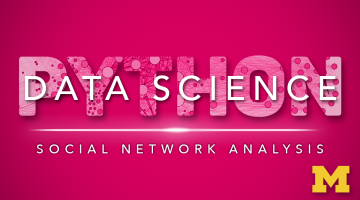
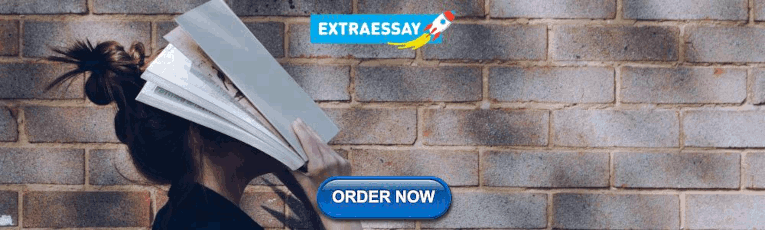
Applied Social Network Analysis in Python
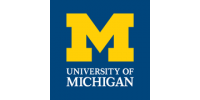
- From www.coursera.org
- Free Access
- Fee-based Certificate
- 4 Sequences
- Intermediate Level
- Subtitles in Korean
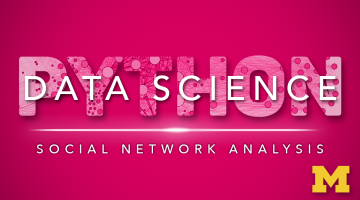
Their employees are learning daily with
Course details, prerequisite, instructors.
Daniel Romero Assistant Professor School of Information
The University of Michigan (UM, UMich or simply Michigan) is a public research university located in Ann Arbor, Michigan, in the United States. Founded in 1817, the university is Michigan's oldest and largest.
The mission of the University of Michigan is to serve the people of Michigan and the world by taking a leadership role in creating, communicating, preserving, and applying academic knowledge, art, and values, and by developing leaders and citizens who will challenge the present and enrich the future.
Coursera is a digital company offering massive open online course founded by computer teachers Andrew Ng and Daphne Koller Stanford University, located in Mountain View, California.
Coursera works with top universities and organizations to make some of their courses available online, and offers courses in many subjects, including: physics, engineering, humanities, medicine, biology, social sciences, mathematics, business, computer science, digital marketing, data science, and other subjects.
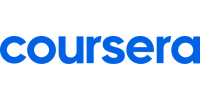
Get certified in
Your browser is ancient! Upgrade to a different browser to experience this site.
Applied Social Network Analysis in Python
Description.
This course will introduce the learner to network analysis through tutorials using the NetworkX library. The course begins with an understanding of what network analysis is and motivations for why we might model phenomena as networks. The second week introduces the concept of connectivity and network robustness. The third week will explore ways of measuring the importance or centrality of a node in a network. The final week will explore the evolution of networks over time and cover models of network generation and the link prediction problem.
This course should be taken after: Introduction to Data Science in Python, Applied Plotting, Charting & Data Representation in Python, and Applied Machine Learning in Python.
based on 2336 ratings

Applied Data Science with Python
U-M Credit Eligible
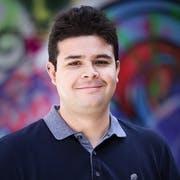
Daniel Romero
Assistant Professor
School of Information
Know someone who would like this course? Share it with them!
Share on Facebook
Share on Twitter
Share on LinkedIn
Search code, repositories, users, issues, pull requests...
Provide feedback.
We read every piece of feedback, and take your input very seriously.
Saved searches
Use saved searches to filter your results more quickly.
To see all available qualifiers, see our documentation .
- Notifications
This repository contains notes, assignments, quizzes and code files from the "Applied Social Network Analysis in Python" course by University of Michigan, on Coursera.
Anacoder1/AppliedSocialNetworkAnalysisInPython
Folders and files, repository files navigation, applied social network analysis in python.
Find the course at https://www.coursera.org/learn/python-social-network-analysis
- Jupyter Notebook 38.1%
- Python 0.5%
If you're seeing this message, that means JavaScript has been disabled on your browser , please Enable JS to make this app work.
