
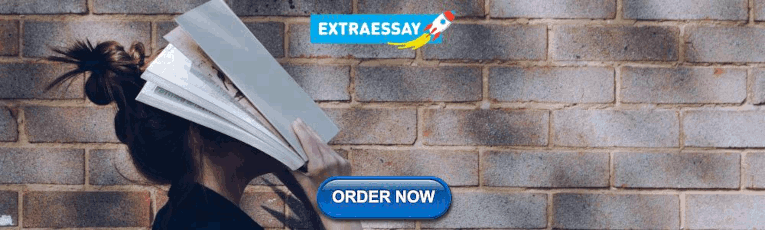
NYU Center for Data Science
Harnessing Data’s Potential for the World
PhD in Data Science
An NRT-sponsored program in Data Science
- Areas & Faculty
- Admission Requirements
- Medical School Track
- NRT FUTURE Program
Advances in computational speed and data availability, and the development of novel data analysis methods, have birthed a new field: data science. This new field requires a new type of researcher and actor: the rigorously trained, cross-disciplinary, and ethically responsible data scientist. Launched in Fall 2017, the pioneering CDS PhD Data Science program seeks to produce such researchers who are fluent in the emerging field of data science, and to develop a native environment for their education and training. The CDS PhD Data Science program has rapidly received widespread recognition and is considered among the top and most selective data science doctoral programs in the world. It has recently been recognized by the NSF through an NRT training grant.
The CDS PhD program model rigorously trains data scientists of the future who (1) develop methodology and harness statistical tools to find answers to questions that transcend the boundaries of traditional academic disciplines; (2) clearly communicate to extract crisp questions from big, heterogeneous, uncertain data; (3) effectively translate fundamental research insights into data science practice in the sciences, medicine, industry, and government; and (4) are aware of the ethical implications of their work.
Our programmatic mission is to nurture this new generation of data scientists, by designing and building a data science environment where methodological innovations are developed and translated successfully to domain applications, both scientific and social. Our vision is that combining fundamental research on the principles of data science with translational projects involving domain experts creates a virtuous cycle: Advances in data science methodology transform the process of discovery in the sciences, and enable effective data-driven governance in the public sector. At the same time, the demands of real-world translational projects will catalyze the creation of new data science methodologies. An essential ingredient of such methodologies is that they embed ethics and responsibility by design.
These objectives will be achieved by a combination of an innovative core curriculum, a novel data assistantship mechanism that provides training of skills transfer through rotations and internships, and communication and entrepreneurship modules. Students will be exposed to a wider range of fields than in more standard PhD programs while working with our interdisciplinary faculty. In particular, we are proud to offer a medical track for students eager to explore data science as applied to healthcare or to develop novel theoretical models stemming from medical questions.
In short, the CDS PhD Data Science program prepares students to become leaders in data science research and prepares them for outstanding careers in academia or industry. Successful candidates are guaranteed financial support in the form of tuition and a competitive stipend in the fall and spring semesters for up to five years.* We invite you to learn more through our webpage or by contacting [email protected] .
*The Ph.D. program also offers students the opportunity to pursue their study and research with Data Science faculty based at NYU Shanghai. With this opportunity, students generally complete their coursework in New York City before moving full-time to Shanghai for their research. For more information, please visit the NYU Shanghai Ph.D. page .
DiscoverDataScience.org
Is a PhD in Data Science Worth It?
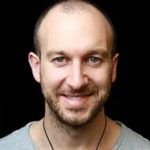
Created by aasif.faizal
The most advanced option you can find is a Data Science PhD, which is an intensive and long-term commitment from which you will graduate at the very top of your field.
The truth is, many who establish thriving careers in data science don’t hold PhDs, and no one would argue that they are necessary to have on the table as one considers their educational options. Estimates for the number of data science PhDs is around one third of all who attend graduate school for data science. For a certain type of person – one who is highly studious, with an aptitude for and interest in research – PhD programs can be excellent experience that will situate you for a highly specialized career.
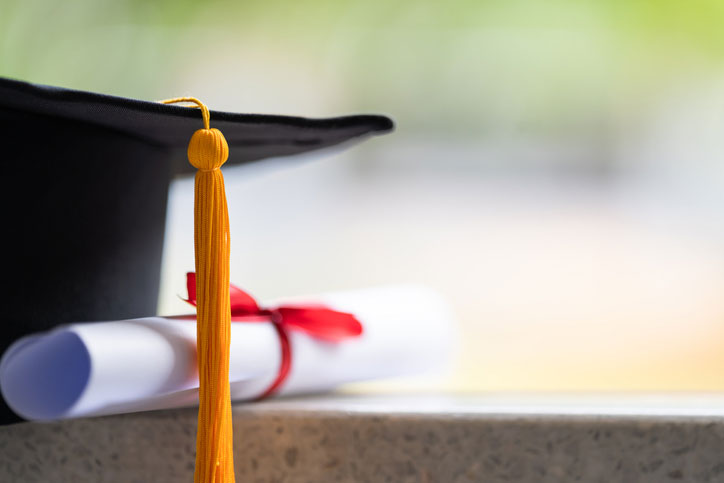
If you’re asking yourself, “Do I need a PhD in Data Science?,” the answer is no. (For a more expansive answer to this question, you can take a look at our article here: “Do I need a PhD in Data Science?”)
