Biogeography.News
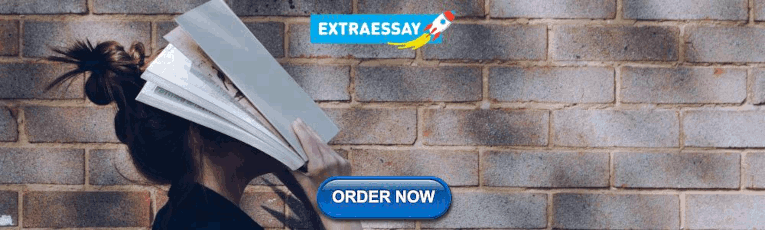
How to write a (great) Perspective article
Like many journals, Journal of Biogeography ( JBI ) provides a specific forum for researchers to put forward new ideas (or dismantle old ones). In JBI , this article type is the Perspective . Our Author Guidelines state that Perspective papers “should be stimulating and reflective essays providing personal perspectives on key research fields and issues within biogeography”.
Across the senior editorial board, we’re always a little surprised that we don’t get more Perspective submissions since most of the biogeographers we know are brimming with personal perspectives, many of which immediately spill out over a coffee, beer or zoom call. Of course, going from a good idea to a finished article is rarely straightforward and writing your first Perspective article can be a daunting prospect – even more so if English is not your native language.
The good news is that writing a Perspective can be exceedingly enjoyable and a refreshing change from the limitations of a standard research article. Moreover, it is not a ‘black box’; there are several general principles that can help you to craft ‘stimulating and reflective essays’. Like research articles, the best Perspectives have a clear U-shaped narrative (Figure 1) that start with a clear justification of why a research area/topic needs re-evaluating and finishing with the potential implications of your new perspective for the development of the field.

One of the best things about Perspective articles is you have enormous flexibility in how you write them. Nevertheless, when planning the article, we find it useful to divide the article into several basic components:
- The Introduction This ought to include an engaging explanation of the problem/challenge you are addressing (this can be conceptual, practical, methodological… anything really!). Generally speaking, the more important/fundamental the problem, the harder it is to convince the referees that your new perspective is valid! But the potential rewards are also greater, so give your best idea a go! Almost by default, you need to contrast your new perspective with the standard or alternative solution/model/explanation, i.e. the “text-book explanation” that most scientists would agree with. This standard explanation needs to be carefully layed-out without creating a ‘straw man’ (e.g. misrepresenting the alternative argument to make your argument look better)! Finally, introduce your new perspective and give a convincing explanation of why you think it is needed.
- Substantiating your new perspective It’s not enough to simply state your new perspective. You also need to provide convincing evidence in favour of, or at the very least consistent with, your argument, citing examples and demonstrating ways in which your new perspective can be applied. This does not need to be an exhaustive synthesis of relevant studies, but it should be sufficient to support your argument and to, at a minimum, demonstrate that existing approaches to the problem are insufficient. Be careful to not cherry pick the literature such that you selectively ignore evidence contrary to your view. Instead, embrace challenging data, and use them to explore limitations and possibilities.
- Conclusions After discussing the evidence it is important to outline the relative strengths of your new perspective as compared to the standard/alternative perspective and to discuss the potential implications of your approach for future developments in the field.
And don’t forget your figures! It’s a decent estimate that a picture is worth a thousand words. A sweet graphic demonstrating the differences between the conventional and your new improved approach will also be worth a whole pile of citations. So, having made a compelling intellectual argument in the text, don’t sell your idea short visually. Design an eye-catching intuitive graphic that’ll get included in social media, in other people’s talks, as well as future papers and text-books. (Advice on preparing figures can be found at https://journalofbiogeographynews.org/2020/07/15/figures-the-art-of-science/ .)
How to get started : When planning a Perspective paper (for any journal), consider starting with a simple plan, e.g. a bullet-pointed outline, that includes: (i) the problem; (ii) the standard approach; (iii) the new perspective; (iv) the key evidence, and; (v) the main conclusion. Of course, there are many other ways to structure an argument and experienced writers will often create a compelling narrative that doesn’t fit into a standard structure. The point is, a strong structure can be a huge help if you are unsure how to start, or to help organize your thoughts. Another tip is, if you’re unsure about the merits of an idea, write to the editorial board. Contact an associate editor in a closely allied field and write to the Reviews Editor, Richard Ladle, and/or the editor-in-chief Michael Dawson < contacts >. We’ll be happy to give you preliminary feedback and guidance.
We hope the short explanation above has shown you that writing a Perspective article is not fiendishly difficult or the preserve of well-seasoned biogeographers with long academic records. A new Perspective is as much about novelty and disruption as it is about experience. Here at Journal of Biogeography we believe that debate and discussion, diverse viewpoints and challenges to orthodoxy are essential if the discipline of biogeography is going to maintain its vibrancy and societal relevance. In this respect we encourage submissions from all biogeographers, but especially early stage researchers and those working in regions of the world historically under-represented in biogeography.
Written by: Richard Ladle Research Highlights Editor
Share this:
Published by biogeography.news.
Contributing to the growth and societal relevance of the discipline of biogeography through dissemination of biogeographical research. View more posts
7 thoughts on “ How to write a (great) Perspective article ”
Can I get to see a sample of perspective type news article, it will be a great help.
Thanks Pragya
Great Post!!! your article is very helpful for me . your ideas of worth are very useful and helpful for me.all the information for worth is very valid. Great post I must admit, keep sharing more…
Thank you for the writing tips.
- Pingback: 13 types d'articles scientifiques publiables - Methodo Recherche
Thanks for your guidance of perspective. — from a fresh neuroscientist
Very Helpful for Newbie here! Thank you! Godspeed!
- Pingback: What’s the Difference Between Journal Writing and Journalist? (Explained) – All The Differences
Leave a Reply Cancel reply
Discover more from biogeography.news.
Subscribe now to keep reading and get access to the full archive.
Type your email…
Continue reading
- Technical Support
- Find My Rep
You are here
New Perspectives
Preview this book.
- Description
- Aims and Scope
- Editorial Board
- Abstracting / Indexing
- Submission Guidelines
New Perspectives aims to provide interdisciplinary insight into politics and international relations, with a focus on Central and Eastern Europe (CEE). Based at the Institute of International Relations Prague , the journal provides a space for innovative perspectives coming from scholars working in the CEE region, and from global scholars engaged with the region and the wider issues that impact it.
New Perspectives encourages empirical, theoretical, conceptual and methodological innovations in scholarship on politics and IR, and aims to widen and deepen explanatory or interpretive frameworks. The journal sees pluralism with respect to approaches and viewpoints as the necessary condition for academic critique. New Perspectives is committed to the effective communication of high-quality original research to wider public audiences, and thereby seeks to foster the creation of useful knowledge, broadly understood.
Each volume centres on blind peer-reviewed research articles. The journal also welcomes review essays, interviews, auto-ethnographic commentaries, collaborative texts, and other non-traditional or creative formats of scholarship. New Perspectives is a member of the Committee on Publication Ethics (COPE).
New Perspectives seeks to attract submissions which address political aspects of regional affairs and their connections to the wider world, from the fields of: International Relations, Political Science, Security Studies and International Political Sociology; International Political Economy; Geography; Sociology; Anthropology; History; Cultural Studies and Legal Studies.
- Clarivate Analytics: Emerging Sources Citation Index (ESCI)
Manuscript Submission Guidelines: New Perspectives
Please read the guidelines below then visit the Journal’s submission site https://mc.manuscriptcentral.com/nps to upload your manuscript. Please note that manuscripts not conforming to these guidelines may be returned . Remember you can log in to the submission site at any time to check on the progress of your paper through the peer review process.
Only manuscripts of sufficient quality that meet the aims and scope of New Perspectives will be reviewed.
There are no fees payable to submit or publish in this Journal. Open Access options are available - see section 3.3 below.
As part of the submission process you will be required to warrant that you are submitting your original work, that you have the rights in the work, and that you have obtained and can supply all necessary permissions for the reproduction of any copyright works not owned by you, that you are submitting the work for first publication in the Journal and that it is not being considered for publication elsewhere and has not already been published elsewhere. Please see our guidelines on prior publication and note that New Perspectives may accept submissions of papers that have been posted on pre-print servers; please alert the Editorial Office when submitting (contact details are at the end of these guidelines) and include the DOI for the preprint in the designated field in the manuscript submission system. Authors should not post an updated version of their paper on the preprint server while it is being peer reviewed for possible publication in the journal. If the article is accepted for publication, the author may re-use their work according to the journal's author archiving policy.
If your paper is accepted, you must include a link on your preprint to the final version of your paper.
- What do we publish? 1.1 Aims & Scope 1.2 Article types 1.3 Writing your paper
- Editorial policies 2.1 Peer review policy 2.2 Authorship 2.3 Acknowledgements 2.4 Declaration of conflicting interests 2.5 Research Data
- Publishing policies 3.1 Publication ethics 3.2 Contributor's publishing agreement 3.3 Open access and author archiving
- Preparing your manuscript 4.1 Formatting 4.2 Artwork, figures and other graphics 4.3 Supplemental material 4.4 Reference style 4.5 English language editing services
- Submitting your manuscript 5.1 ORCID 5.2 Information required for completing your submission 5.3 Permissions
- On acceptance and publication 6.1 Sage Production 6.2 Online First publication 6.3 Access to your published article 6.4 Promoting your article
- Further information
1. What do we publish?
1.1 Aims & Scope
Before submitting your manuscript to New Perspectives please ensure you have read the Aims & Scope .
1.2 Article Types
Research Articles are full-length papers that make an original contribution to research and are the main type of article that we seek. New Perspectives particularly seeks research articles that are methodologically systematic and reflexive; theoretically innovative and compelling; or empirically ground-breaking. Research Articles are normally between 8,000 and 10,000 words, including footnotes and references, with a maximum length including all notes and references is 12,000 words.
Essays present an argument or set out an agenda. They may be based on keynote addresses, be more polemical pieces or represent analytical, interpretive or synthetic work that doesn't fit the research article model. They do not include literature reviews and are written to be read beyond as well as within academia. Essays should be no longer than 6000 words including all notes and references.
Discussions integrate, synthesise or juxtapose scholarship, delineate or develop scholarly debates, or identify new directions in interdisciplinary research on the politics and international relations of Central and Eastern Europe. We encourage discussion papers that are between 10,000 and 12,000 words, although the maximum length including all notes and references is 15,000 words.
Review Essays contextualise several recently published or re-published volumes (3-5 titles per Review Essay) in relation to each other as well as in relation to wider academic scholarship and public political debate and discussion by identifying and critically engaging key themes and strands of thought. Review essays should be between 3,500 and 4,500 words, with a maximum length of 5,000 words including all notes and references.
Fora [on e.g. a book, an event or issue, as a set of responses and rejoinders to a report, proceedings of a roundtable at a conference etc]
- Usually 4-5 contributions responding to something and then a rejoinder or two.
- Sometimes 6 or 7 contributions with no rejoinder is also possible.
Registered Reports, Pre-Data or Post-Data: There are two types of Registered Reports:
- Registered Reports – Pre-Data, i.e., before any data have been gathered
- Registered Reports – Post-Data, i.e., before already existing data have been examined and analysed.
These submissions are reviewed in two stages. In Stage 1, a study proposal is considered for publication prior to data collection and/or analysis. Stage 1 submissions should include a complete Introduction, Methods, and Proposed Analyses. High-quality proposals will be accepted in principle before data collection and/or data analysis commences. Once the study is completed, the author will finish the article including Results and Discussion sections (Stage 2). Publication of the Stage 2 submission is guaranteed as long as the approved Stage 1 protocol is followed and the conclusions are appropriate. Full details can be found here . The Journal’s manuscript requirements should be adhered to for the stage 2 submission.
Cultural Cuts are excerpts from art or cultural products/ texts/ exhibitions that showcase something political in, of or relevant for the region and its international relations in a different way. If you would like to submit or suggest a cultural cut, you should consult the editors and also be ready to write an introduction that helps contextualise the work(s) in question with regard to the journal. [these are usually commissioned/ selected by the Editor/ Deputy, but submissions or suggestions in this regard would also be very welcome.
All three types of articles should be submitted complete with the following:
- an abstract of no more than 200 words, which should describe the main topic, arguments, methods and conclusions of the article
- a list of keywords (minimum 3, maximum 6) for indexing and abstracting purposes
Please note that there is no limit on the number of references allowed in each of these article types.
Nonetheless, all ‘literature review’ elements of any type of article should be kept to a minimum. Paragraphs should be kept as short as possible and generally not exceed 250 words. Likewise, sentences should be kept as short as possible and should not exceed 50 words. The emphasis should be on clear communication, while considering Einstein’s dictum – ‘[Make it] as simple as possible, but not simpler.’
Nonetheless, author’s should bear in mind the journal’s unofficial motto of ‘experiment, express, enjoy’ in their style as well as in the substance of their submissions and we welcome pieces that revel in their writing.
1.3 Writing your paper
The Sage Author Gateway has some general advice and on how to get published , plus links to further resources. Sage Author Services also offers authors a variety of ways to improve and enhance their article including English language editing, plagiarism detection, and video abstract and infographic preparation.
1.3.1 Make your article discoverable
For information and guidance on how to make your article more discoverable, visit our Gateway page on How to Help Readers Find Your Article Online
Back to top
2. Editorial policies
2.1 Peer review policy
Sage does not permit the use of author-suggested (recommended) reviewers at any stage of the submission process, be that through the web-based submission system or other communication. Reviewers should be experts in their fields and should be able to provide an objective assessment of the manuscript. Our policy is that reviewers should not be assigned to a paper if:
• The reviewer is based at the same institution as any of the co-authors
• The reviewer is based at the funding body of the paper
• The author has recommended the reviewer
• The reviewer has provided a personal (e.g. Gmail/Yahoo/Hotmail) email account and an institutional email account cannot be found after performing a basic Google search (name, department and institution).
2.2 Authorship
All parties who have made a substantive contribution to the article should be listed as authors. Principal authorship, authorship order, and other publication credits should be based on the relative scientific or professional contributions of the individuals involved, regardless of their status. A student is usually listed as principal author on any multiple-authored publication that substantially derives from the student’s dissertation or thesis.
Please note that AI chatbots, for example ChatGPT, should not be listed as authors. For more information see the policy on Use of ChatGPT and generative AI tools .
2.3 Acknowledgements
All contributors who do not meet the criteria for authorship should be listed in an Acknowledgements section. Examples of those who might be acknowledged include a person who provided purely technical help, or a department chair who provided only general support.
Please supply any personal acknowledgements separately to the main text to facilitate anonymous peer review.
2.3.1 Third party submissions
Where an individual who is not listed as an author submits a manuscript on behalf of the author(s), a statement must be included in the Acknowledgements section of the manuscript and in the accompanying cover letter. The statements must:
- Disclose this type of editorial assistance – including the individual’s name, company and level of input
- Identify any entities that paid for this assistance
- Confirm that the listed authors have authorized the submission of their manuscript via third party and approved any statements or declarations, e.g. conflicting interests, funding, etc.
Where appropriate, Sage reserves the right to deny consideration to manuscripts submitted by a third party rather than by the authors themselves .
2.4 Declaration of conflicting interests
New Perspectives encourages authors to include a declaration of any conflicting interests and recommends you review the good practice guidelines on the Sage Journal Author Gateway
2.5 Research data
The journal is committed to facilitating openness, transparency and reproducibility of research, and has the following research data sharing policy. For more information, including FAQs please visit the Sage Research Data policy pages
Subject to appropriate ethical and legal considerations, authors are encouraged to:
- share your research data in a relevant public data repository
- include a data availability statement linking to your data. If it is not possible to share your data, we encourage you to consider using the statement to explain why it cannot be shared.
- cite this data in your research
3. Publishing Policies
3.1 Publication ethics
Sage is committed to upholding the integrity of the academic record. We encourage authors to refer to the Committee on Publication Ethics’ International Standards for Authors and view the Publication Ethics page on the Sage Author Gateway
3.1.1 Plagiarism
New Perspectives and Sage take issues of copyright infringement, plagiarism or other breaches of best practice in publication very seriously. We seek to protect the rights of our authors and we always investigate claims of plagiarism or misuse of published articles. Equally, we seek to protect the reputation of the journal against malpractice. Submitted articles may be checked with duplication-checking software. Where an article, for example, is found to have plagiarized other work or included third-party copyright material without permission or with insufficient acknowledgement, or where the authorship of the article is contested, we reserve the right to take action including, but not limited to: publishing an erratum or corrigendum (correction); retracting the article; taking up the matter with the head of department or dean of the author's institution and/or relevant academic bodies or societies; or taking appropriate legal action.
3.1.2 Prior publication
If material has been previously published it is not generally acceptable for publication in a Sage journal. However, there are certain circumstances where previously published material can be considered for publication. Please refer to the guidance on the Sage Author Gateway or if in doubt, contact the Editor at the address given below.
3.2 Contributor's publishing agreement
Before publication, Sage requires the author as the rights holder to sign a Journal Contributor’s Publishing Agreement. Sage’s Journal Contributor’s Publishing Agreement is an exclusive licence agreement which means that the author retains copyright in the work but grants Sage the sole and exclusive right and licence to publish for the full legal term of copyright. Exceptions may exist where an assignment of copyright is required or preferred by a proprietor other than Sage. In this case copyright in the work will be assigned from the author to the society. For more information please visit the Sage Author Gateway
3.3 Open access and author archiving
New Perspectives offers optional open access publishing via the Sage Choice programme and Open Access agreements, where authors can publish open access either discounted or free of charge depending on the agreement with Sage. Find out if your institution is participating by visiting Open Access Agreements at Sage . For more information on Open Access publishing options at Sage please visit Sage Open Access . For information on funding body compliance, and depositing your article in repositories, please visit Sage’s Author Archiving and Re-Use Guidelines and Publishing Policies .
4. Preparing your manuscript for submission
4.1 Formatting
The preferred format for your manuscript is Word. LaTeX files are also accepted. Word and (La)Tex templates are available on the Manuscript Submission Guidelines page of our Author Gateway.
4.2 Artwork, figures and other graphics
For guidance on the preparation of illustrations, pictures and graphs in electronic format, please visit Sage’s Manuscript Submission Guidelines
Figures supplied in colour will appear in colour online regardless of whether or not these illustrations are reproduced in colour in the printed version. For specifically requested colour reproduction in print, you will receive information regarding the costs from Sage after receipt of your accepted article.
4.3 Supplemental material
This journal is able to host additional materials online (e.g. datasets, podcasts, videos, images etc) alongside the full-text of the article. For more information please refer to our guidelines on submitting supplemental files
4.4 Reference style
New Perspectives adheres to the Sage Harvard reference style. View the Sage Harvard guidelines to ensure your manuscript conforms to this reference style.
If you use EndNote to manage references, you can download the Sage Harvard EndNote output file [OR] the Sage Vancouver EndNote output file
4.5 English language editing services
Authors seeking assistance with English language editing, translation, or figure and manuscript formatting to fit the journal’s specifications should consider using Sage Language Services. Visit Sage Language Services on our Journal Author Gateway for further information.
5. Submitting your manuscript
New Perspectives is hosted on Sage Track, a web based online submission and peer review system powered by ScholarOne™ Manuscripts. Visit https://mc.manuscriptcentral.com/nps to login and submit your article online.
IMPORTANT: Please check whether you already have an account in the system before trying to create a new one. If you have reviewed or authored for the journal in the past year it is likely that you will have had an account created. For further guidance on submitting your manuscript online please visit ScholarOne Online Help.
As part of our commitment to ensuring an ethical, transparent and fair peer review process Sage is a supporting member of ORCID, the Open Researcher and Contributor ID . ORCID provides a unique and persistent digital identifier that distinguishes researchers from every other researcher, even those who share the same name, and, through integration in key research workflows such as manuscript and grant submission, supports automated linkages between researchers and their professional activities, ensuring that their work is recognized.
The collection of ORCID IDs from corresponding authors is now part of the submission process of this journal. If you already have an ORCID ID you will be asked to associate that to your submission during the online submission process. We also strongly encourage all co-authors to link their ORCID ID to their accounts in our online peer review platforms. It takes seconds to do: click the link when prompted, sign into your ORCID account and our systems are automatically updated. Your ORCID ID will become part of your accepted publication’s metadata, making your work attributable to you and only you. Your ORCID ID is published with your article so that fellow researchers reading your work can link to your ORCID profile and from there link to your other publications.
If you do not already have an ORCID ID please follow this link to create one or visit our ORCID homepage to learn more
5.2 Information required for completing your submission
You will be asked to provide contact details and academic affiliations for all co-authors via the submission system and identify who is to be the corresponding author. These details must match what appears on your manuscript. The affiliation listed in the manuscript should be the institution where the research was conducted. If an author has moved to a new institution since completing the research, the new affiliation can be included in a manuscript note at the end of the paper. At this stage please ensure you have included all the required statements and declarations and uploaded any additional supplementary files (including reporting guidelines where relevant).
5.3 Permissions
Please also ensure that you have obtained any necessary permission from copyright holders for reproducing any illustrations, tables, figures or lengthy quotations previously published elsewhere. For further information including guidance on fair dealing for criticism and review, please see the Copyright and Permissions page on the Sage Author Gateway
6. On acceptance and publication
6.1 Sage Production
Your Sage Production Editor will keep you informed as to your article’s progress throughout the production process. Proofs will be made available to the corresponding author via our editing portal Sage Edit or by email, and corrections should be made directly or notified to us promptly. Authors are reminded to check their proofs carefully to confirm that all author information, including names, affiliations, sequence and contact details are correct, and that Funding and Conflict of Interest statements, if any, are accurate.
6.2 Online First publication
Online First allows final articles (completed and approved articles awaiting assignment to a future issue) to be published online prior to their inclusion in a journal issue, which significantly reduces the lead time between submission and publication. Visit the Sage Journals help page for more details, including how to cite Online First articles.
6.3 Access to your published article
Sage provides authors with online access to their final article.
6.4 Promoting your article
Publication is not the end of the process! You can help disseminate your paper and ensure it is as widely read and cited as possible. The Sage Author Gateway has numerous resources to help you promote your work. Visit the Promote Your Article page on the Gateway for tips and advice.
7. Further information
Any correspondence, queries or additional requests for information on the manuscript submission process should be sent to Nicholas Michelsen in the New Perspective’s editorial office as follows:
Email: [email protected]
7.1 Appealing the publication decision
Editors have very broad discretion in determining whether an article is an appropriate fit for their journal. Many manuscripts are declined with a very general statement of the rejection decision. These decisions are not eligible for formal appeal unless the author believes the decision to reject the manuscript was based on an error in the review of the article, in which case the author may appeal the decision by providing the Editor with a detailed written description of the error they believe occurred.
If an author believes the decision regarding their manuscript was affected by a publication ethics breach, the author may contact the publisher with a detailed written description of their concern, and information supporting the concern, at [email protected]
- Read Online
- Sample Issues
- Current Issue
- Email Alert
- Permissions
- Foreign rights
- Reprints and sponsorship
- Advertising
Individual Subscription, E-access
Institutional Subscription, E-access
To order single issues of this journal, please contact SAGE Customer Services at 1-800-818-7243 / 1-805-583-9774 with details of the volume and issue you would like to purchase.

