounced in areas such as the hippocampus, amygdala, and temporal lobes. The SHAP values in these regions also exhibited a strong correlation with atrophy ratings from neuroradiologists. Although recent work has shown that explainable machine learning methods may identify spurious correlations in imaging data 24 , we feel that our ability to link regional SHAP distributions to both anatomic atrophy and also semi-quantitative scores of Aβ amyloid, neurofibrillary tangles, and neuritic plaques links our modeling results to a gold standard of postmortem diagnosis. More generally, our approach demonstrates a means by which to assimilate deep learning methodologies with validated clinical evidence in health care.
Our work builds on prior efforts to construct automated systems for the diagnosis of dementia. Previously, we developed and externally validated an interpretable deep learning approach to classify AD using multimodal inputs of MRI and clinical variables 17 . Although this approach provided a novel framework, it relied on a contrived scenario of discriminating individuals into binary outcomes, which simplified the complexity of a real-world setting. Our current work extends this framework by mimicking a memory clinic setting and accounting for cases along the entire cognitive spectrum. Though numerous groups have taken on the challenge of nADD diagnosis using deep learning 18 , 19 , 20 , 25 , 26 , even these tasks were constructed as simple binary classifications between disease subtypes. Given that the practice of medicine rarely reduces to a choice between two pathologies, integrated models with the capability to replicate the differential diagnosis process of experts more fully are needed before deep learning models can be touted as assistive tools for clinical-decision support. Our results demonstrate a strategy for expanding the scope of diagnostic tasks using deep learning, while also ensuring that the predictions of automated systems remain grounded in established medical knowledge.
Interestingly, it should be noted that the performance of a non-imaging model alone approached that of the fusion model. However, the inclusion of neuroimaging data was critical to enable verification of our modeling results by clinical criteria (e.g., cross-correlation with post-mortem neuropathology reports). Such confirmatory data sources cannot be readily assimilated to non-imaging models, thus limiting the ability to independently ground their performance in non-computational standards. Therefore, rather than viewing the modest contribution of neuroimaging to diagnostic accuracy as a drawback, we argue that our results suggest a path towards balancing demands for transparency with the need to build models using routinely collected clinical data. Models such as ours may be validated in high-resource areas where the availability of advanced neuroimaging aids interpretability. As physicians may have difficulty entrusting medical decision-making to black box model in artificial intelligence 27 , grounding our machine learning results in the established neuroscience of dementia may help to facilitate clinical uptake. Nevertheless, we note that our non-imaging model may be best suited for deployment among general practitioners (GPs) and in low-resource settings.
Functionally, we also contend that the flexibility of inputs afforded by our approach is a necessary precursor to clinical adoption at multiple stages of dementia. Given that sub-group analyses suggested significant 4-way diagnostic capacity on multiple combinations of training data (i.e., demographics, clinical variables, and neuropsychological tests), our overall framework is likely adaptable to many variations of clinical practice without requiring providers to significantly alter their typical workflows. For example, GPs frequently perform cognitive screening with or without directly ordering MRI tests 28 , 29 , 30 , whereas memory specialists typically expand testing batteries to include imaging and advanced neuropsychological testing. This ability to integrate along the clinical care continuum, from primary to tertiary care allows our deep learning solution to address a two-tiered problem within integrated dementia care by providing a tool for both screening and downstream diagnosis.
Our study has several limitations. To begin, in cases of mixed dementia, the present models default to a diagnosis of AD whenever this condition is present, thus attributing a single diagnosis to participants with multiple comorbidities. Given the considerable prevalence of mixed dementias 31 , future work may include the possibility of a multi-label classification that may allow for the identification of co-occurring dementing conditions (e.g., LBD and AD, VD and AD) within the same individual. Our cohorts also did not contain any confirmed cases of atypical AD, which is estimated to affect approximately 6% of elderly-onset cases and one-third of patients with early-onset disease 32 . We must also note that MCI is a broad category by itself that includes persons who may or may not progress to dementia. When relevant data becomes available across many cohorts, future investigations could include MCI subjects who are amnestic and non-amnestic, to understand distinct signatures of those who have prodromal AD. We also acknowledge that our study data is predominantly obtained from epidemiologic studies which primarily focus on AD and that variables that optimize the identification of this illness may in fact detract from the accurate diagnosis of certain nADDs. For instance, we noted that the performance of our fusion models was slightly lower than that of the MRI-only model for distinguishing AD from non-parkinsonian dementias such as FTD and VD. We speculate that certain forms of neuropsychological testing such as the MMSE, which have well-known limitations in specificity 33 , may bias predictions towards more common forms of dementia such as AD. Although we validated the various models using data from a population-based cohort (i.e., FHS), it is possible that multimodal analysis frameworks have the potential to decrease diagnostic accuracy for less common dementias. Future modeling efforts may optimize for the identification of these diseases by including additional clinical data tailored to their diagnosis: for instance, the inclusion of motor examination to assess for parkinsonism, FLAIR images for vascular injury, or cognitive fluctuations and sleep behavior abnormalities for LBD. Lastly, although we have compared our model to the performance of individual neurologists and neuroradiologists, future studies may consider comparison to consensus reviews by teams of collaborating clinicians.
In conclusion, our interpretable, multimodal deep learning framework was able to obtain high accuracy signatures of dementia status from routinely collected clinical data, which was validated against data from independent cohorts, neuropathological findings, and expert-driven assessment. Moreover, our approach provides a solution that may be utilized across different practice types, from GPs to specialized memory clinics at tertiary care centers. We envision performing a prospective observational study in memory clinics to confirm our model’s ability to assess dementia status at the same level as the expert clinician involved in dementia care. If confirmed in such a head-to-head comparison, our approach has the potential to expand the scope of machine learning for AD detection and management, and ultimately serve as an assistive screening tool for healthcare practitioners.
Study population
This study was exempted from local institutional review board approval, as all neuroimaging and clinical data were obtained in deidentified format upon request from external study centers, who ensured compliance with ethical guidelines and informed consent for all participants. No compensation was provided to participants.
We collected demographics, medical history, neuropsychological tests, and functional assessments as well as magnetic resonance imaging (MRI) scans from 8 cohorts (Table 1 ), totaling 8916 participants after assessing for inclusion criteria. There were 4550 participants with normal cognition (NC), 2412 participants with mild cognitive impairment (MCI), 1606 participants with Alzheimer’s disease dementia (AD) and 348 participants with dementia due to other causes. The eight cohorts include the Alzheimer’s Disease Neuroimaging Initiative (ADNI) dataset ( n = 1821) 34 , 35 , 36 , the National Alzheimer’s Coordinating Center (NACC) dataset ( n = 4822) 21 , 22 , the frontotemporal lobar degeneration neuroimaging initiative (NIFD) dataset ( n = 253) 37 , the Parkinson’s Progression Marker Initiative (PPMI) dataset ( n = 198) 38 , the Australian Imaging, Biomarker and Lifestyle Flagship Study of Ageing (AIBL) dataset ( n = 661) 39 , 40 , 41 , the Open Access Series of Imaging Studies-3 (OASIS) dataset ( n = 666) 42 , the Framingham Heart Study (FHS) dataset ( n = 313) 43 , 44 , and in-house data maintained by the Lewy Body Dementia Center for Excellence at Stanford University (LBDSU) ( n = 182) 45 .
We labeled the participants according to the clinical diagnosis (See Supplementary Information : Data to clinicians and diagnostic criterion). Subjects were labeled according to the clinical diagnoses provided by each study cohort. We kept MCI diagnoses without further consideration of underlying etiology to simulate a realistic spectrum of MCI presentations. For any subjects with documented dementia and primary diagnosis of Alzheimer’s disease dementia, an AD label was assigned regardless of the presence of additional dementing comorbidities. Subjects with dementia but without confirmed AD diagnosis were labeled as nADD. Notably, we elected to conglomerate all nADD subtypes into a singular label given that subdividing model training across an arbitrary number of prediction tasks ran the risk of diluting overall diagnostic accuracy. The ensemble of these 8 cohorts provided us a considerable number of participants with various forms of dementias as their primary diagnosis, including Alzheimer’s disease dementia (AD, n = 1606), Lewy body dementia (LBD, n = 63), frontotemporal dementia (FTD, n = 193), vascular dementia (VD, n = 21), and other causes of dementia ( n = 237). We provided a full survey of nADD dementias by cohort in the Supplementary Information (Table S9 ).
Data inclusion criterion
Subjects from each cohort were eligible for study inclusion if they had at least one T1-weighted volumetric MRI scan within 6 months of an officially documented diagnosis. We additionally excluded all MRI scans with fewer than 60 slices. For subjects with multiple MRIs and diagnosis records within a 6-month period, we selected the closest pairing of neuroimaging and diagnostic label. Therefore, only one MRI per subject was used. For the NACC and the OASIS cohorts, we further queried all available variables relating to demographics, past medical history, neuropsychological testing, and functional assessments. We did not use the availability of non-imaging features to exclude individuals in these cohorts and used K-nearest neighbor imputation for any missing data fields. Our overall data inclusion workflow may be found in Fig. S9 , where we reported the total number of subjects from each cohort before and after application of the inclusion criterion. See Information Availability by Cohort in the Supplementary Information .
MRI harmonization and preprocessing
To harmonize neuroimaging data between cohorts, we developed a pipeline of preprocessing operations (Fig. S10 ) that was applied in identical fashion to all MRIs used in our study. This pipeline broadly consisted of two phases of registration to a standard MNI-152 template. We describe Phase 1 as follows:
Scan axes were reconfigured to match the standard orientation of MNI-152 space.
Using an automated thresholding technique, a 3D volume-of-interest within the original MRI was identified containing only areas with brain tissue.
The volume-of-interest was skull-stripped to isolate brain pixels.
A preliminary linear registration of the skull-stripped brain to a standard MNI-152 template was performed. This step approximated a linear transformation matrix from the original MRI space to the MNI-152 space.
Phase 2 was designed to fine-tune the quality of linear registration and parcellate the brain into discrete regions. These goals were accomplished by the following steps:
The transformation matrix computed from linear registration in Phase 1 was applied to the original MRI scan.
Skull stripping was once again performed after applying the linear registration computed from the initial volume of interest to isolate brain tissue from the full registered MRI scan.
Linear registration was applied again to alleviate any misalignments to MNI-152 space.
Bias field correction was applied to account for magnetic field inhomogeneities.
The brain was parcellated by applying a nonlinear warp of the Hammersmith Adult brain atlas to the post-processed MRI.
All steps of our MRI-processing pipeline were conducted using FMRIB Software Library v6.0 (FSL) (Analysis Group, Oxford University). The overall preprocessing workflow was inspired by the harmonization protocols of the UK Biobank ( https://git.fmrib.ox.ac.uk/falmagro/UK_biobank_pipeline_v_1 ). We manually inspected the outcome of the MRI pipeline on each scan to filter out cases with poor quality or significant processing artifacts.
Evaluation of MRI harmonization
We further assessed our image harmonization pipeline by clustering the data using the t-distributed stochastic neighbor embedding (tSNE) algorithm 46 . We performed this procedure in order to ensure that (i) input data for all models was free of site-, scanner-, and cohort-specific biases and (ii) such biases could not be learned by a predictive model. To accomplish (i), we performed tSNE using pixel values from post-processed, 8x-downsampled MRI scans. For (ii), we performed tSNE using hidden-layer activations derived from the penultimate layer of a convolutional neural network (CNN) developed for our prediction tasks (see “Model Development” below). For the NACC dataset, we assessed clustering of downsampled MRIs and hidden layer activations based on specific Alzheimer’s Disease Research Centers (ADRCs) and scanner manufacturers (i.e., Siemens, Philips, and General Electric). We also repeated tSNE analysis based on specific cohorts (i.e., NACC, ADNI, FHS, etc.) using all available MRIs across our datasets. We also calculated mutual information scores (MIS) between ADRC ID, scanner brand, and diagnostic labels (NC, MCI, AD, and nADD) in the NACC dataset. This metric calculates the degree of similarity between two sets of labels on a common set of data. As with the tSNE analysis, the MIS calculation helped us to exclude the presence of confounding site- and scanner-specific biases on MRI data.
Harmonization of non-imaging data
To harmonize the non-imaging variables across datasets, we first surveyed the available clinical data in all eight cohorts (See Information Availability by Cohort and Non-Imaging Features Used in Model Development in the Supplementary Information ). We specifically examined information related to demographics, past medical history, neuropsychological test results, and functional assessments. Across a range of clinical features, we found the greatest availability of information in the NACC and the OASIS datasets. Additionally, given that the NACC and the OASIS cohorts follow Uniform Data Set (UDS) guidelines, we were able to make use of validated conversion scales between UDS versions 2.0 and 3.0 to align all cognitive measurements onto a common scale. We supply a full listing of clinical variables along with missing information rates per cohort in Fig. S11 .
Overview of the prediction framework
We developed predictive models to meet two main objectives. The first, which we designated the COG task, was to predict the overall degree of cognitive impairment (either NC, MCI, or dementia [DE]) in each participant based on neuroimaging. To meet this goal, we predicted a continuous 0–2 score (NC: 0, MCI: 1, DE: 2), which we denote as the DEmentia MOdel (DEMO) score. Of note, the COG task may also be regarded as consisting of three separate subtasks: (i) separation of NC from MCI and DE (COG NC task), (ii) separation of MCI from NC and DE (COG MCI task), and (iii) separation of DE from NC and MCI (COG DE task). The second objective, which we designated the ADD task, was to predict whether a participant held a diagnosis of AD or nADD given that they were already predicted as DE in the COG task. For ease of reference, we denoted the probability of a person holding an AD diagnosis as the ALZheimer (ALZ) score. Following the sequential completion of the COG and ADD tasks, we were able to successfully separate AD participants from NC, MCI, and nADD subjects.
MRI-only model
We used post-processed volumetric MRIs as inputs and trained a CNN model. To transfer information between the COG and ADD tasks, we trained a common set of convolutional blocks to act as general-purpose feature extractors. The DEMO and the ALZ scores were then calculated separately by appending respective fully connected layers to the shared convolutional backbone. We conducted the COG task as a regression problem using mean square error loss between the DEMO score and available cognitive labels. We performed the ADD task as a classification problem using binary cross entropy loss between the reference AD label and the ALZ score. The MRI-only model was trained using the NACC dataset and validated on all the other cohorts. To facilitate presentation of results, we pooled data from all the external cohorts (ADNI, AIBL, FHS, LBDSU, NIFD, OASIS, and PPMI), and computed all the model performance metrics.