But is a PhD in Data Science worth it for those who do decide to take it on? The answer, in short, is yes – at least, it can be. This article will explain the greatest rewards of taking on a doctorate program, with information about job options, Data Science PhD salary ranges, and job growth projections. To learn about all of those as well as survey the other degree options for data scientists, read on.
Advantages of a Data Science PhD
So if a PhD in Data Science isn’t necessary to building a high-earning career in big data, what are the advantages of taking on so many years of schooling? To put it simply, the answer is peerless expertise.
It’s true: one can hold just a master’s degree and still find excellent job opportunities in the data sciences, which is why master’s programs are the most popular path for those in the profession. However, it is unquestionable that a doctorate asserts a higher level of mastery and capability than even master’s degree holders have. If you apply for a job with a PhD on your resume, you’ll be instantly asserting that you are as knowledgeable as they come, which in the case of top-ranking (and top-earning) data science positions is exactly what companies are looking for.
Data Science PhD Programs: How They Work
If you think a doctoral degree in Data Science sounds like the right path for you, it’s worth learning about the specifics of a PhD program. Below is an overview of coursework, anticipated duration, and more.
Coursework and Duration
One of the primary differences between a data science PhD and a master’s program is that a doctorate program culminates in testing and a dissertation, while a master’s program does not. Courses in both programs typically include the following:
- Artificial intelligence
- Data management
- Data mining
- Data visualization
- Machine learning
- Software design
Data science PhDs are known for having an especially intensive orientation toward research, especially in the dissertation component of the work. This can extend the duration of a PhD program by several years. While master’s programs typically take two years if students attend them full-time, a PhD program typically adds two or three years of studying to that timeline.
While many who pursue data science PhDs argue that the insight gained from their extensive dissertation work has paid off in the long run, it’s important to ask yourself if you are going to enjoy making such a deep dive into your studies. If the answer is yes, that’s an excellent reason to proceed with your PhD degree. If not, a master’s program may be the more optimal path for you.
The testing process for data science PhDs is also rigorous, with multiple exams along the way to prove competencies in a variety of subjects. These include oral, written, and practical exams. Earning a PhD asserts by default that you have achieved the mastery needed to pass these tests, which is a powerful assertion of your skill and ability from the get-go.
Finding Your Area of Focus
Like with master’s programs, those pursuing data science degrees typically choose a particular area of focus while in school that will lead directly to their professional specialization. This means it’s crucial to get the lay of the land early so that you’re sure you’re picking a path you’re willing to commit to for a long time. (It’s always possible to acquire deeper insight or even pursue new specialties through certification programs, but it’s recommended to start with one focus that tracks with a degree concentration offered by your school.)
Data Science Salaries
The vast field of data science is proving to be an exceptionally fertile ground to grow a career, no matter what focus area you choose. Indeed, according to the Bureau of Labor Statistics , the median annual pay for data scientists overall is an impressive $100,910 per year, well ahead of most other industries. This is an excellent reason to join this burgeoning field, and it’s been enough to motivate droves of people to pursue data science careers of their own.
If you’re impressed by these numbers, consider this: those statistics describe the overall field of data science, not just the jobs of those who hold PhDs. For these highly advanced professionals, the numbers get much higher. Take a look at the job titles in the next section to see the specific wages of high-ranking data science positions.
While the sudden rush of new candidates seeking data science positions may sound daunting, the job growth statistics for data scientists all but guarantee that high-quality jobs will be available in your area of focus. This is because of the exceptional projected growth rate of data science jobs, which the Bureau of Labor Statistics estimates to be an incredible 36% by 2031.
There are few other industries that offer as significant salaries across the board with so many new positions available.
So why are data scientists so in-demand, and why is the field growing so rapidly? The answer has everything to do with the rise of technology in all aspects of our lives, in particular the way it has transformed how we do business. The rate at which new data technology is evolving means constant adaptations within the world of big data to keep up with it. For example, recent leaps in the field of machine learning (ML) has greatly increased data capturing capacities, leading to a greater need for specialized data analysts who can help process the information quickly.
