malayalam language Recently Published Documents
Total documents.
- Latest Documents
- Most Cited Documents
- Contributed Authors
- Related Sources
- Related Keywords
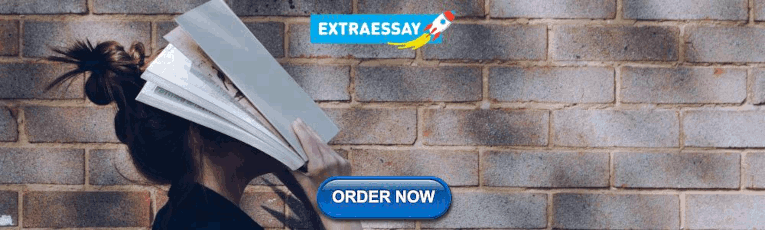
A Bottom-Up Approach applied to Dependency Parsing in Malayalam Language
Physico-chemical characterisation of uttamarasasindura: a valuable kupipakwarasayanafrom rasarajacintamani.
Ayurveda, literally means the science of life is a comprehensive system of health care of great antiquity, based on experiential knowledge and grown with perpetual additions. Rasashatra(Iatrochemistry)is an integrated part of Ayurveda which is based on Rasa (Mercury) and Rasadravyas(Mercury related substances). Rasarajacintamani is a compiled textbook of Rasashastra written in Malayalam language by Vadayattukotta K ParameshwaranPillai.Uttamarasasindura is explained in this textbook along with different types of Rasasinduras. The treatise Rasarajacintamani is reviewed for the specific formulation, Uttamarasasindura. Analytical study is needed to evaluate the proper formation and chemical composition of the medicine.Contexual reviewing revealed that Uttamarasasindura is a multidimensional remedy of clinical practice.
V. Sambasivan’s populist Othello for Kerala’s kathaprasangam
Through the verve and beauty of V. Sambasivan’s (1929–97) recitals for Kerala’s kathaprasangam temple art form, performed solo onstage to harmonium accompaniment, Shakespeare’s Othello has become a lasting part of cultural memory. The veteran storyteller’s energetic Malayalam-language Othello lingers in a YouTube recording, an hour-long musical narrative that sticks faithfully to the bones of Shakespeare’s tragedy while fleshing it out with colourful colloquial songs, verse, dialogue and commentary. Sambasivan consciously indigenized Shakespeare, lending local appeal through familiar stock characters and poetic metaphor. Othello’s ‘moonless night’ or ‘amavasi’ is made bright by Desdemona’s ‘full moon’ or ‘purnima’; Cassio’s lover Bianca is renamed Vasavadatta, after poet Kumaran Asan’s lovelorn courtesan-heroine. Crucially, Sambasivan’s populist introduction of Othello through kathaprasangam marks a progressive phase where Marxism, rather than colonialism, facilitated India’s assimilation of Shakespeare. As part of Kerala’s communist anti-caste movement and mass literacy drive, Sambasivan used the devotional art form to adapt secular world classics into Malayalam, presenting these before thousands of people at venues both sacred and secular. In this article, I interview his son Professor Vasanthakumar Sambasivan, who carries on the family kathaprasangam tradition, as he recalls how his father’s adaptation represents both an artistic and sociopolitical intervention, via Shakespeare.
Of Dictionaries and Dialectics: Locating the Vernacular and the Making of Modern Malayalam
This paper looks at Hermann Gundert’s Malayalam-English dictionary at the juncture of the modernisation of the Malayalam language in the 19th century. Gundert, the then inspector of schools in the Malabar district, saw the dictionary as the first step towards the cause of a universal education through the standardisation of Malayalam language. But what did a dictionary for all and by implication a language for all mean to the Kerala society? For centuries, much of the literary output in Kerala was in Sanskrit language, even as Malayalam continued its sway. The diversity of the language system in Kerala navigated its way through the hierarchies of caste and class tensions, springing up new genres from time to time within these dichotomies. Like many other vernacular languages in India, the Malayalam language system remained as the society it was in, decentralised and plural. This fell into sharp relief against the language systems of modern post-renaissance Europe with its standardised languages and uniform education. The colonial project in India aimed at reconstructing the existing language hierarchies by standardising the vernaculars and replacing Sanskrit as the language of cosmopolitan reach and cultural hegemony with English. Bilingualism and translation was key to this process as it seemed to provide a point of direct cultural linkage between the vernacular Indian cultures and Europe. This paper argues that Gundert’s bilingual dictionary features itself in this attempt at the modernisation of Malayalam by reconstructing the existing hierarchies of Kerala culture through the standardisation of Malayalam and the replacement of Sanskrit with a new cosmopolitan language and cultural values.
A review of the short story collection of Neelamalai
Review of the collection of short stories 'Neelamalai' written by Malayalam writer Urubu One of the notable writers in the world of Malayalam literature, PC Kuttikrishnan, also known as uhd Urubu, wrote a collection of short stories called 'Neelamalai' by. Kuttikrishna Menon is the whole point of Asha php. ‘Urubu’ is his nickname. Eva was born on June 8, 1915 in Ponnani, Kozhikode district. Evangel, Short Story, Drama, Poetry, as has given reassurance to all departments. MP Milk Award. Government of Kerala Award for Best Screenplay. Sahitya Akademi Award. The teacher sometimes lived in the Nilgiris and Wayanad hills, Short story Ava has said. There are a total of 6 short stories in this collection. The titles are set in relation to the central theme of the story. As the stories are centered on the Nilgiris and Wayanad, the technique of setting the beginning of the story with descriptions of places is used in all the stories. The characters in these stories are mostly hill people. Mountain races like Thotawa, Vaduga are shown. On a small scale vulgaris, gpw are shown as human beings. The hill tribesmen who come in male roles are hard workers. He has a hard working body and an owl mind that does not know the outside world. The women who come as female characters are innocent who do not know the outside world. So that he is deceived by others and, cannot break the rules. The characters, who are vulgar and, civilized, plunder the labor and life of the hill people. Pure Malayalam language is used in the stories. The vernacular is also used in the conversation of the hill tribes. vadugha language. Since the stories become the author's experience, it seems to be the best strategy to have the stories set by the teacher. The story is set in the Nilgiris, Wayanad hills, their biographical background, language, culture, customs, etc. The technique used in the stories is good. Having the meaning of the words in their own language helps the reader to understand the story.
Natural language inference for Malayalam language using language agnostic sentence representation
Natural language inference (NLI) is an essential subtask in many natural language processing applications. It is a directional relationship from premise to hypothesis. A pair of texts is defined as entailed if a text infers its meaning from the other text. The NLI is also known as textual entailment recognition, and it recognizes entailed and contradictory sentences in various NLP systems like Question Answering, Summarization and Information retrieval systems. This paper describes the NLI problem attempted for a low resource Indian language Malayalam, the regional language of Kerala. More than 30 million people speak this language. The paper is about the Malayalam NLI dataset, named MaNLI dataset, and its application of NLI in Malayalam language using different models, namely Doc2Vec (paragraph vector), fastText, BERT (Bidirectional Encoder Representation from Transformers), and LASER (Language Agnostic Sentence Representation). Our work attempts NLI in two ways, as binary classification and as multiclass classification. For both the classifications, LASER outperformed the other techniques. For multiclass classification, NLI using LASER based sentence embedding technique outperformed the other techniques by a significant margin of 12% accuracy. There was also an accuracy improvement of 9% for LASER based NLI system for binary classification over the other techniques.
Panchayat Jagratha Samithi role on Violence against Women and Children in Kerala
The Indian state Kerala is renowned for its overall development in various indexes such as human development, equality and education. However, in terms of combating violence against women and girls, state policies do not fulfil their objectives. The total number of crimes against women in Kerala during 2007 was 9381, by the end of 2019, it had risen to 13925, and by October 2020, it was 10124. To prevent all forms of crimes against women, the state established a vigilant committee in 1997 under Kerala Women’s Commission’s supervision. This vigilant committee is known as Jagratha Samithi (in the Malayalam language) and works in every Local Self Government (Panchayat). Therefore, the object of this research is the Jagratha Samithi (JS). The study aims to identify the JS’s role and activities in a panchayat to prevent violence against women and children. The methodology of the research is based on a qualitative study with primary data collected from 40 elected female and male representatives from 35 panchayats from one district in Kerala. The study shows that Jagratha Samithi in a panchayat has a significant role in addressing crimes against women and girls. However, there is a lack of sufficient support from society on its mission.
Pre-trained Word Embeddings for Malayalam Language: A Review
A framework for generating extractive summary from multiple malayalam documents.
Automatic extractive text summarization retrieves a subset of data that represents most notable sentences in the entire document. In the era of digital explosion, which is mostly unstructured textual data, there is a demand for users to understand the huge amount of text in a short time; this demands the need for an automatic text summarizer. From summaries, the users get the idea of the entire content of the document and can decide whether to read the entire document or not. This work mainly focuses on generating a summary from multiple news documents. In this case, the summary helps to reduce the redundant news from the different newspapers. A multi-document summary is more challenging than a single-document summary since it has to solve the problem of overlapping information among sentences from different documents. Extractive text summarization yields the sensitive part of the document by neglecting the irrelevant and redundant sentences. In this paper, we propose a framework for extracting a summary from multiple documents in the Malayalam Language. Also, since the multi-document summarization data set is sparse, methods based on deep learning are difficult to apply. The proposed work discusses the performance of existing standard algorithms in multi-document summarization of the Malayalam Language. We propose a sentence extraction algorithm that selects the top ranked sentences with maximum diversity. The system is found to perform well in terms of precision, recall, and F-measure on multiple input documents.
Siamese Networks for Inference in Malayalam Language Texts
Export citation format, share document.
- Benjamin Bailey Foundation
- Malayalam Research Journal
Malayalam Research Journal is a peer-reviewed, print, bi-lingual non-profitable journal
Benjamin bailey was a remarkable man in the cultural history of kerala., welcome to benjamin bailey foundation.
The Rev. Benjamin Bailey came to Kerala, India from Yorkshire, England at the age of 25 years as a member of Church Missionary Society. He was the founder of CMS station at Kottayam. He lived and worked 34 years in Kerala. Kerala was the second home for him. The multi-faceted activities of Bailey became substantive and incomparable contributions to the cultural history of Kerala. So Bailey was not a mere missionary for Kerala; he was more than that for us; for our language, literature and culture; for the place Kottayam and for our state Kerala. Benjamin Bailey was the first Principal of the first College in India-the ‘Kottayam College’ of CMS, i.e., the CMS College, Kottayam. He was the progenitor of printing and book publishing in Malayalam. He established the first printing office in Kerala in the year of 1821. Read More
Malayalam Research Journal (UGC-CARE Serial No. 41569)
Malayalam Research Journal is published by Benjamin Bailey Foundation since 2008. This is an international bi-lingual Journal dedicated to language, literature and culture. Its International Standard Subscription Number is ISSN-0974-1984. The Journal has been registered with the News paper Registrar of India and the registration number is KERBIL/2008/24527. Articles both in Malayalam and English are published. Three issues are published each year in January-April, May-August and September-December. Certain issues are theme-based and others are miscellany issues. Read More
- Photo Gallery
- Malayalam Research Journal Volume: 12, Issue : 1 January - April, 2019 Read contents
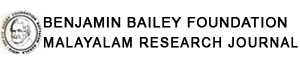
- Malayalam Research Journal
Malayalam Research Journal is published by Benjamin Bailey Foundation since 2008. This is an international bi-lingual Journal dedicated to language, literature and culture. Its International Standard Subscription Number is ISSN-0974-1984. The Journal has been registered with the News paper Registrar of India and the registration number is KERBIL/2008/24527. Articles both in Malayalam and English are published. Three issues are published each year in January-April, May-August and September-December. Certain issues are theme-based and others are miscellany issues. All Universities in Kerala have accorded recognition to Malayalam Research Journal as ‘Referred Journal’.
Malayalam Research Journal welcomes full length research articles, short research notes, review articles, book reviews, thesis reviews (reports), texts having historical importance and essays of a more general nature on the concerned areas of the Journal.
All submissions undergo editorial and peer review. Malayalam Research Journal uses ‘blind review’. Contributors shall not submit the same manuscripts for concurrent consideration of other publications. It may take one to two years to publish an article in the Journal. Authors are expected to revise the manuscripts according to the suggestions, if any, of the editors or reviewers. The Journal is not responsible for the opinions expressed in the articles. Copyright of the published articles is automatically reserved with Malayalam Research Journal. However, authors are permitted to reproduce their own articles for personal use without obtaining permission from the Journal. Others who wish to reproduce articles in Malayalam Research Journal must obtain written permission from the Journal.
Subscription Rates
- Information on journal
- Management Committe
- Editorial Board
- Advisory Board
- Guidelines for contributors
- Guidelines for reviewers
- Subscription
- Photo Gallery
- Benjamin Bailey Foundation
Terms and conditions
Ei aliquip regione.
Lorem ipsum dolor sit amet, nibh omnium in eum, ne per omittam eligendi efficiantur. Eos at mundi dolorem, ad cum omnes utroque fastidii, est fastidii apeirian ea. Ne duo diceret partiendo voluptatum, vel at iudico civibus. Purto erant aliquando ex eos, at vel odio modo. In mel tollit reprehendunt, ut usu praesent posidonium cotidieque. Clita assentior maiestatis sea in, at electram voluptaria mel. Tale nusquam adipisci ad mel, partem civibus no vix, sea no accusata dignissim.
