Technologies
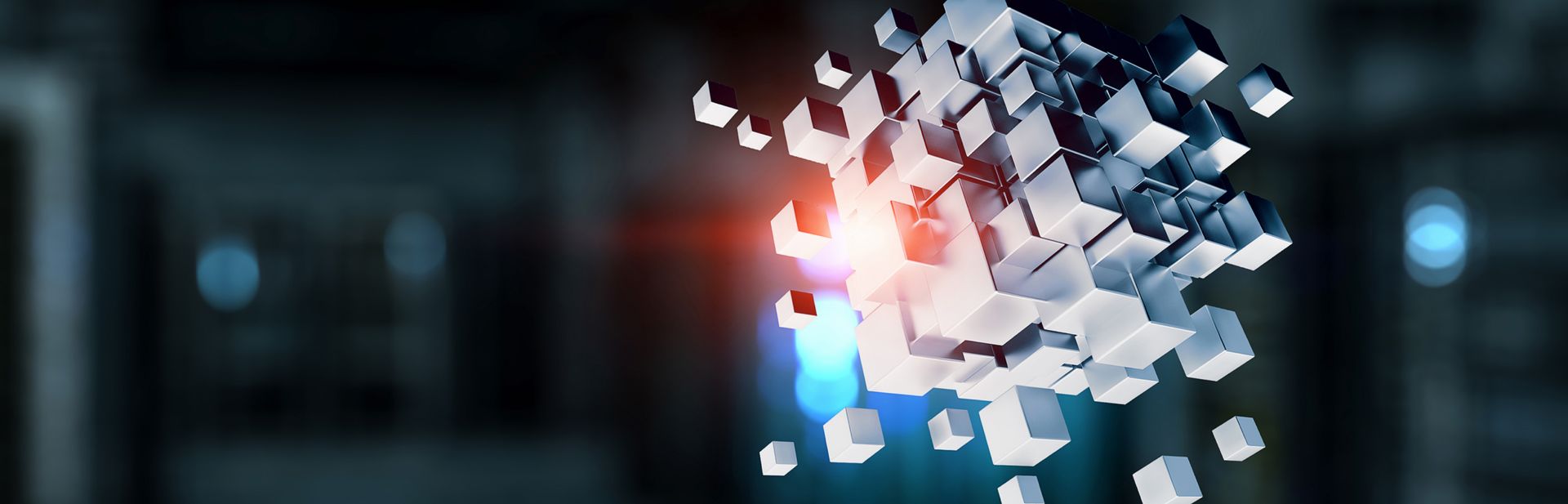
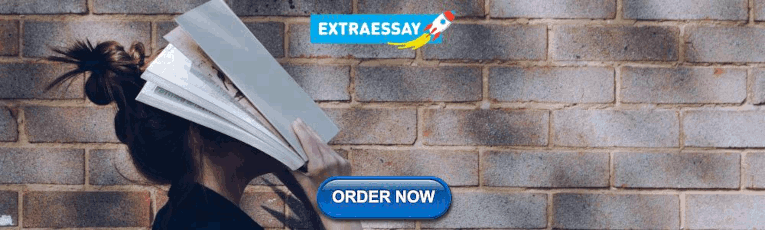
Machine learning in ecommerce: 10 use cases, examples, and best practices
September 5, 2023
Machine learning
- Machine learning consulting
- Artificial intelligence services
- Predictive analytics consulting
- Agriculture
- Manufacturing
- Anomaly detection
- Stock market prediction
Explore the key use cases, benefits, and adoption best practices of this technology to find out how ML is reshaping the way we buy or sell on the web and why online businesses should consider involving machine learning consultants in their ecommerce projects.
Table of contents
- Market stats
- Case studies
ML in ecommerce: market stats
in revenues by implementing ML-based product recommendation systems
+ $1.7 trillion
the value AI, including ML, is expected to create in the retail industry
McKinsey Insights
in customer satisfaction and revenue achieved by ML in ecommerce
in qualified new site visitors due to leveraging social data and machine learning in retail and ecommerce
Top 10 machine learning use cases in ecommerce
From marketing and customer care to logistics and security, ML in ecommerce is paving the way for innovations in various business functions. Let's find out how machine learning algorithms are applied in this field and what advantages ecommerce companies can get from implementing ML in their operations.
Recommendation engines
ML-based recommendation systems are a staple in all major ecommerce platforms and online stores as they drive upselling. These engines can follow two distinct approaches to provide suggestions. Those relying on content-based filtering check a customer's purchase history and recommend other products with characteristics similar to those already bought. Systems using collaborative filtering, on the other hand, will suggest products that have already been ordered and positively rated by other users with similar buying patterns.
Targeted advertising
Similarly to recommendation systems, this technique embraces customization to drive sales. Potential new customers can be segmented and targeted with tailored ads according to a variety of parameters, like their social media interactions, previous online purchases (including those made in virtual stores), Google search history, and other types of big data used in ecommerce . Then, machine learning algorithms process this information to frame customer behavior and purchase patterns, forecasting the products they may like.
Smart search engines
Traditional search engines integrated into ecommerce stores match the keywords used for the site search and those chosen to describe the products in stock. Enhanced with deep learning-based natural language processing capabilities, however, these tools can achieve way deeper context understanding. For example, an ML-driven engine can take into account a broader range of synonyms. It can also adjust the site search process in real time by prioritizing some results according to each user's purchase habits and taste.
Scheme title: Machine learning-driven smart search engine
Pricing optimization
Several ecommerce platforms and online stores leverage machine learning to optimize their pricing strategies and personalize discounts or other promotions. This technique, usually referred to as dynamic pricing, involves periodic and customized price adjustments (even every few minutes, in the case of Amazon) based on personal user data, pricing history of similar products, sales trends, competitors' offers, demand compared to supply, and more.
Scheme title: Static vs dynamic pricing
Chatbots and virtual assistants
Compared to standard, rule-based bots which can only solve simple queries, modern chatbots powered by natural language processing also understand the context in which they operate and, above all, learn from experience, i.e. from previous interactions with other users. This enables chatbots to maintain a realistic conversation and completely replace humans in a variety of customer support tasks. For example, they can clarify available shipping options, ask questions to understand customer preferences, and offer coupons depending on their responses.
Scheme title: How chatbots operate
Contextual shopping
Another significant example of machine learning in the ecommerce industry, specifically applied to route customers towards products while offering them a more interactive experience, comes from contextual shopping solutions. Powered by machine learning and computer vision technologies , these applications identify and highlight specific products or brands that appear in online videos. Sellers can embed links to the related product page, enabling users to purchase such items without leaving multimedia content.
.jpg)
Image title: A fashion show video with an overlay linking to mentioned products
Trend analysis and restocking
ML can help retailers match supply and demand by predicting upcoming ecommerce sales trends and planning out restocking. This also comes in handy for optimizing the catalog, therefore reducing storage utilization and the risk of food spoilage. The variables considered include economic conditions, seasonality-driven purchase patterns, social media sentiment, product reviews, and ratings. All these data points come from various sources, such as social media, ecommerce websites, or POS solutions.
Scheme title: Data sources for inventory optimization Data source: deloitte.com — The age of with Leveraging AI to connect the retail enterprise of the future
Delivery optimization
Machine learning in retail and ecommerce facilitates product distribution via delivery optimization solutions. They process data from prior cases and suggest the best expedition methods and conditions (such as free or one-day delivery) according to customer expectations. As for actual shipping, the process can be accelerated through machine learning-based route planning. These solutions analyze real-time traffic data, weather conditions, and drivers' experience and performance to recommend the fastest route.
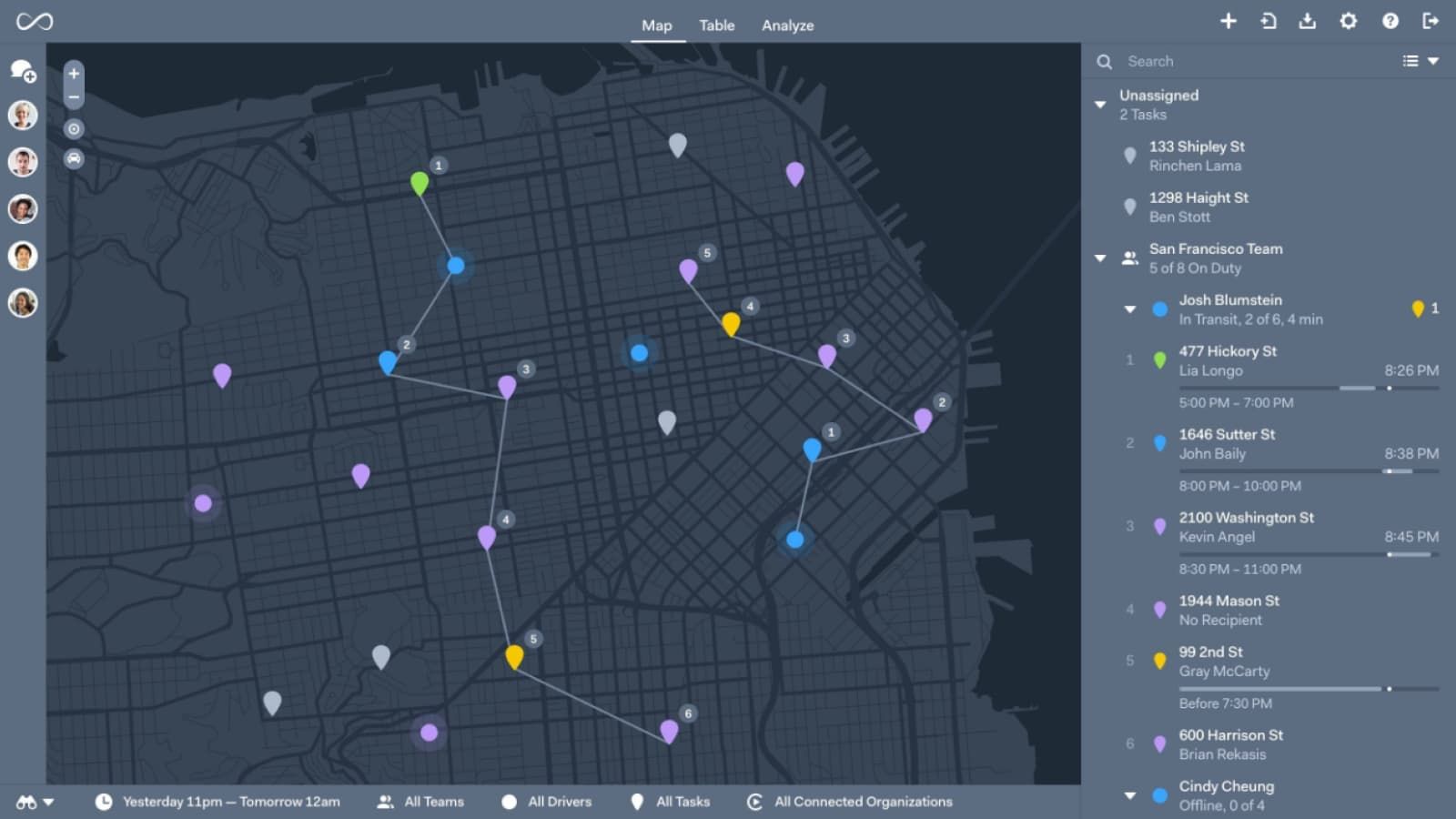
Image title: Onfleet’ route optimization solution Data source: onfleet.com — Power your retail & eCommerce deliveries
Self-driving vehicles
Another embodiment of machine learning aimed at improving product delivery involves deploying self-driving vehicles powered by ML algorithms, deep learning, and computer vision. However, we are still in the realm of experimentation, rather than at a full implementation stage. Still, several pioneering companies like Amazon and Kroger are investing in these technologies with rather promising results, especially to speed up last-mile deliveries and minimize their costs.
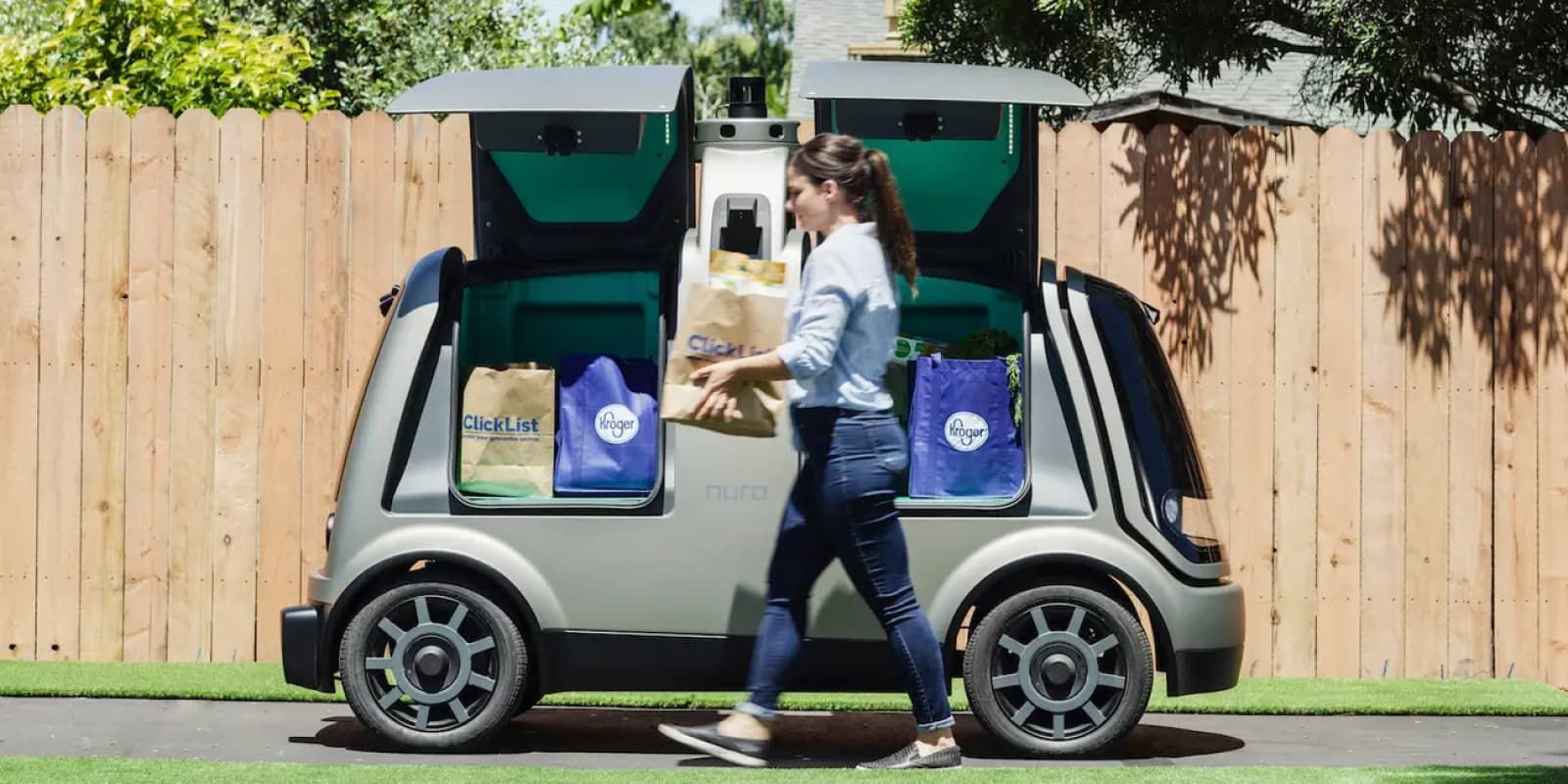
Image title: Kroger’s self-driving vehicle for grocery delivery Data source: businessinsider.com — Kroger has launched self-driving grocery deliveries in Arizona
Fraud detection
Many companies have already turned their gaze to ML as a potential weapon to protect their ecommerce portals from a wide range of criminal actions, including identity theft and fraudulent electronic payments. ML algorithms can spot recurring patterns among the data they process, but also notice if something "breaks the rules". Indeed, ML in fraud detection is commonly deployed to detect anomalies among the credit card accounts under scrutiny (such as an increasing frequency of transactions) that may be signs of fraudulent attempts.
Scheme title: AWS-based fraud detection architecture relying on ML Data source: AWS — Fraud Detection Using Machine Learning
Consult our experts to implement ML the right way
Real-life examples of ml in ecommerce.
Several organizations operating in the industry have already implemented machine learning solutions. Take a look at case studies from major retailers and ecommerce-oriented tech companies.
AiBUY’s shoppable video platform
AiBUY, one of the US leaders in shoppable media technology, partnered with Itransition to enhance its flagship video ecommerce solution with machine learning-based capabilities. The platform integrates CNN-powered image recognition features to detect items across millions of online images and videos and highlight them with customized image overlays. Then, it scans marketing affiliate networks and identifies the closest matching products in their catalogs, enabling viewers to purchase them directly and increasing conversion rates.
eBay’s recommender engine
eBay developed an in-house recommendation system powered by deep neural networks to guide its 150+ million users through 1.5 billion live listings. The engine relies on natural language processing to extract data from item- and user-related textual information and generate embeddings, namely numerical representations of information describing its semantic meaning. Using item-based collaborative filtering, the system matches item and user embeddings to provide suggestions based on past purchases and browsing activity.
.jpg)
Image title: eBay’s recommendation system in action Data source: tech.ebayinc.com — Building a Deep Learning Based Retrieval System for Personalized Recommendations, 2022
Amazon’s anticipatory shipping
Amazon has taken ML-enhanced delivery a step further by implementing an even more innovative "anticipatory shipping" system. The ecommerce leader relies on machine learning algorithms to keep an eye on its customers' purchase habits and online interactions with the platform, forecast their future orders, and transfer these products to a closer warehouse. This means that Amazon will be able to deliver such items in one day and with standard, relatively inexpensive shipping methods as soon as the customer orders them.
Macy’s technology platform
This major American department store chain implemented an extensive tech platform based on Google Cloud to enhance multiple business functions. For instance, Macy’s uses ML-powered predictive analytics to drive its marketing campaigns, which resulted in 10% growth in online sales within the first three months. The company also integrated a recommendation engine and an AI-based virtual agent into its online store. It relied on ML to streamline warehouse management, including inventory allocation, to optimize its pricing strategies.
Anheuser-Busch’s fleet optimization system
To better handle its growing customer network and enhance ecommerce operations, the world's largest brewing company deployed an ML-powered route optimization system across its last-mile teams. The solution processes historical data on traffic patterns, parking information, and weather conditions to calculate the fastest and most cost-efficient routes. Then, it shares such insights (including turn-by-turn navigation and dock locations) with drivers and dispatchers via the app in real time. As a result, the enterprise reduced late deliveries by 80%.
Video title: Anheuser-Busch’s route optimization solution Video source: Wise Systems
Starting with machine learning in ecommerce
When it comes to implementing machine learning in your ecommerce business, you should take into account some general guidelines:
Decide for or against ML
For many companies looking to implement machine learning, the first challenge is deciding whether it’s worth opting for an ML solution (usually more complex and financially demanding) over conventional software. This makes sense if ML adoption delivers good ROI and addresses key inefficiencies that could not be solved with “traditional” technologies. After all, the prospect of a potentially rewarding adoption can foster stakeholders’ and executives’ buy-in despite the cost and complex implementation.
Recommendation
McKinsey highlights the potential of ML in ecommerce for marketing and sales and supply chain management. Accenture, on the other hand, recommends chatbots as a good first investment. All in all, these tools offer a massive boost to the user experience and customer care without requiring huge investments, as you can build your bot on already-existing services and solutions (such as Amazon Lex and Microsoft Bot Framework) or develop one from scratch.
Consider SaaS ecommerce solutions
To enhance your ecommerce site, you can benefit from the embedded ML tools of major online platforms and marketplaces (especially if your business already relies on Amazon, eBay, etc.). Alternatively, you can invest in a fully personalized machine learning-based solution built from scratch. As for the latter case, despite the payoffs of creating proprietary software tailored to your specific needs, you’ll incur large upfront and maintenance costs.
To speed up ML adoption, you can opt for off-the-shelf, ecommerce-oriented SaaS solutions delivered by a cloud provider and offering built-in features for efficient web store management. For instance, platforms like Dynamics 365 Commerce, Adobe Commerce (previously known as Magento), Salesforce Commerce Cloud, SAP Commerce Cloud, and Shopify Plus offer a variety of ML-powered functionalities such as product recommendations and business intelligence.
Complement technology with expertise
Nowadays, most ML-based solutions for customer analytics, pricing optimization, and demand forecasting are carefully designed to foster usability with user-friendly interfaces. Still, your employees can require additional competencies to make the most out of an ML tool’s capabilities. Not to mention, ML-enabled process automation via bots can cause anxiety and resistance to change in your workforce.
You should fill any potential skill gaps and help your staff get familiar with new technologies by investing in corporate training initiatives. Also, consider establishing centers of excellence to coordinate ML adoption and relying on external partnerships to complement your in-house expertise.
Supervise your machine learning models
ML-driven systems suffer from the so-called black box problem, as no one knows exactly how machine learning algorithms and the models they build come to conclusions. This means that a trained machine learning model can behave unexpectedly or provide inaccurate forecasts. For example, a recommendation engine can over-refine until it stops promoting low-selling products completely.
While no ML model can achieve 100% accuracy, training it with good-quality data certainly helps. This means identifying reliable data sources, transforming data sets via ETL processes, and consolidating them in a data lake or data warehouse. This data should be split into training, validation, and test sets to mitigate overfitting issues. Also, monitor your model's operation on the ground and retrain it with newer data sets through multiple iterations according to MLOps best practices to fine-tune its output over time.
Comply with data legislation
Alongside the growing data traffic, the regulations have also tightened significantly, providing an official framework for data protection, not to mention ever-increasing sensitivity among users regarding personal data management and cookie intrusiveness.
Any machine learning solution for ecommerce should be developed in strict compliance with the main ethical standards and legislation applicable to the industry, including GDPR, HIPAA, and PCI DSS. Many online retailers decide to avoid the regulatory burden associated with machine learning implementation and allow ecommerce SaaS providers to handle that.
Partner with Itransition to craft your machine learning solution
Reshaping online shopping with machine learning.
In recent years, artificial intelligence and machine learning in ecommerce has acted as a catalyst for retail digital transformation , enabling businesses to better handle the explosive growth of online shopping in terms of data-driven decision-making , process optimization, and customer experience. However, implementing this technology can take time and effort. To make this journey smoother and avoid missteps, rely on Itransition's experience in ML consulting and development.
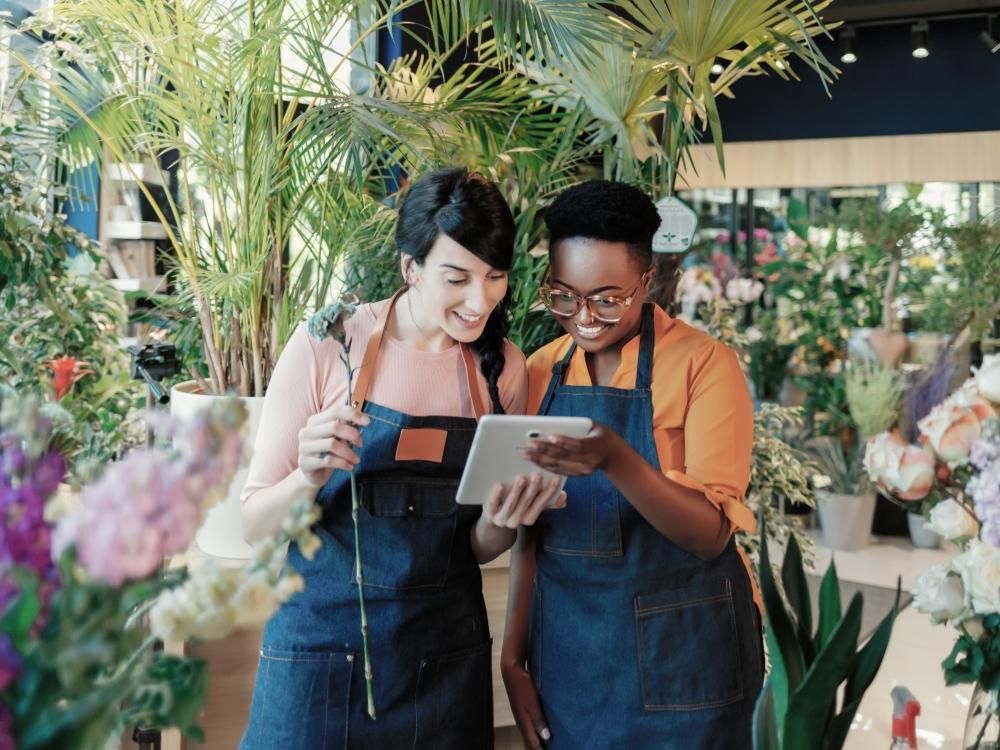
Accelerating ecommerce growth with predictive analytics
In this article, we discuss how ecommerce companies can implement predictive analytics to boost customer loyalty, optimize operations, and increase profits.
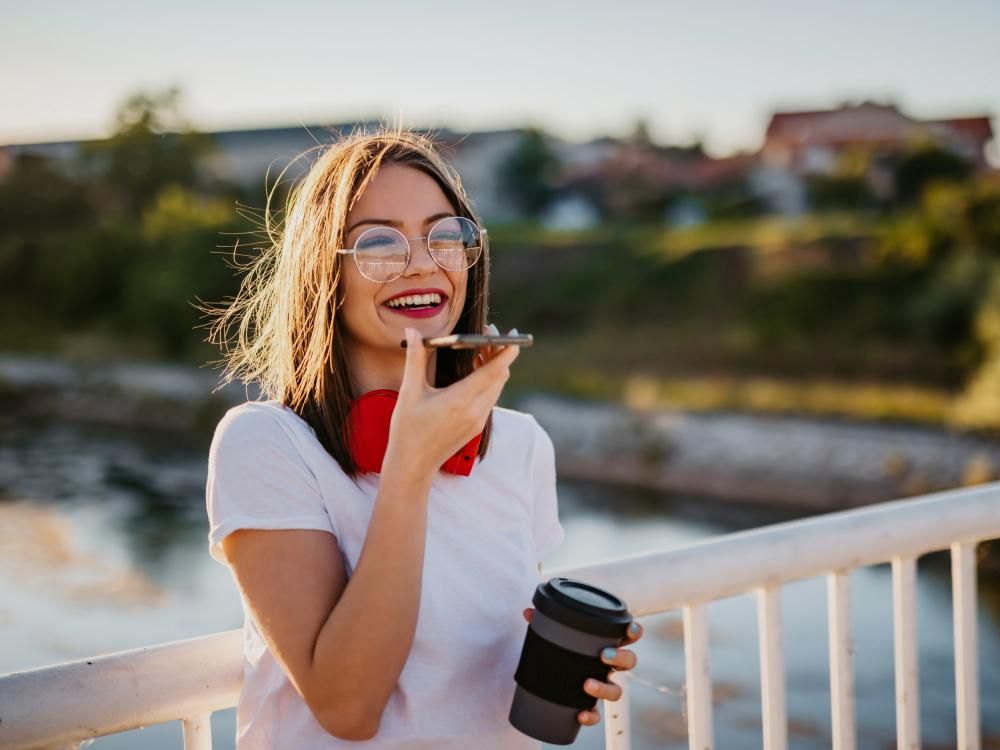
How voice commerce transforms online retail
This article explains the concept of voice commerce, covers its use cases and implementation challenges, and provides some adoption tips.
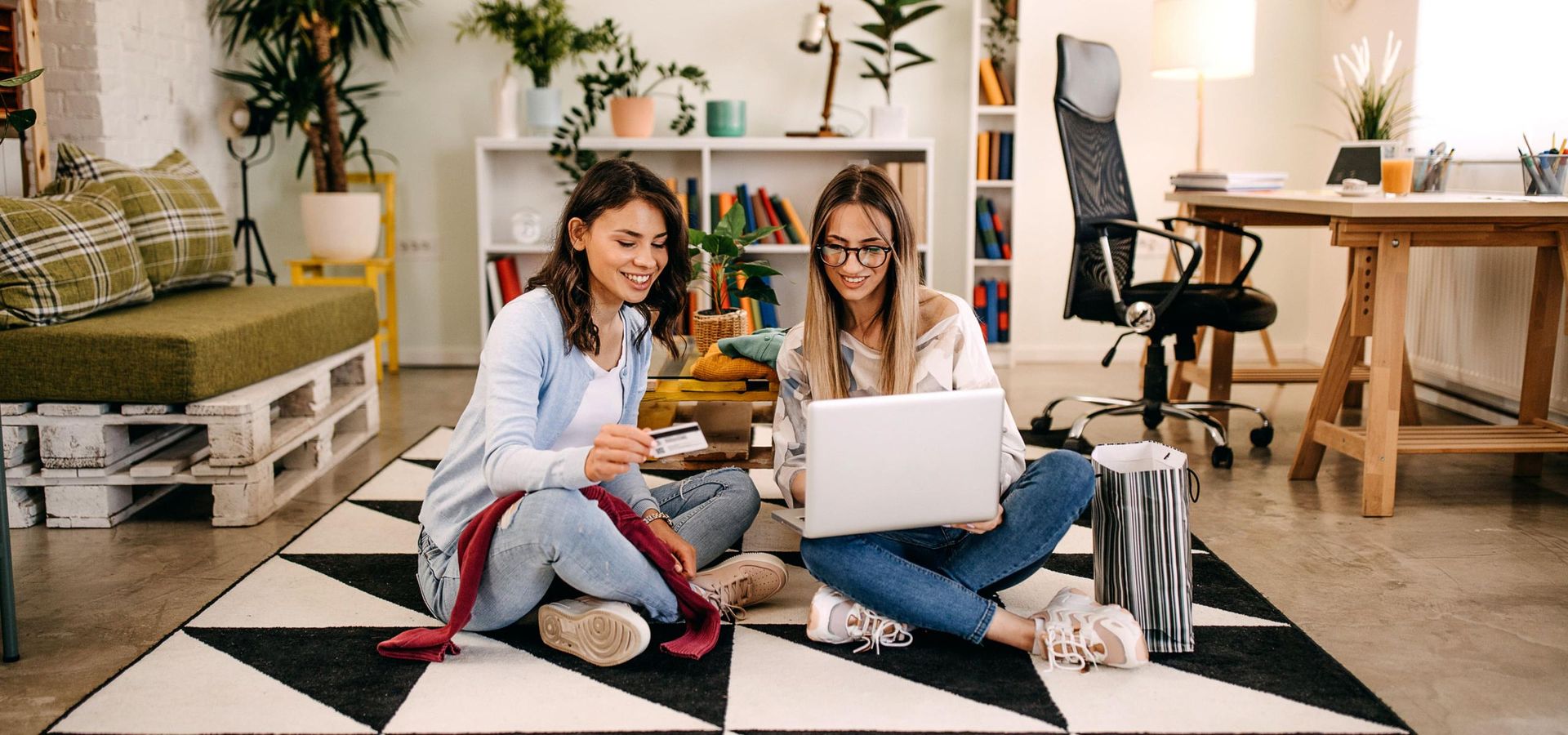
Ecommerce consulting services: key options, platform expertise, and benefits
Discover how retailers can improve their digital commerce strategy and significantly enhance their online store performance with ecommerce consulting services.
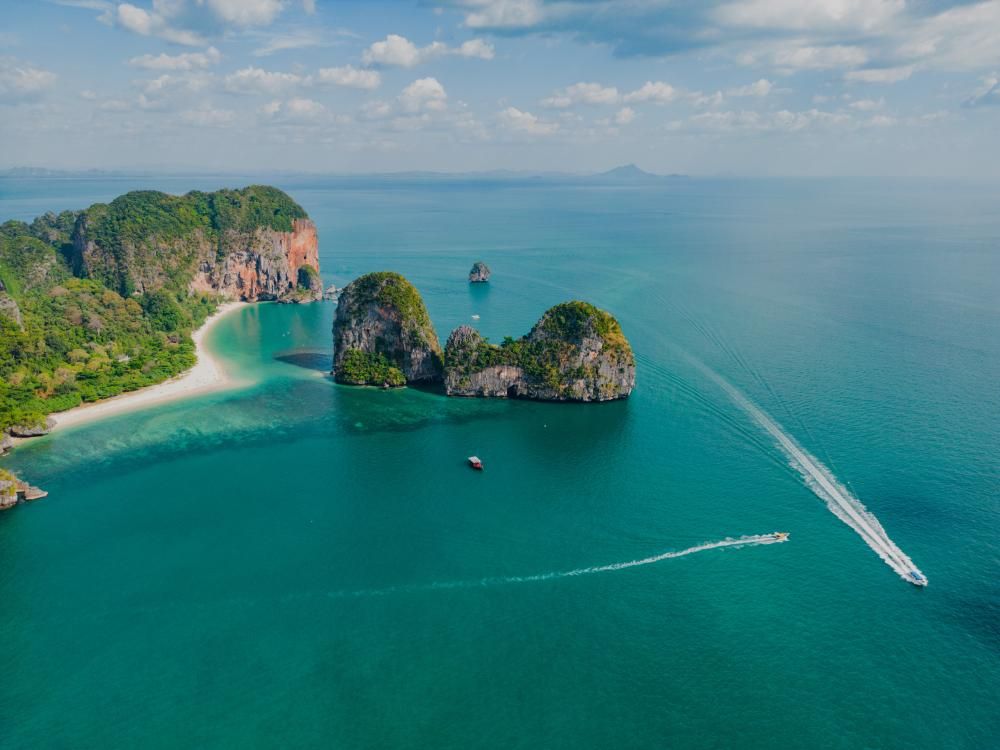
Security for ecommerce: key threats and how to prevent them
Learn about the most pressing security issues and threats for online ecommerce businesses and discover the most effective measures to avert them.
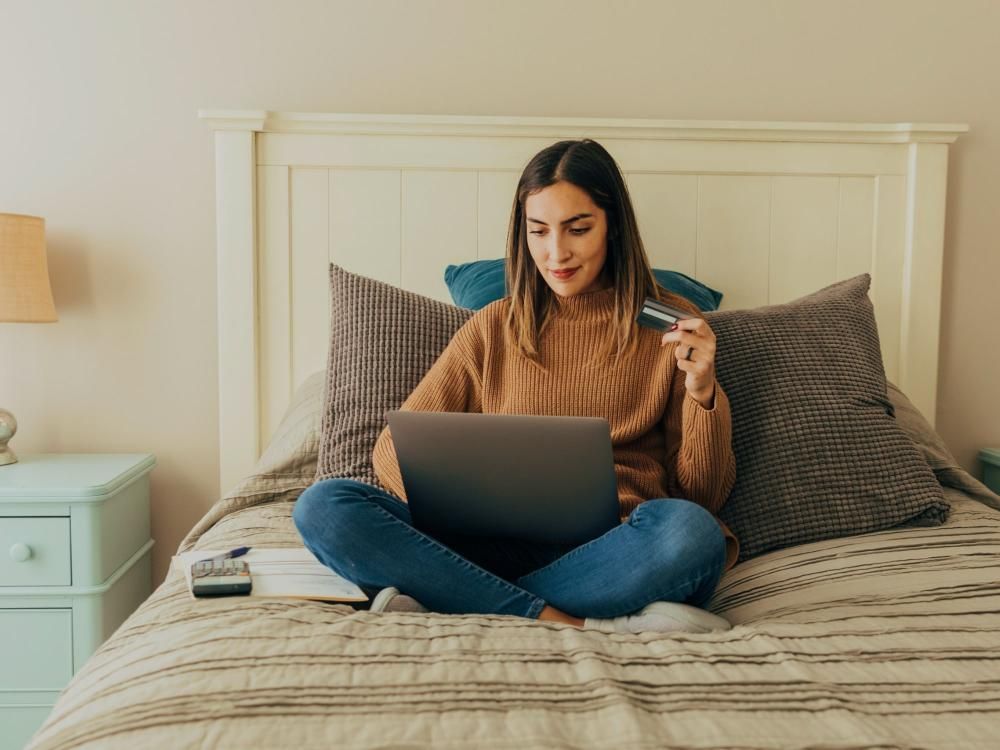
Ecommerce personalization: tactics, examples, and adoption guide
Personalization in ecommerce is indispensable today. Explore powerful tactics for creating unique customer experiences and get practical implementation tips.
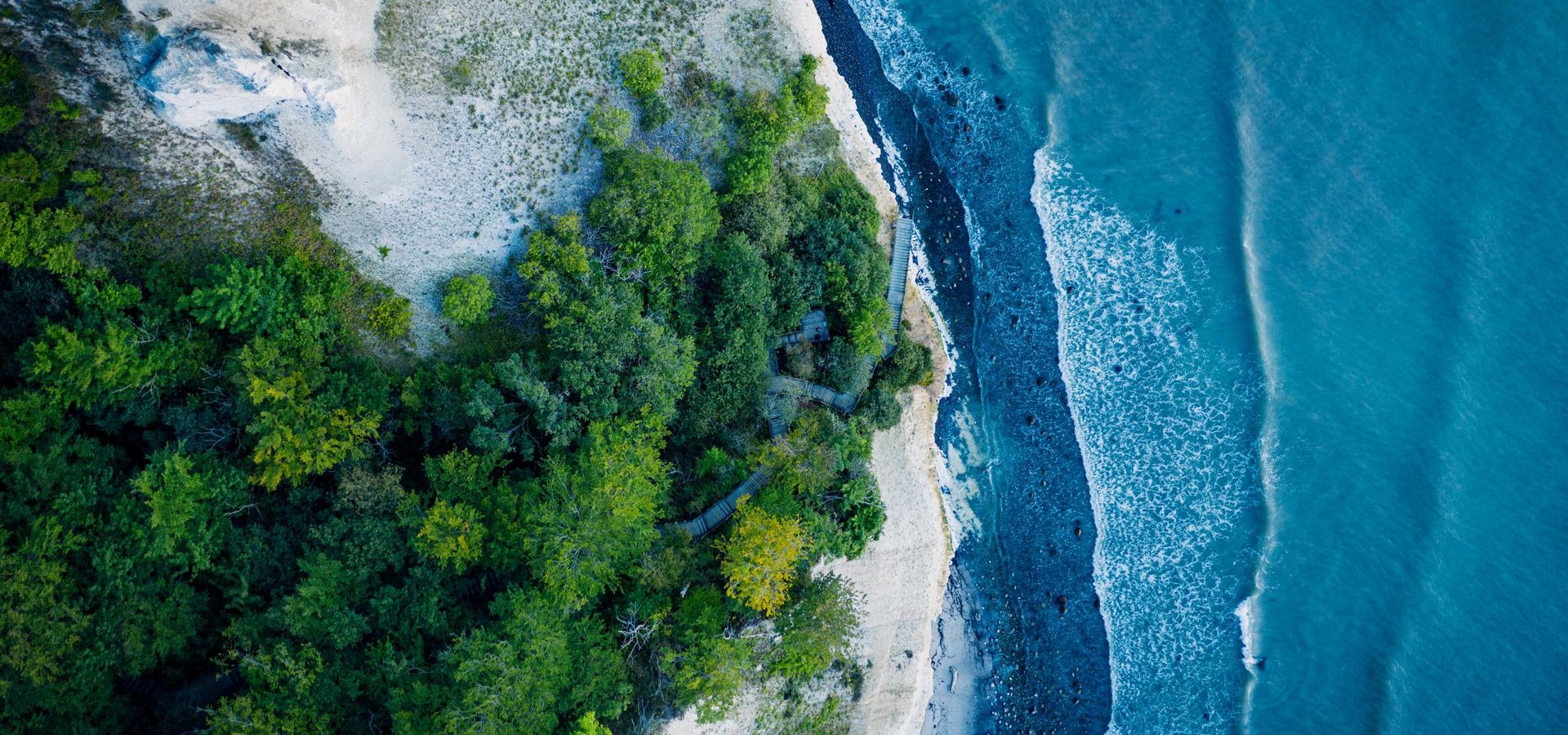
ML services and solutions from certified machine learning experts
Get ML consulting and development services from machine learning experts. Proven expertise in artificial intelligence, machine learning, and data science.
Sales and general inquires
Want to join Itransition?
Please be informed that when you click the Send button Itransition Group will process your personal data in accordance with our Privacy notice for the purpose of providing you with appropriate information.
The total size of attachments should not exceed 10 MB.
Allowed types:
Potential of E-Commerce with Machine Learning: Real-World Use Cases
Understanding the power of machine learning can mean the difference between soaring ahead of the competition or being left in the shadows of obscurity. Welcome to a world where harnessing the potential of data-driven insights and intelligent algorithms holds the promise of transforming your e-commerce endeavors into a resounding success.
Brace yourself as we unravel the vital importance of Machine Learning in E-commerce, revealing how it can truly make or break your journey in the fierce landscape of digital retail. Get ready to discover the strategic weapon that will propel you to the forefront of the competition.
Are you prepared to crack the code? Let’s embark on this transformative journey together!
Introduction to Machine Learning in E-Commerce
In today’s rapidly evolving digital landscape, technology continues to reshape the way businesses operate, and machine learning stands at the forefront of this transformative journey. In the context of e-commerce, machine learning opens up a world of possibilities, offering invaluable opportunities for businesses to optimize their operations, elevate customer experiences, and gain a competitive edge.
What is Machine Learning and What Can It Do For E-Commerce?
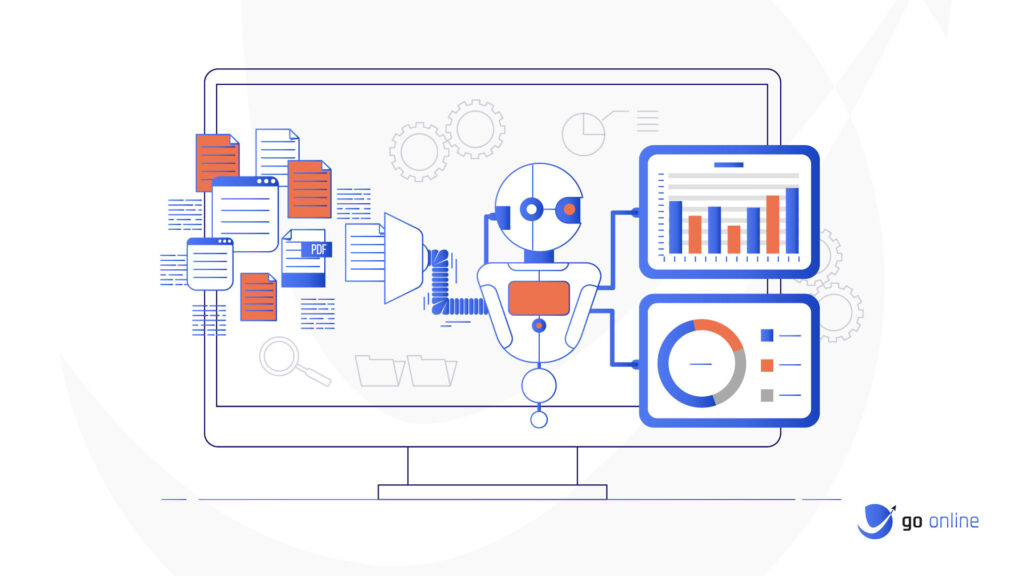
At its core, machine learning is a subset of artificial intelligence that enables computer systems to learn from data patterns and experiences, without the need for explicit programming.
It’s like having an intelligent assistant that can identify hidden insights from vast datasets, predict customer behavior, and make informed decisions based on the information it learns.
Now, you might wonder, “What does this mean for the e-commerce industry?” Well, it means a myriad of exciting prospects that can revolutionize the way e-commerce businesses function and engage with their customers.
5 benefits Of Using Machine Learning In E-Commerce
In the ever-changing digital landscape, machine learning takes the lead as a transformative technology reshaping industries, including e-commerce. Embracing the power of machine learning, online retailers can tap into a world of possibilities, streamlining operations and providing exceptional customer experiences.
From tailored product recommendations and boosted conversion rates to precise and accurate customer segmentation,, reliable demand forecasting, and fortified fraud detection and customer service automation, machine learning unlocks a myriad of advantages, propelling e-commerce businesses to thrive in a fiercely competitive market.
Enchanced Personalization
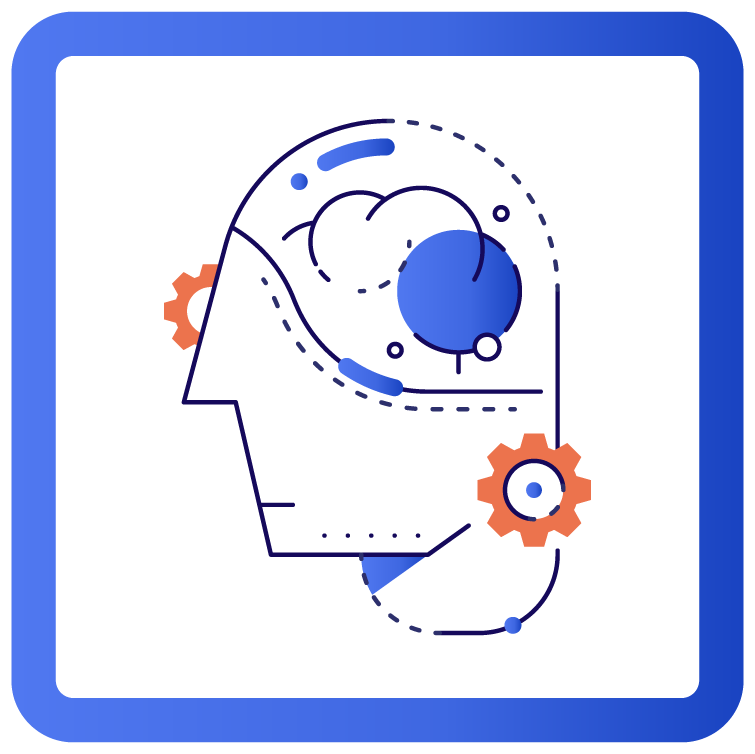
Imagine stepping into a brick-and-mortar store, and the salesperson knows your preferences, likes, and dislikes, providing tailored recommendations just for you. Machine learning brings this level of personalized experience to the digital realm.
By analyzing customer data, such as browsing history, purchase behavior, and demographics, e-commerce platforms can offer highly personalized product recommendations, content, and promotions.
This enhances customer engagement and loyalty, leading to increased sales and repeat business.
Increased Conversion
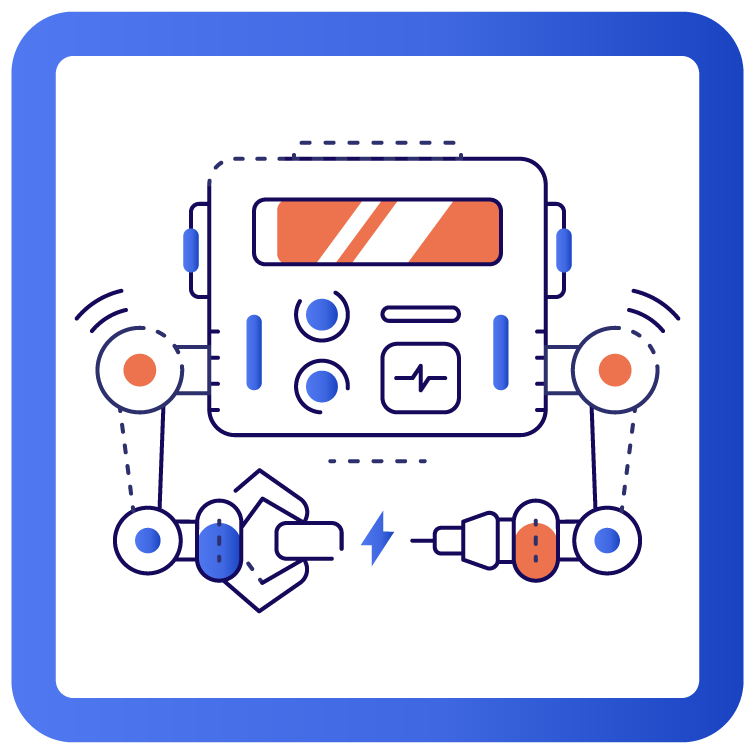
Turning website visitors into customers is a key challenge for e-commerce businesses. Machine learning comes to the rescue by identifying patterns that influence purchase decisions.
By understanding customer behavior and preferences, e-commerce platforms can optimize their website design, product positioning, and marketing strategies, leading to higher conversion rates and improved sales performance.
Efficient Customer Segmentation
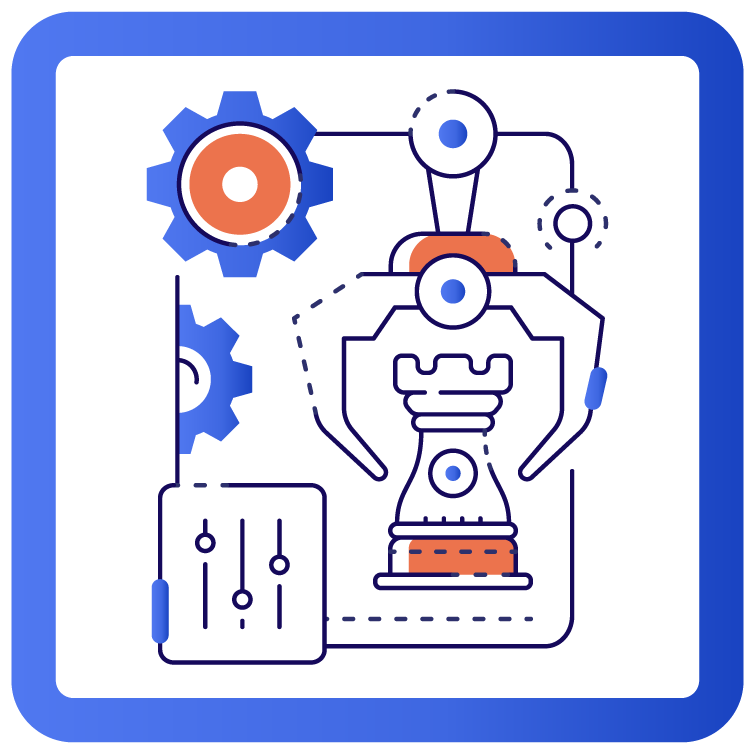
Gone are the days of one-size-fits-all marketing strategies. Machine learning empowers businesses to do more accurate customer segmentation of their customer base more effectively and in real-time. By categorizing customers based on their behaviors and preferences, businesses can target them with personalized marketing campaigns, tailored offers, and relevant content.
This level of segmentation enhances customer engagement and retention, ultimately driving business growth.
Demand Forecasting
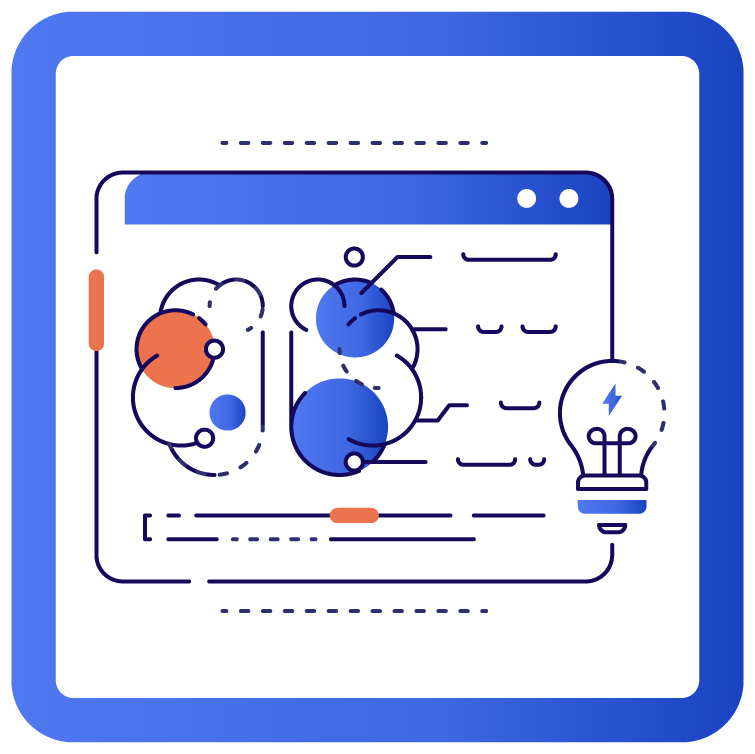
Accurate demand forecasting is a critical aspect of e-commerce operations. Machine learning models analyze historical sales data, seasonal trends, and external factors to predict future demand more accurately.
This foresight enables online businesses to to manage inventory efficiently, optimize pricing strategies, and ensure they meet customer demands effectively.