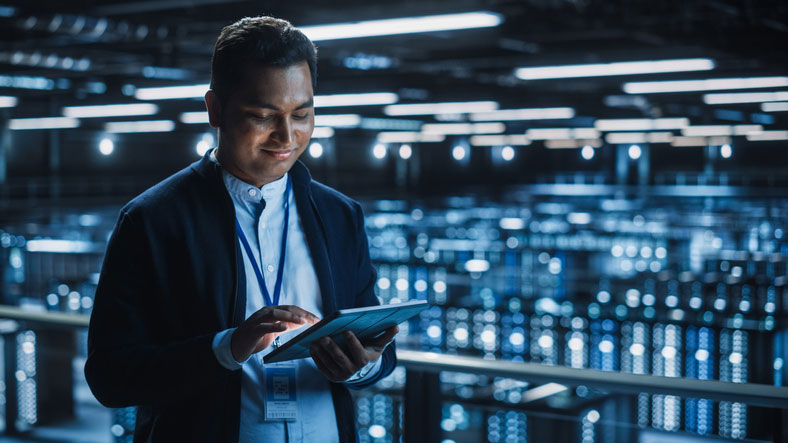
Careers for Data Science PhDs
One of the biggest questions for prospective data science PhD candidates is this: what will it lead to? Indeed, given the rigor of a data science PhD program, it’s important to think through the investment you’re making.
Below are some of the most common positions data scientist PhDs pursue, along with data scientist PhD salary ranges and more.
High Level Data Scientist
Data scientists often pursue more focused concentrations in the field, but their overall functions include collecting and categorizing data so that it can best be leveraged by organizations. Those who hold doctorate degrees in data science are often available for the highest levels of these jobs, which are roles responsible for important decision making functions, oftentimes communicating with executives and other heads of staff on the key insights they’ve acquired in their field.
As you might expect, these high-ranking data science roles earn significant amounts of money. According to the Bureau of Labor Statistics, data scientists earning in the 90th percentile of the field make an annual mean wage of $167,040.
Business Analyst
Business analysts, also often known as management analysts or management consultants, use advanced algorithms to analyze and interpret data that will later be used to guide business strategy. These can be in-house roles at large organizations or consultant positions who are contracted independently on a project basis. Those who excel at business are especially good candidates to pursue this career path.
According to the Bureau of Labor Statistics, management analysts working at the top of their field (in the 90th percentile) earn an annual mean wage of $163,760.
Database Architects
Database architects play a huge role in a business’ data practices, serving as exactly what their name implies – architects who create the virtual structure in which data is stored and organized. It’s imperative that those who hold these roles be highly advanced in their field, as the strength of a business’ database is a crucial factor in the success of its overall operations.
Database architects are highly valued employees and are compensated accordingly. The Bureau of Labor Statistics reports that the top earning database architects in the US make a mean annual wage of $169,500.
Information Security Analysts
The field of cybersecurity is rapidly expanding as new technologies also introduce new types of cyberattacks to databases. Those with rigorous specialization in information security – such as what is conferred by a data science PhD – are ideal candidates to fill these roles. Indeed, companies are unlikely to hire anyone who is not seriously qualified to do this role, as this person will take responsibility for protecting the business’ most vital documents.
The highest earning (90th percentile) information security analysts are reported by the Bureau of Labor Statistics to make a mean annual salary of $165,920.
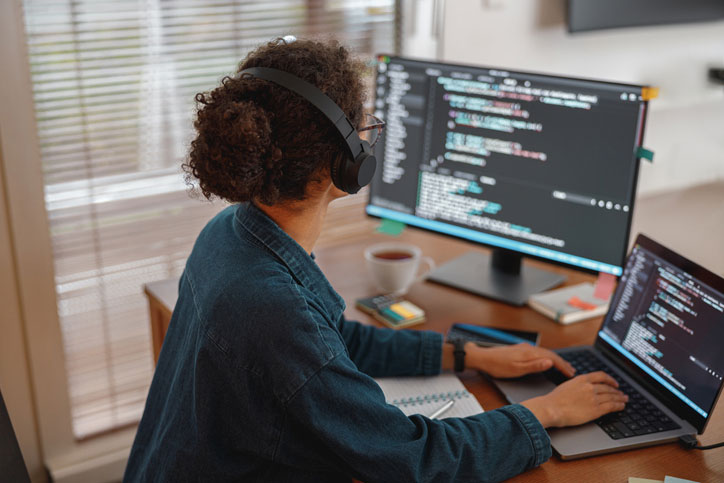
Other Data Science Degree Options
Now that you understand the benefits of a data science PhD program, it’s worth taking stock of the other data science degree and certification options that are available. Good news: all of these degree types have online options, many of which are part-time. This means you can attend school from anywhere, with any schedule.
