Have a language expert improve your writing
Run a free plagiarism check in 10 minutes, generate accurate citations for free.
- Knowledge Base
Methodology
- How to Write a Literature Review | Guide, Examples, & Templates
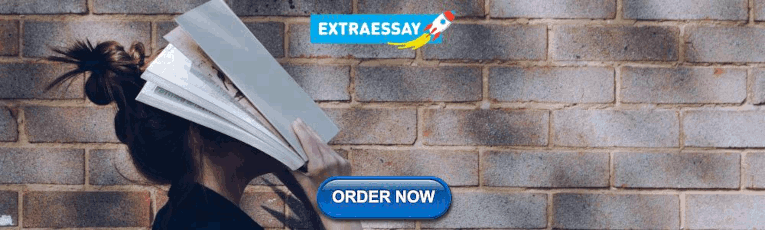
How to Write a Literature Review | Guide, Examples, & Templates
Published on January 2, 2023 by Shona McCombes . Revised on September 11, 2023.
What is a literature review? A literature review is a survey of scholarly sources on a specific topic. It provides an overview of current knowledge, allowing you to identify relevant theories, methods, and gaps in the existing research that you can later apply to your paper, thesis, or dissertation topic .
There are five key steps to writing a literature review:
- Search for relevant literature
- Evaluate sources
- Identify themes, debates, and gaps
- Outline the structure
- Write your literature review
A good literature review doesn’t just summarize sources—it analyzes, synthesizes , and critically evaluates to give a clear picture of the state of knowledge on the subject.
Instantly correct all language mistakes in your text
Upload your document to correct all your mistakes in minutes
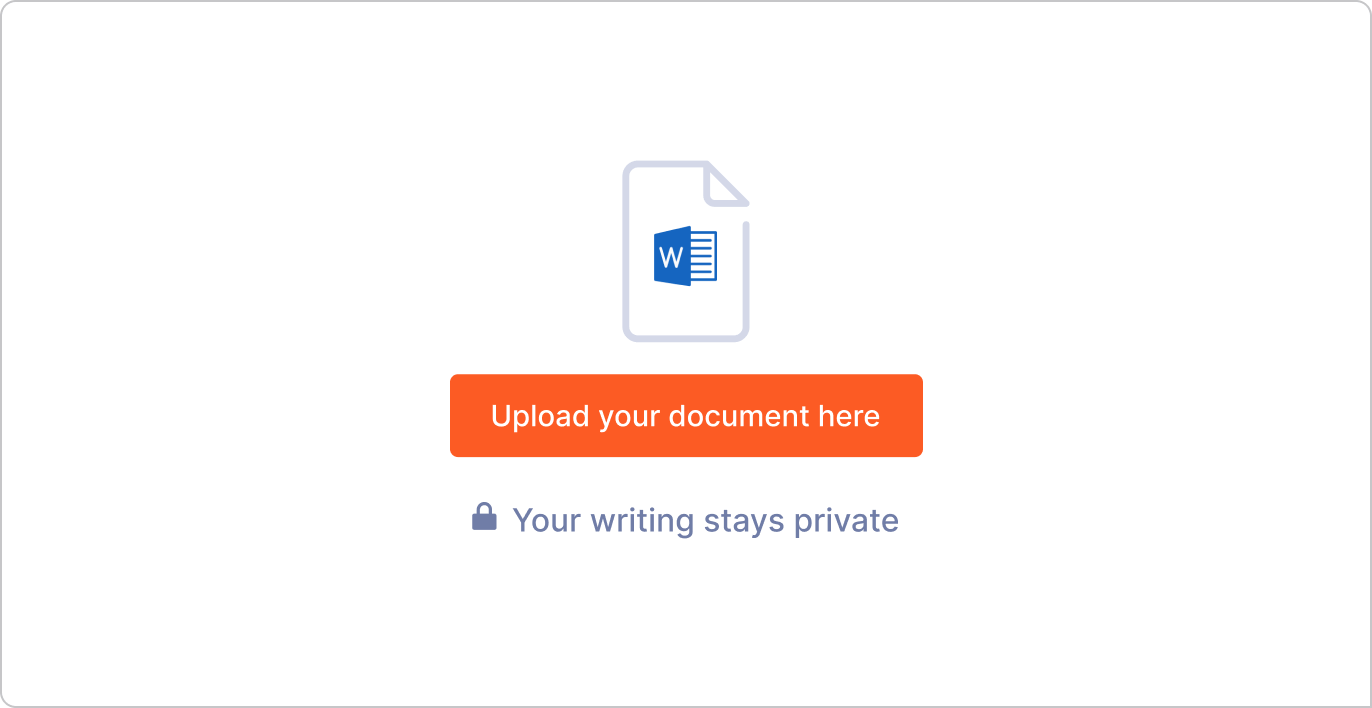
Table of contents
What is the purpose of a literature review, examples of literature reviews, step 1 – search for relevant literature, step 2 – evaluate and select sources, step 3 – identify themes, debates, and gaps, step 4 – outline your literature review’s structure, step 5 – write your literature review, free lecture slides, other interesting articles, frequently asked questions, introduction.
- Quick Run-through
- Step 1 & 2
When you write a thesis , dissertation , or research paper , you will likely have to conduct a literature review to situate your research within existing knowledge. The literature review gives you a chance to:
- Demonstrate your familiarity with the topic and its scholarly context
- Develop a theoretical framework and methodology for your research
- Position your work in relation to other researchers and theorists
- Show how your research addresses a gap or contributes to a debate
- Evaluate the current state of research and demonstrate your knowledge of the scholarly debates around your topic.
Writing literature reviews is a particularly important skill if you want to apply for graduate school or pursue a career in research. We’ve written a step-by-step guide that you can follow below.
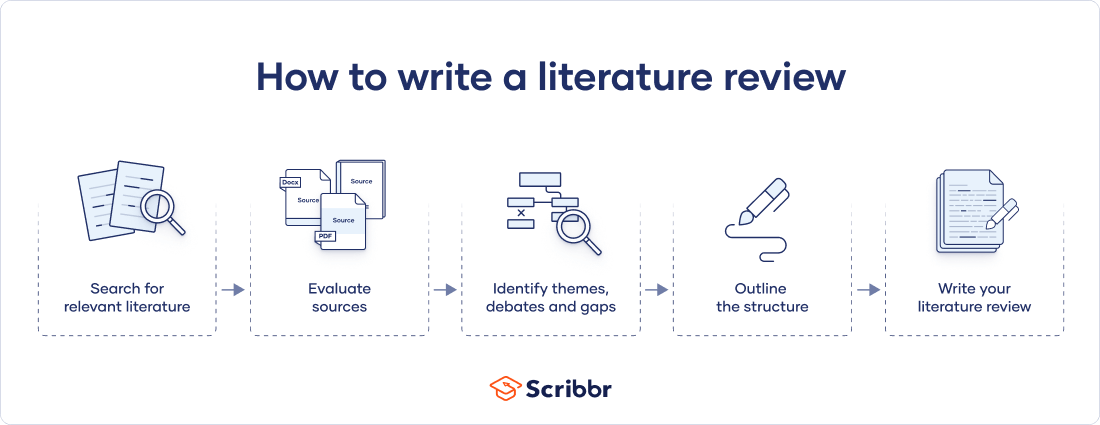
Here's why students love Scribbr's proofreading services
Discover proofreading & editing
Writing literature reviews can be quite challenging! A good starting point could be to look at some examples, depending on what kind of literature review you’d like to write.
- Example literature review #1: “Why Do People Migrate? A Review of the Theoretical Literature” ( Theoretical literature review about the development of economic migration theory from the 1950s to today.)
- Example literature review #2: “Literature review as a research methodology: An overview and guidelines” ( Methodological literature review about interdisciplinary knowledge acquisition and production.)
- Example literature review #3: “The Use of Technology in English Language Learning: A Literature Review” ( Thematic literature review about the effects of technology on language acquisition.)
- Example literature review #4: “Learners’ Listening Comprehension Difficulties in English Language Learning: A Literature Review” ( Chronological literature review about how the concept of listening skills has changed over time.)
You can also check out our templates with literature review examples and sample outlines at the links below.
Download Word doc Download Google doc
Before you begin searching for literature, you need a clearly defined topic .
If you are writing the literature review section of a dissertation or research paper, you will search for literature related to your research problem and questions .
Make a list of keywords
Start by creating a list of keywords related to your research question. Include each of the key concepts or variables you’re interested in, and list any synonyms and related terms. You can add to this list as you discover new keywords in the process of your literature search.
- Social media, Facebook, Instagram, Twitter, Snapchat, TikTok
- Body image, self-perception, self-esteem, mental health
- Generation Z, teenagers, adolescents, youth
Search for relevant sources
Use your keywords to begin searching for sources. Some useful databases to search for journals and articles include:
- Your university’s library catalogue
- Google Scholar
- Project Muse (humanities and social sciences)
- Medline (life sciences and biomedicine)
- EconLit (economics)
- Inspec (physics, engineering and computer science)
You can also use boolean operators to help narrow down your search.
Make sure to read the abstract to find out whether an article is relevant to your question. When you find a useful book or article, you can check the bibliography to find other relevant sources.
You likely won’t be able to read absolutely everything that has been written on your topic, so it will be necessary to evaluate which sources are most relevant to your research question.
For each publication, ask yourself:
- What question or problem is the author addressing?
- What are the key concepts and how are they defined?
- What are the key theories, models, and methods?
- Does the research use established frameworks or take an innovative approach?
- What are the results and conclusions of the study?
- How does the publication relate to other literature in the field? Does it confirm, add to, or challenge established knowledge?
- What are the strengths and weaknesses of the research?
Make sure the sources you use are credible , and make sure you read any landmark studies and major theories in your field of research.
You can use our template to summarize and evaluate sources you’re thinking about using. Click on either button below to download.
Take notes and cite your sources
As you read, you should also begin the writing process. Take notes that you can later incorporate into the text of your literature review.
It is important to keep track of your sources with citations to avoid plagiarism . It can be helpful to make an annotated bibliography , where you compile full citation information and write a paragraph of summary and analysis for each source. This helps you remember what you read and saves time later in the process.
Receive feedback on language, structure, and formatting
Professional editors proofread and edit your paper by focusing on:
- Academic style
- Vague sentences
- Style consistency
See an example
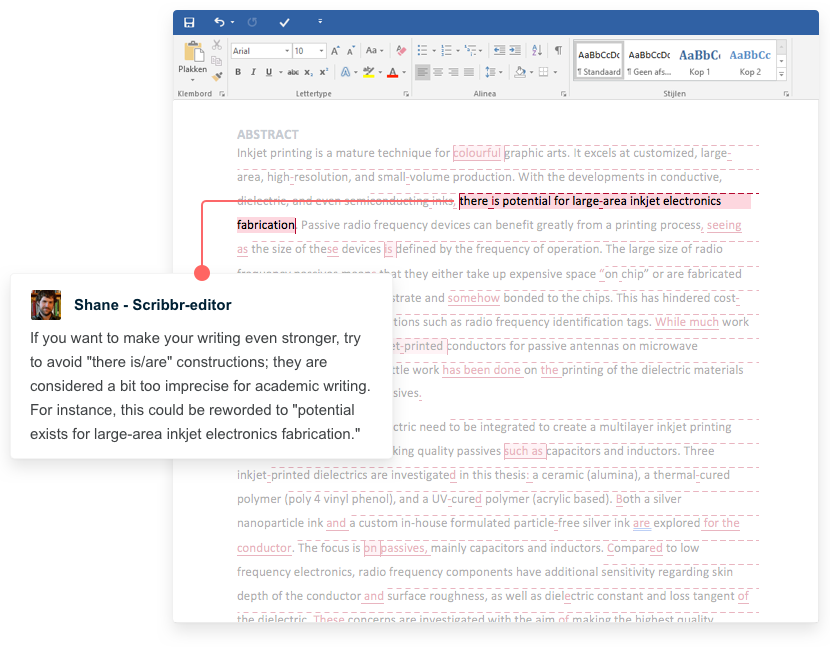
To begin organizing your literature review’s argument and structure, be sure you understand the connections and relationships between the sources you’ve read. Based on your reading and notes, you can look for:
- Trends and patterns (in theory, method or results): do certain approaches become more or less popular over time?
- Themes: what questions or concepts recur across the literature?
- Debates, conflicts and contradictions: where do sources disagree?
- Pivotal publications: are there any influential theories or studies that changed the direction of the field?
- Gaps: what is missing from the literature? Are there weaknesses that need to be addressed?
This step will help you work out the structure of your literature review and (if applicable) show how your own research will contribute to existing knowledge.
- Most research has focused on young women.
- There is an increasing interest in the visual aspects of social media.
- But there is still a lack of robust research on highly visual platforms like Instagram and Snapchat—this is a gap that you could address in your own research.
There are various approaches to organizing the body of a literature review. Depending on the length of your literature review, you can combine several of these strategies (for example, your overall structure might be thematic, but each theme is discussed chronologically).
Chronological
The simplest approach is to trace the development of the topic over time. However, if you choose this strategy, be careful to avoid simply listing and summarizing sources in order.
Try to analyze patterns, turning points and key debates that have shaped the direction of the field. Give your interpretation of how and why certain developments occurred.
If you have found some recurring central themes, you can organize your literature review into subsections that address different aspects of the topic.
For example, if you are reviewing literature about inequalities in migrant health outcomes, key themes might include healthcare policy, language barriers, cultural attitudes, legal status, and economic access.
Methodological
If you draw your sources from different disciplines or fields that use a variety of research methods , you might want to compare the results and conclusions that emerge from different approaches. For example:
- Look at what results have emerged in qualitative versus quantitative research
- Discuss how the topic has been approached by empirical versus theoretical scholarship
- Divide the literature into sociological, historical, and cultural sources
Theoretical
A literature review is often the foundation for a theoretical framework . You can use it to discuss various theories, models, and definitions of key concepts.
You might argue for the relevance of a specific theoretical approach, or combine various theoretical concepts to create a framework for your research.
Like any other academic text , your literature review should have an introduction , a main body, and a conclusion . What you include in each depends on the objective of your literature review.
The introduction should clearly establish the focus and purpose of the literature review.
Depending on the length of your literature review, you might want to divide the body into subsections. You can use a subheading for each theme, time period, or methodological approach.
As you write, you can follow these tips:
- Summarize and synthesize: give an overview of the main points of each source and combine them into a coherent whole
- Analyze and interpret: don’t just paraphrase other researchers — add your own interpretations where possible, discussing the significance of findings in relation to the literature as a whole
- Critically evaluate: mention the strengths and weaknesses of your sources
- Write in well-structured paragraphs: use transition words and topic sentences to draw connections, comparisons and contrasts
In the conclusion, you should summarize the key findings you have taken from the literature and emphasize their significance.
When you’ve finished writing and revising your literature review, don’t forget to proofread thoroughly before submitting. Not a language expert? Check out Scribbr’s professional proofreading services !
This article has been adapted into lecture slides that you can use to teach your students about writing a literature review.
Scribbr slides are free to use, customize, and distribute for educational purposes.
Open Google Slides Download PowerPoint
If you want to know more about the research process , methodology , research bias , or statistics , make sure to check out some of our other articles with explanations and examples.
- Sampling methods
- Simple random sampling
- Stratified sampling
- Cluster sampling
- Likert scales
- Reproducibility
Statistics
- Null hypothesis
- Statistical power
- Probability distribution
- Effect size
- Poisson distribution
Research bias
- Optimism bias
- Cognitive bias
- Implicit bias
- Hawthorne effect
- Anchoring bias
- Explicit bias
A literature review is a survey of scholarly sources (such as books, journal articles, and theses) related to a specific topic or research question .
It is often written as part of a thesis, dissertation , or research paper , in order to situate your work in relation to existing knowledge.
There are several reasons to conduct a literature review at the beginning of a research project:
- To familiarize yourself with the current state of knowledge on your topic
- To ensure that you’re not just repeating what others have already done
- To identify gaps in knowledge and unresolved problems that your research can address
- To develop your theoretical framework and methodology
- To provide an overview of the key findings and debates on the topic
Writing the literature review shows your reader how your work relates to existing research and what new insights it will contribute.
The literature review usually comes near the beginning of your thesis or dissertation . After the introduction , it grounds your research in a scholarly field and leads directly to your theoretical framework or methodology .
A literature review is a survey of credible sources on a topic, often used in dissertations , theses, and research papers . Literature reviews give an overview of knowledge on a subject, helping you identify relevant theories and methods, as well as gaps in existing research. Literature reviews are set up similarly to other academic texts , with an introduction , a main body, and a conclusion .
An annotated bibliography is a list of source references that has a short description (called an annotation ) for each of the sources. It is often assigned as part of the research process for a paper .
Cite this Scribbr article
If you want to cite this source, you can copy and paste the citation or click the “Cite this Scribbr article” button to automatically add the citation to our free Citation Generator.
McCombes, S. (2023, September 11). How to Write a Literature Review | Guide, Examples, & Templates. Scribbr. Retrieved April 2, 2024, from https://www.scribbr.com/dissertation/literature-review/
Is this article helpful?
Shona McCombes
Other students also liked, what is a theoretical framework | guide to organizing, what is a research methodology | steps & tips, how to write a research proposal | examples & templates, what is your plagiarism score.
Thank you for visiting nature.com. You are using a browser version with limited support for CSS. To obtain the best experience, we recommend you use a more up to date browser (or turn off compatibility mode in Internet Explorer). In the meantime, to ensure continued support, we are displaying the site without styles and JavaScript.
- View all journals
- Explore content
- About the journal
- Publish with us
- Sign up for alerts
- CAREER FEATURE
- 04 December 2020
- Correction 09 December 2020
How to write a superb literature review
Andy Tay is a freelance writer based in Singapore.
You can also search for this author in PubMed Google Scholar
Literature reviews are important resources for scientists. They provide historical context for a field while offering opinions on its future trajectory. Creating them can provide inspiration for one’s own research, as well as some practice in writing. But few scientists are trained in how to write a review — or in what constitutes an excellent one. Even picking the appropriate software to use can be an involved decision (see ‘Tools and techniques’). So Nature asked editors and working scientists with well-cited reviews for their tips.
Access options
Access Nature and 54 other Nature Portfolio journals
Get Nature+, our best-value online-access subscription
24,99 € / 30 days
cancel any time
Subscribe to this journal
Receive 51 print issues and online access
185,98 € per year
only 3,65 € per issue
Rent or buy this article
Prices vary by article type
Prices may be subject to local taxes which are calculated during checkout
doi: https://doi.org/10.1038/d41586-020-03422-x
Interviews have been edited for length and clarity.
Updates & Corrections
Correction 09 December 2020 : An earlier version of the tables in this article included some incorrect details about the programs Zotero, Endnote and Manubot. These have now been corrected.
Hsing, I.-M., Xu, Y. & Zhao, W. Electroanalysis 19 , 755–768 (2007).
Article Google Scholar
Ledesma, H. A. et al. Nature Nanotechnol. 14 , 645–657 (2019).
Article PubMed Google Scholar
Brahlek, M., Koirala, N., Bansal, N. & Oh, S. Solid State Commun. 215–216 , 54–62 (2015).
Choi, Y. & Lee, S. Y. Nature Rev. Chem . https://doi.org/10.1038/s41570-020-00221-w (2020).
Download references
Related Articles
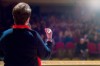
- Research management
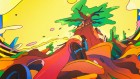
Africa’s postdoc workforce is on the rise — but at what cost?
Career Feature 02 APR 24
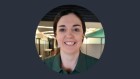
Impact factors are outdated, but new research assessments still fail scientists
World View 02 APR 24
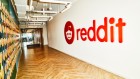
How scientists are making the most of Reddit
Career Feature 01 APR 24
Adopt universal standards for study adaptation to boost health, education and social-science research
Correspondence 02 APR 24
How can we make PhD training fit for the modern world? Broaden its philosophical foundations
Allow researchers with caring responsibilities ‘promotion pauses’ to make research more equitable
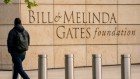
Will the Gates Foundation’s preprint-centric policy help open access?
News 04 APR 24
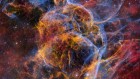
The corpse of an exploded star and more — March’s best science images
News 28 MAR 24
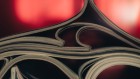
How papers with doctored images can affect scientific reviews
Global Scientist Interdisciplinary Forum & Recruitment
Southern University of Science and Technology, School of Medicine
Shenzhen, Guangdong, China
Research Associate - Neuroscience and Respiratory Physiology
Houston, Texas (US)
Baylor College of Medicine (BCM)
Histology Laboratory Manager
Postdoctoral scholar - research-pediatrics.
Memphis, Tennessee
The University of Tennessee Health Science Center (UTHSC)
Postdoctoral Scholar
Postdoctoral Scholar - PHAST Investigate Novel Roles of Linear Deubiquitinase Otulin In NeuroDegenerato
Memphis, Tennessee (US)
Sign up for the Nature Briefing newsletter — what matters in science, free to your inbox daily.
Quick links
- Explore articles by subject
- Guide to authors
- Editorial policies
When you choose to publish with PLOS, your research makes an impact. Make your work accessible to all, without restrictions, and accelerate scientific discovery with options like preprints and published peer review that make your work more Open.
- PLOS Biology
- PLOS Climate
- PLOS Complex Systems
- PLOS Computational Biology
- PLOS Digital Health
- PLOS Genetics
- PLOS Global Public Health
- PLOS Medicine
- PLOS Mental Health
- PLOS Neglected Tropical Diseases
- PLOS Pathogens
- PLOS Sustainability and Transformation
- PLOS Collections
How to Write a Peer Review

When you write a peer review for a manuscript, what should you include in your comments? What should you leave out? And how should the review be formatted?
This guide provides quick tips for writing and organizing your reviewer report.
Review Outline
Use an outline for your reviewer report so it’s easy for the editors and author to follow. This will also help you keep your comments organized.
Think about structuring your review like an inverted pyramid. Put the most important information at the top, followed by details and examples in the center, and any additional points at the very bottom.

Here’s how your outline might look:
1. Summary of the research and your overall impression
In your own words, summarize what the manuscript claims to report. This shows the editor how you interpreted the manuscript and will highlight any major differences in perspective between you and the other reviewers. Give an overview of the manuscript’s strengths and weaknesses. Think about this as your “take-home” message for the editors. End this section with your recommended course of action.
2. Discussion of specific areas for improvement
It’s helpful to divide this section into two parts: one for major issues and one for minor issues. Within each section, you can talk about the biggest issues first or go systematically figure-by-figure or claim-by-claim. Number each item so that your points are easy to follow (this will also make it easier for the authors to respond to each point). Refer to specific lines, pages, sections, or figure and table numbers so the authors (and editors) know exactly what you’re talking about.
Major vs. minor issues
What’s the difference between a major and minor issue? Major issues should consist of the essential points the authors need to address before the manuscript can proceed. Make sure you focus on what is fundamental for the current study . In other words, it’s not helpful to recommend additional work that would be considered the “next step” in the study. Minor issues are still important but typically will not affect the overall conclusions of the manuscript. Here are some examples of what would might go in the “minor” category:
- Missing references (but depending on what is missing, this could also be a major issue)
- Technical clarifications (e.g., the authors should clarify how a reagent works)
- Data presentation (e.g., the authors should present p-values differently)
- Typos, spelling, grammar, and phrasing issues
3. Any other points
Confidential comments for the editors.
Some journals have a space for reviewers to enter confidential comments about the manuscript. Use this space to mention concerns about the submission that you’d want the editors to consider before sharing your feedback with the authors, such as concerns about ethical guidelines or language quality. Any serious issues should be raised directly and immediately with the journal as well.
This section is also where you will disclose any potentially competing interests, and mention whether you’re willing to look at a revised version of the manuscript.
Do not use this space to critique the manuscript, since comments entered here will not be passed along to the authors. If you’re not sure what should go in the confidential comments, read the reviewer instructions or check with the journal first before submitting your review. If you are reviewing for a journal that does not offer a space for confidential comments, consider writing to the editorial office directly with your concerns.
Get this outline in a template
Giving Feedback
Giving feedback is hard. Giving effective feedback can be even more challenging. Remember that your ultimate goal is to discuss what the authors would need to do in order to qualify for publication. The point is not to nitpick every piece of the manuscript. Your focus should be on providing constructive and critical feedback that the authors can use to improve their study.
If you’ve ever had your own work reviewed, you already know that it’s not always easy to receive feedback. Follow the golden rule: Write the type of review you’d want to receive if you were the author. Even if you decide not to identify yourself in the review, you should write comments that you would be comfortable signing your name to.
In your comments, use phrases like “ the authors’ discussion of X” instead of “ your discussion of X .” This will depersonalize the feedback and keep the focus on the manuscript instead of the authors.
General guidelines for effective feedback
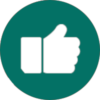
- Justify your recommendation with concrete evidence and specific examples.
- Be specific so the authors know what they need to do to improve.
- Be thorough. This might be the only time you read the manuscript.
- Be professional and respectful. The authors will be reading these comments too.
- Remember to say what you liked about the manuscript!

Don’t
- Recommend additional experiments or unnecessary elements that are out of scope for the study or for the journal criteria.
- Tell the authors exactly how to revise their manuscript—you don’t need to do their work for them.
- Use the review to promote your own research or hypotheses.
- Focus on typos and grammar. If the manuscript needs significant editing for language and writing quality, just mention this in your comments.
- Submit your review without proofreading it and checking everything one more time.
Before and After: Sample Reviewer Comments
Keeping in mind the guidelines above, how do you put your thoughts into words? Here are some sample “before” and “after” reviewer comments
✗ Before
“The authors appear to have no idea what they are talking about. I don’t think they have read any of the literature on this topic.”
✓ After
“The study fails to address how the findings relate to previous research in this area. The authors should rewrite their Introduction and Discussion to reference the related literature, especially recently published work such as Darwin et al.”
“The writing is so bad, it is practically unreadable. I could barely bring myself to finish it.”
“While the study appears to be sound, the language is unclear, making it difficult to follow. I advise the authors work with a writing coach or copyeditor to improve the flow and readability of the text.”
“It’s obvious that this type of experiment should have been included. I have no idea why the authors didn’t use it. This is a big mistake.”
“The authors are off to a good start, however, this study requires additional experiments, particularly [type of experiment]. Alternatively, the authors should include more information that clarifies and justifies their choice of methods.”
Suggested Language for Tricky Situations
You might find yourself in a situation where you’re not sure how to explain the problem or provide feedback in a constructive and respectful way. Here is some suggested language for common issues you might experience.
What you think : The manuscript is fatally flawed. What you could say: “The study does not appear to be sound” or “the authors have missed something crucial”.
What you think : You don’t completely understand the manuscript. What you could say : “The authors should clarify the following sections to avoid confusion…”
What you think : The technical details don’t make sense. What you could say : “The technical details should be expanded and clarified to ensure that readers understand exactly what the researchers studied.”
What you think: The writing is terrible. What you could say : “The authors should revise the language to improve readability.”
What you think : The authors have over-interpreted the findings. What you could say : “The authors aim to demonstrate [XYZ], however, the data does not fully support this conclusion. Specifically…”
What does a good review look like?
Check out the peer review examples at F1000 Research to see how other reviewers write up their reports and give constructive feedback to authors.
Time to Submit the Review!
Be sure you turn in your report on time. Need an extension? Tell the journal so that they know what to expect. If you need a lot of extra time, the journal might need to contact other reviewers or notify the author about the delay.
Tip: Building a relationship with an editor
You’ll be more likely to be asked to review again if you provide high-quality feedback and if you turn in the review on time. Especially if it’s your first review for a journal, it’s important to show that you are reliable. Prove yourself once and you’ll get asked to review again!
- Getting started as a reviewer
- Responding to an invitation
- Reading a manuscript
- Writing a peer review
The contents of the Peer Review Center are also available as a live, interactive training session, complete with slides, talking points, and activities. …
The contents of the Writing Center are also available as a live, interactive training session, complete with slides, talking points, and activities. …
There’s a lot to consider when deciding where to submit your work. Learn how to choose a journal that will help your study reach its audience, while reflecting your values as a researcher…
- Interesting
- Scholarships
- UGC-CARE Journals
How to Write a Best Review Paper to Get More Citation
Review Paper Writing Guide
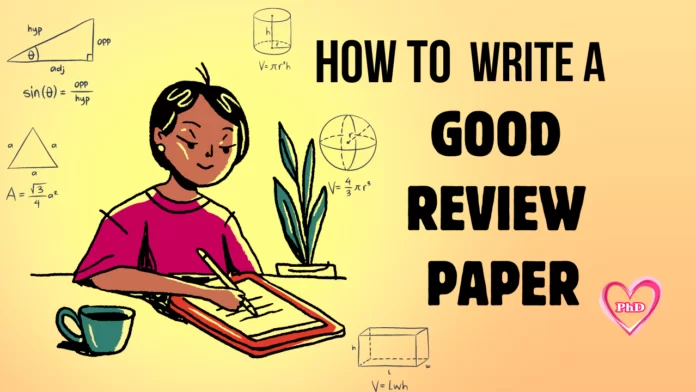
Table of contents
What is a review paper, difference between a review paper and a research paper.
- 6 Types of Review Papers
Purpose of Review Paper
Criteria for good review paper, step-by-step systematic procedure to write a review paper, title, abstract, keywords, introduction, various topics to discuss the critical issues, conclusion and future perspectives, acknowledgment .
Are you new to academia? Do you want to learn how to write a good review paper to get in-depth knowledge about your domain? you are at the right place. In this article, you will learn how to write the best review paper in a step-by-step systematic procedure with a sample review article format to get more Citation.
A review paper, or a literature review , is a thorough, analytical examination of previously published literature. It also provides an overview of current research works on a particular topic in chronological order.
- The main objective of writing a review paper is to evaluate the existing data or results, which can be done through analysis, modeling, classification, comparison, and summary.
- Review papers can help to identify the research gaps, to explore potential areas in a particular field.
- It helps to come out with new conclusions from already published works.
- Any scholar or researcher or scientist who wants to carry out research on a specific theme, first read the review articles relevant to that research area to understand the research gap for arriving at the problem statement.
- Writing a review article provides clarity, novelty, and contribution to the area of research and it demands a great level of in-depth understanding of the subject and a well-structured arrangement of discussions and arguments.
- Some journals publish only review papers, and they do not accept research articles. It is important to check the journal submission guidelines.
The difference between a review paper and a research paper is presented below.
6 T ypes of Review Papers
The review papers are classified in to six main categories based on the theme and it is presented in the figure below.
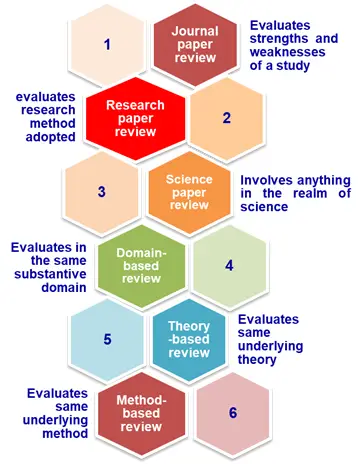
The purpose of a review paper is to assess a particular research question, theoretical or practical approach which provides readers with in-depth knowledge and state-of-the-art understanding of the research area.
The purpose of the review paper can vary based on their specific type and research needs.
- Provide a unified, collective overview of the current state of knowledge on a specific research topic and provide an inclusive foundation on a research theme.
- Identify ambiguity, and contradictions in existing results or data.
- Highlight the existing methodological approaches, research techniques, and unique perceptions.
- Develop theoretical outlines to resolve and work on published research.
- Discuss research gaps and future perspectives.
A good review paper needs to achieve three important criteria. ( Palmatier et al 2017 ).
- First, the area of research should be suitable for writing a review paper so that the author finds sufficient published literature.
- The review paper should be written with suitable literature, detailed discussion, sufficient data/results to support the interpretation, and persuasive language style.
- A completed review paper should provide substantial new innovative ideas to the readers based on the comparison of published works.
Review papers are widely read by many researchers and it helps to get more citations for author. So, it is important to learn how to write a review paper and find a journal to publish .
Time needed: 20 days and 7 hours
The systematic procedural steps to write the best review paper are as follows:
Select a suitable area in your research field formulate clear objectives, and prepare the specific research hypotheses that are to be explored.
Designing your research work is an important step for any researcher. Based on the objectives, develop a clear methodology or protocol to review a review paper.
Thorough analysis and understanding of different published works help the author to identify suitable and relevant data/results that will be used to write the paper.
The degree of analysis to evaluate the collected data varies by extensive review. The examination of trends, patterns, ideas, comparisons, and relationships in the study provides deeper knowledge on that area of research .
Interpretation of results is very important for a good review paper. The author should present the discussion systematically without any ambiguity. The results can be presented in descriptive form, tables, and figures. The new insights should have an in-depth discussion of the topic in line with fundamentals. Finally, the author is expected to present the limitations of the existing study with future perspectives.
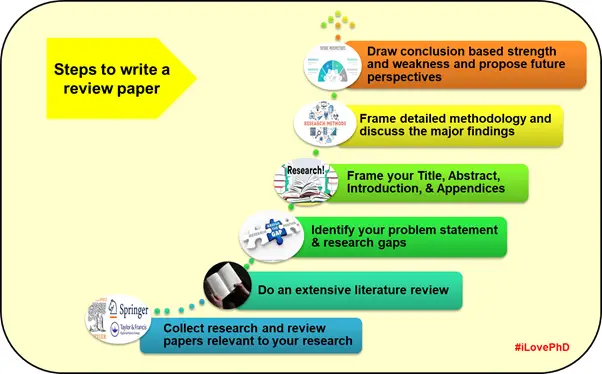
Sample Review Article Format
Write an effective and suitable title, abstract, and keywords relevant to your review paper. This will maximize the visibility of your paper online for the readers to find your work. Your title and abstract should be clear, concise, appropriate, and informative.
Present a detailed introduction to your research which is published in chronological order in your own words. Don’t summarize the published literature. The introduction should encourage the readers to read your paper.
Make sure you present a critical discussion, not a descriptive summary of the topic. If there is contradictory research in your area of research, verify to include an element of debate and present both sides of the argument. A good review paper can resolve the conflict between contradictory works.
The written review paper should achieve your objectives. Hence, the review paper should leave the reader with a clear understanding of the following questions:
What they can understand from the review paper?
What still remains a requirement of further investigation in the research area?
This can include making suggestions for future scope on the theme as part of your conclusion.
The authors can submit a brief acknowledgment of any financial, instrumentation, and academic support received about research work.
Citing references at appropriate places in the article is necessary and important to avoid plagiarism. Each journal has its referencing style. Therefore, the references need to be listed at the end of the manuscript. The number of references in the review paper is usually higher than in a research paper .
I hope this article will give you a clear idea of how to write a review paper. Please give your valuable comments.
iLovePhD Learn to Research Find Journals to Publish
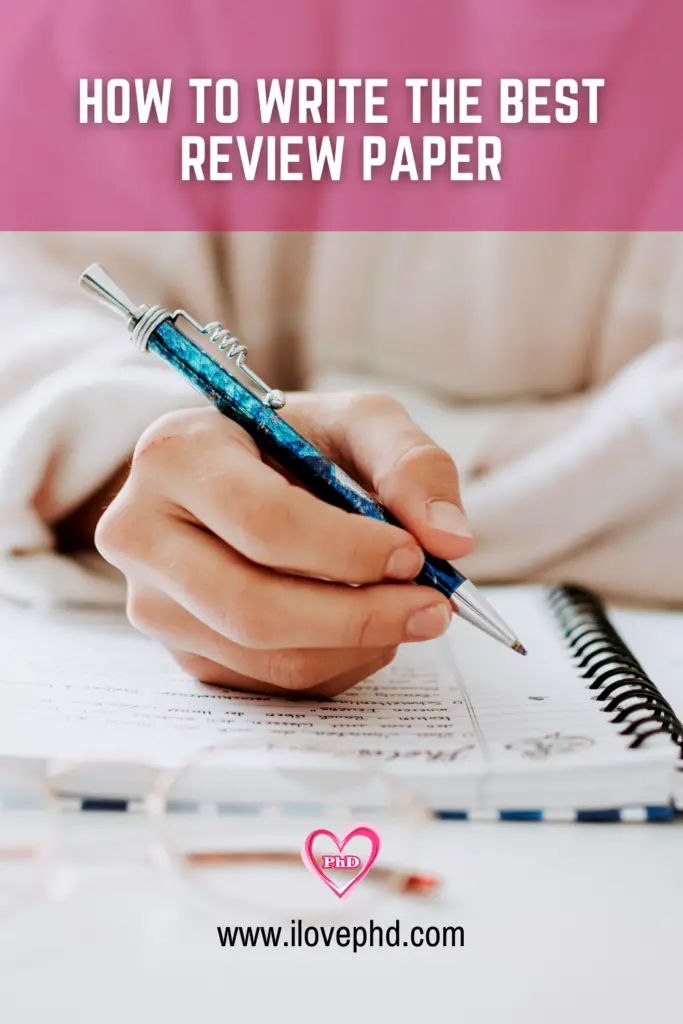
- Academic Citations
- Academic Writing
- academic writing tips
- basics of research
- Citation Boost
- citation management
- Citation Strategies
- easy review paper writing
- effective writing
- example of review paper
- how to write a literature review
- how to write a review article
- how to write a review paper
- improve your review paper
- Literature Review
- literature review tutorial
- Paper Impact
- Paper Writing
- qualitative research methods
- Research Paper
- Research Paper Writing
- research strategies
- review article
- review paper
- review paper writing guide
- Sample Review Paper
- Scholarly writing
- Scientific Review
- step-by-step review paper
- Types of Review Paper
- write a review paper for college
10 Tips to Quickly Publish Research Article with ChatGPT
Top scopus indexed journals in management 2024.
Nice thank you for your clarification
How to write a review paper on the relevant of science to education
This blog is very informative. Is it true that an increase in the number of citations improves the quality and impact of a review paper?
Thanks for your ideas. Really helpful
it was very well information
LEAVE A REPLY Cancel reply
Notify me of follow-up comments by email.
Notify me of new posts by email.
Email Subscription
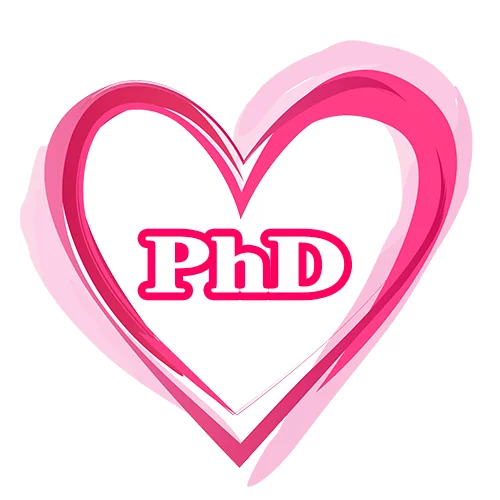
iLovePhD is a research education website to know updated research-related information. It helps researchers to find top journals for publishing research articles and get an easy manual for research tools. The main aim of this website is to help Ph.D. scholars who are working in various domains to get more valuable ideas to carry out their research. Learn the current groundbreaking research activities around the world, love the process of getting a Ph.D.
WhatsApp Channel
Join iLovePhD WhatsApp Channel Now!
Contact us: [email protected]
Copyright © 2019-2024 - iLovePhD
- Artificial intelligence

An official website of the United States government
The .gov means it’s official. Federal government websites often end in .gov or .mil. Before sharing sensitive information, make sure you’re on a federal government site.
The site is secure. The https:// ensures that you are connecting to the official website and that any information you provide is encrypted and transmitted securely.
- Publications
- Account settings
Preview improvements coming to the PMC website in October 2024. Learn More or Try it out now .
- Advanced Search
- Journal List
- PLoS Comput Biol
- v.9(7); 2013 Jul

Ten Simple Rules for Writing a Literature Review
Marco pautasso.
1 Centre for Functional and Evolutionary Ecology (CEFE), CNRS, Montpellier, France
2 Centre for Biodiversity Synthesis and Analysis (CESAB), FRB, Aix-en-Provence, France
Literature reviews are in great demand in most scientific fields. Their need stems from the ever-increasing output of scientific publications [1] . For example, compared to 1991, in 2008 three, eight, and forty times more papers were indexed in Web of Science on malaria, obesity, and biodiversity, respectively [2] . Given such mountains of papers, scientists cannot be expected to examine in detail every single new paper relevant to their interests [3] . Thus, it is both advantageous and necessary to rely on regular summaries of the recent literature. Although recognition for scientists mainly comes from primary research, timely literature reviews can lead to new synthetic insights and are often widely read [4] . For such summaries to be useful, however, they need to be compiled in a professional way [5] .
When starting from scratch, reviewing the literature can require a titanic amount of work. That is why researchers who have spent their career working on a certain research issue are in a perfect position to review that literature. Some graduate schools are now offering courses in reviewing the literature, given that most research students start their project by producing an overview of what has already been done on their research issue [6] . However, it is likely that most scientists have not thought in detail about how to approach and carry out a literature review.
Reviewing the literature requires the ability to juggle multiple tasks, from finding and evaluating relevant material to synthesising information from various sources, from critical thinking to paraphrasing, evaluating, and citation skills [7] . In this contribution, I share ten simple rules I learned working on about 25 literature reviews as a PhD and postdoctoral student. Ideas and insights also come from discussions with coauthors and colleagues, as well as feedback from reviewers and editors.
Rule 1: Define a Topic and Audience
How to choose which topic to review? There are so many issues in contemporary science that you could spend a lifetime of attending conferences and reading the literature just pondering what to review. On the one hand, if you take several years to choose, several other people may have had the same idea in the meantime. On the other hand, only a well-considered topic is likely to lead to a brilliant literature review [8] . The topic must at least be:
- interesting to you (ideally, you should have come across a series of recent papers related to your line of work that call for a critical summary),
- an important aspect of the field (so that many readers will be interested in the review and there will be enough material to write it), and
- a well-defined issue (otherwise you could potentially include thousands of publications, which would make the review unhelpful).
Ideas for potential reviews may come from papers providing lists of key research questions to be answered [9] , but also from serendipitous moments during desultory reading and discussions. In addition to choosing your topic, you should also select a target audience. In many cases, the topic (e.g., web services in computational biology) will automatically define an audience (e.g., computational biologists), but that same topic may also be of interest to neighbouring fields (e.g., computer science, biology, etc.).