Applied social network analysis in python || university of michigan.
In this course, learners will delve into network analysis using the NetworkX library through a series of tutorials. The curriculum is structured to provide a comprehensive understanding of network analysis concepts and methodologies.
The first week introduces the fundamentals of network analysis and discusses the rationale behind modeling phenomena as networks. In the second week, participants explore connectivity and network robustness, gaining insights into how networks function and withstand disruptions. The third week focuses on node centrality, covering various measures to assess the importance of nodes within a network.
Finally, the course delves into the temporal aspect of networks, examining their evolution over time. Additionally, participants will learn about models for network generation and tackle the link prediction problem.
Pre-requisites for this course include Introduction to Data Science in Python, Applied Plotting, Charting & Data Representation in Python, and Applied Machine Learning in Python. By the course's conclusion, learners will have acquired the necessary skills to perform network analysis and apply it to various real-world scenarios effectively.
- Universities
- Learning Packs
- For Educators
- For Corporates
- Course Recommendation
- : +91 22 2655 0659
- : +91 9820465926
- : [email protected]
- Cracking the Code of Linear Algebra: Vectors, Matrices, and Practical Implementations
Linear Algebra Uncovered: Mastering Vectors and Matrices in Your Assignment
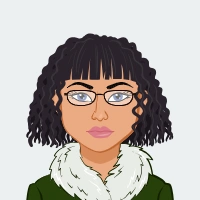
Linear algebra, a fundamental branch of mathematics, serves as a cornerstone in numerous scientific and engineering disciplines, making it an essential knowledge base for individuals embarking on careers in computer science, physics, economics, and engineering alike. The intricate principles that underlie linear algebra are not merely theoretical constructs but practical tools that enable professionals to tackle complex problems and model real-world phenomena. In the following comprehensive exploration, we will navigate through the intricacies of linear algebra, with a specific focus on vectors and matrices—integral components that form the bedrock of this mathematical domain. By unraveling the intricacies of vectors, which represent quantities with both magnitude and direction, and matrices, rectangular arrays of numbers central to linear transformations, we aim to equip readers with a profound understanding of these fundamental elements. This journey into the heart of linear algebra is not merely an academic pursuit; it is a key to unlocking the door to a myriad of applications across diverse scientific and engineering landscapes. From the basics of linear equations to the advanced concepts of eigenvalues and eigenvectors, we will embark on a journey that not only elucidates the theoretical foundations but also unveils the practical applications of linear algebra. As we delve into the mathematical intricacies, it becomes evident that linear algebra is not a mere set of abstract principles but a powerful toolset with far-reaching implications, offering assistance with your math assignment and beyond.
.webp)
This blog post endeavors to demonstrate the significance of linear algebra in shaping the technological landscape, powering innovations in computer graphics, machine learning algorithms, and quantum mechanics. It transcends disciplinary boundaries, proving indispensable in engineering for structural analysis, electrical circuit modeling, and in the field of economics for quantitative modeling and game theory. As we navigate through the complexities of linear algebra, it is imperative to recognize its transformative potential—whether you are manipulating matrices to solve intricate engineering problems or applying eigenvalues to understand the behavior of dynamic systems. The ability to comprehend and wield the principles of linear algebra is not confined to the realm of homework assignments; it is a passport to the frontier of innovation and problem-solving. In essence, this comprehensive exploration serves not only as a guide through the theoretical landscape of linear algebra but as an invitation to appreciate its practical significance in shaping the technological, scientific, and economic landscapes of our interconnected world.
1: The Basics of Linear Algebra
Linear algebra serves as the backbone of various mathematical and scientific disciplines, offering a fundamental framework for understanding and solving complex problems. At its core, linear algebra deals with linear equations and the properties of linear mappings, providing a systematic approach to analyzing and manipulating vectors and matrices. Scalars, vectors, and matrices constitute the essential elements of this mathematical domain, with scalars representing single numerical values, vectors embodying quantities with both magnitude and direction, and matrices serving as rectangular arrays of numbers. Linear equations and systems, intrinsic to linear algebra, play a pivotal role in problem-solving, and mastering their solutions is paramount. As we embark on this journey into the basics of linear algebra, we will explore the operations involving vectors, such as addition, scalar multiplication, and the dot product. Furthermore, we will delve into the realm of matrices, examining their operations, including addition, multiplication, and inversion. The knowledge gained in these fundamental aspects lays the groundwork for more advanced topics like eigenvalues, eigenvectors, and their applications in diverse fields, from computer science to physics and beyond. Through unraveling the basics, we pave the way for a comprehensive understanding of the rich tapestry that is linear algebra.