Fraud DetectionCustomer Service Automation
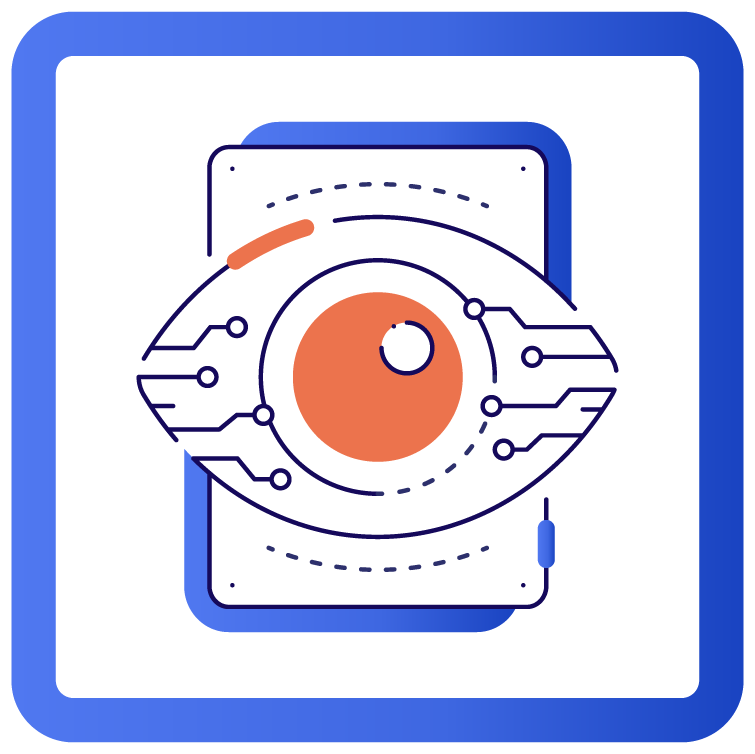
E-commerce businesses often face the challenge of fraud and security threats. Machine learning algorithms act as vigilant guardians by analyzing the transaction data, patterns and user behavior to detect potential fraudulent activities in real-time.
Additionally, machine learning enables customer service automation through chatbots and virtual assistants, providing round-the-clock support and efficient issue resolution.
Key Takeaways:
- Machine learning enables e-commerce platforms to offer personalized experiences to customers.
- Optimizing conversion rates is possible through machine learning-driven data analysis.
- Efficient customer segmentation allows businesses to target customers with personalized marketing strategies.
- Accurate demand forecasting helps businesses manage inventory and optimize pricing effectively.
- Machine learning aids in fraud detection and automates customer service for better efficiency.
Key Use Cases for Machine Learning in E-Commerce
We all know that staying ahead of the competition is the name of the game, and with machine learning, we’ve got the ace up our sleeve. It’s like having an intelligent ally that decodes massive amounts of data, making brilliant moves and insightful decisions, propelling us towards greatness. This powerful innovation is revolutionizing how we sell products, engage customers, and navigate the digital terrain.
From crafting personalized recommendations and finely-tuned marketing strategies to orchestrating seamless inventory management and unearthing fraudulent activities, machine learning is the fuel propelling us to unparalleled success in the e-commerce universe.
In this journey of possibilities, we explore the key use cases where machine learning transforms the ordinary into the extraordinary, creating a remarkable era of efficiency and innovation. So, fasten your seatbelts as we embark on an inspiring voyage to revolutionize e-commerce like never before!
Product Recommendations
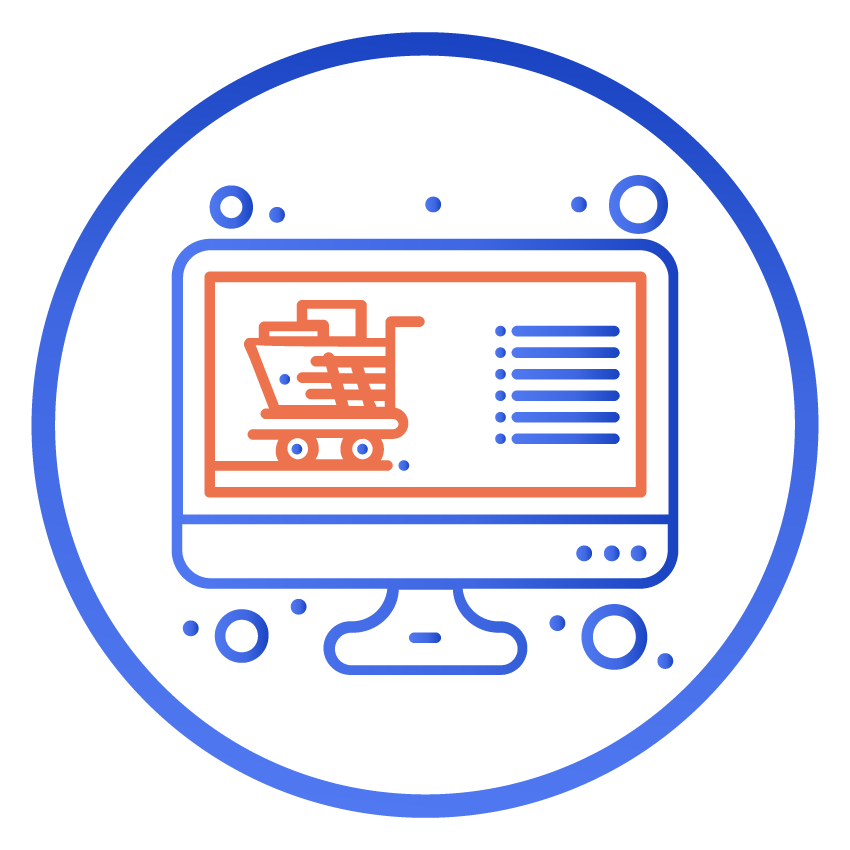
Machine learning revolutionizes product recommendations by understanding each customer’s preferences and behaviors.
By analyzing vast amounts of data, including past purchase history, browsing patterns, and interactions with the website, machine learning algorithms can suggest relevant products to customers.
This personalized approach enhances the online shopping experience, increases the likelihood of making a purchase, and fosters customer loyalty. Whether it’s displaying “Recommended for You” sections or offering complementary products during checkout, product recommendations powered by machine learning create a seamless and engaging online shopping journey.
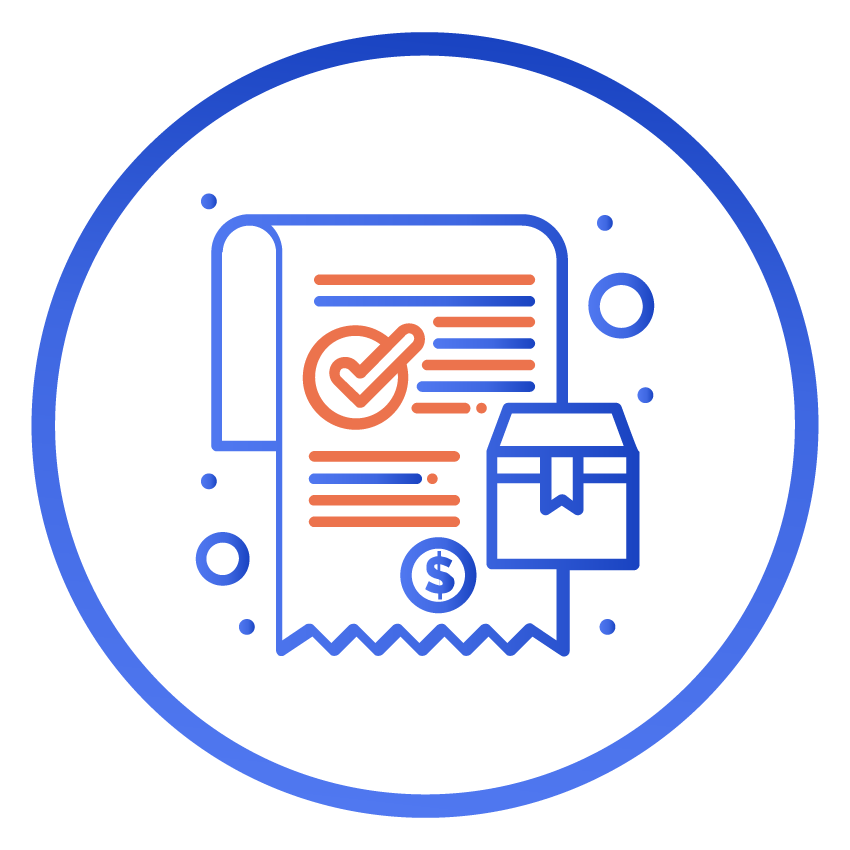
Accurate demand forecasting is critical for e-commerce businesses to optimize inventory levels and meet customer expectations. Machine learning plays a crucial role in this regard by analyzing historical sales data, seasonal patterns, and external factors such as promotions and holidays.
By predicting future demand more accurately, these ecommerce businesses can ensure they have the right products in stock, minimize stockouts, and reduce excess inventory.
This not only saves costs but also improves customer satisfaction by delivering products promptly.
Customer Segmentation
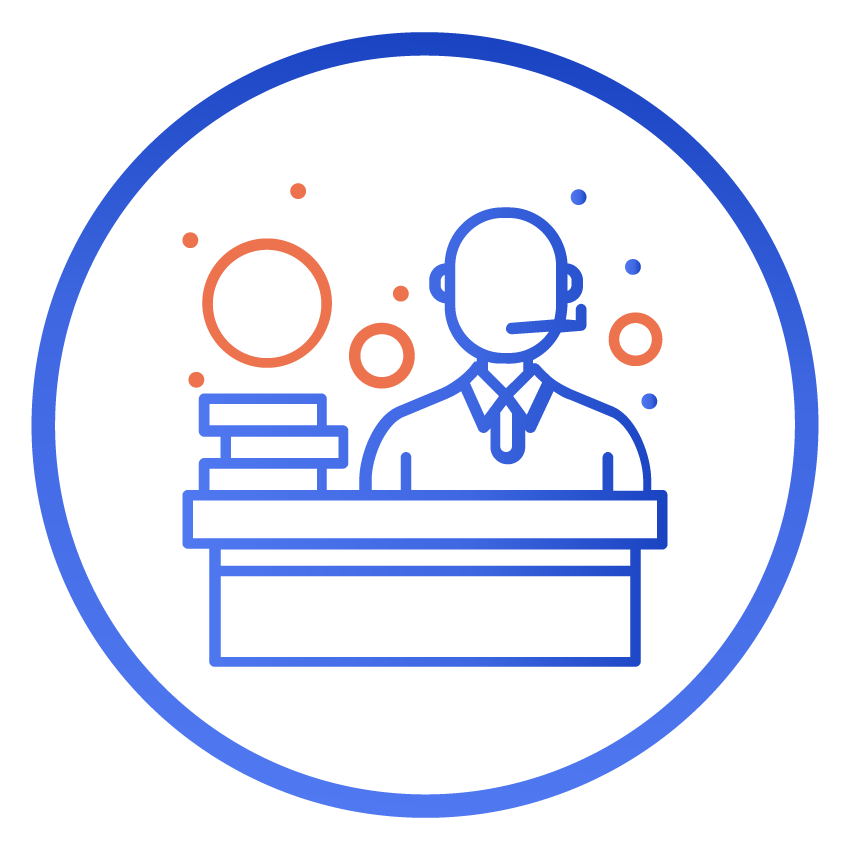
Understanding and segmenting customers based on their behaviors and preferences is vital for targeted marketing and personalized communication.
Machine learning algorithms can group and identify customers with similar characteristics, enabling businesses to tailor their marketing strategies to each segment.
This level of segmentation allows for targeted promotions, personalized content, and relevant offers, leading to higher engagement and conversion rates. Whether it’s segmenting existing customers, based on purchase history or browsing behavior, machine learning empowers businesses to deliver the right message to the right audience.
Fraud Detection
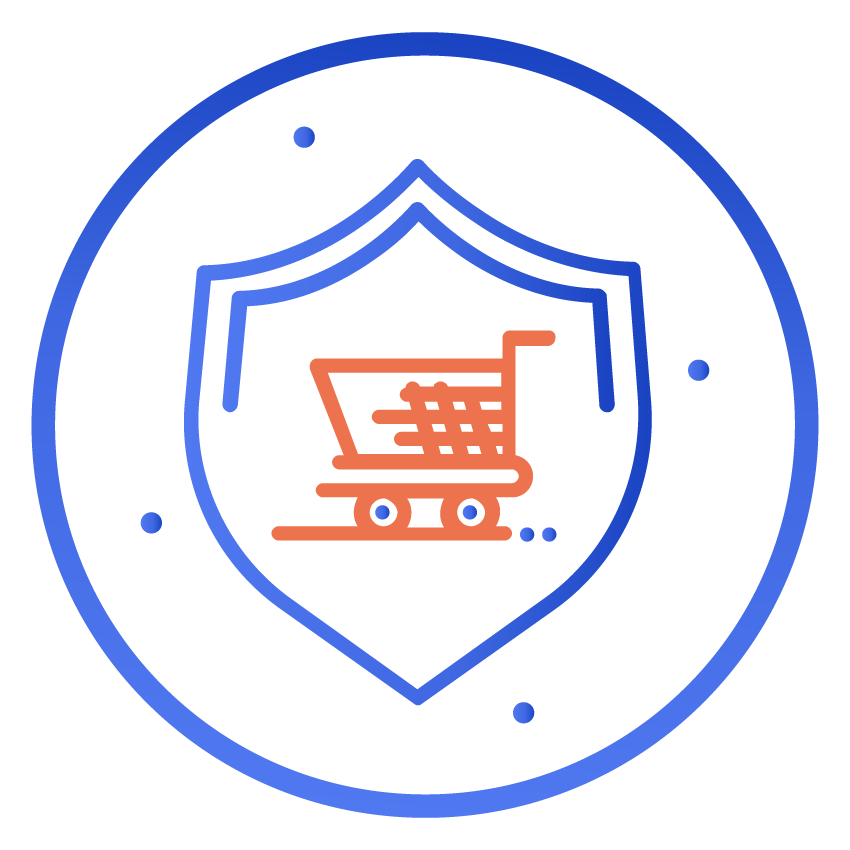
E-commerce platforms are susceptible to fraudulent activities, which can cause significant financial losses and damage customer trust. Machine learning serves as a vigilant guardian by continuously monitoring transactions and user behavior to detect anomalies indicative of fraud.
By analyzing patterns and trends, machine learning algorithms can identify suspicious activities in real-time and trigger security measures to prevent fraudulent transactions.
This proactive approach enhances the an ecommerce platform’s security and protects both businesses and customers from potential threats.
Customer Service Automation
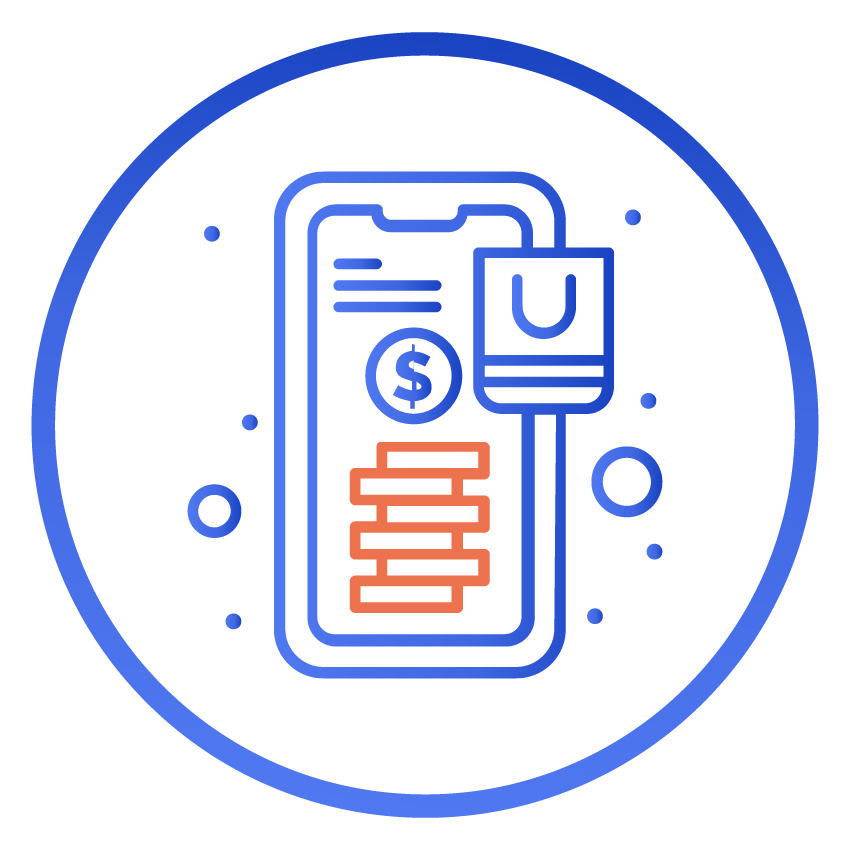
Efficient and responsive customer service is essential for building strong relationships with customers. Machine learning enables businesses to automate customer service through chatbots and virtual assistants.
These AI-driven systems can handle routine queries, provide instant responses, and even assist with order tracking and returns.
By automating these tasks, businesses can significantly reduce response times, improve customer satisfaction, and free up human agents to focus on more complex issues and interactions.
Price Optimization
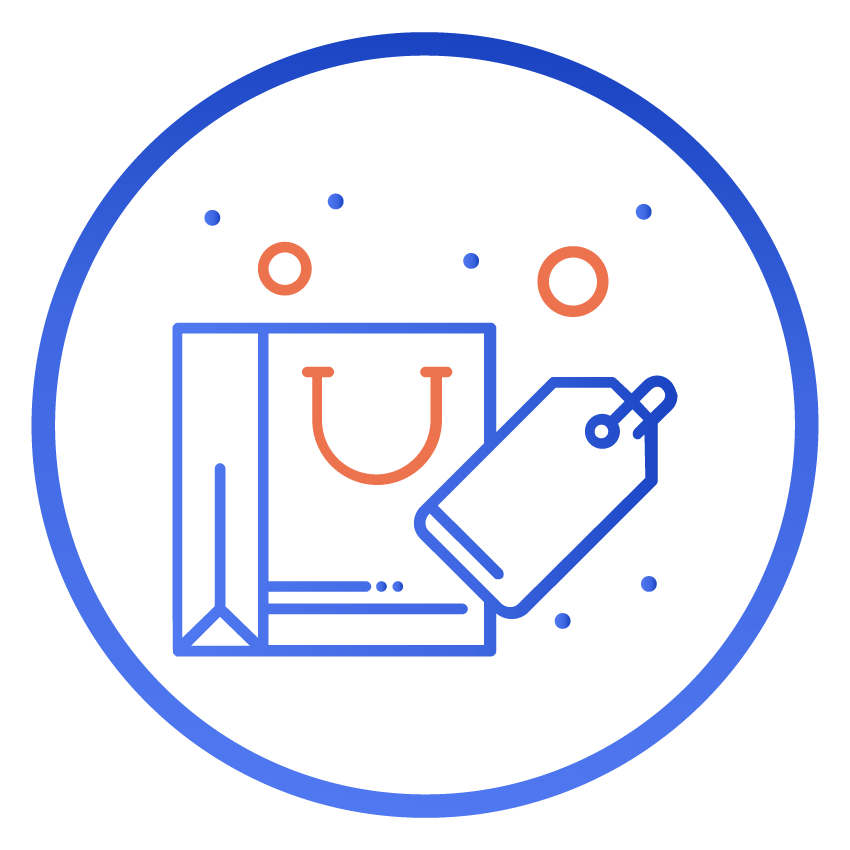
Setting the right prices is crucial for attracting customers while ensuring profitability. Machine learning can optimize pricing strategies by analyzing various factors, including competitor prices, demand elasticity, and customer behavior.
Dynamic pricing algorithms adjust prices in real-time based on these insights, allowing businesses to respond to market fluctuations and increase revenue. This data-driven pricing strategy ensures competitiveness while maintaining profitability in a dynamic market.
Incorporating machine learning in these key use cases empowers e-commerce businesses to deliver personalized experiences, optimize operations, and stay ahead of the competition in a rapidly evolving digital landscape.
Examples of Machine Learning Use Cases in E-Commerce
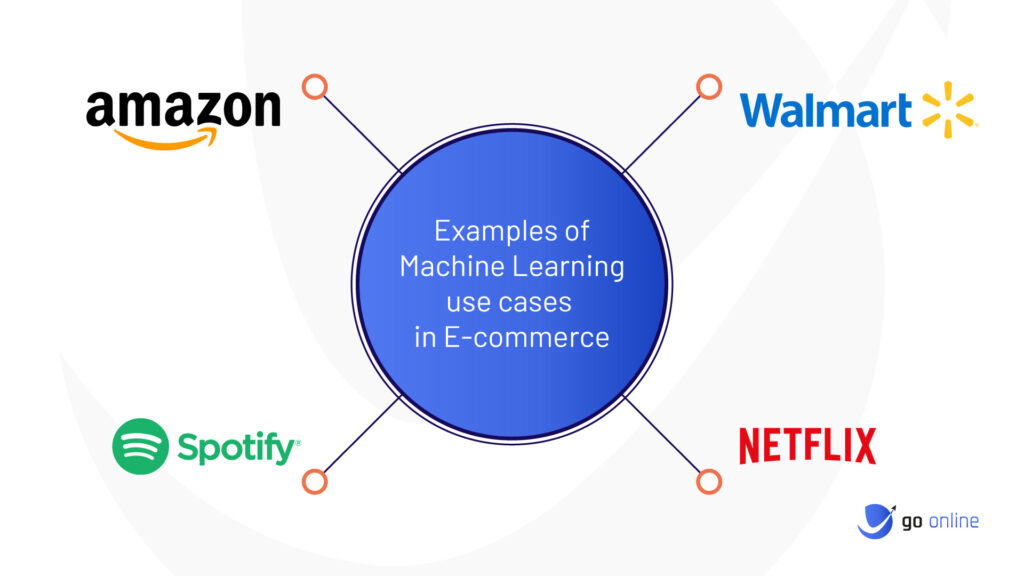
In the ever-evolving landscape of e-commerce, businesses are continuously seeking innovative solutions to elevate their operations, enhance customer experiences, and drive growth. Amidst this pursuit, machine learning emerges as a transformative force, leveraging the potential of data-driven insights and intelligent algorithms to revolutionize the way e-commerce operates.
In this section, we embark on a journey to explore real-world examples of machine learning use cases in e-commerce. These tangible and practical applications illustrate how machine learning empowers businesses to optimize key processes, anticipate customer needs, and craft personalized interactions. By understanding these remarkable use cases, we gain insight into how machine learning shapes the future of online retail, paving the way for unparalleled success.
From enhancing cross-selling opportunities to preventing stockouts, and from optimizing customer segmentation to improving fraud detection, we delve into the specific companies that have harnessed the power of machine learning to create a significant impact in the e-commerce landscape.
Join us as we uncover the innovative strategies employed by leading e-commerce giants to transform their businesses through machine learning. From Amazon’s intelligent product recommendations to Walmart’s proactive inventory management, these examples exemplify the limitless potential of machine learning in revolutionizing e-commerce practices.
Amazon: Enhancing Cross-Selling with Product Recommendations
Amazon, the global e-commerce giant, is renowned for its personalized shopping experience driven by machine learning algorithms. One of their most prominent machine learning use cases is enhancing cross-selling across online stores through product recommendations. When customers browse or purchase a specific product, Amazon strategically displays related or complementary items as suggestions.
For example, when a customer adds a camera to their cart, Amazon’s recommendation engine may showcase relevant accessories like lenses, tripods, or camera bags. By leveraging this smart cross-selling approach, Amazon has seen a substantial increase in average order values and revenue. Customers appreciate the convenience of finding complementary products, while Amazon benefits from increased sales and heightened customer engagement.
Walmart: Preventing Stockouts with Demand Forecasting
Walmart, a global retail giant, utilizes machine learning-based demand forecasting to optimize inventory management and prevent stockouts. With thousands of stores and a vast array of products, efficiently managing inventory is a complex challenge. Walmart’s machine learning algorithms analyze historical sales data, seasonal trends, and external factors like weather patterns and events to accurately predict future demand.
By having a clear understanding of customer preferences and anticipated demand, Walmart ensures that popular products are readily available on their shelves, even during peak seasons or special events. This proactive approach not only maximizes sales opportunities but also enhances customer satisfaction by reducing instances of out-of-stock items.
Spotify: Personalizing Music Recommendations
Although primarily known as a music streaming service, Spotify has a thriving e-commerce component through its personalized music recommendations. Spotify’s machine learning algorithms analyze user listening behavior, genres, and favorite artists to curate personalized playlists and recommend new music.
By using machine learning to understand individual music preferences, Spotify provides a highly personalized and enjoyable listening experience. This not only keeps users engaged with the platform but also encourages them to explore new music and discover emerging artists. As a result, Spotify benefits from increased user retention and a competitive edge in the crowded music streaming industry.
Netflix: Personalizing Movie and TV Show Recommendations
Netflix, the popular video streaming platform, relies heavily on a machine learning algorithm to deliver personalized movie and TV show recommendations to its subscribers. By analyzing user viewing history, movie ratings, social media data, and interactions with the platform, Netflix’s machine learning algorithms create personalized content feeds for each user.
Through this approach, Netflix keeps its vast user base engaged with a curated selection of content tailored to individual preferences. The success of Netflix’s personalized recommendations has been a key driver of its customer retention and global expansion.
Incorporating machine learning in these real-life examples has empowered companies like Amazon, Walmart, Spotify, and Netflix to deliver personalized experiences, optimize operations, less customer churn, and gain a competitive advantage in the ever-evolving world of e-commerce.
What Does This Mean?
In conclusion, machine learning stands as a game-changer in the e-commerce industry, presenting boundless opportunities to elevate businesses to new heights of success. Its transformative impact is evident in the seamless personalized experiences it delivers to customers, resulting in higher conversion rates and increased loyalty.
By harnessing the power of data-driven insights, businesses can optimize their operations, anticipate market demands, and fortify their fraud prevention measures.
The exploration of real-world machine learning use cases in e-commerce showcases the tangible and practical benefits this technology brings to the table. From precision product recommendations to proactive inventory management, machine learning empowers businesses to make informed decisions and stay one step ahead of the competition.
As we delve into these success stories, we gain invaluable insights into how machine learning is reshaping the landscape of online retail, unlocking the potential for unprecedented achievements.
How Amazon Utilized Machine Learning And What Lessons You Can Learn From Them?
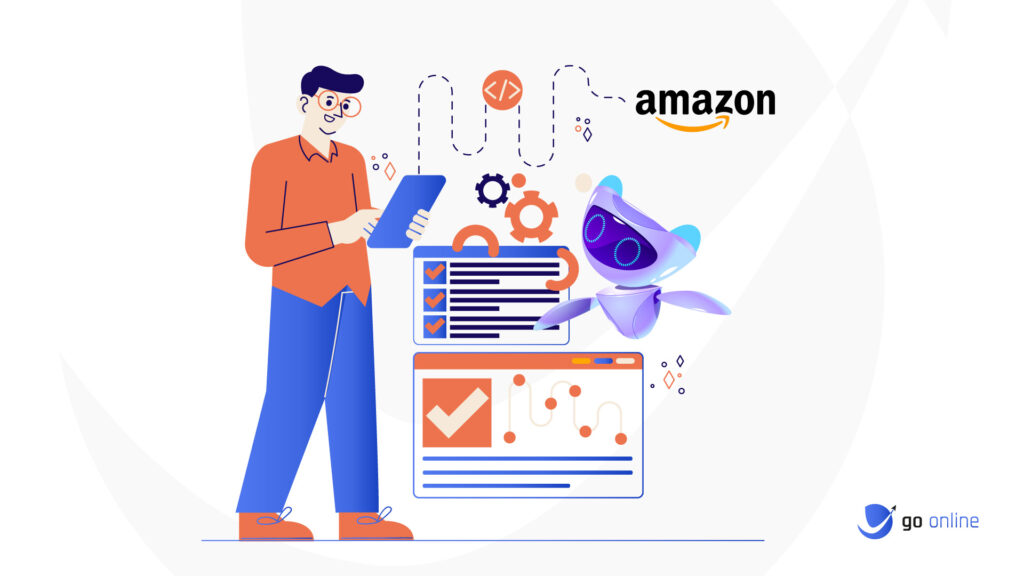
In this section, we delve into a real-life case study showcasing the remarkable impact of machine learning at Amazon, the world’s leading online marketplace.
We will explore how Amazon harnessed the power of machine learning to address key challenges and revolutionize the e-commerce landscape. From personalized product recommendations to demand forecasting and fraud detection, Amazon’s innovative use of machine learning exemplifies the transformative potential of this technology.
Case Study: Amazon – Pioneering Personalization in E-commerce
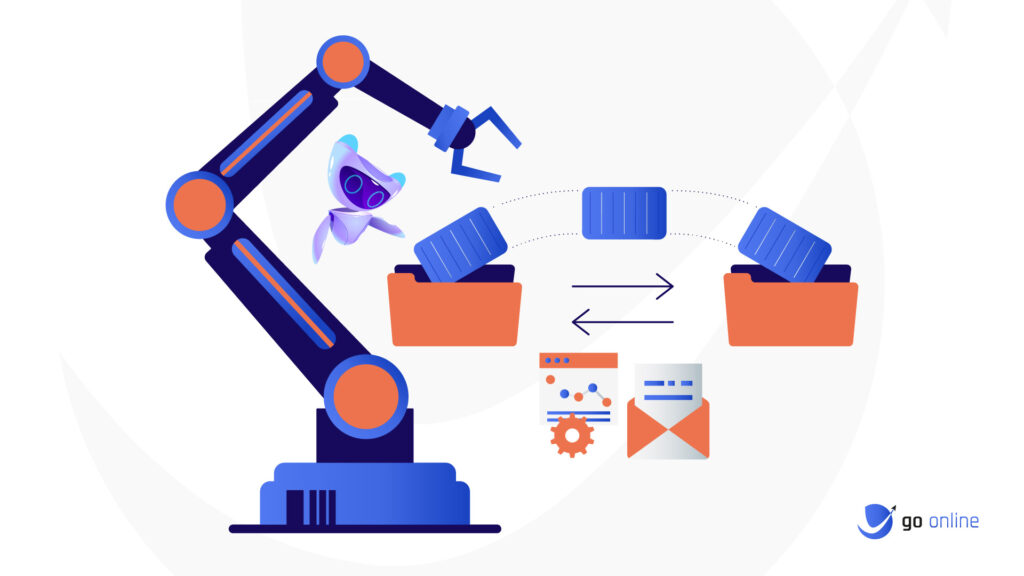
Amazon, the e-commerce giant, faced immense challenges in personalizing the shopping experience for its vast customer base. With millions of products and diverse customer preferences, manually curating tailored product recommendations for each user was an overwhelming task.
Additionally, optimizing inventory management to prevent stockouts and overstocking while meeting customer demand required precise and data-driven strategies.
To address these challenges and maintain their position as an industry leader, Amazon recognized the need to embrace machine learning-driven solutions.
Machine Learning Implementation and Effects Discussing the Motivation for Implementing Machine Learning and Setting Business Goals
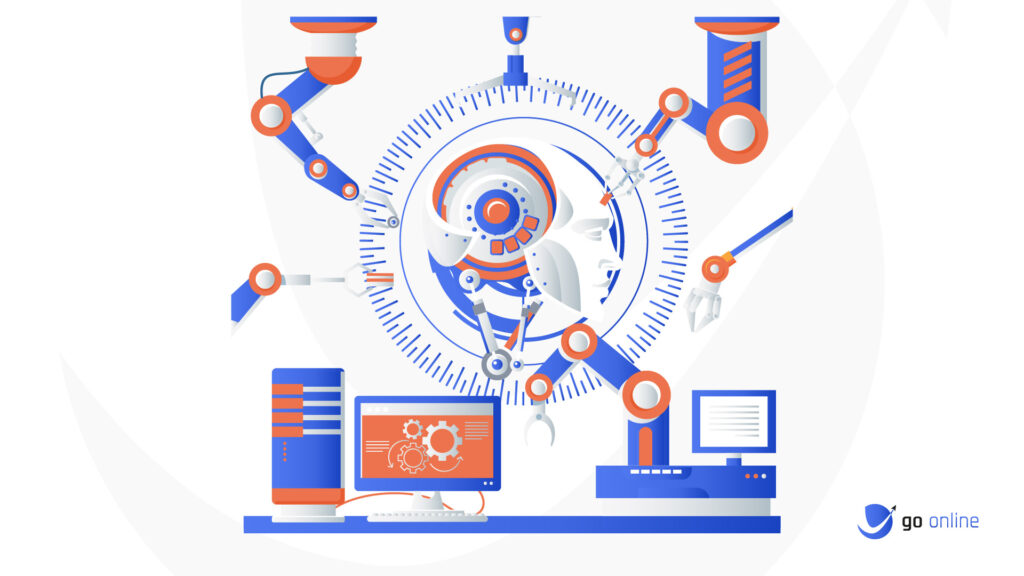
Motivated to elevate customer experiences, improve operational efficiency, and drive revenue growth, Amazon embarked on an ambitious journey to implement machine learning across their e-commerce operations.
The business goals were set as follows:
- Enhanced Personalization: Leverage machine learning algorithms to analyze customer behavior, purchase history, and browsing patterns to deliver highly personalized product recommendations.
- Efficient Demand Forecasting: Utilize machine learning insights to accurately forecast customer demand, optimizing inventory management and minimizing stockouts.
- Robust Fraud Detection: Implement machine learning algorithms to proactively detect and prevent fraudulent activities, safeguarding both Amazon and its customers.
Description of the Machine Learning Implementation Process
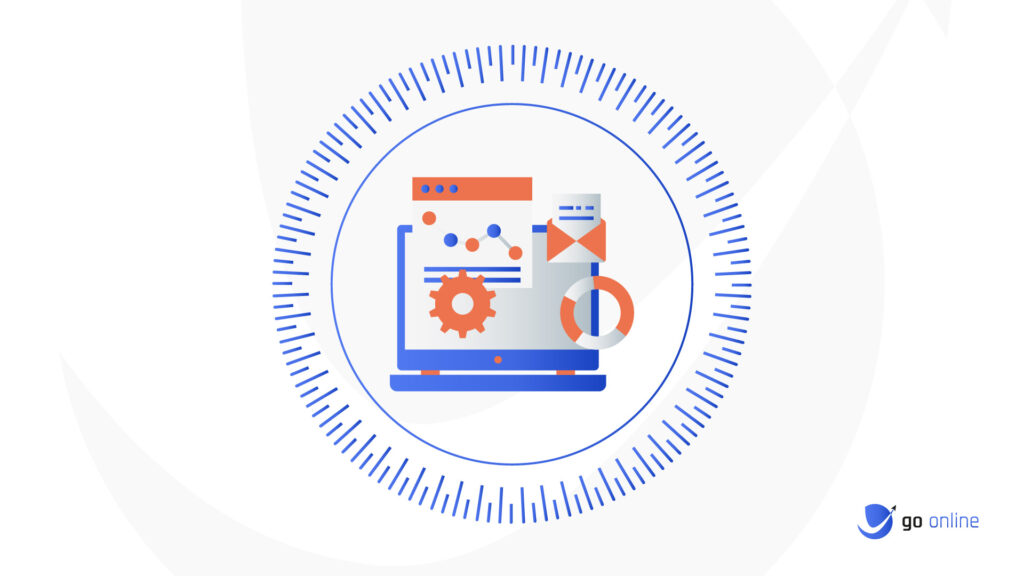
Amazon initiated the machine learning implementation by consolidating and processing vast amounts of customer data from diverse sources. Leveraging cloud-based infrastructure, they streamlined data storage and processing for real-time insights.
For personalized product recommendations, Amazon employed collaborative filtering and deep learning models to understand user preferences and suggest relevant products. Demand forecasting involved time series analysis and regression models to predict future demand based on historical data and external factors.
To address fraud detection, Amazon utilized anomaly detection algorithms and machine learning-based pattern recognition to identify suspicious activities and take preventive measures.
Presentation of the Effects of the Implementation
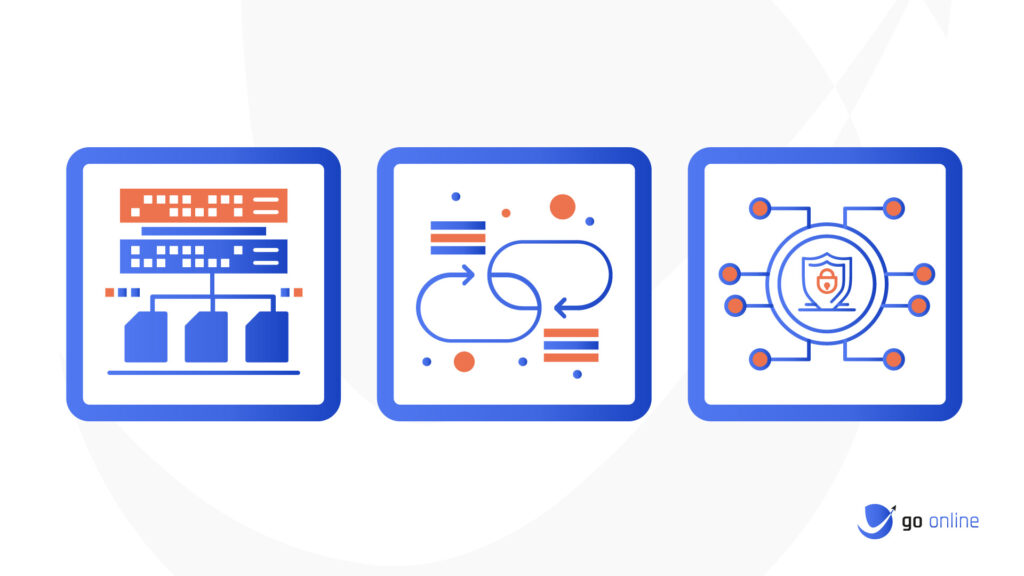
The implementation of machine learning had a profound and transformative impact on Amazon’s e-commerce operations, revolutionizing the way the company interacted with its customers and managed its business processes. Here are some key aspects where the effects were particularly remarkable:
1. Increased Personalization:
With machine learning-powered product recommendations, Amazon witnessed a substantial increase in customer engagement and satisfaction. Customers enjoyed a tailored shopping experience, resulting in higher conversion rates and increased customer loyalty.
2. Efficient Demand Forecasting:
By using data science for accurately predicting customer demand, Amazon significantly improved inventory management. They minimized stockouts and overstocking, leading to reduced costs and improved customer satisfaction.
3. Robust Fraud Detection:
Machine learning-driven fraud detection systems helped Amazon proactively identify and prevent fraudulent activities. This proactive approach protected both the company and its customers, enhancing trust and security.
To wrap up, Amazon’s case study showcases the immense potential of machine learning in driving significant improvements across e-commerce operations. By strategically implementing a machine learning solution, businesses can enhance personalization, optimize demand forecasting, and fortify security, ultimately positioning themselves as leaders in the competitive e-commerce landscape. Embracing machine learning is not just a technological advancement; it’s a transformative tool that empowers all ecommerce companies to thrive and deliver exceptional shopping experiences to their customers.
Machine learning technology has revolutionized the e-commerce industry, offering numerous opportunities to enhance customer experiences and drive business success. By analyzing vast amounts of data, machine learning algorithms enable personalized shopping recommendations, optimized search engines, and efficient fraud detection, fostering trust and loyalty among customers.
This technology also facilitates demand forecasting, enabling businesses to manage inventory effectively and prevent stockouts during peak periods. As a result, machine learning has become a cornerstone for e-commerce growth and competitiveness.
Furthermore, machine learning’s impact on ecommerce sales extends beyond customer-facing aspects, permeating backend operations and supply chain management. With the ability to analyze complex data sets, machine learning optimizes logistics and inventory management, ensuring seamless order fulfillment and minimizing delivery times.
Additionally, it aids in dynamic pricing strategies, allowing e-commerce businesses to adapt to market fluctuations and competitor pricing in real-time, maximizing profitability and competitiveness. Embracing machine learning has become imperative for e-commerce businesses seeking to thrive in a data-driven and hyper-competitive landscape, as it empowers them to stay agile, customer-centric, and at the forefront of innovation.
As this technology continues to evolve, its potential for reshaping the e-commerce industry remains limitless.
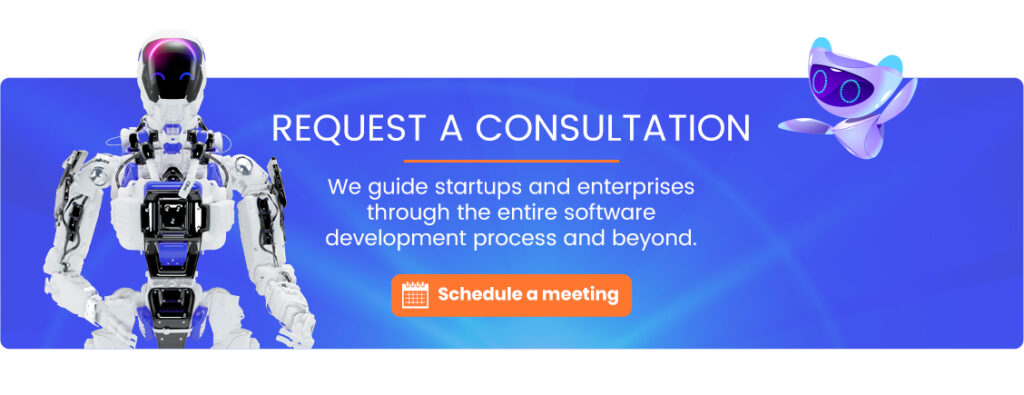
- #.NET Software
- ##cloud advisory
- #accounting
- #aidefectdetection
- #aiecommerce
- #android app
- #application support
- #applications
- #Artificialintelligence
- #Best Practives
- #businessprocessoptimalization
- #cloud based analytics
- #Cloud Computing
- #cloud cost optimization
- #cloud management
- #Cloud Management Outsourcing
- #cloud security
- #CRM software
- #custom app
- #Custom ERP
- #Custom software
- #defectdetection
- #development process
- #e-commerce
- #ERP software development
- #fixed price
- #healthcare
- #hiring automation software
- #Machine Learning
- #machinelearning
- #machinelearninginecommerce
- #management
- #market research
- #medical software
- #minimum viable product
- #modern app
- #Navigation
- #Offshoring
- #one page app
- #online payment
- #outsourcing
- #payment gateways
- #proof of concept
- #qualitycontrol
- #Recruitment Automation Software
- #recruitment tools
- #single page app
- #software house
- #software product
- #time&material
- #web development
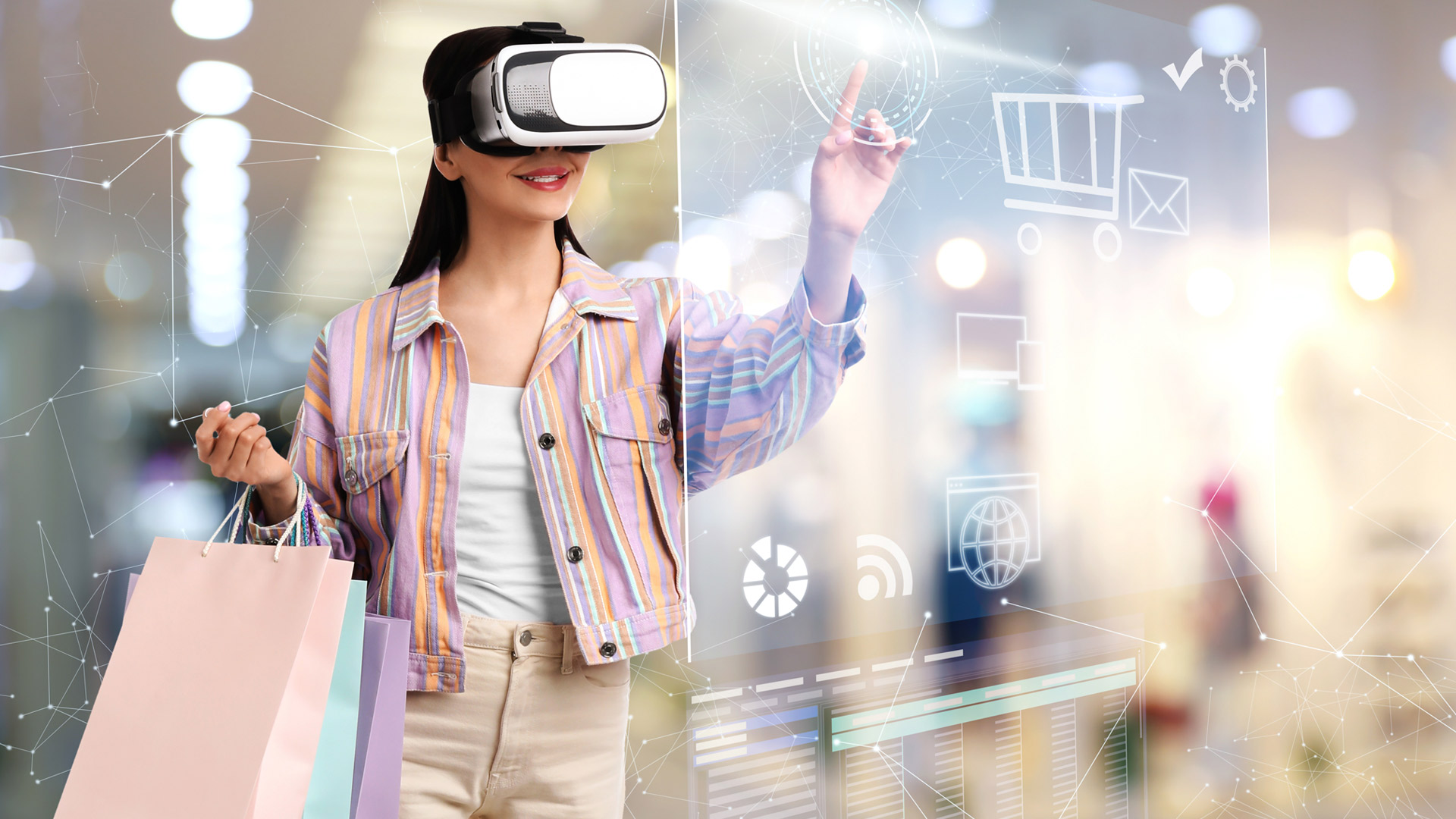
In the ever-evolving realm of modern retail, fueled by the relentless progression of e-commerce, a transformative force takes center stage—virtual try-on technology. This innovation, far from a mere technological catchphrase, represents a fundamental shift in how we approach shopping. Seamlessly merging augmented reality (AR) and virtual reality (VR), it provides consumers with an immersive and […]
In the ever-evolving landscape of e-commerce, the pursuit of success is a constant challenge. With a myriad of products, fierce competition, and rapidly changing consumer preferences, e-commerce businesses must navigate a complex web of decisions to thrive in the digital marketplace. This is where Ecommerce Business Intelligence (eCommerce BI) comes to the rescue, serving as […]
In an era driven by digital innovation and technological advancements, the financial landscape has been undergoing a radical transformation, thanks to the disruptive force known as Financial Technology, or FinTech. The fusion of finance and technology, FinTech, has revolutionized the way we interact with money and has permeated the financial industry across various sectors, sparking […]
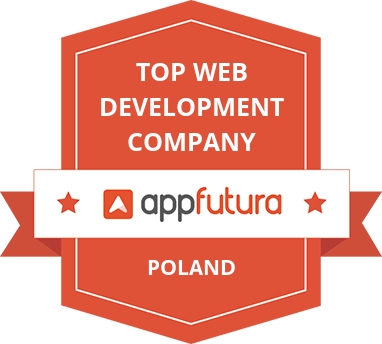
🤝Interested in working with us? Let’s talk about development for equity. 👨💻 [Get in Touch]
- AR & VR Solutions
- Internet of Things Solutions
- Omnichannel Solutions
- Analytics & Business Intelligence
- IT Security
- Business Process Automation
- Cloud Computing Solutions
- eLearning & Online Training
- Social Networking Solutions
- CRM Solutions
- Dashboard & Data Visualization
- Application Development
- Machine Learning Development
- Artificial Intelligence Development
- Chatbot Development
- Robotic Process Automation
- Data Science Consulting
- Natural Language Processing
- Video Analytics
- ChatGPT Application Development
- Software Development
- WordPress Development
- SEO Services
- AWS Consulting
- Google Cloud Consulting
- Database Development
- Devops Consulting Services
- Cloud Application Development
- UI/UX Design
- Ecommerce Development
- Marketplace Development
- Mobile App Development Services
- iPhone App Development
- Hybrid App Development
- Android App Development
- MVP Development
- Microservices Development
- Web Development
- Virtual CTO as a Service
- Development For Equity
- Vision Pro App Development
- Web Portal Development
- Software Product Development
- Software Testing
- Web Application Development
- Hire ASP.NET MVC Developers
- Hire PHP Developers
- Hire iOS & Android Developers
- API Development Services
- Progressive Web App Development
- AngularJS Development
- Reactjs Development
- Vuejs Development
- Nextjs Development
- Nestjs Development
- MongoDB Development
- .NET Development
- CakePHP Development
- Codeigniter Development
- Laravel Development
- Symfony Development
- React Native Development
- Flutter Development
- MERN Stack Development
- MEAN Stack Development
- Full Stack Development
- Java Development