Altera vocibus eleifend
No dico agam error qui, adhuc dicat argumentum sit in. Munere virtute ea ius. Mei an graeco repudiandae disputationi, ex per animal invidunt, probo civibus ne duo. Mea ad officiis temporibus, vim ne idque probatus phaedrum, elit delectus indoctum te has. No sea reprimique necessitatibus, ut usu quas falli.
Automatic Text Classification for Web-Based Malayalam Documents
- Conference paper
- First Online: 05 April 2023
- Cite this conference paper
- Jisha P. Jayan 7 &
- V. Govindaru 7
Part of the book series: Smart Innovation, Systems and Technologies ((SIST,volume 333))
Included in the following conference series:
- International Symposium on Intelligent Informatics
164 Accesses
Text documents are important sources of information that offer insights to administrators, planners, and researchers on what the public likes and does not. They are available in the form of plan documents, government reports, journal write-ups on sports, politics, science, and technology, etc., at web portals. Since its quantity is huge, searching for them on the web is time-consuming. This calls for a real-time tool that would be quicker and effective with categorizations. Comparisons with legacy documents that provide public opinions are constant or changing over a period of time. This study examines existing processes involved in article categorization and puts forth new algorithmic techniques for the automatic classification of Malayalam web-based articles. The performance of new classifiers is evaluated by using precision, recall, accuracy, and F1 score values.
This is a preview of subscription content, log in via an institution to check access.
Access this chapter
- Available as PDF
- Read on any device
- Instant download
- Own it forever
- Available as EPUB and PDF
- Compact, lightweight edition
- Dispatched in 3 to 5 business days
- Free shipping worldwide - see info
- Durable hardcover edition
Tax calculation will be finalised at checkout
Purchases are for personal use only
Institutional subscriptions
K. Kowsari, K.J. Meimandi, M. Heidarysafa, S. Mendu, L. Barnes, D. Brown, Text classification algorithms: a survey. Information 10 (4), 150 (2019). Multidisciplinary Digital Publishing Institute
Google Scholar
G. Mani, Customized news filtering and summarization system based on personal interest. Proc. Eng. 38 , 2214–2221 (2012). Elsevier
N. Chen, D. Blostein, A survey of document image classification: problem statement, classifier architecture and performance evaluation. Int. J. Doc. Anal. Recognit. (IJDAR) 10 (1), 1–16 (2007). Springer. https://doi.org/10.1007/s10032-006-0020-2
V. Gupta, G.S. Lehal, A survey of text mining techniques and applications. J. Emerg. Technol. Web Intell. 1 (1), 60–76 (2009). http://www.jetwi.us/uploadfile/2014/1230/20141230112729939.pdf
Z. Chase, N. Genain, O. Karniol-Tambour. Learning multi-label topic classification of news articles. (2014)
S. Kumar, S. Goel, Enhancing text classification by stochastic optimization method and support vector machine. Int. J. Comput. Sci. Inf. Technol. 6 (4), 3742–3745 (2015). Citeseer
S. Kaur, N.K. Khiva, Online news classification using deep learning technique. Int. Res. J. Eng. Technol. (IRJET) 3 (10), 558–563 (2016)
C.-W. Tsai, X. Chen, Real-Time News Classifier
J.E. Sembodo, E.B. Setiawan, M.A. Bijaksana, Automatic tweet classification based on news category in Indonesian language, in 2018 6th International Conference on Information and Communication Technology (ICoICT) (IEEE, 2018), pp. 389–393
O. Fuks, Classification of News Dataset . (Standford University, 2018). http://cs229.stanford.edu/proj2018/report/183.pdf
A. Patro, M. Patel, R. Shukla, J. Save, Real time news classification using machine learning. Int. J. Adv. Sci. Technol. 29 (9), 620–630 (2020). http://sersc.org/journals/index.php/IJAST/article/view/13254
S. Diab, Optimizing stochastic gradient descent in text classification based on fine-tuning hyper-parameters approach. A case study on automatic classification of global terrorist attacks. arXiv preprint arXiv:1902.06542 (2019)
K. Raghuveer, K.N. Murthy, Text categorization in Indian languages using machine learning approaches, in IICAI (2007), pp. 1864–1883. http://www.languagetechnologies.uohyd.ac.in/knm-publications/text-cat-iicai-2007.pdf
G. Kaur, K. Bajaj, News classification and its techniques: a review. IOSR J. Comput. Eng. (IOSR-JCE) 18 (1), 22–26 (2016)
J. Sreedevi, M.R. Bai, C. Reddy, Newspaper article classification using machine learning techniques. Int. J. Innov. Technol. Explor. Eng. 2278–3075 (2020). https://www.ijitee.org/wp-content/uploads/papers/v9i5/E2753039520.pdf
F. Kabir, S. Siddique, M.R.A. Kotwal, M.N. Huda, Bangla text document categorization using stochastic gradient descent (SGD) classifier, in 2015 International Conference on Cognitive Computing and Information Processing (CCIP) (IEEE, 2015), pp. 1–4. https://doi.org/10.1109/CCIP.2015.7100687
K. Nibeesh, C. Sreejith, P.C. Reghu Raj, Malayalam text classification for efficient news filtering using support vector machine
H. Schütze, C.D. Manning, P. Raghavan, Introduction to Information Retrieval , vol. 39 (Cambridge University Press, Cambridge, 2008)
MATH Google Scholar
J. Chen, H. Huang, S. Tian, Y. Qu, Feature selection for text classification with Naïve Bayes. Exp. Syst. Appl. 36 (3), 5432–5435 (2009). Elsevier
Y. Wang, Z.-O. Wang, A fast KNN algorithm for text categorization, in 2007 international conference on machine learning and cybernetics , vol. 6 (IEEE, 2007), pp. 3436–3441. https://doi.org/10.1109/ICMLC.2007.4370742
W. Zhang, T. Yoshida, X. Tang, Text classification based on multi-word with support vector machine. Knowl. Based Syst. 21 (8), 879–886 (2008). https://doi.org/10.1016/j.knosys.2008.03.044 ; https://www.sciencedirect.com/science/article/pii/S0950705108000968
S.R. Gunn, Support vector machines for classification and regression. Technical Report, School of Electronics and Computer Science, University of Southampton.
J. Han, J. Pei, M. Kamber, Data Mining: Concepts and Techniques (Elsevier, 2011)
L. Pradhan, N.A. Taneja, C. Dixit, M. Suhag, Comparison of text classifiers on news articles. Int. Res. J. Eng. Technol. 4 (3), 2513–2517 (2017). https://www.irjet.net/archives/V4/i3/IRJET-V4I3651.pdf
W. Li, J. Han, J. Pei, CMAR: accurate and efficient classification based on multiple class-association rules, in Proceedings 2001 IEEE International Conference on Data Mining (IEEE, 2001), pp. 369–376. https://doi.org/10.1109/ICDM.2001.989541
C. Jiang, F. Coenen, R. Sanderson, M. Zito, Text classification using graph mining-based feature extraction, in Research and Development in Intelligent Systems , vol. XXVI (Springer, London, 2010), pp. 21–34
D. Huynh, D. Tran, W. Ma, D. Sharma, A new term ranking method based on relation extraction and graph model for text classification, in Proceedings of the Thirty-Fourth Australasian Computer Science Conference , vol. 113 (2011), pp. 145–152. https://crpit.scem.westernsydney.edu.au/confpapers/CRPITV113Huynh.pdf
G.P. Zhang, Neural networks for classification: a survey. IEEE Trans. Syst. Man Cybern. Part C (Appl. Rev.) 30 (4), 451–462 (2000). https://doi.org/10.1109/5326.897072
R.R. Kumar, M. Babu Reddy, P. Praveen, Text classification performance analysis on machine learning. Int. J. Adv. Sci. Technol. 28 (20), 691–697 (2019)
E. Frank, R.R. Bouckaert, Naive Bayes for text classification with unbalanced classes, in European Conference on Principles of Data Mining and Knowledge Discovery (Springer, Berlin, Heidelberg, 2006), pp. 503–510
C.-C. Le, P. Prasad, A. Alsadoon, L. Pham, A. Elchouemi, Text classification: Naïve Bayes classifier with sentiment Lexicon. IAENG Int. J. Comput. Sci. 46 (2), 141–148 (2019). https://researchoutput.csu.edu.au/ws/portalfiles/portal/30550232/30550129_Published_article.pdf
C.H. Sawarkar, Dr. P.N. Mulkalwar, Exploring the use of machine learning for highly accurate text-based information retrieval system. Test Eng. Manag. 81 , 6592–6599 (2019). https://www.testmagzine.biz/index.php/testmagzine/article/view/935/846
G. Ke, Q. Meng, T. Finley, T. Wang, W. Chen, W. Ma, Q. Ye, T.-Y. Liu, Lightgbm: a highly efficient gradient boosting decision tree. Adv. Neural Inf. Process. Syst. 30 (2017). https://doi.org/10.5555/3294996.3295074
T. Zhang, Solving large scale linear prediction problems using stochastic gradient descent algorithms, in Proceedings of the Twenty-First International Conference on Machine Learning (2004), p. 116. https://doi.org/10.1145/1015330.1015332
S. Deb, Naive Bayes vs Logistic Regression (2016). https://medium.com/@sangha_deb/naive-bayes-vs-logistic-regression-a319b07a5d4c
C. Ottesen, Comparison Between Naive Bayes and Logistic Regression (2017). https://dataespresso.com/en/2017/10/24/comparison-between-naive-bayes-and-logistic-regression/
J. Li, G. Huang, C. Fan, Z. Sun, H. Zhu, Key word extraction for short text via word2vec, doc2vec, and textrank. Turk. J. Electr. Eng. Comput. Sci. 27 (3), 1794–1805 (2019). https://journals.tubitak.gov.tr/elektrik/issues/elk-19-27-3/elk-27-3-17-1806-38.pdf
E. Cihan Ates, E. Bostanci, M.S. Guzel, Comparative performance of machine learning algorithms in cyberbullying detection: using Turkish language preprocessing techniques. arXiv e-prints arXiv-2101 (2021)
V. Gupta, V. Gupta, Algorithm for Punjabi text classification. Int. J. Comput. Appl. 37 (11), 30–35 (2012). Citeseer. https://citeseerx.ist.psu.edu/viewdoc/download? doi=10.1.1.681.3035 &rep=rep1 &type=pdf
K. Lee, D. Palsetia, R. Narayanan, Md.M.A. Patwary, A. Agrawal, A. Choudhary, Twitter trending topic classification, in 2011 IEEE 11th International Conference on Data Mining Workshops (IEEE, 2011), pp. 251–258. https://doi.org/10.1109/ICDMW.2011.171
P. Bolaj, S. Govilkar, Text classification for Marathi documents using supervised learning methods. Int. J. Comput. Appl. 155 (8), 6–10 (2016). https://www.ijcaonline.org/archives/volume155/number8/bolaj-2016-ijca-912374.pdf
Download references
Acknowledgement
We acknowledge Consortium for Developing Dependency TreeBanks for Indian Languages and its leader IIIT-Hyderabad, especially Prof. Dipti Misra Sharma and Department of Electronics & Information Technology ( DeitY ), Government of India.
Author information
Authors and affiliations.
Language Computing Department, Centre for Development of Imaging Technology, Thiruvanathapuram, India
Jisha P. Jayan & V. Govindaru
You can also search for this author in PubMed Google Scholar
Corresponding author
Correspondence to Jisha P. Jayan .
Editor information
Editors and affiliations.
School of Computer Science & Engineering (SoCSE), Innovation and Technology (KUDSIT), Kerala University of Digital Sciences, Trivandrum, Kerala, India
Sabu M. Thampi
Dept of Computer Science & Engineering, Indian Inst of Technology Kharagpur, Kharagpur, India
Jayanta Mukhopadhyay
PAN, Systems Research Institute, Warszawa, Poland
Marcin Paprzycki
Department of Computer Science and Information Engineering (CSIE), Providence University, Taichung, Taiwan
Kuan-Ching Li
Rights and permissions
Reprints and permissions
Copyright information
© 2023 The Author(s), under exclusive license to Springer Nature Singapore Pte Ltd.
About this paper
Cite this paper.
Jayan, J.P., Govindaru, V. (2023). Automatic Text Classification for Web-Based Malayalam Documents. In: Thampi, S.M., Mukhopadhyay, J., Paprzycki, M., Li, KC. (eds) International Symposium on Intelligent Informatics. ISI 2022. Smart Innovation, Systems and Technologies, vol 333. Springer, Singapore. https://doi.org/10.1007/978-981-19-8094-7_14
Download citation
DOI : https://doi.org/10.1007/978-981-19-8094-7_14
Published : 05 April 2023
Publisher Name : Springer, Singapore
Print ISBN : 978-981-19-8093-0
Online ISBN : 978-981-19-8094-7
eBook Packages : Engineering Engineering (R0)
Share this paper
Anyone you share the following link with will be able to read this content:
Sorry, a shareable link is not currently available for this article.
Provided by the Springer Nature SharedIt content-sharing initiative
- Publish with us
Policies and ethics
- Find a journal
- Track your research
0494 2631230
ANNOUNCEMENTS
- PG Admission 2024- Application Invited
- Re-QuotationNotice – 10 KVA U.P.S – AMC
- Quotation Notice – Portable Biogas plant
- Dhargas – Bus on contact basis
- Tender – Furniture
- Call for Papers- Sociology Research Journal Sameeksha-Vol.7
- Re-Quotation Notice – Air Conditioner repairing work
- Tender Notice – Air Conditioner
- Final Electoral Roll
- Notification of Election – Student Representative to Nirvahakasamithi
- Executive Committee Election – Draft Voters List published
- Project Assistant -Walk in Interview
Research Scholars-Literature Studies
Ph.d research scholars, neethu.n (ph.d-2016).