Introduction to Linear Algebra
Linear algebra deals with linear equations and the properties of linear mappings. It provides a framework for understanding and solving complex problems through the manipulation of vectors and matrices. We'll begin by exploring the basic concepts and historical background that laid the foundation for linear algebra.
Scalars, Vectors, and Matrices
Scalars, vectors, and matrices form the cornerstone of linear algebra. Scalars are single numerical values, while vectors are quantities with both magnitude and direction. Matrices, rectangular arrays of numbers, encapsulate relationships between vectors. Understanding the properties and operations involving these elements is crucial for mastering linear algebra. The manipulation of scalars, vectors, and matrices provides the foundational skills necessary for more advanced concepts.
Linear Equations and Systems
Linear equations, the heart of linear algebra, lay the groundwork for understanding and solving complex problems. In this section, we delve into the different types of linear equations and their organization into systems. Solving these systems is a fundamental skill, and it sets the stage for tackling more intricate topics in linear algebra. The ability to navigate and manipulate linear equations and systems is key for applications in diverse fields such as physics, computer science, and economics.
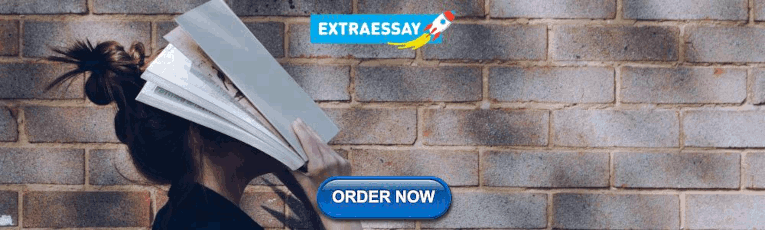
2: Vectors and Vector Spaces
We embark on a comprehensive exploration of vectors and vector spaces, essential components in the realm of linear algebra. Vectors, mathematical entities possessing both magnitude and direction, take center stage as we delve into their operations, including addition, scalar multiplication, and the fundamental dot product. These operations lay the groundwork for understanding the algebraic manipulation of vectors, paving the way for more intricate concepts within the discipline. Moving beyond individual vectors, we venture into the overarching concept of vector spaces, providing a structured environment where vectors can exist and undergo transformations. Topics such as subspaces, linear independence, and basis vectors come under scrutiny, unraveling the intricate properties that govern vector spaces. A firm grasp of these concepts is pivotal for navigating the broader applications of linear algebra, from physics to computer science. As we unravel the intricacies of vectors and their spaces, a deeper understanding of the algebraic structures that define them emerges, equipping learners with the tools needed to tackle advanced challenges in the multifaceted landscape of linear algebra.
Vector Operations
Vectors, mathematical entities representing both magnitude and direction, are fundamental in linear algebra. This section delves into vector operations, exploring additions, scalar multiplications, and the dot product. Understanding these operations is crucial as they form the basis for more advanced concepts, providing a solid foundation for further exploration in linear algebra.
Vector Spaces
Vector spaces are essential mathematical structures that allow vectors to exist and be manipulated. This section explores the properties of vector spaces, covering concepts such as subspaces, linear independence, and basis vectors. A grasp of vector spaces is crucial for understanding the broader applications of linear algebra, providing a framework for solving complex problems across diverse scientific and engineering disciplines.