- NodeJS Development Services
- Python Development
- WebRTC Development Services
- Cardano Development
- Polygon Development
- Solana Development
- Binance Smart Chain Development
- Ethereum Development
- Cosmos Development
- Tezos Development
- Tron Development
- Hyperledger Development
- Polkadot Development
- EOS Development
- Web3 Development
- Crypto Exchange
- Crypto Wallet Development
- Smart Contract Development
- dApp Development
- NFT Marketplace Development
- Metaverse Development
- Dedicated Development Resources
- Proof of Concept (PoC)
- System Audit
- Software Maintenance
- Minimum Viable Product (MVP)
- Product Discovery
- IT Consulting
- Food and Beverage
- Engineering
- Finance & Insurance
- Manufacturing
- Media & Publishing
- Hospitality & Travel
- Online Commerce
- Sports and Entertainment
- Professional Services
- ISVs and Product Development Solutions
- Offshore Outsourcing
- Offshore Development Center
- Engagement Models
- Case Studies
- Testimonials
- Infrastructure
- Software Development Company India
- Content Management Services
- SaaS Development Services
- Design Services
- Game Development
- Ecommerce Development Services
- Web Development Services
- Social Media App Development
- Hire Dedicated Remote Developers
- DevOps Consulting Services
- Cloud Application Development Services
- Frontend Development
- PHP Development
Machine Learning in eCommerce: Use Cases, Examples, & Best Practices
Ml in ecommerce.
Last Updated: 2 May 2024
The current world has experienced changes, especially in technology. And you realize that technology and commerce are coming together each day in a unique way. E-commerce relies much on digital and technological growth. Machine Learning (ML) is a revolutionary force steering ecommerce to new heights. The coming together of ML and ecommerce has brought in several opportunities, always redefining how businesses interact with customers, manage their operations, and help them experience growth. We can use machine learning in eCommerce to help in decision-making to ensure positive impacts in the business environment. This article explores how machine learning has become a game-changer in ecommerce. You will come across real-life examples, use cases, and best practices.
The core of ecommerce is the struggle to achieve operational excellence and improve customer experiences. Machine learning brings the power of analyzing big chunks of data, predicting trends, and providing important insights beyond human ability. Take a situation where there is the personalization of your shopping experience, making it appear as if the store came into existence just for you. Imagine a situation where we have swift and precise customer service. That is machine learning for you when it comes to ecommerce.
ML goes beyond creating futuristic shopping experiences and brings an aspect of solving real-world problems like pricing strategies, managing inventory, and fraud detection. ML ensures the seamless and smooth running of such components.
Brief overview of Machine Learning (ML)
Machine learning is a subset of artificial intelligence (AI) and computer science emphasizing on the use of data and algorithms to imitate the way humans learn, continuously enhancing its precision. ML aims at building computer systems that can learn from data. When you check the traditional programming, you execute tasks following explicit instructions. ML allows computers to make decisions or predictions based on data learning without programming them for particular tasks. Computers can derive insights, recognize patterns, and improve their predictive and decision-making potentials.
ML algorithms can identify patterns and any relationships existing within given data. We use historical data to train the algorithms to make predictions, clarify information, reduce complexities, cluster similar data points, and help create new content. Good examples are in applications like GitHub Copilot, Dall-E 2, and ChatGPT. So, it is possible to interact with ML versatility, including:
- Powering recommendation engines with the news platforms, e-commerce sector, and social media.
- Present in healthcare diagnostics and autonomous cars
- Automating most of the business processes
- Fraud detection
- Spam filtering
- Detecting malware attacks
- Predictive analysis
Amid such good promises, ML faces challenges and complexities. The field needs experts in statistics and mathematics who can choose the applicable algorithms and analyze the results. To maintain accuracy, high-quality data must be fed to the algorithms. The cost and resourcing invested in fine-tuning and running the ML models should be a concern.
Understanding How Machine Learning Works
We have already seen that machine learning involves programming computers to facilitate them learn from experience. The computer can think and make decisions using data as its guide without direct human intervention.
The core of ML is data (or training data), and the more data is gathered, the better. The training data could be images, text, or numbers. You must be careful with the quality and quantity of the data.
The next phase after you gather data is selecting a machine learning model . The model will help you process your gathered data, identify particular patterns, and make predictions. Various models handle the different data sets and classes of tasks.
We can divide ML operations into three main phases. They include:
- Decision-making process: ML algorithms take the gathered data, analyze it, and identify patterns. The patterns then help in carrying out predictions or classifications.
- Error function: ML algorithms check the accuracy of their predictions by comparing them with related examples or actual outcomes.
- Optimization/updating: There is an analysis and evaluation of the error level before updating the first process. Optimization ensures there are fewer errors in future cases and improves accuracy.
Advantages of machine learning
We are currently in the information age, and data is very important. ML powers data-focused generation. You can’t underestimate it. Here are some key important aspects to consider:
Processing of data
ML can manage and interpret big volumes of data. Many digital data across the space comes from sources like sensors, social media, etc. Such data is too complex for traditional methods of data analysis. ML algorithms come in handy to process large amounts of data, identify core patterns, and provide insights to help in decision-making.
A driving force for innovation
ML solutions is the powerhouse for innovation across various industries, enhancing efficiency and creating new opportunities. Some of the areas we can highlight include:
- The healthcare sector. ML algorithms play a big role in predicting disease outbreaks, coming up with personalized patient care plans, and improving the accuracy of medical imaging.
- The finance sector. We can use ML algorithms to determine credit scores, algorithmic trading, and fraud detection.
- The retail sector. ML plays a big role in transforming the supply chains, recommendation systems , and customer service.
- ML also applies in education, entertainment, and agriculture.
Aiding automation
Machine learning places more focus on automation. ML algorithms analyze large volumes of data, improve their processes, and ensure they can handle traditionally manual tasks. Automation helps humans focus on other important and creative tasks, which improves efficiency and innovation.
Types of Machine Learning
We can categorize machine learning algorithms depending on the nature of the learning approach, available data, and the particular task to be carried out. The learning categories may include:
- Unsupervised
- Semi-supervised
- Reinforcement
Supervised Learning: Learning with Guidance
This is the famous type of ML. This method involves the model or algorithms being trained with the use of pre-labeled datasets. The algorithm learns to classify data or predict outcomes through processing the input data and then uses trial and error to refine predictions. Examples include email spam filters for classifying an email as spam/junk or not spam, decision trees, image recognition processes, support vector machines, and linear regression.
Unsupervised Learning: Independent Data Exploration
Unsupervised learning involves training a given model on datasets that aren’t labeled. The algorithms autonomously scan through to identify patterns and relationships and classify such data independently. This method is good for data analysis, dimensionality reduction, pattern/image recognition, and customer segmentation (regarding demographic data, purchasing patterns, etc.).
Semi-Supervised Learning: A Blend of Methods
This learning method combines some elements of supervised and unsupervised learning techniques. There is usage of labeled and unlabeled data during the training. Such algorithm utilizes patterns recognized within a smaller labeled dataset for classifying larger unlabeled data. This method is important in data classification and labeling.
Reinforcement Learning: Learning Through Feedback
Reinforcement Learning involves using algorithms with an agent to learn the art of making decisions in multi-steps following defined rules through interaction with its environment. Depending on the action, the agent or algorithm gets feedback termed as a reward or penalty. The feedback will provide guidance to the learning process. Examples include robotics, resource management, game playing, etc.
Understanding Machine Learning in Ecommerce
Some basic concepts apply when we think of ML in Ecommerce . Here are some of the concepts:
Algorithms : In ecommerce, we use ML algorithms in customer data analysis to determine the key purchasing patterns and preferences.
Data Analysis : The use of ML tools to process large amounts of e-commerce data and develop insights that play a key role in developing strategies and making decisions.
Predictive Analytics : The use of historical data in predicting the future trends. We use such to predict customer behavior, demand, and sales patterns.
Classification Algorithms : Useful in grouping customers according to preferences, purchase history, and browsing history. A good way to implement targeted marketing.
Clustering Techniques : Allows the grouping of similar customers or products to improve the recommendation systems or manage your inventory.
Natural Language Processing (NLP) : Customer service tools and chatbots make use of NLB in handling customer inquiries.
Role & Advantages of ML in Ecommerce
- Data Analysis: We use ML to carry out analysis of the behaviors related to various customers and trends in the market to ensure there is optimal pricing, marketing techniques, and product placement.
- Customer Service: ML is the central pillar of chatbots and virtual assistants for handling customer support and ensure there are personalized recommendations.
- Personalization (or tailor-made marketing): Each customer can have their shopping experience personalized through the use of ML. They get product suggestions depending on their past buys and preferences.
- Inventory Management: You can use ML algorithms to predict demand trends and know when to stock or reduce the restocking volumes.
- Businesses experience enhanced conversions through the process of product recommendations and using on-site search engines.
Use Cases and Examples of Machine Learning in Ecommerce
Personalized product recommendations.
Use Case: Product Recommendations
We have already seen that you can use ML algorithms to study how customers behave, their buying history, and what they prefer. Such analysis helps come up with tailor-made product recommendations. Doing so will see an improvement in conversion rates, sales increase, and the general shopping experience improves.
Example: Amazon’s Product Recommendation System
Those who have interacted or made purchases on Amazon can attest that they get product suggestions that nearly fit what they previously purchased or what they browsed. Amazon’s recommendation system uses collaborative filtering and deep learning techniques to achieve the best outcomes that prove relevant to the user.
Customer Churn Prediction
Use Case: Customer Retention
ML algorithms can determine the customer who is likely to abandon your shop/site depending on how they behave, such as visiting the store less frequently and making smaller purchases. With such, the business takes proactive measures to retain customers.
Example: Netflix’s Customer Churn Prediction
Machine learning is in full use by Netflix, which comes in handy by checking the users’ activity, viewing history, and engagement metrics, which gives the company a clear indication of whether the customer is about to cancel the subscription. In such a scenario, Netflix will send targeted recommendations and offers to ensure they retain the customer(s).
Inventory Management and Demand Prediction
Use Case: Inventory Optimization
Businesses can use ML to optimize their inventory levels by having accurate demand predictions. In such scenarios, there are checks on overstocking or understocking. This is a good measure to save on costs and improve general customer satisfaction.
Example: Walmart’s Inventory Management
ML algorithms help Walmart analyze historical data related to their sales, seasonality of the sales, and other external factors like the effects of weather patterns. The analysis helps predict product demand, which optimizes inventory levels and thereby reduces carrying costs.
Detecting and Preventing Fraud Detection
Use Case: Fraud Prevention
You can use ML algorithms to analyze transactional data, identify any particular patterns and anomalies, and take measures to protect the customer and company against any financial loss.
Example: PayPal’s Fraud Detection
There is use of ML by PayPal in carrying out transaction data analysis related to the behaviors of the users, their transactions and the devices they use. Any weird deviation in the behavior sets the system on alert and calls for investigations to ensure no fraudulent transactions.
Price Optimization
Use Case: Dynamic Pricing
You can use the ML algorithm to adjust a product’s prices by observing factors such as competitor pricing, conditions in the market, and the general demand. It is a good strategy to maximize profits.
Example: The Surge Pricing by Uber
The surge pricing algorithm by Uber will see an increase in fares at the moment when the demand is at its peak. The algorithm studies the traffic conditions, demand by riders, and availability of drivers in a given area in real-time to ensure a balance between supply and demand.
Analyzing Sentiment and Customer Feedback
Use Case: Customer Feedback Analysis
You can use ML algorithms to analyze reviews and feedback from customers and draw insights pointing to customer satisfaction. It is a good way to identify the areas that need improvement.
Example: Airbnb’s Sentiment Analysis
There is the use of ML algorithms by Airbnb to analyze sentiment depending on reviews from guests and the ratings of a particular host. Such analysis helps in coming up with measures to guarantee improved guest experience.
Virtual Assistants and Chatbots
Use Case: Customer Support
ML helps in developing virtual assistants and chatbots to ensure there is efficient and instant customer support to improve the general customer experience. And the outcome is happy customers who keep returning.
Example: IBM Watson Assistant
It uses ML and NLP to interact with customers, collecting and responding to their queries. IBM Watson Assistant helps respond to FAQs, suggest products, and troubleshoot a number of problems.
Customer Segmentation and Targeted Marketing
Use Case: Marketing Campaigns
ML algorithms can group customers based on their demographics, purchasing behavior, and preferences. With such groupings, it is possible to implement marketing campaigns that target a particular group.
Example: Personalized Playlists by Spotify
There is the use of ML by Spotify to categorize users depending on their music flavor. They then personalize playlists and even recommend particular songs depending on every user’s preferences.
Best Practices in Implementing ML in Ecommerce
- You must understand the business needs. Make sure the ML initiatives meet the set business goals to make them effective in the general strategy.
- Observe the quality of data and how it is managed. Ensure the ML models get high-quality and accurate data since their success depends on the data.
- Select the appropriate tools and technologies. Go for ML tools and technologies that will implement your business needs and allow scalability when needed.
- Do you have the skills and expertise? You need ML and data science professionals who will be involved in developing and managing ML solutions.
- Adhere to ethics. Make sure you are observing state laws when it comes to data usage, privacy, and security.
Machine learning has come as a revolution in what people can achieve with the use of technology, especially ecommerce. Online shoppers can enjoy relevance and personalization, thanks to ML, which also improves revenues for online stores. All businesses should consider implementing ML to harness the power of AI and technology.
Want to implement ML in your eCommerce product? Get in touch with our eCommerce development company – Aalpha information systems!
Share This Article:
Written by:.
Pawan Pawar , CEO
CEO -Founder of Aalpha Information Systems India Pvt. Ltd., with 18+ years in software development. I've worked with startups to enterprises, mastering diverse tech skills. Passionate about bridging the gap between vision and reality, my team and I craft customized software solutions to empower businesses. Through this blog, I share insights, industry trends, and expert advice to navigate the ever-evolving tech landscape. Let's unlock the potential of technology and propel your business to new heights. Connect with me on LinkedIn.
Related Posts
Mobile app development timeline.
The field of mobile app development is transforming many industries. From healthcare to education, business, finance, travel & tourism, and agriculture, among many other industries, processes are now simpler and more seamless than before. Many entrepreneurs are now shifting their focus to mobile apps to enhance and accelerate their business growth and scalability. With the many benefits mobile apps have to offer, the question of how long it will take to develop such an app will always come up. Mobile app development is a process that entails several phases, which require different tech tools, resources, and expertise. As a result,…
iOS Application Testing Checklist
The innovation of the iPhone has had a significant impact on the smartphone and the wider tech industry at large. Today, iOS apps have a significant market share, with over 1.5 billion people using iPhones, which equates to 30% of the market share. The number and market share of iPhone users continues to grow with the invention of iOS devices with advanced features. This trend has made iPhone users go for Apple products due to the many benefits they get. Apart from robust security, apple products are known to be effective and reliable in many different ways. Therefore, every iOS…
Questions to Ask a Client When Developing a Mobile App
Mobile applications are taking a better part of our lifestyle every other day. Through such apps, it is now easy for users to connect with others, access valuable information, make purchases, and explore various forms of entertainment and other services. Developing such apps requires expert approaches to enhance the functionality and performance of mobile apps. It is, therefore, the role of developers to ensure they integrate cutting-edge technologies, the latest tools, and resources to develop impressive mobile apps. Ideally, the goal is to ensure clients are satisfied with product delivery. But then, how can a developer ensure they develop mobile…
Get Started with your Project or Take Existing one to Next Level
Proven Development Experience & Happy Clients in 41 Nations are our Pillars of Success
- Domain Expertise
Outsourcing
Email: [email protected].
- Blockchain Development
- E-Learning Online Training India
- IoT Development
- Medical Billing
United States
910 N Market St #45, Wilmington, DE 19801, USA
Sandsvegen 71 6823 Sandane, Norway
Bangalore, India
No. 197, 2nd Floor, 5th Main, 6th Cross Gandhinagar, Bangalore-560009, Karnataka, India
Hubli, India
Block #10, Daimond Corner Opp. Sawai Gandharava Hall, Deshpande Nagar, Hubli-580029, Karnataka India
Follow us on
This website uses cookies and other similar technologies to save and access information on your device ("Cookies"). Please read our Cookie Policy and Privacy Policy and if you accept the Cookies we use, press: "Allow all", and if you want to make a different choice - change the settings in your browser regarding Cookies or use the "Cookie Settings" option below.
These Cookies are necessary for the proper functioning of our website or the provision of services with its help and therefore cannot be switched off. They relate in particular to the possibility of setting preferences in the field of privacy, enabling some functionalities of the website, logging in or filling out forms. If you turn on blocking these Cookies in your browser, our website may stop working or work incorrectly.
These Cookies enable us (and sometimes our partners), for example: to count visits and traffic volumes, check which pages you have visited prior to visiting our website, so that we can analyze and improve the performance and functioning of our website. They help to determine which subpages and sections are the most popular, check how users move around the website and draw conclusions as to its operation. The information collected with the help of these Cookies is aggregated, which makes them anonymous. Turning on blocking of these Cookies will deprive us of this information and limit the possibility of improving the operation of our website.
These Cookies may allow us (and sometimes our partners) to learn about your preferences, and by giving you an additional designation (e.g. ID number) help in adjusting the content of displayed advertisements (on our or other websites) to these preferences. Turning on blocking of these Cookies will not cause that you will not be shown ads, but they will not be selected for you based on how you use? our website.