An official website of the United States government
The .gov means it’s official. Federal government websites often end in .gov or .mil. Before sharing sensitive information, make sure you’re on a federal government site.
The site is secure. The https:// ensures that you are connecting to the official website and that any information you provide is encrypted and transmitted securely.
- Publications
- Account settings
Preview improvements coming to the PMC website in October 2024. Learn More or Try it out now .
- Advanced Search
- Journal List
- Philos Trans R Soc Lond B Biol Sci
- v.364(1516); 2009 Feb 27
Animal camouflage: current issues and new perspectives
Martin stevens.
1 Department of Zoology, University of Cambridge, Downing Street, Cambridge CB2 3EJ, UK
Sami Merilaita
2 Department of Zoology, Stockholm University, 10691 Stockholm, Sweden
1. The importance and history of camouflage research
The study of camouflage has a long history in biology, and the numerous ways of concealment and disguise found in the animal kingdom provided Darwin and Wallace with important examples for illustrating and defending their ideas of natural selection and adaptation. Thus, various forms of camouflage have become classical examples of evolution. In a broader sense, camouflage has been adopted by humans, most notably by the military and hunters, but it has also influenced other parts of society, for example, arts, popular culture and design.
Animals use camouflage to make detection or recognition more difficult, with most examples associated with visual camouflage involving body coloration. However, in addition to coloration, camouflage may make use of morphological structures or material found in the environment, and may even act against senses other than vision ( Ruxton 2009 ). In nature, some of the most striking examples of adaptation can be found with respect to avoiding being detected or recognized, with the strategies employed diverse, and sometimes extraordinary. Such strategies can include using markings to match the colour and pattern of the background, as in various moths (e.g. Kettlewell 1955 ), and to break up the appearance of the body, as in some marine isopods ( Merilaita 1998 ). Camouflage is a technique especially useful if the animal can change colour to match the background on which it is found, such as can some cephalopods ( Hanlon & Messenger 1988 ) and chameleons ( Stuart-Fox et al. 2008 ). Further remarkable examples include insects bearing an uncanny resemblance to bird droppings ( Hebert 1974 ) or fish resembling fallen leaves on a stream bed ( Sazima et al. 2006 ), to even making the body effectively transparent, as occurs in a range of, in particular, aquatic species ( Johnsen 2001 ; Carvalho et al. 2006 ). Examples such as leaf mimicry in butterflies helped convince Wallace (1889) , for example, of the power of natural selection. Other strategies may even stretch to the use of bioluminescence to hide shadows generated in aquatic environments ( Johnsen et al. 2004 ), and include ‘decorating’ the body with items from the general environment, such as do some crabs ( Hultgren & Stachowicz 2008 ). This diversity of camouflage strategies is a testament to the importance of avoiding predation, as this is surely one of the most important selection pressures an organism can face. Concealment represents one of the principal ways to do so.
Camouflage research has for a significant length of time linked biology, art and the military, stemming from the work and influence of Abbott Thayer and Hugh Cott. Indeed, Thayer's ( 1896 , 1909 ) and Cott's (1940) works are still hugely influential and contain a range of untested ideas. However, in spite of its long history and widespread occurrence, research on natural camouflage has not progressed as rapidly as many other areas of adaptive coloration, especially in the last 60–70 years. There are several reasons for this, including that human perceptions have often been used to subjectively assess a range of protective markings, rather than working from the perspective of the correct receiver. In general, the mechanisms of camouflage have often been erroneously regarded as intuitively obvious. Furthermore, many researchers may have found more showy types of animal coloration, for example, aposematism, mimicry and sexual ornamentation, more exciting than the often (but not always) duller colours and patterns used for camouflage. Thus, until recently, the study of natural camouflage has progressed slowly; little had changed in our understanding of how camouflage works since the landmark book of Hugh Cott in 1940. Therefore, many of the striking examples of camouflage, such as those discussed above, have not been formally tested, and the benefit that these different types of concealment bring to animals has rarely been quantified in survival terms and how they specifically work. However, gradually an appreciation of rigorous and objective experimental and analytical methods has increased over descriptive, often subjective, methods in the study of camouflage. Norris & Lowe's (1964) first objective quantification of coloration was important, and in particular, the work by Endler ( 1978 , 1984 ) pioneered and promoted the rigorous study of animal coloration and had a broader influence outside of the field of camouflage.
In the last few years, there has been an explosion of camouflage studies. The renewed interest in concealment has partly arisen following a growing body of research into warning coloration and mimicry, and with increased knowledge of visual perception and computer science. In addition, trying to understand the proximate mechanisms involved in different forms of camouflage includes the need for integrating psychological and ecological factors. This can enable an understanding of the natural selection and constraints imposed on camouflage, which both influence the optimization and evolution of the camouflage strategies. Currently, there are a growing number of researchers interested in camouflage, producing more interdisciplinary links between biology, visual psychology, computer science and art. It is an exciting time to study camouflage, and the contributions to this theme represent this growing interdisciplinary effort.
2. Defining camouflage strategies
During the many years naturalists have been interested in camouflage, a number of different terms have been used to describe the various suggested ways of concealment. This diverse terminology means that, for some phenomena, there are several synonymous names, and that some terms have been used differently by different authors and over time. It is important for clarity to use coherent and consistent terminology, and one aim of this theme issue is to try to clarify this somewhat confusing use of terms. Above, we suggest a list of terms and definitions ( table 1 ). In defining different forms of camouflage, we use the term ‘function’ to describe broadly what the adaptation may do (e.g. breaking up form, distracting attention), and the term ‘mechanism’ to refer to specific perceptual processes (e.g. exploiting edge detection mechanisms, lateral inhibition). Ideally, camouflage strategies should be defined by how they use or exploit specific mechanistic processes. However, one current problem in defining different forms of camouflage is that we do not know enough about the perceptual mechanisms involved. This is clearly a huge area of work for the future.
Terms and definitions relevant to visual camouflage.
With respect to visual camouflage, some authors have argued that defining camouflage types based primarily on appearance is useful. We do not doubt that categorisation of appearances has merits in some circumstances, such as for comparative studies (e.g. Stoner et al. 2003 ). However, others advocate far more extensive uses of descriptive terms. For example, Hanlon (2007) argues that animal camouflage patterns can effectively be defined by three basic pattern classes, ‘uniform’, ‘mottle’ and ‘disruptive’, and that while initially based on appearances in cephalopods, which can adjust their patterning, the grouping seems to apply to other animals as well. We feel this approach is counterproductive and will lead to confusion, particularly because such an approach does not aid the understanding of how different forms of camouflage function or the different visual mechanisms involved and how these, in turn, impose selection on animal coloration. Instead, definitions should be based on what camouflage does (even if the specific visual processes are uncertain). This is crucial because similar pattern types may have entirely different functions in different animals and circumstances. Stripes, for instance, which Hanlon (2007) groups as disruptive could equally well function in background matching, distraction, as warning signals, or with making estimates of speed and trajectory difficult (motion dazzle), depending on the context. In addition, differences in visual perception across animal groups render these subjective categories ineffective because, for example, a pattern may appear mottled to a predator with good visual acuity, or in close proximity, but may appear uniform if an animal is unable to resolve the markings. Camouflage colorations are also more likely to be a continuum and mixture of features, varying much more and along several dimensions than suggested by the three proposed, discrete ‘types’ alone. Finally, defining camouflage based on appearance alone risks confounding camouflage functions with developmental limitations. Instead, aiming to understand functions (and eventually mechanisms) gives much greater insight into the selection imposed on the optimization of anti-predator coloration and how they interrelate and differ.
(a) Definitions
Below, and in table 1 , we define the main forms of concealment, and how they work. We use the term camouflage to describe all forms of concealment, including those strategies preventing detection (crypsis) and recognition (e.g. masquerade). We use ‘cryptic coloration’ and related words to refer to coloration which in the first place prevents detection. In this, we include the terms cryptic (meaning hard to detect/concealed), crypsis and cryptic coloration (e.g. the use of colours and patterns to prevent detection; cf. crypsis versus aposematism). We include several forms of camouflage under crypsis, including countershading, background matching and disruptive coloration. We do not discuss all of these below, but rather outline some of the main disagreements at present.
(b) What is ‘crypsis’?
The use of the term crypsis has caused disagreement over the last few years, but we argue that it comprises all traits that reduce an animal's risk of becoming detected when it is potentially perceivable to an observer. In terms of vision, the term crypsis includes features of physical appearance (e.g. coloration), but also behavioural traits, or both, to prevent detection. To distinguish crypsis from hiding (such as simply being hidden behind an object in the environment), we argue that the features of the animal should reduce the risk of detection when the animal is in plain sight, if those traits are to be considered crypsis. Hiding behind an object, for example, does not constitute crypsis (see also Edmunds 1974 ), because there is no chance of the receiver detecting the animal. We opt for this usage for several reasons. First, this is broadly consistent with the literal and historical terminology; (albeit briefly) Poulton (1890) used the term to describe colours whose ‘object is to effect concealment’; Cott (1940) uses cryptic appearance to ‘encompass modifications of structure, colour, pattern and habit’; and Edmunds (1974) defines the terms crypsis and cryptic, in terms of predators failing to detect prey. By contrast, some researchers have defined crypsis as synonymous with background matching, largely because they rapidly adopted Endler's ( 1978 , 1984 ) definition of crypsis, where an animal should maximize camouflage by matching a random sample of the background at the time and location where the risk of predation is the greatest. However, in recent years, it has become clear that the above definition is wrong on a number of grounds. First, matching a random sample of the background does not necessarily minimize the risk of detection when an animal is found on several backgrounds (cf. ‘compromise camouflage’; Merilaita et al. 1999 , 2001 ; Houston et al. 2007 ; Sherratt et al. 2007 ). Second, the risk of detection can be decreased by disruptive markings, where the emphasis is on specifically breaking up tell-tale features of the animal. Similar points can be made for other camouflage strategies, such as self-shadow concealment (SSC). Finally, matching a random sample on even one background does not guarantee a high level of background matching or crypsis ( Merilaita & Lind 2005 ). This idea of random sample is problematic even on simple backgrounds, because the animal may still be visible due to spatial or phase ‘mismatch’ with important background features, such as edges ( Kelman et al. 2007 ). For these reasons, we simply refer to crypsis as including colours and patterns that prevent detection (but not necessarily recognition).
Despite the above, it is a subject of some debate as to which other forms of camouflage also prevent detection and should therefore be included under crypsis along with background matching (see below). One of the main arguments surrounding what should be included under crypsis regards disruptive coloration, and whether this prevents recognition or detection. While some researchers (e.g. Stobbe & Schaefer 2008 ) assert that disruption prevents recognition of the animal, we argue that disruptive coloration initially prevents detection by breaking up form (which in turn may also influence recognition) and is therefore a type of crypsis. For instance, disruptive coloration seemingly works by breaking up edge information, so that a predator may not detect a prey item because the salient outlines that may give away its presence have been destroyed.
In countershading, an animal possesses a darker surface on the side that typically faces light and a lighter opposite side. Most researchers seem to now agree that the term refers to the appearance of the coloration and not the function, especially as countershading may be involved with several functions. These include compensation of own shadow (SSC), simultaneously matching two different backgrounds in two different directions (background matching), changing the three-dimensional appearance of the animal, protection from UV light and others ( Ruxton et al. 2004 ). For the purposes of this theme issue, the two most relevant functions are SSC, where the creation of shadows is cancelled out by countershading, and ‘obliterative shading’, where the shadow/light cues for three-dimensional form of the animal are destroyed ( Thayer 1896 ). We argue that SSC prevents detection by removing conspicuous shadows, and obliterative shading prevents detection by removing salient three-dimensional information, so group both these under crypsis.
In principle, some of the issues of defining types of camouflage may be cleared up by specifically defining detection. However, at present, there are few good ways of fully defining camouflage object properties correctly with respect to the relevant viewer's perception. Understandably, there is a real issue that distinguishing between detection and recognition in experimental situations is very difficult, and it follows that preventing detection may also lead to a prevention of recognition, e.g. the receiver does not recognize the form of the animal because it does not detect its edges. What matters is what the colour patterning or other camouflage features primarily do. As such, masquerade need not prevent detection but it does prevent recognition, whereas disruptive coloration and SSC, along with background matching, primarily prevent detection.
An additional form of camouflage, distractive markings, is also included under crypsis because they seemingly prevent detection. Although the distractive markings should be detected, the outline of the body or other revealing characteristics, and thus the main part of the animal, is not. However, we note that little work has specifically investigated distractive markings, and that one could also argue that if part of the object is detected, then recognition of the prey is also prevented. Clearly, there is much more work to be done.
(c) Other forms of camouflage
We make a distinction between dazzle or distractive markings and disruptive coloration (cf. Stevens 2007 ) in contrast to Cott (1940) , who fused these different concepts in his description of the function of disruptive coloration. Our use of dazzle coloration is also different from ‘flicker-fusion camouflage’ and startle displays (which involve the sudden appearance of markings, such as spots and bright colours; table 1 ). Although the term masquerade has sometimes been used synonymously with background matching, generally, it seems uncontroversial that masquerade acts against recognition and is therefore a different form of concealment. Motion camouflage is a term for something quite different, where an animal appears not to be moving at all by ‘tricking’ the receiver's visual system by moving in a certain way.
3. Contributions to the theme issue
In this issue there are a range of contributions from researchers spanning multiple disciplines, from behavioural ecology, experimental psychology and computer science, to art history. Hanlon et al. (2009) review and discuss the main camouflage in cephalopods, which have a remarkable ability for rapid colour change. Zylinski et al. (2009) present experiments and discussion about how different forms of camouflage in cuttlefish are produced by features such as edges in the background, and what this can tell us about visual perception in cuttlefish and other animals. Troscianko et al. (2009) apply principles from visual psychology and physiology to discuss various methods involved in visual perception, and how they are important in producing effective camouflage and camouflage breaking. A range of other animals are also capable of colour change, and Stuart-Fox & Moussalli (2009) discuss what these animals, in particular chameleons, can reveal about the proximate and ultimate factors underlying camouflage, signalling strategies and thermoregulation. Théry & Casas (2009) discuss the various functions of spider coloration, webs and decorations, including colour change and concealment. Stevens & Merilaita (2009) synthesize and discuss the principles involved in disruptive coloration, and how disruption relates to other forms of camouflage. One aspect of disruptive coloration is coincident disruption, used to conceal salient body parts such as legs and wings, and Cuthill & Székely (2009) present the first experimental support for this theory with field experiments presenting artificial prey to wild avian predators. Behrens (2009) discusses how art, the military and nature influenced the ideas of Abbott Thayer in producing his theories of camouflage, and how Thayer in turn influenced these fields. Webster et al. (2009) investigate the camouflage and resting orientation of wild moths, using detection experiments with human ‘predators’, showing that the coloration and resting position of the moths produces effective camouflage. Stobbe et al. (2009) present the findings of laboratory predation experiments with avian predators and artificial prey, to investigate the relative importance of colour and luminance in effective camouflage. Rowland (2009) reviews previous work and presents new data to investigate the function of countershading in producing camouflage, with Tankus & Yeshurun (2009) adopting a computer vision approach to illustrate how detection of cylindrical objects may work in predators, and how the countershading of prey animals may inhibit this detection process. Caro (2009) presents a comparative study of black and white coloration in mammals and the various forms of camouflage that may stem from these coloration types. Finally, Ruxton (2009) discusses where and how the principles derived from visual camouflage can be applied to other sensory modalities, and reviews the evidence for non-visual camouflage.
Acknowledgments
We thank Graeme Ruxton for comments and discussion of this paper, and the various contributors to this theme issue for a range of discussion. M.S. was supported by a research fellowship from Girton College, Cambridge, and S.M. by the Swedish Research Council and the Academy of Finland.
One contribution of 15 to a Theme Issue ‘Animal camouflage: current issues and new perspectives’.
- Behrens R.R. Revisiting Abbott Thayer: non-scientific reflections about camouflage in art, war and zoology. Phil. Trans. R. Soc. B. 2009; 364 :497–501. doi:10.1098/rstb.2008.0250 [ PMC free article ] [ PubMed ] [ Google Scholar ]
- Caro T. Contrasting coloration in terrestrial mammals. Phil. Trans. R. Soc. B. 2009; 364 :537–548. doi:10.1098/rstb.2008.0221 [ PMC free article ] [ PubMed ] [ Google Scholar ]
- Carvalho L.N., Zuanon J., Sazima I. The almost invisible league: crypsis and association between minute fishes and shrimps as a possible defence against visually hunting predators. Neotrop. Ichthyol. 2006; 4 :219–224. [ Google Scholar ]
- Cott H.B. Methuen & Co. Ltd; London, UK: 1940. Adaptive coloration in animals. [ Google Scholar ]
- Cuthill I.C., Székely A. Coincident disruptive coloration. Phil. Trans. R. Soc. B. 2009; 364 :489–496. doi:10.1098/rstb.2008.0266 [ PMC free article ] [ PubMed ] [ Google Scholar ]
- Edmunds M. Longman Group Ltd; Harlow, UK: 1974. Defence in animals: a survey of antipredator defences. [ Google Scholar ]
- Endler J.A. A predator's view of animal color patterns. Evol. Biol. 1978; 11 :319–364. [ Google Scholar ]
- Endler J.A. Progressive background matching in moths, and a quantitative measure of crypsis. Biol. J. Linn. Soc. 1984; 22 :187–231. doi:10.1111/j.1095-8312.1984.tb01677.x [ Google Scholar ]
- Hanlon R.T. Cephalopod dynamic camouflage. Curr. Biol. 2007; 17 :400–404. doi:10.1016/j.cub.2007.03.034 [ PubMed ] [ Google Scholar ]
- Hanlon R.T., Messenger J.B. Adaptive coloration in young cuttlefish ( Sepia officinalis L. ): the morphology and development of body patterns and their relation to behavior. Phil. Trans. R. Soc. B. 1988; 320 :437–487. doi:10.1098/rstb.1988.0087 [ Google Scholar ]
- Hanlon R.T., Chiao C.-C., Mäthger L.M., Barbosa A., Buresch K.C., Chubb C. Cephalopod dynamic camouflage: bridging the continuum between background matching and disruptive coloration. Phil. Trans. R. Soc. B. 2009; 364 :429–437. doi:10.1098/rstb.2008.0270 [ PMC free article ] [ PubMed ] [ Google Scholar ]
- Hebert P.D.N. Spittlebug morph mimics avian excrement. Nature. 1974; 150 :352–354. doi:10.1038/250352a0 [ PubMed ] [ Google Scholar ]
- Houston A.I., Stevens M., Cuthill I.C. Animal camouflage: compromise or specialise in a two patch-type environment? Behav. Ecol. 2007; 18 :769–775. doi:10.1093/beheco/arm039 [ Google Scholar ]
- Hultgren K.M., Stachowicz J.J. Alternative camouflage strategies mediate predation risk among closely related co-occuring kelp crabs. Oecologia. 2008; 55 :519–528. doi:10.1007/s00442-007-0926-5 [ PubMed ] [ Google Scholar ]
- Johnsen S. Hidden in plain sight: the ecology and physiology of organismal transparency. Biol. Bull. 2001; 201 :301–318. doi:10.2307/1543609 [ PubMed ] [ Google Scholar ]
- Johnsen S., Widder E.A., Mobley C.D. Propagation and perception of bioluminescence: factors affecting counterillumination as a cryptic strategy. Biol. Bull. 2004; 207 :1–16. doi:10.2307/1543624 [ PubMed ] [ Google Scholar ]
- Kelman E.J., Baddeley R., Shohet A., Osorio D. Perception of visual texture, and the expression of disruptive camouflage by the cuttlefish, Sepia officinalis . Proc. R. Soc. B. 2007; 274 :1369–1375. doi:10.1098/rspb.2007.0240 [ PMC free article ] [ PubMed ] [ Google Scholar ]
- Kettlewell H.B.D. Selection experiments on industrial melanism in the Lepidoptera. Heredity. 1955; 9 :323–342. doi:10.1038/hdy.1955.36 [ Google Scholar ]
- Merilaita S. Crypsis through disruptive coloration in an isopod. Proc. R. Soc. B. 1998; 265 :1059–1064. doi:10.1098/rspb.1998.0399 [ Google Scholar ]
- Merilaita S., Lind J. Background-matching and disruptive coloration, and the evolution of cryptic coloration. Proc. R. Soc. B. 2005; 272 :665–670. doi:10.1098/rspb.2004.3000 [ PMC free article ] [ PubMed ] [ Google Scholar ]
- Merilaita S., Tuomi J., Jormalainen V. Optimization of cryptic coloration in heterogeneous habitats. Biol. J. Linn. Soc. 1999; 67 :151–161. doi:10.1111/j.1095-8312.1999.tb01858.x [ Google Scholar ]
- Merilaita S., Lyytinen A., Mappes J. Selection for cryptic coloration in a visually heterogeneous habitat. Proc. R. Soc. B. 2001; 268 :1925–1929. doi:10.1098/rspb.2001.1747 [ PMC free article ] [ PubMed ] [ Google Scholar ]
- Norris K.S., Lowe C.H. An analysis of background color-matching in amphibians and reptiles. Ecology. 1964; 45 :565–580. doi:10.2307/1936109 [ Google Scholar ]
- Poulton, E. B. 1890 The colours of animals: their meaning and use. Especially considered in the case of insects , 2nd edn. The International Scientific Series. London, UK: Kegan Paul, Trench Trübner, & Co. Ltd.
- Rowland H.M. From Abbot Thayer to the present day: what have we learned about the function of countershading? Phil. Trans. R. Soc. B. 2009; 364 :519–527. doi:10.1098/rstb.2008.0261 [ PMC free article ] [ PubMed ] [ Google Scholar ]
- Ruxton G.D. Non-visual crypsis: a review of the empirical evidence for camouflage to senses other than vision. Phil. Trans. R. Soc. B. 2009; 364 :549–557. doi:10.1098/rstb.2008.0228 [ PMC free article ] [ PubMed ] [ Google Scholar ]
- Ruxton G.D., Speed M.P., Kelly D.J. What, if anything, is the adaptive function of countershading? Anim. Behav. 2004; 68 :445–451. doi:10.1016/j.anbehav.2003.12.009 [ Google Scholar ]
- Sazima I., Carvalho L.N., Mendonça F.P., Zuanon J. Fallen leaves on the water-bed: diurnal camouflage of three night active fish species in an Amazonian streamlet. Neotrop. Ichthyol. 2006; 4 :119–122. [ Google Scholar ]
- Sherratt T.N., Pollitt D., Wilkinson D.M. The evolution of crypsis in replicating populations of web-based prey. Oikos. 2007; 116 :449–460. doi:10.1111/j.0030-1299.2007.15521.x [ Google Scholar ]
- Stevens M. Predator perception and the interrelation between protective coloration. Proc. R. Soc. B. 2007; 274 :1457–1464. doi:10.1098/rspb.2007.0220 [ PMC free article ] [ PubMed ] [ Google Scholar ]
- Stevens M., Merilaita S. Defining disruptive coloration and distinguishing its functions. Phil. Trans. R. Soc. B. 2009; 364 :481–488. doi:10.1098/rstb.2008.0216 [ PMC free article ] [ PubMed ] [ Google Scholar ]
- Stobbe N., Schaefer M.H. Enhancement of chromatic contrast increases predation risk for striped butterflies. Proc. R. Soc. B. 2008; 275 :1535–1541. doi:10.1098/rspb.2008.0209 [ PMC free article ] [ PubMed ] [ Google Scholar ]
- Stobbe N., Dimitrova M., Merilaita S., Schaefer H.M. Chromaticty in the UV/blue range facilitates the search for achromatically background-matching prey in birds. Phil. Trans. R. Soc. B. 2009; 364 :511–517. doi:10.1098/rstb.2008.0248 [ PMC free article ] [ PubMed ] [ Google Scholar ]
- Stoner C.J., Caro T.M., Graham C.M. Ecological and behavioral correlates of coloration in artiodactyls: systematic analyses of conventional hypotheses. Behav. Ecol. 2003; 14 :823–840. doi:10.1093/beheco/arg072 [ Google Scholar ]
- Stuart-Fox D., Moussalli A. Camouflage, communication and thermoregulation: lessons from colour changing organisms. Phil. Trans. R. Soc. B. 2009; 364 :463–470. doi:10.1098/rstb.2008.0254 [ PMC free article ] [ PubMed ] [ Google Scholar ]
- Stuart-Fox D., Moussalli A., Whiting M.J. Predator-specific camouflage in chameleons. Biol. Lett. 2008; 4 :326–329. doi:10.1098/rsbl.2008.0173 [ PMC free article ] [ PubMed ] [ Google Scholar ]
- Tankus A., Yeshurun Y. Computer vision, camouflage breaking and countershading. Phil. Trans. R. Soc. B. 2009; 364 :529–536. doi:10.1098/rstb.2008.0211 [ PMC free article ] [ PubMed ] [ Google Scholar ]
- Thayer A.H. The law which underlies protective coloration. The Auk. 1896; 13 :477–482. [ Google Scholar ]
- Thayer G.H. Macmillan; New York, NY: 1909. Concealing–coloration in the animal kingdom: an exposition of the laws of disguise through color and pattern: being a summary of Abbott H. Thayer's discoveries. [ Google Scholar ]
- Théry M., Casas J. The multiple disguises of spiders: web colour and decorations, body colour and movement. Phil. Trans. R. Soc. B. 2009; 364 :471–480. doi:10.1098/rstb.2008.0212 [ PMC free article ] [ PubMed ] [ Google Scholar ]
- Troscianko T., Benton C.P., Lovell P.G., Tolhurst D.J., Pizlo Z. Camouflage and visual perception. Phil. Trans. R. Soc. B. 2009; 364 :449–461. doi:10.1098/rstb.2008.0218 [ PMC free article ] [ PubMed ] [ Google Scholar ]
- Wallace A.R. Macmillan & Co; London, UK: 1889. Darwinism. An exposition of the theory of natural selection with some of its applications. [ Google Scholar ]
- Webster R.J., Callahan A., Godin J.-G.J., Sherratt T.N. Behaviourally mediated crypsis in two nocturnal moths with contrasting appearance. Phil. Trans. R. Soc. B. 2009; 364 :503–510. doi:10.1098/rstb.2008.0215 [ PMC free article ] [ PubMed ] [ Google Scholar ]
- Zylinski S., Osorio D., Shohet A.J. Perception of edges and visual texture in the camouflage of the common cuttlefish, Sepia officinalis . Phil. Trans. R. Soc. B. 2009; 364 :439–448. doi:10.1098/rstb.2008.0264 [ PMC free article ] [ PubMed ] [ Google Scholar ]

Perspective: How to Share Opinion on Research Articles
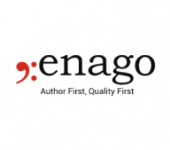
When we think of research articles, most of the time we think of articles that present the results of studies that took a long time to complete. Generally, these articles contain theories, testable hypotheses and extensive methodological justifications for conducting analyses. There are, however, many other types of research articles that are published in scientific journals. One of them, a perspective article, presents an important topic, groundbreaking research, or a different view of an existing issue by an expert in that field of research.
How It All Fits Together
Most of the research articles published by academic journals are original research articles . Journal editors tend to prefer this type of article, especially if it presents important advancements in a research field, or counterintuitive results. Other types of research articles include book reviews, case reports, editorials, interviews, commentaries, profiles, and interviews, and perspectives. Each journal ultimately decides, based on their field specialty, what types of research articles they wish to publish. For example, some social science journals (Comparative Political Studies) do not accept perspective research articles, while others refer to them as letters.
Perspective research articles have an important role in the academic research portfolio. They stimulate further interest about presented topics within the reader audience. They are different from other types of articles because they present a different take on an existing issue, tackle new and trending issues, or emphasize topics that are important, but have been neglected, in the scholarly literature. In some scientific fields they bridge different areas of research that the journal publishes, while in others they bring new issues and ideas to the forefront. In general, their role is to enlighten a general audience about important issues.
Why Write a Perspective?
While the incentive system of academic tenure and promotion emphasizes publication of original research , writing other types of articles is also beneficial for the researchers in the long run. It gives researchers the opportunity to contribute to their discipline in different ways, while at the same time enhancing their own professional work.
A perspective article is a way for young researchers to gain experience in the publications process that can be often arduous and time consuming. It can be a way in which they learn from the publication process while they are working on their original research articles that often take years to complete.
In the case of experienced researchers, writing a perspective article provides them at least two distinct benefits: first, it allows them to step back and reflect on a significant issue that they may know a lot about, but that they have never had the time to address. The second benefit is that the researcher gets the opportunity to give their own authorial voice to a published article that will reach a wide audience.
Pay Attention to Detail
Before one decides to write and submit a perspective research article to an academic journal, it is important to become familiar with the article expectations of the target journal.
Although academic journals hold a similar definition and purpose of a perspective article, there are differences in the technical requirements each journal has. When it comes to the length of the perspective article, some journals have strict limitations while others allow articles to vary the length within a given range. For example, some academic journals in the field of biological sciences and medicine have a limitation of 1,500 and 1,200 words respectively, with defined reference and figure limits. Another journal in the same field has a less restrictive limit of 2,000-4,000 words and a more generous reference limit.
With respect to the structure of the perspective article, journals define their expectations in different terms. Some journals place an emphasis on the structure of the article, requiring sections such as the abstract, introduction, topics and conclusion. Other journals make suggestions on the nature of the title and the specific conceptual connections in the assigned field. Some journals take the time to explain their view and expectation in writing perspective articles, make suggestions and provide lists of things to include and avoid in the perspective article.
Writing a perspective article can have many benefits to authors. Although writing one is less demanding than an original research article, it is recommended that an aspiring author consult the targeted journal for requirements. This will ensure that the journal expectations are met, and that the author has a positive first experience in the writing of this type of research article.
Have you had the experience of writing a perspective article? If yes, then what are the keypoints you kept in mind while doing so? Please let us know your thoughts in the comments section below.
Rate this article Cancel Reply
Your email address will not be published.
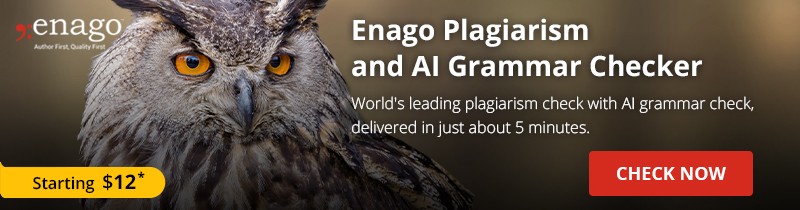
Enago Academy's Most Popular Articles
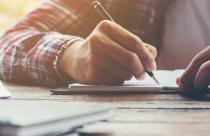
- Reporting Research
Beyond Spellcheck: How copyediting guarantees error-free submission
Submitting a manuscript is a complex and often an emotional experience for researchers. Whether it’s…
- Old Webinars
- Webinar Mobile App
How to Find the Right Journal and Fix Your Manuscript Before Submission
Selection of right journal Meets journal standards Plagiarism free manuscripts Rated from reviewer's POV
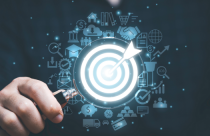
- Manuscripts & Grants
Research Aims and Objectives: The dynamic duo for successful research
Picture yourself on a road trip without a destination in mind — driving aimlessly, not…
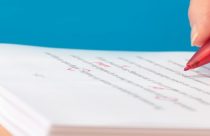
How Academic Editors Can Enhance the Quality of Your Manuscript
Avoiding desk rejection Detecting language errors Conveying your ideas clearly Following technical requirements
Effective Data Presentation for Submission in Top-tier Journals
Importance of presenting research data effectively How to create tables and figures How to avoid…
Top 4 Guidelines for Health and Clinical Research Report
Top 10 Questions for a Complete Literature Review