Data Science Bachelor’s Degree
If you would like to pursue a data science PhD but don’t yet hold a bachelor’s degree in any subject, you will first need to complete a bachelor’s program. If you are in this position, it’s recommended to concentrate on data science during undergraduate school so that you can get a rich introduction to the field, even perhaps finding the area of focus where you’d like to plan your career.
It is possible to start a career in data science with just a bachelor’s degree, though most elect to pursue some level of graduate program, as you will enter the field at a higher level of responsibility, with pay to match. To learn more about bachelor’s in data science degree programs, take a look at our guide here .
Data Science Master’s Degree
A master’s in data science is the most popular path for those entering the field of big data. This degree will give you the expertise needed to find competitive jobs with significant responsibilities and the excellent salaries that draw so many to the data science profession. The coursework for a master’s degree is quite similar to a PhD, minus the intensive testing and the dissertation.
There are numerous fantastic Master’s in Data Science programs that can give you the experience and education needed to find a great position in the field. When choosing a master’s program, be sure it offers a concentration in your intended area of specialty. To take a look at the top online master’s programs available near you, visit our guide here .
Data Science Associates Degree
If you do not have a bachelor’s degree and would like to get your professional life started quickly, an associates degree program can give you the training you need to pursue some entry-level jobs in the world of data science. It’s important to note that these programs on their own are unlikely to give you the expertise needed for a high-earning data science career, but they can offer excellent exposure to the field and provide you with your first work experience.
To learn more about associates in data science degree programs, enjoy our guide here, which will give you all the information you need.
Data Science Certificate
An alternative to a long-term degree program, data science certificates can build a particular area of skill or expertise that can help situate you on a particular career path in data science. Some data science professionals who hold advanced degrees also decide to take on certificate programs to expand on their areas of knowledge or add to their list of specializations.
To learn more about data science certificate programs, visit our comprehensive guide here .
Data Science Bootcamps
Data science bootcamps are likely the fastest possible way to enter the data science profession. These courses – which usually have remote and in-person options – give you a literal crash course in a particular arena of data science, typically over a period of about twelve weeks. You will leave with a developed skill set that usually tracks with a particular type of entry-level job.
Like with most data science opportunities outside of graduate programs, these bootcamps are unlikely to set you up with a high-ranking data science careers, but they can be an excellent way to build your fluency in programming languages or other data science skills.
Data science bootcamps are booming, with plenty of options all over the country. Take a look at our guide here to find the program that is right for you.
Finding the Path That’s Right for You
If you’re feeling overwhelmed by the different opportunities available in the data sciences, don’t worry. While there are indeed many options that are suited to candidates with different skill sets, interests, and backgrounds, the good news is that most of these options are good, and are likely to significantly help you start your career.
For a more elaborate overview of the different program options in data science, take a look at our program guide here for a complete comparison.
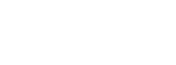
- Related Programs

Ph.D. Specialization in Data Science
The ph.d. specialization in data science is an option within the applied mathematics, computer science, electrical engineering, industrial engineering and operations research, and statistics departments..
Only students already enrolled in one of these doctoral programs at Columbia are eligible to participate in this specialization. Students should fulfill the requirements below in addition to those of their respective department's Ph.D. program. Students should discuss this specialization option with their Ph.D. advisor and their department's director for graduate studies.
Applied Mathematics Doctoral Program
Computer Science Doctoral Program
Decision, Risk, and Operations (DRO) Program
Electrical Engineering Doctoral Program
Industrial Engineering and Operations Research Doctoral Program
Statistics Doctoral Program
The specialization consists of either five (5) courses from the lists below, or four (4) courses plus one (1) additional course approved by the curriculum committee. All courses must be taken for a letter grade and students must pass with a B+ or above. At least three (3) of the courses should come from outside the student’s home department. At least one (1) course has to come from each of the three (3) thematic areas listed below.