The Best Machine Learning Use Cases in e-Commerce
1. recommendation engine (recommender system), 2. personalization of the content on the website, 3. machine learning for dynamic pricing in e-commerce, 4. a/b tests using ai, 5. predictions using machine learning in e-commerce, 6. image processing, 7. improving the quality of the search engine using machine learning in e-commerce, 8. smart chatbots to improve customer service, 9. fraud detection, case studies of machine learning in e-commerce.
- The Best Machine Learning Use Cases in e-Commerce - FAQ

CEO & Co-Founder
Reading time:
E-commerce – is one of the first industries that started using all the benefits of machine learning. Nowadays, there are machine learning applications for almost every area of e-commerce. Machine learning solutions for e-commerce really helps-from inventory management to customer service. Zalando, Asos – companies that have entire artificial intelligence (AI), deep learning departments. They invest a lot of money to have better knowledge on their clients, personalize offers for a particular customer, improve customer experience and automate manual processes.
Recommendation engine and machine learning in the e-commerce industry directly convert into profits and increases the company’s market share with better customer acquisition.

Addepto machine learning consulting team has analyzed which solutions have the biggest potential today. You can find 9 machine learning applications in e-commerce below. They can help monetize your data and improve customer experiences like Asos and Zalando:
Machine Learning in e-commerce has few key use cases. Personalization and recommendation engine is the hottest trend in the global e-fcommerce space. With the use of machine learning algorithms for e-commerce and the processing of huge amounts of data, you can thoroughly analyze the online activity of hundreds of millions of users. On its basis you are able to create product recommendations, tailored to a specific customer or group (auto-segmentation).

Interested in machine learning? Read more: Machine Learning. What it is and why it is essential to business?
How does the recommendation engine work in e-commerce?
Let’s see how the recommendation engine in e-commerce works. By analyzing collected big data on the current traffic on websites, you can determine which sub-pages the client used. You could identify what he was looking for and where he spent most of the time.
Moreover, based on various information: profile of previous customer activity, its preferences (e.g. favorite color), social media data, location and weather – results will be displayed on a personalized page with suggested products that will most likely interest them.

Properly personalized content on the website or mobile application increases conversion and customer engagement. The selection of the best content is possible thanks to machine learning algorithms for e-commerce. Thus, algorithms could find patterns in the data based on the processing of a large amount of structured and unstructured data (including images and text).

Machine learning algorithms in e-commerce take into account various factors such as favorite style and color, image intensity, activity history, preferences, etc. The results on the website are adapted to each individual person’s personal preferences. In this way, recommendations for using machine learning in e-commerce could help you increase your revenues.
Machine Learning in e-commerce can be very helpful in case of dynamic pricing and can improve your KPI’s. This helpfulness comes from ML algorithm ability of learning new patterns from data. As a result, those algorithms continuously learn from new information and detect new demands and trends.
This is why online retailers could use ML models in the e-commerce industry for dynamic pricing. Instead of simple price markdown. E-commerce companies could benefit from predictive models that can allow them to determine the best price for each particular product. You can choose the offer, the optimal price and display real-time discounts, which will also take into account the state of the warehouse.This is done to maximize sales and to optimize inventory.

In fact, Amazon is still the market leader in the area. The company is making significant progress in the use of machine learning for dynamic pricing. Furthermore, they change prices every 10 minutes, which is fifty times more than Walmart and Best Buy, resulting in a 25% increase in profits. [4]
A/B tests enable the product (e.g., website) to be adapted to consumers. Almost 80% of the A / B test variants do not yield positive results. Conducting this process is very hard and laborious, which is why the algorithms of machine learning for e-commerce will definitely help you with:
- Process automation of selecting platform`s (product`s) features that should be changed through the use of a genetic algorithm. That is based on the best-suggested changes to the product that an algorithm may offer. For example, noticing that the bigger “BUY” button on the page increased sales by 1% so we can check whether its further enlargement may improve the results.
- Automatic customer segmentation into groups using unsupervised Machine learning models for e-commerce depending on their characteristics (age, gender, expenses, preferences, etc.) and personalization of the content (product for their needs). For example, for women over 40, the main color of the page will be burgundy, while for men under 20 years old, it will be blue.
- Faster finding optimal options of pages / products through the use of self-learning AI algorithms instead of repetitive and tedious work. Machine learning in e-commerce allows online retailers to shorten orders of magnitude from months to days.

- Predicting whether a given user will make a purchase in a specific product category in real time – so that the seller can react accordingly (eg, call that person or send email with engaging content). It gives you the opportunity to increase conversions while the customer, for example, is considering buying.
- Predicting whether the user will be returning and what purchases he will make at certain times . This will help in matching the right marketing message to that person to increase the conversion of the future purchase and to encourage the person to return.
- Customer lifetime value prediction (CLTV or LTV) – to predict how much money a particular user will spend in your shop. Accurate estimation of the future customer value allows effectively allocate marketing expenses, identificate and care for high-value customers and reduce exposure to losses.
- Customer churn prediction will discover customers who are risky to leave. The implemented machine learning solution in e-commerce will allow you to react quickly to the customers who will probably stop buying from you. Such a system will increase retention rate and will bring you a stable stream of revenue.
- Prediction of client’s size – personalized size recommendations reduce the chargebacks for both the company and customers. Predictions using machine learning in e-commerce reduces company’s or customers’ costs and definitely increases customer satisfaction.
- Prediction of demand for specific product categories – this will help to meet all customer needs and trends in the future. This will cause customers to be happy to return to your online store where most of the goods are available and can be bought immediately.
Retailers invest in AI and image recognition systems to influence customers (buyers) behavior and also for process automatization. Investment into computer vision technology with visual search possibilities could help you to match customers photos e.g. with similar clothes sold online. This could be defined by user’s preferences based on the category of products the person usually buys (what color, what brand) and based on the data from social media (eg Instagram, twitter, facebook, vkontakte).
Another machine learning application in e-commerce could be automatic completion of information about the subject on the basis of the photo (what is the article, what category to add it, what color it has).
Users use search engines to quickly find what they need. They have less and less time and patience to formulate queries, wait for results and analyze them. That is why there is a need for personalized results of search queries.

A personalized search engine could play an increasingly important role. It is based on machine learning models with short-term and long-term user preferences, history or previous queries. In addition, such search engines are able to increase the user’s conversion better than non-personalized search engines based on traditional information retrieval (IR) techniques.
This is especially important for giants like eBay.
With over 800 million items on its website, eBay uses artificial intelligence and data to predict and represent the most relevant search results.
An intelligent chatbot based on NLP and AI can interpret individual users’ questions and respond to them individually. The role of virtual assistants is to imitate the best consultants to be able to help the users of e-stores in the purchase process in the most effective way. For example, help in getting to the products, suggest the best pricing solutions, carry out through the transaction process.
“8 out of 10 consumers who have engaged with a chatbot, report it as an overall positive experience.” – Hiconversion.com
The cost that online stores lose due to fraud continues to increase steadily. Therefore, fraud identification and protection are important processes for all online stores. Machine learning algorithms for e-commerce can improve these processes and make them more effective.
According to a recent Juniper Research research, investment on machine learning in the e-commerce industry would increase by 230 % between 2019 and 2023, with 325,000 retailers worldwide using machine learning algorithms in some form by 2023. [1]
eBay: Machine Learning for language translation
eBay, one of the largest e-commerce platforms in the world, has created a technology called eBay Machine Translation (eMT). This eMT system translates product names with 90% accuracy. Thanks to this machine learning solution, the company’s total sales increased by 10.9%. [2]

“Machine translation at eBay is key in promoting cross-border trade. Our technology helps overcome language barriers and lets buyers order items from foreign countries” – Evgeny Matusov, eBay’s Senior Manager of Machine Translation Science. [2]
Anheuser-Busch: Using machine learning in e-commerce for optimization route planning
The brewing giant has developed a machine learning platform for daily plan routing. As a result, the company noted an increase in productivity and efficiency a couple of months later. The machine learning algorithms for e-commerce also take into account the collective experience of drivers to offer the best delivery time for each customer. [3]

American Eagle Outfitters: Machine learning in e-commerce for visual search engine
American Eagle, a well-known clothing brand, is partnering with Slyce, a promising image recognition startup. Through its mobile app, Slyce provides a visual search engine that allows customers to search for specific clothing based on photos captured by their handheld device’s camera. [5]
E-commerce is an industry where machine learning applications directly affect customer service and business growth. With machine learning applications in e-commerce, you can create business benefits for each department of your e-commerce business.
Moreover, improve customer service, increase efficiency and productivity, improve customer support, and make more informed HR decisions. As machine learning algorithms for e-commerce continue to develop, they will continue to be of great benefit to the e-commerce industry.
Check our case studies to see how e-commerce companies use machine learning and deep learning. Drop us a line and we will explain to you how to use Machine Learning in E-commerce and how companies could benefit from it. We are at your service!
Also check out our machine learning services to learn more.
The Best Machine Learning Use Cases in e-Commerce – FAQ
What is the significance of machine learning in the e-commerce industry.
Machine learning plays a crucial role in e-commerce by enabling businesses to analyze vast amounts of data, personalize customer experiences, optimize pricing strategies, enhance search functionalities, improve customer service through chatbots, detect fraud, and much more.
Recommendation engines analyze user data to understand preferences, browsing history, purchase behavior, and other relevant factors. By leveraging this information, they provide personalized product recommendations to users, enhancing their shopping experience and increasing the likelihood of conversion.
What are the benefits of personalization of website content in e-commerce?
Personalizing website content can lead to increased conversion rates and customer engagement. By tailoring content based on user preferences, browsing history, and behavior, businesses can create a more relevant and compelling shopping experience for their customers, ultimately driving sales and loyalty.
How can machine learning be used for dynamic pricing in e-commerce?
Machine learning algorithms can analyze market trends, competitor pricing, and customer behavior to dynamically adjust prices in real-time. This allows businesses to optimize pricing strategies, maximize revenue, and remain competitive in the market.
What are A/B tests, and how can AI assist in conducting them?
A/B tests involve comparing two or more variants of a product or website to determine which performs better. AI can automate the process of selecting test variants, segmenting customers, and analyzing results, making A/B testing more efficient and effective for e-commerce businesses.
How does machine learning enable predictions in e-commerce?
Machine learning algorithms can predict various outcomes, such as customer purchase behavior, lifetime value, churn, demand for specific products, and more. These predictions empower businesses to make data-driven decisions, optimize marketing strategies, and enhance customer satisfaction.
How is image processing utilized in e-commerce?
Image processing technologies, such as computer vision and visual search, enable businesses to enhance product discovery and recommendation. By analyzing images, these systems can identify products, extract relevant information, and provide personalized recommendations based on user preferences.
What role do chatbots play in improving customer service in e-commerce?
Chatbots powered by natural language processing (NLP) and AI can interact with customers in real-time, providing personalized assistance, answering queries, and guiding them through the purchase process. This improves customer satisfaction, reduces response times, and enhances overall user experience.
How does machine learning contribute to fraud detection in e-commerce?
Machine learning algorithms analyze patterns and anomalies in transaction data to detect fraudulent activities, such as payment fraud, account takeover, and identity theft. By identifying suspicious behavior in real-time, businesses can mitigate risks and protect their customers and assets.
This article is an updated version of the publication from Jun 23, 2021.
- Data-axle.com. 4 case studies on how Machine Learning is helping retailers drive revenue. URL: https://www.data-axle.com/resources/blog/4-case-studies-on-how-machine-learning-is-helping-retailers-drive-revenue/. Accessed June 23, 2021.
- Ebayinc.com. eBay’s Machine Translation Technology Breaks Down Borders. URL: https://www.ebayinc.com/stories/news/ebays-machine-translation-technology-breaks-down-borders/. Accessed June 23, 2021.
- Towardscience.com. Disruption in Retail — AI, Machine Learning & Big Data. URL: https://towardsdatascience.com/disruption-in-retail-ai-machine-learning-big-data-7e9687f69b8f. Accessed June 23, 2021.
- Icicletech.com. Changing the e Commerce Game: 8 Ways AI and ML are Disrupting Online Shopping. URL: https://www.icicletech.com/blog/ai-ml-changing-ecommerce. Accessed June 23, 2021.
- Datadriveninvestor.com. Machine Learning Applications in Retail: 6 Real World Examples from Market Leaders. URL: https://www.datadriveninvestor.com/2019/07/11/machine-learning-applications-in-retail-6-real-world-examples-from-market-leaders/. Accessed June 23, 2021.

AI Consulting
Addepto is an AI consulting company that develops AI-driven services that will enable your company to take full advantage of the gathered data

Machine Learning Consulting
Related articles.

Machine Learning in Energy Sector: Use Cases
Nowadays Artificial intelligence (AI) is helping energy companies analyze massive amounts of data. Most of the data on e...

Machine Learning in Logistics: 10 Use Cases of AI and ML
Artificial Intelligence and machine learning are conquering more and more industries and spheres of our lives, and logis...

Customer Lifetime Value (LTV) Prediction using Machine Learning
In today's customer-focused market, it is very important to know the customer lifetime value (LTV). LTV helps companies ...