Rule 2: Search and Re-search the Literature
After having chosen your topic and audience, start by checking the literature and downloading relevant papers. Five pieces of advice here:
- keep track of the search items you use (so that your search can be replicated [10] ),
- keep a list of papers whose pdfs you cannot access immediately (so as to retrieve them later with alternative strategies),
- use a paper management system (e.g., Mendeley, Papers, Qiqqa, Sente),
- define early in the process some criteria for exclusion of irrelevant papers (these criteria can then be described in the review to help define its scope), and
- do not just look for research papers in the area you wish to review, but also seek previous reviews.
The chances are high that someone will already have published a literature review ( Figure 1 ), if not exactly on the issue you are planning to tackle, at least on a related topic. If there are already a few or several reviews of the literature on your issue, my advice is not to give up, but to carry on with your own literature review,

The bottom-right situation (many literature reviews but few research papers) is not just a theoretical situation; it applies, for example, to the study of the impacts of climate change on plant diseases, where there appear to be more literature reviews than research studies [33] .
- discussing in your review the approaches, limitations, and conclusions of past reviews,
- trying to find a new angle that has not been covered adequately in the previous reviews, and
- incorporating new material that has inevitably accumulated since their appearance.
When searching the literature for pertinent papers and reviews, the usual rules apply:
- be thorough,
- use different keywords and database sources (e.g., DBLP, Google Scholar, ISI Proceedings, JSTOR Search, Medline, Scopus, Web of Science), and
- look at who has cited past relevant papers and book chapters.
Rule 3: Take Notes While Reading
If you read the papers first, and only afterwards start writing the review, you will need a very good memory to remember who wrote what, and what your impressions and associations were while reading each single paper. My advice is, while reading, to start writing down interesting pieces of information, insights about how to organize the review, and thoughts on what to write. This way, by the time you have read the literature you selected, you will already have a rough draft of the review.
Of course, this draft will still need much rewriting, restructuring, and rethinking to obtain a text with a coherent argument [11] , but you will have avoided the danger posed by staring at a blank document. Be careful when taking notes to use quotation marks if you are provisionally copying verbatim from the literature. It is advisable then to reformulate such quotes with your own words in the final draft. It is important to be careful in noting the references already at this stage, so as to avoid misattributions. Using referencing software from the very beginning of your endeavour will save you time.
Rule 4: Choose the Type of Review You Wish to Write
After having taken notes while reading the literature, you will have a rough idea of the amount of material available for the review. This is probably a good time to decide whether to go for a mini- or a full review. Some journals are now favouring the publication of rather short reviews focusing on the last few years, with a limit on the number of words and citations. A mini-review is not necessarily a minor review: it may well attract more attention from busy readers, although it will inevitably simplify some issues and leave out some relevant material due to space limitations. A full review will have the advantage of more freedom to cover in detail the complexities of a particular scientific development, but may then be left in the pile of the very important papers “to be read” by readers with little time to spare for major monographs.
There is probably a continuum between mini- and full reviews. The same point applies to the dichotomy of descriptive vs. integrative reviews. While descriptive reviews focus on the methodology, findings, and interpretation of each reviewed study, integrative reviews attempt to find common ideas and concepts from the reviewed material [12] . A similar distinction exists between narrative and systematic reviews: while narrative reviews are qualitative, systematic reviews attempt to test a hypothesis based on the published evidence, which is gathered using a predefined protocol to reduce bias [13] , [14] . When systematic reviews analyse quantitative results in a quantitative way, they become meta-analyses. The choice between different review types will have to be made on a case-by-case basis, depending not just on the nature of the material found and the preferences of the target journal(s), but also on the time available to write the review and the number of coauthors [15] .
Rule 5: Keep the Review Focused, but Make It of Broad Interest
Whether your plan is to write a mini- or a full review, it is good advice to keep it focused 16 , 17 . Including material just for the sake of it can easily lead to reviews that are trying to do too many things at once. The need to keep a review focused can be problematic for interdisciplinary reviews, where the aim is to bridge the gap between fields [18] . If you are writing a review on, for example, how epidemiological approaches are used in modelling the spread of ideas, you may be inclined to include material from both parent fields, epidemiology and the study of cultural diffusion. This may be necessary to some extent, but in this case a focused review would only deal in detail with those studies at the interface between epidemiology and the spread of ideas.
While focus is an important feature of a successful review, this requirement has to be balanced with the need to make the review relevant to a broad audience. This square may be circled by discussing the wider implications of the reviewed topic for other disciplines.
Rule 6: Be Critical and Consistent
Reviewing the literature is not stamp collecting. A good review does not just summarize the literature, but discusses it critically, identifies methodological problems, and points out research gaps [19] . After having read a review of the literature, a reader should have a rough idea of:
- the major achievements in the reviewed field,
- the main areas of debate, and
- the outstanding research questions.
It is challenging to achieve a successful review on all these fronts. A solution can be to involve a set of complementary coauthors: some people are excellent at mapping what has been achieved, some others are very good at identifying dark clouds on the horizon, and some have instead a knack at predicting where solutions are going to come from. If your journal club has exactly this sort of team, then you should definitely write a review of the literature! In addition to critical thinking, a literature review needs consistency, for example in the choice of passive vs. active voice and present vs. past tense.
Rule 7: Find a Logical Structure
Like a well-baked cake, a good review has a number of telling features: it is worth the reader's time, timely, systematic, well written, focused, and critical. It also needs a good structure. With reviews, the usual subdivision of research papers into introduction, methods, results, and discussion does not work or is rarely used. However, a general introduction of the context and, toward the end, a recapitulation of the main points covered and take-home messages make sense also in the case of reviews. For systematic reviews, there is a trend towards including information about how the literature was searched (database, keywords, time limits) [20] .
How can you organize the flow of the main body of the review so that the reader will be drawn into and guided through it? It is generally helpful to draw a conceptual scheme of the review, e.g., with mind-mapping techniques. Such diagrams can help recognize a logical way to order and link the various sections of a review [21] . This is the case not just at the writing stage, but also for readers if the diagram is included in the review as a figure. A careful selection of diagrams and figures relevant to the reviewed topic can be very helpful to structure the text too [22] .
Rule 8: Make Use of Feedback
Reviews of the literature are normally peer-reviewed in the same way as research papers, and rightly so [23] . As a rule, incorporating feedback from reviewers greatly helps improve a review draft. Having read the review with a fresh mind, reviewers may spot inaccuracies, inconsistencies, and ambiguities that had not been noticed by the writers due to rereading the typescript too many times. It is however advisable to reread the draft one more time before submission, as a last-minute correction of typos, leaps, and muddled sentences may enable the reviewers to focus on providing advice on the content rather than the form.
Feedback is vital to writing a good review, and should be sought from a variety of colleagues, so as to obtain a diversity of views on the draft. This may lead in some cases to conflicting views on the merits of the paper, and on how to improve it, but such a situation is better than the absence of feedback. A diversity of feedback perspectives on a literature review can help identify where the consensus view stands in the landscape of the current scientific understanding of an issue [24] .
Rule 9: Include Your Own Relevant Research, but Be Objective
In many cases, reviewers of the literature will have published studies relevant to the review they are writing. This could create a conflict of interest: how can reviewers report objectively on their own work [25] ? Some scientists may be overly enthusiastic about what they have published, and thus risk giving too much importance to their own findings in the review. However, bias could also occur in the other direction: some scientists may be unduly dismissive of their own achievements, so that they will tend to downplay their contribution (if any) to a field when reviewing it.
In general, a review of the literature should neither be a public relations brochure nor an exercise in competitive self-denial. If a reviewer is up to the job of producing a well-organized and methodical review, which flows well and provides a service to the readership, then it should be possible to be objective in reviewing one's own relevant findings. In reviews written by multiple authors, this may be achieved by assigning the review of the results of a coauthor to different coauthors.
Rule 10: Be Up-to-Date, but Do Not Forget Older Studies
Given the progressive acceleration in the publication of scientific papers, today's reviews of the literature need awareness not just of the overall direction and achievements of a field of inquiry, but also of the latest studies, so as not to become out-of-date before they have been published. Ideally, a literature review should not identify as a major research gap an issue that has just been addressed in a series of papers in press (the same applies, of course, to older, overlooked studies (“sleeping beauties” [26] )). This implies that literature reviewers would do well to keep an eye on electronic lists of papers in press, given that it can take months before these appear in scientific databases. Some reviews declare that they have scanned the literature up to a certain point in time, but given that peer review can be a rather lengthy process, a full search for newly appeared literature at the revision stage may be worthwhile. Assessing the contribution of papers that have just appeared is particularly challenging, because there is little perspective with which to gauge their significance and impact on further research and society.
Inevitably, new papers on the reviewed topic (including independently written literature reviews) will appear from all quarters after the review has been published, so that there may soon be the need for an updated review. But this is the nature of science [27] – [32] . I wish everybody good luck with writing a review of the literature.
Acknowledgments
Many thanks to M. Barbosa, K. Dehnen-Schmutz, T. Döring, D. Fontaneto, M. Garbelotto, O. Holdenrieder, M. Jeger, D. Lonsdale, A. MacLeod, P. Mills, M. Moslonka-Lefebvre, G. Stancanelli, P. Weisberg, and X. Xu for insights and discussions, and to P. Bourne, T. Matoni, and D. Smith for helpful comments on a previous draft.
Funding Statement
This work was funded by the French Foundation for Research on Biodiversity (FRB) through its Centre for Synthesis and Analysis of Biodiversity data (CESAB), as part of the NETSEED research project. The funders had no role in the preparation of the manuscript.
COMMUNICATION IN THE BIOLOGICAL SCIENCES Department of Biology
LITERATURE REVIEW PAPER
WHAT IS A REVIEW PAPER?
CHOOSING A TOPIC
RESEARCHING A TOPIC
HOW TO WRITE THE PAPER
The purpose of a review paper is to succinctly review recent progress in a particular topic. Overall, the paper summarizes the current state of knowledge of the topic. It creates an understanding of the topic for the reader by discussing the findings presented in recent research papers .
A review paper is not a "term paper" or book report . It is not merely a report on some references you found. Instead, a review paper synthesizes the results from several primary literature papers to produce a coherent argument about a topic or focused description of a field.
Examples of scientific reviews can be found in:
Current Opinion in Cell Biology
Current Opinion in Genetics & Development
Annual Review of Plant Physiology and Plant Molecular Biology
Annual Review of Physiology
Trends in Ecology & Evolution
You should read articles from one or more of these sources to get examples of how your paper should be organized.
Scientists commonly use reviews to communicate with each other and the general public. There are a wide variety of review styles from ones aimed at a general audience (e.g., Scientific American ) to those directed at biologists within a particular subdiscipline (e.g., Annual Review of Physiology ).
A key aspect of a review paper is that it provides the evidence for a particular point of view in a field. Thus, a large focus of your paper should be a description of the data that support or refute that point of view. In addition, you should inform the reader of the experimental techniques that were used to generate the data.
The emphasis of a review paper is interpreting the primary literature on the subject. You need to read several original research articles on the same topic and make your own conclusions about the meanings of those papers.
Click here for advice on choosing a topic.
Click here for advice on doing research on your topic.
HOW TO WRITE THE PAPER
Overview of the Paper: Your paper should consist of four general sections:
Review articles contain neither a materials and methods section nor an abstract.
Organizing the Paper: Use topic headings. Do not use a topic heading that reads, "Body of the paper." Instead the topic headings should refer to the actual concepts or ideas covered in that section.
Example
What Goes into Each Section:
Home
How to write a good scientific review article
Affiliation.
- 1 The FEBS Journal Editorial Office, Cambridge, UK.
- PMID: 35792782
- DOI: 10.1111/febs.16565
Literature reviews are valuable resources for the scientific community. With research accelerating at an unprecedented speed in recent years and more and more original papers being published, review articles have become increasingly important as a means to keep up to date with developments in a particular area of research. A good review article provides readers with an in-depth understanding of a field and highlights key gaps and challenges to address with future research. Writing a review article also helps to expand the writer's knowledge of their specialist area and to develop their analytical and communication skills, amongst other benefits. Thus, the importance of building review-writing into a scientific career cannot be overstated. In this instalment of The FEBS Journal's Words of Advice series, I provide detailed guidance on planning and writing an informative and engaging literature review.
© 2022 Federation of European Biochemical Societies.
Publication types
Purdue Online Writing Lab Purdue OWL® College of Liberal Arts
Writing a Literature Review
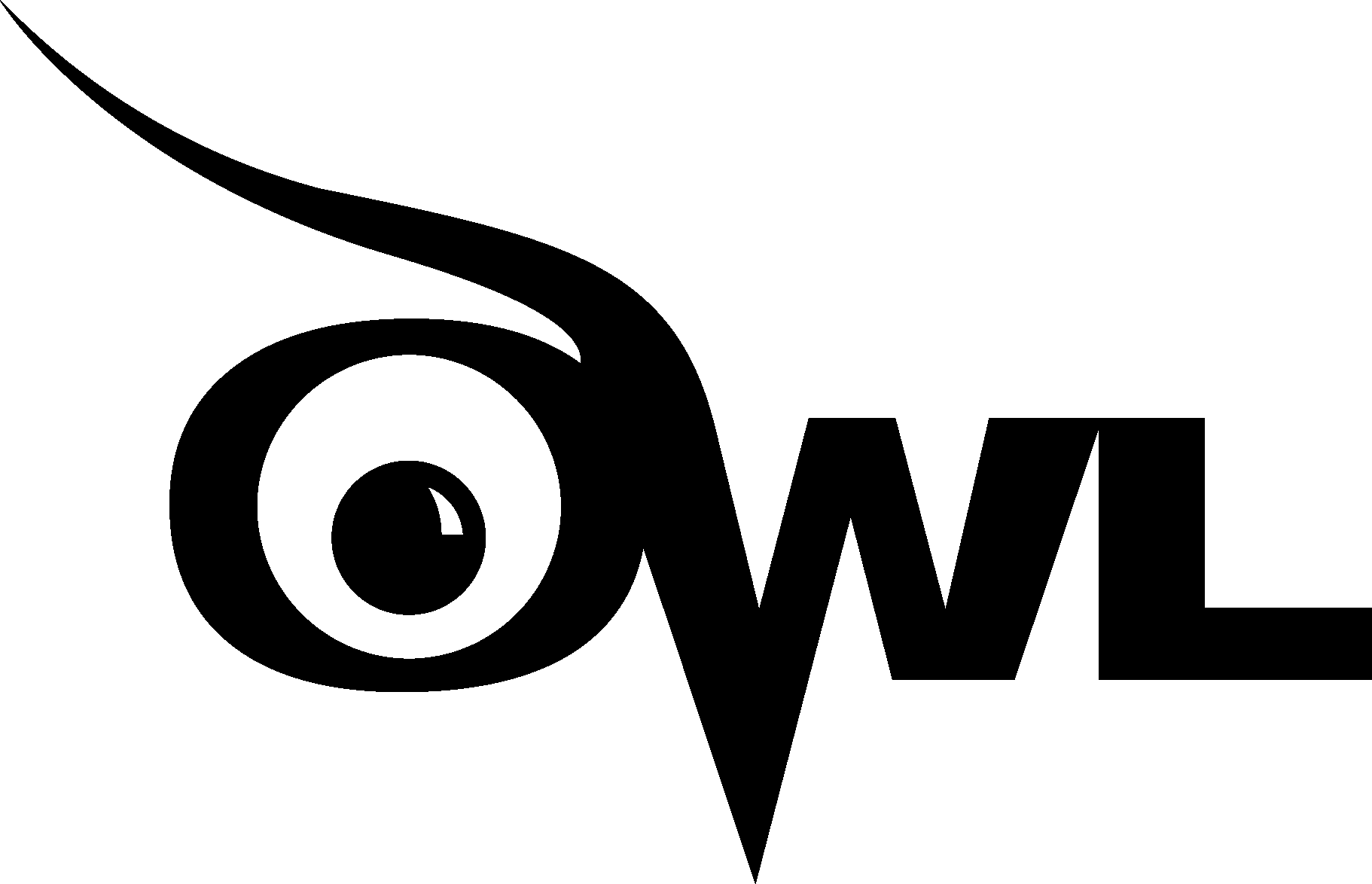
Welcome to the Purdue OWL
This page is brought to you by the OWL at Purdue University. When printing this page, you must include the entire legal notice.
Copyright ©1995-2018 by The Writing Lab & The OWL at Purdue and Purdue University. All rights reserved. This material may not be published, reproduced, broadcast, rewritten, or redistributed without permission. Use of this site constitutes acceptance of our terms and conditions of fair use.
A literature review is a document or section of a document that collects key sources on a topic and discusses those sources in conversation with each other (also called synthesis ). The lit review is an important genre in many disciplines, not just literature (i.e., the study of works of literature such as novels and plays). When we say “literature review” or refer to “the literature,” we are talking about the research ( scholarship ) in a given field. You will often see the terms “the research,” “the scholarship,” and “the literature” used mostly interchangeably.
Where, when, and why would I write a lit review?
There are a number of different situations where you might write a literature review, each with slightly different expectations; different disciplines, too, have field-specific expectations for what a literature review is and does. For instance, in the humanities, authors might include more overt argumentation and interpretation of source material in their literature reviews, whereas in the sciences, authors are more likely to report study designs and results in their literature reviews; these differences reflect these disciplines’ purposes and conventions in scholarship. You should always look at examples from your own discipline and talk to professors or mentors in your field to be sure you understand your discipline’s conventions, for literature reviews as well as for any other genre.
A literature review can be a part of a research paper or scholarly article, usually falling after the introduction and before the research methods sections. In these cases, the lit review just needs to cover scholarship that is important to the issue you are writing about; sometimes it will also cover key sources that informed your research methodology.
Lit reviews can also be standalone pieces, either as assignments in a class or as publications. In a class, a lit review may be assigned to help students familiarize themselves with a topic and with scholarship in their field, get an idea of the other researchers working on the topic they’re interested in, find gaps in existing research in order to propose new projects, and/or develop a theoretical framework and methodology for later research. As a publication, a lit review usually is meant to help make other scholars’ lives easier by collecting and summarizing, synthesizing, and analyzing existing research on a topic. This can be especially helpful for students or scholars getting into a new research area, or for directing an entire community of scholars toward questions that have not yet been answered.
What are the parts of a lit review?
Most lit reviews use a basic introduction-body-conclusion structure; if your lit review is part of a larger paper, the introduction and conclusion pieces may be just a few sentences while you focus most of your attention on the body. If your lit review is a standalone piece, the introduction and conclusion take up more space and give you a place to discuss your goals, research methods, and conclusions separately from where you discuss the literature itself.
Introduction:
- An introductory paragraph that explains what your working topic and thesis is
- A forecast of key topics or texts that will appear in the review
- Potentially, a description of how you found sources and how you analyzed them for inclusion and discussion in the review (more often found in published, standalone literature reviews than in lit review sections in an article or research paper)
- Summarize and synthesize: Give an overview of the main points of each source and combine them into a coherent whole
- Analyze and interpret: Don’t just paraphrase other researchers – add your own interpretations where possible, discussing the significance of findings in relation to the literature as a whole
- Critically Evaluate: Mention the strengths and weaknesses of your sources
- Write in well-structured paragraphs: Use transition words and topic sentence to draw connections, comparisons, and contrasts.
Conclusion:
- Summarize the key findings you have taken from the literature and emphasize their significance
- Connect it back to your primary research question
How should I organize my lit review?
Lit reviews can take many different organizational patterns depending on what you are trying to accomplish with the review. Here are some examples:
- Chronological : The simplest approach is to trace the development of the topic over time, which helps familiarize the audience with the topic (for instance if you are introducing something that is not commonly known in your field). If you choose this strategy, be careful to avoid simply listing and summarizing sources in order. Try to analyze the patterns, turning points, and key debates that have shaped the direction of the field. Give your interpretation of how and why certain developments occurred (as mentioned previously, this may not be appropriate in your discipline — check with a teacher or mentor if you’re unsure).
- Thematic : If you have found some recurring central themes that you will continue working with throughout your piece, you can organize your literature review into subsections that address different aspects of the topic. For example, if you are reviewing literature about women and religion, key themes can include the role of women in churches and the religious attitude towards women.
- Qualitative versus quantitative research
- Empirical versus theoretical scholarship
- Divide the research by sociological, historical, or cultural sources
- Theoretical : In many humanities articles, the literature review is the foundation for the theoretical framework. You can use it to discuss various theories, models, and definitions of key concepts. You can argue for the relevance of a specific theoretical approach or combine various theorical concepts to create a framework for your research.
What are some strategies or tips I can use while writing my lit review?
Any lit review is only as good as the research it discusses; make sure your sources are well-chosen and your research is thorough. Don’t be afraid to do more research if you discover a new thread as you’re writing. More info on the research process is available in our "Conducting Research" resources .
As you’re doing your research, create an annotated bibliography ( see our page on the this type of document ). Much of the information used in an annotated bibliography can be used also in a literature review, so you’ll be not only partially drafting your lit review as you research, but also developing your sense of the larger conversation going on among scholars, professionals, and any other stakeholders in your topic.
Usually you will need to synthesize research rather than just summarizing it. This means drawing connections between sources to create a picture of the scholarly conversation on a topic over time. Many student writers struggle to synthesize because they feel they don’t have anything to add to the scholars they are citing; here are some strategies to help you:
- It often helps to remember that the point of these kinds of syntheses is to show your readers how you understand your research, to help them read the rest of your paper.
- Writing teachers often say synthesis is like hosting a dinner party: imagine all your sources are together in a room, discussing your topic. What are they saying to each other?
- Look at the in-text citations in each paragraph. Are you citing just one source for each paragraph? This usually indicates summary only. When you have multiple sources cited in a paragraph, you are more likely to be synthesizing them (not always, but often
- Read more about synthesis here.
The most interesting literature reviews are often written as arguments (again, as mentioned at the beginning of the page, this is discipline-specific and doesn’t work for all situations). Often, the literature review is where you can establish your research as filling a particular gap or as relevant in a particular way. You have some chance to do this in your introduction in an article, but the literature review section gives a more extended opportunity to establish the conversation in the way you would like your readers to see it. You can choose the intellectual lineage you would like to be part of and whose definitions matter most to your thinking (mostly humanities-specific, but this goes for sciences as well). In addressing these points, you argue for your place in the conversation, which tends to make the lit review more compelling than a simple reporting of other sources.
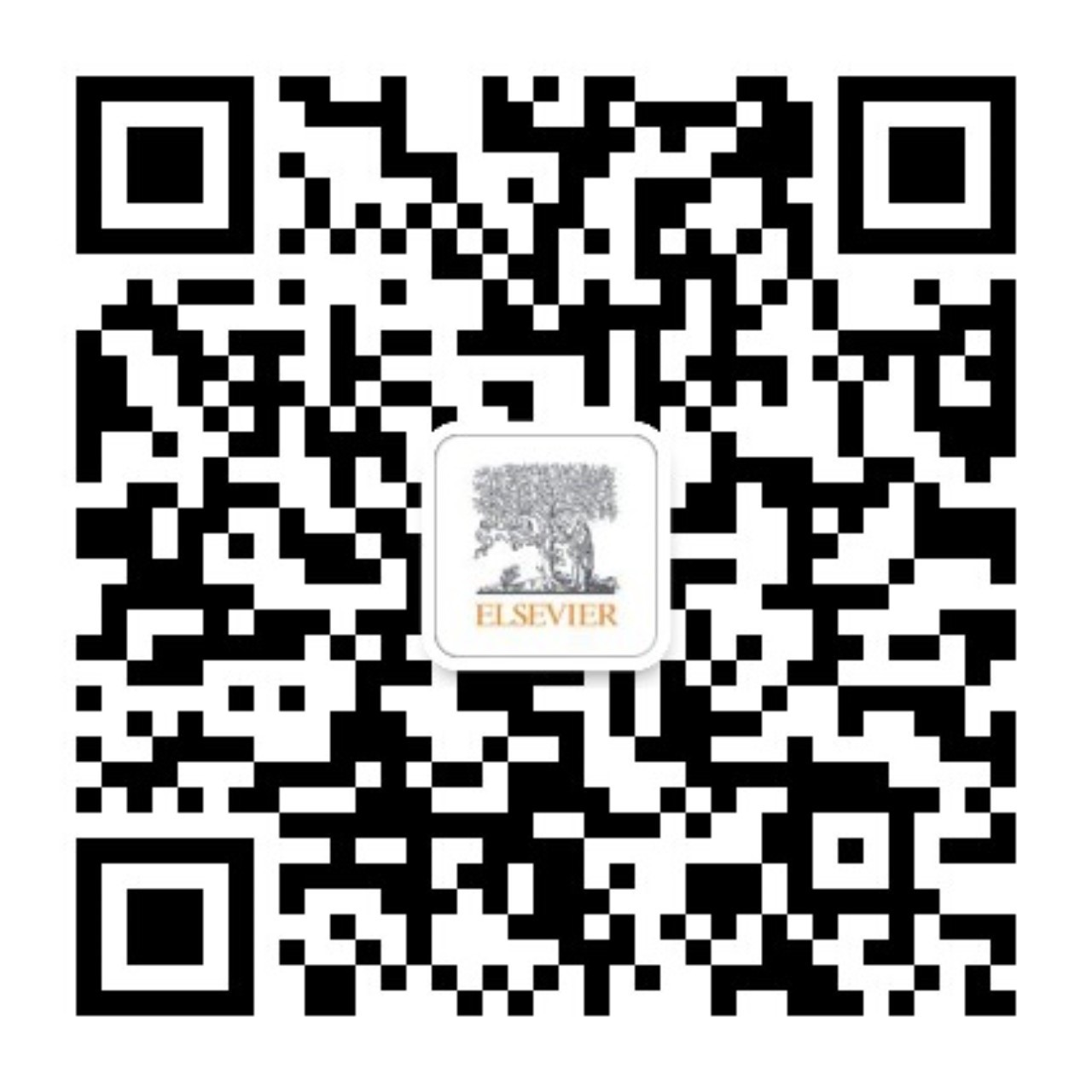
- Research Process
Writing a good review article
- 3 minute read
- 71.5K views
Table of Contents
As a young researcher, you might wonder how to start writing your first review article, and the extent of the information that it should contain. A review article is a comprehensive summary of the current understanding of a specific research topic and is based on previously published research. Unlike research papers, it does not contain new results, but can propose new inferences based on the combined findings of previous research.
Types of review articles
Review articles are typically of three types: literature reviews, systematic reviews, and meta-analyses.
A literature review is a general survey of the research topic and aims to provide a reliable and unbiased account of the current understanding of the topic.
A systematic review , in contrast, is more specific and attempts to address a highly focused research question. Its presentation is more detailed, with information on the search strategy used, the eligibility criteria for inclusion of studies, the methods utilized to review the collected information, and more.
A meta-analysis is similar to a systematic review in that both are systematically conducted with a properly defined research question. However, unlike the latter, a meta-analysis compares and evaluates a defined number of similar studies. It is quantitative in nature and can help assess contrasting study findings.
Tips for writing a good review article
Here are a few practices that can make the time-consuming process of writing a review article easier:
- Define your question: Take your time to identify the research question and carefully articulate the topic of your review paper. A good review should also add something new to the field in terms of a hypothesis, inference, or conclusion. A carefully defined scientific question will give you more clarity in determining the novelty of your inferences.
- Identify credible sources: Identify relevant as well as credible studies that you can base your review on, with the help of multiple databases or search engines. It is also a good idea to conduct another search once you have finished your article to avoid missing relevant studies published during the course of your writing.
- Take notes: A literature search involves extensive reading, which can make it difficult to recall relevant information subsequently. Therefore, make notes while conducting the literature search and note down the source references. This will ensure that you have sufficient information to start with when you finally get to writing.
- Describe the title, abstract, and introduction: A good starting point to begin structuring your review is by drafting the title, abstract, and introduction. Explicitly writing down what your review aims to address in the field will help shape the rest of your article.
- Be unbiased and critical: Evaluate every piece of evidence in a critical but unbiased manner. This will help you present a proper assessment and a critical discussion in your article.
- Include a good summary: End by stating the take-home message and identify the limitations of existing studies that need to be addressed through future studies.
- Ask for feedback: Ask a colleague to provide feedback on both the content and the language or tone of your article before you submit it.
- Check your journal’s guidelines: Some journals only publish reviews, while some only publish research articles. Further, all journals clearly indicate their aims and scope. Therefore, make sure to check the appropriateness of a journal before submitting your article.
Writing review articles, especially systematic reviews or meta-analyses, can seem like a daunting task. However, Elsevier Author Services can guide you by providing useful tips on how to write an impressive review article that stands out and gets published!
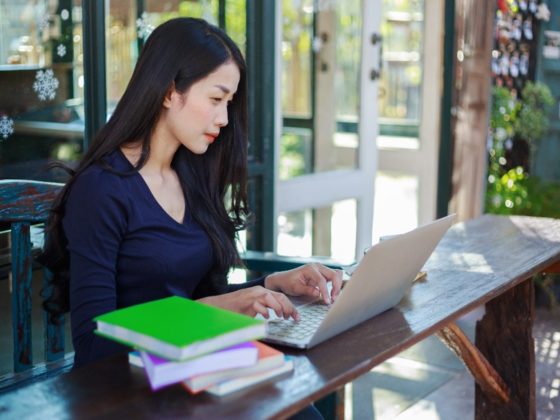
- Manuscript Preparation
What are Implications in Research?
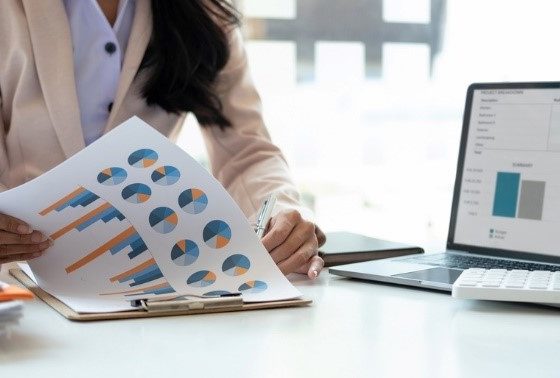
How to write the results section of a research paper
You may also like.
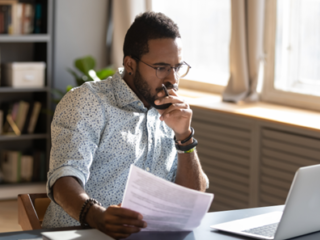
Descriptive Research Design and Its Myriad Uses
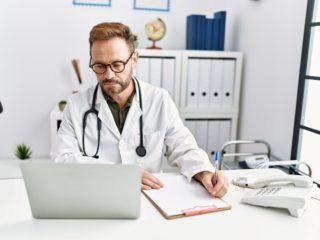
Five Common Mistakes to Avoid When Writing a Biomedical Research Paper
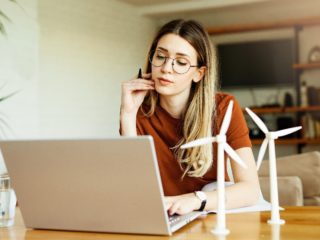
Making Technical Writing in Environmental Engineering Accessible
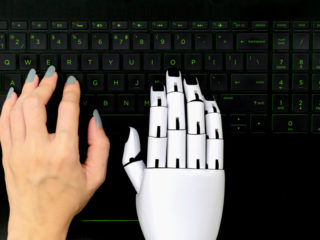
To Err is Not Human: The Dangers of AI-assisted Academic Writing
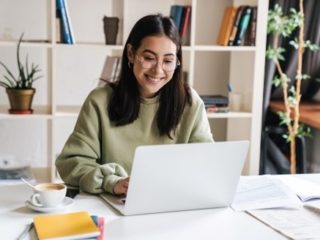
When Data Speak, Listen: Importance of Data Collection and Analysis Methods
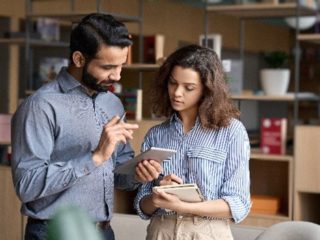
Choosing the Right Research Methodology: A Guide for Researchers
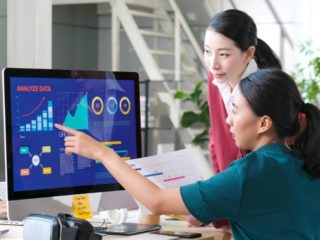
Why is data validation important in research?
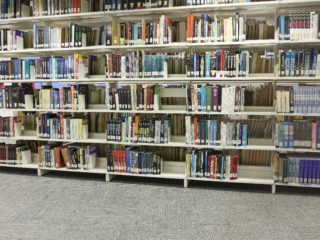
Scholarly Sources: What are They and Where can You Find Them?
Input your search keywords and press Enter.
- Open access
- Published: 29 August 2022
Enhancing health and wellness by, for and with Indigenous youth in Canada: a scoping review
- Udoka Okpalauwaekwe 1 ,
- Clifford Ballantyne 2 ,
- Scott Tunison 3 &
- Vivian R. Ramsden 4
BMC Public Health volume 22 , Article number: 1630 ( 2022 ) Cite this article
8139 Accesses
9 Citations
3 Altmetric
Metrics details
Indigenous youth in Canada face profound health inequities which are shaped by the rippling effects of intergenerational trauma, caused by the historical and contemporary colonial policies that reinforce negative stereotypes regarding them. Moreover, wellness promotion strategies for these youth are replete with individualistic Western concepts that excludes avenues for them to access holistic practices grounded in their culture. Our scoping review explored strategies, approaches, and ways health and wellness can be enhanced by, for, and with Indigenous youth in Canada by identifying barriers/roadblocks and facilitators/strengths to enhancing wellness among Indigenous youth in Canada.
We applied a systematic approach to searching and critically reviewing peer-reviewed literature using the Preferred Reporting Items for Systematic Reviews and Meta-Analyses extension for Scoping Reviews [PRISMA-ScR] as a reporting guideline. Our search strategy focused on specific keywords and MeSH terms for three major areas: Indigenous youth, health, and Canada. We used these keywords, to systematically search the following electronic databases published in English between January 01, 2017, to May 22, 2021: Medline [Ovid], PubMed, ERIC, Web of Science, Scopus, and iportal. We also used hand-searching and snowballing methods to identify relevant articles. Data collected were analysed for contents and themes.
From an initial 1695 articles collated, 20 articles met inclusion criteria for this review. Key facilitators/strengths to enhancing health and wellness by, for, and with Indigenous youth that emerged from our review included: promoting culturally appropriate interventions to engage Indigenous youth; using strength-based approaches; reliance on the wisdom of community Elders; taking responsibility; and providing access to wellness supports. Key barriers/roadblocks included: lack of community support for wellness promotion activities among Indigenous youth; structural/organizational issues within Indigenous communities; discrimination and social exclusion; cultural illiteracy among youth; cultural discordance with mainstream health systems and services; and addictions and risky behaviours.
This scoping review extracted 20 relevant articles about ways to engage Indigenous youth in health and wellness enhancement. Our findings demonstrate the importance of promoting health by, and with Indigenous youth, by engaging them in activities reflexive of their cultural norms, rather than imposing control measures that are incompatible with their value systems.
Peer Review reports
Introduction
The term ‘Indigenous’ is internationally recognized to describe a distinct group of people that live within or are attached to geographically distinct ancestral territories [ 1 , 2 ]. In Canada, the term Indigenous is an inclusive term used to refer to the First Nations, Métis, and Inuit people, each of which has unique histories, cultural traditions, languages, and beliefs [ 3 , 4 , 5 ]. Indigenous peoples are the fastest-growing population in Canada, with a population estimated at 1.8 million, which is 5.1% of the Canadian population [ 6 , 7 ]. Within this population, 63% identify as First Nation, 33% as Métis, and 4% as Inuit [ 6 , 7 ]. Indigenous youth are the youngest population in Canada, with over 50% of Indigenous youth under 25 years [ 7 ]. Projections of Indigenous peoples in Canada have estimated a 33.3 to 78.7% increase in Indigenous populations, with the youth making up the largest proportion of the Indigenous population by 2041 [ 6 , 7 ].
Before European contact in North America, Indigenous peoples in Canada lived and thrived with their cultures, languages, and distinct ways of knowing [ 2 ]. However, Indigenous peoples in Canada rank lower in almost every health determinant when compared with non-Indigenous Canadians [ 8 , 9 , 10 ]. A report on health disparities in Saskatoon, Saskatchewan, described First Nations peoples to be “more likely to experience poor health outcomes in essentially every indicator possible” (page 27) [ 11 ]. This greater burden of ill health among Indigenous peoples in Canada has been attributed to systemic racism (associated with differences in power, resources, capacities, and opportunities) [ 9 , 10 , 12 , 13 ] and intergenerational trauma (stemming from the past and ongoing legacy of colonization such as experienced through the Indian residential and Day school systems, the Sixties Scoop, and the ongoing waves of Indigenous child and youth apprehensions seen in the foster and child care structures that remove Indigenous children from their family, community and traditional lands) [ 3 , 9 , 10 , 12 , 13 , 14 , 15 , 16 , 17 ]. These traumatic historical events, along with ongoing inequities, such as: socioeconomic and environmental dispossession; loss of language; disruption of ties to Indigenous families, community, land and cultural traditions; have been reported to exacerbate drastically and cumulatively the physical, mental, social and spiritual health of Indigenous peoples in Canada, creating “soul wounds” (3 p.208) that require interventions beyond the Westernized biomedical models of health and healing [ 3 , 9 , 10 , 12 , 13 , 14 , 15 , 16 , 17 , 18 , 19 , 20 , 21 ].
In the same way, Indigenous youth in Canada face some of the most profound health inequities when compared with non-Indigenous youth which can be further shaped by the rippling effects of intergenerational trauma caused by the historical and contemporary colonial policies that reinforce or legitimize negative stereotypes regarding Indigenous youth in Canada [ 2 , 10 , 14 , 20 , 22 , 23 , 24 , 25 , 26 , 27 ]. When compared with their non-Indigenous peers, Indigenous youth in Canada have been reported to be more likely to have higher rates of chronic conditions [e.g., diabetes, obesity, chronic respiratory diseases, heart diseases, etc.] [ 14 ], discrimination [ 28 , 29 ], youth incarceration and state care [ 12 , 20 , 30 ], poverty [ 31 ], homelessness [ 32 ], higher adverse mental health conditions [ 20 , 33 , 34 , 35 , 36 , 37 ], higher suicide rates [ 33 , 38 , 39 ], and lower overall life expectancies [ 24 , 40 , 41 , 42 ].
Indigenous peoples’ perception of health and wellness is shaped by their worldview and traditional knowledge [ 43 , 44 ]. While the Western concept of health broadly defines health as the state of complete physical, mental, social well-being, and not merely the absence of disease [ 45 ], Indigenous peoples understand health in a holistic way [ 26 ] that seeks balance between the physical, mental, emotional, and spiritual aspects of an Indigenous person in reciprocal relationships with their families, communities, the land, the environment, their ancestors, and future generations [ 46 , 47 , 48 ]. Unfortunately, this holistic concept of health and wellness opposes the individualistic and biomedically focused Western worldview of health, which is a dominant lens commonly used in health research, projects, and programs involving Indigenous communities [ 46 ]. This practice further perpetuates the legacy of colonization and excludes avenues for Indigenous communities to access holistic healing practices “grounded in their culture” [ 43 , 49 , 50 ]. For example, health research involving Indigenous peoples in Canada tends to focus on Indigenous health deficits and identified social determinants in the communities, more often and without proper representation [ 43 ]. Additionally, there is the imposition of research on rather than with youth [ 43 , 44 ]; and the failure to acknowledge Indigenous worldviews in research, to ensure in benefits them [ 43 ].
Authentically engaging with Indigenous youth has been cited by Indigenous scholars as one of the ways of achieving and enhancing wellness by, for, and with youth [ 51 , 52 ]. This is characterized by meaningful and sustained involvement of the youth in program planning, development, and decision-making to promote self-confidence and positive relationships [ 53 ]. Authentic engagement involves working with rather than on youth as research partners or program planning participants [ 54 ]. This shift to working with rather than on implies respect for the knowledge of the lived experiences of the youth involved [ 54 , 55 , 56 ] and is based on meaningful relationships built over time among all involved [ 53 , 57 , 58 ]. Research has shown that engaging youth (Indigenous or non-Indigenous) as partners in a project/program fosters a sense of belonging, self-determination, and self-actualization within their community; thus, enhancing community wellness [ 54 , 56 , 58 , 59 ].
This paper explores what is known in the peer-reviewed literature about strategies, approaches, and ways to engage Indigenous youth in health and wellness enhancement. Our main objective is to use information gathered from this review to inform youth engagement strategies, by considering the facilitators/strengths and barriers/roadblocks to enhancing wellness with Indigenous youth. We define facilitators in this context as factors that improve, enhance, strengthen, or motivate a journey to health, wellness, and self-determination. These are considered ‘strengths’ in the language of Indigenous peoples as they support equitable strength-based pathways towards reconciliation. Conversely, barriers are roadblocks, and demotivating factors or processes that limit and challenge Indigenous peoples’ access to achieving health and wellness. Our overarching research question was, in what ways can Indigenous youth enhance health and wellness for themselves, their family, and the Indigenous communities where they live?
Sub-questions included:
What factors do Indigenous youth in Canada identify as facilitators/strengths to enhancing health and wellness?
What factors do Indigenous youth in Canada identify as barriers/roadblocks to enhancing health and wellness?
Methodology and methods
Scoping reviews help provide an overview of the research available on a given area of interest where evidence is emerging [ 60 ]. While there are several accepted approaches to such reviews, this scoping review was undertaken using the Joanna Briggs Institute (JBI) Guideline for scoping reviews [ 61 ]. This approach was based on the Arksey and O’Malley methodological framework [ 62 ], which was further advanced by Levac et al. [ 60 ], and Peter et al. [ 61 ]. Our search strategy focused on primary sources that elucidated youth-driven, youth-led, or youth-engaged strategies carried out by, for, and with Indigenous youth to enhance health and wellness. We chose to explore all health programs and research inquiry that explore health challenges on the physical, mental, emotional, and spiritual aspects of an Indigenous person to encompass the definition of health and wellness as defined and understood from an Indigenous perspective. This scoping review is reported in accordance with the guidelines provided in the Preferred Reporting Items for Systematic Reviews and Meta-Analyses (PRISMA) extension for Scoping Reviews (PRISMA-ScR) [ 63 ]. See Supplementary material file 1 for PRISMA-SCR checklist.
Protocol registration and reporting information
There was no pre-published or registered protocol before the commencement of this study.
Eligibility criteria
Types of studies.