Email: [email protected]
Topic: Women centred discource analysis; A study on periodicals of 19th centuary
Research Guide: Dr. C Ganesh
Academic Achievements
- Got Nakshthra scholarship in P.G 2014.
- Paper presented at National seminar in 2016 June 10 in Malayalam University (Titled THINA and ECOLOGY)
- Presented paper on Researchmethodology in the National seminar at Malayalam University, 2016 october 28.
Sruthi.T (Ph.D-2014)
Email: [email protected]
Topic: Modernism in malayalm criticism
Research Guide: Dr. T Anithakumri
- Presented paper in National seminar at catholicate college, Pathanamthitta on New Malayalam Poetry in 2013 August.
- Presented paper in National seminar at Malayalam University on the topic Cyber Space in Malayalam Short Story (2016 october 28)
- Published an article in the book named as Prakashavarshangal edited by Dr. Jitheesh (2017)
- Got Nakshathra scholarship, 2014.
- Got Kuttettan Literay Award, 2014.
- Got Gireesh Puthenjery poetry Award, 2016.
Archana Mohan (Ph.D-2016)
Email: [email protected]
Topic: Femine Uses in Malayalam Fictions: A Study in the light of selected short stories
Research Guide: Dr. T Anithakumari
- Paper presented in the National seminar at Malayalam University, 18.03.2016.
- Paper presented in the National seminar at Malayalam University, 14.07.2016.
Jishila.K (Ph.D-2016)
Email: [email protected]
Topic: Modern Poem and Other Arts: A Study based on selected poems
Research Guide: Dr. E Radhakrishnan
- Presented paper in National seminar at Malayalam Univer sity on the topic Universities and Literary Researches.
Athira.C.C (Ph.D-2016)
Email: [email protected]
Topic: Cultural Rsistence in Venmani Poems: A Critical Study.
Research Guide: Dr. Anwar A
- Presented paper titled as Ancient and Medieval Champus: Re-thinking and Analysis in National seminar at S.S.U.S Payyannur centre.
- Got Nakshthra Scholarship 2014-15
Dheena.PP (Ph.D-2016)
Email: [email protected]
Topic: Eco criticism and eco language system: A Study based on selected malayalam novels
Research Guide: Dr. N.V Muhammed Rafi
Resmi TN (Ph.D-2017)
Topic: Literature and history of literature: A Study on selected texts on history of literature
- Presented a paper titled as Feministic Linguistic Approach in Sarah Joseph’s novels conducted by Dept. of Linguistics, Malayalam University, 2017 March 1.
Aiswarya V Gopal (Ph.D-2016)
Email: [email protected]
Topic: Identity Crisis in Pathmarajan’s Shortstories: An Inquiry in to the Narratology of his selected works
Research Guide: Dr. NV Muhammed Rafi
M.Phil Research scholars
Devi.n (m.phil-2016).
Email: [email protected]
Topic: Narratology in the works of Sumamgala
Guide: Dr. T Anithakumari
- Presented a paper in National Seminar at Sri Krishna College, Guruvayoor on the Novel Khasakkinte Ithihasam (2017, March 13)
Susmitha.KS (M.Phil-2016)
Email: [email protected]
Topic: Historiography in Works of N.S Madhavan: A Study based on selected short stories
Guide: Dr. Roshni Swapna
- Presented a paper in National Seminar at Sri Krishna College, Guruvayoor on the topic: Stardome in Modern Malayalam Drama (2017, March 13)
Reshma.KM (M.Phil-2016)
Email: [email protected]
Topic: A Study on short stories of T.K.C Vaduthala and C Ayyappan
Sharmiya Noorudheen (M.Phil-2016)
Email: [email protected]
Topic: Caricature in Payyan stories: A Critical Study
Guide: Dr. E Radhakrishnan
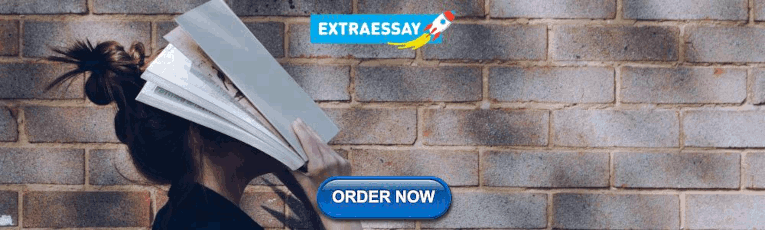
Academic Achievements:
- Presented a paper titled as -The Power in Perumpara,the drama of P.M Taj: A Critical Study- in National Seminar Sri Krishna College, Guruvayoor (2017, March 13)
Dhyana.V (M.Phil-2016)
Email: [email protected]
Topic: Contextual Analysis of epics based on queer theory Special reference to Sree Mahabharatham Kilippattu
Guide: Dr. N.V Muhammed Rafi
- Presented a paper titled as -Post modern Theatre- in National Seminar Sri Krishna College, Guruvayoor (2017, March 13)
- Presented a paper in National Seminar in Malayalam University conducted by Dept.of Literary Studies on Translation in 2016
- Presented a paper titled as - Popular Cinema and Politics of Language- in National Seminar in Malayalam University
- Topscorer in Post Graduation, Dept. of Literary Studies, Malayalam University
- Open access
- Published: 26 April 2022
Social media text analytics of Malayalam–English code-mixed using deep learning
- S. Thara ORCID: orcid.org/0000-0003-3202-7083 1 &
- Prabaharan Poornachandran 1
Journal of Big Data volume 9 , Article number: 45 ( 2022 ) Cite this article
4637 Accesses
15 Citations
1 Altmetric
Metrics details
Zigzag conversational patterns of contents in social media are often perceived as noisy or informal text. Unrestricted usage of vocabulary in social media communications complicates the processing of code-mixed text. This paper accentuates two major aspects of code mixed text: Offensive Language Identification and Sentiment Analysis for Malayalam–English code-mixed data set. The proffered framework addresses 3 key points apropos these tasks—dependencies among features created by embedding methods (Word2Vec and FastText), comparative analysis of deep learning algorithms (uni-/bi-directional models, hybrid models, and transformer approaches), relevance of selective translation and transliteration and hyper-parameter optimization—which ensued in F1-Scores (model’s accuracy) of 0.76 for Forum for Information Retrieval Evaluation (FIRE) 2020 and 0.99 for European Chapter of the Association for Computational Linguistics (EACL) 2021 data sets. A detailed error analysis was also done to give meaningful insights. The submitted strategy turned in the best results among the benchmarked models dealing with Malayalam–English code-mixed messages and it serves as an important step towards societal good.
Introduction
Social networking has been proliferating worldwide, over the last decade, concurrent with remarkable advancements in communication technologies. Research in Indian languages has evinced keen interest [ 1 ]. In India, social media enthusiasts originate from regions of diverse languages and multi-cultural backgrounds [ 2 ]. Indian civilization was endowed with an enriched linguistic heritage. Britain’s 200-year colonization enabled India to become the second largest English-speaking population. Footnote 1 Malayalam, a Dravidian Footnote 2 language mainly spoken in the southern parts of India, is the official language of the Union Territories—Lakshadweep and Pondicherry, and the state Kerala, India. A deeply agglutinative language, the global Malayalam-speaking population is nearly 38 million [ 3 ]. The alphabets in Malayalam are constituted of the Vatteluttu Footnote 3 alpha-syllabic scripts that belong to a family of the Abugida Footnote 4 writing system. Social media enthusiasts often adopt the Roman script as language scripts due to its ease of input. Hence, majority of the data content in social media, available for under-resourced languages, is code-mixed [ 4 ].
The prime objective of this research has been to identify sentiment polarity and repulsive content in Malayalam–English code-mixed blogs in social media. This study highlights the latent interspersed offensive language and sentiment polarity content in the Dravidian languages, on social media. Also, the research is evaluated by various techniques and outperforms well with the published results [ 5 ]. Sentiment analysis (SA) has been an active area of research since the advent of the current millennium. Code-mixed texts in social media have spurred the demand for SA [ 6 , 7 , 8 ]. SA entails the identification of subjective opinions or responses on a given topic, product or service in e-commerce. Progressive evolutions in communications and networking technologies have motivated consumers to share their personal opinions and critiques of retail products and services in real time.
The ensuing fallout of aggressive, harmful posts on social websites is undeniable. Nowadays, people openly voice their disdain towards a government policy or specific individuals, by posting abusive critical bulletins. The deluge of derisive fictitious messages must be detected and suppressed in any communicative forum. Such falsified posts profusely hurt people’s sentiments, causing mental trauma, and distress [ 9 , 10 , 11 ]. Unrestrained proclivity towards dissemination of fake news and derogatory contents calls for their automatic detection and proscription from media platforms [ 12 ]. To some extent, denigratory communications have been forbidden in the English language. Nevertheless, prevention of resentful Indian language blogs, in the code-mixed domain, is in early infancy stages [ 13 ].
The exigency of this problem is critical in societal domains such as health care, politics, e-retail, and movie review. In the prevailing digital era, people prefer the social media for news updates, and interaction with friends. Media blogs are replete with natural language content. Governmental agencies [ 14 ] have enacted strict laws to deal with proliferation of hateful text through social media and mobile apps. Misinformation on the prevention and cure of the COVID-19 [ 15 ] pandemic have serious repercussions on public health, leading to avoidable mental trauma, and distress [ 16 ]. Besides, as major business, entertainment, and political activities have been confined to online settings, the deceptive corpus is wildly rampant. Politicians exchange views on latest partisan developments, inviting the citizens to comment, share ideas apropos their political agenda. Public penchant for online entertainment and e-commerce has transformed retail business to movies online and recurrent impulsive shopping. Principal schemes for SA and offensive language identification (OLI) emanated from the computational linguistics domain [ 17 , 18 ], exploiting the syntactic structure and pragmatic features of code-mixed semantics. Machine learning [ 19 ] flourished via the n-gram (sequence of written symbols of length n , where n can be 1, 2 or 3) word and char features. Character n-gram models incorporate information about the internal structure of the word in terms of character n-gram embedding. Term frequency–Inverse document frequency (TF-IDF) [ 20 ] was applied as feature extraction method for the SA and OLI tasks; other measures have leaned on deep learning [ 21 , 22 ] and ensemble approaches [ 23 , 24 ].
A major research gap, pinpointing a limited data set [ 3 , 25 , 26 ] called for the creation of a code-mixed corpus due to the unavailability of an openly accessible gold standard data set. Inadequate multi-lingual code-mixed data for fine-tuning pretrained models is another challenge. These under-resourced morphologically rich languages (Malayalam, Tamil, Telugu, Kannada) lack pre-trained models to process low-resource languages. Few state-of-the-art (SOTA) models were adopted to address the research problem of identification of sentiment polarity and offensive content in Malayalam–English code-mixed which yielded F1-scores (measure of a model’s accuracy) of 0.76 and 0.99, respectively, for FIRE 2020 and EACL 2021 data sets of social media text analytics. These were the best results scored for both the data set [ 27 ]. The novelty lies in the selective translation and transliteration stages [ 28 ], concurrent with optimization of hyper-parameters (learning rate, epochs, optimizers etc.) and up-sampling strategy. Selective translation and transliteration are prime concepts wherein Romanized sentences are converted to their native language where the semantic meaning is preserved. A systematic comparison of deep learning models—Convolution Neural Networks (CNN), Long Short Term Memory (LSTM), Gated Recurrent Unit (GRU), Bidirectional LSTM (BiLSTM), and Bidirectional GRU (BiGRU) returned good results, as a testimony to the propriety of preprocessing of inputs to the proposed model. This research was undertaken for the societal good as unwarranted pejorative posts exacerbate traumatic impairment of a person’s mental health.
Challenges encountered in the run-up to both the tasks were:
short-length messages.
informal words like plz/pls—please; lvl-level.
abbreviations like cr—crore; fdfs—first day first show, bgm— back-groundmusic.
spelling variations like w8/wt – wait; wtng/w8ing/wting,—waiting; avg—average.
time stamps, various formats are used to mention time, like 3:02, 3 min 3, 3.03 min.
repetition of characters in words for example coooool, sooooooooperb/supeerb, fansss, maaaaaaaasss.
multiple ways to represent numbers for example 3.7k, 3700, one lac, one lakh, 2 Million, 2 M.
Key contributions of this paper
A fine-grained analysis of sentiment and offensive content identification, with several deep learning algorithms for the Malayalam–English code-mixed data set.
Highlight the propriety of selective translation and transliteration in code-mixed data set.
Achievement of benchmark results—overall 2% increase in the F1-score (measure of a model’s accuracy on a data set).
Extensive experimental studies with detailed error analysis which stimulate self-directed research.
This paper is organized as follows—section " Related works " presents an overview of the related works. Section " Proposed approach " describes the proposed approach which includes data description, data preprocessing, feature extraction methods, and deep learning approaches. Section " Experimental Setup " discusses the experimental setup for verifications of the proffered design, which include the best hyper-parameter configuration. Section " Results and discussions " discusses results and the inferences from this study. Section " Limitations " gives glimpses of limitations of proposed method. Section " Conclusion " concludes the paper with closing remarks. Section " Future work " draws attention to future research in this area.