Non-imaging model
In addition to an MRI-only model, we developed a range of traditional machine learning classifiers using all available non-imaging variables shared between the NACC and the OASIS datasets. We first compiled vectors of demographics, past medical history, neuropsychological test results, and functional assessments. We scaled continuous variables by their mean and standard deviations and one-hot encoded categorical variables. These non-imaging data vectors were then passed as input to CatBoost, XGBoost, random forest, decision tree, multi-layer perceptron, support vector machine and K-nearest neighbor algorithms. Like the MRI-only model, each non-imaging model was sequentially trained to complete the COG and the ADD tasks by calculating the DEMO and the ALZ scores, respectively. We ultimately found that a CatBoost model yielded the best overall performance per area-under-receiver-operating-characteristic curve (AUC) and area-under-precision-recall curve (AP) metrics. We, therefore, selected this algorithm as the basis for follow-up analyses.
To mimic a clinical neurology setting, we developed a non-imaging model using data that is routinely collected for dementia diagnosis. A full listing of the variables used as input may be found in our Supplementary Information . While some features such as genetic status (APOE ε4 allele) 47 , or cerebrospinal fluid measures 10 have great predictive value, we have purposefully not included them for model development because they are not part of the standard clinical work-up of dementia.
To infer the extent to which completeness of non-imaging datasets influenced model performance, we conducted multiple experiments using different combinations of clinical data variables. The following combinations were input to the CatBoost algorithm for comparison: (1) demographic characteristics alone, (2) demographic characteristics and neuropsychological tests, (3) demographic characteristics and functional assessments, (4) demographic characteristics and past medical history, (5) demographic characteristics, neuropsychological tests and functional assessments, (6) demographic characteristics, neuropsychological tests and past medical history, and (7) demographic characteristics, neuropsychological tests, past medical history, and functional assessments.
Fusion model
To best leverage every aspect of the available data, we combined both MRI and non-imaging features into a common “fusion” model for the COG and the ADD tasks. The combination of data sources was accomplished by concatenating the DEMO and the ALZ scores derived from the MRI-only model to lists of clinical variables. The resultant vectors were then given as input to traditional machine learning classifiers as described above. Based on the AUC and the AP metrics, we ultimately found that a CNN linked with CatBoost model yielded the highest performance in discriminating different cognitive categories; the combination of CNN and CatBoost models was thus used as the final fusion model for all further experiments. Similarly, to our procedure with the non-imaging model, we studied how MRI features interacted with different subsets of demographic, past medical history, neuropsychological, and functional assessment variables. As with our non-imaging model, development and validation of fusion models was limited to NACC and OASIS only given limited availability of non-imaging data in other cohorts.
Training strategy and data splitting
We trained all models on the NACC dataset using cross validation. NACC was randomly divided into 5 folds of equal size with constant ratios of NC, MCI, AD, and nADD cases. We trained the model on 3 of the 5 folds and used the remaining two folds for validation and testing, respectively. Each tuned model was also tested on the full set of available cases from external datasets. Performance metrics for all models were reported as a mean across five folds of cross validation along with standard deviations and 95% confident intervals. A graphical summary of our cross-validation strategy may be found within Fig. S12 . Prior to training, we also set aside two specialized cohorts within NACC for neuropathologic validation and head-to-head comparison with clinicians. In the former case, we identified 74 subjects from whom post-mortem neuropathological data was available within 2 years of an MRI scan. In the latter, we randomly selected 100 age- and sex-matched groups of patients (25 per diagnostic category) to provide simulated cases to expert clinicians.
SHAP analysis
SHAP is a unified framework for interpreting machine learning models which estimates the contribution of each feature by averaging over all possible marginal contributions to a prediction task 23 . Though initially developed for game theory applications 48 , this approach may be used in deep learning-based computer vision by considering each image voxel or a network node as a unique feature. By assigning SHAP values to specific voxels or by mapping internal network nodes back to the native imaging space, heatmaps may be constructed over input MRIs.
Though a variety of methods exist for estimating SHAP values, we implemented a modified version of the DeepLIFT algorithm 49 , which computes SHAP by estimating differences in model activations during backpropagation relative to a standard reference. We established this reference by integrating over a “background” of training MRIs to estimate a dataset-wide expected value. For each testing example, we then calculated SHAP values for the overall CNN model as well as for specific internal layers. Two sets of SHAP values were estimated for the COG and ADD tasks, respectively. SHAP values calculated over the full model were directly mapped back to native MRI pixels whereas those derived for internal layers were translated to the native imaging space via nearest neighbor interpolation.
Network analysis
We sought to perform a region-by-region graph analysis of SHAP values to determine whether consistent differences in ADD and nADD populations could be demonstrated. To visualize the relationship of SHAP scores across various brain regions, we created graphical representations of inter-region SHAP correlations within the brain. We derived region-specific scores by averaging voxel-wise SHAP values according to their location within the registered MRI. Subsequently, we constructed acyclic graphs in which nodes were defined as specific brain regions and edges as inter-regional correlations measured by Spearman’s rank correlation and Pearson correlation coefficient, separately. To facilitate visualization and convey structural information, we manually aligned the nodes to a radiographic projection of the brain.
Once correlation values were calculated between every pair of nodes, we filtered out the edges with p value larger than 0.05 and ranked the remaining edges according to the absolute correlation value. We used only the top N edges ( N = 100 for sagittal view, N = 200 for axial view) for the graph. We used color to indicate the sign of correlation and thickness to represent the magnitude of correlation. We used the following formula to derive the thickness:
where the threshold is defined as the minimum of the absolute value of all selected edges’ correlation value. The radius of nodes represents the weighted degree of the node which is defined as the sum of the edge weights for edges incident to that node. More specifically, we calculated the radius using the following equation:
In the above equation, we used 20 as a bias term to ensure that every node has at-least a minimal size to be visible on the graph. Note as well that the digit inside each node represents the index of the region name. Derivation of axial and sagittal nodes from the Hammersmith atlas is elaborated in Table S5 .
Neuropathologic validation
Neuropathologic evaluations are considered to be the gold standard for confirming the presence and severity of neurodegenerative diseases 50 . We validated our model’s ability to identify regions of high risk of dementia by comparing the spatial distribution of the model-derived scores with post-mortem neuropathological data from NACC, FHS, and ADNI study cohorts, derived from the National Institute on Aging Alzheimer’s Association guidelines for the neuropathologic assessment of AD 51 . Hundred and ten participants from NACC ( n = 74), ADNI ( n = 25) and FHS ( n = 11) who met the study inclusion criteria, had MRI scans taken within 2 years of death and with neuropathologic data were included in the neuropathologic validation. The data was harmonized in the format of the Neuropathology Data Form Version 10 of the NACC established by the National Institute on Aging. The neuropathological lesions of AD (i.e., amyloid β deposits (Aβ), neurofibrillary tangles (NFTs), and neuritic plaques (NPs)) were assessed in the entorhinal, hippocampal, frontal, temporal, parietal, and occipital cortices. The regions were based on those proposed for standardized neuropathological assessment of AD and the severity of the various pathologies were classified into four semi-quantitative score categories (0 = None, 1 = Mild, 2 = Moderate, 3 = Severe) 52 . Based on the NIA-AA protocol, the severity of neuropathologic changes were evaluated using a global “ABC” score which incorporates histopathologic assessments of amyloid β deposits by the method of Thal phases: 53 (A), staging of neurofibrillary tangles (B) silver-based histochemistry 54 , or phospho-tau immunohistochemistry 55 , and scoring of neuritic plaques (C). Spearman’s rank correlation was used to correlate the DEMO score predictions with the A, B, C scores, and ANOVA and Tukey’s tests were used to assess the differences in the mean DEMO scores across the different levels of the scoring categories. Lastly, a subset of the participants from ADNI ( n = 25) and FHS ( n = 11) had regional semi-quantitative Aβ, NFT, and NP scores, which was also used to validate the model predictions.
We sought to test our model’s predictions against the diagnostic acumen of clinicians who are involved in care of patients with dementia. We recruited an international cohort of practicing neurologists and neuroradiologists to participate in simulated diagnostic tasks using a subset of NACC cases (see “Training strategy and data splitting” above). Neurologists were provided with 100 cases that included imaging data (T1-weighted brain MRI scans) and non-imaging data (demographics, medical history, neuropsychological tests, and functional assessments) and asked to provide diagnostic impressions of NC, MCI, AD, and nADD. Notably, the model was not directly compared to neurologists for the ADD task given that our framework only performs this prediction on patients internally identified as demented. Due to this computational pre-selection, it was not feasible to consistently compare a common cohort of persons with neurologists who also must perform a differential diagnosis of NC, MCI, AD, and nADD. Neuroradiologists were provided with imaging data (T1-weighted brain MRI scans), age, and gender from 50 known DE cases and then asked to provide diagnostic impressions of AD or nADD. For each case, the neuroradiologists also answered a questionnaire to grade the extent of atrophy in each sub-region of the brain on a scale of 0 to 4, where higher values indicate greater atrophy. A case sample and example questionnaires provided to neurologists and neuroradiologists, respectively, may be found within the Supplementary Information (Data to clinicians and diagnostic criterion). For both groups of clinicians, we also calculated inter-annotator agreement using Cohen’s kappa (κ). Additionally, to compare our machine learning models to neuropsychological assessments, we performed the COG NC , COG DE , and ADD tasks using all possible whole number cutoffs of neuropsychiatric test scores available in the NACC dataset. Following this approach, we performed simple thresholding for binary classifications.
Performance metrics
We presented the performance by computing the mean and the standard deviation over the model runs. We generated receiver operating characteristic (ROC) and precision-recall (PR) curves based on model predictions on the NACC test data as well as on the other datasets. For each ROC and PR curve, we also computed the area under curve (AUC & AP) values. Additionally, we computed sensitivity, specificity, F1-score and Matthews correlation coefficient on each set of model predictions. The F1-score considers both precision and recall of a test whereas the MCC is a balanced measure of quality for dataset classes of different sizes of a binary classifier. We also calculated inter-annotator agreement using Cohen’s kappa (κ), as the ratio of the number of times two experts agreed on a diagnosis. We computed average pairwise κ for each sub-group task that provided an overall measure of agreement between the neurologists and the neuroradiologists, respectively.
Statistical analysis
We used one-way ANOVA test and the χ2 test for continuous and categorical variables, respectively to assess the overall levels of differences in the population characteristics between NC, MCI, AD, and nADD groups across the study cohorts. To validate our CNN model, we evaluated whether the presence and severity of the semi-quantitative neuropathology scores across the neuropathological lesions of AD (i.e., amyloid β deposits (Aβ), neurofibrillary tangles (NFTs), and neuritic plaques (NPs)) reflected the DEMO score predicted by the CNN model. We stratified the lesions based on A, B, and C scores and used Spearman’s rank correlation to assess their relationship with the DEMO scores. Next using one-way ANOVA analysis, we evaluated the differences in the mean DEMO scores across the different levels of the scoring categories for the A, B, and C scores. We used the Tukey-Kramer test to identify the pairwise statistically significant differences in the mean DEMO score between the levels of scoring categories (0–3). Similarly, to analyze the correspondence between SHAP values and a known marker of neurodegenerative disease, we correlated SHAPs with the radiologist impressions of atrophy. Utilizing the segmentation maps derived from each participant, we calculated regional SHAP averages on each of the 50 test cases given to neuroradiologists with the 0–4 regional atrophy scales assigned by the clinicians. We calculated Pearson’s correlation coefficients with two-tailed p values that indicates the probability that an uncorrelated system producing Pearson’s correlation coefficient as extreme as the observed value in the neuroanatomic regions known to be implicated in AD pathology. All statistical analyses were conducted at a significance level of 0.05. Confidence intervals for model performance were calculated by assuming a normal distribution of AUC and AP values across cross-validation experiments using t-student distribution with 4 degrees of freedom.
Computational hardware and software
We processed all MRIs and non-imaging data on a computing workstation with Intel i9 14-core 3.3 GHz processor, and 4 NVIDIA RTX 2080Ti GPUs. Python (version 3.7.7) was used for software development. Each deep learning model was developed using PyTorch (version 1.5.1), and plots were generated using the Python library matplotlib (version 3.1.1) and numpy (version 1.18.1) was used for vectorized numerical computation. Other Python libraries used to support data analysis include pandas (version 1.0.3), scipy (version 1.3.1), tensorflow (version 1.14.0), tensorboardX (version 1.9), torchvision (version 0.6) and scikit-learn (version 0.22.1). Using a single 2080Ti GPU, the average run time for training the deep learning model was 10 h, and inference task took less than a minute. All clinicians reviewed MRIs using 3D Slicer (version 4.10.2) ( https://www.slicer.org/ ) and recorded impressions in REDCap (version 11.1.3). Additionally, statistics for neuropathology analysis were completed using SAS (version 9.4).
Reporting summary
Further information on research design is available in the Nature Research Reporting Summary linked to this article.
Data availability
Data from ADNI, AIBL, NACC, NIFD, OASIS and PPMI can be downloaded from publicly available resources. Data from FHS and LBDSU are available upon request and will be subjected to institutional approval. Source data for figures are provided with this paper. Source data are provided with this paper.
Code availability
Python scripts are made available on GitHub ( https://github.com/vkola-lab/ncomms2022 ).
Nichols, E. et al. Global, regional, and national burden of Alzheimer’s disease and other dementias, 1990–2016: a systematic analysis for the Global Burden of Disease Study 2016. Lancet Neurol. 18 , 88–106 (2019).
Article Google Scholar
Mehta, K. M. & Yeo, G. W. Systematic review of dementia prevalence and incidence in United States race/ethnic populations. Alzheimer’s Dement. 13 , 72–83 (2017).
James, B. D. et al. Contribution of Alzheimer disease to mortality in the United States. Neurology 82 , 1045–1050 (2014).
Article CAS PubMed PubMed Central Google Scholar