A priori inclusion criteria for articles in this study included: 1] peer-reviewed journal articles reporting health and wellness programs, initiatives, and/or strategies among Indigenous youth in Canada, and 2] peer-reviewed journal articles published between January 01, 2017, to May 22, 2021. We chose a 5-year time frame to limit our findings to the most updated peer-reviewed literature which could provide implications for the growing body of work done in the field of Indigenous research among youth. Systematic reviews, meta-analyses, study protocols, opinion pieces, and narrative reviews were excluded.
Participants
Peer-reviewed studies involving Indigenous youth (First Nations, Métis, and Inuit) in Canada were eligible for inclusion. We considered the fluidity of definitions for youth by age range as literature sources generally defined youth in stages between adolescence to early adulthood [ 6 , 64 , 65 ]. In Canada, the Government of Canada uses several age brackets to identify youth depending on context, program, or policies in question. For example, Statistics Canada defines youth as between 15 to 29 years [ 6 ], Health Canada in the first State of Youth Report defined youth as between 12 to 30 years [ 65 ] when referring to statistical reports, and as between 13 to 36 years when referring to youth-led programs and policies [ 65 ]. However, for the purposes of this review we defined and referred to Indigenous youth or young people as between 10 to 24 years to be more representative of a broader definition of youth which is in keeping with Indigenous peoples’ worldviews, languages, and cultures and more representative of a broader definition of youth as offered by Sawyer et al. [ 64 ].
Information sources and search strategy
With the assistance of an Academic Reference Librarian, search terms were identified, which were categorized and combined into three conceptual MeSH terms that we adapted for the database-specific search strategy. These terms included: Indigenous youth (including synonyms and MeSH terms), health (including synonyms and MeSH terms) and Canada. Thus, studies were then identified for this scoping review by searching electronic databases and hand-searching reference lists of included articles.
Initially, the following databases (Medline (Ovid), PubMed, ERIC, Web of Science and Scopus) were used to identify relevant articles published between January 1, 2017, and April 30, 2021. This constituted our first search. We then carried out a second search (updated search) on May 22, 2021, using the same search queries on the same library databases; in addition, we included the University of Saskatchewan’s Indigenous Studies Portal (iPortal) [ 66 ] to ensure we had as many hits as possible for our search query on focused studies with Indigenous communities. To ensure exhaustiveness, we employed hand-searching techniques and snowballing methods to identify articles relevant to the research questions by reviewing reference lists of relevant articles that met the eligibility criteria. Following this, all the identified articles were collated in Endnote Reference Manager version X9.3 [ 67 ] and exported, after removing duplicates, into Distiller SR [ 68 ], a web-based systematic review and meta-analysis software. The syntax used on electronic databases and the University of Saskatchewan’s iPortal to identify potentially relevant articles for inclusion into this review study is outlined in Table 1 .
Selection of sources of evidence
Two iterative stages were employed to select sources of evidence for this review study. First, we created screening, coding, and data extraction forms using Distiller SR [ 68 ] for each stage. In the first stage, UO screened titles and abstracts of all articles using the following keywords: Indigenous youth; health; wellness; engagement and Canada. In the second stage, UO independently screened and reviewed the full-text articles (FTAs) of citations included from the first stage. The questions in Table 2 were used to screen the eligibility for inclusion of the article for data extraction. A second reviewer (ST) also independently reviewed and screened every 10th FTA citation from the first phase to check inter-rater reliability.
Data charting process and data items
Data were extracted using a pre-designed data extraction form on DistillerSR [ 68 ]. All extracted data were exported into Microsoft Excel [ 69 ] for data cleaning and analysis. The title fields used to extract data from included articles are shown in Table 3 .
Critical appraisal of individual sources of evidence
Conjointly, UO and CB appraised each article included considering characteristics and methodological quality using the JBI Critical Appraisal Tool for qualitative and quantitative studies [ 70 ]. The JBI Critical Appraisal Tool was designed to evaluate the rigour, trustworthiness, relevance, and potential for bias in study designs, conduct, and analysis [ 70 ]. Results on the critical appraisals are summarized in Supplementary material file 2 .
Synthesis of results
We categorized findings in this review as facilitators/strengths and barriers/roadblocks to enhancing wellness by, for, and with Indigenous youth, further describing how youth described wellness promotion. We met weekly via videoconference to discuss, review, and revisit our study evaluation protocol to ensure we adhered strictly to the scoping review guidelines.
As a result of our literature search, 1671 articles from five library databases and 24 articles through hand-search and snowball methods were identified. Of the 1695 articles, 253 were excluded as duplicates on EndNote vX9.3 using the ‘remove duplicates’ function on the software. Another 1227 articles were excluded following screening of title and abstracts on Distiller SR which we had fed with a series of screening questions (see Table 2 ) that were reviewed independently by two reviewers (UO and ST). Inter-rater reliability (Cohen’s kappa) calculated was 0.886, standard error = 0.147, p -value = 0.001. Where there were conflicts in article inclusion ratings, a third reviewer (CB), was brought in to discuss and provide a resolution. This left 215 articles for full-text article (FTA) screening. After reviewing 215 FTAs, a further 195 articles were excluded, leaving 20 articles for inclusion into the final review. Articles were excluded in the eligibility stage for the following reasons, 1) articles not focused on Indigenous youth or Indigenous communities, 2) articles not focused on Indigenous health and/or wellness, 3) articles not primarily focused in Canadian settings, 4) articles not written in English, 5) articles considered irrelevant or not applicable to addressing the research objectives or research questions of our study, 6) articles other than original research (i.e., we excluded review studies, opinion papers, and conference abstracts). A flowchart of article selection can be found in Fig. 1 .
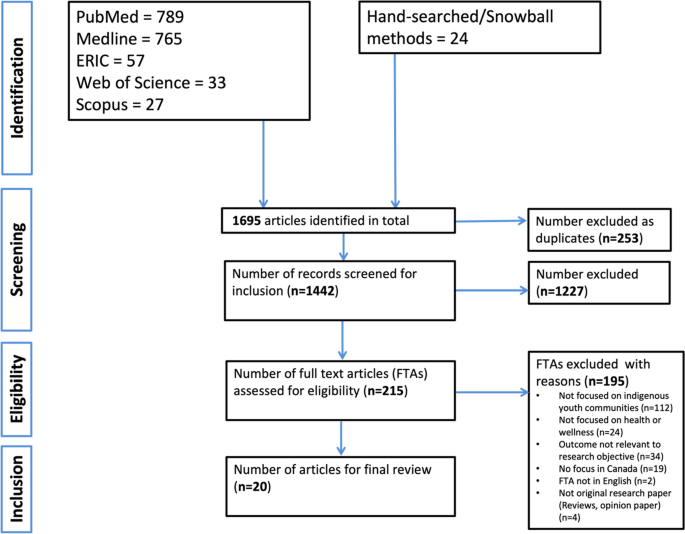
PRISMA flowchart showing selection of articles for scoping review
Characteristics of sources of evidence
The general and methodological characteristics of all 20 included articles are summarized in Table 4 . Of these, one study was published in 2017, two in 2018, eleven in 2019, four in 2020 and two in 2021. Five (25%) studies that were included were set in the province of Ontario, four (20%) in the province of Saskatchewan, three (15%) in the Northwest Territories and two in the province of Alberta. Fifty percent (10/20) of the studies recruited or focused on Indigenous (First Nations, Métis, and Inuit) people as study participants, seven (35%) studies recruited or concentrated on First Nations peoples only, and three (15%), on Inuit peoples only. Sixteen (80%) articles were qualitative studies, three (15%) used mixed methods, and one (5%) was a quantitative study. Eleven (55%) studies used participatory research approaches (which included photovoice, community-based participatory research (CBPR) or participatory action research (PAR)) in their study designs, seven (35%) integrated Indigenous research methods (e.g., the two-eyed seeing approach) into their study design, and five (25%) studies used descriptive or inferential evaluation strategies in their study design. Interviews, focus-group discussions, and discussion circles were the most common data collection methodology used in 17 (85%) of the studies included. Youth were commonly engaged in non-cultural activities in twelve (60%) of the studies and employed a youth-adult co-led strategy in 16 (80%) of the included studies.
Results of individual sources of evidence
All included studies provided answers relevant to one or more of the research questions with the potential for changing practice and strategies for engagement. All the included studies explored, investigated, or evaluated issues addressing health and wellness among Indigenous youth in Canada. The age range of youth involved in included studies ranged between 11 to 24 years. All studies utilized fun and interactive strategies to engage youth in their respective studies with the outcomes aimed at promoting health, developing capacity in youth participants and engaging youth in collaborating on sustainable outcomes for and with their communities [ 5 , 8 , 40 , 44 , 57 , 71 , 72 , 73 , 74 , 75 , 76 , 77 , 78 , 79 , 80 , 81 , 82 , 83 , 84 ], save for one [ 16 ]. The summary of individual sources of evidence is described in Table 5 .
The key facilitators/strengths and barriers/roadblocks to enhancing health and wellness by, for, and with Indigenous youth that emerged from the included studies are described in Table 6 , in descending order of major themes for the frequency of citation by included articles per theme. The facilitators/strengths and barriers/roadblocks have also been categorized into sub-themes under five major themes for facilitators/strengths and six major themes for barriers/roadblocks. Health outcomes/programs examined by included studies included suicide prevention [ 40 ], mental health promotion [ 71 , 74 ], HIV prevention [ 75 ], wellness promotion through youth empowerment and cultural activism [5, 8, 16, 57, 72,,76, 77, 78,79, 80], social health [ 76 , 83 ], land-based healing and wellness [ 77 , 82 ], art-media based therapy and wellness [ 44 , 73 , 81 , 84 ]. An overview of the facilitators/strengths and barriers/roadblocks to enhancing health and wellness by, for, and with Indigenous youth is presented in Fig. 2 .
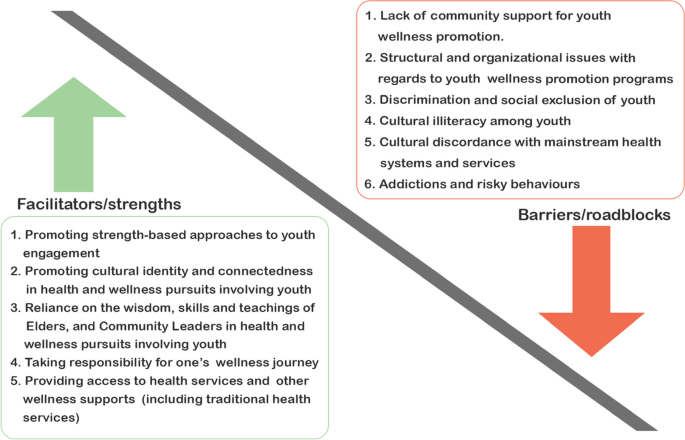
Summary of facilitators/strengths and barriers/roadblocks to enhancing wellness by, for and with Indigenous youth
Facilitators/strengths to enhancing health and wellness by, for, and with indigenous youth
Five major themes emerged and were identified as facilitators/strengths to enhancing health and wellness by, for, and with Indigenous youth in Canada. The most identified facilitator/strength of health and wellness among Indigenous youth in Canada, identified in 19 [95%] of the included studies, was the promotion of strength-based approaches to engaging with youth in the community [ 5 , 8 , 16 , 44 , 57 , 71 , 72 , 73 , 74 , 75 , 76 , 77 , 78 , 79 , 80 , 81 , 82 , 83 , 84 ]. A number of sub-themes also emerged from this major theme to include: peer-mentoring [ 5 , 8 , 44 , 57 , 71 , 73 , 74 , 75 , 76 , 79 , 80 , 81 , 82 , 83 , 84 ]; engaging youth in programs that developed and promoted self-determination, capacity building and empowerment [ 5 , 8 , 44 , 57 , 72 , 73 , 74 , 76 , 77 , 78 , 79 , 80 , 82 , 83 , 84 ]; building positive relationships and social connections with others, nature and the environment [ 5 , 8 , 44 , 57 , 72 , 73 , 76 , 77 , 79 , 80 , 81 , 82 , 83 , 84 ]; showing kindness to one another [ 5 , 16 , 44 , 57 , 77 , 79 , 80 , 81 , 83 ]; and engaging youth in cultural activities [ 57 , 76 , 82 , 83 ] that stimulate or encourage mutual learning, enhance critical consciousness and cause transformative change [ 5 , 8 , 75 , 76 , 79 , 81 ]. The next most common facilitator identified in 16 [80%] of included studies was enhancing cultural identity and connectedness through youth engagement in cultural activities [ 8 , 16 , 40 , 44 , 57 , 71 , 72 , 75 , 76 , 77 , 79 , 80 , 81 , 82 , 83 , 84 ]. Other facilitators included: reliance on the wisdom, skills, and teachings of community Elders, Traditional Knowledge Keepers and community leaders in the pursuit of health and wellness promotion with Indigenous youth [ 5 , 16 , 44 , 72 , 77 , 79 , 80 , 81 , 83 , 84 ]; taking responsibility for one’s journey to wellness [ 44 , 57 , 72 , 74 , 79 , 80 , 82 , 83 ]; and providing access to health services and other wellness supports (including traditional health services) for youth in Indigenous communities [ 76 , 78 ]. A summary of the facilitators/strengths is provided in Fig. 2 .
Barriers/roadblocks to enhancing health and wellness by, for, and with indigenous youth
Six major themes emerged and identified as barriers/roadblocks to enhancing health and wellness by, for and with Indigenous youth in Canada. The most identified barrier/roadblock to enhancing health and wellness identified in 55% (11/20) of the included articles was a lack of community support [including social, financial, and organizational support] for wellness promotion strategies among Indigenous youth [ 5 , 44 , 57 , 72 , 74 , 75 , 76 , 77 , 78 , 80 , 81 ]. Structural and organizational issues within Indigenous communities regarding wellness promotion strategies were identified as the second most common barrier/roadblock to enhancing wellness in 50% [10/20] of included studies [ 5 , 8 , 72 , 73 , 76 , 77 , 78 , 81 , 82 , 83 ]. These structural and organizational issues included: Indigenous community problems or concerns affecting the sustainability of instituted wellness programs/strategies [ 5 , 8 , 78 , 81 ]; dogmatism and debates about definitions regarding traditions of health among Indigenous communities [ 72 , 77 , 82 , 83 ]; social and structural instability within communities (e.g., leadership concerns) [ 8 , 76 , 83 ]; modest to low capacity of service providers (e.g. vendors, health service centers, social service centers, etc.) to meet the demands of communities [ 73 , 78 , 81 ]; and the misperception of a lack of control for self-governance in Indigenous communities [ 81 ]. Discrimination and social exclusion of Indigenous youth were also identified as a barrier/roadblock to enhancing wellness in eight (40%) studies included [ 5 , 8 , 44 , 57 , 74 , 76 , 80 , 83 ]. Forms of discrimination and social exclusion identified as subthemes included: Racism (e.g., personal, interpersonal, structural and systemic racism) [ 5 , 8 , 76 , 80 , 83 ]; low self-esteem and a low view of self-identity leading to self-deprecation and self-exclusion from engaging in youth activities [ 8 , 44 , 76 , 80 , 83 ]; mental health stigmatization [ 73 , 74 , 76 ]; lack of inclusivity of traditional Indigenous activities into Canadian teaching institutions [ 76 , 77 ]; and all forms of bullying, abuse and hunger [ 57 , 80 ]. Other barriers/roadblocks included: cultural illiteracy among Indigenous youth [ 44 , 57 , 73 , 74 , 75 , 83 , 84 ]; friction between Western and Traditional methods of promoting health and wellness [ 5 , 74 , 76 , 77 ]; and risky behaviours such as gang activity, substance use/abuse and addictions [ 44 , 57 , 75 , 76 , 80 ]. A summary of the barriers/roadblocks is provided in Fig. 2 .
Scoping reviews determine the extent, range, and quality of evidence on any chosen topic [ 60 , 61 , 62 , 63 ]. In addition, they can be used to map and describe what is known about an identified topic to identify existing gaps in the literature regarding the chosen topic [ 60 , 61 , 62 , 63 ]. In this scoping review, the peer-reviewed evidence regarding facilitators/strengths and barriers/roadblocks to enhancing health and wellness by, for and with Indigenous youth in Canada were mapped and synthesized. Key facilitators/strengths highlighted included: promoting culturally appropriate interventions [ 8 , 16 , 40 , 44 , 57 , 71 , 72 , 75 , 76 , 77 , 79 , 80 , 81 , 82 , 83 , 84 ] using strength-based approaches [ 5 , 8 , 16 , 44 , 57 , 71 , 72 , 73 , 74 , 75 , 76 , 77 , 78 , 79 , 80 , 81 , 82 , 83 , 84 ]. Key barriers to enhancing health and wellness by, for and with Indigenous youth identified in this review were the lack of community support for wellness promotion activities among Indigenous youth [ 5 , 44 , 57 , 72 , 74 , 75 , 76 , 77 , 78 , 80 , 81 ] and structural/organizational issues within Indigenous communities [ 5 , 8 , 72 , 73 , 76 , 77 , 78 , 81 , 82 , 83 ].
Strength-based approaches empower community members, academic researchers, and policymakers to effect community change while focusing on what has worked in the past and the community vision for success in the future [ 79 ]. This is contrasted with the common narrative in most studies exploring Indigenous health and wellness that focused on why and where the community has failed to thrive [ 79 ]. Promoting strength-based interventions by, for, and with Indigenous youth works in parallel with ensuring that health interventions are culturally appropriate [ 44 , 79 ] because Indigenous epistemologies or ways of knowing see reality as intricate processes of interdependent relationships between humans, nature, and the spirit world [ 44 , 77 ]. As such, wellness promotion in Indigenous communities should emphasize support for their traditional values such as respect, trust, non-judgement, and relationality, all of which support cultural revitalization [ 26 , 71 ].
Conversely, wellness promotion in Indigenous communities should disavow the use of Western-based epistemologies that embrace and emphasize control over risk factors and health [ 44 , 79 ]. The definition and perception of health and wellness by Indigenous peoples are starkly different from the Western perspective of health promotion [ 44 , 79 ] which was found in our study to be a barrier/roadblock to enhancing health and wellness by, for and with Indigenous youth [ 8 , 43 , 44 , 76 ]. Because of these contrasting and conflicting views on health and wellness, research carried out with Indigenous communities must be grounded in their culture. Elder Jim Dumont – a professor of Native Studies and a member of the Shawanaga First Nation on Eastern Georgian Bay, when describing the role of Indigenous culture in facilitating wellness among Indigenous peoples, defined Indigenous culture as a “ facilitator to spiritual expression” [ 85 p.11]. He described Indigenous culture as “an expression of the life-ways, the spiritual, psychological, social, and material practice of the Indigenous worldview, which attends to the whole person’s spiritual desire to live life to the fullest” [ 85 p.9]. This was the way of life for Indigenous peoples before colonization [ 2 ]. Back then, Indigenous peoples honoured and utilized traditional methods and practices connected to their respective unceded homelands to promote and sustain health and wellness by themselves within their respective communities [ 2 , 16 , 86 ]. These cultural practices provided and promoted health and wellness for the community, the peoples, the lands, and the environment [ 2 ].
Furthermore, Indigenous wellness promotion by, for and with Indigenous youth should go beyond making mainstream health promotion strategies more culturally appropriate. Indigenous wellness promotion should also invite youth as partners and co-researchers to authentically engage with the community, acknowledging their needs while working together with them to identify opportunities for change (which should include shared power and responsibilities in the relationship dynamic). This must be the fundamental principle for any work done by, for, or with Indigenous communities (i.e., authentic engagement) [ 54 , 55 , 59 ]. Authentic engagement is working and walking with rather than on communities [ 54 ] in a way that encourages respectful, compassionate, and genuine interest in the work undertaken by all partners involved [ 54 , 55 , 57 , 87 , 88 ]. In authentically engaging with Indigenous communities, emphasis should be placed on connecting with , rather than controlling, community members [ 44 , 89 ]. By doing so, enhances a community’s ability to answer their issues by identifying their community strengths and assets, considering opportunities for change, and co-creating meaningful solutions to mitigate them.
The Tri-Council Policy Statement (TCPS) on Ethical Conduct for Research involving Humans indicates in Chapter 9 that, where research involves First Nations, Métis, and Inuit peoples and their communities, they are to have a role in shaping and co-creating research that affects them; with respect being given to the autonomy of these communities and the individuals within them to decide to participate [ 90 ]. Our study showed that where youth were engaged as partners and co-researchers, promoted self-determination, capacity building and ultimately enhanced wellness [ 8 , 40 , 44 , 57 , 72 , 74 , 75 , 76 , 77 , 79 , 84 ].
From the outcomes of this review, youth were engaged as partners or co-researcher in 55% of the included articles using research approaches such as community-based participatory research [CBPR], photovoice, visual voice, participatory videography, performative arts, participatory narrative, and storytelling methods [ 8 , 40 , 44 , 57 , 72 , 74 , 75 , 76 , 77 , 79 , 84 ]. This review demonstrated that these methods helped foster an environment for transformative learning, reciprocal transfer of expertise, shared decision-making, and co-ownership of the research processes [ 8 , 40 , 44 , 57 , 72 , 74 , 75 , 76 , 77 , 79 , 84 ]. For example, Goodman et al. identified that through photovoice, youth identified how racism negatively influenced the types of social supports and relationships formed in their community, leading to improved access to mental health-promoting social programs [ 76 ]. Anang et al. reported that engaging Indigenous youth as co-researchers in exploring ways to promote suicide prevention revitalized awareness of their cultural identity, which was identified as a protective factor to youth suicide [ 40 ]. A group of First Nation girls involved in the Girl Power Program designed to build and foster empowerment using youth participatory action research approach indicated that working as co-researchers/co-creators in the program empowered them to find healing from wounded spirits, which helped enhance positive changes towards wellness through āhkamēyimowin (perseverance) [ 57 ]. Thus, we can conclude from our study that engaging youth as partners in research processes optimizes their personal experiences and gives them a voice which can stimulate action.
Engaging Indigenous youth in the co-creation of wellness strategies should also involve community Elders, Traditional Knowledge Keepers, and other Indigenous community leaders. This review demonstrated that reliance on the wisdom of Elders, Traditional Knowledge Keepers and Indigenous community leaders facilitated and enhanced wellness among Indigenous youth [ 5 , 16 , 44 , 72 , 77 , 79 , 80 , 81 , 83 , 84 , 91 ]. Elders, Traditional Knowledge Keepers, and Indigenous community leaders play a central role in increasing awareness related to the community’s histories, languages, knowledge, and ways of knowing [ 91 , 92 ]. For non-Indigenous researchers and allies, Elders and Traditional Knowledge Keepers can provide formal and informal teachings on: histories of the Indigenous community in question, their world views, languages in the community, arts, crafts and songs, value systems in the nation/community; knowledge of traditional plants and medicines; clan teachings in the nation/community; ceremonial knowledge or protocols; and understanding of wellness in the community that can increase cultural awareness and build Indigenous research competencies for non-Indigenous researchers and allies [ 91 , 92 , 93 ]. Hence, engaging Elders, Knowledge Keepers and Indigenous community leaders in youth wellness programs can provide an avenue for mutual learning, guiding non-Indigenous researchers/allies towards cultural appropriateness in co-developing youth-driven wellness strategies.
Practical implications
Overall, this review emphasized the importance of promoting wellness among Indigenous youth using ‘ culture as strength ’ rather than imposing control measures on Indigenous values. The historical experiences of Indigenous youth have revealed traumatic and distressful pasts propagated by the cumulative intergenerational impacts of colonization which evolved from Residential Schools, Day Schools, and the Sixties Scoop [ 15 , 16 , 33 , 94 , 95 ]. The 2015 Truth and Reconciliation Commission of Canada’s 96 Calls-to-Action stressed the need to decolonize mainstream health promotion strategies and embrace the promotion of self-determination in the use of and access to traditional knowledge, therapies, and healing practices Indigenous peoples [ 95 , 96 ]. This review provided a foundation for authentically engaging Indigenous youth in the co-creation of culturally appropriate wellness promotion strategies/programs driven and sustained by authentically engaged Indigenous youth in the community. Considering the number of qualitative studies we found in our review, a meta-synthesis of qualitative studies may guide future directions based on the findings in our study to further pursue to understand, appraise, summarize, and combine qualitative evidence to address the specific research questions particularly around the influences and experiences of cultural connectedness and wellness among Indigenous youth in Canada. Nonetheless, this review also contributes to the growing literature identifying strength-based approaches to enhancing health and wellness among Indigenous peoples in Canada.
Study limitations
This review aimed to provide an entire scope of all original studies published in peer-reviewed journals to allow for as broad a scope of literature synthesis as possible. However, this study is not without limitations. First, the search was limited to multiple library databases, including the University of Saskatchewan’s Indigenous Studies Portal (iPortal) [ 66 ]. Although this review produced many peer-reviewed and original studies, there is a potential that other relevant articles and reports were missed because we did not search the grey literature. Secondly, because this review was limited to peer-reviewed articles published in English, it is possible that potentially relevant studies in other languages were omitted. Moreover, the outcomes of this review are limited to the nature of the data reported in the articles included in the review. Additionally, we acknowledge the differences and nuances in Indigenous practices, values and culture which limits the generalizability of our review findings. Lastly, some of the studies in the scoping review utilized Indigenous study designs and methods that could not be appropriately evaluated using the JBI Critical Appraisal Tools [ 70 ].
This scoping review identified ways health and wellness can be enhanced by, for, and with Indigenous youth by identifying facilitators/strengths and barriers/roadblocks to enhancing health and wellness among Indigenous youth from identified studies published between January 1, 2017, and May 22, 2021. The outcomes of this review showed that promoting culturally based and appropriate interventions using strength-based approaches were key facilitators/strengths to enhancing health and wellness among Indigenous youth. Thus, the outcomes demonstrate the continued need to promote programs grounded in culture as a part of enhancing health and wellness while authentically engaging Indigenous youth in health and wellness strategies, interventions, and programs.
Availability of data and materials
Data sharing is not applicable to this article as no datasets were generated or analyzed during the current study.
Abbreviations
Community-based participatory research
First Nations
Full-Text Articles
University of Saskatchewan’s Indigenous Studies Portal
Joanna Briggs Institute
Medical Subject Headings
Participatory action research
Preferred Reporting Items for Systematic Reviews and Meta Analyses
Preferred Reporting Items for Systematic Reviews and Meta-Analyses extension for Scoping Reviews
United Nations Department of Economic and Social Affairs (UNDESA). Who are Indigenous peoples. 2020. Available from: https://www.un.org/esa/socdev/unpfii/documents/5session_factsheet1.pdf . Assessed 02 Feb 2022.
Google Scholar
Government of Canada. Indigenous peoples and communities. 2021. Available from: https://www.rcaanc-cirnac.gc.ca/eng/1100100013785/1529102490303 . Assessed 02 Feb 2022.
Allen L, Hatala A, Ijaz S, Courchene ED, Bushie EB. Indigenous-led health care partnerships in Canada. CMAJ. 2020;192(9):E208–16. https://doi.org/10.1503/cmaj.190728 .
Article PubMed PubMed Central Google Scholar
Latimer M, Sylliboy JR, MacLeod E, Rudderham S, Francis J, Hutt-MacLeod D, et al. Creating a safe space for first nations youth to share their pain. Pain Rep. 2018;3(Suppl 1):e682. https://doi.org/10.1097/PR9.0000000000000682 .
Lopresti S, Willows ND, Storey KE, McHugh TF. Indigenous youth mentorship program: key implementation characteristics of a school peer mentorship program in Canada. Health Promot Int. 2021;36(4):913–23. https://doi.org/10.1093/heapro/daaa090 .
Article PubMed Google Scholar
Statistics Canada. Projections of the Indigenous populations and households in Canada, 2016 to 2041: overview of data sources, methods, assumptions and scenarios. 2021. Ottawa, ON. Cat. No. 17-2-0001. Available from: https://www150.statcan.gc.ca/n1/pub/17-20-0001/172000012021001-eng.htm . Assessed 02 Feb 2022.
Statistics Canada. Indigenous population in Canada – Projections to 2041. 2021. Ottawa, ON. Cat. No. 17-2-0001. Available from: https://www150.statcan.gc.ca/n1/pub/11-627-m/11-627-m2021066-eng.htm . Assessed 02 Feb 2022.
Merati N, Salsberg J, Saganash J, Iserhoff J, Moses KH, Law S. Cree youth engagement in health planning. Int J Indig Health. 2020;15(1):73–89. https://doi.org/10.32799/ijih.v15i1.33985 .
Article Google Scholar
Adelson N. The embodiment of inequity: health disparities in Aboriginal Canada. Can J Public Health. 2005;96(Suppl 2):S45–61. https://doi.org/10.1007/BF03403702 .
National Collaborating Centre for Indigenous Health (NCCIH). An overview of Aboriginal health in Canada. 2012. Available from: http://www.nccah-ccnsa.ca/Publications/Lists/Publications/Attachments/101/abororiginal_health_web.pdf . Assessed 02 Feb 2022.
Lemstra M, Neudorf C. Health disparity in Saskatoon: analysis to intervention summary. 2008. Available from: https://www.alliancehealth.ca/wp-content/uploads/2019/06/HD-Summary.pdf . Assessed 02 Feb 2022.
Kirmayer LJ, Brass G. Addressing global health disparities among indigenous peoples. Lancet. 2016;388(10040):105–6. https://doi.org/10.1016/s0140-6736(16)30194-5 .
Kirmayer LJ, Gone JP, Moses J. Rethinking historical trauma. Transcult Psychiatry. 2014;51(3):299–319. https://doi.org/10.1177/1363461514536358 .
Phillips-Beck W, Sinclair S, Campbell R, Star L, Cidro J, Wicklow B, et al. Early-life origins of disparities in chronic diseases among indigenous youth: pathways to recovering health disparities from intergenerational trauma. J Dev Orig Health Dis. 2019;10(1):115–22. https://doi.org/10.1017/S2040174418000661 .
Article CAS PubMed Google Scholar
Barker B, Sedgemore K, Tourangeau M, Lagimodiere L, Milloy J, Dong H, et al. Intergenerational trauma: the relationship between residential schools and the child welfare system among young people who use drugs in Vancouver, Canada. J Adolesc Health. 2019;65(2):248–54. https://doi.org/10.1016/j.jadohealth.2019.01.022 .
Gray AP, Cote W. Cultural connectedness protects mental health against the effect of historical trauma among Anishinabe young adults. Public Health. 2019;176:77–81. https://doi.org/10.1016/j.puhe.2018.12.003 .
Wilk P, Maltby A, Cooke M, Forsyth J. The effect of parental residential school attendance and parental involvement on indigenous youth's participation in sport and physical activity during school. Int J Indig Health. 2019;14(2):133–49. https://doi.org/10.32799/ijih.v14i2.31929 .
Frohlich KL, Ross N, Richmond C. Health disparities in Canada today: some evidence and a theoretical framework. Health Policy. 2006;79(2–3):132–43. https://doi.org/10.1016/j.healthpol.2005.12.010 .
Greenwood ML, de Leeuw SN. Social determinants of health and the future well-being of Aboriginal children in Canada. Paediatr Child Health. 2012;17(7):381–4. https://doi.org/10.1093/pch/17.7.381 .
Kim PJ. Social determinants of health inequities in indigenous Canadians through a life course approach to colonialism and the residential school system. Health Equity. 2019;3(1):378–81. https://doi.org/10.1089/heq.2019.0041 .
King J, Masotti P, Dennem J, Hadani S, Linton J, Lockhart B, et al. The culture is prevention project: adapting the cultural connectedness scale for multi-tribal communities. Am Indian Alsk Native Ment Health Res. 2019;26(3):104–35. https://doi.org/10.5820/aian.2603.2019.104 .
Smylie J, O'Brien K, Beaudoin E, Daoud N, Bourgeois C, George EH, et al. Long-distance travel for birthing among indigenous and non-indigenous pregnant people in Canada. CMAJ. 2021;193(25):E948–e55. https://doi.org/10.1503/cmaj.201903 .
Pollock NJ, Healey GK, Jong M, Valcour JE, Mulay S. Tracking progress in suicide prevention in indigenous communities: a challenge for public health surveillance in Canada. BMC Public Health. 2018;18(1):1320. https://doi.org/10.1186/s12889-018-6224-9 .
Pollock NJ, Mulay S, Valcour J, Jong M. Suicide rates in Aboriginal communities in Labrador, Canada. Am J Public Health. 2016;106(7):1309–15. https://doi.org/10.2105/AJPH.2016.303151 .
Lucente G, Kurzawa J, Danseco E. Moving towards racial equity in the child and youth mental health sector in Ontario, Canada. Adm Policy Ment Health. 2021:1–4. https://doi.org/10.1007/s10488-021-01153-3 .
Sasakamoose J, Scerbe A, Wenaus I, Scandrett A. First Nation and Métis youth perspectives of health. Qual Inq. 2016;22(8):636–50. https://doi.org/10.1177/1077800416629695 .
Wood L, Kamper D, Swanson K. Spaces of hope? Youth perspectives on health and wellness in indigenous communities. Health Place. 2018;50:137–45. https://doi.org/10.1016/j.healthplace.2018.01.010 .
Bauer GR, Mahendran M, Braimoh J, Alam S, Churchill S. Identifying visible minorities or racialized persons on surveys: can we just ask? Can J Public Health. 2020;111(3):371–82. https://doi.org/10.17269/s41997-020-00325-2 .
Janzen B, Karunanayake C, Rennie D, Katapally T, Dyck R, McMullin K, et al. Racial discrimination and depression among on-reserve first nations people in rural Saskatchewan. Can J Public Health. 2018;108(5–6):e482–e7. https://doi.org/10.17269/cjph.108.6151 .
Kirmayer LJ, Brass GM, Tait CL. The mental health of Aboriginal peoples: transformations of identity and community. Can J Psychiatr. 2000;45(7):607–16. https://doi.org/10.1177/070674370004500702 .
Article CAS Google Scholar
Victor J, Linds W, Episkenew J-A, Goulet L, Benjoe D, Brass D, et al. Kiskenimisowin (self-knowledge): co-researching wellbeing with Canadian first nations youth through participatory visual methods. Int J Indig Health. 2016;11(1):262–78. https://doi.org/10.18357/ijih111201616020 .
Ansloos JP, Wager AC. Surviving in the cracks: a qualitative study with indigenous youth on homelessness and applied community theatre. Int J Qual Stud Educ. 2019;33(1):50–65. https://doi.org/10.1080/09518398.2019.1678785 .
Bombay A, McQuaid RJ, Schwartz F, Thomas A, Anisman H, Matheson K. Suicidal thoughts and attempts in first nations communities: links to parental Indian residential school attendance across development. J Dev Orig Health Dis. 2019;10(1):123–31. https://doi.org/10.1017/S2040174418000405 .
Gabel C, Pace J, Ryan C. Using photovoice to understand intergenerational influences on health and well-being in a southern Labrador Inuit community. Int J Indig Health. 2016;11(1):75–91. https://doi.org/10.18357/ijih111201616014 .
Grande AJ, Elia C, Peixoto C, Jardim PTC, Dazzan P, Veras AB, et al. Mental health interventions for suicide prevention among indigenous adolescents: a systematic review protocol. BMJ Open. 2020;10(5):e034055. https://doi.org/10.1136/bmjopen-2019-034055 .
Hartshorn KJ, Whitbeck LB, Prentice P. Substance use disorders, comorbidity, and arrest among indigenous adolescents. Crime Delinq. 2015;61(10):1311–32. https://doi.org/10.1177/0011128712466372 .
Boksa P, Hutt-MacLeod D, Clair L, Brass G, Bighead S, MacKinnon A, et al. Demographic and clinical presentations of youth using enhanced mental health services in six indigenous communities from the ACCESS open minds network. Can J Psychiatr. 2022;67(3):179–91. https://doi.org/10.1177/07067437211055416 .
Abraham ZK, Sher L. Adolescent suicide as a global public health issue. Int J Adolesc Med Health. 2017;31(4):20170036. https://doi.org/10.1515/ijamh-2017-0036 .
Ansloos J. Rethinking indigenous suicide. Int J Indig Health. 2018;13(2):8–28. https://doi.org/10.18357/ijih.v13i2.32061 .
Anang P, Naujaat Elder EH, Gordon E, Gottlieb N, Bronson M. Building on strengths in Naujaat: the process of engaging Inuit youth in suicide prevention. Int J Circumpolar Health. 2019;78(2):1508321. https://doi.org/10.1080/22423982.2018.1508321 .
Barker B, Goodman A, DeBeck K. Reclaiming indigenous identities: culture as strength against suicide among indigenous youth in Canada. Can J Public Health. 2017;108(2):e208–e10. https://doi.org/10.17269/cjph.108.5754 .
Lemstra M, Rogers M, Moraros J, Grant E. Risk indicators of suicide ideation among on-reserve first nations youth. Paediatr Child Health. 2013;18(1):15–20. https://doi.org/10.1093/pch/18.1.15 .
Plazas PC, Cameron BL, Milford K, Hunt LR, Bourque-Bearskin L, Santos SA. Engaging indigenous youth through popular theatre: knowledge mobilization of indigenous peoples’ perspectives on access to healthcare services. Action Res. 2019;17(4):492–509. https://doi.org/10.1177/1476750318789468 .
Sanchez-Pimienta CE, Masuda J, M'Wikwedong indigenous friendship C. From controlling to connecting: M'Wikwedong as a place of urban indigenous health promotion in Canada. Health Promot Int. 2021;36(3):703–13. https://doi.org/10.1093/heapro/daaa066 .
Leonardi F. The definition of health: towards new perspectives. Int J Health Serv. 2018;48(4):735–48. https://doi.org/10.1177/0020731418782653 .
Snowshoe A, Crooks CV, Tremblay PF, Hinson RE. Cultural connectedness and its relation to mental wellness for first nations youth. J Prim Prev. 2017;38(1–2):67–86. https://doi.org/10.1007/s10935-016-0454-3 .
Auger M, Howell T, Gomes T. Moving toward holistic wellness, empowerment and self-determination for indigenous peoples in Canada: can traditional indigenous health care practices increase ownership over health and health care decisions? Can J Public Health. 2016;107(4–5):e393–e8. https://doi.org/10.17269/cjph.107.5366 .
Brady M. Cultural considerations in play therapy with Aboriginal children in Canada. First Peoples Child Fam Rev. 2015;10(2):95–109.
Rabbitskin N, Ermine, W, Walsh, K, Gilbert, L; in Interagency Coalition on AIDS and Development publications. Case Study: Sturgeon Lake traditional health program. 2010. Available from: http://www.icad-cisd.com/our-resources/icad-publications-and-resources/case-study-sturgeon-lake-traditional-health-program/ . Assessed 23 Sept 2021.
Sturgeon Lake First Nation. Sturgeon Lake First Nation wholistic influenza pandemic planning. 2007. Available from: https://fnpa.ca/wp-content/uploads/2020/03/Sturgeon-Lake-First-Nation-Wholistic-Influenza-Plan.pdf . Assessed 23 Sept 2021.
Sturgeon Lake First Nation. Sturgeon Lake First Nation of Saskatchewan. Available from: http://www.slfn.ca/ . Accessed 23 Sept 2021.
Government of Canada. Sturgeon Lake First Nation-connectivity profile. 2020. Available from: https://www.aadnc-aandc.gc.ca/eng/1357840942279/1360166692704 . Assessed 23 Sept 2021.
Gaspar C. Āhkamēyimowin: walking together [Dissertation, University of Saskatchewan]. 2019. Available from: https://core.ac.uk/download/pdf/226154411.pdf . Assessed 23 Sept 2021.
Ramsden VR, Salsberg J, Herbert CP, Westfall JM, LeMaster J, Macaulay AC. Patient- and community-oriented research: how is authentic engagement identified in grant applications? Can Fam Physician. 2017;63(1):74–6.
PubMed PubMed Central Google Scholar
Ramsden VR, Rabbitskin N, Westfall JM, Felzien M, Braden J, Sand J. Is knowledge translation without patient or community engagement flawed? Fam Pract. 2017;34(3):259–61. https://doi.org/10.1093/fampra/cmw114 .
Woolf SH, Zimmerman E, Haley A, Krist AH. Authentic engagement of patients and communities can transform research, practice, and policy. Health Aff (Millwood). 2016;35(4):590–4. https://doi.org/10.1377/hlthaff.2015.1512 .
Gaspar C, Sundown S, Kingfisher S, Thornton R, Bighead S, Girl Power program participants of Sturgeon Lake First Nation, et al. āhkamēyimowin (perseverance): walking together: codesigned research project resulted in empowering first nations girls. Can Fam Physician. 2019;65(12):930–2.
Wu HC, Kornbluh M, Weiss J, Roddy L. Measuring and understanding authentic youth engagement: the youth-adult partnership rubric. Afterschool Matters. 2016;23:8–17.
Allen ML, Salsberg J, Knot M, LeMaster JW, Felzien M, Westfall JM, et al. Engaging with communities, engaging with patients: amendment to the NAPCRG 1998 policy statement on responsible research with communities. Fam Pract. 2017;34(3):313–21. https://doi.org/10.1093/fampra/cmw074 .
Levac D, Colquhoun H, O'Brien KK. Scoping studies: advancing the methodology. Implement Sci. 2010;5:69. https://doi.org/10.1186/1748-5908-5-69 .
Peters MD, Godfrey CM, Khalil H, McInerney P, Parker D, Soares CB. Guidance for conducting systematic scoping reviews. Int J Evid Based Healthc. 2015;13(3):141–6. https://doi.org/10.1097/xeb.0000000000000050 .
Arksey H, O'Malley L. Scoping studies: towards a methodological framework. Int J Soc Res Methodol. 2005;8(1):19–32. https://doi.org/10.1080/1364557032000119616 .
Tricco AC, Lillie E, Zarin W, O'Brien KK, Colquhoun H, Levac D, et al. PRISMA extension for scoping reviews (PRISMA-ScR): checklist and explanation. Ann Intern Med. 2018;169(7):467–73. https://doi.org/10.7326/m18-0850 .
Sawyer SM, Azzopardi PS, Wickremarathne D, Patton GC. The age of adolescence. Lancet Child Adolesc Health. 2018;2(3):223–8. https://doi.org/10.1016/s2352-4642(18)30022-1 .
Government of Canada. Canada’s first state of youth report: for youth, with youth, by youth. Ottawa: Canadian Heritage; 2021. Available from: https://www.canada.ca/en/canadian-heritage/campaigns/state-youth/report.html . Assessed 02 Feb 2022
University of Saskatchewan. University of Saskatchewan Indigenous studies portal research tool. Available at: https://iportal.usask.ca/ . Assessed 23 Sept 2021.