Related works
Researchers have relied on various methods for the complex task of discernment of sentimental offensive language in the code-mixed domain. Initially researchers developed an engineering approach for SA [ 29 ], by leveraging a handful of metadata, lexical and sentiment features, to design a model. Several works [ 30 ] proposed a combination of Naive Bayes and SVM (NBSVM), for classification of code-mixed data sets. The preprocessing stages included tokenization, hashtag segmentation, URL removals, and lower-casing of sentences. F1-scores of 0.72, 0.65, 0.76 were obtained for negative, neutral, and positive classes, respectively. A unique Enhanced Language Representation with Informative Entities (ERNIE) model [ 31 ], was proposed by Liu et al. [ 32 ] and applied to code-mixed data sets of Hindi and English. An adversarial training was applied while training, along with XLM-RoBERTa model (XLM-R), for a multilingual model; it achieved F1-scores of 0.799, 0.769 and 0.689 for positive, negative, and neutral classes, respectively. Table 1 summarises few articles. It is divided into Data set, Methodology, Limitations and results.
Research in OLI [ 38 ] has been also facilitated by making the corpus available. Chakravarthi et al. [ 12 ] used TF-IDF vectors, along with character level n-grams, towards feature engineering process. Character n-gram models incorporate the internal structure of the word in terms of character n-gram embedding. The four main developed models were—LSTM, LR, XGBoost and attention networks. Traditional ML classifiers with these features produced good F1-score of 0.78, on par with deep learning models [ 39 ].
The background studies of SA and OLI in code-mixed corpora address the diverse paths ranging from feature engineering to task modeling. The relevance of this probe was attested by the linguistically diverse code-mixed corpora. Multi-lingual languages are semantically complex, bereft of sophisticated models in the Dravidian code-mixed domain, for the management of SA and OLD [ 27 , 40 ]. As shown in the above studies, no relevant probe was conducted with selective translation and transliteration incorporating hyper-parameter optimization. This paper undertook a pioneering attempt, for assessment of the viability of selective transliteration and translation preprocessed comments [ 41 ], factoring in class imbalances, together with hyper-parameter optimization which augmented the proffered approach’s weighted accuracy (F1-score). Hyper-parameter optimization was shown to be propitious for the final step of tag prediction. An extensive comparative study of several deep learning approaches was conducted; despite data scarcity for code-mixed corpus, the proposed approach attained the best score of 0.76 for the FIRE 2020 and 0.99 for the EACL 2021 data sets.
Proposed approach
This section covers a brief on the data set, and feature extraction methods—Word2Vec [ 42 ] and FastText [ 43 ]. Discussions of SOTA deep learning approaches follows next, inclusive of the requisite hyper-parameters. The section concludes with sentiment and offensive comments/posts prediction. Figure 1 unveils an overview of the proposed methodology, where the different stages are the bilingual code-mixed data set, requisite data preprocessing steps, feature engineering techniques (Word2Vec and FastText), optimized hyper-parameter values for selecting deep learning approaches for modeling and finally prediction.
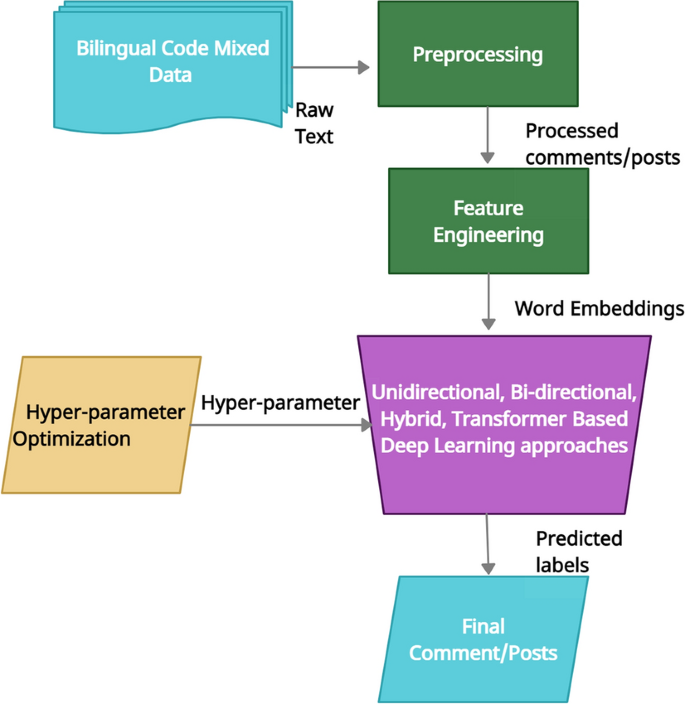
Flow diagram of the proposed work
Data set description
For the experimental study, the data sets for SA and OLI tasks were retrieved from organizers of the FIRE 2020 Footnote 5 and EACL 2021, Footnote 6 who conducted pioneering structured shared tasks in Malayalam–English code-mixed data sets. Hence, the corresponding data sets can be considered as the standard/benchmark data sets for their respective tasks. The comments/posts of the SA, OLI tasks contain more than one sentence, but the average length of sentences of the code-mixed data sets is 1 for both the tasks. Each comment/post is annotated with its corresponding class label. All the tasks can be considered as a fine-grained analysis, as the comments are scrutinized at a finer level.
The code-mixed data set for SA is classified into 5 classes: Positive, Negative, Unknown state, Mixed feelings, and not-Malayalam. The shared task for SA is constituted of 4851 code-mixed social media comments/posts in the training set, 540 comments in the validation data set and 1348 comments in the test data set. The OLD data set is classified into 5 categories: Not offensive (NF), Offensive Targeted Insult Individual (OTII), not Malayalam (NM), Offensive Targeted Insult Group (OTIG) and Offensive Untargetede (OUT). The shared task for offensive language detection (OLD) is comprised of 16,010 code-mixed social media comments/posts in the training set, 1999 comments/posts in the validation set and 2001 comments in the test data set. Since both the SA and OLD tasks involve a fine-grained approach, the availability of training data per class, is minimal. This gives rise to class imbalance problems (non-uniform distribution of classes in the data set), for the two code-mixed data sets, depicting real-world scenarios (Table 2 presents statistics for the SA stats, Table 3 for statistics of the OLD data set). Random up-sampling technique was used to address the class imbalance problems for both the tasks. Hence, minority classes were sampled repeatedly, so that all classes in each of the data sets have an equal number of samples. Percentage distribution of each class in all the three sets (training, validation and test) are shown separately.
Data set preprocessing
Sentimental and Offensive language code-mixed FIRE 2020 and EACL 2021 data sets are constituted of comments/posts from YouTube channel; spelling mistakes and commonly used internet jargon are widely observed within both the data sets. Preprocessing in Malayalam–English code-mixed data set is a challenging task; hence, both the data sets went through the sequence of step shown in Fig. 2 . The pseudo code for basic preprocessing is shown in Algorithm 1.
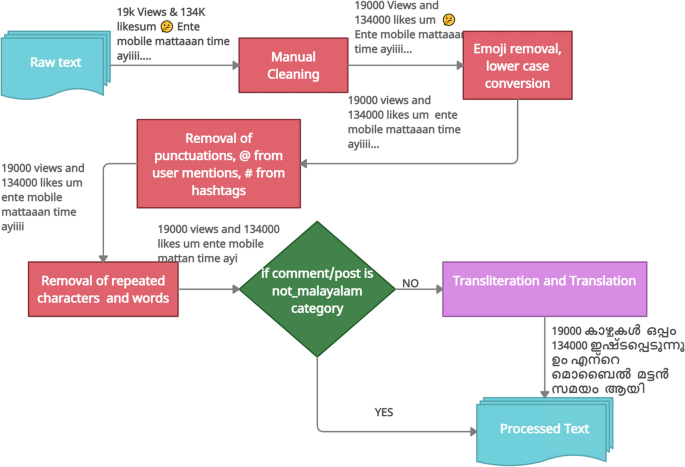
Various stages of preprocessing
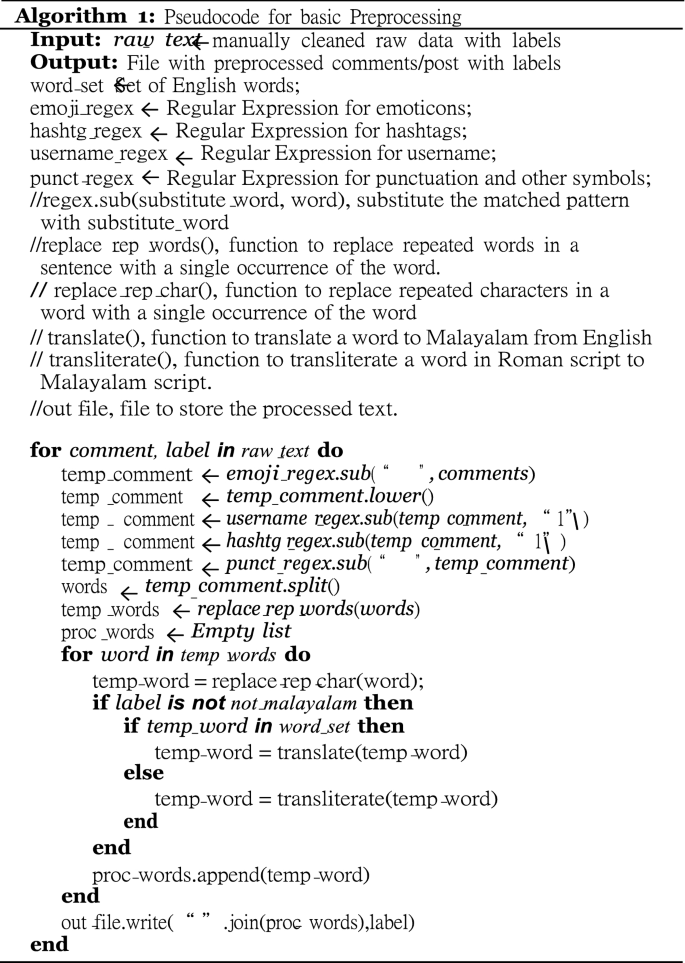
Selective translation and transliteration
To convert code-mixed text into a native script, we cannot rely on neural translation systems, particularly in tweets where users are prone to write informally, using multiple languages. Besides, translation of Romanized non-English language words into a particular language does not make any sense. In many cases, proper translation of words from English to a non-English language would not be available. We propose selective transliteration and translation of the text as a solution to this problem. In effect, the process of conversion of Romanized text (for example, Manglish) is to transliterate the words in the native Malayalam language in text into Malayalam and translate the English words in the text into Malayalam selectively. This separation of English words from native language words is carried out using a big corpus of English words from NLTK Footnote 7 corpus. The idea of this selective conversion is based on the observation that in the Romanized native language, users tend to use English words only when the word-meaning is better conveyed with the English word, or when the corresponding native language word is not commonly used in regular conversations. For example, Malayalam-users prefer the word “movie” over much its corresponding Malayalam word. During the basic phase, the raw text of the not-Malayalam category are left unchanged; otherwise, the comments/posts are transliterated and translated. The pseudo code for transliteration and translation is shown in Algorithm 2. Google API was used for translation. Footnote 8
Feature extraction method
Humans can intuitively deal with natural language or text data. A computer’s inability to handle such data call for the numerical representation of texts. A method is needed that can capture the syntactic and semantic relationships among words, along with a clear understanding of the contexts in which they are used in. Such methods are called Word embeddings [ 45 ], wherein each word is mapped to an N-dimensional real vector. One of the early methods to form vector representations of a word is called one-hot encoded vector, where a “1” is assigned for the index position of the word and “0” placed elsewhere. Word2Vec and FastText are other word embedding models discussed in the paper.
The Word2Vec embedding method marshals algorithmic schemes such as Continuous Bag of Words (CBOW) [ 42 ] and Skip-gram [ 42 ] for the derivation of real-valued vectors. These models create embeddings based on the co-occurrence of words; for e.g., ‘She walked by the riverbank, and went to the bank to deposit money’; if we take the word “bank” and the corpus contains more information about riverbanks rather than the financial institution, then the embeddings of the bank will be inclined towards river and stream, instead of finance, lender, etc. Another key limitation is that Word2Vec does not keep track of the position of a word in the sentence i.e., word ordering information is not preserved. Word2Vec ignores the internal structure of the word.
