
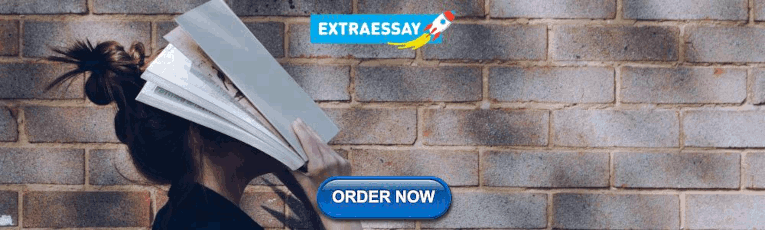
Best 151+ Quantitative Research Topics for STEM Students

In today’s rapidly evolving world, STEM (Science, Technology, Engineering, and Mathematics) fields have gained immense significance. For STEM students, engaging in quantitative research is a pivotal aspect of their academic journey. Quantitative research involves the systematic collection and interpretation of numerical data to address research questions or test hypotheses. Choosing the right research topic is essential to ensure a successful and meaningful research endeavor.
In this blog, we will explore 151+ quantitative research topics for STEM students. Whether you are an aspiring scientist, engineer, or mathematician, this comprehensive list will inspire your research journey. But we understand that the journey through STEM education and research can be challenging at times. That’s why we’re here to support you every step of the way with our Engineering Assignment Help service.
What is Quantitative Research in STEM?
Table of Contents
Quantitative research is a scientific approach that relies on numerical data and statistical analysis to draw conclusions and make predictions. In STEM fields, quantitative research encompasses a wide range of methodologies, including experiments, surveys, and data analysis. The key characteristics of quantitative research in STEM include:
- Data Collection: Systematic gathering of numerical data through experiments, observations, or surveys.
- Statistical Analysis: Application of statistical techniques to analyze data and draw meaningful conclusions.
- Hypothesis Testing: Testing hypotheses and theories using quantitative data.
- Replicability: The ability to replicate experiments and obtain consistent results.
- Generalizability: Drawing conclusions that can be applied to larger populations or phenomena.
Importance of Quantitative Research Topics for STEM Students
Quantitative research plays a pivotal role in STEM education and research for several reasons:
1. Empirical Evidence
It provides empirical evidence to support or refute scientific theories and hypotheses.
2. Data-Driven Decision-Making
STEM professionals use quantitative research to make informed decisions, from designing experiments to developing new technologies.
3. Innovation
It fuels innovation by providing data-driven insights that lead to the creation of new products, processes, and technologies.
4. Problem Solving
STEM students learn critical problem-solving skills through quantitative research, which are invaluable in their future careers.
5. Interdisciplinary Applications
Quantitative research transcends STEM disciplines, facilitating collaboration and the tackling of complex, real-world problems.
Also Read: Google Scholar Research Topics
Quantitative Research Topics for STEM Students
Now, let’s explore important quantitative research topics for STEM students:
Biology and Life Sciences
Here are some quantitative research topics in biology and life science:
1. The impact of climate change on biodiversity.
2. Analyzing the genetic basis of disease susceptibility.
3. Studying the effectiveness of vaccines in preventing infectious diseases.
4. Investigating the ecological consequences of invasive species.
5. Examining the role of genetics in aging.
6. Analyzing the effects of pollution on aquatic ecosystems.
7. Studying the evolution of antibiotic resistance.
8. Investigating the relationship between diet and lifespan.
9. Analyzing the impact of deforestation on wildlife.
10. Studying the genetics of cancer development.
11. Investigating the effectiveness of various plant fertilizers.
12. Analyzing the impact of microplastics on marine life.
13. Studying the genetics of human behavior.
14. Investigating the effects of pollution on plant growth.
15. Analyzing the microbiome’s role in human health.
16. Studying the impact of climate change on crop yields.
17. Investigating the genetics of rare diseases.
Let’s get started with some quantitative research topics for stem students in chemistry:
1. Studying the properties of superconductors at different temperatures.
2. Analyzing the efficiency of various catalysts in chemical reactions.
3. Investigating the synthesis of novel polymers with unique properties.
4. Studying the kinetics of chemical reactions.
5. Analyzing the environmental impact of chemical waste disposal.
6. Investigating the properties of nanomaterials for drug delivery.
7. Studying the behavior of nanoparticles in different solvents.
8. Analyzing the use of renewable energy sources in chemical processes.
9. Investigating the chemistry of atmospheric pollutants.
10. Studying the properties of graphene for electronic applications.
11. Analyzing the use of enzymes in industrial processes.
12. Investigating the chemistry of alternative fuels.
13. Studying the synthesis of pharmaceutical compounds.
14. Analyzing the properties of materials for battery technology.
15. Investigating the chemistry of natural products for drug discovery.
16. Analyzing the effects of chemical additives on food preservation.
17. Investigating the chemistry of carbon capture and utilization technologies.
Here are some quantitative research topics in physics for stem students:
1. Investigating the behavior of subatomic particles in high-energy collisions.
2. Analyzing the properties of dark matter and dark energy.
3. Studying the quantum properties of entangled particles.
4. Investigating the dynamics of black holes and their gravitational effects.
5. Analyzing the behavior of light in different mediums.
6. Studying the properties of superfluids at low temperatures.
7. Investigating the physics of renewable energy sources like solar cells.
8. Analyzing the properties of materials at extreme temperatures and pressures.
9. Studying the behavior of electromagnetic waves in various applications.
10. Investigating the physics of quantum computing.
11. Analyzing the properties of magnetic materials for data storage.
12. Studying the behavior of particles in plasma for fusion energy research.
13. Investigating the physics of nanoscale materials and devices.
14. Analyzing the properties of materials for use in semiconductors.
15. Studying the principles of thermodynamics in energy efficiency.
16. Investigating the physics of gravitational waves.
17. Analyzing the properties of materials for use in quantum technologies.
Engineering
Let’s explore some quantitative research topics for stem students in engineering:
1. Investigating the efficiency of renewable energy systems in urban environments.
2. Analyzing the impact of 3D printing on manufacturing processes.
3. Studying the structural integrity of materials in aerospace engineering.
4. Investigating the use of artificial intelligence in autonomous vehicles.
5. Analyzing the efficiency of water treatment processes in civil engineering.
6. Studying the impact of robotics in healthcare.
7. Investigating the optimization of supply chain logistics using quantitative methods.
8. Analyzing the energy efficiency of smart buildings.
9. Studying the effects of vibration on structural engineering.
10. Investigating the use of drones in agricultural practices.
11. Analyzing the impact of machine learning in predictive maintenance.
12. Studying the optimization of transportation networks.
13. Investigating the use of nanomaterials in electronic devices.
14. Analyzing the efficiency of renewable energy storage systems.
15. Studying the impact of AI-driven design in architecture.
16. Investigating the optimization of manufacturing processes using Industry 4.0 technologies.
17. Analyzing the use of robotics in underwater exploration.
Environmental Science
Here are some top quantitative research topics in environmental science for students:
1. Investigating the effects of air pollution on respiratory health.
2. Analyzing the impact of deforestation on climate change.
3. Studying the biodiversity of coral reefs and their conservation.
4. Investigating the use of remote sensing in monitoring deforestation.
5. Analyzing the effects of plastic pollution on marine ecosystems.
6. Studying the impact of climate change on glacier retreat.
7. Investigating the use of wetlands for water quality improvement.
8. Analyzing the effects of urbanization on local microclimates.
9. Studying the impact of oil spills on aquatic ecosystems.
10. Investigating the use of renewable energy in mitigating greenhouse gas emissions.
11. Analyzing the effects of soil erosion on agricultural productivity.
12. Studying the impact of invasive species on native ecosystems.
13. Investigating the use of bioremediation for soil cleanup.
14. Analyzing the effects of climate change on migratory bird patterns.
15. Studying the impact of land use changes on water resources.
16. Investigating the use of green infrastructure for urban stormwater management.
17. Analyzing the effects of noise pollution on wildlife behavior.
Computer Science
Let’s get started with some simple quantitative research topics for stem students:
1. Investigating the efficiency of machine learning algorithms for image recognition.
2. Analyzing the security of blockchain technology in financial transactions.
3. Studying the impact of quantum computing on cryptography.
4. Investigating the use of natural language processing in chatbots and virtual assistants.
5. Analyzing the effectiveness of cybersecurity measures in protecting sensitive data.
6. Studying the impact of algorithmic trading in financial markets.
7. Investigating the use of deep learning in autonomous robotics.
8. Analyzing the efficiency of data compression algorithms for large datasets.
9. Studying the impact of virtual reality in medical simulations.
10. Investigating the use of artificial intelligence in personalized medicine.
11. Analyzing the effectiveness of recommendation systems in e-commerce.
12. Studying the impact of cloud computing on data storage and processing.
13. Investigating the use of neural networks in predicting disease outbreaks.
14. Analyzing the efficiency of data mining techniques in customer behavior analysis.
15. Studying the impact of social media algorithms on user behavior.
16. Investigating the use of machine learning in natural language translation.
17. Analyzing the effectiveness of sentiment analysis in social media monitoring.
Mathematics
Let’s explore the quantitative research topics in mathematics for students:
1. Investigating the properties of prime numbers and their distribution.
2. Analyzing the behavior of chaotic systems using differential equations.
3. Studying the optimization of algorithms for solving complex mathematical problems.
4. Investigating the use of graph theory in network analysis.
5. Analyzing the properties of fractals in natural phenomena.
6. Studying the application of probability theory in risk assessment.
7. Investigating the use of numerical methods in solving partial differential equations.
8. Analyzing the properties of mathematical models for population dynamics.
9. Studying the optimization of algorithms for data compression.
10. Investigating the use of topology in data analysis.
11. Analyzing the behavior of mathematical models in financial markets.
12. Studying the application of game theory in strategic decision-making.
13. Investigating the use of mathematical modeling in epidemiology.
14. Analyzing the properties of algebraic structures in coding theory.
15. Studying the optimization of algorithms for image processing.
16. Investigating the use of number theory in cryptography.
17. Analyzing the behavior of mathematical models in climate prediction.
Earth Sciences
Here are some quantitative research topics for stem students in earth science:
1. Investigating the impact of volcanic eruptions on climate patterns.
2. Analyzing the behavior of earthquakes along tectonic plate boundaries.
3. Studying the geomorphology of river systems and erosion.
4. Investigating the use of remote sensing in monitoring wildfires.
5. Analyzing the effects of glacier melt on sea-level rise.
6. Studying the impact of ocean currents on weather patterns.
7. Investigating the use of geothermal energy in renewable power generation.
8. Analyzing the behavior of tsunamis and their destructive potential.
9. Studying the impact of soil erosion on agricultural productivity.
10. Investigating the use of geological data in mineral resource exploration.
11. Analyzing the effects of climate change on coastal erosion.
12. Studying the geomagnetic field and its role in navigation.
13. Investigating the use of radar technology in weather forecasting.
14. Analyzing the behavior of landslides and their triggers.
15. Studying the impact of groundwater depletion on aquifer systems.
16. Investigating the use of GIS (Geographic Information Systems) in land-use planning.
17. Analyzing the effects of urbanization on heat island formation.
Health Sciences and Medicine
Here are some quantitative research topics for stem students in health science and medicine:
1. Investigating the effectiveness of telemedicine in improving healthcare access.
2. Analyzing the impact of personalized medicine in cancer treatment.
3. Studying the epidemiology of infectious diseases and their spread.
4. Investigating the use of wearable devices in monitoring patient health.
5. Analyzing the effects of nutrition and exercise on metabolic health.
6. Studying the impact of genetics in predicting disease susceptibility.
7. Investigating the use of artificial intelligence in medical diagnosis.
8. Analyzing the behavior of pharmaceutical drugs in clinical trials.
9. Studying the effectiveness of mental health interventions in schools.
10. Investigating the use of gene editing technologies in treating genetic disorders.
11. Analyzing the properties of medical imaging techniques for early disease detection.
12. Studying the impact of vaccination campaigns on public health.
13. Investigating the use of regenerative medicine in tissue repair.
14. Analyzing the behavior of pathogens in antimicrobial resistance.
15. Studying the epidemiology of chronic diseases like diabetes and heart disease.
16. Investigating the use of bioinformatics in genomics research.
17. Analyzing the effects of environmental factors on health outcomes.
Quantitative research is the backbone of STEM fields, providing the tools and methodologies needed to explore, understand, and innovate in the world of science and technology . As STEM students, embracing quantitative research not only enhances your analytical skills but also equips you to address complex real-world challenges. With the extensive list of 155+ quantitative research topics for stem students provided in this blog, you have a starting point for your own STEM research journey. Whether you’re interested in biology, chemistry, physics, engineering, or any other STEM discipline, there’s a wealth of quantitative research topics waiting to be explored. So, roll up your sleeves, grab your lab coat or laptop, and embark on your quest for knowledge and discovery in the exciting world of STEM.
I hope you enjoyed this blog post about quantitative research topics for stem students.
Related Posts
8 easiest programming language to learn for beginners.
There are so many programming languages you can learn. But if you’re looking to start with something easier. We bring to you a list of…
10 Online Tutoring Help Benefits
Do you need a computer science assignment help? Get the best quality assignment help from computer science tutors at affordable prices. They always presented to help…

200+ Experimental Quantitative Research Topics For STEM Students In 2023

STEM means Science, Technology, Engineering, and Math, which is not the only stuff we learn in school. It is like a treasure chest of skills that help students become great problem solvers, ready to tackle the real world’s challenges.
In this blog, we are here to explore the world of Research Topics for STEM Students. We will break down what STEM really means and why it is so important for students. In addition, we will give you the lowdown on how to pick a fascinating research topic. We will explain a list of 200+ Experimental Quantitative Research Topics For STEM Students.
And when it comes to writing a research title, we will guide you step by step. So, stay with us as we unlock the exciting world of STEM research – it is not just about grades; it is about growing smarter, more confident, and happier along the way.
What Is STEM?
Table of Contents
STEM is Science, Technology, Engineering, and Mathematics. It is a way of talking about things like learning, jobs, and activities related to these four important subjects. Science is about understanding the world around us, technology is about using tools and machines to solve problems, engineering is about designing and building things, and mathematics is about numbers and solving problems with them. STEM helps us explore, discover, and create cool stuff that makes our world better and more exciting.
Why STEM Research Is Important?
STEM research is important because it helps us learn new things about the world and solve problems. When scientists, engineers, and mathematicians study these subjects, they can discover cures for diseases, create new technology that makes life easier, and build things that help us live better. It is like a big puzzle where we put together pieces of knowledge to make our world safer, healthier, and more fun.
- STEM research leads to new discoveries and solutions.
- It helps find cures for diseases.
- STEM technology makes life easier.
- Engineers build things that improve our lives.
- Mathematics helps us understand and solve complex problems.
How to Choose a Topic for STEM Research Paper
Here are some steps to choose a topic for STEM Research Paper:
Step 1: Identify Your Interests
Think about what you like and what excites you in science, technology, engineering, or math. It could be something you learned in school, saw in the news, or experienced in your daily life. Choosing a topic you’re passionate about makes the research process more enjoyable.
Step 2: Research Existing Topics
Look up different STEM research areas online, in books, or at your library. See what scientists and experts are studying. This can give you ideas and help you understand what’s already known in your chosen field.
Step 3: Consider Real-World Problems
Think about the problems you see around you. Are there issues in your community or the world that STEM can help solve? Choosing a topic that addresses a real-world problem can make your research impactful.
Step 4: Talk to Teachers and Mentors
Discuss your interests with your teachers, professors, or mentors. They can offer guidance and suggest topics that align with your skills and goals. They may also provide resources and support for your research.
Step 5: Narrow Down Your Topic
Once you have some ideas, narrow them down to a specific research question or project. Make sure it’s not too broad or too narrow. You want a topic that you can explore in depth within the scope of your research paper.
Here we will discuss 200+ Experimental Quantitative Research Topics For STEM Students:
Qualitative Research Topics for STEM Students:
Qualitative research focuses on exploring and understanding phenomena through non-numerical data and subjective experiences. Here are 10 qualitative research topics for STEM students:
- Exploring the experiences of female STEM students in overcoming gender bias in academia.
- Understanding the perceptions of teachers regarding the integration of technology in STEM education.
- Investigating the motivations and challenges of STEM educators in underprivileged schools.
- Exploring the attitudes and beliefs of parents towards STEM education for their children.
- Analyzing the impact of collaborative learning on student engagement in STEM subjects.
- Investigating the experiences of STEM professionals in bridging the gap between academia and industry.
- Understanding the cultural factors influencing STEM career choices among minority students.
- Exploring the role of mentorship in the career development of STEM graduates.
- Analyzing the perceptions of students towards the ethics of emerging STEM technologies like AI and CRISPR.
- Investigating the emotional well-being and stress levels of STEM students during their academic journey.
Easy Experimental Research Topics for STEM Students:
These experimental research topics are relatively straightforward and suitable for STEM students who are new to research:
- Measuring the effect of different light wavelengths on plant growth.
- Investigating the relationship between exercise and heart rate in various age groups.
- Testing the effectiveness of different insulating materials in conserving heat.
- Examining the impact of pH levels on the rate of chemical reactions.
- Studying the behavior of magnets in different temperature conditions.
- Investigating the effect of different concentrations of a substance on bacterial growth.
- Testing the efficiency of various sunscreen brands in blocking UV radiation.
- Measuring the impact of music genres on concentration and productivity.
- Examining the correlation between the angle of a ramp and the speed of a rolling object.
- Investigating the relationship between the number of blades on a wind turbine and energy output.
Research Topics for STEM Students in the Philippines:
These research topics are tailored for STEM students in the Philippines:
- Assessing the impact of climate change on the biodiversity of coral reefs in the Philippines.
- Studying the potential of indigenous plants in the Philippines for medicinal purposes.
- Investigating the feasibility of harnessing renewable energy sources like solar and wind in rural Filipino communities.
- Analyzing the water quality and pollution levels in major rivers and lakes in the Philippines.
- Exploring sustainable agricultural practices for small-scale farmers in the Philippines.
- Assessing the prevalence and impact of dengue fever outbreaks in urban areas of the Philippines.
- Investigating the challenges and opportunities of STEM education in remote Filipino islands.
- Studying the impact of typhoons and natural disasters on infrastructure resilience in the Philippines.
- Analyzing the genetic diversity of endemic species in the Philippine rainforests.
- Assessing the effectiveness of disaster preparedness programs in Philippine communities.
Read More
- Frontend Project Ideas
- Business Intelligence Projects For Beginners
Good Research Topics for STEM Students:
These research topics are considered good because they offer interesting avenues for investigation and learning:
- Developing a low-cost and efficient water purification system for rural communities.
- Investigating the potential use of CRISPR-Cas9 for gene therapy in genetic disorders.
- Studying the applications of blockchain technology in securing medical records.
- Analyzing the impact of 3D printing on customized prosthetics for amputees.
- Exploring the use of artificial intelligence in predicting and preventing forest fires.
- Investigating the effects of microplastic pollution on aquatic ecosystems.
- Analyzing the use of drones in monitoring and managing agricultural crops.
- Studying the potential of quantum computing in solving complex optimization problems.
- Investigating the development of biodegradable materials for sustainable packaging.
- Exploring the ethical implications of gene editing in humans.
Unique Research Topics for STEM Students:
Unique research topics can provide STEM students with the opportunity to explore unconventional and innovative ideas. Here are 10 unique research topics for STEM students:
- Investigating the use of bioluminescent organisms for sustainable lighting solutions.
- Studying the potential of using spider silk proteins for advanced materials in engineering.
- Exploring the application of quantum entanglement for secure communication in the field of cryptography.
- Analyzing the feasibility of harnessing geothermal energy from underwater volcanoes.
- Investigating the use of CRISPR-Cas12 for rapid and cost-effective disease diagnostics.
- Studying the interaction between artificial intelligence and human creativity in art and music generation.
- Exploring the development of edible packaging materials to reduce plastic waste.
- Investigating the impact of microgravity on cellular behavior and tissue regeneration in space.
- Analyzing the potential of using sound waves to detect and combat invasive species in aquatic ecosystems.
- Studying the use of biotechnology in reviving extinct species, such as the woolly mammoth.
Experimental Research Topics for STEM Students in the Philippines
Research topics for STEM students in the Philippines can address specific regional challenges and opportunities. Here are 10 experimental research topics for STEM students in the Philippines:
- Assessing the effectiveness of locally sourced materials for disaster-resilient housing construction in typhoon-prone areas.
- Investigating the utilization of indigenous plants for natural remedies in Filipino traditional medicine.
- Studying the impact of volcanic soil on crop growth and agriculture in volcanic regions of the Philippines.
- Analyzing the water quality and purification methods in remote island communities.
- Exploring the feasibility of using bamboo as a sustainable construction material in the Philippines.
- Investigating the potential of using solar stills for freshwater production in water-scarce regions.
- Studying the effects of climate change on the migration patterns of bird species in the Philippines.
- Analyzing the growth and sustainability of coral reefs in marine protected areas.
- Investigating the utilization of coconut waste for biofuel production.
- Studying the biodiversity and conservation efforts in the Tubbataha Reefs Natural Park.
Capstone Research Topics for STEM Students in the Philippines:
Capstone research projects are often more comprehensive and can address real-world issues. Here are 10 capstone research topics for STEM students in the Philippines:
- Designing a low-cost and sustainable sanitation system for informal settlements in urban Manila.
- Developing a mobile app for monitoring and reporting natural disasters in the Philippines.
- Assessing the impact of climate change on the availability and quality of drinking water in Philippine cities.
- Designing an efficient traffic management system to address congestion in major Filipino cities.
- Analyzing the health implications of air pollution in densely populated urban areas of the Philippines.
- Developing a renewable energy microgrid for off-grid communities in the archipelago.
- Assessing the feasibility of using unmanned aerial vehicles (drones) for agricultural monitoring in rural Philippines.
- Designing a low-cost and sustainable aquaponics system for urban agriculture.
- Investigating the potential of vertical farming to address food security in densely populated urban areas.
- Developing a disaster-resilient housing prototype suitable for typhoon-prone regions.
Experimental Quantitative Research Topics for STEM Students:
Experimental quantitative research involves the collection and analysis of numerical data to conclude. Here are 10 Experimental Quantitative Research Topics For STEM Students interested in experimental quantitative research:
- Examining the impact of different fertilizers on crop yield in agriculture.
- Investigating the relationship between exercise and heart rate among different age groups.
- Analyzing the effect of varying light intensities on photosynthesis in plants.
- Studying the efficiency of various insulation materials in reducing building heat loss.
- Investigating the relationship between pH levels and the rate of corrosion in metals.
- Analyzing the impact of different concentrations of pollutants on aquatic ecosystems.
- Examining the effectiveness of different antibiotics on bacterial growth.
- Trying to figure out how temperature affects how thick liquids are.
- Finding out if there is a link between the amount of pollution in the air and lung illnesses in cities.
- Analyzing the efficiency of solar panels in converting sunlight into electricity under varying conditions.
Descriptive Research Topics for STEM Students
Descriptive research aims to provide a detailed account or description of a phenomenon. Here are 10 topics for STEM students interested in descriptive research:
- Describing the physical characteristics and behavior of a newly discovered species of marine life.
- Documenting the geological features and formations of a particular region.
- Creating a detailed inventory of plant species in a specific ecosystem.
- Describing the properties and behavior of a new synthetic polymer.
- Documenting the daily weather patterns and climate trends in a particular area.
- Providing a comprehensive analysis of the energy consumption patterns in a city.
- Describing the structural components and functions of a newly developed medical device.
- Documenting the characteristics and usage of traditional construction materials in a region.
- Providing a detailed account of the microbiome in a specific environmental niche.
- Describing the life cycle and behavior of a rare insect species.
Research Topics for STEM Students in the Pandemic:
The COVID-19 pandemic has raised many research opportunities for STEM students. Here are 10 research topics related to pandemics:
- Analyzing the effectiveness of various personal protective equipment (PPE) in preventing the spread of respiratory viruses.
- Studying the impact of lockdown measures on air quality and pollution levels in urban areas.
- Investigating the psychological effects of quarantine and social isolation on mental health.
- Analyzing the genomic variation of the SARS-CoV-2 virus and its implications for vaccine development.
- Studying the efficacy of different disinfection methods on various surfaces.
- Investigating the role of contact tracing apps in tracking & controlling the spread of infectious diseases.
- Analyzing the economic impact of the pandemic on different industries and sectors.
- Studying the effectiveness of remote learning in STEM education during lockdowns.
- Investigating the social disparities in healthcare access during a pandemic.
- Analyzing the ethical considerations surrounding vaccine distribution and prioritization.
Research Topics for STEM Students Middle School
Research topics for middle school STEM students should be engaging and suitable for their age group. Here are 10 research topics:
- Investigating the growth patterns of different types of mold on various food items.
- Studying the negative effects of music on plant growth and development.
- Analyzing the relationship between the shape of a paper airplane and its flight distance.
- Investigating the properties of different materials in making effective insulators for hot and cold beverages.
- Studying the effect of salt on the buoyancy of different objects in water.
- Analyzing the behavior of magnets when exposed to different temperatures.
- Investigating the factors that affect the rate of ice melting in different environments.
- Studying the impact of color on the absorption of heat by various surfaces.
- Analyzing the growth of crystals in different types of solutions.
- Investigating the effectiveness of different natural repellents against common pests like mosquitoes.
Technology Research Topics for STEM Students
Technology is at the forefront of STEM fields. Here are 10 research topics for STEM students interested in technology:
- Developing and optimizing algorithms for autonomous drone navigation in complex environments.
- Exploring the use of blockchain technology for enhancing the security and transparency of supply chains.
- Investigating the applications of virtual reality (VR) and augmented reality (AR) in medical training and surgery simulations.
- Studying the potential of 3D printing for creating personalized prosthetics and orthopedic implants.
- Analyzing the ethical and privacy implications of facial recognition technology in public spaces.
- Investigating the development of quantum computing algorithms for solving complex optimization problems.
- Explaining the use of machine learning and AI in predicting and mitigating the impact of natural disasters.
- Studying the advancement of brain-computer interfaces for assisting individuals with
- disabilities.
- Analyzing the role of wearable technology in monitoring and improving personal health and wellness.
- Investigating the use of robotics in disaster response and search and rescue operations.
Scientific Research Topics for STEM Students
Scientific research encompasses a wide range of topics. Here are 10 research topics for STEM students focusing on scientific exploration:
- Investigating the behavior of subatomic particles in high-energy particle accelerators.
- Studying the ecological impact of invasive species on native ecosystems.
- Analyzing the genetics of antibiotic resistance in bacteria and its implications for healthcare.
- Exploring the physics of gravitational waves and their detection through advanced interferometry.
- Investigating the neurobiology of memory formation and retention in the human brain.
- Studying the biodiversity and adaptation of extremophiles in harsh environments.
- Analyzing the chemistry of deep-sea hydrothermal vents and their potential for life beyond Earth.
- Exploring the properties of superconductors and their applications in technology.
- Investigating the mechanisms of stem cell differentiation for regenerative medicine.
- Studying the dynamics of climate change and its impact on global ecosystems.
Interesting Research Topics for STEM Students:
Engaging and intriguing research topics can foster a passion for STEM. Here are 10 interesting research topics for STEM students:
- Exploring the science behind the formation of auroras and their cultural significance.
- Investigating the mysteries of dark matter and dark energy in the universe.
- Studying the psychology of decision-making in high-pressure situations, such as sports or
- emergencies.
- Analyzing the impact of social media on interpersonal relationships and mental health.
- Exploring the potential for using genetic modification to create disease-resistant crops.
- Investigating the cognitive processes involved in solving complex puzzles and riddles.
- Studying the history and evolution of cryptography and encryption methods.
- Analyzing the physics of time travel and its theoretical possibilities.
- Exploring the role of Artificial Intelligence in creating art and music.
- Investigating the science of happiness and well-being, including factors contributing to life satisfaction.
Practical Research Topics for STEM Students
Practical research often leads to real-world solutions. Here are 10 practical research topics for STEM students:
- Developing an affordable and sustainable water purification system for rural communities.
- Designing a low-cost, energy-efficient home heating and cooling system.
- Investigating strategies for reducing food waste in the supply chain and households.
- Studying the effectiveness of eco-friendly pest control methods in agriculture.
- Analyzing the impact of renewable energy integration on the stability of power grids.
- Developing a smartphone app for early detection of common medical conditions.
- Investigating the feasibility of vertical farming for urban food production.
- Designing a system for recycling and upcycling electronic waste.
- Studying the environmental benefits of green roofs and their potential for urban heat island mitigation.
- Analyzing the efficiency of alternative transportation methods in reducing carbon emissions.
Experimental Research Topics for STEM Students About Plants
Plants offer a rich field for experimental research. Here are 10 experimental research topics about plants for STEM students:
- Investigating the effect of different light wavelengths on plant growth and photosynthesis.
- Studying the impact of various fertilizers and nutrient solutions on crop yield.
- Analyzing the response of plants to different types and concentrations of plant hormones.
- Investigating the role of mycorrhizal in enhancing nutrient uptake in plants.
- Studying the effects of drought stress and water scarcity on plant physiology and adaptation mechanisms.
- Analyzing the influence of soil pH on plant nutrient availability and growth.
- Investigating the chemical signaling and defense mechanisms of plants against herbivores.
- Studying the impact of environmental pollutants on plant health and genetic diversity.
- Analyzing the role of plant secondary metabolites in pharmaceutical and agricultural applications.
- Investigating the interactions between plants and beneficial microorganisms in the rhizosphere.
Qualitative Research Topics for STEM Students in the Philippines
Qualitative research in the Philippines can address local issues and cultural contexts. Here are 10 qualitative research topics for STEM students in the Philippines:
- Exploring indigenous knowledge and practices in sustainable agriculture in Filipino communities.
- Studying the perceptions and experiences of Filipino fishermen in coping with climate change impacts.
- Analyzing the cultural significance and traditional uses of medicinal plants in indigenous Filipino communities.
- Investigating the barriers and facilitators of STEM education access in remote Philippine islands.
- Exploring the role of traditional Filipino architecture in natural disaster resilience.
- Studying the impact of indigenous farming methods on soil conservation and fertility.
- Analyzing the cultural and environmental significance of mangroves in coastal Filipino regions.
- Investigating the knowledge and practices of Filipino healers in treating common ailments.
- Exploring the cultural heritage and conservation efforts of the Ifugao rice terraces.
- Studying the perceptions and practices of Filipino communities in preserving marine biodiversity.
Science Research Topics for STEM Students
Science offers a diverse range of research avenues. Here are 10 science research topics for STEM students:
- Investigating the potential of gene editing techniques like CRISPR-Cas9 in curing genetic diseases.
- Studying the ecological impacts of species reintroduction programs on local ecosystems.
- Analyzing the effects of microplastic pollution on aquatic food webs and ecosystems.
- Investigating the link between air pollution and respiratory health in urban populations.
- Studying the role of epigenetics in the inheritance of acquired traits in organisms.
- Analyzing the physiology and adaptations of extremophiles in extreme environments on Earth.
- Investigating the genetics of longevity and factors influencing human lifespan.
- Studying the behavioral ecology and communication strategies of social insects.
- Analyzing the effects of deforestation on global climate patterns and biodiversity loss.
- Investigating the potential of synthetic biology in creating bioengineered organisms for beneficial applications.
Correlational Research Topics for STEM Students
Correlational research focuses on relationships between variables. Here are 10 correlational research topics for STEM students:
- Analyzing the correlation between dietary habits and the incidence of chronic diseases.
- Studying the relationship between exercise frequency and mental health outcomes.
- Investigating the correlation between socioeconomic status and access to quality healthcare.
- Analyzing the link between social media usage and self-esteem in adolescents.
- Studying the correlation between academic performance and sleep duration among students.
- Investigating the relationship between environmental factors and the prevalence of allergies.
- Analyzing the correlation between technology use and attention span in children.
- Studying how environmental factors are related to the frequency of allergies.
- Investigating the link between parental involvement in education and student achievement.
- Analyzing the correlation between temperature fluctuations and wildlife migration patterns.
Quantitative Research Topics for STEM Students in the Philippines
Quantitative research in the Philippines can address specific regional issues. Here are 10 quantitative research topics for STEM students in the Philippines
- Analyzing the impact of typhoons on coastal erosion rates in the Philippines.
- Studying the quantitative effects of land use change on watershed hydrology in Filipino regions.
- Investigating the quantitative relationship between deforestation and habitat loss for endangered species.
- Analyzing the quantitative patterns of marine biodiversity in Philippine coral reef ecosystems.
- Studying the quantitative assessment of water quality in major Philippine rivers and lakes.
- Investigating the quantitative analysis of renewable energy potential in specific Philippine provinces.
- Analyzing the quantitative impacts of agricultural practices on soil health and fertility.
- Studying the quantitative effectiveness of mangrove restoration in coastal protection in the Philippines.
- Investigating the quantitative evaluation of indigenous agricultural practices for sustainability.
- Analyzing the quantitative patterns of air pollution and its health impacts in urban Filipino areas.
Things That Must Keep In Mind While Writing Quantitative Research Title
Here are few things that must be keep in mind while writing quantitative research tile:
1. Be Clear and Precise
Make sure your research title is clear and says exactly what your study is about. People should easily understand the topic and goals of your research by reading the title.
2. Use Important Words
Include words that are crucial to your research, like the main subjects, who you’re studying, and how you’re doing your research. This helps others find your work and understand what it’s about.
3. Avoid Confusing Words
Stay away from words that might confuse people. Your title should be easy to grasp, even if someone isn’t an expert in your field.
4. Show Your Research Approach
Tell readers what kind of research you did, like experiments or surveys. This gives them a hint about how you conducted your study.
5. Match Your Title with Your Research Questions
Make sure your title matches the questions you’re trying to answer in your research. It should give a sneak peek into what your study is all about and keep you on the right track as you work on it.
STEM students, addressing what STEM is and why research matters in this field. It offered an extensive list of research topics , including experimental, qualitative, and regional options, catering to various academic levels and interests. Whether you’re a middle school student or pursuing advanced studies, these topics offer a wealth of ideas. The key takeaway is to choose a topic that resonates with your passion and aligns with your goals, ensuring a successful journey in STEM research. Choose the best Experimental Quantitative Research Topics For Stem Students today!
Related Posts
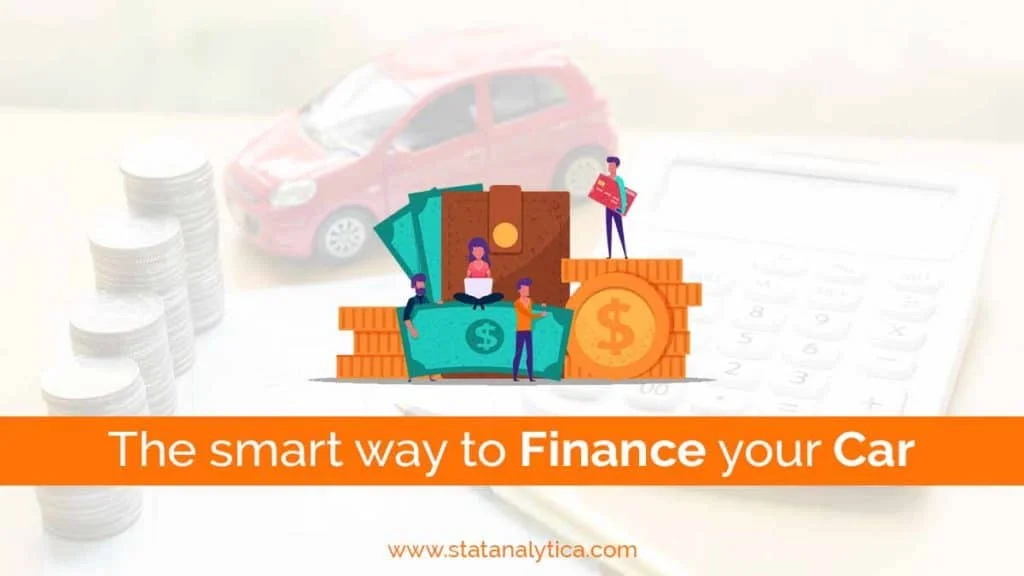
Step by Step Guide on The Best Way to Finance Car
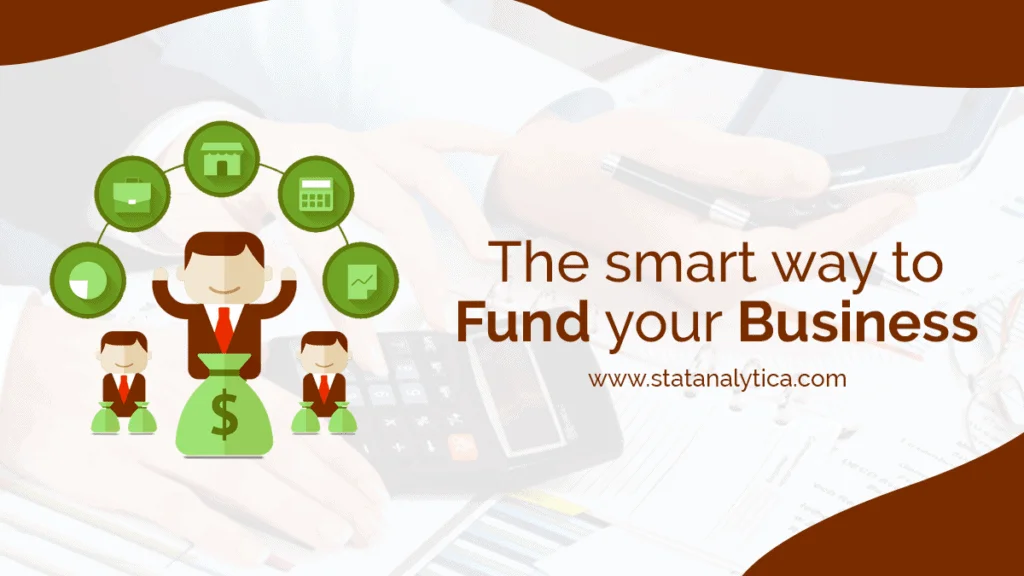
The Best Way on How to Get Fund For Business to Grow it Efficiently
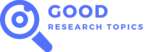
190+ Best Quantitative Research Topics for STEM Students 2024
Dive into a world of quantitative research topics for STEM students! It’s all about unveiling the secrets of biology, decoding the language of particles, and taking a data-driven ride into the unknown.