Clarivate Analytics. MacOS Endnote X9 version 9.2. [commercial computer software]. New York: Thomson Reuters Corporation; 2019.
Evidence Partners Incorporated. Distiller SR. Systematic Review and Literature Review Software. Ottawa: Evidence Partners Incorporated; 2021.
Microsoft Corporation. Microsoft Excel version 16.32. [commercial computer software]. New York: Microsoft; 2016.
Joanna Briggs Institute. Joanna Briggs Institute critical appraisal tools. 2014. Available from: https://joannabriggs.org/ebp/critical_appraisal_tools . Accessed 23 May 2021.
Crooks CV, Exner-Cortens D, Burm S, Lapointe A, Chiodo D. Two years of relationship-focused mentoring for first nations, Metis, and Inuit adolescents: promoting positive mental health. J Prim Prev. 2017;38(1–2):87–104. https://doi.org/10.1007/s10935-016-0457-0 .
Gaudet JC, Chilton C. Milo Pimatisiwin project: healthy living for Mushkegowuk youth. Int J Indig Health. 2018;13(1):20–40. https://doi.org/10.18357/ijih.v13i1.30264 .
Lys C, Gesink D, Strike C, Larkin J. Body mapping as a youth sexual health intervention and data collection tool. Qual Health Res. 2018;28(7):1185–98. https://doi.org/10.1177/1049732317750862 .
Etter M, Goose A, Nossal M, Chishom-Nelson J, Heck C, Joober R, et al. Improving youth mental wellness services in an indigenous context in Ulukhaktok, Northwest Territories: ACCESS open minds project. Early Interv Psychiatry. 2019;13(Suppl 1):35–41. https://doi.org/10.1111/eip.12816 .
Flicker S, Wilson C, Monchalin R, Oliver V, Prentice T, Jackson R, et al. “Stay strong, stay sexy, stay native”: storying indigenous youth HIV prevention activism. Action Res. 2017;17(3):323–43. https://doi.org/10.1177/1476750317721302 .
Goodman A, Snyder M, Wilson K, Whitford J. Healthy spaces: exploring urban indigenous youth perspectives of social support and health using photovoice. Health Place. 2019;56:34–42. https://doi.org/10.1016/j.healthplace.2019.01.004 .
Hatala AR, Morton D, Njeze C, Bird-Naytowhow K, Pearl T. Re-imagining miyo-wicehtowin: human-nature relations, land-making, and wellness among indigenous youth in a Canadian urban context. Soc Sci Med. 2019;230:122–30. https://doi.org/10.1016/j.socscimed.2019.04.012 .
Hutt-MacLeod D, Rudderham H, Sylliboy A, Sylliboy-Denny M, Liebenberg L, Denny JF, et al. Eskasoni first Nation's transformation of youth mental healthcare: partnership between a Mi'kmaq community and the ACCESS open minds research project in implementing innovative practice and service evaluation. Early Interv Psychiatry. 2019;13(Suppl 1):42–7. https://doi.org/10.1111/eip.12817 .
Lines LA, Yellowknives Dene First Nation Wellness Division, Jardine CG. Connection to the land as a youth-identified social determinant of indigenous peoples’ health. BMC Public Health. 2019;19(1):176. https://doi.org/10.1186/s12889-018-6383-8 .
Loebach J, Tilleczek K, Chaisson B, Sharp B. Keyboard warriors? Visualising technology and well-being with, for and by indigenous youth through digital stories. Vis Stud. 2019;34(3):281–97. https://doi.org/10.1080/1472586x.2019.1691050 .
Camargo Plazas P, Cameron BL, Milford K, Hunt LR, Bourque-Bearskin L, Santos SA. Engaging indigenous youth through popular theatre: knowledge mobilization of indigenous peoples’ perspectives on access to healthcare services. Action Res. 2018;17(4):492–509. https://doi.org/10.1177/1476750318789468 .
Hatala AR, Bird-Naytowhow K. Performing pimatisiwin: the expression of indigenous wellness identities through community-based theater. Med Anthropol Q. 2020;34(2):243–67. https://doi.org/10.1111/maq.12575 .
Njeze C, Bird-Naytowhow K, Pearl T, Hatala AR. Intersectionality of resilience: a strengths-based case study approach with indigenous youth in an urban Canadian context. Qual Health Res. 2020;30(13):2001–18. https://doi.org/10.1177/1049732320940702 .
Saini M, Roche S, Papadopoulos A, Markwick N, Shiwak I, Flowers C, et al. Promoting Inuit health through a participatory whiteboard video. Can J Public Health. 2020;111(1):50–9. https://doi.org/10.17269/s41997-019-00189-1 .
Canadian Institutes of Health Research (CIHR). Elder Jim Dumont, National Native Addictions Partnership Foundation in: Honouring our strengths; Indigenous culture as intervention in addictions treatment project - University of Saskatchewan. 2014. Available from: https://cyfn.ca/wp-content/uploads/2016/10/Honouring-our-strengths-Culture-as-treatment-resource-guide.pdf . Assessed 23 Sept 2021.
Fletcher S, Mullett J. Digital stories as a tool for health promotion and youth engagement. Can J Public Health. 2016;107(2):e183–e7. https://doi.org/10.17269/cjph.107.5266 .
Ramsden VR. Integrated primary health services model research team. Learning with the community. Evolution to transformative action research. Can Fam Physician. 2003;49(2):195–7 200–2.
CAS PubMed PubMed Central Google Scholar
Ramsden VR, McKay S, Crowe J. The pursuit of excellence: engaging the community in participatory health research. Glob Health Promot. 2010;17(4):32–42. https://doi.org/10.1177/1757975910383929 .
Ramsden VR, Cave AJ. Participatory methods to facilitate research. Can Fam Physician. 2002;48(3):548–9 553–4.
Canadian Institutes of Health Research (CIHR), Natural Sciences and Engineering Research Council of Canada (NSERC), Social Sciences and Humanities Research Council of Canada (SSHRC). Tri-Council policy statement. Ethical conduct for research involving humans. Ottawa: Government of Canada; 2018. Available from: https://ethics.gc.ca/eng/documents/tcps2-2018-en-interactive-final.pdf . Assessed 17 Jan 2022
Kanewiyakiho ED, Sawchuk KF, Ramsden VR. Ask before you ask: co-developing meaningful research questions with indigenous elders. Can Fam Physician. 2021;67(12):947–8. https://doi.org/10.46747/cfp.6712947 .
Flicker S, O'Campo P, Monchalin R, Thistle J, Worthington C, Masching R, et al. Research done in “a good way”: the importance of indigenous Elder involvement in HIV community-based research. Am J Public Health. 2015;105(6):1149–54. https://doi.org/10.2105/AJPH.2014.302522 .
Rowe G, Straka S, Hart M, Callahan A, Robinson D, Robson G. Prioritizing indigenous elders’ knowledge for intergenerational well-being. Can J Aging. 2020;39(2):156–68.
Cooke MJ, Wilk P, Paul KW, Gonneville SL. Predictors of obesity among Métis children: socio-economic, behavioural and cultural factors. Can J Public Health. 2013;104(4):e298–303. https://doi.org/10.17269/cjph.104.3765 .
Truth and Reconciliation Commission of Canada (TRC). Honoring the truth, reconciling for the future: Summary of the Final Report of the Truth and Reconciliation Commission of Canada. 2015. Available from: https://ehprnh2mwo3.exactdn.com/wp-content/uploads/2021/01/Executive_Summary_English_Web.pdf . Assessed 23 Sept 2021.
Truth and Reconciliation Commission of Canada (TRC). Truth and Reconciliation Commission of Canada: Calls to Action. 2015. Available from: https://www2.gov.bc.ca/assets/gov/british-columbians-our-governments/indigenous-people/aboriginal-peoples-documents/calls_to_action_english2.pdf . Assessed 23 Sept 2021.
Download references
Acknowledgements
Not applicable.
Funding provided by the Saskatchewan Health Research Foundation (SHRF) and the Canadian Institute of Health Research (CIHR)/Saskatchewan Center for Patient-Oriented Research (SCPOR) as part of the SHRF Leader Award held by Dr. Ramsden.
Author information
Authors and affiliations.
Health Sciences Program, College of Medicine, University of Saskatchewan, Saskatoon, Saskatchewan, S7N 5E5, Canada
Udoka Okpalauwaekwe
Sturgeon Lake Youth Center, Sturgeon Lake First Nation, Sturgeon Lake, Saskatchewan, S0J 2E1, Canada
Clifford Ballantyne
University of Saskatchewan, Saskatoon, Saskatchewan, S7N 0X1, Canada
Scott Tunison
Research Division, Department of Academic Family Medicine, University of Saskatchewan, Saskatoon, Saskatchewan, S7M 3Y5, Canada
Vivian R. Ramsden
You can also search for this author in PubMed Google Scholar
Contributions
UO contributed to the conceptualization, methodology, design, data analysis, original draft writing, review, editing and revision of this study. CB was involved in and contributed to the methodology, data analysis, original draft review and editing of this study. ST was involved in and contributed to the conceptualization, methodology, data analysis, review and editing of several versions of this study. VRR was involved in and contributed to the conceptualization, methodology, data analysis, review and editing of this study. All authors (UO, CB, ST, and VRR) read and approved the final the final manuscript.
Corresponding authors
Correspondence to Udoka Okpalauwaekwe or Vivian R. Ramsden .
Ethics declarations
Ethics approval and consent to participate, consent for publication, competing interests.
The authors report no conflict of interest.
Additional information
Publisher’s note.
Springer Nature remains neutral with regard to jurisdictional claims in published maps and institutional affiliations.
Supplementary Information
Additional file 1: supplementary material file 1..
PRISMA-ScR Checklist.
Additional file 2: Supplementary Material File 2.
Summary of critical appraisals of individual sources of evidence.
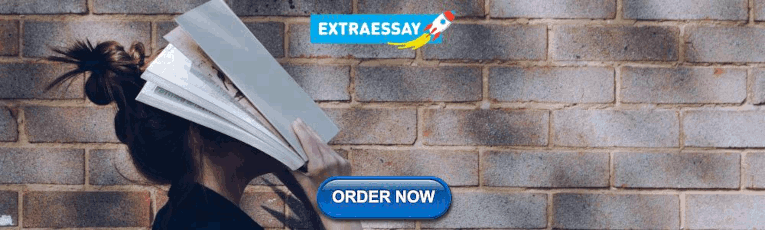
Additional file 3: Supplementary Material File 3.
List of Terminology.
Rights and permissions
Open Access This article is licensed under a Creative Commons Attribution 4.0 International License, which permits use, sharing, adaptation, distribution and reproduction in any medium or format, as long as you give appropriate credit to the original author(s) and the source, provide a link to the Creative Commons licence, and indicate if changes were made. The images or other third party material in this article are included in the article's Creative Commons licence, unless indicated otherwise in a credit line to the material. If material is not included in the article's Creative Commons licence and your intended use is not permitted by statutory regulation or exceeds the permitted use, you will need to obtain permission directly from the copyright holder. To view a copy of this licence, visit http://creativecommons.org/licenses/by/4.0/ . The Creative Commons Public Domain Dedication waiver ( http://creativecommons.org/publicdomain/zero/1.0/ ) applies to the data made available in this article, unless otherwise stated in a credit line to the data.
Reprints and permissions
About this article
Cite this article.
Okpalauwaekwe, U., Ballantyne, C., Tunison, S. et al. Enhancing health and wellness by, for and with Indigenous youth in Canada: a scoping review. BMC Public Health 22 , 1630 (2022). https://doi.org/10.1186/s12889-022-14047-2
Download citation
Received : 14 April 2022
Accepted : 18 August 2022
Published : 29 August 2022
DOI : https://doi.org/10.1186/s12889-022-14047-2
Share this article
Anyone you share the following link with will be able to read this content:
Sorry, a shareable link is not currently available for this article.
Provided by the Springer Nature SharedIt content-sharing initiative
- Indigenous youth
- Authentic engagement
- Culture as treatment
- Wellness promotion
BMC Public Health
ISSN: 1471-2458
- Submission enquiries: [email protected]
- General enquiries: [email protected]
Help | Advanced Search
Computer Science > Human-Computer Interaction
Title: advancing explainable autonomous vehicle systems: a comprehensive review and research roadmap.
Abstract: Given the uncertainty surrounding how existing explainability methods for autonomous vehicles (AVs) meet the diverse needs of stakeholders, a thorough investigation is imperative to determine the contexts requiring explanations and suitable interaction strategies. A comprehensive review becomes crucial to assess the alignment of current approaches with the varied interests and expectations within the AV ecosystem. This study presents a review to discuss the complexities associated with explanation generation and presentation to facilitate the development of more effective and inclusive explainable AV systems. Our investigation led to categorising existing literature into three primary topics: explanatory tasks, explanatory information, and explanatory information communication. Drawing upon our insights, we have proposed a comprehensive roadmap for future research centred on (i) knowing the interlocutor, (ii) generating timely explanations, (ii) communicating human-friendly explanations, and (iv) continuous learning. Our roadmap is underpinned by principles of responsible research and innovation, emphasising the significance of diverse explanation requirements. To effectively tackle the challenges associated with implementing explainable AV systems, we have delineated various research directions, including the development of privacy-preserving data integration, ethical frameworks, real-time analytics, human-centric interaction design, and enhanced cross-disciplinary collaborations. By exploring these research directions, the study aims to guide the development and deployment of explainable AVs, informed by a holistic understanding of user needs, technological advancements, regulatory compliance, and ethical considerations, thereby ensuring safer and more trustworthy autonomous driving experiences.
Submission history
Access paper:.
- HTML (experimental)
- Other Formats
References & Citations
- Google Scholar
- Semantic Scholar
BibTeX formatted citation

Bibliographic and Citation Tools
Code, data and media associated with this article, recommenders and search tools.
- Institution
arXivLabs: experimental projects with community collaborators
arXivLabs is a framework that allows collaborators to develop and share new arXiv features directly on our website.
Both individuals and organizations that work with arXivLabs have embraced and accepted our values of openness, community, excellence, and user data privacy. arXiv is committed to these values and only works with partners that adhere to them.
Have an idea for a project that will add value for arXiv's community? Learn more about arXivLabs .
- Search Menu
- Author Guidelines
- Submission Site
- Open Access
- About International Studies Review
- About the International Studies Association
- Editorial Board
- Advertising and Corporate Services
- Journals Career Network
- Self-Archiving Policy
- Dispatch Dates
- Journals on Oxford Academic
- Books on Oxford Academic

Article Contents
Introduction, the analytical framework: linking climate change, vulnerability, and conflict, methodology: a systematic review, pathways between climate change and violent conflict in the mena region, evaluating the “pathways” framework in the mena region.
- < Previous
Climate Change and Violent Conflict in the Middle East and North Africa

- Article contents
- Figures & tables
- Supplementary Data
Kyungmee Kim, Tània Ferré Garcia, Climate Change and Violent Conflict in the Middle East and North Africa, International Studies Review , Volume 25, Issue 4, December 2023, viad053, https://doi.org/10.1093/isr/viad053
- Permissions Icon Permissions
Previous research has demonstrated that climate change can escalate the risks for violent conflict through various pathways. Existing evidence suggests that contextual factors, such as migration and livelihood options, governance arrangements, and existing conflict dynamics, can influence the pathways through which climate change leads to conflict. This important insight leads to an inquiry to identify sets of conditions and processes that make climate-related violent conflict more likely. In this analytic essay, we conduct a systematic review of scholarly literature published during the period 1989–2022 and explore the climate-conflict pathways in the Middle East and North Africa (MENA) region. Through the systematic review of forty-one peer-reviewed publications in English, we identify that society’s ability to cope with the changing climate and extreme weather events is influenced by a range of factors, including preceding government policies that led to the mismanagement of land and water and existing conflict dynamics in the MENA region. Empirical research to unpack the complex and diverse relationship between the climate shocks and violent conflict in the MENA region needs advancing. Several avenues for future research are highlighted such as more studies on North Africa and the Gulf region, with focus on the implications of floods and heatwaves, and exploring climate implications on non-agriculture sectors including the critical oil sector.
Investigaciones previas que han demostrado que el cambio climático puede llegar a aumentar la probabilidad del riesgo de conflictos violentos a través de diversos mecanismos. Las pruebas existentes sugieren que los factores contextuales, tales como la migración y las opciones de medios de subsistencia, los acuerdos de gobernanza y la dinámica de conflicto existente, pueden influir en las vías a través de las cuales el cambio climático conduce a los conflictos. Esta percepción motiva una investigación con el objetivo de identificar una serie de condiciones y procesos que hacen que incrementan la probabilidad de conflictos violentos relacionados con el clima. En este ensayo analítico, llevamos a cabo una revisión sistemática de la literatura académica publicada durante el período entre 1989 y 2022. El estudio explora las vías de conflicto climático en la región de Oriente Medio y el Norte de África (MENA, por sus siglas en inglés). A través de la revisión sistemática de 41 publicaciones en inglés revisadas por expertos, fenómenos meteorológicos extremos está influenciada por una serie de factores, que incluyen tanto las políticas gubernamentales precedentes que condujeron a la mala gestión de la tierra y el agua como la dinámica de conflicto existente en la región MENA. Es esencial avanzar en la investigación empírica para poder desentrañar la compleja y diversa relación existente entre las perturbaciones climáticas y los conflictos violentos en la región de Oriente Medio y el Norte de África. Destacamos varias vías de investigación futura, como la realización de un mayor número estudios sobre el norte de África y la región del Golfo, con un enfoque en las implicaciones de las inundaciones y las olas de calor, así como la exploración de las implicaciones climáticas en los sectores no agrícolas, incluido el sector petrolero, de crítica importancia.
Des travaux de recherche antérieurs ont montré que le changement climatique pouvait aggraver les risques de conflits violents de bien des façons. Les éléments probants existants indiquent que les facteurs contextuels, comme les possibilités d'immigration et de moyens de subsistance, les arrangements gouvernementaux et les dynamiques de conflit existantes, peuvent avoir une incidence sur les mécanismes par lesquels le changement climatique peut créer des conflits. Cette information importante nous pousse à chercher les ensembles de conditions et de processus qui augmentent la probabilité des conflits violents en lien avec le climat. Dans cet article analytique, nous conduisons un examen systématique de la littérature académique publiée entre 1989 et 2022 pour nous intéresser aux liens entre climat et conflits dans la région du Moyen-Orient et de l'Afrique du Nord (MENA). En examinant de façon systématique 41 publications en anglais vérifiées par des pairs, nous remarquons que la capacité d'une société à gérer l’évolution du climat et les phénomènes météorologiques extrêmes est liée à un éventail de facteurs, y compris les politiques précédentes du gouvernement qui ont engendré une mauvaise gestion des terres et de l'eau et les dynamiques de conflit existantes dans la région MENA. La recherche empirique pour décortiquer la relation complexe et plurielle entre les crises climatiques et les conflits violents dans la région MENA doit avancer. Plusieurs pistes de recherches ultérieures sont présentées, comme davantage d’études dans la région de l'Afrique du Nord et du Golfe, en se concentrant plus particulièrement sur les implications des inondations et des vagues de chaleur, et l'analyse des conséquences climatiques sur les secteurs hors agriculture, notamment le secteur décisif du pétrole.
Climate change contributes to conflict risk and undermines livelihoods and human security. The impact of climate change overburdens countries in demanding security environments and exacerbates political instability, which may lead to violent conflict. Researchers have sought to explain the relationship between climate change and violent conflict and climate change as a growing factor for security risks ( Gleditsch 2012 ; Meierding 2013 ; Sakaguchi, Varughese, and Auld 2017 ; Ide 2018 ; Van Baalen and Mobjörk 2018 ). There is a greater consensus that climate change has an impact on human security and sustaining peace ( Abrahams 2020 ; Black et al. 2022 ; Morales-Muñoz et al. 2022 ). The evidence has been gathered on the physical changes in diverse livelihood systems and human migration and the negative effects on human adaptation capacities ( IPCC 2022 ). The debate may have to move on from whether climate change has been the primary cause of a war or not ( Verhoeven 2011 ; e.g., Selby et al. 2017 ). Our understanding of what context climate change matters for conflict and security and how relevant factors play out in local contexts should be based on comprehensive and systematic research that considers various scales, time periods, and localities.
Moreover, existing evidence suggests that climate-related security risks are context specific, and there are multiple pathways by which climate change influences the onsets and patterns of armed conflict ( Brzoska and Fröhlich 2016 ; Mobjörk, Krampe, and Tarif 2020 ). The “climate insecurity pathway” framework assumes that climate change may not be the only contributor to violent conflict but also other factors leading to insecurity such as internal and international migration, livelihood options, and governance arrangements ( Van Baalen and Mobjörk 2018 ). Existing conflict dynamics and security environments can exacerbate climate-related security risks. This analytic essay contributes to the debate on how climate change affects the risk of violent conflict by conducting a systematic review of the literature directly or indirectly linking climate change of violent conflict focusing on the Middle East and North Africa (MENA), a region that has been severely impacted by both. 1 By conducting a systematic literature review, we are particularly interested in synthesizing existing evidence to better understand the climate-conflict links in the MENA region. We included forty-one peer-reviewed articles published between 1989 and 2022 in the analysis. Based on the review, we conclude that the relationship between climate change and violent conflict is predominantly indirect and diverse, highlighting the need to avoid oversimplified assumptions. Climate change’s contribution to conflict risk in the MENA region is further mediated by political economy, institutional weaknesses, elite competition, and existing socio-political relations. A careful examination of evidence is crucial for comprehensive climate security discussions in general and policy considerations for the MENA region. The following systematic review of literature showcases the linkages between climate exposure and various sources of vulnerability in the MENA region.
Climate Exposure and Social Vulnerability in the MENA Region
The MENA region is facing major security challenges from its vulnerability to climate change and violent conflict. The region is the world’s most water-stressed region, hosting thirteen of the world’s twenty most water-stressed countries, with currently over 82 percent of its terrain covered in desert ( Sieghart and Betre 2018 ). Indeed, water rationing and the limitation of water supplies are already a reality in parts of Algeria, Lebanon, Iraq, Palestine, and Jordan ( Sowers, Vengosh, and Weinthal 2011 ). Recent climate science predicts an average global warming of 1.5°C under the business-as-usual scenario, while in the MENA region, it is expected to increase up to 4°C ( Gaub and Lienard 2021 ). Furthermore, the level of mean precipitation is also expected to decrease in the region ( Zittis , et al. 2020 ). By the end of the century, about half of the MENA population could be annually exposed to super- and ultra-extreme heatwaves ( Zittis et al. 2021 ). In essence, the region is likely to become drier and experience extremely high temperatures, followed by extreme and chronic water shortages becoming more frequent.
Many countries in the MENA region are vulnerable to the effects of climate change due to their weak adaptive capacity ( Sowers et al. 2011 ; Namdar, Karami, and Keshavarz 2021 ). The adaptive capacity to climate change varies across the MENA region. While oil-exporting Gulf states have the financial resources for investments in water desalination and wastewater technologies, others suffer from a lack of financial resources and water conservation policies ( Sowers et al. 2011 ). The adverse effect of climate change on agricultural productivity is likely to affect the livelihood conditions of rural populations and may contribute to rural-to-urban migration in some cases ( Waha et al. 2017 ). Changes in precipitation and extreme weather events can reduce the region’s agriculture yields, as up to 70 percent of the crops are rain-fed ( Waha et al. 2017 ). Climate change impacts present a threat to food security in the MENA region and exacerbate the vulnerability to global food price volatility, including Egypt and Lebanon. Countries with a high level of imported grain dependency witness significant inflations in cereal prices that can be a source of political instability ( Tanchum 2021 ). Food price volatility has contributed to political stability in the past, especially during the Arab Spring, and the combined effect of reduced water discharge with the demographic trend of the youth bulge could present a challenge to the political stability of a region ( Borghesi and Ticci 2019 ).
Over the past decade, several of the world’s deadliest conflicts flared up in the MENA region, particularly in Syria, Yemen, Iraq, and Turkey ( Palik et al. 2020 ). The intractable conflict between Israel and Palestine has caused immense human suffering and disrupted regional stability. These conflicts are linked to long-running inequalities and grievances and economic and political instability, which make conflict resolution exceptionally challenging. Deterioration of the physical environment and land degradation further exacerbate risks of communal conflict and political instability in the future. Violent conflict, on the other hand, has been destructive to the adjoining environment. For instance, the effect of intense armed conflict has been significant in Syria’s already declining land and water resources ( Mohamed, Anders, and Schneider 2020 ). Environmental degradation leading to water and food insecurity has adversely affected the livelihoods of the population.
The linkages between conflict and the environment are an integral component that constitutes peace and security in the MENA region. The arid natural environment of the region and the changing climate are part of consideration when analyzing conflict in the region ( Smith and Krampe 2019 ). This article focuses on the MENA region and analyzes the role of climate-related environmental factors in violent conflict by drawing evidence from existing research. This systematic review provides an overview of conditions and processes in the climate-conflict nexus. The findings demonstrate that indirect pathways between climate change and violent conflict that are found in other regions such as East Africa, South Asia, and Southeast Asia, and West Africa are also applicable to the MENA region. In addition, downstream impacts of water development projects such as dams and irrigation projects in transboundary river basins, weaponization of water by armed groups, and the government’s mismanagement of water and land have particularly affected vulnerability to climate change in the MENA region. Climate change exacerbates water scarcity in the MENA region, which in turn can incentivize policies such as unilaterally building water storages and weaponization of water as an instrument for leverage during armed conflicts. These MENA region-specific dimensions of climate-conflict pathways appear to be influenced by the region’s internal politics, relations between neighboring countries, and conflict dynamics.
The article is organized in the following order. We present the analytical framework of a set of pathways that connects climate change and violent conflict and then an outline of the methodology for a systematic review, which includes the operationalization of the variables and the sampling strategy. This is followed by the description of the methodology for conducting a systematic review. The review of literature is organized into four categories that are specified in the analytical framework, and then a synthesized analysis is detailed. Finally, we conclude by summarizing policy and research relevant implications from the finding in the MENA regional contexts with a set of recommendations.
The climate-conflict nexus is complex. Climate change has implications for various forms of interstate and intrastate conflict, including communal violence, insurgencies, mass civil resistance campaigns, protests, and interpersonal disputes ( Hendrix et al. 2023 ). Specific contexts of environment, socio-political systems, and pre-existing conflict matter when examining the connection between climate-related environmental changes and conflict. The analytical framework is based on a premise that the relationship between climate change and conflict is mediated by social, political, and ecological vulnerability ( Daoudy 2021 ). When climate impacts contribute to social outcomes such as deteriorating livelihood conditions, migration, escalation of armed groups’ tactics, and elite capture, risks of violent conflict can increase. The following outlines four “pathways” between climate change and conflict ( Figure 1 ).

A framework of climate insecurity pathways
The deterioration of livelihood conditions is a centerpiece in linking environmental changes and violent conflict. Climate-exposed sectors such as agriculture, forestry, fishery, energy, and tourism are highly likely to suffer from economic damages from climate change ( IPCC 2022 , SPM-11). Consequently, people whose livelihoods are dependent on the natural environment are subjected to additional economic burdens due to the changing climate or climate shocks. Extreme weather events such as droughts, heatwaves, sandstorms, flooding, and long-term changes in the environment can affect the income from the aforementioned sectors ( IPCC 2022 , SPM-11). Populations with low adaptive capacity including marginalized groups are disproportionately affected and vulnerable to short-term economic damages related to climate change ( IPCC 2022 , SPM-8). Demographic changes may accelerate the deterioration of livelihood conditions. Population growth in the MENA region has been rapid from 105 million in 1960 to 486 million in 2021 ( World Bank 2022 ), which means more land and water are required for livelihoods. Climate change can worsen coastal erosion and decline tin he productivity of coastal plains in Israel and Morocco, which are important for food production. Sea-level rise has negative impacts on deltas, coastal plains, and human settlements, and tourism and industrial activities are also expected to decline due to heatwaves and worsening water shortages ( Sowers et al. 2011 ).
Existing studies focus on various socio-economic outcomes of climate and environmental changes and their implications on conflict mobilization. Agriculture, fisheries, and livestock sectors are particularly susceptible to the loss of income due to climate shocks such as prolonged droughts ( von Uexkull 2014 ; Schmidt and Pearson 2016 ). Loss of income due to the deterioration of livelihood conditions can lead individuals to seek alternative sources of livelihood, and some may turn to illicit activities, including joining non-state armed groups ( Barnett and Adger 2007 , 644; Seter 2016 , 5).
Another category of social outcomes includes changes in migration and mobility patterns. Migration is one of the climate adaptation strategies, and subsequent socioeconomic and political impacts of migration can be linked to conflict. Declining livelihood conditions can trigger rural-to-urban migration in search for alternative livelihoods ( Rüttinger et al. 2015 , 27). Long-term climate change and weather shocks may accelerate environmental degradation and declining livelihood conditions. The increased migration flow accelerates urbanization and creates instability in hosting cities with inadequate infrastructure for public services ( Balsari, Dresser, and Leaning 2020 ).
Changing migratory patterns of pastoralist or agropastoral groups, influenced by the availability of grazing land and water, can be linked to clashes with other communities ( Abroulaye et al. 2015 ; Mohammed Ali 2019 ). Violent communal clashes and livestock raiding, which have become increasingly lethal, are linked to intensified competition over scarce resources for pastoralist populations ( Detges 2014 ). For instance, farmer-herder conflicts in the Sahel region have become increasingly lethal during recent decades, especially in areas with a higher population and livestock density.
Previous research also focuses on the role of elites who have leveraged social outcomes of climate change for their benefit. Here, elite actors include traditional elites, privileged groups with economic and political power, and even armed group leaders. More frequent and intense climate-related extreme weather events can provide additional opportunities for local elites to capture resources. When climate-induced disasters such as droughts and floods cause humanitarian crises, their basic needs and post-disaster reconstruction would bring in additional resources to the disaster-hit regions, which can be exploited by local elites. Humanitarian aid delivery often needs to cooperate with local elites, whose influence over the aid provision can further strengthen the client-patronage relationship, which is a source of tension ( Uson 2017 ). Elite capture of resources, particularly land, is likely to generate strains within and between communities ( Zaman 1991 ). Local grievances over land rights can be exploited in intercommunal conflict or national conflicts ( Chavunduka and Bromley 2011 ). National elites can exploit local grievances of a population segment that are closely related to climate change. Inadequate government responses to Cyclone Bhola in 1970 led to a devastating human toll in the Bay of Bengal and contributed to the rise of the independence movement, which subsequently led to the secession of Bangladesh ( Busby 2022 , 181).
Changing environmental conditions by climate change may influence armed group tactics and behaviors. Armed groups have utilized the local grievances for a recruitment drive for the youth ( Benjaminsen and Ba 2019 ). Climate change also affects the way of wars are to be fought. In warm climates, prolonged and unpredictable rainy seasons can alter the fighting season and patterns. Due to the reduced water availability in some areas, the strategic importance of water access points and infrastructure may have become more salient. Armed groups can escalate the conflict by weaponizing water by flooding farmland and cities or depriving the population of water ( King 2015 ). Amid droughts and unreliable rainfalls, armed groups may consider water weaponization as a more effective tactic in order to influence and control communities already experiencing water scarcity.
The analytical framework of climate-conflict pathways is applied to analyze findings from existing research relevant to the MENA region. The following details a method of a systematic review of the literature.
This paper leverages from existing evidence by conducting a systematic review of existing studies. Systematic review method has been extensively employed in examining the linkage between climate change and violent conflict ( Ide 2018 ; Nordqvist and Krampe 2018 ; Van Baalen and Mobjörk 2018 ; Tarif 2022 ). Systematic reviews differ from a traditional sense of literature review in a way that it is “focused” and “systematic”; it zooms on a specific research question; and is based on pre-established sets of principles for literature selection. Systematic and focused nature of the review is helpful to “locate previous research, select relevant literature, evaluate contributions and analyses, and synthesize data” ( Denyer and Tranfield 2009 , 671). This approach is particularly useful to yield new insights and provide clarification on frequently debated issues ( Dacombe 2018 , 155). In addition, the method is a highly relevant policy tool that promotes evidence-based policymaking.
We have used the following set of principles for locating, selecting, and evaluating the literature. A Boolean search string containing keywords was composed with keywords from climate change and violent conflict. 2 Search words for climate-related environmental conditions include terms related to the effects of extreme weather events or long-term environmental changes on nature-based livelihoods and water and food insecurity, involuntary displacement, which are adopted from previous research done in a similar scope ( Nordqvist and Krampe 2018 ; Van Baalen and Mobjörk 2018 ; Tarif 2022 ). Several social outcomes are theorized as consequences of climate change such as internal and cross-border migration and elite exploitation of changing environmental conditions. In the paper, violent conflict is defined as the situation when one or more actors engaged in violence against hostile groups due to incompatibilities. This broad definition allows include interstate wars, terrorism to communal clashes involving violence. The definition does not include protests and non-violent actions, which are a crucial class of social phenomena leading to political instability and violence. We paid attention to this element in the analysis but excluded studies exclusively focusing on non-violent conflict (e.g., Ide et al. 2021 ). We used specific keywords relevant to conflict actors and types of conflict in the MENA region.
The Boolean search string was used in searching the abstracts of existing studies in English published during 1989–2020 from Web of Science, a major database of scholarly literature. From the search results, we read the abstracts and selected items with relevance to the relationship between climate-related environmental changes and conflict. The initial screening found 141 articles, which then were reviewed manually for their relevance to the inquiry (see the Online Appendix). In the screening process, we excluded a number of studies that focused on the impact of armed conflict on the environment and studies that did not explicitly focus on violent conflict. Similarly, studies that do not explicitly focus on climate change as in long-term climate trends, climate hazards, and weather events were excluded. Another set of articles that were removed from the list were commentaries and reviews that were not based on either qualitative or quantitative empirical material. While all the selected articles either have at least one country in the MENA region or adapt a regional focus on the MENA, the specific definition of these regions varies. In our literature review, we adhere to a specific list of countries that we recognize as part of the region. 3 After the screening, we manually searched the bibliographies of the selected articles and included eleven relevant articles. In total, forty-one articles are reviewed with a focus on a set of categories stemmed from the analytical framework for explaining the relationship between climate-related environmental change and violent conflict ( Figure 2 ).

Peer-reviewed articles reviewed
The geographical focus of the reviewed studies demonstrates that much of the scholarship focuses on Syria and Iraq. In contrast, North African countries and Gulf countries have received relatively limited attention ( Figure 3 ). The high number of research works focusing on Syria can be explained by the high profile of the contested linkage between climate change and the Syrian civil war. While media narratives have regarded Syria as a prime example of an armed conflict fuelled by climate change and several prominent public figures have publicized it as an illustration of the nexus, it is worth noting that scholarly research has presented differing perspectives on the direct causative role of climate change in conflict escalation ( Miller 2015 ; “Climate Wars - Syria” with Thomas Friedman 2017 ; VICE 2017 ).

The distribution of geographical focus of the reviewed studies
Source: a map drawn by authors.
In this section, we discuss existing explanations from previous research that connect climate-related environmental changes and violent conflict in the MENA region. The linkages between the environmental changes related to climate change and violent conflict constitute a complex chain of events (e.g., Gleditsch 1998 ). Most empirical research contributes to examine parts of the chain under specific temporal and spatial scopes, and this is one reason why it is important to consider the broader implication of each piece of evidence, which then can contribute to the better understanding of the climate-conflict pathways as a larger phenomenon. For clarity and focus, we organized a set of findings from previous studies under four pre-determined analytical categories: worsening livelihood conditions, migration and mobility, armed groups, and elite exploitation. As explained earlier, these categories are not mutually exclusive; rather, explanations under different categories are interlinked and can mutually reinforce each other in different stages of mobilization and conflict.
Direct Link between Climate Change and Violent Conflict
Scholars have examined whether climate impacts such as warmer temperatures and precipitation anomalies are statistically correlated to violent conflict, and several studies have focused on specific countries within the MENA region ( Feizi, Janatabadi, and Torshizi 2019 ; Döring 2020 ; Helman and Zaitchik 2020 ; Helman, Zaitchik, and Funk 2020 ; Sofuoglu and Ay 2020 ; Linke and Ruether 2021 ). Findings from existing research on the direct impact of climate-related factors on violent conflict and political instability suggest that the relationship is not always linear and varied in specific country contexts ( Helman and Zaitchik 2020 ; Helman et al. 2020 ). Water scarcity, for instance, is not only associated with increased communal conflict but also cooperation ( Döring 2020 ). Warming did not unitarily increase or decrease conflict risk—warmer temperatures increased risks of violence in Africa but decreased in the Middle East, and warming did not have a linear effect but had a greater effect on conflict risk in warmer regions ( Helman et al. 2020 ). Increased temperatures and rainfall anomalies are positively associated with political instability in the MENA region ( Helman and Zaitchik 2020 ; Sofuoglu and Ay 2020 ). These findings caution against generalized or simplistic assumptions about the relationship between climate change and violent conflict.
Studies have found an insignificant relationship between water scarcity and violent conflict. Precipitation levels and droughts do not have a direct impact on communal violence in a model including the Middle East and Africa ( Döring 2020 ). The same study also found that communal conflict is more likely to occur in areas with lower rainfalls and limited groundwater availability. Groundwater is less affected by short-term droughts, but prolonged droughts and unsustainable extraction can lead to groundwater shortages, which is the case in northern Syria ( Kelley et al. 2015 ) and Yemen ( Weiss 2015 ). Rainfall variability does not seem to have significantly affected the intensity of civil war violence during the 2011–2019 Syrian civil war ( Linke and Ruether 2021 ). The discussion on climate change’s impact on armed group tactics and behavior is followed in the later part of the paper.
Droughts and water scarcity seem to be a source of social disputes and non-violent conflict ( Feizi et al. 2019 ; Bijani et al. 2020 ; Ide et al. 2021 ). Whether the tension over water scarcity escalates to non-violent conflict or not seems to be contingent on the pre-existing negative socio-political relationships between groups and the types of political systems ( Ide et al. 2021 ). In Iran, irregular rainfalls and water scarcity at the local level are linked to interpersonal conflict and communal tensions and can degrade state legitimacy and contribute to political instability ( Feizi et al. 2019 ; Bijani et al. 2020 ).
Evidence from existing studies on the direct climate-conflict link also alludes to the need to further explore the mechanisms between physical environmental changes and social outcomes. Both large- N and small- N studies can contribute to the understanding of the underlying mechanisms or indirect pathways connecting climate change and conflict. The following sections discuss livelihoods, migration, inadequate management, and armed group behaviors as the pathways between climate-related environmental changes and violent conflict.
Deteriorating Livelihood Conditions
Several studies evaluating the worsening livelihood mechanism in the MENA region focus on the relationship between droughts’ impacts on agriculture and conflict. Severe and frequent droughts due to climate change may affect the region’s food security and livelihoods. In the MENA countries, agriculture, fisheries, and livestock accounts for roughly 15 percent of the total population’s livelihood ( World Bank 2023 ). Agriculture dependency is one of the best predictors of violent conflict ( von Uexkull et al. 2016 ). Indeed, evidence from a study focusing on the MENA region and Africa shows a consistent result that conflict risk is higher in areas where the population depends on agriculture for their livelihoods ( Helman and Zaitchik 2020 ).
Droughts’ impact on agriculture is an important area of research in the implications of the changing climate on the deterioration of livelihood conditions. During the last three decades, droughts in the MENA region have become more frequent and severe. Three out of four most severe multi-year droughts in the Fertile Crescent region referring to parts of Iraq, Syria, Lebanon, Palestine, Israel, Jordan, and Egypt occurred during 1990–2015 ( Kelley et al. 2015 , 3243). The sub-region has historically witnessed periodic droughts; therefore, their agricultural systems are to a degree adaptive to drought conditions and low rainfalls. More frequent and intensifying droughts and drying conditions may jeopardize the population’s adaptive capacity, leading to far-reaching and consequential disruptions in societies. In particular, the 2007–2008 drought severely affected the agricultural production in the Fertile Crescent region. Annual wheat production in Iraq during 2008–2009 declined by 35 percent ( Selby 2019 , 264). Jordan and the West Bank in Palestine also experienced a reduction in agricultural production after the 2007–2008 drought ( Feitelson and Tubi 2017 ). However, none of these countries experienced the same extent of “shock” as in Syria, whose effects some refer to as the “collapse” of the agricultural sector. The 2007–2008 drought is considered “the worst drought in the instrumental record, causing widespread crop failure” and decimation of livestock populations in northeast Syria ( Kelley et al. 2015 , 3241).
A dozen of the reviewed authors have probed the linkage between the 2007 and 2008 multi-year droughts and their impacts on agricultural and livestock production and the Syrian conflict using quantitative and qualitative methods ( De Châtel 2014 ; Gleick 2014 ; Kelley et al. 2015 ; Eklund and Thompson 2017 ; Selby et al. 2017 ; Ide 2018 ; Karnieli et al. 2019 ; Ash and Obradovich 2020 ; Daoudy 2020a , 2021 ; Eklund et al. 2022 ). These reviewed research works have disagreed on what extent the drought’s contribution to the sharp decline in agricultural production and rural livelihood in Syria. Kelley et al. (2015 ) is one of the major empirical studies that argues for the linkage between the multi-year drought and the political instability, which argument is similar to Gleick (2014 ). Other studies have refuted the causal linkage between the drought and the Syrian civil war, but their core reasons for arguing against it have varied.
Several authors point out that the impact of climate shocks on livelihoods is mediated by water governance decisions. This argument downplays the role of climate change as the main driver of livelihood deterioration rather than a contributing factor. Despite being affected by similar rainfall deficits during 2007–2008, farmers in northern Syria generally experienced far worse consequences in productivity compared to northwest Iraq and southeast Turkey ( Eklund and Thompson 2017 ). Turkey’s substantial investment in water infrastructure and placing policies for better management during the 1990s and 2000s seem to have reduced their vulnerability to droughts ( Kelley et al. 2015 ; Eklund and Thompson 2017 ). On the contrary, the Syrian regime’s agricultural expansion policy, unsustainable groundwater use, and economic policy have exacerbated the population’s drought vulnerability. Agricultural expansion schemes in Syria more than doubled the irrigated area from 650,000 ha in 1985 to 1.4 million ha in 2005, driven by “a vision of development through agrarian modernization” ( Selby 2019 , 268). The policy overlooked physical limitations of groundwater resources by over-extracting water from aquifers at a rate of 300 percent or more than the basin’s yield and depleting aquifers prior to the 2007–2008 drought ( Selby 2019 , 266). Groundwater depletion in the region has a major effect on drought vulnerability because groundwater is an important source of water during low rainfall years ( Kelley et al. 2015 ).