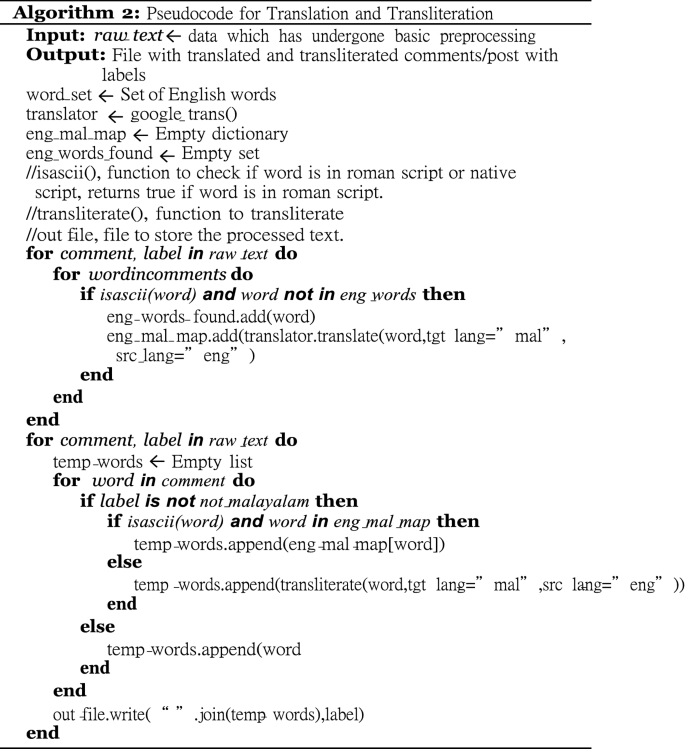
Words that belongs to morphologically rich languages are better handled by character level information (character n-grams). Each word is represented as a bag of character n-grams. We can resolve Out of Vocabulary (OOV) words using this method. FastText is an algorithm which follows character n-gram based model. For each word, the algorithm considers character n-grams for example unigram, bigrams, trigram, five grams etc. We can find shorter and longer n-grams. Shorter n-grams helps to identify the structure of a word; longer n-grams are good to capture its semantic information. Gensim Footnote 9 implementation of Word2Vec and FastText was used to custom train the word embedding model with the two code-mixed data sets. Word2Vec and FastText were trained separately on these two data sets. The learned embeddings were saved in a text file. The Python NumPy Footnote 10 package was used to convert the word embeddings in the text file to a matrix; this word embedding matrix was then used to initialize the weights of Keras Embedding Layer; Footnote 11 the Embedding Layer’s trainable attribute was set to false so that the word embeddings remain constant throughout the training phase.
Deep learning approaches
In this section the major focus is on the proposed deep learning models used for the prediction of sentiments and offensive content in the Malayalam–English code-mixed domain.
For this paper’s study, 1D-CNN was used, which is fed with spatially dropped word embeddings; 1-D CNN was used with a kernel size of 1, and 256 filters. The output from the 1-D CNN is down sampled, using 1-D global max pooling. The condensed representation after pooling, is made to pass through two feedforward neural networks, with a dropout layer in between, to avoid over-fitting. The final feed-forward layer acts as a classification layer. Illustrations in given in Fig. 3 a.
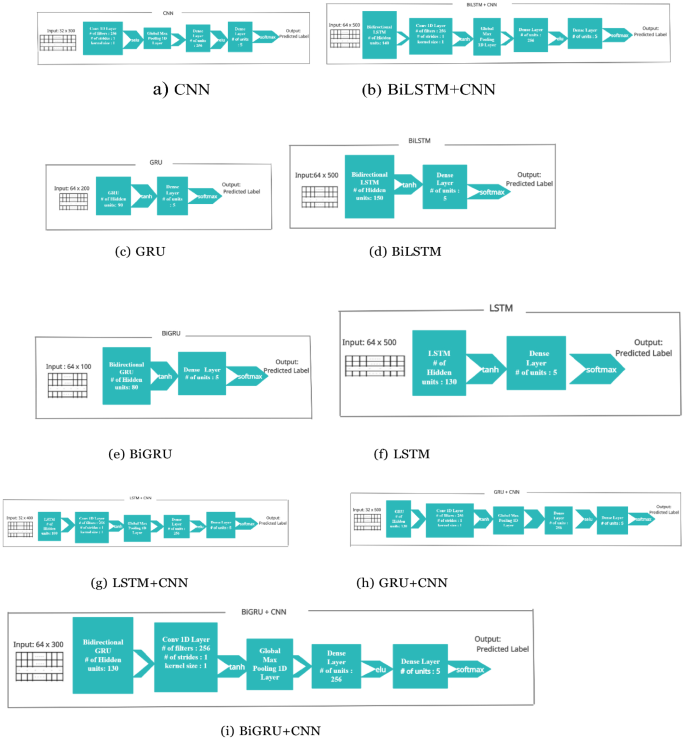
Layer configuration and parameter settings of various deep learning models used in the proposed approach
In this paper’s investigation, word embeddings were made to pass through a 1D Spatial Dropout layer, which is used to expand the dropout value over the entire feature map. After dropout, the word embeddings are then fed into the LSTM. We have considered the output from the last time step of the LSTM as encoded representation of the input string, followed by, passing the LSTM output through a dropout layer, and then, fed into a feed-forward neural network. The feed forward neural network acts as the classification layer. Pictorial representation is given in Fig. 3 f.
In order to reduce overfitting, the word embeddings are fed into the GRU, after a spatial dropout. The last hidden state of an input sentence is made to pass through a dropout layer, followed by the classification layer as shown in Fig. 3 c.
BiLSTM yields a more powerful and richer representation of the input sentence, compared to unidirectional LSTM. As shown in Fig. 3 d using a single LSTM after spatial dropout, the input sentence is fed to the LSTM, in both the forward and backward directions. The hidden states of the last time step, from both the directions of the input, are concatenated and fed into a dropout layer, which is followed by a feed forward layer that acts as the classification layer.
In Fig. 3 e the input sentence is made to pass through a spatial dropout layer, before being fed into the BiGRU where the GRU is fed with the input sentence in both the directions. After dropout, the hidden state from the last time step is conveyed to a classification layer.
BiLSTM + CNN and LSTM + CNN
BiLSTM + CNN/LSTM + CNN were inspired from the encoder-decoder architecture [ 46 ]. Designed to overcome the vanishing gradient problem [ 47 ]. Figure 3 b and g gives the pictorial view.
BiGRU + CNN and GRU + CNN
BiGRU + CNN and GRU + CNN were also inspired from the encoder–decoder architecture. GRU and BiGRU act as encoders and the 1-D CNN as the decoder as shown in Fig. 3 h and i.
XLM-Roberta (XLM-R)
XLM-R is a transformer-based, multilingual, masked language model [ 48 ], pre-trained on text in 100 languages. It delivers SOTA performance on cross-lingual classification, sequence labeling and answering questions. XLM-R base was used for classification tasks, Footnote 12 in this study.
Experimental setup
This section addresses different combinations of hyper-parameters used by deep learning approaches for empirical investigations. Key configuration elements of the experimental platform, to train the deep learning models, were as follows: Python 3.7.9 version, NVIDIA Graphical Processing Unit (GPU) driver version 460.32.03, Compute Unified Device Architecture (CUDA) 11.2 and Tesla K80 GPU with 12 GB memory.
Hyper-parameters for deep learning models
Design of a neural network architecture calls for tuning of diverse combinations of hyper-parameters. This ensues in optimization of the hyperparametric values, using the Grid-Search technique. The crux of any neural network is to define the right combination of parameters that yields high performance and low error rate. The following Table 4 convey the standard values of hyper-parameters, which were utilized and tuned for experimental evaluations:
Optimal hyper-parameter configuration
Iterative applications of the Grid-Search scheme enabled the identification of the optimal hyper-parameters that yielded SOTA weighted F1-scores, on the two code-mixed data sets. The finally selected hyper-parameters were the ones that exhibited best performance for the deep learning models. All the hyper-parameters were tuned on training and development data. On the sensible selection of optimal hyper-parameters, those parameters were exercised for evaluation on the test data. Table 5 displays a overview of the optimal hyper-parameter values for the best performing models.
Results and discussions
This section summarizes the results and analysis of simple deep learning models, bidirectional models, hybrid models, and transformers. Efficacy of the proposed system was evaluated in terms of the F1-score, Footnote 13 on a held-out test data, using the Sklearn Footnote 14 machine learning tool.
Table 6 evince the results of SOTA deep learning models for OLI in code-mixed Malayalam–English data set. The experimental evaluations show that the Recurrent Neural Network(RNN) variants, LSTM and GRU, performed better than CNNs. LSTM and GRU enhanced F1-score by marginal values of 1.15% and 1.50%, respectively, when compared with CNN, RNNs are outfitted to capture sequential information, unlike the CNNs that handle information of local context only. Bidirectional networks enable models to discern dependencies on either side of the code-mixed text, unlike unidirectional models that apprehend information only from the precedent input. Bi-directional layers can learn forward and backward features from an input sequence. Thus, bidirectional models such as BiGRU exceeded the F1-scores of unidirectional models (CNN, LSTM, GRU) by 1.55%, 0.68%, and 0.78%, respectively. Among the hybrid models, GRU + CNN and BiLSTM + CNN turned in the highest F1-score of 0.9969. Several other hybrid and bidirectional models shown in Table 4 yielded results on par with the best result.
Table 7 results verify that for OLI, the proposed models performed better than the conventional models. The strength of the handcrafted transliteration and translation features cleared the way for attainment of the augmented F1-score. All the models were fine-tuned to derive optimal hyper-parameters, ensuing in a marginal outperformance by all the proposed methodologies over the published studies of contemporaneous approaches.
Figures 4 and 5 present the accuracy and loss curves respectively of the training data set. Initially, the accuracy curve shows that as the number of epochs increases, the accuracy curve grows fast; at some point, all the models converge into a single line. Hence, with the rise in the number of epochs, accuracy of the models increases, reaching saturation after a few additional epochs. The loss curves, notably high at the get-go, follow steep descents after a few epochs, reaching a plateau with increased number of epochs. However, neither oscillations nor further decrease in loss was observed with additional increase in the number of epochs. As a rule, smaller the value of the loss (closer to 0) the better the performance of the deep learning models on the test data.
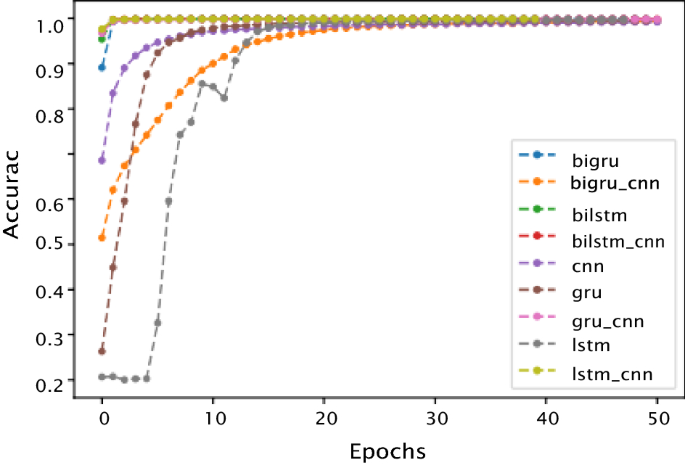
Accuracy vs epochs
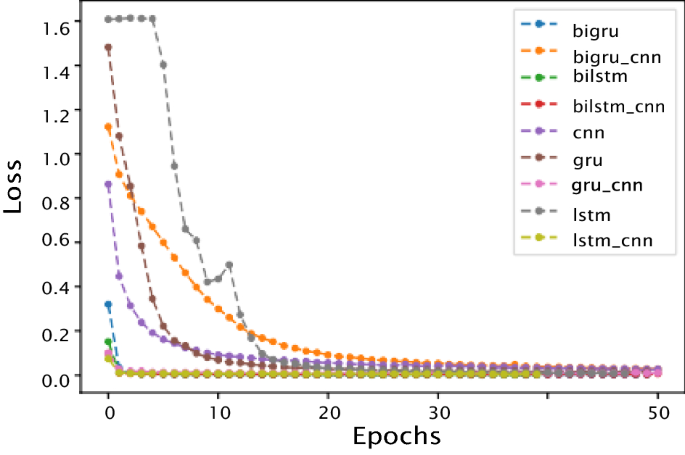
Loss vs epochs
Albeit each model can be appraised for its accuracy, this does not furnish any insight into the aptness of this model. Figure 6 displays the Confusion Matrices which deal with the test data that validate the performance of a model. In each matrix, the x-axis represents the predicted labels, whereas the y-axis represents the veritable true labels. The diagonal elements portray the majority of normalized values, for which the predicted label and true label are equal. Matrix values in the upper and lower triangles are misclassified samples, with respect to classes in each row and column. The figure verifies the small misclassification rate for the models, which signify many correct predictions. In Table 8 , the proposed methodologies of GRU + CNN, BiLSTM + CNN and BiGRU show the lowest misclassification rate of 0.003 due to high F1-score, as compared to CNN, which has the highest misclassification rate of 0.01 supervened by a low F1-score. Hence, they have a strong performance edge over CNNs. In addition, the class-wise accuracy for each of the proposed deep learning models is outstanding. Interestingly, although these proposed models rarely confuse between NM and NF, few misclassifications were observed for the other 3 classes (OTIG, OTII, OUT).