Sign-up to read more
Subscribe for free to get unrestricted access to all our resources on research writing and academic publishing including:
- 2000+ blog articles
- 50+ Webinars
- 10+ Expert podcasts
- 50+ Infographics
- 10+ Checklists
- Research Guides
We hate spam too. We promise to protect your privacy and never spam you.
I am looking for Editing/ Proofreading services for my manuscript Tentative date of next journal submission:
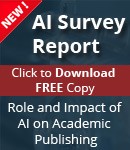
What should universities' stance be on AI tools in research and academic writing?
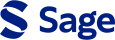
- Request new password
- Create a new account
Doing Research in the Real World
Student resources, chapter 2: theoretical perspectives and research methodologies.
- Checklist for Using Theory
Thank you for visiting nature.com. You are using a browser version with limited support for CSS. To obtain the best experience, we recommend you use a more up to date browser (or turn off compatibility mode in Internet Explorer). In the meantime, to ensure continued support, we are displaying the site without styles and JavaScript.
- View all journals
- Explore content
- About the journal
- Publish with us
- Sign up for alerts
- Perspective
- Published: 06 March 2024
Artificial intelligence and illusions of understanding in scientific research
- Lisa Messeri ORCID: orcid.org/0000-0002-0964-123X 1 na1 &
- M. J. Crockett ORCID: orcid.org/0000-0001-8800-410X 2 , 3 na1
Nature volume 627 , pages 49–58 ( 2024 ) Cite this article
21k Accesses
3 Citations
708 Altmetric
Metrics details
- Human behaviour
- Interdisciplinary studies
- Research management
- Social anthropology
Scientists are enthusiastically imagining ways in which artificial intelligence (AI) tools might improve research. Why are AI tools so attractive and what are the risks of implementing them across the research pipeline? Here we develop a taxonomy of scientists’ visions for AI, observing that their appeal comes from promises to improve productivity and objectivity by overcoming human shortcomings. But proposed AI solutions can also exploit our cognitive limitations, making us vulnerable to illusions of understanding in which we believe we understand more about the world than we actually do. Such illusions obscure the scientific community’s ability to see the formation of scientific monocultures, in which some types of methods, questions and viewpoints come to dominate alternative approaches, making science less innovative and more vulnerable to errors. The proliferation of AI tools in science risks introducing a phase of scientific enquiry in which we produce more but understand less. By analysing the appeal of these tools, we provide a framework for advancing discussions of responsible knowledge production in the age of AI.
This is a preview of subscription content, access via your institution
Access options
Access Nature and 54 other Nature Portfolio journals
Get Nature+, our best-value online-access subscription
24,99 € / 30 days
cancel any time
Subscribe to this journal
Receive 51 print issues and online access
185,98 € per year
only 3,65 € per issue
Rent or buy this article
Prices vary by article type
Prices may be subject to local taxes which are calculated during checkout
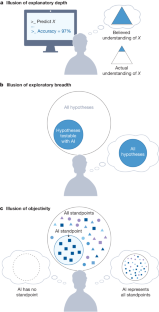
Similar content being viewed by others
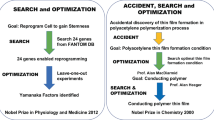
Nobel Turing Challenge: creating the engine for scientific discovery
Hiroaki Kitano
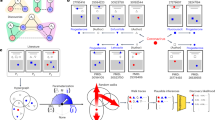
Accelerating science with human-aware artificial intelligence
Jamshid Sourati & James A. Evans
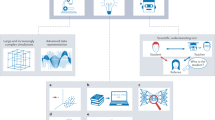
On scientific understanding with artificial intelligence
Mario Krenn, Robert Pollice, … Alán Aspuru-Guzik
Crabtree, G. Self-driving laboratories coming of age. Joule 4 , 2538–2541 (2020).
Article CAS Google Scholar
Wang, H. et al. Scientific discovery in the age of artificial intelligence. Nature 620 , 47–60 (2023). This review explores how AI can be incorporated across the research pipeline, drawing from a wide range of scientific disciplines .
Article CAS PubMed ADS Google Scholar
Dillion, D., Tandon, N., Gu, Y. & Gray, K. Can AI language models replace human participants? Trends Cogn. Sci. 27 , 597–600 (2023).
Article PubMed Google Scholar
Grossmann, I. et al. AI and the transformation of social science research. Science 380 , 1108–1109 (2023). This forward-looking article proposes a variety of ways to incorporate generative AI into social-sciences research .
Gil, Y. Will AI write scientific papers in the future? AI Mag. 42 , 3–15 (2022).
Google Scholar
Kitano, H. Nobel Turing Challenge: creating the engine for scientific discovery. npj Syst. Biol. Appl. 7 , 29 (2021).
Article PubMed PubMed Central Google Scholar
Benjamin, R. Race After Technology: Abolitionist Tools for the New Jim Code (Oxford Univ. Press, 2020). This book examines how social norms about race become embedded in technologies, even those that are focused on providing good societal outcomes .
Broussard, M. More Than a Glitch: Confronting Race, Gender, and Ability Bias in Tech (MIT Press, 2023).
Noble, S. U. Algorithms of Oppression: How Search Engines Reinforce Racism (New York Univ. Press, 2018).
Bender, E. M., Gebru, T., McMillan-Major, A. & Shmitchell, S. On the dangers of stochastic parrots: can language models be too big? in Proc. 2021 ACM Conference on Fairness, Accountability, and Transparency 610–623 (Association for Computing Machinery, 2021). One of the first comprehensive critiques of large language models, this article draws attention to a host of issues that ought to be considered before taking up such tools .
Crawford, K. Atlas of AI: Power, Politics, and the Planetary Costs of Artificial Intelligence (Yale Univ. Press, 2021).
Johnson, D. G. & Verdicchio, M. Reframing AI discourse. Minds Mach. 27 , 575–590 (2017).
Article Google Scholar
Atanasoski, N. & Vora, K. Surrogate Humanity: Race, Robots, and the Politics of Technological Futures (Duke Univ. Press, 2019).
Mitchell, M. & Krakauer, D. C. The debate over understanding in AI’s large language models. Proc. Natl Acad. Sci. USA 120 , e2215907120 (2023).
Kidd, C. & Birhane, A. How AI can distort human beliefs. Science 380 , 1222–1223 (2023).
Birhane, A., Kasirzadeh, A., Leslie, D. & Wachter, S. Science in the age of large language models. Nat. Rev. Phys. 5 , 277–280 (2023).
Kapoor, S. & Narayanan, A. Leakage and the reproducibility crisis in machine-learning-based science. Patterns 4 , 100804 (2023).
Hullman, J., Kapoor, S., Nanayakkara, P., Gelman, A. & Narayanan, A. The worst of both worlds: a comparative analysis of errors in learning from data in psychology and machine learning. In Proc. 2022 AAAI/ACM Conference on AI, Ethics, and Society (eds Conitzer, V. et al.) 335–348 (Association for Computing Machinery, 2022).
Rudin, C. Stop explaining black box machine learning models for high stakes decisions and use interpretable models instead. Nat. Mach. Intell. 1 , 206–215 (2019). This paper articulates the problems with attempting to explain AI systems that lack interpretability, and advocates for building interpretable models instead .
Crockett, M. J., Bai, X., Kapoor, S., Messeri, L. & Narayanan, A. The limitations of machine learning models for predicting scientific replicability. Proc. Natl Acad. Sci. USA 120 , e2307596120 (2023).
Article CAS PubMed PubMed Central Google Scholar
Lazar, S. & Nelson, A. AI safety on whose terms? Science 381 , 138 (2023).
Article PubMed ADS Google Scholar
Collingridge, D. The Social Control of Technology (St Martin’s Press, 1980).
Wagner, G., Lukyanenko, R. & Paré, G. Artificial intelligence and the conduct of literature reviews. J. Inf. Technol. 37 , 209–226 (2022).
Hutson, M. Artificial-intelligence tools aim to tame the coronavirus literature. Nature https://doi.org/10.1038/d41586-020-01733-7 (2020).
Haas, Q. et al. Utilizing artificial intelligence to manage COVID-19 scientific evidence torrent with Risklick AI: a critical tool for pharmacology and therapy development. Pharmacology 106 , 244–253 (2021).
Article CAS PubMed Google Scholar
Müller, H., Pachnanda, S., Pahl, F. & Rosenqvist, C. The application of artificial intelligence on different types of literature reviews – a comparative study. In 2022 International Conference on Applied Artificial Intelligence (ICAPAI) https://doi.org/10.1109/ICAPAI55158.2022.9801564 (Institute of Electrical and Electronics Engineers, 2022).
van Dinter, R., Tekinerdogan, B. & Catal, C. Automation of systematic literature reviews: a systematic literature review. Inf. Softw. Technol. 136 , 106589 (2021).
Aydın, Ö. & Karaarslan, E. OpenAI ChatGPT generated literature review: digital twin in healthcare. In Emerging Computer Technologies 2 (ed. Aydın, Ö.) 22–31 (İzmir Akademi Dernegi, 2022).
AlQuraishi, M. AlphaFold at CASP13. Bioinformatics 35 , 4862–4865 (2019).
Jumper, J. et al. Highly accurate protein structure prediction with AlphaFold. Nature 596 , 583–589 (2021).
Article CAS PubMed PubMed Central ADS Google Scholar
Lee, J. S., Kim, J. & Kim, P. M. Score-based generative modeling for de novo protein design. Nat. Computat. Sci. 3 , 382–392 (2023).
Gómez-Bombarelli, R. et al. Design of efficient molecular organic light-emitting diodes by a high-throughput virtual screening and experimental approach. Nat. Mater. 15 , 1120–1127 (2016).
Krenn, M. et al. On scientific understanding with artificial intelligence. Nat. Rev. Phys. 4 , 761–769 (2022).
Extance, A. How AI technology can tame the scientific literature. Nature 561 , 273–274 (2018).
Hastings, J. AI for Scientific Discovery (CRC Press, 2023). This book reviews current and future incorporation of AI into the scientific research pipeline .
Ahmed, A. et al. The future of academic publishing. Nat. Hum. Behav. 7 , 1021–1026 (2023).
Gray, K., Yam, K. C., Zhen’An, A. E., Wilbanks, D. & Waytz, A. The psychology of robots and artificial intelligence. In The Handbook of Social Psychology (eds Gilbert, D. et al.) (in the press).
Argyle, L. P. et al. Out of one, many: using language models to simulate human samples. Polit. Anal. 31 , 337–351 (2023).
Aher, G., Arriaga, R. I. & Kalai, A. T. Using large language models to simulate multiple humans and replicate human subject studies. In Proc. 40th International Conference on Machine Learning (eds Krause, A. et al.) 337–371 (JMLR.org, 2023).
Binz, M. & Schulz, E. Using cognitive psychology to understand GPT-3. Proc. Natl Acad. Sci. USA 120 , e2218523120 (2023).
Ornstein, J. T., Blasingame, E. N. & Truscott, J. S. How to train your stochastic parrot: large language models for political texts. Github , https://joeornstein.github.io/publications/ornstein-blasingame-truscott.pdf (2023).
He, S. et al. Learning to predict the cosmological structure formation. Proc. Natl Acad. Sci. USA 116 , 13825–13832 (2019).
Article MathSciNet CAS PubMed PubMed Central ADS Google Scholar
Mahmood, F. et al. Deep adversarial training for multi-organ nuclei segmentation in histopathology images. IEEE Trans. Med. Imaging 39 , 3257–3267 (2020).
Teixeira, B. et al. Generating synthetic X-ray images of a person from the surface geometry. In Proc. IEEE Conference on Computer Vision and Pattern Recognition 9059–9067 (Institute of Electrical and Electronics Engineers, 2018).
Marouf, M. et al. Realistic in silico generation and augmentation of single-cell RNA-seq data using generative adversarial networks. Nat. Commun. 11 , 166 (2020).
Watts, D. J. A twenty-first century science. Nature 445 , 489 (2007).
boyd, d. & Crawford, K. Critical questions for big data. Inf. Commun. Soc. 15 , 662–679 (2012). This article assesses the ethical and epistemic implications of scientific and societal moves towards big data and provides a parallel case study for thinking about the risks of artificial intelligence .
Jolly, E. & Chang, L. J. The Flatland fallacy: moving beyond low–dimensional thinking. Top. Cogn. Sci. 11 , 433–454 (2019).
Yarkoni, T. & Westfall, J. Choosing prediction over explanation in psychology: lessons from machine learning. Perspect. Psychol. Sci. 12 , 1100–1122 (2017).
Radivojac, P. et al. A large-scale evaluation of computational protein function prediction. Nat. Methods 10 , 221–227 (2013).
Bileschi, M. L. et al. Using deep learning to annotate the protein universe. Nat. Biotechnol. 40 , 932–937 (2022).
Barkas, N. et al. Joint analysis of heterogeneous single-cell RNA-seq dataset collections. Nat. Methods 16 , 695–698 (2019).
Demszky, D. et al. Using large language models in psychology. Nat. Rev. Psychol. 2 , 688–701 (2023).
Karjus, A. Machine-assisted mixed methods: augmenting humanities and social sciences with artificial intelligence. Preprint at https://arxiv.org/abs/2309.14379 (2023).
Davies, A. et al. Advancing mathematics by guiding human intuition with AI. Nature 600 , 70–74 (2021).
Peterson, J. C., Bourgin, D. D., Agrawal, M., Reichman, D. & Griffiths, T. L. Using large-scale experiments and machine learning to discover theories of human decision-making. Science 372 , 1209–1214 (2021).
Ilyas, A. et al. Adversarial examples are not bugs, they are features. Preprint at https://doi.org/10.48550/arXiv.1905.02175 (2019)
Semel, B. M. Listening like a computer: attentional tensions and mechanized care in psychiatric digital phenotyping. Sci. Technol. Hum. Values 47 , 266–290 (2022).
Gil, Y. Thoughtful artificial intelligence: forging a new partnership for data science and scientific discovery. Data Sci. 1 , 119–129 (2017).
Checco, A., Bracciale, L., Loreti, P., Pinfield, S. & Bianchi, G. AI-assisted peer review. Humanit. Soc. Sci. Commun. 8 , 25 (2021).
Thelwall, M. Can the quality of published academic journal articles be assessed with machine learning? Quant. Sci. Stud. 3 , 208–226 (2022).
Dhar, P. Peer review of scholarly research gets an AI boost. IEEE Spectrum spectrum.ieee.org/peer-review-of-scholarly-research-gets-an-ai-boost (2020).
Heaven, D. AI peer reviewers unleashed to ease publishing grind. Nature 563 , 609–610 (2018).
Conroy, G. How ChatGPT and other AI tools could disrupt scientific publishing. Nature 622 , 234–236 (2023).
Nosek, B. A. et al. Replicability, robustness, and reproducibility in psychological science. Annu. Rev. Psychol. 73 , 719–748 (2022).
Altmejd, A. et al. Predicting the replicability of social science lab experiments. PLoS ONE 14 , e0225826 (2019).
Yang, Y., Youyou, W. & Uzzi, B. Estimating the deep replicability of scientific findings using human and artificial intelligence. Proc. Natl Acad. Sci. USA 117 , 10762–10768 (2020).
Youyou, W., Yang, Y. & Uzzi, B. A discipline-wide investigation of the replicability of psychology papers over the past two decades. Proc. Natl Acad. Sci. USA 120 , e2208863120 (2023).
Rabb, N., Fernbach, P. M. & Sloman, S. A. Individual representation in a community of knowledge. Trends Cogn. Sci. 23 , 891–902 (2019). This comprehensive review paper documents the empirical evidence for distributed cognition in communities of knowledge and the resultant vulnerabilities to illusions of understanding .
Rozenblit, L. & Keil, F. The misunderstood limits of folk science: an illusion of explanatory depth. Cogn. Sci. 26 , 521–562 (2002). This paper provided an empirical demonstration of the illusion of explanatory depth, and inspired a programme of research in cognitive science on communities of knowledge .
Hutchins, E. Cognition in the Wild (MIT Press, 1995).
Lave, J. & Wenger, E. Situated Learning: Legitimate Peripheral Participation (Cambridge Univ. Press, 1991).
Kitcher, P. The division of cognitive labor. J. Philos. 87 , 5–22 (1990).
Hardwig, J. Epistemic dependence. J. Philos. 82 , 335–349 (1985).
Keil, F. in Oxford Studies In Epistemology (eds Gendler, T. S. & Hawthorne, J.) 143–166 (Oxford Academic, 2005).
Weisberg, M. & Muldoon, R. Epistemic landscapes and the division of cognitive labor. Philos. Sci. 76 , 225–252 (2009).
Sloman, S. A. & Rabb, N. Your understanding is my understanding: evidence for a community of knowledge. Psychol. Sci. 27 , 1451–1460 (2016).
Wilson, R. A. & Keil, F. The shadows and shallows of explanation. Minds Mach. 8 , 137–159 (1998).
Keil, F. C., Stein, C., Webb, L., Billings, V. D. & Rozenblit, L. Discerning the division of cognitive labor: an emerging understanding of how knowledge is clustered in other minds. Cogn. Sci. 32 , 259–300 (2008).
Sperber, D. et al. Epistemic vigilance. Mind Lang. 25 , 359–393 (2010).
Wilkenfeld, D. A., Plunkett, D. & Lombrozo, T. Depth and deference: when and why we attribute understanding. Philos. Stud. 173 , 373–393 (2016).
Sparrow, B., Liu, J. & Wegner, D. M. Google effects on memory: cognitive consequences of having information at our fingertips. Science 333 , 776–778 (2011).
Fisher, M., Goddu, M. K. & Keil, F. C. Searching for explanations: how the internet inflates estimates of internal knowledge. J. Exp. Psychol. Gen. 144 , 674–687 (2015).
De Freitas, J., Agarwal, S., Schmitt, B. & Haslam, N. Psychological factors underlying attitudes toward AI tools. Nat. Hum. Behav. 7 , 1845–1854 (2023).
Castelo, N., Bos, M. W. & Lehmann, D. R. Task-dependent algorithm aversion. J. Mark. Res. 56 , 809–825 (2019).
Cadario, R., Longoni, C. & Morewedge, C. K. Understanding, explaining, and utilizing medical artificial intelligence. Nat. Hum. Behav. 5 , 1636–1642 (2021).
Oktar, K. & Lombrozo, T. Deciding to be authentic: intuition is favored over deliberation when authenticity matters. Cognition 223 , 105021 (2022).
Bigman, Y. E., Yam, K. C., Marciano, D., Reynolds, S. J. & Gray, K. Threat of racial and economic inequality increases preference for algorithm decision-making. Comput. Hum. Behav. 122 , 106859 (2021).
Claudy, M. C., Aquino, K. & Graso, M. Artificial intelligence can’t be charmed: the effects of impartiality on laypeople’s algorithmic preferences. Front. Psychol. 13 , 898027 (2022).
Snyder, C., Keppler, S. & Leider, S. Algorithm reliance under pressure: the effect of customer load on service workers. Preprint at SSRN https://doi.org/10.2139/ssrn.4066823 (2022).
Bogert, E., Schecter, A. & Watson, R. T. Humans rely more on algorithms than social influence as a task becomes more difficult. Sci Rep. 11 , 8028 (2021).
Raviv, A., Bar‐Tal, D., Raviv, A. & Abin, R. Measuring epistemic authority: studies of politicians and professors. Eur. J. Personal. 7 , 119–138 (1993).
Cummings, L. The “trust” heuristic: arguments from authority in public health. Health Commun. 29 , 1043–1056 (2014).
Lee, M. K. Understanding perception of algorithmic decisions: fairness, trust, and emotion in response to algorithmic management. Big Data Soc. 5 , https://doi.org/10.1177/2053951718756684 (2018).
Kissinger, H. A., Schmidt, E. & Huttenlocher, D. The Age of A.I. And Our Human Future (Little, Brown, 2021).
Lombrozo, T. Explanatory preferences shape learning and inference. Trends Cogn. Sci. 20 , 748–759 (2016). This paper provides an overview of philosophical theories of explanatory virtues and reviews empirical evidence on the sorts of explanations people find satisfying .
Vrantsidis, T. H. & Lombrozo, T. Simplicity as a cue to probability: multiple roles for simplicity in evaluating explanations. Cogn. Sci. 46 , e13169 (2022).
Johnson, S. G. B., Johnston, A. M., Toig, A. E. & Keil, F. C. Explanatory scope informs causal strength inferences. In Proc. 36th Annual Meeting of the Cognitive Science Society 2453–2458 (Cognitive Science Society, 2014).
Khemlani, S. S., Sussman, A. B. & Oppenheimer, D. M. Harry Potter and the sorcerer’s scope: latent scope biases in explanatory reasoning. Mem. Cognit. 39 , 527–535 (2011).
Liquin, E. G. & Lombrozo, T. Motivated to learn: an account of explanatory satisfaction. Cogn. Psychol. 132 , 101453 (2022).
Hopkins, E. J., Weisberg, D. S. & Taylor, J. C. V. The seductive allure is a reductive allure: people prefer scientific explanations that contain logically irrelevant reductive information. Cognition 155 , 67–76 (2016).
Weisberg, D. S., Hopkins, E. J. & Taylor, J. C. V. People’s explanatory preferences for scientific phenomena. Cogn. Res. Princ. Implic. 3 , 44 (2018).
Jerez-Fernandez, A., Angulo, A. N. & Oppenheimer, D. M. Show me the numbers: precision as a cue to others’ confidence. Psychol. Sci. 25 , 633–635 (2014).
Kim, J., Giroux, M. & Lee, J. C. When do you trust AI? The effect of number presentation detail on consumer trust and acceptance of AI recommendations. Psychol. Mark. 38 , 1140–1155 (2021).
Nguyen, C. T. The seductions of clarity. R. Inst. Philos. Suppl. 89 , 227–255 (2021). This article describes how reductive and quantitative explanations can generate a sense of understanding that is not necessarily correlated with actual understanding .
Fisher, M., Smiley, A. H. & Grillo, T. L. H. Information without knowledge: the effects of internet search on learning. Memory 30 , 375–387 (2022).
Eliseev, E. D. & Marsh, E. J. Understanding why searching the internet inflates confidence in explanatory ability. Appl. Cogn. Psychol. 37 , 711–720 (2023).
Fisher, M. & Oppenheimer, D. M. Who knows what? Knowledge misattribution in the division of cognitive labor. J. Exp. Psychol. Appl. 27 , 292–306 (2021).
Chromik, M., Eiband, M., Buchner, F., Krüger, A. & Butz, A. I think I get your point, AI! The illusion of explanatory depth in explainable AI. In 26th International Conference on Intelligent User Interfaces (eds Hammond, T. et al.) 307–317 (Association for Computing Machinery, 2021).
Strevens, M. No understanding without explanation. Stud. Hist. Philos. Sci. A 44 , 510–515 (2013).
Ylikoski, P. in Scientific Understanding: Philosophical Perspectives (eds De Regt, H. et al.) 100–119 (Univ. Pittsburgh Press, 2009).
Giudice, M. D. The prediction–explanation fallacy: a pervasive problem in scientific applications of machine learning. Preprint at PsyArXiv https://doi.org/10.31234/osf.io/4vq8f (2021).
Hofman, J. M. et al. Integrating explanation and prediction in computational social science. Nature 595 , 181–188 (2021). This paper highlights the advantages and disadvantages of explanatory versus predictive approaches to modelling, with a focus on applications to computational social science .
Shmueli, G. To explain or to predict? Stat. Sci. 25 , 289–310 (2010).
Article MathSciNet Google Scholar
Hofman, J. M., Sharma, A. & Watts, D. J. Prediction and explanation in social systems. Science 355 , 486–488 (2017).
Logg, J. M., Minson, J. A. & Moore, D. A. Algorithm appreciation: people prefer algorithmic to human judgment. Organ. Behav. Hum. Decis. Process. 151 , 90–103 (2019).
Nguyen, C. T. Cognitive islands and runaway echo chambers: problems for epistemic dependence on experts. Synthese 197 , 2803–2821 (2020).
Breiman, L. Statistical modeling: the two cultures. Stat. Sci. 16 , 199–215 (2001).
Gao, J. & Wang, D. Quantifying the benefit of artificial intelligence for scientific research. Preprint at arxiv.org/abs/2304.10578 (2023).
Hanson, B. et al. Garbage in, garbage out: mitigating risks and maximizing benefits of AI in research. Nature 623 , 28–31 (2023).
Kleinberg, J. & Raghavan, M. Algorithmic monoculture and social welfare. Proc. Natl Acad. Sci. USA 118 , e2018340118 (2021). This paper uses formal modelling methods to demonstrate that when companies all rely on the same algorithm to make decisions (an algorithmic monoculture), the overall quality of those decisions is reduced because valuable options can slip through the cracks, even when the algorithm performs accurately for individual companies .
Article MathSciNet CAS PubMed PubMed Central Google Scholar
Hofstra, B. et al. The diversity–innovation paradox in science. Proc. Natl Acad. Sci. USA 117 , 9284–9291 (2020).
Hong, L. & Page, S. E. Groups of diverse problem solvers can outperform groups of high-ability problem solvers. Proc. Natl Acad. Sci. USA 101 , 16385–16389 (2004).
Page, S. E. Where diversity comes from and why it matters? Eur. J. Soc. Psychol. 44 , 267–279 (2014). This article reviews research demonstrating the benefits of cognitive diversity and diversity in methodological approaches for problem solving and innovation .
Clarke, A. E. & Fujimura, J. H. (eds) The Right Tools for the Job: At Work in Twentieth-Century Life Sciences (Princeton Univ. Press, 2014).
Silva, V. J., Bonacelli, M. B. M. & Pacheco, C. A. Framing the effects of machine learning on science. AI Soc. https://doi.org/10.1007/s00146-022-01515-x (2022).
Sassenberg, K. & Ditrich, L. Research in social psychology changed between 2011 and 2016: larger sample sizes, more self-report measures, and more online studies. Adv. Methods Pract. Psychol. Sci. 2 , 107–114 (2019).
Simon, A. F. & Wilder, D. Methods and measures in social and personality psychology: a comparison of JPSP publications in 1982 and 2016. J. Soc. Psychol. https://doi.org/10.1080/00224545.2022.2135088 (2022).
Anderson, C. A. et al. The MTurkification of social and personality psychology. Pers. Soc. Psychol. Bull. 45 , 842–850 (2019).
Latour, B. in The Social After Gabriel Tarde: Debates and Assessments (ed. Candea, M.) 145–162 (Routledge, 2010).
Porter, T. M. Trust in Numbers: The Pursuit of Objectivity in Science and Public Life (Princeton Univ. Press, 1996).
Lazer, D. et al. Meaningful measures of human society in the twenty-first century. Nature 595 , 189–196 (2021).
Knox, D., Lucas, C. & Cho, W. K. T. Testing causal theories with learned proxies. Annu. Rev. Polit. Sci. 25 , 419–441 (2022).
Barberá, P. Birds of the same feather tweet together: Bayesian ideal point estimation using Twitter data. Polit. Anal. 23 , 76–91 (2015).
Brady, W. J., McLoughlin, K., Doan, T. N. & Crockett, M. J. How social learning amplifies moral outrage expression in online social networks. Sci. Adv. 7 , eabe5641 (2021).
Article PubMed PubMed Central ADS Google Scholar
Barnes, J., Klinger, R. & im Walde, S. S. Assessing state-of-the-art sentiment models on state-of-the-art sentiment datasets. In Proc. 8th Workshop on Computational Approaches to Subjectivity, Sentiment and Social Media Analysis (eds Balahur, A. et al.) 2–12 (Association for Computational Linguistics, 2017).
Gitelman, L. (ed.) “Raw Data” is an Oxymoron (MIT Press, 2013).
Breznau, N. et al. Observing many researchers using the same data and hypothesis reveals a hidden universe of uncertainty. Proc. Natl Acad. Sci. USA 119 , e2203150119 (2022). This study demonstrates how 73 research teams analysing the same dataset reached different conclusions about the relationship between immigration and public support for social policies, highlighting the subjectivity and uncertainty involved in analysing complex datasets .
Gillespie, T. in Media Technologies: Essays on Communication, Materiality, and Society (eds Gillespie, T. et al.) 167–194 (MIT Press, 2014).
Leonelli, S. Data-Centric Biology: A Philosophical Study (Univ. Chicago Press, 2016).
Wang, A., Kapoor, S., Barocas, S. & Narayanan, A. Against predictive optimization: on the legitimacy of decision-making algorithms that optimize predictive accuracy. ACM J. Responsib. Comput. , https://doi.org/10.1145/3636509 (2023).
Athey, S. Beyond prediction: using big data for policy problems. Science 355 , 483–485 (2017).
del Rosario Martínez-Ordaz, R. Scientific understanding through big data: from ignorance to insights to understanding. Possibility Stud. Soc. 1 , 279–299 (2023).
Nussberger, A.-M., Luo, L., Celis, L. E. & Crockett, M. J. Public attitudes value interpretability but prioritize accuracy in artificial intelligence. Nat. Commun. 13 , 5821 (2022).
Zittrain, J. in The Cambridge Handbook of Responsible Artificial Intelligence: Interdisciplinary Perspectives (eds. Voeneky, S. et al.) 176–184 (Cambridge Univ. Press, 2022). This article articulates the epistemic risks of prioritizing predictive accuracy over explanatory understanding when AI tools are interacting in complex systems.
Shumailov, I. et al. The curse of recursion: training on generated data makes models forget. Preprint at arxiv.org/abs/2305.17493 (2023).
Latour, B. Science In Action: How to Follow Scientists and Engineers Through Society (Harvard Univ. Press, 1987). This book provides strategies and approaches for thinking about science as a social endeavour .
Franklin, S. Science as culture, cultures of science. Annu. Rev. Anthropol. 24 , 163–184 (1995).
Haraway, D. Situated knowledges: the science question in feminism and the privilege of partial perspective. Fem. Stud. 14 , 575–599 (1988). This article acknowledges that the objective ‘view from nowhere’ is unobtainable: knowledge, it argues, is always situated .
Harding, S. Objectivity and Diversity: Another Logic of Scientific Research (Univ. Chicago Press, 2015).
Longino, H. E. Science as Social Knowledge: Values and Objectivity in Scientific Inquiry (Princeton Univ. Press, 1990).
Daston, L. & Galison, P. Objectivity (Princeton Univ. Press, 2007). This book is a historical analysis of the shifting modes of ‘objectivity’ that scientists have pursued, arguing that objectivity is not a universal concept but that it shifts alongside scientific techniques and ambitions .
Prescod-Weinstein, C. Making Black women scientists under white empiricism: the racialization of epistemology in physics. Signs J. Women Cult. Soc. 45 , 421–447 (2020).
Mavhunga, C. What Do Science, Technology, and Innovation Mean From Africa? (MIT Press, 2017).
Schiebinger, L. The Mind Has No Sex? Women in the Origins of Modern Science (Harvard Univ. Press, 1991).
Martin, E. The egg and the sperm: how science has constructed a romance based on stereotypical male–female roles. Signs J. Women Cult. Soc. 16 , 485–501 (1991). This case study shows how assumptions about gender affect scientific theories, sometimes delaying the articulation of what might be considered to be more accurate descriptions of scientific phenomena .
Harding, S. Rethinking standpoint epistemology: What is “strong objectivity”? Centen. Rev. 36 , 437–470 (1992). In this article, Harding outlines her position on ‘strong objectivity’, by which clearly articulating one’s standpoint can lead to more robust knowledge claims .
Oreskes, N. Why Trust Science? (Princeton Univ. Press, 2019). This book introduces the reader to 20 years of scholarship in science and technology studies, arguing that the tools the discipline has for understanding science can help to reinstate public trust in the institution .
Rolin, K., Koskinen, I., Kuorikoski, J. & Reijula, S. Social and cognitive diversity in science: introduction. Synthese 202 , 36 (2023).
Hong, L. & Page, S. E. Problem solving by heterogeneous agents. J. Econ. Theory 97 , 123–163 (2001).
Sulik, J., Bahrami, B. & Deroy, O. The diversity gap: when diversity matters for knowledge. Perspect. Psychol. Sci. 17 , 752–767 (2022).
Lungeanu, A., Whalen, R., Wu, Y. J., DeChurch, L. A. & Contractor, N. S. Diversity, networks, and innovation: a text analytic approach to measuring expertise diversity. Netw. Sci. 11 , 36–64 (2023).
AlShebli, B. K., Rahwan, T. & Woon, W. L. The preeminence of ethnic diversity in scientific collaboration. Nat. Commun. 9 , 5163 (2018).
Campbell, L. G., Mehtani, S., Dozier, M. E. & Rinehart, J. Gender-heterogeneous working groups produce higher quality science. PLoS ONE 8 , e79147 (2013).
Nielsen, M. W., Bloch, C. W. & Schiebinger, L. Making gender diversity work for scientific discovery and innovation. Nat. Hum. Behav. 2 , 726–734 (2018).
Yang, Y., Tian, T. Y., Woodruff, T. K., Jones, B. F. & Uzzi, B. Gender-diverse teams produce more novel and higher-impact scientific ideas. Proc. Natl Acad. Sci. USA 119 , e2200841119 (2022).
Kozlowski, D., Larivière, V., Sugimoto, C. R. & Monroe-White, T. Intersectional inequalities in science. Proc. Natl Acad. Sci. USA 119 , e2113067119 (2022).
Fehr, C. & Jones, J. M. Culture, exploitation, and epistemic approaches to diversity. Synthese 200 , 465 (2022).
Nakadai, R., Nakawake, Y. & Shibasaki, S. AI language tools risk scientific diversity and innovation. Nat. Hum. Behav. 7 , 1804–1805 (2023).
National Academies of Sciences, Engineering, and Medicine et al. Advancing Antiracism, Diversity, Equity, and Inclusion in STEMM Organizations: Beyond Broadening Participation (National Academies Press, 2023).
Winner, L. Do artifacts have politics? Daedalus 109 , 121–136 (1980).
Eubanks, V. Automating Inequality: How High-Tech Tools Profile, Police, and Punish the Poor (St. Martin’s Press, 2018).
Littmann, M. et al. Validity of machine learning in biology and medicine increased through collaborations across fields of expertise. Nat. Mach. Intell. 2 , 18–24 (2020).
Carusi, A. et al. Medical artificial intelligence is as much social as it is technological. Nat. Mach. Intell. 5 , 98–100 (2023).
Raghu, M. & Schmidt, E. A survey of deep learning for scientific discovery. Preprint at arxiv.org/abs/2003.11755 (2020).
Bishop, C. AI4Science to empower the fifth paradigm of scientific discovery. Microsoft Research Blog www.microsoft.com/en-us/research/blog/ai4science-to-empower-the-fifth-paradigm-of-scientific-discovery/ (2022).
Whittaker, M. The steep cost of capture. Interactions 28 , 50–55 (2021).
Liesenfeld, A., Lopez, A. & Dingemanse, M. Opening up ChatGPT: Tracking openness, transparency, and accountability in instruction-tuned text generators. In Proc. 5th International Conference on Conversational User Interfaces 1–6 (Association for Computing Machinery, 2023).
Chu, J. S. G. & Evans, J. A. Slowed canonical progress in large fields of science. Proc. Natl Acad. Sci. USA 118 , e2021636118 (2021).
Park, M., Leahey, E. & Funk, R. J. Papers and patents are becoming less disruptive over time. Nature 613 , 138–144 (2023).
Frith, U. Fast lane to slow science. Trends Cogn. Sci. 24 , 1–2 (2020). This article explains the epistemic risks of a hyperfocus on scientific productivity and explores possible avenues for incentivizing the production of higher-quality science on a slower timescale .
Stengers, I. Another Science is Possible: A Manifesto for Slow Science (Wiley, 2018).
Lake, B. M. & Baroni, M. Human-like systematic generalization through a meta-learning neural network. Nature 623 , 115–121 (2023).
Feinman, R. & Lake, B. M. Learning task-general representations with generative neuro-symbolic modeling. Preprint at arxiv.org/abs/2006.14448 (2021).
Schölkopf, B. et al. Toward causal representation learning. Proc. IEEE 109 , 612–634 (2021).
Mitchell, M. AI’s challenge of understanding the world. Science 382 , eadm8175 (2023).
Sartori, L. & Bocca, G. Minding the gap(s): public perceptions of AI and socio-technical imaginaries. AI Soc. 38 , 443–458 (2023).
Download references
Acknowledgements
We thank D. S. Bassett, W. J. Brady, S. Helmreich, S. Kapoor, T. Lombrozo, A. Narayanan, M. Salganik and A. J. te Velthuis for comments. We also thank C. Buckner and P. Winter for their feedback and suggestions.
Author information
These authors contributed equally: Lisa Messeri, M. J. Crockett
Authors and Affiliations
Department of Anthropology, Yale University, New Haven, CT, USA
Lisa Messeri
Department of Psychology, Princeton University, Princeton, NJ, USA
M. J. Crockett
University Center for Human Values, Princeton University, Princeton, NJ, USA
You can also search for this author in PubMed Google Scholar
Contributions
The authors contributed equally to the research and writing of the paper.
Corresponding authors
Correspondence to Lisa Messeri or M. J. Crockett .
Ethics declarations
Competing interests.
The authors declare no competing interests.
Peer review
Peer review information.
Nature thanks Cameron Buckner, Peter Winter and the other, anonymous, reviewer(s) for their contribution to the peer review of this work.
Additional information
Publisher’s note Springer Nature remains neutral with regard to jurisdictional claims in published maps and institutional affiliations.
Rights and permissions
Springer Nature or its licensor (e.g. a society or other partner) holds exclusive rights to this article under a publishing agreement with the author(s) or other rightsholder(s); author self-archiving of the accepted manuscript version of this article is solely governed by the terms of such publishing agreement and applicable law.
Reprints and permissions
About this article
Cite this article.
Messeri, L., Crockett, M.J. Artificial intelligence and illusions of understanding in scientific research. Nature 627 , 49–58 (2024). https://doi.org/10.1038/s41586-024-07146-0
Download citation
Received : 31 July 2023
Accepted : 31 January 2024
Published : 06 March 2024
Issue Date : 07 March 2024
DOI : https://doi.org/10.1038/s41586-024-07146-0
Share this article
Anyone you share the following link with will be able to read this content:
Sorry, a shareable link is not currently available for this article.
Provided by the Springer Nature SharedIt content-sharing initiative
This article is cited by
Ai is no substitute for having something to say.
Nature Reviews Physics (2024)
Perché gli scienziati si fidano troppo dell'intelligenza artificiale - e come rimediare
Nature Italy (2024)
Why scientists trust AI too much — and what to do about it
Nature (2024)
By submitting a comment you agree to abide by our Terms and Community Guidelines . If you find something abusive or that does not comply with our terms or guidelines please flag it as inappropriate.
Quick links
- Explore articles by subject
- Guide to authors
- Editorial policies
Sign up for the Nature Briefing newsletter — what matters in science, free to your inbox daily.

Search form
Doing more, but learning less: the risks of ai in research.