Weiss (2015 ) makes a similar observation in Yemen, indicating that governance issues are mainly responsible for groundwater depletion in the country rather than climate-related environmental changes. Factors related to agrarian political economy and governance capacities further affect the vulnerability. The government’s capacity to deal with environmental changes and their impact on local economies and livelihoods is pointed out to be a key mediating factor in the linkage between climate change and violent conflict. The issues related to mismanagement and elite exploitation of climate change are further discussed in the later section of the article.
A few studies found differing climate impacts based on gender and ethnicity. Vulnerability to climate change varies between communities and countries, and intersectional identities of the affected people such as gender, age, and ethnicity influence their capacity to adapt to climate change and resilience ( Thomas et al. 2019 ). Evidence from Iran shows how women are forced to carry the “double burden” of doing off-farm work activities such as weeding or thinning cotton for minimal wages, in addition to the regular household and on-farm tasks ( Keshavarz, Karami, and Vanclay 2013 ). In Syria, the mechanization of agriculture has led to a significant loss of rural employment and disproportionately affected women ( Selby 2019 , 267). The disproportionate effect on women is related to structural gender inequality restricting women’s economic opportunities and wealth accumulation ( Selby 2019 ). This finding aligns with previous literature linking gender and climate change indicating that women are often worse affected by climate impacts due to restrictive norms and rights ( Denton 2002 ). In Israel, pastoralists are often disadvantaged due to the Israeli state’s resource allocation policies prioritizing farmers. In the northern Negev region, the state’s land appropriation disproportionately affected agri-pastoralist Bedouin tribes during the early 1900s. This has led to higher vulnerability of the Bedouins during droughts ( Tubi and Feitelson 2016 ). A similar pattern of marginalization is found in Hasakah, a region in northern Syria, where the state turned open range lands into farmlands ( Selby 2019 ). The findings on differing vulnerability and impacts on livelihoods are based on a handful of studies, and intersectional approaches are generally absent in most studies reviewed in the analytic essay.
Changes in Migration and Mobility Patterns
Migration represents a critical adaptation strategy for populations affected by climate-induced environmental changes. Existing research examines various linkages between climate-induced environmental changes and migration in the MENA region. The main discussions are related to the contribution of climate shocks in internal and international migration and migration as a source of political instability and conflict. Existing evidence in the reviewed studies does not fully confirm that climate shocks and changing climate conditions are the primary drivers of internal or international migration. The link between displacement and violent conflict seems to be contested as well.
One of the predominant narratives links climate, migration, and insecurity theorizes worsening of livelihood conditions due to climate change has led to distressed migration of rural population to urban or peri-urban areas, which can contribute to greater political instability ( Gleick 2014 ; Kelley et al. 2015 ; Feitelson and Tubi 2017 ; Ash and Obradovich 2020 ). This argument gained prominence after out-migration from drought-affected regions in northern Syria in 2008 and the agricultural sector collapse in 2010 preceded the 2011 uprising.
Several studies focus on empirically examining the migration patterns after the 2007–2008 droughts in the Levant ( De Châtel 2014 ; Gleick 2014 ; Kelley et al. 2015 ; Ash and Obradovich 2020 ). There seems to be a wide-ranging estimation of the scale of internal migration in Syria during this time ( Ide 2018 ). While acknowledging the multiple factors contributing to migration, researchers have debated on the number of displaced people in northern Syria and Iraq amid the 2007–2008 drought. While Gleick (2014 , 334) and Kelley et al . ( 2015 , 3241–2) estimate ∼1.5 million people to be internally displaced, others suggest 40–60,000 households or ∼ 300,000 displaced people ( Selby et al. 2017 , 254). Several methods are employed in estimating drought-induced migration. For instance, Ash and Obradovich (2020 ) used nightlight intensity as a proxy measure for population change, which seemed to detect the changes in population in drought-affected regions. Satellite imagery can be analyzed for measuring agricultural land use, which can be a proxy indicator for out-migration ( Eklund et al. 2022 ). Others relied on official statistics and survey data, which are based on a combination of census, fieldwork, and expert assessment (e.g., OCHA 2009 ). Nightlight intensity and satellite imagery are effective measurements of population changes, but remote sensing data provide little context about who moved, to where, and why. Fieldwork-based studies such as De Châtel (2014 ) provide insights into the socio-economic circumstances of migrants and their political orientation. A UN rapid assessment report is based on various UN-led field reports and assessments during 2006–2008 and supplies valuable on-the-ground information including changing migration patterns, children’s school enrollment, and water availability ( OCHA 2009 ). The evidence indicates that migration after the drought was indeed significant, although we cannot exactly say the scale of it. The question is whether these migrants play a role in the subsequent uprising and civil war.
Critics of this narrative argue that the Syrian uprising emerged due to political discontent, economic recession, youth unemployment, discrimination, and injustice, not because of the mass climate migrants ( De Châtel 2014 ; Selby et al. 2017 ; Daoudy 2020a ). Eklund et al. (2022 ) suggest migration triggered by the 2007–2008 droughts did not play a significant role in the uprising because migrants were likely to have returned as early as 2010 based on the satellite images showing full recovery of agricultural activities in drought-affected areas ( Eklund et al. 2022 ). Rural-to-urban migration in the MENA region is rather influenced by pre-existing socio-economic conditions and political decisions. For example, in Syria, the introduction of neoliberal agrarian policies by the government generated a significant degree of insecurity in the rural populations and prompted rural-to-urban migration ( De Châtel 2014 ; Selby 2019 ). And region’s demographic trend has a much greater and long-lasting impact on the pressure in urban areas. For instance, the urban population in Syria is estimated to have grown from 8.9 million in 2002 to 13.8 million in 2010, and most migrants lived in informal settlements with poor infrastructure and no jobs ( Kelley et al. 2015 ).
The narratives on climate change and migration in the MENA region in existing literature reflect how countries perceive climate-induced migration as a source of conflict and insecurity. Jordan, for instance, fears the influx of migration from the MENA region, mostly Palestine, Iraq, and Syria, would worsen the country’s water scarcity and thus security ( Weinthal, Zawahri, and Sowers 2015 ). Fears of “climate refugees” from Africa have shaped Israel’s discriminatory discourses and practices against African refugees and Bedouin communities inside the country ( Weinthal et al. 2015 ). Media reports have suggested that climate shocks in the MENA regions, where asylum seekers and irregular migrants originated from, have affected their decision to migrate ( O'Hagan 2015 ). More than 2.2 million migrants without legal permits have amassed at EU external borders during 2009–2017, and most migrants during this period were from the MENA region ( Cottier and Salehyan 2021 , 2).
Findings from existing research refute the idea of climate shocks would trigger refugee flows from the MENA region. Climate shocks and precipitation deficits are not linked to the increase of out-migration from the MENA region to Europe ( Abel et al. 2019 ; Cottier and Salehyan 2021 ). Severe droughts and drier weather conditions in the MENA region are associated with the reduced migration flow to Europe, which is contradictory from the popular media narrative about “climate refugees” ( Cottier and Salehyan 2021 ). This finding alone suggests that migration can be an “investment,” because the extra income generated from additional rain reduces financial barriers to emigrating ( Cottier and Salehyan 2021 , 6). The correlation between rainfall variability and asylum-seeking flows has been found during 2010–2012 when the Arab Spring swept a dozen MENA countries but not during other periods between 2006 and 2015 ( Abel et al. 2019 ). This finding demonstrates that the impact of climate change on generating asylum-seeking flows seems to be conditional on the origin country’s political stability.
Armed Group’s Tactical Considerations
Existing research specifically focusing on how climate change affects armed groups’ tactics is sparse in the MENA region (exception of Linke and Ruether 2021 ), but several research works demonstrate that armed groups may escalate their tactics due to the increased environmental stress on water and agricultural land. Changing climate conditions and weather shocks adversely affect water availability for agriculture. This trend underscores the notion that the strategic importance of controlling water and water infrastructure could emerge as an effective instrument for exerting pressure to local populations in times of armed conflicts. Previous research supplies evidence on how water is weaponized by armed groups, which is a case of escalation of tactics ( Grech-Madin 2020 ). Water weaponization is defined as the “intentional or unintentional damage or destruction of (sensitive) components of the water infrastructure like dams, treatment plants, pumping stations, piping and canal systems, sewage plants, reservoirs, wells, etc” ( von Lossow 2016 , 84).
Water has been used as both a target and a weapon by state and non-state actors. Existing studies focus on how non-state armed groups and government militaries have strategically attacked or captured water and other environmental infrastructure ( King 2015 ; von Lossow 2016 ; Sowers, Weinthal, and Zawahri 2017 ; Gleick 2019 ; Daoudy 2020b ). Water scarcity in the region is an incentivizing factor for government troops and armed groups to use water to incur damage to the local population. Attacks on water pipes, sanitation and desalination plants, water treatment, pumping and distribution facilities, and dams have occurred in Syria, Libya, and Yemen during civil wars. Targeting of water infrastructure also occurs in protracted conflict situations such as the Israel and Palestine conflict when Israel was accused of attacking wells in Gaza City ( von Lossow 2016 , 84). Particular attention has been drawn to rebel groups’ ability to use water for strategic but as well psychological terrorism ( King 2015 ).
The weaponization of water is not limited to targeting water infrastructure during wartime. Increasing water scarcity and the importance of water access influence the strategic calculation by armed groups on when and where they would deploy violence ( King 2015 ). Non-state armed groups such as the Islamic State in Syria and Iraq are known to have fought over the control of water infrastructure in the Euphrates and Tigris Rivers as part of their expansion strategy ( von Lossow 2016 ). Armed groups fight more intensely during the growing season, which is linked to tax revenue from agricultural harvest and control of the population who rely on farming ( Linke and Ruether 2021 , 116).
Armed groups can also use water as a tool of governance. By providing water and electricity to the local population, the Islamic State achieved ideological credibility as well as legitimacy over the local population, which was a core component of the IS claim of statehood ( King 2015 ; von Lossow 2016 ). Supplying water is a crucial governance function, so armed groups can obstruct water infrastructure to damage the conflict party’s control and legitimacy.
Elite Exploitation
Previous research demonstrates how elite exploitation is linked to protests and violent conflict by focusing on corruption, elite capture of disaster relief, and elite bias in the MENA region. Political patronage and ethnic, tribal, and religious networks for political mobilization shape elite behavior in the region. Political patronage is not unique to the MENA region, but clientelism explains the viability of political networks of some political elites in the MENA region who maintained power through providing resources and preferential treatment in return for votes, loyalty, and compliance ( Herb 1999 ; Haddad 2012 ). Social fabrics of the MENA are woven with diverse ethnic, tribal, and religious groups, and these minorities have also been part of political cleavage structures ( Belge and Karakoç 2015 ). Political mobilization along ethnic, tribal, and religious lines has been effective in the contexts when these identities are contested ( Yiftachel 1996 ). In the following, three main findings from existing research are outlined.
Climate change may increase opportunities for elites to appropriate humanitarian aid for their benefit, and elite exploitation can worsen the conflict risk amid climate-induced disasters and environmental scarcities. The risk of politicization of humanitarian and development aid has been extensively studied ( Doocy and Lyles 2018 ; Alqatabry and Butcher 2020 ). In situations of climate-induced disasters, local and central elites can have a significant influence on the planning and distribution of humanitarian aid. Political elites can be biased in their relationship with local elites, and this elite bias can have implications for local-level politics ( Brosché and Elfversson 2012 ). After the 2007–2008 drought in Syria, the Assad government directed the UN-led relief efforts to almost entirely focus on the Arab district of Al-Shaddadi, although the Kurdish communities were equally or worse affected ( Selby 2019 , 270). Unequal aid distribution can increase intercommunal tensions during droughts. State intervention can reduce the risk of conflict amid climate-related natural disasters. Tubi and Feitelson (2016 ) demonstrate how proactive relief provisions during droughts have reduced communal violence between Bedouin herders and Jewish farmers in Israel. The findings from Tubi and Feitelson (2016 ) confirm that the state’s capacity to adapt and absorb shocks remains essential for the inhabitants’ perceived marginal benefits and the opportunity cost of conflict ( Post et al. 2016 ).
Powerful elites compete over acquiring land and water resources from weak and vulnerable groups. Mismanagement and corruption in the public sector are other factors that affect the population’s access to water and basic services, which are simultaneously hampered by climate change ( Kim and Swain 2017 ). In Yemen, most communal conflict occurs over water and land when tribal elites compete with one another ( Weiss 2015 ). In southern Iraq, a large volume of water is illegally diverted for commercial farms owned by elites, which worsens water scarcity ( Mason 2022 ). Donor-funded projects for repairing Basra’s aging water infrastructure after the 2003 invasion, worth 2 billion USD over nearly two decades, were succumbed to widespread corruption ( Mason 2022 ). Bureaucratic procedures endow opportunities for officials to extort bribes such as well-licensing in Syria and water development project licensing in Lebanon ( De Châtel 2014 ; Mason and Khawlie 2016 ). In Syria, the government’s requirement to annually renew well licences was an opportunity for security personnel and local officials to collect bribes ( De Châtel 2014 , 12). Protestors in Dara’a, Syria initially demanded to end corruption in the water sector ( De Châtel 2014 ). In Iraq, the epidemic of corruption in the water sector endowed youth and urban poor grievances against the state, which led to widespread protests ( Human Rights Watch 2019 ).
Although the MENA region is a climate change hotspot, governance failures, and mismanagement account for declining water access ( Mason and Khawlie 2016 ; Selby et al. 2017 ; Daoudy 2021 ). Elites in the MENA region have leveraged climate change to explain some of the governance failures in the water and agriculture sectors. The Syrian state and security apparatus have exploited the narratives around climate change by portraying Syria as a “naturally water-scarce” country, although the reality on the ground shows a man-made water crisis due to corruption and inefficient management by the government authorities ( De Châtel 2014 , 9). Similarly, the Lebanese government blamed climate change for the reduction of water flow in the Hasbani Basin, while civil society representatives accused the government of “systematically neglecting their concerns” about water access ( Mason and Khawlie 2016 , 1352–3).
Tensions over transboundary water sharing may continue to rise in the MENA region ( Bulloch and Darwish 1993 ; Amery 2002 ). The Euphrates River and Tigris River are important water sources for Turkey, Iraq, Syria, and Iran, and Turkey controls the water flow through the investment in the Southeastern Anatolia Project consisting of twenty-two large reservoirs and nineteen hydroelectric power stations on the upper tributaries of the Euphrates and Tigris Rivers. Karnieli et al. (2019 ) argue that Turkey’s transboundary investment and dam filling to be the primary driver of 2007–2008 droughts in Syria instead of climate change. This might be inconsequential because Turkey released additional water to Syria during the drought (see Kibaroglu and Scheumann 2011 , 297). As long as the downstream countries, Syria, Iraq, and Iran, see their domestic water problems to be attributed to the upstream dams in Turkey (e.g., Al-Muqdadi et al. 2016 ), transboundary rivers can be a source of interstate tension—although it is unlikely to develop into a full-scale armed conflict ( Bencala and Dabelko 2008 ). The impact of climate change in transboundary water governance is still an under-researched area that deserves more attention. Another area that can be a subject for further research is a growing sub-national competition over water such as brewing tension within Iraq due to the Kurdish Regional Government’s dam building plans ( Tinti 2023 ).
Existing evidence demonstrates that climate impacts, particularly droughts and drying trends, contribute to armed conflict in various ways. This section weighs in on the findings from the analysis to evaluate the overall framework of pathways to climate insecurity in the MENA region. The synthesis of findings highlights consensus and disagreement in existing studies and identifies the areas for further research.
Water scarcity in the MENA region is apparent at multiple scales, from domestic to transboundary, and has various implications for social vulnerability and political stability. The region’s water insecurity is as much driven by governance challenges as climatic and environmental trends. Severe droughts in the Levant during 2007–2009 appear to have led to the decline in agricultural production in the affected areas, but the drought vulnerability is mediated by groundwater availability, the viability of irrigation systems, and the capacity of water infrastructure ( Kelley et al. 2015 ). Decades of mismanagement of water resources and institutional failings undermine adaptive capacities in the region, demonstrated in examples from Lebanon, Yemen, Syria, and Iraq ( Weiss 2015 ; Mason and Khawlie 2016 ; Selby 2019 ; Mason 2022 ).
The depletion of groundwater in parts of the MENA region is largely attributed to the government’s unsustainable agricultural and water policies. Groundwater offers an important source of reserve during droughts, and the unsustainable use of groundwater adversely affects farmers’ drought vulnerability. Government subsidies on fuels encouraged farmers to install diesel pumps to use groundwater for irrigation, without consideration for sustainability in Yemen and Syria ( Weiss 2015 ; Selby 2019 ). These governments’ agricultural and economic policies resulted in farmers growing more water-intensive crops such as cotton and citrus fruits, which accelerated groundwater depletion. Political elites used fuel subsidies to ensure support from farmers at the expense of the environment. These unsustainable water and agricultural policies are not technical “mismanagement” but embedded in a much larger political context and ideology ( Daoudy 2021 , 13). Considering political factors in climate vulnerability is an important aspect to understand the climate-conflict nexus in the MENA region.
This analytic essay also looks into the important debate about the contribution of droughts in the Syrian uprising and subsequent civil war. Fourteen out of thirty-nine existing studies focus on the Syrian conflict and examine various linkages between the conflict and climate-related environmental factors. The popular narrative portrays the Syrian civil war as a climate conflict that is triggered by climate-induced agricultural collapse resulting in mass displacement ( Gleick 2014 ; Werrell, Femia, and Sternberg 2015 ). Research refutes this narrative by contesting the empirical foundations. Drought-displaced people in urban or peri-urban areas did not participate in street protests ( De Châtel 2014 ), and a significant proportion of the displaced returned to northern Syria before the revolution began ( Eklund and Thompson 2017 ; Eklund et al. 2022 ). Reviewing the literature demonstrates that attributing the onset of the Syrian civil war solely to climate change lacks empirical substantiation. Nevertheless, climate-related environmental changes, such as falling groundwater levels, have significant impact on natural resources and livelihoods, which can consequently undermine human and environment security.
Internal migration is more prominent than international migration in the research focusing on climate-induced mobility in the MENA region. This is similar to other studies with different regional focus (e.g., Burrows and Kinney 2016 ). The disruption of the rural livelihoods appears to be a strong push factor in Syria, which can be worsened by droughts ( Fröhlich 2016 ). Data on migration seem to be a challenge in unpacking this complex phenomenon. It is challenging to disentangle environmental changes from economic drivers in migration decision-making. Satellite-based data provide reasonable proxy measures for in- and out-migration in locations (e.g., Ash and Obradovich 2020 ), but they do not offer insights on who moved from where to where and why. More studies incorporating qualitative data are needed to further the understanding of climate-induced internal migration.
There is clear evidence that armed groups have escalated their tactics by weaponizing water in the MENA region. Several studies demonstrate how armed groups escalate their tactics by weaponizing water. Such a wartime trend indicates a heightened risk for civilians and long-term consequences by destructing key water infrastructures. This finding is highly policy relevant for strengthening and enforcing international laws for civilian protection during armed conflict (see Grech-Madin 2021 ). In relation to the armed group’s tactics, more research is needed to unpack the role of climate-related environmental factors in the armed group’s recruitment and tactical decisions.
The findings on differing vulnerability and gendered impacts on livelihoods are based on a handful of studies, and intersectional approaches are generally absent in most studies reviewed in the analytic essay. How climate shocks have varying impacts on people based on their gender, age, livelihoods, ethnicity, and combinations of these identities is missing. If marginalization and grievances are key processes of climate-induced conflict, how climate change affects different segments of the population differently needs better understanding.
The relationship between climate change and violent conflict is primarily indirect and varied, cautioning against generalized assumptions. How climate change influences the risk of violent conflict in the MENA region is mediated by political economy, institutional shortcomings, and elite competition. The risk of violent conflict is contingent on pre-existing negative socio-political relationships, types of political systems, and different climate vulnerabilities of various social groups. Gendered climate vulnerabilities need better understanding for establishing the linkage between climate vulnerability and insecurity. Carefully examining existing evidence is important for both over general climate security discussions as well as for the policy discussions on the MENA region, which has remained a focal point of scholarly and policy debates concerning climate security ( Daoudy, Sowers, and Weinthal 2022 , 7).
Disentangling specific climate impacts is also crucial for enhancing government’s climate adaptation and disaster mitigation policies in the MENA region. Civil society representatives from the MENA region have been concerned that states and political elites blame climate change to legitimize inequalities and to devoid accountability ( Selby et al. 2017 ; Kausch 2022 ). As existing research demonstrated, water and food insecurity in the region is driven by a lack of state capacity to properly manage natural resources and the integrity of public institutions in the MENA region.
Future research should pay attention to other types of climate hazards, including floods, heatwaves, and dust storms. Existing research primarily focuses on droughts and precipitation deficits, failing to account for heatwaves and flooding, which also are common in the MENA region. Floods are understudied despite their severe humanitarian impact. For instance, heavy flooding forced more than 84,000 people to displacement in Yemen, 13,000 people in Iran, and 5,000 people in northern Iraq in 2021 ( IDMC 2023 ). How flooding affects livelihood conditions and social vulnerability would be considerably different from droughts. Studies from other regions suggest floods are not associated with communal violence ( Petrova 2022 ). Ultra-heatwaves are likely to worsen without substantial government interventions ( Zittis et al. 2021 ), and their impact on oil exploitation, tourism, and urban areas demands more research. Oil and tourism industries are economic backbones of several MENA countries, and adverse impact on these sectors is likely lead to ripple effects on the society. A decrease in oil production due to extreme heatwaves and dust storms will affect public service provisions by the governments, which can be a source of instability as previous research points out (e.g., Mason 2022 ).
Future research should look at non-violent conflicts, especially protests linked to climate change in the MENA region. There is already a substantial debate on the role of food security in political stability, such as in the Arab Spring ( Werrell and Femia 2013 ; Schilling et al. 2020 ). And few studies focus on under what conditions droughts and floods can lead to non-violent conflicts such as political unrest and protests ( Ide, Kristensen, and Bartusevičius 2021 ; Ide et al. 2021 ). Youth climate activists in the region have demanded their respective governments to take proactive climate actions ( Altaeb 2022 ). Climate change is becoming a politically salient topic, and the MENA region’s civil society has voiced its concerns about the inaction and growing uncertainty about the future. How the region’s climate activism interacts with politics appears to be an important area for future research.
The narrative about climate change and conflict in the MENA region is shaped by both scientific projections but also a “long history of colonial and postcolonial scholarship invoking environmental determinism as an explanation for underdevelopment” ( Daoudy et al. 2022 , 7). This calls for more “open” and critical approaches in researching the climate-conflict nexus in the region. The evidence from existing studies shows that current water and food insecurity in the MENA region are outcomes of domestic politics and institutional shortcomings rather than past climate change. This highlights the importance of governance reforms for enhancing adaptative capacity in the region ( Sowers et al. 2011 ). Improved understanding of how vulnerability to climate change interacts with political systems, institutions, and social relations can inform policy development. This enhanced understanding can equip relevant stakeholders to more effectively anticipate, prevent, and respond to the intricate web of risks entwining climate change and violent conflict, while concurrently enhancing resilience-building efforts.
We adopt SIPRI’s definition of the MENA region, which includes Bahrain, Egypt, Iran, Iraq, Israel, Jordan, Kuwait, Lebanon, Oman, Palestine, Qatar, Saudi Arabia, Syria, Turkey, the United Arab Emirates (UAE), North Yemen (–1990), South Yemen (–1990) and Yemen; (NA) Algeria, Libya, Morocco, and Tunisia. See “Regional coverage,” See SIPRI databases at https://www.sipri.org/databases/regional-coverage .
The search string was the following: AB=((climat* OR "climat* change" OR "climat* variability" OR rainfall OR precipitation OR drought OR "water scarcity" OR "land degradation" OR weather OR disaster OR temperature OR warming OR "sea level rise" OR desertification OR famine OR “soil erosion” OR flood*) AND (conflict OR jihad* OR armed OR insurgen* OR rebel* OR terror* OR violen* OR war) AND ("middle east*" OR “north africa*” OR MENA OR algeria OR bahrain OR egypt OR iran OR Iraq OR israel OR jordan OR kuwait OR lebanon OR libya OR morocco OR oman OR palestin* OR qatar OR “saudi arabia” OR syria OR tunisia OR “united arab emirates” OR yemen OR “western sahara”)).
Here, we use SIPRI’s definition of the MENA region, which includes Bahrain, Egypt, Iran, Iraq, Israel, Jordan, Kuwait, Lebanon, Oman, Palestine, Qatar, Saudi Arabia, Syria, Turkey, the United Arab Emirates (UAE), North Yemen (–1990), South Yemen (–1990) and Yemen; (NA) Algeria, Libya, Morocco, and Tunisia.
Author’s note : This work is supported by funding from the Swedish Ministry for Foreign Affairs as part of SIPRI’s Climate Change and Security Project and the Norwegian Ministry of Foreign Affairs for SIPRI’s Climate-Related Security and Development Risks Project. We would like to thank two anonymous reviewers for their constructive feedback for improving the manuscript. We are indebted to Florian Krampe, Farah Hegazi, and Kheira Tarif for their helpful comments throughout the writing process.
Abel Guy J. , Brottrager Michael , Cuaresma Jesus Crespo , Muttarak Raya . 2019 . “ Climate, Conflict and Forced Migration .” Global Environmental Change—Human and Policy Dimensions . 54 : 239 – 49 .
Google Scholar
Abrahams Daniel. 2020 . “ Conflict in Abundance and Peacebuilding in Scarcity: Challenges and Opportunities in Addressing Climate Change and Conflict .” World Development . 132 : 104998 .
Abroulaye Sanfo , Issa Savadogo , Abalo Kulo E , Nouhoun Zampaligre . “ 2015 . ” Climate Change: A Driver of Crop Farmers-Agro Pastoralists Conflicts in Burkina Faso . International Journal of Applied Science and Technology . 5 : 92 – 104 .
Almazroui Mansour , Saeed Fahad , Saeed Sajjad , Islam M. Nazrul , Ismail Muhammad , Klutse Nana Ama Browne , Siddiqui Muhammad Haroon . 2020 . “ Projected Change in Temperature and Precipitation Over Africa from CMIP6 .” Earth Systems and Environment . 4 : 455 – 75 .
Al-Muqdadi Sameh W. , Omer Mohammed F. , Abo Rudy , Naghshineh Alice . 2016 . “ Dispute over Water Resource Management—Iraq and Turkey .” Journal of Environmental Protection . 7 : 1096 – 103 .
Alqatabry Hameed , Butcher Charity . 2020 . “ Humanitarian Aid in Yemen: Collaboration or Co-Optation? .” Journal of Peacebuilding & Development . 15 : 250 – 5 .
Altaeb Malak. 2022 . A Silenced MENA Youth Climate Activism Under COP 27 . The Tahrir Institute for Middle East Policy . Accessed March 8, 2023. https://timep.org/2022/11/09/a-silenced-mena-youth-climate-activism-under-cop-27/ .
Amery H.A. 2002 . “ Water Wars in the Middle East: A Looming Threat .” Geographical Journal . 168 : 313 – 23 .
Ash Konstantin , Obradovich Nick . 2020 . “ Climatic Stress, Internal Migration, and Syrian Civil War Onset .” Journal of Conflict Resolution . 64 : 3 – 31 .
Balsari Satchit , Dresser Caleb , Leaning Jennifer . 2020 . “ Climate Change, Migration, and Civil Strife .” Current Environmental Health Reports . 7 : 404 – 14 .
Barnett Jon , Neil Adger W . 2007 . “ Climate Change, Human Security and Violent Conflict .” Political Geography . 26 : 639 – 55 .
Belge Ceren , Karakoç Ekrem . 2015 . “ Minorities in the Middle East: Ethnicity, Religion, and Support for Authoritarianism .” Political Research Quarterly . 68 : 280 – 92 .
Bencala Karin R. , Dabelko Geoffrey D. . 2008 . “ Water Wars: Obscuring Opportunities .” Journal of International Affairs . 61 : 21 .
Benjaminsen Tor A. , Ba Boubacar . 2019 . “ Why Do Pastoralists in Mali Join Jihadist Groups? A Political Ecological Explanation .” The Journal of Peasant Studies . 46 : 1 – 20 .
Bijani Masoud , Hayati Dariush , Azadi Hossein , Tanaskovik Vjekoslav , Witlox Frank . 2020 . “ Causes and Consequences of the Conflict among Agricultural Water Beneficiaries in Iran .” Sustainability . 12 ( 16 ): 6630 .
Black Richard , Busby Joshua , Dabelko Geoffrey D. , de Coning Cedric , Maalim Hafsa , McAllister Claire , Ndiloseh Melvis , et al. 2022 . Environment of Peace: Security in a New Era of Risk . Stockholm : Stockholm International Peace Research Institute . Accessed April 29, 2022. https://www.sipri.org/publications/2022/other-publications/environment-peace-security-new-era-risk .
Google Preview
Borghesi Simone , Ticci Elisa . 2019 . “ Climate Change in the MENA Region: Environmental Risks, Socioeconomic Effects and Policy Challenges for the Future .” In Midterranean Yearbook , Barcelona : European Institute of the Mediterranean .
Brosché Johan , Elfversson Emma . 2012 . “ Communal Conflict, Civil War, and the State: Complexities, Connections, and the Case of Sudan .” African Journal on Conflict Resolution . 12 ( 1 ): 33 – 60 .
Brzoska Michael , Fröhlich Christiane . 2016 . “ Climate Change, Migration and Violent Conflict: Vulnerabilities, Pathways and Adaptation Strategies .” Migration and Development . 5 : 190 – 210 .
Bulloch John , Darwish Adel . 1993 . Water Wars: Coming Conflicts in the Middle East . London : St Dedmundsbury Press .
Burrows Kate , Kinney Patrick . 2016 . “ Exploring the Climate Change, Migration and Conflict Nexus .” International Journal of Environmental Research and Public Health . 13 : 442 – 59 .
Busby Joshua W. 2022 . States and Nature: The Effects of Climate Change on Security . 1st ed. Cambridge : Cambridge University Press . Accessed April 11, 2022. https://www.cambridge.org/core/product/identifier/9781108957922/type/book .
Chavunduka Charles , Bromley Daniel W. . 2011 . “ Climate, Carbon, Civil War and Flexible Boundaries: Sudan’s Contested Landscape .” Land Use Policy . 28 : 907 – 16 .
“Climate Wars - Syria” with Thomas Friedman . 2017 . Accessed August 8, 2023. https://www.youtube.com/watch?v=i31v1z–3Z8 .
Cottier Fabien , Salehyan Idean . 2021 . “ Climate Variability and Irregular Migration to the European Union .” Global Environmental Change . 69 : 102275 .
Dacombe Rod. 2018 . “ Systematic Reviews in Political Science: What Can the Approach Contribute to Political Research? ” Political Studies Review . 16 : 148 – 57 .
Daoudy Marwa. 2020a . The Origins of the Syrian Conflict: Climate Change and Human Security . 1st ed. Cambridge : Cambridge University Press . Accessed June 3, 2022. https://www.cambridge.org/core/product/identifier/9781108567053/type/book .
Daoudy Marwa. . 2020b . “ Water Weaponization in the Syrian Conflict: Strategies of Domination and Cooperation .” International Affairs . 96 : 1347 – 66 .
Daoudy Marwa. . 2021 . “ Rethinking the Climate–Conflict Nexus: A Human–Environmental–Climate Security Approach .” Global Environmental Politics . 21 ( 3 ): 4 – 25 .
Daoudy Marwa , Sowers Jeannie , Weinthal Erika . 2022 . “ What Is Climate Security? Framing Risks around Water, Food, and Migration in the Middle East and North Africa .” WIREs Water . 9 ( 3 ): e1582 . Accessed February 20, 2023. https://onlinelibrary.wiley.com/doi/10.1002/wat2.1582 .
De Châtel Francesca. 2014 . “ The Role of Drought and Climate Change in the Syrian Uprising: Untangling the Triggers of the Revolution .” Middle Eastern Studies . 50 : 521 – 35 .
Denton Fatma. 2002 . “ Climate Change Vulnerability, Impacts, and Adaptation: Why Does Gender Matter? ” Gender & Development . 10 : 10 – 20 .
Denyer David , Tranfield David , eds. 2009 . “ Producing a Systematic Review .” In The Sage Handbook of Organizational Research Methods . Thousand Oaks, CA : Sage Publications Ltd .
Detges Adrien. 2014 . “ Close-Up on Renewable Resources and Armed Conflict: The Spatial Logic of Pastoralist Violence in Northern Kenya .” Political Geography . 42 : 57 – 65 .
Doocy Shannon , Lyles Emily . 2018 . “ Humanitarian Needs in Government Controlled Areas of Syria .” PLoS Currents . Accessed January 24, 2020. http://currents.plos.org/disasters/?p=35351 .
Döring Stefan. 2020 . “ Come Rain, or Come Wells: How Access to Groundwater Affects Communal Violence .” Political Geography . 76 : 102073 .
Eklund Lina , Theisen Ole Magnus , Baumann Matthias , Tollefsen Andreas Forø , Kuemmerle Tobias , Nielsen Jonas Østergaard . 2022 . “ Societal Drought Vulnerability and the Syrian Climate-Conflict Nexus Are Better Explained by Agriculture than Meteorology .” Communications Earth & Environment . 3 : 85 .
Eklund Lina , Thompson Darcy . 2017 . “ Differences in Resource Management Affects Drought Vulnerability across the Borders between Iraq, Syria, and Turkey .” Ecology and Society . 22 ( 4 ): 11 .
Feitelson Eran , Tubi Amit . 2017 . “ A Main Driver or an Intermediate Variable? Climate Change, Water and Security in the Middle East .” Global Environmental Change . 44 : 39 – 48 .
Feizi Mehdi , Heidarzadeh Janatabadi Najmeh , Torshizi Ahmad Saradari . 2019 . “ Rainfall and Social Disputes in Iran .” Water Policy . 21 : 880 – 93 .
Fröhlich Christiane J. 2016 . “ Climate Migrants as Protestors? Dispelling Misconceptions about Global Environmental Change in Pre-Revolutionary Syria .” Contemporary Levant . 1 : 38 – 50 .
Gaub Florence , Lienard Clémentine . 2021 . Arab Climte Future: Of Risks and Readiness . LU: Publications Office .
Gleditsch Nils Petter . 1998 . “ Armed Conflict and the Environment: A Critique of the Literature .” Journal of Peace Research . 35 : 381 – 400 .
Gleditsch Nils Petter . 2012 . “ Whither the Weather? Climate Change and Conflict .” Journal of Peace Research . 49 : 3 – 9 .
Gleick Peter H. 2014 . “ Water, Drought, Climate Change, and Conflict in Syria .” Weather, Climate, and Society . 6 : 331 – 40 .
Gleick Peter H. . 2019 . “ Water as a Weapon and Casualty of Armed Conflict: A Review of Recent Water-Related Violence in Iraq, Syria, and Yemen .” WIREs Water . 6 ( 4 ): e1351 . Accessed November 16, 2021. https://onlinelibrary.wiley.com/doi/10.1002/wat2.1351 .
Grech-Madin Charlotte. 2020 . “ The Water Taboo: Restraining the Weaponisation of Water in International Conflict .” PhD Dissertation . Uppsala University .
Grech-Madin Charlotte. . 2021 . “ Water and Warfare: The Evolution and Operation of the Water Taboo .” International Security . 45 : 84 – 125 .
Haddad Bassam. 2012 . Business Networks in Syria: The Political Economy of Authoritarian Resilience . Stanford, CA : Stanford University Press .
Helman David , Zaitchik Benjamin F. . 2020 . “ Temperature Anomalies Affect Violent Conflicts in African and Middle Eastern Warm Regions .” Global Environmental Change—Human and Policy Dimensions . 63 : 102118 .
Helman David , Zaitchik Benjamin F. , Funk Chris . 2020 . “ Climate Has Contrasting Direct and Indirect Effects on Armed Conflicts .” Environmental Research Letters . 15 : 104017 .
Hendrix Cullen S. , Koubi Vally , Selby Jan , Siddiqi Ayesha , von Uexkull Nina . 2023 . “ Climate Change and Conflict .” Nature Reviews Earth & Environment . 4 : 144 – 8 .
Herb Michael. 1999 . All in the Family: Absolutism, Revolution, and Democracy in the Middle Eastern Monarchies . Albany : State University of New York Press .
Human Rights Watch . 2019 . “ Basra Is Thirsty: Iraq’s Failure to Manage the Water Crisis .” Human Rights Watch . Accessed November 23, 2022. https://www.hrw.org/report/2019/07/22/basra-thirsty/iraqs-failure-manage-water-crisis .
Ide Tobias. 2018 . “ Climate War in the Middle East? Drought, the Syrian Civil War and the State of Climate-Conflict Research .” Current Climate Change Reports . 4 : 347 – 54 .
Ide Tobias , Kristensen Anders , Bartusevičius Henrikas . 2021 . “ First Comes the River, Then Comes the Conflict? A Qualitative Comparative Analysis of Flood-Related Political Unrest .” Journal of Peace Research . 58 : 83 – 97 .
Ide Tobias , Lopez Miguel Rodriguez , Fröhlich Christiane , Scheffran Jürgen . 2021 . “ Pathways to Water Conflict during Drought in the MENA Region .” Journal of Peace Research . 58 : 568 – 82 .
IDMC . 2023 . “ Global Internal Displacement Dataset .” IDMC . Accessed March 23, 2023. https://www.internal-displacement.org/home .
IPCC . 2022 . "Summary for Policymakers." in Climate Change 2022: Impacts, Adaptation and Vulnerability , edited by Pörtner H.-O. , Roberts D.C. , Tignor M. , Poloczanska E.S. , Mintenbeck K. , Alegría A. , Craig M. , Langsdorf S. , Löschke S. , Möller V. , Okem A. , Rama B. . London; New York : Cambridge University Press .
Karnieli Arnon , Shtein Alexandra , Panov Natalya , Weisbrod Noam , Tal Alon . 2019 . “ Was Drought Really the Trigger Behind the Syrian Civil War in 2011? ” Water . 11 : 1564 .
Kausch Kristina. 2022 . “ Middle Eastern Civil Society’s Struggles With the Primacy of Geopolitics—Global Civil Society in a Geopolitical Age: How Great Power Competition Is Reshaping Civic Activism .” Carnegie Europe . Accessed March 23, 2023. https://carnegieeurope.eu/2022/11/30/middle-eastern-civil-society-s-struggles-with-primacy-of-geopolitics-pub-88490 .
Kelley Colin P. , Mohtadi Shahrzad , Cane Mark A. , Seager Richard , Kushnir Yochanan . 2015 . “ Climate Change in the Fertile Crescent and Implications of the Recent Syrian Drought .” Proceedings of the National Academy of Sciences of the United States of America . 112 : 3241 – 6 .
Keshavarz Marzieh , Karami Ezatollah , Vanclay Frank . 2013 . “ The Social Experience of Drought in Rural Iran .” Land Use Policy . 30 : 120 – 9 .
Kibaroglu Aysegul , Scheumann Waltina . 2011 . “ Euphrates-Tigris Rivers System: Political Rapprochement and Transboundary Water Cooperation .” In Turkey’s Water Policy , edited by Kramer Annika , Kibaroglu Aysegul , Scheumann Waltina . Berlin : Springer . Accessed June 3, 2022. http://link.springer.com/10.1007/978-3-642-19636-2_16 .
Kim Kyungmee , Swain Ashok . 2017 . “ Crime, Corruption, Terrorism and Beyond: A Typology of Water Crime .” In The Human Face of Water Security , edited by Devlaeminck D. , Adeel Z. , Sandford R. . New York : Springer .
King Marcus DuBois . 2015 . “ The Weaponization of Water in Syria and Iraq .” The Washington Quarterly . 38 : 153 – 69 .
Linke Andrew M , Ruether Brett . 2021 . “ Weather, Wheat, and War: Security Implications of Climate Variability for Conflict in Syria .” Journal of Peace Research . 58 : 114 – 31 .
Mason Michael. 2022 . “ Infrastructure under Pressure: Water Management and State-Making in Southern Iraq .” Geoforum . 132 : 52 – 61 .
Mason Michael , Khawlie Mohamad . 2016 . “ Fluid Sovereignty: State-Nature Relations in the Hasbani Basin, Southern Lebanon .” Annals of the American Association of Geographers . 106 : 1344 – 59 .
Meierding Emily. 2013 . “ Climate Change and Conflict: Avoiding Small Talk about the Weather .” International Studies Review . 15 : 185 – 203 .
Miller Brandon. 2015 . “ Is the Syrian Conflict Linked to Climate Change? ” CNN . Accessed August 8, 2023. https://www.cnn.com/2015/11/23/world/is-the-syrian-conflict-linked-to-climate-change/index.html .
Mobjörk Malin , Krampe Florian , Tarif Kheira . 2020 . Pathways of Climate Insecurity: Guidance for Policymakers . Stockholm : Stockholm International Peace Research Institute . Policy Brief .
Mohamed Mohamed Ali , Anders Julian , Schneider Christoph . 2020 . “ Monitoring of Changes in Land Use/Land Cover in Syria from 2010 to 2018 Using Multitemporal Landsat Imagery and GIS .” Land . 9 : 226 .
Mohammed Ali Ibrahim Mustafa . 2019 . “ The Ecological, Socio-Economic and Political Constraints on Pastoralists’ Access to Water, Blue Nile State (Sudan) .” Nomadic Peoples . 23 : 282 – 302 .
Morales-Muñoz Héctor , Bailey Arwen , Löhr Katharina , Caroli Giulia , Villarino Ma. Eliza J. , LoboGuerrero Ana María , Bonatti Michelle , et al. 2022 . “ Co-Benefits Through Coordination of Climate Action and Peacebuilding: A System Dynamics Model .” Journal of Peacebuilding & Development . 17 : 304 – 23 .
Namdar Razieh , Karami Ezatollah , Keshavarz Marzieh . 2021 . “ Climate Change and Vulnerability: The Case of MENA Countries .” ISPRS International Journal of Geo-Information . 10 : 794 .
Nordqvist Pernilla , Krampe Florian . 2018 . Climate Change and Violent Conflict: Sparse Evidence from South Asia and South East Asia .Stockholm: Stockholm International Peace Research Institute. SIPRI Insight for Peace and Security.
OCHA . 2009 . Syria Drought Response Plan . Damascus : United Nations .