Ready for a deep dive into the quantitative wonders of Science, Technology, Engineering, and Math? Our “Quantitative Research Topics for STEM Students” lineup is like a playground for your curious minds.
Imagine it as a buffet of cool ideas waiting for your unique spin. Whether you love crunching numbers to reveal data mysteries or untangling relationships between different things, these topics are your VIP pass to the science party!
So, grab a seat, gear up that brainpower, and let’s turn STEM research into an adventure. Picture these ideas as your scientific rollercoaster – twists, turns, and maybe even a couple of “aha!” moments. Let the quantitative fun kick-off!
Table of Contents
The Importance of Quantitative Research in STEM
Check out the importance of quantitative research in STEM.
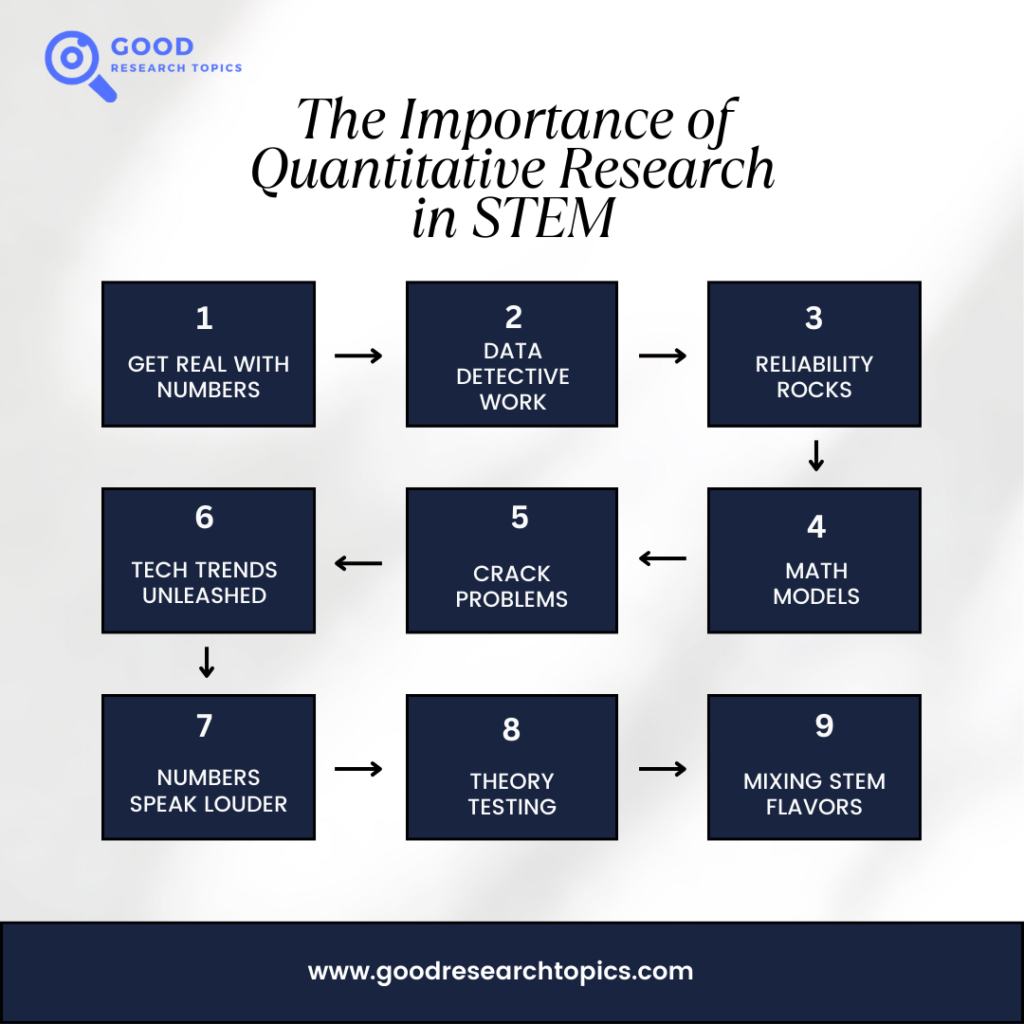
1. Get Real with Numbers
Quantitative research in STEM isn’t just about jargon and equations; it’s the cool way of saying, “Let’s measure things objectively!” It gives us the numbers to express complex stuff and makes experiments the rockstars of replicability.
2. Data Detective Work
Ever wanted to be a detective? Well, quantitative research in STEM lets us play detective with data. We dig into big datasets, spot trends, and unveil the secrets that numbers hide. It’s like solving a mystery, but with graphs and charts!
3. Reliability Rocks
Picture this: your research is like a superhero, reliable and always ready for action. Thanks to quantitative methods, experiments can be repeated with superhero-like consistency, making our findings more trustworthy than ever.
4. Math Models – The Superheroes of STEM
Move over, superheroes; mathematical models are here! Quantitative research helps us create these powerful models that predict and explain all the cool and crazy things happening in the natural world. It’s like having a mathematical superhero team!
5. Crack Problems with Quantitative Kung Fu
Forget about traditional problem-solving. In STEM, we use quantitative kung fu! We quantify variables, analyze relationships, and kick problems to the curb with efficient and powerful moves. It’s basically the Bruce Lee of research!
6. Tech Trends Unleashed
Want to be at the forefront of technology? Quantitative research is the ticket. It doesn’t just guide us in designing cool tech; it helps us optimize what we already have. It’s the GPS for navigating the fast-paced world of STEM innovation.
7. Numbers Speak Louder in Policy Land
Policymakers love a good story, especially if it’s told in numbers. Quantitative research speaks their language, helping shape policies based on hard evidence. It’s like turning data into a blockbuster movie with a powerful impact!
8. Theory Testing, Like a Boss
In STEM, we don’t just talk theories; we test them like bosses. Quantitative research brings theories down to earth, making sure they’re not just cool ideas but proven, tested, and ready to roll.
9. Mixing STEM Flavors
STEM isn’t a solo act; it’s a band. Quantitative research lets us mix and match data from different instruments, creating a symphony of insights. It’s like blending different STEM flavors to cook up something amazing!
Choosing the Right Quantitative Research Topic
Choosing the perfect quantitative research topic is like embarking on a thrilling adventure – it’s all about excitement, challenges, and finding something that truly lights up your STEM-loving heart. So, let’s dive into the wild ride of “Choosing the Right Quantitative Research Topic.”
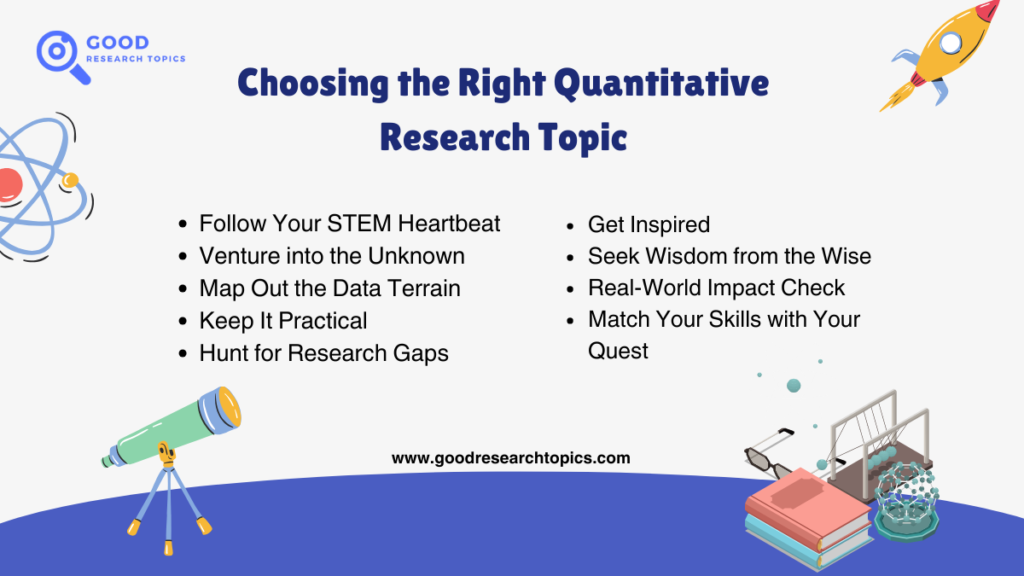
1. Follow Your STEM Heartbeat
First things first, what makes your STEM-loving heart race? Is it the allure of cracking genetic codes or navigating the intricate world of algorithms? Choose a topic that makes you go, “Wow, I want to know more!”
2. Venture into the Unknown
Don’t fear the unknown; embrace it! The most fascinating questions often lurk in uncharted territories. Think of your research topic as a treasure waiting to be discovered in the vast landscape of STEM.
3. Map Out the Data Terrain
A good adventure needs a map, right? Similarly, ensure there’s enough data to guide you. Having solid and accessible data turns your research journey into a well-prepared expedition.
4. Keep It Practical
Consider the practical side. Can you realistically embark on experiments, gather data, or dive into analyses within your available resources and timeframe? Let’s keep this adventure doable!
5. Hunt for Research Gaps
Explore the landscape of existing research. Are there areas where quantitative exploration is scarce? Becoming a gap-filler not only makes you a research superhero but also adds a unique twist to your journey.
6. Get Inspired
Think of reading research papers and attending seminars as your STEM version of gathering allies for your quest. Surround yourself with inspiration – it’s like finding magical artifacts for your research toolkit.
7. Seek Wisdom from the Wise
Wise mentors, professors, or seasoned experts are like the Gandalfs of your STEM journey. Seek their counsel. They’ve been through quests and can guide you with their sage advice.
8. Real-World Impact Check
Consider the real-world impact of your research. How can your findings make a dent in solving problems or pushing the boundaries of knowledge in your STEM realm? It’s like giving your research a superhero cape!
9. Match Your Skills with Your Quest
Choose a topic that aligns with your skills and strengths. Think of it as selecting a character for a video game – you want one that matches your style and abilities for a victorious and enjoyable quest.
Remember, your quantitative research topic isn’t just a research project – it’s your personal STEM expedition, waiting for your unique exploration and discovery. Let the adventure begin!
Quantitative Research Topics for STEM Students
Check out quantitative research topics in physics.
Biology Research Topics
Chemistry research topics , physics research topics, mathematics research topics, computer science research topics, engineering research topics, environmental science research topics, biomedical engineering research topics, aerospace engineering research topics, mechanical engineering research topics, list of 125+ quantitative research topics for stem students.
Alright, let’s wrap this up in a more laid-back way. So, quantitative research for STEM students? It’s basically like handing them the keys to a scientific playground. These topics aren’t just a snooze-fest of numbers and graphs; think of it as a superhero origin story, but for budding scientists.
Picture this: students diving into data like it’s a treasure map, figuring out the secrets of biology or decoding the funky dance moves of particles. It’s not just studying; it’s like getting a backstage pass to the cool, mysterious world of science.
Why bother? Because this isn’t just about acing exams. It’s about becoming the superhero of your scientific turf. You’re not just learning stuff; you’re becoming a detective—asking the big questions, collecting clues, and maybe stumbling upon some mind-blowing discoveries.
So, cheers to the quantitative research journey! It’s not just a college thing; it’s the ticket to becoming the cool, problem-solving scientist everyone wants on their team. Ready for the adventure? Let’s dive in!
Frequently Asked Questions (FAQs)
Are there specific resources for stem students engaging in quantitative research.
Yes, there are specialized software tools, academic journals, and online platforms dedicated to quantitative research in STEM. Explore these resources for comprehensive support.
How can I overcome common pitfalls in quantitative research?
Mitigating pitfalls involves thorough planning, robust methodology, and staying aware of potential biases. Learning from the experiences of others can also be invaluable.
Related Posts
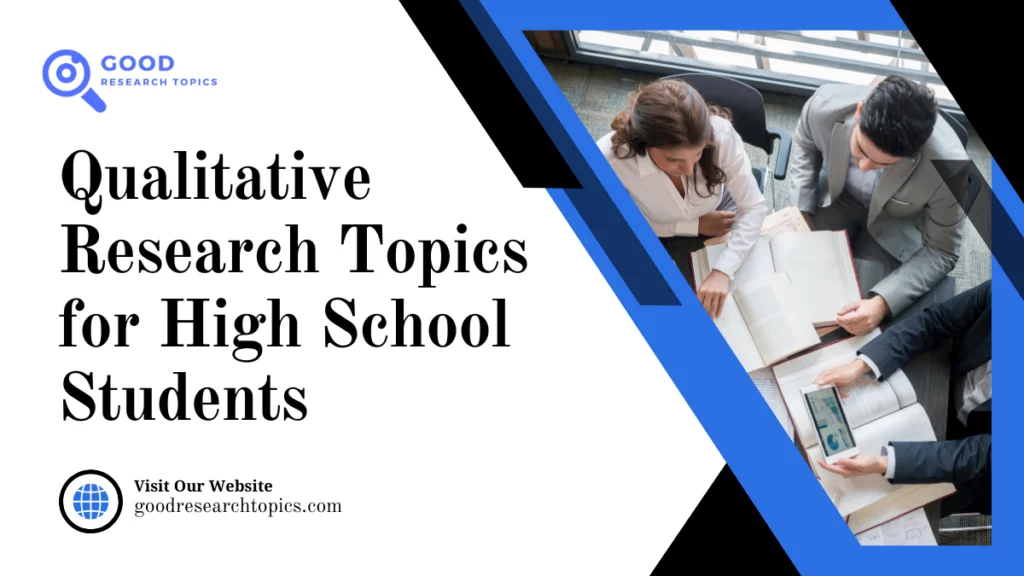
100+ Most Qualitative Research Topics For High School Students In 2024
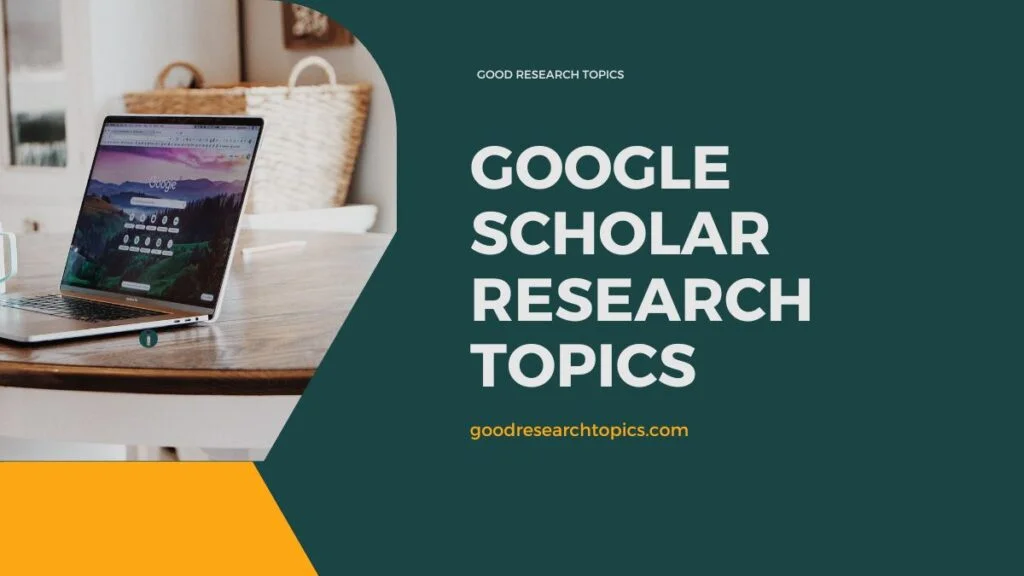
99+ Astonishing Google Scholar Research Topics: The Road Less Traveled
Leave a comment cancel reply.
Your email address will not be published. Required fields are marked *
Save my name, email, and website in this browser for the next time I comment.

Top 127+ Great Quantitative Research Topics For STEM Students
Are you a STEM enthusiast eager to dive into quantitative research but uncertain about the best topics to explore? Look no further! In this comprehensive guide, we’ll navigate through the top 27+ Quantitative Research Topics for STEM Students.
There are we give the best topics for future scientists, engineers, and math whizzes! Are you curious about diving into the fantastic world of quantitative research? Well, you’re in for an exciting way! Today, we’re going to explore some super cool Quantitative Research Topics for STEM Students like you. But first, what’s all this talk about “quantitative research”? Don’t worry; it’s not as tricky as it sounds!
Quantitative research simply means using numbers and data to study things. For example, solving a math problem or conducting a science experiment where you count, measure, or analyze stuff to learn more. Cool, right? Now, let’s talk about STEM. No, not the plant stem, but STEM subjects – Science, Technology, Engineering, and Mathematics. These subjects are like the crucial part of knowledge!
So, here’s the exciting part! We’ve got a bunch of fascinating topics lined up for you to explore in these STEM fields using numbers, stats, and math. From studying how robots help doctors predict climate change to finding ways to make renewable energy work better in cities, these topics will make your brain more creative!
Also Like To Know: Sk Project Ideas
Table of Contents
What Is Experimental Quantitative Research Topics For STEM Students
Experimental quantitative research topics for STEM students involve conducting investigations using numbers and measurements to find answers to questions related to science, technology, engineering, and mathematics. These topics help students gather data through controlled experiments and use mathematical analysis to understand how things work or solve problems in subjects like biology, physics, chemistry, or mathematics. For example, they might explore topics like testing how different temperatures affect plant growth or analyzing the relationship between force and motion using simple experiments and numbers.
How Do You Identify A Quantitative Research Title?
Here are 7 easy steps to identify a quantitative research title:
- Define Your Research Area: Start by identifying the general subject or field you want to study. For instance, it could be related to science, education, psychology, etc.
- Focus on a Specific Topic: Narrow down your field of interest to a specific area or problem. For example, if you’re interested in psychology, you might want to focus on the effects of social media on teenagers’ mental health.
- Identify Variables: Determine the variables or factors you want to measure or investigate. In quantitative research, these are typically measurable quantities or numerical data.
- Formulate a Research Question: Develop a clear and concise research question that reflects what you want to study. Ensure it is specific and can be addressed using quantitative methods.
- Consider the Population or Sample: Determine the population you want to study or the sample you’ll collect data from. This helps in shaping the scope of your research.
- Quantifiable Outcome: Ensure that the research title suggests an outcome that can be measured numerically. Quantitative research aims to gather numerical data and analyze it statistically.
- Review and Refine: After formulating a tentative title, review it to ensure it aligns with the research objectives, is clear and concise, and accurately reflects the focus of your study. Make any necessary refinements to improve clarity and precision.
List of Best 127+ Great Quantitative Research Topics For STEM Students
Here are the 127+ Great Quantitative Research Topics For STEM Students:
Best Mathematics Quantitative Research Topics For STEM Students
- Applications of Machine Learning in Mathematical Problem Solving
- Chaos Theory and Its Applications in Nonlinear Systems
- Algorithmic Trading Strategies and Mathematical Modeling
- Data Compression Techniques: Efficiency and Accuracy Trade-offs
- Exploring Applications of Topological Data Analysis
- Analyzing Random Matrix Theory in Statistical Physics
- Mathematical Models for Climate Change Predictions
- Analyzing Cryptocurrency Trends Using Mathematical Models
- Investigating Mathematical Models for Social Networks
- Studying Mathematical Foundations of Quantum Computing
Easy Quantitative Research Topics For STEM Students In Physics
- Quantum Entanglement and Its Applications in Communication
- Plasma Physics: Understanding Fusion Reactors
- Superconductivity and Its Practical Applications
- Statistical Mechanics in Complex Systems
- Applications of String Theory in Cosmology
- Gravitational Wave Detection and Interpretation
- Quantum Field Theory and Particle Interactions
- Quantum Computing: Designing Error-Correcting Codes
- Analyzing Exoplanet Data Using Astrophysical Models
- Studying Black Hole Physics and Information Paradox
- Computational Chemistry for Drug Design and Discovery
- Quantum Chemistry: Exploring Molecular Properties
- Applications of Nanomaterials in Renewable Energy
- Analyzing Chemical Reaction Kinetics
- Environmental Impact Assessment of Chemical Pollutants
- Polymer Chemistry: Designing Advanced Materials
- Studying Catalysis and Surface Chemistry
- Exploring Electrochemical Energy Storage Systems
- Bioinorganic Chemistry: Metalloprotein Modeling
- Investigating Supramolecular Chemistry for Functional Materials
Biology Quantitative Research Topics For STEM Students
- Systems Biology: Modeling Cellular Signaling Networks
- Computational Neuroscience: Brain Network Analysis
- Population Genetics and Evolutionary Dynamics
- Mathematical Modeling of Infectious Diseases
- Studying Protein Folding Using Computational Methods
- Ecological Niche Modeling for Biodiversity Conservation
- Quantitative Analysis of Gene Regulatory Networks
- Metagenomics: Analyzing Microbial Communities
- Bioinformatics Applications in Personalized Medicine
- Integrative Biology: Understanding Multi-Omics Data
Engineering
- Robotics and Autonomous Systems: Motion Planning Algorithms
- Finite Element Analysis for Structural Engineering
- Machine Learning in Image Processing and Computer Vision
- Control Systems Engineering in Autonomous Vehicles
- Renewable Energy Grid Integration and Optimization
- Optimization of Transportation Networks
- Analyzing Fluid Dynamics in Aerospace Engineering
- Materials Science: Quantum Mechanics in Materials Design
- Sustainable Infrastructure Planning and Design
- Cyber-Physical Systems: Security and Resilience
Computer Science Quantitative Research Topics For STEM Students
- Big Data Analytics: Scalable Algorithms for Data Processing
- Natural Language Processing for Sentiment Analysis
- Blockchain Technology: Security and Consensus Algorithms
- Quantum Computing Algorithms and Complexity
- Developing Explainable AI Models for Decision Support
- Privacy-Preserving Techniques in Data Mining
- Network Security: Intrusion Detection Systems
- Cloud Computing: Resource Allocation and Optimization
- Human-Robot Interaction and Collaboration
- Advancements in Quantum Cryptography Protocols
Earth and Environmental Sciences
- Climate Modeling: Predicting Regional Climate Changes
- Geographical Information Systems (GIS) in Environmental Analysis
- Hydrology and Water Resource Management Modeling
- Remote Sensing: Image Analysis for Environmental Monitoring
- Seismology: Studying Earthquake Patterns Using Data Analysis
- Oceanography: Analyzing Ocean Currents and Climate Impacts
- Environmental Impact Assessment of Renewable Energy Projects
- Soil Science: Quantifying Soil Erosion and Nutrient Dynamics
- Air Quality Modeling and Pollution Analysis
- Ecosystem Services Valuation Using Quantitative Methods
Agriculture and Food Sciences
- Precision Agriculture: Using Data Analytics for Crop Management
- Genetics and Genomics in Crop Improvement Strategies
- Quantitative Analysis of Food Supply Chains
- Agricultural Policy Analysis and Economic Modeling
- Nutritional Analysis Using Quantitative Methods
- Modeling Pesticide Use and Environmental Impact
- Aquaculture: Optimization of Fish Farming Practices
- Soil Fertility Modeling and Nutrient Management
- Food Safety Assessment Using Quantitative Techniques
- Sustainable Agriculture: Systems Modeling and Optimization
Health Sciences and Medicine: quantitative research topics in nursing
- Epidemiology: Modeling Disease Transmission Dynamics
- Healthcare Analytics: Predictive Modeling for Patient Outcomes
- Pharmacokinetics and Drug Dosage Optimization
- Health Informatics: Quantitative Analysis of Electronic Health Records
- Medical Imaging Analysis Using Quantitative Techniques
- Health Economics: Cost-Benefit Analysis of Healthcare Policies
- Genomic Medicine: Analyzing Genetic Data for Disease Risk Prediction
- Public Health Policy Evaluation Using Quantitative Methods
- Biostatistics: Designing Clinical Trials and Statistical Analysis
- Computational Anatomy for Disease Diagnosis and Treatment
Psychology and Social Sciences
- Quantitative Analysis of Social Network Dynamics
- Behavioral Economics: Decision-Making Models
- Psychometrics: Measurement Models in Psychological Testing
- Quantitative Study of Human Cognition and Memory
- Social Media Analytics: Sentiment Analysis and Trends
- Sociology: Modeling Social Movements and Cultural Dynamics
- Educational Data Mining and Learning Analytics
- Quantitative Research in Political Science and Policy Analysis
- Consumer Behavior Analysis Using Quantitative Methods
- Quantitative Approaches to Studying Emotion and Personality
Astronomy and Astrophysics
- Cosmic Microwave Background Radiation: Analyzing Anisotropies
- Time-domain Astronomy: Statistical Analysis of Variable Stars
- Gravitational Lensing: Quantifying Distortions in Cosmic Images
- Stellar Evolution Modeling and Simulations
- Exoplanet Atmosphere Characterization Using Quantitative Methods
- Galaxy Formation and Evolution: Statistical Approaches
- Multimessenger Astronomy: Data Fusion Techniques
- Dark Matter and Dark Energy: Analyzing Cosmological Models
- Astrophysical Jets: Modeling High-Energy Particle Emissions
- Supernova Studies: Quantitative Analysis of Stellar Explosions
Linguistics and Language Sciences
- Computational Linguistics: Natural Language Generation Models
- Phonetics and Speech Analysis Using Quantitative Techniques
- Sociolinguistics: Statistical Analysis of Dialect Variation
- Syntax and Grammar Modeling in Linguistic Theory
- Quantitative Study of Language Acquisition in Children
- Corpus Linguistics: Quantifying Textual Data
- Language Typology and Universals: Cross-Linguistic Analysis
- Psycholinguistics: Quantitative Study of Language Processing
- Machine Translation: Improving Accuracy and Efficiency
- Quantitative Approaches to Historical Linguistics
Business and Economics: quantitative research topics in education
- Financial Risk Management: Quantitative Modeling of Risks
- Econometrics: Statistical Methods in Economic Analysis
- Marketing Analytics: Consumer Behavior Modeling
- Quantitative Analysis of Macroeconomic Policies
- Operations Research: Optimization in Supply Chain Management
- Quantitative Methods in Corporate Finance
- Labor Economics: Analyzing Employment Trends Using Data
- Economic Impact Assessment of Policy Interventions
- Quantitative Analysis of Market Dynamics and Competition
- Behavioral Finance: Quantifying Psychological Aspects in Financial Decision-Making
Education and Pedagogy
- Educational Data Mining for Adaptive Learning Systems
- Quantitative Analysis of Learning Outcomes and Student Performance
- Technology Integration in Education: Assessing Efficacy
- Assessment and Evaluation Models in Educational Research
- Quantitative Study of Teacher Effectiveness and Practices
- Cognitive Load Theory: Quantifying Learning Processes
- Educational Psychology: Quantitative Analysis of Motivation
- Online Education: Analytics for Engagement and Success
- Curriculum Development: Quantitative Approaches to Design
- Educational Policy Analysis Using Quantitative Methods
Communication and Media Studies
- Media Effects Research: Quantitative Analysis of Influence
- Computational Journalism: Data-driven Storytelling
- Social Media Influence Metrics and Analysis
- Quantitative Study of Public Opinion and Opinion Formation
- Media Content Analysis Using Statistical Methods
- Communication Network Analysis: Quantifying Connections
- Quantitative Approaches to Media Bias Assessment
- Entertainment Analytics: Audience Behavior Modeling
- Digital Media Consumption Patterns: Statistical Analysis
- Crisis Communication: Quantitative Assessment of Responses
quantitative research topics for accounting students in the Philippines
Here are ten quantitative research topics suitable for accounting students in the Philippines:
- “Impact of Tax Reforms on Small and Medium Enterprises (SMEs) in the Philippines: A Quantitative Analysis”
- “Financial Performance Evaluation of Philippine Banks: A Comparative Study Using Ratios and Metrics”
- “Effectiveness of Internal Control Systems in Mitigating Fraud: A Quantitative Assessment in Philippine Organizations”
- “Analysis of Corporate Governance Practices and Financial Performance: Evidence from Philippine Listed Companies”
- “Determinants of Audit Quality: A Quantitative Study of Auditing Practices in the Philippines”
- “The Role of Accounting Information Systems in Enhancing Organizational Efficiency: A Quantitative Investigation”
- “Factors Influencing Financial Reporting Quality in the Philippines: A Quantitative Approach”
- “Assessing the Impact of International Financial Reporting Standards (IFRS) Adoption on Philippine Firms”
- “Analysis of Factors Affecting Financial Literacy among Filipino College Students: A Quantitative Study”
- “Cash Flow Management Practices and Financial Sustainability of SMEs in the Philippines: A Quantitative Analysis”
What are the 10 examples of research titles in school quantitative?
Here are ten examples of quantitative research titles suitable for school-related studies:
- “Impact of Technology Integration on Academic Performance: A Quantitative Analysis”
- “Effects of Classroom Size on Student Learning Outcomes: A Quantitative Study”
- “Parental Involvement and Student Achievement: A Quantitative Investigation”
- “Assessing the Efficacy of Different Teaching Methods in Mathematics Education”
- “Correlation between Student Engagement and Standardized Test Scores”
- “Bullying in Schools: Quantitative Analysis of Prevalence and Impact on Academic Performance”
- “Examining the Relationship between Socioeconomic Status and Reading Proficiency”
- “Effectiveness of School Counseling Programs on Student Mental Health: A Quantitative Approach”
- “Analyzing the Impact of Extracurricular Activities on Student Success Metrics”
- “Evaluation of a New Grading System: Quantitative Comparison with Traditional Grading Scales”
Best experimental quantitative research topics for stem students in the Philippines
The following are the best quantitative research topics for stem students:
Biology Quantitative Research Topics
In the realm of Biology, quantitative research delves into the numerical aspects of living organisms, ecosystems, and genetics, aiding in understanding diverse biological phenomena.
Chemistry Quantitative Research Topics
Chemistry’s quantitative research explores numerical relationships within chemical reactions, material properties, and various compounds, offering insights into chemical phenomena through measurable data.
Physics Quantitative Research Topics
In Physics, quantitative research scrutinizes measurable physical quantities and their interactions, exploring fundamental principles and phenomena of the natural world.
Mathematics Quantitative Research Topics
Mathematics, in its quantitative research, investigates numerical patterns, structures, and mathematical theories, exploring the quantifiable aspects of various mathematical concepts.
We’ve explored the wonders of using numbers, data, and math to unravel the mysteries of science, technology, engineering, and mathematics. Quantitative research isn’t about mind-boggling formulas or complex theories. It’s about using simple math and statistics to understand the world around us. Whether it’s predicting the impact of climate change, exploring how robots aid healthcare, or figuring out ways to make our cities greener, each topic we’ve discussed holds the potential for groundbreaking discoveries.
Now, as you continue your academic journey, keep this curiosity alive. Embrace the joy of asking questions, experimenting, and exploring. Your passion for STEM subjects can lead to amazing things – from inventing new technologies to finding solutions for global challenges.
So, what’s next for you? Pick a topic that excites you, dive into the world of quantitative research, and let your imagination soar! Who knows, maybe you’ll be the one to discover something incredible that changes the world.
Leave a Comment Cancel Reply
Your email address will not be published. Required fields are marked *
Save my name, email, and website in this browser for the next time I comment.
- Write my thesis
- Thesis writers
- Buy thesis papers
- Bachelor thesis
- Master's thesis
- Thesis editing services
- Thesis proofreading services
- Buy a thesis online
- Write my dissertation
- Dissertation proposal help
- Pay for dissertation
- Custom dissertation
- Dissertation help online
- Buy dissertation online
- Cheap dissertation
- Dissertation editing services
- Write my research paper
- Buy research paper online
- Pay for research paper
- Research paper help
- Order research paper
- Custom research paper
- Cheap research paper
- Research papers for sale
- Thesis subjects
- How It Works
55 Brilliant Research Topics For STEM Students
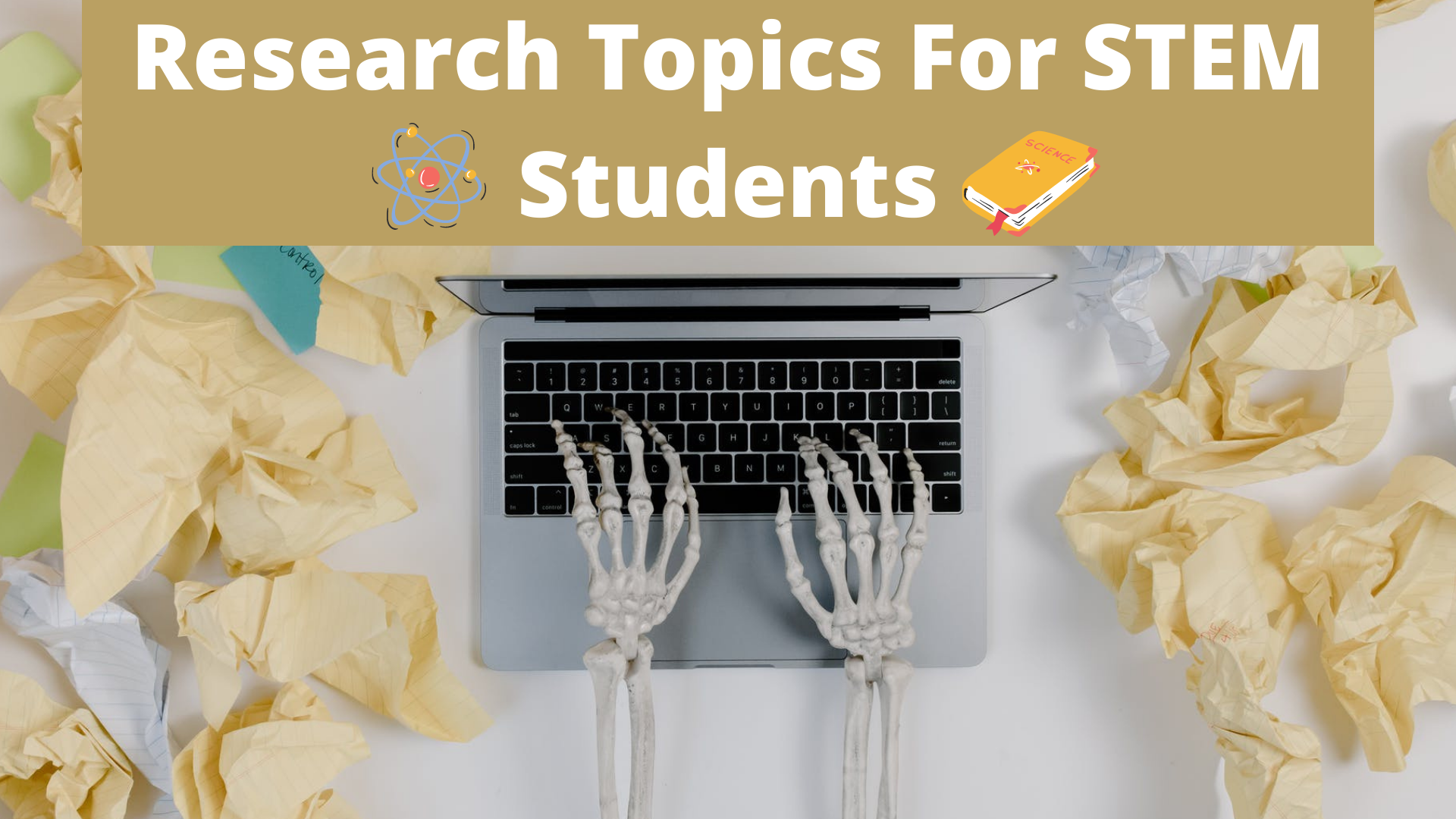
Primarily, STEM is an acronym for Science, Technology, Engineering, and Mathematics. It’s a study program that weaves all four disciplines for cross-disciplinary knowledge to solve scientific problems. STEM touches across a broad array of subjects as STEM students are required to gain mastery of four disciplines.
As a project-based discipline, STEM has different stages of learning. The program operates like other disciplines, and as such, STEM students embrace knowledge depending on their level. Since it’s a discipline centered around innovation, students undertake projects regularly. As a STEM student, your project could either be to build or write on a subject. Your first plan of action is choosing a topic if it’s written. After selecting a topic, you’ll need to determine how long a thesis statement should be .
Given that topic is essential to writing any project, this article focuses on research topics for STEM students. So, if you’re writing a STEM research paper or write my research paper , below are some of the best research topics for STEM students.
List of Research Topics For STEM Students
Quantitative research topics for stem students, qualitative research topics for stem students, what are the best experimental research topics for stem students, non-experimental research topics for stem students, capstone research topics for stem students, correlational research topics for stem students, scientific research topics for stem students, simple research topics for stem students, top 10 research topics for stem students, experimental research topics for stem students about plants, research topics for grade 11 stem students, research topics for grade 12 stem students, quantitative research topics for stem high school students, survey research topics for stem students, interesting and informative research topics for senior high school stem students.
Several research topics can be formulated in this field. They cut across STEM science, engineering, technology, and math. Here is a list of good research topics for STEM students.
- The effectiveness of online learning over physical learning
- The rise of metabolic diseases and their relationship to increased consumption
- How immunotherapy can improve prognosis in Covid-19 progression
For your quantitative research in STEM, you’ll need to learn how to cite a thesis MLA for the topic you’re choosing. Below are some of the best quantitative research topics for STEM students.
- A study of the effect of digital technology on millennials
- A futuristic study of a world ruled by robotics
- A critical evaluation of the future demand in artificial intelligence
There are several practical research topics for STEM students. However, if you’re looking for qualitative research topics for STEM students, here are topics to explore.
- An exploration into how microbial factories result in the cause shortage in raw metals
- An experimental study on the possibility of older-aged men passing genetic abnormalities to children
- A critical evaluation of how genetics could be used to help humans live healthier and longer.
Experimental research in STEM is a scientific research methodology that uses two sets of variables. They are dependent and independent variables that are studied under experimental research. Experimental research topics in STEM look into areas of science that use data to derive results.
Below are easy experimental research topics for STEM students.
- A study of nuclear fusion and fission
- An evaluation of the major drawbacks of Biotechnology in the pharmaceutical industry
- A study of single-cell organisms and how they’re capable of becoming an intermediary host for diseases causing bacteria
Unlike experimental research, non-experimental research lacks the interference of an independent variable. Non-experimental research instead measures variables as they naturally occur. Below are some non-experimental quantitative research topics for STEM students.
- Impacts of alcohol addiction on the psychological life of humans
- The popularity of depression and schizophrenia amongst the pediatric population
- The impact of breastfeeding on the child’s health and development
STEM learning and knowledge grow in stages. The older students get, the more stringent requirements are for their STEM research topic. There are several capstone topics for research for STEM students .