Palmqvist, S. et al. Discriminative accuracy of plasma phospho-tau217 for Alzheimer disease vs other neurodegenerative disorders. Jama 324 , 772–781 (2020).
Article CAS PubMed Google Scholar
Nordberg, A. PET imaging of amyloid in Alzheimer’s disease. lancet Neurol. 3 , 519–527 (2004).
Bohnen, N. I., Djang, D. S., Herholz, K., Anzai, Y. & Minoshima, S. Effectiveness and safety of 18F-FDG PET in the evaluation of dementia: a review of the recent literature. J. Nucl. Med. 53 , 59–71 (2012).
Mattsson, N. et al. Predicting diagnosis and cognition with 18F-AV-1451 tau PET and structural MRI in Alzheimer’s disease. Alzheimer’s Dement. 15 , 570–580 (2019).
Ossenkoppele, R. et al. Associations between tau, Aβ, and cortical thickness with cognition in Alzheimer disease. Neurology 92 , e601–e612 (2019).
Sevigny, J. et al. The antibody aducanumab reduces Aβ plaques in Alzheimer’s disease. Nature 537 , 50–56 (2016).
Article ADS CAS PubMed Google Scholar
McKhann, G. M. et al. The diagnosis of dementia due to Alzheimer’s disease: recommendations from the National Institute on Aging-Alzheimer’s Association workgroups on diagnostic guidelines for Alzheimer’s disease. Alzheimer’s Dement. 7 , 263–269 (2011).
Knopman, D. et al. Practice parameter: diagnosis of dementia (an evidence-based review): report of the Quality Standards Subcommittee of the American Academy of Neurology. Neurology 56 , 1143–1153 (2001).
Beach, T. G., Monsell, S. E., Phillips, L. E. & Kukull, W. Accuracy of the clinical diagnosis of Alzheimer disease at National Institute on Aging Alzheimer Disease Centers, 2005–2010. J. Neuropathol. Exp. Neurol. 71 , 266–273 (2012).
Article PubMed Google Scholar
Dall, T. M. et al. Supply and demand analysis of the current and future US neurology workforce. Neurology 81 , 470–478 (2013).
Article PubMed PubMed Central Google Scholar
Dall, T. M. Physician workforce shortages: what do the data really say? Acad. Med 90 , 1581–1582 (2015).
Pedersen, M. et al. Artificial intelligence for clinical decision support in neurology. Brain Commun. 2 , fcaa096 (2020).
Lu, D., Popuri, K., Ding, G. W., Balachandar, R. & Beg, M. F. Multimodal and multiscale deep neural networks for the early diagnosis of Alzheimer’s disease using structural MR and FDG-PET images. Sci. Rep. 8 , 1–13 (2018).
Google Scholar
Qiu, S. et al. Development and validation of an interpretable deep learning framework for Alzheimer’s disease classification. Brain 143 , 1920–1933 (2020).
Wada, A. et al. Differentiating Alzheimer’s disease from dementia with Lewy bodies using a deep learning technique based on structural brain connectivity. Magn. Reson. Med. Sci. 18 , 219 (2019).
Nemoto, K. et al. Differentiating dementia with lewy bodies and Alzheimer’s disease by deep learning to structural MRI. J. Neuroimaging 31 , 579–587 (2021).
Ma, D. et al. Differential diagnosis of frontotemporal dementia, Alzheimer’s disease, and normal aging using a multi-scale multi-type feature generative adversarial deep neural network on structural magnetic resonance images. Front. Neurosci. 14 , 853 (2020).
Beekly, D. L. et al. The National Alzheimer’s Coordinating Center (NACC) database: the uniform data set. Alzheimer Dis. Associated Disord. 21 , 249–258 (2007).
Beekly, D. L. et al. The national Alzheimer’s coordinating center (NACC) database: an Alzheimer disease database. Alzheimer Dis. Associated Disord. 18 , 270–277 (2004).
Lundberg, S. M. & Lee, S.-I. A Unified Approach to Interpreting Model Predictions: in Proceedings of the 31st International Conference on Neural Information Processing Systems . 4768–4777 (2017).
DeGrave, A. J., Janizek, J. D. & Lee, S.-I. AI for radiographic COVID-19 detection selects shortcuts over signal. Nat. Mach. Intell. 3 , 610–619 (2021).
Wang, Y. et al. Classification of subcortical vascular cognitive impairment using single MRI sequence and deep learning convolutional neural networks. Front. Neurosci. 13 , 627 (2019).
Castellazzi, G. et al. A machine learning approach for the differential diagnosis of Alzheimer and vascular dementia fed by MRI selected features. Front. Neuroinform. 14 , 25 (2020).
Wang, F., Kaushal, R. & Khullar, D. Should health care demand interpretable artificial intelligence or accept “black box” medicine? Ann. Intern. Med. 172 , 59–60 (2020).
Weiner, M. & Khachaturian, Z. The use of MRI and PET for clinical diagnosis of dementia and investigation of cognitive impairment: a consensus report. Alzheimer’s Assoc. Chic., IL 1 , 1–15 (2005).
Kara, S. et al. Guidelines, training and quality assurance: influence on general practitioner MRI referral quality. J. Prim. Health Care 11 , 235–242 (2019).
Article ADS PubMed Google Scholar
Bernstein, A. et al. Dementia assessment and management in primary care settings: a survey of current provider practices in the United States. BMC Health Serv. Res . 19 , 919 (2019).
Zekry, D., Hauw, J. J. & Gold, G. Mixed dementia: epidemiology, diagnosis, and treatment. J. Am. Geriatrics Soc. 50 , 1431–1438 (2002).
Graff-Radford, J. et al. New insights into atypical Alzheimer’s disease in the era of biomarkers. Lancet Neurol. 20 , 222–234 (2021).
Wind, A. W. et al. Limitations of the Mini‐Mental State Examination in diagnosing dementia in general practice. Int. J. Geriatr. psychiatry 12 , 101–108 (1997).
Jack, C. R. Jr et al. The Alzheimer’s disease neuroimaging initiative (ADNI): MRI methods. J. Magn. Reson. Imaging. 27 , 685–691 (2008).
Mueller, S. G. et al. Ways toward an early diagnosis in Alzheimer’s disease: the Alzheimer’s Disease Neuroimaging Initiative (ADNI). Alzheimer’s Dement. 1 , 55–66 (2005).
Petersen, R. C. et al. Alzheimer’s Disease Neuroimaging Initiative (ADNI): clinical characterization. Neurology 74 , 201–209 (2010).
Boxer, A. L. et al. Frontotemporal degeneration, the next therapeutic frontier: molecules and animal models for frontotemporal degeneration drug development. Alzheimers Dement 9 , 176–188 (2013).
Marek, K. et al. The parkinson progression marker initiative (PPMI). Prog. Neurobiol. 95 , 629–635 (2011).
Article PubMed Central Google Scholar
Ellis, K., Ames, D., Martins, R., Hudson, P. & Masters, C. The Australian biomarkers lifestyle and imaging flagship study of ageing. Acta Neuropsychiatr. 18 , 285 (2006).
PubMed Google Scholar
Ellis, K. A. et al. The Australian Imaging, Biomarkers and Lifestyle (AIBL) study of aging: methodology and baseline characteristics of 1112 individuals recruited for a longitudinal study of Alzheimer’s disease. Int Psychogeriatr. 21 , 672–687 (2009).
Ellis, K. A. et al. Addressing population aging and Alzheimer’s disease through the Australian imaging biomarkers and lifestyle study: collaboration with the Alzheimer’s Disease Neuroimaging Initiative. Alzheimers Dement 6 , 291–296 (2010).
LaMontagne, P. J. et al. OASIS-3: Longitudinal neuroimaging, clinical, and cognitive dataset for normal aging and Alzheimer disease. medRxiv , https://doi.org/10.1101/2019.12.13.19014902 (2019).
Mahmood, S. S., Levy, D., Vasan, R. S. & Wang, T. J. The Framingham Heart Study and the epidemiology of cardiovascular disease: a historical perspective. lancet 383 , 999–1008 (2014).
Massaro, J. M. et al. Managing and analysing data from a large-scale study on Framingham Offspring relating brain structure to cognitive function. Stat. Med. 23 , 351–367 (2004).
Linortner, P. et al. White matter hyperintensities related to Parkinson’s disease executive function. Mov. Disord. Clin. Pr. 7 , 629–638 (2020).
Van der Maaten, L. & Hinton, G. Visualizing data using t-SNE. J. Mach. Learn. Res. 9 (2008).
Choudhury, P., Ramanan, V. K. & Boeve, B. F. APOE ɛ4 allele testing and risk of Alzheimer disease. JAMA 325 , 484–485 (2021).
Shapley, L. S. 17. A value for n-person games . (Princeton University Press, 2016).
Shrikumar, A., Greenside, P. & Kundaje, A. Learning important features through propagating activation differences: in. Int. Conf. Mach. Learn. 70 , 3145–3153 (2017).
Besser, L. M. et al. The revised national Alzheimer’s coordinating center’s neuropathology form-available data and new analyses. J. Neuropathol. Exp. Neurol. 77 , 717–726 (2018).
Montine, T. J. et al. National Institute on Aging–Alzheimer’s Association guidelines for the neuropathologic assessment of Alzheimer’s disease: a practical approach. Acta Neuropathologica 123 , 1–11 (2012).
Hyman, B. T. et al. National Institute on Aging–Alzheimer’s Association guidelines for the neuropathologic assessment of Alzheimer’s disease. Alzheimer’s Dement. 8 , 1–13 (2012).
Thal, D. R., Rub, U., Orantes, M. & Braak, H. Phases of A beta-deposition in the human brain and its relevance for the development of AD. Neurology 58 , 1791–1800 (2002).
Braak, H. & Braak, E. Neuropathological stageing of Alzheimer-related changes. Acta Neuropathologica 82 , 239–259 (1991).
Braak, H., Alafuzoff, I., Arzberger, T., Kretzschmar, H. & Del Tredici, K. Staging of Alzheimer disease-associated neurofibrillary pathology using paraffin sections and immunocytochemistry. Acta Neuropathol. 112 , 389–404 (2006).
Download references
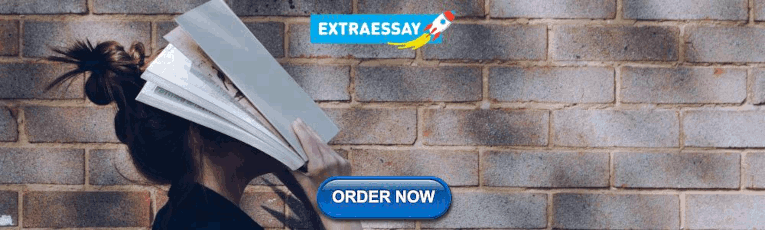
Acknowledgements
This project was supported by grants from the Karen Toffler Charitable Trust (VBK), the Michael J. Fox Foundation (KLP), the Lewy Body Dementia Association (KLP), the Alzheimer’s Drug Discovery Foundation (RA), the American Heart Association (20SFRN35460031, VBK), and the National Institutes of Health (R01-HL159620 [VBK], R21-CA253498 [VBK], RF1-AG062109 [RA], RF1-AG072654 [RA], U19-AG065156 [KLP], P30-AG066515 [KLP], R01-NS115114 [KLP], K23-NS075097 [KLP], U19-AG068753 [RA] and P30-AG013846 [RA, VBK]). We acknowledge the efforts of several investigators from the ADNI, AIBL, FHS, LBDSU, NACC, NIFD, OASIS, and PPMI studies for providing access to data.
Author information
These authors contributed equally: Shangran Qiu, Matthew I. Miller.
Authors and Affiliations
Department of Medicine, Boston University School of Medicine, Boston, MA, USA
Shangran Qiu, Matthew I. Miller, Joyce C. Lee, Chonghua Xue, Yunruo Ni, Yuwei Wang & Vijaya B. Kolachalama
Department of Physics, College of Arts & Sciences, Boston University, Boston, MA, USA
Shangran Qiu
Department of Anatomy and Neurobiology, Boston University School of Medicine, Boston, MA, USA
Prajakta S. Joshi, Chonghua Xue, Phillip H. Hwang & Rhoda Au
Department of General Dentistry, Boston University School of Dental Medicine, Boston, MA, USA
Prajakta S. Joshi
The Framingham Heart Study, Boston University School of Medicine, Boston, MA, USA
Prajakta S. Joshi, Jesse Mez & Rhoda Au
School of Public Health and Tropical Medicine, Tulane University, New Orleans, LA, USA
Ileana De Anda-Duran
Department of Radiology, College of Medicine, University of Nebraska Medical Center, Omaha, NE, USA
Justin A. Cramer
Department of Neurology, Boston University School of Medicine, Boston, MA, USA
Brigid C. Dwyer, Michelle C. Kaku, Sarah O’Shea, Marie-Helene Saint-Hilaire, E. Alton Sartor, Aneeta R. Saxena, Ludy C. Shih, Courtney E. Takahashi, Shuhan Zhu, Michael L. Alosco, Jesse Mez & Rhoda Au
Department of Neurology, Peking Union Medical College Hospital, Peking Union Medical College, Chinese Academy of Medical Sciences, Beijing, China
Honglin Hao, Jing Yuan & Yan Zhou
Department of Neurological Sciences, College of Medicine, University of Nebraska Medical Center, Omaha, NE, USA
Sachin Kedar, Daniel L. Murman, Arun Swaminathan & Olga Taraschenko
Department Neurology, Emory University School of Medicine, Atlanta, GA, USA
Sachin Kedar
Department Ophthalmology, Emory University School of Medicine, Atlanta, GA, USA
Department of Radiology, Lahey Hospital & Medical Center, Burlington, MA, USA
Peter H. Lee, Aaron B. Paul, Juan E. Small & Maximilian J. Smith
Department of Radiology, Boston University School of Medicine, Boston, MA, USA
Asim Z. Mian
Department of Radiology, Peking Union Medical College Hospital, Peking Union Medical College, Chinese Academy of Medical Sciences, Beijing, China
Boston University Alzheimer’s Disease Research Center, Boston, MA, USA
Michael L. Alosco, Jesse Mez, Thor D. Stein, Rhoda Au & Vijaya B. Kolachalama
Department of Pathology and Laboratory Medicine, Boston University School of Medicine, Boston, MA, USA
Thor D. Stein
Boston VA Healthcare System, Boston, MA, USA
Bedford VA Healthcare System, Bedford, MA, USA
Department of Neurology, Stanford University, Palo Alto, CA, USA
Kathleen L. Poston
Department of Epidemiology, Boston University School of Public Health, Boston, MA, USA
Department of Computer Science, Boston University, Boston, MA, USA
Vijaya B. Kolachalama
Faculty of Computing & Data Sciences, Boston University, Boston, MA, USA
You can also search for this author in PubMed Google Scholar
Contributions
S.Q. and M.I.M. contributed equally to this work. S.Q. and M.I.M. performed literature search. S.Q. designed and developed the deep learning framework, performed image processing, and constructed the models. S.Q., P.S.J., and J.C.L., performed data collection, data harmonization and statistical analysis. C.X., Y.N., Y.W., I.D.A., and P.H.H. assisted with data collection and performed sub-group analyses. S.Q., M.I.M., P.S.J., J.C.L., and C.X. generated the figures and tables. P.S.J. and S.Q. performed the analysis on the neuropathology data. J.A.C., B.C.D., H.H., M.C.K., S.K., P.H.L., A.Z.M., D.L.M., S.O., A.B.P., M-H.S-H., E.A.S., A.R.S., L.C.S., J.E.S., M.J.S., A.S., C.E.T., O.T., H.Y., J.Y., Y.Z., S.Z. and K.L.P. are the practicing clinicians who reviewed the cases. M.L.A., J.M., T.D.S, K.L.P., and R.A. provided the clinical relevance context. K.L.P. and R.A. provided access to data. M.I.M. and V.B.K. wrote the manuscript. All authors reviewed and approved the manuscript. V.B.K. conceived, designed, and directed the entire study.
Corresponding author
Correspondence to Vijaya B. Kolachalama .
Ethics declarations
Competing interests.
V.B.K. reports honoraria from invited scientific presentations to industry not exceeding $5000/year. He also serves as a consultant to Davos Alzheimer’s Collaborative. R.A. is a scientific advisor to Signant Health and consultant to Biogen. K.L.P. reports honoraria from invited scientific presentations to universities and professional societies not exceeding $5,000/year and has received consulting fees from Curasen. The remaining authors declare no competing interests.
Peer review
Peer review information.
Nature Communications thanks Arman Eshaghi and the other anonymous reviewer(s) for their contribution to the peer review of this work. Peer review reports are available.
Additional information
Publisher’s note Springer Nature remains neutral with regard to jurisdictional claims in published maps and institutional affiliations.
Supplementary information
Supplementary information, peer review file, reporting summary, source data, source data, rights and permissions.
Open Access This article is licensed under a Creative Commons Attribution 4.0 International License, which permits use, sharing, adaptation, distribution and reproduction in any medium or format, as long as you give appropriate credit to the original author(s) and the source, provide a link to the Creative Commons license, and indicate if changes were made. The images or other third party material in this article are included in the article’s Creative Commons license, unless indicated otherwise in a credit line to the material. If material is not included in the article’s Creative Commons license and your intended use is not permitted by statutory regulation or exceeds the permitted use, you will need to obtain permission directly from the copyright holder. To view a copy of this license, visit http://creativecommons.org/licenses/by/4.0/ .
Reprints and permissions
About this article
Cite this article.
Qiu, S., Miller, M.I., Joshi, P.S. et al. Multimodal deep learning for Alzheimer’s disease dementia assessment. Nat Commun 13 , 3404 (2022). https://doi.org/10.1038/s41467-022-31037-5
Download citation
Received : 21 August 2021
Accepted : 06 May 2022
Published : 20 June 2022
DOI : https://doi.org/10.1038/s41467-022-31037-5
Share this article
Anyone you share the following link with will be able to read this content:
Sorry, a shareable link is not currently available for this article.
Provided by the Springer Nature SharedIt content-sharing initiative
This article is cited by
Multimodal diagnosis model of alzheimer’s disease based on improved transformer.
BioMedical Engineering OnLine (2024)
Comparing a pre-defined versus deep learning approach for extracting brain atrophy patterns to predict cognitive decline due to Alzheimer’s disease in patients with mild cognitive symptoms
- Ida Arvidsson
- Olof Strandberg
- Niklas Mattsson-Carlgren
Alzheimer's Research & Therapy (2024)
Leveraging electronic health records and knowledge networks for Alzheimer’s disease prediction and sex-specific biological insights
- Alice S. Tang
- Katherine P. Rankin
- Marina Sirota
Nature Aging (2024)
An explainable machine learning approach for Alzheimer’s disease classification
- Abbas Saad Alatrany
- Dhiya Al-Jumeily
Scientific Reports (2024)
Towards a digitally connected body for holistic and continuous health insight
- Philipp Gutruf
Communications Materials (2024)
By submitting a comment you agree to abide by our Terms and Community Guidelines . If you find something abusive or that does not comply with our terms or guidelines please flag it as inappropriate.
Quick links
- Explore articles by subject
- Guide to authors
- Editorial policies
Sign up for the Nature Briefing: Translational Research newsletter — top stories in biotechnology, drug discovery and pharma.