What Are the Top 10 Challenges of Machine Learning?
On our blog, we talk about machine learning every week. That’s no surprise. After all, machine learning consulting is ...
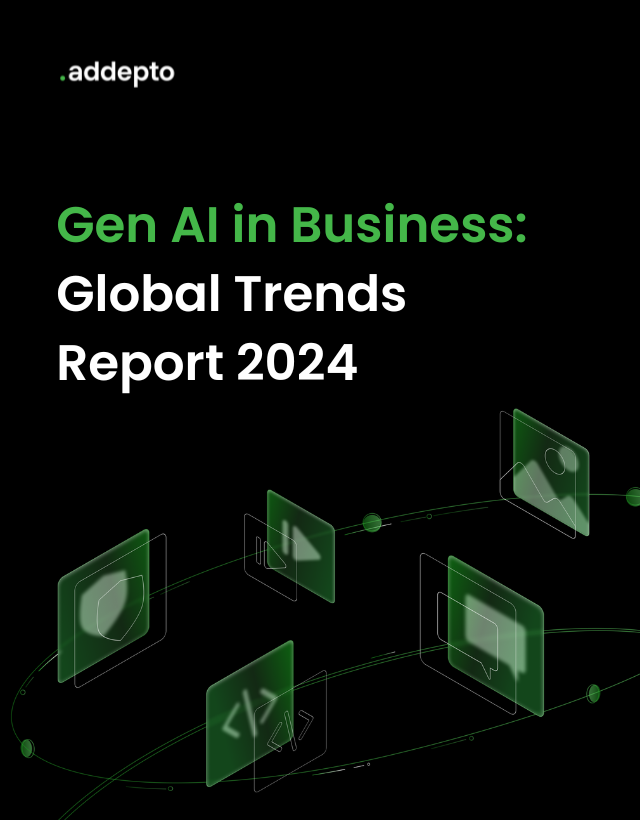
Report: “Generative AI in Business 2024”. Unlock the Future of Business Innovation!
Discover the latest industry insights, challenges, opportunities, and real-world case studies on integrating Gen AI, all compiled in one comprehensive report!
Machine Learning in eCommerce: 12 Ways to Boost the Online Shopping Experience
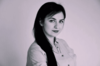
eCommerce is one of the most rapidly growing industries. The market generated 5.2 trillion U.S. dollars in revenue in 2021.
Along with growth come a lot of challenges. You must either innovate or risk losing your footing.
eCommerce machine learning can turn your business upside down (in a good way, don’t worry). Higher revenues, reduced losses, improved customer satisfaction, and lower returns are just a few benefits that AI and ML can bring.
According to Servion , AI will power 95% of customer interactions by 2025. Machine learning improves the performance of an eCommerce business through an impeccable customer experience.
For instance, implementing AI-powered sizing assistance helped Asos multiply revenue by 300% and cut losses in half caused by product returns.
In this article, we talk about how to build a successful eCommerce business by implementing machine learning.
You’ll discover:
- Future of eCommerce and machine learning
- Benefits of machine learning in eCommerce
- Use cases of machine learning for eCommerce
- Useful eCommerce platforms and tools
- 6-step process for incorporating machine learning algorithm
All in all, it’s time to revamp your eCommerce business with machine learning and artificial intelligence.
Future of eCommerce and Machine Learning
It is no surprise that the latest technologies are always finding ways into eCommerce and online stores. Machine learning is no exception.
So what AI/ML can bring to the retail industry?
Machine learning in eCommerce helps businesses become more agile while taking the customer experience to a new level.
In 57% of cases, companies use machine learning and artificial intelligence to improve their customer experience. Personalized design and product recommendations, customer demand prediction, and inventory and delivery management are three areas with the biggest potential for machine learning in the retail industry.
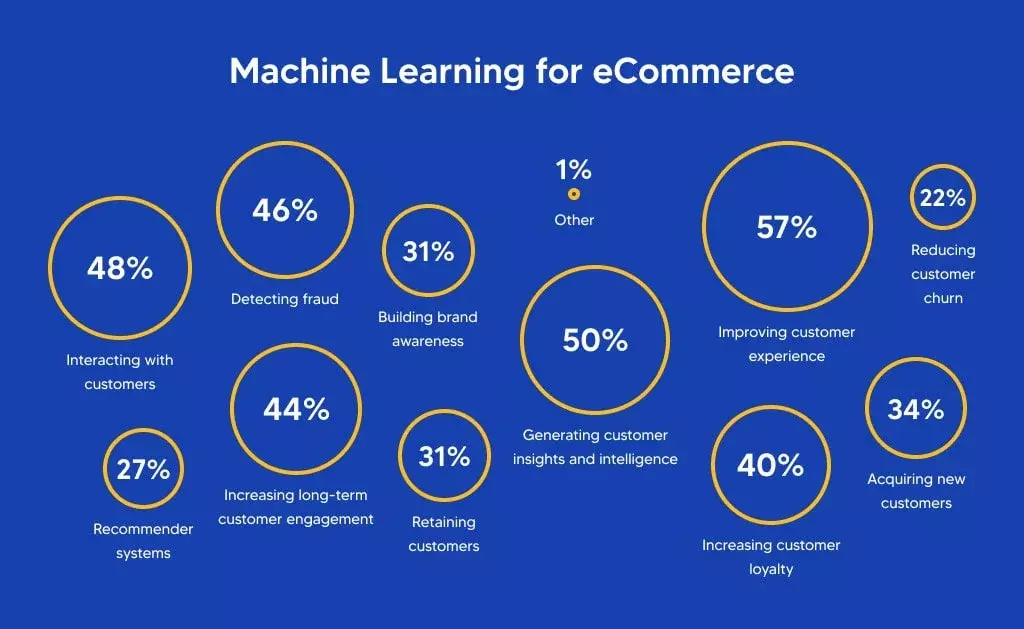
Thanks to machine learning implementation, customers can get timely predictions regarding their businesses, insight into customer behavior, and the ability to identify what influences their buying decisions, as well as how much inventory they need for the upcoming holiday season.
Machine learning in eCommerce brings many benefits, which is why companies are searching for the help of a software development company like MLSDev to implement the technology.
Want to implement machine learning in eCommerce?
Get in touch with MLSDev to discuss your eCommerce operations and requirements. We’ll help you decide how to build a solution to improve customer experience and boost eCommerce sales.
Business Benefits of eCommerce Machine Learning
eCommerce businesses accumulate a lot of data. Machine learning uses data to make business, demand, and growth predictions. The possible applications of the technology are truly limitless.
Personalization
Machine learning for eCommerce allows companies to tailor the customer experience to their preferences and expectations. As a result, customers see more relevant recommended products, targeted ads, and even search results when they type a query in the search bar on your website.
Interactivity
Deep learning can also be used to create chatbots. These chatbots provide 24/7 customer support, improve the recommendation engine, boost eCommerce sales, and save money for a business. Another possible implementation is contextual shipping features to let customers ship whatever they want anytime.
Logistics and inventory management is another sphere where machine learning can make a huge difference. Implementation of the technology allows the data to be used to forecast demand. Real-time analytics allow for streamlined product delivery.
Another area is warehouse technology , where self-driving vehicles can speed up the picking process, reduce the strain on human workers, and automate error-prone operations.
Machine learning in eCommerce can also strengthen the security of operations. Fraud and anomaly detection tools powered by machine learning can ensure the safety of transactions, the protection of user data, and compliance with regulations.
Increased conversions
Higher conversions are what all merchants want, and implementing machine learning in eCommerce can help you achieve this goal. There are a few ways the technology can do this, and we will review them in more detail further in the article. Now, let’s just say that more relevant search results, personalized product recommendations, and highly targeted ads all contribute to higher conversion rates. In the end, you get better revenue.
Better marketing campaigns
eCommerce stores have access to a lot of information. With the help of machine learning, businesses can make sense of customer data in order to create tailored marketing campaigns.
For example, machine learning knows that when a potential customer visits an online store, searches for certain products, and adds them to the shopping cart but doesn’t ultimately proceed with the purchase. The next time customers visit your store, they will be recommended products from the category they’ve browsed before.
You can also easily segment your customers and target them with relevant special offers, discounts, and promotions via email or other marketing channels.
Improved in-house operational efficiencies
The importance of machine learning for eCommerce doesn’t only lie in boosting sales; the technology is also effective at reducing expenses.
Real-time insight into a business allows employees to operate better while spending less time on tasks. For example, deep learning can be used to forecast demand. Thus, you’ll be able to replenish your inventory so that you won’t run out of products but, at the same time, won’t have too many products in stock either, especially if we’re talking about products with a limited shelf life.
More informed decisions
- Will customers be interested in a new product?
- Should you increase prices for some products?
- Is there something wrong with your checkout process?
Whatever questions you have and decisions you need to make, eCommerce machine learning will provide you with the necessary data to make data-driven decisions.
As you can see, the benefits of machine learning for eCommerce are numerous, and there are quite a few areas where it can be used.
12 Use Cases of Machine Learning in eCommerce
Now that you know about the benefits of deep learning, let’s talk about some areas where it can be implemented.
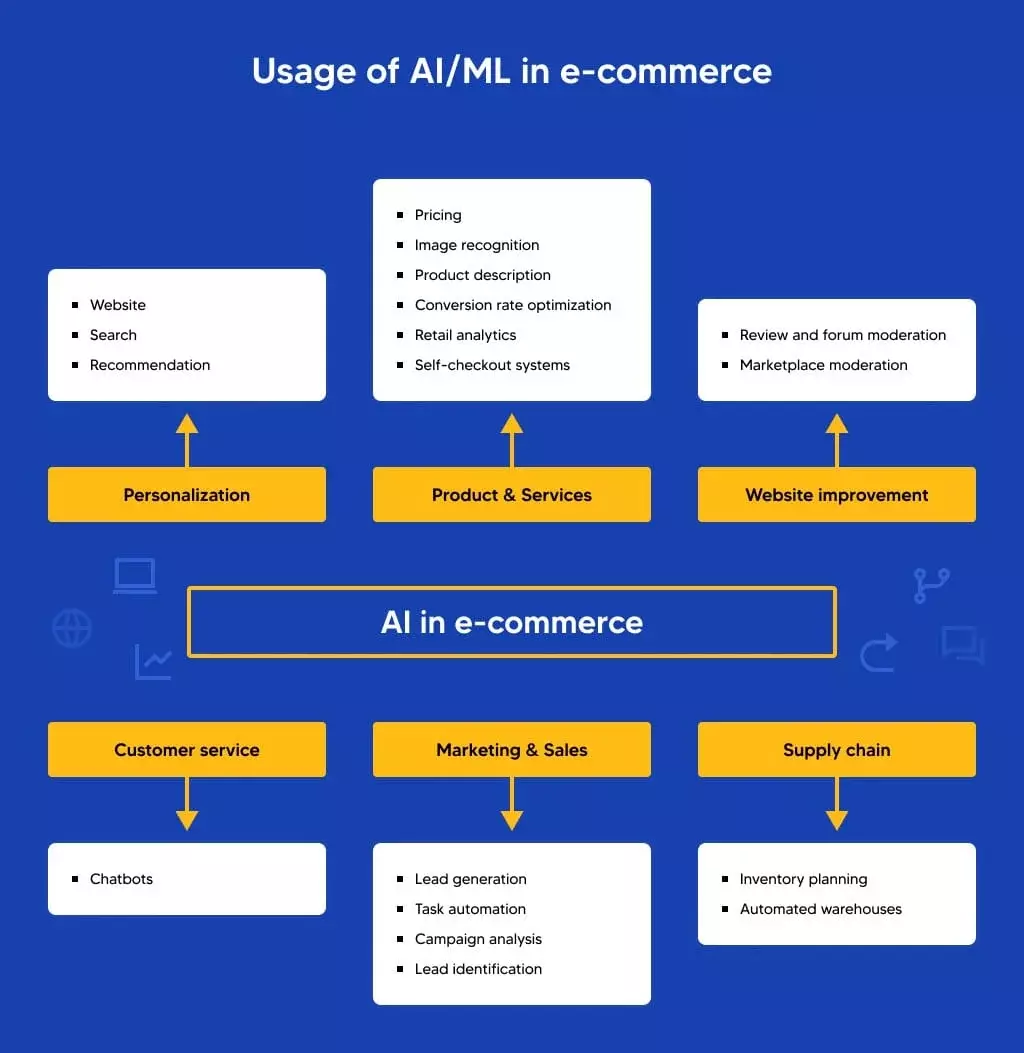
Today, you can use machine learning to provide customers with a personalized shopping experience, provide more relevant site search results, detect fraud, predict customer churn, and improve customer service. However, possible uses of machine learning for eCommerce don’t end there.
We’ve collected twelve use cases to illustrate the capabilities of this technology. Let’s take a close look at them.
1. Personalized shopping
There is no secret that a personalized customer experience boosts eCommerce sales.
According to research by Google , existing customers are 40% more likely to spend more than planned when merchants provide them with a highly personalized shopping experience.
What does it take to create a personalized shopping experience?
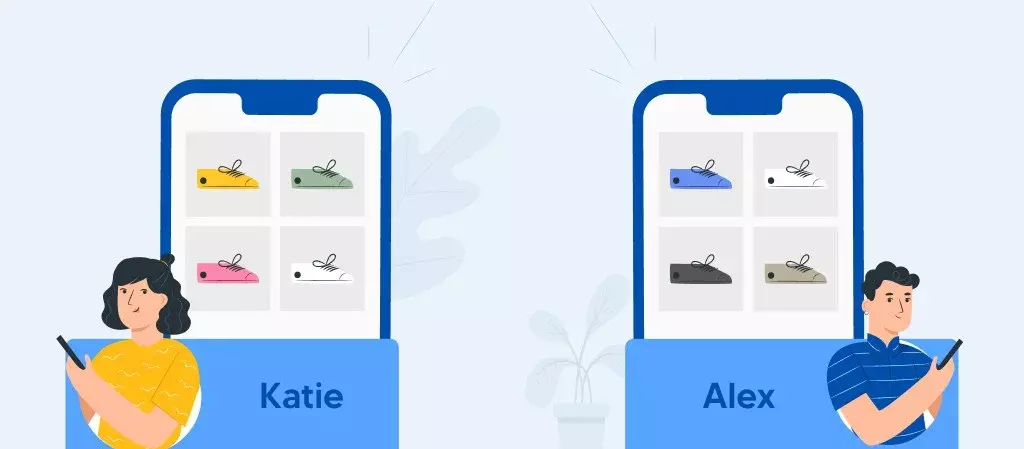
We usually mean personalized product recommendations when we talk about machine learning in eCommerce. eCommerce stores make recommendations based on historical data, preferences, interests, purchase history, and behavior.
However, you can take personalization even further, for example, by using customers’ location data and real-time weather forecasts, you can show products and banners that suit their current weather conditions.
You can also show a different message for different segments to target new customers. For example, for first-time visitors, you can offer 10% off of their first purchase. For return customers, you can offer free shipping or use the personal information you gathered during the first purchase to send them an exclusive discount for their birthday.
As you can see, there is no limit to what machine learning eCommerce personalization can help you do and achieve.
2. Omnichannel marketing & ML
Omnichannel commerce is focused on providing a seamless experience across all sales channels, such as a website, social media, and brick-and-mortar stores. Through omnichannel marketing, online businesses increase customer retention, purchase rate, and engagement.
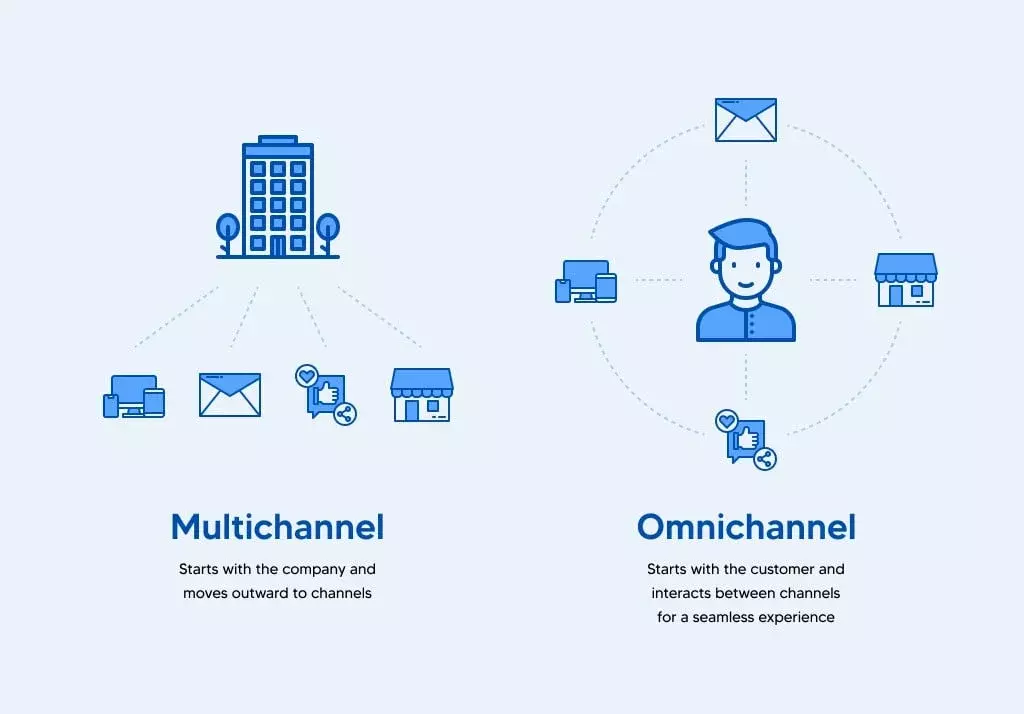
eCommerce machine learning can help you gather information across all sales channels and analyze how your customers behave over time. This will help you form a more unified image of your existing customers and choose a better communication strategy.
The data can be used to provide customers with a personalized customer experience at every channel and touchpoint.
3. Wise recommendations
As mentioned, product recommendations are integral to creating a personalized shopping experience. eCommerce recommendation algorithms can process past sales data, recognize recurring purchase patterns, and predict what products might grab customers’ attention.
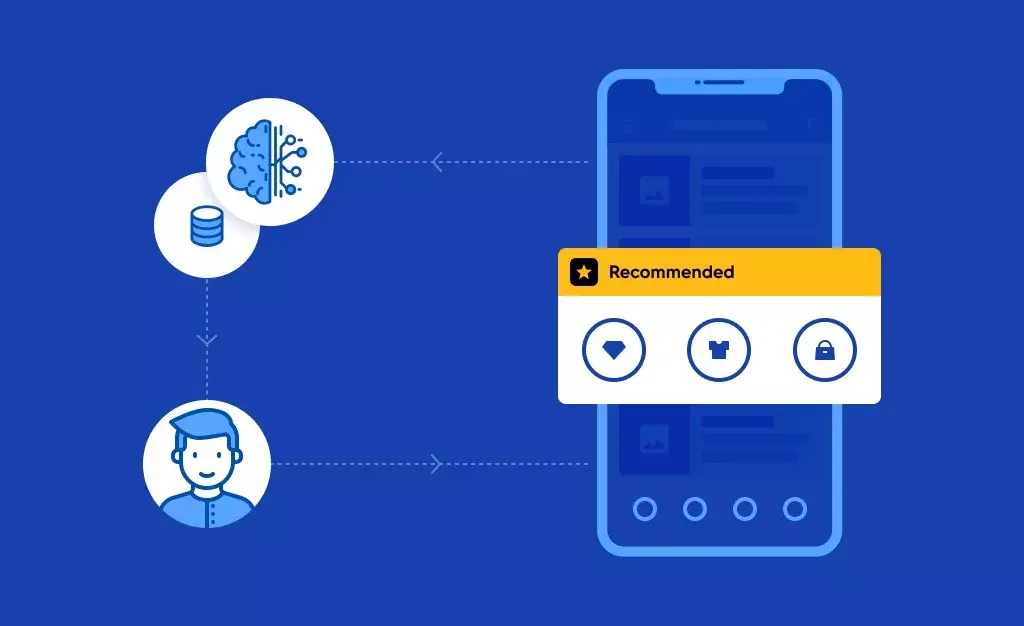
Recommendation engines usually use content-based and collaborative filtering approaches:
- Content-based recommendations analyze a customer’s purchase history and recommend other products with similar characteristics to those purchased before such as price, product type, etc.
- Collaborative filtering recommendations consider a customer’s behavior and compare it to the behavior of similar customers. Recommendations are then provided based on the products that have already been ordered and positively rated by other customers with similar buying patterns
5. Optimized pricing
Pricing is one of the key factors that influences the buying decisions of 47% of customers . This is the main reason eCommerce companies optimize product prices, taking into account internal trends such as demand, and external trends, such as competitors’ prices.
As a result, businesses can increase customer interest by offering more attractive prices for low-demand products or increase prices for high-demand products that competitors have run out of stock.
Dynamic pricing is an effective way to beat the competition and ensure customers will return to your online store for repeat purchases.
6. Optimized search results
Around 30% of eCommerce visitors use the internal site search to find the products they’re interested in. Those visitors who use site search are 2-3 times more likely to convert into customers.
Machine learning and artificial intelligence have made these searches even smarter. AI-powered search solutions like Algolia are typo-tolerant, have an autocomplete feature, and have a language-based option for tweaking the configuration of plural handling, stop words, optional words, etc. eCommerce search machine learning can also analyze customers’ behavior and tweak search results to include products that have the highest chance of grabbing their attention.
7. Visual search
Image recognition technology based on machine learning allows visitors to search for a product using an image. After the customer feeds in the photo, the image recognition engine matches the input information with the product database, then selects and displays the closest matches.
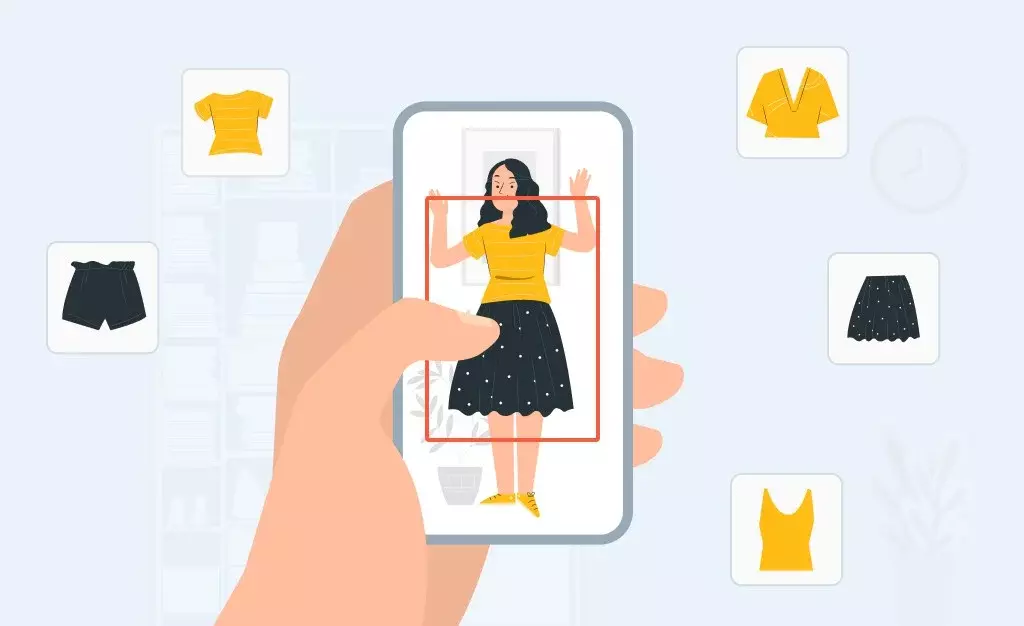
Visual search is a must-have for eCommerce businesses targeting Millennials and Gen Z. According to statistics, 62% of these generations use this feature over all other search methods. Visual search is also extremely effective for the clothing and make-up segments specifically.
8. Supply and demand management
Product inventory management is one of the most challenging tasks in eCommerce. Businesses need to understand how many products they need to meet demand. The task becomes even more challenging during the holiday season when sales can increase by up to 10% .
When you make the wrong predictions, you lose money.
Retailers can lose nearly half of intended purchases when customers encounter stock outages, which equals 4% of sales. Due to inventory shortages, a billion-dollar retailer could lose $40 million in sales during a single year.
Machine learning in eCommerce can help solve this challenge. Predictive machine learning algorithms analyze the data from past purchases and customer purchase patterns to predict future demand based on different factors.
As mentioned, there is seasonal demand and incidental demand. The first one is easier to predict, as it depends on the seasonal changes in consumer behavior. Incidental demand is caused by third-party factors such as changes in the economy or even in the epidemiologic situation on the other side of the world. This demand is much harder to predict, as many factors are involved.
9. Fraud detection & prevention
Fraud is the bane of the eCommerce industry. In the first half of 2022, the industry lost $3.56 billion to online fraud , which is an almost 50% increase compared to the same time period a year ago.
eCommerce fraud detection machine learning has the power to detect and prevent fraud. Machine learning algorithms spot recurring patterns among the datasets they process. They can also take note when something breaks the rules. For example, an unusual frequency of transactions can be a sign of fraudulent activity.
Aside from credit card fraud, machine learning can also prevent other types of fraudulent activity, such as Return to Origin fraud when criminals abuse the refund policy or account takeover, which is considered the most sophisticated type of eCommerce fraud.
10. Delivery optimization and autonomous vehicles
Machine learning can also streamline the processes of product shipping and delivery. For example, by analyzing information about traffic, weather conditions, and driver performance, the technology can accurately calculate the time of delivery and recommend the best route.
Amazon is taking this approach a hundred steps further with anticipatory shipping. The company uses machine learning to track customers’ purchase habits and predict future orders in order to transfer products to the closest warehouses. As a result, Amazon can provide one-day delivery with inexpensive shipping costs.
11. Better inventory management
As discussed, machine learning helps online stores better control product inventory; but what should you do if the system predicts a significant drop in sales and you already have these products in stock? Machine learning algorithms can help you decide how to boost sales with upselling, cross-selling, special offers, discounts, etc.
12. Predictions via ML
The technology can be used to understand your audience better and predict their future behaviors.
Here are a few things that ML can help you with:
- Predict Customer Lifetime Value (CLV) to better understand the value of customers.
- Predict the possibility of a purchase based on previous purchases and behavior. Based on the data, you can better understand when to send an incentive in the form of a discount, special offer, or related products to increase average order value
- Predict customer returns and purchases to better craft marketing messaging. For example, customers that are more likely to return might need less attention from your side, while visitors less likely to become repeat customers might require your special attention and additional incentives.
Useful eCommerce Machine Learning Tools
There are thousands of solutions with machine learning for eCommerce. Businesses can use the power of ML and AI for data-driven decisions, high-accuracy predictions, and a better customer experience.
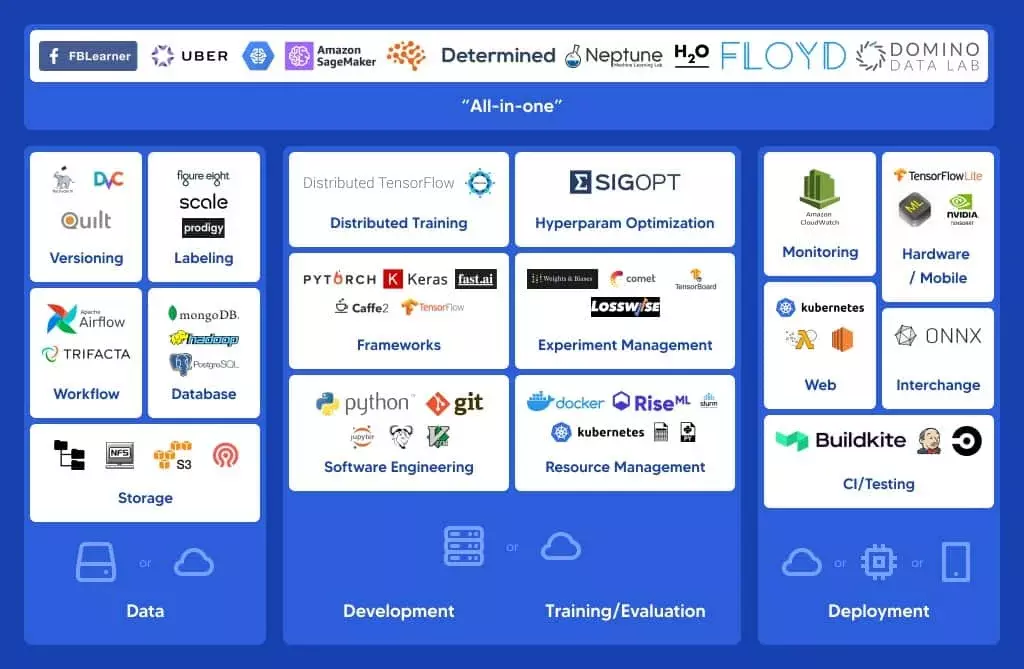
Here are some of the tools you might be interested in exploring:
- Deloitte Trellis – ML-powered platform designed to solve retail’s challenges around demand forecasting, replenishment, procurement, pricing, and promotions.
- Choice AI – this solution employs AI and ML algorithms that analyze customer data to predict fraud probability.
- Granify – analyzes customer behavior in real-time based on numerous attributes to provide the right information at the right moment for each shopper.
- Wiser – a dynamic pricing tool for eCommerce that keeps track of competitors’ prices to provide customers with the best offers.
- Syte – an eCommerce visual search platform that helps shoppers find the products they love.
- Algolia – one of the most popular platforms with powerful eCommerce search algorithms that help businesses maximize the speed of searches and the relevance of search results.
6-Step Process for Utilizing Machine Learning in eCommerce
You already know how ML can help solve business problems. You probably have some ideas about how these solutions can boost your eCommerce business.
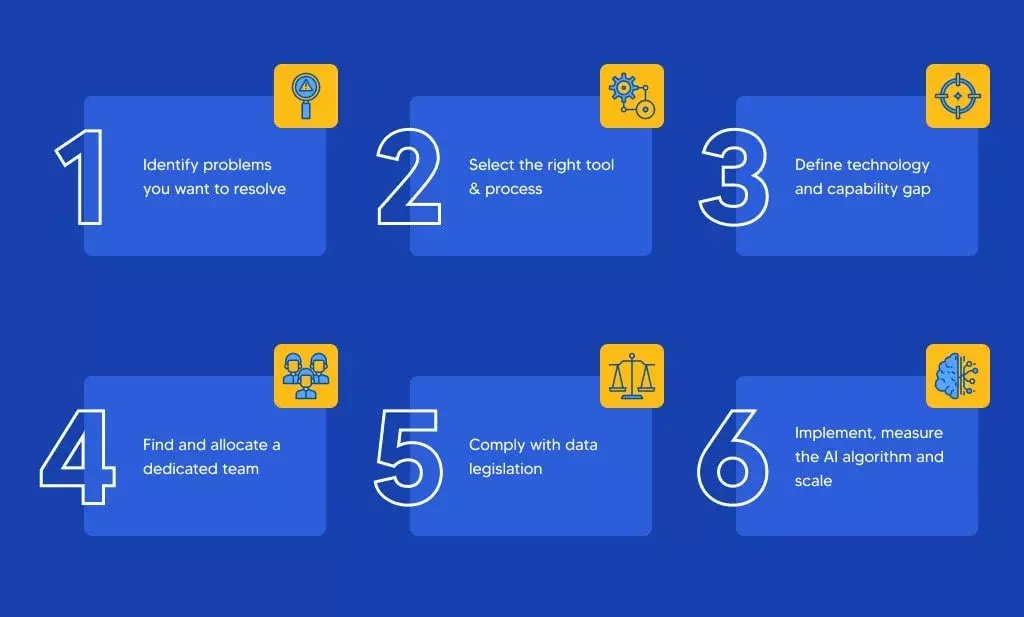
So, how can you get started with adopting this technology?
You can either choose one of the ready-to-go solutions or opt for custom development.
Let’s explore a 6-step process to implement machine learning in eCommerce.
Software development outsourcing is a challenging process. Read the guide Outsource Web Development Wisely: Useful Tips to learn about the best outsourcing locations, how to find the best team, and why development cost shouldn’t be the main deciding factor.
1. Identify problems you want to resolve
Let’s be honest, you can’t adopt technology just for the sake of technology. You need to understand your problems and what you want to achieve with an eCommerce machine learning solution.
The first thing to do is to find identifiable goals. For example, you may find that you have a high checkout abandonment rate. Your aim could be to reduce the bounce rate by offering highly personalized special offers and discounts. This is just one example of a specific goal you can achieve with machine learning.
You can either do the research yourself or hire a company that provides business analysis services to help you better understand how to strengthen your business.
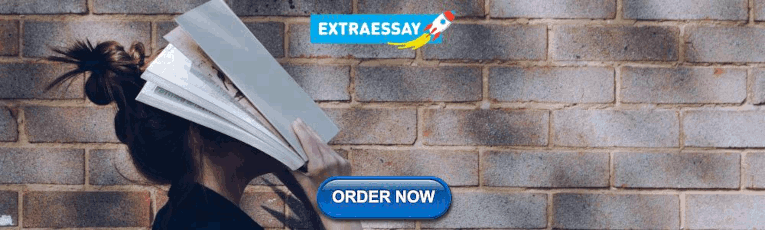
2. Select the right tool & process
A few options are available if you want to adopt machine learning for eCommerce. For instance, you can choose one of the ready-to-use solutions, some of which were mentioned earlier in this article. As well, you can invest in developing a fully customized ML-based eCommerce solution from scratch.
The first option is more useful for businesses with straightforward requirements. Big and enterprise eCommerce companies with specific business requirements might benefit more from custom development.
3. Define technology and capability gap
Machine learning solutions have different barriers to adoption. While some of them are straightforward and easy to use, others require an in-depth knowledge of machine learning and software development technologies .
Before making a decision, consider your business’s capabilities and tech resources. You might need to hire a development company to implement a ready-to-use technology in your operations.
4. Find and allocate a dedicated team
If you build an eCommerce machine learning solution from scratch, you’ll need to find a technology partner to help you with this task. You can hire in-house developers, an outsource team to work on your project part-time, or a dedicated development team with the ultimate focus on your project.
5. Comply with data legislation
Over the past few years, data legislation has increased significantly. For example, in the European Union, you must comply with GDPR (General Data Protection Regulation) and there is a CCPA (California Consumer Privacy Act) in the USA.
Any ML solution should be developed in compliance with these regulations. You’ll be charged huge fines if you’re guilty of breaching them.
6. Implement, measure the AI algorithm and scale
When you’re just starting out with adopting a new machine learning solution, you should start small in order to analyze a specific eCommerce data-set first. This way, you will be able to better test the insights, predictions, and results delivered. You can also find a company providing software testing services and have them test your solution before rolling it out.
When the performance of your new solution satisfies your requirements, you can further scale and expand said solution. Since machine learning deals with a lot of data, you need to ensure your servers can withstand a growing load. So, finding someone who provides DevOps services is the next step to take.
Final Takeaways: Implementing Machine Learning for eCommerce
eCommerce machine learning solutions help companies make data-driven decisions, provide a better customer experience, increase conversion levels, and boost revenue.
Machine learning in eCommerce can help you achieve several goals:
- Reduce abandonment rate
- Improve conversions
- Fix product inventory issues
- Improve demand prediction
- Add dynamic pricing capabilities
- Get better insight into customer behavior
- Provide a personalized shopping experience
- Reduce customer support expenses
- Provide better website searches
- Implement visual
- and much more…
Want to adopt an ML-based solution for your eCommerce business and need help with the task? Drop us a line and we’ll be glad to discuss your project and provide you with a rough project estimate.
Looking for an expert in adopting machine learning in eCommerce?
Get in touch to get a full elaboration on your concept accompanied by a detailed consultation from our business analyst and software architect. All is done to ensure the success and efficiency of using machine learning in your business.
Frequently asked questions
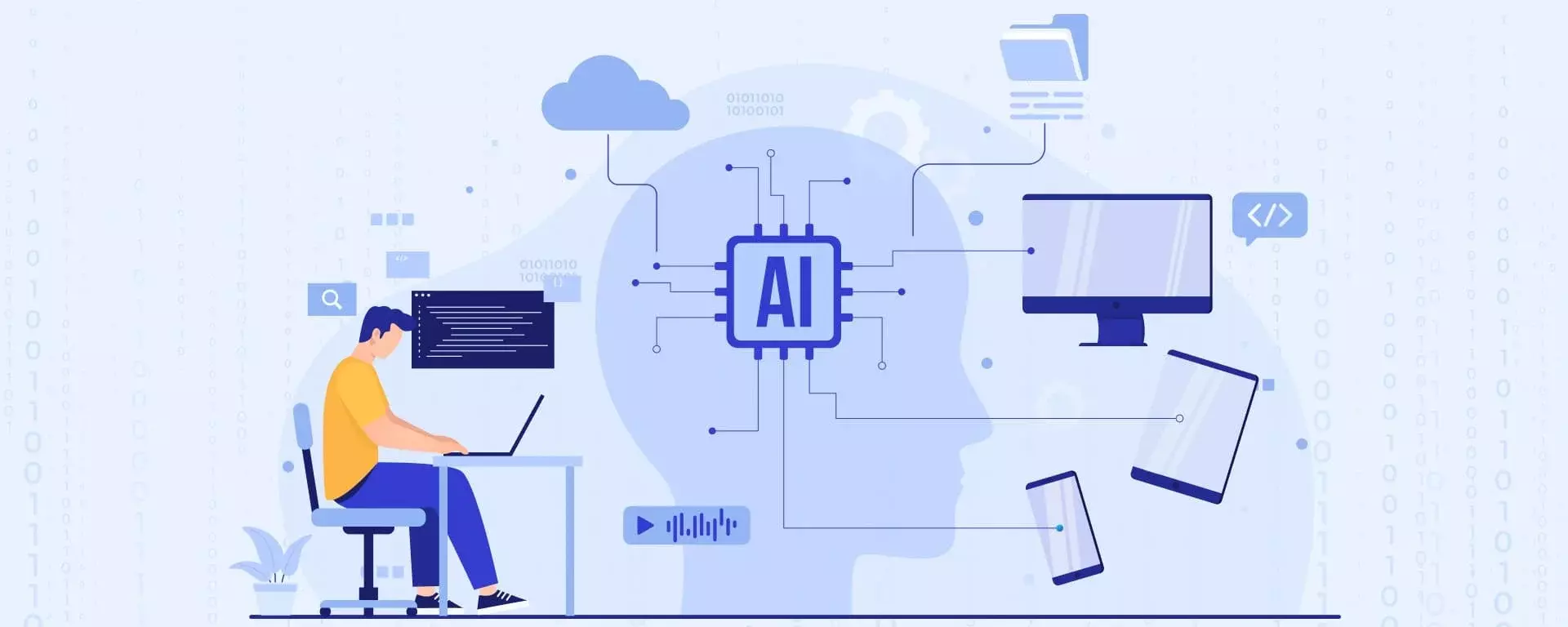
- Searchanise /
Everything You Need to Know About Ecommerce Machine Learning in 2024
Ecommerce machine learning statistics, machine learning in ecommerce, how can ecommerce machine learning benefit online store owners, ai and machine learning in ecommerce, searchanise personalised ai search, machine learning use cases in ecommerce, machine learning ecommerce case studies in the real-world, boosted conversion rates, targeted marketing, informed business decisions, efficient inventory management.
- Machine Learning: Ecommerce machine learning algorithms classify customers using demographics, behavior, and purchase patterns, forming the basis for creating focused marketing campaigns. Precision in segmentation is achieved by employing clustering algorithms.
- Artificial Intelligence: AI guides the overall marketing strategy, ensuring that segmentation is aligned with business objectives and that marketing efforts are coherent across various channels.
- Machine Learning: ML in eCommerce examines past sales data and external variables to anticipate future demand, frequently employing regression models and time-series analysis.
- Artificial Intelligence: AI oversees the holistic approach to inventory management, considering supply chain strategies, supplier relationships, and long-term business objectives.
- Results Based on Behavior: AI Personalization ensures that the search results for your users are tailored to their behavior. This means that their interactions with your store, such as viewing products, adding products to the cart, and completing purchases, influence the search outcomes.
- Factors Analyzed by AI Personalization: AI personalization takes into account various factors, including those mentioned above, plus the category and type of the product and customer interest in specific brands. For example, if a customer purchases a laptop from a particular brand, the AI will prioritize showing a mouse from the same brand when the customer searches for one.
- Flexibility in Adjusting Priorities: AI Personalization offers the flexibility to adjust the priority of customer actions, allowing you to fine-tune the search experience based on the importance assigned to different customer interactions, ranging from low to very high.
Product Recommendation Engine
Smart search, dynamic pricing, hidden markov models, leverage the power of ai with searchanise, related posts.
- Prompt Library
- DS/AI Trends
- Stats Tools
- Interview Questions
- Generative AI
- Machine Learning
- Deep Learning
E-commerce Machine Learning Use Cases: Examples
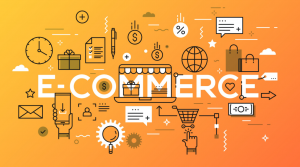
In e-commerce , machine learning can be used to improve a number of decisions thereby resulting in creating a positive business impact. Not only does it help e-commerce organizations increase conversion rates and find the best deals for their customers, but it also helps them understand the customer better. This blog post will look at various different use cases where AI /machine learning and deep learning have been used in eCommerce .
Table of Contents
What are some key machine learning use cases in eCommerce?
Here are some key areas in eCommerce where AI/machine learning can be leveraged:
- Product recommendation : One of the key use cases where machine learning has been used is to provide product recommendations for ecommerce websites. eCommerce businesses rely on product recommendations to drive more sales. Product recommendation helps increase onsite engagement and also boosts ecommerce conversions significantly. A product recommendation system helps increase sales because it provides personalized recommendations for each customer based on their purchase history & preferences (price range, color preference, size, style preference, etc.). It also allows eCommerce brands to upsell/ cross-sell existing customers through personalized promotions which are otherwise not possible without knowledge of shopping. Recommendation engines are designed based on a collaborative filtering algorithm that uses historical purchase data of customers who bought similar products or their buying patterns. There are different algorithms like user-based, item-to-item matrix factorization which can be used depending upon the business requirement. This helps eCommerce platforms provide more relevant product suggestions to their customers. A few examples include Amazon’s ‘Customers Who Bought This Item Also Bought’, Flipkart’s ‘Recommended For You’ feature among others. One of the challenges in product recommendation is the lack of traceability of users if he/she is not logged in. In e-commerce websites, given that the users might be untrackable as they are not logged in, and they can have rapidly changing shopping preferences, providing recommendations based purely on the interactions that happen in the current session is an extremely important and challenging problem. Many methods have been explored that leverage the sequence of interactions that occur during a session, including session-based k-NN (k-Nearest Neighbours) algorithms like V-SkNN and neural approaches like GRU4Rec. In addition, the approaches based on transformers architectures based on NLP techniques have demonstrated significant advantages over sequential and CNN-based approaches. T
- Pricing recommendation : Pricing is an important aspect of eCommerce and machine learning can be used to recommend the best prices for products. This use case was famously implemented by Netflix when it recommended different rental prices for its users based on their past viewing behavior. There are various ways in which machine learning can be used for pricing recommendation such as regression analysis, gradient boosting, Bayesian optimization, etc.
- Fraud detection : Fraudulent activities are a big concern for ecommerce businesses and machine learning can be used to detect them early. Machine learning models can be trained to identify typical patterns associated with fraudulent activities like abnormally high order values or customers placing orders from new IP addresses. Some eCommerce platforms like Alibaba & Amazon have built-in fraud detection systems that use machine learning algorithms.
- Delivery time prediction : eCommerce businesses face the challenge of accurately predicting delivery times for their products. This is important to ensure that customers are not left disappointed and also to manage expectations properly. Machine learning can be used to predict delivery times by taking into account factors like order size, item weight, customer location, etc.
- Personalized shopping experience based on customer segmentation : eCommerce businesses can use machine learning to identify different customer segments and then personalize the shopping experience for them. Customer segmentation can be done on the basis of demographics (location, age, gender), psychographics (lifestyle, interests), or buyer persona (needs & wants). Amazon does this very well with its ‘Customers Who Bought This Item Also Bought’ feature which recommends similar items based on a customer’s purchase history.
- Automated eCommerce chatbots : Chat is one of the most popular ways to engage with eCommerce customers and this use is expected to grow by 30% over the next few years. Machine learning can be used in eCommerce chatbot systems for a number of purposes such as personalizing the shopping experience, answering customer queries, generating reports & alerts based on user behavior, etc. There are cloud tools such as Amazon Lex , Amazon Kendra , Google Dialogflow , Azure bot engine , etc., which can be used to build your custom chatbots.
- Demand forecasting : eCommerce businesses like e-commerce marketplaces face the challenge of accurately forecasting demand for their products. Forecasting helps eCommerce companies to manage inventory, plan logistics & warehouse space accordingly, and also decide on pricing strategies. Machine learning can be used in eCommerce demand forecasting systems by taking into account factors like historical data (item sales volume), seasonality, holidays or festivals calendar, weather conditions, etc.
- Campaign optimization : eCommerce businesses use marketing campaigns to attract new customers and increase sales. Machine learning can be used to optimize these campaigns by identifying the most effective channels (email, SMS, social media) & target audience for each campaign. It can also be used to determine the best time of day or week to run a particular campaign.
- Product comparisons : Product comparison engines are a special case of a product recommendation system, in which a product detail page displays alternative choices in a table containing informative product specifications. There can be three different aspects of such a product comparison system including the following: A. Fast retrieval to narrow down products B. Further, shortlist the product from narrowed down results with high precision C. Rank the result. Fast retrieval can be achieved through algorithms such as K-NN over the embedding space.
- Product categorization : eCommerce businesses need to categorize their products so that they can be easily found by customers. This is often done using machine learning algorithms such as k-means clustering, latent Dirichlet allocation (LDA), or support vector machines (SVM). These algorithms can be used to automatically group similar products together based on factors like product features, customer reviews, etc.
- Predicting customer churn : eCommerce businesses face the challenge of predicting which of their customers are likely to churn i.e., stop doing business with them in the future. This is important for eCommerce companies because it allows them to take proactive measures to prevent churn and retain more customers. Machine learning can be used in eCommerce churn prediction models by taking into account factors like customer purchase history, demographic information, social media data, etc.
- Product search : eCommerce businesses rely on product search engines to help their customers find the products they are looking for. Product search engines use a variety of techniques to rank products including indexing of product data, ranking algorithms, and evaluating user feedback. Machine learning can be used in product search engines to improve the accuracy of results by taking into account factors like the popularity of a product, its price, customer reviews, etc.
- Product Question & Answering system : eCommerce businesses can use e-commerce chatbots to answer questions about the product like how it is different from another similar item or specific questions related to the products and their attributes. Customer spends a lot of time looking for answers in relation to a specific product on the product page and this is where chatbots can reduce this time providing valuable information in question and answer format.
- Customer lifetime value (LTV) prediction : Measuring customer future purchases and lifetime value is an important performance indicator for marketing management and budgeting. When the customer and firm have no formal agreements, this approach is nearly impossible. As a result, future purchases must be forecast based almost entirely on previous purchases. There are different solution approaches including statistics-based solutions and machine learning based solutions that can be used to solve the problem of predicting future purchases. A series of probabilistic models also termed “buy-till-you-die (BYTD)” models link simple past purchase summary statistics with a theoretically well-grounded behavior. The Pareto/NBD model, which assumes a Poisson purchase process and an exponentially distributed lifetime, is the most widely known BTYD framework. Then there are machine learning algorithms such as random forest which can be used to predict customer purchases and lifetime value.
eCommerce Machine Learning use cases are a great way to improve the e-commerce customer experience and drive more sales. There are several types of machine learning use cases such as product recommendation, product search, customer churn prediction, product categorization, etc. eCommerce businesses can also utilize chatbots for answering questions about the products they sell as well as using machine learning algorithms to predict future purchases or lifetime value of their customers that have no formal agreements with them. If you like to learn more, please feel free to reach out.
Recent Posts
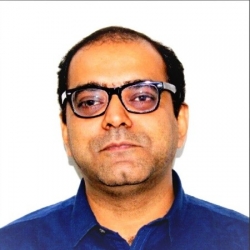
- Autoencoder vs Variational Autoencoder (VAE): Differences - May 8, 2024
- Linear Regression T-test: Formula, Example - May 7, 2024
- Feature Engineering in Machine Learning: Python Examples - May 3, 2024
Ajitesh Kumar
Leave a reply cancel reply.
Your email address will not be published. Required fields are marked *
- Search for:
- Excellence Awaits: IITs, NITs & IIITs Journey
ChatGPT Prompts (250+)
- Generate Design Ideas for App
- Expand Feature Set of App
- Create a User Journey Map for App
- Generate Visual Design Ideas for App
- Generate a List of Competitors for App
- Autoencoder vs Variational Autoencoder (VAE): Differences
- Linear Regression T-test: Formula, Example
- Feature Engineering in Machine Learning: Python Examples
- Feature Selection vs Feature Extraction: Machine Learning
- Model Selection by Evaluating Bias & Variance: Example
Data Science / AI Trends
- • Prepend any arxiv.org link with talk2 to load the paper into a responsive chat application
- • Custom LLM and AI Agents (RAG) On Structured + Unstructured Data - AI Brain For Your Organization
- • Guides, papers, lecture, notebooks and resources for prompt engineering
- • Common tricks to make LLMs efficient and stable
- • Machine learning in finance
Free Online Tools
- Create Scatter Plots Online for your Excel Data
- Histogram / Frequency Distribution Creation Tool
- Online Pie Chart Maker Tool
- Z-test vs T-test Decision Tool
- Independent samples t-test calculator
Recent Comments
I found it very helpful. However the differences are not too understandable for me
Very Nice Explaination. Thankyiu very much,
in your case E respresent Member or Oraganization which include on e or more peers?
Such a informative post. Keep it up
Thank you....for your support. you given a good solution for me.
Ecommerce Machine Learning Brings the Future to the Present of Online Shopping
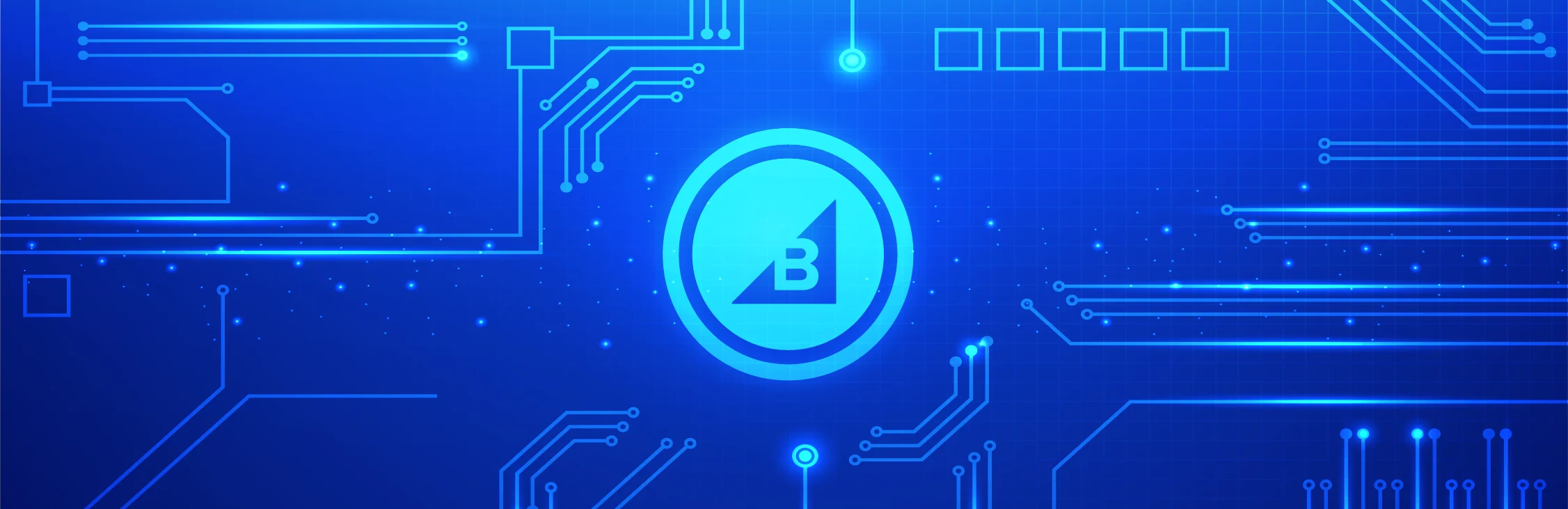
Learn more about our modern, flexible ecommerce platform.
One of the primary roles of emerging technology is to make humans more efficient and more effective. Technological advances help us to do more while using fewer resources, enhancing products and services and fueling innovation.
Ecommerce retailers are no different, having seen a wealth of evolution since the industry matured. This includes the introduction of machine learning, which has spun out of artificial intelligence to become a key application of that technology.
Specifically, ecommerce machine learning is the creation and refinement of applications and algorithms by artificial intelligence that “learns” from a steady flow of data. It involves artificial intelligence not just executing on a data set, but using data to test and change reactions to data.
It’s an evolving field for online retailers and ecommerce stores, but is starting to impact customers and users and new ways.
Much of the ad targeting on Amazon is handled by machine learning and even Netflix is using the technology. Approximately 75 percent of suggested viewing on the platform is fed to consumers by machine learning.
How Does Machine Learning Work?
By definition, humans are not involved in machine learning once a system is deployed. The technology is primarily machine learning algorithms that become more accurate and effective as more data is introduced. The application analyzes the data and outcomes and applies these “learnings” to new data sets.
Much like humans, machine learning involves trial and error before optimization is achieved. Unlike humans, machines aren’t hindered by little things like sleep and can operate 24/7.
Business Benefits of Ecommerce Machine Learning
Machine learning offers ecommerce companies new opportunities for providing an optimized customer experience for online stores. From the public-facing site to the logistics of fulfillment, machine learning is helping the ecommerce industry better meet customer needs.
Increased conversions.
Machine learning may boost conversion rates by optimizing on-site search engines and delivering informed product recommendations on ecommerce websites. Applying AI to both create “smarter” results that are more in line with how the customer acts and thinks.
Natural language processing more accurately predicts what customers are searching for. Product recommendations can be altered based on a customer’s purchase history. Both lead to customers finding what they want — quickly.
Create more relevant marketing campaigns.
Ecommerce platforms have more data than ever before to draw from. This is data that can be fed to an algorithm that shows what interests different customers or visitors to your website. That allows for more accurate customer segmentation. You can split your prospects based on their interests. That lets you target them with far more relevant marketing material.
Improve in-house operational efficiencies.
Algorithms can also deliver real-time insights to help you make your other operations more efficient. This is often found within logistics or supply chains where stock levels and inventory are monitored in real time. Machine learning can monitor customer demand to determine what products need to be ordered and when and can even predict future demand.
Make more informed decisions.
At the center of machine learning is analysis. Understanding data and how it impacts your business is key to fully leveraging the power of machine learning. A quality algorithm processes and understands large amounts of data quickly. This leads to better decision making.
Use Cases for Ecommerce Machine Learning
Machine learning can significantly enhance the online shopping experience, for the customer and the company. From product selection to inventory to security, machine learning makes the shopping experience smoother for all.
Personalization.
Modern consumers don’t want to be made to feel like just another number. They want a shopping experience personalized to their tastes. Machine learning can do this by analyzing past behavior to better predict specific products customers may be interested in.
A tailored experience means more eyeballs on products that customers have a proven affinity for. This can mean higher conversion rates.
Site search.
Consumers are used to getting the quality experience that Google provides — although many ecommerce site search experiences fall short. Machine learning can shrink that gap by using intelligent algorithms to include a recommendation engine or visual search, making it more likely to connect customers with what they’re looking for.
Managing supply and demand.
Inventory management and optimizing supply chains is hard — but machine learning can ease some of that burden. Accurately forecasting demand for certain products based on a variety of data-driven factors means you keep up with consumer demand, without wasting resources on unnecessary warehouse space.
Churn prediction.
Churn prediction is the rate at which consumers abandon a brand. It’s easier to sell to existing customers than new customers, so having an effective retention marketing strategy is key. Machine learning can optimize customer churn by accurately predicting when customers may be on the verge of leaving your platform.
Fraud detection.
Fraud detection and protection are essential parts of any ecommerce platform. The amount of data collected that is stored or in motion is massive and makes for a valuable target for hackers. Machine learning can better analyze transactions to understand what are genuine transactions and what are potentially fraudulent.
Improved customer service.
Ecommerce platforms are open 24/7 and expect customer support to do the same. That’s, of course, not always possible, but machine learning can make that possible by offering automated responses to basic customer service inquiries before referring more complicated issues to humans. This increases customer satisfaction and encourages purchases.
Dynamic pricing.
Pricing is not constant and, with the ever-fluctuating state of global markets, can change quite frequently. A machine learning solution that takes changes in materials, shipping and production costs into account and automatically alters pricing ensures that profit margins are maintained.
Product recommendations.
Customer data is invaluable for ecommerce companies. Leveraging purchase history, social media, searches or cart activity enables platforms to recommend similar products and encourage visitors to make additional purchases.
Automated chatbots for customer service.
As mentioned above, staffing customer service 24/7 isn’t realistic for most companies, although customers expect answers to questions around the clock. A chatbot powered by machine learning is a good solution to give customers a quality user experience without placing a major burden on staff.
How to Adopt Machine Learning in Your Ecommerce Business
It’s one thing to understand machine learning on a surface level. It’s another to execute on an effective business strategy. This process will help do just that.
Get familiar with everything machine learning.
Before you can leverage machine learning effectively, you must fully understand its capabilities. That means putting in the time researching the present state of the technology and understanding automation and deep learning.
Look into the AI-enabled solutions others are using and how they’re leveraging these platforms.
Leverage third-party expertise.
It’s very likely that you don’t have the requisite experience on staff to deliver a fully optimized machine learning solution. You may need to bring in outside experts — either a consultant or new staff member — to manage adoption across the organization.
Identify problems you want machine learning to improve.
Problems should be specific and easily identifiable. Having a grand problem you want to solve (“increase sales”) isn’t enough. They should be more granular, like “increase retention rates among first-time buyers.”
Applications of machine learning should be well-reasoned and well-researched.
Understand your technology and capability gap.
This step is best taken in concert with the previous one. When defining your machine learning goals, take your organization’s capabilities into account. Don’t dream bigger than your staffing or tech resources allow.
Create a team dedicated to implementing machine learning technology.
A project of this size must have dedicated resources, including manpower. Assembling the knowledge and skill sets to execute on a vision is a crucial step in ensuring a project delivers on its goals.
Measure and scale.
It’s advisable to start small and scale as more data is compiled that shows what works and what doesn’t. Once the initial implementation meets expectations, further applications can be explored.
How to Increase Ecommerce Sales
Explore our collection of free resources designed to help you scale smarter and accelerate your online growth from $1 million to $100 million.
The Final Word
How machine learning was initially viewed was the realm of science fiction. Although it’s not quite as grandiose as some of those depictions, machine learning is now a part of everyday life. Ecommerce companies that don’t embrace this technology are in danger of falling behind competitors that do.
It doesn’t solve all your problems, but it can make experiences and operations more efficient and, ultimately, deliver a better customer experience.
FAQs About Ecommerce Machine Learning
Can machine learning predict if a customer will purchase something, what is the difference between machine learning and artificial intelligence, should i use machine learning in my ecommerce business, browse additional resources.

DataScienceVerse
Best Data Science Blogs
DataScienceVerse is designed to help out analysts by producing the best blogs for data science with effectively tackles other AI-related problems. DataScienceVerse is a place to meet and trade thoughts about insights of data science articles to read with step by step solutions. DataScienceVerse Teams plan and priority is to provide the solutions of one of the most common and unique data science problems and tackle the big data problems by helping data scientists to stay up to the minute with latest libraries and technology
DataScienceVerse is designed to help out analysts by producing the best blogs for data science with effectively tackles other AI-related problems. DataScienceVerse is a place to meet and trade thoughts about insights of data science articles to read with step by step solutions. DataScienceVerse Teams plan and priority is to provide the solutions of one of the most common and unique data science problems and tackle the big data problems by helping data scientists to stay up to the minute with latest libraries and technology.

Machine Learning e-Commerce Use Cases
Table of Contents
Machine learning in e-commerce is a trend of retail digital transformation and is the deployment of self-learning computer algorithms that can automatically enhance their performance via experience.
Machine learning algorithms can be specifically trained by analyzing big and vast datasets, identifying recurrent patterns, relationships, and anomalies throughout all of the data, and creating mathematical models corresponding to such associations.
In the eCommerce business, recommendation engines and machine learning boost a company’s market share through improved customer acquisition.
e-Commerce Machine Learning Examples
I’ll start by giving you three exemplary models of machine learning in e-commerce .
- Asos : Asos was able to reduce return-related losses by two times while increasing revenues by 300% .
- Uniqlo : After searching their cellphones, Uniqlo connects clients to the locations that sell the desired items closest to them.
- Arcbazar : With the pricing recommendation that aids customers in making decisions , Arcbazar boosted customer happiness.
Most retail chains, regardless of size, have problems and objectives. Here are some methods that machine learning in eCommerce can assist you with improving your products and begin raising margins immediately! We truly hope you and your business will find them useful.
Here is a comprehensive list of areas where machine learning can be put to use right away:
Product Feed Recommender Engine
Amazon’s recommendation engine, which is totally based on machine learning, is one of the main reasons it continues to rule the eCommerce market. A few significant use cases for e-commerce machine learning exist. In the e-commerce field, personalization and recommendation engines are the hottest things.
By using machine learning algorithms for e-commerce and the processing of large volumes of data, you can comprehensively examine the online behavior of hundreds of millions of people.
You can use it as the foundation for creating product suggestions that are specific to a certain clientele or demographic (auto-segmentation).
How does the e-commerce recommendation engine operate?
Let’s have a look at how the e-commerce recommendation engine operates. The evaluation of vast amounts of information obtained on the current website traffic can reveal which sub-pages the client used. You could tell what he was seeking and where he was spending the majority of his time.
Additionally, results will be provided on a tailored page with suggested products that would most likely interest customers based on a number of factors, including location, weather, social media data, previous customer activity, and preferences (such as preferred color).
Businesses like Netflix, YouTube, Amazon , and others employ Python-based recommendation engines to make it simple to access their microservices.
You may also share your comments and propose products that nearby customers have bought by using recommendation systems. And that’s just one illustration of how machine learning may be successfully used in e-commerce.
Personalization of Content on the Website
Properly tailored content enhances conversion and user engagement on the website or mobile application. Machine learning algorithms for e-commerce enable the selection of the finest range. As a result, algorithms may detect patterns in data after processing vast amounts of both organized and unstructured data (including images and text).