Below are some simple quantitative research topics for stem students.
- How population impacts energy-saving strategies
- The application of an Excel table processor capabilities for cost calculation
- A study of the essence of science as a sphere of human activity
Correlations research is research where the researcher measures two continuous variables. This is done with little or no attempt to control extraneous variables but to assess the relationship. Here are some sample research topics for STEM students to look into bearing in mind how to cite a thesis APA style for your project.
- Can pancreatic gland transplantation cure diabetes?
- A study of improved living conditions and obesity
- An evaluation of the digital currency as a valid form of payment and its impact on banking and economy
There are several science research topics for STEM students. Below are some possible quantitative research topics for STEM students.
- A study of protease inhibitor and how it operates
- A study of how men’s exercise impacts DNA traits passed to children
- A study of the future of commercial space flight
If you’re looking for a simple research topic, below are easy research topics for STEM students.
- How can the problem of Space junk be solved?
- Can meteorites change our view of the universe?
- Can private space flight companies change the future of space exploration?
For your top 10 research topics for STEM students, here are interesting topics for STEM students to consider.
- A comparative study of social media addiction and adverse depression
- The human effect of the illegal use of formalin in milk and food preservation
- An evaluation of the human impact on the biosphere and its results
- A study of how fungus affects plant growth
- A comparative study of antiviral drugs and vaccine
- A study of the ways technology has improved medicine and life science
- The effectiveness of Vitamin D among older adults for disease prevention
- What is the possibility of life on other planets?
- Effects of Hubble Space Telescope on the universe
- A study of important trends in medicinal chemistry research
Below are possible research topics for STEM students about plants:
- How do magnetic fields impact plant growth?
- Do the different colors of light impact the rate of photosynthesis?
- How can fertilizer extend plant life during a drought?
Below are some examples of quantitative research topics for STEM students in grade 11.
- A study of how plants conduct electricity
- How does water salinity affect plant growth?
- A study of soil pH levels on plants
Here are some of the best qualitative research topics for STEM students in grade 12.
- An evaluation of artificial gravity and how it impacts seed germination
- An exploration of the steps taken to develop the Covid-19 vaccine
- Personalized medicine and the wave of the future
Here are topics to consider for your STEM-related research topics for high school students.
- A study of stem cell treatment
- How can molecular biological research of rare genetic disorders help understand cancer?
- How Covid-19 affects people with digestive problems
Below are some survey topics for qualitative research for stem students.
- How does Covid-19 impact immune-compromised people?
- Soil temperature and how it affects root growth
- Burned soil and how it affects seed germination
Here are some descriptive research topics for STEM students in senior high.
- The scientific information concept and its role in conducting scientific research
- The role of mathematical statistics in scientific research
- A study of the natural resources contained in oceans
Final Words About Research Topics For STEM Students
STEM topics cover areas in various scientific fields, mathematics, engineering, and technology. While it can be tasking, reducing the task starts with choosing a favorable topic. If you require external assistance in writing your STEM research, you can seek professional help from our experts.
Leave a Reply Cancel reply

200 Best Quantitative Research Topics for STEM Students
Explore a diverse range of engaging quantitative research topics for STEM students. From unraveling mysteries in science to designing innovative technologies, discover ideas to ignite your curiosity and drive innovation
Hey, STEM enthusiasts! Ever wondered how science and technology wizards uncover secrets and create cool stuff? That’s where quantitative research swoops in! It’s like your magic wand for diving into the mysteries of science, tech, engineering, and math.
In this guide, we’ve whipped up a batch of awesome research topics tailored just for you. So, get ready to roll up your sleeves, explore, and unleash your inner genius!
Table of Contents
Quantitative Research Topics for STEM Students
Check out quantitative research topics for STEM students
- How does temperature affect plant growth?
- Exploring biodiversity in local ecosystems.
- The impact of pollution on aquatic life.
- Comparing natural and chemical pesticides.
- Studying the relationship between diet and fruit fly lifespan.
- Testing reactions of metals with acids.
- Studying pH levels of household substances.
- Investigating factors affecting rusting.
- Comparing effectiveness of cleaning agents.
- Exploring properties of different plastics.
- Investigating pendulum length and swing time.
- Studying forces affecting rolling objects.
- Exploring light reflection with mirrors.
- Testing ball speed on different surfaces.
- Efficiency of simple machines like levers.
Mathematics
- Analyzing patterns in the Fibonacci sequence.
- Studying prime number properties.
- Exploring geometric shapes and their uses.
- Investigating circle circumference and diameter.
- Analyzing data using graphs and charts.
Engineering
- Designing and testing bridge strength.
- Studying insulation materials for buildings.
- Investigating aerodynamics with different shapes.
- Designing water filtration systems.
- Analyzing renewable energy source efficiency.
Computer Science
- Creating a program for math problem solving.
- Developing a language learning app.
- Studying sorting algorithms’ effects on data.
- Designing a recycling education game.
- Investigating password encryption security.
Environmental Science
- Monitoring air quality in various locations.
- Studying deforestation’s impact on wildlife.
- Investigating recycling’s waste reduction effects.
- Analyzing water conservation method effectiveness.
- Studying climate change effects on ecosystems.
- Studying rock properties.
- Investigating erosion’s impact on landscapes.
- Analyzing soil formation.
- Studying earthquakes’ effects on infrastructure.
- Investigating mineral properties.
- Observing moon phases.
- Studying planets in our solar system.
- Recording star and constellation movements.
- Investigating light pollution effects on stargazing.
- Studying meteor shower patterns.
- Testing treatments’ effects on bacteria.
- Studying genetics’ role in disease.
- Analyzing lifestyle impacts on health.
- Testing pain relief method effectiveness.
- Investigating mental health factors.
Neuroscience
- Studying exercise effects on brain function.
- Investigating music’s impact on mood.
- Analyzing food effects on brain health.
- Studying brain waves during activities.
- Investigating sleep’s effects on memory.
- Testing therapy effects on mental health.
- Studying personality-behavior relationship.
- Analyzing stress’ impact on cognition.
- Studying parenting styles’ effect on development.
- Investigating social media’s mental health impact.
- Analyzing inflation’s purchasing power impact.
- Investigating interest rate-consumer spending relationship.
- Studying government policy-economic impact.
- Analyzing globalization-income inequality link.
- Investigating stock market-economic growth relationship.
Agriculture
- Testing different fertilizers’ effects on plants.
- Investigating soil composition’s impact on crops.
- Analyzing climate change effects on agriculture.
- Studying pest control method effectiveness.
- Investigating crop rotation benefits.
Physics Education
- Creating simple physics experiments for kids.
- Studying teaching method effectiveness in physics.
- Designing physics-based games.
- Investigating student motivation-physic learning link.
- Studying hands-on activity-physic understanding link.
Chemistry Education
- Conducting simple chemistry experiments.
- Studying interactive demo impact on learning.
- Creating virtual chemistry labs.
- Investigating peer tutoring benefits.
- Analyzing teacher enthusiasm-student engagement link.
Biology Education
- Developing creative biology knowledge assessment.
- Studying lab experience impact on understanding.
- Creating outdoor field trips for ecosystem exploration.
- Investigating cooperative learning benefits.
- Analyzing teacher feedback-student performance link.
Mathematics Education
- Designing strategies for difficult math concepts.
- Studying student attitude-math learning link.
- Creating tech-based math lessons.
- Investigating homework-academic performance link.
- Analyzing teacher expectation-achievement link.
Engineering Education
- Designing project-based learning for engineering.
- Developing hands-on activities for student engagement.
- Creating industry partnerships for education enhancement.
- Implementing mentoring for student support.
- Studying internship impact on student success.
Computer Science Education
- Developing programming tutorials for beginners.
- Creating engaging activities for student involvement.
- Designing online resource hubs.
- Developing gamified learning platforms.
- Investigating gender gap bridging strategies.
Environmental Science Education
- Creating outdoor learning experiences.
- Developing environmental education programs.
- Engaging students in research projects.
- Implementing place-based education.
- Studying fieldwork impact on engagement.
Geology Education
- Using geospatial tech to map geology.
- Organizing field trips to geological sites.
- Creating virtual reality geology experiences.
- Implementing inquiry-based geology learning.
- Studying teacher knowledge impact on understanding.
Astronomy Education
- Visiting planetariums.
- Exploring astronomy career paths.
- Using multimedia for solar system learning.
- Peer teaching astronomy concepts .
- Correcting astronomy misconceptions.
Medicine Education
- Using simulation for medical skill practice.
- Learning clinical skills through hands-on practice.
- Collaborating for improved patient care.
- Training in cultural competency for diverse care.
- Receiving feedback for skill and knowledge enhancement.
Neuroscience Education
- Teaching brain concepts using brain-based methods.
- Exploring neuroscience careers.
- Using interactive brain models for learning.
- Inquiry-based brain learning.
- Correcting neuroscience misconceptions.
Psychology Education
- Using varied assessment methods.
- Engaging students with interactive activities.
- Experiencing psychology concepts in real life.
- Leading peer discussions for deeper understanding.
- Connecting with instructors for enhanced learning.
Economics Education
- Playing economic simulations for learning.
- Learning about student attitudes toward economics.
- Gaining financial literacy.
- Applying economics to real-world situations.
- Using economics for informed decision-making.
Agriculture Education
- Learning about agriculture through hands-on activities.
- Exploring agriculture careers.
- Understanding agriculture basics.
- Gaining agricultural experience through internships.
- Practicing sustainable farming for a greener future.
Physics Engineering
- Choosing materials for engineering projects.
- Designing products for engineering success.
- Understanding manufacturing processes.
- Building for a sustainable future.
- Making every penny count in engineering.
Chemistry Engineering
- Transforming ideas into reality with chemical processes.
- Understanding material properties in engineering.
- Controlling chemical reactions for desired outcomes.
- Keeping chemical processes safe.
- Using energy wisely for a greener future.
Electrical Engineering
- Lighting up the world with circuit designs.
- Understanding electrical properties.
- Distributing power effectively.
- Ensuring signal clarity with electromagnetic interference.
- Building for a reliable future.
Mechanical Engineering
- Engineering the future with mechanical designs.
- Choosing the right materials for mechanical systems.
- Slowing things down with friction management.
- Understanding thermal effects in mechanical engineering.
- Making things strong with design parameters.
Civil Engineering
- Building strong foundations with construction materials.
- Engineering durable infrastructure.
- Considering environmental impact in civil engineering.
- Designing for earthquake resilience.
- Building sustainably with cost-effective solutions.
Environmental Engineering
- Cleaning up the environment with pollution control.
- Balancing industry and nature with environmental regulations.
- Purifying water with wastewater treatment.
- Breathing clean air with air pollution control.
- Powering a green future with renewable energy.
Software Engineering
- Making programs that work with software development.
- Keeping software simple for usability.
- Ensuring software quality with testing.
- Maintaining software for longevity.
- Documenting software for understanding.
Data Science
- Teaching computers to learn with machine learning.
- Ensuring data quality for accurate analysis.
- Visualizing data for easy understanding.
- Preparing data for analysis.
- Protecting data for privacy and security.
Cybersecurity
- Keeping hackers out with intrusion detection.
- Establishing cybersecurity policies for protection.
- Protecting against online dangers with cyber threat prevention.
- Keeping data secure with social engineering awareness.
- Ensuring data protection with encryption.
Network Engineering
- Understanding network protocols for data transfer.
- Mapping network topology for efficient communication.
- Managing network congestion for smooth operation.
- Securing networks against threats.
- Scaling networks for growth and demand.
Human-Computer Interaction
- Designing user-friendly interfaces for ease of use.
- Enhancing user experience for satisfaction.
- Listening to user feedback for improvements.
- Using mobile devices effectively for convenience.
- Ensuring accessibility for all users.
- Implementing control algorithms for robot movement.
- Designing robots for specific tasks with robot design.
- Providing sensory feedback for robot understanding.
- Developing autonomous robots for independence.
- Considering ethical implications of robotics for societal impact.
These topics are designed to be engaging and relatable, making research more accessible and enjoyable for STEM students!
And there you have it, the grand finale of our journey into the realm of Quantitative Research Topics for STEM students! These aren’t just research topics; they’re like secret codes unlocking the mysteries of science.
Whether you’re diving into the cosmos with physics, peeking into the microscopic wonders of biology, crunching numbers with mathematics, or riding the tech wave, these topics are more than just projects—they’re like treasure maps leading you to scientific wonders.
As we wrap up, think of these Quantitative Research Topics as not just academic challenges but as your personal invitations to be scientific explorers. Each topic is a ticket to understanding the world a bit better, and who knows, maybe even changing it! So, keep that scientific curiosity alive, grab your virtual lab goggles, and let the quantitative research adventure continue to unfold!
Frequently Asked Questions
What are some common data collection techniques in quantitative research.
Common techniques include surveys, experiments, statistical modeling, and data visualization.
Where can I access resources for my quantitative research project?
University libraries, online databases, and academic journals are valuable resources for research materials and data.
Leave a Comment Cancel Reply
Your email address will not be published. Required fields are marked *
Save my name, email, and website in this browser for the next time I comment.

80 Experimental Quantitative Research Topics for STEM Students
Dive into a captivating world of quantitative research topics for STEM students! Fuel your scientific curiosity and sharpen your analytical skills as you navigate through this carefully curated collection. Picture it as your personal roadmap, guiding you through the thrilling landscapes of Science, Technology, Engineering, and Mathematics.
Picture yourself as a scientific adventurer, standing at the intersection of curiosity and precision. The vast expanse of STEM awaits, and the quantitative research frontier is your ticket to uncharted territories where data becomes your trusted guide. So, fellow scholars, buckle up as we embark on a journey designed to not only pique your curiosity but also propel you into the heart of STEM exploration.
Think of this collection as more than just a list of topics; it’s your backstage pass to a rollercoaster of analytical adventures. Watch as numbers pirouette and graphs spin tales of discovery. Get ready to unravel the mysteries of the quantitative realm, where each topic is a portal to transformative magic for aspiring scientists and researchers.
Consider this your invitation to the captivating universe of quantitative research in STEM—it’s not just a collection; it’s your VIP access to an exploration that promises to be both thrilling and enlightening. Let the journey begin!
Table of Contents
The Power of Experimental Quantitative Research
Here’s an in-depth look at the power of experimental quantitative research for STEM students:
Cause-and-Effect Quest
Ever wonder why things happen? Experimental quantitative research is like the superhero of answers. It dives deep into cause-and-effect relationships, shaking things up in a controlled setting to reveal the real power players.
Stats Showdown
Think of it as a statistical showdown. This research isn’t just crunching numbers; it’s unleashing statistical wizards like ANOVA and regression analysis. These magic tools make sure the conclusions are not just guesses but rock-solid findings.
Precision Masters
Precision is the name of the game. Experimental designs let researchers measure stuff with surgical precision. No blurry lines here—just clear, accurate measurements that make other research methods jealous.
Do It Again, Sam
One experiment, two experiments, three! The cool thing about experimental research is its replay button. When you get similar results over and over, it’s like hitting “repeat” on a killer playlist. It boosts the credibility of your findings and lets you say, “Yep, we’re onto something big.”
No Sneaky Variables Allowed
Ever had unwanted guests crash your party? Experimental research kicks out those sneaky variables that could mess up your results. It’s like having bouncers at the door, ensuring only the main players get in.
Quantify Everything
If you can measure it, you can study it. Experimental research loves turning everything into numbers. It’s not just about changes; it’s about quantifiable, concrete outcomes that you can hold up and say, “Yep, we nailed it.”
Hypothesis Hustle
It’s like a detective story. Researchers cook up hypotheses, design experiments to test them, and then play detective with the results. It’s not just a guessing game; it’s a strategic hunt for answers.
Smart Decision DJ
Picture this: you’re making decisions with a DJ booth of data. The insights from experimental research guide decisions, whether it’s improving a product, tweaking a lesson plan, or steering a business strategy. It’s like having a crystal ball, but way more reliable.
Knowledge Thrill
Ever get a thrill from connecting the dots? Experimental research is the ultimate dot connector. It doesn’t just stop at one study—it builds on existing knowledge, refines theories, and pushes the boundaries of what we know.
Real-World Magic
Finally, it’s not just about fancy theories. Experimental research brings real-world magic. From making better gadgets to crafting smarter policies, it’s the real deal that transforms ideas into practical solutions. So, let the experimental adventure begin!
Quantitative Research Topics for STEM Students
Check out experimental quantitative research topics for stem students:-
- How does temperature affect plant growth?
- Exploring urban insect diversity.
- How does pollution impact fish populations?
- Studying genetic variations in fruit flies.
- Which fertilizer boosts tomato yield best?
- How does climate change affect bird migration?
- Does diet influence gut bacteria?
- Testing herbal remedies for headaches.
- Effects of deforestation on bird habitats.
- Investigating pesticides’ impact on bees.
- How fast does sugar dissolve in water?
- Exploring everyday materials’ properties.
- Does acidity differ among fruit juices?
- Testing various cleaning agents’ efficiency.
- Reaction rates of baking soda and vinegar.
- Synthesizing aspirin in the lab.
- Analyzing aspirin purity through titration.
- How does temperature affect crystal growth?
- Conductivity testing of different metals.
- Effects of air pollution on metal corrosion.
- How does ramp angle affect toy car distance?
- Exploring solar panel efficiency in various lighting.
- Studying different magnet strengths.
- How do sound waves behave in materials?
- Analyzing water flow through different pipes.
- Testing friction across various surfaces.
- How do colors of light differ in properties?
- Studying engine efficiency across types.
- Exploring electron behavior in circuits.
- Analyzing lens properties across types.
Mathematics
- Patterns in the times tables.
- Relationship between circle circumference and diameter.
- Distribution of prime numbers.
- Efficiency of equation-solving methods.
- Properties of different triangles.
- Accuracy of estimating pi methods.
- Fibonacci sequence’s relationship with nature.
- Efficiency of Sudoku-solving methods.
- Properties of different types of graphs.
- Probability in various games of chance.
Engineering
- Paper airplane design impact on flight.
- Strength of different bridge types.
- Wind turbine efficiency in power generation.
- Material properties for building bridges.
- Efficiency of water filtration system designs.
- Durability testing of building materials.
- Paper tower design impact on strength.
- Wing design impact on airplane lift.
- Roller coaster design efficiency.
- Properties of different gear types.
Environmental Science
- pH’s effect on aquatic life.
- Deforestation’s impact on soil erosion.
- Recycling program effectiveness in waste reduction.
- Air pollution’s effect on plant growth.
- Oil spill cleanup methods’ efficiency.
- Water quality testing in local water bodies.
- Climate change’s impact on bird migration.
- Ocean acidification’s effect on coral reefs.
- Composting methods’ efficiency.
- Urbanization’s effect on air quality.
Health Sciences
- Exercise impact on heart rate.
- Diet’s effect on blood sugar levels.
- Sterilization methods’ effectiveness.
- Sleep’s role in memory retention.
- Stress’s effect on immune function.
- Pain relief method comparison.
- Music’s impact on mood.
- Diet’s influence on acne.
- Caffeine’s effect on reaction time.
- Smoking cessation method effectiveness.
Computer Science
- File size’s impact on download time.
- Sorting algorithm efficiency comparison.
- Antivirus software effectiveness testing.
- Social media’s effect on mental health.
- Password security analysis.
- Programming language efficiency comparison.
- Screen time’s impact on eye strain.
- Spam email blocking method comparison.
- Video game’s effect on cognitive function.
- Search algorithm efficiency comparison.
These topics offer a plethora of engaging research avenues for STEM students to explore!
What is a quantitative study related to stem strand?
Absolutely, let’s add a touch of excitement and a dash of curiosity to the concept of quantitative studies in STEM:
Ever wondered how effective our STEM superhero training is? Join us on a quest where numbers reveal the superpowers our young heroes are gaining – from learning to engagement, it’s a STEM adventure in the making!
Math Mysteries
Imagine embarking on a quest to crack the code of the most enchanting math teaching methods. We’re using numbers to unlock the secrets and find out which teaching methods make math an epic journey!
Tech Time Warp
Step into a time warp to explore the impact of technology in STEM classrooms. We’re crunching numbers to unveil the connection between tech wizardry and students’ fascinating journey through the STEM universe!
STEM Jam Sessions
Ever been curious about the magic of STEM extracurricular activities? Get ready to rock with the impact – we’re turning up the volume on how these activities groove with students’ interests, skills, and future STEM dreams.
RoboRevolution
Join the revolution and dance with the impact of robotics! We’re using numbers to unravel the story of how our robotic buddies are shaping students’ understanding of engineering, problem-solving, and teamwork.
Physics Fiesta
Picture a fiesta where traditional teaching methods face off against hands-on learning in physics. Get ready for a showdown – we’re crunching the numbers to see which teaching style turns physics into the ultimate party!
STEM Sisters
Lights, camera, mentorship! Discover the magic mentorship brings to aspiring female scientists . We’re using numbers to capture the story of how mentorship shapes dreams and success in the world of STEM.
Chemical Magic
Imagine a magical realm where virtual and real labs compete in chemistry education. We’re diving into the data to see which type of magic – virtual or hands-on – creates the most enchanting learning experience.
Rocket Launchers
Buckle up for an odyssey into the impact of STEM education on career paths. We’re using numbers to capture the essence of how STEM education propels students into exciting career trajectories.
Internship Adventure
Join the internship adventure where STEM students become workplace explorers. We’re using numbers to capture the spirit of how internships prepare our STEM enthusiasts for the real-world adventure.
These engaging examples turn the exploration of STEM concepts into thrilling quests, magical showdowns, and epic adventures, making the world of quantitative studies in STEM feel more like a captivating journey!
Hey future STEM explorers, let’s wrap up this quantitative research journey with some serious excitement! Picture this: you’re in a massive theme park of ideas. From tiny molecular mysteries to epic cosmic adventures, STEM is basically your ultimate rollercoaster ride.
This isn’t your average math class – it’s like being a science superhero. You’re not just learning; you’re decoding secrets, analyzing data like a wizard, and dropping knowledge bombs left and right.
Quantitative research is like your trusty sidekick, helping you navigate the crazy jungle of data. It’s not just about acing tests; it’s about painting your own graffiti on the walls of STEM greatness. Your research isn’t just making you smarter; it’s adding a funky beat to the STEM jam.
So get pumped, future STEM rockstars – every formula you conquer, every discovery you make, it’s like you’re dropping the mic in the concert of science. Game on, champs!
Frequently Asked Questions
What is the key difference between quantitative and qualitative research.
Quantitative research focuses on numerical data and statistical analysis, while qualitative research emphasizes understanding human behavior and motivations.
Are there interdisciplinary research opportunities in STEM?
Absolutely! Many groundbreaking discoveries occur at the intersection of STEM disciplines, so don’t hesitate to explore interdisciplinary topics.
Leave a Comment Cancel Reply
Your email address will not be published. Required fields are marked *
Save my name, email, and website in this browser for the next time I comment.

189+ Good Quantitative Research Topics For STEM Students
Quantitative research is an essential part of STEM (Science, Technology, Engineering, and Mathematics) fields. It involves collecting and analyzing numerical data to answer research questions and test hypotheses.
In 2023, STEM students have a wealth of exciting research opportunities in various disciplines. Whether you’re an undergraduate or graduate student, here are quantitative research topics to consider for your next project.
If you are looking for the best list of quantitative research topics for stem students, then you can check the given list in each field. It offers STEM students numerous opportunities to explore and contribute to their respective fields in 2023 and beyond.
Whether you’re interested in astrophysics, biology, engineering, mathematics, or any other STEM field.
Also Read: Most Exciting Qualitative Research Topics For Students
What Is Quantitative Research
Table of Contents
Quantitative research is a type of research that focuses on the organized collection, analysis, and evaluation of numerical data to answer research questions, test theories, and find trends or connections between factors. It is an organized, objective way to do study that uses measurable data and scientific methods to come to results.
Quantitative research is often used in many areas, such as the natural sciences, social sciences, economics, psychology, education, and market research. It gives useful information about patterns, trends, cause-and-effect relationships, and how often things happen. Quantitative tools are used by researchers to answer questions like “How many?” and “How often?” “Is there a significant difference?” or “What is the relationship between the variables?”
In comparison to quantitative research, qualitative research uses non-numerical data like conversations, notes, and open-ended surveys to understand and explore the ideas, experiences, and points of view of people or groups. Researchers often choose between quantitative and qualitative methods based on their research goals, questions, and the type of thing they are studying.
How To Choose Quantitative Research Topics For STEM
Here’s a step-by-step guide on how to choose quantitative research topics for STEM:
Step 1:- Identify Your Interests and Passions
Start by reflecting on your personal interests within STEM. What areas or subjects in STEM excite you the most? Choosing a topic you’re passionate about will keep you motivated throughout the research process.
Step 2:- Review Coursework and Textbooks
Look through your coursework, textbooks, and class notes. Identify concepts, theories, or areas that you found particularly intriguing or challenging. These can be a source of potential research topics.
Step 3:- Consult with Professors and Advisors
Discuss your research interests with professors, academic advisors, or mentors. They can provide valuable insights, suggest relevant topics, and guide you toward areas with research opportunities.
Step 4:- Read Recent Literature
Explore recent research articles, journals, and publications in STEM fields. This will help you identify current trends, gaps in knowledge, and areas where further research is needed.
Step 5:- Narrow Down Your Focus
Once you have a broad area of interest, narrow it down to a specific research focus. Consider questions like:
- What specific problem or phenomenon do you want to investigate?
- Are there unanswered questions or controversies in this area?
- What impact could your research have on the field or society?
Step 6:- Consider Resources and Access
Assess the resources available to you, including access to laboratories, equipment, databases, and funding. Ensure that your chosen topic aligns with the resources you have or can access.
Step 7:- Think About Practicality
Consider the feasibility of conducting research on your chosen topic. Are the data readily available, or will you need to collect data yourself? Can you complete the research within your available time frame?
Step 8:- Define Your Research Question
Formulate a clear and specific research question or hypothesis. Your research question should guide your entire study and provide a focus for your data collection and analysis.
Step 9:- Conduct a Literature Review
Dive deeper into the existing literature related to your chosen topic. This will help you understand the current state of research, identify gaps, and refine your research question.
Step 10:- Consider the Impact
Think about the potential impact of your research. How does your topic contribute to the advancement of knowledge in your field? Does it have practical applications or implications for society?
Step 11:- Brainstorm Research Methods
Determine the quantitative research methods and data collection techniques you plan to use. Consider whether you’ll conduct experiments, surveys, data analysis, simulations, or use existing datasets.
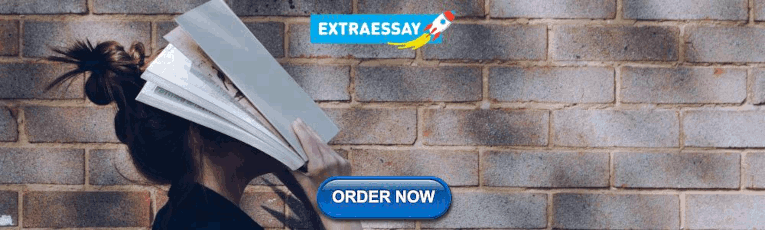
Step 12:- Seek Feedback
Share your research topic and ideas with peers, advisors, or mentors. They can provide valuable feedback and help you refine your research focus.
Step 13:- Assess Ethical Considerations
Consider ethical implications related to your research, especially if it involves human subjects, sensitive data, or potential environmental impacts. Ensure that your research adheres to ethical guidelines.
Step 14:- Finalize Your Research Topic
Once you’ve gone through these steps, finalize your research topic. Write a clear and concise research proposal that outlines your research question, objectives, methods, and expected outcomes.
Step 15:- Stay Open to Adjustments
Be open to adjusting your research topic as you progress. Sometimes, new insights or challenges may lead you to refine or adapt your research focus.
Following are the most interesting quantitative research topics for stem students. These are given below.
Quantitative Research Topics In Physics and Astronomy
- Quantum Computing Algorithms : Investigate new algorithms for quantum computers and their potential applications.
- Dark Matter Detection Methods : Explore innovative approaches to detect dark matter particles.
- Quantum Teleportation : Study the principles and applications of quantum teleportation.
- Exoplanet Characterization : Analyze data from telescopes to characterize exoplanets.
- Nuclear Fusion Modeling : Create mathematical models for nuclear fusion reactions.
- Superconductivity at High Temperatures : Research the properties and applications of high-temperature superconductors.
- Gravitational Wave Analysis : Analyze gravitational wave data to study astrophysical phenomena.
- Black Hole Thermodynamics : Investigate the thermodynamics of black holes and their entropy.
Quantitative Research Topics In Biology and Life Sciences
- Genome-Wide Association Studies (GWAS) : Conduct GWAS to identify genetic factors associated with diseases.
- Pharmacokinetics and Pharmacodynamics : Study drug interactions in the human body.
- Ecological Modeling : Model ecosystems to understand population dynamics.
- Protein Folding : Research the kinetics and thermodynamics of protein folding.
- Cancer Epidemiology : Analyze cancer incidence and risk factors in specific populations.
- Neuroimaging Analysis : Develop algorithms for analyzing brain imaging data.
- Evolutionary Genetics : Investigate evolutionary patterns using genetic data.
- Stem Cell Differentiation : Study the factors influencing stem cell differentiation.
Engineering and Technology Quantitative Research Topics
- Renewable Energy Efficiency : Optimize the efficiency of solar panels or wind turbines.
- Aerodynamics of Drones : Analyze the aerodynamics of drone designs.
- Autonomous Vehicle Safety : Evaluate safety measures for autonomous vehicles.
- Machine Learning in Robotics : Implement machine learning algorithms for robot control.
- Blockchain Scalability : Research methods to scale blockchain technology.
- Quantum Computing Hardware : Design and test quantum computing hardware components.
- IoT Security : Develop security protocols for the Internet of Things (IoT).
- 3D Printing Materials Analysis : Study the mechanical properties of 3D-printed materials.
Quantitative Research Topics In Mathematics and Statistics
Following are the best Quantitative Research Topics For STEM Students in mathematics and statistics.
- Prime Number Distribution : Investigate the distribution of prime numbers.
- Graph Theory Algorithms : Develop algorithms for solving graph theory problems.
- Statistical Analysis of Financial Markets : Analyze financial data and market trends.
- Number Theory Research : Explore unsolved problems in number theory.
- Bayesian Machine Learning : Apply Bayesian methods to machine learning models.
- Random Matrix Theory : Study the properties of random matrices in mathematics and physics.
- Topological Data Analysis : Use topology to analyze complex data sets.
- Quantum Algorithms for Optimization : Research quantum algorithms for optimization problems.
Experimental Quantitative Research Topics In Science and Earth Sciences
- Climate Change Modeling : Develop climate models to predict future trends.
- Biodiversity Conservation Analysis : Analyze data to support biodiversity conservation efforts.
- Geographic Information Systems (GIS) : Apply GIS techniques to solve environmental problems.
- Oceanography and Remote Sensing : Use satellite data for oceanographic research.
- Air Quality Monitoring : Develop sensors and models for air quality assessment.
- Hydrological Modeling : Study the movement and distribution of water resources.
- Volcanic Activity Prediction : Predict volcanic eruptions using quantitative methods.
- Seismology Data Analysis : Analyze seismic data to understand earthquake patterns.
Chemistry and Materials Science Quantitative Research Topics
- Nanomaterial Synthesis and Characterization : Research the synthesis and properties of nanomaterials.
- Chemoinformatics : Analyze chemical data for drug discovery and materials science.
- Quantum Chemistry Simulations : Perform quantum simulations of chemical reactions.
- Materials for Renewable Energy : Investigate materials for energy storage and conversion.
- Catalysis Kinetics : Study the kinetics of chemical reactions catalyzed by materials.
- Polymer Chemistry : Research the properties and applications of polymers.
- Analytical Chemistry Techniques : Develop new analytical techniques for chemical analysis.
- Sustainable Chemistry : Explore green chemistry approaches for sustainable materials.
Computer Science and Information Technology Topics
- Natural Language Processing (NLP) : Work on NLP algorithms for language understanding.
- Cybersecurity Analytics : Analyze cybersecurity threats and vulnerabilities.
- Big Data Analytics : Apply quantitative methods to analyze large data sets.
- Machine Learning Fairness : Investigate bias and fairness issues in machine learning models.
- Human-Computer Interaction (HCI) : Study user behavior and interaction patterns.
- Software Performance Optimization : Optimize software applications for performance.
- Distributed Systems Analysis : Analyze the performance of distributed computing systems.
- Bioinformatics Data Mining : Develop algorithms for mining biological data.
Good Quantitative Research Topics Students In Medicine and Healthcare
- Clinical Trial Data Analysis : Analyze clinical trial data to evaluate treatment effectiveness.
- Epidemiological Modeling : Model disease spread and intervention strategies.
- Healthcare Data Analytics : Analyze healthcare data for patient outcomes and cost reduction.
- Medical Imaging Algorithms : Develop algorithms for medical image analysis.
- Genomic Medicine : Apply genomics to personalized medicine approaches.
- Telemedicine Effectiveness : Study the effectiveness of telemedicine in healthcare delivery.
- Health Informatics : Analyze electronic health records for insights into patient care.
Agriculture and Food Sciences Topics
- Precision Agriculture : Use quantitative methods for optimizing crop production.
- Food Safety Analysis : Analyze food safety data and quality control.
- Aquaculture Sustainability : Research sustainable practices in aquaculture.
- Crop Disease Modeling : Model the spread of diseases in agricultural crops.
- Climate-Resilient Agriculture : Develop strategies for agriculture in changing climates.
- Food Supply Chain Optimization : Optimize food supply chain logistics.
- Soil Health Assessment : Analyze soil data for sustainable land management.
Social Sciences with Quantitative Approaches
- Educational Data Mining : Analyze educational data for improving learning outcomes.
- Sociodemographic Surveys : Study social trends and demographics using surveys.
- Psychometrics : Develop and validate psychological measurement instruments.
- Political Polling Analysis : Analyze political polling data and election trends.
- Economic Modeling : Develop economic models for policy analysis.
- Urban Planning Analytics : Analyze data for urban planning and infrastructure.
- Climate Policy Evaluation : Evaluate the impact of climate policies on society.
Environmental Engineering Quantitative Research Topics
- Water Quality Assessment : Analyze water quality data for environmental monitoring.
- Waste Management Optimization : Optimize waste collection and recycling programs.
- Environmental Impact Assessments : Evaluate the environmental impact of projects.
- Air Pollution Modeling : Model the dispersion of air pollutants in urban areas.
- Sustainable Building Design : Apply quantitative methods to sustainable architecture.
Quantitative Research Topics Robotics and Automation
- Robotic Swarm Behavior : Study the behavior of robot swarms in different tasks.
- Autonomous Drone Navigation : Develop algorithms for autonomous drone navigation.
- Humanoid Robot Control : Implement control algorithms for humanoid robots.
- Robotic Grasping and Manipulation : Study robotic manipulation techniques.
- Reinforcement Learning for Robotics : Apply reinforcement learning to robotic control.
Quantitative Research Topics Materials Engineering
- Additive Manufacturing Process Optimization : Optimize 3D printing processes.
- Smart Materials for Aerospace : Research smart materials for aerospace applications.
- Nanostructured Materials for Energy Storage : Investigate energy storage materials.
- Corrosion Prevention : Develop corrosion-resistant materials and coatings.
Nuclear Engineering Quantitative Research Topics
- Nuclear Reactor Safety Analysis : Study safety aspects of nuclear reactor designs.
- Nuclear Fuel Cycle Analysis : Analyze the nuclear fuel cycle for efficiency.
- Radiation Shielding Materials : Research materials for radiation protection.
Quantitative Research Topics In Biomedical Engineering
- Medical Device Design and Testing : Develop and test medical devices.
- Biomechanics Analysis : Analyze biomechanics in sports or rehabilitation.
- Biomaterials for Medical Implants : Investigate materials for medical implants.
Good Quantitative Research Topics Chemical Engineering
- Chemical Process Optimization : Optimize chemical manufacturing processes.
- Industrial Pollution Control : Develop strategies for pollution control in industries.
- Chemical Reaction Kinetics : Study the kinetics of chemical reactions in industries.
Best Quantitative Research Topics In Renewable Energy
- Energy Storage Systems : Research and optimize energy storage solutions.
- Solar Cell Efficiency : Improve the efficiency of photovoltaic cells.
- Wind Turbine Performance Analysis : Analyze and optimize wind turbine designs.
Brilliant Quantitative Research Topics In Astronomy and Space Sciences
- Astrophysical Simulations : Simulate astrophysical phenomena using numerical methods.
- Spacecraft Trajectory Optimization : Optimize spacecraft trajectories for missions.
- Exoplanet Detection Algorithms : Develop algorithms for exoplanet detection.
Quantitative Research Topics In Psychology and Cognitive Science
- Cognitive Psychology Experiments : Conduct quantitative experiments in cognitive psychology.
- Emotion Recognition Algorithms : Develop algorithms for emotion recognition in AI.
- Neuropsychological Assessments : Create quantitative assessments for brain function.
Geology and Geological Engineering Quantitative Research Topics
- Geological Data Analysis : Analyze geological data for mineral exploration.
- Geological Hazard Prediction : Predict geological hazards using quantitative models.
Top Quantitative Research Topics In Forensic Science
- Forensic Data Analysis : Analyze forensic evidence using quantitative methods.
- Crime Pattern Analysis : Study crime patterns and trends in urban areas.
Great Quantitative Research Topics In Cybersecurity
- Network Intrusion Detection : Develop quantitative methods for intrusion detection.
- Cryptocurrency Analysis : Analyze blockchain data and cryptocurrency trends.
Mathematical Biology Quantitative Research Topics
- Epidemiological Modeling : Model disease spread and control in populations.
- Population Genetics : Analyze genetic data to understand population dynamics.
Quantitative Research Topics In Chemical Analysis
- Analytical Chemistry Methods : Develop quantitative methods for chemical analysis.
- Spectroscopy Analysis : Analyze spectroscopic data for chemical identification.
Mathematics Education Quantitative Research Topics
- Mathematics Curriculum Analysis : Analyze curriculum effectiveness in mathematics education.
- Mathematics Assessment Development : Develop quantitative assessments for mathematics skills.
Quantitative Research Topics In Social Research
- Social Network Analysis : Analyze social network structures and dynamics.
- Survey Research : Conduct quantitative surveys on social issues and trends.
Quantitative Research Topics In Computational Neuroscience
- Neural Network Modeling : Model neural networks and brain functions computationally.