- Alzheimer's disease & dementia
- Arthritis & Rheumatism
- Attention deficit disorders
- Autism spectrum disorders
- Biomedical technology
- Diseases, Conditions, Syndromes
- Endocrinology & Metabolism
- Gastroenterology
- Gerontology & Geriatrics
- Health informatics
- Inflammatory disorders
- Medical economics
- Medical research
- Medications
- Neuroscience
- Obstetrics & gynaecology
- Oncology & Cancer
- Ophthalmology
- Overweight & Obesity
- Parkinson's & Movement disorders
- Psychology & Psychiatry
- Radiology & Imaging
- Sleep disorders
- Sports medicine & Kinesiology
- Vaccination
- Breast cancer
- Cardiovascular disease
- Chronic obstructive pulmonary disease
- Colon cancer
- Coronary artery disease
- Heart attack
- Heart disease
- High blood pressure
- Kidney disease
- Lung cancer
- Multiple sclerosis
- Myocardial infarction
- Ovarian cancer
- Post traumatic stress disorder
- Rheumatoid arthritis
- Schizophrenia
- Skin cancer
- Type 2 diabetes
- Full List »
share this!
April 18, 2024
This article has been reviewed according to Science X's editorial process and policies . Editors have highlighted the following attributes while ensuring the content's credibility:
fact-checked
peer-reviewed publication
trusted source
New therapy shows promise for a rare childhood dementia
by University of Sydney
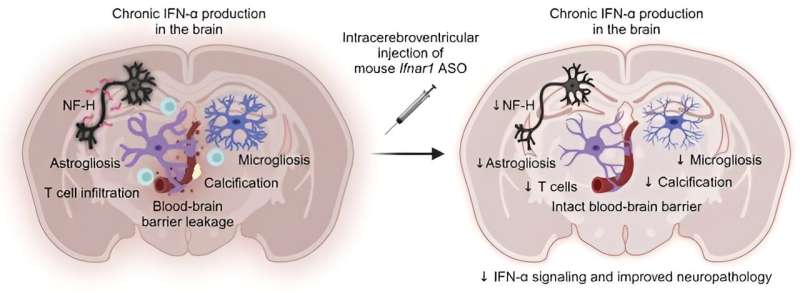
New RNA-targeted therapy can halt the progression of a specific type of childhood dementia in mice and holds potential to reverse some of the detrimental effects of this rare disease.
Scientists from the University of Sydney's Charles Perkins Center and the School of Life and Environmental Sciences have shown that a new RNA-targeted therapy can halt the progression of a specific type of childhood dementia in mice and holds potential to reverse some of the detrimental effects of this rare disease. The research is published in the Journal of Clinical Investigation .
Aicardi-Goutières Syndrome (AGS) is among the more than 100 rare neurodegenerative genetic disorders identified as causing childhood dementia. A few thousand worldwide, including several in Australia, have AGS.
The underlying cause of AGS is interferon, the body's own defense against infection. In AGS, excessive production of interferon in the brain leads to neuroinflammation and neurodegeneration.
Although many newborns with AGS show no symptoms, they typically develop severe neurological issues and lifelong physical impairments within the first two years of life.
With no cure currently available, existing treatments for AGS focus on symptom management. The urgent need for an effective therapeutic approach has prompted researchers to explore innovative solutions.
The new approach targets the interferon alpha receptor using antisense oligonucleotides. These therapies are designed to seek out, bind to, and degrade messenger RNA (mRNA) in a highly specific manner, reducing the amount of disease-causing protein. Antisense oligonucleotide therapies have shown potential in treating various neurological diseases, including SOD1-dependent ALS, a fatal motor neuron disease.
In a study, researchers found that when mice received the treatment directly into their central nervous system, it significantly reduced the interferon response in the brain, reducing both neuroinflammation and neuronal damage and restored the integrity of the blood-brain barrier.
Dr. Barney Viengkhou, lead author of the new paper, stated, "The fact that we observed the treatment's effectiveness in halting and even reversing some neuropathological features in older mice with more advanced disease is particularly exciting."
Patrick Winters, Research Director of the Aicardi-Goutières Syndrome Advocacy Association (AGSAA), expressed his enthusiasm for the findings, saying, "As a passionate advocate for Aicardi-Goutières syndrome, I celebrate the findings in this study. This new drug therapy offers real hope for managing this disease effectively. It's a potential breakthrough that may lighten the burden for those battling this rare and serious condition. This discovery signals a brighter future for AGS."
Seeking the molecular mechanism behind AGS
For the past 10 years, the University of Sydney research team led by Associate Professor Markus Hofer has been investigating the molecular mechanisms underlying AGS development.
"Our understanding of the pathological processes involved in AGS has evolved significantly," said Associate Professor Hofer. "We learn more with every study and with the experiences shared by families affected by AGS."
"This research marks a crucial step forward in the quest for effective treatments for this devastating condition."
This study was conducted in partnership with Ionis Pharmaceuticals, who developed the treatment, and Biogen, with further research underway. With animal studies now complete, the findings look set to inform human trials in the future.
New hope for families in the future
Pediatric neurologist Professor Russell Dale, who cares for 10 children with AGS at the Children's Hospital at Westmead, remarked, "We sadly have few options for children who are deteriorating and losing developmental skills. This targeted ASO therapy approach provides hope for these families in the future."
Associate Professor Hofer added, "It's possible that in the future this treatment strategy could be applicable to similar conditions."
This is especially crucial given the alarming statistics surrounding childhood dementia.
Childhood dementia claims more young lives each year in Australia than childhood cancer. It affects 1 in 2,900 births, and about 1,400 young Australians are currently living with childhood dementia.
"This groundbreaking research has the potential to change the lives of children with dementia caused by AGS across the world, and the learnings will spill over to therapy development for many other types of childhood dementia as well, says Dr. Kris Elvidge, Head of Research at the Childhood Dementia Initiative.
"There is an urgent need to develop not only new innovative treatments but also to provide appropriate care and support for families affected by childhood dementia ."
Explore further
Feedback to editors