(© stock.adobe.com)
Artificial intelligence (AI) is widely heralded for its potential to enhance productivity in scientific research. But with that promise come risks that could narrow scientists’ ability to better understand the world, according to a new paper co-authored by a Yale anthropologist.
Some future AI approaches, the authors argue, could constrict the questions researchers ask, the experiments they perform, and the perspectives that come to bear on scientific data and theories.
All told, these factors could leave people vulnerable to “illusions of understanding” in which they believe they comprehend the world better than they do.
The paper published March 7 in Nature .
“ There is a risk that scientists will use AI to produce more while understanding less,” said co-author Lisa Messeri, an anthropologist in Yale’s Faculty of Arts and Sciences. “We’re not arguing that scientists shouldn’t use AI tools, but we’re advocating for a conversation about how scientists will use them and suggesting that we shouldn’t automatically assume that all uses of the technology, or the ubiquitous use of it, will benefit science.”
The paper, co-authored by Princeton cognitive scientist M. J. Crockett, sets a framework for discussing the risks involved in using AI tools throughout the scientific research process, from study design through peer review.
“ We hope this paper offers a vocabulary for talking about AI’s potential epistemic risks,” Messeri said.
Added Crockett: “To understand these risks, scientists can benefit from work in the humanities and qualitative social sciences.”
Messeri and Crockett classified proposed visions of AI spanning the scientific process that are currently creating buzz among researchers into four archetypes:
- In study design, they argue, “AI as Oracle” tools are imagined as being able to objectively and efficiently search, evaluate, and summarize massive scientific literatures, helping researchers to formulate questions in their project’s design stage.
- In data collection, “AI as Surrogate” applications, it is hoped, allow scientists to generate accurate stand-in data points, including as a replacement for human study participants, when data is otherwise too difficult or expensive to obtain.
- In data analysis, “AI as Quant” tools seek to surpass the human intellect’s ability to analyze vast and complex datasets.
- And “AI as Arbiter” applications aim to objectively evaluate scientific studies for merit and replicability, thereby replacing humans in the peer-review process.
The authors warn against treating AI applications from these four archetypes as trusted partners, rather than simply tools , in the production of scientific knowledge. Doing so, they say, could make scientists susceptible to illusions of understanding, which can crimp their perspectives and convince them that they know more than they do.
The efficiencies and insights that AI tools promise can weaken the production of scientific knowledge by creating “monocultures of knowing,” in which researchers prioritize the questions and methods best suited to AI over other modes of inquiry, Messeri and Crockett state. A scholarly environment of that kind leaves researchers vulnerable to what they call “illusions of exploratory breadth,” where scientists wrongly believe that they are exploring all testable hypotheses, when they are only examining the narrower range of questions that can be tested through AI.
For example, “Surrogate” AI tools that seem to accurately mimic human survey responses could make experiments that require measurements of physical behavior or face-to-face interactions increasingly unpopular because they are slower and more expensive to conduct, Crockett said.
The authors also describe the possibility that AI tools become viewed as more objective and reliable than human scientists, creating a “monoculture of knowers” in which AI systems are treated as a singular, authoritative, and objective knower in place of a diverse scientific community of scientists with varied backgrounds, training, and expertise. A monoculture, they say, invites “illusions of objectivity” where scientists falsely believe that AI tools have no perspective or represent all perspectives when, in truth, they represent the standpoints of the computer scientists who developed and trained them.
“ There is a belief around science that the objective observer is the ideal creator of knowledge about the world,” Messeri said. “But this is a myth. There has never been an objective ‘knower,’ there can never be one, and continuing to pursue this myth only weakens science.”
There is substantial evidence that human diversity makes science more robust and creative, the authors add.
“ Acknowledging that science is a social practice that benefits from including diverse standpoints will help us realize its full potential,” Crockett said. “Replacing diverse standpoints with AI tools will set back the clock on the progress we’ve made toward including more perspectives in scientific work.”
It is important to remember AI’s social implications, which extend far beyond the laboratories where it is being used in research, Messeri said.
“ We train scientists to think about technical aspects of new technology,” she said. “We don’t train them nearly as well to consider the social aspects, which is vital to future work in this domain.”
Science & Technology
Social Sciences
Media Contact
Bess Connolly : [email protected] ,

What have the bots learned about us?

Engineering a heart conduit

Day of Service 2024 winning t-shirt design announced

Uncovering new patterns behind economic transformation in Africa
- Show More Articles
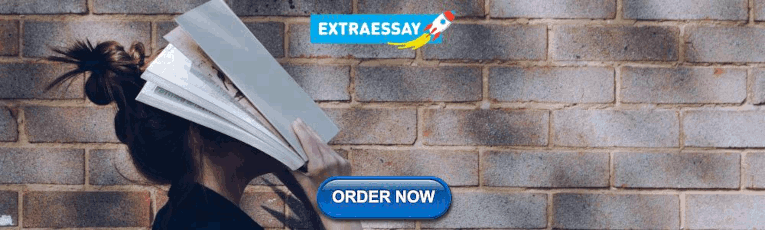
Deep learning for misinformation detection on online social networks: a survey and new perspectives
- Review Paper
- Published: 29 September 2020
- Volume 10 , article number 82 , ( 2020 )
Cite this article
- Md Rafiqul Islam ORCID: orcid.org/0000-0001-7209-3881 1 ,
- Shaowu Liu 1 ,
- Xianzhi Wang 2 &
- Guandong Xu 1
33k Accesses
123 Citations
6 Altmetric
Explore all metrics
Recently, the use of social networks such as Facebook, Twitter, and Sina Weibo has become an inseparable part of our daily lives. It is considered as a convenient platform for users to share personal messages, pictures, and videos. However, while people enjoy social networks, many deceptive activities such as fake news or rumors can mislead users into believing misinformation. Besides, spreading the massive amount of misinformation in social networks has become a global risk. Therefore, misinformation detection (MID) in social networks has gained a great deal of attention and is considered an emerging area of research interest. We find that several studies related to MID have been studied to new research problems and techniques. While important, however, the automated detection of misinformation is difficult to accomplish as it requires the advanced model to understand how related or unrelated the reported information is when compared to real information. The existing studies have mainly focused on three broad categories of misinformation: false information, fake news, and rumor detection. Therefore, related to the previous issues, we present a comprehensive survey of automated misinformation detection on (i) false information, (ii) rumors, (iii) spam, (iv) fake news, and (v) disinformation. We provide a state-of-the-art review on MID where deep learning (DL) is used to automatically process data and create patterns to make decisions not only to extract global features but also to achieve better results. We further show that DL is an effective and scalable technique for the state-of-the-art MID. Finally, we suggest several open issues that currently limit real-world implementation and point to future directions along this dimension.
Similar content being viewed by others
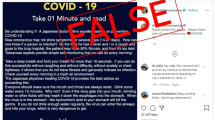
Fake news, disinformation and misinformation in social media: a review
Esma Aïmeur, Sabrine Amri & Gilles Brassard
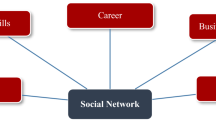
Online social networks security and privacy: comprehensive review and analysis
Ankit Kumar Jain, Somya Ranjan Sahoo & Jyoti Kaubiyal
Social Media Account Hacking Using Kali Linux-Based Tool BeEF
Avoid common mistakes on your manuscript.
1 Introduction
On online social networks such as Facebook Footnote 1 , Twitter Footnote 2 , and Sina Weibo Footnote 3 , people share their opinions, videos, and news on their various activities (Gao and Liu 2014 ; Islam et al. 2018a ). While people enjoy social networks, many deceptive activities such as fake news, or rumors can mislead users into believing misinformation (Kumar et al. 2016 ). Therefore, MID in social networks has gained a great deal of attention and is considered an emerging area of research interest recently (Wu et al. 2019 ; Goswami and Kumar 2016 ). However, the automated detection of misinformation is difficult to accomplish as it requires the advanced model to understand how related or unrelated the reported information is when compared to real information (Wu et al. 2019 ). Also, to solve many complex MID problems, academia and industry researchers have applied DL to a large number of applications to make decisions (Xu et al. 2019 ; Yenala et al. 2018 ; Yin et al. 2020 ). Therefore, this survey seeks to provide such a systematic review of current research on MID based on DL techniques.
Social network (SN) sites are a dynamic platform that is now being utilized for different purposes such as education, business, medical purposes, telemarketing, but also, unfortunately, unlawful activities (Vartapetiance and Gillam 2014 ; Wu et al. 2019 ; Naseem et al. 2020 ). Generally, people use SN to socialize with their interested friends and colleagues. Additionally, it is utilized as a channel to speak with clients, and its information can be valuable for identifying new patterns in business insights (Bindu et al. 2017 ). Figure 1 illustrates the rapid information exchange between users regardless of their location. For example, on online social media, businesses share their product marketing, organizations share their daily activities, celebrities share news on their various activities, and government bodies share information on their various responsibilities. As a result, businesses can offer discounts on their products based on observations of current market demand, customer feedback, etc. Besides, they have realized that online marketing is spreading faster than manual marketing (Acquisti and Gross 2009 ; Tsui 2017 ; Nguyen et al. 2017a ; Quah and Sriganesh 2008 ). Similarly, celebrities use SN to increase their public exposure, and the government uses it to collect public opinions. However, it also opens the door for unlawful activities which harm society, business markets, healthcare systems, etc. where incorrect or misleading information is intentionally or unintentionally spread (Bharti et al. 2017 ; Sun et al. 2018 ; Gao and Liu 2014 ). Therefore, SN has attracted a lot of attention and is considered to be a developing interdisciplinary research area that aims to analyze, combine, explore, and adjust techniques to investigate SN data globally. Although existing studies might consider the concept of misinformation in a different view, we consider MID in social media is a timely matter of concern (Sharma et al. 2019 ; Shu et al. 2020 ).
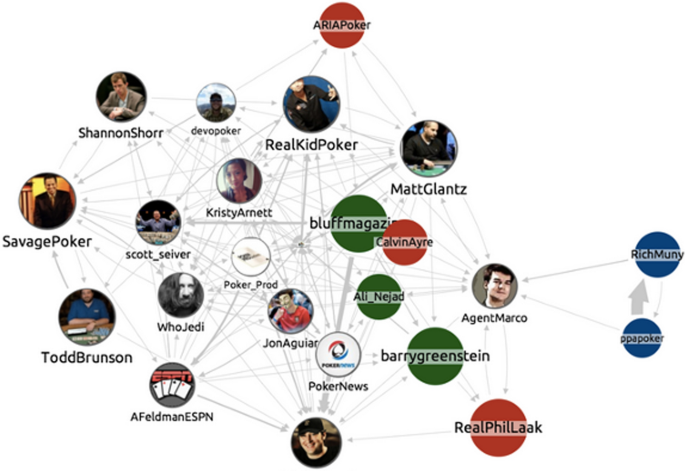
Social relationships between different users
Misinformation is inaccurate information which is created to misguide the readers (Fernandez and Alani 2018 ; Zhang et al. 2018a ). There are numerous terms related to misinformation including fake news, rumors, spam, and disinformation, which usually contain numerical, categorical, textual, image, etc., data and used to initiate terrible outcomes (Ma et al. 2016 ; Bharti et al. 2017 ; Helmstetter and Paulheim 2018 ). Due to the high dependency on social media, many dishonest people get a chance to spread misinformation via a false account (Kumar and Shah 2018 ; Shu et al. 2019c ). Additionally, the information they provide is well written, long, and well referenced. So, the readers trust their activities. However, the spread of misinformation will be ineffective if people can identify the different types of misbehavior including fake reviews, false information, and rumors. And, identifying misinformation using SN data may provide early feedback on emerging issues, such as stock movement, political gossip, social issues, and business performance (Habib et al. 2019 ). In this regard, various techniques have been applied to differentiate genuine and fraudulent information or users over the past years (Islam et al. 2018a ; De Choudhury et al. 2013a ; De Choudhury et al. 2013b ). However, it is very difficult for traditional methods to analyze all these types of misinformation. Therefore, deep learning-based detection approaches can be designed to fit various types of features for MID.
The development of machine learning (ML) and DL techniques have attracted significant attention for different purposes both from industry and research communities (LeCun et al. 2015 ; Islam et al. 2019 ). In particular, DL-based detection approaches have become a major source of MID. For example, a large volume of research works have explored on automatic MID (Jain et al. 2016 ; Qazvinian et al. 2011 ; Zhang et al. 2016 ), as well as related terms, e.g., rumor (Sampson et al. 2016 ; Wu et al. 2017 ), fake information (Kwon et al. 2017 ; Ma et al. 2016 ; Ruchansky et al. 2017 ; Shu et al. 2017 ; Wu et al. 2017 ), and spam detection (Hu et al. 2013 ; Yin et al. 2018 ; Li and Liu 2018 ; Markines et al. 2009 ; Wang et al. 2011 ). Therefore, the success of DL for MID both in academia and industry requires a systematic review to better understand the scenarios of the existing problem and current research issues. Although there have been attempts to review and summarize the literature on MID in a very nice way, there are still enough spaces to review the literature on misinformation in a broader way. For example, in an existing survey, Shu et al. ( 2017 ) shows a fascinating association between psychological concept, fake news, and social network with data mining techniques. The literature surveyed by Zubiaga et al. ( 2018 ), Yu et al. ( 2017b ), and Zhang et al. ( 2015 ) illustrates a related problem of rumor detection, where they differentiate between unverified and verified information, wherein the unverified information may remain unresolved or may turn out to be true or false. Additionally, Kumar and Shah ( 2018 ) addressed a broader scope of false information on the web which presents the existing work, current progress, and future directions together. However, from the existing studies, we find that (1) there is no clear boundary definition between misinformation, disinformation, and false information, and (2) there are no DL method-based systematic reviews on MID whether different types of misinformation problems have been summarized under each DL technique. We wish to emphasize that misinformation is getting more and more complex, making the conventional machine learning techniques incapable of detecting them. For example, due to the recent advances in large-scale pre-trained models (e.g., BERT, GPT-3) and adversarial learning, programs can generate misinformation in an automated and difficult to detect manner. This calls for the need of using high capacity models, such as DL. Therefore, although existing reviews are important in their own right, much like the detection of scam email (Saberi et al. 2007 ), fake followers (Cresci et al. 2015 ), or false web links (Lake 2014 ), we decide to focus on MID to provide detailed discussions of DL techniques and their limitations.
The existing surveys covered a broad range of techniques used for MID. However, given the increasing popularity of using DL methods to detect misinformation, we believe our survey provides a timely review of the use of DL techniques. For example, we reviewed how different MID problems are covered under various DL techniques, which were not covered in existing surveys. We hope this survey can benefit researchers to deep insight between related techniques and these issues. Moreover, despite the promising outcomes of DL techniques, we focus on some open issues such as data volume, data quality, explainability, domain complexity, interpretability, feature enrichment, model privacy, incorporating expert knowledge, and temporal modeling, which are necessary to understand the advances in this domain. In summary, the main contributions of our survey are as follows:
We present a state-of-the-art systematic review of the existing problems, solutions, and validation of MID in online social networks based on various DL techniques.
To identify the recent and future trends of MID research, we analyze the key strengths and limitations of the existing various techniques and describe the state-of-the-art DL as an emerging technique on massive social network data.
We provide some open issues that contribute to this new exciting field based on DL techniques.
In the rest of the paper, first, we present the MID with the formal problem definition, types, impacts, and DL with the associated challenges. We then present the state-of-the-art DL techniques for MID. Further, to encourage researchers with rigorous evaluation and comparison, we include a list of open issues that outline promising directions for future research. Finally, we present the conclusion.
2 Background
2.1 misinformation detection.
In this section, we discuss the formal definition of misinformation, types, and its impact on SN. But we do not discuss in this section how to undertake MID, what techniques are used, and what techniques are effective. The introduction of this paper has shown that DL is now an emerging technique that plays a critical role in MID. As our main task is to review the learning process of DL for MID, in the following sections, we discuss the importance of DL for MID, overview its existing performance, and provide some open issues to work in the future.
2.1.1 What is misinformation?
Misinformation is a false statement to lead people astray by hiding the correct facts. It is also referred to as deception, ambiguity, falsehoods, etc. (Zhang et al. 2016 ). It generates feelings of mistrust that subsequently weaken relationships, which is a negative violation of expectations (Wu et al. 2019 ; Ma et al. 2018 ). Additionally, people do not expect to receive misinformation from their close friends, relatives, or strangers. Instead, they expect truthful communication. For example, some people were involved in a Facebook discussion on a recently published product where there are both fake users and real users. The real users discuss the product’s features honestly. However, fake users praise the product regardless of their true opinion.
Problem definition Suppose you have been given a restaurant review of E among N users to analyze user feedback a, which contains both genuine feedback and false reviews created by restaurant owners. It is very difficult to distinguish the false review from the true ones. Therefore, the researcher’s role is to identify the real and false reviews.
According to Wu et al. ( 2019 ), in the following section, we provide five major terms related to MID, namely rumor, fake news, false information, spam, and disinformation as shown in Fig. 2 . We then describe a brief literature review of existing DL techniques for MID in Sect. 3 .
2.1.2 Types of misinformation
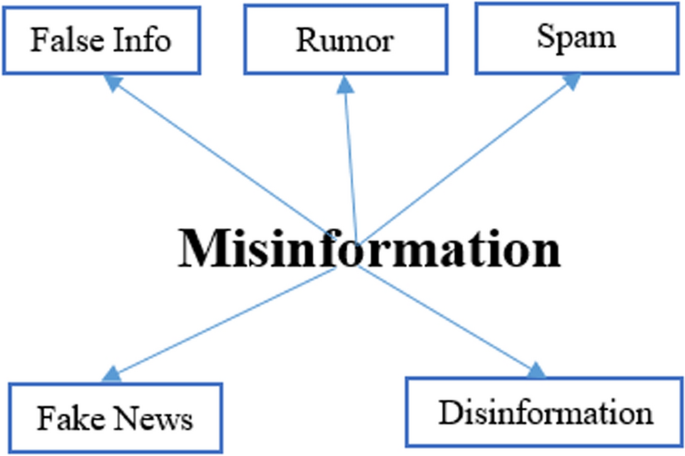
Key types related to misinformation
There are many terms related to misinformation such as rumor, fake news, false information, spam, and disinformation. Rumor is a story of circulating information from person to person whose veracity status is doubtful (Lin et al. 2019 ). Fake news is a news article that intentionally misleads the readers, and it is verifiably false (Shu et al. 2018 ). Misinformation can be broadly used to treat information as False information (Kumar and Shah 2018 ). Spam can be referred as an unsolicited message which sends over the internet for spreading malware, advertising, etc. (Rayana and Akoglu 2015 ; Hu et al. 2013 ). Disinformation is a piece of inaccurate information that is spread intentionally to mislead people (Galitsky 2015 ). Although misinformation and disinformation both refer to incorrect/false information, a big difference between them lies in the intention - without the intent misinformation is spread to deceive while disinformation is spread with the intent (Kumar et al. 2016 ; Hernon 1995 ; Fallis 2014 ). Several studies have been forwarded for misinformation identification on social media (Kumar and Shah 2018 ; Wu et al. 2019 ). Some works treat a microblog post an object, obtain the credibility of the post, and aggregate to the event level (Jin et al. 2017a ), (Jindal et al.), (Qazvinian et al. 2011 ; Gupta et al. 2013 ). Additionally, some work extracts various features from the event level and identifies whether an event belongs to misinformation (Kwon et al. 2017 ; Ma et al. 2016 ; Zhang et al. 2016 ). Moreover, some other works extract more effective hand-crafted features, including conflict viewpoints (Jin et al. 2017c ), temporal properties (Kwon et al. 2017 ; Ma et al. 2019 ), users’ feedback (Shu et al. 2020 ), and signals tweets containing skepticism (Zhao et al. 2015 ). Therefore, to better understand misinformation in social media, Fig. 2 illustrates the five related terms of misinformation such as false information, rumors, fake news, spam, and disinformation. In this section, we define and describe each type of misinformation, respectively.
False information False information is a broader concept of misinformation. Intentionally, it is interchangeably used to define as a correct information. In social networks, some unscrupulous people exploit this for their interests by ensuring that the basic patterns of the original information are correct (Kumar and Shah 2018 ; Habib et al. 2019 ). For example, we generally expect honest users to provide positive reviews to good products and negative reviews to bad products, whereas dishonest users may not follow this behavior. Existing studies estimated that 20% of the reviews on Yelp are fake (Donfro 2013 ). This large number of fake reviews, which are getting more and more difficult to detect, call for the use of advanced DL techniques to extract meaningful features and to identify the review as fake or real accurately.
Rumor A rumor is a story of doubtful truth that is easy to spread widely online (Zubiaga et al. 2017 ). The rumor is spread by dishonest business people for their benefit (Zubiaga et al. 2018 , 2016b ). For example, a rumor spreads on social media that recently the price of salt and onion had increased in Bangladesh and some shops quickly increased their prices Footnote 4 , Footnote 5 . In this regard, some people purchased more of these products than they needed. Additionally, Fig. 3 depicts an interesting rumor campaign about “Saudi Arabia’s first female robot citizen beheaded,” which shows how popular index patterns are overwhelmed by propaganda terms expressing doubts and disagreements like “fake news.”
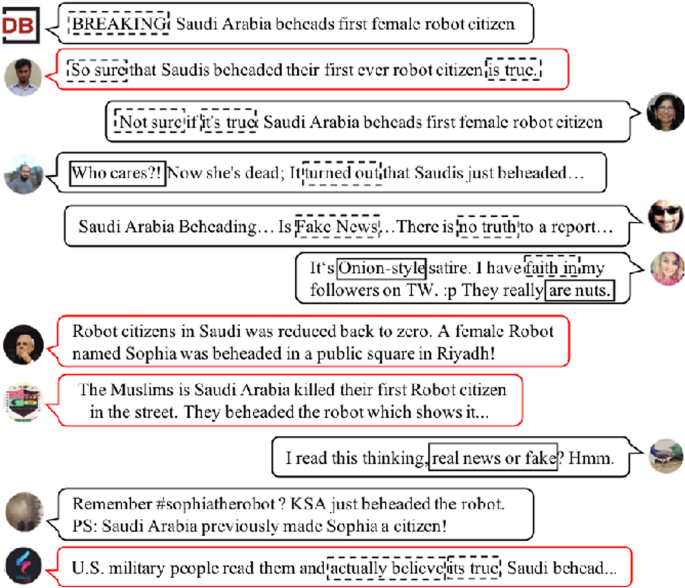
Sample responses to a rumor claim. Figure courtesy by Ma et al. ( 2019 )
Fake news Fake news is a modified version of an original news story which is spread intentionally and very difficult to identify (Cui et al. 2019 ). It mimics traditional news and spreads easily on social media, reaches a large number of people quickly, and deceives many (Kumar et al. 2019 ; Shu et al. 2017 ).
Spam Spam is an unwanted message that generally contains irrelevant or inappropriate information to mislead users (Yilmaz and Durahim 2018 ). It is difficult to distinguish spam from real messages, as spammers hack users’ information (Çıtlak et al. 2019 ).
Disinformation Disinformation is a subset of misinformation, which is false or misleading information. It is intentionally spread online to deceive others, and its impact has continued to grow (Hernon 1995 ; Galitsky 2015 ). Misinformation is conveyed in the honest but mistaken belief that the relayed incorrect facts are true. However, disinformation defines false facts that are conceived to deliberately deceive an audience. One recent disinformation example is pure alcohol that can cure the coronavirus infections during the COVID-19 pandemic situation. However, pure alcohol can be very harmful to the human body Footnote 6 , Footnote 7 .
In summary, we compare the five related terms of misinformation as shown in Table 1 . For example, on characteristics, disinformation provides misleading features that also have a specific objective. Additionally, different types of misinformation have different categories of side effects. On integrity, we use sure and not sure levels to evaluate five different types of misinformation.
2.1.3 Impact of misinformation
Misinformation can affect every aspect of life such as the social, political, economic, stock market, emergency response during natural disasters, and crisis events. It aims to intentionally or unintentionally mislead public opinions, influence political elections, and threaten public security and social stability (Wu et al. 2019 ). Most of the time, it reveals fabricated information related to fictional issues rather than relevant information (Fernandez and Alani 2018 ). Nowadays, it has become easier to spread misinformation quickly due to social network platforms such as Facebook, Twitter, and Sina Weibo. In particular, when people engage in conversation, one can share information that is stated to be factual but that may not always be true. Additionally, fraudulent users share misleading information to look for personal gain in some way. For example, concerning political issues, some view being a misled resident as more regrettable than being an uninformed resident. Misguided residents express their opinions with certainty and thus influence in elections. This kind of deception originates from speakers not continually being forthright and clear.
With the advent of SN and technological advances around the world, there has been a great explosion of misinformation (Kumar et al. 2016 ; Sharma et al. 2019 ; Goswami and Kumar 2016 ). In the last few decades, several studies have been conducted to measure the impact of misinformation such as rumors, fake reviews, and fake news. For example, Friggeri et al. ( 2014 ) studied the spread of rumors on Facebook, and (Willmore) analyzed the use of fake election news articles on Facebook. Another work by Zubiaga et al. ( 2018 ) discussed how rumors spread quickly on social media (Twitter) and how this is becoming a threat to many people. They stated that misinformation has a significant negative impact on the workplace and daily life. For example, an organization can undermine reliable evidence through a purposeful deception campaign. In detail, tobacco companies utilized falsehood in half of the twentieth century to diminish the reliability of studies that showed the connection between smoking and lung disease (Brandt 2012 ). In the clinical field, misinformation can quickly prompt life endangerment as found in the case of the public’s negative observation toward vaccines to treat diseases.
Overall, in the context of misinformation, existing studies focus on the text content mostly, whereas a few of them investigated image/video content (Jin et al. 2016 ; Gupta et al. 2014 ). Although many techniques used for MID, all the approaches have not been proved effective yet (Zhang et al. 2016 ; Shu et al. 2017 ). Additionally, existing approaches have some challenges for MID, e.g. data volume, data quality, domain complexity, interpretability, feature enrichment, model privacy, incorporating expert knowledge, temporal modeling, dynamic, etc. (Liu and Xu 2016 ; Ma et al. 2015 ). Therefore, we attempt to introduce DL as an emerging state-of-the-art technique for MID.
2.2 Deep learning
The term deep learning (DL) was first introduced to the machine learning community by Dechter ( 1986 ) and to artificial neural networks based on a Boolean threshold by Aizenberg ( 1999 ). In the field of ML in artificial intelligence, DL is an emerging technique which is used in various applications including computer vision (Wang and Yeung 2013 ), speech recognition (Hinton et al. 2012 ), natural language processing (Young et al. 2018 ), anomaly detection (Du et al. 2017 ), portfolio optimization (Vo et al. 2019 ), healthcare monitoring (Islam et al. 2018b ), personality mining (Vo et al. 2018 ), novelty detection in robot behavior, traffic monitoring, visual data processing, social network analysis, etc. Nowadays, it is becoming increasingly used for processing data and creating patterns to assist the decision-making process. Furthermore, this state-of-the-art method helps to improve learning execution, expand the scope of the research area, and simplify the measuring procedure.
Over the few decades, various techniques have been proposed to solve many problems (fake news, misinformation, anomaly detection, etc.) in the online social network. Researchers are constantly finding and investigating research gaps in various domains and attempting to solve these problems using various techniques. Deep learning is one such technique and has become increasingly popular, being explored in a large number of domains with various neural networks such as convolutional neural networks (CNN) (Abdel-Hamid et al. 2014 ; Kim 2014 ), recurrent neural networks (RNN) (Cho et al. 2014b ; Li and Wu 2015 ), and long short-term memory (LSTM) (Sun et al. 2018 ), which are introduced to help other researchers explore their knowledge in different applications.
Deep learning serves as the key to performing complex tasks of higher levels of sophistication. However, to successfully build and deploy them proves to be a challenge for data scientists and engineers all over the world (Liu and Wu 2020 ; Hardy et al. 2016 ). Although data training takes a little longer, testing can be done in a very short time. To accelerate DL processing, DL frameworks combine the implementation of modularized DL algorithms, optimization techniques, distribution techniques, and support to infrastructures (Chalapathy and Chawla 2019 ; David and Netanyahu 2015 ). They are developed to simplify the implementation process and boost system-level development and research. Table 2 shows some popular DL frameworks such as Caffe, Torch, TensorFlow, MXNet, and CNTK, which allow researchers to develop tools and can offer a better level of abstraction and simplify difficult programming challenges. Each framework is built differently for different purposes. It can be observed from Table 2 that most of the frameworks are implemented in Python, which is the most common language for DL architecture design. It can make programming more efficient and easier by simplifying the programming process.
3 Deep learning for misinformation detection: state-of-the-art
Misinformation detection is defined as an observation that deviates greatly from other observations and thereby arouses suspicion that it was generated by a different mechanism. In Sect. 2.1.2 , we have discussed different terms related to misinformation with examples. It is observed that the same type of problem has been solved by many techniques. Although many techniques are being used to detect misinformation in social network data, DL is one of the better approaches to use. However, the same type of misinformation problems has been solved with various DL techniques (Table 3 ). Additionally, these types of DL techniques are dependent on different data characteristics and used to automatically identify misinformation. Therefore, we have divided the DL techniques into three main categories based on the model as follows: (1) discriminative models, (2) generative models, and (3) hybrid models. All three categories have a large number of architectural models that are commonly used for MID. However, due to differences in performance, we only discussed 12 models namely convolutional neural networks (CNN), recurrent neural networks (RNN), recursive neural networks (RvNN), restricted Boltzmann machines (RBM), deep Boltzmann machines (DBM), deep belief networks (DBN), variational autoencoders (VAE), convolutional restricted Boltzmann machines (CRBM), convolutional recurrent neural networks (CRNN), ensemble-based fusion (EBF), and long short-term memory (LSTM), as shown in Fig. 4 . We discuss each model that uses for MID, respectively.
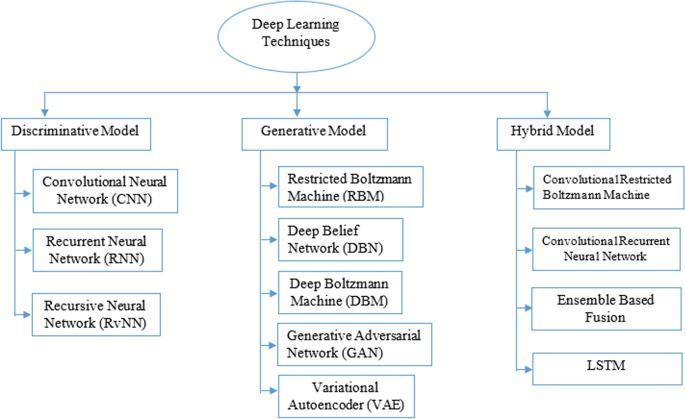
Classification of deep learning models
3.1 Discriminative model for detecting misinformation
A variety of discriminative models used social content and context-based features for MID. In recent years, to tackle the problem of misinformation, several studies have been conducted and revealed some promising preliminary results. Therefore, we briefly review the three discriminative models namely CNN, RNN, and RvNN, respectively. It is noted that the discriminative-based models have demonstrated significant advances in text classification and analysis.
Convolutional Neural Network (CNN) CNN is one of the most popular and widely used models for the state-of-the-art of many computer vision tasks (LeCun et al. 2010 ). However, recently, it has been extensively applied in the NLP community as well (Jacovi et al. 2018 ). For example, Chen et al. ( 2017 ) introduced a convolutional neural network-based classification method with single and multi-word embedding for identifying both rumor and stance tweets. Kumar et al. ( 2019 ) introduced both a CNN and a bidirectional LSTM ensembled network with an attention mechanism to solve MID. Additionally, Yang et al. ( 2018 ) stated that online social media is continually growing in popularity and genuine users are being attacked by many fraudulent users. They informed that fake news is written to intentionally mislead users. In their paper, they applied the TI-CNN model to identify the explicit and latent features from the text and image information. They demonstrated that their model solves the fake news detection problem effectively.
Recurrent Neural Network (RNN) RNN utilizes the sequential information in the network which is essential in many applications where the embedded structure in the data sequence conveys useful knowledge (Alkhodair et al. 2020 ). The advantage of RNN is its ability to better capture contextual information. To detect rumors, existing methods rely on handcrafted features to employ machine learning algorithms that require a huge manual effort. To guard against this issue, the earliest adoption of RNNs for rumor detection is reported in Ma et al. ( 2016 ) and recurrent neural networks with attention mechanism in Chen et al. ( 2018 ) and Jin et al. ( 2017b ). Figure 5 shows the RNN architecture used for the fake news detection proposed by (Shu et al. 2019a ). Authors have proposed different RNN architectures, namely tanh-RNN, LSTM and Gated Recurrent Unit (GRU) (Cho et al. 2014a ). Among the proposed architectures, GRU has obtained the best results in both the datasets considered, with 0.88 and 0.91 accuracy, respectively. Ma et al. ( 2016 ) proposed a RNN model to learn and that captures variations in relevant information in posts over time. Additionally, they described that RNN utilizes the sequential information in the network where the embedded structure in the data sequence conveys useful knowledge. They demonstrated that their proposed model can capture more data from hidden layers which give better results than the other models.
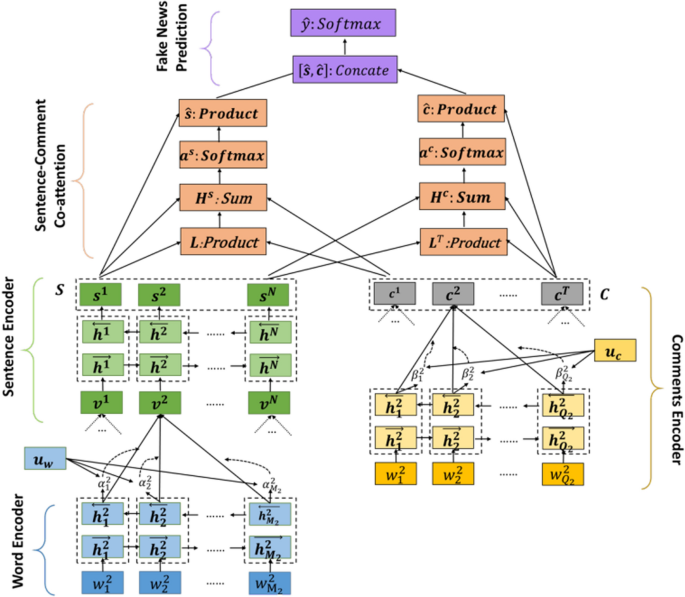
RNN architecture used for fake news detection. Figure courtesy by Shu et al. ( 2019a )
Recursive Neural Network (RvNN) Researchers are more concerned to identify unscrupulous users in SN and want to protect genuine users from fraudulent behavior (Guo et al. 2019 ). Therefore, RvNN is one of the most widely used and successful networks for many natural language processing (NLP) tasks (Socher et al. 2013 ; Zubiaga et al. 2016a ). This architecture processes objects that can make predictions in a hierarchical structure and classifies the outputs using compositional vectors. To reproduce the patterns of the input layer to the output layer, this network is trained by auto-association. Also, this model analyzes a text word by word and stores the semantics of all the previous texts in a fixed-sized hidden layer (Cho et al. 2014b ). For instance, Zubiaga et al. ( 2016b ) proposed a RvNN architecture for handling the input of different modalities. Ma et al. ( 2018 ) proposed a model that collects tweets from Twitter and extracts features from discriminating information. It follows a non-sequential pattern to present a more robust identification of the various types of rumour-related content structures.
3.2 Generative model for detecting misinformation
Over the last few decades, online social media platforms have become the main target space of deceptive opinions where deceptive opinions (such as rumor, spam, troll, fake news) are deliberately written to sound authentic. Several existing works for MID are based on syntactic and lexical patterns or features of opinion. Therefore, in this section, the successful use of five generative models on various classification applications, namely RBM, DBN, DBM, GAN, and VAE are discussed.
Restricted Boltzmann Machine (RBM) RBM is a generative stochastic artificial neural network. It can learn a probability distribution over its set of inputs (Liao et al. 2016 ). Although learning is impractical in general Boltzmann machines, it can be quite efficient in an architecture called the restricted Boltzmann machine. However, it does not allow intra-layer connections between hidden units (Papa et al. 2015 ). Therefore, this method of stacking RBMs makes it possible to train many layers of hidden units efficiently. RBMs have been applied in various applications, but very few works have been addressed in the context of MID. However, in the last few decades, researchers are attempting to fit this method to identify fake, rumour, spam, etc. on social media platforms. For instance, Da Silva et al. ( 2018 ), da Silva et al. ( 2016 ), and Silva et al. ( 2015 ) applied RBMs to automatically extract the features related to spam detection.
Deep Belief Network (DBN) DBN is a generative graphical model composed of multiple layers of latent variables (hidden units). It connects between the layers but not between units within each layer. DBNs can be viewed as a composition of simple, unsupervised networks such as restricted Boltzmann machines (RBMs) or autoencoders, where each subnetwork’s hidden layer serves as the visible layer for the next. There are already many works that have used this network (Li et al. 2018b ; Yepes et al. 2014 ; Alom et al. 2015 ; Selvaganapathy et al. 2018 ). For example, Tzortzis and Likas ( 2007 ) stated that spam is an unexpected message which contains inappropriate information and first applied to fit DBNs for spam detection. In another paper, Wei et al. ( 2018 ) proposed a DBN-based method to identify false data injection attacks in the smart grid. They demonstrated that the DBN-based method achieves a better result than the traditional SVM-based approach.
Deep Boltzmann Machine (DBM) DBM is a type of binary pair-wise markov random field with multiple layers of hidden random variables. This is a network of symmetrically coupled stochastic binary units which have been used to detect malicious activities (Zhang et al. 2012 ; Dandekar et al. 2017 ). For example, Jindal et al. used a multimodal benchmark dataset for fake news detection. They presented results from a Deep Boltzmann Machine-based multimodal DL model (Srivastava and Salakhutdinov 2012 ). Zhang et al. ( 2012 ) generated a model based on DBMs to detect spoken queries. They presented that their proposed method achieved 10.3% improvement compared to that with the previous Gaussian model.
Generative Adverserial Network (GAN) GAN is a class of ML systems (Goodfellow et al. 2014 ). Given a training set, this technique learns to generate new data with the same statistics as the training set. When considering earlier studies, we see that the widespread rumors usually result from the deliberate dissemination of information which is generally aimed at forming a consensus on rumor news events. Ma et al. ( 2019 ) proposed a generative adversarial network model to make automated rumor detection more robust and efficient and is designed to identify powerful features related to uncertain or conflicting voice production and rumors. Figure 6 illustrates the structure of a deep generative adversarial learning model for rumors detection proposed by Ma et al. ( 2019 ).
Variational Autoencoder (VAE) VAE models make strong assumptions concerning the distribution of latent variables. The use of a variational approach for latent representation learning results in an additional loss component and a specific estimator for the training algorithm called the stochastic gradient variational bayes (SGVB) estimator. Qian et al. ( 2018 ) proposed a generative conditional VAE model to extract new patterns by analyzing a user’s past meaningful responses on true and false news articles and played a vital role in detecting misinformation on social media. Wu et al. ( 2017 ) explored whether the knowledge from the historical data analysis can benefit rumor detection. The result of their study was that similar rumors always produce the same behaviors.