- Brain Connectivity Analysis : Analyze functional and structural brain connectivity.
Best Topics In Transportation Engineering
- Traffic Flow Modeling : Model and optimize traffic flow in urban areas.
- Public Transportation Efficiency : Analyze the efficiency of public transportation systems.
Good Quantitative Research Topics In Energy Economics
- Energy Policy Analysis : Evaluate the economic impact of energy policies.
- Renewable Energy Cost-Benefit Analysis : Assess the economic viability of renewable energy projects.
Quantum Information Science
- Quantum Cryptography Protocols : Develop and analyze quantum cryptography protocols.
- Quantum Key Distribution : Study the security of quantum key distribution systems.
Human Genetics
- Genome Editing Ethics : Investigate ethical issues in genome editing technologies.
- Population Genomics : Analyze genomic data for population genetics research.
Marine Biology
- Coral Reef Health Assessment : Quantitatively assess the health of coral reefs.
- Marine Ecosystem Modeling : Model marine ecosystems and biodiversity.
Data Science and Machine Learning
- Machine Learning Explainability : Develop methods for explaining machine learning models.
- Data Privacy in Machine Learning : Study privacy issues in machine learning applications.
- Deep Learning for Image Analysis : Develop deep learning models for image recognition.
Environmental Engineering
Robotics and automation, materials engineering, nuclear engineering, biomedical engineering, chemical engineering, renewable energy, astronomy and space sciences, psychology and cognitive science, geology and geological engineering, forensic science, cybersecurity, mathematical biology, chemical analysis, mathematics education, quantitative social research, computational neuroscience, quantitative research topics in transportation engineering, quantitative research topics in energy economics, topics in quantum information science, amazing quantitative research topics in human genetics, quantitative research topics in marine biology, what is a common goal of qualitative and quantitative research.
A common goal of both qualitative and quantitative research is to generate knowledge and gain a deeper understanding of a particular phenomenon or topic. However, they approach this goal in different ways:
1. Understanding a Phenomenon
Both types of research aim to understand and explain a specific phenomenon, whether it’s a social issue, a natural process, a human behavior, or a complex event.
2. Testing Hypotheses
Both qualitative and quantitative research can involve hypothesis testing. While qualitative research may not use statistical hypothesis tests in the same way as quantitative research, it often tests hypotheses or research questions by examining patterns and themes in the data.
3. Contributing to Knowledge
Researchers in both approaches seek to contribute to the body of knowledge in their respective fields. They aim to answer important questions, address gaps in existing knowledge, and provide insights that can inform theory, practice, or policy.
4. Informing Decision-Making
Research findings from both qualitative and quantitative studies can be used to inform decision-making in various domains, whether it’s in academia, government, industry, healthcare, or social services.
5. Enhancing Understanding
Both approaches strive to enhance our understanding of complex phenomena by systematically collecting and analyzing data. They aim to provide evidence-based explanations and insights.
6. Application
Research findings from both qualitative and quantitative studies can be applied to practical situations. For example, the results of a quantitative study on the effectiveness of a new drug can inform medical treatment decisions, while qualitative research on customer preferences can guide marketing strategies.
7. Contributing to Theory
In academia, both types of research contribute to the development and refinement of theories in various disciplines. Quantitative research may provide empirical evidence to support or challenge existing theories, while qualitative research may generate new theoretical frameworks or perspectives.
Conclusion – Quantitative Research Topics For STEM Students
So, selecting a quantitative research topic for STEM students is a pivotal decision that can shape the trajectory of your academic and professional journey. The process involves a thoughtful exploration of your interests, a thorough review of the existing literature, consideration of available resources, and the formulation of a clear and specific research question.
Your chosen topic should resonate with your passions, align with your academic or career goals, and offer the potential to contribute to the body of knowledge in your STEM field. Whether you’re delving into physics, biology, engineering, mathematics, or any other STEM discipline, the right research topic can spark curiosity, drive innovation, and lead to valuable insights.
Moreover, quantitative research in STEM not only expands the boundaries of human knowledge but also has the power to address real-world challenges, improve technology, and enhance our understanding of the natural world. It is a journey that demands dedication, intellectual rigor, and an unwavering commitment to scientific inquiry.
What is quantitative research in STEM?
Quantitative research in this context is designed to improve our understanding of the science system’s workings, structural dependencies and dynamics.
What are good examples of quantitative research?
Surveys and questionnaires serve as common examples of quantitative research. They involve collecting data from many respondents and analyzing the results to identify trends, patterns
What are the 4 C’s in STEM?
They became known as the “Four Cs” — critical thinking, communication, collaboration, and creativity.
Similar Articles

Top 19 Tips & Tricks On How To Improve Grades?
Do you want to improve your grades? If yes, then don’t worry! In this blog, I have provided 19 tips…

How To Study For Final Exam – 12 Proven Tips You Must Know
How To Study For Final Exam? Studying for the final exam is very important for academic success because they test…
Leave a Comment Cancel Reply
Your email address will not be published. Required fields are marked *
This site uses Akismet to reduce spam. Learn how your comment data is processed .

60+ Best Quantitative Research Topics for STEM Students: Dive into Data
Embark on a captivating journey through the cosmos of knowledge with our curated guide on Quantitative Research Topics for STEM Students. Explore innovative ideas in science, technology, engineering, and mathematics, designed to ignite curiosity and shape the future.
Unleash the power of quantitative research and dive into uncharted territories that go beyond academics, fostering innovation and discovery.
Hey, you future scientists, tech wizards, engineering maestros, and math superheroes – gather ’round! We’re about to dive headfirst into the rad world of quantitative research topics, tailor-made for the rockstars of STEM.
In the crazy universe of science, technology, engineering, and math (STEM), quantitative research isn’t just a nerdy term—it’s your VIP pass to an interstellar adventure. Picture this: you’re strapping into a rocket ship, zooming through the cosmos, and decoding the universe’s coolest secrets, all while juggling numbers like a cosmic DJ.
But here’s the real scoop: finding the ultimate research topic is like picking the juiciest star in the galaxy. It’s about stumbling upon something so mind-blowing that you can’t resist plunging into the data. It’s about choosing questions that make your STEM-loving heart do the cha-cha.
In this guide, we’re not just your sidekicks; we’re your partners in crime through the vast jungle of quantitative research topics. Whether you’re a rookie gearing up for your first lab escapade or a seasoned explorer hunting for a new thrill, think of this article as your treasure map, guiding you to the coolest STEM discoveries.
From the teeny wonders of biology to the brain-bending puzzles of physics, the cutting-edge vibes of engineering, and the downright gorgeous dance of mathematics – we’ve got your back.
So, buckle up, fellow STEM enthusiasts! We’re setting sail on a cosmic adventure through the groovy galaxy of quantitative research topics. Get ready to unravel the secrets of science and tech, one sizzling digit at a time.
Stick around for a ride that’s part data, part disco, and all STEM swagger!
Table of Contents
Benefits of Choosing Quantitative Research
Embarking on the quantitative research journey is like stepping into a treasure trove of benefits across a spectrum of fields. Let’s dive into the exciting advantages that make choosing quantitative research a game-changer:
Numbers That Speak Louder
Quantitative research deals in cold, hard numbers. This means your data isn’t just informative; it’s objective, measurable, and has a voice of its own.
Statistical Swagger
Crunching numbers isn’t just for show. With quantitative research, statistical tools add a touch of pizzazz, boosting the validity of your findings and turning your study into a credible performance.
For the Masses
Quantitative research loves a crowd. Larger sample sizes mean your discoveries aren’t just for the lucky few – they’re for everyone. It’s the science of sharing the knowledge wealth.
Data Showdown
Ready for a duel between variables? Quantitative research sets the stage for epic battles, letting you compare, contrast, and uncover cause-and-effect relationships in the data arena.
Structured and Ready to Roll
Think of quantitative research like a well-organized party. It follows a structured plan, making replication a breeze. Because who doesn’t love a party that’s easy to recreate?
Data Efficiency Dance
Efficiency is the name of the game. Surveys, experiments, and structured observations make data collection a dance – choreographed, smooth, and oh-so-efficient.
Data Clarity FTW
No decoding needed here. Quantitative research delivers crystal-clear results. It’s like reading a good book without the need for interpretation – straightforward and to the point.
Spotting Trends Like a Pro
Ever wish you had a crystal ball for trends? Quantitative analysis is the next best thing. It’s like having a trend-spotting superpower, revealing patterns that might have otherwise stayed hidden.
Bias Be Gone
Quantitative research takes bias out of the equation. Systematic data collection and statistical wizardry reduce researcher bias, leaving you with results that are as unbiased as a judge at a talent show.
Key Components of a Quantitative Research Study
Launching into a quantitative research study is like embarking on a thrilling quest, and guess what? You’re the hero of this research adventure! Let’s unravel the exciting components that make your study a blockbuster:
Quest-Starter: Research Question or Hypothesis
It’s your “once upon a time.” Kick off your research journey with a bang by crafting a captivating research question or hypothesis. This is the spark that ignites your curiosity.
Backstory Bonanza: Literature Review
Think of it as your research Netflix binge. Dive into existing literature for the backstory. It’s not just research – it’s drama, plot twists, and the foundation for your epic tale.
Blueprint Brilliance: Research Design
Time to draw up the plans for your study castle. Choose your research design – is it a grand experiment or a cunning observational scheme? Your design is the architectural genius behind your research.
Casting Call: Population and Sample
Who’s in your star-studded lineup? Define your dream cast – your target population – and then handpick a sample that’s ready for the research red carpet.
Gear Up: Data Collection Methods
Choose your research tools wisely – surveys, experiments, or maybe a bit of detective work. Your methods are like the gadgets in a spy movie, helping you collect the data treasures.
The Numbers Game: Variables and Measures
What’s in the spotlight? Identify your main characters – independent and dependent variables. Then, sprinkle in some measures to add flair and precision to your study.
Magic Analysis Wand: Data Analysis Techniques
Enter the wizardry zone! Pick your magic wand – statistical methods, tests, or software – and watch as it unravels the mysteries hidden in your data.
Ethical Superhero Cape: Ethical Considerations
Every hero needs a moral compass. Clearly outline how you’ll be the ethical superhero of your study, protecting the well-being and secrets of your participants.
Grand Finale: Results and Findings
It’s showtime! Showcase your results like the grand finale of a fireworks display. Tables, charts, and statistical dazzle – let your findings steal the spotlight.
Wrap-Up Party: Conclusion and Implications
Bring out the confetti! Summarize your findings, discuss their VIP status in the research world, and hint at the afterparty – how your results shape the future.
Behind-the-Scenes Blooper Reel: Limitations and Future Research
No Hollywood film is perfect. Share the bloopers – the limitations of your study – and hint at the sequel with ideas for future research. It’s all part of the cinematic journey.
Roll Credits: References
Give a shout-out to the supporting cast! Cite your sources – it’s the credits that add credibility to your blockbuster.
Bonus Scene: Appendix
Think of it as the post-credits scene. Tuck in any extra goodies – surveys, questionnaires, or behind-the-scenes material – for those eager to dive deeper into your research universe.
By weaving these storylines together, your quantitative research study becomes a cinematic masterpiece, leaving a lasting impact on the grand stage of academia. Happy researching, hero!
Quantitative Research Topics for STEM Students
Check out the best quantitative research topics for STEM students:-
- Investigating the Effects of Different Soil pH Levels on Plant Growth.
- Analyzing the Impact of Pesticide Exposure on Bee Populations.
- Studying the Genetic Variability in Endangered Species.
- Quantifying the Relationship Between Temperature and Microbial Growth in Water.
- Analyzing the Effects of Ocean Acidification on Coral Reefs.
- Investigating the Correlation Between Pollinator Diversity and Crop Yield.
- Studying the Role of Gut Microbiota in Human Health and Disease.
- Quantifying the Impact of Antibiotics on Soil Microbial Communities.
- Analyzing the Effects of Light Pollution on Nocturnal Animal Behavior.
- Investigating the Relationship Between Altitude and Plant Adaptations in Mountain Ecosystems.
- Measuring the Speed of Light Using Interferometry Techniques.
- Investigating the Quantum Properties of Photons in Quantum Computing.
- Analyzing the Factors Affecting Magnetic Field Strength in Electromagnets.
- Studying the Behavior of Superfluids at Ultra-Low Temperatures.
- Quantifying the Efficiency of Energy Transfer in Photovoltaic Cells.
- Analyzing the Properties of Quantum Dots for Future Display Technologies.
- Investigating the Behavior of Particles in High-Energy Particle Accelerators.
- Studying the Effects of Gravitational Waves on Space-Time.
- Quantifying the Frictional Forces on Objects at Different Surfaces.
- Analyzing the Characteristics of Dark Matter and Dark Energy in the Universe.
Engineering
- Optimizing the Design of Wind Turbine Blades for Maximum Efficiency.
- Investigating the Use of Smart Materials in Structural Engineering.
- Analyzing the Impact of 3D Printing on Prototyping in Product Design.
- Studying the Behavior of Composite Materials Under Extreme Temperatures.
- Evaluating the Efficiency of Water Treatment Plants in Removing Contaminants.
- Investigating the Aerodynamics of Drones for Improved Flight Control.
- Quantifying the Effects of Traffic Flow on Roadway Maintenance.
- Analyzing the Impact of Vibration Damping in Building Structures.
- Studying the Mechanical Properties of Biodegradable Polymers in Medical Devices.
- Investigating the Use of Artificial Intelligence in Autonomous Robotic Systems.
Mathematics
- Exploring Chaos Theory and Its Applications in Nonlinear Systems.
- Modeling the Spread of Infectious Diseases in Population Dynamics.
- Analyzing Data Mining Techniques for Predictive Analytics in Business.
- Studying the Mathematics of Cryptography Algorithms for Data Security.
- Quantifying the Patterns in Stock Market Price Movements Using Time Series Analysis.
- Investigating the Applications of Fractal Geometry in Computer Graphics.
- Analyzing the Behavior of Differential Equations in Climate Modeling.
- Studying the Optimization of Supply Chain Networks Using Linear Programming.
- Investigating the Mathematical Concepts Behind Machine Learning Algorithms.
- Quantifying the Patterns of Prime Numbers in Number Theory.
- Investigating the Chemical Mechanisms Behind Enzyme Catalysis.
- Analyzing the Thermodynamic Properties of Chemical Reactions.
- Studying the Kinetics of Chemical Reactions in Different Solvents.
- Quantifying the Concentration of Pollutants in Urban Air Quality.
- Evaluating the Effectiveness of Antioxidants in Food Preservation.
- Investigating the Electrochemical Properties of Batteries for Energy Storage.
- Studying the Behavior of Nanomaterials in Drug Delivery Systems.
- Analyzing the Chemical Composition of Exoplanet Atmospheres Using Spectroscopy.
- Quantifying Heavy Metal Contamination in Soil and Water Sources.
- Investigating the Correlation Between Chemical Exposure and Human Health.
Computer Science
- Analyzing Machine Learning Algorithms for Natural Language Processing.
- Investigating Quantum Computing Algorithms for Cryptography Applications.
- Studying the Efficiency of Data Compression Methods for Big Data Storage.
- Quantifying Cybersecurity Threats and Vulnerabilities in IoT Devices.
- Evaluating the Impact of Cloud Computing on Distributed Systems.
- Investigating the Use of Artificial Intelligence in Autonomous Vehicles.
- Analyzing the Behavior of Neural Networks in Deep Learning Applications.
- Studying the Performance of Blockchain Technology in Supply Chain Management.
- Quantifying User Behavior in Social Media Analytics.
- Investigating Quantum Machine Learning for Enhanced Data Processing.
These additional project ideas provide a diverse range of opportunities for STEM students to engage in quantitative research and explore various aspects of their respective fields. Each project offers a unique avenue for discovery and contribution to the world of science and technology.
What is an example of a quantitative research?
Quantitative research is a powerful investigative approach, wielding numbers to shed light on intricate relationships and phenomena. Let’s dive into an example of quantitative research to understand its workings:
Research Question
What is the correlation between the time students devote to studying and their academic grades?
Students who invest more time in studying are likely to achieve higher grades.
Research Design
Imagine a researcher embarking on a journey within a high school. They distribute surveys to students, inquiring about their weekly study hours and their corresponding grades in core subjects.
Data Analysis
Equipped with statistical tools, our researcher scrutinizes the collected data. Lo and behold, a significant positive correlation emerges—students who dedicate more time to studying generally earn higher grades.
With data as their guide, the researcher concludes that indeed, a relationship exists between study time and academic grades. The more time students commit to their studies, the brighter their academic stars tend to shine.
This example merely scratches the surface of quantitative research’s potential. It can delve into an extensive array of subjects and investigate complex hypotheses. Here are a few more examples:
- Assessing a New Drug’s Effectiveness: Quantifying the impact of a novel medication in treating a specific illness.
- Socioeconomic Status and Crime Rates: Investigating the connection between economic conditions and criminal activity.
- Analyzing the Influence of an Advertising Campaign on Sales: Measuring the effectiveness of a marketing blitz on product purchases.
- Factors Shaping Customer Satisfaction: Using data to pinpoint the elements contributing to customer contentment.
- Government Policies and Employment Rates: Evaluating the repercussions of new governmental regulations on job opportunities.
Quantitative research serves as a potent beacon, illuminating the complexities of our world through data-driven inquiry. Researchers harness its might to collect, analyze, and draw valuable conclusions about a vast spectrum of phenomena. It’s a vital tool for unraveling the intricacies of our universe.
As we bid adieu to our whirlwind tour of quantitative research topics tailor-made for the STEM dreamers, it’s time to soak in the vast horizons that science, technology, engineering, and mathematics paint for us.
We’ve danced through the intricate tango of poverty and crime, peeked into the transformative realm of cutting-edge technologies, and unraveled the captivating puzzles of quantitative research. But these aren’t just topics; they’re open invitations to dive headfirst into the uncharted seas of knowledge.
To you, the STEM trailblazers, these research ideas aren’t mere academic pursuits. They’re portals to curiosity, engines of innovation, and blueprints for shaping the future of our world. They’re the sparks that illuminate the trail leading to discovery.
As you set sail on your research odyssey, remember that quantitative research isn’t just about unlocking answers—it’s about nurturing that profound sense of wonder, igniting innovation, and weaving your unique thread into the fabric of human understanding.
Whether you’re stargazing, decoding the intricate language of genes, engineering marvels, or tackling global challenges head-on, realize that your STEM and quantitative research journey is a perpetual adventure.
May your questions be audacious, your data razor-sharp, and your discoveries earth-shattering. Keep that innate curiosity alive, keep exploring, and let the spirit of STEM be your North Star, guiding you towards a future that’s not just brighter but brilliantly enlightened.
And with that, fellow adventurers, go forth, embrace the unknown, and let your journey in STEM be the epic tale that reshapes the narrative of tomorrow!
Frequently Asked Questions
How can i ensure the ethical conduct of my quantitative research project.
To ensure ethical conduct, obtain informed consent from participants, maintain data confidentiality, and adhere to ethical guidelines established by your institution and professional associations.
Are there any software tools recommended for data analysis in STEM research?
Yes, there are several widely used software tools for data analysis in STEM research, including R, Python, MATLAB, and SPSS. The choice of software depends on your specific research needs and familiarity with the tools.
Leave a Comment Cancel Reply
Your email address will not be published. Required fields are marked *
Save my name, email, and website in this browser for the next time I comment.

An official website of the United States government
The .gov means it’s official. Federal government websites often end in .gov or .mil. Before sharing sensitive information, make sure you’re on a federal government site.
The site is secure. The https:// ensures that you are connecting to the official website and that any information you provide is encrypted and transmitted securely.
- Publications
- Account settings
Preview improvements coming to the PMC website in October 2024. Learn More or Try it out now .
- Advanced Search
- Journal List
- CBE Life Sci Educ
- v.21(1); Spring 2022
Integrating Critical Approaches into Quantitative STEM Equity Work
Meaghan i. pearson.
† Combined Program in Education and Psychology, University of Michigan, Ann Arbor, MI 48109
Sarah D. Castle
‡ Program in Mathematics Education, Michigan State University, East Lansing, MI 48824
Rebecca L. Matz
§ Center for Academic Innovation, University of Michigan, Ann Arbor, MI 48109
Benjamin P. Koester
ǁ Department of Physics, University of Michigan, Ann Arbor, MI 48109
W. Carson Byrd
¶ National Center for Institutional Diversity, University of Michigan, Ann Arbor, MI 48109
The recent anti-racist movements in the United States have inspired a national call for more research on the experiences of racially marginalized and minoritized students in science, technology, engineering, and mathematics (STEM) fields. As researchers focused on promoting diversity, equity, and inclusion, we contend that STEM education must, as a discipline, grapple with how analytic approaches may not fully support equity efforts. We discuss how researchers and educational practitioners should more critically approach STEM equity analyses and why modifying our approaches matters for STEM equity goals. Engaging with equity as a process rather than a static goal, we provide a primer of reflective questions to assist researchers with framing, analysis, and interpretation of student-level data frequently used to identify disparities and assess course-level and programmatic interventions. This guidance can inform analyses conducted by campus units such as departments and programs, but also across universities and the scientific community to enhance how we understand and address systemic inequity in STEM fields.
INTRODUCTION
Over the past 2 years, the world watched as police in the United States killed Black, Hispanic and Latinx Americans ( Egbuonu, 2020 ); hate crimes toward Asians, Asian Americans, and Pacific Islanders surged ( Gover et al. , 2020 ); and disproportionate numbers of Black, Hispanic and Latinx, and Native American people fell victim to the COVID-19 pandemic ( Alcendor, 2020 ). Questions arose across countries, institutions, and communities about how these devastating and brutal events reflect underlying systemic inequities that exist across the globe (e.g., Collins et al. , 2021 ). Higher education in particular witnessed another reinvestment in diversity, equity, and inclusion (DEI) efforts with reinvigorated commitments to faculty cluster hires, curricular revisions, and changes to admissions criteria ( Heinecke and Beach 2020 ; Peoples and Dillard, 2020 ). History has shown, however, that diversity initiatives can serve as mechanisms for institutions to send out signals of advancement without actually translating to systemic changes ( Ahmed, 2012 ; Ray, 2019a , b ; Johnson, 2020 ; Thomas 2020 ).
Likewise, science, technology, engineering, and mathematics (STEM) fields are focusing significant attention toward improving DEI in reflection on student enrollment and success data marred by gendered, racialized, and classed patterns ( Asai, 2020 ; Griffin et al. , 2020 ; McGee, 2020a , b ). Quantitative analyses in and focused on STEM education that rely on commonly available demographic variables (herein we consider gender, race, income, and parental education) are ever more present ( Li et al. , 2020 ). The key issue we address here with STEM equity analyses is that demographic variables are often used automatically in “assessing student success” without situating these student characteristics in relation to the overlapping structural inequities that shape students’ experiences and academic performance.
Because of this tendency, there are often mismatches between the outcomes of equity analyses and how they are interpreted to inform institutional efforts that promote DEI in STEM. By inadequately grappling with the theoretical framing of students’ identities in relation to inequities, current methodological approaches guiding equity analyses can contribute to individualizing inequality ( Byrd, 2021 ). As a consequence, educational initiatives are developed that prioritize changing students and not the STEM environments that perpetuate such inequalities.
STEM equity analyses are conducted by researchers in many different settings across academia—for example, STEM departments and programs do assessment work, institutional research offices conduct internal studies, and even individual instructors may be equipped to investigate their own courses. These analyses are also conducted by federal agencies, policy institutes, and corporations. That is, whether explicitly or implicitly described as “equity analyses” within a department, program, or organization, STEM equity analyses are part and parcel of everyday assessment and evaluation in STEM. Therefore, our intention here is to provide information that is applicable for researchers across the broader STEM community. Fostering an authentic discussion of issues raised by these questions will lead to better analyses that support justice for students who have historically been excluded from and continue to experience marginalization in STEM fields.
The purpose of this essay is twofold. We first discuss the importance of integrating critical perspectives in STEM equity research that relies on quantitative analyses. Then, through a series of critical questions, we aim to engender reflection and conversation with researchers and practitioners who do STEM equity research so that we all can use quantitative data more responsibly and accurately. This discussion was sparked by our own challenges in working with institutional data to better represent and understand the experiences and outcomes of marginalized and minoritized students 1 in STEM through the interdisciplinary Sloan Equity and Inclusion in STEM Introductory Courses (SEISMIC) collaboration. We (the authors) represent the fields of chemistry and biology education, physics and astronomy, mathematics and mathematics education, educational psychology, and sociology and engage in quantitative research regularly in our positions as faculty, staff, and graduate students. We identify mostly as cis-heterosexual men and women, and all of us, except for one author, identify as white. Nonetheless, we all identify with and embrace the need for our fields and institutions to continually improve research and decision making aimed at tackling campus inequalities and injustices.
WHAT ARE CRITICAL APPROACHES TO RESEARCH?
As researchers, the lens through which we view the world has implications for the research questions we seek to explore and our methodological and analytical choices. Those who receive their academic training in STEM fields are generally socialized to adopt a postpositivist lens ( Guba and Lincoln, 1994 ; Harding, 2006 ). Postpositivists assume that objectivity is achievable, where truths about the world remain credible if those seeking it follow the scientific method. This rationale works when observing scientific and mathematical phenomena like gravity or volcanic eruptions but breaks down when studying human experiences. Humans are situated in social contexts that have been shaped by historical events, structural forces, and interaction with other human beings. Thus, humans are more than objects of inquiry; humans and their experiences are a by-product of the structures in which they are embedded ( Horkheimer, 1972 ; Bohman, 2005 ; Devetak, 2005 ).
In contrast to postpositivism, a critical lens assumes that what can be known about the world is socially constructed. Critical theorists separate themselves from traditional theorists across fields ( Bohman, 2005 ). Critical theories explore how historical events and society have shaped present-day experiences and understandings of how the world functions ( Horkheimer, 1972 ). So, whereas traditional theoretical approaches place the phenomenon of interest at the center of analysis, critical theorists seek to place societal contexts that shape a phenomenon as the focal point. Currently, several critical perspectives—that is, feminist, race, queer, disability, and decolonial theories—are accepted in the critical canon ( Bell, 1995 ; hooks, 2000 ; Watson, 2005 ; Siebers, 2008 ; Mignolo, 2012 ). The common theme in these theoretical perspectives is their assertion that society has produced oppressive structures (e.g., patriarchy, racism, sexism, colonialism, and ableism) that harm those who are not white, cisgendered, male, heterosexual, able-bodied, wealthy, and Western individuals. As a result, there exists the need for society and those in it to interrogate how those oppressive ideologies show up in ourselves and connect to oppressive structures in the world around us. From there, we can begin to imagine new strategies for how to make life better for those who find themselves on the margins ( Bohman, 2005 ; Devetak, 2005 ; hooks, 2000 ).
WHY ARE CRITICAL APPROACHES NEEDED IN STEM EQUITY RESEARCH?
Before we can discuss why a critical approach is needed, we must first define the goal of conducting STEM equity research. Over the past 2 years, we have seen increases in the numbers of people wanting to participate in research practices that focus on equity efforts in STEM, many of whom aim to increase the representation of marginalized and minoritized students in STEM fields for the betterment of our institutions and the U.S. economy ( President’s Council of Advisors on Science and Technology, 2020 ). However, placing the United States and our institutions as the motivations for our work and not the students, who often find themselves pushed out of the fields they once found joy in ( McGee, 2020a ), is a striking concern we must all sit with. We argue that engaging in critical research is an effort to re-center the students, to create safer and healthier environments for them to pursue their passions.
STEM equity researchers must grapple with the historical events that have shaped what STEM fields look like today. STEM environments have led to scientific discoveries and innovation, but these environments also have a history of reproducing systemic inequities that harm individuals ( Graves, 2001 ; Roberts, 2011 ; Wilder, 2013 ; Gholson, 2016 ; Joseph et al. , 2019 ; Saini, 2019 ; Cech and Waidzunas, 2021 ; Reinholz and Ridgway, 2021 ). Additionally, it is important to acknowledge that STEM fields have a history of conducting research, creating theories, and making measurements that primarily centered white, cisgendered, male, heterosexual, able-bodied, wealthy individuals ( Harding, 2006 ). Consequently, the prioritization of individuals from privileged groups in STEM has produced research and policies that are susceptible to the structural inequities and personal biases that have historically harmed and excluded marginalized and minoritized communities.
Thus, we argue that the necessity of integrating critical approaches is directly linked to the need for revision in STEM education. Kiese Laymon, a Black Mississippian writer and professor, notes that “revision is a dynamic practice of revisitation, premised on ethically reimagining the ingredients, scope, and primary audience of one’s initial vision” ( Laymon, 2021 , para. 16). Laymon (2021) also argues that the current racial inequities we see in American society are a product of America’s failure to wrestle with and acknowledge its history of “anti-black terror” (para. 28). These arguments apply to STEM equity research, in which our inability to confront our institutions’ historical legacy of slavery, indigenous erasure, and exclusion of those who were not white, cisgendered, male, heterosexual, able-bodied, wealthy in our research and institutions is tied to the lack of representation we see in STEM fields. We assert that STEM equity researchers must commit to an act of revision in which we reflect on our motivations, historical and societal influences, and research processes in the hopes that we can imagine and work toward a more equitable future.
WHAT DO CRITICAL APPROACHES LOOK LIKE IN QUANTITATIVE RESEARCH?
For the purposes of this essay, we outline how to incorporate critical approaches in quantitative STEM equity research. Quantitative analyses are pivotal tools for examining DEI in STEM, but it is imperative to understand that researchers’ positions in relation to race, gender, socioeconomic status, where they are located within universities, academic training, and other characteristics can shape how they approach data and analyses. Researchers employing quantitative methods within a postpositivist framework tend to eschew acknowledging how the positionality of researchers impacts analytic decision making (Zuberi and Bonilla Silva, 2008; Gillborn et al. , 2018 ; López et al. , 2018 ). Additionally, centering individual demographic variables (e.g., race, gender, and ability) instead of structural inequities positions marginalized and minoritized students as solely responsible for their lack of representation in STEM fields. These unrecognized beliefs can lead to misinterpretations of people’s experiences, which in turn negatively affects campus decision making and policies ( Sultana, 2007 ).
Critical quantitative (QuantCrit) approaches are helpful for those interested in studying ways to improve the experiences of marginalized and minoritized students from a quantitative standpoint. The foundational elements of QuantCrit are tied to critical race theory but are also aligned with other perspectives of the critical canon. Critical race theory explores where and how racism prevents people of color from accessing social and economic opportunities ( Bell, 1995 ; Ladson-Billings, 2009 ). Critical race theorists are also interested in subverting deficit-framing projections by documenting the ways that people of color actively resist and cultivate joy despite racist structures ( Devetak, 2005 ; Harper, 2010 ; Delgado and Stefancic, 2017 ). Relying on critical race theory, QuantCrit theory allows researchers to revise traditional notions of viewing relationships among racial groups as causal, instead seeing them as a reflection of historical and existing structural racism that differentially affects racial and ethnic groups ( Zuberi, 2001 ).
Scholars who use QuantCrit: 1) grapple with the historical and present-day reality of racism; 2) recognize how the practice of naively using statistics can uphold white supremacy (e.g., achievement gaps); 3) interrogate how social categorizations such as race and ethnicity are varied, contested, and fluid over time ( Omi and Winant, 2015 ) and how these shifts can impact analyses and interpretations; 4) integrate the voices of racially marginalized and minoritized individuals through qualitative and mixed-methods approaches to account for limitations in quantitative interpretations; and 5) embrace research methods, including quantitative approaches, to pursue equity goals that align with a social justice liberatory agenda (see Gillborn et al. , 2018 ). Recently, those who employ QuantCrit approaches have begun to extend these tenets beyond focusing solely on racism, incorporating how individuals are impacted by overlapping structural inequities ( Crenshaw, 1989 ; Collins, 2000 ; Jang, 2018 ). Accordingly, using QuantCrit approaches provides researchers with the ability to use statistical practices as an analytical tool for improving the social conditions of marginalized and minoritized populations.
CRITICAL QUESTIONS: INTEGRATING QUANTCRIT APPROACHES IN STEM EQUITY ANALYSES
As STEM equity researchers who were originally trained in a postpositivist paradigm, we understand firsthand that learning how to be a QuantCrit researcher is difficult. As described, STEM fields have a history of pushing out marginalized and minoritized students ( Gholson, 2016 ; Joseph et al. , 2019 ; McGee, 2020a , b ; Cech and Waidzunas, 2021 ; Reinholz and Ridgway, 2021 ) and using harmful statistical approaches that contribute to negative perceptions of students ( Zuberi, 2001 ; Zuberi and Bonilla-Silva, 2008 ). Instead of repeating harm, we as STEM education researchers can revise our paradigm to reflect more equity-centered approaches. Relying on Gutiérrez’s (2013) sociopolitical framework and critical perspectives ( Crenshaw, 1989 ; Bohman, 2005 ; Cooper, 2018 ), we define equity as the process of reckoning with how historical events have shaped and continue to reinforce unequal power imbalances in a given context and actively working to dismantle those power imbalances so that society can restructure itself to better sustain and empower all. Importantly, this definition emphasizes continual adaptation as a goal of equity in order to accommodate changing perspectives of how we understand power, inequality, and injustice in our work.
Gutiérrez (2002) similarly argues that equity is a process, rather than a static goal, reflective of individual, institutional, and societal processes. As institutions and fields evolve through space (i.e., geographic location, institution, and classroom) and time, there will always be a need to reimagine new equitable practices. Therefore, here, we use the structure of questions rather than asserting definitive guidelines to follow, reifying our commitment to equity as a process with no universal, one-size-fits-all approach to equity analyses. These questions can assist researchers in adjusting their methodological approaches to the contexts of the educational environments in their studies as well as in delineating which data are collected and selected for analyses. To this end, imperfection and improvement represent the norm of equity analyses and can provide clarifications with each iteration. As a result, we advise that researchers use the following eight questions (summarized in Table 1 ) as self-reflective tools, rather than as an exhaustive list of questions to consider with every analysis.
Critical questions: A guide to integrating critical approaches in STEM equity quantitative analyses
How Does Lived Experience Affect How One Approaches Research?
The lenses through which researchers view the world are influenced by their lived experiences accumulated through a multitude of interpersonal interactions and exposure to and engagement with different research perspectives, methodologies, and theories. When using quantitative approaches, researchers often implicitly regard themselves as objective observers, with numbers viewed as neutral ( Guba and Lincoln, 1994 ). This practice becomes especially concerning in social and educational research, as scholars have uncovered how numbers and data have been used to reinforce social inequities. For instance, Zuberi and Bonilla-Silva (2008) highlight how “statistical analysis was developed to explain the racial inferiority of colonial and second-class citizens in the new imperial era” (p. 5). These harmful statistical practices still permeate education research today, with researchers studying achievement gaps in higher education without adequate explanation of structural barriers ( Ladson-Billings, 2006 ; Gutiérrez, 2008 ). It is important to realize that researchers are human beings who are situated in societal contexts that privilege some groups over others ( Bohman, 2005 ). As a result, researchers are prone to have conscious and unconscious biases that influence the development of research questions and decision-making practices for measurement and analyses ( Harding, 1992 ).
A common practice in qualitative research is to write positionality statements ( Secules et al. , 2021 ). In these statements, researchers discuss how their backgrounds and experiences impacted their academic trajectories and relationships to research. Harding (1992) argues that being upfront about one’s biases, values, and experiences reflects “strong objectivity,” because it allows the audience to understand how a researcher’s lived experience and personal biases might impact the study. Given that positionality statements are not a common practice in quantitative research, we understand that researchers might be hesitant to include them in their work. However, a core component of being a critical scholar is constantly reflecting on how society influences one’s view of the world and in return how one chooses to do research ( Guba and Lincoln, 1994 ). By grappling with their positionality, researchers can better understand the strengths and limitations of their lenses and thus their work. Before and throughout the research process, we advise that researchers take time to reflect on, and perhaps write about, their positionality. Additionally, researchers should assess whether their lived experiences or academic training prepared them to conduct their research.
What Theoretical Assumptions Are Present in Conceptualizations of Equity Practices?
Before analyzing data, researchers should first assess their definitions of equity. Rodriguez et al. (2012) outlined, for example, three different equity models using the language of parity, fairness, and individuality. Equity models based on parity focus on getting minoritized students to obtain similar levels of academic success as majority groups members, and equity models based on fairness aim to get different groups of students to achieve similar levels of progress on tasks and assignments ( Rodriguez et al. , 2012 ). An inherent problem in these models is the assumption that academic success is contingent upon the behaviors and beliefs of majority groups. The equity of parity model also does not account for the historical trauma and discrimination that has hindered marginalized and minoritized students’ academic success in STEM programs. Individual students have their own sets of privileges and disadvantages that influence their needs and experiences in STEM learning environments. Therefore, STEM equity models should accept that conceptualizations of equity will vary across groups and situations, and not neatly align with cut-and-dried societal hierarchies.
Rodriguez et al. (2012) advocate for researchers to use equity models of individuals in which researchers attend to the factors that have harmed marginalized and minoritized students’ access to STEM fields and develop conceptualizations of success for each individual group. Going further, Gutiérrez (2013) argues that focusing on individual groups is not enough, rather that equitable practices within STEM contexts must contend with the ways that identity and power manifest in our courses and institutions. First, Gutiérrez (2013) describes how equity for an identity group can fluctuate depending on the context and time frame. As a result, researchers should unpack their justifications for focusing on a specific identity group when conceptualizing equity. For example, researchers will explain that they are studying an identity group (e.g., women or students of color) due to their lack of representation in STEM fields. However, each institution, department, and classroom has its own set of historical origins and structural factors that have shaped the present-day experiences for each identity group. Equity models should describe and embrace these variations ( Hancock, 2007 ).
Additionally, Gutiérrez (2013) comments on how STEM skill sets (e.g., quantitative literacy) are perceived as necessary tools for professional and personal development, creating a system in which individuals who fail to adopt these skill sets are rendered less valuable. These ideological assumptions shape STEM learning spaces as sociopolitical institutions wherein marginalized and minoritized students are blamed for their lack of representation without addressing systemic inequities. Thus, when focusing on ways to center equity in STEM analyses, we suggest that researchers avoid using language that solely focuses on marginalized and minoritized students in relation to their academic outcomes and more so on how their educational experiences are shaped by history, power, and context.
What Analytical and Interpretive Choices Can Be Made to Focus on Excellence?