Study finds that human neuron signals flow in one direction
24 minutes ago

A common pathway in the brain that enables addictive drugs to hijack natural reward processing identified

Scientists identify airway cells that sense aspirated water and acid reflux

Environment may influence metacognitive abilities more than genetics

Contracting RSV before age two can cause long-term lung changes and impairment

Mutations in noncoding DNA become functional in some cancer-driving genes

Novel cell therapy treatments offer promise to immune-compromised children

DNA vaccine against Zika performs well in tests on mice
2 hours ago

Human cases of bird flu 'an enormous concern': WHO
3 hours ago

Perfect balance: How the brain fine-tunes its sensitivity
4 hours ago
Related Stories

Genetic 'whiteout' offers hope to children living with dementia
Apr 17, 2023

Devastating causes of childhood dementia revealed
Sep 18, 2023

Childhood dementia: Insights from the eyes
Nov 30, 2020

Dementia protein changes discovered in terminal childhood disease
Jan 10, 2022

Accumulated DNA damage linked to neurodegeneration in a rare pediatric disorder
Oct 15, 2021

Enzyme therapy shows promise for childhood dementia
Oct 11, 2022
Recommended for you
Let us know if there is a problem with our content.
Use this form if you have come across a typo, inaccuracy or would like to send an edit request for the content on this page. For general inquiries, please use our contact form . For general feedback, use the public comments section below (please adhere to guidelines ).
Please select the most appropriate category to facilitate processing of your request
Thank you for taking time to provide your feedback to the editors.
Your feedback is important to us. However, we do not guarantee individual replies due to the high volume of messages.
E-mail the story
Your email address is used only to let the recipient know who sent the email. Neither your address nor the recipient's address will be used for any other purpose. The information you enter will appear in your e-mail message and is not retained by Medical Xpress in any form.
Newsletter sign up
Get weekly and/or daily updates delivered to your inbox. You can unsubscribe at any time and we'll never share your details to third parties.
More information Privacy policy
Donate and enjoy an ad-free experience
We keep our content available to everyone. Consider supporting Science X's mission by getting a premium account.
E-mail newsletter
New therapy shows promise for a rare childhood dementia
Scientists from the University of Sydney’s Charles Perkins Centre and the School of Life and Environmental Sciences have shown that a new RNA-targeted therapy can halt the progression of a specific type of childhood dementia in mice and holds potential to reverse some of the detrimental effects of this rare disease.
Aicardi-Goutières Syndrome (AGS) is among the more than 100 rare neurodegenerative genetic disorders identified as causing childhood dementia. A few thousand worldwide, including several in Australia, have AGS.
The underlying cause of AGS is interferon, the body’s own defence against infection. In AGS, excessive production of interferon in the brain leads to neuroinflammation and neurodegeneration.
Although many newborns with AGS show no symptoms, they typically develop severe neurological issues and lifelong physical impairments within the first two years of life.
With no cure currently available, existing treatments for AGS focus on symptom management. The urgent need for an effective therapeutic approach has prompted researchers to explore innovative solutions.
The new approach targets the interferon alpha receptor using antisense oligonucleotides. These therapies are designed to seek out, bind to, and degrade messenger RNA (mRNA) in a highly specific manner, reducing the amount of disease-causing protein. Antisense oligonucleotide therapies have shown potential in treating various neurological diseases, including SOD1-dependent ALS, a fatal motor neuron disease.
In a study, published in The Journal of Clinical Investigation , researchers found that when mice received the treatment directly into their central nervous system, it significantly reduced the interferon response in the brain, reducing both neuroinflammation and neuronal damage and restored the integrity of the blood-brain barrier.
Dr. Barney Viengkhou, lead author of the new paper, stated, “The fact that we observed the treatment’s effectiveness in halting and even reversing some neuropathological features in older mice with more advanced disease is particularly exciting.”
Patrick Winters, Research Director of the Aicardi-Goutières Syndrome Advocacy Association (AGSAA), expressed his enthusiasm for the findings, saying, “As a passionate advocate for Aicardi-Goutières syndrome, I celebrate the findings in this study. This new drug therapy offers real hope for managing this disease effectively. It’s a potential breakthrough that may lighten the burden for those battling this rare and serious condition. This discovery signals a brighter future for AGS.”
Seeking the molecular mechanism behind AGS
For the past 10 years, the University of Sydney research team led by Associate Professor Markus Hofer has been investigating the molecular mechanisms underlying AGS development.
“Our understanding of the pathological processes involved in AGS has evolved significantly,” said Associate Professor Hofer. “We learn more with every study and with the experiences shared by families affected by AGS.”
“This research marks a crucial step forward in the quest for effective treatments for this devastating condition.”
This study was conducted in partnership with Ionis Pharmaceuticals, who developed the treatment, and Biogen, with further research underway. With animal studies now complete, the findings look set to inform human trials in the future.
New hope for families in the future
Paediatric neurologist Professor Russell Dale, who cares for 10 children with AGS at the Children's Hospital at Westmead, remarked, “We sadly have few options for children who are deteriorating and losing developmental skills. This targeted ASO therapy approach provides hope for these families in the future.”
Associate Professor Hofer added, “It’s possible that in the future this treatment strategy could be applicable to similar conditions.”
This is especially crucial given the alarming statistics surrounding childhood dementia.
Childhood dementia claims more young lives each year in Australia than childhood cancer. It affects one in 2,900 births, and around 1,400 young Australians are currently living with childhood dementia.
“This groundbreaking research has the potential to change the lives of children with dementia caused by AGS across the world, and the learnings will spill over to therapy development for many other types of childhood dementia as well, says Dr Kris Elvidge, Head of Research at the Childhood Dementia Initiative.
“There is an urgent need to develop not only new innovative treatments but also to provide appropriate care and support for families affected by childhood dementia.”
Declaration: Parts of this study were supported by a grant from the National Health and Medical Research Council Australia (NHMRC) Ideas grant. Ionis Pharmaceuticals provided the drug used and participated in the study. A number of authors are employed by Ionis Pharmaceuticals and Biogen. Ethical approval was granted by the University of Sydney Animal Care and Ethics Committee (2014/688).
Media Office
- 02 8627 0246
- [email protected]
Related articles
Evolution wired human brains to act like supercomputers, how a cat got people talking about rare neurological diseases, scientists discover spiral-shaped signals that organise brain activity.
Switch language:
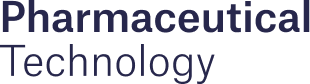
Inflammation among current dementia research focus, says Alchemab CSO
Alchemab CSO Jane Osbourn also observes recent ‘step change’ in collaboration and consortia around dementia.
- Share on Linkedin
- Share on Facebook
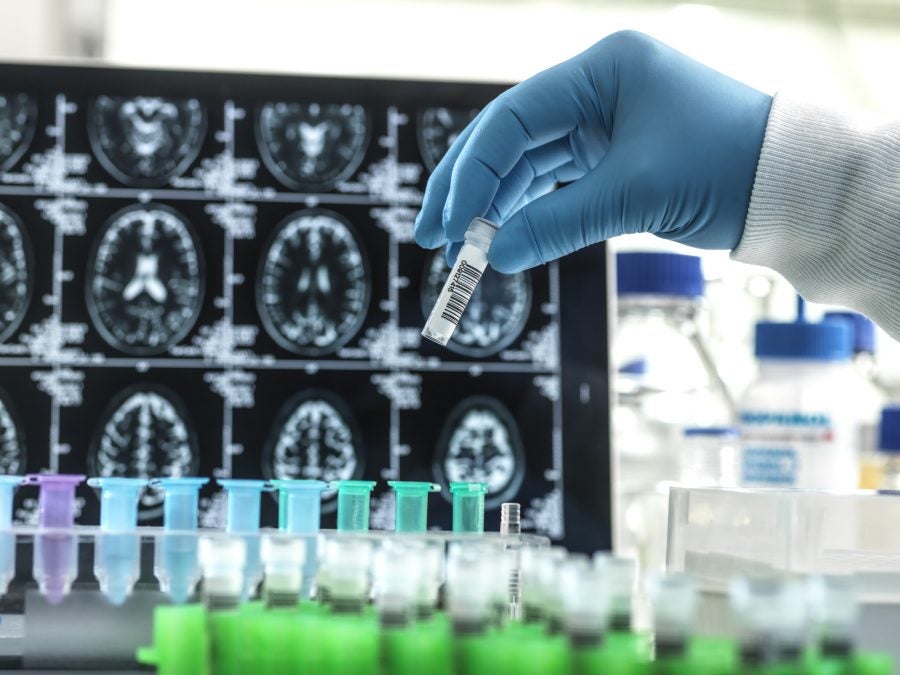
Inflammation, cell metabolism and neuronal health are current key areas of focus for dementia researchers, according to Jane Osbourn, chief scientific officer (CSO) at Alchemab.
Osbourn was speaking on a panel discussing novel approaches to treat neurodegeneration at the 2024 Anglonordic Life Science Conference being held in London, UK on 18 April.
Go deeper with GlobalData

Dementia Associated With Alzheimers Disease Drugs in Development by...
Premium insights.
The gold standard of business intelligence.
Find out more
She outlined that the past five to 10 years have led towards a better understanding of dementia’s causes.
“Obviously one cause is toxic protein aggregation, which has been a hypothesis for decades and is being addressed, but we’re starting to see more understanding of other causes such as inflammation, cell metabolism and neuronal health, and I think those are the areas that will now form the next wave of products that can then be used in combinations.
“I think understanding the causes, and what we are doing as an industry about them – such as whether we can do anything about neuronal health or making the brain more resilient against inflammation – are just some of the questions under consideration at this time.”
On the collaborative nature of being able to get cohorts together, Osbourn added: “I think in recent times there’s been a step change in collaboration and consortia, and getting patients to enable biomarkers, diagnosis and trials, which is going to really help in gaining further insights.”
How well do you really know your competitors?
Access the most comprehensive Company Profiles on the market, powered by GlobalData. Save hours of research. Gain competitive edge.
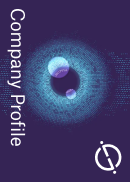
Your download email will arrive shortly
Not ready to buy yet? Download a free sample
We are confident about the unique quality of our Company Profiles. However, we want you to make the most beneficial decision for your business, so we offer a free sample that you can download by submitting the below form
Osbourn highlighted the impact of ageing dementia populations.
“Ageing is a risk for neurodegeneration, and we’re all becoming much more aware of the significance of that in terms of the impact on patients, carers, and society. I think there’s a massive societal risk around the dementia population ageing in terms of how they integrate with society.
“Society is built for people who are able, have memory, can use online tools – and one of my personal concerns is around a need to look more closely at how we are supporting people through dementia; not just by drugs but in a more holistic way.”
Speciality pharmaceutical group Essential Pharma recently completed the acquisition of Reminyl oral capsules from Johnson & Johnson company Janssen Pharmaceutica.
Reminyl is an established central nervous system (CNS) drug for mild to moderately severe dementia of the Alzheimer type.
Sabirnetug is a humanised, monoclonal antibody under clinical development by Acumen Pharmaceuticals and currently in Phase I for Dementia Associated With Alzheimer’s Disease.
Sign up for our daily news round-up!
Give your business an edge with our leading industry insights.
More Relevant
Ftortiazinon by gamaleya federal research center of epidemiology and microbiology for urinary tract infections: likelihood of approval, sofnobrutinib by carna biosciences for skin inflammation: likelihood of approval, sabirnetug by acumen pharmaceuticals for dementia associated with alzheimer's disease: likelihood of approval, 2x-111 by smerud medical research international for human epidermal growth factor receptor 2 positive breast cancer (her2+ breast cancer): likelihood of approval, sign up to the newsletter: in brief, your corporate email address, i would also like to subscribe to:.
Pharma Technology Focus : Pharmaceutical Technology Focus (monthly)
Thematic Take (monthly)
I consent to Verdict Media Limited collecting my details provided via this form in accordance with Privacy Policy
Thank you for subscribing
View all newsletters from across the GlobalData Media network.
Scientists Identify Link Between Sitting and Dementia
N ew insights into the medical mysteries behind dementia have been revealed this week, with two studies identifying drivers of the brain-degenerating condition.
One study, released on September 11 in the journal General Psychiatry , shows that the shortening of little caps on the end of chromosomes may be linked to increased dementia risk. Another, published in the journal JAMA on September 12, reveals that spending more time sedentary, such as sitting down, may also increase the risk.
These studies may help scientists to further understand the mechanisms behind what causes dementia to develop, and therefore how to stop it.
How Aging Is Linked to Dementia
Dementia is a term for the symptoms of a decline in brain function, as a result of damage to or changes in the brain. Alzheimer's disease is a form of dementia, caused by an abnormal build-up of two types of protein: amyloid and tau. One of the major drivers of dementia development is age, as it is much more common in the elderly.
This may be somewhat explained by the findings of the General Psychiatry paper, which discovered that dementia risk increases with the shortening of the telomeres. These telomeres are little caps on the ends of chromosomes that prevent the functional genes from being lost during replication. As a person ages, their telomeres become shorter and shorter as a result of many years of cell division and chromosome replication.
According to the paper, data from UK Biobank—a large biomedical database—reveals that of patients between the ages of 37 and 73, those with shorter telomeres in their leucocytes (a type of white blood cell) were 14 percent more likely to be diagnosed with dementia than those with the longest telomeres, and 28 percent more likely to be diagnosed with Alzheimer's disease.
"We found that LTL [leucocyte telomere length] acts as an aging biomarker associated with the risk of dementia," the authors wrote. "Furthermore, we also observed linear associations of LTL with total and regional brain structure. These findings highlight telomere length as a potential biomarker of brain health."
"Since LTL is largely inherited, individuals who inherit shorter LTL may be predisposed to dementia, making LTL an appealing predictive biomarker for dementia. In addition, shorter LTL is widely regarded as an indicator of poorer neuropsychological condition, so measurement of LTL might be considered as an option offered to the public to motivate healthy lifestyle choices in the general population."
How Sedentary Behavior is Linked to Dementia
The JAMA paper reveals that another driver of dementia may be a sedentary lifestyle.
Also using UK Biobank data, as well as accelerometer data from study participants, the researchers found that people aged over 60 who spend more than 10 hours a day engaging in sedentary behaviors, such as sitting, are at a higher risk of dementia compared with those who are sedentary for less time.
"The link between sedentary behavior was nonlinear, so that at lower amounts of sitting time, there was no significant increase in risk," study author David Raichlen, professor of biological sciences and anthropology at the USC Dornsife College of Letters, Arts and Sciences, told Newsweek . "After about 10 hours per day of sedentary behavior, risks increased significantly. Ten hours per day of sedentary behavior was associated with an 8 percent increase in risk of dementia and 12 hours per day was associated with a 63 percent increase in risk of dementia."
Sedentary behavior was defined by the authors as any waking behavior characterized by "a low energy expenditure while in a sitting or reclining posture," Raichlen said.
"We were surprised to find that the risk of dementia begins to rapidly increase after 10 hours spent sedentary each day, regardless of how the sedentary time was accumulated," study author Gene Alexander, professor of psychology and psychiatry at the Evelyn F. McKnight Brain Institute at the University of Arizona and Arizona Alzheimer's Disease Research Center, said in a statement.
"This suggests that it is the total time spent sedentary that drove the relationship between sedentary behavior and dementia risk, but importantly lower levels of sedentary behavior, up to around 10 hours, were not associated with increased risk."
They also found that even if this sedentary time is broken up by periods of activity, it's only the total time spent sitting that appears to have an impact on dementia risk.
"Many of us are familiar with the common advice to break up long periods of sitting by getting up every 30 minutes or so to stand or walk around," Raichlen said in the statement. "We wanted to see if those types of patterns are associated with dementia risk. We found that once you take into account the total time spent sedentary, the length of individual sedentary periods didn't really matter."
The exact reason why sedentary lifestyles may be linked to dementia risk is unclear, the authors said, with more research being required to fully understand the mechanisms behind this association.
"Our study was not focused on mechanisms but it is possible that reductions in cerebral blood flow or links between sedentary behavior and cardiometabolic disease factors may play a role in increased risk for dementia. Future work will focus on identifying these mechanisms," Raichlen said.
The link between telomere length and dementia also needs more research, the General Psychiatry authors explain, including looking at telomere length in other cell types, and how dementia risk changes with changes in telomere length.
"Several limitations must be taken into account," the authors wrote. "LTL was measured only once at baseline in nearly 470,000 participants. Based on the results of the current study, we were unable to identify whether changes in LTL impact the chances of dementia development.
"Dementia diagnoses were obtained from electronic health records only, so some dementia cases may not have been fully covered; likewise, we inevitably omitted some undiagnosed dementia and less severe dementia cases as they might not have been mentioned in the electronic health records," they said. "Finally, given the nature of an observational study design, conclusions of causality should be made with caution."
Do you have a tip on a science story that Newsweek should be covering? Do you have a question about dementia? Let us know via [email protected].
Related Articles
- Earth May Now Be Outside the 'Safe Operating Space' for Humans
- MIT Neuroscientist Shares Exercise They Do to Improve Memory
- Gastroenterologist Shares the First Thing He Does When Patients Are Bloated
- China Aims To Replicate Human Brain in Bid To Dominate Global AI
- Scientists Link Ultraprocessed Foods to Depression
Start your unlimited Newsweek trial
- History and Mission
- Structure and Cores
- Annual Report
- Media Guide
- Guiding Principles for Diversity
- Land Acknowledgement
- Volunteer for a study
- Open Studies
- Brain Donation
- Classes and Series
- Precious Memories Choir
- Mind Readers Book Club
- What is Alzheimer's disease?
- COVID-19 Brain Health Resource Guide
- Resources for People with Dementia and Care Partners
- How to get a diagnosis
- Raising awareness of Alzheimer’s disease in Wisconsin’s Indian Country
- Dementia Matters
- Dementia Matters COVID-19 Special Series
- Biomarker videos
- Request a Talk
- Professional Education
- Black Leaders for Brain Health
- Research Services and Resources
- Apply for Resources
- Data Dictionary
- Developmental Projects
- Acknowledging the Center
- IEA Innovation Fund
- Funded Pilot Grants, 2006-2019
- Internal Center Links
- Referring Patients to Research
- Updates in Dementia Care E-newsletter
- Dr. Daniel I. Kaufer Lecture
- How to join a research lab
- REC Scholar Program
- Research Day 2024
- Junior Fellowship
- Professional Development Award
- Lightning Funding Award
- Health Reporting Internship
Addressing higher Alzheimer's rates in African Americans
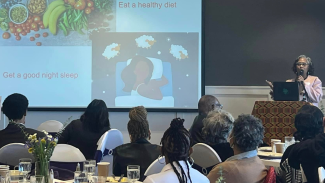
Nearly 200 people attended the annual Solomon Carter Fuller Brain Health Brunch on April 6, 2024, at TPC Wisconsin in Madison. The yearly event aims to build awareness of Alzheimer’s disease in the African American community. Several studies have shown Alzheimer’s disease hits African Americans at a higher rate than other ethnic and racial groups, with African Americans experiencing Alzheimer’s disease at up to twice the rate as white Americans.
The educational event is named after Dr. Solomon Carter Fuller, the first African American psychiatrist and a pioneer in Alzheimer’s disease research. Dr. Fuller worked closely with Dr. Alois Alzheimer in Germany in the early 1900s and wrote some of the earliest and most comprehensive scientific papers published in English about dementia.
The Precious Memories choir performed at this year’s event, and actor Esun Morales portrayed Dr. Solomon Carter Fuller for photo ops with attendees at a vintage photo booth.
Dr. Lisa Barnes, the Alla V. and Solomon Jesmer professor of gerontology and geriatric medicine within the Department of Neurological Sciences at Rush University in Chicago, offered the keynote address.
The Wisconsin Alzheimer's Disease Research Center (ADRC) and the African Americans Fighting Alzheimer's in Midlife (AA-FAIM) study presented the event.
“ Alzheimer's Disease Research Center holds annual Alzheimer's Brunch ,” aired on WKOW 27 on April 6, 2024.
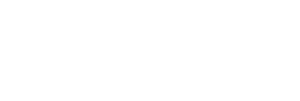
Information For
Participants & Families Brain Donors & Families Caregivers Researchers Providers Media Employees
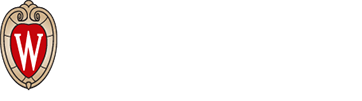
Contact Us Join a Research Lab Subscribe to E-news Give