The architecture of the generative adversarial learning model. Figure courtesy by Ma et al. ( 2019 )
3.3 Hybrid model for detecting misinformation
The tasks of detecting misinformation (such as fake news, rumor, spam, troll, false information, and disinformation) have been made in a variety of ways. A lot of research works have been done using various DL models separately. However, to increase the performance of individual models, the need for hybrid models are immense. Therefore, over the last few decades, hybrid DL has been considered an emerging technique for various purposes. In this section, we review some related works on MID based on the deep hybrid model. The hybrid model consists of CRBM, CRNN, EBF, and LSTM.
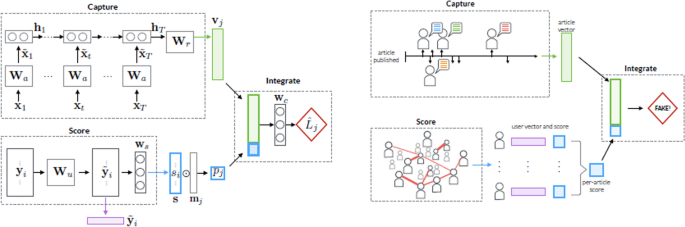
An illustration of the hybrid model. Figure courtesy by Ruchansky et al. ( 2017 )
Convolutional Recurrent Neural Network (CRNN) Currently, researchers are increasingly focusing on applying CNN and RNN models in a hybrid way to achieve better performance in various applications. They argue that real-world data are structured sequences, with spatio-temporal sequences. For example, several works utilized a blend of CNN and RNN such as spatial and temporal regularities (Lin et al. 2019 ; Xu et al. 2019 ; Wang et al. 2019 ). Their models can process time-shifting visual contributions for variable length expectations. These neural network architectures combine a CNN for visual element extraction with an RNN for grouping learning. Besides, such models have been effectively utilized for fake news, rumor, false information, and spammer detection. For example, to identify rumor for events on social media platform, Lin et al. ( 2019 ) proposed a novel rumor detection method based on a hierarchical recurrent convolutional neural network. They use the RCNN model to learn contextual information and utilize the bidirectional GRU network to learn time period information. Figure 7 shows the structure of a deep hybrid model for fake news detection proposed by Ruchansky et al. ( 2017 ). Xu et al. ( 2019 ) proposed a CRNN model to extract data from textual overlays, for example, captions, key ideas, or scene level summaries for rumor detection on Sina Weibo. They proposed this CRNN model to create training data intended for textual overlays regularly occurring in the online sina weibo platform. Zhang et al. ( 2018c ) proposed an approach called deceptive review identification by recurrent convolutional neural network (DRI-RCNN) to identify the deceptive review of the content. They compared the neural network approaches (RvNN, LB-SVM, CNN, and RCNN, GRNN) to the widely used conventional strategies. Their experimental results demonstrated that the neural network approaches outperform the conventional techniques for all datasets.
Convolutional Restricted Boltzman Machine (CRBM) An extension of the RBM model, called the convolutional RBM (CRBM), was developed by Norouzi ( 2009 ). He informed that CRBM, like the RBM, is a two-layer model in which visible and hidden arbitrary factors are organized as matrices. He proposed a way to make a Boltzmann machine and convolutional limited Boltzmann machine, forming a deep network to improve its presentation for both image processing and feature extraction. He also provided a simple and intuitive training method that jointly optimizes all RBMs in the network, which works well in practice. For instance, Norouzi et al. ( 2009 ) proposed a CRBM model for learning features specific to an object class. In which associations are nearby and loads are shared with the spatial structure of pictures and stack them over one another to construct a multilayer progressive system of exchanging, separating, and pooling.
Ensemble-Based Fusion To study profile information, Wang ( 2017 ) proposed a hybrid model where he used speaker profiles as a part of the input data. He also made the first large-scale fake news detection benchmark dataset with speaker details information such as location of speech, party affiliation, job title, credit history, as well as topic. Tschiatschek et al. ( 2018 ) investigated the vital problem of leveraging crowd signals to detect fake news. They analyzed user’s flagging behaviors and applied novel algorithms detective to perform Bayesian inference to detect fake news. Their experiments performed well in identifying a genuine user’s flagging behavior. Zhang et al. ( 2018b ) explored a new idea to detect fake news on social media. They identified some deceptive words which can be used by these online fake users and harm offline society. Shu et al. ( 2018 ) discussed that social media has become a popular network for sharing misinformation and presented FakeNewsNet as fake news data respiratory for further analysis. Roy et al. ( 2018 ) presented misinformation and applied many various DL techniques such as CNN, Bi-LSTM, and MLP to detect fake news. They claimed that the rate of misinformation is increasing rapidly.
LSTM Density Mixture Model Although traditional methods have used lexical features to detect fake news automatically, the hybrid deep neural network has received a lot of attention globally. For example, Ruchansky et al. ( 2017 ) stated that fake news detection has gained a great deal of attention both from the research and academic communities. In their work, they identified three types of fake news: (1) the text of an article, (2) the user response it receives, and (3) the source on which users promote it. They analyzed that fake news has the importance to affect public opinion. Existing studies have mostly focused on tailoring solutions to one particular problem with their limited success. However, Ruchansky et al. ( 2017 ) proposed a hybrid model combining all their characteristics to predict the more accurate and automated result. Similarly, Long et al. ( 2017 ) proposed a novel method to incorporate speaker profiles into an attention-based LSTM model for fake news detection. Additionally, several studies described that LSTM-based hybrid model is proven to work better for long sentences and attention models are also proposed to weigh the importance of different words in their context (Tang et al. 2015 ; Prova et al. 2019 ).
In another study, Kudugunta and Ferrara ( 2018 ) stated that bots have been used to sway political elections by distorting online discourse, to manipulate the stock market that may have caused health epidemics. They applied LSTM-based architecture that exploits both content and metadata to detect bots. They claimed that their model can achieve an extremely high accuracy exceeding 0.96 AUC. Yenala et al. ( 2018 ) identified that the automatic detection and filtering of inappropriate messages or comments have become an important problem for improving the quality of conversations with users as well as virtual agents. They proposed a novel hybrid DL model to automatically identify the inappropriate language. Zhao et al. ( 2015 ) created a hybrid model namely C-LSTM where they combined CNN and LSTM for the sentiment analysis of movie reviews and question-type classification.
4 Discussion with open issues and future research
Over the few years, several researchers have applied the recent developments and easy access of DL techniques to fake news, rumor, spam, etc. in the online social networks. It enables frameworks to process, handle, and adapt a lot of information. It is now being utilized the most in business insight frameworks and predictive analytics, as well as in increasingly advanced learning management systems (LMS). Therefore, we followed various DL methods inspired by the guidelines of Pouyanfar et al. ( 2018 ), Pouyanfar and Chen ( 2016 ), Perozzi et al. ( 2014 ), and Savage et al. ( 2014 ). The development of DL can potentially benefit to MID research. However, existing studies are not directly comparable to each other due to the lack of large-scale publicly available datasets. There are still numerous improvements that can be made to the models. Furthermore, DL is one of the most effective methods for the present development of innovation in the world. This method has given computers remarkable power, for example, the capacity to perceive discourse similar to a human, to prepare a model with no requirement for feature extraction or data labeling. It is currently being utilized to guide and improve a wide range of key procedures. As previously stated there are many reasons to apply DL to MID, we summarize some of the strengths of the deep learning-based model in the following.
First, deep learning techniques are more robust and effective than state-of-the-art baseline approaches and have shown their strength in various applications, in particular, misinformation (fake, spam, rumor, false information, disinformation, troll, etc.) and detection (Wu et al. 2019 ; Zhang et al. 2016 ).
Second, deep learning architecture can be easily adapted to a new problem, e.g., using CNNs, RNNs, or LSTM, GAN, DBN, etc., which is valuable for MID.
Third, deep learning techniques are highly flexibile especially with the advent of much popular deep learning frameworks such as Tensorflow, Keras, Caffe, PyTorch, and Theano.
Fourth, deep learning techniques can deal with complex interaction patterns and precisely reflect users’ preferences.
4.1 Existing dataset
The establishment of unique solutions for MID has often been dependent on limited and quality datasets. Therefore, to encourage future research work, we highlighted some recent and quality datasets related to the misinformation task in Table 4 . Such datasets are needed to understand the reasons for applying DL techniques to MID and collectively improve the state-of-the-art. Although several established techniques have been used for MID in different domains, they are not similar to each other. Due to various research directions, the data collections could vary significantly. Moreover, the available data resource from existing research work is also hard for the collection. For instance, some datasets mainly focus on personal issues while others consist of political, business, and socially relevant issues. Additionally, datasets may vary depending on what sorts of text contents are incorporated, what labels are given, how labels are gathered, whether fraudulent information is recorded, etc.
4.2 Open issues
In this section, we summarise some limitations which we identified and proposed some ideas to address these limitations:
Semantics Understanding Misinformation which is fabricated or manipulated to mislead users. It is very difficult for a machine to completely understand such semantics. Existing studies (Shu et al. 2019a ; Braşoveanu and Andonie 2019 ) for MID covers various kinds of language styles. However, the understanding of semantic features is necessary to distinguish between different weapons and to improve the performance of MID.
Multimodal Data for Misinformation In the existing literature, there are several studies related to MID such as rumors detection, fake news detection, and spam detection based on multi-modal features are exist. According to the previous studies (Wang et al. 2018 ; Farajtabar et al. 2017 ; Jin et al. 2017b ), we specify that misinformation on social media takes the form of text, images, or videos and the information in different modalities can provide clues for MID. However, how to extract these prominent features from each modality is challenging. Also, comprehensive and large-scale datasets are needed for MID.
Content Validation Due to misinformation, users are often confronted with misleading, confusing, controversial issues that need to be addressed very well. However, it is also true that too beautifully identifiable misinformation is difficult. Therefore, to easily identify incorrect information on online social media, we need a very good quality fact-checker and a special tool for crowdsourcing content validation can be developed
Spreader Identification Identifying the influential spreaders in social networks is a very important topic, which is conducive to deeply understand the role of nodes in information diffusion and epidemic spreading among a population. However, existing techniques are not able to quantify the nodal spreading capability correctly nor can they differentiate the influence of various nodes.
Misinformation Identification At present, many types of misidentification methods have been introduced in the existing research. However, most of the research works (a) tend to focus on alerting users but give no explanation as to why this is misinformation; (b) focus more on directly engaged users for the detection of misinformation. But if the users are not directly related, some users play an effective role in spreading misinformation on online social media. As they are not directly related, identifying them is a very difficult job.
Anomalous and Normal User Identification As the number of people who depend on online social media are growing, dishonest users try to exploit this opportunity. In most cases, dishonest people do this for their benefit (Zhao et al. 2014 ; Feng and Hirst 2013 ). Although researchers have used many methods to identify dishonest users, many more approaches can be investigated, for example, perhaps a new technique or modified version of an existing technique could be developed.
Bridging Echo Chamber Social media echo chambers play an important rule in spreading the presence of misinformation. One of the strategies for MID is to bridge conflicting echo chambers so that opposing opponents can be exchanged and considered. Therefore, data-driven models are an effective means which are needed to bridge these echo chambers. Also, researchers need to research to reduce the polarization effectiveness.
Mining Disinformation The widespread of disinformation can cause detrimental societal effects. Therefore, mining disinformation is desired to prevent a large number of people to be affected. From the discussion of existing studies, we find a recent improvement for disinformation in SN. However, due to its diversity, complexity, multi-modality, and costs of fact-checking, it is still non-trivial. Additionally, it is often unrealistic to obtain abundant labeled data. Existing studies argued that due to overfitting on small labeled datasets, the performance is largely limited (Wei and Wan 2017 ; Kim et al. 2018 ). In addition, models learned on one domain may be biased and might not perform well on a different target domain. Therefore, advanced DL strategies such as reinforcement learning can be utilized to tackle this problem, explore more information and better detect disinformation.
Misinformation Dynamic The spread of misinformation on social networking sites mainly depends on the content of the information, the impact of the users’ behavior, and the network structure. Most studies on misinformation analyzed the various effects of static data but have not analyzed the effects of topology on real-time data (Wei and Wan 2017 ; Kim et al. 2018 ). Therefore, we need to consider dynamic model to capture the uncertainty of user behavior to reduce the spread of fake news and misinformation.
4.3 Future direction
As with anything, there are both good and bad aspects of technology dependence. Spreading misinformation in SN is one such example. There have been a lot of research works on MID, and good results have been achieved by various effective techniques. However, we have to keep in mind that the current age is knowledge-based and technology-dependent. Therefore, researchers have to think deeply about how their research can transform people’s wellbeing in a technology-dependent era. Thus, in this paper, we have discussed some of the effective roles that elimination of misinformation can have on online SN with DL techniques. Moreover, we focused on the impact, characteristics, and detection of misinformation using DL techniques. In summary, the following are several findings of this article and possible future works:
One of the important tasks of DL is that it can work with large-sized data which the other techniques cannot. However, DL also has difficulty to find and process massive datasets, and generally to train the model, DL networks require a lot of time. In today’s competitive age, it is worthwhile to research how to train large data in a short time with DL. We believe DL should be investigated in the future.
Most current studies show that researchers can analyze static data on a given topic and predict the positive or negative aspects of that topic. However, it is high time to analyze dynamic data.
The practice of detecting false facts from SN data is very popular and is benefiting people greatly. However, this involves descriptive research which is explainable MID, not just predictive research. With MID, if a new part can be added such as the description of why it is false, then maybe that research will be even more effective and acceptable to people.
Deep reinforcement learning is a new area of machine learning that enables an agent to learn in a good interactive environment by experimenting with feedback from it own experience. So, if we combine reinforcement learning with DL to detect false facts, then better results can be obtained.
Deep learning faces the over-fitting problem which impacts the execution of the model in real-life situations.
5 Conclusion
In this survey, we reviewed various research works on MID in social networks. In particular, we took a comprehensive view of five related terms of misinformation: false information, rumor, spam, fake news, and disinformation and discussed how misinformation misleads people on social networks. We also discussed the importance of earlier works as this may be helpful to other researchers who wish to investigate this area. Comparing with the most existing detection approaches, we considered DL is an efficient and effective technique to measure the misinformation problem on online social networks. We emphasized that DL is now the leading technique to solve MID problems because it helps in identifying false facts perfectly. The result and performance are excellent and it is similar to human performance. In all respects, DL is one of the best techniques to analyze social network data. We also demonstrated that DL can be utilized to improve MID given unlabeled and imbalanced data. However, there are several challenges (data volume, data quality, domain complexity, interpretability, explainability, feature enrichment, federated inference, model privacy, incorporating expert knowledge, temporal modeling, etc.) which need to be improved in further research work. Therefore, deep learning-based MID is still an active research topic and needs to be extended in future research. This paper can benefit others who will choose to investigate DL models.
Existing research works used DL and have done extensive work for MID. Most of the existing research works have discussed the connection of one user to another in SN, their historical activities, etc. in spreading false facts. However, there are very few studies that have incorporated user mental health conditions with the user’s historical activity. Although in the above section, we introduced some future directions, one of the main future directions of our research is to expand modeling. Therefore, First, we can analyze user connections and their historical activity on SN where the user can reflect how they relate to the spread of fake news. Second, we can incorporate the human mental condition with the user’s historical data, which can better analyze the user’s activity, since the tendency to spread false information is related to the user’s human mental condition.
https://www.facebook.com/
https://twitter.com/
https://weibo.com/
https://www.thedailystar.net/country/rumour-salt-price-raising-spreads-among-consumers-1829260
https://www.dhakatribune.com/business/2019/11/19/rumour-drives-groceries-out-of-salt-stock-across-country
https://ec.europa.eu/info/live-work-travel-eu/health/coronavirus-response/fighting-disinformation/tackling-coronavirus-disinformation-en
https://www.lowyinstitute.org/the-interpreter/disinformation-and-coronavirus
Abadi M, Agarwal A, Barham P, Brevdo E, Chen Z, Citro C, Corrado GS, Davis A, Dean J, Devin M, et al. (2015) Tensorflow: large-scale machine learning on heterogeneous systems, 2015. Software available from tensorflow. org 1
Abdel-Hamid O, Mohamed, A.r., Jiang, H., Deng, L., Penn, G., Yu, D., (2014) Convolutional neural networks for speech recognition. IEEE/ACM Trans Audio Speech Lang Process 22:1533–1545
Acquisti A, Gross R (2009) Predicting social security numbers from public data. Proc Nat Acad Sci 106:10975–10980
Google Scholar
Aiello LM, Petkos G, Martin C, Corney D, Papadopoulos S, Skraba R, Göker A, Kompatsiaris I, Jaimes A (2013) Sensing trending topics in twitter. IEEE Trans Multimedia 15:1268–1282
Aizenberg IN (1999) Neural networks based on multi-valued and universal binary neurons: theory, application to image processing and recognition. In: International conference on computational intelligence, Springer, pp 306–316
Alkhodair SA, Ding SH, Fung BC, Liu J (2020) Detecting breaking news rumors of emerging topics in social media. Inf Process Manag 57:102018
Alom MZ, Bontupalli V, Taha TM (2015) Intrusion detection using deep belief networks. In: 2015 national aerospace and electronics conference (NAECON), IEEE, pp 339–344
Bathla G, Aggarwal H, Rani R (2018) Improving recommendation techniques by deep learning and large scale graph partitioning. Int J Adv Comput Sci Appl 9:403–409
Bharti SK, Pradhan R, Babu KS, Jena SK (2017) Sarcasm analysis on twitter data using machine learning approaches. In: Trends in social network analysis. Springer, pp 51–76
Bindu P, Thilagam PS, Ahuja D (2017) Discovering suspicious behavior in multilayer social networks. Comput Hum Behav 73:568–582
Brandt AM (2012) Inventing conflicts of interest: a history of tobacco industry tactics. Am J Public Health 102:63–71
Braşoveanu AM, Andonie R (2019) Semantic fake news detection: a machine learning perspective. In: International work-conference on artificial neural networks, Springer, pp 656–667
Chalapathy R, Chawla S (2019) Deep learning for anomaly detection: a survey. arXiv preprint arXiv:1901.03407
Chen T, Li X, Yin H, Zhang J (2018) Call attention to rumors: Deep attention based recurrent neural networks for early rumor detection. In: Pacific-Asia conference on knowledge discovery and data mining, Springer, pp 40–52
Chen YC, Liu ZY, Kao HY (2017) Ikm at semeval-2017 task 8: vonvolutional neural networks for stance detection and rumor verification. In: Proceedings of the 11th international workshop on semantic evaluation (SemEval-2017), pp 465–469
Cho K, van Merriënboer B, Gulcehre C, Bahdanau D, Bougares F, Schwenk H, Bengio Y, (2014a) Learning phrase representations using RNN encoder–decoder for statistical machine translation. In: Proceedings of the 2014 conference on empirical methods in natural language processing (EMNLP), Association for Computational Linguistics, Doha, Qatar. pp 1724–1734. https://www.aclweb.org/anthology/D14-1179 , 10.3115/v1/D14-1179
Cho K, Van Merriënboer B, Gulcehre C, Bahdanau D, Bougares F, Schwenk H, Bengio Y (2014b) Learning phrase representations using rnn encoder-decoder for statistical machine translation. arXiv preprint arXiv:1406.1078
Chollet F (2018) Deep learning mit Python und Keras: Das Praxis-Handbuch vom Entwickler der Keras-Bibliothek. MITP-Verlags GmbH & Co, KG
Çıtlak O, Dörterler M, Doğru İA (2019) A survey on detecting spam accounts on twitter network. Soc Netw Anal Min 9:35
Collobert R, Bengio S, Mariéthoz J (2002) Torch: a modular machine learning software library. Technical Report, Idiap
Collobert R, Kavukcuoglu K, Farabet C (2011) Torch7: a matlab-like environment for machine learning. In: BigLearn, NIPS workshop
Concone F, Re GL, Morana M, Ruocco C (2019) Twitter spam account detection by effective labeling. In: ITASEC
Cresci S, Di Pietro R, Petrocchi M, Spognardi A, Tesconi M (2015) Fame for sale: efficient detection of fake twitter followers. Decis Support Syst 80:56–71
Cui L, Wang S, Lee D (2019) Same: sentiment-aware multi-modal embedding for detecting fake news. In: Proceedings of the 2019 IEEE/ACM international conference on advances in social networks analysis and mining, pp 41–48
Da Silva LA, Da Costa KA, Papa JP, Rosa G, De Albuquerque VHC (2018) Fine-tuning restricted boltzmann machines using quaternions and its application for spam detection. IET Netw 8:101–105
Dai JJ, Wang Y, Qiu X, Ding D, Zhang Y, Wang Y, Jia X, Zhang LC, Wan Y, Li Z, Wang J, Huang S, Wu Z, Wang Y, Yang Y, She B, Shi D, Lu Q, Huang K, Song G (2019) Bigdl: A distributed deep learning framework for big data. In: Proceedings of the ACM symposium on cloud computing, Association for Computing Machinery. pp 50–60. https://arxiv.org/pdf/1804.05839.pdf , 10.1145/3357223.3362707
Dandekar A, Zen RA, Bressan S (2017) Generating fake but realistic headlines using deep neural networks. In: International conference on database and expert systems applications, Springer, pp 427–440
David OE, Netanyahu NS (2015) Deepsign: Deep learning for automatic malware signature generation and classification. In: 2015 international joint conference on neural networks (IJCNN), IEEE, pp 1–8
De Choudhury M, Counts S, Horvitz E (2013a) Predicting postpartum changes in emotion and behavior via social media. In: Proceedings of the SIGCHI conference on human factors in computing systems, ACM, pp 3267–3276
De Choudhury M, Gamon M, Hoff A, Roseway A (2013b) âĂIJmoon phrasesâĂİ: a social media faciliated tool for emotional reflection and wellness. In: 2013 7th international conference on pervasive computing technologies for healthcare and workshops, IEEE, pp 41–44
Dechter R (1986) Learning while searching in constraint-satisfaction problems. University of California, Computer Science Department, Cognitive Systems âĂȩ
Dhamani N, Azunre P, Gleason JL, Corcoran C, Honke G, Kramer S, Morgan J (2019) Using deep networks and transfer learning to address disinformation. arXiv preprint arXiv:1905.10412
Donfro J (2013) A whopping 20% of yelp reviews are fake
Du M, Li F, Zheng G, Srikumar V (2017) Deeplog: Anomaly detection and diagnosis from system logs through deep learning. In: Proceedings of the 2017 ACM SIGSAC conference on computer and communications security, ACM, pp 1285–1298
Fallis D (2014) A functional analysis of disinformation. In: Conference 2014 proceedings
Farajtabar M, Yang J, Ye X, Xu H, Trivedi R, Khalil E, Li S, Song L, Zha H (2017) Fake news mitigation via point process based intervention. In: Proceedings of the 34th international conference on machine learning, vol 70, pp 1097–1106
Feng VW, Hirst G (2013) Detecting deceptive opinions with profile compatibility. In: Proceedings of the sixth international joint conference on natural language processing, pp 338–346
Fernandez M, Alani H (2018) Online misinformation: challenges and future directions. Companion Proc Web Conf 2018:595–602
Friggeri A, Adamic L, Eckles D, Cheng J (2014) Rumor cascades. In: Eighth international AAAI conference on weblogs and social media
Galitsky B (2015) Detecting rumor and disinformation by web mining. In: 2015 AAAI Spring symposium series
Gao H, Liu H (2014) Data analysis on location-based social networks. Mobile Soc Netw 11:165–194
Gong M, Gao Y, Xie Y, Qin A (2020) An attention-based unsupervised adversarial model for movie review spam detection. In: IEEE transactions on multimedia
Goodfellow I, Pouget-Abadie J, Mirza M, Xu B, Warde-Farley D, Ozair S, Courville A, Bengio Y (2014) Generative adversarial nets. In: Advances in neural information processing systems, pp 2672–2680
Goswami A, Kumar A (2016) A survey of event detection techniques in online social networks. Soc Netw Anal Min 6:107
Guo B, Ding Y, Yao L, Liang Y, Yu Z (2019) The future of misinformation detection: new perspectives and trends. arXiv preprint arXiv:1909.03654
Guo H, Cao J, Zhang Y, Guo J, Li J (2018) Rumor detection with hierarchical social attention network. In: Proceedings of the 27th ACM international conference on information and knowledge management, ACM, pp 943–951
Gupta A, Kumaraguru P, Castillo C, Meier P (2014) Tweetcred: Real-time credibility assessment of content on twitter. In: International Conference on Social Informatics, Springer, pp 228–243
Gupta A, Lamba H, Kumaraguru P, Joshi A (2013) Faking sandy: characterizing and identifying fake images on twitter during hurricane sandy. In: Proceedings of the 22nd international conference on World Wide Web, ACM, pp 729–736
Habib A, Asghar MZ, Khan A, Habib A, Khan A (2019) False information detection in online content and its role in decision making: a systematic literature review. Soc Netw Anal Min 9:50
Hardy W, Chen L, Hou S, Ye Y, Li X (2016) Dl4md: a deep learning framework for intelligent malware detection. In: Proceedings of the international conference on data mining (DMIN), the steering committee of the world congress in computer science, computer âĂȩ, p 61
Helmstetter S, Paulheim H (2018) Weakly supervised learning for fake news detection on twitter. In: 2018 IEEE/ACM international conference on advances in social networks analysis and mining (ASONAM), IEEE, pp 274–277
Hernon P (1995) Disinformation and misinformation through the internet: findings of an exploratory study. Gov Inf Q 12:133–139
Hinton G, Deng L, Yu D, Dahl G, Mohamed Ar, Jaitly N, Senior A, Vanhoucke V, Nguyen P, Kingsbury B, et al. (2012) Deep neural networks for acoustic modeling in speech recognition. In: IEEE Signal processing magazine, p 29
Horne BD, Adali S (2017) This just in: fake news packs a lot in title, uses simpler, repetitive content in text body, more similar to satire than real news. In: Eleventh international AAAI conference on web and social media
Hu X, Tang J, Zhang Y, Liu H (2013) Social spammer detection in microblogging. In: Twenty-third international joint conference on artificial intelligence
Islam MR, Kabir MA, Ahmed A, Kamal ARM, Wang H, Ulhaq A (2018a) Depression detection from social network data using machine learning techniques. Health Inf Sci Syst 6:8
Islam MR, Kamal ARM, Sultana N, Islam R, Moni MA, et al. (2018b) Detecting depression using k-nearest neighbors (knn) classification technique. In: 2018 international conference on computer, communication, chemical, material and electronic engineering (IC4ME2), IEEE, pp 1–4
Islam MR, Miah SJ, Kamal ARM, Burmeister O (2019) A design construct of developing approaches to measure mental health conditions. In: Australasian journal of information systems, p 23
Jacovi A, Shalom OS, Goldberg Y (2018) Understanding convolutional neural networks for text classification. arXiv preprint arXiv:1809.08037
Jain S, Sharma V, Kaushal R (2016) Towards automated real-time detection of misinformation on twitter. In: 2016 international conference on advances in computing. Communications and informatics (ICACCI), IEEE, pp 2015–2020
Jia Y, Shelhamer E, Donahue J, Karayev S, Long J, Girshick R, Guadarrama S, Darrell T (2014) Caffe: convolutional architecture for fast feature embedding. In: Proceedings of the 22nd ACM international conference on Multimedia, ACM, pp 675–678
Jia Y, Song X, Zhou J, Liu L, Nie L, Rosenblum DS (2016) Fusing social networks with deep learning for volunteerism tendency prediction. In: Thirtieth AAAI conference on artificial intelligence
Jin D, Ge M, Li Z, Lu W, He D, Fogelman-Soulie F (2017a) Using deep learning for community discovery in social networks. In: 2017 IEEE 29th international conference on tools with artificial intelligence (ICTAI), IEEE, pp 160–167
Jin Z, Cao J, Guo H, Zhang Y, Luo J (2017b) Multimodal fusion with recurrent neural networks for rumor detection on microblogs. In: Proceedings of the 25th ACM international conference on multimedia, pp 795–816
Jin Z, Cao J, Guo H, Zhang Y, Wang Y, Luo J (2017c) Detection and analysis of 2016 us presidential election related rumors on twitter. In: International conference on social computing, behavioral-cultural modeling and prediction and behavior representation in modeling and simulation, Springer, pp 14–24
Jin Z, Cao J, Zhang Y, Zhou J, Tian Q (2016) Novel visual and statistical image features for microblogs news verification. IEEE Trans Multimedia 19:598–608
Jindal S, Sood R, Singh R, Vatsa M, Chakraborty T (xxxx) Newsbag: a multimodal benchmark dataset for fake news detection
Ketkar N (2017) Introduction to pytorch. In: Deep learning with python. Springer, pp 195–208
Kim J, Tabibian B, Oh A, Schölkopf B, Gomez-Rodriguez M (2018) Leveraging the crowd to detect and reduce the spread of fake news and misinformation. In: Proceedings of the eleventh ACM international conference on web search and data mining, pp 324–332
Kim Y (2014) Convolutional neural networks for sentence classification. arXiv preprint arXiv:1408.5882
King DE (2009) Dlib-ml: a machine learning toolkit. J Mach Learn Res 10:1755–1758
Kochkina E, Liakata M, Zubiaga A (2018) All-in-one: Multi-task learning for rumour verification. arXiv preprint arXiv:1806.03713
Kudugunta S, Ferrara E (2018) Deep neural networks for bot detection. Inf Sci 467:312–322
Kumar S, Asthana R, Upadhyay S, Upreti N, Akbar M (2019) Fake news detection using deep learning models: a novel approach. Trans Emerg Telecommun Technol 5:e3767
Kumar S, Shah N (2018) False information on web and social media: a survey. arXiv preprint arXiv:1804.08559
Kumar S, West R, Leskovec J (2016) Disinformation on the web: Impact, characteristics, and detection of wikipedia hoaxes. In: Proceedings of the 25th international conference on World Wide Web, pp 591–602
Kwon S, Cha M, Jung K (2017) Rumor detection over varying time windows. PloS ONE 12:69
Lake JM (2014) Fake web addresses and hyperlinks. US Patent 8,799,465
LeCun Y, Bengio Y, Hinton G (2015) Deep learning. Nature 521:436
LeCun Y, Kavukcuoglu K, Farabet C (2010) Convolutional networks and applications in vision. In: Proceedings of 2010 IEEE international symposium on circuits and systems, IEEE, pp 253–256
Li C, Liu S (2018) A comparative study of the class imbalance problem in twitter spam detection. Concurr Comput Pract Exp 30:e4281
Li L, Cai G, Chen N (2018a) A rumor events detection method based on deep bidirectional gru neural network. In: 2018 IEEE 3rd international conference on image. Vision and computing (ICIVC), IEEE, pp 755–759
Li X, Wu X (2015) Constructing long short-term memory based deep recurrent neural networks for large vocabulary speech recognition. In: 2015 IEEE international conference on acoustics. Speech and signal processing (ICASSP), IEEE, pp 4520–4524
Li Y, Nie X, Huang R (2018b) Web spam classification method based on deep belief networks. Expert Syst Appl 96:261–270
Liao L, Jin W, Pavel R (2016) Enhanced restricted boltzmann machine with prognosability regularization for prognostics and health assessment. IEEE Trans Industr Electron 63:7076–7083
Lin X, Liao X, Xu T, Pian W, Wong KF (2019) Rumor detection with hierarchical recurrent convolutional neural network. In: CCF international conference on natural language processing and chinese computing, Springer, pp 338–348
Liu Q, Yu F, Wu S, Wang L (2018) Mining significant microblogs for misinformation identification: an attention-based approach. ACM Trans Intell Syst Technol 9:1–20
Liu Y, Wu YFB (2018) Early detection of fake news on social media through propagation path classification with recurrent and convolutional networks. In: Thirty-second AAAI conference on artificial intelligence
Liu Y, Wu YFB (2020) Fned: a deep network for fake news early detection on social media. ACM Trans Inf Syst 38:1–33
Liu Y, Xu S (2016) Detecting rumors through modeling information propagation networks in a social media environment. IEEE Trans Comput Soc Syst 3:46–62
Long Y, Lu Q, Xiang R, Li M, Huang CR (2017) Fake news detection through multi-perspective speaker profiles. In: Proceedings of the eighth international joint conference on natural language processing, vol 2, pp 252–256
Ma J, Gao W, Mitra P, Kwon S, Jansen BJ, Wong KF, Cha M (2016) Detecting rumors from microblogs with recurrent neural networks. In: Ijcai, pp 3818–3824
Ma J, Gao W, Wei Z, Lu Y, Wong KF (2015) Detect rumors using time series of social context information on microblogging websites. In: Proceedings of the 24th ACM international on conference on information and knowledge management, pp 1751–1754
Ma J, Gao W, Wong KF (2018) Rumor detection on twitter with tree-structured recursive neural networks. In: Proceedings of the 56th annual meeting of the association for computational linguistics, vol 1, pp 1980–1989
Ma J, Gao W, Wong KF (2019) Detect rumors on twitter by promoting information campaigns with generative adversarial learning. In: The World Wide Web Conference, ACM, pp 3049–3055
Markines B, Cattuto C, Menczer F (2009) Social spam detection. In: Proceedings of the 5th international workshop on adversarial information retrieval on the web, pp 41–48
Mitra T, Gilbert E (2015) Credbank: A large-scale social media corpus with associated credibility annotations. In: ICWSM, pp 258–267
Mukherjee A, Venkataraman V, Liu B, Glance NS (2013) What yelp fake review filter might be doing? In: Icwsm, pp 409–418
Nan CJ, Kim KM, Zhang BT (2015) Social network analysis of tv drama characters via deep concept hierarchies. In: 2015 IEEE/ACM international conference on advances in social networks analysis and mining (ASONAM), IEEE, pp 831–836
Naseem U, Razzak I, Musial K, Imran M (2020) Transformer based deep intelligent contextual embedding for twitter sentiment analysis. Future Gener Comput Syst 6:91
Nguyen DT, Al Mannai KA, Joty S, Sajjad H, Imran M, Mitra P (2017a) Robust classification of crisis-related data on social networks using convolutional neural networks. In: Eleventh international AAAI conference on web and social media
Nguyen TN, Li C, Niederée C (2017b) On early-stage debunking rumors on twitter: Leveraging the wisdom of weak learners. In: International conference on social informatics, Springer, pp 141–158
Norouzi M (2009) Convolutional restricted Boltzmann machines for feature learning. Ph.D. thesis. School of Computing Science-Simon Fraser University
Norouzi M, Ranjbar M, Mori G (2009) Stacks of convolutional restricted boltzmann machines for shift-invariant feature learning. In: 2009 IEEE conference on computer vision and pattern recognition, IEEE, pp 2735–2742
Papa JP, Rosa GH, Marana AN, Scheirer W, Cox DD (2015) Model selection for discriminative restricted boltzmann machines through meta-heuristic techniques. J Comput Sci 9:14–18
Parvat A, Chavan J, Kadam S, Dev S, Pathak V (2017) A survey of deep-learning frameworks. In: 2017 international conference on inventive systems and control (ICISC), IEEE, pp 1–7
Perozzi B, Al-Rfou R, Skiena S (2014) Deepwalk: Online learning of social representations. In: Proceedings of the 20th ACM SIGKDD international conference on Knowledge discovery and data mining, ACM, pp 701–710
Pierri F, Piccardi C, Ceri S (2020) A multi-layer approach to disinformation detection on twitter. arXiv preprint arXiv:2002.12612
Popat K, Mukherjee S, Yates A, Weikum G (2018) Declare: debunking fake news and false claims using evidence-aware deep learning. arXiv preprint arXiv:1809.06416
Pouyanfar S, Chen SC (2016) Semantic event detection using ensemble deep learning. In: 2016 IEEE international symposium on multimedia (ISM), IEEE, pp 203–208
Pouyanfar S, Sadiq S, Yan Y, Tian H, Tao Y, Reyes MP, Shyu ML, Chen SC, Iyengar S (2018) A survey on deep learning: algorithms, techniques, and applications. ACM Comput Surv 51:92
Prova AA, Akter T, Islam MR, Uddin MR, Hossain T, Hannan M, Hossain MS (2019) Analysis of online marketplace data on social networks using lstm. In: 2019 5th international conference on advances in electrical engineering (ICAEE), IEEE, pp 381–385
Qazvinian V, Rosengren E, Radev DR, Mei Q (2011) Rumor has it: Identifying misinformation in microblogs. In: Proceedings of the conference on empirical methods in natural language processing, Association for. Computational Linguistics, pp 1589–1599
Qian F, Gong C, Sharma K, Liu Y (2018) Neural user response generator: Fake news detection with collective user intelligence. In: IJCAI, pp 3834–3840
Quah JT, Sriganesh M (2008) Real-time credit card fraud detection using computational intelligence. Expert Syst Appl 35:1721–1732
Rayana S, Akoglu L (2015). Collective opinion spam detection: Bridging review networks and metadata. In: Proceedings of the 21th acm sigkdd international conference on knowledge discovery and data mining, pp 985–994
Roy A, Basak K, Ekbal A, Bhattacharyya P (2018) A deep ensemble framework for fake news detection and classification. arXiv preprint arXiv:1811.04670
Ruchansky N, Seo S, Liu Y (2017) Csi: A hybrid deep model for fake news detection. In: Proceedings of the 2017 ACM on conference on information and knowledge management, ACM, pp 797–806
Saberi A, Vahidi M, Bidgoli BM (2007) Learn to detect phishing scams using learning and ensemble? methods. In: 2007 IEEE/WIC/ACM international conferences on web intelligence and intelligent agent technology-workshops, IEEE, pp 311–314
Sampson J, Morstatter F, Wu L, Liu H (2016) Leveraging the implicit structure within social media for emergent rumor detection. In: Proceedings of the 25th ACM international on conference on information and knowledge management, pp 2377–2382
Savage D, Zhang X, Yu X, Chou P, Wang Q (2014) Anomaly detection in online social networks. Soc Netw 39:62–70
Selvaganapathy S, Nivaashini M, Natarajan H (2018) Deep belief network based detection and categorization of malicious urls. Inf Secur J Global Perspect 27:145–161
Shahariar G, Biswas S, Omar F, Shah FM, Hassan SB (2019) Spam review detection using deep learning. In: 2019 IEEE 10th annual information technology, electronics and mobile communication conference (IEMCON), IEEE, pp 0027–0033
Sharma K, Qian F, Jiang H, Ruchansky N, Zhang M, Liu Y (2019) Combating fake news: a survey on identification and mitigation techniques. arXiv preprint arXiv:1901.06437
Shi S, Wang Q, Chu X (2018) Performance modeling and evaluation of distributed deep learning frameworks on gpus. In: 2018 IEEE 16th international conference on dependable, autonomic and secure computing, 16th international conference on pervasive intelligence and computing, 4th international conference on big data intelligence and computing and cyber science and technology congress (DASC/PiCom/DataCom/CyberSciTech), IEEE, pp 949–957
Shu K, Cui L, Wang S, Lee D, Liu H (2019a) defend: Explainable fake news detection. In: Proceedings of the 25th ACM SIGKDD international conference on knowledge discovery and data mining, pp 395–405
Shu K, Mahudeswaran D, Wang S, Lee D, Liu H (2018) Fakenewsnet: a data repository with news content, social context and dynamic information for studying fake news on social media. arXiv preprint arXiv:1809.01286
Shu K, Sliva A, Wang S, Tang J, Liu H (2017) Fake news detection on social media: a data mining perspective. ACM SIGKDD Explor Newslett 19:22–36
Shu K, Wang S, Lee D, Liu H (2020) Mining disinformation and fake news: concepts, methods, and recent advancements. arXiv preprint arXiv:2001.00623
Shu K, Wang S, Liu H (2019b) Beyond news contents: The role of social context for fake news detection. In: Proceedings of the twelfth ACM international conference on web search and data mining, pp 312–320
Shu K, Zhou X, Wang S, Zafarani R, Liu H (2019c) The role of user profiles for fake news detection. In: Proceedings of the 2019 IEEE/ACM international conference on advances in social networks analysis and mining, pp 436–439
Silva L, Ribeiro P, Rosa G, Costa K, Papa JP (2015) Parameter setting-free harmony search optimization of restricted boltzmann machines and its applications to spam detection. In: 12th international conference applied computing, pp 142–150
da Silva LA, da Costa KAP, Ribeiro PB, de Rosa GH, Papa JP (2016) Learning spam features using restricted boltzmann machines. IADIS International Journal on Computer Science & Information Systems 11
Silverman C, Strapagiel L, Shaban H, Hall E, Singer-Vine J (2016) Hyperpartisan facebook pages are publishing false and misleading information at an alarming rate. Buzzfeed News 20:68
Socher R, Perelygin A, Wu J, Chuang J, Manning CD, Ng A, Potts C (2013) Recursive deep models for semantic compositionality over a sentiment treebank. In: Proceedings of the 2013 conference on empirical methods in natural language processing, pp 1631–1642
Srivastava N, Salakhutdinov RR (2012) Multimodal learning with deep boltzmann machines. In: Advances in neural information processing systems, pp 2222–2230
Sun X, Zhang C, Ding S, Quan C (2018) Detecting anomalous emotion through big data from social networks based on a deep learning method. Multimedia Tools Appl 5:1–22
Tacchini E, Ballarin G, Della Vedova ML, Moret S, de Alfaro L (2017) Some like it hoax: automated fake news detection in social networks. arXiv preprint arXiv:1704.07506
Tang D, Qin B, Liu T (2015) Document modeling with gated recurrent neural network for sentiment classification. In: Proceedings of the 2015 conference on empirical methods in natural language processing, pp 1422–1432
Tschiatschek S, Singla A, Gomez Rodriguez M, Merchant A, Krause A (2018) Fake news detection in social networks via crowd signals. In: Companion of the the web conference 2018 on the web conference 2018, international world wide web conferences steering committee, pp 517–524
Tsui D (2017) Predicting stock price movement using social media analysis. Technical Report. Stanford University, Technical Report
Tzortzis G, Likas A (2007) Deep belief networks for spam filtering. In: 19th IEEE international conference on tools with artificial intelligence (ICTAI 2007), IEEE, pp 306–309
Van Merriënboer B, Bahdanau D, Dumoulin V, Serdyuk D, Warde-Farley D, Chorowski J, Bengio Y (2015) Blocks and fuel: frameworks for deep learning. arXiv preprint arXiv:1506.00619
Vartapetiance A, Gillam L (2014) Deception detection: dependable or defective? Soc Netw Anal Min 4:166
Vlachos A, Riedel S (2014) Fact checking: Task definition and dataset construction. In: Proceedings of the ACL 2014 workshop on language technologies and computational social science, pp 18–22
Vo NN, He X, Liu S, Xu G (2019) Deep learning for decision making and the optimization of socially responsible investments and portfolio. Decis Support Syst 124:113097
Vo NN, Liu S, He X, Xu G (2018) Multimodal mixture density boosting network for personality mining. In: Pacific-Asia conference on knowledge discovery and data mining, Springer. pp 644–655
Wang D, Irani D, Pu C (2011) A social-spam detection framework. In: Proceedings of the 8th annual collaboration, electronic messaging, anti-abuse and Spam conference, pp 46–54
Wang N, Yeung DY (2013) Learning a deep compact image representation for visual tracking. In: Advances in neural information processing systems, pp 809–817
Wang W, Zhang F, Luo X, Zhang S (2019) Pdrcnn: precise phishing detection with recurrent convolutional neural networks. Secur Commun Netw 9:72
Wang WY (2017) “ liar, liar pants on fire”: a new benchmark dataset for fake news detection. arXiv preprint arXiv:1705.00648
Wang Y, Ma F, Jin Z, Yuan Y, Xun G, Jha K, Su L, Gao J (2018) Eann: Event adversarial neural networks for multi-modal fake news detection. In: Proceedings of the 24th ACM sigkdd international conference on knowledge discovery and data mining, pp 849–857
Wei L, Gao D, Luo C (2018) False data injection attacks detection with deep belief networks in smart grid. In: 2018 Chinese automation congress (CAC), IEEE, pp 2621–2625
Wei W, Wan X (2017) Learning to identify ambiguous and misleading news headlines. arXiv preprint arXiv:1705.06031
Willmore A (xxxx) This analysis shows how viral fake election news stories outperformed real news on facebook
Wu L, Li J, Hu X, Liu H (2017) Gleaning wisdom from the past: Early detection of emerging rumors in social media. In: Proceedings of the 2017 SIAM international conference on data mining, SIAM, pp 99–107
Wu L, Morstatter F, Carley KM, Liu H (2019) Misinformation in social media: definition, manipulation, and detection. ACM SIGKDD Explor Newslett 21:80–90
Wu L, Rao Y, Yu H, Wang Y, Nazir A (2018) False information detection on social media via a hybrid deep model. In: International conference on social informatics, Springer, pp 323–333
Xu Y, Wang C, Dan Z, Sun S, Dong F (2019) Deep recurrent neural network and data filtering for rumor detection on sina weibo. Symmetry 11:1408
Yang Y, Zheng L, Zhang J, Cui Q, Li Z, Yu PS (2018) Ti-cnn: Convolutional neural networks for fake news detection. arXiv preprint arXiv:1806.00749
Yenala H, Jhanwar A, Chinnakotla MK, Goyal J (2018) Deep learning for detecting inappropriate content in text. Int J Data Sci Anal 6:273–286
Yepes AJ, MacKinlay A, Bedo J, Garvani R, Chen Q (2014) Deep belief networks and biomedical text categorisation. In: Proceedings of the Australasian language technology association. Workshop, pp 123–127
Yilmaz CM, Durahim AO (2018) Spr2ep: a semi-supervised spam review detection framework. In: 2018 IEEE/ACM international conference on advances in social networks analysis and mining (ASONAM), IEEE, pp 306–313
Yin J, Zhou Z, Liu S, Wu Z, Xu G (2018) Social spammer detection: a multi-relational embedding approach. In: Pacific-Asia conference on knowledge discovery and data mining, Springer, pp 615–627
Yin J, Li Q, Liu S, Wu Z, Xu G (2020) Leveraging Multi-level Dependency of Relational Sequences for Social Spammer Detection. arXiv preprint arXiv:2009.06231
Young T, Hazarika D, Poria S, Cambria E (2018) Recent trends in deep learning based natural language processing. In: IEEE computational intelligence magazine, vol 13, pp 55–75
Yu F, Liu Q, Wu S, Wang L, Tan T et al. (2017a) A convolutional approach for misinformation identification
Yu S, Li M, Liu F (2017b) Rumor identification with maximum entropy in micronet. Complexity 2017
Zhang H, Alim MA, Li X, Thai MT, Nguyen HT (2016) Misinformation in online social networks: detect them all with a limited budget. ACM Trans Inf Syst 34:1–24
Zhang H, Kuhnle A, Smith JD, Thai MT (2018a) Fight under uncertainty: Restraining misinformation and pushing out the truth. In: 2018 IEEE/ACM international conference on advances in social networks analysis and mining (ASONAM), IEEE, pp 266–273
Zhang J, Cui L, Fu Y, Gouza FB (2018b) Fake news detection with deep diffusive network model. arXiv preprint arXiv:1805.08751
Zhang Q, Lipani A, Liang S, Yilmaz E (2019) Reply-aided detection of misinformation via bayesian deep learning. In: The world wide web conference, pp 2333–2343
Zhang Q, Zhang S, Dong J, Xiong J, Cheng X (2015) Automatic detection of rumor on social network. In: Natural language processing and Chinese computing. Springer, pp 113–122
Zhang W, Du Y, Yoshida T, Wang Q (2018c) Dri-rcnn: an approach to deceptive review identification using recurrent convolutional neural network. Inf Process Manag 54:576–592
Zhang Y, Salakhutdinov R, Chang HA, Glass J (2012) Resource configurable spoken query detection using deep boltzmann machines. In: 2012 IEEE international conference on acoustics. Speech and signal processing (ICASSP), IEEE, pp 5161–5164
Zhao J, Cao N, Wen Z, Song Y, Lin YR, Collins C (2014) Fluxflow: visual analysis of anomalous information spreading on social media. IEEE Trans Visual Comput Graphics 20:1773–1782
Zhao Z, Resnick P, Mei Q (2015) Enquiring minds: Early detection of rumors in social media from enquiry posts. In: Proceedings of the 24th international conference on world wide web, pp 1395–1405
Zhou C, Sun C, Liu Z, Lau F (2015) A c-lstm neural network for text classification. arXiv preprint arXiv:1511.08630
Zubiaga A, Aker A, Bontcheva K, Liakata M, Procter R (2018) Detection and resolution of rumours in social media: a survey. ACM Comput Surv 51:1–36
Zubiaga A, Kochkina E, Liakata M, Procter R, Lukasik M (2016a) Stance classification in rumours as a sequential task exploiting the tree structure of social media conversations. arXiv preprint arXiv:1609.09028
Zubiaga A, Liakata M, Procter R (2017) Exploiting context for rumour detection in social media. In: International conference on social informatics, Springer, pp 109–123
Zubiaga A, Liakata M, Procter R, Hoi GWS, Tolmie P (2016b) Analysing how people orient to and spread rumours in social media by looking at conversational threads. PLoS ONE 11:e0150989
Download references
Author information
Authors and affiliations.
Advanced Analytics Institute (AAi), University of Technology Sydney (UTS), Sydney, Australia
Md Rafiqul Islam, Shaowu Liu & Guandong Xu
School of Computer Science, University of Technology Sydney (UTS), Sydney, Australia
Xianzhi Wang
You can also search for this author in PubMed Google Scholar
Corresponding author
Correspondence to Guandong Xu .
Additional information
Publisher's note.
Springer Nature remains neutral with regard to jurisdictional claims in published maps and institutional affiliations.
Rights and permissions
Reprints and permissions
About this article
Islam, M.R., Liu, S., Wang, X. et al. Deep learning for misinformation detection on online social networks: a survey and new perspectives. Soc. Netw. Anal. Min. 10 , 82 (2020). https://doi.org/10.1007/s13278-020-00696-x
Download citation
Received : 28 March 2020
Revised : 11 September 2020
Accepted : 12 September 2020
Published : 29 September 2020
DOI : https://doi.org/10.1007/s13278-020-00696-x
Share this article
Anyone you share the following link with will be able to read this content:
Sorry, a shareable link is not currently available for this article.
Provided by the Springer Nature SharedIt content-sharing initiative
- Deep learning
- Neural network
- Misinformation detection
- Decision making
- Online social networks
- Find a journal
- Publish with us
- Track your research
Understanding different research perspectives
Introduction
In this free course, Understanding different research perspectives , you will explore the development of the research process and focus on the steps you need to follow in order to plan and design a HR research project.
The course comprises three parts:
- The first part (Sections 1 and 2) discusses the different perspectives from which an issue or phenomenon can be investigated and outlines how each of these perspectives generates different kinds of knowledge about the issue. It is thus concerned with what it means to ‘know’ in research terms.
- The second part (Sections 3 to 7) identifies the different elements (e.g. methodologies, ethics) of a business research project. In order to produce an effective project, these different elements need to be integrated into a research strategy. The research strategy is your plan of action and will include choices regarding research perspectives and methodologies.
- The third part (Sections 8 to 10) highlights the main methodologies that can be used to investigate a business issue.
This overview of the research perspectives will enable you to take the first steps to develop a work-based project – namely, identifying a research problem and developing the research question(s) you want to investigate.
By the end of this course you should have developed a clear idea of what you want to investigate; in which context you want to do this (e.g. in your organisation, in another organisation, with workers from different organisations); and what are the specific questions you want to address.
This OpenLearn course is an adapted extract from the Open University course B865 Managing research in the workplace .
Learning outcomes
After studying this course, you should be able to:
understand the different perspectives from which a problem can be investigated
consider the researcher’s involvement in the research process as insider and/or outsider
reflect on the importance of ethical processes in research
understand the development of a research strategy and how this is translated into a research design
identify a research problem to be investigated.
1 Objective and subjective research perspectives
Research in social science requires the collection of data in order to understand a phenomenon. This can be done in a number of ways, and will depend on the state of existing knowledge of the topic area. The researcher can:
- Explore a little known issue. The researcher has an idea or has observed something and seeks to understand more about it (exploratory research).
- Connect ideas to understand the relationships between the different aspects of an issue, i.e. explain what is going on (explanatory research).
- Describe what is happening in more detail and expand the initial understanding (explicatory or descriptive research).
Exploratory research is often done through observation and other methods such as interviews or surveys that allow the researcher to gather preliminary information.
Explanatory research, on the other hand, generally tests hypotheses about cause and effect relationships. Hypotheses are statements developed by the researcher that will be tested during the research. The distinction between exploratory and explanatory research is linked to the distinction between inductive and deductive research. Explanatory research tends to be deductive and exploratory research tends to be inductive. This is not always the case but, for simplicity, we shall not explore the exceptions here.
Descriptive research may support an explanatory or exploratory study. On its own, descriptive research is not sufficient for an academic project. Academic research is aimed at progressing current knowledge.
The perspective taken by the researcher also depends on whether the researcher believes that there is an objective world out there that can be objectively known; for example, profit can be viewed as an objective measure of business performance. Alternatively the researcher may believe that concepts such as ‘culture’, ‘motivation’, ‘leadership’, ‘performance’ result from human categorisation of the world and that their ‘meaning’ can change depending on the circumstances. For example, performance can mean different things to different people. For one it may refer to a hard measure such as levels of sales. For another it may include good relationships with customers. According to this latter view, a researcher can only take a subjective perspective because the nature of these concepts is the result of human processes. Subjective research generally refers to the subjective experiences of research participants and to the fact that the researcher’s perspective is embedded within the research process, rather than seen as fully detached from it.
On the other hand, objective research claims to describe a true and correct reality, which is independent of those involved in the research process. Although this is a simplified view of the way in which research can be approached, it is an important distinction to think about. Whether you think about your research topic in objective or subjective terms will determine the development of the research questions, the type of data collected, the methods of data collection and analysis you adopt and the conclusions that you draw. This is why it is important to consider your own perspective when planning your project.
Subjective research is generally referred to as phenomenological research. This is because it is concerned with the study of experiences from the perspective of an individual, and emphasises the importance of personal perspectives and interpretations. Subjective research is generally based on data derived from observations of events as they take place or from unstructured or semi-structured interviews. In unstructured interviews the questions emerged from the discussion between the interviewer and the interviewee. In semi-structured interviews the interviewer prepares an outline of the interview topics or general questions, adding more as needs emerged during the interview. Structured interviews include the full list of questions. Interviewers do not deviate from this list. Subjective research can also be based on examinations of documents. The researcher will attribute personal interpretations of the experiences and phenomena during the process of both collecting and analysing data. This approach is also referred to as interpretivist research. Interpretivists believe that in order to understand and explain specific management and HR situations, one needs to focus on the viewpoints, experiences, feelings and interpretations of the people involved in the specific situation.
Conversely, objective research tends to be modelled on the methods of the natural sciences such as experiments or large scale surveys. Objective research seeks to establish law-like generalisations which can be applied to the same phenomenon in different contexts. This perspective, which privileges objectivity, is called positivism and is based on data that can be subject to statistical analysis and generalisation. Positivist researchers use quantitative methodologies, which are based on measurement and numbers, to collect and analyse data. Interpretivists are more concerned with language and other forms of qualitative data, which are based on words or images. Having said that, researchers using objectivist and positivist assumptions sometimes use qualitative data while interpretivists sometimes use quantitative data. (Quantitative and qualitative methodologies will be discussed in more detail in the final part of this course.) The key is to understand the perspective you intend to adopt and realise the limitations and opportunities it offers. Table 1 compares and contrasts the perspectives of positivism and interpretivism.
Some textbooks include the realist perspective or discuss constructivism, but, for the purpose of your work-based project, you do not need to engage with these other perspectives. This course keeps the discussion of research perspectives to a basic level.
Search and identify two articles that are based on your research topic. Ideally you may want to identify one article based on quantitative and one based on qualitative methodologies.
Now answer the following questions:
- In what ways are the two studies different (excluding the research focus)?
- Which research perspective do the author/s in article 1 take in their study (i.e. subjective or objective or in other words, phenomenological/interpretivist or positivist)?
- What elements (e.g. specific words, sentences, research questions) in the introduction reveal the approach taken by the authors?
- Which research perspective do the author/s in article 2 take in their study (i.e. subjective or objective, phenomenological/interpretivist or positivist)?
- What elements (e.g. specific words, sentences, research questions) in the introduction and research questions sections reveal the approach taken by the authors?
This activity has helped you to distinguish between objective and subjective research by recognising the type of language and the different ways in which objectivists/positivists and subjectivists/interpretivists may formulate their research aims. It should also support the development of your personal preference on objective or subjective research.
2 The researcher as an outsider or an insider
The researcher’s perspective is not only related to philosophical questions of subjectivity and objectivity but also to the researcher’s position with respect to the subject researched. This is particularly relevant for work-based projects where researchers are looking at their own organisation, group or community. In relation to the researcher’s position, s/he can be an insider or an outsider. Here the term ‘insider’ will include the semi-insider position, and the term ‘outsider’ will include the semi-outsider position. If you belong to the group you want to study, you become an ‘insider-researcher’. For example, if you want to conduct your research project with HR managers and you are a HR manager yourself you will have a common language and a common understanding of the issues associated with doing the same job. While, on one hand, the insider perspective allows special sensitivity, empathy and understanding of the matters, which may not be so clear to an outsider, it may also lead to greater bias or to a research direction that is more important to the researcher. On the other hand, an outsider-researcher would be more detached, less personal, but also less well-informed.
Rabe (2003) suggests that once outsider and insider perspectives in research are examined, three concepts can lead to a better understanding. First, the outsider and insider can be understood by considering the concept of power : there is power involved in the relationship between the researcher and the people and organisations participating in the research. As researchers are gathering data from the research participants, they have the power to represent those participants in any way they choose. The research participants have less power, although they can choose what to say to the researchers. This has different implications for insiders and outsiders. It is obvious that in the case of work-based projects conducted in your organisation, the ways in which you choose to represent your colleagues and your organisation places you in a position of power.
Second, insider and outsider perspectives can be understood in the context of knowledge : the insider has inside knowledge that the outsider does not have. If you conduct research in your organisation, institution or profession you will have access to inside knowledge that an outsider will not be able to gain.
The third way in which the insider/outsider concept can be understood is by considering the role of the researcher in the field of anthropology . In fact, anthropologists approach those being studied (e.g. remote cultures, tribes, social groups) as outsiders. As researchers experience the life of those studied by living with them, they acquire an insider’s perspective. The goal is to obtain both insider and outsider knowledge and to maintain the appropriate detachment. This approach applies to participatory research in general, not only anthropology.
Figure 1 reports the various stages you are expected to follow in order to complete and write up your research report.