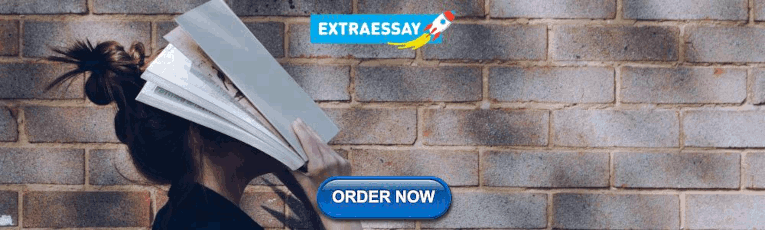
Specialization Requirements
- COMS 4231 Analysis of Algorithms I
- COMS 6232 Analysis of Algorithms II
- COMS 4111 Introduction to Databases
- COMS 4113 Distributed Systems Fundamentals
- EECS 6720 Bayesian Models for Machine Learning
- COMS 4771 Machine Learning
- COMS 4772 Advanced Machine Learning
- IEOR E6613 Optimization I
- IEOR E6614 Optimization II
- IEOR E6711 Stochastic Modeling I
- EEOR E6616 Convex Optimization
- STAT 6301 Probability Theory I
- STAT 6201 Theoretical Statistics I
- STAT 6101 Applied Statistics I
- STAT 6104 Computational Statistics
- STAT 5224 Bayesian Statistics
- STCS 6701 Foundations of Graphical Models (joint with Computer Science)
Information Request Form
Ph.d. specialization committee.
- View All People
- Faculty of Arts and Sciences Professor of Statistics
- The Fu Foundation School of Engineering and Applied Science Professor of Computer Science
Richard A. Davis
- Faculty of Arts and Sciences Howard Levene Professor of Statistics
Vineet Goyal
- The Fu Foundation School of Engineering and Applied Science Associate Professor of Industrial Engineering and Operations Research
Garud N. Iyengar
- The Fu Foundation School of Engineering and Applied Science Vice Dean of Research
- Tang Family Professor of Industrial Engineering and Operations Research
Gail Kaiser
Rocco a. servedio, clifford stein.
- Data Science Institute Interim Director
- The Fu Foundation School of Engineering and Applied Science Wai T. Chang Professor of Industrial Engineering and Operations Research and Professor of Computer Science
John Wright
- The Fu Foundation School of Engineering and Applied Science Associate Professor of Electrical Engineering
- Data Science Institute Associate Director for Academic Affairs
Doctor of Philosophy in Data Science
Developing future pioneers in data science
The School of Data Science at the University of Virginia is committed to educating the next generation of data science leaders. The Ph.D. in Data Science is designed to impart the skills and knowledge necessary to enable research and discovery in data science methods. Because the end goal is to extract knowledge and enable discovery from complex data, the program also boasts robust applied training that is geared toward interdisciplinary collaboration. Doctoral candidates will master the computational and mathematical foundations of data science, and develop competencies in data engineering, software development, data policy and ethics.
Doctoral students in our program apprentice with faculty and pursue advanced research in an interdisciplinary, collaborative environment that is often focused on scientific discovery via data science methods. By serving as teaching assistants for the School’s undergraduate and graduate programs, they learn to be adroit educators and hone their critical thinking and communication skills.
LEARNING OUTCOMES
Pursuing a Ph.D. in Data Science will prepare you to become an expert in the field and work at the cutting edge of a new discipline. According to LinkedIn’s most recent Emerging Jobs Report, data science is booming and data scientist is one of the top three fastest growing jobs. A Ph.D. in Data Science from the University of Virginia opens career paths in academia, industry or government. Graduates of our program will:
- Understand data as a generic concept, and how data encodes and captures information
- Be fluent in modern data engineering techniques, and work with complex and large data sets
- Recognize ethical and legal issues relevant to data analytics and their impact on society
- Develop innovative computational algorithms and novel statistical methods that transform data into knowledge
- Collaborate with research teams from a wide array of scientific fields
- Effectively communicate methods and results to a variety of audiences and stakeholders
- Recognize the broad applicability of data science methods and models
Graduates of the Ph.D. in Data Science will have contributed novel methodological research to the field of data science, demonstrated their work has impactful interdisciplinary applications and defended their methods in an open forum.
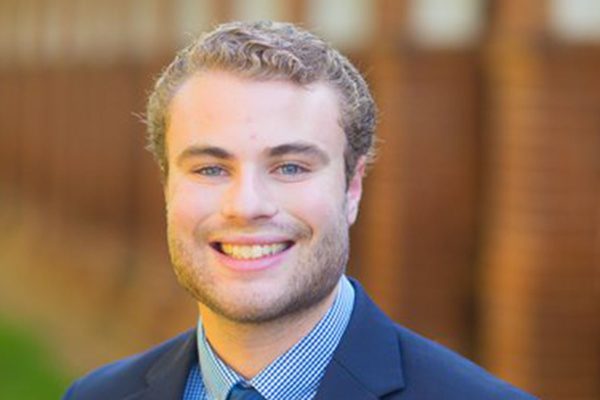
A Week in the Life: First-Year Ph.D. Student
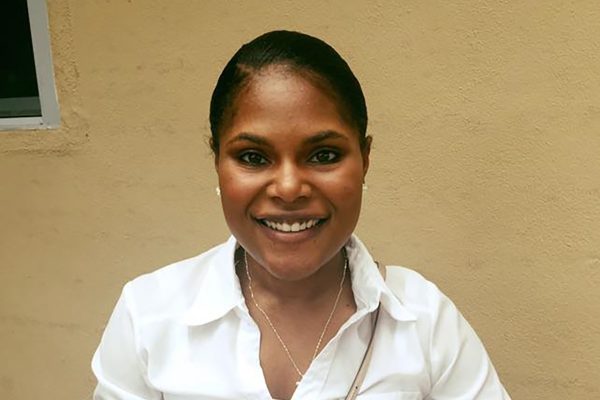
Ph.D. Student Profile: Jade Preston
Ph.D. Student Profile: Beau LeBlond
Get the latest news.