This website may not display properly if you are using any version of Internet Explorer Feedback, questions or accessibility issues: [email protected]
Privacy Notice | © 2024 Board of Regents of the University of Wisconsin System

An official website of the United States government
The .gov means it’s official. Federal government websites often end in .gov or .mil. Before sharing sensitive information, make sure you’re on a federal government site.
The site is secure. The https:// ensures that you are connecting to the official website and that any information you provide is encrypted and transmitted securely.
- Publications
- Account settings
Preview improvements coming to the PMC website in October 2024. Learn More or Try it out now .
- Advanced Search
- Journal List
- Palliat Care Soc Pract
A UK qualitative study of living and dying with dementia in the last year of life
Jacqueline crowther.
Academic Palliative and Supportive Care Studies Group (APSCSG) and Primary Care and Mental Health, University of Liverpool, Liverpool, UK
Siobhan Horton
St Luke’s Hospice, Winsford, UK
Kenneth Wilson
Department of Psychiatry, University of Liverpool, Liverpool, UK
Mari Lloyd-Williams
Professor, Primary Care and Mental Health, Liverpool Health Partners and University of Liverpool, Brownlow Hill, Liverpool L69 3GB, UK
Background:
Dementia is a life-limiting illness, but the trajectory of dying can be difficult to establish and care at end of life can be variable and problematic.
This UK study was carried out to explore the end-of-life-care experiences of people with dementia from the perspective of their family carers. In-depth interviews were conducted with 40 bereaved family carers of people with dementia.
Forty family carers (male n = 9, female n = 31) age range: 18–86 years were interviewed. Issues with poor communication were common. The hard work of caring and issues regarding unpredictability of living and dying with dementia were also commonplace within the study. Only three patients were referred for specialist palliative care support at the end of life, all of whom had a dual diagnosis of dementia and cancer.
Conclusion:
This qualitative study has identified that there are several gaps in the end-of-life care of people with dementia, and frequently, there is poor communication during the last year of life. The need for high-quality integrated care for people dying with dementia with appropriate support during the last year of life is identified. COVID-19 has disproportionately affected people with dementia, and in the post-pandemic era, there is an urgent need to ensure every person dying with dementia is supported to die in their preferred place and that family members are supported and enabled to be treated as the ‘expert’ in terms of their knowledge of their relatives’ care and preferences.
What is already Known About the topic
- Dementia is a life-limiting illness, but the diagnosis of dying can be difficult.
- Many patients die with dementia in hospital or in care homes.
What this paper adds
- This qualitative study of family carers revealed that communication with the person with dementia and also with professionals was a major issue.
- Family carers felt worn down by the constant ‘battles’ they perceived they experienced in negotiating the healthcare system on behalf of the person with dementia.
- Dying of dementia was traumatic for family carers of those interviewed with little support and privacy and frequently no support from specialist palliative care.
Implications for practice theory or policy
- This study suggests that specialist or generalist palliative care is frequently not available for those with dementia dying in hospital or in care homes.
- Sensitive communication and respect for family carers is a basic necessity of good end-of-life care.
- Where advance care plans are not in place, family carers should be consulted and considered within the clinical decision-making process
Introduction
It is estimated that there are approximately 820,000 people with dementia in the United Kingdom, 1 and the incidence and prevalence of dementia will continue to rise with an ageing population. It is predicted that deaths from dementia in the United Kingdom will increase from around 59,000 per year in 2014 to around 220,000 per year by 2040. 2
Research suggests that people with dementia receive poorer end-of-life care, 3 – 5 inadequate pain relief, and limited access to palliative and hospice care. 6 – 9 Studies exploring end-of-life care for people with dementia have focused mainly on 24-h care environments, that is, hospital, nursing, and residential care, and frequently, the focus of research has been with paid carers and their understanding and approach to care towards the end of life. There is relatively little research and literature exploring personal experiences of family carers who have cared for a loved one with dementia up to and including death. Dementia is a challenging illness, and in the later stages, there is not only cognitive deterioration but also physical deterioration in terms of mobility, issues with nutrition, faecal and urinary incontinence, and in the later stages becoming bedfast.
In a study to explore decision-making at the end of life, interviewing carers and professionals, uncertainty and poor communication were reported as the main areas of difficulty. 10 A further qualitative study of professionals, along with people with dementia and family carers, reported seven key areas namely timely planning of discussions around end of life; recognition of patient being at the end of life and provision of supportive care; co-ordination of care; effective working relationships with primary-care teams; managing hospitalisation; continuing care after death; and valuing staff and ongoing learning. 11
Longitudinal studies are particularly valuable to capture experiences at different time points and a study from the Netherlands 12 to determine what should be available to enable good palliative care for people with dementia at the end of life, interviewed 10 family carers of people with dementia in focus groups at three time points. Family carers wanted familiarity at the end of life of having familiar people caring for the person with dementia and also wished care to be at home if at all possible and communication with professionals that they knew and trusted.
The aim of our study and this paper was to explore the direct personal experiences of a diverse group of family carers who had cared for people with dementia in the last year of life and up to death.
We report additional analysis of our national study 4 which recruited bereaved family carers from Scotland, England, and Wales. Participants were recruited via organizations, for example, Alzheimer’s Society and Age UK, social media, and community networks in order to obtain a broad range of experiences of care during the last year of life, and this paper reports additional analysis from the original study. As data collection and analysis progressed recruitment became more focused on certain under represented groups and locations, that is, male carers, semi-rural and rural areas. To facilitate this process, organisations such as the Alzheimer’s Society, Age UK, and Alzheimer’s Scotland were contacted and invited to publicise the appeal through newsletters and carers groups held within local branches. Full ethical approval from University of Liverpool ethics committee was obtained (RETH000206). Inclusion criteria included unpaid carer of someone with dementia in their last year of life and at the time of death; aged 18 years or over; death to have occurred within the last 5 years in any care environment (family home, care home, and hospital). Exclusion criteria included paid/formal carers; under 18 years of age; death occurred more than 5 years previously.
Family carers were invited to contact the first author if they were interested in participating in the study and were sent an information pack which included an information sheet and reply letter to invite the first author to contact them. Following written consent being obtained, interviews were conducted with participants and digitally recorded and transcribed verbatim. Interviews lasted between 30 and 80 min. Interviews were conducted face to face usually at the participants home, with a small number being conducted by telephone. The interview process was informed by narrative interviewing, 13 with the unstructured narrative interview designed to provide an opportunity for the participant to give a detailed account of a particular experience, event, or point in time.
Participants were encouraged to tell ‘their story’ as a narrative with minimal interruption from the first author which ensured events and issues important and significant to the participant were reported. The participants were encouraged to talk about the diagnosis and illness trajectory; what support was offered and how effective was support in the last year of life; were palliative care needs addressed; to discuss what happened in the last few weeks of life. This method was utilised in preference to a semi-structured interview design would have only allowed participants to select isolated parts from their own story and in effect filling in or completing pre-existing narratives by the first author. Field notes were collected to supplement the interpretation and analysis. The study was ongoing for 3 years with 18 months of this time spent on data collection. This prolonged period of time in the field helped the researcher who was an experienced mental health practitioner to acclimatise to researching an area of extreme sensitivity. It also supported development of skills that would help with conducting and understanding interviews and facilitated reflexivity which contributed to incorporating issues and theories from previous interviews into subsequent interviews. The field notes added to the context, nature, and circumstances of the interviews carried out.
During the research process and throughout interviews, the researcher attempted to create a climate of openness with participants in an attempt to reduce researcher effect. All participants were fully aware of the nature and purpose of the study. All transcripts were returned to the participants for verification and to ensure that participants’ narratives were recorded correctly. There were no requests for amendments following this process; however, many participants stated they had valued taking part and appreciated having a copy of their interview.
The study adopted a qualitative approach informed by phenomenology 14 and grounded theory. 15 , 16 Phenomenology as a philosophical stance due to the lived experiences being sought and grounded theory informed the study in an attempt to interpret and explain these experiences.
Analysis of the data using comparative analysis 15 commenced from the outset of data collection and transcripts were read by all authors and re-read by the first author. Line-by-line coding of transcripts, memo writing, development of categories, and emergence of themes followed and emerging themes were compared, discussed, and agreed by all authors who were part of the study team. Data saturation began to occur when recruitment reached 35 participants and a further five participants were recruited to ensure saturation had been fully achieved.
Participants were recruited from the North West of England: n = 21, North East of England: n = 4, Wales: n = 9 and Scotland: n = 6 and included participants living in urban, rural, and semi-rural areas. Thirty-one female and nine male family carers who had cared for people with dementia with an age range of 69–96 years (mean age 81.5 years) participated and included all socio-economic backgrounds. Efforts were made to recruit family carers where death had occurred in different care settings; however, the majority of participants had experienced death in hospital or care home with only four participants caring for a person with dementia within their family home until the end of life. No deaths had occurred in a hospice. Within the study, 22 people with dementia had died in Hospital, 14 within care homes and four within the family home ( Table 1 ) and the majority of family carers had experienced care in several different care settings in the year prior to death. Participants described how they had cared for the person with dementia for between 18 months to 20 years before death with a mean of 5.1 years and median of 5 years in a caring role. The deaths had occurred between 3 months and 5 years (mean 1.7 years, median 1.3 years) prior to the interviews.
Information regarding participants (reused with permission from Crowther et al. 17 ).
The mean length of time from formal diagnosis to death was 4.5 years; however, most participants reported cognitive problems for some time prior to diagnosis. Four participants were family carers for people who had a dual diagnosis of cancer and dementia. Of these, three experienced specialist palliative care services: one in a day hospice: one hospital-based and one a community-based specialist palliative care service. From the evidence of the interviews, no patient with a dementia-only diagnosis received specialist palliative care.
As a result of constant comparison of individual transcripts, 23 categories emerged from the data. Over a period of time, data were reduced further, and the following key themes began to emerge: communication; family carers as experts; the hard work of caring; and living and dying with dementia in the face of uncertainty. An example of an interview coding is included in Table 2 .
Examples of initial coding from text and memos.
Communication
Difficulties with communication in its broadest sense were a major theme to emerge from this study. Family carers knew the person and had established non-verbal means of communication
I knew when she was cold she would physically put her arms together and when she was too hot she would be unbuttoning her blouse I tried to get the staff to understand we had to look for non-verbal . . . when they don’t speak at all you’re having to work out what their needs are. (Family carer 36)
Family carers searched their creative knowledge of their loved ones in attempts to communicate and connect with the enduring self. This would involve playing music or having certain activities on a television channel they knew the person with dementia once enjoyed.
there was nothing we could give him, he had a television, we put it onto what we thought he enjoyed . . . in the summer he always liked to watch cricket we would tend to put that on . . . he liked hymns, certain singers, we tried to do this. (Family carer 39)
Just ‘being’ with the person with dementia became increasingly important for some family carers as the disease advanced and communication skills diminished.
Visiting . . . I couldn’t wait to get there . . . I was there every afternoon all afternoon for a fortnight . . . I went in everyday I held her hand . . . she knew me — I held her hand. (Family carer 40) I’d stay right through to evening . . . sit with mum just sit . . . talk to her . . . put the tv on . . . put some music on or I’d just sit there . . . (Family carer 31)
Communicating about care issues as the person with dementia deteriorated was another source of distress. The language used to inform family carers that the person with dementia was in the terminal phase was not always clear and added to the chaos and uncertainty experienced.
‘he’s very, very poorly, still very poorly’ they said. (Family carer 30)
Occasionally, a deterioration was reported and interpreted to mean the person with dementia was close to death. There appeared to be a subliminal message for family carers within the words used.
“about half past ten the staff nurse rang B and said “her breathing’s become a bit distressed” I said “right I’ll come now.” (Family carer 22)
A number of participants expressed concerns in relation to different languages and different cultures within UK hospitals and care homes. The quote below was the experience of a daughter whose father was Polish and reverting to his native language as dementia progressed – he had attended a memory clinic in the last year of life and the following were the daughter’s recollection of the clinic visit
he couldn’t write his name . . . my dad was Polish- the doctor was from another country . . . you can imagine . . . it were like never the twain shall meet. She wouldn’t let us stay in the room with him while she asked him these questions and we’d have to stand outside, we could hear what she was saying and she was getting quite agitated my dad wasn’t responding . . . he didn’t know how to respond . . . basically she wasn’t interested in him . . . she said there was no point giving him any tablets. (Family carer 21)
As dementia progresses, people often revert to the use of their native language and may totally loose their skills to speak or understand their second or third language. The importance of being able to communicate in the native language in order to facilitate assessment of cognitive deficits is demonstrated below.
she was lovely and spoke Welsh to him, she went through this rigmarole of questions and drawing then she said “will you write me a sentence?” . . . I thought “what’s going to happen here?” he said “of course I will” he picked up the paper, he wrote a sentence in Welsh do you know what that sentence was translated?.” it’s wonderful to have a young lady like you to visit me. (Family carer 39)
As dementia progressed, the ability to converse became more limited, and some family carers went to great lengths to choose 24-h care facilities which employed staff who could speak the native language of the person with dementia.
I really wanted him to go to a Welsh speaking care home I wanted people there who spoke to him in his mother tongue, that was very important. Not all of them did speak Welsh, but most of them understood Welsh and would know enough words to communicate. (Family carer 39)
Informal family carers as experts in care
Family carers within the study believed they were the “experts “ in care and in care preferences of their relative – in most cases, they had lived with, known, and cared for the person with dementia for many years prior to diagnosis and had witnessed the changes in the person with dementia following the diagnosis. Participants expressed specific wishes in relation to aspects of care. Informal family carers believed their level of knowledge and skill acquired, over many years of caring, was devalued, negated, and frequently ignored by professionals. Some expressed explicit wishes relating to the care of the person with dementia. The following quote was a patient with dementia and a cancer diagnosis – his wife found it unbearable that the oncologists insisted on informing her husband of his diagnosis at every clinic visit. Due to his dementia, he had no memory of previously being told his diagnosis but had sufficient insight to be devastated each time he was told his cancer diagnosis.
it was my belief he’d not got the mental capacity to know, every time he was told it would be like telling him again. She (oncologist) was really quite . . . not aggressive . . . it was her opinion he should know that was it I think relatives know - well I knew my husband better than anybody else, we’d been married for forty five years and it wasn’t appropriate to tell him . . . (Family carer 32)