In e-commerce sites, machine learning algorithms take into account a number of variables, including preferred color and design, image quality, profiling, preference, etc. Each person’s specific tastes are taken into account while determining the website’s findings. In this manner, suggestions for employing machine learning in eCommerce may assist you in boosting your sales.
Why should you personalize content using machine learning?
Only artificial intelligence (AI), and more specifically, machine learning, can give high levels of personalization online. To customize the user experience for each site visitor, algorithms examine client data and behavior.
Dynamic Pricing
Pricing strategy is crucial in today’s cutthroat marketplaces. Businesses are well aware that before making a purchase decision, consumers want to check prices for the same goods on various e-commerce platforms.
With the help of machine learning, retailers may calibrate pricing based on factors such as supply and demand, the minimum price, operating costs, competition, etc. To accomplish everything manually would be challenging. For improved real-time dynamic pricing, machine learning automates enormous volumes of data collection, processing, and analysis.
A/B testing with AI
Although it might be challenging, A/B testing is a helpful tool in internet marketing. Consider that you wish to compare two versions of a product page.
You see, if you modify multiple things, you can never be completely certain of what will result in the subsequent positive or negative change. However, if you only make one modification, it can be so slight that it goes unnoticed.
What KPIs should be tracked too?
Yes, the most obvious metrics to monitor are conversion and purchase rates. However, factors such as the amount of time spent on the page, the number of clicks, and the return rate are also significant.
Using machine learning in e-commerce and AI simplifies this testing process.
The software automatically creates versions based on previous data and decides which components should be reviewed. Depending on the outcomes of tests, it can update website elements dynamically. For instance, showing pages differently for various locations or demographics.
Results for Enhanced Search
It is essential for an e-commerce platform to keep all necessary products in stock, but even more significant is for customers to easily find the items they’re looking for. Therefore, the effectiveness of the search feature is crucial in determining whether or not your clients stay on your platform.
Keywords drive searches, but not all customers are experts at using them. Machine learning in eCommerce has a way of helping customers quickly find products. Machine learning algorithms recognize patterns in search, purchases, clicks, and preferences to produce improved search results.
Customers can quickly locate the things they’re seeking thanks to search results based on machine learning, which also recommends related and pertinent products.
Machine Learning e-Commerce Fraud Detection and Prevention
First-time online buyers frequently have concerns about the safety of e-commerce platforms. Additionally, fraudulent operations involving user data, transactional information, etc., target specific e-commerce businesses. Such data breaches hurt not just the company’s reputation but also its clients, forcing it to close its doors.
Therefore, it has become crucial for businesses to invest in cutting-edge technology like machine learning and artificial intelligence to identify and stop fraud.
Machine learning can quickly evaluate vast volumes of data and detect odd behavior in fraud detection, helping to prevent fraud. And this also implies that automated data processing and anomaly detection will be quicker and more effective than human-performed manual analysis.
Machine Learning in e-Commerce| Case Studies
Several pro-guides of eCommerce machine learning examples are provided below.
ARCBAZAR : A FINELY TUNED PRICE RECOMMENDATION SYSTEM FOR IMPROVING CUSTOMER RETENTION IS ARCBAZAR.
One of the eCommerce machine learning published studies is the recommender system for our client. The world’s largest platform for architectural and design competitions, Arcbazar, has worked with Greenie as a technical partner for many years.
Recently, we developed a recommendation system based on machine learning for them to improve their client experience by recommending pricing for competitions.
The technology analyses historical data from more than 15,000 strategic initiatives to establish consumer intent. Following that, it gives customers the go-ahead to reward the winning entry from the competition with a fair and appropriate incentive.
HELLOAVA : Personalized Product Recommendations for Improving Customer Experience
The goal of the personalized skincare platform HelloAva is to choose the best products for particular skin types. Customers must create a user profile and answer a survey about their skin in order to receive recommendations. The data were analyzed by the ML system, which then assigned a consultant based on data from dermatologists and selected by aestheticians from a pool of 2,000 products.
BOOHOO : Aiming for Better Product Discoverability Through Independent Research
This added an image recognition-based visual search feature to their mobile website. Customers upload photographs, and the system searches the inventory for items that are comparable.
The numbers were outstanding, even during testing. In comparison to situations where consumers didn’t use the new function, the conversion rate rose by 100%. The number of pageviews rose by 135%. The average order value also increased by 12%. This is how a rise in sales was caused by just one ML-based feature.
ASOS : ONE ML SOLUTION FOR 3X VALUE
Finally, one of the biggest online clothing retailers, Asos, integrated machine learning (ML) into its website to better assist clients in choosing the appropriate size. The new sizing tool was known as Fit Assistance. In short, customers must disclose information about their preferred fit, size, weight, gender, thigh and waist measurements, and size for well-known brands.
Similar Posts

R and Python debate: My Personal Experience and Insight on which one is the Best
Data science is a field that’s constantly evolving and growing, and with it comes a never-ending debate: R and Python? Both languages have their own set of strengths and weaknesses, and choosing the right one for your data science projects can be a daunting task. In this article, I will share my personal experience and…

Declarative Machine Learning: An Advancement Even Your Child Should Do
Declarative Machine Learning (DML) is a type of machine learning that uses a declarative programming language to define and train models. This approach is based on the idea that the programmer should only have to define the goal of the machine learning task and the model should be able to learn from the data and…

Data Science For Absolute Beginners – Avoid 6 Common Mistakes
We’ll talk about six common mistakes to avoid for Data Science Absolute Beginners. So, are you new to data science? In that case, you most likely can’t wait to begin exploring the realm of machine learning and predictive analytics. We are all aware of how competitive the field of data science is right now. Some…

Comprehensive Step-by-Step Guide to Linear Regression in R
Welcome to our comprehensive step-by-step guide to Linear Regression in R. In this article, we will take you through the basics of Linear Regression, its applications, and how to use R to build Linear Regression models. We will cover everything from understanding the theory and assumptions behind Linear Regression to data preparation and exploration, building…

Let’s Find out How To Apply Natural Language Processing to Machine Learning
Natural Language Processing (NLP) is a powerful tool used to analyze and manipulate language. It can be used to help train Machine Learning algorithms and improve their accuracy. In this article, we will discuss how to apply NLP to Machine Learning, and the various techniques that can be used to do so. We will also…