O'Hagan Ellie Mae . 2015 . “ Mass Migration Is No ‘Crisis’: It's the New Normal as the Climate Changes .” The Guardian . Accessed February 6, 2023. https://www.theguardian.com/commentisfree/2015/aug/18/mass-migration-crisis-refugees-climate-change .
Palik Júlia , Aas Rustad Siri , Berg Harpviken Kristian , Methi Fredrik . 2020 . 35 Conflict Trends in the Middle East . Oslo : Prio . Prio Paper .
Petrova Kristina. 2022 . “ Floods, Communal Conflict and the Role of Local State Institutions in Sub-Saharan Africa .” Political Geography . 92 : 102511 .
Post Riley , Hudson Darren , Mitchell Donna , Bell Patrick , Perliger Arie , Williams Ryan . 2016 . “ Rethinking the Water-Food-Climate Nexus and Conflict: An Opportunity Cost Approach .” Applied Economic Perspectives and Policy . 38 : 563 – 77 .
Rüttinger Lukas , Smith Dan , Stang Gerald , Tänzler Dennis , Vivekananda Janani . 2015 . A New Climate for Peace: Taking Action on Climate and Fragility Risks . Berlin : Adelphi; International Alert; Woodrow Wilson International Center for Scholars; European Union Institute for Security Studies . Accessed February 24, 2021. https://climate-diplomacy.org/sites/default/files/2020-11/NewClimateForPeace_FullReport_small_0.pdf .
Sakaguchi Kendra , Varughese Anil , Auld Graeme . 2017 . “ Climate Wars? A Systematic Review of Empirical Analyses on the Links between Climate Change and Violent Conflict .” International Studies Review . 19 : 622 – 45 .
Schilling Janpeter , Hertig Elke , Tramblay Yves , Scheffran Jürgen . 2020 . “ Climate Change Vulnerability, Water Resources and Social Implications in North Africa .” Regional Environmental Change . 20 : 15 .
Schmidt Matthias , Pearson Olivia . 2016 . “ Pastoral Livelihoods under Pressure: Ecological, Political and Socioeconomic Transitions in Afar (Ethiopia) .” Journal of Arid Environments . 124 : 22 – 30 .
Selby Jan. 2019 . “ Climate Change and the Syrian Civil War, Part II: The Jazira’s Agrarian Crisis .” Geoforum . 101 : 260 – 74 .
Selby Jan , Dahi Omar S. , Fröhlich Christiane , Hulme Mike . 2017 . “ Climate Change and the Syrian Civil War Revisited .” Political Geography . 60 : 232 – 44 .
Seter Hanne. 2016 . “ Connecting Climate Variability and Conflict: Implications for Empirical Testing .” Political Geography . 53 : 1 – 9 .
Sieghart Lia Carol , Betre Mahlette . 2018 . Challenges and Opportunities for the World’s Most Water Stressed Region . Washington, DC : World Bank . Quick Note Series .
Smith Dan , Krampe Florian . 2019 . “ Climate-Related Security Risks in the Middle East .” In Routledge Handbook on Middle East Security , edited by Jägerskog A. , Schulz M. , Swain A. . London : Routledge .
Sofuoglu Emrah , Ay Ahmet . 2020 . “ The Relationship between Climate Change and Political Instability: The Case of MENA Countries (1985:01–2016:12) .” Environmental Science and Pollution Research . 27 : 14033 – 43 .
Sowers Jeannie , Vengosh Avner , Weinthal Erika . 2011 . “ Climate Change, Water Resources, and the Politics of Adaptation in the Middle East and North Africa .” Climatic Change . 104 : 599 – 627 .
Sowers Jeannie L , Weinthal Erika , Zawahri Neda . 2017 . “ Targeting Environmental Infrastructures, International Law, and Civilians in the New Middle Eastern Wars .” Security Dialogue . 48 : 410 – 30 .
Tanchum Michaël. 2021 . The Fragile State of Food Security in the Maghreb: Implication of the 2021 Cereal Grains Crisis in Tunisia, Algeria, and Morocco . Washington, DC : The Middle East Instisute .
Tarif Kheira. 2022 . Climate Change and Violent Conflict in West Africa: Assessing the Evidence . Stockholm International Peace Research Institute . Accessed April 4, 2022. https://www.sipri.org/publications/2022/sipri-insights-peace-and-security/climate-change-and-violent-conflict-west-africa-assessing-evidence .
Thomas Kimberley , Dean Hardy R. , Lazrus Heather , Mendez Michael , Orlove Ben , Rivera-Collazo Isabel , Timmons Roberts J. , et al. 2019 . “ Explaining Differential Vulnerability to Climate Change: A Social Science Review .” WIREs Climate Change . 10 : e565 .
Tinti Alessandro. 2023 . “ Scales of Justice. Large Dams and Water Rights in the Tigris–Euphrates Basin .” Policy and Society . 42 ( 2 ): 184 – 96 .
Tubi Amit , Feitelson Eran . 2016 . “ Drought and Cooperation in a Conflict Prone Area: Bedouin Herders and Jewish Farmers in Israel’s Northern Negev, 1957–1963 .” Political Geography . 51 : 30 – 42 .
von Lossow Tobias. 2016 . “ The Rebirth of Water as a Weapon: IS in Syria and Iraq .” The International Spectator . 51 : 82 – 99 .
von Uexkull Nina. 2014 . “ Sustained Drought, Vulnerability and Civil Conflict in Sub-Saharan Africa .” Political Geography . 43 : 16 – 26 .
von Uexkull Nina , Croicu Mihai , Fjelde Hanne , Buhaug Halvard . 2016 . “ Civil Conflict Sensitivity to Growing-Season Drought .” Proceedings of the National Academy of Sciences . 113 : 12391 – 6 .
Uson Maria , Angelina M. 2017 . “ Natural Disasters and Land Grabs: The Politics of Their Intersection in the Philippines Following Super Typhoon Haiyan .” Canadian Journal of Development Studies/Revue canadienne d’études du développement . 38 : 414 – 30 .
Van Baalen Sebastian , Mobjörk Malin . 2018 . “ Climate Change and Violent Conflict in East Africa: Integrating Qualitative and Quantitative Research to Probe the Mechanisms .” International Studies Review . 20 : 547 – 75 .
Verhoeven Harry. 2011 . “ Climate Change, Conflict and Development in Sudan: Global Neo-Malthusian Narratives and Local Power Struggles: Climate Change, Conflict and Development in Sudan .” Development and Change . 42 : 679 – 707 ..
VICE . 2017 . “ Assad’s Syria & The Cost of Climate Change .” Video . Accessed August 8, 2023. https://video.vice.com/en_us/video/hbo-assad-syria-climate-change-cost/58ac53d2d081c04e5f9e37b0 .
Waha Katharina , Krummenauer Linda , Adams Sophie , Aich Valentin , Baarsch Florent , Coumou Dim , Fader Marianela , et al. 2017 . “ Climate Change Impacts in the Middle East and Northern Africa (MENA) Region and Their Implications for Vulnerable Population Groups .” Regional Environmental Change . 17 : 1623 – 38 .
Weinthal Erika , Zawahri Neda , Sowers Jeannie . 2015 . “ Securitizing Water, Climate, and Migration in Israel, Jordan, and Syria .” International Environmental Agreements: Politics, Law and Economics . 15 : 293 – 307 .
Weiss Matthew I. 2015 . “ A Perfect Storm: The Causes and Consequences of Severe Water Scarcity, Institutional Breakdown and Conflict in Yemen .” Water International . 40 : 251 – 72 .
Werrell Caitlin E. , Femia Francesco . 2013 . The Arab Spring and Climate Change . Washington, DC : Center for American Progress .
Werrell Caitlin E. , Femia Francesco , Sternberg Troy . 2015 . “ Did We See It Coming?: State Fragility, Climate Vulnerability, and the Uprisings in Syria and Egypt .” SAIS Review of International Affairs . 35 : 29 – 46 .
World Bank . 2022 . “ Population, Total - Middle East & North Africa .” The World Bank . Accessed November 5, 2021. https://data.worldbank.org/indicator/SP.POP.TOTL?locations=ZQ .
World Bank . 2023 . “ Employment in Agriculture, Female (% of Male Employment) (Modeled ILO Estimate) .” Accessed March 23, 2023. https://data.worldbank.org/indicator/SL.AGR.EMPL.MA.ZS?view=chart .
Yiftachel Oren. 1996 . “ The Internal Frontier: Territorial Control and Ethnic Relations in Israel .” Regional Studies . 30 : 493 – 508 .
Zaman M.Q. 1991 . “ Social Structure and Process in Char Land Settlement in the Brahmaputra–Jamuna Floodplain .” Man . 26 : 673 .
Zittis George , Hadjinicolaou Panos , Almazroui Mansour , Bucchignani Edoardo , Driouech Fatima , El Rhaz Khalid , Kurnaz Levent , et al. 2021 . “ Business-as-Usual Will Lead to Super and Ultra-Extreme Heatwaves in the Middle East and North Africa .” NPJ Climate and Atmospheric Science . 4 : 20 .
Zittis George , Hadjinicolaou Panos , Klangidou Marina , Proestos Yiannis , Lelieveld Jos . 2019 . “ A Multi-Model, Multi-Scenario, and Multi-Domain Analysis of Regional Climate Projections for the Mediterranean .” Regional Environmental Change . 19 : 2621 – 35 .
Email alerts
Citing articles via.
- Recommend to your Library
Affiliations
- Online ISSN 1468-2486
- Print ISSN 1521-9488
- Copyright © 2024 International Studies Association
- About Oxford Academic
- Publish journals with us
- University press partners
- What we publish
- New features
- Open access
- Institutional account management
- Rights and permissions
- Get help with access
- Accessibility
- Advertising
- Media enquiries
- Oxford University Press
- Oxford Languages
- University of Oxford
Oxford University Press is a department of the University of Oxford. It furthers the University's objective of excellence in research, scholarship, and education by publishing worldwide
- Copyright © 2024 Oxford University Press
- Cookie settings
- Cookie policy
- Privacy policy
- Legal notice
This Feature Is Available To Subscribers Only
Sign In or Create an Account
This PDF is available to Subscribers Only
For full access to this pdf, sign in to an existing account, or purchase an annual subscription.
- Open access
- Published: 01 December 2021
Feature selection revisited in the single-cell era
- Pengyi Yang ORCID: orcid.org/0000-0003-1098-3138 1 , 2 , 3 ,
- Hao Huang 1 , 2 &
- Chunlei Liu 2
Genome Biology volume 22 , Article number: 321 ( 2021 ) Cite this article
10k Accesses
30 Citations
31 Altmetric
Metrics details
Recent advances in single-cell biotechnologies have resulted in high-dimensional datasets with increased complexity, making feature selection an essential technique for single-cell data analysis. Here, we revisit feature selection techniques and summarise recent developments. We review their application to a range of single-cell data types generated from traditional cytometry and imaging technologies and the latest array of single-cell omics technologies. We highlight some of the challenges and future directions and finally consider their scalability and make general recommendations on each type of feature selection method. We hope this review stimulates future research and application of feature selection in the single-cell era.
Introduction
High-throughput biotechnologies are at the centre of modern molecular biology, where typically a sheer number of biomolecules are measured in cells and tissues. While significantly higher coverage of molecules is achieved by high-throughput biotechnologies compared to traditional biochemical assays, the variation in sample quality, reagents and workflow introduces profound technical variation in the data. The high dimensionality, redundancy and noise commonly found in these large-scale molecular datasets create significant challenges in their analysis and can lead to a reduction in model generalisability and reliability. Feature selection, a class of computational techniques for data analytics and machine learning, is at the forefront in dealing with these challenges and has been an essential driving force in a wide range of bioinformatics applications [ 1 ].
Until recently, the global molecular signatures generated from most high-throughput biotechnologies have been the average profiles of mixed populations of cells from tissues, organs or patients, and feature selection techniques have been predominately applied to such ‘bulk’ data. However, the recent development of technologies that enables the profiling of various molecules (e.g. DNA, RNA, protein) in individual cells at the omics scale has revolutionised our ability to study various molecular programs and cellular processes at the single-cell resolution [ 2 ]. The accumulation of large-scale and high-dimensional single-cell data has seen renewed interests in developing and the need for applying feature selection techniques to such data given their increased scale and complexity compared to their bulk counterparts.
To foster research in feature selection in the new era of single-cell sciences, we set out to revisit the feature selection literature, summarise its advancement in the last decade and recent development in the field of deep learning and review its current applications in various single-cell data types. We then discuss some key challenges and opportunities that we hope would inspire future research and development in this fast-growing interdisciplinary field. Finally, we consider the scalability and applicability of each type of feature selection methods and make general recommendations to their usage.
Basics of feature selection techniques
Feature selection refers to a class of computational methods where the aim is to select a subset of useful features from the original feature set in a dataset. When dealing with high-dimensional data, feature selection is an effective strategy to reduce the feature dimension and redundancy and can alleviate issues such as model overfitting in downstream analysis. Different from dimension reduction methods (e.g. principal component analysis) where features in a dataset are combined and/or transformed to derive a lower feature dimension, feature selection methods do not alter the original features in the dataset but only identify and select features that satisfy certain pre-defined criteria or optimise certain computational procedures [ 3 ]. The application of feature selection in bioinformatics is widespread [ 1 ]. Some of the most popular research directions include selecting genes that can discriminate complex diseases such as cancers from microarray data [ 4 , 5 ], selecting protein markers that can be used for disease diagnosis and prognostic prediction from mass spectrometry-based proteomics data [ 6 ], identifying single nucleotide polymorphisms (SNPs) and their interactions that are associated with specific phenotypes or diseases in genome-wide association studies (GWAS) [ 7 ], selecting epigenetic features that mark cancer subtypes [ 8 ] and selecting DNA structural properties for predicting genomic regulatory elements [ 9 ]. Traditionally, feature selection techniques fall into one of the three categories including filters, wrappers and embedded methods (Fig. 1 ). In this section, we revisit the key properties and defining characteristics of the three categories of feature selection methods. Please refer to [ 10 ] for a comprehensive survey of feature selection methods.
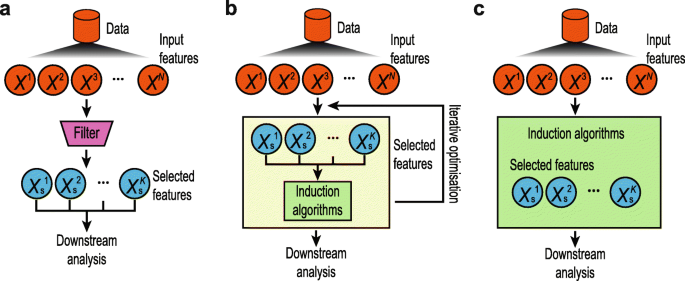
Schematic illustrations of typical filter ( a ), wrapper ( b ) and embedded methods ( c ) in feature selection
Filter methods typically rank the features based on certain criteria that may facilitate other subsequent analyses (e.g. discriminating samples) and select those that pass a threshold judged by the filtering criteria (Fig. 1 A). In bioinformatics applications, commonly used criteria are univariate methods such as t statistics, on which most ‘differential expression’ (DE) methods for biological data analysis are built [ 11 ], and multivariate methods that take into account relationships among features [ 12 ]. The main advantages of filter methods lie in their simplicity, requiring less computational resources in general and ease of applications in practice [ 13 ]. However, filter methods typically select features independent from the induction algorithms (e.g. classification algorithms) that are applied for downstream analyses, and therefore, the selected features may not be optimal with respect to the induction algorithms in the subsequent applications.
In comparison, wrappers utilise the performance of the induction algorithms to guide the feature selection process and therefore may lead to features that are more conducive to the induction algorithm used for optimisation in downstream analyses [ 14 ] (Fig. 1 B). A key aspect of wrapper methods is the design of the feature optimisation algorithms that maximise the performance of the induction algorithms. Since the feature dimensions are typically very high in bioinformatics applications, exhaustive search is often impractical. To this end, various greedy algorithms, such as forward and backward selection [ 15 ], and nature-inspired algorithms, such as the genetic algorithm (GA) [ 16 ] and the particles swarm optimisation (PSO) [ 17 ], were employed to speed up the optimisation and feature selection processes. Nevertheless, since the induction algorithms are included to iteratively evaluate feature subsets, wrappers are typically computationally intensive compared to filter methods.
While filters and wrappers separate feature selection from downstream analysis, embedded methods typically perform feature selection as part of the induction algorithm itself [ 18 ] (Fig. 1 C). Akin to wrappers, embedded methods optimise selected features with respect to an induction model and therefore may lead to more suited features for the induction algorithm in subsequent tasks such as sample classification. Since the embedded methods perform feature selection and induction simultaneously, it is also generally more computationally efficient than wrapper methods albeit less so when compared to filter methods [ 19 ]. Nevertheless, as feature selection is part of the induction algorithm in embedded methods, they are often specific to the algorithmic design and less generic compared to filters and wrappers. Popular choices of embedded methods in bioinformatics applications include tree-based methods [ 20 , 21 ] and shrinkage-based methods such as LASSO [ 22 ].
Advance of feature selection in the past decade
Besides the astonishing increase in the number of feature selection techniques in the last decade, we have also seen a few notable trends in their development. Here, we summarise three aspects that have shown proliferating research in various fields and applications, including bioinformatics.
First, a variety of approaches have been proposed for ensemble feature selection, including those for filters [ 23 , 24 ], wrappers [ 25 ] and embedded methods such as tree-based ensembles [ 26 ]. Ensemble learning is a well-established approach where instead of building a single model, multiple ‘base’ models are combined to perform tasks [ 27 ]. Supervised ensemble classification models are popular among bioinformatics applications [ 28 ] and have recently seen their increasing integration with deep learning models [ 29 ]. Similar to their counterpart in supervised learning, ensemble feature selection methods, typically, rely on either perturbation to the dataset or hyperparameters of the feature selection algorithms for creating ‘base selectors’ from which the ensemble could be derived [ 30 ]. Examples include using different subsets of samples for creating multiple filters or using different learning parameters in an induction algorithm of a wrapper method. Key attributes of ensemble feature selection methods are that they generally achieve better generalisability in sample classification [ 31 ] and higher reproducibility in feature selection [ 32 , 33 ]. Although these improvements in performance typically come with a cost on computational efficiency, ensemble feature selection methods are increasingly popular given the increasing computational capacity in the last decade and the parallelisation in some of their implementations [ 34 , 35 , 36 ].
Second, various hybrid methods have been proposed to combine filters, wrappers and embedded methods [ 37 ]. While these methods closely resemble ensemble approaches, they do not rely on data or model perturbations but instead use heterogeneous feature selection algorithms for creating a consensus [ 38 ]. Typically, these include combining different filter algorithms or different types of feature selection algorithms (e.g. stepwise combination of filter and wrapper). Generally, hybrid methods are motivated by the aim of taking advantage of the strengths of individual methods while alleviating or avoiding their weaknesses [ 39 ]. For example, in bioinformatics applications, several methods combine filters with wrappers in that filters are first applied to reduce the number of features from high dimension to a moderate number so that wrappers can be employed more efficiently for generating the final set of features [ 40 , 41 ]. As another example, genes selected by various feature selection methods are used for training a set of support vector machines (SVMs) for achieving better classification accuracy using microarray data [ 42 ]. While many hybrid feature selection algorithms are intuitive and numerous studies have reported favourable results compared to their individual components, a fundamental issue of these methods is their ad hoc nature, complicating the formal analysis of their underlying properties, such as theoretical algorithmic complexity and scalability.
Third, a recent evolution in feature selection has been its development and implementation using deep learning models. These include models based on perturbation [ 43 , 44 ], such as randomly excluding features to test their impact on the neural network output, and gradient propagation, where the gradient from the trained neural network is backpropagated to determine the importance of the input feature [ 45 , 46 ]. These deep learning feature selection models share a common concept of ‘saliency’ which was initially designed for interpreting black-box deep neural networks by highlighting input features that are relevant for the prediction of the model [ 47 ]. Some examples in bioinformatics applications include a deep feature selection model that uses a neural network with a weighted layer to select key input features for the identification and understanding regulatory events [ 48 ]; and a generative adversarial network approach for identifying genes that are associated with major depressive disorders using gradient-based methods [ 49 ]. While feature selection methods that are based on deep learning generally require significantly more computational resources (e.g. memory) and may be slower than traditional methods (especially when compared to filter methods), their capabilities for identifying complex relationships (e.g. non-linearity, interaction) among features have attracted tremendous attention in recent years.
Feature selection in the single-cell era
Until recently, the global molecular signatures generated from most biotechnologies are the average profiles from mixed populations of cells, masking the heterogeneity of cell and tissue types, a foundational characteristic of multicellular organisms [ 50 ]. Breakthroughs in global profiling techniques at the single-cell resolution, such as single-cell RNA-sequencing (scRNA-seq), single-cell Assay for Transposase Accessible Chromatin using sequencing (scATAC-seq) [ 51 ] and cellular indexing of transcriptomes and epitopes by sequencing (CITE-seq) [ 52 ], have reshaped many of our long-held views on multicellular biological systems. These advances of single-cell technologies create unprecedented opportunities for studying complex biological systems at resolutions that were previously unattainable and have led to renewed interests in feature selection for analysing such data. Below we review some of the latest developments and applications of feature selection across various domains in the single-cell field. Table 1 summarises the methods and their applications with additional details included in Additional file 1 : Table S1.
Feature selection in single-cell transcriptomics
By far, the most widely applied single-cell omics technologies are single-cell transcriptomics [ 53 ] made popular by an array of scRNA-seq protocols [ 54 ]. Given the availability of a huge amount of scRNA-seq data and the large number of genes profiled in these datasets, a similar characteristic of their bulk counterparts, most of recent feature selection applications in single-cell transcriptomics have been concentrated on gene selection from scRNA-seq data for various upstream pre-processing and downstream data analyses.
Among these, some of the most popular methods are univariate filters designed for identifying differential distributed genes, including t statistics or ANOVA based DE methods [ 55 , 56 ] and other statistical approaches such as differential variability (DV) [ 57 ] and differential proportion (DP) [ 58 ]. While differential distribution-based methods can often identify genes that are highly discriminative for downstream analysis, they require labels such as cell types to be pre-defined, limiting their applicability when such information is not available. A less restrictive and widely used alternative approach is to filter for highly variable genes (HVGs), which is implemented in various methods including the popular Seurat package [ 59 ]. Other methods that do not require label information include SCMarker which relies on testing the number of modalities of each gene through its expression profile [ 60 ], M3Drop which models the relationship between mean expression and dropout rate [ 61 ], and OGFSC, a variant of HVGs, based on modelling coefficient of variance of genes across cells [ 62 ]. Many scRNA-seq clustering algorithms also implement HVGs and its variants for gene filtering to improve the clustering of cells [ 63 ]. Besides the above univariate filters, recent research has also explored multivariate approaches. Examples include COMET which relies on a modified hypergeometric test for filtering gene pairs [ 64 ] and a multinomial method for gene filtering using the deviance statistic [ 65 ].
While filters are the most common options for pre-processing and feature selection from single-cell transcriptomics data, the application of wrapper methods is gaining much attention with a range of approaches built and extends on classic methods with the primary goal of facilitating downstream analyses such as cell type classification. Some examples include the application of classic methods such as greedy-based optimisation of entropy [ 66 ], nature-inspired optimisation such as using GA [ 67 , 68 ], and their hybrid with filters [ 69 , 70 , 71 ] or embedded methods [ 72 ]. More advanced methods include active learning-based feature selection using SVM as a wrapper [ 73 ] and optimisation based on data projection [ 74 ]. The impact of optimal feature selection using wrapper methods on improving cell type classification is well demonstrated through these studies.
Due to the simplicity in their application, the popularity of embedded methods is growing quickly in the last few years especially in studies that treat feature selection as a key goal in their analyses. These include the discovery of the minimum marker gene combinations using tree-based models [ 75 ], discriminative learning of DE genes using logistic regression models [ 76 ], regulatory gene signature identification using LASSO [ 77 ] and marker gene selection based on compressed sensing optimisation [ 78 ].
Lastly, several studies have compared the effect of various feature selection methods on the clustering of cell types [ 63 ] and investigated factors that affect feature selection in cell lineage analysis [ 79 ]. Together, these studies demonstrate the utility and flexibility of feature selection techniques in a wide range of tasks in single-cell transcriptomic data analyses.
Feature selection in single-cell epigenomics
Besides single-cell transcriptomic profiling, another fast-maturing single-cell omics technology is single-cell epigenomics profiling using scATAC-seq [ 51 ]. In particular, scATAC-seq measures genome-wide chromatin accessibility and therefore can provide a clue regarding the activity of epigenomic regulatory elements and their transcription factor binding motifs in single cells. Such data can offer additional information that is not accessible to scRNA-seq technologies and hence can complement and significantly enrich scRNA-seq data for characterising cell identity and gene regulatory networks (GRNs) in single cells [ 80 ]. Although most application of feature selection has been on investigating single-cell transcriptomes, recent studies have broadened the view to single-cell epigenomics primarily through their application in scATAC-seq data analysis. These analyses enable us to expand the gene expression analysis to also include regulatory elements such as enhancers and silencers in understanding molecular and cellular processes.
Feature selection methods could be directly applied to scATAC-seq data for identifying differential accessible chromatin regions or one can summarise scATAC-seq data to the gene level using tools such as those reviewed in [ 81 ] and then feature selection be performed for selecting ‘differentially accessible genes’ (DAGs) using such summarised data. For instance, Scasat, a tool for classifying cells using scATAC-seq data, implements both information gain and Fisher’s exact test for filtering and selecting differential accessible chromatin regions [ 82 ]. Similarly, scATAC-pro, a pipeline for scATAC-seq analysis at the chromatin level, employs Wilcoxon test as the default for filtering differential accessible chromatin regions, while also implements embedded methods such as logistic regression and negative binomial regression-based models as alternative options [ 83 ]. Another example is SnapATAC [ 84 ] which performs differential accessible chromatin analysis using the DE method implemented in edgeR [ 85 ]. In contrast, Kawaguchi et al. [ 86 ] summarised scATAC-seq data to the gene level using SCANPY [ 87 ] and performed embedded feature selection using either logistic LASSO or random forests to identify DAGs [ 86 ]. Muto et al. [ 88 ] performed filter-based differential analysis on both chromatin and gene levels based on Cicero estimated gene activity scores [ 89 ]. Finally, DUBStepR [ 71 ], a hybrid approach that combines a correlation-based filter and a regression-based wrapper for gene selection from scRNA-seq data, can also be applied to scATAC-seq data. Collectively, these methods and tools demonstrate the utility and impact of feature selection on scATAC data for cell-type identification, motif analysis, regulatory element and gene interaction detection among other applications.
Feature selection for single-cell surface proteins
Owing to the recent advancement in flow cytometry and related technologies such as mass cytometry [ 90 , 91 ], and single-cell multimodal sequencing technologies such as CITE-seq [ 52 ], surface proteins of the cells have now also become increasingly accessible at the single-cell resolution.
A key application of feature selection methods to flow and mass cytometry data has been for finding optimal protein markers for cell gating [ 92 ]. A representative example is GateFinder which implements a random forest-based feature selection procedure for optimising stepwise gating strategies on each given dataset [ 93 ]. Besides automated gating, several studies have also explored the use of feature selection for improving model performance on sample classification. For example, in their study, Hassan et al. [ 94 ] demonstrated the utility of shrinkage-based embedded models for classifying cancer samples. Another application of feature selection techniques was recently demonstrated by Tanhaemami et al. [ 95 ] for discovering signatures from label-free single cells. In particular, the authors employed a GA for feature selection and verified its utility in predicting lipid contents in algal cells under different conditions. Together, these studies illustrate the wide applicability of feature selection methods in a wide range of challenges in flow and mass cytometry data analysis.
Recent advancement in single-cell multimodal sequencing technologies such as CITE-seq and other related techniques such as RNA expression and protein sequencing (REAP-seq) [ 96 ] has enabled the profiling of both surface proteins and gene expressions at the single-cell level. While still at its infancy, feature selection techniques have already found their use in such data. One example is the application of a random forest-based approach for selecting marker proteins that can distinguish closely related cell types profiled using CITE-seq from PBMCs isolated from the blood of healthy human donors [ 97 ]. Another example is the use of a greedy forward feature selection wrapper that maximises a logistic regression model for identifying surface protein markers for each cell type from a given CITE-seq dataset [ 98 ].
Feature selection in single-cell imaging data
Other widely accessible data at the single-cell resolution are imaging-related data types such as those generated by image cytometry [ 99 ] and various single-cell imaging techniques [ 100 ]. Although the application of feature selection methods in this domain is very diverse, the following examples provide a snapshot of different types of feature selection techniques used for single-cell imaging data analysis.
To classify cell states using imaging flow cytometry data, Pischel et al. [ 101 ] employed a set of filters, including mutual information maximisation, maximum relevance minimum redundancy and Fisher score, for feature selection and demonstrated their utility on apoptosis detection. To predict cell cycle phases, Hennig et al. [ 102 ] implemented two embedded feature selection techniques, gradient boosting and random forest, for selecting the most predictive features from image cytometry data. These implementations are included in the CellProfiler, open-source software for imaging flow cytometry data analysis. To improve data interpretability of single-cell imaging data, Peralta and Saeys [ 103 ] proposed a clustering-based method for selecting representative features from each cluster and thus significantly reducing data dimensionality. To classify cell phenotypes, Doan et al. [ 104 ] implemented supervised and weakly supervised deep learning models in a framework called Deepometry for feature selection from imaging cytometry data. To classify cells according to their response to insulin stimulation, Norris et al. [ 105 ] used a random forest approach for ranking the informativeness of various temporal features extracted from time-course live-cell imaging data. Finally, to select spatially variable genes from imaging data generated by multiplexed single-molecule fluorescence in situ hybridization (smFISH), Svensson et al. [ 106 ] introduced a model based on the Gaussian process regression that decomposes expression and spatial information for gene selection.
Upcoming domains and future opportunities
The works reviewed above covers some of the most popular single-cell data types. Nevertheless, the technological advances in the single-cell field are extending our capability at a breakneck speed, enabling many other data modalities [ 107 ] as well as the spatial locations [ 108 ] of individual cells to be captured in high-throughput. For instance, recent development in single-cell DNA-sequencing provides the opportunity to analyse SNPs and copy-number variations (CNVs) in individual cells from cancer and normal tissues [ 109 , 110 ], and single-cell proteomics seems now on the horizon [ 111 , 112 ], holding great promises to further transform the single-cell field. Given the high feature-dimensionality of such data (e.g. numbers of SNPs, proteins and spatial locations), we anticipate feature selection techniques to be readily adopted for these single-cell data types when they become more available.
Another fast-growing capability in the single-cell field is increasingly towards multimodality. CITE-seq and REAP-seq are examples where both the gene expression and the surface proteins are measured in each individual cell. Nevertheless, many more recent techniques now also enable other combinations of modalities to be profiled at the single-cell level (Fig. 2 ). Some examples include ASAP-seq for profiling gene expression, chromatin accessibility and protein levels [ 113 ]; scMT-seq for profiling gene expression and DNA methylation [ 114 ] and its extension, scNMT-seq, for gene expression, chromatin accessibility and DNA methylation [ 115 ]; SHARE-seq and SNARE-seq for gene expression and chromatin accessibility [ 116 , 117 ]; scTrio-seq for CNVs, DNA methylation and gene expression [ 118 ]; and G&T-seq for genomic DNA and gene expression [ 119 ]. Given the complexity in the data structure in these single-cell multimodal data, feature selection methods that can facilitate integrative analysis of multiple data modalities are in great need. While some preliminary works have emerged recently [ 120 ], research on integrative feature selection is still at its infancy and requires significant innovation in their design and implementation.
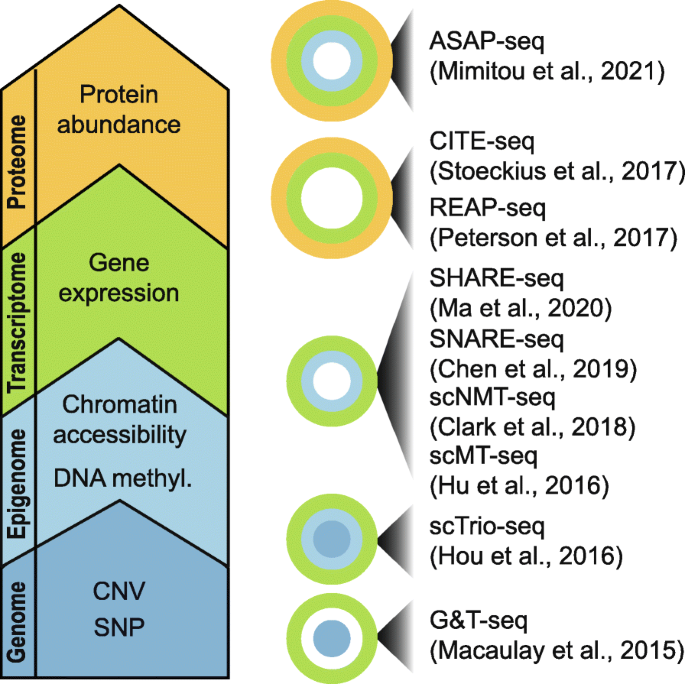
A schematic summary of some recent multimodal single-cell omics technologies
On the design of feature selection techniques in the single-cell field, most current studies directly use one of the three main types of methods (i.e. filters, wrappers and embedded methods). While we found a small number of them employed hybrid approaches (e.g. [ 71 , 72 ]), most are relatively straightforward combinations (such as stepwise application of filter and then wrapper methods) as have been used previously for bulk data analyses. The application of ensemble and deep learning-based feature selection methods is even sparser in the field. One ensemble feature selection method is EDGE which uses a set of weak learners to vote for important genes from scRNA-seq data [ 121 ], and the current literature on deep learning-based feature selection in single cells are a study for identifying regulatory modules from scRNA-seq data through autoencoder deconvolution [ 122 ]; and another for identifying disease-associated gene from scRNA-seq data using gradient-based methods [ 49 ]. Owing to the non-linear nature of the deep learning models, feature selection methods that are based on deep learning are well-suited to learn complex non-linear relationships among features. Given the widespread non-linearity relationships, such as gene-gene and protein-protein interactions, and interactions among genomic regulatory elements and their target genes in biological systems, and hence the data derived from them, we anticipate more research to be conducted on developing and adopting deep learning-based feature selection techniques in the single-cell field in the near future.
Applicability considerations
The works we have reviewed above showcase diverse feature selection strategies and promising future directions in single-cell data analytics. In practice, scalability and robustness are critical in choosing feature selection techniques and are largely dependent on the algorithm structure and implementation. Here, we discuss several key aspects specific to the utility and applicability of feature selection methods with the goal of guiding the choice of methods from each feature selection category for readers who are interested in their application.
Scalability towards the feature dimension
A key aspect in the applicability of a feature selection method rests upon its scalability to large datasets. Univariate filter algorithms are probably the most efficient in terms of scalability towards the feature dimension since, in general, the computation time of these algorithms increases linearly with the number of features. We therefore recommend univariate filters as the first choice when working with datasets with very high feature dimensions. In comparison, wrapper algorithms generally do not scale well with respect to the number of features due to their frequent reliance on combinatorial optimisation and therefore will remain applicable to datasets with a relatively small number of features. While other factors such as available computational resources and specific algorithm implementations also affect the choice of methods, wrapper algorithms are generally applied to datasets with up to a few hundred features. Embedded methods offer a good trade-off and both tree- and shrinkage-based methods computationally scale well with the number of features [ 19 ]. Nevertheless, like wrapper methods, embedded methods rely on an induction algorithm for feature selection and therefore are sensitive to model overfitting when dealing with data with a small sample size. We recommend choosing embedded methods for datasets with up to a few thousand features when the sample size (e.g. number of cells) is moderate or large. Similarly, hybrid algorithms that combine the filter with wrappers or filter with embedded methods also make a useful compromise and can be applied to the dataset with relatively high to very high feature dimensions, depending on the reduced feature dimension following the filtering step.
Scalability towards the sample size
With the advance of biotechnologies, the number of cells profiled in an experiment is growing exponentially. Hence, apart from the feature dimensionality, the scalability of the feature selection algorithm towards the sample size, typically in terms of the number of cells, is also a central determinant of its applicability to large-scale single-cell datasets. Although classic feature selection algorithms such as filters scale linearly towards the feature dimension, this does not necessarily mean they also scale linearly with the increasing number of cells [ 55 ]. To this end, the choice is more dependent on the specific implementation of the feature selection algorithms. Methods that purely rely on estimating variabilities (e.g. HVGs) without using cell type labels and fitting models generally scale better due to the extra steps taken by the latter for learning various data characteristics (e.g. zero-inflation). Another aspect to note is the memory usage. Most filter methods require the entire dataset to be loaded into the computer memory before feature selection can be performed. This can be an issue when the size of the dataset exceeds the size of the computer memory. Interestingly, deep learning-based feature selection methods could be better suited for analysing datasets with a very large number of cells. This is due to the unique characteristic of these methods where the neural network can be trained using small batches of input data sequentially and therefore alleviates the need to load the entire dataset into the computer memory.
Robustness and interpretability
Besides algorithm scalability, robustness and interpretability are also important criteria for assessing and selecting feature selection methods. This is especially crucial when the downstream applications are to identify reproducible biomarkers, where the selection of robust and stable features is essential, or to characterise gene regulatory networks, where model interpretability will be highly desirable. A key property of ensemble feature selection methods is their robustness to noise and slight variations in the data, which leads to better reproducibility in selected features [ 32 , 33 ]. We thus recommend exploring ensemble feature selection methods when the task is related to identify reproducible biomarkers such as marker genes for cells of a given type. In terms of interpretability, complex models, while often offering better performance in downstream analyses such as cell classification, may not be the most appropriate choices given the difficulties in their model interpretation. To this end, simpler models such as tree-based methods can provide clarity, for example, to how selected features are used to classify a cell and hence can facilitate the characterisation of gene regulatory networks underlying cell identity. Notably, however, significant progress has been made to improve interpretability especially for deep learning models [ 123 ]. Given the increasing importance of downstream analyses that involves biomarker discovery and pathway/network characterisation in single-cell research, we anticipate increasing efforts to be devoted to improving the robustness and interpretability of advanced methods such as deep learning models in feature selection applications.
Other considerations
Finally, the choice of feature selection methods also depends on other factors such as programming language, computing platform, parallelisation and whether they are well documented and easy to use. While most recent methods are implemented using popular programming languages such as R and Python which are well supported in various computing platforms including Windows, macOS and Linux/Unix and its variants, their difficulty in application varies and requires different levels of expertise from interacting with a simple graphical user interface to more complex execution that involves programming (e.g. loading packages in the R programming environment). Methods that optimise for computation speed may use C/C++ as their programming language and may also offer parallelisation. However, these methods are often computing platform-specific and may require more expertise from a specific operating system and programming language from users for their application. Lastly, the quality of the documentation of methods can have a significant impact on their ease of use. Methods that have comprehensive documentations with testable examples could help popularise their application. To this end, methods that are implemented under standardised framework such as Bioconductor [ 124 ] generally provide well-documented usages and examples known as ‘vignette’ for supporting users and therefore can be a practical consideration in their choices.
Conclusions
The explosion of single-cell data in recent years has led to a resurgence in the development and application of feature selection techniques for analysing such data. In this review, we revisited and summarised feature selection methods and their key development in the last decade. We then reviewed the recent literature for their applications in the single-cell field, summarising achievements so far and identifying missing aspects in the field. Based on these, we propose several research directions and discuss practical considerations that we hope will spark future research in feature selection and their application in the single-cell era.
Availability of data and materials
Not applicable.
Saeys Y, Inza I, Larranaga P. A review of feature selection techniques in bioinformatics. Bioinformatics. 2007;23(19):2507–17. https://doi.org/10.1093/bioinformatics/btm344 .
Article CAS PubMed Google Scholar
Efremova M, Teichmann SA. Computational methods for single-cell omics across modalities. Nature Methods. 2020;17(1):14–7. https://doi.org/10.1038/s41592-019-0692-4 .
Guyon I, Elisseeff A. An introduction to variable and feature selection. Journal of Machine Learning Research. 2003;3:1157–82.
Google Scholar
Lazar C, Taminau J, Meganck S, Steenhoff D, Coletta A, Molter C, et al. A survey on filter techniques for feature selection in gene expression microarray analysis. IEEE/ACM Transactions on Computational Biology and Bioinformatics. 2012;9(4):1106–19. https://doi.org/10.1109/TCBB.2012.33 .
Article PubMed Google Scholar
Bolón-Canedo V, Sánchez-Marono N, Alonso-Betanzos A, Benítez JM, Herrera F. A review of microarray datasets and applied feature selection methods. Information Sciences. 2014;282:111–35. https://doi.org/10.1016/j.ins.2014.05.042 .
Article Google Scholar
Levner I. Feature selection and nearest centroid classification for protein mass spectrometry. BMC Bioinformatics. 2005;6(1):1–14. https://doi.org/10.1186/1471-2105-6-68 .
Article CAS Google Scholar
Yang P, Ho JW, Zomaya AY, Zhou BB. A genetic ensemble approach for gene-gene interaction identification. BMC Bioinformatics. 2010;11(1):1–15. https://doi.org/10.1186/1471-2105-11-524 .
Model F, Adorjan P, Olek A, Piepenbrock C. Feature selection for DNA methylation based cancer classification. Bioinformatics. 2001;17(Suppl 1):S157–64. https://doi.org/10.1093/bioinformatics/17.suppl_1.S157 .
Gan Y, Guan J, Zhou S. A comparison study on feature selection of DNA structural properties for promoter prediction. BMC Bioinformatics. 2012;13(1):1–12. https://doi.org/10.1186/1471-2105-13-4 .
Chandrashekar G, Sahin F. A survey on feature selection methods. Computers & Electrical Engineering. 2014;40(1):16–28. https://doi.org/10.1016/j.compeleceng.2013.11.024 .
Ritchie ME, Phipson B, Wu D, Hu Y, Law CW, Shi W, et al. limma powers differential expression analyses for RNA-sequencing and microarray studies. Nucleic Acids Research. 2015;43(7):e47–7. https://doi.org/10.1093/nar/gkv007 .
Ding C, Peng H. Minimum redundancy feature selection from microarray gene expression data. Journal of Bioinformatics and Computational Biology. 2005;3(02):185–205. https://doi.org/10.1142/S0219720005001004 .
Bommert A, Sun X, Bischl B, Rahnenführer J, Lang M. Benchmark for filter methods for feature selection in high-dimensional classification data. Computational Statistics & Data Analysis. 2020;143:106839. https://doi.org/10.1016/j.csda.2019.106839 .