3: Matrices and Matrix Operations
In the realm of linear algebra, Matrices and Matrix Operations stand as pivotal components, offering a concise yet potent framework for representing and manipulating complex mathematical structures. Matrices, defined as rectangular arrays of numbers, serve as versatile tools applicable across various disciplines. In this chapter, we delve into the fundamental aspects of matrices, elucidating their notation, basic properties, and the intrinsic role they play in encapsulating linear transformations. Matrix Operations, a cornerstone of this exploration, encompass addition, multiplication, and inversion, unlocking a plethora of applications. Matrix multiplication, a particularly pivotal operation, serves as the linchpin for solving systems of linear equations and understanding intricate transformations. As we navigate through the intricacies of these operations, their properties and applications unravel, showcasing how matrices serve as indispensable tools in fields ranging from physics to computer science. This chapter not only unveils the elegance of matrix mathematics but also lays the groundwork for comprehending advanced topics, underscoring the enduring significance of matrices in the broader landscape of linear algebra.
Introduction to Matrices
Matrices, rectangular arrays of numbers, form a core element in linear algebra. They offer a concise representation for linear transformations and play a vital role in various mathematical applications. This se ction introduces the fundamental concept of matrices, detailing their notation, basic properties, and their significance in modeling diverse mathematical structures.
Matrix Operations
Matrix operations, encompassing addition, multiplication, and inversion, constitute fundamental aspects of linear algebra. In this section, we delve into the properties and applications of these operations. Matrix multiplication, a key element, is thoroughly explored for its pivotal role in manipulating and transforming matrices, forming the backbone of numerous mathematical and computational processes. Understanding matrix operations is essential for mastering the broader applications of linear algebra in scientific and engineering domains.
4: Eigenvalues and Eigenvectors
In the realm of linear algebra, Eigenvalues and Eigenvectors stand out as pivotal concepts with far-reaching applications. Eigenvalues are scalar values that represent the scaling factor by which a matrix transforms an Eigenvector, a non-zero vector that remains in the same direction after the transformation. Understanding Eigenvalues and Eigenvectors provides profound insights into the behavior of linear transformations and is instrumental in various scientific and engineering domains. The significance of these concepts extends to diagonalization, a process that simplifies the representation of matrices and finds application in solving differential equations and analyzing complex systems. Whether employed in physics, computer science, or engineering, Eigenvalues and Eigenvectors facilitate the comprehension and manipulation of intricate mathematical structures. This chapter explores the fundamental principles underlying Eigenvalues and Eigenvectors, offering a detailed examination of their computation, properties, and applications. As we uncover the intricacies of these eigenpairs, we gain a deeper understanding of the inherent patterns and symmetries present in linear transformations, unlocking a powerful analytical toolset for tackling diverse problems across disciplines.
Understanding Eigenvalues and Eigenvectors
Eigenvalues and eigenvectors are powerful concepts in linear algebra with applications in various fields, including physics, computer science, and engineering. We'll explain the meaning of eigenvalues and eigenvectors and demonstrate how to compute them. Understanding these concepts provides insight into the behavior of linear transformations.
Diagonalization and Applications
Diagonalization is a process that simplifies the representation of certain matrices. We'll explore how to diagonalize matrices and discuss applications in areas such as differential equations and data analysis. Diagonalization provides a powerful tool for solving complex problems by simplifying the underlying mathematical structures.
5: Applications of Linear Algebra
Applications of linear algebra extend across diverse fields, showcasing its pervasive influence in solving real-world problems. In computer science, linear algebra serves as a cornerstone for algorithms in machine learning, computer graphics, and data analysis. The manipulation of vectors and matrices enables efficient data representation and computation, underpinning the foundations of artificial intelligence. In engineering and physics, linear algebra finds applications in modeling and analyzing complex systems, ranging from structural engineering to quantum mechanics. The ability to express physical phenomena through matrices facilitates the understanding and prediction of various scientific phenomena. Moreover, linear algebra is not confined to the realms of technology and science; it plays a vital role in economics and social sciences. Economic modeling, game theory, and social network analysis leverage linear algebra concepts to formulate and solve intricate problems, illustrating its significance in understanding complex relationships and dynamics within societal structures. These diverse applications underscore the interdisciplinary nature of linear algebra, positioning it as an invaluable tool for addressing challenges in fields as varied as technology, science, economics, and social sciences.