As is demonstrated in the quote below, one of the most complex but important areas faced in caring for those with advancing disease is deciding when and how far to intervene when patients deteriorate. Including family carers in such decisions and listening to their views, where possible, can support the decision-making process and help arrive at solutions based on knowledge of that person and what maybe in their best interests.
they said about feeding him through his nose, I said “no” I knew he’d be traumatised, I knew he’d pull it out, he wouldn’t like being held down having that done so I said “absolutely no, no way that” When I go back in the afternoon they’d done it, he’d been very traumatised, I was furious. (Family carer 07)
The difficulty for family carers in hospitals when staff did not take notice of family carers’ views is illustrated below from the daughter of a patient who was sectioned (detained in a psychiatric hospital against their will) and admitted to a psychogeriatric ward
. . . he got put into ward X when he was sectioned, . . . “you’re in here Mr. H because you’ve hurt your wife” and I used to think, why are they saying that to him–how can you say that to somebody whose mind is like that . . ., It would make him so angry and he’d say “I do not, I do not hurt my wife!” you know and I feel me dad really, really suffered because he would not have hurt a fly and to keep being told . . . He’d say “this is punishment” he’d sit on ward X with his head in his hands and say “ ooh, they’ve said I’ve hurt P (wife), I love P, I’d never hurt P” you know and they were telling him all the time. It was terrible, it was absolutely dreadful and then he went into the nursing home thank God but I do feel for these people who haven’t got any family–what happens to them? (Family carer 09)
The hard work of caring
All interviews were retrospective accounts of events which had occurred months or some years previously, but recollections were vivid and emotional. Powerful words such as “fight,” “battles,” and “traumatic,” illustrated the difficulties confronting families and the hard work involved. Choice of words and language used were indicative of the enduring effect of the experiences and memories for the study participants.
then began the battle to get him out of hospital, it was a battle . . . they knew I wanted to get him home as soon as possible, it took 21 days to get him home, they told me it’s within the time limit. I pointed out to them S died 21 days after he came home so it may have seemed within their time limits, but it wasn’t within our time limits . . . we lost valuable time where he could have been at home didn’t we? (Family carer 32) I’d have to put him to bed, I think as a daughter, I think that’s been one of the hardest things which I have got over now, it would have made me cry so much it was just so upsetting. This fantastic man who’d done so much for so many other people, just become this shell terribly frightened, he just didn’t know where he was, kept asking for his own mother, I think quite a few times he thought I was his mum putting him to bed which for a daughter to think your father thinks you’re his mother was so very, very upsetting . . . (Family carer 03)
There was also the difficulties perceived by the attitude of hospital staff towards patients with dementia and pressure to move patients quickly to a care home. This very event for many families was traumatic, and the importance of going to a care home the family had chosen and wanted was important as illustrated below
. . . there was quite a bit of pressure actually from the local NHS here basically to get him out which I resisted because I wanted him to go into this particular nursing home, they wanted us to put him somewhere else . . . and wait for a bed . . . I felt that it was much better in one stage. (Family carer 39)
Living and dying with uncertainty and unpredictability – the last days
The level of uncertainty family carers of people with dementia may experience as death approached or appeared to approach caused distress to many family carers. Although told many times that a person with dementia may be near death, death could sometimes occur suddenly and unexpectedly. Uncertainty and unpredictability persisted sometimes over many months and occasionally years. Some family carers found themselves in situations where they were advised by medical staff the person with dementia was close to death only to see them recover, and for some, this occurred on several occasions during the disease trajectory and was a source of considerable anxiety and distress.
doctors were saying “he’s very poorly”, she was saying “you don’t know my dad, I’ll come in tomorrow, he’s going to be as right as rain, he’ll be off this mask” They were sort of like “right, right ok” that’s what happened to us so many times, then it got to Monday morning they said “he’s not going to last 24 hours. . . . (Family carer 20) he was a fighter that time he went in with pneumonia he wasn’t expected to come out . . . but he did. (Family carer 37)
Most family carers experienced death within a hospital setting and although for some, the care experience was good, it was common for people with dementia to die on an open busy ward with the promise of transfer to a side room not materialising.
it was horrible the way me dad died, absolutely horrible . . . it was dreadful . . . if he’d have been in a side room . . . had a little bit of dignity where it could have been quiet . . . (Family carer 9)
Of the four patients who had a dual diagnosis of cancer and dementia, one participant reported that her mother who had lung cancer and dementia had received day care in a local hospice while at home but died in a care home. Individual experiences of specialist palliative care services varied with one referral to a hospital team advising the family they were unable to help – this person died at home 4 weeks later. Only one family experienced palliative care in a hospital setting for a patient who had a diagnosis of gastric cancer and dementia. This participant was the only participant to mention the possibility of her father going to a hospice for end-of-life care, although this did not happen and he died on a hospital ward.
The one thing that I would have liked is for him to perhaps not have to stay so long in a clinical setting, he didn’t like hospitals at all and possibly, I think, we did discuss this with the doctors. . . . I think they thought that it wasn’t worth arranging to move him to a hospice or whatever because he wouldn’t survive . . . (Family carer 7)
Our study aimed to explore the experience of dementia in the last year of life in a diverse group of bereaved family carers from the UK in an attempt to identify and interpret what were the specific perceived needs of people with dementia and of their families at the end of life. Although a retrospective account, in all cases, carers gave an emotional and vivid account of events leading up to the death of the person with dementia. Communication in its broadest sense was the overarching issue of concern within this study and communication impacted on every aspect of care at the end of life.
As previously reported 18 , 19 communication issues were the main areas of concern in this study and were perceived as problematic by family carers in a range of circumstances, environments, and contexts and influenced every experience of care received. It was evident how difficulties with communication impacted on care and in this study, the experiences of communication were mostly negative. Non-verbal messages such as body language or para-verbal messages including tone and volume of voice have equal importance to verbal components of communication. 20 All aspects of communication influenced family carers’ experiences of end-of-life care. It is acknowledged that for many who work in health and social care in the United Kingdom, English is not their first language. Family carers experienced difficulties communicating with professionals in some circumstances and expressed concerns regarding the person with dementia who already had compromised communication skills as a result of their illness. For Welsh speaking family carers in Wales who participated in the study in particular, there was concern regarding the lack of access to support and care within their own native language and lack of access to care that was culturally acceptable. Sensitive communication and respect for family carers is a basic essential of good end-of-life care as is ensuring that family carers understand what is being said and listening to the family carers views and opinions.
Carers as experts
An expert is defined as ‘a person who is very knowledgeable about or skilful in a particular area’. Development of competent skills and extended knowledge about a particular subject, place, issue, or person is crucial to being assigned the title ‘expert’. There appeared to be a combination of experts in this study. Those who were professionals providing a service in which they were viewed as experts by lay people (i.e., the family carers interviewed for the study) and lay people (the family carers themselves) who considered themselves as experts in the care of the person with dementia. There was evidence of conflict and criticism mainly attributable to lack of acknowledgement and value perceived to be placed upon informal family carers expert knowledge and skills by professionals. Family carers felt they knew the person with dementia extremely well and based proxy decisions and choices on vast prior knowledge. They felt qualified as experts in care and found themselves, at times, in conflict and confrontation with professionals. Family carers considered themselves as expert in terms of knowledge and care of their loved ones 21 and what was in their best interests, while professionals appeared to consider themselves the experts in relation to dementia, disease trajectory, symptom management, and treatments. Families of people with dementia have frequently been living with the condition for a number of years prior to diagnosis and may have been making decisions based on their knowledge and previous wishes expressed by the person with dementia. These may, or may not, have been documented in a care plan. It was clear from this study that key areas of decision-making which created difficulties for the expert family carers and professionals alike included artificial hydration and nutrition, communication, and hospital admission. 22
Two recent papers based on a qualitative study of 30 bereaved carers of patients who had died of Lewy Body Disease also reported the lack of communication between health care teams and families and difficulty predicting death timing were two frequently expressed challenges. 23 , 24 Within our study, carers perceived professionals as inflexible; policy and procedures appeared to be followed regardless of information shared by informal family carers. It should be noted, that in this further analysis of a retrospective study, none of the participants in the study had an Advanced Care Plan (ACP); 25 , 26 however, a recent paper 27 reported that of over 6000 patients presenting with an acute medical emergency, only 4.8% had an ACP available for admitting medical team. In the study, 27 although 9.5% of patients over 80 and 12.6% of patients over 90 had an ACP, it is clear that the vast majority of older people, many of whom will have dementia as primary or co-morbidity do not have any documentation to state their wishes. Advance care planning (ACP) is a process of reflection on and communication of a person’s future health care wishes; 28 however, the process itself can be challenging as a recent systematic review reported and uncertainty with decision-making requires strategies and support to aid this complex process. 29 Since introduction of the Mental Capacity Act in the United Kingdom in 2005, 30 there has been increasing focus on ACP and treatment directives. This is in anticipation of loss of capacity and ability to make informed choices regarding treatments and wishes as capacity is lost and death may be approaching. In dementia, timing is crucial, as the person with dementia can be involved at an earlier phase but not necessarily later due to cognitive decline; however, for people newly diagnosed with dementia, it can be difficult to anticipate future care needs. What appeared most challenging for family carers within this study was the fact they felt they were not being listened to nor recognised by professionals.
The effects and impact of being a family carer for people with dementia has already been reported in the literature. 31 , 32 It became apparent that there were different elements to the hard work of caring namely the practical hard work and organisation of care; the physical hard work and the caring tasks required on a regular basis to support daily living and the emotional hard work related to burden, stress, anxiety, and guilt. The constant ‘battles’ family carers described when trying to negotiate the world of professional care was a drain on their emotional resources and such situations impacted on family carers causing stress and distress. 33 These findings are also echoed by a study which found that bereaved and current carers found it exhausting to co-ordinate care for the person with dementia especially regarding end-of-life care. 34
Uncertainty has been described as a common experience during illness, and dementia is no exception. 35 , 36 It could be argued that dying with dementia may be challenged with more uncertainty due to the potential length and unpredictable nature of the disease trajectory. The narratives shared within this study appeared to be fraught with chaos. Nothing within the last year of life including death itself appeared to be ordered or predictable. Issues regarding prognostication and dying in life-limiting illness remain problematic, and transfer from nursing home to hospital at end of life is common. 37 Many people with dementia also have co-morbidities. 38 It was of note that specialist palliative care services were rarely involved, and involvement in this study appeared limited to those with a dual diagnosis of dementia and cancer. Palliative care services for people with dementia were and are being developed but remain limited 17 , 39 and the debate regarding early introduction of palliative care in dementia continues. Currently, in the United Kingdom, less than 1% of people with dementia in the United Kingdom die within a hospice. In the United Kingdom, Admiral Nurses who are specialist nurses supporting people with dementia and their families are working in many areas including acute hospitals and can support both families and staff who are caring for patients with dementia at the end of life. Our findings in this study echo those of Bolt et al. 40 who found family members wanted to be recognised as an important caregiver and that a lack of person-centred care was an issue for many family members throughout the dementia illness.
Strengths and limitations
To our knowledge, this is one of the largest qualitative studies exploring the needs of patients with dementia at the end of life from the perspective of a diverse sample of bereaved family carers. Although every effort was made to include people from different ethnic backgrounds, this was not possible which limits the extent to which findings can be applied in other populations. The self-selecting nature of the sample could represent family carers with a more difficult experience wishing to participate and to share their experiences and those family carers who wished to participate may have been more engaged in caring for the person with dementia than the average family carer, and their desire to be heard, therefore greater. Conversely, it is possible that people with worse experiences of end-of-life care may not have wished to re-live the experience in an interview and that those interviewed for the study were possibly portraying better experiences of care. This was a United Kingdom–based study, and we acknowledge that findings may not be generalizable to other countries where provision of care is different.
Conclusion and recommendations
This large qualitative study of family carers revealed that communication in its broadest sense with the person with dementia and with professionals was a major issue. Family carers felt worn down by the constant ‘battles’ they experienced in negotiating the healthcare system on behalf of the person with dementia. Dying of dementia was frequently traumatic for family carers with little support and privacy and no support from specialist palliative care. Despite many publications and research on palliative care and dementia, 41 it is still important that all research is based on the experience of patients or their family carers who at the end of life are the advocates and the voice.
This paper is being written as we hopefully begin to emerge from the COVID-19 Pandemic which has seen a disproportionate number of people with dementia die of COVID-19. COVID-19 has disproportionately affected people with dementia, and in the post-pandemic era, there is an urgent need to ensure every person dying with dementia is supported to die in their preferred place and that family members are supported and enabled to be treated as the ‘expert’ in terms of their knowledge of their relatives’ care and preferences.
Communication with family members and determining their views regarding care at the end of life is essential as is ensuring people with dementia can die in their usual place of care among familiar surroundings. Although there is a belief that an ACP appears integral to aid delivery of good end of life for people with dementia, their completion can be problematic and very few people currently have a completed ACP. In such situations, it is essential to discuss with and include close family carers as partners in the care of the person with dementia as they know the person. If end-of-life decisions have not previously been discussed, family carers will be able to advise what may be the best care based on prior knowledge of what the person with dementia may or may not want. It is unclear whether the increase in numbers of people with dementia dying at home in the United Kingdom will continue, but what remains clear is the need for excellent communication along with support and respect for carers acknowledging their expertise, which with co-ordinated care will allow those with dementia to die in comfort and with dignity in the location of their choice.
Acknowledgments
The authors thank St Luke’s Hospice Winsford for a research grant which supported this study, and they thank all the family carers who so generously gave their time to be interviewed.
Ethics approval and consent to participate: Full ethical approval was awarded to this study by the University of Liverpool ethics committee. The authors are accountable for all aspects of the work in ensuring that questions related to the accuracy or integrity of any part of the work are appropriately investigated and resolved. All data collected for this study was with the full informed consent of all participants. Full ethical approval from University of Liverpool ethics committee was obtained for this study (RETH000206).
Consent for publication: All family carers who participated in the study consented to the publication of anonymised qualitative data.
Author contribution(s): Jacqueline Crowther: Data curation; Formal analysis; Investigation; Methodology; Writing – review & editing.
Kenneth Wilson: Formal analysis; Project administration; Writing – review & editing.
Mari Lloyd-Williams: Conceptualisation; Formal analysis; Funding acquisition; Investigation; Methodology; Supervision; Writing – original draft; Writing – review & editing.