A popular practice in STEM equity research is to observe the achievement gaps existing between majority and marginalized and minoritized students ( Gouvea, 2021 ). A wealth of research explores how students who belong to underserved racial, gender, ability, and socioeconomic groups underperform academically in comparison to their privileged counterparts (e.g., Bastedo and Jaquette, 2011 ; Matz et al. , 2017 ; Whitcomb and Singh, 2021 ). Focusing solely on gaps is harmful, because it centers students’ identities as the reasons behind their academic failures. Additionally, the research on achievement gaps over the years has not substantially improved the experiences of marginalized and minoritized students in STEM; in fact, research has shown that overreliance on the documentation of these gaps has contributed to negative societal constructions of the academic abilities of students from minoritized backgrounds ( Gutiérrez, 2008 ; Martin, 2012 ). Embracing critical approaches in STEM equity research necessitates that researchers use proactive approaches wherein efforts are pushed toward addressing what institutions can do to better support students.
Rather than framing analyses with gaps, those conducting equity analyses should focus on how different factors positively relate to advancements, gains, and excellence of students ( Gutiérrez, 2008 ; Harper, 2010 ). For example, research shows that LGBTQ+ students experience fewer stressors when they attend colleges that have academic studies, policies, and student clubs supportive of LGBTQ+ individuals ( Woodford et al. , 2018 ). By shifting the onus to institutional components, Woodford et al. (2018) showcase how university programs and policies are directly tied to the success of LGBTQ+ students. Practitioners can use these findings to create supportive institutional and classroom environments for LGBTQ+ students.
We further caution against excellence-based approaches that solely center grades or degree attainment. Despite increases in STEM degrees conferred to racially minoritized students, there still exists a lack of representation in STEM fields ( Fry et al. , 2021 ). Furthermore, the financial and psychological burdens that racially marginalized and minoritized students report while enrolled in and after college suggests that “success” postgraduation is not equitable across all groups ( Keels et al ., 2017 ; Davis et al. , 2020 ; McGee, 2020b ). Students of color often conceptualize “success” as tied to their ability to give back to their communities, which is different from traditional conceptualizations of success ( McGee and Martin, 2011 ; Pérez Huber et al. , 2018 ; Lopez, 2020 ; McGee, 2020a ). As a result, we recommend that researchers adopt definitions of excellence within STEM contexts based on the conceptualizations of their population of interest and then use those definitions in analyses ( Pérez Huber et al. , 2018 ; Weatherton and Schussler, 2021 ).
What Theoretical Linkages Exist between the Constructs and Demographic Variables of Interest?
Too often, quantitative STEM equity analyses are conducted with a “kitchen sink” approach in which full combinatorics are used to study intersections of student constructed identities. Including several independent demographic variables without adequately accounting for past research or theoretical linkages among them hinders the interpretation of research findings. How and why these demographic variables are used in analyses impacts conversations about what inequities look like, for whom, and what should be done.
Recently, scholars have begun to discuss the need for a “race re-imaging” wherein commonly used measures such as motivation or institutional support are re-evaluated and adapted to fit the lived experiences of racially marginalized and minoritized experiences historically left out of educational psychology research ( DeCuir-Gunby and Schutz, 2014 ; Lopez, 2020 ; Matthews and López, 2020 ). Research indicates that racially marginalized and minoritized students’ value in STEM is strengthened through their ability to understand how STEM educational skill sets can uplift their communities ( McGee and Bentley, 2017 ; Gray et al. , 2020 ), countering the individualistic culture of STEM learning environments ( Battey and Leyva, 2016 ; Carter, 2017 ). Therefore, STEM utility and motivational measures that ignore social justice and community engagement may miss out on the ways that STEM and racial identities intersect ( McGee, 2020a ; Miller-Cotto and Lewis, 2020 ). As STEM equity researchers, we can apply these ideals to questions of pre-existing assumptions we may hold about the relationships between our constructs of interest and different demographic variables (i.e., gender, socioeconomic status, ability, and sexuality) selected for our studies before running analyses. Then, if deficit-based theoretical linkages emerge, we recommend researchers find outside studies promoting strength-based approaches or adopt qualitative or mixed approaches that can better speak to the associations between the demographic variables and constructs of interest.
What Should Be Considered when Using Standardized Test Scores as a Metric for “Prior Preparation”?
Standardized test scores (ACT/Scholastic Aptitude Test [SAT]) must be incorporated cautiously considering who is and has been most likely to do well on them given structural inequalities that privilege certain families over others ( Rothstein, 2004 ; Soares, 2007 ; Zwick, 2013 ; Carnevale et al. , 2020 ). As researchers focused on equity, we must acknowledge the racist origins of standardized assessments. In the early 1900s, standardized assessments were intentionally used by eugenicists as justifications for racial purity in American educational systems ( Lemann, 2000 ; Harris et al. , 2011 ; Soares, 2007 ). Today, standardized assessments are still used in admissions decisions and placement into undergraduate STEM courses, even though research has shown that they are weak and inadequate predictors of college retention for racially minoritized students ( Sedlacek, 2004 ). Although many institutions have either modified admissions policies to be test optional or completely eliminated standardized tests in admissions review due to the impact of the COVID-19 pandemic, how long institutions will continue with such policies and what possible alternative assessments may be used in place of ACT and SAT test scores remains to be seen. Further, students who come from economically privileged families have access to high schools and test preparation resources that increase their chances of doing well on standardized assessments. The economic privileges continue once these students enter higher education ( Borg et al. , 2012 ; Carnevale et al. , 2020 ). As a result, causal linkages between standardized assessments and degree attainment generally fail to account for wealth as a confounding variable. Therefore, we encourage the use of other metrics to capture the academic preparation of students.
College course grades and high school grade point average (GPA), while also imperfect measures, are stronger predictors for student adjustment and success in college over standardized test scores ( Byrd et al. , 2014 ; Koester et al. , 2016 ; Galla et al. , 2019 ). Unlike standardized assessment scores, a student’s high school course grades and college course work better reflect the level of mastery for a given subject. Additionally, researchers could consider using Advancement Placement (AP) scores. The AP program provides high school students with the chance to engage in college-level curricula ( Kolluri, 2018 ). Research has shown that passing AP tests is related to positive college outcomes across students from different racial and socioeconomic backgrounds ( Dougherty et al. , 2006 ; Fischer et al. , 2021 ). It is important to note that even these metrics are not perfect indicators of academic preparation, given the intersecting inequalities that exist in K–12 educational systems (see Lewis and Diamond, 2015 ). Therefore, researchers should account for the current limitations that exist in how we assess students’ prior academic preparation.
What Measures Capture Structural Inequalities That Exist in STEM Higher Education?
Equity analyses that only use individual-level variables provide great insight into how academic outcomes vary across different social groups. However, interpretations that come from these types of analyses often place sole responsibility on minoritized and marginalized students to persevere through systemic barriers ( McGee, 2020a ). Using complex multilevel models, researchers have assessed the impacts of various structural components on student outcomes, such as campus and classroom climate, policies, and institutional characteristics (e.g., selectivity and public vs. private status; Espinosa, 2011 ; Leath and Chavous, 2018 ; Ohland et al. , 2018). For example, Espinosa (2011) found that women of color who attended private colleges were more likely than their peers enrolled at public institutions to persist in their STEM programs. Espinosa (2011) attributes the positive effect of private institutions to the large amounts of educational resources available that counteract a lack of academic preparation among women of color. Espinosa (2011) further showcases that experiences for women of color vary based on the STEM contexts in which they are situated. In contrast, Leath and Chavous (2018) show that Black women enjoy college less when they feel like they must conceal their racial and ethnic identity. Leath and Chavous (2018) demonstrate how tumultuous racial climates contribute to Black women’s college experiences. These studies allow researchers to gain insight into the underlying mechanisms and structural components (e.g., type of college and racist campus climate) that contribute to student experiences, persistence, and success. Also, these researchers illustrate a story in which the institution is held accountable for variations in student outcomes ( Hancock, 2007 ).
There are, however, limitations to these approaches. For one, institutional climate measures may aggregate students’ perceptions about how well students from different backgrounds get along with one another. Although these measures are reflective of structural components, they still rely on individual-level perceptions and do not generally account for different conceptualizations of climate across social groups. Second, multilevel models require large sample sizes at the individual level to maintain statistical power ( Snijders, 2005 ), but researchers attempting to collect information from a diverse set of participants are often blocked by a lack of financial resources and time ( Hancock, 2007 ). As a result, researchers may be forced to make difficult analytical decisions like aggregating multiple social groups together (e.g., combining non-white students) that gloss over the variation between different groups of students and hinder our understanding of how structural inequalities on campus, and in STEM programs specifically, differentially impact their experiences and outcomes. Additionally, because STEM equity researchers often find themselves working with institutional data, individual variables may be the only ones available, and as we describe in more detail later, may not include documentation about how these data were collected, which can influence modeling strategies and subsequent interpretations.
As a result, we emphasize that models based only on individual-level variables (e.g., race, gender, and ability) can only suggest variations across existing groups; they are not explanations for the underlying mechanisms that influence these variations. Ultimately, much work remains in delineating the best practices for integrating structural features into analyses and appropriately contextualizing them within STEM equity research. In the meantime, we recommend that researchers do their best to incorporate structural components in analyses wherever possible.
How Do Changes in Institutional Categories for Demographic Variables over Time Affect Analyses?
When working with institutional data, researchers may need to track how their institutions’ social categories have changed over time ( Viano and Baker, 2020 ; Byrd, 2021 ). Categorizations like gender, race and ethnicity, income, and parental education are not fixed; these categories fluctuate over time, even if slowly. As a broad example, the race and ethnicity categories on the U.S. census that inform data collection across society have changed with every census administration ( Brown, 2020 ). At one time, for example, Irish immigrants were not viewed as “white” due to a few factors including their socioeconomic position and religious beliefs, but as the Irish gained economic mobility in a deepening Jim Crow era, their ascension to whiteness was solidified in the United States ( Omi and Winant, 2015 ). Here we see that race is not static, but a by-product of social and political change.
In addition to race and ethnicity, gender categorizations in the United States have also evolved, with social surveys moving beyond binary conceptualizations and shifting toward more gender-inclusive (i.e., transgender, gender non-conforming, nonbinary) categories ( Westbrook and Saperstein, 2015 ). Indeed, such variation has always existed ( D’Ignazio and Klein, 2020 ), and it is important to note that individual perceptions of social categorizations are also subject to change ( Freeman et al. , 2011 ). Similarly, sometimes the same information is collected about students in multiple contexts (e.g., when both the financial aid and registrar’s office have information about students’ first- or continuing-generation status). Identifying areas of discordance from different data-collection mechanisms over time can more properly contextualize analyses, particularly when merging multiple data sets for the same students.
Consequently, when working with secondary data sources, we recommend that researchers seek to obtain information about how social categorizations were solicited and defined as well as how they may have changed over time. Including this information in studies, even if only as supplemental material, will help to produce research that is better contextualized. Researchers should also reach out to campus offices that maintain and analyze student-level data for additional student information that may not be included in existing data sets to improve clarity about how groups are constructed and how this might influence analyses and interpretations.
Are Quantitative Analyses the Best Tools for Answering the Proposed Research Questions?
Using qualitative and mixed-methods approaches, scholars have provided in-depth commentary on the ways that systemic inequities have shaped marginalized and minoritized students’ experiences in STEM contexts ( McGee and Bentley, 2017 ; Allaire, 2019 ). At the same time, the rise of big data has encouraged educational systems to examine the experiences of marginalized and minoritized students at the macro level ( Daniel, 2019 ). Although quantitative approaches offer unique benefits, some research questions require alternative approaches to better capture the lived experiences of minoritized students ( Covarrubias, 2011 ). For example, Jack (2019) studied the experiences of low-income students at elite institutions, showing that those who graduated from private high schools were able to navigate elite institutions better than their low-income peers who attended public high schools in their communities. A common practice in educational research tends to clump the experiences of low-income students together when studying inequity. Jack (2019) demonstrates how qualitative research has the power to capture variations within groups not easily noticeable when groups are combined in quantitative analyses. Therefore, before conducting analyses, we recommend that researchers first identify the main goals of a research project and assess whether quantitative analyses are most applicable and viable given the data available or to be collected, regardless of sample size.
Although higher education has contributed to the advancement of society, our institutions have also participated in creating and reproducing systemic inequities ( Patton, 2016 ). Our institutions, as well as the research community, can and should play a role in making the experiences of all students more equitable by first examining, with the students themselves, what those experiences are that can inform campus decision making. The misuse of quantitative data in STEM equity analyses can, even when unintended, reinforce deficit interpretations about marginalized and minoritized students and mask the role of systemic inequities. Integrating critical approaches in STEM equity analyses can provide insight into how institutions bear responsibility for the lack of diversity, representation, and differential experiences in STEM fields reflecting an unequal opportunity structure on our campuses.
In this essay, we aimed to inspire those conducting STEM equity research from a quantitative perspective to commit to an act of revision ( Laymon, 2021 ). As researchers in STEM education, we understand that career success is often dependent upon one’s ability to adopt beliefs that research and numbers are objective. We also know that researchers are encouraged to search for “silver bullets” or universal approaches in their work. In fact, we still fail at upholding all of the recommendations we have offered. However, understanding the value that statistical practices have in equity policy initiatives, we are committed to working through present-day limitations that come with the quantification of human experiences. By being upfront where our work falls short, we get closer to discovering new analytical approaches that can be used for liberatory purposes. Finally, we hope to contribute to a critical discourse and prompt reflection in an effort to make a meaningful impact that ultimately promotes equity and inclusion on our campuses and in STEM fields.
Acknowledgments
We thank Juniar Lucien for organizational contributions and insightful feedback. Additionally, this work was supported by the SEISMIC project (seismicproject.org), which is funded by the Alfred P. Sloan Foundation. SEISMIC is managed at the University of Michigan for the participating institutions, which include Arizona State University, Indiana University, Michigan State University, Purdue University, University of California Davis, University of California Irvine, University of California Santa Barbara, University of Michigan, University of Minnesota, and University of Pittsburgh.
1 For the purposes of this paper, we define minoritized and marginalized students as those who belong to identity groups that have been impacted by structural inequities (e.g., racism, sexism, and ableism) and are less represented in STEM in comparison to the American population ( National Science Foundation, 2021 ).
- Ahmed, S. (2012). On being included: Racism and diversity in institutional life . Durham, NC: Duke University Press. [ Google Scholar ]
- Alcendor, D. J. (2020). Racial disparities-associated COVID-19 mortality among minority populations in the US . Journal of Clinical Medicine , 9 ( 8 ), 2442. [ PMC free article ] [ PubMed ] [ Google Scholar ]
- Allaire, F. S. (2019). Navigating uncharted waters: First-generation native Hawaiian college students in STEM . Journal of College Student Retention: Research, Theory & Practice , 21 ( 3 ), 305–325. [ Google Scholar ]
- Asai, D. J. (2020). Race matters . Cell , 181 ( 4 ), 754–757. 10.1016/j.cell.2020.03.044 [ PubMed ] [ CrossRef ] [ Google Scholar ]
- Bastedo, M. N., Jaquette, O. (2011). Running in place: Low-income students and the dynamics of higher education stratification . Educational Evaluation and Policy Analysis , 33 ( 3 ), 318–339. [ Google Scholar ]
- Battey, D., Leyva, L. A. (2016). A Framework for understanding whiteness in mathematics education . Journal of Urban Mathematics Education , 9 ( 2 ), 49–80. [ Google Scholar ]
- Bell, D. A. (1995). Who’s afraid of critical race theory? University of Illinois Law Review , 1995 ( 4 ), 893–910. [ Google Scholar ]
- Bohman, J. (2005). Critical theory . In Zalta, E. N. (Ed.), Stanford Encyclopedia of Philosophy (Spring 2021 Edition) . Retrieved May 15, 2021, from https://plato.stanford.edu/archives/spr2021/entries/critical-theory/
- Borg, J. R., Borg, M. O., Stranahan, H. A. (2012). Closing the achievement gap between high-poverty schools and low-poverty schools . Research in Business and Economics Journal , 5 , 1–24. [ Google Scholar ]
- Brown, A. (2020, February 25). The changing categories the U.S. census has used to measure race . Fact Tank: News in the Numbers . Retrieved May 15, 2021, from www.pewresearch.org/fact-tank/2020/02/25/the-changing-categories-the-u-s-has-used-to-measure-race
- Byrd, W. C. (2021). Behind the diversity numbers: Achieving racial equity on campus . Cambridge, MA: Harvard Education Press. [ Google Scholar ]
- Byrd, W. C., Brunn-Bevel, R. J., Sexton, P. R. (2014). “We don’t all look alike”: The academic performance of Black students at elite colleges . Du Bois Review: Social Science Research on Race , 11 ( 2 ), 353–385. [ Google Scholar ]
- Carnevale, A. P., Schmidt, P., Strohl, J. (2020). The merit myth: How our colleges favor the rich and divide America . New York, NY: New Press. [ Google Scholar ]
- Carter, L. (2017). Neoliberalism and STEM education: Some Australian policy discourse . Canadian Journal of Science, Mathematics and Technology Education , 17 ( 4 ), 247–257. [ Google Scholar ]
- Cech, E. A., Waidzunas, T. J. (2021). Systemic inequalities for LGBTQ professionals in STEM . Science Advances , 7 ( 3 ), 1–9. [ PMC free article ] [ PubMed ] [ Google Scholar ]
- Collins, F. S., Adams, A. B., Aklin, C., Archer, T. K., Bernard, M. A., Boone, E., ... & Wolinetz, C. (2021). Affirming NIH’s commitment to addressing structural racism in the biomedical research enterprise . Cell , 184 ( 12 ), 3075–3079. 10.1016/j.cell.2021.05.014 [ PubMed ] [ CrossRef ] [ Google Scholar ]
- Collins, P. H. (2000). Black feminist thought: Knowledge, consciousness, and the politics of empowerment . New York, NY: Routledge. [ Google Scholar ]
- Cooper, B. (2018). Intersectionality . In Disch, L., Hawkesworth, M. (Eds.), The Oxford handbook of feminist theory (pp. 385–406). New York, NY: Oxford University Press. [ Google Scholar ]
- Covarrubias, A. (2011). Quantitative intersectionality: A critical race analysis of the Chicana/o educational pipeline . Journal of Latinos and Education , 10 ( 2 ), 86–105. [ Google Scholar ]
- Crenshaw, K. (1989). Demarginalizing the intersection of race and sex: A black feminist critique of antidiscrimination doctrine, feminist theory and antiracist politics . University of Chicago Legal Forum , 1989 , 139–167. [ Google Scholar ]
- Daniel, B. K. (2019). Big Data and data science: A critical review of issues for educational research . British Journal of Educational Technology , 50 ( 1 ), 101–113. [ Google Scholar ]
- Davis, C. H. F., III, Mustaffa, J. B., King, K., Jama, A. (2020). Legislation, policy, and the Black student debt crisis . Washington, DC: National Association for the Advancement of Colored People. [ Google Scholar ]
- DeCuir-Gunby, J. T., Schutz, P. A. (2014). Researching race within educational psychology contexts . Educational Psychologist , 49 ( 4 ), 244–260. [ Google Scholar ]
- Delgado, R., Stefancic, J. (2017). Critical race theory: An introduction . New York, NY: NYU Press. [ Google Scholar ]
- Devetak, R. (2005). Critical theory . Theories of International Relations , 3 , 137–160. [ Google Scholar ]
- D’Ignazio, C., Klein, L. F. (2020). Data feminism . Cambridge, MA: MIT Press. [ Google Scholar ]
- Dougherty, C., Mellor, L., Jian, S. (2006). The relationship between Advanced Placement and college graduation (2005 AP Study Series, Report 1) . Austin, TX: National Center for Educational Accountability. [ Google Scholar ]
- Egbuonu, O. (Producer, director, & writer). (2020, June 26). Breonna Taylor and George Floyd both deserve justice. But justice for Black women is elusive . NBCNews.com. Retrieved June 26, 2021, from www.nbcnews.com/think/opinion/breonna-taylor-george-floyd-both-deserve-justice-justice-black-women-ncna1232190
- Espinosa, L. (2011). Pipelines and pathways: Women of color in undergraduate STEM majors and the college experiences that contribute to persistence . Harvard Educational Review , 81 ( 2 ), 209–241. [ Google Scholar ]
- Fischer, C., Witherspoon, E., Nguyen, H., Feng, Y., Fiorini, S., Vincent-Ruz, P., ... & Schunn, C. D., (2021, June 9). Advanced Placement course credit and undergraduate student success in gateway science courses (OSF Preprints) . 10.31219/osf.io/6zhqw [ CrossRef ]
- Freeman, J. B., Penner, A. M., Saperstein, A., Scheutz, M., Ambady, N. (2011). Looking the part: Social status cues shape race perception . PloS ONE , 6 ( 9 ), e25107. [ PMC free article ] [ PubMed ] [ Google Scholar ]
- Fry, R., Kennedy, B., Funk, C. (2021). April 1). STEM jobs see uneven progress in increasing gender and ethnic diversity . Retrieved May 15, 2021, from www.pewresearch.org/science/2021/04/01/stem-jobs-see-uneven-progress-in-increasing-gender-racial-and-ethnic-diversity/
- Galla, B. M., Shulman, E. P., Plummer, B. D., Gardner, M., Hutt, S. J., Goyer, J. P., … & Duckworth, A. L. (2019). Why high school grades are better predictors of on-time college graduation than are admissions test scores: The roles of self-regulation and cognitive ability . American Educational Research Journal , 56 ( 6 ), 2077–2115. [ Google Scholar ]
- Gholson, M. L. (2016). Clean corners and algebra: A critical examination of the constructed invisibility of Black girls and women in mathematics . Journal of Negro Education , 85 ( 3 ), 290–301. [ Google Scholar ]
- Gillborn, D., Warmington, P., Demack, S. (2018). QuantCrit: Education, policy, “Big Data” and principles for a critical race theory of statistics . Race Ethnicity and Education , 21 ( 2 ), 158–179. 10.1080/13613324.2017.1377417 [ CrossRef ] [ Google Scholar ]
- Gouvea, J. S. (2021). Antiracism and the problems with “achievement gaps” in STEM education . CBE—Life Sciences Education , 20 ( 1 ), fe2. [ PMC free article ] [ PubMed ] [ Google Scholar ]
- Gover, A. R., Harper, S. B., Langton, L. (2020). Anti-Asian hate crime during the COVID-19 pandemic: Exploring the reproduction of inequality . American Journal of Criminal Justice , 45 ( 4 ), 647–667. 10.1007/s12103-020-09545-1 [ PMC free article ] [ PubMed ] [ CrossRef ] [ Google Scholar ]
- Graves, J. L. Jr. (2001). The emperor’s new clothes: Biological theories of race at the millennium . New Brunswick, NJ: Rutgers University Press. [ Google Scholar ]
- Gray, D. L., McElveen, T. L., Green, B. P., Bryant, L. H. (2020). Engaging Black and Latinx students through communal learning opportunities: A relevance intervention for middle schoolers in STEM elective classrooms . Contemporary Educational Psychology , 60 , 101833. [ Google Scholar ]
- Griffin, K. A., Baker, V. L., O’Meara, K. (2020). Doing, caring, and being: “Good” mentoring and its role in the socialization of graduate students of color in STEM . In Weidman, J. C., DeAngelo, L. (Eds.), Socialization in higher education and the early career: Theory, research and application (pp. 223–239). Cham, Switzerland: Springer. [ Google Scholar ]
- Guba, E. G., Lincoln, Y. S. (1994). Competing paradigms in qualitative research . In Denzin, N. K., Lincoln, Y. S. (Eds.), Handbook of qualitative research (pp. 105–117). Thousand Oaks, CA: Sage. [ Google Scholar ]
- Gutiérrez, R. (2002). Enabling the practice of mathematics teachers in context: Toward a new equity research agenda . Mathematical Thinking and Learning , 4 ( 2–3 ), 145–187. [ Google Scholar ]
- Gutiérrez, R. (2008). Research commentary: A gap-gazing fetish in mathematics education? Problematizing research on the achievement gap . Journal for Research in Mathematics Education , 39 ( 4 ), 357–364. 10.5951/jresematheduc.39.4.0357 [ CrossRef ] [ Google Scholar ]
- Gutiérrez, R. (2013). The sociopolitical turn in mathematics education . Journal for Research in Mathematics Education , 44 ( 1 ), 37–68. 10.5951/jresematheduc.44.1.0037 [ CrossRef ] [ Google Scholar ]
- Hancock, A.-M. (2007). When multiplication doesn’t equal quick addition: Examining intersectionality as a research paradigm . Perspectives on Politics , 5 ( 1 ), 63–79. [ Google Scholar ]
- Harding, S. (1992). Rethinking standpoint epistemology: What is “strong objectivity”? Centennial Review , 36 ( 3 ), 437–470. [ Google Scholar ]
- Harding, S. (2006). Science and social inequality: Feminist and postcolonial issues . Urbana, IL: University of Illinois Press. [ Google Scholar ]
- Harper, S. R. (2010). An anti-deficit achievement framework for research on students of color in STEM . New Directions for Institutional Research , 2010 ( 148 ), 63–74. 10.1002/ir.362 [ CrossRef ] [ Google Scholar ]
- Harris, P., Smith, B. M., Harris, J. (2011). The myths of standardized tests: Why they don’t tell you what you think they do . Lanham, MD: Rowman & Littlefield. [ Google Scholar ]
- Heinecke, W. F., Beach, S. (2020). Social Justice Interventions in Higher Education . In Mullen, C. A. (Ed.), Handbook of social justice interventions in education (pp. 1–25). Cham, Switzerland: Springer International Publishing. 10.1007/978-3-030-29553-0_115-1 [ CrossRef ] [ Google Scholar ]
- hooks, b. (2000). Feminist theory: From margin to center . Boston, MA: South End Press. [ Google Scholar ]
- Horkheimer, M. (1972). Critical theory: Selected essays (Translated by O’Connell, M. J., & others). New York, NY: Continuum. [ Google Scholar ]
- Jack, A. A. (2019). The privileged poor: How elite colleges are failing disadvantaged students . Cambridge, MA: Harvard University Press. [ Google Scholar ]
- Jang, S. T. (2018). The implications of intersectionality on Southeast Asian female students’ educational outcomes in the United States: A critical quantitative intersectionality analysis . American Educational Research Journal , 55 ( 6 ), 1268–1306. [ Google Scholar ]
- Johnson, M. (2020). Undermining racial justice: How one university embraced inclusion and inequality . Ithaca, NY: Cornell University Press. [ Google Scholar ]
- Joseph, N. M., Hailu, M. F., Matthews, J. S. (2019). Normalizing Black girls’ humanity in mathematics classrooms . Harvard Educational Review , 89 ( 1 ), 132–155. [ Google Scholar ]
- Keels, M., Durkee, M., Hope, E. (2017). The psychological and academic costs of school-based racial and ethnic microaggressions . American Educational Research Journal , 54 ( 6 ), 1316–1344. [ Google Scholar ]
- Koester, B. P., Grom, G., McKay, T. A. (2016). Patterns of gendered performance difference in introductory STEM courses . Retrieved from arXiv:1608.07565 [Physics].
- Kolluri, S. (2018). Advanced Placement: The dual challenge of equal access and effectiveness . Review of Educational Research , 88 ( 5 ), 671–711. [ Google Scholar ]
- Ladson-Billings, G. (2006). From the achievement gap to the education debt: Understanding achievement in US schools . Educational Researcher , 35 ( 7 ), 3–12. [ Google Scholar ]
- Ladson-Billings, G. (2009). Critical race theory in education . In Apple, M. W., Au, W., Gandin, L. A. (Eds.), Routledge international handbook of critical education (pp. 110–122). New York, NY: Routledge. [ Google Scholar ]
- Laymon, K. (2021, May 17). What we owe and are owed: Kiese Laymon on Black revision, repayment, and renewal . Vox . Retrieved September 16, 2021, from www.vox.com/the-highlight/22419450/kiese-laymon-justice-fairness-black-america [ Google Scholar ]
- Leath, S., Chavous, T. (2018). Black women’s experiences of campus racial climate and stigma at predominantly White institutions: Insights from a comparative and within-group approach for STEM and non-STEM majors . Journal of Negro Education , 87 ( 2 ), 125–139. [ Google Scholar ]
- Lemann, N. (2000). The big test: The secret history of the American meritocracy . New York, NY: Farrar, Straus and Giroux. [ Google Scholar ]
- Lewis, A. E., Diamond, J. B. (2015). Despite the best intentions: How racial inequality thrives in good schools . New York, NY: Oxford University Press. [ Google Scholar ]
- Li, Y., Wang, K., Xiao, Y., Froyd, J. E. (2020). Research and trends in STEM education: A systematic review of journal publications . International Journal of STEM Education , 7 ( 1 ), 1–16. 10.1186/s40594-020-00207-6 [ CrossRef ] [ Google Scholar ]
- Lopez, J. D. (2020). Examining construct validity of the scale of Native Americans giving back . Journal of Diversity in Higher Education , 14 ( 4 ), 513–529. [ Google Scholar ]
- López, N., Erwin, C., Binder, M., Chavez, M. J. (2018). Making the invisible visible: Advancing quantitative methods in higher education using critical race theory and intersectionality . Race Ethnicity and Education , 21 ( 2 ), 180–207. 10.1080/13613324.2017.1375185 [ CrossRef ] [ Google Scholar ]
- Martin, D. B. (2012). Learning mathematics while Black . Educational Foundations , 26 , 47–66. [ Google Scholar ]
- Matthews, J. S., López, F. (2020). Race-reimaging educational psychology research: Investigating constructs through the lens of race and culture , Contemporary Educational Psychology , 61 , 1–7. 10.1016/j.cedpsych.2020.101878 [ CrossRef ] [ Google Scholar ]
- Matz, R. L., Koester, B. P., Fiorini, S., Grom, G., Shepard, L., Stangor, C. G., … & McKay, T. A. (2017). Patterns of gendered performance differences in large introductory courses at five research universities . AERA Open , 3 ( 4 ), 2332858417743754. [ Google Scholar ]
- McGee, E. O. (2020a). Black, brown, bruised: How racialized STEM education stifles innovation . Cambridge, MA: Harvard Education Press. [ Google Scholar ]
- McGee, E. O. (2020b). Interrogating structural racism in STEM higher education . Educational Researcher , 49 ( 9 ), 633–644. [ Google Scholar ]
- McGee, E. O., Bentley, L. (2017). The troubled success of Black women in STEM . Cognition and Instruction , 35 ( 4 ), 265–289. [ Google Scholar ]
- McGee, E. O., Martin, D. B. (2011). “You would not believe what I have to go through to prove my intellectual value!” Stereotype management among academically successful Black mathematics and engineering students . American Educational Research Journal , 48 ( 6 ), 1347–1389. [ Google Scholar ]
- Mignolo, W. D. (2012). Local histories/global designs . Princeton, NJ: Princeton University Press. [ Google Scholar ]
- Miller-Cotto, D., Lewis, N. Jr. (2020, August 6). Am I a “math person”? How classroom cultures shape math identity among Black and Latinx students . OSF Preprints , 10.31219/osf.io/hcqst [ CrossRef ]
- National Science Foundation, National Center for Science and Engineering Statistics. (2021). Women, minorities, and persons with disabilities in science and engineering (Special report NSF 21–321) . Alexandria, VA. Retrieved May 15, 2021, from https://ncses.nsf.gov/pubs/nsf21321/report [ Google Scholar ]
- Ohland, M. W., Brawner, C. E., Camacho, M. M., Layton, R. A., Long, R. A., Lord, S. M., Wasburn, M. H. (2011). Race, gender, and measures of success in engineering education . Journal of Engineering Education , 100 ( 2 ), 225–252. [ Google Scholar ]
- Omi, M., Winant, H. (2015). Racial formation in the United States (3rd edition). New York: Routledge. [ Google Scholar ]
- Patton, L. D. (2016). Disrupting postsecondary prose: Toward a critical race theory of higher education . Urban Education , 51 ( 3 ), 315–342. [ Google Scholar ]
- Peoples, W., Dillard, A. D. (2020, September 2). 5 Lessons from a race-and-ethnicity requirement . Chronicle of Higher Education . Retrieved October 1, 2021, from https://community.chronicle.com/news/2402-5-lessons-from-a-race-and-ethnicity-requirement [ Google Scholar ]
- Pérez Huber, L., Velez, V. N., Solorzano, D. (2018). More than “papelitos”: A QuantCrit counterstory to critique Latina/o degree value and occupational prestige . Race Ethnicity and Education , 21 ( 2 ), 208–230. [ Google Scholar ]
- President’s Council of Advisors on Science and Technology. (2020). Recommendations for strengthening American leadership in industries of the future . Washington, DC. Retrieved April 2, 2021, from https://science.osti.gov/-/media/_/pdf/about/pcast/202006/PCAST_June_2020_Report.pdf?la=en&hash=019A4F17C79FDEE5005C51D3D6CAC81FB31E3ABC [ Google Scholar ]
- Ray, V. E. (2019a). The still furious passage of the Black graduate student . In Byrd, W. C., Brunn-Bevel, R. J., Ovink, S. M. (Eds.), Intersectionality and higher education (pp. 88–104). New Brunswick, NJ: Rutgers University Press. [ Google Scholar ]
- Ray, V. E. (2019b). A theory of racialized organizations . American Sociological Review , 84 ( 1 ), 26–53. [ Google Scholar ]
- Reinholz, D. L., Ridgway, S. W. (2021). Access needs: Centering students and disrupting ableist norms in STEM . CBE—Life Sciences Education , 20 ( 3 ), es8. [ PMC free article ] [ PubMed ] [ Google Scholar ]
- Roberts, D. (2011). Fatal invention: How science, politics, and big business re-create race in the twenty-first century . New York, NY: New Press. [ Google Scholar ]
- Rodriguez, I., Brewe, E., Sawtelle, V., Kramer, L. H. (2012). Impact of equity models and statistical measures on interpretations of educational reform . Physical Review Special Topics—Physics Education Research , 8 ( 2 ), 020103. 10.1103/PhysRevSTPER.8.020103 [ CrossRef ] [ Google Scholar ]
- Rothstein, J. M. (2004). College performance predictions and the SAT . Journal of Econometrics , 121 ( 1 ), 297–317. [ Google Scholar ]
- Saini, A. (2019). Superior: The return of race science . Boston, MA: Beacon Press. [ Google Scholar ]
- Secules, S., McCall, C., Mejia, J. A., Beebe, C., Masters, A. S. L., Sánchez-Peña, M., Svyantek, M. (2021). Positionality practices and dimensions of impact on equity research: A collaborative inquiry and call to the community . Journal of Engineering Education , 110 ( 1 ), 19–43. [ Google Scholar ]
- Sedlacek, W. E. (2004). Beyond the big test: Noncognitive assessment in higher education . Hoboken, NJ: Wiley. [ Google Scholar ]
- Siebers, T. (2008). Disability theory . Ann Arbor, MI: University of Michigan Press. [ Google Scholar ]
- Snijders, T. A. (2005). Power and sample size in multilevel linear models . In Everitt, B., Howell, D. (Eds.), Encyclopedia of statistics in behaviorial science . Hoboken, NJ: Wiley [ Google Scholar ]
- Soares, J. A. (2007). The power of privilege: Yale and America’s elite colleges . Stanford, CA: Stanford University Press. [ Google Scholar ]
- Sultana, F. (2007). Reflexivity, positionality and participatory ethics: Negotiating fieldwork dilemmas in international research . ACME: An International Journal for Critical Geographies , 6 ( 3 ), 374–385. [ Google Scholar ]
- Thomas, J. M. (2020). Diversity regimes: Why talk is not enough to fix racial inequality at universities . New Brunswick, NJ: Rutgers University Press. [ Google Scholar ]
- Viano, S., Baker, D. J. (2020). How administrative data collection and analysis can better reflect racial and ethnic identities . Review of Research in Education , 44 ( 1 ), 301–331. [ Google Scholar ]
- Watson, K. (2005). Queer theory . Group Analysis , 38 ( 1 ), 67–81. [ Google Scholar ]
- Weatherton, M., Schussler, E. E. (2021). Success for all? A call to re-examine how student success is defined in higher education . CBE—Life Sciences Education , 20 ( 1 ), es3. [ PMC free article ] [ PubMed ] [ Google Scholar ]
- Westbrook, L., Saperstein, A. (2015). New categories are not enough: Rethinking the measurement of sex and gender in social surveys . Gender & Society , 29 ( 4 ), 534–560. [ Google Scholar ]
- Whitcomb, K. M., Singh, C. (2021). Underrepresented minority students receive lower grades and have higher rates of attrition across STEM disciplines: A sign of inequity? International Journal of Science Education , 43 ( 7 ), 1054–1089. 10.1080/09500693.2021.1900623 [ CrossRef ] [ Google Scholar ]
- Wilder, C. S. (2013). Ebony & ivy: Race, slavery, and the troubled history of America’s universities . New York, NY: Bloomsbury Press. [ Google Scholar ]
- Woodford, M. R., Kulick, A., Garvey, J. C., Sinco, B. R., Hong, J. S. (2018). LGBTQ policies and resources on campus and the experiences and psychological well-being of sexual minority college students: Advancing research on structural inclusion . Psychology of Sexual Orientation and Gender Diversity , 5 ( 4 ), 445–456. [ Google Scholar ]
- Zuberi, T. (2001). Thicker than blood: How racial statistics lie . Minneapolis, MN: University of Minnesota Press. [ Google Scholar ]
- Zuberi, T. (2008). Deracializing social statistics: Problems in the quantification of race . In Zuberi, T., Bonilla-Silva, E. (Eds.), White methods, white logic: Racism and methodology (pp. 137–146). Lanham, MD: Rowman and Littlefield. [ Google Scholar ]
- Zuberi, T., Bonilla-Silva, E. (2008). White logic, white methods: Racism and methodology . Lanham, MD: Rowman & Littlefield. [ Google Scholar ]
- Zwick, R. (2013). Is the SAT a “wealth test”? The link between educational achievement and socioeconomic status . In Zwick, R. (Ed.), Rethinking the SAT: The future of standardized testing in university admissions (pp. 225–238). New York, NY: Routledge. [ Google Scholar ]
Advertisement
Trends and Hot Topics of STEM and STEM Education: a Co-word Analysis of Literature Published in 2011–2020
- Published: 23 February 2023
Cite this article
- Ying-Shao Hsu ORCID: orcid.org/0000-0002-1635-8213 1 , 2 ,
- Kai-Yu Tang ORCID: orcid.org/0000-0002-3965-3055 3 &
- Tzu-Chiang Lin ORCID: orcid.org/0000-0003-3842-3749 4 , 5
939 Accesses
3 Citations
Explore all metrics
This study explored research trends in science, technology, engineering, and mathematics (STEM) education. Descriptive analysis and co-word analysis were used to examine articles published in Social Science Citation Index journals from 2011 to 2020. From a search of the Web of Science database, a total of 761 articles were selected as target samples for analysis. A growing number of STEM-related publications were published after 2016. The most frequently used keywords in these sample papers were also identified. Further analysis identified the leading journals and most represented countries among the target articles. A series of co-word analyses were conducted to reveal word co-occurrence according to the title, keywords, and abstract. Gender moderated engagement in STEM learning and career selection. Higher education was critical in training a STEM workforce to satisfy societal requirements for STEM roles. Our findings indicated that the attention of STEM education researchers has shifted to the professional development of teachers. Discussions and potential research directions in the field are included.