Linear Algebra in Computer Science
Linear algebra is pivotal in computer science, permeating various realms from computer graphics to machine learning. Vectors, matrices, and eigenvalues form the backbone of algorithms, facilitating tasks such as image processing, data analysis, and artificial intelligence. The efficient manipulation of these mathematical entities empowers computer scientists to develop robust and sophisticated applications, making linear algebra an indispensable tool in the digital landscape.
Engineering and Physics Applications
In engineering and physics, linear algebra serves as a cornerstone for modeling physical systems and solving intricate equations. Structural analysis, electrical circuits, and quantum mechanics leverage matrix representations, vector spaces, and eigenvalues to describe complex phenomena. Engineers and physicists harness the power of linear algebra to unravel the intricacies of the physical world, enabling advancements in technology, infrastructure, and our understanding of fundamental principles governing the universe.
Economic and Social Applications
Linear algebra extends its influence beyond the realms of science and technology, finding crucial applications in economics and social sciences. Economic modeling relies on linear algebra to formulate and solve systems of equations, guiding decision-making processes. Game theory utilizes matrices and strategic interactions for modeling competitive scenarios. In social network analysis, linear algebra facilitates the understanding of complex relationships and patterns within interconnected systems. The impact of linear algebra in these domains underscores its versatility as a tool for deciphering and addressing real-world challenges in economic and social contexts.
In conclusion, delving into the intricacies of linear algebra is an intellectually rewarding journey that unveils the mathematical framework governing numerous scientific and engineering disciplines. From the foundational concepts of scalars, vectors, and matrices to the advanced realms of eigenvalues and eigenvectors, this exploration equips learners with a versatile toolkit for problem-solving. The significance of linear algebra extends beyond mere academic exercises, finding practical applications in fields like computer science, engineering, physics, economics, and social sciences. Whether deciphering complex algorithms in computer graphics, unraveling the behavior of physical systems in engineering and physics, or modeling economic interactions in social sciences, the principles of linear algebra prove indispensable. Mastery of this subject is not merely about excelling in homework assignments; it's about acquiring a profound understanding of the fundamental structures that govern our quantitative world. As students embark on this intellectual journey, they gain not only mathematical prowess but also a powerful lens through which to comprehend and manipulate the intricate relationships underlying diverse phenomena. Linear algebra thus emerges as a cornerstone of analytical thinking, empowering individuals to unravel the complexities of our dynamic and interconnected world.
Post a comment...
Cracking the code of linear algebra: vectors, matrices, and practical implementations submit your assignment, attached files.
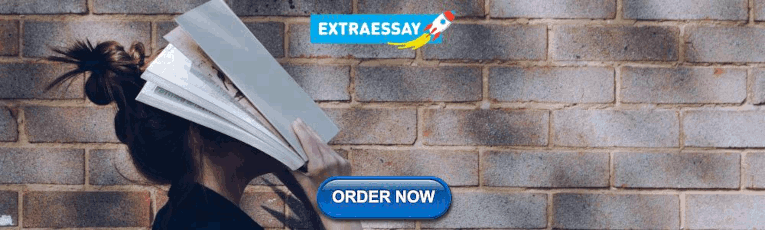
IMAGES
VIDEO
COMMENTS
Applied Social Network Analysis in Python. This repository contains a collection of the assignments used in the course Applied Social Network Analysis in Python, part of Applied Data Science using Python Specialization from University of Michigan offered by Coursera. This course will introduce the learner to network analysis through tutorials ...
This course will introduce the learner to network analysis through tutorials using the NetworkX library. The course begins with an understanding of what network analysis is and motivations for why we might model phenomena as networks. The second week introduces the concept of connectivity and network robustness.
Applied-Social-Network-Analysis-in-Python-Course-work-Quizzes-and-Assignments-This Repository contain my Assignments and Quizzes of Applied Social Network Analysis in Python Course on Coursera. About. No description or website provided. Topics.
There are 4 modules in this course. This course will introduce the learner to network analysis through tutorials using the NetworkX library. The course begins with an understanding of what network analysis is and motivations for why we might model phenomena as networks. The second week introduces the concept of connectivity and network robustness.
Explore network analysis with Python in this 4-week course by the University of Michigan. Learn about network robustness, node centrality, and network evolution. ... The assignment will give you an opportunity to use NetworkX to analyze a networked dataset of employees in a small company. ... Start your review of Applied Social Network Analysis ...