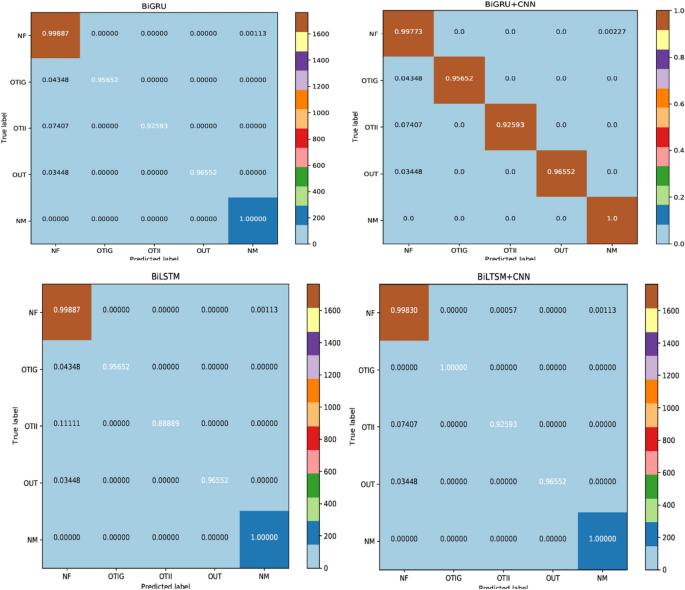
Confusion matrices of various models on offensive detection task
Data summarized in Table 9 reaffirm the augmented F1-score of SA of the proposed system as being comparable to the extant benchmark criteria. F1-scores for SA, as compared to OLI tasks, are relatively low, limited by data availability. GRU gave impressive results among all the experimented models. GRU works better than CNN as the latter does not consider long term dependencies in the sentences, which is important in text analytics. GRUs are simpler in nature due to the presence of additional update and forget gates in LSTM. Unlike LSTM, GRUs are able to avoid being overfitted, as reflected in the F1-scores of the proposed deep learning models. The lowest misclassification rate of 0.236 which can be ascribed to GRUs having the highest F1-score.
Top 3 existing approaches versus proposed model for SA are shown in Table 10 . Compared to all other systems, the proposed GRU model achieved notable improvement in F1-score, achieving a marginal 2% improvement. The key to this lies in the obligatory preprocessing stages of transliteration and translation. Supervened by the class imbalance problem, corpus of code-mixed language can be worked around, by up-sampling, to avert performance degradation.
Figure 7 portrays how each of the five hyper-parameters are varied for the best experiment, which reportedly has the highest F1-score and accuracy for SA. As the number of epochs increases, the model is more capable of generalizing the learning. Usage of a large number of epochs ensue in overfitting problem on the training set, and the model would perform poorly for validation or test set. The maximum F1-score was attained at epoch 130. Simultaneous dropout is used as a better regularization technique, to avoid overfitting. The figure shows that the model reaches the highest performance value with a dropout value of 0.34. Next, the optimizer minimizes the loss function of the model. Among the five popular optimizers, Adam was the preferred choice as it gave the highest classification performance. Also, we have learning rate where it is responsible for the optimization of the weights. The classification performance of the model became stable when the value of the learning rate was 0.0001. Finally, the maximum performance was achieved at a window size of 9.
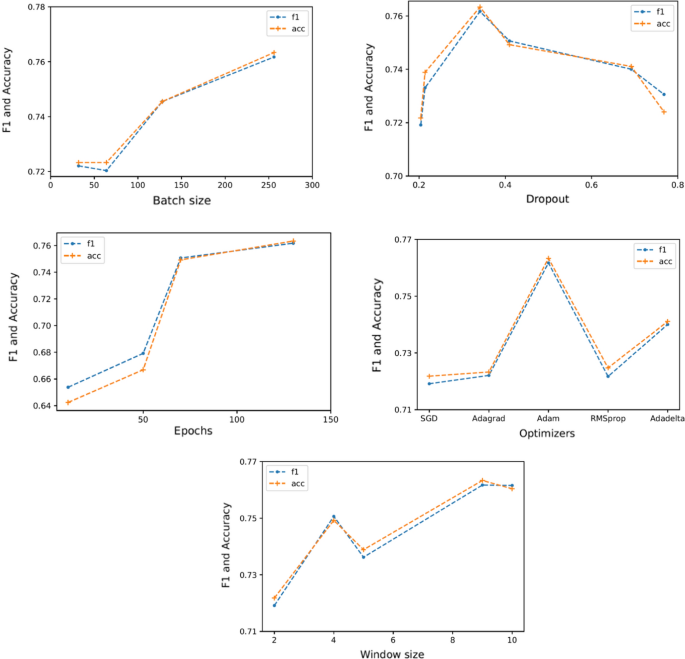
Relationship between hyper-parameters and performance metrics (F1-score (f1) and Accuracy (acc))
Table 11 presents the results of the models, with and without translation and transliteration, to draw attention to their relevance as the key preprocessing step in SA. An increase in the weighted F1-score value on the data set was seen with translation and transliteration, when compared to data set without translation and transliteration. This is a direct consequence of the rich features extracted, before translation and transliteration, from the data set of a single language corpus by the word embedding models, in contrast to a corpora of multiple languages.
A detailed error analysis of the proposed models, conducted to derive insightful corollaries, is shown in Table 12 . As the posts in the not-Malayalam class were either in Roman or native scripts, the proposed models were able to clearly discern this class from rest of the classes. Comments are often misclassified as positive in the SA task, as majority of the data set belongs to positive class. After the positive class, Unknown state class has a greater number of examples in the code-mixed data set. Hence, unknown state has a good class-wise accuracy, after NM and positive classes. Few negative classes were mis-classified as positive which may be due to micro aggressive [ 51 ] comments posted by people. Such subtle comments complicate the analysis for researchers, in the discernment of the true nature, quantification, and automatic extraction of micro aggressions. For instance:
Mara paazhu mega mairananil ninnum ethil koodutal pratheeshikaruthu 1980 kalile rajanikanthinu padikkunnu verum chavaru mairan….
True label: Negative
Predicted label: Positive
In the above example, albeit the comments are not explicitly negative, they clearly express disapproval of the movie.
Maoist alla avnte achn vare namml adich odikkum ikkaaa
True label: unknown state
In the above example Sarcasm not detected. It was observed that the models could not capture sarcasm. It is a complex linguistic phenomena.
Lalettante stunt…. kandirunnupovum aarum… Entha oru style. Mammootty
thapassirunnal polum ettante aduthu ethillaaa….
True label : Mixed feelings
Predicted label: Negative
In above examples, system failed to predict correctly, as they did not possess the required world knowledge. Here the word thapassirunnal is a colloquial metaphor, which is used for mocking someone but the system failed to understand it.
Movie ok aanu. Entertaining movie
True label: Positive
Predicted label: Mixed feelings
Length of the sentences played a vital role in predicting correctly. When the length of the sentence is too short or too long the classifiers failed to predict it.
The proposed method can benefit humankind in societal perspectives. Albeit access restricted to people who know or understand English, as the Internet is rife with digital information, any small gain or acquired information must be disseminated to commoners unfamiliar or unable to access technology.
Limitations
As the training data for SA was less than for OLD, in the code-mixed dataset, adoption of transfer learning [ 52 ] from a multilingual model would be preferable as it can further enhance the performance.
This work reviewed significant research of the Malayalam–English code-mixed language, accessible in the public domain. Several deep learning models were exploited for two basic tasks: SA and OLI. The proffered method achieved impressive F1-scores, in spite of the intricacies of code-mixed language, as compared to monolingual language. Asymmetrical distribution of code-mixed data sets among different classes apropos. SA and OLD tasks, call for an up-sampling work around strategy. This research highlighted the aptness of translation and transliteration preprocessing. These major offerings coupled with data up-sampling and word embeddings, led to benchmark results for deep learning methods. As observed the proposed model achieved the best score for both the tasks. Empirical analysis of deep learning models yielded marginal improvements of 1.63% in accuracy and 1.55% in F1-score for OLD, while enhancements of 12.08% in accuracy and 9.86% in F1-score for SA were turned in. The readers are anticipated to make informed choices in their selection of the deep learning model, or perhaps, a combination of models that may be a judicious choice.
Future work
Extant work of code-mixed languages can be broadened to handle more than two languages, for multilingual societies. Despite advancements reported in the code-mixed domain, limited availability of sentimental analysis data, call for improvement in a model’s accuracy [F1-scores]. Data augmentation by selective addition of class-specific data is expected to lower the misclassification rate. On the design section, ensemble approach should be probed to ascertain its relevance and efficacy.
Availability of data and materials
Not applicable. For any collaboration, please contact the authors.
https://en.wikipedia.org/wiki/History_of_the_British_Raj .
https://www.britannica.com/topic/Dravidian-languages .
https://en.wikipedia.org/wiki/Vatteluttu .
https://en.wikipedia.org/wiki/Abugida .
https://dravidian-codemix.github.io/2020/ .
https://competitions.codalab.org/competitions/27654 .
https://www.nltk.org/ .
https://pypi.org/project/google-trans-new/ .
https://www.kaggle.com/pierremegret/gensim-word2vec-tutorial .
https://pypi.org/project/numpy/ .
https://keras.io/api/layers/corelayers/embedding/ .
https://huggingface.co/transformers/ .
https://pypi.org/project/scikit-learn/ .
https://scikit-learn.org/ .
Reinsel D, Gantz J, Rydning J. The digitization of the world from edge to core. Framingham: International Data Corporation; 2018. p. 16.
Google Scholar
Kemp S. Hootsuite: Digital in 2018: essential insights into internet, social media, mobile, and ecommerce use around the world. 2018, 1–153.
Chakravarthi BR, Priyadharshini R, Muralidaran V, Jose N, Suryawanshi S, Sherly E, McCrae JP. Dravidiancodemix: Sentiment analysis and offensive language identification dataset for Dravidian languages in code-mixed text. arXiv preprint arXiv:2106.09460 , 2021.
Thara S, Poornachandran P. Transformer based language identification for Malayalam–English code-mixed text. IEEE Access. 2021;9:118837–50.
Article Google Scholar
Saha D, Paharia N, Chakraborty D, Saha P, Mukherjee A. Hate-alert@ dravidianlangtech-eacl2021: Ensembling strategies for transformer-based offensive language detection. arXiv preprint arXiv:2102.10084 , 2021.
Severyn A, Uryupina O, Plank B, Moschitti A, Filippova K. Opinion mining on YouTube. 2014.
Mabrouk A, D´ıaz Redondo RP, Kayed M. Deep learning-based sentiment classification: a comparative survey. IEEE Access. 2020; 8:85616–38
Agarwal B, Nayak R, Mittal N, Patnaik S. Deep learning-based approaches for sentiment analysis. Berlin: Springer; 2020.
Book Google Scholar
Patwa P, Aguilar G, Kar S, Pandey S, Pykl S, Gamb¨ack B, Chakraborty T, Solorio T, Das A. Semeval-2020 task 9: overview of sentiment analysis of code-mixed tweets. In Proceedings of the Fourteenth Workshop on Semantic Evaluation. 2020; 774–790.
Thara S, Krishna A. Aspect sentiment identification using random Fourier features. Int J Intell Syst Appl. 2018;10:32–9.
Aparna TS, Simran K, Premjith B, and Soman KP. Aspect-based sentiment analysis in Hindi: Comparison of machine/deep learning algorithms. In Inventive Computation and Information Technologies. Springer; 2021, 81–91.
Chakravarthi BR, Anand Kumar M, McCrae JP, Premjith B, Soman KP, Mandl T. Overview of the track on hasoc-offensive language identification-Dravidiancodemix. In FIRE (Working Notes). 2020; 112–120.
Ranjan P, Raja B, Priyadharshini R, Balabantaray RC. A comparative study on code-mixed data of Indian social media vs formal text. In 2016 2nd international conference on contemporary computing and informatics (IC3I). IEEE, 2016; 608–611.
Bayer J, Petra B´. Hate speech and hate crime in the EU and the evaluation of online content regulation approaches. Policy Report European Parliament, 2020.
Lohani R, Suresh V, Varghese EG, Thara S. An analytical overview of the state-wise impact of covid-19 in India. ICT Analysis and Applications. 2022; 845– 853.
Imran M, Qazi U, Ofli F. Tbcov: two billion multilingual covid-19 tweets with sentiment, entity, geo, and gender labels. Data. 2022;7(1):8.
Pratapa S, Bhat G, Choudhury M, Sitaram S, Dandapat S, Bali K. Language modeling for code-mixing: the role of linguistic theory based synthetic data. In Proceedings of the 56th Annual Meeting of the Association for Computational Linguistics (Volume 1: Long Papers). 2018; pp. 1543–1553.
Scotton CM, Jake J. Duelling languages. Grammatical structure in codeswitching. Oxford: Clarendon Press; 1993.
Pathak V, Joshi M, Joshi P, Mundada M, Joshi T. Kbcnmujal@ hasoc-dravidian-codemix-fire2020: Using machine learning for detection of hate speech and offensive code-mixed social media text. arXiv preprint arXiv:2102.09866 , 2021.
Balaji NN, Bharathi B. Ssncse nlp@ hasoc-dravidian-codemix-fire2020: Offensive language identification on multilingual code mixing text. In FIRE (Working Notes). 2020; 370–376.
Angel J, Aroyehun ST, Tamayo A, Gelbukh A. NLP-CIC at SemEval-2020 task 9: Analysing sentiment in code-switching language using a simple deep-learning classifier. In Proceedings of the Fourteenth Workshop on Semantic Evaluation, pages 957–962, Barcelona (online), December 2020. International Committee for Computational Linguistics.
Banerjee S, Ghannay S, Rosset S, Vilnat A, Rosso P. LIMSI UPV at SemEval-2020 task 9: Recurrent convolutional neural network for code-mixed sentiment analysis. In Proceedings of the Fourteenth Workshop on Semantic Evaluation, pages 1281–1287, Barcelona (online), December 2020. International Committee for Computational Linguistics.
Baroi SJ, Singh N, Das R, Singh TD. NITS-Hinglish-sentimix at semeval-2020 task 9: Sentiment analysis for code-mixed social media text using an ensemble model, 2020.
Singh A, Parmar SP. Voice@SRIB at SemEval-2020 tasks 9 and 12: Stacked ensembling method for sentiment and offensiveness detection in social media. In Proceedings of the Fourteenth Workshop on Semantic Evaluation, pages 1331– 1341, Barcelona (online), December 2020. International Committee for Computational Linguistics.