This is a preview of subscription content, log in via an institution to check access.
Access this article
Price includes VAT (Russian Federation)
Instant access to the full article PDF.
Rent this article via DeepDyve
Institutional subscriptions
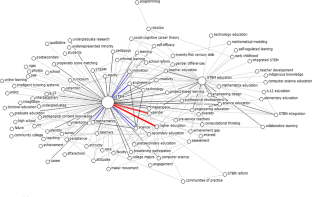
Similar content being viewed by others
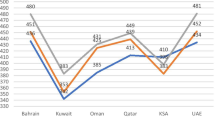
A systematic review of STEM education research in the GCC countries: trends, gaps and barriers
Fatma Kayan-Fadlelmula, Abdellatif Sellami, … Salman Umer
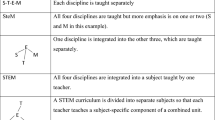
Mathematics and interdisciplinary STEM education: recent developments and future directions
Merrilyn Goos, Susana Carreira & Immaculate Kizito Namukasa
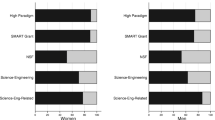
The influence of STEM definitions for research on women’s college attainment
Catherine A. Manly, Ryan S. Wells & Suzan Kommers
Data Availability
The datasets generated during and/or analysed during the current study are available from the corresponding author on reasonable request.
Akgunduz, D. (2016). A Research about the placement of the top thousand students placed in STEM fields in Turkey between the years 2000 and 2014. EURASIA Journal of Mathematics, Science and Technology Education, 12 (5), 1365–1377.
Article Google Scholar
Appianing, J., & Van Eck, R. N. (2018). Development and validation of the Value-Expectancy STEM Assessment Scale for students in higher education. International Journal of STEM Education , 5 , article 24.
Assefa, S. G., & Rorissa, A. (2013). A bibliometric mapping of the structure of STEM education using co-word analysis. Journal of the American Society for Information Science and Technology, 64 (12), 2513–2536.
Belland, B. R., Walker, A. E., Kim, N. J., & Lefler, M. (2017). Synthesizing results from empirical research on computer-based scaffolding in STEM education: A meta-analysis. Review of Educational Research, 87 (2), 309–344.
Brotman, J. S., & Moore, F. M. (2008). Girls and science: A review of four themes in the science education literature. Journal of Research in Science Teaching, 45 (9), 971–1002.
Brown, R. E., & Bogiages, C. A. (2019). Professional development through STEM integration: How early career math and science teachers respond to experiencing integrated STEM tasks. International Journal of Science and Mathematics Education, 17 (1), 111–128.
Burt, B. A., Williams, K. L., & Palmer, G. J. M. (2019). It takes a village: The role of emic and etic adaptive strengths in the persistence of black men in engineering graduate programs. American Educational Research Journal, 56 (1), 39–74.
Callon, M., Courtial, J. P., & Laville, F. (1991). Co-word analysis as a tool for describing the network of interactions between basic and technological research: The case of polymer chemistry. Scientometrics, 22 (1), 155–205.
Carlisle, D. L. & Weaver, G. C. (2018). STEM education centers: Catalyzing the improvement of undergraduate STEM education. International Journal of STEM Education, 5 , article 47.
Chang, D. F., & ChangTzeng, H. C. (2020). Patterns of gender parity in the humanities and STEM programs: The trajectory under the expanded higher education system. Studies in Higher Education, 45 (6), 1108–1120.
Charleston, L. J. (2012). A qualitative investigation of African Americans’ decision to pursue computing science degrees: Implications for cultivating career choice and aspiration. Journal of Diversity in Higher Education, 5 (4), 222–243.
Charleston, L. J., George, P. L., Jackson, J. F. L., Berhanu, J., & Amechi, M. H. (2014). Navigating underrepresented STEM spaces: Experiences of black women in US computing science higher education programs who actualize success. Journal of Diversity in Higher Education, 7 (3), 166–176.
Chien, Y. H., & Chu, P. Y. (2018). The different learning outcomes of high school and college students on a 3D-printing STEAM engineering design curriculum. International Journal of Science and Mathematics Education, 16 (6), 1047–1064.
Dehdarirad, T., Villarroya, A., & Barrios, M. (2014). Research trends in gender differences in higher education and science: A co-word analysis. Scientometrics, 101 (1), 273–290.
Dickerson, D. L., Eckhoff, A., Stewart, C. O., Chappell, S., & Hathcock, S. (2014). The examination of a pullout STEM program for urban upper elementary students. Research in Science Education, 44 (3), 483–506.
Eccles, J., Adler, T. F., Futterman, R., Goff, S. B., Kaczala, C. M., Meece, J., & Midgley, C. (1983). Expectancies, values and academic behaviors. In J. T. Spence (Ed.), Achievement and Achievement Motives . W. San Francisco: H. Freeman.
Ellison, S., & Allen, B. (2018). Disruptive innovation, labor markets, and Big Valley STEM School: Network analysis in STEM education. Cultural Studies of Science Education, 13 (1), 267–298.
Erdogan, N., Navruz, B., Younes, R., & Capraro, R. M. (2016). Viewing how STEM project-based learning influences students’ science achievement through the implementation lens: A latent growth modeling. Eurasia Journal of Mathematics, Science and Technology Education, 12 (8), 2139–2154.
European Commission, Directorate-General for Education, Youth, Sport and Culture (2016). Does the EU need more STEM graduates? Final report . Retrieve from https://data.europa.eu/doi/10.2766/000444
Fredricks, J. A., Hofkens, T., Wang, M. T., Mortenson, E., & Scott, P. (2018). Supporting girls’ and boys’ engagement in math and science learning: A mixed methods study. Journal of Research in Science Teaching, 55 (2), 271–298.
Fry, R., Kennedy, B., & Funk, C. (2021). Stem jobs see uneven progress in increasing gender, racial and ethnic diversity. Retrieve from https://www.pewresearch.org/science/wp-content/uploads/sites/16/2021/03/PS_2021.04.01_diversity-in-STEM_REPORT.pdf
Ganley, C. M., George, C. E., Cimpian, J. R., & Makowski, M. B. (2018). Gender equity in college majors: Looking beyond the STEM/non-STEM dichotomy for answers regarding female participation. American Educational Research Journal, 55 (3), 453–487.
Gehrke, S., & Kezar, A. (2019). Perceived outcomes associated with engagement in and design of faculty communities of practice focused on STEM reform. Research in Higher Education, 60 (4), 844–869.
Gilmore, J., Vieyra, M., Timmerman, B., Feldon, D., & Maher, M. (2015). The relationship between undergraduate research participation and subsequent research performance of early career STEM graduate students. Journal of Higher Education, 86 (6), 834–863.
Godwin, A., Potvin, G., Hazari, Z., & Lock, R. (2016). Identity, critical agency, and engineering: An affective model for predicting engineering as a career choice. Journal of Engineering Education, 105 (2), 312–340.
Han, S., Yalvac, B., Capraro, M. M., & Capraro, R. M. (2015). In-service teachers’ implementation and understanding of STEM project based learning. Eurasia Journal of Mathematics Science and Technology Education, 11 (1), 63–76.
Heras, M., Ruiz-Mallén, I., & Gallois, S. (2020). Staging science with young people: Bringing science closer to students through stand-up comedy. International Journal of Science Education, 42 (12), 1968–1987.
Hernandez, P. R., Estrada, M., Woodcock, A., & Schultz, P. W. (2017). Protégé perceptions of high mentorship quality depend on shared values more than on demographic match. Journal of Experimental Education, 85 (3), 450–468.
Hinojo Lucena, F. J., Lopez Belmonte, J., Fuentes Cabrera, A., Trujillo Torres, J. M., & Pozo Sanchez, S. (2020). Academic effects of the use of flipped learning in physical education. International journal of Environmental Research and Public Health , 17 (1), article 276.
Holmes, K., Gore, J., Smith, M., & Lloyd, A. (2018). An integrated analysis of school students’ aspirations for STEM careers: Which student and school factors are most predictive? International Journal of Science and Mathematics Education, 16 (4), 655–675.
Huang, X., & Qiao, C. (2022). Enhancing computational thinking skills through artificial intelligence education at a STEAM high school. Science & Education . https://doi.org/10.1007/s11191-022-00392-6
Hughes, R. M., Nzekwe, B., & Molynearx, K. J. (2013). The single sex debate for girls in science: A comparison between two informal science programs on middle school students’ STEM identity formation. Research in Science Education, 43 , 1979–2007.
Hughes, B. S., Corrigan, M. W., Grove, D., Andersen, S. B., & Wong, J. T. (2022). Integrating arts with STEM and leading with STEAM to increase science learning with equity for emerging bilingual learners in the United States. International Journal of STEM Education , 9 , article 58.
Johnson, A. M. (2019). “I can turn it on when I need to”: Pre-college integration, culture, and peer academic engagement among black and Latino/a engineering students. Sociology of Education, 92 (1), 1–20.
Kayan-Fadlelmula, F., Sellami, A., Abdelkader, N., & Umer, S. (2022). A systematic review of STEM education research in the GCC countries: Trends, gaps and barriers. International Journal of STEM Education, 9 , article 2.
Kelly, R., Mc Garr, O., Leahy, K., & Goos, M. (2020). An investigation of university students and professionals’ professional STEM identity status. Journal of Science Education and Technology, 29 (4), 536–546.
Kezar, A., Gehrke, S., & Bernstein-Sierra, S. (2017). Designing for success in STEM communities of practice: Philosophy and personal interactions. The Review of Higher Education, 40 (2), 217–244.
Kezar, A., Gehrke, S., & Bernstein-Sierra, S. (2018). Communities of transformation: Creating changes to deeply entrenched issues. The Journal of Higher Education, 89 (6), 832–864.
Kricorian, K., Seu, M., Lpoez, D., Ureta, E., & Equils, O. (2020). Factors influencing participation of underrepresented students in STEM fields: Matched mentors and mindsets. International Journal of STEM Education, 7 , article 16.
Ku, C. J., Hsu, Y. S., Chang, M. C., & Lin, K. Y. (2022). A model for examining middle school students’ STEM integration behavior in a national technology competition. International Journal of STEM Education, 9 (1), 3.
Leydesdroff, L. (1989). Words and co-words as indicators of intellectual organization. Research Policy, 18 (4), 209–223.
Li, Y., Wang, K., Xiao, Y., & Froyd, J. E. (2020a). Research and trends in STEM education: A systematic review of journal publications. International Journal of STEM Education, 7 , article 11.
Li, Y., Wang, K., Xiao, Y., Froyd, J. E., Nite, S. B. (2020b). Research and trends in STEM education: A systematic analysis of publicly funded projects. International Journal of STEM Education, 7 , article 17.
Lin, T. C., Lin, T. J., & Tsai, C. C. (2014). Research trends in science education from 2008 to 2012: A systematic content analysis of publications in selected journals. International Journal of Science Education, 36 (8), 1346–1372.
Lin, T. J., Lin, T. C., Potvin, P., & Tsai, C. C. (2019). Research trends in science education from 2013 to 2017: A systematic content analysis of publications in selected journals. International Journal of Science Education, 41 (3), 367–387.
Lin, T. C., Tang, K. Y., Lin, S. S., Changlai, M. L., & Hsu, Y. S. (2022). A co-word analysis of selected science education literature: Identifying research trends of scaffolding in two decades (2000–2019). Frontiers in Psychology, 13 , 844425.
Liu, J. S., & Lu, L. Y. (2012). An integrated approach for main path analysis: Development of the Hirsch index as an example. Journal of the American Society for Information Science and Technology, 63 (3), 528–542.
Liu, C. Y., & Wu, C. J. (2022). STEM without art: A ship without a sail. Thinking Skills and Creativity, 43 , 100977.
Lou, S. H., Shih, R. C., Diez, C. R., & Tseng, K. H. (2011). The impact of problem-based learning strategies on STEM knowledge integration and attitudes: An exploratory study among female Taiwanese senior high school students. International Journal of Technology and Design Education, 21 (2), 195–215.
Lynch, S. J., Burton, E. P., Behrend, T., House, A., Ford, M., Spillane, N., Matray, S., Han, E., & Means, B. (2018). Understanding inclusive STEM high schools as opportunity structures for underrepresented students: Critical components. Journal of Research in Science Teaching, 55 (5), 712–748.
Maass, K., Geiger, V., Ariza, M. R., & Goos, M. (2019). The Role of mathematics in interdisciplinary STEM education. ZDM-Mathematics Education, 51 (6), 869–884.
Mansfield, K. C. (2014). How listening to student voices informs and strengthens social justice research and practice. Educational Administration Quarterly, 50 (3), 392–430.
Margot, K. C., & Kettler, T. (2019). Teachers’ perception of STEM integration and education: A systematic literature review. International Journal of STEM education , 6 , article 2.
Marín-Marín, J. A., Moreno-Guerrero, A. J., Dúo-Terrón, P., & López-Belmonte, J. (2021). STEAM in education: A bibliometric analysis of performance and co-words in Web of Science. International Journal of STEM Education , 8 , article 41.
Martín-Páez, T., Aguilera, D., Perales-Palacios, F. J., & Vílchez-González, J. M. (2019). What are we talking about when we talk about STEM education? A Review of Literature. Science Education, 103 (4), 799–822.
Google Scholar
McGee, E. O. (2020). Interrogating structural racism in STEM higher education. Educational Researcher, 49 (9), 633–644.
Meho, L. I., & Yang, K. (2006). A new era in citation and bibliometric analyses: Web of Science, Scopus, and Google Scholar. arXiv preprint cs/0612132 .
Mejias, S., Thompson, N., Sedas, R. M., Rosin, M., Soep, E., Peppler, K., Roche, J., Wong, J., Hurley, M., Bell, P., & Bevan, B. (2021). The trouble with STEAM and why we use it anyway. Science Education, 105 (2), 209–231.
Micari, M., Van Winkle, Z., & Pazos, P. (2016). Among friends: The role of academic-preparedness diversity in individual performance within a small-group STEM learning environment. International Journal of Science Education, 38 (12), 1904–1922.
Millar, V. (2020). Trends, issues and possibilities for an interdisciplinary STEM curriculum. Science & Education, 29 (4), 929–948.
Nadelson, L. S., Callahan, J., Pyke, P., Hay, A., Dance, M., & Pfiester, J. (2013). Teacher STEM perception and preparation: Inquiry-based STEM professional development for elementary teachers. Journal of Educational Research, 106 (2), 157–168.
Nakatoh, T., & Hirokawa, S. (2019, July). Evaluation index to find relevant papers: Improvement of focused citation count. In International Conference on Human-Computer Interaction (pp. 555–566). Springer, Cham.
National Science Technology Council. (2012). Coordinating federal science, technology, engineering, and mathematics (STEM) education investments: Progress report. Retrieved from https://obamawhitehouse.archives.gov/sites/default/files/microsites/ostp/nstc_federal_stem_education_coordination_report.pdf
National Science Technology Council. (2013). Federal Science, Technology, Engineering, and Mathematics (STEM) Education 5-Year Strategic Plan. Retrieved from https://obamawhitehouse.archives.gov/sites/default/files/microsites/ostp/stem_stratplan_2013.pdf
Ong, M., Smith, J. M., & Ko, L. T. (2018). Counterspaces for women of color in STEM higher education: Marginal and central spaces for persistence and success. Journal of Research in Science Teaching, 55 (2), 206–245.
Organisation for Economic Cooperation and Development, OECD (2021). Education at A Glance 2021. Retrieve from https://read.oecd.org/10.1787/b35a14e5-en?format=pdf
Perez-Felkner, L., Felkner, J. S., Nix, S., & Magalhaes, M. (2020). The puzzling relationship between international development and gender equity: The case of STEM postsecondary education in Cambodia. International Journal of Educational Development, 72 , 102102.
Perignat, E., & Katz-Buonincontro, J. (2019). STEAM in practice and research: An integrative literature review. Thinking Skills and Creativity, 31 , 31–43.
Quigley, C. F., & Herro, D. (2016). “Finding the joy in the unknown”: Implementation of steam teaching practices in middle school science and math classrooms. Journal of Science Education and Technology, 25 (3), 410–426.
Ramey, K. E., & Stevens, R. (2019). Interest development and learning in choice-based, in-school, making activities: The case of a 3D printer. Learning, Culture and Social Interaction, 23 , 100262.
Salami, M. K., Makela, C. J., & de Miranda, M. A. (2017). Assessing changes in teachers’ attitudes toward interdisciplinary STEM teaching. International Journal of Technology and Design Education, 27 (1), 63–88.
Sanders, M. (2009). Integrative STEM education primer. The Technology Teacher, 68 (4), 20–26.
Saorín, J. L., Melian-Díaz, D., Bonnet, A., Carrera, C. C., Meier, C., & De La Torre-Cantero, J. (2017). Makerspace teaching-learning environment to enhance creative competence in engineering students. Thinking Skills and Creativity, 23 , 188–198.
Simon, R. M., Wagner, A., & Killion, B. (2017). Gender and choosing a STEM major in college: Femininity, masculinity, chilly climate, and occupational values. Journal of Research in Science Teaching, 54 (3), 299–323.
Stolle-McAllister, K., Domingo, M. R. S., & Carrillo, A. (2011). The Meyerhoff way: How the Meyerhoff scholarship program helps black students succeed in the sciences. Journal of Science Education and Technology, 20 (1), 5–16.
Thomas, B., & Watters, J. J. (2015). Perspectives on Australian, Indian and Malaysian approaches to STEM education. International Journal of Educational Development, 45 , 42–53.
Tosun, C. (2022). Analysis of the last 40 years of science education research via bibliometric methods. Science & Education . https://doi.org/10.1007/s11191-022-00400-9
Van Eck, N. J., & Waltman, L. (2010). Software survey: VOSviewer, a computer program for bibliometric mapping. Scientometrics, 84 (2), 523–538.
Vencent-Ruz, P., & Schunn, C. D. (2017). The increasingly important role of science competency beliefs for science learning in girls. Journal of Research in Science Teaching, 54 (6), 790–822.
Wang, S., Chen, Y., Lv, X., & Xu, J. (2022). Hot topics and frontier evolution of science education research: A bibliometric mapping from 2001 to 2020. Science & Education . https://doi.org/10.1007/s11191-022-00337-z
Weeden, K. A., Gelbgiser, D., & Morgan, S. L. (2020). Pipeline dreams: Occupational plans and gender differences in STEM major persistence and completion. Sociology of Education, 93 (4), 297–314.
Wigfield, A., & Eccles, J. S. (2000). Expectancy-value theory of achievement motivation. Contemporary Educational Psychology, 25 (1), 68–81.
Download references
Author information
Authors and affiliations.
Graduate Institute of Science Education, National Taiwan Normal University, No. 88, Ting-Jou Rd., Sec. 4, Taipei City, 116, Taiwan
Ying-Shao Hsu
Institute for Research Excellence in Learning Sciences, National Taiwan Normal University, No. 88, Ting-Jou Rd., Sec. 4, Taipei City, 116, Taiwan
Graduate Institute of Library & Information Science, National Chung Hsing University, 145 Xingda Rd., South Dist., Taichung City, 402, Taiwan
Kai-Yu Tang
Center for Liberal Arts, National Kaohsiung University of Science and Technology, No. 415, Jiangong Rd., Sanmin Dist, Kaohsiung City, 807618, Taiwan
Tzu-Chiang Lin
Center for Teacher Education, National Kaohsiung University of Science and Technology, No. 415, Jiangong Rd., Sanmin Dist, Kaohsiung City, 807618, Taiwan
You can also search for this author in PubMed Google Scholar
Corresponding author
Correspondence to Tzu-Chiang Lin .
Ethics declarations
Ethical approval and consent to participate.
This study involves neither human participants’ data nor relevant biological material. Ethics approval and informed consent are hence not applicable.
Conflict of Interest
The authors declare that they have no conflict of interest.
Additional information
Publisher's note.
Springer Nature remains neutral with regard to jurisdictional claims in published maps and institutional affiliations.
Rights and permissions
Springer Nature or its licensor (e.g. a society or other partner) holds exclusive rights to this article under a publishing agreement with the author(s) or other rightsholder(s); author self-archiving of the accepted manuscript version of this article is solely governed by the terms of such publishing agreement and applicable law.
Reprints and permissions
About this article
Hsu, YS., Tang, KY. & Lin, TC. Trends and Hot Topics of STEM and STEM Education: a Co-word Analysis of Literature Published in 2011–2020. Sci & Educ (2023). https://doi.org/10.1007/s11191-023-00419-6
Download citation
Accepted : 26 January 2023
Published : 23 February 2023
DOI : https://doi.org/10.1007/s11191-023-00419-6
Share this article
Anyone you share the following link with will be able to read this content:
Sorry, a shareable link is not currently available for this article.
Provided by the Springer Nature SharedIt content-sharing initiative
- STEM education
- Co-word analysis
- Research trends
- Find a journal
- Publish with us
- Track your research

171+ Brilliant Quantitative Research Topics For STEM Students

STEM means science, technology, engineering, and mathematics. These all are the most interesting fields of study for computer science students. There are lots of quantitative research topics for stem students.
By practicing these projects STEm students can easily boost their skills in their field. Also, you will easily get the best job in their relevant field. If you are seriously looking for the most interesting and best topics in quantitative research for STEM students, do not look further.
Stop your research here because here you are finding the best quantitative research topics for the students whether you are a nursing student, a psychology student, or looking for any field. Here you get all the topics that are most helpful for you. Let’s grab here all knowledgeable topics.
Also Like To Read: 100+ Best Accounting Research Topics For Students In 2024
Table of Contents
What Is Quantitative Research Topics In STEM
Quantitative research involves collecting and analyzing numerical data to understand phenomena, test hypotheses, and measure outcomes. Here are some key things to know about quantitative research topics in STEM (science, technology, engineering, and math) fields:
- Quantitative research is used widely across STEM disciplines to test objective theories and examine relationships between measurable variables. This allows for statistical analysis.
- Common quantitative research methods in STEM include experiments, observational studies, surveys, and analysis of existing statistical data. Researchers precisely measure variables and outcomes to collect numerical data.
- STEM research topics suited to quantitative methods include examining the effectiveness of an educational intervention, comparing factors that influence electricity usage, optimizing chemical reactions, analyzing properties of materials or manufactured products, and modeling climate phenomena.
- Strong quantitative STEM research questions focus on measurable independent and dependent variables, such as “How does the timing of active learning breaks affect test scores in elementary school students?” or “What welding parameters produce joints with the highest tensile strength?”
- Quantitative STEM research aims to collect generalizable, replicable data. Variables and conditions must be carefully controlled, and bias minimized. Randomized experiments are ideal.
How To Choose Best Quantitative Research Topics For STEM Students
These are the following steps to choose the best topics in quantitative research for STEM students.
- Identify your research interests and passion in STEM.
- Explore recent STEM literature for gaps or trends.
- Consult with professors, mentors, or peers for guidance.
- Narrow down topics based on feasibility and resources.
- Ensure the research question is specific and testable.
- Consider the potential impact and relevance of your topic.
- Review and refine your research topic before finalizing.
Best Quantitative Research Topics For STEM Students
Below are some best quantitative research topics for STEM students.
Mathematics Research Topics
- The distribution of prime numbers.
- Group theory and its applications.
- Non-commutative rings and their properties.
- Diophantine equations and Fermat’s Last Theorem.
- Applications of algebraic structures in cryptography.
- Bayesian analysis of real-world data.
- Regression analysis in economic forecasting.
- Statistical methods in clinical trials.
- The properties of perfect numbers.
- Number theory and its practical applications.
- Cryptography and code-breaking techniques.
- Analyzing statistical anomalies in financial markets.
- Chaos theory and its implications in mathematics.
- Analyzing patterns in fractals.
- The Riemann Hypothesis and its significance.
Physics Research Topics
- Quantum entanglement and quantum communication.
- Behavior of particles in Bose-Einstein condensates.
- The physics of superconductivity.
- Properties of black holes and their role in the universe.
- Cosmic microwave background radiation.
- Formation and evolution of galaxies.
- The Higgs boson and particle physics.
- Exploring the Standard Model’s limitations.
- Properties of neutrinos and their role in the universe.
- Quantum teleportation and its practical applications.
- The physics of string theory.
- Gravitational waves and their detection.
- Magnetic monopoles in particle physics.
- The behavior of quarks and gluons.
- The search for dark matter in the universe.
Chemistry Research Topics
- Chemical kinetics and reaction mechanisms.
- Catalysis in chemical reactions.
- Kinetics of enzyme-substrate interactions.
- Applications of nanomaterials in drug delivery.
- Nanoscale characterization techniques.
- Environmental impact of nanotechnology.
- Mass spectrometry techniques for chemical analysis.
- Chromatography in pharmaceutical analysis.
- Electrochemical methods for sensor development.
- Green chemistry and sustainable practices.
- Chemical thermodynamics and phase equilibria.
- Polymer chemistry and its industrial applications.
- Quantum chemistry and molecular modeling.
- Supramolecular chemistry and self-assembly.
- Analyzing chemical reactions at the atomic level.
Biology Research Topics
- Epigenetics and gene regulation.
- Genome sequencing and personalized medicine.
- Genetics of inherited diseases.
- Impact of climate change on ecosystems.
- Biodiversity and conservation efforts.
- Effects of pollution on aquatic ecosystems.
- Antibiotic resistance in bacteria.
- Role of microbiota in human health.
- Viral replication mechanisms.
- Evolutionary biology and speciation.
- Behavioral ecology and animal communication.
- Neurobiology of memory and learning.
- Molecular biology of cancer.
- Genomic imprinting and its significance.
- Evolution of drug resistance in pathogens.
Engineering Research Topics
- Artificial intelligence in robotics.
- Autonomous vehicle technology.
- Challenges of human-robot collaboration.
- Efficiency of solar cell technologies.
- Wind turbine design and optimization.
- Biofuels for sustainable energy.
- Earthquake-resistant structural materials.
- Composite materials in construction.
- Sustainability in building designs.
- Aerospace materials and their properties.
- Biomedical engineering advancements.
- Transportation system optimization.
- Space exploration technologies.
- Smart cities and urban planning.
- Materials for clean energy production.
Computer Science Research Topics
- Deep learning algorithms for image recognition.
- Natural language processing for chatbots.
- Ethical considerations in AI development.
- Data mining techniques for business insights.
- Predictive modeling in healthcare analytics.
- Impact of big data on decision-making.
- Blockchain technology for secure transactions.
- Detection and prevention of cyber threats.
- Role of machine learning in cybersecurity.
- Quantum computing and its potential.
- Human-computer interaction and user experience.
- Distributed computing and cloud computing.
- Internet of Things (IoT) applications.
- Bioinformatics and genomic data analysis.
- Virtual reality and augmented reality technologies.
Earth Sciences Quantitative Research Topics For High School Students
- Plate tectonics and earthquake prediction.
- Mineral exploration and resource management.
- Impact of geological processes on the environment.
- Climate modeling and climate change predictions.
- Effects of El Niño and La Niña phenomena.
- Role of clouds in climate regulation.
- Ocean circulation patterns and climate impact.
- Marine biodiversity and conservation.
- Effects of ocean acidification on marine ecosystems.
- Geothermal energy exploration and utilization.
- Volcanic eruptions and their monitoring.
- Remote sensing in Earth sciences.
- Geological hazards and risk assessment.
- Geological survey techniques.
- Geographical information systems (GIS) in environmental analysis.
- Carbon capture and sequestration.
Nursing Quantitative Research Topics For STEM Students
- Nursing Education and Curriculum Development
- Patient Outcomes and Quality of Care
- Healthcare Technology and Informatics
- Nursing Workforce and Staffing
- Chronic Disease Management
- Pain Management and Palliative Care
- Infection Control and Prevention
- Mental Health and Psychiatric Nursing
- Maternal and Child Health
- Health Disparities and Cultural Competence
So, these are the best quantitative research topics for STEM students.
Why Quantitative Research Topics Beneficial For STEM Students
These are the major reasons why beneficial quantitative research topics for STEM students.
- Quantitative research topics provide practical data analysis skills.
- They foster critical thinking and problem-solving abilities.
- Quantitative research enhances statistical literacy , crucial in STEM fields.
- It encourages hypothesis testing and evidence-based decision-making.
- STEM students gain proficiency in data collection and measurement.
- Quantitative studies contribute to scientific advancement and innovation.
- They prepare STEM students for research and industry demands.
With a final of 171+ quantitative research topics for stem students in various STM areas, students have plenty of options to explore and contribute to the advancement of knowledge in their chosen subjects.
Quantitative research not only tests their understanding but also imparts them with valuable analytical skills. So, dive into the fascinating world of STM research and unlock the potential to make meaningful discoveries. Quantitative Research is a summary of STM topics, providing endless opportunities to stock up and explore.
If you are passionate about mathematics, physics, chemistry, biology, engineering, computer science, or earth science, there is a quantitative research topic waiting for you to explore and expand your understanding of the world. I hope you liked this post about quantitative research topics.
Good Frequently Asked Questions
What is the significance of quantitative research topics in stem fields.
Quantitative research topics are essential in STEM to provide data-driven insights and support evidence-based decision-making.
How can I select a suitable quantitative research topic for my STEM project?
A well-defined quantitative research question should be specific, measurable, and relevant to address a scientific problem.
What are the key elements of a well-defined quantitative research question?
In quantitative STEM research, ensuring data reliability and validity is crucial for the accuracy of findings and conclusions.
Leave a Reply Cancel reply
Your email address will not be published. Required fields are marked *
Save my name, email, and website in this browser for the next time I comment.
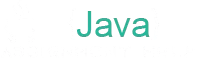
199+ Engaging Quantitative Research Topics for STEM Students
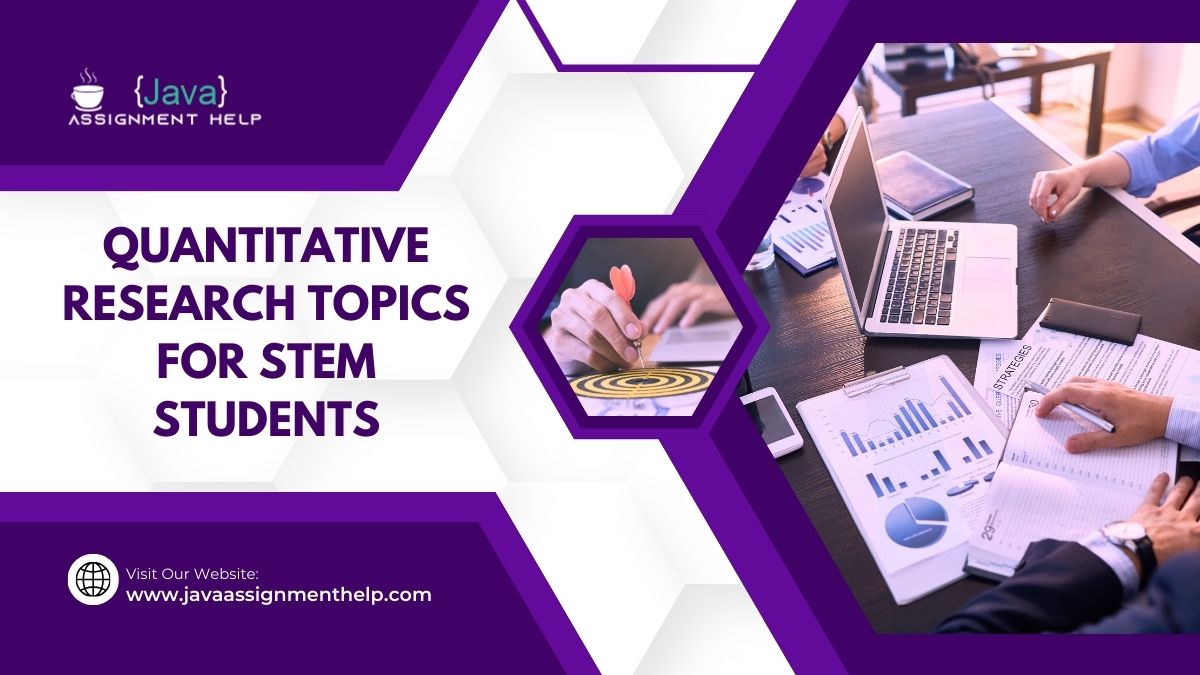
Explore diverse quantitative research topics for STEM students, spanning climate change, AI, robotics, and more. Find engaging projects for high school and beyond.
Quantitative studies allow you to investigate real-world problems and make groundbreaking discoveries using cold, hard facts.
Whether you’re curious about developing new sustainable materials, modeling climate patterns, or optimizing computer algorithms, quantitative methods provide the tools to find concrete answers.
This guide will open your eyes to all sorts of compelling quantitative research areas perfectly suited for inquisitive STEM students like yourself.
Get ready to geek out over cutting-edge ideas from multiple scientific disciplines! Conducting your own quantitative research is an awesome opportunity to strengthen vital skills like statistical analysis, objective reasoning, and solving complex equations.
Plus, you’ll get to satisfy your inner science nerd by pushing technological and scientific frontiers. The possibilities are endless when you’ve got empirical data as your foundation.
Let’s uncover some numerical nuggets and find a quantitative topic that sparks your passion for mathematical and scientific exploration!
Why Choose Quantitative Research?
Why choose quantitative research? Here’s the lowdown on its perks:
- Numbers Don’t Lie: Quantitative research deals with hard data, making analysis unbiased and credible. Plus, it’s easy to replicate by other researchers.
- Broad Insights: With big sample sizes, findings can apply to larger groups. Perfect for understanding widespread trends or testing big hypotheses.
- Testing, Testing: Got a hunch? Quantitative research lets you test hypotheses with structured methods, finding cause-and-effect or spotting correlations.
- Quick and Efficient: Streamlined surveys or existing data mean faster collection and analysis compared to other methods.
- Clear as Day: Results are presented through stats, charts, and graphs, making them simple to understand and share—even with non-experts.
Keep in mind
- Numbers Rule: Quantitative research is all about crunching data and measuring impacts.
- Scope’s the Limit: It’s great for objective results, but might not dig deep into human stories or motivations.
In short, if you’re all about measuring trends or figuring out cause-and-effect, quantitative research is your go-to. Easy as pie!
Quantitative Research Topics for STEM Students
Check out quantiative research topics for STEM students:-
- The impact of environmental factors on species biodiversity.
- Quantifying the efficacy of different fertilizers on crop yield.
- Analyzing genetic variations in disease susceptibility among populations.
- The effect of pH levels on enzyme activity.
- Pollution levels and aquatic ecosystem health.
- Population dynamics in specific ecosystems.
- Temperature changes and species migration patterns.
- Conservation strategies on endangered species recovery.
- Climate change effects on plant phenology.
- Antibiotic use and antibiotic resistance in bacteria.
- Kinetics of chemical reactions under varying conditions.
- Energy efficiency of different fuel sources.
- Composition of atmospheric pollutants in urban vs. rural areas.
- Effectiveness of water purification methods.
- Solubility of compounds in different solvents.
- Catalyst efficiency in chemical reactions.
- Correlation between pH levels and acid rain formation.
- Concentration of heavy metals in soil samples.
- Temperature effects on reaction rates.
- Material composition and mechanical properties.
- Projectile motion trajectories.
- Mass and gravitational force relationship.
- Efficiency of renewable energy technologies.
- Effects of friction on object motion.
- Temperature and conductivity in semiconductors.
- Light behavior in different mediums.
- Efficiency of insulation materials.
- Air resistance effects on falling objects.
- Properties of materials under pressure.
- Methods for soundproofing efficiency.
Mathematics
- Population growth models.
- Distribution of prime numbers.
- Relationship between math aptitude and academic performance.
- Efficiency of algorithms for optimization problems.
- Class size and student learning outcomes.
- Socioeconomic status and math achievement.
- Effectiveness of teaching methods.
- Voting patterns in elections.
- Income inequality distribution.
- Stock market trend prediction models.
Engineering
- Material efficiency in structural engineering.
- Road design and traffic congestion.
- Aerodynamic properties of aircraft designs.
- Water distribution systems in urban areas.
- Vibration effects on building structures.
- Bridge design and resilience to natural disasters.
- Cooling systems efficiency in electronics.
- Urbanization impact on heat island effect.
- Energy consumption in buildings.
- Waste management and recycling methods.
Computer Science
- Sorting algorithm efficiency.
- Performance of machine learning algorithms.
- Coding proficiency and software development productivity.
- Encryption algorithms for data security.
- Network traffic pattern analysis.
- Software complexity and system reliability.
- Cyberattacks impact on network performance.
- Error correction codes in communication systems.
- Parallel computing architecture efficiency.
- Cloud computing scalability for large datasets.
Environmental Science
- Deforestation rates and ecosystem impact.
- Air pollution and respiratory health.
- Renewable energy policies and greenhouse gas emissions.
- Wastewater treatment plant efficiency.
- Ocean acidification effects on coral reefs.
- Temperature rise and sea level change correlation.
- Plastic pollution impact on marine biodiversity.
- Conservation strategies effectiveness.
- Land use changes and ecosystem services relationship.
- Carbon capture and storage technologies efficiency.
- Galaxy distribution in the universe.
- Properties of exoplanets discovered through transit methods.
- Stellar mass and black hole formation.
- Telescope design efficiency.
- Gravitational lensing effects in galaxy clusters.
- Solar activity and geomagnetic storms correlation.
- Propulsion systems efficiency for interplanetary travel.