Why to Migrate Web Development and Ace in Data Science Fast
Nowadays many web developers are beginning to wonder if they should migrate from web development to data science as a future career. The industry will continue to be more data-driven in the future. So, is it accurate to say that changing careers to data science may help businesses satisfy their needs? Web development jobs appear…
Leave a Reply Cancel reply
Your email address will not be published. Required fields are marked *
Save my name, email, and website in this browser for the next time I comment.
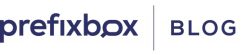
Prefixbox Blog
eCommerce search dedicated to you
12 Best Machine Learning Strategies for E-commerce Businesses
In today’s world of E-commerce, it’s impossible to run a successful online shop without leveraging Machine Learning.
Check out this article to find out how to incorporate artificial intelligence into your e-Business in these easy ways:
- For price optimization
- For search result optimization
- For product recommendation
- And many more
Let’s get started!
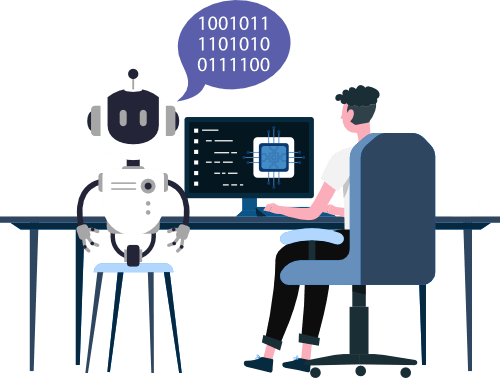
How machine learning works – AI Use cases in E-commerce
1. pricing optimization, 2. segmenting, personalization and targeting customers, 3. search results optimization, 4. product recommendations, customer lifetime value prediction, predicting if a customer will make a purchase, predicting customer return (and purchases), customer churn prediction, client size prediction, 6. site search autocomplete, 7. a/b tests using ai, 8. chatbots for automated customer support, 9. inventory management, 10. omnichannel marketing boosting with ml, 11. image processing and recognition, 12. fraud protection.
Today, when global E-commerce sales amount to $4.88 trillion , it’s an innovator’s game.
According to Gartner , up to 80% of customer interactions are managed by AI today.
Introducing AI into sales, marketing, even inventory management is both a natural next step in the evolution of commerce and an absolute must for those who want to keep up and make cost-effective decisions.
And in this article, we are going to show you how you could effectively be leveraging AI in your E-commerce business.
Let’s kick off with the answer to….
If we want to define machine learning in the simplest terms, it is the study and creation of algorithms that can learn from given sets of data and extrapolate from those to predict trends and outcomes .
This is a necessary function of any algorithm-based system that has to deal with large, frequently changing data sets – from natural language searches (where wording, typos, and new phrases are common) to facial recognition (where the system basically never meets the same input twice, but still has to determine the key features). Machine learning is used in email filtering, weather forecasts, diagnosing disease and predicting socioeconomic trends.
E-commerce is a field in dire need of machine learning. Even a small webshop can create millions of relevant data points daily that a single human, or even a team of experts could never hope to fully comprehend and utilize.
But, a machine can do it.
It will not only monitor these data sets, changes and trends in them, but make connections.
An AI based on machine learning will reason . Deduce, based on experience.
For example, it can help with…
Price is, unsurprisingly, one of the most important factors when considering a purchase. In fact, price is one of the major drivers for at least 47% of customers according to BigCommerce .
If your prices are high – or just higher than your competitors’ – your cart abandonment rate can increase, and even more so if you don’t set shipping prices correctly. If your store offers international shipping, you also have to display prices based on the location of the customer.
There are many other factors that may influence your prices – supply and demand, which promotions are running, what time of the day or year it is and so on.
Via machine learning, these factors could be assessed in a fraction of a second and your site will display dynamic pricing: showing the customer the most up-to-date price for them based on the aforementioned factors.
This ensures prices are instantly understood (no “how much is that in my currency” , “does that include shipping” etc.), with no surprises , and no need for you to manually change them.
In traditional brick-and-mortar stores, segmenting was done solely by salesmen, who approached the customers. By observing and asking a few questions, they could gather more than enough information about demographics, their needs and doubts, and their overall mood based on non-verbal communication.
This made it possible to immediately address any concerns about the products, matching the words and expressions used by the customer, being able to upsell them on the spot, and reinforcing their purchase intent.
When the customer has to deal with an online experience, all of this has to be replaced, and scaled.
Segmentation has to be based on behavior that can be measured.
And on-site search is a great place to start.
When searching, shoppers use natural language , which gives clues about their background and native language. Based on the results they click on, an algorithm can determine what they are looking for and offer more relevant results, which encourages them to make a purchase.
And based on what other customers in the store previously bought, in conjunction with this particular search, cross- and upsell offers could be made with great confidence. Part of the demographic information is readily available as soon as someone signs up – most times you will know their age, location and whatever data you ask for.
You can also monitor their behavior on your site – what content do they read, what materials do the download, how often do they return and how often do they make a purchase.
You can also keep track of the kind of emails they open and when are they most likely to make a purchase during a day, a week or year.
There is an incredible amount of data generated from all these interactions – and machine learning is what makes it possible to assess behaviors to ascertain patterns. These patterns help you make sense of data sets and make more effective marketing decisions. For example, you can create ultra-targeted campaigns with the most relevant messages, and increase your conversion rate by offering customers exactly what they are looking for.
Providing search results based on keywords is just the very first, most basic step in on-site search. In order to provide shoppers with the best experience possible, your search should go much deeper than that.
Given a large enough data set, you could determine which results are better for people in certain locations, how to optimize E-commerce search result filters , and which products best match their needs based on their previous behaviour.
By analyzing the data and figuring out what items go together , you can also recommend similar products, and even cross-sell items that are frequently bought together by your users.
Machine learning is able to pinpoint the trends and patterns needed to automatically determine these.
Leveraging this leads to higher click-through rates on your results pages, higher conversion rates, and a higher average order value.
It’s worth noting that filtering results in real-time based on user inputs, via faceted search , paired with AI-based recommendations is currently the single most effective method of providing relevant results.
But let’s stay for a minute with recommendations…
If you’re curious about how effective automatic product recommendations are, look no further than Netflix. According to McKinsey , 75% of what people watch on the streaming platform is suggested to them via an algorithm that analyzes user behavior. (The same is true for 35% of purchases at Amazon.)
You might think that determining which products go well together is easy – for example, simply track what people watch after they finish Futurama, and if it’s Rick and Morty, recommend that, and that’s it.
But you should also take into account the demographics – people in non-English speaking countries might go for movies and series in their native language with more enthusiasm. The preferences of young and older audiences will differ, as will the taste of those living in East Coast metropolitan areas versus those in rural European towns.
And this doesn’t even account for personal taste of genres, styles, lengths, eras etc.
To be able to offer ultra-targeted products to your customer, you have to work with a very large number of variables, each weighed differently.
Learn more about the most important related search features to increase conversion rates & revenue by 6%.
It is virtually impossible to do this without a machine learning algorithm – even if you have just a few hundred products.
As you can see, knowing your customers is a bit like predicting the future.
To prove the importance of providing the shopper with the right product suggestions throughout the customer journey, check how significantly Rossmann’s conversion rate and average order value improved by utilizing AI-backed recommendations:
5. Predictions About Your Customers
Machine learning can tell you a lot of things about the people who visit your site and make a purchase – even things like how likely they are to buy from you again or what they might be interested in.
Check out what machine learning can predict.
To fine-tune your communication and messages, it’s useful to know how much money a customer is likely to spend in your store over a given time period.
If you can estimate a lifetime value based on behavior, you can also make your marketing more cost-effective and targeted.
You can also identify customers who are the most valuable and deserve special attention.
Imagine this: you have an online store for office supplies. You have a customer who orders the same amount of ink cartridges from you about every 6 months.
Let’s say 5 months pass, and this customer logs into your site, but doesn’t order anything. Logically, this could mean that they are checking the prices in order to budget the next order, or they might be comparing your prices to your competitors’.
As a store owner, you most likely will never learn that they’ve logged in and done this.
However, the AI will notice it.
And it can deduce that this is the right time to provide a little incentive to increase average order value – as the customer is a returning, but hesitant one, maybe it’s time to fire up a workflow that provides them with a personal discount for their next order, thanking them for their loyalty.
If, based on their behavior, a customer is likely to return to your store later, then an entirely different marketing message may resonate better with them .
Algorithms can predict, on the long term, whether this is the case and can initiate longer workflows with messages that target loyalty and reinforce branding.
Retaining existing customers is one of the most important tasks if you want to keep your marketing budget from bleeding. Acquiring new customers is much most cost-intensive.
A machine learning algorithm can determine which shoppers are the most likely to abandon your site – based on things behaviour such as: returning to your store less frequently, smaller purchases, etc.
When the AI realizes this is the case, it can initiate workflows targeted at retention, giving shoppers incentives to stay.
Based on average order value and purchase frequency, along with other information like number of employees, and company type, an algorithm can estimate the size of the client for you .
This gives you great insight into deciphering which potential customers to pay special attention to. You can make more personalized offers and give them deals that are more cost-efficient in the long term.
As you can see, machine learning really tells your future by predicting what your customers will or won’t do.
Another forward-looking application of machine learning that deserves its own subsection is…
A truly useful autocomplete has to learn, instead of scouring through different product attributes and description.
It has to understand the natural language of the users instead of the often technical and machine-like phrases of database entries.
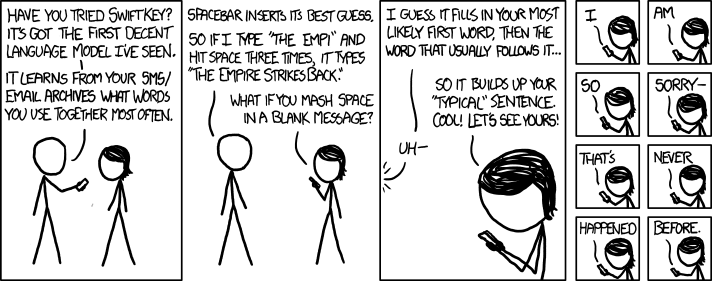
E-commerce search best practices dictate that online stores use an artificial intelligence-based autocomplete as it streamlines the shopping journey and provides shoppers with a sense of comfort as this is what they’ve come to expect.
So, natural language processing and machine learning have to understand the kind of language and phrases your customers use, how often they use them, if the results are satisfactory for those phrases, and even common typos along with the correct spelling.
Learn more about the most important search autocomplete features to decrease zero-result pages by 31% and increase cart value by 19%.
This way, every user can feel that the search engine is truly there to help and pays attention to the smallest details, all without being annoying.
Speaking of details…
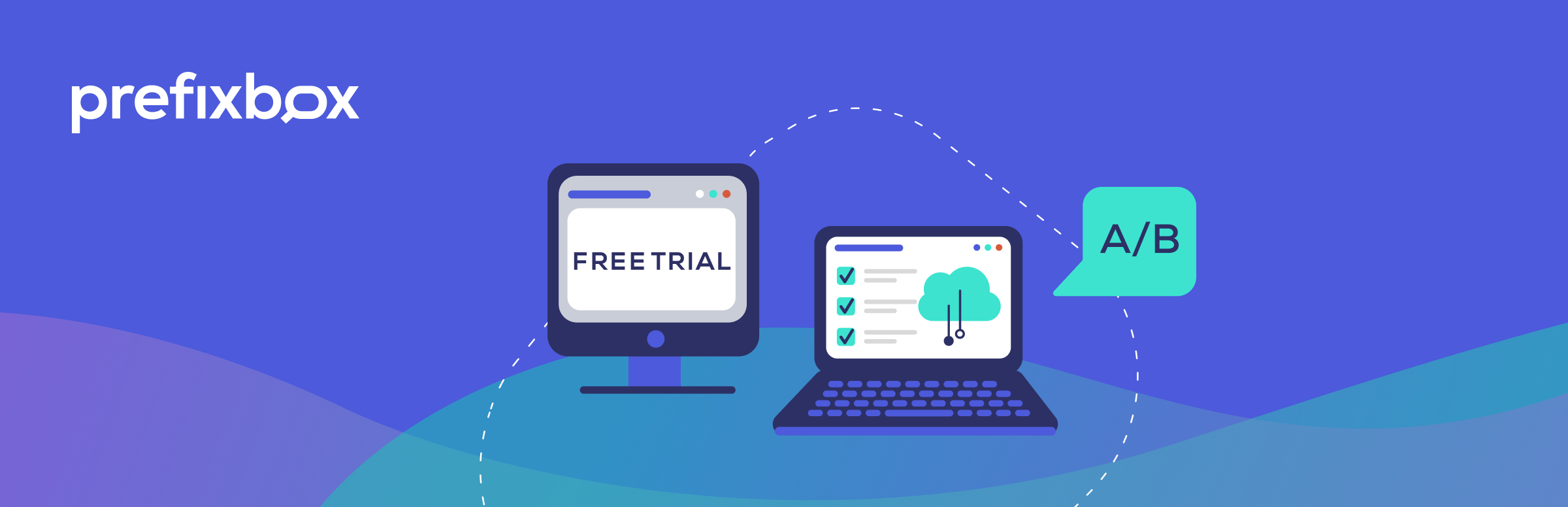
Try Prefixbox for Free
Increase your site’s shopping experience for increased conversion rate and revenue!
Thank you for your request!
We will be in touch soon to:
- Understand your requirements
- Discuss the integration process
A/B testing is a powerful tool in online marketing, but it can also be tricky.
Let’s say you want to run an A/B test for a product page.
Firstly: what do you change? The display of pricing? The location of your CTAs? The background color?
You see, if you change more than one thing, you can’t really be sure what causes the next positive or negative change.
But, if you change only one thing, the change may be so small it can’t even be registered.
And what KPIs should you track?
Yes, conversion and purchase rate are the most obvious numbers to track.
But time spent on page, number of clicks, and return rate are also important.
Machine learning and AI makes this testing process easier:
- Based on historical data, it decides which elements should be tested and automatically creates variants.
- It can dynamically change page elements based on test results. For example, displaying pages differently for different demographics or locations.
It can find the optimal versions much faster, because it can take all the variables into account and find the connections between even small changes.
With customer support, there rarely is an optimal choice. If you want to solve all issues with manpower, your support team will be huge and expensive – and not at all efficient, as many times they will be dealing with things that could be solved by redirecting the customer to an FAQ page.
On the other hand, you can’t completely automate support , because a lot of issues will require human assistance, and your customers will quickly get annoyed if they can’t get it.
The solution to this problem is often implementing a chatbot based on machine learning.
These chatbots are able to maintain a conversation with the customer. Not only by using previously defined answers, but also via AI – they’re able to learn about natural language from every conversation.
Of course the chatbot will require time to learn, to get to know the products and services as well as the customers and their way of communication.
It will never pass a Turing test, but it could, in time, identify opportunities for upsell, create customized coupons, and open tickets for humans in customer support to handle.
Keep in mind, however…
A professional custom chatbot can cost anywhere between $30,000 and $300,000, depending on the desired functionality.
Speaking about money well spent…
Artificial Intelligence recently opened another avenue of sales for E-commerce stores by combining online shopping with chatbot assistance and messaging apps. This new means of shopping called conversational commerce , can not only enhance the customer experience, but improve conversion rates, and increase online sales overall. Check the video for more details:
You know how a smart fridge reminds you you’re out of milk and puts it on your grocery list for you?
ML does that for E-commerce, times a billion.
Well, a trillion actually, as around 7% of the US GDP (more than $1.1 trillion) is tied up in cash in inventory along with accounts receivable and accounts payable.
Inventory management and logistics is nothing to sneeze at in terms of complexity.
If you run a successful online store, you have to monitor your stocks, reorder items, predict trends in demand, coordinate contractors, deal with manufacturers, suppliers, mailing services, and manage your revenues accordingly.
This is exactly the kind of job machine learning was made for.
By monitoring all your inventory, and even predicting future trends in supply, demand and even cash flow, you can be sure that with AI, you won’t be the airline that overbooks flights or flies empty.
You will be the airline that plans their next year schedule with absolute confidence.
Let’s get the obvious out of the way: omnichannel marketing brings you higher retention and conversion rates and boosts your revenue. But only if you use the available channels wisely.
Of course, you could just hire a marketing team, where there are dedicated people for social, email marketing , content creation, and tell everyone to give it 100%.
But is there a better way to do it?
Actually, yes.
Analyzing the data your customers create when interacting with your activities on those channels is majorly important.
Based on customer behavior – ads that perform well, content that’s frequently read, email open rates – machine learning algorithms can analyze your messages and display them in a way that ensures every customer gets the perfect one.
Online real estate became more important than television and radio partly because of how results can be tracked and campaigns can be optimized.
ML is the natural next step in this evolution.
Image recognition can be a great tool for an online store with thousands of products in their inventory.
Ideally, it enables a customer to just upload a photo they snapped of a given product at home or at the store. The system then processes it on the store’s servers, and instantly displays a response with availability, current price, shipping info so the shopper knows where to buy it.
More often than not, this leads to a purchase as it’s the most convenient shopping method.
Beauty.com, for example, was able to increase sales by 15% after implementing their visual search feature.
Another possible use of image processing is to give ultra-targeted recommendations. For example, processing a photo of the customer and then applying clothes available in store, so they can see how different styles would look on them.
Fraud is inseparable from commerce as a whole, and E-commerce is especially vulnerable.
Online shopping offers huge opportunities for those who want to take advantage of automated systems, which is why it is important to have algorithms in place that can detect fraudulent activity.
Integrating a CAPTCHA is not enough . You also have to monitor behavior and look at how certain people use your site.
With machine learning, you can identify repetitive patterns that don’t match with human behaviour; such as: filling in forms too quickly, opening dozens of pages for split seconds, entering multiple different sets of information rapidly at checkout, etc.
Introducing artificial intelligence in E-commerce processes is not something futuristic or even a highly complex task. There are applications and services readily available for most functions that have been developed by people over the past decade.
It’s not always cheap, but it’s worth the cost.
- It will help you understand your customers and audience better
- Increase your sales and average order value
- Cut unnecessary work
- Give you deep insight that no human ever could
You don’t have to hand over everything at once to the machine of course. Start small. Implement a smart site search solution, start using recommendations based on machine learning – and get to the rest when you are ready.
But get moving, because the analogue methods are phasing out fast.
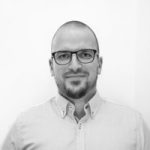
Balazs is an Online Marketing Manager at Prefixbox, a leading AI search solution provider for E-commerce sites. He is a marketing enthusiast from Budapest, who is interested in new technologies and solutions and believes in the power of search.
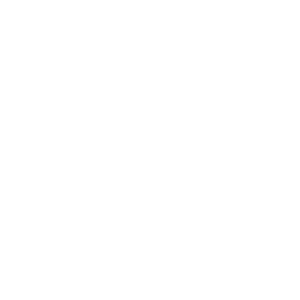
Home » Machine Learning in E-commerce : Top 11 Use Cases & Benefits
- Machine Learning
Machine Learning in E-commerce : Top 11 Use Cases & Benefits
- March 6, 2023
Introduction
Machine learning is a quickly developing field changing how e-commerce businesses operate. Businesses looking to improve their operations and give customers a better experience have many options thanks to incorporating machine learning algorithms into e-commerce platforms. Machine learning use cases in eCommerce are numerous and diverse, including product recommendations for fraud detection. In this blog, we will explore the top use cases of machine learning in e-commerce, and the key benefits businesses can expect from this technology.
This blog will give you useful insights into the power of machine learning in eCommerce , whether you’re an e-commerce company looking to enhance your operations or an e-commerce developer hoping to optimize your website. E-commerce businesses can improve customer experience, optimize operations, and acquire a competitive edge in the market by utilizing machine learning algorithms. Let’s explore machine learning in eCommerce and understand how companies may use this technology to their advantage.
The eCommerce industry is one of the most rapidly growing sectors of the economy, fueled by advances in technology and digital solutions. According to Statista , the number of users in the eCommerce market is projected to reach 284.6 million by 2025, with user penetration expected to be 83.6% by that time.
Additionally, eMarketer research reveals that global retail eCommerce sales will surpass $5 trillion in 2022, a fifth of overall retail sales, and reach $7 trillion by 2025. This growth can be attributed to integrating machine learning into eCommerce, making it a powerful tool for driving success in the sector.
Let’s explore how machine learning can perform in eCommerce and how it can improve business performance.
Track and analyze your sales data with our machine learning-powered analytics dashboard, and use insights to optimize your e-commerce strategy.
Artificial Intelligence(AI) Marketing Tools for 2023
Benefits of Machine Learning in E-Commerce
E-commerce businesses can reap numerous rewards from incorporating Machine Learning into their operations. Here are 11 key advantages of using Machine Learning in e-commerce:
1. Improved Customer Retention and Satisfaction
Nobody will contest the fact that maintaining relationships with current clients is simpler and less expensive than finding new ones. Loyal consumers are crucial for your business’s growth and development. The only thing that unites client acquisition and retention solutions are that both situations will benefit from improving the customer experience.
By using predictive analytics to understand customer preferences and behavior, machine learning can assist e-commerce organizations in increasing customer satisfaction and retention. Machine learning algorithms can identify trends in customer behavior and provide personalized suggestions for goods and services that cater to their individual needs by evaluating vast volumes of data. Higher client happiness, greater customer loyalty, and repeat business may arise.
Customer satisfaction and retention are becoming increasingly important as competition in the e-commerce market grows.
2. Increasing Sales and Marketing Success
You’ve likely already seen that several marketing strategies that were effective a few years ago have completely lost their effectiveness. If you don’t develop the ability to foresee trends and modify your plan, marketing budgets become true money pits.
Machine learning enables your company to increase client lifetime value and retention through more personalized marketing, predict customer churn, and dynamically change prices without sacrificing profit margins.
With the growth of big data, machine learning is becoming a more crucial tool for e-commerce businesses looking to improve marketing and boost sales.
3. Automating Back Office Task
Many routine tasks simply prevent your employees from focusing on the more difficult and analytical jobs. It is simple to delegate document management, customer service, and warehouse management to machine learning to cut operating expenses and losses from returns.
As companies look to streamline their operations and boost productivity, machine learning is becoming increasingly popular for automating back-office chores.
Get started today and launch your online store with our expert Ecommerce development services !
4. More Effective Fraud Detection and Data Protection
Why is it so important to catch fraud? Because this is a component that fosters trust! A client who lost faith in your service will never return. When building profiles, customers entrust you with their sensitive financial information. Therefore, preventing fraud is of utmost importance, and using machine learning to identify eCommerce fraud lets you foresee hostile behavior and protect your brand.
As the risk of fraud and data breaches increases, machine learning for fraud detection and protection is taking on more significance in the e-commerce sector.
5. Product Recommendations and Personalization Improvements
Machine learning can improve project recommendations and customize the shopping experience for customers of e-commerce enterprises. Machine learning algorithms can create customized product and service suggestions by evaluating vast volumes of customer data to find trends in client behavior. Increased consumer engagement, higher conversion rates, and greater customer satisfaction may result.
As consumers demand a more individualized buying experience, personalization and customization are becoming more and more significant in the market.
6. Pricing and Inventory Management Optimization
By analyzing large amounts of data to find patterns in consumer behavior and industry trends, machine learning may assist e-commerce enterprises in optimizing pricing and inventory management. Through better decision-making around pricing, inventory control, and product sourcing, firms may be able to operate more effectively and generate more revenue.
Businesses are increasingly utilizing machine learning to optimize their pricing and inventory management methods as the e-commerce market’s level of competitiveness rises.
Top 15 eCommerce Trends to Increase Sales and Conversions in 2023
Top 11 Use Cases of Machine Learning (ML) in E-Commerce
Here are the top 11 use cases of machine learning in e-commerce:
1. Machine Learning for Product Suggestions
- Customer behavior analysis.
- Purchase history analysis.
- Product similarity analysis.
- Dynamic pricing optimization.
2. Personalization for Customers
- Customer segmentation.
- Personalized product recommendations.
- Personalized marketing campaigns.
- Personalized email campaigns.
3. Use of Chatbots for Purchasing Assistance
- 24/7 customer support.
- Automated product recommendations.
- Resolving simple customer issues.
- Reducing customer service costs.
4. Detection of Fraudulent Activities
In today’s world of ever-increasing cybersecurity risks, You might believe that eCommerce fraud is a thing of the past in this day and age of cybersecurity awareness. Unfortunately, you’d be wrong. The size of the eCommerce fraud detection and prevention market is growing steadily, with recent reports estimating that it will reach over $69 billion by 2025.
Market size for e-commerce fraud prevention and detection worldwide from 2020 to 2025 (in U.S. dollars per billion)
Source: Statista
- Real-time fraud detection.
- Pattern analysis.
- Anomaly detection.
- Protection of customer information.
5. Automated Pricing
- Market trend analysis.
- Increased profits.
6. Automated Payment Processing
- Streamlined payment process.
- Reduced manual processing time.
- Improved accuracy.
- Increased customer satisfaction.
7. Forecasting of Sales
- Sales trend analysis.
- Seasonality analysis.
- Product demand forecasting.
- Inventory management optimization.
8. Prediction of Stock Performance
- Stock price prediction.
- Risk assessment.
- Improved investment decisions.
9. Retention of Customers
- Personalized customer retention strategies.
- Improved customer satisfaction.
- Increased customer loyalty
10. Search Engine Optimization
- Keyword analysis.
- Content optimization.
- Improved search rankings.
- Increased website traffic.
11. Image and Video Analysis
- Object recognition.
- Image and video classification.
- Improved product search.
- Improved product categorization.
Looking for a reliable Ecommerce development company ? Look no further – contact us today!
The Future of Machine Learning in E-commerce
The future of machine learning in e-commerce is promising and holds immense potential for businesses to improve their operations and provide better customer experiences. This section discusses the future of machine learning in e-commerce, including any emerging trends and how companies can prepare for them.
Here are some key trends for the Future of Machine Learning (ML) in E-commerce
1. Increased Personalization
Based on user behavior and preferences, machine learning algorithms will continue to be utilized to tailor the customer experience and deliver more focused recommendations.
2. Improved Customer Support
To make these chatbots even more advanced and intelligent, machine learning techniques will be deployed. Chatbots will still play a big part in providing round-the-clock customer care.
3. Streamlined Inventory Management
Inventory management will be improved using machine learning algorithms, which will result in less goods being retained and faster, more accurate deliveries.
4. Automated Decision-Making
Using machine learning algorithms to automate decision-making in areas like pricing, marketing, and product suggestions will increase, giving employees more time to focus on other tasks.
5. Enhanced Fraud Detection
Machine learning algorithms will detect fraud and safeguard client data more efficiently, lowering the risk of fraud for businesses and boosting customer confidence.
6. Predictive Customer Experience
Machine learning will play a critical role in predicting customer behavior and preferences in the future of e-commerce. This will allow businesses to create a more personalized shopping experience for customers and better predict their needs and wants.
7. Intelligent Supply Chain Management
Machine learning algorithms will be used to optimize supply chain operations and improve inventory management. This will result in reduced costs, improved delivery times, and enhanced customer satisfaction.
8. Virtual Personal Shopping Assistants
Machine learning will power virtual personal shopping assistants to help customers find desired products, place orders, and track deliveries. This will result in a more convenient and personalized shopping experience for customers.
9. Predictive Marketing
Machine learning will analyze customer behavior and make predictive marketing decisions. This will result in more effective marketing campaigns, improved customer engagement, and higher conversion rates.
Overall, new and creative uses for machine learning in e-commerce are constantly being developed, making the future of this technology promises. E-commerce companies can enhance their operations, offer better consumer experiences, and remain competitive as machine learning advances.
Supply Chain Predictive Analytics For Your Business
The eCommerce industry is directly impacted by machine learning technology, and this impact will continue to grow. Machine learning will continue to help the industry as technology advances, from customer experience to inventory management and efficiency.
So, whether you market B2C or B2B locally or worldwide, Syndell can assist you in developing a machine learning model tailored to your particular company’s requirements. It will raise client happiness and engagement levels, eventually enhancing sales for you.
AI and machine learning for eCommerce are the ideal pairings in which both sides gain from the other’s presence.
With various machine learning algorithms, artificial intelligence plays a part in eCommerce by improving the comfort and effectiveness of the buyer’s trip.
Machine learning applications directly impact the quality of the customer experience and the expansion of businesses in the eCommerce sector.
If you are looking to hire machine learning developers , you can rely on their expertise in providing top-notch machine learning development services or Considering implementing machine learning in your online store or ecommerce store. We will contact you if you fill out the contact form .
How can machine learning be applied to online shopping?
Machine learning may increase conversion rates by streamlining on-site search engines and making knowledgeable product recommendations on e-commerce websites. Using AI to generate “smarter” results is like following how the client behaves and thinks.
What kind of algorithm is used in eCommerce?
The Neural Collaborative Filtering Model is a popular method for applying the Matrix Factorization algorithm to neural networks (NCF). The NCF is a distinctive deep learning-based architecture for recommendation engines.
How to apply Machine Learning/AI in eCommerce?
Machine Learning can be applied in eCommerce by integrating algorithms into various processes such as product recommendations, predictive search, fraud detection, customer segmentation, inventory management, and chatbots/virtual assistants to automate and improve the customer shopping experience.
How much does integrating ML/AI in eCommerce cost?
The cost of integrating AI/ML in eCommerce can vary depending on several factors, such as the complexity of the project, the type of algorithms used, and the size of the data sets. On average, the cost of a small to medium-sized AI/ML project can range from a few thousand to several hundred thousand dollars.
What is eCommerce Machine Learning AI's role in the future of online shopping?
Machine learning AI is likely to play a significant role in shaping the future of online shopping by providing more personalized and efficient shopping experiences for customers. AI/ML can automate and optimize various eCommerce processes, such as product recommendations, predictive search, fraud detection, and customer segmentation. This can help eCommerce companies remain competitive and improve customer satisfaction, leading to increased sales and revenue.
Hiren Sanghvi
Power of business intelligence in revolutionizing modern supply chains, generative ai in ecommerce: how to boost revenue growth and sales in 10 ways, harnessing the power of business intelligence for your organization.
Start Your Dream Project with Our Expertise
Alternative Ways to Connect with Us
+1-858-275-3535 , +1-214-600-2592
[email protected]
hiren.k.sanghvi
Mobile app development
Advanced Technology Development
Full Stack Development
Web app development
Digital Marketing
Quick Links
Hire Developers
304 Shikhar Complex, Srimali Society, Navrangpura, Ahmedabad – 380009
600 North Broad Street, Suite 5 #3424, Middletown, DE – 19709
Bloc, 17 Marble St, Manchester, M2 3AW, United Kingdom
Application of Machine Learning in Customer Services and E-commerce
- Conference paper
- First Online: 29 May 2023
- Cite this conference paper
- G. Aarthi 13 ,
- R. Karthikha 14 ,
- Sharmila Sankar 13 ,
- S. Sharon Priya 13 ,
- D. Najumnissa Jamal 15 &
- W. Aisha Banu 13
Part of the book series: Lecture Notes in Networks and Systems ((LNNS,volume 662))
Included in the following conference series:
- International Conference on Data Management, Analytics & Innovation
410 Accesses
1 Citations
Nowadays, machine learning (ML) plays the important role in the E-commerce industry and its customer relations to perform different kinds of tasks such as prediction of purchases, segmentation of customers according to their reviews/sentiments, and recommendation of products to the active users. Various ML algorithms are implemented to get trained with data patterns to perform the above-mentioned tasks. In this paper, the customer segmentation and recommendation of women’s clothing based on the reviews are presented. The comparative study is done using five different ML algorithms, namely regression analysis, Naïve Bayes, decision trees, support vector machines, and clustering analysis. The results show that the Naïve Bayes algorithm has better performance when compared to other algorithms by showing better accuracy.
This is a preview of subscription content, log in via an institution to check access.
Access this chapter
- Available as PDF
- Read on any device
- Instant download
- Own it forever
- Available as EPUB and PDF
- Compact, lightweight edition
- Dispatched in 3 to 5 business days
- Free shipping worldwide - see info
Tax calculation will be finalised at checkout
Purchases are for personal use only
Institutional subscriptions
Archer N, Yuan Y (2000) Managing business‐to‐business relationships throughout the e‐commerce procurement life cycle. Internet Res
Google Scholar
Ryding D (2010) The impact of new technologies on customer satisfaction and business-to-business customer relationships: evidence from the soft drinks industry. J Retail Consum Serv 17(3):224–228
Article Google Scholar
Osterwalder A, Pigneur Y (2003) Modelling customer relationships in ebusiness illustrated through the mobile industry. BLED 2003 Proc 41
Morris MH, Pitt LF, Honeycutt ED (2001) Business-to-business marketing: a strategic approach. Sage
Helander N, Ulkuniemi P (2012) Customer perceived value in the software business. J High Technol Managem Res 23(1):26–35
Pearson S (2016) Building brands directly: creating business value from customer relationships. Springer
Ragins EJ, Greco AJ (2003) Customer relationship management and e-business: more than a software solution. Rev Bus 24(1):25
Xu D, Ruan C, Korpeoglu E, Kumar S, Achan K (2021) Theoretical understandings of product embedding for e-commerce machine learning. In: Proceedings of the 14th ACM international conference on web search and data mining, pp 256–264
Yang HW, Pan ZG, Wang XZ, Xu B (2004) A personalized product selection assistance based on e-commerce machine learning. In: Proceedings of 2004 international conference on machine learning and cybernetics (IEEE Cat. No. 04EX826). IEEE 4:2629–2633
Sabbeh SF (2018) Machine-learning techniques for customer retention: a comparative study. Int J Adv Comp Sci Appl 9(2)
Policarpo LM, da Silveira DE, da Rosa Righi R, Stoffel RA, da Costa CA, Barbosa JLV, Arcot T et al (2021) Machine learning through the lens of e-commerce initiatives: an up-to-date systematic literature review. Comp Sci Rev 41:100414
Wong AN, Marikannan BP (2020) Optimising e-commerce customer satisfaction with machine learning. J Phys Conf Ser 1712(1):012044. IOP Publishing
Pang H, Zhang W (2021) Decision support model of e-commerce strategic planning enhanced by machine learning. Inform Syst e-Business Manage 1–17
Capalbo V, Ghiani G, Manni E (2021) The role of optimization and machine learning in e-commerce logistics in 2030. Int J Econom Manage Eng 15(3):290–294
Shen B (2021) E-commerce customer segmentation via unsupervised machine learning. In: The 2nd international conference on computing and data science, pp 1–7
Dayton CM (1992) Logistic regression analysis. Stat 474:574
Liu CJ, Huang TS, Ho PT, Huang JC, Hsieh CT (2020) Machine learning-based e-commerce platform repurchase customer prediction model. PLoS ONE 15(12):e0243105
Monil P, Darshan P, Jecky R, Vimarsh C, Bhatt BR (2020) Customer segmentation using machine learning. Int J Res Appl Sci Eng Technol (IJRASET) 8(6):2104–2108
Singh A, Thakur N, Sharma A (2016) A review of supervised machine learning algorithms. In: 2016 3rd international conference on computing for sustainable global development (INDIACom). IEEE, pp 1310–1315
Mahesh B (2020) Machine learning algorithms—a review. Int J Sci Res (IJSR) 9:381–386 [Internet]
Ekelik H, Şenol EMİR, A comparison of machine learning classifiers for evaluation of remarketing audiences in e-commerce. Eskişehir Osmangazi Üniversitesi İktisadi ve İdari Bilimler Dergisi 16(2):341–359
Ray S (2019) A quick review of machine learning algorithms. In: 2019 International conference on machine learning, big data, cloud and parallel computing (COMITCon). IEEE, pp 35–39
Khan A, Baharudin B, Lee LH, Khan K (2010) A review of machine learning algorithms for text-documents classification. J Adv Inform Technol 1(1):4–20
Choudhary R, Gianey HK (2017) A comprehensive review on supervised machine learning algorithms. In: 2017 International conference on machine learning and data science (MLDS). IEEE, pp 37–43
Sarker IH (2021) Machine learning: algorithms, real-world applications and research directions. SN Comp Sci 2(3):1–21
MathSciNet Google Scholar
Dhall D, Kaur R, Juneja M (2020) Machine learning: a review of the algorithms and their applications. Proc ICRIC 2019:47–63
Praveena M, Jaiganesh V (2017) A literature review on supervised machine learning algorithms and boosting process. Int J Comp Appl 169(8):32–35
Kotsiantis SB, Zaharakis I, Pintelas P (2007) Supervised machine learning: a review of classification techniques. Emerg Artif Intell Appl Comp Eng 160(1):3–24
Santana CJ, Aguiar P, Bastos-Filho CJ (2018) Customer segmentation in a travel agency dataset using clustering algorithms. In: 2018 IEEE Latin American conference on computational intelligence (LA-CCI). IEEE, pp 1–6
Duong GHT, Nguyen-Thi TA (2021) A review: preprocessing techniques and data augmentation for sentiment analysis. Comput Soc Netw 8(1):1–16
Alexandropoulos SAN, Kotsiantis SB, Vrahatis MN (2019) Data preprocessing in predictive data mining. Knowl Eng Rev 34
Dwivedi SK, Rawat B (2015) A review paper on data preprocessing: a critical phase in web usage mining process. In: 2015 International conference on green computing and internet of things (ICGCIoT). IEEE, pp 506–510
Huang J, Li YF, Xie M (2015) An empirical analysis of data preprocessing for machine learning-based software cost estimation. Inf Softw Technol 67:108–127
Haixiang G, Yijing L, Shang J, Mingyun G, Yuanyue H, Bing G (2017) Learning from class-imbalanced data: Review of methods and applications. Expert Syst Appl 73:220–239
Ali H, Salleh MNM, Hussain K, Ahmad A, Ullah A, Muhammad A, Khan M et al (2019) A review on data preprocessing methods for class imbalance problem. Int J Eng Technol 8:390–397
Famili A, Shen WM, Weber R, Simoudis E (1997) Data preprocessing and intelligent data analysis. Intell Data Anal 1(1):3–23
Download references
Author information
Authors and affiliations.
Computer Science and Engineering, B. S. Abdur Rahman Crescent Institute of Science and Technology, Chennai, India
G. Aarthi, Sharmila Sankar, S. Sharon Priya & W. Aisha Banu
Electrical and Electronics Engineering, B. S. Abdur Rahman Crescent Institute of Science and Technology, Chennai, India
R. Karthikha
Electronics and Instrumentation Engineering, B. S. Abdur Rahman Crescent Institute of Science and Technology, Chennai, India
D. Najumnissa Jamal
You can also search for this author in PubMed Google Scholar
Corresponding author
Correspondence to G. Aarthi .
Editor information
Editors and affiliations.
Tata Consultancy Services Ltd., Pune, India
Neha Sharma
Society for Data Science, Pune, India
Faculty of Engineering, A. K. Choudhury School of Information Technology, Kolkata, West Bengal, India
Amlan Chakrabarti
Faculty of Computer Science, Technion—Israel Institute of Technology, Haifa, Israel
Alfred M. Bruckstein
Rights and permissions
Reprints and permissions
Copyright information
© 2023 The Author(s), under exclusive license to Springer Nature Singapore Pte Ltd.
About this paper
Cite this paper.
Aarthi, G., Karthikha, R., Sankar, S., Priya, S.S., Jamal, D.N., Banu, W.A. (2023). Application of Machine Learning in Customer Services and E-commerce. In: Sharma, N., Goje, A., Chakrabarti, A., Bruckstein, A.M. (eds) Data Management, Analytics and Innovation. ICDMAI 2023. Lecture Notes in Networks and Systems, vol 662. Springer, Singapore. https://doi.org/10.1007/978-981-99-1414-2_56
Download citation
DOI : https://doi.org/10.1007/978-981-99-1414-2_56
Published : 29 May 2023
Publisher Name : Springer, Singapore
Print ISBN : 978-981-99-1413-5
Online ISBN : 978-981-99-1414-2
eBook Packages : Intelligent Technologies and Robotics Intelligent Technologies and Robotics (R0)
Share this paper
Anyone you share the following link with will be able to read this content:
Sorry, a shareable link is not currently available for this article.
Provided by the Springer Nature SharedIt content-sharing initiative
- Publish with us
Policies and ethics
- Find a journal
- Track your research
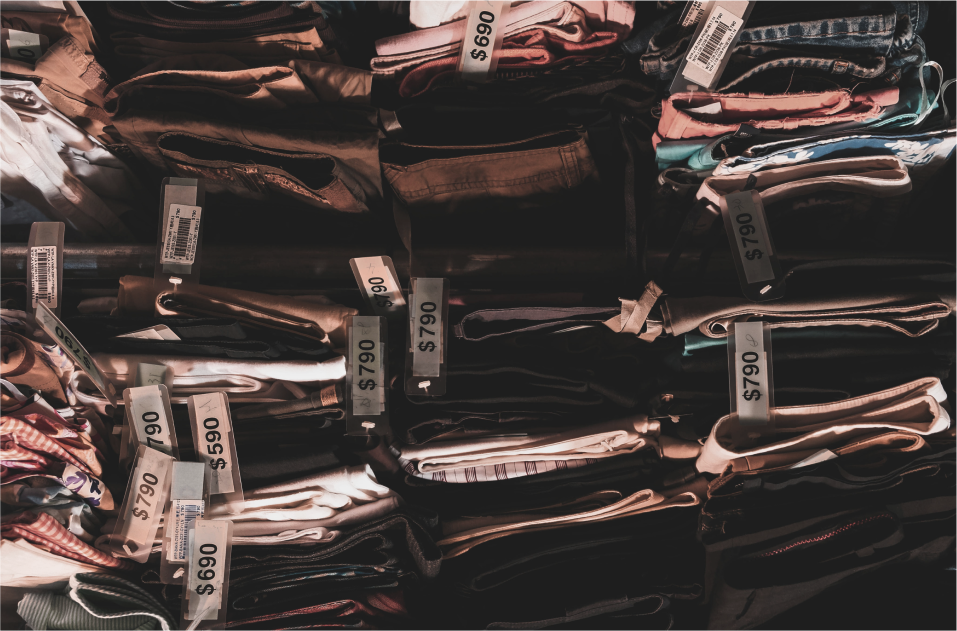
Mon, Jun 1, 2020
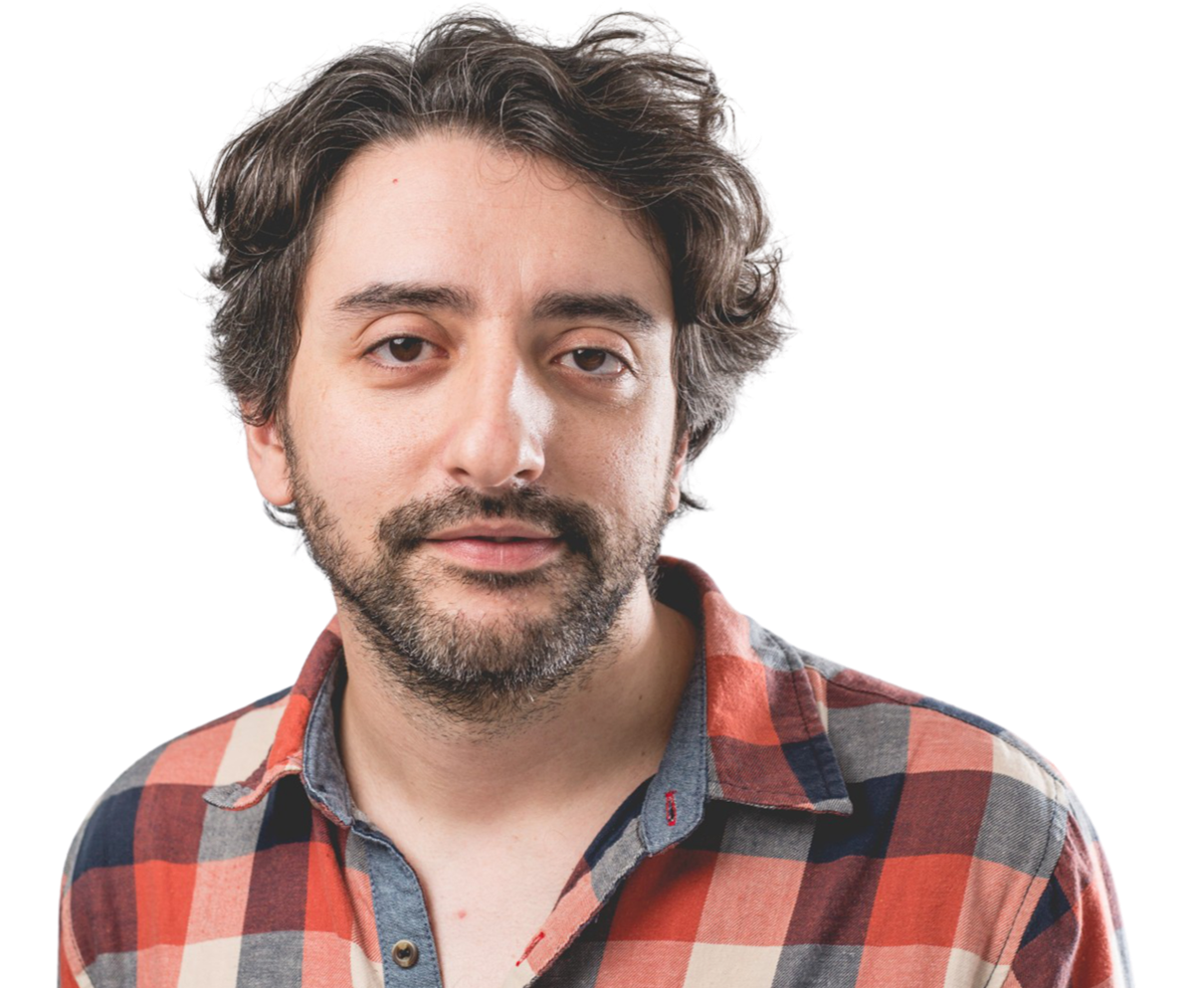
We have previously discussed how a data-driven price optimization is no longer an option for retailers, but a question of how and when to do it . With a world that's moving towards changing prices more and more dynamically, static pricing strategies can't keep up, and data-driven approaches have arrived to stay. In this post, we'll be focusing on how to perform data-driven price optimization , using a case study to showcase what can be done using sales data to predict demand and optimize prices.
Having read our previous post , you may now ask yourself:
- Is price optimization with Machine Learning worth the investment in my case?
- How do I know if the quality of my sales data is good enough?
- Or putting it simply: price optimization, here I go! But am I ready?
To address these and other questions, we will demonstrate a practical example using the tools we've developed in the past years while excelling in our client's pricing strategies. To do so, we will take the publicly available dataset from the Brazilian marketplace Olist as our use case foundation.
This will be a glimpse of what you can achieve by sharing your sales data with us. We could perform an opportunity summary report in line with this use case, and uncover your own opportunities regarding price optimization. So, fasten your seatbelt and get ready for the take-off.
Analyzing an E-Commerce's Sales Data
While diving into the practical example and explain how we solve these kinds of problems, let's refresh some price optimization concepts and introduce the work pipeline we will follow. There are different ways to tackle a price optimization problem and different strategies to perform a Machine Learning approach for this task.
Our price optimization pipeline will be based on six phases or steps:
- Data Collection and Integration : build a data asset that integrates all the relevant information for demand forecasting.
- Data analysis : generate explanatory data analysis reports and categorize items according to demand patterns.
- Anomaly detection report : generate an anomaly detection report to identify inconsistent fields, detect out-of-stock issues, observe sales distribution, and detect outliers.
- Demand forecasting : estimate the demand curves for each one of the items, i.e., understand the elasticity of the demand.
- Experimentation : elaborate the strategy on how to perform exploration and exploitation.
- Optimization : optimize the price for the items, subject to a list of specific business constraints.
In the remainder of the post we will describe each one of these steps using the Olist dataset as an explanatory example.
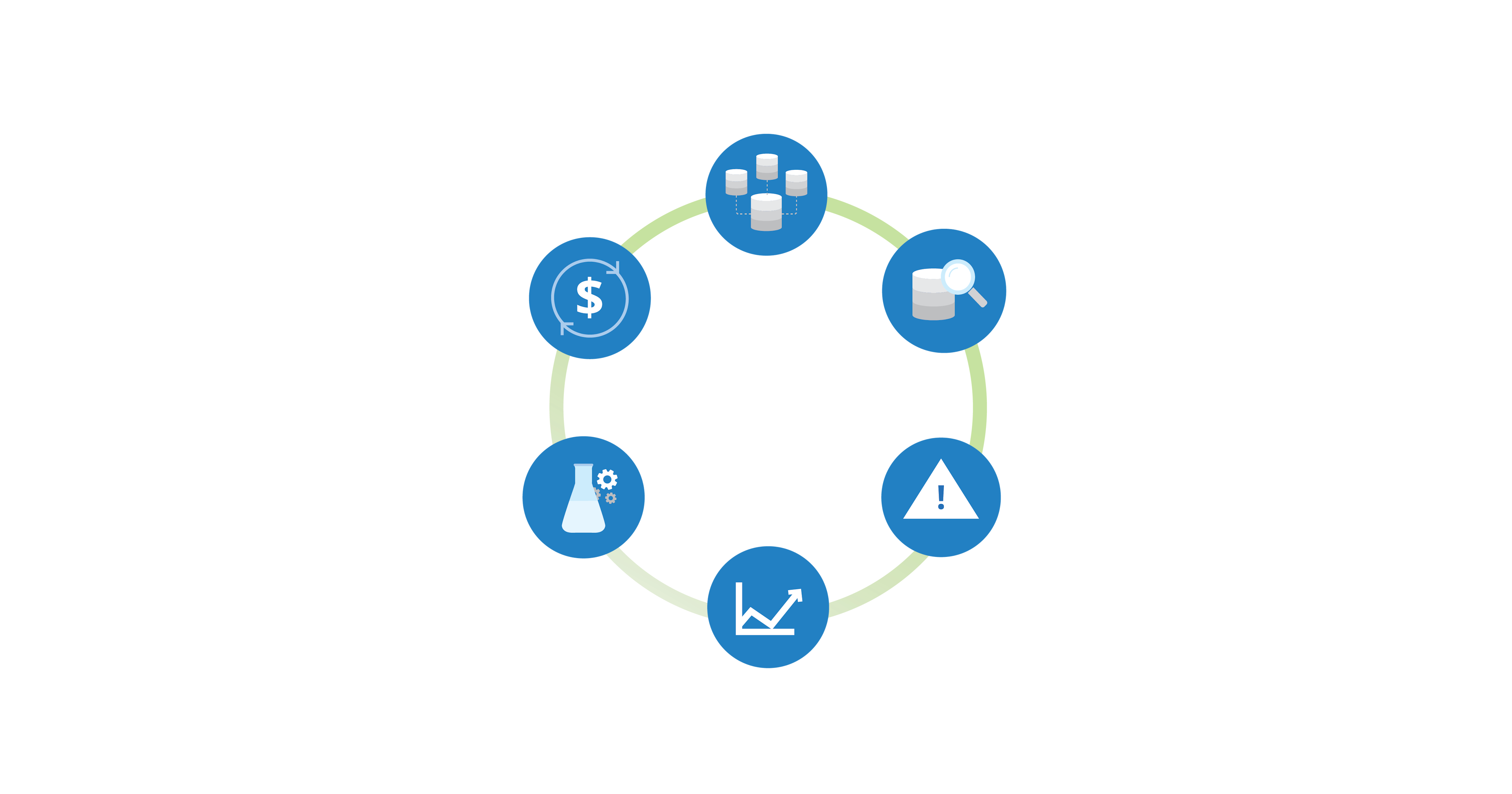
1. Data Collection and Integration
The first step is to build our data asset, taking different sources of information that will be used to train our demand forecasting models.
The cornerstone to begin working on any price optimization project is the sales dataset , i.e., to have the sales information of your business. Whether you are an e-commerce or a brick-and-mortar store, sales information is the first asset we have to focus on, and where our analysis will begin.
What do we mean by the sales dataset? To put it simply, we need access to the sales information of the business segment that the company is willing to optimize the pricing strategy for. If you already know beforehand which segment this is, great! You are one step ahead. But if you don't, no worries, we can help you decide which is the best segment to start based on your sales information, as we will see in our example.
Usually, companies choose to store their sales information in one of the following structures:
- By sales transactions: each transaction detail with its item identifier, the timestamp, the number of items sold, and its sell price. Other data fields that can be useful to complement this information are the payment method (cash, credit card, etc.) and the shipping information.
- By daily aggregation: some companies store their sales information this way because they need to have an accurate cash flow.
Complementing your sales data
Naturally, sales data won't be the only source of information we will consider when addressing the price optimization task. As demand is affected by several factors (such as the price, competitor's price, season, holidays, and macroeconomic variables), this is only one of the many factors to consider.
At this point, the knowledge of a business expert will play a big part to jointly work on a data structure that works for each specific problem.
Once again, don't worry if you are not storing all this data currently. We can quickly provide you with several of these public datasets.
Here we list other sources of information that are usually considered when collecting data:
- Items information: data such as category, sub-category, description, number of photos, photos, and general characteristics can be used to profile each item and relate it with others.
- Macroeconomics: different indicators such as GDP, unemployment rate, consumer confidence, and currency exchange. It is crucial to keep in mind that these indicators are all gathered during different periods. Some of them are calculated quarterly, some others monthly, and some others daily.
- Stores or seller information: location, size, etc.
- Holidays and events: certain holidays and events can have a direct impact on the number of sales of different items, e.g., Valentine's Day, Christmas, Mother's Day, etc.
- Item's reviews: reviews performed by customers can also be included in our forecasting model.
- Competitor(s)' price: the presence of one or several strong competitors can have a direct impact on the demand of our items, so having information about how they are pricing can potentially be used as insights for our models.
- Customer traffic: number of visitors (e-commerce and brick-and-mortar), average time on page, and number of clicks (e-commerce only).
- Other business-specific information .
2. Data Analysis
Now that the basics are clear let's dive into the Olist e-commerce data. We always start working on an exploratory data analysis of the sales data to gain insights about the different available items, their trends in the sales, and the pricing history as well.
For the Olist example , the sales data is stored as transactions, divided into three different datasets:
- order-items : which includes the items and sell price for each order.
- orders : contains all the information related to the orders, such as customer, seller, order status, order timestamp, and the estimated delivery date.
- order-payments : it includes the payment information for each order.
After processing these three datasets, we can build a standard sales dataset, as shown in the following figure, which aggregates item-seller sales per day:
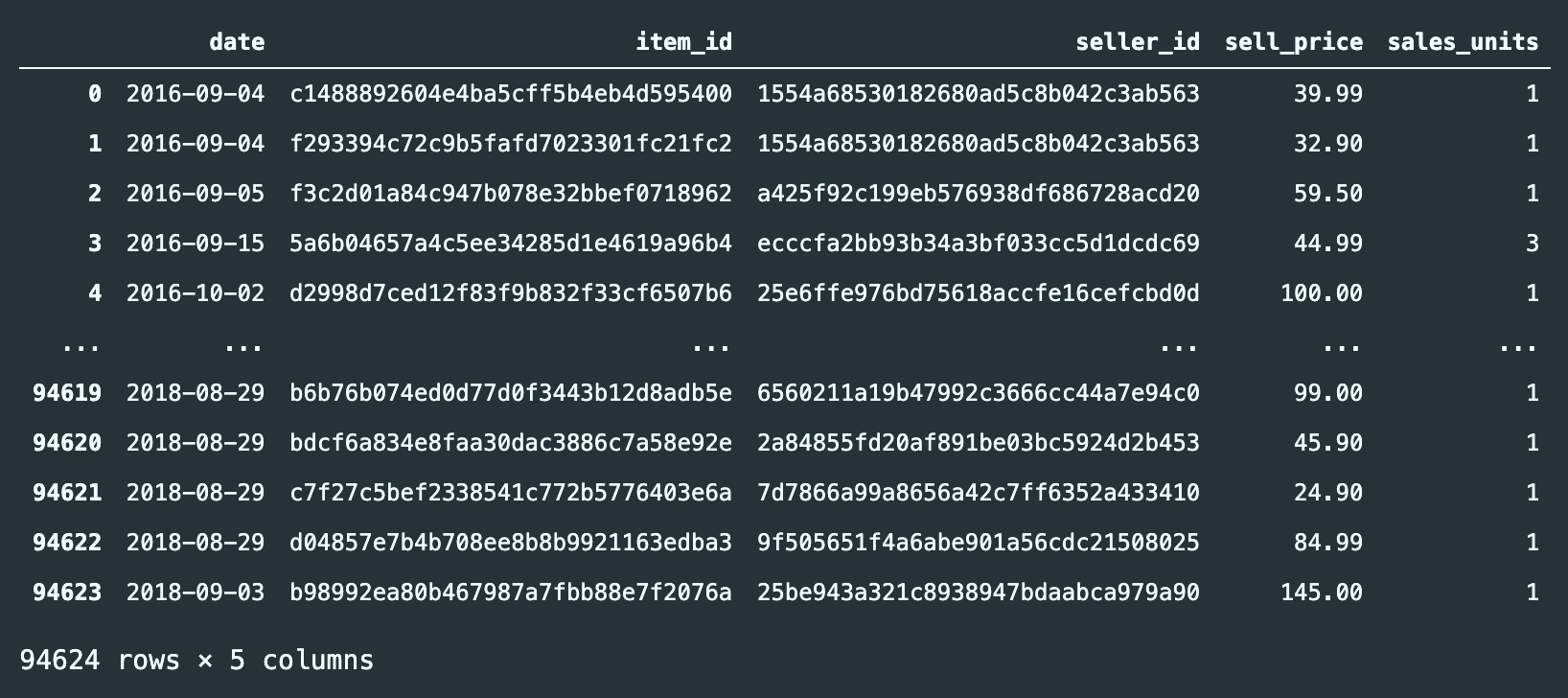
Once we define the sales dataset, we should question ourselves how many items should we choose to begin the optimization? Or even further: are all items ready to be optimized?
To begin with, we will set a threshold on the number of historical sales observations. To estimate the future demand for a given item, we need historical sales data. Therefore, we will start considering those articles that have , at minimum, ten weeks with at least 1 unit sold.
Using this threshold, in our Olist use case, we will have a universe of 1,197 items. On the other hand, we could think of a price automation solution for those unique items that are sold only once or very few times.
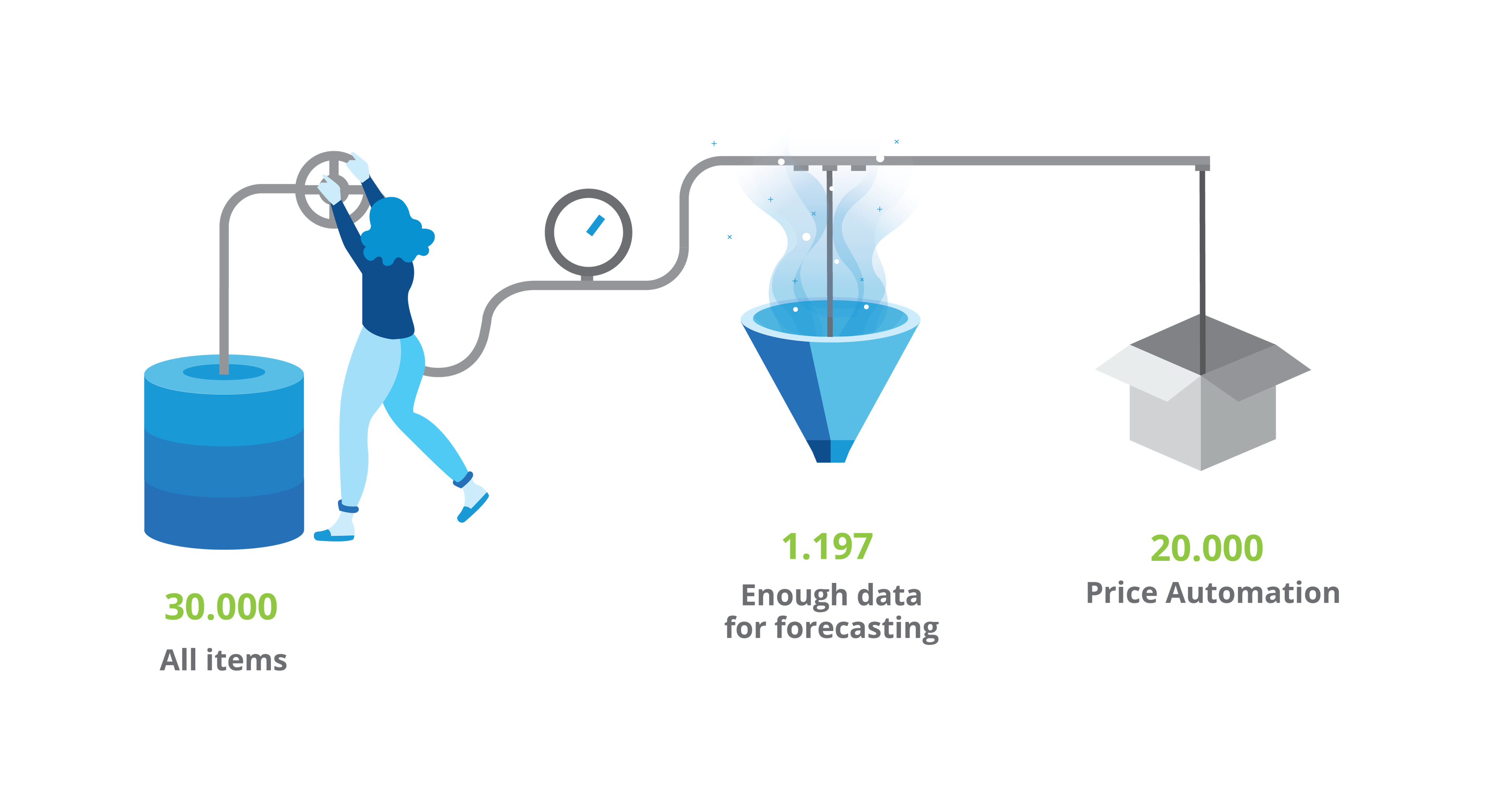
Moreover, this subset of items can be further categorized. In particular, we will classify items based on demand history and the number of different prices that have historically been used. The shape of the demand history will have a direct impact on the modeling efforts, and so will the number of price changes on the experimentation strategy. Therefore, we will divide our approach into two different stages to select the first set of items:
- Study the variability of the demand history concerning timing and magnitude.
- Study the number of price changes and timing.
Variability of the demand history
At this first stage we will study the variability of the demand history, as we are looking to identify those items that have a simpler selling pattern than others. This approach will not only impact the final accuracy of the sales forecasting but also the feature engineering and modeling efforts.
To perform this categorization, we will use the one proposed by Syntetos et al. (2005) , in which the demands are divided into four different categories:
- Intermittent , for items with infrequent demand occurrences but regular in magnitude
- Lumpy , for items whose demand is intermittent and highly variable in magnitude
- Smooth , for items whose demand is both frequent and regular in magnitude
- Erratic , for items whose demand is frequent but highly variable in magnitude
In the following figure, we show how each of these demands would look like in a typical scenario, plotting the demand magnitude to the time.
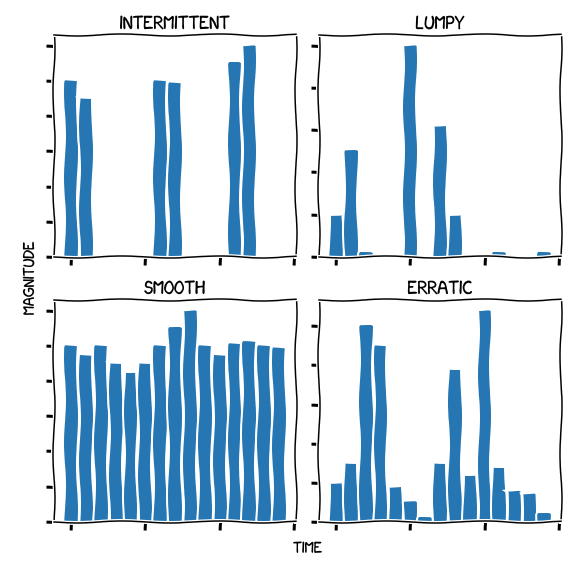
Smooth and intermittent demands are easier to model than lumpy and erratic . In particular, lumpy items are hard to model, because its demand shape is both erratic and irregular.
Instead of looking at the shape of each item's demand, we can calculate two magnitudes that will help us to classify all items at once:
- How spaced the sales are over time. Calculated as the mean inter-demand interval (p) .
- A measure of how bumpy the sales are. Calculated as the squared coefficient of variation of demand sizes ( C V 2 CV^2 C V 2 ) .
Although we'll not dive into the details, we can use both values to perform a scatter plot of the items and cluster them into the four mentioned categories. This classification will also help us to define the level of aggregation of our data. In the following figures, we compare how the items are classified when aggregating the sales data daily and weekly.
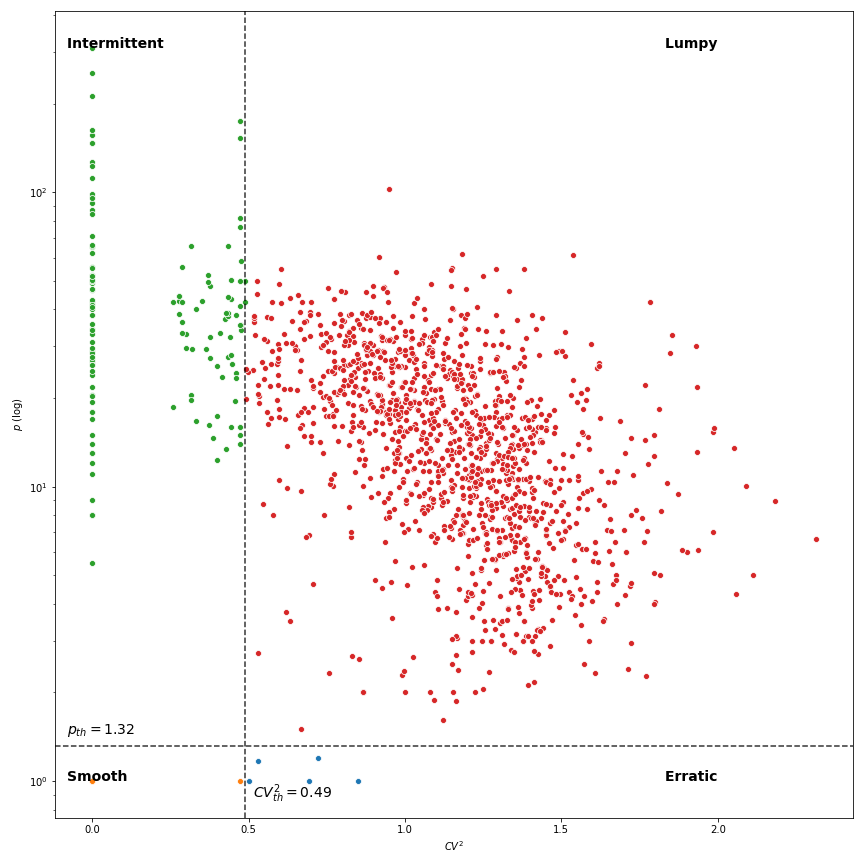
As you can see, a weekly aggregation of the data provides us a higher number of items to consider given our classification. This is also shown in the table below.
Items classification according to demand variability
We will aggregate our data by week and we will select those items that fall in the smooth , erratic, and intermittent categories (629 items), leaving out of the scope those with a lumpy demand (568 items) for which we should think of another level of aggregation.
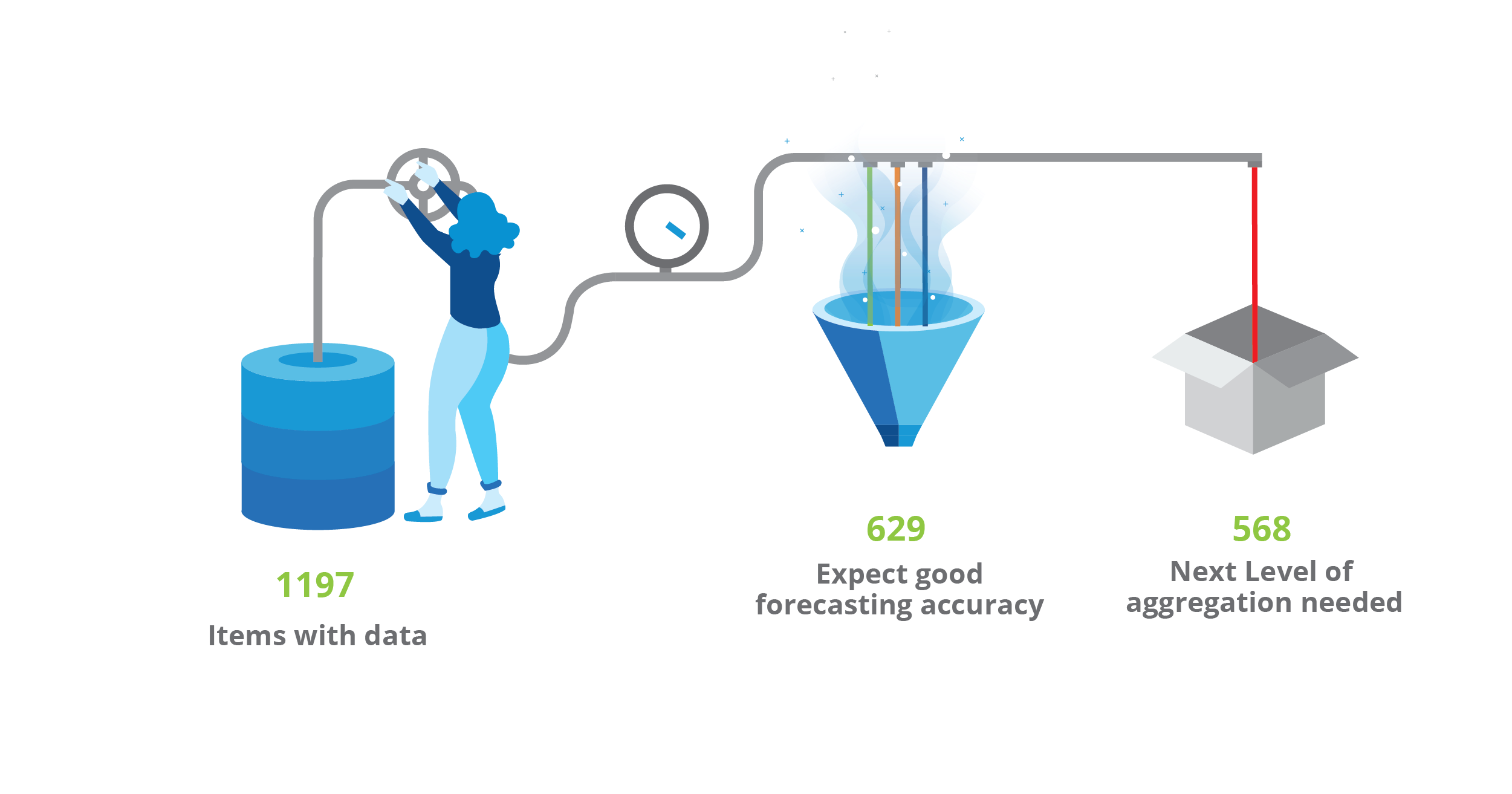
Number of price changes and timing
Another important aspect to consider for each one of the items is how many prices have been historically tried and during which period of time.
The more information we have about the demand changes relative to the price, the less the exploration efforts needed, as we will see later on.
These are the filters that we are considering for the Olist dataset:
- Have historically changed their price at least three times in the 2-year span
- Each of these prices has at least four days of sales.
With these constraints, we will be able to filter those items that have a significant amount of information. This allows us to estimate the demand curves within our historical sales, i.e., we want to select those items in which we have information about the sales behavior at different points in time, using different prices.
If we only filter by the number of price changes and timing specified in 1. and 2. the number of valid items is reduced to 144.
Items selection
We are now ready to join both conditions together —pattern shape of historical demand & the number of price changes and timing— to reach the final number of items that, without doing any other further experimentation, are ready to optimize. Taking both conditions into consideration, we reach a universe of 51 items that are ready to start .
As mentioned before, the rest of the items won't be discarded, but further price exploration will have to take place to generate meaningful datapoints. The price exploration strategy will be explained in the "Experimentation setup" section.
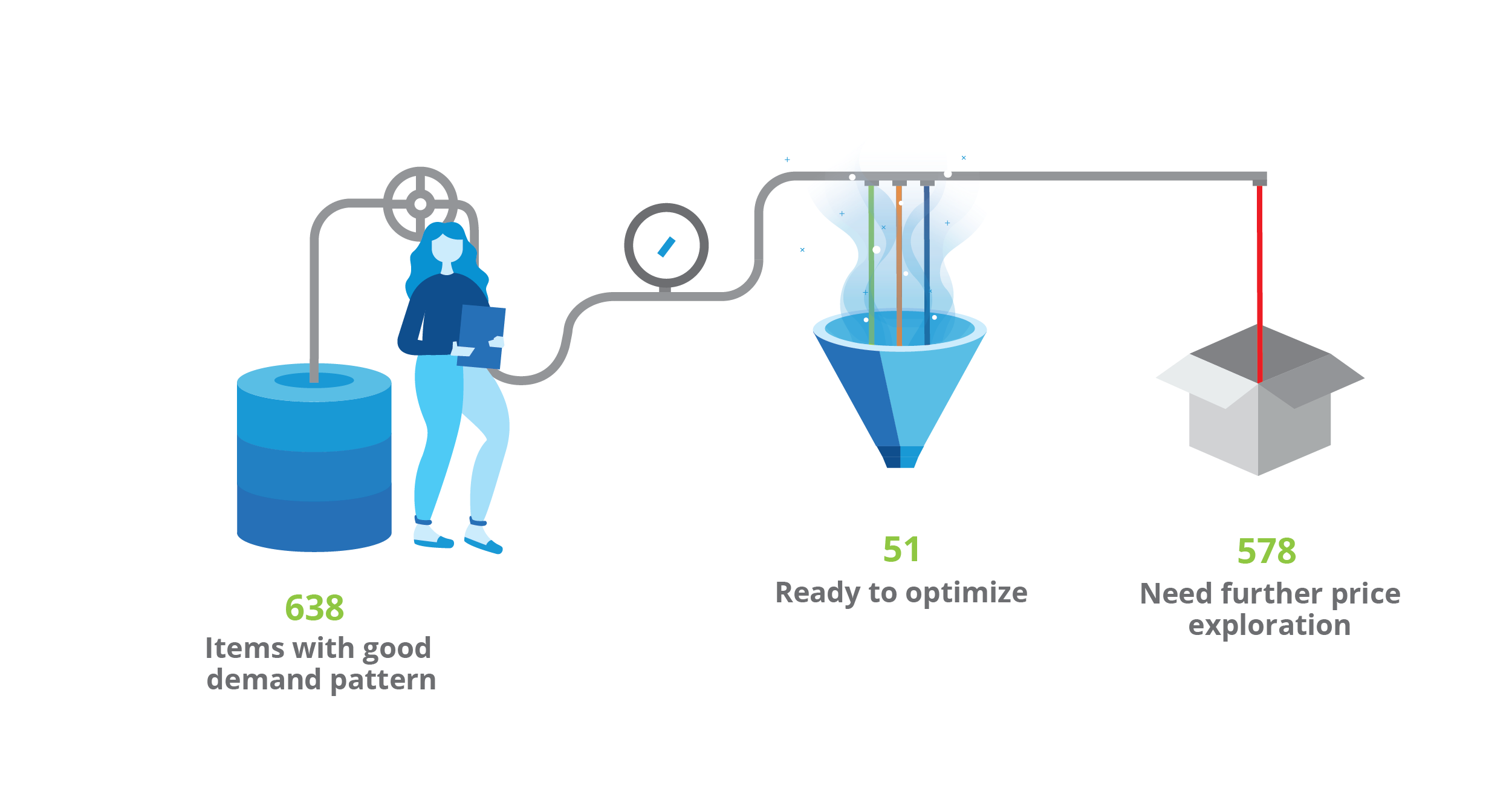
3. Anomaly detection
From our experience working with multiple datasets and several retailers, we have been able to develop an anomaly detection report. This report provides useful information to the data science team working with the dataset and provides meaningful insights to the business team. It has been of great help to identify missing information or misconceptions of the available data.
Anomaly detection involves identifying the differences, deviations, and exceptions from the norm in the data.
In a typical retail scenario, our anomaly detection report consists of three main topics:
- Identifying missing and inconsistent fields
- Detecting and handling out-of-stocks
- Observing sales distribution and detecting outliers
We will dig into the details of each one of these topics in the following sections.
1. Identify missing and inconsistent fields
1.1 missing fields.
Though there is a lot of meaningful information in the sales information of Olist , there are missing features that are essential to tackle our price optimization problem. For example, we don't have information about the manufacturer's suggested retail price (MSRP) of the items nor about its costs.
Let's say that we want to optimize our prices to maximize profit . Let's take a look then to the definition of the profit function:
p r o f i t = ∑ i N Q i ( p i ) × ( p i − c i ) \mathrm{profit} = \sum_i^N Q_i(p_i) \times (p_i-c_i) profit = ∑ i N Q i ( p i ) × ( p i − c i )
Q represents the demand, p the price, and c the cost, and the sum is performed over our universe of items ( N ). As you can see, the cost is needed to calculate the profit, and so is to perform the optimization. If we don't have this information, we can assume a zero cost for the items, and then optimize our prices to maximize revenue instead of profit:
r e v e n u e = ∑ i N Q i ( p i ) × p i \mathrm{revenue} = \sum_i^N Q_i(p_i) \times p_i revenue = ∑ i N Q i ( p i ) × p i
On the other hand, the MSRP can be used to compare the selling prices against the manufacturer's suggestion. It can be useful when analyzing each item's historical pricing strategy and its effects on the demand.
Also, we don't have much information about the items themselves. We lack information such as the item's description, images, name, sub-category, or brand, which are of great help to relate and cluster similar items. Nevertheless, as we will see, we can still get meaningful results.
1.2 Inconsistent fields
We can also run a series of inconsistency checks between the features. For example, if we have the sales data aggregated by date and not by order or basket, we can check if the " Total Sales " for a particular item/date is equal to the number of units sold times the price .
We would all expect this to be true, but what if:
- there have been refunds on that given date
- some units were sold with a privileged discount
- some customer paid with a different currency
We need to make sure that all of these details are correctly recorded, and if not, try to detect these anomalies automatically to take specific actions.
Moreover, many times retailers have business-specific rules which can be checked in a data-driven way to make sure they are being applied or not. Does any of the following business rules sound familiar to you?
- Prices for the same item must be consistent across stores
- No more than 40% discount from MSRP is allowed
- Sell price must always be lower than the competitor's price
- Different price promotions cannot overlap
With our anomaly detection report, you can specify the business rules, and we can check these quickly, easily, and automatically.
Going back to the Olist sample dataset, we don't have information about the MSRP, the cost of each item, or any specific business rule. However, we could still check for certain general inconsistent fields such as the following:
- Do we have negative values on sales units or prices?