Subscribe to receive updates from the School of Data Science.
- Prospective Student
- School of Data Science Alumnus
- UVA Affiliate
- Industry Member
How I Became a Data Scientist Despite Having Been a Math Major
Caution: the following post is laden with qualitative extrapolation of anecdotes and impressions. Perhaps ironically (though perhaps not), it is not a data driven approach to measuring the efficacy of math majors as data scientists. If you have a differing opinion, I would greatly appreciate you to carefully articulate it and share it with the world.
I recently started my third “real” job since finishing school; at my first and third jobs I have been a “data scientist”. I was a math major in college (and pretty good at it) and spent a year in the math Ph.D. program at the University of Virginia (and performed well there as well). These two facts alone would not have equipped me for a career in data science. In fact, it remains unclear to me that those two facts alone would have prepared me for any career (with the possible exception of teaching) without significantly more training.
“There has never been a better time to be a mathematician”?
When I was in college Business Week published an article declaring “There has never been a better time to be a mathematician.” At the time, I saw an enormous disconnect between the piece and what I was being taught in math classes (and thus what I considered to be a “mathematician”). I have come across other pieces lauding this as the age of the mathematicians, and more often than not, I’ve wondered if the author knew what students actually studied in math departments.
Background on Me
The math courses I had as an undergraduate were:
- Linear algebra
- Discrete math
- Differential equations (ODEs and numerical)
- Theory of statistics 1
- Numerical analysis 1 (numerical linear algebra) and 2 (quadrature)
- Abstract algebra
- Number theory
- Real analysis
- Complex analysis
- Intermediate analysis (point set topology)
My program also required a one semester intro to C++ and two semesters of freshman physics. In my year as a math Ph.D. student, I took analysis, algebra, and topology classes; had I stayed in the program, my future coursework would have been similar: pure math where homework problems consistent almost exclusively of proofs done with pen and paper (or in LaTeX).
What is Data Science?
Though my current position occasionally requires mathematical proof, I suspect that is rare among data scientist. While the “data science” demarcation problem is challenging (and I will not seek to solve it here), it seems evident that my curriculum lacked preparation in many essential areas of data science. Chief among these are programming skill, knowledge of experimental statistics, and experience with math modeling.
Data Science Requires Programming and Engineering
Few would argue that programming ability is not a key skill of data science. As Drew Conway has argued , a data scientist need not have a degree in computer science, but “Being able to manipulate text files at the command-line, understanding vectorized operations, thinking algorithmically; these are the hacking skills that make for a successful data hacker.” Many of my undergrad peers, having briefly seen C++ freshman year and occasionally used Mathematica to solve ODEs for homework assignments, would have been unaware that manipulation of a file from the command-line was even possible, much less have been able to write a simple sed script; there was little difference with my grad school classmates.
Many data science positions require even more than the ability to solve problems with code. As Trey Causey has recently explained , many positions require understanding of software engineering skills and tools such as writing reusable code, using version control, software testing, and logging. Though I gained a fair bit of programming skill in college, these skills, now essential in my daily work, remained foreign to me until years later.
Data Science Requires Applied Statistics
My math training had a lack of statistics courses. Though my brief exposure to mathematical statistics has been valuable in picking up machine learning, experimental statistics was missing altogether. Many data science teams are interested in questions of causal inference and design and analysis of experiments; some would make these essential skills for a data scientist. I learned nothing about these topics in math departments. Moreover, machine learning, also a cornerstone of data science, is not a subject I could have even defined until after I was finished with my math coursework; at the end of college, I would have said artificial intelligence was mostly about rule-based systems in Lisp and Prolog.
Data Science Involves Very Applied Math
Even if statistics had play a more prominent role in my coursework, those who have studied statistics know there is often a gulf between understanding textbook statistics and being able to effectively apply statistical models and methods to real world problems. This is only an aspect of a bigger issue: mathematical (including statistical) modeling is an extraordinarily challenging problem, but instruction on effectively model real world problems is absent from many math programs. To this day, defining my problem in mathematical terms one of the hardest problems I face; I am certain that I am not alone on this. Though I am now armed with a wide variety of mathematical models, it is rarely clear exactly which model can or should be applied in a given situation.