- Asteroid collisions impact on planetary surfaces.
- Dark matter effects on galaxy rotation curves.
- Mars terraforming feasibility.
Materials Science
- Crystal structures analysis using X-ray diffraction.
- Material composition and mechanical properties correlation.
- Energy storage materials efficiency.
- Nanomaterials performance in biomedical applications.
- Temperature effects on material conductivity.
- Material defects and fracture toughness correlation.
- Coating materials efficiency for corrosion protection.
- Material processing techniques impact on grain size distribution.
- Material properties and electronic band structure relationship.
- Composite materials durability under extreme conditions.
Health Sciences
- Vaccine effectiveness in disease prevention.
- Patient outcomes in clinical trials.
- Lifestyle factors and chronic diseases correlation.
- Effectiveness of screening methods for early disease detection.
- Air pollution effects on respiratory illness prevalence.
- Sleep quality and cognitive function correlation.
- Socioeconomic factors impact on healthcare access.
- Mindfulness meditation stress reduction effectiveness.
- Genetic predisposition and disease susceptibility correlation.
- Telemedicine interventions for patient outcomes improvement.
Social Sciences
- Crime rates in urban vs. rural areas.
- Education levels and income inequality relationship.
- Parenting styles and child development outcomes correlation.
- Social media usage and mental health outcomes.
- Diversity initiatives impact on workplace productivity.
- Cultural norms and gender inequality correlation.
- Intervention programs effectiveness for reducing substance abuse.
- Political ideology and voting behavior correlation.
- Income inequality impact on social mobility.
- Public policy interventions effectiveness in addressing homelessness.
- Class size impact on academic achievement.
- Teaching methods effectiveness on student learning outcomes.
- Parental involvement and student success relationship.
- Standardized testing efficiency in assessing proficiency.
- Technology integration impact on classroom engagement.
- Teacher experience and student performance correlation.
- School funding disparities impact on educational equity.
- Early childhood education interventions impact on academic success.
- Socioeconomic status and educational attainment correlation.
- Interventions effectiveness for reducing school dropout rates.
- Personality traits impact on career success.
- Childhood trauma effects on adult mental health outcomes.
- Cognitive-behavioral therapy effectiveness in anxiety disorders treatment.
- Mindfulness-based interventions stress reduction effectiveness.
- Social media usage effects on self-esteem and body image.
- Parenting styles and child behavior problems correlation.
- Cultural differences impact on emotion regulation strategies.
- Sleep quality and mood disorders correlation.
- Exercise effects on depression symptoms.
- Interventions for preventing suicide effectiveness.
- Inflation rates and economic growth relationship.
- Government spending impact on GDP growth.
- Taxation policies efficiency in reducing income inequality.
- Trade policies effects on employment rates.
- Interest rates and investment behavior correlation.
- Education levels and income mobility correlation.
- Technological innovation impact on productivity growth.
- Monetary policy interventions effectiveness in stabilizing the economy.
- Globalization impact on income distribution.
- Fiscal stimulus programs efficiency in economic recovery.
Political Science
- Voter turnout rates in different electoral systems.
- Political ideology and policy preferences correlation.
- Campaign strategies impact on election outcomes.
- Media coverage impact on public opinion formation.
- Gerrymandering effects on electoral competitiveness.
- Political polarization impact on social cohesion.
- Lobbying efforts impact on legislative decision-making.
- Government transparency and public trust correlation.
- Electoral reform impact on political representation.
- International diplomacy effectiveness in conflict resolution.
- Geological formations and earthquake activity correlation.
- Soil erosion rates in different landscapes.
- Groundwater remediation techniques efficiency.
- Volcanic eruptions impact on climate patterns.
- Tectonic plate movements and mountain formation correlation.
- Fossil fuel extraction and land subsidence correlation.
- Sea level rise impact on coastal erosion rates.
- Landslide prevention measures effectiveness.
- Climate change impact on glacier retreat.
- Geological hazard mitigation strategies efficiency.
Oceanography
- Ocean circulation patterns analysis using numerical models.
- Ocean temperature and marine biodiversity relationship.
- Fishing practices efficiency on fish stocks.
- Ocean acidification impact on coral reef ecosystems.
- Sea level rise and coastal erosion correlation.
- Ocean currents and climate patterns correlation.
- Plastic pollution impact on marine ecosystems.
- Marine protected areas effectiveness in conservation.
- Offshore oil drilling impact on marine life.
- Desalination techniques efficiency for freshwater production.
- Treatments effectiveness for specific diseases.
- Patient outcomes in clinical trials analysis.
- Vaccination campaigns impact on disease prevalence.
- Genetic mutations and disease susceptibility correlation.
- Medication adherence impact on treatment success.
- Healthcare disparities impact on patient outcomes.
- Telemedicine interventions impact on remote patient care.
- Hospital-acquired infections impact on patient morbidity.
- Healthcare delivery systems efficiency in improving population health.
Biomedical Engineering
- Prosthetic limb functionality analysis for amputees.
- Biomaterial properties and tissue regeneration correlation.
- Drug delivery systems efficiency for targeted therapies.
- Wearable medical devices impact on patient monitoring.
- Biomechanical forces and orthopedic injuries correlation.
- Medical imaging techniques impact on diagnostic accuracy.
- Bioinformatics tools impact on genomic analysis.
- Neural implants efficiency for restoring sensory function.
- Tissue engineering approaches impact on organ transplantation.
- Rehabilitation robotics efficiency for motor recovery.
Nanotechnology
- Nanoparticle size and drug delivery efficiency correlation.
- Nanomaterial toxicity in biological systems analysis.
- Nanosensors efficiency for detecting environmental pollutants.
- Nanocoatings impact on material durability and corrosion resistance.
- Surface roughness and nanomaterial adhesion correlation.
- Nanoparticle structure and electronic properties correlation.
- Nanotechnology impact on renewable energy technologies.
- Nanomedicine approaches efficiency for cancer therapy.
- Nanofluids impact on heat transfer in engineering applications.
- Nanofabrication techniques efficiency for manufacturing nanostructures.
These topics offer a diverse range of research opportunities for STEM students across various disciplines.
What are the best topics in quantitative research for STEM students?
Exploring STEM is like opening a treasure chest of wonders with numbers! Check out these awesome areas:
Health and Medicine
- Ever wondered how they test new medicines? It’s fascinating stuff!
- See how things like food, exercise, and sleep impact our health—it’s like cracking a secret code!
- Explore why people end up in hospitals and what we can learn from it.
- Curious about how climate change might affect animals and plants? Let’s find out!
- Is planting trees really helping our planet? Time to investigate!
- Which clean energy source is the coolest? Solar? Wind? Let’s compare and see!
Technology and Engineering
- Testing out new materials to see how strong they are is like being a science superhero!
- Wi-Fi vs. 5G—ever wondered which is faster? Let’s put them to the test!
- Making machines run smoother and greener? Count me in!
What is the best topic for research in stem?
There is not any single best topic for research in STEM. Here’s what to consider:
- Your Passion: What in STEM gets you excited? Follow your interests!
- Real-World Impact: Aim to tackle important problems or make big discoveries.
- Practical Stuff: Make sure you have the resources and time for your project.
Here’s how to start your search
- Read Cool Stuff: Check out top STEM journals for ideas and see what’s hot.
- Join the Chat: Attend conferences and webinars to learn and meet experts.
- Talk it Out: Discuss ideas with professors—they’re there to help!
And here are some hot topics to consider
- Saving the Planet: Think renewable energy, climate change solutions, or eco-friendly materials.
- Health Breakthroughs: Explore personalized medicine, gene editing, or new ways to fight diseases.
- Future Tech: Dive into artificial intelligence, robotics, or the mysteries of space and astronomy.
Hope this helps you find your STEM passion!
What is quantitative research related to stem students?
Quantitative research is like a secret weapon for STEM students. It helps them explore science using numbers and stats, making their findings solid and reliable. Here’s why:-
- It’s Objective: Numbers don’t lie, so it keeps things fair and unbiased.
- Testing Ideas: Got a hunch? Quantitative research helps you test your theories properly.
- Big Picture: With lots of data, you can make conclusions that matter to more than just your study group.
Now, here are some cool areas where STEM students can get into it:
- Environmental Science: Measure climate change impacts or track biodiversity loss.
- Public Health: Look at how diets affect diseases or see if vaccines really work.
- Engineering: Figure out which materials make things stronger or find the best renewable energy sources.
And a few tips for STEM students diving into quantitative research:
- Find the Data: Check out government databases or online sources for info on your topic.
- Think Beyond Science: Consider how your research might affect society or the environment.
- Get Advice: Talk to your professors for guidance on your project.
With quantitative research, STEM students can unlock new discoveries and make a real impact on the world.
In the world of STEM, think of quantitative research like an artist’s brush, adding precise details to our big picture of knowledge.
It’s the toolbox that lets STEM students dive deep into the mysteries of our universe using numbers and stats.
The stuff we’ve been talking about isn’t just boring digits; they’re like secret passages leading to exciting discoveries.
Whether it’s understanding climate change, exploring AI, or playing with data, quantitative research helps STEM students find cool insights.
As we wrap up, remember it’s not just about crunching numbers; it’s about uncovering stories.
STEM students are like modern adventurers, armed with tools to explore new ideas.
So, embrace the challenges, the unknowns, and the fun possibilities. With quantitative research, you can find answers to new questions and shape the future of STEM.
And always remember, awesome discoveries start with a simple question and a curious mind.
So, ask boldly, explore eagerly, and let your love for learning guide you. The world is waiting for your breakthroughs, one data point at a time.
Frequently Asked Questions
How do i choose the right quantitative research topic.
When selecting your topic, think about what interests you, what’s trending in STEM, and where there are gaps in research that pique your curiosity. Find something that genuinely grabs your attention.
Where can I find data for quantitative research in STEM?
Data sources can vary based on your topic. You can access academic databases, government research agencies, and even conduct experiments or surveys, depending on your specific research focus.
Leave a Comment Cancel reply
- Open access
- Published: 16 May 2018
A study of the correlation between STEM career knowledge, mathematics self-efficacy, career interests, and career activities on the likelihood of pursuing a STEM career among middle school students
- Karen A. Blotnicky 1 ,
- Tamara Franz-Odendaal 2 ,
- Frederick French 3 &
- Phillip Joy 4
International Journal of STEM Education volume 5 , Article number: 22 ( 2018 ) Cite this article
125k Accesses
114 Citations
13 Altmetric
Metrics details
A sample of 1448 students in grades 7 and 9 was drawn from public schools in Atlantic Canada to explore students’ knowledge of science and mathematics requirements for science, technology, engineering, and mathematics (STEM) careers. Also explored were their mathematics self-efficacy (MSE), their future career interests, their preferences for particular career activities, and their likelihood to pursue a STEM career.
Analysis revealed that while older students had more knowledge about mathematics/science requirements for STEM careers, this knowledge was lacking overall. Also, students with higher MSE were more knowledgeable about STEM career requirements. Furthermore, students with higher MSE and STEM career knowledge were more likely to choose a STEM career. Students with greater interest in technical and scientific skills were also more likely to consider a STEM career than those who preferred career activities that involved practical, productive, and concrete activities.
Conclusions
The results of this study show that students in middle school have a limited STEM career knowledge with respect to subject requirements and with respect to what sort of activities these careers involve. Furthermore, students with low MSE have a declining interest in STEM careers. Our data thus support the need to improve access to knowledge to facilitate students’ understanding of STEM careers and the nature of STEM work. Exposure of students to STEM careers can enhance their interest in pursuing careers involving science, technology, engineering, and mathematics.
Globally, youth vary considerably in their level of science, technology, engineering, and mathematics (STEM) career knowledge, their career interests, and their intentions of pursuing a STEM career. STEM career knowledge, defined as a student’s familiarity with a particular STEM career, varies considerably based on the school’s STEM career guidance. The level of STEM career knowledge an individual has will directly affect one’s intentions of pursuing a STEM career in the future (Compeau 2016 ; Nugent et al. 2015 ; Zhang and Barnett 2015 ). Without adequate knowledge, there is a risk that students will dismiss a STEM-based career path as a potential option for their future. Consequently, student interest in a particular STEM career will wane, which will negatively influence their desire to participate in activities that serve to increase STEM career knowledge and awareness. Indeed, interventions have shown that equipping students with STEM career knowledge early increases their motivation to take more science and mathematics courses in high school (Harackiewicz et al. 2012 ).
Students’ career interest and their preferred future career activities will also affect their intention of pursuing a STEM career. A key predictor of STEM career interest at the end of high school is interest at the start of high school (Sadler et al. 2012 ). However, the positive attitudes towards science identified in youth age 10 sharply declines by age 14 (Murphy and Beggs 2005 ; Tai et al. 2006 ); the junior high school years are typically ages 12–14 years. An extensive study in 2015, surveying 24,000 students, showed that occupational intentions change dramatically between the 9th and 11th grade and that the relationship between STEM intention and motivation is highly time-sensitive (Mangu et al. 2015 ).
Both STEM career knowledge and career interests are also influenced by society at large. These society influencers include role models that students are exposed to either in person or through the media, the individual students interact with on a daily basis such as teachers, family members, and peers, as well as students’ extracurricular experiences (Dabney et al. 2012 ; Harackiewicz et al. 2012 ; Nugent et al. 2015 ; Sahin et al. 2014 ; Sahin et al. 2015 ; Schumacher et al. 2009 ; Sjaastad 2012 ; Steinke et al. 2009 ; Zhang and Barnett 2015 ). Collectively, these influencing factors predict the self-efficacy (i.e., one’s belief in one’s ability) youth hold about their career options as well as their outcome expectancies (Mangu et al. 2015 ). Self-efficacy is considered a major predictor guiding the selection of majors during high school and post-secondary education (Heilbronner 2009 ; Kelly et al. 2013 ).
The grades 7 through 9 years (12–15-year-olds) are the key time period for influencing STEM career interest and for building this self-efficacy with respect to mathematics and science. Thus, it is during the junior high (middle) school age that a student’s beliefs about competency and interests begin to solidify (Simpkins et al. 2006 ). It is at this time that student engagement activities and career knowledge should be at its highest. Social cognitive career theory (Lent 2005 ) acknowledges and hypothesizes that career interests, choice, and personal goals form a complex human agency process that includes performance, self-efficacy, and outcome expectations. For example, self-efficacy is positively related to student academic performance and science self-efficacy has been shown to impact student selection of science-related activities, which impacts their ultimate success and helps maintain interests (Britner and Pajares 2006 ; Parker et al. 2014 ; Richardson et al. 2012 ).
Early interest in STEM topics is an excellent predictor for later learning and eventual career interests and choice (DeBacker and Nelson 1999 ). Contextual and individual variables influence these social cognitive variables including factors such as parental, teacher, and peer cultural expectations (Lent et al. 1994 ). Nugent et al. ( 2015 ) found support for the social cognitive career theory (Lent et al. 1994 ) as a framework for examining STEM learning and career orientation outcomes by providing a way in which to view the socio-contextual, motivational, and instructional factors that can impact youth STEM interests.
Although 88% of parents believe they can help guide their children’s learning, less than 28% actually discuss the value of a STEM education with their children (“Let’s Talk Science Canada Annual Report,” 2015 ). Recent studies have also indicated that junior high students have an unclear view about engineering (Compeau 2016 ; Karatas et al. 2011 ) and science (Masnick et al. 2010 ) yet these are critical years in which to build STEM interest. The present paper builds on our previous study (Franz-Odendaal et al. 2016 ) and explores students’ knowledge of STEM career mathematics/science requirements and their mathematics self-efficacy (MSE) and how these shape students’ career interests and preferred career activities. Differences among grade 7 and 9 students with respect to career interests and activities, and the likelihood of pursuing a STEM career will be examined. While gender differences are important because STEM stereotypes are heavily biased towards males, these differences are beyond the scope of the current study. This study will examine who, what, and how youth are influenced in STEM career choice.
This study captured five main areas of interest: student knowledge of mathematics and science requirements that lead to STEM careers, MSE, career interests, career activity preferences, and their correlation with the likelihood to consider pursuing a STEM career among youth. Based on the literature, the following research questions were developed to guide this research.
RQ1: What is the correlation between grade level and students’ knowledge of high school requirements for STEM careers?
RQ2: What is the correlation between MSE and students’ knowledge of high school requirements for STEM careers?
RQ3: What is the correlation between MSE and students’ career interests and/or their preference for particular career activities?
RQ4: What is the association between student preferences for career interests and preferred career activities with grade level?
RQ5: What are the relationships between the following factors and the likelihood that students will choose a STEM career: grade level, MSE, student knowledge of mathematics/science requirements for post-secondary study for STEM careers, career interests and preferred career activities?
These research questions have not been explored in the context of Atlantic Canada, thus making this study relevant to the education system within Canada and globally.
Grade 7 and 9 students in the four Canadian Atlantic provinces (New Brunswick, Nova Scotia, Prince Edward Island, and Newfoundland) completed an online survey during their school hours. This research was approved by the university research ethics board. Permission to collect data in the schools was obtained from school board superintendents and parents. Schools were purposefully chosen from school families in geographic areas across Atlantic Canada. English and French language schools were included in the study. Data were weighted to ensure that the sample was representative by grade level, from each of the four Atlantic provinces. A total sample size of 1448 students was obtained across all four provinces in Atlantic Canada: New Brunswick (33%), Nova Scotia (38.4%), Prince Edward Island (6.5%), and Newfoundland-Labrador (22.1%). The sample was split almost evenly between grade 7 (48%) and grade 9 (52%). The sample was balanced with respect to gender (58% female to 42% male). Students ranged in age from 11 to 20 years with an average age of 13.5 years and a median age of 14 years (SD = 1.1). Grade 7 students had an average age of 12.6 years (SD = .6) with a median age of 13 years. Grade 9 students had an average age of 14.5 years (SD = .6) with a median age of 14 years.
Five different measures were used in this study. These included measures of STEM career knowledge, MSE, career activity preferences, career interests, and likelihood to choose to pursue a STEM career. These measures were incorporated into the study based on earlier reviews that found that studies’ examining factors influencing career choice have been criticized for failing to account for the complexity of career choices and career decision-making (Patton and McMahon 2006 ) and for being too static in their view of career development (Hirshi 2011 ).
STEM career knowledge score
A STEM career knowledge (SCK) score was created to capture students’ knowledge about the requirements for high school mathematics and science in STEM careers. Students were presented with 12 STEM careers and asked to indicate whether they believed that the training for each of the careers required having taken mathematics or science in high school. Students could respond “yes” if they believed the career required high school mathematics or science based on their knowledge of the entrance requirements for Canadian colleges and universities. They could respond “no” if they believed that the career did not require high school mathematics or science, or they could choose “uncertain” if they were not sure that high school mathematics and/or science were required for that career. The list included careers students are commonly exposed to (such as veterinarian, pharmacist, and oral hygienist) as well as careers that are likely less familiar to them (such as mechanical engineer, geologist, and land surveyor). The list included mechanical engineer, computer hardware designer, pharmacist, medical technologist, geologist, veterinarian, oil industry engineer, physiotherapist, oral hygienist, nutritionist, land surveyor, and ophthalmologist. The list was provided to students in no particular order.
A score was calculated to capture students’ knowledge based on these responses. “Yes” responses were scored as “1,” “uncertain” responses as “0,” and “no” scored as “− 1.” The responses were then summed to obtain a basic SCK score per student. The SCK score was calculated only for students who had rated at least one third of the careers in the list. The SCK was validated using confirmatory factor analysis (CFA) and reliability analysis.
Mathematics Self-Efficacy Scale
In an attempt to offer a more complete perspective on the process of career decision-making, Hackett and Bertz (Hackett and Betz 1981 ) drew on the work of Bandura ( 1977 ) to introduce the concept of self-efficacy to the career development literature noting its potential to help understand the complexity of career decision-making such as the underrepresentation of women in traditional male-dominated career fields. Self-efficacy referred to the belief that a person had in their own ability to successfully perform a particular behavior based on their perception of their capability and the likelihood of their achieving success in that activity.
The second measure used in this analysis was a MSE scale. Students were asked to describe their experiences in mathematics by rating each of the following statements on a scale ranging from (1) Strongly disagree to (5) Strongly agree: I get good grades in mathematics; I learn quickly in mathematics; I look forward to my mathematics class; I feel tense doing mathematics problems; I feel helpless doing mathematics problems. Negatively phrased items were reverse-coded to maintain consistency in the MSE scale. Students’ responses to these five statements about their experiences in mathematics were then coded into dichotomies to create five separate measures. Dichotomies were created by assigning a value of “1” to those who were most comfortable with Likert scale ratings of 4 or 5 to the statements and assigning a value of 0 to those who were less confident and comfortable with Likert scale ratings of 1 through 3. These five measures were then summed to get a single MSE scale that would reflect higher measures for those who were the most confident and comfortable with mathematics. The MSE scale was validated using confirmatory factor analysis (CFA) and reliability analysis. The resulting MSE score ranged from 0 to 5. The MSE scale was then divided into two subgroups to create an MSE score for further analysis. The MSE score consisted of those with low MSE (scores of 0 through 3) and high MSE (scores of 4 or 5). The goal in using this breakout was to identify students who were the most comfortable and confident in their mathematics experiences.
Career activities and career interests
Social Cognitive Career Theory (SCCT) (Lent et al. 2010 ) has continued to evolve to include person and environmental and socio-demographic variables as well as interest and career choice models. The SCCT argues that people develop interests (actively likes and dislikes) largely on the basis of their beliefs about their self-efficacy and the outcomes their efforts could achieve. Ultimately, people become interested in activities they believe they can perform well. Therefore, people develop goals to pursue academic and career activities that are consistent with their interests as well as with their self-efficacy and outcome expectations (Sheu et al. 2010 ). Thus, career activities and career interests are highly correlated. For these reasons, student ratings of self-perceptions of their career interests, and also their career activities, were included.
The SCCT has been found to support self-efficacy and outcome expectations as significant predictors of interest, that interests partially mediate the relation of self-efficacy and outcome expectations, and that self-efficacy relates to outcome expectations across Holland’s (1997) broad occupational themes as utilized in the current study (Sheu et al. 2010 ). This alignment was felt to provide a rationale for the use of student ratings of interests, activities, and Holland’s broad occupational themes as a comprehensive way of gaining insight into the complexity of career decision-making of junior high students.
Hollands’ Theory of Career Choice and Development (Holland 1973 ) focused on six basic personality types: realistic (practical); investigative (analytical, curious); artistic (expressive, original); social (working/helping others); enterprising (goal oriented); and conventional (ordered). Individuals are not limited to one personality type and many exhibit characteristics on more than one type. Holland ( 1973 ) argued that everyone has career decisions to make at various stages of their lives. As well, he argued that everyone can serve as both a coach and/or a player in those decisions depending on their role, situation, and knowledge. Reflecting on the life stage, the environment, and the knowledge one has of their own particular type of preferred approach to life plus knowledge of the interaction among a variety of factors such as the cultural, social, academic, and family influences on the decisions that each individual makes about their life career. These are not perfect, single, nor static events and depend on self and other perceptions of a wide range of factors. However, at a point in time, they represent what each person conceptualizes as a satisfying career for them. Holland ( 1973 ) argued that his theory of careers was really intended to help practitioners, researchers, and students in education and social science to address a fuller understanding of vocational choice and to be helpful in professional counseling. Miller ( 1998 ) stated that Holland’s theory can be used to help individuals explore career choices. More recently, Olitsky ( 2014 ) used Holland’s theory of career and educational choice when researching the earnings of STEM majors, indicating that the underlying theory is still relevant. Since career interests and career activities are highly correlated, they were measured separately.
The third measure used in this analysis was a ranking of the preferred career activities using Holland’s Theory of Career Choice and Development (1973). Students were asked to rank six different career activities from (1) Most favorite to (6) Least favorite. Each of the career activities was then analyzed based on the percentage of students who rated it in their top 2 favorites. The career activities studied in this research included the following: (1) artistic, unusual, and creative activities; (2) working on practical, productive, and concrete activities; (3) taking responsibility, providing leadership, and convincing others; (4) things being organized into routines and having an order; (5) learning by reading, study, analysis, or investigation; (6) helping others and being concerned for the welfare of others.
The fourth measure used in this analysis was a ranking of career interests also based on Holland’s Theory of Career Choice and Development (Holland 1973 ). Students were asked to rank six different career interests from (1) Most favorite to (6) Least favorite. Each of the career interests was then analyzed based on the percentage of students who rated it in their top 2 favorites. These interests were (1) working with people; (2) creative skills and expression; (3) technical and scientific skills; (4) manual and mechanical skills; (5) leading, persuading, and directing others; and (6) routines and adhering to standards of performance.
Likelihood to pursue a STEM career
The final measure used in this analysis was the likelihood that students would consider choosing a STEM career in their future. Students were asked how likely they would be to choose a career that is science-related (including science, engineering, health, or technology). Likelihood was measured using the following Likert scale: (1) Very unlikely, (2) Somewhat unlikely, (3) Somewhat likely, and (4) Very likely. This scale was recoded into a dichotomous variable for use in bivariate logistic regression: Students who were somewhat likely or very likely to choose a STEM career were coded as “1,” and those who were somewhat unlikely or very unlikely to choose a STEM career were coded as “0.”
Data analysis
Data were analyzed using the SPSS software (IBM Corp 2013 ). Descriptive statistics were used to provide an overall analysis of the data. Various statistical tests were selected based on the level of data measurement and data distributions (McDaniel et al. 2014 ; Hair Jr. et al. 2010 ). t tests were used to explore differences in average ratings between groups. Chi-square was used to analyze associations between nominal and ordinal variables. Analysis of variance (ANOVA) was used to evaluate significant differences between average ratings and measures involving categorical variables with more than two response levels (McDaniel et al. 2014 ). Logistic regression was used to explore research questions involving interval and ratio-scaled variables (Hair Jr. et al. 2010 ). Brown-Forsyth exact tests were used with ANOVA to compensate for violations of homogeneity of variance (IBM Corp 2013 ). Bonferroni post hoc tests were used to detect significant differences between groups for significant ANOVA results (IBM Corp 2013 ; Hair Jr. et al. 2010 ). Data were weighted to reflect the population of students by grade level and province across Atlantic Canada.
Bivariate logistic regression was conducted to explore the relative contribution of the following factors on the likelihood that students would choose a STEM career: SCK score, MSE score, grade level, career interests, and career activities. Grade level, career interest, and career activities were coded as dichotomies for the regression analysis as follows: grade level (grade 9 = 1, grade 7 = 0), career interests (rated in top 2 favorites = 1, not rated in top 2 favorites = 0), career activities (rated in top 2 favorites = 1, not rated in top 2 favorites = 0).
Three regressions were created to explore the research questions. The first analysis regressed grade level, SCK score, and MSE score against the likelihood to pursue a STEM career. Two more regressions were conducted: one to regress career activities and a second to regress career interests against the likelihood to pursue a STEM career as a dependent variable. Measures for career activity and career interests showed a high level of multicollinearity between the two sets of variables. Separating these predictors into two different regressions eliminated problems with multicollinearity.
We first describe the results for each of the measures used in this study and then answer our research questions (RQ1–5).
Student knowledge of mathematics and science requirements for STEM careers
We assessed student’s knowledge of high school requirements for STEM careers, by asking students to indicate whether a career required mathematics and/or science (Table 1 ). Mechanical engineer was noted by 71.4% of students as having a high school mathematics/science requirement. Two careers (land surveyor and ophthalmologist) were noted by less than half of the students as requiring high school mathematics or science. Five careers were classified as requiring mathematics and science by 65.6 to 68.2% (veterinarian, geologist, medical technologist, pharmacist, computer hardware engineer). Four of the careers were listed as requiring mathematics and science by 51.8 to 58.6% of the students (nutritionist, oral hygienist, physiotherapist, oil industry engineer). What is notable in the students’ responses are that most students seemed confident of their career classification in that they answered “yes” or “no” and not the option of “uncertain,” indicating that they were confident in their choice. The percentage of students saying that they were uncertain if a career required mathematics or science for post-secondary study was low and ranged from 12.5 to 32.6% across all of the careers with half of the uncertain responses ranging from 12.5 to 13% of students. Table 1 shows the results of high school mathematics/science requirements for STEM careers.
Students’ responses were summed to obtain an overall SCK score. A factor analysis of the career ratings was used to ensure it was unidimensional, and reliability of the score was measured using Cronbach’s alpha. The confirmatory factor analysis was statistically significant (KMO = .961, p < .01). Cronbach’s alpha was .95 which meets the criterion for reliability.
The SCK score ranged from − 12 to + 12, with an average score of 4.6 (SD = 7.6; Fig. 1 ). The average SCK score was low, indicating a lack of familiarity with the mathematics and/or science requirements of STEM careers. Approximately 8% of students did not correctly classify any of the careers as having a high school mathematics and/or science requirement. Only 36.4% of students had high SCK scores having correctly classified 10 to 12 careers. The top quartile of students scored 11 or better while the bottom quartile scored 0 or less than 0 out of the 12-point score. A summary of the SCK score is in Fig. 1 .
STEM career knowledge (SCK) score
Overall, these results suggest that STEM career knowledge is limited among middle school students. Results also reveal that students seem to be unaware of their limited knowledge regarding STEM career preparation.
Students’ mathematics self-efficacy
In order to determine whether MSE was correlated with students STEM career knowledge (RQ2) and/or between MSE and career interests and/or preferred career activities (RQ3), we first determined the MSE scale for the cohort. The MSE scale ranged from 0 (No self-efficacy) to 5 (High self-efficacy). The distribution of the Math Self-Efficacy Scale is shown in Fig. 2 .
Mathematics Self-Efficacy (MSE) Scale
A confirmatory factor analysis of the measures in the MSE scale indicated that it was unidimensional and reliable. The factor analysis was statistically significant (KMO = .698, p < .01). Cronbach’s alpha was .72 which is acceptable for a scale analysis. These results suggest that over half of the students had a relatively high MSE and about one third of students had low MSE.
Career activities and interests
In order to assess students’ preferred career activities and their career interests, students were asked to select their favorites. Students were presented with a list of six career activities and asked to indicate which activities were in their top 2 favorites. These measures were recoded into dichotomies for further analysis. There was a very even spread of students rating career activities in their top 2 favorites, ranging from 32.1 to 45.9%. Most of the activities were listed in their top 2 favorites by about one third of the students. The results revealed that artistic, unusual, and creative activities were most commonly listed in the top 2 favorite career activities. The career activity with the lowest rating was helping others and being concerned for their welfare. The results are summarized in Table 2 .
Students were also presented with a list of six career interests and asked to indicate which career interests were in their top 2 favorites. These measures were recoded into dichotomies for further analysis. The percentage of students rating career interests as their top 2 favorites ranged from 21.9% for routines and adhering to standards to working with people at 49.8%. The results are summarized in Table 3 .
Likelihood of choosing a STEM career
Next, we assessed whether students were interested in pursuing a STEM career. Nearly 70% percent of students surveyed revealed that they were either somewhat likely or very likely to pursue a STEM career. On a scale of (1) Very unlikely to (4) Very likely, an average rating of 2.9/4 indicated that students were somewhat likely to pursue a STEM career. The results appear in Table 4 .
The association of grade level and STEM career knowledge
The first research question (RQ1) explored the correlation of grade level and STEM career knowledge. There was a statistically significant difference in the average SCK score by grade, with grade 9 students scoring higher than grade 7 students (5.7 vs 3.3, t = − 5.69, df = 1209.7, p < .01). While it is good to see that students appear to acquire more knowledge of STEM career requirements in middle school grades, it is concerning that students in grade 9 still had a low average SCK score since this is the year in which students begin to choose subject classes in Atlantic Canada. This indicates that more work is needed to ensure students have the correct information about STEM career requirements in time for them to make informed decisions about high school course selection.
Correlation between mathematics self-efficacy and the STEM career knowledge
The second research question (RQ2) focused on whether there is a correlation between students with higher MSE and knowledge of STEM career requirements. An analysis of variance revealed that students with high self-efficacy (MSE scale = 4 and 5) had significantly higher SCK scores than students who did not score as highly in the MSE scale (BF = 8.7, df = 5, p < .01). Students with high MSE had a SCK score of 6.6 out of 12, while students with lower MSE scores had average SCK scores ranging from 2.8 to 4.8. The results are shown in Table 5 .
These results for RQ2 show that students who report more confidence and comfort in mathematics tend to be more knowledgeable about mathematics/science requirements for STEM careers. This is a correlation only and cannot be interpreted as a causal relationship since survey data cannot be used to measure causality.
Correlation between mathematics self-efficacy and students’ preferred career activities and their career interests
Our third research question (RQ3) explored whether there was a correlation between MSE and students’ career interests and preferred career activity. There were statistically significant differences by students’ preferred career activities for the MSE scale. MSE scale totals were sorted into two groups to create an MSE score for further analysis. Those with low MSE scale totals (0 through 3) were assigned an MSE score of 0, and those with high MSE scale totals (4 and 5) were assigned an MSE score of 1. A chi-square analysis revealed that only one career activity differed significantly based on students’ MSE scores. Reading, study, analysis, and investigation was listed in the top 2 favorites for career activities by 36.5% of students who had high MSE scores (between 4 and 5) when compared to 28.4% of students with low MSE (0 through 3) ( χ 2 = 7.979, df = 1, p < .01). The remaining career activities did not differ significantly based on students’ MSE. The results are summarized in Table 6 .
These results show that most of the preferred career activities had no correlation at all with MSE scores. However, reading, study, analysis, and investigation are the hallmarks of a mathematics-, science-, or technology-based activity. Therefore, it is reasonable that students who are confident and comfortable with mathematics would also enjoy reading, study, analysis, and investigation.
In order to explore whether there is a correlation between MSE and student’s career interests, a chi-square analysis was conducted. The chi-square analysis revealed that only one career interest differed significantly based on students’ MSE score. This career interest was technical and scientific skills. This career interest was listed in the top 2 favorites for career activities by 43.8% of students with high MSE score (between 4 and 5) compared to 36.0% of students with low MSE score (0 through 3) ( χ 2 = 6.558, df = 1, p = .01). The remaining career interests did not differ significantly based on students’ MSE scores. As with the results for career activities, these results show that most of the career interests were not significantly correlated with MSE and all of the career interests were rated in the top 2 favorites by less than half of the students. It is reasonable that students who are confident and comfortable with mathematics would also be interested in careers involving technical and scientific skills. The results are summarized in Table 7 .
The correlation between grade level and students’ career interests and preferred career activities
The fourth research question (RQ4) addressed whether grade level was associated with student preferences for career interests and activities. There were statistically significant differences by grade regarding some of the career interests, thereby satisfying the first part of the fourth research question. More grade 7 than grade 9 students listed manual and mechanical skills in their top 2 favorites (36.5 vs 29.4%, χ 2 = 6.84, df = 1, p < .01), as well as creative skills and expression (45.4 vs 37.0%, χ 2 = 8.73, df = 1, p < .01). More grade 9 than grade 7 students ranked “working with people” in their top 2 favorites (52.8 vs 46.8%, χ 2 = 4.21, df = 1, p < .05). These results are summarized in Table 8 .
More grade 7 than grade 9 students listed practical, productive, and concrete activities in their top 2 favorites (42.1 vs 34.7%; χ 2 = 6.9, df = 1, p < .01). More grade 9 than grade 7 students rated helping others and being concerned for their welfare in their top 2 favorite career activities (34.9 vs 28.6%; χ 2 = 5.4, df = 1, p < .05) as well as having things organized into routines and having order (39.4 vs 29.4%; χ 2 = 13.2, df = 1, p < .01). There were no statistically significant differences by grade level for the other career activities studied.
This trend is similar to that emerging in the analysis of career interests. In general, students in the higher grade focused more on activities involving helping others and being less attracted to careers that involved practical applications or routines. The results are summarized in Table 9 .
The correlations between students’ STEM career knowledge, mathematics self-efficacy, and grade level on their likelihood to choose a STEM career
The fifth research question (RQ5) focused on how several aspects might relate to students’ likelihood of choosing a STEM career. These areas included grade level, MSE, knowledge of STEM careers, and preferences for various career interests and activities.
First, a logistic regression was conducted to determine whether or not grade level, STEM knowledge, and MSE score were associated with students’ likelihood to pursue a STEM career. The hypothesized regression model was likelihood of choosing a STEM career (ODDS) = f (grade level, STEM knowledge score, MSE score). A test of the full regression model against an intercept-only model was statistically significant ( χ 2 = 76.85, df = 3, p < .01). The regression was strong with a McFadden’s R 2 = .85.
The regression analysis correctly classified 70.6% of all cases and 95.3% of those who were likely to choose a STEM career. The regression revealed that students with stronger SCK scores were marginally more likely to pursue a STEM career than were students with weaker SCK scores (odds ratio = 1.04, probability = .51). However, students with high MSE scores were 1.3 times more likely to pursue a STEM career than were those who had lower MSE scores (probability = .56). Grade level was not a statistically significant predictor of the likelihood of pursuing a STEM career.
These results showed that students’ knowledge of STEM careers and their self-efficacy in mathematics were statistically significant factors in the likelihood that they would pursue a STEM career, while STEM career knowledge was a modest contributor. Also, students in grade 9 were not more likely to pursue a STEM career than were students in Grade 7. However, research has shown that occupational intentions change dramatically between 9th and 11th grades and the relationship between STEM intention and motivation is very time-sensitive (Mangu et al. 2015 , p.55). The results are summarized in Table 10 .
These regression results reveal that individual student characteristics, MSE, and SCK are better predictors of the likelihood to pursue STEM careers than student grade level. Individual strengths and weaknesses, as well as students’ knowledge and competency, are better indicators of future career paths than grade level.
The correlation between students’ career interests and their likelihood to pursue a STEM career
A second logistic regression was conducted to explore whether or not students’ preferred career interests was correlated with their likelihood to pursue a STEM career. Six career interests were explored in the analysis. The hypothesized regression model was likelihood of choosing a STEM career (ODDS) = f (manual and mechanical skills; technical and scientific skills; creative skills and expression; working with people; leading, persuading, and directing others; routines and adhering to standards). A test of the full regression model against an intercept-only model was statistically significant ( χ 2 = 119.94, df = 6, p < .01). The regression was reasonably strong with a McFadden’s R 2 = .73. The regression analysis correctly classified 72% of all cases and 96.6% of those who were likely to choose a STEM career.