3 Deciding what to research
Selecting an appropriate research topic is the first step towards a satisfactory project. For some people, choosing the topic is easy because they have a very specific interest in an area. For example, you might be interested in studying training and development systems in multinational companies (MNCs), or you might have a pressing work issue you want to address, like why the career progression of women in the construction industry is slower than that of men in the same industry. Alternatively, your sponsoring organisation might like you to carry out a specific project that will benefit the organisation itself, for example, it might need a specific HR policy to be developed.
While you may be among those who already have clear ideas, for other learners the process of choosing the research topic can be daunting and frustrating. So how can you generate research ideas? Where should you start? It is easiest to start from your organisational context, if you are currently in employment or if you are volunteering. Is there anything that is bothering you, your colleagues or your department? Is there a HR issue that could be addressed or further developed? Talking about your project with colleagues and family may also help as they may have suggestions that you have not considered.
The following case study describes a process that might help you to identify opportunities in your daily activities that could lead to a suitable topic.
Lucy works as a HR assistant manager for a large manufacturer of confectionery that operates at a national level. The company has three factories and a head office. While the company has a centralised HR function based at the head office, Lucy is based at one of the factories (the largest of the three) that employs 176 workers. The HR department at the factory comprises three HR experts: the manager and two assistants. Their focus is mainly concerned with the training and development of the on-site staff, with the recruitment of factory workers, grievances, disciplinary and day-to-day HR management. It excludes general issues such as salaries, benefits, pensions, recruitment and development of managerial staff and more centralised aspects that are managed by the HR function at head office level.