- Is the same item sold at different prices on the same date?
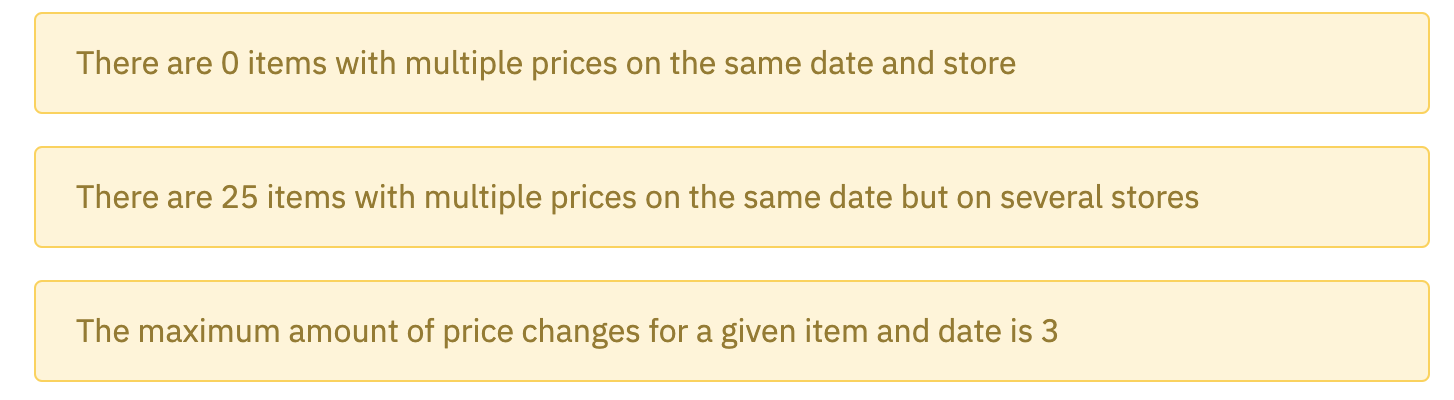
2. Detecting and handling out-of-stocks
Another chapter in our anomaly detection report for retailers consists of detecting out-of-stock issues. This is very important because of two main reasons:
- Revenue loss: having out-of-stock issues implies losing sales and sometimes even clients. Therefore, pricing and inventory management decisions have to be taken jointly.
- Demand curve estimation: to correctly estimate a demand curve for each item/store, we need to identify the causes for which we observe zero sales for certain items in certain moments. In particular, we need to distinguish between zero demand and zero supply.
Ideally, we could incorporate inventory data and flag days as out-of-stock if the stock level for a specific item is equal to zero. Nevertheless, from our experience working with retailers, we have learned that inventory data is not necessarily 100% accurate . For example, in a brick-and-mortar store, we may observe zero sales for consecutive days, even when the inventory data suggests there should be five or even ten items in-store. Still, these items are not properly displayed to the public.
Therefore, we have designed different heuristics according to the client's data to correctly identify out-of-stock days and better estimate the demand curves. Some of these heuristics are complex and client-specific, while others are simple and straightforward.
As a preliminary approach, we can identify per item/store long gaps of zero sales and assume these are out-of-stock problems. This definition of out-of-stock will depend on the consecutive zeros threshold we define. For example, we can determine the consecutive zeros threshold as 30 days; this is to say that an item will be considered as out-of-stock if there are no sales in 30 consecutive days. We can visualize the out-of-stock periods as the shadowed area on the following plot:
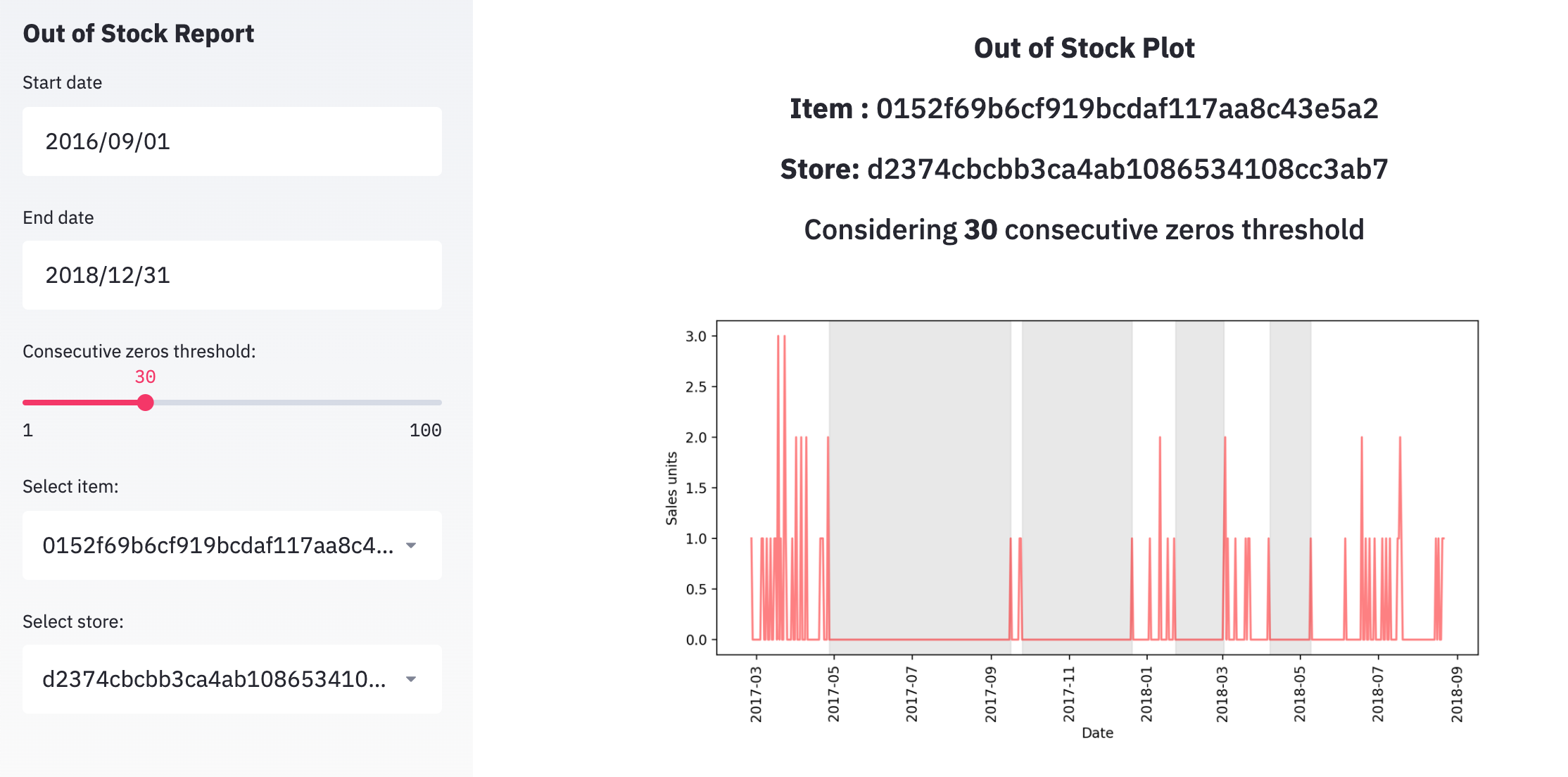
Given this definition, we can estimate the revenue lost as a consequence of out-of-stock problems, e.g., assuming that the sales during the out-of-stock periods would have been equal to an average date.
Furthermore, we could also download a report with the estimated revenue lost for each item/seller on each out-of-stock period. The Out-of-Stock Report would look something like this:
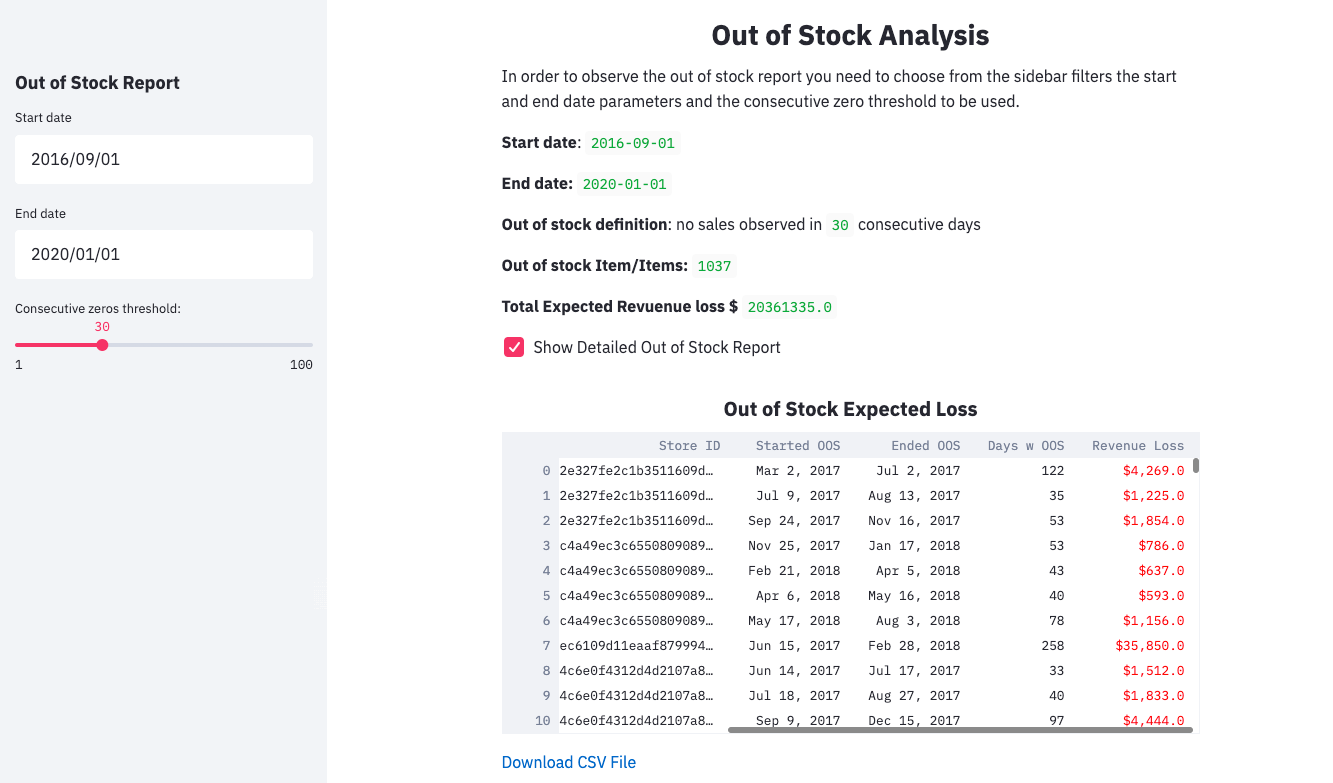
Even though this report gives us some insights into the impact that out-of-stocks is having on our revenue, these are just preliminary estimations. To better approximate the revenue loss and to identify the out-of-stock days better, we can use the same Machine Learning models we use to perform the forecast of the demands.
Let's picture this with an example. Imagine our Machine Learning model estimates that 53 units would've been sold of a specific item, on a particular date, given a certain price and conditions, and we observe that only 1 unit has been sold. This would raise an alert since it could be that a specific item has potentially run out-of-stock.
Finally, but not least important, once the Machine Learning models are trained, they can also be used in a proactive way instead of reactive. Several weeks in advance, we could estimate how many units will be sold and generate stock replenishment recommendations to avoid out-of-stock issues.
3. Observe sales distribution and detect outliers
To detect outliers, we can plot the daily sales distribution per seller or store by day of the week, month, or year. In particular, we can observe the distribution and detect outliers by generating different visualizations of the data .
For example, we can generate a boxplot to observe the distribution of units sold by day of the week. These plots are useful to understand how many units are sold on average, by a seller or store, per item on each category group.
As shown in the following figure, we can observe from the boxes sizes that, on average, all days have a similar behavior where most sellers per day sell only one unit per item. Nevertheless, there seem to be several outliers (dots that are far from the normal distribution). If we hover over the plot, for example, on the point which is farther away on the Friday category, we can see that it corresponds to a particular seller who sold 25 units for a specific item on Friday, 24th November 2017.
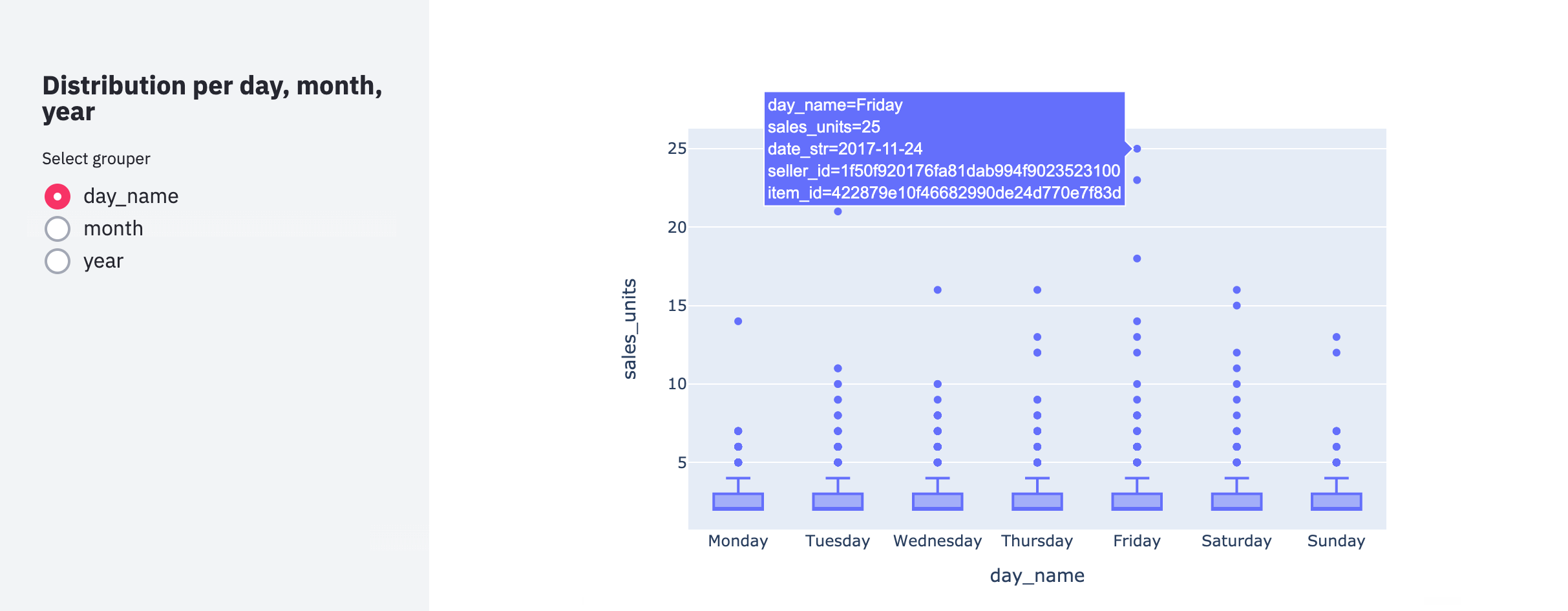
Does that date ring a bell to you? Why could you sell a totally different amount of units than what you usually sell? BINGO! That was Black Friday!
Even though we were considering national holidays as features for our demand estimation, from the data, we have learned that it may be relevant to include Black Friday as a special date.
However, we may also wonder what happened on Black Friday in other years? Was it also the date with the highest sales in 2018? Or what about Black Friday for other sellers? Did their sales increase dramatically too? By having a look at the boxplot by year, we can check what happened in 2018:
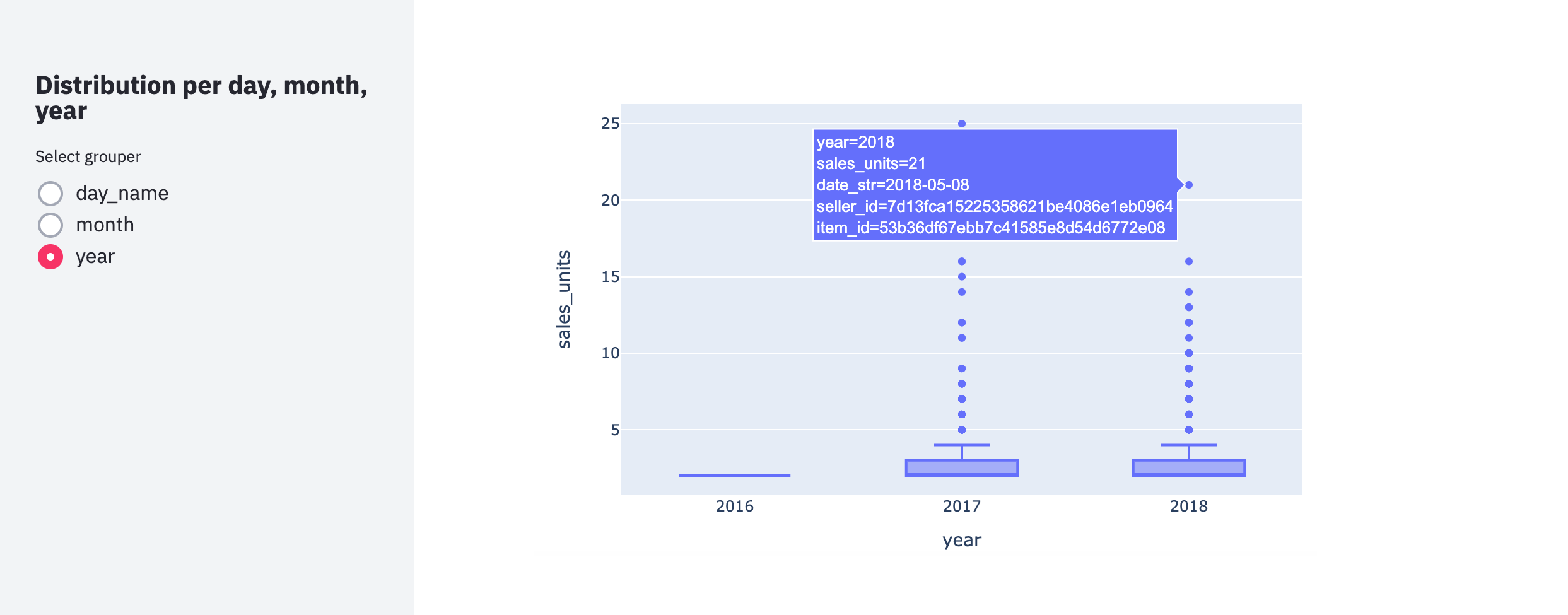
For 2016, we have almost no observations (data started in September 2016), but if we compare 2017 and 2018, the distribution seems very similar. Nevertheless, the seller who sold the maximum amount of units per item in 2018 was a different seller and did so on a different date. In particular, it was on May 8, 2018. What could have happened on that specific date?
When looking in detail at the list of holidays and events for Brazil in 2018, we can see that May 8 corresponds to the week before Sunday of Mother's Day (May 13). Also, this makes total sense when we see that the category of the item is marked as "watches gifts."
Taking consideration of holidays is important to detect and classify the outliers into different groups, i.e., those potentially related to errors in the data ingestion process —and that need to be treated as misleading— and those strictly associated with special events.
For sure, we need to continue exploring the data in order to gain more insights. We could, for example, observe the location for that particular outlier seller and check the sales distribution of the nearest stores to see whether it was one specific event that affected several stores or just this one. Or we could check if a sudden decrease in price can explain the increase in sales by plotting both variables together, as shown in the following plot.
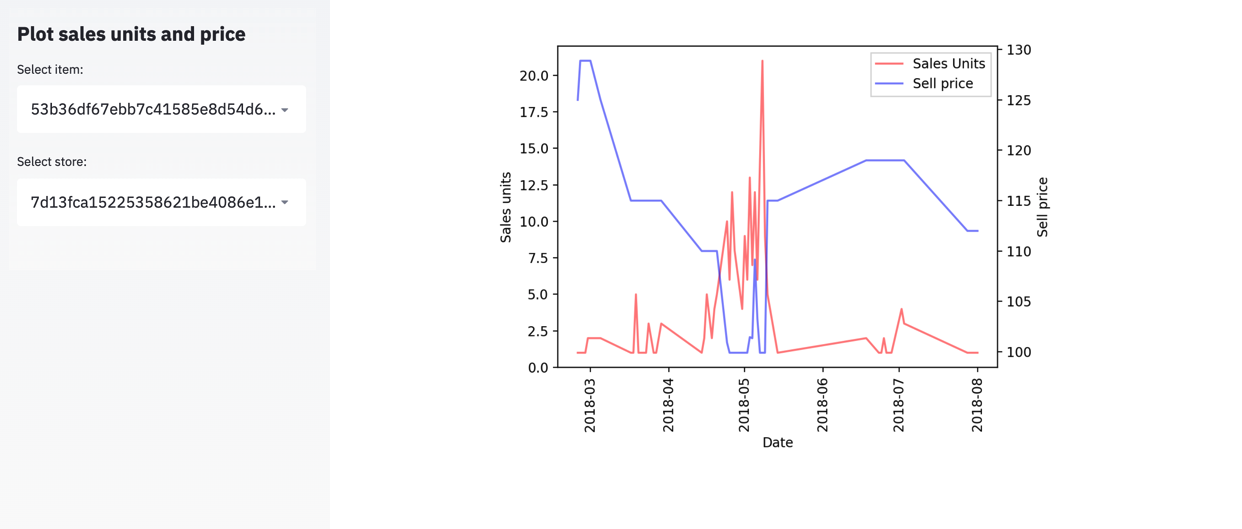
Following our intuition, this sudden increase in sales may be explained by a decrease in price. But we need to continue thinking of possible features that could help us better understand and explain the price elasticity of demand. This is where our Machine Learning models come into play . By including several different features, we can learn from the data which variables are mostly affecting the demand for each item . By modeling this particular item, we would have learned that it is not only the reduction in price that explained the increase in sales but also the days until Mother's Day, the day of the week, and several other variables.
Finally, it's important to stress the relevance of business expertise to help point out those relevant dates when you know sales go up or down. The Machine Learning models help you validate and test new hypotheses.
4. Modeling the demand
Having our data ready, we proceed to train the demand forecasting models. Our goal is to be able to build the demand curve for each item. The models will learn the relation between the demand and several factors such as the price, holidays/events, and macroeconomics.
During this phase, the collaboration with the business team is particularly important. Business insights help to validate the selected features and ensures that we are not missing any important aspect that can be potentially used to forecast the demand.
Below we show an example of the demand curve for an item in the garden tools category.
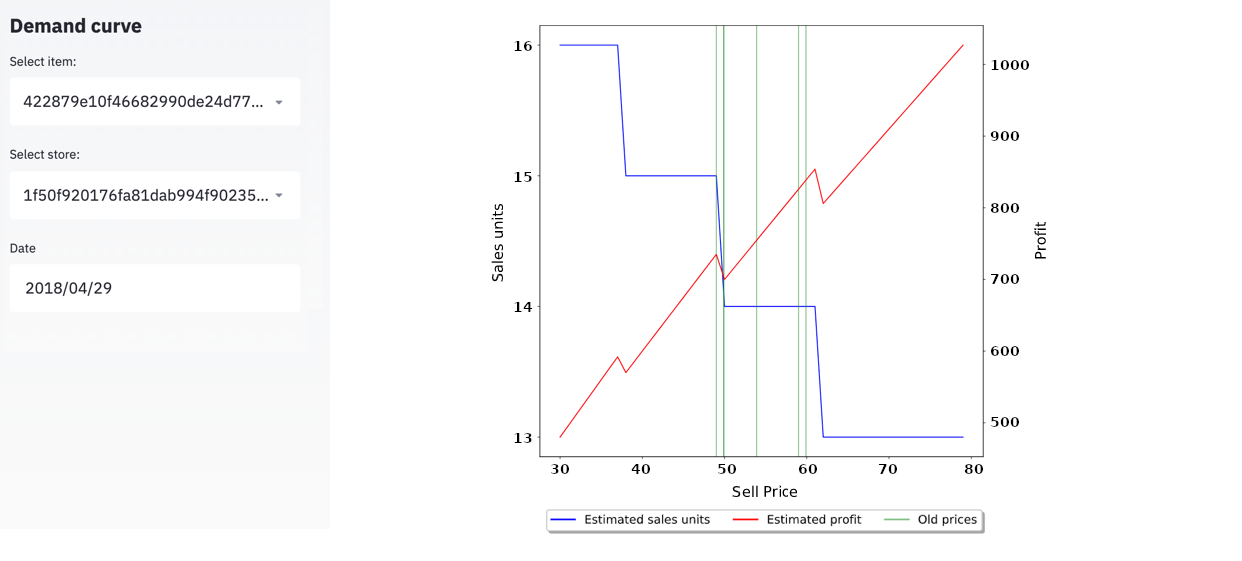
This plot shows the predicted demand curve (blue line) for a particular date (April 29, 2018). The vertical green lines are the historically tested prices (from where our model learned the price elasticity). The red line shows the expected profit (assuming item cost equal to zero, as we don't have this information).
What insights do we get from the estimated demand curve? In this case, we can see that between $50 and $60, the number of units sold would be equal to 14, meaning that in this particular price range and week, the demand curve is completely inelastic. Nevertheless, if we increase the price somewhere close to $63, the number of units sold will decrease, one unit less will be sold but still obtain higher revenue. According to our model estimations, without having a retailer and business background, we would recommend starting with a price between $63 and $ 70 to explore how the demand behaves on this point.
Once we have information about the demand using this price, we can use the latest information to feed the model and then decide which is the following price to set. The advantage of trying prices far from the historically tested ones would be that we may find a new optimum price (exploring). Still, trying prices that have never been tested before brings more uncertainty as the accuracy of the model in those regions is lower.
Moreover, explainability is a desired feature of the models in price optimization. Most of the time, it provides new business insights to retailers and validates that the model is learning correctly. Our models can tell us which features were the most relevant to fit the data. Below we show the top-10 feature importance for the model associated with our garden tool item.
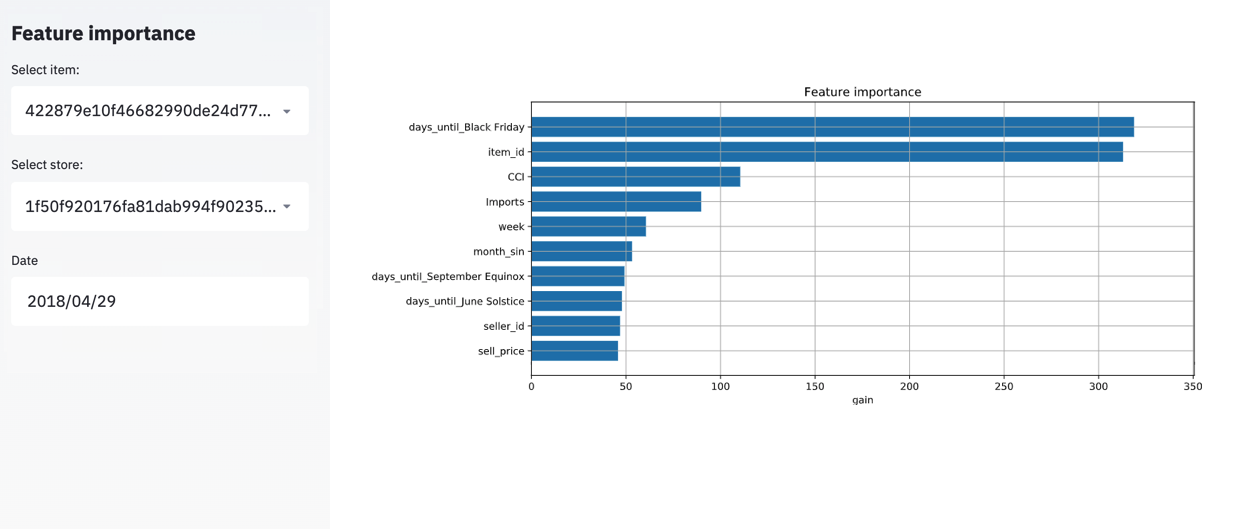
The most important features according to the model are:
- Days until Black Friday
- Consumer Confidence Index
- Total imports in USD
- Week number of the year
- Month number of the year
- Days until Spring
- Days until Winter
As you can see, Black Friday is the most important event for this retailer. This means that each time the feature was used, the model achieves a greater increase in its accuracy. Also, the Consumer Confidence Index (CCI) and the total imports in USD (Imports) are the most relevant macroeconomic factors for this particular item. Furthermore, the beginning of Winter and Spring seasons also play an important role in explaining the demand for our garden tool item.
5. Experimental setup
Once we have our demand curves, we need to decide what exact prices we will try. This is to say, we have to plan what concrete actions we will take, and these actions or strategies will depend on our experimental setup. Three main questions need to be answered to define the experimental setup:
1. How are we going to measure the profit gain for the business as usual?
We need to define a measure against which we will be compared in order to evaluate the progress of our pricing system. We can have different options here, for example:
Control store: we can start by comparing our store's performance against a control one, that will keep using the same business-as-usual pricing strategy. This scenario usually applies to brick-and-mortar stores.
Synthetic control: when we lack a reference or control metric to compare against, we can synthesize one using other available metrics. This is known as synthetic control and provides us a way to measure the profit gain with respect to the business-as-usual even when there is no clear control measure. This method can be used for both e-commerce and brick-and-mortar retail companies.
2. Which price change frequency is best suited in my case?
We need to define the frequency of the price changes, that is if we are going to change the prices hourly, daily, weekly, etc. This will depend on two major factors:
Is your business Brick-and-mortar or e-commerce?
Brick-and-mortar stores, due to the operational limitations of physically changing price tags, usually use weekly or bi-weekly frequencies.
Some of these companies use digital price tags that remove this limitation and let them change prices more frequently. However, if the frequency is too high, customers may feel that the prices in the store are unfair, so this should be taken cautiously.
On the other hand, e-commerce retailers usually select their prices more dynamically, changing prices in the hour-daily range. Often, customers are more likely to accept these higher pricing frequencies since they see this behavior in big players like Amazon.
How accurate are our models for each price change frequency?
The other major factor to consider is the ability of the models to accurately predict the sales units in different time windows. This will depend on various factors, such as the shapes of the demands and the amount of historically price changes, as we discussed in the previous section.
So as a general rule, we should choose the frequency in which our models are accurate enough to perform the predictions, and that maximizes our objective function (e.g., profit) while remaining operationally viable.
3. What will be the Exploration vs. Exploitation strategy.
During exploration , we try new unseen prices that will add crucial information to the data about the shape of the demand curve. On the other hand, during exploitation , we exploit the information that we already know from the demand curves and try to maximize our objective function.
Different strategies can be applied depending on the necessities of the retailer and the quality of the data regarding historical prices. In the Olist dataset , we have 51 items ready to start exploitation. The rest of the items have very few prices tried or very few historical sales and will require some level of exploration.
Here below, we list some recommendations that are useful when defining the exploration strategy:
- If we count on the MSRP, start at this price, and make variations around this price to see how the demand behaves.
- If not, start setting a price near the ones that have been historically tried and make variations around this price to see how the demand behaves.
- With the business team, set a reasonable threshold to the upper and lower boundaries of the exploration price range.
6. Optimization
Once all these questions have been answered, you have everything set to optimize your pricing strategy, as many other retailers are already doing.
Optimizing your pricing strategy means defining your function to be maximized and consider any restrictions to include as constraints. In most scenarios, the objective function to be maximized will be the overall profit subject to the stock level or sales velocity preferred. However, you may be willing to maximize sales volume or customer’s lifetime value. The definition of the optimization problem must be clearly defined with the client.
All in all, we show the opportunity summary report that showcases several promising lines of work.
Opportunity summary report
Final thoughts and concluding remarks.
We have shown how a typical price optimization pipeline would look like, and how we would asses the potential opportunities for a pricing model.
In this particular example, working with the publicly available sales data of the Brazilian marketplace Olist and with no further information nor insights from their side, we have shown that there is room for improvement regarding their pricing decisions.
Combining their available dataset and other publicly available information, such as holidays/events data and macroeconomic series, we have been able to estimate demand curves for a subset of the items which would allow them to take optimal pricing decisions . The remaining items should undergo an exploration phase where new prices would be tried in order to be able to estimate their demand curves accurately. The exploration strategy is generally decided jointly with the client.
For this example, given the data available and our past experience, we would suggest performing weekly price changes during the exploration phase. Furthermore, we have shown that there is plenty of room for inventory management improvement since there seems to be an important amount of revenue lost due to understock.
Finally, during this analysis, we have focused on working with items that have been sold several times during the two years time span. Nevertheless, it is essential to note that there are thousands of items that have been sold only once. For those items, we could think of a price automation solution that could also help your company boost its profit.
In summary, we have shown that price optimization results are immediate and disruptive for companies using traditional pricing. We have also demonstrated that demand forecasting can also be used in other areas of operations, including assortment optimization, marketing, and inventory planning. In other words, investing in a good demand forecasting system opens the door for new opportunities that can be developed component by component based on business priorities.
We can show you how this works, and help you find the opportunities you may be missing.
Get the latest news about price optimization
Subscribe to our pricing with ML newsletter.
Wondering how AI can help you?
Video Analytics
Demand Forecasting
Price Optimization
Product Matching
Predictive Maintenance
AI On The Edge
Transformers
Data Engineering
Collections
Open Source
Partnerships
Terms and Conditions
© 2024 . All rights reserved.
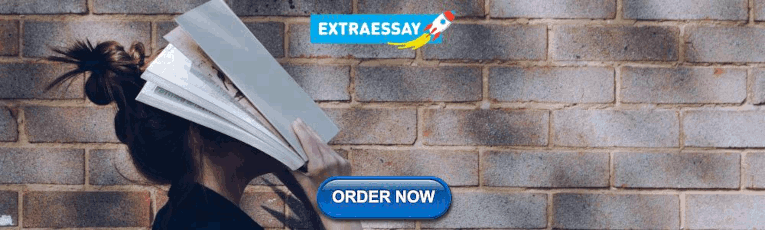
IMAGES
VIDEO
COMMENTS
Machine learning in ecommerce: 10 use cases, examples, and best practices. Machine learning in ecommerce involves the adoption of systems powered by self-learning algorithms to forecast sales trends, fine-tune marketing strategies, streamline inventory management and order deliveries, personalize the shopping experience, and mitigate online ...
1. Amazon. The first thing to say about Amazon machine learning use case in ecommerce is that Amazon uses ML for personalized product recommendations. Their recommendation engine is powerful enough to be considered as the tool providing a huge part of the total business revenue.
To wrap up, Amazon's case study showcases the immense potential of machine learning in driving significant improvements across e-commerce operations. By strategically implementing a machine learning solution, businesses can enhance personalization, optimize demand forecasting, and fortify security, ultimately positioning themselves as leaders ...
Machine learning is a subset of artificial intelligence (AI) and computer science emphasizing on the use of data and algorithms to imitate the way humans learn, continuously enhancing its precision. ML aims at building computer systems that can learn from data. When you check the traditional programming, you execute tasks following explicit ...
Case studies of Machine Learning in E-commerce. According to a recent Juniper Research research, investment on machine learning in the e-commerce industry would increase by 230 % between 2019 and 2023, with 325,000 retailers worldwide using machine learning algorithms in some form by 2023. [1] eBay: Machine Learning for language translation
7. Visual search. Image recognition technology based on machine learning allows visitors to search for a product using an image. After the customer feeds in the photo, the image recognition engine matches the input information with the product database, then selects and displays the closest matches. Visual Search.
Machine Learning: Ecommerce machine learning algorithms classify customers using demographics, behavior, and purchase patterns, ... This case study shows that a product recommendation engine based on machine learning for eCommerce isn't confined to just product industries - it also seamlessly applies to service industries. ...
Use Case: Product Recommendations. Machine learning algorithms analyze customer behavior, purchase history, and preferences to provide personalized product recommendations. This enhances the ...
Here are some key areas in eCommerce where AI/machine learning can be leveraged: Product recommendation: One of the key use cases where machine learning has been used is to provide product recommendations for ecommerce websites. eCommerce businesses rely on product recommendations to drive more sales. Product recommendation helps increase ...
This leads to better decision making. Use Cases for Ecommerce Machine Learning. Machine learning can significantly enhance the online shopping experience, for the customer and the company. From product selection to inventory to security, machine learning makes the shopping experience smoother for all. Personalization.
of compute and data that were available. With the progress made on e-commerce platforms this problem began to attract the attention of machine learning researchers as well. 2.1 Distribution fitting approach A series of probabilistic models link simple past purchase summary statistics with a theoretically well-grounded behavioral [8].
Machine learning, recognized for its transformative potential across various domains within e-commerce, has proven effective in addressing pivotal challenges such as personalized recommendations , customer churn prediction , demand forecasting , and sentiment analysis . In light of this, our study embarks on a transformative journey aimed at ...
Machine learning in e-commerce is a trend of retail digital transformation and is the deployment of self-learning computer algorithms that can automatically enhance their performance via experience.. Machine learning algorithms can be specifically trained by analyzing big and vast datasets, identifying recurrent patterns, relationships, and anomalies throughout all of the data, and creating ...
An AI based on machine learning will reason. Deduce, based on experience. For example, it can help with…. 1. Pricing Optimization. Price is, unsurprisingly, one of the most important factors when considering a purchase. In fact, price is one of the major drivers for at least 47% of customers according to BigCommerce.
Intelligent performance evaluation of e-commerce express services using machine learning: A case study with quantitative analysis. Author links open overlay panel Huwei Liu a 1, ... Perspective on the e-commerce. E-commerce, as a marketing approach that has surged in popularity over the past decade, is rapidly gaining prominence alongside the ...
Greetings, LinkedIn community! 👋. I'm excited to share a captivating case study on how TechTrends, an innovative e-commerce company, utilized machine learning to supercharge its marketing ...
Here are 11 key advantages of using Machine Learning in e-commerce: 1. Improved Customer Retention and Satisfaction. Nobody will contest the fact that maintaining relationships with current clients is simpler and less expensive than finding new ones. Loyal consumers are crucial for your business's growth and development.
Abstract. Nowadays, machine learning (ML) plays the important role in the E-commerce industry and its customer relations to perform different kinds of tasks such as prediction of purchases, segmentation of customers according to their reviews/sentiments, and recommendation of products to the active users. Various ML algorithms are implemented ...
Merchant rating impacts more than I thought it would be, the higher the rating, the more customer confident to buy the product. Customer usually be confident if the merchant's rating is 4.0 minimum.
Predicting User Behavior in e-Commerce Using. Machine Learning. Rumen Ketipov 1, Vera Angelova 1, Lyubka Doukovska 1, Roman. Schnalle 2. 1 Institute of Information and Communication Technologies ...
commerce Using Machine Learning: A Case Study . and Comparative Analysis . Jagdeep Sharma . School of Management and Commerce, RIMT University, Mandi Gobindgarh, India. [email protected] .
In summary, our machine learning model, built using Logistic Regression, is off to a good start, achieving an accuracy score of 0.79 and delivering a strong 0.80 precision and recall for customer ...
1. Data Collection and Integration. The first step is to build our data asset, taking different sources of information that will be used to train our demand forecasting models. The cornerstone to begin working on any price optimization project is the sales dataset, i.e., to have the sales information of your business.