I suspect that many people, even technical people, are uncertain as to what academic math is beyond undergraduate calculus. Mathematicians mostly work in the logical manipulation of abstractly defined structures. These structures rarely bear any necessary relationship to physical entities or data sets outside the abstractly defined domain of discourse. Though some might argue I am speaking only of “pure” mathematics, this is often true of what is formally known as “applied mathematics”. John D. Cook has made similar observations about the limitations of pure and applied math (as proper disciplines) in dubbing himself a “very applied mathematician”. Very applied mathematics is “an interest in the grubby work required to see the math actually used and a willingness to carry it out. This involves not just math but also computing, consulting, managing, marketing, etc.” These skills are conspicuously absent from most math curricula I am familiar with.
Math → Data Science
Given this description of how my schooling left me woefully unprepared for a career in data science, one might ask how I have had two jobs with that title. I can think of several (though probably not all) reasons.
First, the academic study of mathematics provides much of the theoretical underpinnings of data science. Mathematics underlies the study of machine learning, statistics, optimization, data structures, analysis of algorithms, computer architecture, and other important aspects of data science. Knowledge of mathematics (potentially) allows the learner to more quickly grasp each of these fields. For example, learning how principle component analysis —a math model that can be applied and interpreted by someone without formal mathematical training—works will be significantly easier for someone with earlier exposure linear algebra. On a meta-level, training in mathematics forces students to think carefully and solve hard problems; these skills are valuable in many fields, including data science.
My second reason is connect to the first: I unwittingly took a number of courses that later played important roles in my data science toolkit. For example, my current work in Bayesian inference has been made possible by my knowledge of linear algebra, numerical analysis, stochastic processes, measure theory, and mathematical statistics.
Third, I did a minor in computer science as an undergraduate. That provided a solid foundation for me when I decided to get serious about building programming skill in 2010. Though my academic exposure to computer science lacked any software engineer skills, I left college with a solid grasp of basic data structures, analysis of algorithms, complexity theory, and a handful of programming languages.
Fourth, I did a masters degree in operations research (after my year as a math PhD student convinced me pure math wasn’t for me). This provided me with experience in math modeling, a broad knowledge of mathematical optimization (central to machine learning), and the opportunity to take graduate-level machine learning classes. 1
Fifth, my insatiable curiosity in computers and problem solving has played a key role in my career success. Eager to learn something about computer programming, I taught myself PHP and SQL as a high school student (to make Tolkien fan sites, incidentally). Having been given small Mathematica-based homework assignments in freshman differential equations, I bought and read a book on programming Mathematica. Throughout college and grad school, I often tried—and sometimes succeeded—to write programs to solve homework problems that professors expected to be solved by hand. This curiosity has proven valuable time and time again as I’ve been required to learn new skills and solve technical problems of all varieties. I’m comfortable jumping in to solve a new problem at work, because I’ve been doing that on my own time for fifteen years.
Sixth, I have been been fortunate enough to have employers who have patiently taught me and given me the freedom to learn on my own. I have learned an enormous amount in my two and a half year professional career, and I don’t anticipate slowing down any time soon. As Mat Kelcey has said: always be sure you’re not the smartest one in the room. I am very thankful for three jobs where I’ve been surrounded by smart people who have taught me a lot, and for supervisors who trust me enough to let me learn on my own.
Finally, 2 it would be hard for me to overvalue the four and a half years of participation in the data science community on Twitter. Through Twitter, I have the ear of some of data science’s brightest minds (most of whom I’ve never met in person), and I’ve built a peer network that has helped me find my current and last job. However, I mostly want to emphasize the pedagogical value of Twitter. Every day, I’m updated on the release of new software tools for data science, the best new blog posts for our field, and the musings of of some of my data science heros. Of course, I don’t read every blog post or learn every software tool. But Twitter helps me to recognize which posts are most worth my time, and because of Twitter, I know something instead of nothing about Theano, Scalding, and dplyr. 3
Conclusions
I don’t know to what extent my experience generalizes 4 , in either the limitations of my education or my analysis of my success, but I am obviously not going to let that stop me from drawing some general conclusions.
Hiring Data Scientists
For those hiring data scientists, recognize that mathematics as taught might not be the same mathematics you need from your team. Plenty of people with PhDs in mathematics would be unable to define linear regression or bloom filters. At the same time, recognize that math majors are taught to think well and solve hard problems; these skills shouldn’t be undervalued. Math majors are also experienced in reading and learning math! They may be able to read academic papers and understand difficult (even if new) mathematical more quickly than a computer scientist or social scientist. Given enough practice and training, they would probably be excellent programmers.