Social network analysis is the process of investigating social structures through the use of networks and graph theory. This article introduces data scientists to the theory of social networks, with a short introduction to graph theory, information spread and influence maximization [6]. It dives into Python code with NetworkX [8] constructing and.
The applications of such analysis include marketing influence maximization, fraud detection or recommender systems. There are multiple tools and techniques that can be applied on network datasets, but they need to be chosen wisely, taking into account the problem's and the network's unique properties.
Find helpful learner reviews, feedback, and ratings for Applied Social Network Analysis in Python from University of Michigan. Read stories and highlights from Coursera learners who completed Applied Social Network Analysis in Python and wanted to share their experience. ... Do note that the assignment in week 4 does pull together the whole ...
Editor. The University of Michigan (UM, UMich or simply Michigan) is a public research university located in Ann Arbor, Michigan, in the United States. Founded in 1817, the university is Michigan's oldest and largest. The mission of the University of Michigan is to serve the people of Michigan and the world by taking a leadership role in ...
{"payload":{"allShortcutsEnabled":false,"fileTree":{"Week 4":{"items":[{"name":"Assignment 4.ipynb","path":"Week 4/Assignment 4.ipynb","contentType":"file"},{"name ...
Description. This course will introduce the learner to network analysis through tutorials using the NetworkX library. The course begins with an understanding of what network analysis is and motivations for why we might model phenomena as networks. The second week introduces the concept of connectivity and network robustness.
APPLIED SOCIAL NETWORK ANALYSIS IN PYTHON Summary Many complex structures can be modeled by networks. Studying the structure of a network can allows us to answer questions about complex phenomena. In this course, we will explore different network techniques to study the structure of social networks.
Completed Applied social network analysis in python by University of Michigan. Mainly focused on the following points mentioned below : 1. Represent and manipulate networked data using the Network ...
This assignment has three objectives: • Apply Python concepts to read and. assistwithbeow in python. Assignment 4 (Social Network Analysis) This assignment is worth 20 points, and is individual effort. Problem Definition In this Assignment, we are going to use Amazon Product Co-purchase data to make Book Recommendations using Social Network ...
Deep learning is the subset of machine learning methods based on artificial neural networks (ANNs) with representation learning.The adjective "deep" refers to the use of multiple layers in the network. Methods used can be either supervised, semi-supervised or unsupervised.. Deep-learning architectures such as deep neural networks, deep belief networks, recurrent neural networks, convolutional ...
Offered by University of Michigan. This course will introduce the learner to network analysis through tutorials using the NetworkX library. ... Enroll for free.
This repository contains notes, assignments, quizzes and code files from the "Applied Social Network Analysis in Python" course by University of Michigan, on Coursera. Resources. Readme Activity. Stars. 5 stars Watchers. 4 watching Forks. 3 forks Report repository Releases No releases published. Packages 0.
Master Python social network analysis on Coursera. Learn network analysis techniques. Enroll now for advanced skills!
There are 4 modules in this course. This course will introduce the learner to network analysis through tutorials using the NetworkX library. The course begins with an understanding of what network analysis is and motivations for why we might model phenomena as networks. The second week introduces the concept of connectivity and network robustness.
In social network analysis, linear algebra facilitates the understanding of complex relationships and patterns within interconnected systems. The impact of linear algebra in these domains underscores its versatility as a tool for deciphering and addressing real-world challenges in economic and social contexts.
Completing my 9-month assignment at Aramco's Finance PPMD, I leave enriched by the experience and the people. It was a period of significant growth, challenging projects, and learning opportunities.
Prior to that, I was involved in technical and vocational training, sharing my knowledge on topics like electronic citizenship and social network marketing with over 200 participants. My technical skills are broad and deep, encompassing Python programming, data analysis, machine learning, and artificial intelligence.
Computer science is the study of computation, information, and automation. Computer science spans theoretical disciplines (such as algorithms, theory of computation, and information theory) to applied disciplines (including the design and implementation of hardware and software). Though more often considered an academic discipline, computer science is closely related to computer programming.