Kohavi R, John GH. Wrappers for feature subset selection. Artificial Intelligence. 1997;97(1-2):273–324. https://doi.org/10.1016/S0004-3702(97)00043-X .
Aha, D. W. & Bankert, R. L. A comparative evaluation of sequential feature selection algorithms. In Learning From Data, 199–206 (Springer, 1996).
Li L, Weinberg CR, Darden TA, Pedersen LG. Gene selection for sample classification based on gene expression data: study of sensitivity to choice of parameters of the GA/KNN method. Bioinformatics. 2001;17(12):1131–42. https://doi.org/10.1093/bioinformatics/17.12.1131 .
Yang P, Xu L, Zhou BB, Zhang Z, Zomaya AY. A particle swarm based hybrid system for imbalanced medical data sampling. BMC Genomics. 2009;10(Suppl 3):S34. https://doi.org/10.1186/1471-2164-10-S3-S34 .
Article PubMed PubMed Central Google Scholar
Lal, T. N., Chapelle, O., Weston, J. & Elisseeff, A. Embedded methods. In Feature Extraction, 137–165 (Springer, 2006).
Bolón-Canedo V, Sánchez-Maroño N, Alonso-Betanzos A. A review of feature selection methods on synthetic data. Knowledge and Information Systems. 2013;34(3):483–519. https://doi.org/10.1007/s10115-012-0487-8 .
Deng, H. & Runger, G. Feature selection via regularized trees. In The 2012 International Joint Conference on Neural Networks (IJCNN), 1–8 (IEEE, 2012).
Breiman L. Random forests. Machine Learning. 2001;45(1):5–32. https://doi.org/10.1023/A:1010933404324 .
Tibshirani R. Regression shrinkage and selection via the lasso. Journal of the Royal Statistical Society: Series B (Methodological). 1996;58:267–88.
Saeys, Y., Abeel, T. & Van de Peer, Y. Robust feature selection using ensemble feature selection techniques. In Joint European Conference on Machine Learning and Knowledge Discovery in Databases, 313–325 (Springer, 2008).
Abeel T, Helleputte T, Van de Peer Y, Dupont P, Saeys Y. Robust biomarker identification for cancer diagnosis with ensemble feature selection methods. Bioinformatics. 2010;26(3):392–8. https://doi.org/10.1093/bioinformatics/btp630 .
Yang, P., Liu, W., Zhou, B. B., Chawla, S. & Zomaya, A. Y. Ensemble-based wrapper methods for feature selection and class imbalance learning. In Pacific-Asia conference on knowledge discovery and data mining, 544–555 (Springer, 2013).
Tuv E, Borisov A, Runger G, Torkkola K. Feature selection with ensembles, artificial variables, and redundancy elimination. The Journal of Machine Learning Research. 2009;10:1341–66.
Dietterich, T. G. Ensemble methods in machine learning. In International Workshop on Multiple Classifier Systems, 1–15 (Springer, 2000).
Yang P, Hwa Yang Y. B Zhou, B. & Y Zomaya, A. A review of ensemble methods in bioinformatics. Current Bioinformatics. 2010;5(4):296–308. https://doi.org/10.2174/157489310794072508 .
Cao Y, Geddes TA, Yang JYH, Yang P. Ensemble deep learning in bioinformatics. Nature Machine Intelligence. 2020;2:500–8.
Bolón-Canedo V, Alonso-Betanzos A. Ensembles for feature selection: a review and future trends. Information Fusion. 2019;52:1–12. https://doi.org/10.1016/j.inffus.2018.11.008 .
Brahim AB, Limam M. Ensemble feature selection for high dimensional data: a new method and a comparative study. Advances in Data Analysis and Classification. 2018;12(4):937–52. https://doi.org/10.1007/s11634-017-0285-y .
Yang, P., Zhou, B. B., Yang, J. Y.-H. & Zomaya, A. Y. Stability of feature selection algorithms and ensemble feature selection methods in bioinformatics. Biological Knowledge Discovery Handbook, 333–352 (2013).
Pes B. Ensemble feature selection for high-dimensional data: a stability analysis across multiple domains. Neural Computing and Applications. 2020;32(10):5951–73. https://doi.org/10.1007/s00521-019-04082-3 .
Hijazi, N. M., Faris, H. & Aljarah, I. A parallel metaheuristic approach for ensemble feature selection based on multi-core architectures. Expert Systems with Applications 115290 (2021).
Tsai C-F, Sung Y-T. Ensemble feature selection in high dimension, low sample size datasets: Parallel and serial combination approaches. Knowledge-Based Systems. 2020;203:106097. https://doi.org/10.1016/j.knosys.2020.106097 .
Soufan O, Kleftogiannis D, Kalnis P, Bajic VB. Dwfs: a wrapper feature selection tool based on a parallel genetic algorithm. PloS one. 2015;10(2):e0117988. https://doi.org/10.1371/journal.pone.0117988 .
Article CAS PubMed PubMed Central Google Scholar
Chen C-W, Tsai Y-H, Chang F-R, Lin W-C. Ensemble feature selection in medical datasets: combining filter, wrapper, and embedded feature selection results. Expert Systems. 2020;37:e12553.
Seijo-Pardo B, Porto-Díaz I, Bolón-Canedo V, Alonso-Betanzos A. Ensemble feature selection: homogeneous and heterogeneous approaches. Knowledge-Based Systems. 2017;118:124–39. https://doi.org/10.1016/j.knosys.2016.11.017 .
Jovic´, A., Brkic´, K. & Bogunovic´, N. A review of feature selection methods with applications. In 2015 38th international convention on information and communication technology, electronics and microelectronics (MIPRO), 1200–1205 (Ieee, 2015).
Yang P, Zhou BB, Zhang Z, Zomaya AY. A multi-filter enhanced genetic ensemble system for gene selection and sample classification of microarray data. BMC Bioinformatics. 2010;11(S1):1–12. https://doi.org/10.1186/1471-2105-11-S1-S5 .
Chuang L-Y, Yang C-H, Wu K-C, Yang C-H. A hybrid feature selection method for dna microarray data. Computers in Biology and Medicine. 2011;41(4):228–37. https://doi.org/10.1016/j.compbiomed.2011.02.004 .
Nanni L, Brahnam S, Lumini A. Combining multiple approaches for gene microarray classification. Bioinformatics. 2012;28(8):1151–7. https://doi.org/10.1093/bioinformatics/bts108 .
Ribeiro, M. T., Singh, S. & Guestrin, C. “Why should I trust you?” explaining the predictions of any classifier. In Proceedings of the 22nd ACM SIGKDD International Conference on Knowledge Discovery and Data mining, 1135–1144 (2016).
Bach S, Binder A, Montavon G, Klauschen F, Müller KR, Samek W. On pixel-wise explanations for non-linear classifier decisions by layer-wise relevance propagation. PloS One. 2015;10(7):e0130140. https://doi.org/10.1371/journal.pone.0130140 .
Simonyan, K., Vedaldi, A. & Zisserman, A. Deep inside convolutional networks: visualising image classification models and saliency maps. In In Workshop at International Conference on Learning Representations (Citeseer, 2014).
Shrikumar, A., Greenside, P. & Kundaje, A. Learning important features through propagating activation differences. In International Conference on Machine Learning, 3145–3153 (PMLR, 2017).
Cancela B, Bolón-Canedo V, Alonso-Betanzos A, Gama J. A scalable saliency-based feature selection method with instance-level information. Knowledge-Based Systems. 2020;192:105326. https://doi.org/10.1016/j.knosys.2019.105326 .
Li Y, Chen C-Y, Wasserman WW. Deep feature selection: theory and application to identify enhancers and promoters. Journal of Computational Biology. 2016;23(5):322–36. https://doi.org/10.1089/cmb.2015.0189 .
Bahrami M, Maitra M, Nagy C, Turecki G, Rabiee HR, Li Y. Deep feature extraction of single-cell transcriptomes by generative adversarial network. Bioinformatics. 2021;37(10):1345–51. https://doi.org/10.1093/bioinformatics/btaa976 .
Buettner F, Natarajan KN, Casale FP, Proserpio V, Scialdone A, Theis FJ, et al. Computational analysis of cell-to-cell heterogeneity in single-cell rna-sequencing data reveals hidden subpopulations of cells. Nature Biotechnology. 2015;33(2):155–60. https://doi.org/10.1038/nbt.3102 .
Cusanovich DA, Daza R, Adey A, Pliner HA, Christiansen L, Gunderson KL, et al. Multiplex single-cell profiling of chromatin accessibility by combinatorial cellular indexing. Science. 2015;348(6237):910–4. https://doi.org/10.1126/science.aab1601 .
Stoeckius M, Hafemeister C, Stephenson W, Houck-Loomis B, Chattopadhyay PK, Swerdlow H, et al. Simultaneous epitope and transcriptome measurement in single cells. Nature Methods. 2017;14(9):865–8. https://doi.org/10.1038/nmeth.4380 .
Aldridge S, Teichmann SA. Single cell transcriptomics comes of age. Nature Communications. 2020;11:1–4.
Mereu E, Lafzi A, Moutinho C, Ziegenhain C, McCarthy DJ, Álvarez-Varela A, et al. Benchmarking single-cell RNA-sequencing protocols for cell atlas projects. Nature Biotechnology. 2020;38(6):747–55. https://doi.org/10.1038/s41587-020-0469-4 .
Soneson C, Robinson MD. Bias, robustness and scalability in single-cell differential expression analysis. Nature Methods. 2018;15(4):255–61. https://doi.org/10.1038/nmeth.4612 .
Vans, E., Patil, A. & Sharma, A. Feats: feature selection-based clustering of single-cell rna-seq data. Briefings in bioinformatics bbaa306.
Lin, Y. et al. scclassify: sample size estimation and multiscale classification of cells using single and multiple reference. Molecular Systems Biology 16, e9389 (2020).
Korthauer KD, Chu LF, Newton MA, Li Y, Thomson J, Stewart R, et al. A statistical approach for identifying differential distributions in single-cell rna-seq experiments. Genome Biology. 2016;17(1):1–15. https://doi.org/10.1186/s13059-016-1077-y .
Stuart T, Butler A, Hoffman P, Hafemeister C, Papalexi E, Mauck WM III, et al. Comprehensive integration of single-cell data. Cell. 2019;177(7):1888–902. https://doi.org/10.1016/j.cell.2019.05.031 .
Wang F, Liang S, Kumar T, Navin N, Chen K. Scmarker: ab initio marker selection for single cell transcriptome profiling. PLoS Computational Biology. 2019;15(10):e1007445. https://doi.org/10.1371/journal.pcbi.1007445 .
Andrews TS, Hemberg M. M3drop: dropout-based feature selection for scrnaseq. Bioinformatics. 2019;35(16):2865–7. https://doi.org/10.1093/bioinformatics/bty1044 .
Hao J, Cao W, Huang J, Zou X, Han Z-G. Optimal gene filtering for single-cell data (ogfsc)—a gene filtering algorithm for single-cell rna-seq data. Bioinformatics. 2019;35(15):2602–9. https://doi.org/10.1093/bioinformatics/bty1016 .
Su K, Yu T, Wu H. Accurate feature selection improves single-cell RNA-seq cell clustering. Briefings in Bioinformatics. 2021;22(5). https://doi.org/10.1093/bib/bbab034 .
Delaney C, Schnell A, Cammarata LV, Yao-Smith A, Regev A, Kuchroo VK, et al. Combinatorial prediction of marker panels from single-cell transcriptomic data. Molecular systems biology. 2019;15(10):e9005. https://doi.org/10.15252/msb.20199005 .
Townes FW, Hicks SC, Aryee MJ, Irizarry RA. Feature selection and dimension reduction for single-cell RNA-seq based on a multinomial model. Genome Biology. 2019;20(1):1–16. https://doi.org/10.1186/s13059-019-1861-6 .
Lall, S., Ghosh, A., Ray, S. & Bandyopadhyay, S. sc-REnF: an entropy guided robust feature selection for clustering of single-cell rna-seq data. bioRxiv (2020).
Aliee H, Theis FJ. Autogenes: automatic gene selection using multi-objective optimization for RNA-seq deconvolution. Cell Systems. 2021;12(7):706–715.e4. https://doi.org/10.1016/j.cels.2021.05.006 .
Gupta S, Verma AK, Ahmad S. Feature selection for topological proximity prediction of single-cell transcriptomic profiles in drosophila embryo using genetic algorithm. Genes. 2021;12(1):28. https://doi.org/10.3390/genes12010028 .
Zhang, J. & Feng, J. Gene selection for single-cell RNA-seq data based on information gain and genetic algorithm. In 2018 14th International Conference on Computational Intelligence and Security (CIS), 57–61 (IEEE, 2018).
Zhang, J., Feng, J. & Yang, X. Gene selection for scRNA-seq data based on information gain and fruit fly optimization algorithm. In 2019 15th International Conference on Computational Intelligence and Security (CIS), 187–191 (IEEE, 2019).
Ranjan B, Sun W, Park J, Mishra K, Schmidt F, Xie R, et al. DUBStepR is a scalable correlation-based feature selection method for accurately clustering single-cell data. Nature Communications. 2021;12(1):5849. https://doi.org/10.1038/s41467-021-26085-2 .
Yuan F, Pan XY, Zeng T, Zhang YH, Chen L, Gan Z, et al. Identifying cell-type specific genes and expression rules based on single-cell transcriptomic atlas data. Frontiers in Bioengineering and Biotechnology. 2020;8:350. https://doi.org/10.3389/fbioe.2020.00350 .
Chen, X., Chen, S. & Thomson, M. Active feature selection discovers minimal gene-sets for classifying cell-types and disease states in single-cell mRNA-seq data. arXiv preprint arXiv:2106.08317 (2021).
Dumitrascu B, Villar S, Mixon DG, Engelhardt BE. Optimal marker gene selection for cell type discrimination in single cell analyses. Nature Communications. 2021;12(1):1–8. https://doi.org/10.1038/s41467-021-21453-4 .
Aevermann, B. D. et al. A machine learning method for the discovery of minimum marker gene combinations for cell-type identification from single-cell RNA sequencing. Genome Research, gr–275569 (2021).
Ntranos V, Yi L, Melsted P, Pachter L. A discriminative learning approach to differential expression analysis for single-cell RNA-seq. Nature Methods. 2019;16(2):163–6. https://doi.org/10.1038/s41592-018-0303-9 .
Huynh, N. P., Kelly, N. H., Katz, D. B., Pham, M. & Guilak, F. Single cell RNA sequencing reveals heterogeneity of human MSC chondrogenesis: Lasso regularized logistic regression to identify gene and regulatory signatures. bioRxiv 854406 (2019).
Vargo AH, Gilbert AC. A rank-based marker selection method for high throughput scRNA-seq data. BMC Bioinformatics. 2020;21(1):1–51. https://doi.org/10.1186/s12859-020-03641-z .
Chen B. Herring, C. A. & Lau, K. S. pyNVR: investigating factors affecting feature selection from scRNA-seq data for lineage reconstruction. Bioinformatics. 2019;35(13):2335–7. https://doi.org/10.1093/bioinformatics/bty950 .
Buenrostro JD, Wu B, Litzenburger UM, Ruff D, Gonzales ML, Snyder MP, et al. Single-cell chromatin accessibility reveals principles of regulatory variation. Nature. 2015;523(7561):486–90. https://doi.org/10.1038/nature14590 .
Chen H, Lareau C, Andreani T, Vinyard ME, Garcia SP, Clement K, et al. Assessment of computational methods for the analysis of single-cell ATAC-seq data. Genome Biology. 2019;20(1):1–25. https://doi.org/10.1186/s13059-019-1854-5 .
Baker SM, Rogerson C, Hayes A, Sharrocks AD, Rattray M. Classifying cells with scasat, a single-cell ATAC-seq analysis tool. Nucleic acids research. 2019;47(2):e10–0. https://doi.org/10.1093/nar/gky950 .
Yu W, Uzun Y, Zhu Q. Chen, C. & Tan, K. scATAC-pro: a comprehensive workbench for single-cell chromatin accessibility sequencing data. Genome Biology. 2020;21(1):1–17. https://doi.org/10.1186/s13059-020-02008-0 .
Fang R, Preissl S, Li Y, Hou X, Lucero J, Wang X, et al. Comprehensive analysis of single cell atac-seq data with snapatac. Nature communications. 2021;12(1):1–15. https://doi.org/10.1038/s41467-021-21583-9 .
Robinson MD. McCarthy, D. J. & Smyth, G. K. edgeR: a bioconductor package for differential expression analysis of digital gene expression data. Bioinformatics. 2010;26(1):139–40. https://doi.org/10.1093/bioinformatics/btp616 .
Kawaguchi RK, et al. Exploiting marker genes for robust classification and characterization of single-cell chromatin accessibility. BioRxiv. 2021.
Wolf FA, Angerer P, Theis FJ. SCANPY: large-scale single-cell gene expression data analysis. Genome Biology. 2018;19(1):1–5. https://doi.org/10.1186/s13059-017-1382-0 .
Muto Y, Wilson PC, Ledru N, Wu H, Dimke H, Waikar SS, et al. Single cell transcriptional and chromatin accessibility profiling redefine cellular heterogeneity in the adult human kidney. Nature Communications. 2021;12(1):1–17. https://doi.org/10.1038/s41467-021-22368-w .
Pliner HA, Packer JS, McFaline-Figueroa JL, Cusanovich DA, Daza RM, Aghamirzaie D, et al. Cicero predicts cis-regulatory DNA interactions from single-cell chromatin accessibility data. Molecular Cell. 2018;71(5):858–71. https://doi.org/10.1016/j.molcel.2018.06.044 .
Brummelman J, Haftmann C, Núñez NG, Alvisi G, Mazza EMC, Becher B, et al. Development, application and computational analysis of high-dimensional fluorescent antibody panels for single-cell flow cytometry. Nature Protocols. 2019;14(7):1946–69. https://doi.org/10.1038/s41596-019-0166-2 .
Spitzer MH, Nolan GP. Mass cytometry: single cells, many features. Cell. 2016;165(4):780–91. https://doi.org/10.1016/j.cell.2016.04.019 .
Saeys Y, Van Gassen S, Lambrecht BN. Computational flow cytometry: helping to make sense of high-dimensional immunology data. Nature Reviews Immunology. 2016;16(7):449–62. https://doi.org/10.1038/nri.2016.56 .
Aghaeepour N, Simonds EF, Knapp DJHF, Bruggner RV, Sachs K, Culos A, et al. GateFinder: projection-based gating strategy optimization for flow and mass cytometry. Bioinformatics. 2018;34(23):4131–3. https://doi.org/10.1093/bioinformatics/bty430 .
Hassan, S. S., Ruusuvuori, P., Latonen, L. & Huttunen, H. Flow cytometry-based classification in cancer research: a view on feature selection. Cancer Informatics 14, CIN–S30795 (2015).
Tanhaemami M, Alizadeh E, Sanders CK, Marrone BL, Munsky B. Using flow cytometry and multistage machine learning to discover label-free signatures of algal lipid accumulation. Physical Biology. 2019;16(5):055001. https://doi.org/10.1088/1478-3975/ab2c60 .
Peterson VM, Zhang KX, Kumar N, Wong J, Li L, Wilson DC, et al. Multiplexed quantification of proteins and transcripts in single cells. Nature Biotechnology. 2017;35(10):936–9. https://doi.org/10.1038/nbt.3973 .
Kim HJ, Lin Y, Geddes TA, Yang JYH, Yang P. CiteFuse enables multi-modal analysis of CITE-Seq data. Bioinformatics. 2020;36(14):4137–43. https://doi.org/10.1093/bioinformatics/btaa282 .
Hao Y, Hao S, Andersen-Nissen E, Mauck WM III, Zheng S, Butler A, et al. Integrated analysis of multimodal single-cell data. Cell. 2021;184(13):3573–3587.e29. https://doi.org/10.1016/j.cell.2021.04.048 .
Weissleder R, Lee H. Automated molecular-image cytometry and analysis in modern oncology. Nature Reviews Materials. 2020;5(6):409–22. https://doi.org/10.1038/s41578-020-0180-6 .
Stender AS, Marchuk K, Liu C, Sander S, Meyer MW, Smith EA, et al. Single cell optical imaging and spectroscopy. Chemical Reviews. 2013;113(4):2469–527. https://doi.org/10.1021/cr300336e .
Pischel D, Buchbinder JH, Sundmacher K, Lavrik IN, Flassig RJ. A guide to automated apoptosis detection: how to make sense of imaging flow cytometry data. PloS One. 2018;13(5):e0197208. https://doi.org/10.1371/journal.pone.0197208 .
Hennig H, Rees P, Blasi T, Kamentsky L, Hung J, Dao D, et al. An open-source solution for advanced imaging flow cytometry data analysis using machine learning. Methods. 2017;112:201–10. https://doi.org/10.1016/j.ymeth.2016.08.018 .
Peralta D, Saeys Y. Robust unsupervised dimensionality reduction based on feature clustering for single-cell imaging data. Applied Soft Computing. 2020;93:106421. https://doi.org/10.1016/j.asoc.2020.106421 .
Doan, M. et al. Deepometry, a framework for applying supervised and weakly supervised deep learning to imaging cytometry. Nature Protocols 1–24 (2021).
Norris, D. et al. Signaling heterogeneity is defined by pathway architecture and intercellular variability in protein expression. iScience 24, 102118 (2021).
Svensson V, Teichmann SA, Stegle O. SpatialDE: identification of spatially variable genes. Nature Methods. 2018;15(5):343–6. https://doi.org/10.1038/nmeth.4636 .
Macaulay IC, Ponting CP, Voet T. Single-cell multiomics: multiple measurements from single cells. Trends in Genetics. 2017;33(2):155–68. https://doi.org/10.1016/j.tig.2016.12.003 .
Burgess DJ. Spatial transcriptomics coming of age. Nature Reviews Genetics. 2019;20(6):317–7. https://doi.org/10.1038/s41576-019-0129-z .
Velazquez-Villarreal EI, Maheshwari S, Sorenson J, Fiddes IT, Kumar V, Yin Y, et al. Single-cell sequencing of genomic DNA resolves sub-clonal heterogeneity in a melanoma cell line. Communications Biology. 2020;3(1):1–8. https://doi.org/10.1038/s42003-020-1044-8 .
Luquette LJ, Bohrson CL, Sherman MA, Park PJ. Identification of somatic mutations in single cell DNA-seq using a spatial model of allelic imbalance. Nature Communications. 2019;10(1):1–14. https://doi.org/10.1038/s41467-019-11857-8 .
Marx V. A dream of single-cell proteomics. Nature Methods. 2019;16(9):809–12. https://doi.org/10.1038/s41592-019-0540-6 .
Kelly RT. Single-cell proteomics: progress and prospects. Molecular & Cellular Proteomics. 2020;19(11):1739–48. https://doi.org/10.1074/mcp.R120.002234 .
Mimitou, E. P. et al. Scalable, multimodal profiling of chromatin accessibility, gene expression and protein levels in single cells. Nature Biotechnology 1–13 (2021).
Hu Y, Huang K, An Q, du G, Hu G, Xue J, et al. Simultaneous profiling of transcriptome and DNA methylome from a single cell. Genome Biology. 2016;17(1):1–11. https://doi.org/10.1186/s13059-016-0950-z .
Clark SJ, Argelaguet R, Kapourani CA, Stubbs TM, Lee HJ, Alda-Catalinas C, et al. scNMT-seq enables joint profiling of chromatin accessibility DNA methylation and transcription in single cells. Nature Communications. 2018;9(1):1–9. https://doi.org/10.1038/s41467-018-03149-4 .
Ma S, Zhang B, LaFave LM, Earl AS, Chiang Z, Hu Y, et al. Chromatin potential identified by shared single-cell profiling of RNA and chromatin. Cell. 2020;183(4):1103–16. https://doi.org/10.1016/j.cell.2020.09.056 .
Chen S, Lake BB, Zhang K. High-throughput sequencing of the transcriptome and chromatin accessibility in the same cell. Nature Biotechnology. 2019;37(12):1452–7. https://doi.org/10.1038/s41587-019-0290-0 .
Hou Y, Guo H, Cao C, Li X, Hu B, Zhu P, et al. Single-cell triple omics sequencing reveals genetic, epigenetic, and transcriptomic heterogeneity in hepatocellular carcinomas. Cell Research. 2016;26(3):304–19. https://doi.org/10.1038/cr.2016.23 .
Macaulay IC, Haerty W, Kumar P, Li YI, Hu TX, Teng MJ, et al. G&t-seq: parallel sequencing of single-cell genomes and transcriptomes. Nature Methods. 2015;12(6):519–22. https://doi.org/10.1038/nmeth.3370 .
Liang S, Mohanty V, Dou J, Miao Q, Huang Y, Müftüoğlu M, et al. Single-cell manifold-preserving feature selection for detecting rare cell populations. Nature Computational Science. 2021;1(5):374–84. https://doi.org/10.1038/s43588-021-00070-7 .
Sun X, Liu Y, An L. Ensemble dimensionality reduction and feature gene extraction for single-cell RNA-seq data. Nature Communications. 2020;11(1):1–9. https://doi.org/10.1038/s41467-020-19465-7 .
Kinalis S, Nielsen FC, Winther O, Bagger FO. Deconvolution of autoencoders to learn biological regulatory modules from single cell mRNA sequencing data. BMC Bioinformatics. 2019;20(1):1–9. https://doi.org/10.1186/s12859-019-2952-9 .
Samek, W. et al. Explainable artificial intelligence: understanding, visualizing and interpreting deep learning models. arXiv:1708.08296 (2017).
Gentleman RC, Carey VJ, Bates DM, Bolstad B, Dettling M, Dudoit S, et al. Bioconductor: open software development for computational biology and bioinformatics. Genome Biology. 2004;5(10):R80. https://doi.org/10.1186/gb-2004-5-10-r80 .
Download references
Acknowledgements
The authors thank the feedback from the members of the Sydney Precision Bioinformatics Alliance.
Peer review information
Barbara Cheifet was the primary editor of this article and managed its editorial process and peer review in collaboration with the rest of the editorial team.
Review history
The review history is available as Additional file 2 .
P.Y. is supported by a National Health and Medical Research Council Investigator Grant (1173469).
Author information
Authors and affiliations.
School of Mathematics and Statistics, University of Sydney, Sydney, NSW, 2006, Australia
Pengyi Yang & Hao Huang
Computational Systems Biology Group, Children’s Medical Research Institute, University of Sydney, Westmead, NSW, 2145, Australia
Pengyi Yang, Hao Huang & Chunlei Liu
Charles Perkins Centre, University of Sydney, Sydney, NSW, 2006, Australia
Pengyi Yang
You can also search for this author in PubMed Google Scholar
Contributions
P.Y. conceptualised this work. The authors reviewed the literature, drafted the manuscript and wrote and edited the manuscript. The authors read and approved the final manuscript.
Authors’ information
Twitter handles: @PengyiYang82 (Pengyi Yang); @haohuang1999 (Hao Huang); @ChunleiLiu0 (Chunlei Liu).
Corresponding author
Correspondence to Pengyi Yang .
Ethics declarations
Ethics approval and consent to participate, consent for publication, competing interests.
The authors declare that they have no competing interests.
Additional information
Publisher’s note.
Springer Nature remains neutral with regard to jurisdictional claims in published maps and institutional affiliations.
Supplementary information
Additional file 1: table s1..
A list of studies that applied feature selection techniques to the single-cell field.
Additional file 2:
Review history.
Rights and permissions
Open Access This article is licensed under a Creative Commons Attribution 4.0 International License, which permits use, sharing, adaptation, distribution and reproduction in any medium or format, as long as you give appropriate credit to the original author(s) and the source, provide a link to the Creative Commons licence, and indicate if changes were made. The images or other third party material in this article are included in the article's Creative Commons licence, unless indicated otherwise in a credit line to the material. If material is not included in the article's Creative Commons licence and your intended use is not permitted by statutory regulation or exceeds the permitted use, you will need to obtain permission directly from the copyright holder. To view a copy of this licence, visit http://creativecommons.org/licenses/by/4.0/ . The Creative Commons Public Domain Dedication waiver ( http://creativecommons.org/publicdomain/zero/1.0/ ) applies to the data made available in this article, unless otherwise stated in a credit line to the data.
Reprints and permissions
About this article
Cite this article.
Yang, P., Huang, H. & Liu, C. Feature selection revisited in the single-cell era. Genome Biol 22 , 321 (2021). https://doi.org/10.1186/s13059-021-02544-3
Download citation
Received : 26 July 2021
Accepted : 15 November 2021
Published : 01 December 2021
DOI : https://doi.org/10.1186/s13059-021-02544-3
Share this article
Anyone you share the following link with will be able to read this content:
Sorry, a shareable link is not currently available for this article.
Provided by the Springer Nature SharedIt content-sharing initiative
Genome Biology
ISSN: 1474-760X
- Submission enquiries: [email protected]
- General enquiries: [email protected]
Fake news, disinformation and misinformation in social media: a review
- Original Article
- Published: 09 February 2023
- Volume 13 , article number 30 , ( 2023 )
Cite this article
- Esma Aïmeur ORCID: orcid.org/0000-0001-7414-5454 1 ,
- Sabrine Amri ORCID: orcid.org/0000-0002-5009-4573 1 &
- Gilles Brassard ORCID: orcid.org/0000-0002-4380-117X 1
56k Accesses
38 Citations
160 Altmetric
31 Mentions
Explore all metrics
Online social networks (OSNs) are rapidly growing and have become a huge source of all kinds of global and local news for millions of users. However, OSNs are a double-edged sword. Although the great advantages they offer such as unlimited easy communication and instant news and information, they can also have many disadvantages and issues. One of their major challenging issues is the spread of fake news. Fake news identification is still a complex unresolved issue. Furthermore, fake news detection on OSNs presents unique characteristics and challenges that make finding a solution anything but trivial. On the other hand, artificial intelligence (AI) approaches are still incapable of overcoming this challenging problem. To make matters worse, AI techniques such as machine learning and deep learning are leveraged to deceive people by creating and disseminating fake content. Consequently, automatic fake news detection remains a huge challenge, primarily because the content is designed in a way to closely resemble the truth, and it is often hard to determine its veracity by AI alone without additional information from third parties. This work aims to provide a comprehensive and systematic review of fake news research as well as a fundamental review of existing approaches used to detect and prevent fake news from spreading via OSNs. We present the research problem and the existing challenges, discuss the state of the art in existing approaches for fake news detection, and point out the future research directions in tackling the challenges.
Similar content being viewed by others
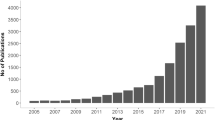
The disaster of misinformation: a review of research in social media
Sadiq Muhammed T & Saji K. Mathew
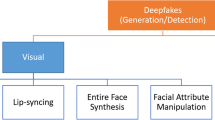
Deepfakes generation and detection: state-of-the-art, open challenges, countermeasures, and way forward
Momina Masood, Mariam Nawaz, … Hafiz Malik
The Power of Consumers on Social Media: A Case Study of Balenciaga’s Crisis Communication
Avoid common mistakes on your manuscript.
1 Introduction
1.1 context and motivation.
Fake news, disinformation and misinformation have become such a scourge that Marcia McNutt, president of the National Academy of Sciences of the United States, is quoted to have said (making an implicit reference to the COVID-19 pandemic) “Misinformation is worse than an epidemic: It spreads at the speed of light throughout the globe and can prove deadly when it reinforces misplaced personal bias against all trustworthy evidence” in a joint statement of the National Academies Footnote 1 posted on July 15, 2021. Indeed, although online social networks (OSNs), also called social media, have improved the ease with which real-time information is broadcast; its popularity and its massive use have expanded the spread of fake news by increasing the speed and scope at which it can spread. Fake news may refer to the manipulation of information that can be carried out through the production of false information, or the distortion of true information. However, that does not mean that this problem is only created with social media. A long time ago, there were rumors in the traditional media that Elvis was not dead, Footnote 2 that the Earth was flat, Footnote 3 that aliens had invaded us, Footnote 4 , etc.
Therefore, social media has become nowadays a powerful source for fake news dissemination (Sharma et al. 2019 ; Shu et al. 2017 ). According to Pew Research Center’s analysis of the news use across social media platforms, in 2020, about half of American adults get news on social media at least sometimes, Footnote 5 while in 2018, only one-fifth of them say they often get news via social media. Footnote 6
Hence, fake news can have a significant impact on society as manipulated and false content is easier to generate and harder to detect (Kumar and Shah 2018 ) and as disinformation actors change their tactics (Kumar and Shah 2018 ; Micallef et al. 2020 ). In 2017, Snow predicted in the MIT Technology Review (Snow 2017 ) that most individuals in mature economies will consume more false than valid information by 2022.
Recent news on the COVID-19 pandemic, which has flooded the web and created panic in many countries, has been reported as fake. Footnote 7 For example, holding your breath for ten seconds to one minute is not a self-test for COVID-19 Footnote 8 (see Fig. 1 ). Similarly, online posts claiming to reveal various “cures” for COVID-19 such as eating boiled garlic or drinking chlorine dioxide (which is an industrial bleach), were verified Footnote 9 as fake and in some cases as dangerous and will never cure the infection.
Social media outperformed television as the major news source for young people of the UK and the USA. Footnote 10 Moreover, as it is easier to generate and disseminate news online than with traditional media or face to face, large volumes of fake news are produced online for many reasons (Shu et al. 2017 ). Furthermore, it has been reported in a previous study about the spread of online news on Twitter (Vosoughi et al. 2018 ) that the spread of false news online is six times faster than truthful content and that 70% of the users could not distinguish real from fake news (Vosoughi et al. 2018 ) due to the attraction of the novelty of the latter (Bovet and Makse 2019 ). It was determined that falsehood spreads significantly farther, faster, deeper and more broadly than the truth in all categories of information, and the effects are more pronounced for false political news than for false news about terrorism, natural disasters, science, urban legends, or financial information (Vosoughi et al. 2018 ).
Over 1 million tweets were estimated to be related to fake news by the end of the 2016 US presidential election. Footnote 11 In 2017, in Germany, a government spokesman affirmed: “We are dealing with a phenomenon of a dimension that we have not seen before,” referring to an unprecedented spread of fake news on social networks. Footnote 12 Given the strength of this new phenomenon, fake news has been chosen as the word of the year by the Macquarie dictionary both in 2016 Footnote 13 and in 2018 Footnote 14 as well as by the Collins dictionary in 2017. Footnote 15 \(^,\) Footnote 16 Since 2020, the new term “infodemic” was coined, reflecting widespread researchers’ concern (Gupta et al. 2022 ; Apuke and Omar 2021 ; Sharma et al. 2020 ; Hartley and Vu 2020 ; Micallef et al. 2020 ) about the proliferation of misinformation linked to the COVID-19 pandemic.
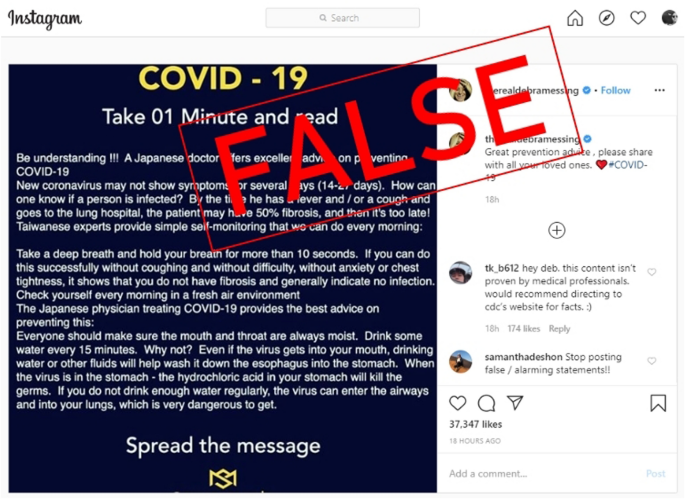
Fake news example about a self-test for COVID-19 source: https://cdn.factcheck.org/UploadedFiles/Screenshot031120_false.jpg , last access date: 26-12-2022
The Gartner Group’s top strategic predictions for 2018 and beyond included the need for IT leaders to quickly develop Artificial Intelligence (AI) algorithms to address counterfeit reality and fake news. Footnote 17 However, fake news identification is a complex issue. (Snow 2017 ) questioned the ability of AI to win the war against fake news. Similarly, other researchers concurred that even the best AI for spotting fake news is still ineffective. Footnote 18 Besides, recent studies have shown that the power of AI algorithms for identifying fake news is lower than its ability to create it Paschen ( 2019 ). Consequently, automatic fake news detection remains a huge challenge, primarily because the content is designed to closely resemble the truth in order to deceive users, and as a result, it is often hard to determine its veracity by AI alone. Therefore, it is crucial to consider more effective approaches to solve the problem of fake news in social media.
1.2 Contribution
The fake news problem has been addressed by researchers from various perspectives related to different topics. These topics include, but are not restricted to, social science studies , which investigate why and who falls for fake news (Altay et al. 2022 ; Batailler et al. 2022 ; Sterret et al. 2018 ; Badawy et al. 2019 ; Pennycook and Rand 2020 ; Weiss et al. 2020 ; Guadagno and Guttieri 2021 ), whom to trust and how perceptions of misinformation and disinformation relate to media trust and media consumption patterns (Hameleers et al. 2022 ), how fake news differs from personal lies (Chiu and Oh 2021 ; Escolà-Gascón 2021 ), examine how can the law regulate digital disinformation and how governments can regulate the values of social media companies that themselves regulate disinformation spread on their platforms (Marsden et al. 2020 ; Schuyler 2019 ; Vasu et al. 2018 ; Burshtein 2017 ; Waldman 2017 ; Alemanno 2018 ; Verstraete et al. 2017 ), and argue the challenges to democracy (Jungherr and Schroeder 2021 ); Behavioral interventions studies , which examine what literacy ideas mean in the age of dis/mis- and malinformation (Carmi et al. 2020 ), investigate whether media literacy helps identification of fake news (Jones-Jang et al. 2021 ) and attempt to improve people’s news literacy (Apuke et al. 2022 ; Dame Adjin-Tettey 2022 ; Hameleers 2022 ; Nagel 2022 ; Jones-Jang et al. 2021 ; Mihailidis and Viotty 2017 ; García et al. 2020 ) by encouraging people to pause to assess credibility of headlines (Fazio 2020 ), promote civic online reasoning (McGrew 2020 ; McGrew et al. 2018 ) and critical thinking (Lutzke et al. 2019 ), together with evaluations of credibility indicators (Bhuiyan et al. 2020 ; Nygren et al. 2019 ; Shao et al. 2018a ; Pennycook et al. 2020a , b ; Clayton et al. 2020 ; Ozturk et al. 2015 ; Metzger et al. 2020 ; Sherman et al. 2020 ; Nekmat 2020 ; Brashier et al. 2021 ; Chung and Kim 2021 ; Lanius et al. 2021 ); as well as social media-driven studies , which investigate the effect of signals (e.g., sources) to detect and recognize fake news (Vraga and Bode 2017 ; Jakesch et al. 2019 ; Shen et al. 2019 ; Avram et al. 2020 ; Hameleers et al. 2020 ; Dias et al. 2020 ; Nyhan et al. 2020 ; Bode and Vraga 2015 ; Tsang 2020 ; Vishwakarma et al. 2019 ; Yavary et al. 2020 ) and investigate fake and reliable news sources using complex networks analysis based on search engine optimization metric (Mazzeo and Rapisarda 2022 ).
The impacts of fake news have reached various areas and disciplines beyond online social networks and society (García et al. 2020 ) such as economics (Clarke et al. 2020 ; Kogan et al. 2019 ; Goldstein and Yang 2019 ), psychology (Roozenbeek et al. 2020a ; Van der Linden and Roozenbeek 2020 ; Roozenbeek and van der Linden 2019 ), political science (Valenzuela et al. 2022 ; Bringula et al. 2022 ; Ricard and Medeiros 2020 ; Van der Linden et al. 2020 ; Allcott and Gentzkow 2017 ; Grinberg et al. 2019 ; Guess et al. 2019 ; Baptista and Gradim 2020 ), health science (Alonso-Galbán and Alemañy-Castilla 2022 ; Desai et al. 2022 ; Apuke and Omar 2021 ; Escolà-Gascón 2021 ; Wang et al. 2019c ; Hartley and Vu 2020 ; Micallef et al. 2020 ; Pennycook et al. 2020b ; Sharma et al. 2020 ; Roozenbeek et al. 2020b ), environmental science (e.g., climate change) (Treen et al. 2020 ; Lutzke et al. 2019 ; Lewandowsky 2020 ; Maertens et al. 2020 ), etc.
Interesting research has been carried out to review and study the fake news issue in online social networks. Some focus not only on fake news, but also distinguish between fake news and rumor (Bondielli and Marcelloni 2019 ; Meel and Vishwakarma 2020 ), while others tackle the whole problem, from characterization to processing techniques (Shu et al. 2017 ; Guo et al. 2020 ; Zhou and Zafarani 2020 ). However, they mostly focus on studying approaches from a machine learning perspective (Bondielli and Marcelloni 2019 ), data mining perspective (Shu et al. 2017 ), crowd intelligence perspective (Guo et al. 2020 ), or knowledge-based perspective (Zhou and Zafarani 2020 ). Furthermore, most of these studies ignore at least one of the mentioned perspectives, and in many cases, they do not cover other existing detection approaches using methods such as blockchain and fact-checking, as well as analysis on metrics used for Search Engine Optimization (Mazzeo and Rapisarda 2022 ). However, in our work and to the best of our knowledge, we cover all the approaches used for fake news detection. Indeed, we investigate the proposed solutions from broader perspectives (i.e., the detection techniques that are used, as well as the different aspects and types of the information used).
Therefore, in this paper, we are highly motivated by the following facts. First, fake news detection on social media is still in the early age of development, and many challenging issues remain that require deeper investigation. Hence, it is necessary to discuss potential research directions that can improve fake news detection and mitigation tasks. However, the dynamic nature of fake news propagation through social networks further complicates matters (Sharma et al. 2019 ). False information can easily reach and impact a large number of users in a short time (Friggeri et al. 2014 ; Qian et al. 2018 ). Moreover, fact-checking organizations cannot keep up with the dynamics of propagation as they require human verification, which can hold back a timely and cost-effective response (Kim et al. 2018 ; Ruchansky et al. 2017 ; Shu et al. 2018a ).