For some time, Lucy has received feedback through the appraisal system and exit interviews that shop floor workers are dissatisfied by the lack of progression to supervisory level positions within the company. In fact, the company had no career progression plans nor a structured assessment of training needs for factory workers, who make up the majority of employees. Training was provided on-site by supervisors, managers and HR managers and off-site by external consultants when a specific skill or knowledge was needed. Equally employees could request to attend a course by choosing from a list of courses provided on a yearly basis. However, although the company was keen for employees to attend training courses, these were not systematically recorded on the employee file nor did they fit in a wider career plan.
Lucy is doing a part-time postgraduate diploma in HRM at The Open University and she has to complete a research project in order to gain CIPD membership. She has fully considered this issue and thought that it could become a good project. It did not come to her mind immediately but was the result of talks with her HR colleagues and her partner who helped her to see an opportunity where she could not see it.
Lucy talked to supervisors and factory workers and she analysed the organisational documents (appraisal records and exit interview records). Having searched the literature on blue-collar worker career development and training she compiled a loose structure (semi-structured) for interviews to be conducted with shop floor employees. At the end of her course she submitted a project which included the development of an online programme that managed a record system for each employee. The system brought together all the training courses completed as well as the performance records of each employee. It became much easier for managers and supervisors to identify the training courses attended by each employee as well as future training needs.
When people are employed in a job, or on a placement, and are undertaking research in the employing organisation they can be defined as (insider) practitioner–researchers. While the position of insider brings advantages in terms of knowledge and access to information and resources, there may be political issues to consider in undertaking and writing up the project. For example, a controversial or sensitive issue might emerge in the collection of data and the insider researcher has to consider carefully how to present it in their writing. Furthermore, while the organisation or some of its members may initially be willing to collaborate, resistance to full participation may be experienced during some stages of the project. If you are carrying out research in your own organisation it is therefore important to consider, in addition to its feasibility, any political issues likely to emerge and whether your status may affect the process of undertaking the research (Anderson, 2013).
Another way that can help you to identify a topic of investigation is through reading a HR magazine (such as People Management , Personnel Today or HR Magazine ), a journal article or even a newspaper. What topics do they include? Are you interested in any of them? Would the topic appeal to your organisation? Could it be developed into a feasible project?
If you develop an idea, however rudimentary, it is worth writing down the topic and a short sentence that captures some aspects of the topic. For example if the topic is ‘diversity in organisations’, you might want to write a note such as ‘relationship between diversity policy and practices’. From here you can start to develop a map of ideas that will help to identify keywords that can be used to do a more in-depth search of the literature. Figure 2 shows the first step in developing the idea.
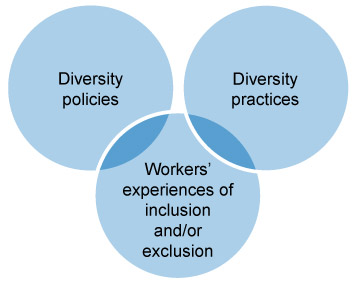
The figure shows three general approaches that you might take in developing a research project on diversity in organisations. You may decide to focus on one of these aspects and you could brainstorm it and add elements such as relationships with the various other aspects, national and organisational context, organisational sector, legislation, and aspects of diversity (e.g. age, gender, sexuality, race, etc.). Obviously this is an example but you can apply this process to any topic.
If you have an idea of a topic for your project, take five minutes to think about the possible perspectives from which it can be investigated. Having done this, take a sheet of paper and write the topic in the middle of the page. Alternatively, if you are still uncertain about the topic you want to investigate, you might want to think about the role of the HR practitioner and the various activities associated with this role. As you consider the various aspects of the role of HR practitioner, you may realise that one of these can become your chosen topic.
Starting from this core idea, now draw a mind map or a spider plan focusing on your chosen topic.

Mind mapping gives you a way to illustrate the various elements of an issue or topic and helps to clarify how these elements are linked to each other. A map is a good way to visualise, structure and organise ideas.
Now that you have identified your research topic you can move on to work on the research focus. Activity 3 will help you with this.
Develop a research statement of approximately 600 words explaining your chosen topic, the research problem and how you are thinking of investigating it.
You could start by using the mind map you developed in Activity 2. If you wished, you could do some online research around your topic to get a clearer focus on your own project. You might also read a few sources such as newspaper or magazine articles that are particularly relevant to your topic. These sources may yield some additional aspects for you to investigate. If your topic has been widely researched, try not to feel overwhelmed by the amount of existing information. Focus on one or two articles that appear more closely related to your topic and try to identify the specific area you want to focus on.
Once you have researched the topic, consider the research problem and how this can be investigated. Would you need to interview specific people? Would a questionnaire be more appropriate if you need to access a large number of individuals? Would you need to observe work practices in a specific organisation?
Your statement is a draft research proposal and should include:
- an overview of the topic area with an explanation of why you think this needs to be researched
- the focus of the research and the problem it addresses (and possibly the research questions if you have a clear idea of them at this stage, however, this is not essential if you are still developing the focus of your research)
- how the problem can be investigated (i.e. questionnaire, interviews, secondary data).
You may want to show this proposal to colleagues and other contacts who may be interested in order to gain their feedback about the focus and feasibility.
There is no feedback on this activity.
The next section discusses the ethical issues that need to be considered when doing a research project.
4 Research ethics
Ethics is a fundamental aspect of research and of professional work. Ethics refers to the science of morals and rules of behaviour. It is concerned with the concept of right and wrong conduct in all stages of doing research. However, while the idea of right and wrong conduct may seem straightforward, on reflection you will realise how complex ethics is. Ethics is obviously applied to many aspects of life, not just research, and, in business, topics such as ethics and social responsibility and ethical trading are often brought to people’s attention by the media.
As the meaning of what is ethical behaviour is often subjective and may have controversial elements, think about the following questions and make some notes:
- What does ethics in research mean for you?
- Why is ethical behaviour important for you?
- Why should ethics matter in research?
Research ethics is concerned with the prevention of any harm which may occur during the course of research. This is particularly important if your research involves human participants. Harm refers to psychological as well as physical harm. Human rights and the law must be respected by researchers with regard to the safety and wellbeing of their participants at all times. Research ethics is also concerned with identifying high standards of research conduct and putting them into practice. Cameron and Price (2009) suggest that researcher conduct is guided by a number of different obligations:
- Legal obligations which apply not only to the country in which researchers conduct the project, but also where they collect and store data.
- Professional obligations which are established by professional bodies (e.g. British Psychological Society, The Law Society, CIPD) to guide the conduct of its members.
- Cultural obligations which refer to informal rules regulating the behaviours of people within the society in which they live.
- Personal obligations which include the behavioural choices that individuals make of their own will.
In planning and carrying out a research project researchers should consider their responsibilities to the participants and respondents, to those sponsoring the research, and to the wider research community (Cameron and Price, 2009, p. 121). Before embarking on a research project it is worth identifying all stakeholders and considering your responsibilities towards them.
Generally universities and professional bodies have a list of principles or a code of ethics conduct that governs the research process. The principles to be followed in conducting research with human participants, and which you must follow when collecting data for your research project, are outlined below:
- Informed consent : Potential participants should always be informed in advance, and in understandable terms, of any potential benefits, risks, inconvenience or obligations associated with the research that might reasonably be expected to influence their willingness to participate. This should normally involve the use of an information sheet about the research and what participation will involve, and a signed consent form. Sufficient time shall be allowed for a potential participant to consider their decision from receiving the information sheet to giving their consent. In the case of children (individuals under 16 years of age) informed consent should be given by parents or guardians. An incentive to participate (e.g. a prize or a small payment) should be offered only after consent has been given. Participants should be informed clearly that they have a right to withdraw their consent at any time, that any data that they have provided will be destroyed if they so request and that there will be no resultant adverse consequences.
- Openness and integrity : Researchers should be open and honest about the purpose and content of their research and behave in a professional manner at all times. Covert collection of data should only take place where it is essential to achieve the research results required, where the research objective has strong scientific merit and where there is an appropriate risk management and harm alleviation strategy. Participants should be given opportunities to access the outcomes of research in which they have participated and debriefed, if appropriate, after they have provided data.
- Protection from harm : Researchers must make every effort to minimise the risks of any harm, either physical or psychological. Researchers shall comply with the requirements of the UK Data Protection Act 1998, the Freedom of Information Act 2000 and any other relevant legal frameworks governing the management of personal information in the UK or in any other country in which the research may be conducted. Where research involves children or other vulnerable groups, an appropriate level of disclosure should be obtained from the Disclosure and Barring Service for all researchers in contact with participants.
- Confidentiality : Except where explicit written consent is given, researchers should respect and preserve the confidentiality of participants’ identities and data at all times. The procedures by which this is to be achieved should be specified in the research protocol (an outline of the research topic and strategy).
- Professional codes of practice and ethics : Where the subject of a research project falls within the domain of a professional body with a published code of practice and ethical guidelines, researchers should explicitly state their intention to comply with the code and guidelines in the project protocol. Research within the UK NHS should always be conducted in compliance with an ethical protocol approved by the appropriate NHS Research Ethics Committee.
This guidance has been adapted from, and reflects the principles of, the Open University Research Ethics Guideline. The CIPD’s Code of Professional Conduct provides more information on professional standards in the field of HR.
Look at your research topic mind map and the research statement you wrote for Activity 3. Did you consider ethics? Regardless of whether or not you included ethics in your earlier outline of your research topic, consider what ethical factors could prevent you from conducting a research project on the chosen topic. Write your notes in the space provided below.
Describe at least two types of risks that could be encountered in HR research.
What is informed consent? What factors would you want to know before agreeing to participate in a research study? What should be included in an informed consent form?
This activity added the ethical dimension to the research topic, which was likely to be missed out in a previous outline of the project topic and aim(s). It also compels you to reflect on the specific ethical risks of HR research. There are risks associated with most HR research (e.g. stress can be induced by an interview or questionnaire questions) and it is important to consider them before finalising the research proposal. The activity also encouraged you to consider the elements that should be included in the informed consent form.
The next section considers the research question.
5 Developing research questions
By now you should have a clear direction for your research project. It is now necessary to think about the sorts of questions that you need to formulate in order to define your research project. Will they be ‘what’, ‘how’ or ‘why’ type questions? An important point to bear in mind, as discussed above, is that the wording of a question can be central in defining the scope and direction of the study, including the methodology. Your research question(s) do not necessarily have to be expressed as question(s); they can be statements of purpose. The research question is so called because it is a problem or issue that needs to be solved or addressed. Here are some examples of research questions that focus on different areas of HR. They are expressed as questions but they can easily be changed to research statements if the researcher prefers to present them in that way.
- How do the personal experiences and stories of career development processes among HR professionals in the UK and in Romania differ?
- To what extent do NHS managers engage with age diversity?
- How are organisational recruitment and selection practices influenced by the size of the organisation (e.g. small and medium-sized organisations, large national companies, multinational corporations)?
- In what ways, and for what reasons, does the internal perception of the organisational culture vary in relation to the culture that the organisation portrays in official documents?
- How are absenteeism levels linked to employees’ performance?
The process of producing clearly defined and focused research questions is likely to take some time. You will continue to tweak your research questions in the next few weeks even after you have written your research proposal, read the relevant literature and processed the information. The research questions above are very different but they also have common elements. The next activity invites you to think about research questions, both those in the examples above and your own, which may still be very tentative.
Make notes on the following questions:
- What constitutes a research question?
- What are the main pieces of information that a research question needs to contain?
This activity helped you to focus further on your research aims. What you wrote will guide you towards the development of your research questions. Figure 4 shows that research questions can contain a number of elements.

You should now note down possible research questions for your project. You might have only one question or possibly two. You do not need too many questions because you need to be realistic about what you can achieve in the project’s time frame. At the end of this course you will come back to your question(s) and finalise them.
6 Research strategy
A research strategy introduces the main components of a research project such as the research topic area and focus, the research perspective (see Sections 1 and 2), the research design, and the research methods (these are discussed below). It refers to how you propose to answer the research questions set and how you will implement the methodology.
In the first part of this course, you started to identify your research topic, to develop your research statement and you thought about possible research question(s). While you might already have clear research questions or objectives, it is possible that, at this stage, you are uncertain about the most appropriate strategy to implement in order to address those questions. This section looks briefly at a few research strategies you are likely to adopt.
Figure 5 shows the four main types of research strategy: case study, qualitative interviews, quantitative survey and action-oriented research. It is likely that you will use one of the first three; you are less likely to use action-oriented research.
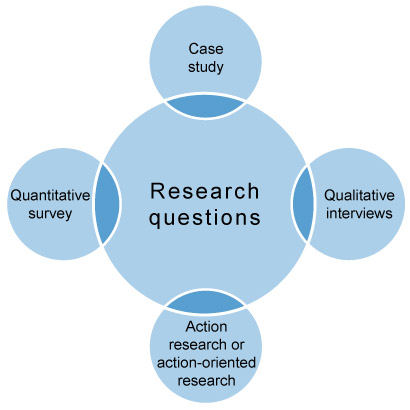
Here is what each of these strategies entails:
- Case Study : This focuses on an in-depth investigation of a single case (e.g. one organisation) or a small number of cases. In case study research generally, information is sought from different sources and through the use of different types of data such as observations, survey, interviews and analysis of documents. Data can be qualitative, quantitative or a mix of both. Case study research allows a composite and multifaceted investigation of the issue or problem.
- Qualitative interviews : There are different types of qualitative interviews (e.g. structured, semi-structured, unstructured) and this is the most widely used method for gathering data. Interviews allow access to rich information. They require extensive planning concerning the development of the structure, decisions about who to interview and how, whether to conduct individual or group interviews, and how to record and analyse them. Interviewees need a wide range of skills, including good social skills, listening skills and communication skills. Interviews are also time-consuming to conduct and they are prone to problems and biases that need to be minimised during the design stage.
- Quantitative survey : This is a widely used method in business research and allows access to significantly high numbers of participants. The availability of online sites enables the wide and cheap distribution of surveys and the organisation of the responses. Although the development of questions may appear easy, to develop a meaningful questionnaire that allows the answering of research questions is difficult. Questionnaires need to appeal to respondents, cannot be too long, too intrusive or too difficult to understand. They also need to measure accurately the issue under investigation. For these reasons it is also advisable, when possible, to use questionnaires that are available on the market and have already been thoroughly validated. This is highly recommended for projects such as the one you need to carry out for this course. When using questionnaires decisions have to be made about the size of the sample and whether and when this is representative of the whole population studied. Surveys can be administered to the whole population (census), for example to all employees of a specific organisation.
- Action-oriented research : This refers to practical business research which is directed towards a change or the production of recommendations for change. Action-oriented research is a participatory process which brings together theory and practice, action and reflection. The project is often carried out by insiders. This is because it is grounded in the need to actively involve participants in order for them to develop ownership of the project. After the project, participants will have to implement the change.
Action-oriented research is not exactly action research, even though they are both grounded in the same assumptions (e.g. to produce change). Action research is a highly complex approach to research, reflection and change which is not always achievable in practice (Cameron and Price, 2009). Furthermore action researchers have to be highly skilled and it is unlikely that for this specific project you will be involved in action research. For these reasons this overview focuses on the less pure action-oriented research strategy. If you are interested in exploring this strategy and action research further, you might want to read Chapter 14 of Cameron and Price (2009).
It is possible for you to choose a strategy that includes the use of secondary data. Secondary data is data that has been collected by other people (e.g. employee surveys, market research data, census). Using secondary data for your research project needs to be justified in that it meets the requirements of the research questions. The use of secondary data has obvious benefits in terms of saving money and time. However, it is important to ascertain the quality of the data and how it was collected; for example, data collected by government agencies would be good quality but it may not necessary meet the needs of your project.
It is important to note that there should be consistency between the perspective (subjective or objective) and the methodology employed. This means that the type of strategy adopted needs to be coherent and that its various elements need to fit in with each other, whether the research is grounded on primary or secondary data.
Now watch this video clip in which Dr Rebecca Hewett, Prof Mark Saunders, Prof Gillian Symon and Prof David Guest discuss the importance of setting the right research question, what strategy they adopted to come up with specific research questions for their projects, and how they refined these initial research questions to focus their research.

Make notes on how you might apply some of these strategies to develop your own research question.
7 Research design
In planning your project you need to think about how you will design and conduct the study as well as how you will present and write up the findings. The design is highly dependent upon the research strategy. It refers to the practical choices regarding how the strategy is implemented in practice. You need to think about what type of data (evidence) would best address the research questions; for example, when considering case study research, questions of design will address the choice of the specific methods of data collection, e.g. if observation, what to observe and how to record it? For how long? Which department or work environment to observe? If interviews are chosen, you need to ask yourself what type? How many? With whom? How long should they be? How will I record them? Where will they be conducted?
The following list (adapted from Cameron and Price, 2009) shows some of the different types of data, or sources of evidence, available to draw on:
- observations
- conversations
- statistics (e.g. government)
- focus groups
- organisational records
- documents (e.g. organisational policies)
- secondary data.
8 Research methodology
The most important methodological choice researchers make is based on the distinction between qualitative and quantitative data. As mentioned previously, qualitative data takes the form of descriptions based on language or images, while quantitative data takes the form of numbers.
Qualitative data is richer and is generally grounded in a subjective and interpretivist perspective. However, while this is generally the case, it is not always so. Qualitative research supports an in-depth understanding of the situation investigated and, due to time constraints, it generally involves a small sample of participants. For this reason the findings are limited to the sample studied and cannot be generalised to other contexts or to the wider population. Popular methods based on qualitative data include semi-structured or unstructured interviews, participant observations and document analysis. Qualitative analysis is generally more time-consuming than quantitative analysis.
Quantitative data, on the other hand, might be easier to collect and analyse and it is based on a large sample of participants. Quantitative methods are based on data that can be ‘objectively’ measured with numbers. The data is analysed through numerical comparisons and statistical analysis. For this reason it appears more ‘scientific’ and may appeal to people who seek clear answers to specific causal questions. Quantitative analysis is often quicker to carry out as it involves the use of software. Owing to the large number of respondents it allows generalisation to a wider group than the research sample. Popular methods based on quantitative data include questionnaires and organisational statistical records among others.
The choice of which methodology to use will depend on your research questions, the formulation of which is consequently informed by your research perspective. Generally, unstructured or semi-structured interviews produce qualitative data and questionnaires produce quantitative data, but such a distinction is not always applicable. In fact, language-based data can often be translated into numbers; for example, by reporting the frequency of certain key words. Questionnaires can produce quantitative as well as qualitative data; for example, multiple choice questions produce quantitative data, while open questions produce qualitative data.
Go back to the two papers you started reading in Activity 1 and read the methodology sections (they may be called methods or something similar) of both papers.
Now answer the following questions.
- What type of method(s) have the author/s in article 1 used to collect data?
- What method of analysis have these author/s used?
- What type of method(s) have the author/s in article 2 used to collect data?
- What methods of analysis have these author/s used?
- How do you think the methods used in both papers address the initial research aims or questions?
- Why do you think the methods used in both papers are appropriate to address these initial research aims or questions?
If you have chosen two papers based on different methodologies, you should reflect on the link between the ways in which the purposes of the studies were developed and the specific methods that the authors chose to address those questions. In the articles there should be a fit between research questions and methodology for collecting and analysing the data. The activity should have helped you to familiarise yourself with processes of planning a research methodology that fits the research question.
This course so far has given you an overview of the research strategy, design and possible methodologies for collecting data. What you have learned should be enough for you to have developed a clear idea of the general research strategy you want to adopt before you move on to develop your methodology for collecting data and review the methods in detail so that you are clear about the benefits and limitations of each before you collect your data.
9 Further development of your research questions
Before you embark on collecting data for your project, it is necessary to have a clear and specific focus for your research – i.e. the research aim, purpose or question(s). Section 5 gave you some examples of research questions. As a research question does not necessarily need to be expressed as a question (it is called a question because it is a problem to be solved), a study can have one general research aim or question and a few secondary aims or questions. It may also have only one research aim or question. In the case of quantitative studies, a few hypotheses might be developed. These will emerge from gaps in the literature but would have to derive from, and be linked to, an overall research purpose. Section 10 discusses research hypotheses.
The clear development of one or more research questions will guide the development of your data collection process and the tool(s) or instrument(s) you will use. Your research question should emerge from a specific need to acquire greater knowledge about a phenomenon or a situation. Such need may be a personal one as well as a contextual and organisational need. Box 1 gives an example of this.
Box 1 An example of how to develop a research question
As a consequence of government cuts, your arts organisation has to re-structure and this is causing stress and tension among staff. You are involved in the planning of the change initiative and want to develop an organisational change programme that minimises stress and conflict. In order to do so you need to know more about people’s views, at the various organisational levels.
What type of questions would help you to:
- understand the context
- demonstrate to the various research stakeholders (e.g. organisational members and research participants or supervisor, etc.) what you intend to do.
Perhaps you would like to make some notes of your initial ideas and think about how you could apply this process to developing your own research question.
Reading around the topic will help you to achieve greater focus, as will discussing your initial questions with colleagues or supervisor. In the example above, assuming the literature has been searched and several articles on change management and business restructuring have been read, you are likely to have developed clearer ideas about what you want to investigate and how you want to investigate it. Figure 6 shows what the main research question and the sub-questions or objectives might be:

This example is very well developed and would constitute a much larger project than the work-based project you might be doing. However the development of a general question and more specific questions focusing on different aspects should give you an idea of the relationship between the main question and the sub-questions.
In developing your research questions you also need to be concerned with issues of feasibility in terms of access and time. You do not need to be over ambitious but you need to realistically evaluate how difficult it would be to get the data you are planning in the time you have available before submitting the project at the end of the course. You need to plan a project that is neither too broad nor too narrow in scope and one that can be carried out in the available time.
Revisit your research topic and look back at the notes you have made about the topic (including the mind map you developed earlier in the course). Expand and amend where you need to.
Now think about what you need to do to re-write your statement as a research question and write the question in the space provided below.
Write a maximum of four sub-questions or research objectives that will help you to answer the main research question given above. In formulating the sub-questions make sure you consider the scope and the feasibility of the project. Write your questions in the space provided below.
10 Research hypotheses
For quantitative studies a research question can be further focused into a hypothesis. This is not universally the case – especially in exploratory research when little is known and so it is difficult to develop hypotheses – however it is generally the case in explanatory projects. A hypothesis usually makes a short statement concerning the relationship between two or more aspects or variables; the research thus aims to verify the hypothesis through investigation. According to Verma and Beard (1981, p. 184) ‘in many cases hypotheses are hunches that the researcher has about the existence of relationships between variables’. A hypothesis differs from a research question in several ways. The main difference is that a question is specific and asks about the relationship between different aspects of a problem or issue, whereas a hypothesis suggests a possible answer to the problem, which can then be tested empirically. You will now see how a research question (RQ) may be formulated as a research hypothesis (RH).
- RQ: Does motivation affect employees’ performance?
This is a well-defined research question; it explores the contribution of motivation to the work performance of employees. The question omits other possible causes, such as organisational resources and market conditions. This question could be turned into a research hypothesis by simply changing the emphasis:
- RH: Work motivation is positively related to employees’ performance.
The key elements of a hypothesis are:
- The variables used in a hypothesis must all be empirically measurable (e.g. you need to be able to measure motivation and performance objectively).
- A hypothesis should provide an answer (albeit tentatively) to the question raised by the problem statement.
- A hypothesis should be as simple as possible.
If you are planning to do an explanatory quantitative study, you will need to develop hypotheses. You will develop your hypotheses once you have read and reviewed the literature and have become familiar with previous knowledge about the topic.
In this free course, Understanding different research perspectives , the various perspectives (subjective/objective and interpretivist/positivist) that a researcher can take in investigating a problem have been discussed, as well as the issues that need to be considered in planning the project (ethics, research design, research strategy and research methodology). This fits with the first two stages of the overall research process as shown in Table 2.
The activities in this course have guided you through these first two processes.
Acknowledgements
This free course was written by Cinzia Priola.
Except for third party materials and otherwise stated (see terms and conditions ), this content is made available under a Creative Commons Attribution-NonCommercial-ShareAlike 4.0 Licence .
The material acknowledged below is Proprietary and used under licence (not subject to Creative Commons Licence). Grateful acknowledgement is made to the following sources for permission to reproduce material in this free course:
Course Image: © Kristian Sekulic/iStockphoto.com
Figure 2: © Yuri/iStockphoto.com
4. Research Ethics: CIPD’s Code of Professional Conduct: courtesy of Chartered Institute of Personnel and Development (CIPD)
7. Research and Design: extract adapted from: Cameron, S. and Price, D. (2009) Business Research Methods: A Practical Approach , London, CIPD
Every effort has been made to contact copyright owners. If any have been inadvertently overlooked, the publishers will be pleased to make the necessary arrangements at the first opportunity.
Don’t miss out
If reading this text has inspired you to learn more, you may be interested in joining the millions of people who discover our free learning resources and qualifications by visiting The Open University – www.open.edu/ openlearn/ free-courses .
Copyright © 2016 The Open University
- Search for: Toggle Search
‘You Transformed the World,’ NVIDIA CEO Tells Researchers Behind Landmark AI Paper
Of GTC ’s 900+ sessions, the most wildly popular was a conversation hosted by NVIDIA founder and CEO Jensen Huang with seven of the authors of the legendary research paper that introduced the aptly named transformer — a neural network architecture that went on to change the deep learning landscape and enable today’s era of generative AI.
“Everything that we’re enjoying today can be traced back to that moment,” Huang said to a packed room with hundreds of attendees, who heard him speak with the authors of “ Attention Is All You Need .”
Sharing the stage for the first time, the research luminaries reflected on the factors that led to their original paper, which has been cited more than 100,000 times since it was first published and presented at the NeurIPS AI conference. They also discussed their latest projects and offered insights into future directions for the field of generative AI.
While they started as Google researchers, the collaborators are now spread across the industry, most as founders of their own AI companies.
“We have a whole industry that is grateful for the work that you guys did,” Huang said.
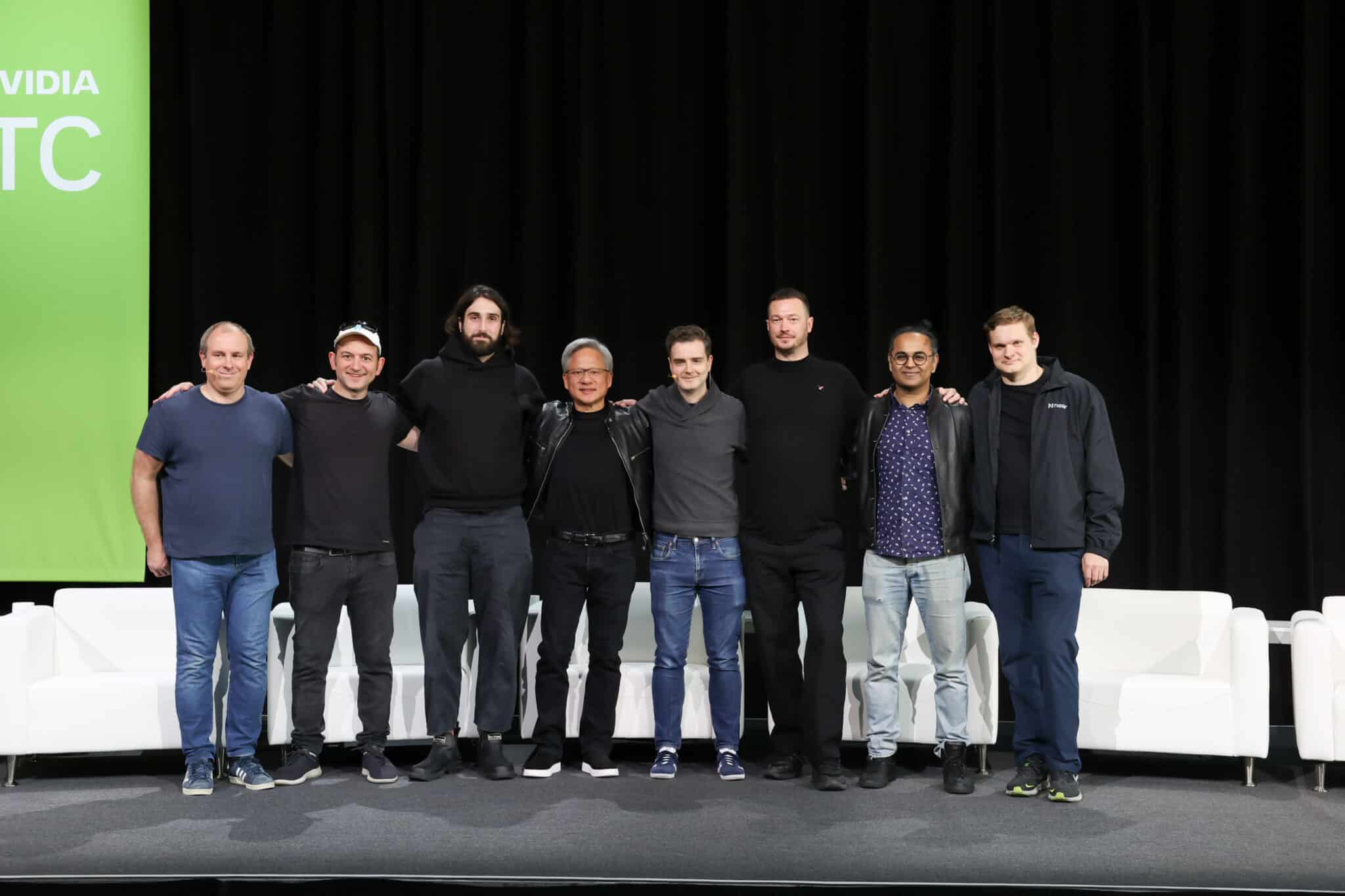
Origins of the Transformer Model
The research team initially sought to overcome the limitations of recurrent neural networks , or RNNs, which were then the state of the art for processing language data.
Noam Shazeer, cofounder and CEO of Character.AI, compared RNNs to the steam engine and transformers to the improved efficiency of internal combustion.
“We could have done the industrial revolution on the steam engine, but it would just have been a pain,” he said. “Things went way, way better with internal combustion.”
“Now we’re just waiting for the fusion,” quipped Illia Polosukhin, cofounder of blockchain company NEAR Protocol.
The paper’s title came from a realization that attention mechanisms — an element of neural networks that enable them to determine the relationship between different parts of input data — were the most critical component of their model’s performance.
“We had very recently started throwing bits of the model away, just to see how much worse it would get. And to our surprise it started getting better,” said Llion Jones, cofounder and chief technology officer at Sakana AI.
Having a name as general as “transformers” spoke to the team’s ambitions to build AI models that could process and transform every data type — including text, images, audio, tensors and biological data.
“That North Star, it was there on day zero, and so it’s been really exciting and gratifying to watch that come to fruition,” said Aidan Gomez, cofounder and CEO of Cohere. “We’re actually seeing it happen now.”
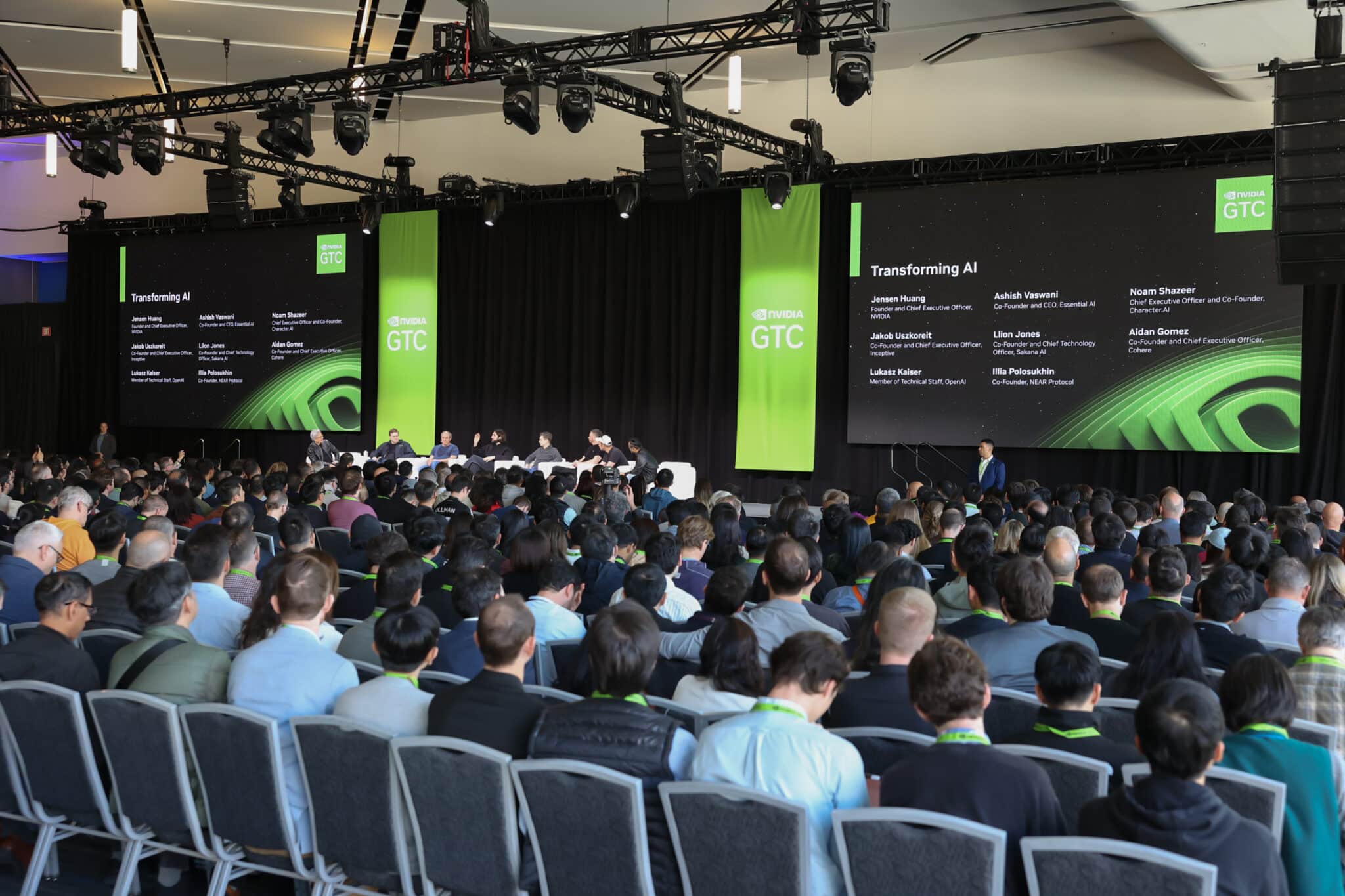
Envisioning the Road Ahead
Adaptive computation, where a model adjusts how much computing power is used based on the complexity of a given problem, is a key factor the researchers see improving in future AI models.
“It’s really about spending the right amount of effort and ultimately energy on a given problem,” said Jakob Uszkoreit, cofounder and CEO of biological software company Inceptive. “You don’t want to spend too much on a problem that’s easy or too little on a problem that’s hard.”
A math problem like two plus two, for example, shouldn’t be run through a trillion-parameter transformer model — it should run on a basic calculator, the group agreed.
They’re also looking forward to the next generation of AI models.
“I think the world needs something better than the transformer,” said Gomez. “I think all of us here hope it gets succeeded by something that will carry us to a new plateau of performance.”
“You don’t want to miss these next 10 years,” Huang said. “Unbelievable new capabilities will be invented.”
The conversation concluded with Huang presenting each researcher with a framed cover plate of the NVIDIA DGX-1 AI supercomputer, signed with the message, “You transformed the world.”
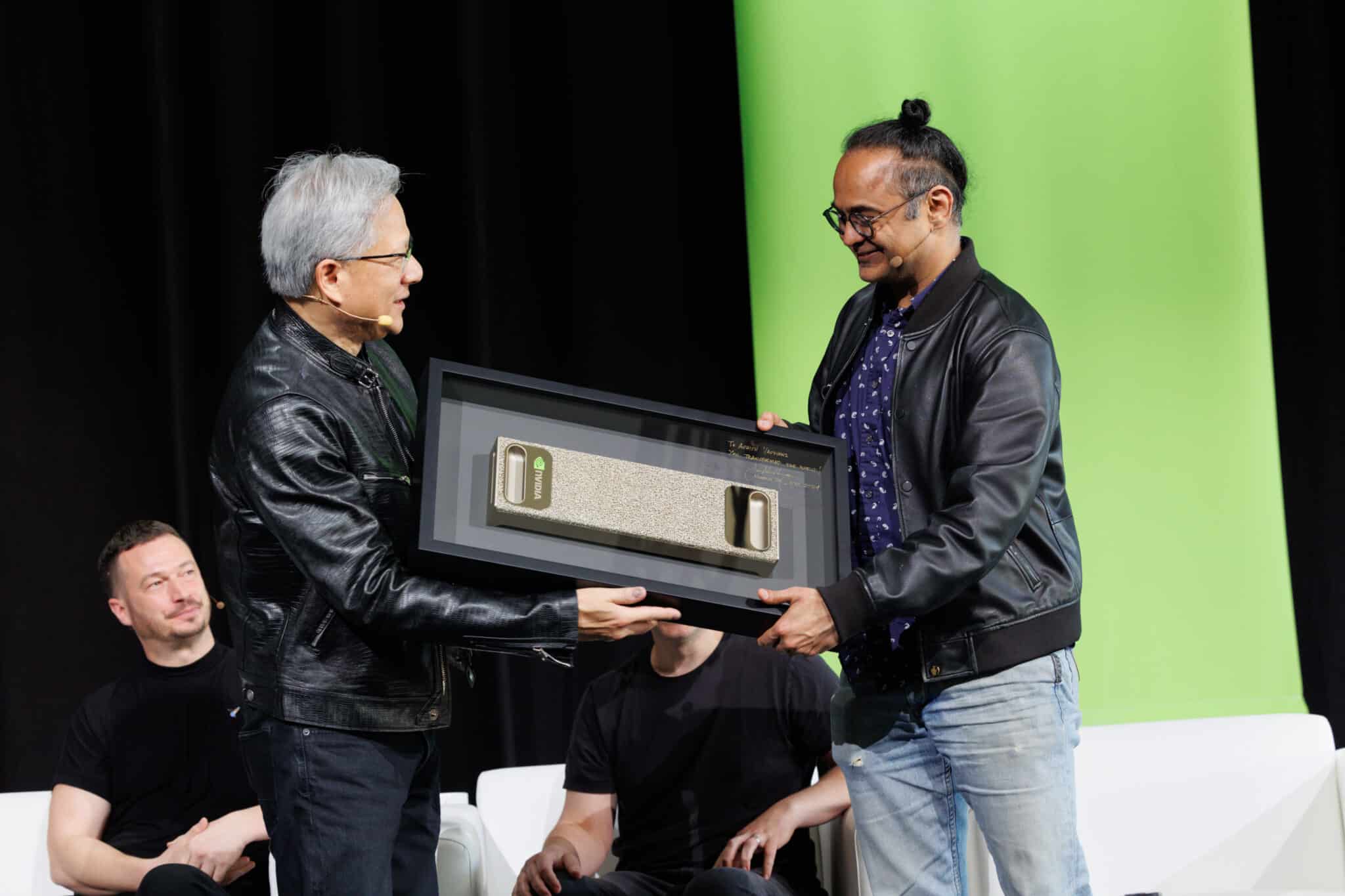
There’s still time to catch the session replay by registering for a virtual GTC pass — it’s free.
To discover the latest in generative AI, watch Huang’s GTC keynote address:
NVIDIA websites use cookies to deliver and improve the website experience. See our cookie policy for further details on how we use cookies and how to change your cookie settings.
Share on Mastodon
ORIGINAL RESEARCH article
This article is part of the research topic.
Psychological Transformation in Technology-Enhanced Learning Environments (TELEs): Focus on Teachers and Learners
Developing Community of Inquiry using Educational Blog in Higher Education of Bangladesh Perspective Provisionally Accepted
- 1 Institute of Education and Research (IER), University of Dhaka, Bangladesh
The final, formatted version of the article will be published soon.
Web 2.0 tools such as blogs, wikis, social networking, podcasting etc. have received concentration in educational research over the last decade. Blogs enable students to reflect their learning experiences, disseminate ideas, and participate in analytical thinking. The Community of Inquiry (CoI) framework has been widely utilized in educational research to understand and enhance online and blended learning environments. There is insufficient research evidence to demonstrate the impact of educational blogging utilizing the CoI model as a framework. This paper explores how blogs can be used to support collaborative learning and how such interaction upholds CoI through enhancing critical thinking and meaningful learning in the context of Higher Education (HE). An exploratory mixed method research approach has been followed in this study. A convenience sampling method was employed to choose 75 undergraduate students from Dhaka University for a 24-week blogging project. Every publication on the blog was segmented into meaningful units. Whole texts of posts and comments are extracted from the blog and the transcripts are analyzed in Qualitative manner considering CoI framework, more specifically, through the lens of cognitive, social, and teaching presence. In addition, the semi-structured questionnaire is used to collect data from students whether blogging expediated students' learning or not. The research findings indicate that cognitive presence, namely the exploration component, is dominant in blog-based learning activity. Moreover, this research has demonstrated that blogs build reliable virtual connections among students through exchanging ideas and information and by offering opportunities for reflective practice and asynchronous feedback. This study also revealed challenges related to blogging in the context of developing countries, including lack of familiarity with blogs, restricted internet connectivity, limited access to devices, and low levels of social interaction. It is recommended that different stakeholders including policy makers, curriculum developers and teachers may take the initiative to synchronize the utilization of educational blogs with the formal curriculum, guaranteeing that blog activities supplement and improve traditional teaching-learning activities.
Keywords: web 2.0, Community of inquiry (COI), Blog, Collaborative Learning, Learning outcome
Received: 26 Sep 2023; Accepted: 25 Mar 2024.
Copyright: © 2024 Chowdhury and Siddique. This is an open-access article distributed under the terms of the Creative Commons Attribution License (CC BY) . The use, distribution or reproduction in other forums is permitted, provided the original author(s) or licensor are credited and that the original publication in this journal is cited, in accordance with accepted academic practice. No use, distribution or reproduction is permitted which does not comply with these terms.
* Correspondence: Mr. Sabbir A. Chowdhury, University of Dhaka, Institute of Education and Research (IER), Dhaka, Bangladesh
People also looked at
Intermittent fasting linked to higher risk of cardiovascular death, research suggests
Intermittent fasting, a diet pattern that involves alternating between periods of fasting and eating, can lower blood pressure and help some people lose weight , past research has indicated.
But an analysis presented Monday at the American Heart Association’s scientific sessions in Chicago challenges the notion that intermittent fasting is good for heart health. Instead, researchers from Shanghai Jiao Tong University School of Medicine in China found that people who restricted food consumption to less than eight hours per day had a 91% higher risk of dying from cardiovascular disease over a median period of eight years, relative to people who ate across 12 to 16 hours.
It’s some of the first research investigating the association between time-restricted eating (a type of intermittent fasting) and the risk of death from cardiovascular disease.
The analysis — which has not yet been peer-reviewed or published in an academic journal — is based on data from the Centers for Disease Control and Prevention’s National Health and Nutrition Examination Survey collected between 2003 and 2018. The researchers analyzed responses from around 20,000 adults who recorded what they ate for at least two days, then looked at who had died from cardiovascular disease after a median follow-up period of eight years.
However, Victor Wenze Zhong, a co-author of the analysis, said it’s too early to make specific recommendations about intermittent fasting based on his research alone.
“Practicing intermittent fasting for a short period such as 3 months may likely lead to benefits on reducing weight and improving cardiometabolic health,” Zhong said via email. But he added that people “should be extremely cautious” about intermittent fasting for longer periods of time, such as years.
Intermittent fasting regimens vary widely. A common schedule is to restrict eating to a period of six to eight hours per day, which can lead people to consume fewer calories, though some eat the same amount in a shorter time. Another popular schedule is the "5:2 diet," which involves eating 500 to 600 calories on two nonconsecutive days of the week but eating normally for the other five.
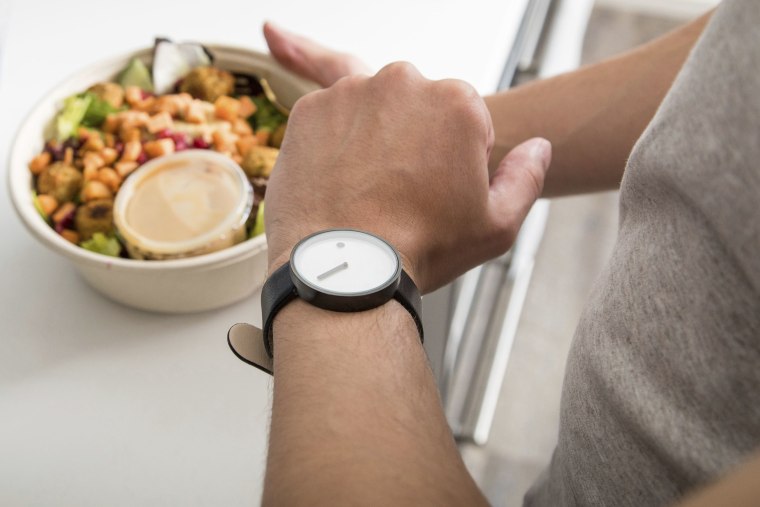
Zhong said it’s not clear why his research found an association between time-restricted eating and a risk of death from cardiovascular disease. He offered an observation, though: People who limited their eating to fewer than eight hours per day had less lean muscle mass than those who ate for 12 to 16 hours. Low lean muscle mass has been linked to a higher risk of cardiovascular death .
Cardiovascular and nutrition experts who were not involved in the analysis offered several theories about what might explain the results.
Dr. Benjamin Horne, a research professor at Intermountain Health in Salt Lake City, said fasting can increase stress hormones such as cortisol and adrenaline, since the body doesn’t know when to expect food next and goes into survival mode. That added stress may raise the short-term risk of heart problems among vulnerable groups, he said, particularly elderly people or those with chronic health conditions.
Horne’s research has shown that fasting twice a week for four weeks, then once a week for 22 weeks may increase a person’s risk of dying after one year but decrease their 10-year risk of chronic disease.
“In the long term, what it does is reduces those risk factors for heart disease and reduces the risk factors for diabetes and so forth — but in the short term, while you’re actually doing it, your body is in a state where it’s at a higher risk of having problems,” he said.
Even so, Horne added, the analysis “doesn’t change my perspective that there are definite benefits from fasting, but it’s a cautionary tale that we need to be aware that there are definite, potentially major, adverse effects.”
Intermittent fasting gained popularity about a decade ago, when the 5:2 diet was touted as a weight loss strategy in the U.K. In the years to follow, several celebrities espoused the benefits of an eight-hour eating window for weight loss, while some Silicon Valley tech workers believed that extreme periods of fasting boosted productivity . Some studies have also suggested that intermittent fasting might help extend people’s lifespans by warding off disease .
However, a lot of early research on intermittent fasting involved animals. In the last seven years or so, various clinical trials have investigated potential benefits for humans, including for heart health.
“The purpose of intermittent fasting is to cut calories, lose weight,” said Penny Kris-Etherton, emeritus professor of nutritional sciences at Penn State University and a member of the American Heart Association nutrition committee. “It’s really how intermittent fasting is implemented that’s going to explain a lot of the benefits or adverse associations.”
Dr. Francisco Lopez-Jimenez, a cardiologist at Mayo Clinic, said the timing of when people eat may influence the effects they see.
“I haven’t met a single person or patient that has been practicing intermittent fasting by skipping dinner,” he said, noting that people more often skip breakfast, a schedule associated with an increased risk of heart disease and death .
The new research comes with limitations: It relies on people’s memories of what they consumed over a 24-hour period and doesn’t consider the nutritional quality of the food they ate or how many calories they consumed during an eating window.
So some experts found the analysis too narrow.
“It’s a retrospective study looking at two days’ worth of data, and drawing some very big conclusions from a very limited snapshot into a person’s lifestyle habits,” said Dr. Pam Taub, a cardiologist at UC San Diego Health.
Taub said her patients have seen “incredible benefits” from fasting regimens.
“I would continue doing it,” she said. “For people that do intermittent fasting, their individual results speak for themselves. Most people that do intermittent fasting, the reason they continue it is they see a decrease in their weight. They see a decrease in blood pressure. They see an improvement in their LDL cholesterol.”
Kris-Etherton, however, urged caution: “Maybe consider a pause in intermittent fasting until we have more information or until the results of the study can be better explained,” she said.

Aria Bendix is the breaking health reporter for NBC News Digital.
- Share full article
Advertisement
Supported by
Study About Purported Ancient ‘Pyramid’ in Indonesia Is Retracted
The study, based on research featured in a Netflix documentary, fueled debate over a site that is used for Islamic and Hindu rituals.

By Mike Ives
Reporting from Seoul
The American publisher of a study that challenged scientific orthodoxy by claiming that an archaeological site in Indonesia may be the world’s “oldest pyramid” says it has been retracted.
The October 2023 study in the journal Archaeological Prospection made the explosive claim that the deepest layer of the site, Gunung Padang, appears to have been “sculpted” by humans up to 27,000 years ago.
The study’s critics say that it incorrectly dated the human presence at Gunung Padang based on radiocarbon measurements of soil from drilling samples, not artifacts. The journal’s American publisher, Wiley, cited that exact reasoning in the retraction notice it issued on Monday.
Gunung Padang is widely considered a dormant volcano, and archaeologists say that ceramics recovered there so far suggest that humans have been using it for several hundred years or more — not anything close to 27,000 years. The pyramids of Giza in Egypt are only about 4,500 years old.
The retraction, based on a monthslong investigation, said that the study was flawed because its soil samples “were not associated with any artifacts or features that could be reliably interpreted as anthropogenic or ‘man-made.’”
Some archaeologists said in interviews that they welcomed the retraction. But the study’s authors called it “unjust,” saying in a statement on Wednesday that their soil samples had been “unequivocally established as man-made constructions or archaeological features,” in part because the soil layers included artifacts.
“We urge the academic community, scientific organizations, and concerned individuals to stand with us in challenging this decision and upholding the principles of integrity, transparency, and fairness in scientific research and publishing,” the authors wrote.
The study ’s lead author, Danny Hilman Natawidjaja, an earthquake geologist, did not immediately respond to a request for comment. Neither did Wiley or the editors of Archaeological Prospection, Eileen Ernenwein and Gregory Tsokas.
One prominent supporter of Mr. Natawidjaja’s research, the journalist Graham Hancock, said in a statement he did not see the retraction as “fair, justified or good science.” He said that instead of issuing a retraction, the journal should have published critiques of the paper, a move he said would have allowed readers to make up their own minds.
“Science should not be about suppression,” said Mr. Hancock, who interviewed Mr. Natawidjaja for an episode about Gunung Padang on “ Ancient Apocalypse, ” his 2022 Netflix documentary series.
The Society for American Archaeology has said that Mr. Hancock’s Netflix show “devalues the archaeological profession on the basis of false claims and disinformation.” He has vigorously rejected that argument, arguing that archaeologists should be more open to theories that challenge academic orthodoxy. Netflix did not respond to a request for comment on the retraction.
People from Indonesia have long traveled to Gunung Padang, a hilltop site dotted with stone terraces, to hold Islamic and Hindu rituals. A domestic narrative portraying it as a very, very old pyramid had support, and financing, from the central government during the administration of President Susilo Bambang Yudhoyono, who left office in 2014. His successor, President Joko Widodo, cut off the funding.
Archaeologists said in interviews on Wednesday that they welcomed the retraction.
One of them, Noel Hidalgo Tan, an archaeologist in Bangkok who had relayed his concerns about the study to Wiley, said that he considered the retraction “entirely appropriate” because the study’s evidence did not support its conclusions.
“It was unfortunate that the paper had to get to this stage,” said Dr. Tan, who works at the Southeast Asian Regional Center for Archaeology and Fine Arts. “But it was better to be retracted than to have nothing said about it at all.”
Dwi Ratna Nurhajarini, the head of the Cultural Heritage Conservation Office in West Java Province, the location of the site, said the study’s conclusions should be re-examined in light of the retraction.
“The structures at Gunung Padang are indeed layered and terraced, reminiscent of civilizations from Indonesia’s distant past,” she said by phone on Wednesday. “But their age might not be as old as suggested.”
Rin Hindryati contributed reporting.
Mike Ives is a reporter for The Times based in Seoul, covering breaking news around the world. More about Mike Ives
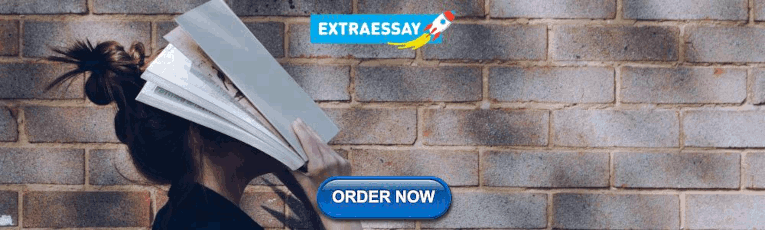
IMAGES
VIDEO
COMMENTS
Perspective, opinion, and commentary articles are scholarly articles which express a personal opinion or a new perspective about existing research on a particular topic. These do not require original research, and are, therefore, less time-consuming than original research articles. However, the author needs to have in-depth knowledge of the topic.
Like many journals, Journal of Biogeography (JBI) provides a specific forum for researchers to put forward new ideas (or dismantle old ones).In JBI, this article type is the Perspective.Our Author Guidelines state that Perspective papers "should be stimulating and reflective essays providing personal perspectives on key research fields and issues within biogeography".
Abstract: Behavior and attitude of students in the new normal perspectives have an. impact in their learning process. It contributes to self-determination in the new normal. classes and framework ...
The general prevalence of gout is 1-4% of the general population. In western countries, it occurs in 3-6% in men and 1-2% in women. In some countries, prevalence may increase up to 10%. Prevalence rises up to 10% in men and 6% in women more than 80 years old. Annual incidence of gout is 2.68 per 1000 persons.
Furthermore, Spoorthy et al. (2020) conducted a review on the gendered impact of Covid-19 and found that 68.7-85.5% of medical staff is composed of women, and the mean age ranged between 26 and 40 years. Also, women are more likely to be affect by anxiety, depression, and distress ( Lai et al., 2020; Zanardo et al., 2020 ).
This paper provides a different perspective by examining relevant challenges and providing examples of some less-talked-about yet essential topics, such as hybrid systems, redefining advanced manufacturing, basic building blocks of new manufacturing, ecosystem readiness, and technology scalability.
This information will provide support in the new direction of research and hopefully provide insight into how to continue future research that includes perspectives from a cross-cultural lens. ... Wiggins, V. (2023). The Importance of Including Students' Perspectives in Research to Further Understand New Learning Environments. In: Chan, R.Y ...
In a recent paper published in Child Development Perspectives, Volling and colleagues (Cabrera et al., 2018) argued that fathers are parents too and there was a need for expanding conceptualizations of parenting to include fathers and a focus on the father-child relationship.In addition, Palkovitz (2019) recently published an article in the Journal of Family Theory and Review arguing that it ...
Putting time in perspective: A valid, reliable individual-differences metric. Journal of Personality and Social Psychology, 77, 1271-1288. Google Scholar; Zimbardo P. G., Boyd J. N. (2008). The time paradox: The new psychology of time that will change your life. New York: The Free Press. Google Scholar
New Perspectives is committed to the effective communication of high-quality original research to wider public audiences, and thereby seeks to foster the creation of useful knowledge, broadly understood. Each volume centres on blind peer-reviewed research articles. The journal also welcomes review essays, interviews, auto-ethnographic ...
Before submitting your manuscript to New Perspectives please ensure you have read the Aims & Scope. 1.2 Article Types. Research Articles are full-length papers that make an original contribution to research and are the main type of article that we seek. New Perspectives particularly seeks research articles that are methodologically systematic ...
Animal camouflage: current issues and new perspectives. 1. The importance and history of camouflage research. The study of camouflage has a long history in biology, and the numerous ways of concealment and disguise found in the animal kingdom provided Darwin and Wallace with important examples for illustrating and defending their ideas of ...
Abstract. In this paper we describe the development of a theory and a new measure of military leadership. Based on a definition of military leadership and Yukl's (2013) three metacategories of ...
A perspective article is a way for young researchers to gain experience in the publications process that can be often arduous and time consuming. It can be a way in which they learn from the publication process while they are working on their original research articles that often take years to complete. In the case of experienced researchers ...
Indigenous Peoples and contexts have offered valuable insights to enrich management and organization theories and literature. Yet, despite their growing prevalence and impact, these insights have not been compiled and synthesized comprehensively. With this article, we provide a systematic and thorough analysis of Indigenous management and organization studies research published over a 90-year ...
Employability skills. Datasets. Templates and checklists. Chapter 2: Theoretical Perspectives and Research Methodologies. Chapter 3: Selecting and Planning Research Proposals and Projects. Chapter 4: Research Ethics. Chapter 5: Searching, Critically Reviewing and Using the Literature. Chapter 6: Research Design: Quantitative Methods.
The visions of AI Oracles, Surrogates, Quants and Arbiters appear in papers authored both by scientists without AI expertise and by researchers who study AI, either through interdisciplinary ...
Svitlana Bulbeniuk. Olha Naumenko. Preview abstract. Restricted access Research article First published December 18, 2023 pp. 91-107. xml GET ACCESS. Table of contents for New Perspectives, 32, 1, Mar 01, 2024.
Each volume centres on blind peer-reviewed research articles. The journal also welcomes review essays, interviews, auto-ethnographic commentaries, collaborative texts, and other non-traditional or creative formats of scholarship. New Perspectives is a member of the Committee on Publication Ethics (COPE).
The paper, co-authored by Princeton cognitive scientist M. J. Crockett, sets a framework for discussing the risks involved in using AI tools throughout the scientific research process, from study design through peer review. " We hope this paper offers a vocabulary for talking about AI's potential epistemic risks," Messeri said.
Introduction. With its negative consequences for wellbeing, bullying is a major public health concern affecting the lives of many children and adolescents (Holt et al. 2014; Liu et al. 2014 ). Bullying can take many different forms and include aggressive behaviours that are physical, verbal or psychological in nature (Wang, Iannotti, and Nansel ...
On online social networks such as Facebook Footnote 1, Twitter Footnote 2, and Sina Weibo Footnote 3, people share their opinions, videos, and news on their various activities (Gao and Liu 2014; Islam et al. 2018a).While people enjoy social networks, many deceptive activities such as fake news, or rumors can mislead users into believing misinformation (Kumar et al. 2016).
Understanding different research perspectives Introduction. In this free course, Understanding different research perspectives, you will explore the development of the research process and focus on the steps you need to follow in order to plan and design a HR research project. The course comprises three parts: The first part (Sections 1 and 2) discusses the different perspectives from which an ...
A version of this article appears in print on , Section A, Page 2 of the New York edition with the headline: A Psychedelics Reporter With a New Perspective. Order Reprints | Today's Paper ...
Of GTC's 900+ sessions, the most wildly popular was a conversation hosted by NVIDIA founder and CEO Jensen Huang with seven of the authors of the legendary research paper that introduced the aptly named transformer — a neural network architecture that went on to change the deep learning landscape and enable today's era of generative AI. ...
The New German-speaking School of Balkankompetenzen: Knowledge production and truth claims in post-colonial post-communist context. Albert Doja. Preview abstract. xml PDF / EPUB. Free access Research article First published December 30, 2021 pp. 119-139.
Web 2.0 tools such as blogs, wikis, social networking, podcasting etc. have received concentration in educational research over the last decade. Blogs enable students to reflect their learning experiences, disseminate ideas, and participate in analytical thinking. The Community of Inquiry (CoI) framework has been widely utilized in educational research to understand and enhance online and ...
China has produced a huge number of top A.I. engineers in recent years. New research shows that, by some measures, it has already eclipsed the United States. By Paul Mozur and Cade Metz Paul Mozur ...
Horne's research has shown that fasting twice a week for four weeks, then once a week for 22 weeks may increase a person's risk of dying after one year but decrease their 10-year risk of ...
The American publisher of a study that challenged scientific orthodoxy by claiming that an archaeological site in Indonesia may be the world's "oldest pyramid" says it has been retracted.