Chakravarthi BR, Muralidaran V, Priyadharshini R, McCrae JP. Corpus creation for sentiment analysis in code-mixed Tamil-English text. arXiv preprint arXiv:2006.00206 , 2020.
Chakravarthi BR, Jose N, Suryawanshi S, Sherly E, McCrae JP. A sentiment analysis dataset for code-mixed Malayalam–English. arXiv preprint arXiv:2006.00210 , 2020.
Chakravarthi BR, Chinnappa D, Priyadharshini R, Madasamy AK, Sivanesan S, Navaneethakrishnan SC, Thavareesan S, Vadivel D, Ponnusamy R, Kumaresan PK. Developing successful shared tasks on offensive language identification for Dravidian languages. arXiv e-prints, pages arXiv–2111, 2021.
Shekhar S, Garg H, Agrawal R, Shivani S, Sharma B. Hatred and trolling detection transliteration framework using hierarchical lstm in code-mixed social media text. Complex & Intelligent Systems. 2021; pages 1–14.
Advani L, Lu C, Maharjan S. C1 at SemEval-2020 task 9: SentiMix: Sentiment analysis for code-mixed social media text using feature engineering. In Proceedings of the Fourteenth Workshop on Semantic Evaluation, pages 1227–1232, Barcelona (online), December 2020. International Committee for Computational Linguistics.
Javdan S, Shangipourataei T, Minaei-Bidgoli B. Iust at semeval-2020 task 9: Sentiment analysis for code-mixed social media text using deep neural networks and linear baselines, 2020.
Sun Y, Wang S, Li Y, Feng S, Tian H, Wu H, Wang H. Ernie 2.0: a continual pre-training framework for language understanding. Proc the AAAI Confer Artif Intell. 2020;34(5):8968–75.
Liu J, Chen X, Feng S, Wang S, Ouyang X, Sun Y, Huang Z, Su W. Kk2018 at SemEval-2020 task 9: Adversarial training for code-mixing sentiment classification. In Proceedings of the Fourteenth Workshop on Semantic Evaluation, pages 817–823, Barcelona (online), December 2020. International Committee for Computational Linguistics.
Dowlagar S, Mamidi R. Cmsaone@dravidian-codemix-fire2020: a meta embedding and transformer model for code-mixed sentiment analysis on social media text, 2021.
Kumar A, Agarwal H, Bansal K, Modi A. Baksa at semeval-2020 task 9: Bolstering cnn with self-attention for sentiment analysis of code mixed text, 2020.
Gundapu S, Mamidi R. gundapusunil at semeval-2020 task 9: syntactic semantic lstm architecture for sentiment analysis of code-mixed data, 2020.
Braaksma B, Scholtens R, van Suijlekom S, Wang R, U ¨ stu¨n A. Fissa at semeval-2020 task 9: fine-tuned for feelings, 2020.
Srivastava A, Vardhan VH. Hcms at semeval-2020 task 9: a neural approach to sentiment analysis for code-mixed texts, 2020.
Zampieri M, Malmasi S, Nakov P, Rosenthal S, Farra N, Kumar R. Predicting the type and target of offensive posts in social media. In Proceedings of the 2019 Conference of the North American Chapter of the Association for Computational Linguistics: Human Language Technologies, Volume 1 (Long and Short Papers). 2019; 1415–1420.
Sreelakshmi K, Premjith B, and Soman Kp. Amrita cen nlp@ dravidianlangtech-eacl2021: deep learning-based offensive language identification in Malayalam, Tamil and Kannada. In Proceedings of the First Workshop on Speech and Language Technologies for Dravidian Languages. 2021; 249–254.
Sengupta A, Bhattacharjee SK, Akhtar MS, Chakraborty T. Does aggression lead to hate? Detecting and reasoning offensive traits in hinglish code-mixed texts. Neurocomputing. 2021.
Chopra S, Sawhney R, Mathur P, Shah RR. Hindi-English hate speech detection: author profiling, debiasing, and practical perspectives. In Proceedings of the AAAI Conference on Artificial Intelligence, volume 34, 2020; 386–393.
Mikolov T, Chen K, Corrado G, Dean J. Efficient estimation of word representations in vector space, 2013.
Bojanowski P, Grave E, Joulin A, Mikolov T. Enriching word vectors with subword information. Trans Assoc Comput Linguist. 2017;5:135–46.
Chakravarthi BR, Priyadharshini R, Anand Kumar M, Krishnamurthy P, Sherly E, editors. Proceedings of the First Workshop on Speech and Language Technologies for Dravidian Languages, Kyiv, April 2021. Association for Computational Linguistics.
Levy O, Goldberg Y. Dependency-based word embeddings. In Proceedings of the 52nd Annual Meeting of the Association for Computational Linguistics (Volume 2: Short Papers). 2014; 302–308.
Sutskever I, Vinyals O, Le QV. Sequence to sequence learning with neural networks, 2014.
Hochreiter S. The vanishing gradient problem during learning recurrent neural nets and problem solutions. Int J Uncertain Fuzziness Knowl Based Syst. 1998;6(2):107–16.
Article MathSciNet Google Scholar
Conneau A, Khandelwal K, Goyal N, Chaudhary V, Wenzek G, Guzm´an F, Grave E, Ott M, Zettlemoyer L, Stoyanov V. Unsupervised cross-lingual representation learning at scale, 2020.
Jayanthi SM, Gupta A. Sj aj@ dravidianlangtech-eacl2021: Task- adaptive pre-training of multilingual bert models for offensive language identification. arXiv preprint arXiv:2102.01051 , 2021.
Sharif O, Hossain E, Hoque MM. Nlp-cuet@ dravidianlangtech-eacl2021: Offensive language detection from multilingual code-mixed text using transformers. arXiv preprint arXiv:2103.00455 , 2021.
Breitfeller L, Ahn E, Jurgens D, Tsvetkov Y. Finding microaggressions in the wild: a case for locating elusive phenomena in social media posts. In Proceedings of the 2019 Conference on Empirical Methods in Natural Language Pro- cessing and the 9th International Joint Conference on Natural Language Processing (EMNLP-IJCNLP), pages 1664–1674, Hong Kong, China, November 2019. Association for Computational Linguistics.
Pan SJ, Yang Q. A survey on transfer learning. IEEE Trans Knowl Data Eng. 2009;22(10):1345–59.
Download references
Acknowledgements
Not applicable.
Not applicable. This research received no specific grant from any funding agency.
Author information
Authors and affiliations.
Department of Computer Science and Engineering, Amrita Vishwa Vidyapeetham, Amritapuri, India
S. Thara & Prabaharan Poornachandran
You can also search for this author in PubMed Google Scholar
Contributions
ST is the first author role, she performed the literature review, implemented the proposed model, carried out the experiments and wrote the manuscript. PP has a supervisory role, he oversaw the completion of the work. Both authors read and approved the final manuscript.
Corresponding author
Correspondence to S. Thara .
Ethics declarations
Ethics approval and consent to participate.
The author confirms the sole responsibility for this manuscript.
Consent for publication
Competing interests.
The authors declare that there is no conflict of interest.
Additional information
Publisher's note.
Springer Nature remains neutral with regard to jurisdictional claims in published maps and institutional affiliations.
Rights and permissions
Open Access This article is licensed under a Creative Commons Attribution 4.0 International License, which permits use, sharing, adaptation, distribution and reproduction in any medium or format, as long as you give appropriate credit to the original author(s) and the source, provide a link to the Creative Commons licence, and indicate if changes were made. The images or other third party material in this article are included in the article's Creative Commons licence, unless indicated otherwise in a credit line to the material. If material is not included in the article's Creative Commons licence and your intended use is not permitted by statutory regulation or exceeds the permitted use, you will need to obtain permission directly from the copyright holder. To view a copy of this licence, visit http://creativecommons.org/licenses/by/4.0/ .
Reprints and permissions
About this article
Cite this article.
Thara, S., Poornachandran, P. Social media text analytics of Malayalam–English code-mixed using deep learning. J Big Data 9 , 45 (2022). https://doi.org/10.1186/s40537-022-00594-3
Download citation
Received : 27 October 2021
Accepted : 28 March 2022
Published : 26 April 2022
DOI : https://doi.org/10.1186/s40537-022-00594-3
Share this article
Anyone you share the following link with will be able to read this content:
Sorry, a shareable link is not currently available for this article.
Provided by the Springer Nature SharedIt content-sharing initiative
- Deep neural network
- Dravidian languages
- Natural Language Processing
- Multilingual language
Malayalam handwritten character recognition using convolutional neural network
Ieee account.
- Change Username/Password
- Update Address
Purchase Details
- Payment Options
- Order History
- View Purchased Documents
Profile Information
- Communications Preferences
- Profession and Education
- Technical Interests
- US & Canada: +1 800 678 4333
- Worldwide: +1 732 981 0060
- Contact & Support
- About IEEE Xplore
- Accessibility
- Terms of Use
- Nondiscrimination Policy
- Privacy & Opting Out of Cookies
A not-for-profit organization, IEEE is the world's largest technical professional organization dedicated to advancing technology for the benefit of humanity. © Copyright 2024 IEEE - All rights reserved. Use of this web site signifies your agreement to the terms and conditions.
Academia.edu no longer supports Internet Explorer.
To browse Academia.edu and the wider internet faster and more securely, please take a few seconds to upgrade your browser .
Enter the email address you signed up with and we'll email you a reset link.
- We're Hiring!
- Help Center
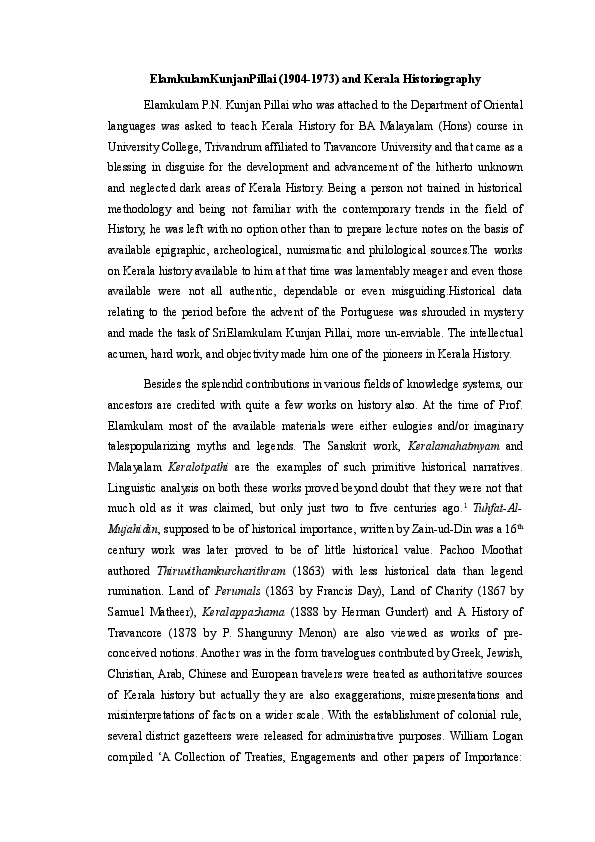
ElamkulamKunjanPillai (1904-1973) and Kerala Historiography

ElamkulamKunjanPillai (1904-1973) -Attempted to rewrite history of Kerala on the basis of epic-graphic and literary sources-
Related Papers
Malayalam Research Journal
Shiju Sam Varughese
Editor's introduction to the special issue on 'Reimagining Kerala’s Engagements with Modernity’, Malayalam Research Journal Volume no. 13, Issue no.: 1 (May–August), 2020. ISSN 09741984
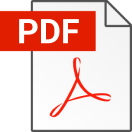
The Indian Economic & Social History Review
Sivan Goren
Department of English Payyanur College, Payyanur
A.c. Sreehari
The articles are an ensemble of literary and cultural studies based in Kerala and which address the crises of the cultural and political project called modernity.
Studies in People's History
Kesavan Veluthat
The formation of regions is as complex a process as the formation of countries or nations. Geography has much to do with it in some cases, languages in others. But political events, commercial networks and tribal or caste dominance can also play their part. History, actual or imagined, may be invoked to create regional consciousness. This article examines how owing to complex factors Kerala came to be identified as a definite region by the sixteenth century, so identified not only by its mainstream tradition but also by people external to it.