Studying Math
For those studying math, recognize that the field you love, in its formal sense, may be keeping you away from enjoyable and lucrative careers. Most of your math professors have spent their adult lives solving math problems on paper or on a chalkboard. They are inexperienced and, possibly, unknowledgeable about very applied mathematics . A successful career in pure mathematics will be very hard and will require you to be very good. While there seem to be lots of jobs in teaching, they will rarely pay well.
If you’re still an student, you have a great opportunity to take control of your career path. Consider taking computer science classes (e.g. data structures, algorithms, software engineering, machine learning) and statistics classes (e.g. experimental design, data analysis, data mining).
For both students and graduates, recognize your math knowledge becomes very marketable when combined skills such as programming and machine learning; there are a wealth of good books, MOOCs, and blog posts that can help you learn these things. More over, the barrier to entry for getting started with production quality tools has never been lower. Don’t let your coursework be the extent of your education. There is so much more to learn! 5
Update (Oct. 2017) : I gave a talk based on this post
Update (Mar. 2018) : I get a lot of emails with questions about this post, so I wrote FAQ post trying to answer some of them.
At the same time, my academic training in operations research failed me, in some aspects, for a successful career in operations research. For example, practical math modeling was not sufficiently emphasized and the skills of computer programming and software development were undervalued. ↩︎
Of course, I have plenty of data science skills left to learn. My knowledge of experimental design is still pretty fuzzy. I still struggle with effective mathematical modeling. I haven’t deployed a large scale machine learning system to production. I suck at software logging. I have no idea how deep learning works. ↩︎
I have successfully answered more than one interview question by regurgitating knowledge gleaned from tweets. ↩︎
Among other reasons, I didn’t really plan to get where I am today. I changed majors no fewer than three times in college (physics, CS, and math) and essentially dropped out of two PhD programs! ↩︎
For example, install Anaconda and start playing with some of these IPython notebooks . ↩︎
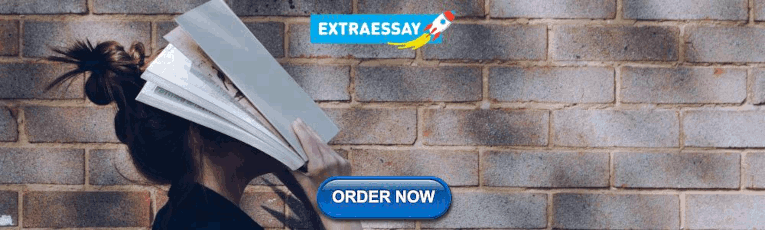
IMAGES
VIDEO
COMMENTS
There are pros/cons to starting a PhD after taking a break and swimming in money from your job in industry. The pay as a grad student sucks! You're paid a barely livable wage with shit health insurance. Huge opportunity cost to consider, especially with a 401k. My employer plasters PhD or Dr on everything.
10 subscribers in the TheDailyUnfold community. Welcome to The Daily Unfold, your go-to source for the latest news and current events from around the…
An NRT-sponsored program in Data Science Overview Overview Advances in computational speed and data availability, and the development of novel data analysis methods, have birthed a new field: data science. This new field requires a new type of researcher and actor: the rigorously trained, cross-disciplinary, and ethically responsible data scientist. Launched in Fall 2017, the …
Below are some of the most common positions data scientist PhDs pursue, along with data scientist PhD salary ranges and more. High Level Data Scientist Data scientists often pursue more focused concentrations in the field, but their overall functions include collecting and categorizing data so that it can best be leveraged by organizations.
Students should discuss this specialization option with their Ph.D. advisor and their department's director for graduate studies. The specialization consists of either five (5) courses from the lists below, or four (4) courses plus one (1) additional course approved by the curriculum committee. All courses must be taken for a letter grade and ...
A Ph.D. in Data Science from the University of Virginia opens career paths in academia, industry or government. Graduates of our program will: Understand data as a generic concept, and how data encodes and captures information. Be fluent in modern data engineering techniques, and work with complex and large data sets.
Data Science teams are usually using both languages as some prefer R and others Python. In the end, it doesn't really matter as some models have to be reimplemented in a compiled language (Java, Go) to make faster predictions in production. Python enables you to do the analysis, develop the model from scratch and run it in production.
As Drew Conway has argued, a data scientist need not have a degree in computer science, but "Being able to manipulate text files at the command-line, understanding vectorized operations, thinking algorithmically; these are the hacking skills that make for a successful data hacker.".