The regression revealed that students who rated technical and scientific skills in their top 2 favorite career interests were 5.4 times more likely to pursue a STEM career (probability = .84). Students who rated working with people in their top 2 favorites were 1.5 times more likely to pursue a STEM career (probability = .61). Students who rated creative and expressive skills in their top 2 favorite career interests were less likely to pursue a STEM career than those who rated creative and expressive skills highly. Their odds of pursuing a STEM career were only .70 of those who did not rate creativity and expressiveness among their favorite career interests. Their probability of pursuing a STEM career was .41. The remaining career interests were not statistically significant predictors of the likelihood of pursuing a STEM career (manual or mechanical skills; leading, persuading, or directing others; routines and adhering to standards). These results provide evidence for the fifth research question in that three out of the six career interests measured did have a statistically significant correlation with the likelihood that a student would consider pursuing a STEM career. The results are summarized in Table 11 .
These results indicate that student preference for technical and scientific skills and careers involving working with people enhance the likelihood of pursuing a STEM career, while students who prefer careers involving creative skills and expression are less likely to do so. While a focus group could better explore the students’ preferences for creativity and creative careers, this level of detail is not possible in large sample survey-based research and is outside of the scope of this study. Other career interests that focus on mechanical, manual, or routine activities, or those involving leadership, do not predict the likelihood of students pursuing a STEM career and are not significantly correlated with STEM career choice.
A third logistic regression analysis was conducted to determine whether students’ career activity preferences were correlated whether or not they were likely to pursue a STEM career. Six career activities were explored in the analysis. The hypothesized regression model was likelihood of choosing a STEM career (ODDS) = f (practical, productive, concrete activities; reading, study, analysis, and investigation; artistic, unusual, and creative activities; taking responsibility, providing leadership, and convincing; and helping others and being concerned for their welfare). A test of the full regression model against an intercept-only model was statistically significant ( χ 2 = 32.883, df = 6, p < .01). The regression was reasonably strong with a McFadden’s R 2 = .78. The regression analysis correctly classified 72% of all cases, and 100% of those who were likely to choose a STEM career.
The regression revealed that students who preferred career activities involving reading, study, analysis, and investigation were 1.8 times more likely to pursue a STEM career (probability = .65) than those who did not prefer such activities. Students’ rating career activities involving routines and having an order were 1.5 times more likely (probability = .60) to pursue a STEM career than those who did not prefer such activities, while students with preferences for practical, productive, and concrete career activities were 1.5 times more likely to pursue a STEM career (probability = .60) compared to those who did not prefer such activities. The remaining career activities were not statistically significant predictors of the likelihood to pursue a STEM career (artistic, unusual, and creative activities; taking responsibility, providing leadership, and convincing others; helping others and being concerned for their welfare). These results revealed three out of the six career activities measured did have a statistically significant correlation with the likelihood that a student would consider pursuing a STEM career. The results are summarized in Table 12 .
These results stand in contrast to those for students’ career interests and the likelihood of pursuing a STEM career. Unlike the career interest analysis, students seeking routine career activities are more likely to pursue a STEM career. Also, students who ranked career interests involving helping others were more likely to pursue STEM careers, but this analysis showed that student preference for career activities involving helping others and being concerned for their welfare was not a statistically significant indicator of their likelihood to pursue a STEM career. Further, these results differ somewhat in terms of students’ preferences for practical activities. While career interests involving manual or mechanical skills were not statistical indicators of the likelihood of pursuing a STEM career, career activities involving practical, productive, and concrete activities were statistically significant. The career activity involving reading, study, analysis, and investigation was also statistically linked to students’ likelihood to pursue a STEM career, which seems reasonable given that such activities are at the heart of many STEM careers.
Youth vary widely in their career knowledge, interest, and intentions. Factors investigated in the present study examined STEM career knowledge, MSE, career activities, career interests, and the likelihood of students to pursue a STEM career.
Knowledge and self-efficacy
Results of the present study align with recent findings by Compeau et al. ( 2016 ), Nugent et al. ( 2015 ), and Zhang and Barnett ( 2015 ) show that self-efficacy along with knowledge of STEM careers are significant factors in whether or not adolescents pursue STEM careers. Findings also indicated that career knowledge is limited among middle school students and students seem to be unaware of their limited knowledge regarding STEM preparation. While approximately 70% of students reported that mathematics was an important requirement for a career in mechanical engineering, computer hardware design, and pharmacy, 50% or less were aware that it was also important in careers for ophthalmology, land surveyor, nutrition, and oral hygienist.
The issue of self-efficacy takes on particular significance as students progress through high school. Previous research by Murphy and Beggs ( 2005 ), Heilbronner ( 2009 ), and Mangu et al. ( 2015 ) have noted how young women have a lower self-efficacy in STEM during high school years. Previous research has also shown that interest in STEM and motivation to pursue STEM activities tends to wane over time for all high school students. The results of the current study agree with earlier findings that lower levels of MSE exist; we found approximately 34% of participants had low MSE scale totals. These findings raise concerns about the combined effects of students’ low MSE and their declining interest in STEM from early through to later grades and on the numbers of graduating high school students who will be inclined to choose a STEM career.
Results of the current study demonstrated that students in grades 7 and 9 had a broad range of favorite career activities with the majority (approximately 46%) stating that their strongest preference was for artistic and creative types of activities. Also, all of the possible activities were selected by at least one third of the group. Interestingly, approximately 50% of participants selected their career interest as being “working with people,” but relative to career activities, only one third of participants selected “helping people.” However, this is not surprising given that one can have an interest but may not want to have a career working in that activity. For example, one may be interested in art, but have no interest, or lack sufficient talent, to pursue a career in the field (Holland 1973 ). Also, middle school students may not be able to discriminate between the nuances between career activities and interests in the way that older students and young adults would. Although a focus group study may be able to further elucidate this issue, this is beyond the scope of the current study.
Working with others and participating in creative types of activities are important findings that relate to current issues in education in Canada. A recent study (Ayar and Yalvac 2016 ) found that many STEM careers are team-based, creative, and require technical, scientific, and problem solving skills. However, in Canada, many post-secondary programs continue to focus more on memorizing and replicating science content knowledge. Further study of this possible implication would be worthwhile.
While approximately 70% of participants stated they were likely to choose a STEM career, 30% were less likely to do so. Not all students have the financial means to pursue a career interest. In addition, career interest and motivation are highly time sensitive (Mangu et al. 2015 , p.55). Considering findings from studies such as Ayar and Yalvac ( 2016 ), as well as what we know about the decline in interest in STEM careers as students mature, these results suggest that there is room for increasing awareness, STEM career interest, and providing for better knowledge acquisition in the area of STEM careers. As well, our data suggests that alternative ways of teaching and evaluating STEM courses should be considered. Perhaps a greater emphasis on authentic means of teaching and evaluating STEM content that involves collaboration, problem solving, and application of STEM knowledge might serve to engage learners in more meaningful ways, thereby enabling continued motivation and interest in STEM careers as students progress through secondary and post-secondary education. Does a higher MSE lead students to consider pursuing STEM careers and lead them to becoming more informed about the career requirements or do students who have higher knowledge of STEM careers become more competent in mathematics? Are these factors simply correlational and reflect students who are high in both measures or low in both measures? While these questions cannot be answered in this research, it is interesting to note that MSE may play a role, or be a leading indicator, for STEM career knowledge.
Influence of mathematics self-efficacy on career knowledge, interest and activities
Our results indicate that while there is a relationship between career knowledge and MSE, we did not find a relationship among career interests or activities with MSE. The assumption that having a positive sense of mathematics skill would correlate with STEM career interests and activities was not supported. Follow-up research involving interviews with participants about their understanding of career interests, activities, and MSE, would provide more an in-depth understanding. Based on findings by Simpkins et al. ( 2006 ) it was expected that there would be a relationship among interests, activities and MSE as their findings indicated that in junior high beliefs about competency and interests begin to solidify. Further research may help to uncover reasons for not seeing such a correlation in this analysis.
Influence of grade level on STEM career knowledge, interest, and activities
Results indicate that there were significant differences between grade 7 and grade 9 students in the present study relative to STEM career knowledge. Overall, students in grade 9 were more knowledgeable than grade 7 students about STEM careers. The differences formed an interesting and consistent pattern that more grade 7 students expressed interest in manual and mechanical skills than grade 9 students who tended to have more interest in working with people. Further, more grade 7 students expressed interests in practical and concrete types of activities while more grade 9 students expressed interests in helping people and being concerned for their welfare. Reasons for this shift are not clearly understood. As noted by Lent ( 2005 ), career interest, choice, and personal goals form a complex chain involving performance, self-efficacy, and outcome expectations. As well, socio-cultural factors also need to be considered along with opportunity for exposure (Fouad and Smith 1996 ; Kuncel et al. 2005 ; Lent et al. 1994 ). As with the relationship among self-efficacy, knowledge, interests, and activities, in-depth research involving student interviews may result in greater understanding of the reasons for these shifts and their impact on later careers.
Factors influencing positive statements involving the likelihood of choosing a STEM career
Regression analyses revealed that participants with stronger STEM career knowledge were slightly more likely to pursue a STEM career and that students with higher MSE scores were also slightly more likely to choose a STEM career. Also, grade level was not a differentiating factor, which was anticipated given the small distance between the experiences of grade 7 versus grade 9 students. As noted in many previous studies (Lent et al. 1994 ; Kuncel et al. 2005 ), knowledge of STEM careers and self-efficacy in mathematics are statistically significant factors in the likelihood that participants will pursue STEM careers.
Interest in technical and scientific skills is a strong predictor of the likelihood of pursuing a STEM career with those who indicated a preference for technical and scientific skills being 5.4 times more likely to indicate the likelihood of choosing a STEM career compared to those who rated working with people as their stronger interest. Indeed, preferences for practical, productive, and concrete activities also indicated a stronger likelihood of pursuing STEM careers than those who do not prefer such activities. Implications of these findings point to improving methods for providing information on the skills and nature of the work in STEM careers particularly in fields such as engineering and technology (which have an important focus on team work, problem solving, and creativity) as well as on technical and scientific skills.
Overall, results of the present study show that career knowledge is limited among middle school students and that they have a declining interest in STEM and have low MSE scores. Students are interested in careers that involve a wide variety of activities but do not appear to relate these activities to STEM careers. Our results point to the importance of finding and expanding on ways to increase authentic learning opportunities in secondary school in Atlantic Canada such that students are better able to participate in collaboration, problem solving, and the application of scientific knowledge in their classes. Such learning opportunities would ensure that students have access to more information on the actual nature of work in the STEM field and what is required to pursue these careers. This strategy would also serve as a motivator to those who are not aware that STEM careers involve people skills, creativity, and problem solving.
Abbreviations
Confirmatory factor analysis
- Mathematics self-efficacy
Science, technology, engineering, and mathematics
Ayar, MC, & Yalvac, B. (2016). Lessons learned: authenticity, interdisciplinarity, and mentoring for STEM learning environments. International Journal of Education in Mathematics, Science and Technology , 4 (1), 30–43.
Article Google Scholar
Bandura, A. (1977). Self-efficacy: toward a unifying theory of behavior change. Psychological Review , 84 , 191–215.
Britner, SL, & Pajares, F. (2006). Sources of science self-efficacy beliefs of middle school students. Journal of Research in Science Teaching , 43 (5), 485–499.
Compeau, S. (2016). The calling of an engineer: High school students’ perceptions of engineering. Retrieved from http://qspace.library.queensu.ca/jspui/handle/1974/13924
Google Scholar
Dabney, KP, Tai, RH, Almarode, JT, Miller-Friedmann, JL, Sonnert, G, Sadler, PM, Hazari, Z. (2012). Out-of-school time science activities and their association with career interest in STEM. International Journal of Science Education, Part B , 2 (1), 63–79.
DeBacker, TK, & Nelson, RM. (1999). Variations on an expectancy-value model of motivation in science. Contemporary Educational Psychology , 24 (2), 71–94.
Fouad, NA, & Smith, PL. (1996). A test of a social cognitive model for middle school students: math and science. Journal of Counseling Psychology , 43 (3), 338.
Franz-Odendaal, TA, Blotnicky, K, French, F, Joy, P. (2016). Experiences and perceptions of STEM subjects, careers, and engagement in STEM activities among middle school students in the maritime provinces. Canadian Journal of Science, Mathematics and Technology Education , 16 (2), 153–168.
Hackett, G, & Betz, NE. (1981). A self-efficacy approach to the career development of women. Journal of Vocational Behavior , 18 , 326–336.
Hair Jr, JF, Blacks, WC, Barry, BJ, Anderson, RE (2010). Multivariate data analysis, 7/e . Upper Saddle River, NJ: Prentice-Hall, Inc.
Harackiewicz, JM, Rozek, CS, Hulleman, CS, Hyde, JS. (2012). Helping parents to motivate adolescents in mathematics and science an experimental test of a utility-value intervention. Psychological Science , 956797611435530 .
Heilbronner, NN (2009). Pathways in STEM: factors affecting the retention and attrition of talented men and women from the STEM pipeline . ERIC Retrieved from http://eric.ed.gov/?id=ED513162 .
Hirshi, A. (2011). Career-choice readiness in adolescence: developmental trajectories and individual differences. Journal of Vocational Behavior , 79 , 340–348.
Holland, JL (1973). Making vocational choices: a theory of careers . Englewood Cliffs: Prentice-Hall, Inc.
IBM Corp. Released 2013. IBM SPSS Statistics for Windows, version 22.0. Armonk, NY: IBM Corp.
Karatas, FO, Micklos, A, Bodner, GM. (2011). Sixth-grade students’ views of the nature of engineering and images of engineers. Journal of Science Education and Technology , 20 (2), 123–135.
Kelly, K, Dampier, DA, Carr, K. (2013). Willing, able, and unwanted: high school girl’s potential selves in computing. Journal of Women and Minorities in Science and Engineering , 19 (1) Retrieved from http://www.dl.begellhouse.com/journals/00551c876cc2f027,2d4a5d286768c212,26d9b12a206d2bc6.html .
Kuncel, NR, Credé, M, Thomas, LL. (2005). The validity of self-reported grade point averages, class ranks, and test scores: a meta-analysis and review of the literature. Review of Educational Research , 75 (1), 63–82.
Lent, R. W. (2005). A Social Cognitive View of Career Development and Counseling. Retrieved from http://psycnet.apa.org/psycinfo/2004-21312-005
Lent, RW, Brown, SD, Hackett, G. (1994). Toward a unifying social cognitive theory of career and academic interest, choice, and performance. Journal of Vocational Behavior , 45 (1), 79–122.
Lent, RW, Paixao, MA, da Silva, JT, Leitao, LM. (2010). Predicting occupational interest and choice aspirations in Portuguese high school students: a test of social cognitive career theory. Journal of Vocational Behavior , 76 , 244–251.
Let's Talk Science Annual Report. Spotlight on Science Learning - Exploring Parental Influence: Shaping Teen Decisions Regarding Science Education. (2015). Retrieved from http://letstalkscience.ca/Portals/0/Documents/RPS/Spotlight/LTS-Exploring-parental-influence-EN.pdf
Mangu, DM, Lee, AR, Middleton, JA, Nelson, JK (2015). Motivational factors predicting STEM and engineering career intentions for high school students. In Frontiers in education conference (FIE), 2015. 32614 2015. IEEE , (pp. 1–8). IEEE Retrieved from http://ieeexplore.ieee.org/xpls/abs_all.jsp?arnumber=7344065 .
Masnick, AM, Valenti, SS, Cox, BD, Osman, CJ. (2010). A multidimensional scaling analysis of students’ attitudes about science careers. International Journal of Science Education , 32 (5), 653–667.
McDaniel, C, Gates, R, Sivaramakrishnan, S, Main, K (2014). Marketing research essentials , 2/ce (). Mississauga, ON: Wiley & Sons Canada.
Miller, MJ. (1998). Broading the use of Holland’s hexagon with specific implications for career counselors. Journal of Employment Counseling , 35 , 2–5.
Murphy, C, & Beggs, J. (2005). Coteaching as an approach to enhance science learning and teaching in primary schools. Counterpoints , 294 , 207–231.
Nugent, G, Barker, B, Welch, G, Grandgenett, N, Wu, C, Nelson, C. (2015). A model of factors contributing to STEM learning and career orientation. International Journal of Science Education , 37 (7), 1067–1088.
Olitsky, NH. (2014). How do academic achievement and gender affect the earnings of STEM majors? A propensity score matching approach. Research in Higher Education , 55 , 245–271.
Parker, PD, Marsh, HW, Ciarrochi, J, Marshall, S, Abduljabbar, AS. (2014). Juxtaposing math self efficacy and self-concept as predictors of long-term achievement outcomes. Educational Psychology , 34 (1), 29–48.
Patton, W, & McMahon, M (2006). Career development and systems theory , (2nd ed., ). Pacific Grove, CA: Brooks/Cole.
Richardson, M, Abraham, C, Bond, R. (2012). Psychological correlates of university students’ academic performance: a systematic review and meta-analysis. Psychological Bulletin , 138 (2), 353.
Sadler, PM, Sonnert, G, Hazari, Z, Tai, R. (2012). Stability and volatility of STEM career interest in high school: a gender study. Science Education , 96 (3), 411–427.
Sahin, A, Ayar, MC, Adiguzel, T. (2014). STEM related after-school program activities and associated outcomes on student learning. Educational Sciences: Theory and Practice , 14 (1), 309–322.
Sahin, A, Gulacar, O, Stuessy, C. (2015). High school students’ perceptions of the effects of international science Olympiad on their STEM career aspirations and twenty-first century skill development. Research in Science Education , 45 (6), 785–805.
Schumacher, MM, Stansbury, KN, Johnson, MN, Floyd, SR, Reid, CE, Noland, MP, Leukefeld, CG. (2009). The young women in science program: a five-year follow-up of an intervention to change science attitudes, academic behavior, and career aspirations. Journal of Women and Minorities in Science and Engineering , 15 (4) Retrieved from http://www.dl.begellhouse.com/journals/00551c876cc2f027,293840bd7415e516,4ac4cd3d67f80a79.html .
Sheu, HB, Lent, RW, Brown, SD, Miller, MJ, Hennessy, KD, Duggy, RD. (2010). Testing the choice model of social cognitive career theory across Holland themes: a meta-analytic path analysis. Journal of Vocational Behavior , 76 , 252–264.
Simpkins, SD, Davis-Kean, PE, Eccles, JS. (2006). Math and science motivation: a longitudinal examination of the links between choices and beliefs. Developmental Psychology , 42 (1), 70.
Sjaastad, J. (2012). Sources of inspiration: the role of significant persons in young people’s choice of science in higher education. International Journal of Science Education , 34 (10), 1615–1636.
Steinke, J, Lapinski, M, Long, M, Van Der Maas, C, Ryan, L, Applegate, B. (2009). Seeing oneself as a Scientist: media influences and adolescent girl’s science career-possible selves. Journal of Women and Minorities in Science and Engineering , 15 (4) Retrieved from http://www.dl.begellhouse.com/journals/00551c876cc2f027,293840bd7415e516,0b5caaa338bc00a0.html .
Tai, R. H., Liu, C. Q., Maltese, A. V., & Fan, X. (2006). Planning early for careers in science. Life Sciences, 1, 0–2.
Zhang, L, & Barnett, M. (2015). How high school students envision their STEM career pathways. Cultural Studies of Science Education , 10 (3), 637–656.
Download references
Acknowledgements
Funding was provided to author by a grant from the Natural Sciences and Engineering Research Council of Canada. We are grateful to the individuals who helped our team obtain parental consent forms and parents for providing consent for the children to participate in this study.
Availability of data and materials
University ethics approval does not include release of the raw data. Data was collected from minor-aged children under the strict condition of anonymity and cannot be shared. Please contact the corresponding author for more information.
Author information
Authors and affiliations.
Department of Business Administration and Tourism and Hospitality Management, Mount Saint Vincent University, Halifax, NS, B3M 2J6, Canada
Karen A. Blotnicky
Department of Biology, Mount Saint Vincent University, Halifax, NS, B3M 2J6, Canada
Tamara Franz-Odendaal
Department of Education, Mount Saint Vincent University, Halifax, NS, B3M 2J6, Canada
Frederick French
School of Health and Human Performance, Dalhousie University, Halifax, NS, B3H 4R2, Canada
Phillip Joy
You can also search for this author in PubMed Google Scholar
Contributions
The second author conceptualized the broad goals of the study, and all four authors were part of a research team that designed all the study components and executed the research. The first author was primarily responsible for designing the methodology and quantitative data analysis, while the fourth author assisted with the data analysis. The second and third authors were primarily responsible for conducting the literature review, developing the theoretical underpinnings of the research, and interpreting the results of the analysis from a theoretical perspective. All authors read and approved the final manuscript.
Corresponding author
Correspondence to Karen A. Blotnicky .
Ethics declarations
Ethics approval and consent to participate.
This approval was granted by the Research Ethics Board of Mount Saint Vincent University in Halifax, NS Canada.
Competing interests
The authors declare that they have no competing interests.
Publisher’s Note
Springer Nature remains neutral with regard to jurisdictional claims in published maps and institutional affiliations.
Rights and permissions
Open Access This article is distributed under the terms of the Creative Commons Attribution 4.0 International License ( http://creativecommons.org/licenses/by/4.0/ ), which permits unrestricted use, distribution, and reproduction in any medium, provided you give appropriate credit to the original author(s) and the source, provide a link to the Creative Commons license, and indicate if changes were made.
Reprints and permissions
About this article
Cite this article.
Blotnicky, K.A., Franz-Odendaal, T., French, F. et al. A study of the correlation between STEM career knowledge, mathematics self-efficacy, career interests, and career activities on the likelihood of pursuing a STEM career among middle school students. IJ STEM Ed 5 , 22 (2018). https://doi.org/10.1186/s40594-018-0118-3
Download citation
Received : 28 July 2017
Accepted : 24 April 2018
Published : 16 May 2018
DOI : https://doi.org/10.1186/s40594-018-0118-3
Share this article
Anyone you share the following link with will be able to read this content:
Sorry, a shareable link is not currently available for this article.
Provided by the Springer Nature SharedIt content-sharing initiative
- STEM career
- Technical skills
- Career knowledge
- Career awareness
- Career interests
- Career activities
- Subject requirements
- Announcements
- In The News
Thursday, March 28, 2024
WVU recognizes four faculty members as 2024 Foundation Outstanding Teachers
Each spring, West Virginia University recognizes selected faculty members for their exceptional and innovative teaching. This year, the 2024 WVU Foundation Awards for Outstanding Teaching honor four faculty members:
• Jake Follmer , assistant professor in the School of Education, College of Applied Human Sciences;
• David Miller , professor of mathematics and assistant director of undergraduate studies in the School of Mathematical and Data Sciences, Eberly College of Arts and Sciences;
• Chris Ramezan , assistant professor of cybersecurity in the Department of Management Information Systems and coordinator of the Business Cybersecurity Management program, John Chambers College of Business and Economics; and
• David Smith , teaching associate professor of journalism, Reed College of Media.
Established in 1985 by the WVU Foundation, the Outstanding Teaching Awards recognize faculty who are particularly effective and inspiring teachers, as well as those who have established patterns of exceptional innovation in their teaching methods, course and curriculum design, and instructional tools.
“Our faculty members are without question some of the most dedicated professionals in higher education today,” said Provost and Vice President for Academic Affairs Maryanne Reed. “Each of these award recipients is a prime example of the kind of innovative teacher who engages and inspires students to achieve excellence in their studies. This year’s Foundation Outstanding Teachers are more than deserving of this prestigious award for their dedication to their craft and to our students’ success.”
At the core of Follmer’s instruction are active and structured learning approaches combined with models of deeper learning. He utilizes these student-centered pedagogical approaches to learning because they have been found to broadly improve all students’ overall learning and to uniquely improve the learning of underrepresented students (e.g., first generation and low-income students).
Follmer combines these approaches with efficacy-promoting instructional methods; open, inclusive and challenging learning environments; and course designs that prioritize student transfer and application of course material. Follmer’s approach to teaching and learning can be found across his courses — from undergraduate offerings in research methods to graduate courses in applied statistical methods and research and evaluation. In fact, he has been so successful in teaching the data analyses courses that advisers from across the college and the University routinely send their students to take his courses. Students consistently provide very high evaluation scores and written praise of Follmer’s teaching approach. Also noteworthy is that Follmer’s students finish his graduate data analyses courses with significant gains in their conceptual and procedural knowledge as well as self-efficacy related to quantitative methods.
Despite his teaching and learning success, Follmer continually engages in an iterative process to improve his courses, documenting and assessing the impact of different instructional interventions. He has also published at least eight scholarship of teaching and learning products documenting the impact of his teaching and serves as a co-principal investigator on the National Science Foundation’s Improving Undergraduate STEM Education: Engaged Learning Track grant for which the purpose is to develop instructional interventions that promote learning and retention in STEM programs. Follmer is also completing an American Educational Research Association fellowship program through which he is investigating the impact of deeper learning pedagogy on successful college pathways for first-generation learners.
Miller started his higher education career at WVU in 2006. He is known for his innovative work establishing and directing the Emerging Scholars Program in the calculus and differential equations courses. The ESP began under the National Science Foundation’s Louis Stokes Alliance for Minority Participation grant that has received continual funding — $6 million dollars to date — since Miller joined WVU. Miller serves as one of the co-principal investigators on the Kentucky-West Virginia LSAMP grant at the University of Kentucky and as the grant’s campus coordinator at WVU. He personally recruits racially and ethnically minoritized STEM students, as well as first-generation STEM major students, into WVU ESP courses and integrates inquiry-based learning with active group learning.
Miller begins with individual and group activities that build conceptual and procedural foundations and follows with challenging problems that deepen student understanding and ignite whole-class discussions. The three mathematics and science education articles about the WVU ESP in calculus demonstrate the significant positive impact of the program on academic performance, retention rates and graduation rates for underrepresented students in STEM disciplines. Miller found that the average learning gains among students in the ESP calculus courses are significantly higher than those demonstrated by students in traditional calculus courses. He also found that the work in these courses removes the retention gaps between traditional and underrepresented calculus students and that the overall and STEM graduation rates for underrepresented students that have taken ESP calculus classes are higher than underrepresented students in traditional calculus classes.
Miller is also recognized for his long-standing, exceptional teaching effectiveness in traditional college algebra courses and upper-level mathematics courses like Introduction to the Concepts of Mathematics and Introduction to Analysis and Topology at WVU. He is known for creating engaging and meaningful active learning classroom experiences that make mathematics accessible and understandable to a wide range of students. When he began coordinating the college algebra courses, Miller employed instructional materials that minimize students’ cognitive load on their short-term or working memory. For example, using worked-example worksheets, students are better able to easily learn from expert solutions to solve other algebra problems. This shift in instruction has helped students improve their overall letter grade in the course. Miller has disseminated his findings on this effective teaching strategy in four publications in mathematics and science education journals.
The high student evaluations and open-ended comments, as well as strong letters of support from current and past ESP students, indicate Miller fosters a collaborative learning environment in which mathematics is accessible through practice, and where students share ideas and solve problems together and ultimately enjoy and succeed in math courses.
Ramezan is recognized for his innovative curriculum contributions to the business cybersecurity undergraduate and graduate course offerings, which includes 12 unique courses over five years. His cutting-edge courses on topics such as machine learning, security architecture and industrial control system security make WVU one of the only business school cybersecurity programs to offer these types of courses.
Ramezan has also designed business cybersecurity program courses to align with 10 different industry cybersecurity certification exams and created hundreds of problem-solving and applied simulation exam questions that better prepare students for those exams. He has also led countless experiential learning projects on real cybersecurity problems with a variety of community partners to provide students with real-world cybersecurity experience and developed a series of innovative cyber exercises through which students hack real systems within a safe environment. In addition, Ramezan has developed over 100 hours of high-quality online video lectures and lab walkthroughs.
Ramezan is also recognized for designing cutting-edge, hands-on, interactive cybersecurity lab assignments on a variety of topics, such as network architecture, applied machine learning, penetration testing and cybersecurity operations. In addition, Ramezan envisioned the Karen Evans Cybersecurity Lab in Reynolds Hall, a teaching and research lab modeled as a security operations center which enables cybersecurity students to safely hone and develop their cybersecurity skills. He also partnered with the U.S. Cyber Command, Joint Force Headquarters Department of Defense Information Network, Marshall University, and the Civil-Military Innovation Institute to assist with creating the Cybersecurity Center of Excellence in Critical Infrastructure and has led WVU efforts with the NATO Locked Shields exercise, the largest live-fire cybersecurity exercise in the world. This work has also warranted Ramezan being named the Academic Lead of the 2024 NATO Locked Shields exercise by the Chief of Staff of the Joint Force Headquarters. Ramezan also helped the U.S. national team earn a 4th place ranking in the 2020 NATO Locked Shields exercise, one of the highest rankings the team has ever received in the competition.
His high student evaluations and open-ended comments indicate Ramezan is a talented and dedicated teacher and have earned him the Chambers College Awards of Distinction in both Teaching and Service, as well as the American Legion Department of West Virginia Higher Education Educator of the Year Award in 2023. He provides significant student support for their own development and recognition — including for a recent Truman Scholar, awardees of the National Cyber-Forensics and Training Alliance scholarship and an Air Force Chief’s Council scholarship recipient — and has developed significant employment pathways for WVU cybersecurity undergraduate and graduate students. Ramezan has also recently published two teaching related articles in high-quality journals. Unsurprisingly, Ramezan’s efforts have helped grow the University’s Master of Science in Business Cybersecurity Management program by 400% since 2019.
Before joining WVU, Smith had a 10-year career as a visual storyteller in journalism and strategic communications. He is recognized for a highly successful approach to teaching and learning that is student-centered, hands-on, adaptive and grounded in real-world experiences. One reason for his continued success in the classroom is his ability to consistently strike a balance between nurturing students’ ambitions and celebrating their achievements while providing them honest feedback in ways that develop their resiliency.
Smith is also recognized for integrating modern technologies and international elements whenever possible into his courses, and for his innovative development of courses on media tools and storytelling, immersive storytelling, social video digital publication, visual brand storytelling, extended reality, drone journalism, and augmented and virtual reality. In these courses, students learn and apply new skills, such as studying for and passing the Federal Aviation Administration’s drone pilot’s license certification and contributing to compelling community projects across Appalachia.
In addition, Smith’s study abroad programs and international partnerships have given students exposure to diverse cultural narratives and global media trends. These teaching efforts have also translated into grant funding, including as co-principal investigator on a project supported by the Department of Education’s Rehabilitation Services Administration award to develop immersive technologies curriculum for vocational rehabilitation counselors-in-training.
Smith has been recognized by his students with outstanding evaluations of his teaching and letters of support, as well as awards. He has been recognized by his colleagues with the Reed College Teaching Award, a Public Relations Society of America — West Virginia Crystal Award for a video produced for the town of Pineville’s brand launch, and an Association for Education in Journalism and Mass Communication Great Ideas for Teachers award. His students have likewise earned regional and national awards, including for two consecutive years the Broadcast Education Association’s first-place award in Emerging Media and Technology Best in Festival award for international multimedia storytelling, BEA’s first-place award in Interactive Media and Emerging Technologies, BEA’s third-place award in Interactive Media and Emerging Technologies, and multiple Telly Awards for projects produced in the 100 Days of Appalachia digital video class and the community branding class, as well as significant publications in “100 Days in Appalachia” and student photos published in The Washington Post.
Faculty members must be nominated by their college leadership in order to be eligible for the Foundation Award for Outstanding Teaching. Each of the honorees will receive $5,000 in professional development monies from the WVU Foundation.
Award recipients will be recognized during a faculty and staff awards reception at Blaney House in April.
Read more about these and other awards.
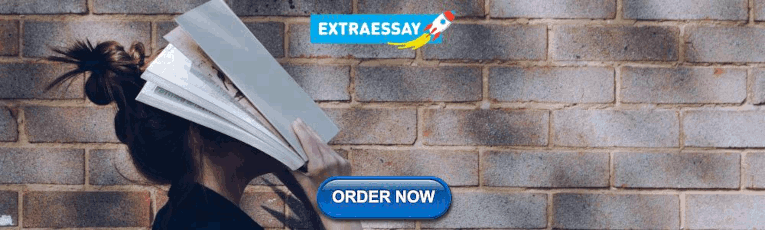
IMAGES
VIDEO
COMMENTS
Chemistry. Let's get started with some quantitative research topics for stem students in chemistry: 1. Studying the properties of superconductors at different temperatures. 2. Analyzing the efficiency of various catalysts in chemical reactions. 3. Investigating the synthesis of novel polymers with unique properties. 4.
Here are 10 practical research topics for STEM students: Developing an affordable and sustainable water purification system for rural communities. Designing a low-cost, energy-efficient home heating and cooling system. Investigating strategies for reducing food waste in the supply chain and households.
Biomedical Engineering Research Topics. 1. Optimizing algorithms for medical image analysis in diagnostic imaging. 2. Quantifying the efficiency of prosthetic and orthopedic devices in rehabilitation. 3. Analyzing the quantitative aspects of drug delivery systems in personalized medicine. 4.
Biology Quantitative Research Topics For STEM Students. Systems Biology: Modeling Cellular Signaling Networks. Computational Neuroscience: Brain Network Analysis. Population Genetics and Evolutionary Dynamics. Mathematical Modeling of Infectious Diseases. Studying Protein Folding Using Computational Methods.
Below are some examples of quantitative research topics for STEM students in grade 11. A study of how plants conduct electricity; How does water salinity affect plant growth? A study of soil pH levels on plants; Research Topics For Grade 12 STEM Students. Here are some of the best qualitative research topics for STEM students in grade 12.
Robotics. Implementing control algorithms for robot movement. Designing robots for specific tasks with robot design. Providing sensory feedback for robot understanding. Developing autonomous robots for independence. Considering ethical implications of robotics for societal impact. These topics are designed to be engaging and relatable, making ...
Dive into a captivating world of quantitative research topics for STEM students! Fuel your scientific curiosity and sharpen your analytical skills as you navigate through this carefully curated collection. Picture it as your personal roadmap, guiding you through the thrilling landscapes of Science, Technology, Engineering, and Mathematics.
Following are the best Quantitative Research Topics For STEM Students in mathematics and statistics. Prime Number Distribution: Investigate the distribution of prime numbers. Graph Theory Algorithms: Develop algorithms for solving graph theory problems. Statistical Analysis of Financial Markets: Analyze financial data and market trends.
Embark on a captivating journey through the cosmos of knowledge with our curated guide on Quantitative Research Topics for STEM Students. Explore innovative ideas in science, technology, engineering, and mathematics, designed to ignite curiosity and shape the future. Unleash the power of quantitative research and dive into uncharted territories ...
With the rapid increase in the number of scholarly publications on STEM education in recent years, reviews of the status and trends in STEM education research internationally support the development of the field. For this review, we conducted a systematic analysis of 798 articles in STEM education published between 2000 and the end of 2018 in 36 journals to get an overview about developments ...
Taking publicly funded projects in STEM education as a special lens, we aimed to learn about research and trends in STEM education. We identified a total of 127 projects funded by the Institute of Education Sciences (IES) of the US Department of Education from 2003 to 2019. Both the number of funded projects in STEM education and their funding amounts were high, although there were ...
Health Sciences Quantitative Research Topics For STEM Students. Quantifying the impact of sleep duration on mental health. Analyzing the effectiveness of preventive health programs in reducing disease incidence. Investigating the correlation between physical activity and cardiovascular health. Assessing the relationship between dietary habits ...
Quantitative analyses are pivotal tools for examining DEI in STEM, but it is imperative to understand that researchers' positions in relation to race, gender, socioeconomic status, where they are located within universities, academic training, and other characteristics can shape how they approach data and analyses.
This study explored research trends in science, technology, engineering, and mathematics (STEM) education. Descriptive analysis and co-word analysis were used to examine articles published in Social Science Citation Index journals from 2011 to 2020. From a search of the Web of Science database, a total of 761 articles were selected as target samples for analysis. A growing number of STEM ...
With a final of 171+ quantitative research topics for stem students in various STM areas, students have plenty of options to explore and contribute to the advancement of knowledge in their chosen subjects. Quantitative research not only tests their understanding but also imparts them with valuable analytical skills.
This study used a systematic review and meta-analysis as a method to investigate whether STEM enactment in Asia effectively enhances students' learning outcomes. Verifiable examples of science, technology, engineering, and mathematics (STEM) education, effectively being applied in Asia, are presented in this study. The study involved 4768 students from 54 studies.
October 17, 2023 by Sumit. Explore diverse quantitative research topics for STEM students, spanning climate change, AI, robotics, and more. Find engaging projects for high school and beyond. Quantitative studies allow you to investigate real-world problems and make groundbreaking discoveries using cold, hard facts.
Here are 8 key points on how to do experimental research effectively. 1. Clear Research Focus. Begin by defining a clear and focused research question. A well-defined question provides a purpose and direction for your experiment, guiding your choices in variables and methodology. 2.
This research was aiming at trying out the implementation of STEM approach to teach the topics of electricity in Science and Statistics in Mathematics to grade IX students by using real life ...
The study method entails two main factors, purpose and the research synthesis process of the study. This study aims to explore the understanding and awareness of the teachers and students about STEM education; the challenges faced by them and their solutions in the light of literature review and research amalgamation and the abilities and skills to overcome these challenges.
A sample of 1448 students in grades 7 and 9 was drawn from public schools in Atlantic Canada to explore students' knowledge of science and mathematics requirements for science, technology, engineering, and mathematics (STEM) careers. Also explored were their mathematics self-efficacy (MSE), their future career interests, their preferences for particular career activities, and their ...
As students in previous research have described LLPs as "cultural phenomena" (Wawrzynski et al., 2009, p. 156), we utilized phenomenological case study methods stemming from a social-constructivist epistemology to address our research questions. Students of color in a STEM LLP served as the phenomenon and the specific LLP served as a case ...
Here are the top quantitative research topics for STEM students in the Philippines in 2024. 1. Impact of Climate Change on Farming. Analyze how changing weather affects the growth of crops like rice and corn in different parts of the Philippines. Use numbers to find ways and suggest ways farmers can adapt. 2.
Further, this quantitative-correlational study involves 255 freshman students, of which 115 have non-STEM academic background from the SHS. Results reveal that students with a SHS STEM background ...
Students consistently provide very high evaluation scores and written praise of Follmer's teaching approach. Also noteworthy is that Follmer's students finish his graduate data analyses courses with significant gains in their conceptual and procedural knowledge as well as self-efficacy related to quantitative methods.