Journal of the American Oriental Society
Editor, University of Chitral Journal of Linguistics and Literature
Devika Jayakumari
T T Sreekumar
Novel, as a literary genre has always struggled to capture the anxieties embedded in national histories. The politics, ideologies and cultural conflicts of nations have always been part of the history of novel. Infact there need not even be a separate category called novels of national history. Every novel is woven by the threads and strands of the nation in making. The spatio-temporality of the genre in its European origin and its colonial dissemination, made the novel an integral unit of the nation state. Most novels of national history of the colonial period thus write new representations of colonial histories, sometimes even by inventing scores of intricate discursive methods. The novels of C. V. Raman Pillai in Malayalam confront the colonial dilemma by inventing many linguistic time-spaces within a single historical periphery that ranges from pristine Sanskrit to the most local vernacular expression 1. Raman Pillai was infact one of the first writers in Malayalam who had to face the trauma of reconceiving locales as nations. This is why heperhaps painted his Travancore rather ahistorically as asovereign nation state, a historical replica of the colonial state whose domination it was facing at the time. In his historical novels, he takes the pain to avoid any reference to colonialism and Travancore's subordination to the British, while he has authored several articles decrying colonialism as the root cause of the political problems that Travancore was facing. Bewildering diversity of Malayalam dialects that Raman Pillai uses in his works were for him only one of the tools and raw materials in the kind of imaginary nation building exercise that he ventured into through his novels 2. Such meta nationalist politics was an inseparable aspect of both nationalist and literary histories of the colonial era. Raman Pillai imagined Travancore state as one giant state in opposition to the furtive threats against it, existing outside the socioeconomic history of the world at that time. In its illusoriness and in its unaccountability to the colonial history, Raman Pillai's Travancore was actually representing the inferiority of the very subjugation that he was being silent about. His conception and use of novel was rooted in its capacity as a medium that could contain this unspeakable politics as well. In this manner, through his novels, Raman Pillai could dodge and forget the violence, the injustices and unethicality of the state of Travancore and imagine that he could conquer the inferiority instilled by colonialism by staying close to the general socio-cultural politics of nation 3. On the other hand S.K Pottekad and ThakazhiSivasankara Pillai, two prominent novelists who followed Raman Pillai's generation, did not have these conflicts while writing their novels. OruDeshathinte Katha [The Story of a desham (loacle)]' by Pottekad (1971) orKayar ['The Coir] by ThakazhiSivasankara Pillai (1978/2013) and Tattakam by Kovilan (1995) are grounded in the liberal landscape of postcolonialism. They also reinvented counter-national histories, albeit through different narratives that sought to overcome the garrulousness of nationalist politics through the ironical interpolation of the histories of the small locales (desham). K. P Appan, the foremost literary critic of high modernism in Malayalam, showers eloquent praises on S K Pottekad's material historicizing and his 'objective subjectivism' that makes him craft his novels as though they were 'Kerala's monologues'. But Appan compares the historical objectivity in Pottekad's narratives to the objectivism in journalism and eventually goes on to make a parallel to Dickens's fictional landscape. An aesthetic methodology rooted in the writing of regional histories as against the grand narratives of nation building that was evident in Pottekad was thus mostly overlooked.
Rupkatha Journal on Interdisciplinary Studies in Humanities
Niveditha Kalarikkal
Shiju Sam Varughese , Satheese Chandra Bose
The southwest coast of India has always been a significant site within the global network of relations through trade and exchange of ideas, commodities, technologies, skills and labour. The much longer history of colonial experience makes Kerala’s engagement with modernity polyvalent and complex. Without understanding the multiple space-times of this region, it is impossible to make sense of the complexities of Kerala modernity beyond its general description as ‘Malayalee modernity’. From the colonial pepper trade and Narayana Guru’s philosophical engagement with the question of caste, to the seemingly disparate elements that weave together an ‘eclectic past’ through the Muziris Heritage Project; from the debates on women’s sexuality around the Suryanelli rape case, to the gendered constitution of public space during the mass annual Attukal Pongala ritual; from the changes in state attitude towards providing piped water supply to how Cochin port’s inter-War history has scripted the story of urban modernity; from the shaping of the public sphere to the radical Left politics of the 1970s and the emergence of popular janapriya literature—this book analyses the ideas, spaces and practices that intricately weave the region’s experiences of modernity. Kerala Modernity emphasizes the methodological need to re-examine the idea of ‘region’ as a discursive category to explore Kerala’s regional modernity apart from Eurocentric and nation-centric frames of analyses. The interdisciplinary presentation—complete with a Dalit critique of modernity in the Foreword—will be an important contribution to literature on Kerala and the debates on alternative modernities in South Asia. It will be of interest to students and scholars of history, sociology and literary and cultural studies, as well as the interested general reader. CONTENTS List of Tables, Figures and Map FOREWORD : Gopal Guru Acknoweldgements INTRODUCTION / Situating an Unbound Region: Reflections on Kerala Modernity Satheese Chandra Bose and Shiju Sam Varughese CHAPTERS 01. The Routes of Pepper: Colonial Discourses around the Spice-trade in Malabar Vinod Kottayil Kalidasan 02. Colonial Intellectuals, Public Sphere and the Promises of Modernity: Reading Parangodeeparinayam Shiju Sam Varughese 03. (Re)construction of ‘the Social’ for Making a Modern Kerala: Reflections on Narayana Guru’s Social Philosophy Satheese Chandra Bose 04. Port Building and Urban Modernity: Cochin, 1920–45 Justin Mathew 05. At the End of the Story: Popular-Fiction, Readership and Modernity in Literary Malayalam Ancy Bay 06. Contemporaneity and the Collective: The Reportage in Amma Ariyaan Ameet Parameswaran 07. The Politics of Sexuality and Caste: Looking through Kerala’s Public Space Carmel Christy K.J. 08. Attukal Pongala: Myth and Modernity in a Ritualistic Space Darshana Sreedhar 09. The Pipe Dreams of Development: Institutionalising Drinking Water Supply in Kerala S. Mohammed Irshad 10. Archaeology and the New Imaginations of the Past: Understanding the Muziris Heritage Project Rachel A. Varghese REFERENCES NOTES ON CONTRIBUTORS INDEX
Pius Malekandathil
intensification of maritime trade in the Indian Ocean from the eleventh century onwards and the hectic commercial transactions that happened in Koualm Mali (Quilon), Shingly (Cranganore), Pantalayani Kollam, 1 were followed by gradual proliferation of subsidiary markets and aṅṅāḍis in inland parts of Kerala. 2 Very often, many of these vibrant markets appeared in and around the headquarters of the fourteen divisions of the Cēra rulers, and later when the chieftains of these divisions started asserting autonomy and power of varying degrees on the collapse of the Cēras of Mahōdayapuram in the twelfth century, 3 many of these markets grew along with the augmentation of consumption requirements of the rulers and the evolving political houses. The nuclei of several of the primary and secondary towns of present-day Kerala can be traced back to the major sites of power and wealth that each chieftain and his political house conveniently set up or banked upon during this period in each division in an effort to carve out an independent principality. The collapse of the Cēras of Mahōdayapuram was followed not only by the evolution of smaller principalities and political structures of various nomenclatures like svarūpaṃs, nāḍuvāḻis, dēśavāḻis, kaimals, karttās, etc. (indicating the fragmenting nature of authority-structure) across the length and breadth of Kerala, 4 but also by the evolution in
RELATED PAPERS
Revista Brasileira de Psicologia do Esporte
Marlizete Vargas
Revista de Administração da Unimep
Adriana Camargo
Current Cardiology Reviews
Thach Nguyen
Pharmacognosy Journal
Chitra Khanwelkar
Remote Sensing
Terence Kubar
Physics Letters B
Yolanda Guerrero
The Journal of Clinical Endocrinology & Metabolism
Mariana Costanzo
Axel Fernando
Journal of Cell Science
Klemens Rottner
Journal of Translational Medicine
gonneke winter-warnars
Frontiers in Psychology
Revista Iberoamericana De Educacion
Yanelis Rodríguez
RePEc: Research Papers in Economics
Coccia Mario
E-Jurnal Manajemen Universitas Udayana
Siti Nurhaliza
Attila Husar
办莫道克大学Murdoch毕业证书 澳洲大学文凭学历证书
Béatrice Daille
European Journal of Dental Education
Alison Bullock
نشریه مطالعات بین رشته ای هنرهای تجسمی دانشگاه نیشابور
maryam fadaei tehrani
UNE毕业证书 新英格兰大学毕业证
Shubha Ambardekar
Amazing Solar
Giovanni Contel
Journal of Power Sources
RELATED TOPICS
- We're Hiring!
- Help Center
- Find new research papers in:
- Health Sciences
- Earth Sciences
- Cognitive Science
- Mathematics
- Computer Science
- Academia ©2024
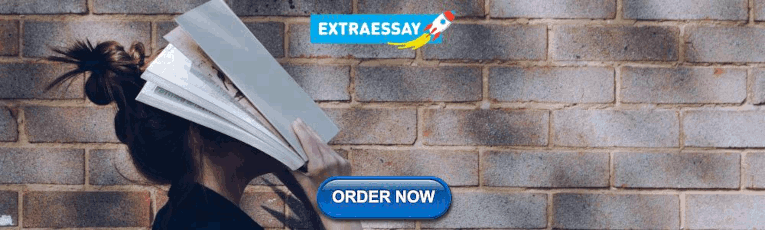
IMAGES
VIDEO
COMMENTS
This vigilant committee is known as Jagratha Samithi (in the Malayalam language) and works in every Local Self Government (Panchayat). Therefore, the object of this research is the Jagratha Samithi (JS). The study aims to identify the JS's role and activities in a panchayat to prevent violence against women and children.
Malayalam Research Journal (UGC-CARE Serial No. 41569) Malayalam Research Journal is published by Benjamin Bailey Foundation since 2008. This is an international bi-lingual Journal dedicated to language, literature and culture. Its International Standard Subscription Number is ISSN-0974-1984. The Journal has been registered with the News paper ...
Profile. Malayalam Research Journal is published by Benjamin Bailey Foundation since 2008. This is an international bi-lingual Journal dedicated to language, literature and culture. Its International Standard Subscription Number is ISSN-0974-1984. The Journal has been registered with the News paper Registrar of India and the registration number ...
Malayalam Research Journal Vol. 13, Issue. 2 May-August 2020 4800 The paper attempts to retrieve the history of dalit Christianity in Malayalam Research Journal Vol. 13, Issue. 2 May-August 2020 Malabar, which has so far been obliterated by the existing studies on the Basal Mission.
Machine learning approach to suffix separation on a sandhi rule annotated malayalam data set. South Asia Research 40, 2 (2020), 231 - 249. Google Scholar Cross Ref; Sebastian M. P. and Kumar G. S.. 2019. Verb phrases alignment technique for english-malayalam parallel corpus in statistical machine translation special issue on MTIL 2017.
Google Scholar provides a simple way to broadly search for scholarly literature. Search across a wide variety of disciplines and sources: articles, theses, books, abstracts and court opinions.
Abstract The paper introduces the study of quasimorphemes in Malayalam, a Dravidian language labeled as a creole, as a potentially feasible method to evaluate the presence of a 'Faculty of Language' in human beings. It describes the principle ... Nevertheless, the method outlined here is an important area of Biolinguistics research ...
In this paper, we propose an NER system for Malayalam using neural networks. Neural networks are exceptionally powerful tools for learning representations of data with multiple levels of abstraction.
This paper discusses the concept and method of causative sentence handling in Malayalam to English and English to Malayalam Machine Translation Systems. A Rule-based system is proposed here to ...
The research article discusses text categorization for Indian languages using corpus-based ML approaches. The research involved ten Indian languages, including Malayalam. Experiments with various machine learning methods were conducted for these languages. A review of news classification is carried out by . The paper focuses on various steps ...
In my paper, I would discuss the role of two women writers of the early twentieth century who paved the way for subsequent feminist writings in Malayalam literature. In the course of the paper, I intend to engage with the ways these writers articulated the concept of womanhood in a comparative framework, and critically analyse the responses ...
The primary objective of the Malayalam University is the promotion of study and research of Malayalam language, literature and Kerala culture. The territorial limits of the University extend to the whole of India. ... Presented paper in National seminar at Malayalam University on the topic Cyber Space in Malayalam Short Story (2016 october 28)
This video explains structure of a research paper. It details each section and contents of a scientific article with illustration. Tips to Teachers, Students...
This paper provides an overview of important contributions and advances in offline as well as online handwritten character recognition of Malayalam scripts. : Architecure of a general handwritten ...
Zigzag conversational patterns of contents in social media are often perceived as noisy or informal text. Unrestricted usage of vocabulary in social media communications complicates the processing of code-mixed text. This paper accentuates two major aspects of code mixed text: Offensive Language Identification and Sentiment Analysis for Malayalam-English code-mixed data set. The proffered ...
Optical Character Recognition is the process of converting an input text image into a machine encoded format. Different methods are used in OCR for different languages. The main steps of optical character recognition are pre-processing, segmentation and recognition. Recognizing handwritten text is harder than recognizing printed text. Convolutional Neural Network has shown remarkable ...
This paper tries to examine one of the Malayalam movies, Chemeen, through the lens of Marxist Feminism. The primary text chosen for the study is the movie, Chemeen, an adaptation of Thakazhi ...
Malayalam Research Journal (Issue Editor) Meena T. Pillai. 2021. Special issue on 'Gendering the Spectres of Keralam's Many Modernities' Two Volumes. See Full PDF. Download PDF.
Malayalam Research Journal "Kerala's Engagements with Modernity: Genealogies and Geographies" ... Engagements and other papers of Importance: Relating to British Affairs in Malabar' in 1879. His 'Malabar', the invaluable work on the history of Kerala, published in two volumes in1887, is considered to be an authentic manual on the ...
This research paper on "The Portrayal of Queerness in Malayalam Films" is based on. three films namely, Deshadanakkili Karayarilla (1986), Chanthupottu (2005), and Moothon. (2019), which carry ...
This video will provide explanations on Thesis Writing Format & Styles of Referencing in Malayalam - Research Aptitude - UGC NET Classes Malayalam. This vide...
This research paper thus analysis how internet memes have been used to communicate political satire. The study deals with the impact of internet memes on digital natives and digital migrants in India.
This paper critically analyses the politics of representation using the example of tribals in Malayalam films as it has evolved over the past decades and attempts to trace a whole gamut of ...