Funding: The authors disclosed receipt of the following financial support for the research, authorship, and/or publication of this article: Research grant St Luke’s Hospice Winsford, Cheshire, UK.
Conflict of interest statement: The authors declared no potential conflicts of interest with respect to the research, authorship, and/or publication of this article.
Availability of data and materials: Raw data as in qualitative interviews is available by contact with authors
Contributor Information
Jacqueline Crowther, Academic Palliative and Supportive Care Studies Group (APSCSG) and Primary Care and Mental Health, University of Liverpool, Liverpool, UK.
Siobhan Horton, St Luke’s Hospice, Winsford, UK.
Kenneth Wilson, Department of Psychiatry, University of Liverpool, Liverpool, UK.
Mari Lloyd-Williams, Professor, Primary Care and Mental Health, Liverpool Health Partners and University of Liverpool, Brownlow Hill, Liverpool L69 3GB, UK.
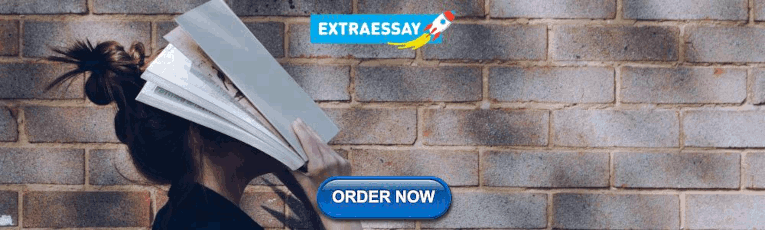
IMAGES
COMMENTS
INTRODUCTION. Dementia is a common public health problem. 1 Worldwide, approximately 47 million people have dementia and this number is expected to increase to 131 million by 2050. 1 Reductions in age-adjusted incidence of dementia have occurred in the United States (US) and other developed countries over the last 20 years, perhaps associated with increasing formal educational attainment.
The number of older people, including those living with dementia, is rising, as younger age mortality declines. However, the age-specific incidence of dementia has fallen in many countries, probably because of improvements in education, nutrition, health care, and lifestyle changes. Overall, a growing body of evidence supports the nine potentially modifiable risk factors for dementia modelled ...
1. Introduction. Alzheimer's disease (AD) (named after the German psychiatric Alois Alzheimer) is the most common type of dementia and can be defined as a slowly progressive neurodegenerative disease characterized by neuritic plaques and neurofibrillary tangles (Figure 1) as a result of amyloid-beta peptide's (Aβ) accumulation in the most affected area of the brain, the medial temporal ...
Dementia is a syndrome that involves severe loss of cognitive abilities as a result of disease or injury. Dementia caused by traumatic brain injury is often static, whereas dementia caused by ...
Alzheimer's disease (AD) is the most common form of dementia, and accounts for over 60% of cases. Aging is the biggest risk factor for dementia. According to the World Health Organization (WHO ...
Observations: Dementia is an acquired loss of cognition in multiple cognitive domains sufficiently severe to affect social or occupational function. In the United States, Alzheimer disease, one cause of dementia, affects 5.8 million people. Dementia is commonly associated with more than 1 neuropathology, usually Alzheimer disease with ...
Importance Worldwide, 47 million people live with dementia and, by 2050, the number is expected to increase to 131 million.. Observations Dementia is an acquired loss of cognition in multiple cognitive domains sufficiently severe to affect social or occupational function. In the United States, Alzheimer disease, one cause of dementia, affects 5.8 million people.
On 4 October 2022, the WHO released a dementia research blueprint to support implementation of the Global action plan on the public health response to dementia in 2017-2025, representing a first ...
In 2022, multiple research milestones have occurred in the field of dementia. Therapeutics went through a fundamental transition, with amyloid-β (Aβ) targeted drugs gradually moving from clinical trials into real-world application. Encouraging findings on biomarkers and risk factors also marked the ongoing shift towards precise diagnosis and early prevention.
Dementia, not a particular disease but rather a gathering of conditions which ascribes the debilitation of atleast two cerebrum capacities, cognitive decline and memory judgment has became ubiquous all over the world. Achieving accuracy and timely diagnosis is a major challenge in dementia detection. The recent advancements in neuroimaging techniques has setup some benchmarks. The amalgamation ...
Abstract and Figures. Dementia is a neurodegenerative disorder characterized by progressive and continuous loss of cognitive functions. The neuropsychiatric symptoms include apathy; agitation and ...
Modelling of the UK change suggests a 57% increase in the number of people with dementia from 2016 to 2040, 70% of that expected if age-specific incidence rates remained steady, 10 such that by 2040 there will be 1·2 million UK people with dementia.
psychologists who perform evaluations of dementia, MCI, and age-related cognitive change. Although the DSM-5 uses the terms mild neurocognitive disorder and major neurocognitive disorder, the broader research and practice literature typically uses the terms mild cognitive impairment and dementia, and this document follows that convention.
Four themes emerged from the research synthesis regarding what. intervention strategies can improve the quality of life for patients with dementia: 1) Validating the Patient's Sense of Self; 2) The Relationship between Caregiver and Patient. 3) The Impact on Patient's Daily Routine and 4) Depression and Mental Health.
Mild cognitive impairment and dementia: strategies for prevention. Evidence supports the existence of prodromal stages of dementia, including preclinical disease and the early clinical stage of MCI. 8,9 The hallmark of MCI is the presence of cognitive deficits beyond what is expected for age but not severe enough to cause disruption of daily life. 10,11 MCI represents a common prodrome of ...
The final set of selected papers consisted of 75 research papers, among these final selected articles, each of the data modalities (image, clinical-variables, voice) contained 25 papers. ... Aside from image-based ML techniques for dementia prediction, several research studies have utilized clinical-variable data with ML algorithms to predict ...
1 INTRODUCTION. Alzheimer's disease (AD) is a neurodegenerative disorder characterized by progressive cognitive and functional decline. Intervening at early stages of the disease is key to maximizing treatment benefit; thus, the development and testing of AD therapeutics targeted at the asymptomatic or "preclinical" stage of the disease holds much promise. 1-4 Preclinical AD refers to the ...
Here the authors present a deep learning framework for dementia diagnosis, which can identify persons with normal cognition, mild cognitive impairment, Alzheimer's disease, and dementia due to ...
It affects 1 in 2,900 births, and about 1,400 young Australians are currently living with childhood dementia. "This groundbreaking research has the potential to change the lives of children with ...
A total of 5,712 dementia-free participants at visit 5 (mean age 75.4 ± 5.1 years; 22% Black race; 58% female) were included in our analysis. The median follow-up was 5.5 years. The minimum cumulative PPI use was 112 days, and the maximum use was 20.3 years. There were 585 cases of incident dementia identified during follow-up.
Nurses in neurological wards face numerous challenges when caring for patients with dementia, particularly those who also present other acute illnesses. However, studies focusing on this area are limited. This study aimed to explore the difficulties and strategies in caring for patients with dementia among nurses working in a neurological ward.
Caring for elderly people with dementia is associated with well-documented increases in burden, distress, and decrements in mental health and wellbeing. More severe behavioural, cognitive, and functional impairments in a patient are associated with higher levels of burden and distress. Distress increases with care hours per week, number of tasks, and declining coping and support resources ...
Lancet. Commission on dementia prevention, intervention, and care life-course model of nine factors (less education, hypertension, hearing impairment, smoking, obesity, depression, physical inactivity, diabetes, and infrequent social contact). • Modifying 12 risk factors might prevent or delay up to 40% of dementias.
Dr. Barney Viengkhou, lead author of the new paper, stated, "The fact that we observed the treatment's effectiveness in halting and even reversing some neuropathological features in older mice with more advanced disease is particularly exciting." ... Head of Research at the Childhood Dementia Initiative. "There is an urgent need to ...
Inflammation, cell metabolism and neuronal health are current key areas of focus for dementia researchers, according to Jane Osbourn, chief scientific officer (CSO) at Alchemab. Osbourn was speaking on a panel discussing novel approaches to treat neurodegeneration at the 2024 Anglonordic Life Science Conference being held in London, UK on 18 April.
Dementia is a clinical diagnosis requiring new functional dependence on the basis of progressive cognitive decline. It is estimated that 1.3% of the entire UK population, or 7.1% of those aged 65 or over, have dementia. Applying these to 2013 population estimates gives an estimated number of 19,765 people living with dementia in Northern Ireland.
How Sedentary Behavior is Linked to Dementia. The JAMA paper reveals that another driver of dementia may be a sedentary lifestyle.. Also using UK Biobank data, as well as accelerometer data from ...
The educational event is named after Dr. Solomon Carter Fuller, the first African American psychiatrist and a pioneer in Alzheimer's disease research. Dr. Fuller worked closely with Dr. Alois Alzheimer in Germany in the early 1900s and wrote some of the earliest and most comprehensive scientific papers published in English about dementia.
Two recent papers based on a qualitative study of 30 bereaved carers of patients who had died of Lewy Body Disease also reported the lack of communication between health care teams ... Despite many publications and research on palliative care and dementia, 41 it is still important that all research is based on the experience of patients or ...
Midlife, not your 70s or 80s, is when brain changes start to occur that can pave the way toward dementia, Alzheimer's disease and cognitive decline later, according to a growing body of research ...