Our work focuses primarily on understanding the “fake news” problem, its related challenges and root causes, and reviewing automatic fake news detection and mitigation methods in online social networks as addressed by researchers. The main contributions that differentiate us from other works are summarized below:
We present the general context from which the fake news problem emerged (i.e., online deception)
We review existing definitions of fake news, identify the terms and features most commonly used to define fake news, and categorize related works accordingly.
We propose a fake news typology classification based on the various categorizations of fake news reported in the literature.
We point out the most challenging factors preventing researchers from proposing highly effective solutions for automatic fake news detection in social media.
We highlight and classify representative studies in the domain of automatic fake news detection and mitigation on online social networks including the key methods and techniques used to generate detection models.
We discuss the key shortcomings that may inhibit the effectiveness of the proposed fake news detection methods in online social networks.
We provide recommendations that can help address these shortcomings and improve the quality of research in this domain.
The rest of this article is organized as follows. We explain the methodology with which the studied references are collected and selected in Sect. 2 . We introduce the online deception problem in Sect. 3 . We highlight the modern-day problem of fake news in Sect. 4 , followed by challenges facing fake news detection and mitigation tasks in Sect. 5 . We provide a comprehensive literature review of the most relevant scholarly works on fake news detection in Sect. 6 . We provide a critical discussion and recommendations that may fill some of the gaps we have identified, as well as a classification of the reviewed automatic fake news detection approaches, in Sect. 7 . Finally, we provide a conclusion and propose some future directions in Sect. 8 .
2 Review methodology
This section introduces the systematic review methodology on which we relied to perform our study. We start with the formulation of the research questions, which allowed us to select the relevant research literature. Then, we provide the different sources of information together with the search and inclusion/exclusion criteria we used to select the final set of papers.
2.1 Research questions formulation
The research scope, research questions, and inclusion/exclusion criteria were established following an initial evaluation of the literature and the following research questions were formulated and addressed.
RQ1: what is fake news in social media, how is it defined in the literature, what are its related concepts, and the different types of it?
RQ2: What are the existing challenges and issues related to fake news?
RQ3: What are the available techniques used to perform fake news detection in social media?
2.2 Sources of information
We broadly searched for journal and conference research articles, books, and magazines as a source of data to extract relevant articles. We used the main sources of scientific databases and digital libraries in our search, such as Google Scholar, Footnote 19 IEEE Xplore, Footnote 20 Springer Link, Footnote 21 ScienceDirect, Footnote 22 Scopus, Footnote 23 ACM Digital Library. Footnote 24 Also, we screened most of the related high-profile conferences such as WWW, SIGKDD, VLDB, ICDE and so on to find out the recent work.
2.3 Search criteria
We focused our research over a period of ten years, but we made sure that about two-thirds of the research papers that we considered were published in or after 2019. Additionally, we defined a set of keywords to search the above-mentioned scientific databases since we concentrated on reviewing the current state of the art in addition to the challenges and the future direction. The set of keywords includes the following terms: fake news, disinformation, misinformation, information disorder, social media, detection techniques, detection methods, survey, literature review.
2.4 Study selection, exclusion and inclusion criteria
To retrieve relevant research articles, based on our sources of information and search criteria, a systematic keyword-based search was carried out by posing different search queries, as shown in Table 1 .
We discovered a primary list of articles. On the obtained initial list of studies, we applied a set of inclusion/exclusion criteria presented in Table 2 to select the appropriate research papers. The inclusion and exclusion principles are applied to determine whether a study should be included or not.
After reading the abstract, we excluded some articles that did not meet our criteria. We chose the most important research to help us understand the field. We reviewed the articles completely and found only 61 research papers that discuss the definition of the term fake news and its related concepts (see Table 4 ). We used the remaining papers to understand the field, reveal the challenges, review the detection techniques, and discuss future directions.
3 A brief introduction of online deception
The Cambridge Online Dictionary defines Deception as “ the act of hiding the truth, especially to get an advantage .” Deception relies on peoples’ trust, doubt and strong emotions that may prevent them from thinking and acting clearly (Aïmeur et al. 2018 ). We also define it in previous work (Aïmeur et al. 2018 ) as the process that undermines the ability to consciously make decisions and take convenient actions, following personal values and boundaries. In other words, deception gets people to do things they would not otherwise do. In the context of online deception, several factors need to be considered: the deceiver, the purpose or aim of the deception, the social media service, the deception technique and the potential target (Aïmeur et al. 2018 ; Hage et al. 2021 ).
Researchers are working on developing new ways to protect users and prevent online deception (Aïmeur et al. 2018 ). Due to the sophistication of attacks, this is a complex task. Hence, malicious attackers are using more complex tools and strategies to deceive users. Furthermore, the way information is organized and exchanged in social media may lead to exposing OSN users to many risks (Aïmeur et al. 2013 ).
In fact, this field is one of the recent research areas that need collaborative efforts of multidisciplinary practices such as psychology, sociology, journalism, computer science as well as cyber-security and digital marketing (which are not yet well explored in the field of dis/mis/malinformation but relevant for future research). Moreover, Ismailov et al. ( 2020 ) analyzed the main causes that could be responsible for the efficiency gap between laboratory results and real-world implementations.
In this paper, it is not in our scope of work to review online deception state of the art. However, we think it is crucial to note that fake news, misinformation and disinformation are indeed parts of the larger landscape of online deception (Hage et al. 2021 ).
4 Fake news, the modern-day problem
Fake news has existed for a very long time, much before their wide circulation became facilitated by the invention of the printing press. Footnote 25 For instance, Socrates was condemned to death more than twenty-five hundred years ago under the fake news that he was guilty of impiety against the pantheon of Athens and corruption of the youth. Footnote 26 A Google Trends Analysis of the term “fake news” reveals an explosion in popularity around the time of the 2016 US presidential election. Footnote 27 Fake news detection is a problem that has recently been addressed by numerous organizations, including the European Union Footnote 28 and NATO. Footnote 29
In this section, we first overview the fake news definitions as they were provided in the literature. We identify the terms and features used in the definitions, and we classify the latter based on them. Then, we provide a fake news typology based on distinct categorizations that we propose, and we define and compare the most cited forms of one specific fake news category (i.e., the intent-based fake news category).
4.1 Definitions of fake news
“Fake news” is defined in the Collins English Dictionary as false and often sensational information disseminated under the guise of news reporting, Footnote 30 yet the term has evolved over time and has become synonymous with the spread of false information (Cooke 2017 ).
The first definition of the term fake news was provided by Allcott and Gentzkow ( 2017 ) as news articles that are intentionally and verifiably false and could mislead readers. Then, other definitions were provided in the literature, but they all agree on the authenticity of fake news to be false (i.e., being non-factual). However, they disagree on the inclusion and exclusion of some related concepts such as satire , rumors , conspiracy theories , misinformation and hoaxes from the given definition. More recently, Nakov ( 2020 ) reported that the term fake news started to mean different things to different people, and for some politicians, it even means “news that I do not like.”
Hence, there is still no agreed definition of the term “fake news.” Moreover, we can find many terms and concepts in the literature that refer to fake news (Van der Linden et al. 2020 ; Molina et al. 2021 ) (Abu Arqoub et al. 2022 ; Allen et al. 2020 ; Allcott and Gentzkow 2017 ; Shu et al. 2017 ; Sharma et al. 2019 ; Zhou and Zafarani 2020 ; Zhang and Ghorbani 2020 ; Conroy et al. 2015 ; Celliers and Hattingh 2020 ; Nakov 2020 ; Shu et al. 2020c ; Jin et al. 2016 ; Rubin et al. 2016 ; Balmas 2014 ; Brewer et al. 2013 ; Egelhofer and Lecheler 2019 ; Mustafaraj and Metaxas 2017 ; Klein and Wueller 2017 ; Potthast et al. 2017 ; Lazer et al. 2018 ; Weiss et al. 2020 ; Tandoc Jr et al. 2021 ; Guadagno and Guttieri 2021 ), disinformation (Kapantai et al. 2021 ; Shu et al. 2020a , c ; Kumar et al. 2016 ; Bhattacharjee et al. 2020 ; Marsden et al. 2020 ; Jungherr and Schroeder 2021 ; Starbird et al. 2019 ; Ireton and Posetti 2018 ), misinformation (Wu et al. 2019 ; Shu et al. 2020c ; Shao et al. 2016 , 2018b ; Pennycook and Rand 2019 ; Micallef et al. 2020 ), malinformation (Dame Adjin-Tettey 2022 ) (Carmi et al. 2020 ; Shu et al. 2020c ), false information (Kumar and Shah 2018 ; Guo et al. 2020 ; Habib et al. 2019 ), information disorder (Shu et al. 2020c ; Wardle and Derakhshan 2017 ; Wardle 2018 ; Derakhshan and Wardle 2017 ), information warfare (Guadagno and Guttieri 2021 ) and information pollution (Meel and Vishwakarma 2020 ).
There is also a remarkable amount of disagreement over the classification of the term fake news in the research literature, as well as in policy (de Cock Buning 2018 ; ERGA 2018 , 2021 ). Some consider fake news as a type of misinformation (Allen et al. 2020 ; Singh et al. 2021 ; Ha et al. 2021 ; Pennycook and Rand 2019 ; Shao et al. 2018b ; Di Domenico et al. 2021 ; Sharma et al. 2019 ; Celliers and Hattingh 2020 ; Klein and Wueller 2017 ; Potthast et al. 2017 ; Islam et al. 2020 ), others consider it as a type of disinformation (de Cock Buning 2018 ) (Bringula et al. 2022 ; Baptista and Gradim 2022 ; Tsang 2020 ; Tandoc Jr et al. 2021 ; Bastick 2021 ; Khan et al. 2019 ; Shu et al. 2017 ; Nakov 2020 ; Shu et al. 2020c ; Egelhofer and Lecheler 2019 ), while others associate the term with both disinformation and misinformation (Wu et al. 2022 ; Dame Adjin-Tettey 2022 ; Hameleers et al. 2022 ; Carmi et al. 2020 ; Allcott and Gentzkow 2017 ; Zhang and Ghorbani 2020 ; Potthast et al. 2017 ; Weiss et al. 2020 ; Tandoc Jr et al. 2021 ; Guadagno and Guttieri 2021 ). On the other hand, some prefer to differentiate fake news from both terms (ERGA 2018 ; Molina et al. 2021 ; ERGA 2021 ) (Zhou and Zafarani 2020 ; Jin et al. 2016 ; Rubin et al. 2016 ; Balmas 2014 ; Brewer et al. 2013 ).
The existing terms can be separated into two groups. The first group represents the general terms, which are information disorder , false information and fake news , each of which includes a subset of terms from the second group. The second group represents the elementary terms, which are misinformation , disinformation and malinformation . The literature agrees on the definitions of the latter group, but there is still no agreed-upon definition of the first group. In Fig. 2 , we model the relationship between the most used terms in the literature.
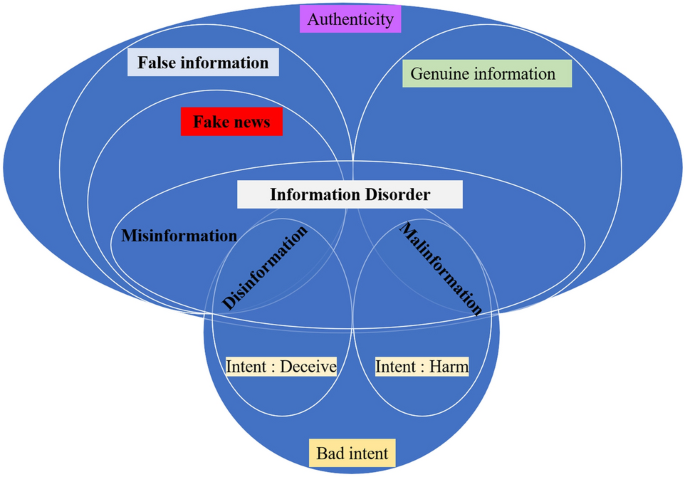
Modeling of the relationship between terms related to fake news
The terms most used in the literature to refer, categorize and classify fake news can be summarized and defined as shown in Table 3 , in which we capture the similarities and show the differences between the different terms based on two common key features, which are the intent and the authenticity of the news content. The intent feature refers to the intention behind the term that is used (i.e., whether or not the purpose is to mislead or cause harm), whereas the authenticity feature refers to its factual aspect. (i.e., whether the content is verifiably false or not, which we label as genuine in the second case). Some of these terms are explicitly used to refer to fake news (i.e., disinformation, misinformation and false information), while others are not (i.e., malinformation). In the comparison table, the empty dash (–) cell denotes that the classification does not apply.
In Fig. 3 , we identify the different features used in the literature to define fake news (i.e., intent, authenticity and knowledge). Hence, some definitions are based on two key features, which are authenticity and intent (i.e., news articles that are intentionally and verifiably false and could mislead readers). However, other definitions are based on either authenticity or intent. Other researchers categorize false information on the web and social media based on its intent and knowledge (i.e., when there is a single ground truth). In Table 4 , we classify the existing fake news definitions based on the used term and the used features . In the classification, the references in the cells refer to the research study in which a fake news definition was provided, while the empty dash (–) cells denote that the classification does not apply.
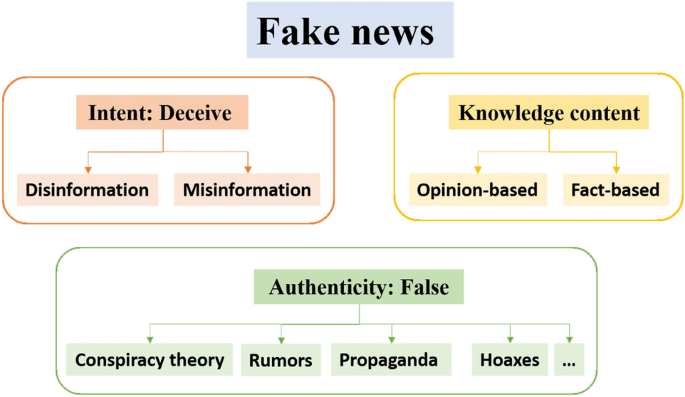
The features used for fake news definition
4.2 Fake news typology
Various categorizations of fake news have been provided in the literature. We can distinguish two major categories of fake news based on the studied perspective (i.e., intention or content) as shown in Fig. 4 . However, our proposed fake news typology is not about detection methods, and it is not exclusive. Hence, a given category of fake news can be described based on both perspectives (i.e., intention and content) at the same time. For instance, satire (i.e., intent-based fake news) can contain text and/or multimedia content types of data (e.g., headline, body, image, video) (i.e., content-based fake news) and so on.
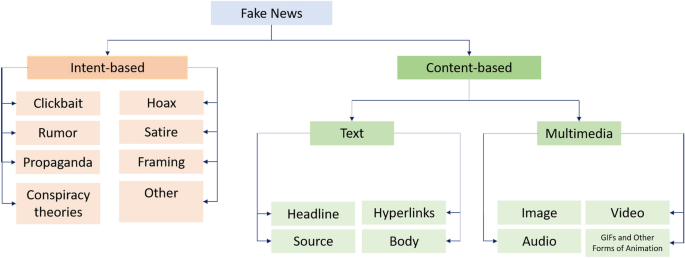
Fake news typology
Most researchers classify fake news based on the intent (Collins et al. 2020 ; Bondielli and Marcelloni 2019 ; Zannettou et al. 2019 ; Kumar et al. 2016 ; Wardle 2017 ; Shu et al. 2017 ; Kumar and Shah 2018 ) (see Sect. 4.2.2 ). However, other researchers (Parikh and Atrey 2018 ; Fraga-Lamas and Fernández-Caramés 2020 ; Hasan and Salah 2019 ; Masciari et al. 2020 ; Bakdash et al. 2018 ; Elhadad et al. 2019 ; Yang et al. 2019b ) focus on the content to categorize types of fake news through distinguishing the different formats and content types of data in the news (e.g., text and/or multimedia).
Recently, another classification was proposed by Zhang and Ghorbani ( 2020 ). It is based on the combination of content and intent to categorize fake news. They distinguish physical news content and non-physical news content from fake news. Physical content consists of the carriers and format of the news, and non-physical content consists of the opinions, emotions, attitudes and sentiments that the news creators want to express.
4.2.1 Content-based fake news category
According to researchers of this category (Parikh and Atrey 2018 ; Fraga-Lamas and Fernández-Caramés 2020 ; Hasan and Salah 2019 ; Masciari et al. 2020 ; Bakdash et al. 2018 ; Elhadad et al. 2019 ; Yang et al. 2019b ), forms of fake news may include false text such as hyperlinks or embedded content; multimedia such as false videos (Demuyakor and Opata 2022 ), images (Masciari et al. 2020 ; Shen et al. 2019 ), audios (Demuyakor and Opata 2022 ) and so on. Moreover, we can also find multimodal content (Shu et al. 2020a ) that is fake news articles and posts composed of multiple types of data combined together, for example, a fabricated image along with a text related to the image (Shu et al. 2020a ). In this category of fake news forms, we can mention as examples deepfake videos (Yang et al. 2019b ) and GAN-generated fake images (Zhang et al. 2019b ), which are artificial intelligence-based machine-generated fake content that are hard for unsophisticated social network users to identify.
The effects of these forms of fake news content vary on the credibility assessment, as well as sharing intentions which influences the spread of fake news on OSNs. For instance, people with little knowledge about the issue compared to those who are strongly concerned about the key issue of fake news tend to be easier to convince that the misleading or fake news is real, especially when shared via a video modality as compared to the text or the audio modality (Demuyakor and Opata 2022 ).
4.2.2 Intent-based Fake News Category
The most often mentioned and discussed forms of fake news according to researchers in this category include but are not restricted to clickbait , hoax , rumor , satire , propaganda , framing , conspiracy theories and others. In the following subsections, we explain these types of fake news as they were defined in the literature and undertake a brief comparison between them as depicted in Table 5 . The following are the most cited forms of intent-based types of fake news, and their comparison is based on what we suspect are the most common criteria mentioned by researchers.
Clickbait refers to misleading headlines and thumbnails of content on the web (Zannettou et al. 2019 ) that tend to be fake stories with catchy headlines aimed at enticing the reader to click on a link (Collins et al. 2020 ). This type of fake news is considered to be the least severe type of false information because if a user reads/views the whole content, it is possible to distinguish if the headline and/or the thumbnail was misleading (Zannettou et al. 2019 ). However, the goal behind using clickbait is to increase the traffic to a website (Zannettou et al. 2019 ).
A hoax is a false (Zubiaga et al. 2018 ) or inaccurate (Zannettou et al. 2019 ) intentionally fabricated (Collins et al. 2020 ) news story used to masquerade the truth (Zubiaga et al. 2018 ) and is presented as factual (Zannettou et al. 2019 ) to deceive the public or audiences (Collins et al. 2020 ). This category is also known either as half-truth or factoid stories (Zannettou et al. 2019 ). Popular examples of hoaxes are stories that report the false death of celebrities (Zannettou et al. 2019 ) and public figures (Collins et al. 2020 ). Recently, hoaxes about the COVID-19 have been circulating through social media.
The term rumor refers to ambiguous or never confirmed claims (Zannettou et al. 2019 ) that are disseminated with a lack of evidence to support them (Sharma et al. 2019 ). This kind of information is widely propagated on OSNs (Zannettou et al. 2019 ). However, they are not necessarily false and may turn out to be true (Zubiaga et al. 2018 ). Rumors originate from unverified sources but may be true or false or remain unresolved (Zubiaga et al. 2018 ).
Satire refers to stories that contain a lot of irony and humor (Zannettou et al. 2019 ). It presents stories as news that might be factually incorrect, but the intent is not to deceive but rather to call out, ridicule, or to expose behavior that is shameful, corrupt, or otherwise “bad” (Golbeck et al. 2018 ). This is done with a fabricated story or by exaggerating the truth reported in mainstream media in the form of comedy (Collins et al. 2020 ). The intent behind satire seems kind of legitimate and many authors (such as Wardle (Wardle 2017 )) do include satire as a type of fake news as there is no intention to cause harm but it has the potential to mislead or fool people.
Also, Golbeck et al. ( 2018 ) mention that there is a spectrum from fake to satirical news that they found to be exploited by many fake news sites. These sites used disclaimers at the bottom of their webpages to suggest they were “satirical” even when there was nothing satirical about their articles, to protect them from accusations about being fake. The difference with a satirical form of fake news is that the authors or the host present themselves as a comedian or as an entertainer rather than a journalist informing the public (Collins et al. 2020 ). However, most audiences believed the information passed in this satirical form because the comedian usually projects news from mainstream media and frames them to suit their program (Collins et al. 2020 ).
Propaganda refers to news stories created by political entities to mislead people. It is a special instance of fabricated stories that aim to harm the interests of a particular party and, typically, has a political context (Zannettou et al. 2019 ). Propaganda was widely used during both World Wars (Collins et al. 2020 ) and during the Cold War (Zannettou et al. 2019 ). It is a consequential type of false information as it can change the course of human history (e.g., by changing the outcome of an election) (Zannettou et al. 2019 ). States are the main actors of propaganda. Recently, propaganda has been used by politicians and media organizations to support a certain position or view (Collins et al. 2020 ). Online astroturfing can be an example of the tools used for the dissemination of propaganda. It is a covert manipulation of public opinion (Peng et al. 2017 ) that aims to make it seem that many people share the same opinion about something. Astroturfing can affect different domains of interest, based on which online astroturfing can be mainly divided into political astroturfing, corporate astroturfing and astroturfing in e-commerce or online services (Mahbub et al. 2019 ). Propaganda types of fake news can be debunked with manual fact-based detection models such as the use of expert-based fact-checkers (Collins et al. 2020 ).
Framing refers to employing some aspect of reality to make content more visible, while the truth is concealed (Collins et al. 2020 ) to deceive and misguide readers. People will understand certain concepts based on the way they are coined and invented. An example of framing was provided by Collins et al. ( 2020 ): “suppose a leader X says “I will neutralize my opponent” simply meaning he will beat his opponent in a given election. Such a statement will be framed such as “leader X threatens to kill Y” and this framed statement provides a total misrepresentation of the original meaning.
Conspiracy Theories
Conspiracy theories refer to the belief that an event is the result of secret plots generated by powerful conspirators. Conspiracy belief refers to people’s adoption and belief of conspiracy theories, and it is associated with psychological, political and social factors (Douglas et al. 2019 ). Conspiracy theories are widespread in contemporary democracies (Sutton and Douglas 2020 ), and they have major consequences. For instance, lately and during the COVID-19 pandemic, conspiracy theories have been discussed from a public health perspective (Meese et al. 2020 ; Allington et al. 2020 ; Freeman et al. 2020 ).
4.2.3 Comparison Between Most Popular Intent-based Types of Fake News
Following a review of the most popular intent-based types of fake news, we compare them as shown in Table 5 based on the most common criteria mentioned by researchers in their definitions as listed below.
the intent behind the news, which refers to whether a given news type was mainly created to intentionally deceive people or not (e.g., humor, irony, entertainment, etc.);
the way that the news propagates through OSN, which determines the nature of the propagation of each type of fake news and this can be either fast or slow propagation;
the severity of the impact of the news on OSN users, which refers to whether the public has been highly impacted by the given type of fake news; the mentioned impact of each fake news type is mainly the proportion of the negative impact;
and the goal behind disseminating the news, which can be to gain popularity for a particular entity (e.g., political party), for profit (e.g., lucrative business), or other reasons such as humor and irony in the case of satire, spreading panic or anger, and manipulating the public in the case of hoaxes, made-up stories about a particular person or entity in the case of rumors, and misguiding readers in the case of framing.
However, the comparison provided in Table 5 is deduced from the studied research papers; it is our point of view, which is not based on empirical data.
We suspect that the most dangerous types of fake news are the ones with high intention to deceive the public, fast propagation through social media, high negative impact on OSN users, and complicated hidden goals and agendas. However, while the other types of fake news are less dangerous, they should not be ignored.
Moreover, it is important to highlight that the existence of the overlap in the types of fake news mentioned above has been proven, thus it is possible to observe false information that may fall within multiple categories (Zannettou et al. 2019 ). Here, we provide two examples by Zannettou et al. ( 2019 ) to better understand possible overlaps: (1) a rumor may also use clickbait techniques to increase the audience that will read the story; and (2) propaganda stories, as a special instance of a framing story.
5 Challenges related to fake news detection and mitigation
To alleviate fake news and its threats, it is crucial to first identify and understand the factors involved that continue to challenge researchers. Thus, the main question is to explore and investigate the factors that make it easier to fall for manipulated information. Despite the tremendous progress made in alleviating some of the challenges in fake news detection (Sharma et al. 2019 ; Zhou and Zafarani 2020 ; Zhang and Ghorbani 2020 ; Shu et al. 2020a ), much more work needs to be accomplished to address the problem effectively.
In this section, we discuss several open issues that have been making fake news detection in social media a challenging problem. These issues can be summarized as follows: content-based issues (i.e., deceptive content that resembles the truth very closely), contextual issues (i.e., lack of user awareness, social bots spreaders of fake content, and OSN’s dynamic natures that leads to the fast propagation), as well as the issue of existing datasets (i.e., there still no one size fits all benchmark dataset for fake news detection). These various aspects have proven (Shu et al. 2017 ) to have a great impact on the accuracy of fake news detection approaches.
5.1 Content-based issue, deceptive content
Automatic fake news detection remains a huge challenge, primarily because the content is designed in a way that it closely resembles the truth. Besides, most deceivers choose their words carefully and use their language strategically to avoid being caught. Therefore, it is often hard to determine its veracity by AI without the reliance on additional information from third parties such as fact-checkers.
Abdullah-All-Tanvir et al. ( 2020 ) reported that fake news tends to have more complicated stories and hardly ever make any references. It is more likely to contain a greater number of words that express negative emotions. This makes it so complicated that it becomes impossible for a human to manually detect the credibility of this content. Therefore, detecting fake news on social media is quite challenging. Moreover, fake news appears in multiple types and forms, which makes it hard and challenging to define a single global solution able to capture and deal with the disseminated content. Consequently, detecting false information is not a straightforward task due to its various types and forms Zannettou et al. ( 2019 ).
5.2 Contextual issues
Contextual issues are challenges that we suspect may not be related to the content of the news but rather they are inferred from the context of the online news post (i.e., humans are the weakest factor due to lack of user awareness, social bots spreaders, dynamic nature of online social platforms and fast propagation of fake news).
5.2.1 Humans are the weakest factor due to the lack of awareness
Recent statistics Footnote 31 show that the percentage of unintentional fake news spreaders (people who share fake news without the intention to mislead) over social media is five times higher than intentional spreaders. Moreover, another recent statistic Footnote 32 shows that the percentage of people who were confident about their ability to discern fact from fiction is ten times higher than those who were not confident about the truthfulness of what they are sharing. As a result, we can deduce the lack of human awareness about the ascent of fake news.
Public susceptibility and lack of user awareness (Sharma et al. 2019 ) have always been the most challenging problem when dealing with fake news and misinformation. This is a complex issue because many people believe almost everything on the Internet and the ones who are new to digital technology or have less expertise may be easily fooled (Edgerly et al. 2020 ).
Moreover, it has been widely proven (Metzger et al. 2020 ; Edgerly et al. 2020 ) that people are often motivated to support and accept information that goes with their preexisting viewpoints and beliefs, and reject information that does not fit in as well. Hence, Shu et al. ( 2017 ) illustrate an interesting correlation between fake news spread and psychological and cognitive theories. They further suggest that humans are more likely to believe information that confirms their existing views and ideological beliefs. Consequently, they deduce that humans are naturally not very good at differentiating real information from fake information.
Recent research by Giachanou et al. ( 2020 ) studies the role of personality and linguistic patterns in discriminating between fake news spreaders and fact-checkers. They classify a user as a potential fact-checker or a potential fake news spreader based on features that represent users’ personality traits and linguistic patterns used in their tweets. They show that leveraging personality traits and linguistic patterns can improve the performance in differentiating between checkers and spreaders.
Furthermore, several researchers studied the prevalence of fake news on social networks during (Allcott and Gentzkow 2017 ; Grinberg et al. 2019 ; Guess et al. 2019 ; Baptista and Gradim 2020 ) and after (Garrett and Bond 2021 ) the 2016 US presidential election and found that individuals most likely to engage with fake news sources were generally conservative-leaning, older, and highly engaged with political news.
Metzger et al. ( 2020 ) examine how individuals evaluate the credibility of biased news sources and stories. They investigate the role of both cognitive dissonance and credibility perceptions in selective exposure to attitude-consistent news information. They found that online news consumers tend to perceive attitude-consistent news stories as more accurate and more credible than attitude-inconsistent stories.
Similarly, Edgerly et al. ( 2020 ) explore the impact of news headlines on the audience’s intent to verify whether given news is true or false. They concluded that participants exhibit higher intent to verify the news only when they believe the headline to be true, which is predicted by perceived congruence with preexisting ideological tendencies.
Luo et al. ( 2022 ) evaluate the effects of endorsement cues in social media on message credibility and detection accuracy. Results showed that headlines associated with a high number of likes increased credibility, thereby enhancing detection accuracy for real news but undermining accuracy for fake news. Consequently, they highlight the urgency of empowering individuals to assess both news veracity and endorsement cues appropriately on social media.
Moreover, misinformed people are a greater problem than uninformed people (Kuklinski et al. 2000 ), because the former hold inaccurate opinions (which may concern politics, climate change, medicine) that are harder to correct. Indeed, people find it difficult to update their misinformation-based beliefs even after they have been proved to be false (Flynn et al. 2017 ). Moreover, even if a person has accepted the corrected information, his/her belief may still affect their opinion (Nyhan and Reifler 2015 ).
Falling for disinformation may also be explained by a lack of critical thinking and of the need for evidence that supports information (Vilmer et al. 2018 ; Badawy et al. 2019 ). However, it is also possible that people choose misinformation because they engage in directionally motivated reasoning (Badawy et al. 2019 ; Flynn et al. 2017 ). Online clients are normally vulnerable and will, in general, perceive web-based networking media as reliable, as reported by Abdullah-All-Tanvir et al. ( 2019 ), who propose to mechanize fake news recognition.
It is worth noting that in addition to bots causing the outpouring of the majority of the misrepresentations, specific individuals are also contributing a large share of this issue (Abdullah-All-Tanvir et al. 2019 ). Furthermore, Vosoughi et al. (Vosoughi et al. 2018 ) found that contrary to conventional wisdom, robots have accelerated the spread of real and fake news at the same rate, implying that fake news spreads more than the truth because humans, not robots, are more likely to spread it.
In this case, verified users and those with numerous followers were not necessarily responsible for spreading misinformation of the corrupted posts (Abdullah-All-Tanvir et al. 2019 ).
Viral fake news can cause much havoc to our society. Therefore, to mitigate the negative impact of fake news, it is important to analyze the factors that lead people to fall for misinformation and to further understand why people spread fake news (Cheng et al. 2020 ). Measuring the accuracy, credibility, veracity and validity of news contents can also be a key countermeasure to consider.
5.2.2 Social bots spreaders
Several authors (Shu et al. 2018b , 2017 ; Shi et al. 2019 ; Bessi and Ferrara 2016 ; Shao et al. 2018a ) have also shown that fake news is likely to be created and spread by non-human accounts with similar attributes and structure in the network, such as social bots (Ferrara et al. 2016 ). Bots (short for software robots) exist since the early days of computers. A social bot is a computer algorithm that automatically produces content and interacts with humans on social media, trying to emulate and possibly alter their behavior (Ferrara et al. 2016 ). Although they are designed to provide a useful service, they can be harmful, for example when they contribute to the spread of unverified information or rumors (Ferrara et al. 2016 ). However, it is important to note that bots are simply tools created and maintained by humans for some specific hidden agendas.
Social bots tend to connect with legitimate users instead of other bots. They try to act like a human with fewer words and fewer followers on social media. This contributes to the forwarding of fake news (Jiang et al. 2019 ). Moreover, there is a difference between bot-generated and human-written clickbait (Le et al. 2019 ).
Many researchers have addressed ways of identifying and analyzing possible sources of fake news spread in social media. Recent research by Shu et al. ( 2020a ) describes social bots use of two strategies to spread low-credibility content. First, they amplify interactions with content as soon as it is created to make it look legitimate and to facilitate its spread across social networks. Next, they try to increase public exposure to the created content and thus boost its perceived credibility by targeting influential users that are more likely to believe disinformation in the hope of getting them to “repost” the fabricated content. They further discuss the social bot detection systems taxonomy proposed by Ferrara et al. ( 2016 ) which divides bot detection methods into three classes: (1) graph-based, (2) crowdsourcing and (3) feature-based social bot detection methods.
Similarly, Shao et al. ( 2018a ) examine social bots and how they promote the spread of misinformation through millions of Twitter posts during and following the 2016 US presidential campaign. They found that social bots played a disproportionate role in spreading articles from low-credibility sources by amplifying such content in the early spreading moments and targeting users with many followers through replies and mentions to expose them to this content and induce them to share it.
Ismailov et al. ( 2020 ) assert that the techniques used to detect bots depend on the social platform and the objective. They note that a malicious bot designed to make friends with as many accounts as possible will require a different detection approach than a bot designed to repeatedly post links to malicious websites. Therefore, they identify two models for detecting malicious accounts, each using a different set of features. Social context models achieve detection by examining features related to an account’s social presence including features such as relationships to other accounts, similarities to other users’ behaviors, and a variety of graph-based features. User behavior models primarily focus on features related to an individual user’s behavior, such as frequency of activities (e.g., number of tweets or posts per time interval), patterns of activity and clickstream sequences.
Therefore, it is crucial to consider bot detection techniques to distinguish bots from normal users to better leverage user profile features to detect fake news.
However, there is also another “bot-like” strategy that aims to massively promote disinformation and fake content in social platforms, which is called bot farms or also troll farms. It is not social bots, but it is a group of organized individuals engaging in trolling or bot-like promotion of narratives in a coordinated fashion (Wardle 2018 ) hired to massively spread fake news or any other harmful content. A prominent troll farm example is the Russia-based Internet Research Agency (IRA), which disseminated inflammatory content online to influence the outcome of the 2016 U.S. presidential election. Footnote 33 As a result, Twitter suspended accounts connected to the IRA and deleted 200,000 tweets from Russian trolls (Jamieson 2020 ). Another example to mention in this category is review bombing (Moro and Birt 2022 ). Review bombing refers to coordinated groups of people massively performing the same negative actions online (e.g., dislike, negative review/comment) on an online video, game, post, product, etc., in order to reduce its aggregate review score. The review bombers can be both humans and bots coordinated in order to cause harm and mislead people by falsifying facts.
5.2.3 Dynamic nature of online social platforms and fast propagation of fake news
Sharma et al. ( 2019 ) affirm that the fast proliferation of fake news through social networks makes it hard and challenging to assess the information’s credibility on social media. Similarly, Qian et al. ( 2018 ) assert that fake news and fabricated content propagate exponentially at the early stage of its creation and can cause a significant loss in a short amount of time (Friggeri et al. 2014 ) including manipulating the outcome of political events (Liu and Wu 2018 ; Bessi and Ferrara 2016 ).
Moreover, while analyzing the way source and promoters of fake news operate over the web through multiple online platforms, Zannettou et al. ( 2019 ) discovered that false information is more likely to spread across platforms (18% appearing on multiple platforms) compared to real information (11%).
Furthermore, recently, Shu et al. ( 2020c ) attempted to understand the propagation of disinformation and fake news in social media and found that such content is produced and disseminated faster and easier through social media because of the low barriers that prevent doing so. Similarly, Shu et al. ( 2020b ) studied hierarchical propagation networks for fake news detection. They performed a comparative analysis between fake and real news from structural, temporal and linguistic perspectives. They demonstrated the potential of using these features to detect fake news and they showed their effectiveness for fake news detection as well.
Lastly, Abdullah-All-Tanvir et al. ( 2020 ) note that it is almost impossible to manually detect the sources and authenticity of fake news effectively and efficiently, due to its fast circulation in such a small amount of time. Therefore, it is crucial to note that the dynamic nature of the various online social platforms, which results in the continued rapid and exponential propagation of such fake content, remains a major challenge that requires further investigation while defining innovative solutions for fake news detection.
5.3 Datasets issue
The existing approaches lack an inclusive dataset with derived multidimensional information to detect fake news characteristics to achieve higher accuracy of machine learning classification model performance (Nyow and Chua 2019 ). These datasets are primarily dedicated to validating the machine learning model and are the ultimate frame of reference to train the model and analyze its performance. Therefore, if a researcher evaluates their model based on an unrepresentative dataset, the validity and the efficiency of the model become questionable when it comes to applying the fake news detection approach in a real-world scenario.
Moreover, several researchers (Shu et al. 2020d ; Wang et al. 2020 ; Pathak and Srihari 2019 ; Przybyla 2020 ) believe that fake news is diverse and dynamic in terms of content, topics, publishing methods and media platforms, and sophisticated linguistic styles geared to emulate true news. Consequently, training machine learning models on such sophisticated content requires large-scale annotated fake news data that are difficult to obtain (Shu et al. 2020d ).
Therefore, datasets are also a great topic to work on to enhance data quality and have better results while defining our solutions. Adversarial learning techniques (e.g., GAN, SeqGAN) can be used to provide machine-generated data that can be used to train deeper models and build robust systems to detect fake examples from the real ones. This approach can be used to counter the lack of datasets and the scarcity of data available to train models.
6 Fake news detection literature review
Fake news detection in social networks is still in the early stage of development and there are still challenging issues that need further investigation. This has become an emerging research area that is attracting huge attention.
There are various research studies on fake news detection in online social networks. Few of them have focused on the automatic detection of fake news using artificial intelligence techniques. In this section, we review the existing approaches used in automatic fake news detection, as well as the techniques that have been adopted. Then, a critical discussion built on a primary classification scheme based on a specific set of criteria is also emphasized.
6.1 Categories of fake news detection
In this section, we give an overview of most of the existing automatic fake news detection solutions adopted in the literature. A recent classification by Sharma et al. ( 2019 ) uses three categories of fake news identification methods. Each category is further divided based on the type of existing methods (i.e., content-based, feedback-based and intervention-based methods). However, a review of the literature for fake news detection in online social networks shows that the existing studies can be classified into broader categories based on two major aspects that most authors inspect and make use of to define an adequate solution. These aspects can be considered as major sources of extracted information used for fake news detection and can be summarized as follows: the content-based (i.e., related to the content of the news post) and the contextual aspect (i.e., related to the context of the news post).
Consequently, the studies we reviewed can be classified into three different categories based on the two aspects mentioned above (the third category is hybrid). As depicted in Fig. 5 , fake news detection solutions can be categorized as news content-based approaches, the social context-based approaches that can be divided into network and user-based approaches, and hybrid approaches. The latter combines both content-based and contextual approaches to define the solution.
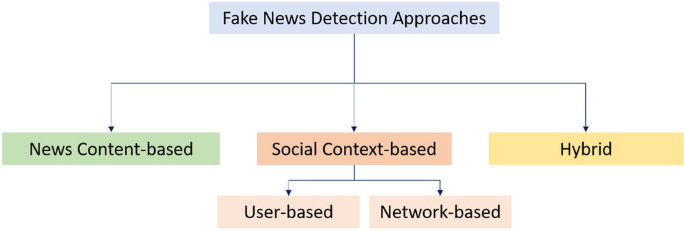
Classification of fake news detection approaches
6.1.1 News Content-based Category
News content-based approaches are fake news detection approaches that use content information (i.e., information extracted from the content of the news post) and that focus on studying and exploiting the news content in their proposed solutions. Content refers to the body of the news, including source, headline, text and image-video, which can reflect subtle differences.
Researchers of this category rely on content-based detection cues (i.e., text and multimedia-based cues), which are features extracted from the content of the news post. Text-based cues are features extracted from the text of the news, whereas multimedia-based cues are features extracted from the images and videos attached to the news. Figure 6 summarizes the most widely used news content representation (i.e., text and multimedia/images) and detection techniques (i.e., machine learning (ML), deep Learning (DL), natural language processing (NLP), fact-checking, crowdsourcing (CDS) and blockchain (BKC)) in news content-based category of fake news detection approaches. Most of the reviewed research works based on news content for fake news detection rely on the text-based cues (Kapusta et al. 2019 ; Kaur et al. 2020 ; Vereshchaka et al. 2020 ; Ozbay and Alatas 2020 ; Wang 2017 ; Nyow and Chua 2019 ; Hosseinimotlagh and Papalexakis 2018 ; Abdullah-All-Tanvir et al. 2019 , 2020 ; Mahabub 2020 ; Bahad et al. 2019 ; Hiriyannaiah et al. 2020 ) extracted from the text of the news content including the body of the news and its headline. However, a few researchers such as Vishwakarma et al. ( 2019 ) and Amri et al. ( 2022 ) try to recognize text from the associated image.
Most researchers of this category rely on artificial intelligence (AI) techniques (such as ML, DL and NLP models) to improve performance in terms of prediction accuracy. Others use different techniques such as fact-checking, crowdsourcing and blockchain. Specifically, the AI- and ML-based approaches in this category are trying to extract features from the news content, which they use later for content analysis and training tasks. In this particular case, the extracted features are the different types of information considered to be relevant for the analysis. Feature extraction is considered as one of the best techniques to reduce data size in automatic fake news detection. This technique aims to choose a subset of features from the original set to improve classification performance (Yazdi et al. 2020 ).
Table 6 lists the distinct features and metadata, as well as the used datasets in the news content-based category of fake news detection approaches.
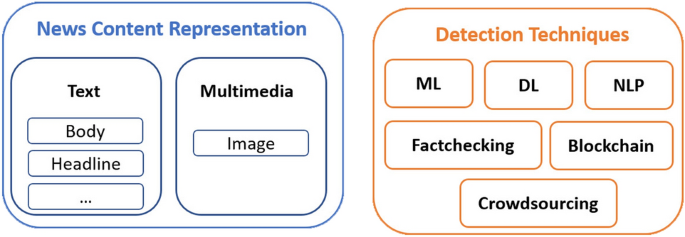
News content-based category: news content representation and detection techniques
6.1.2 Social Context-based Category
Unlike news content-based solutions, the social context-based approaches capture the skeptical social context of the online news (Zhang and Ghorbani 2020 ) rather than focusing on the news content. The social context-based category contains fake news detection approaches that use the contextual aspects (i.e., information related to the context of the news post). These aspects are based on social context and they offer additional information to help detect fake news. They are the surrounding data outside of the fake news article itself, where they can be an essential part of automatic fake news detection. Some useful examples of contextual information may include checking if the news itself and the source that published it are credible, checking the date of the news or the supporting resources, and checking if any other online news platforms are reporting the same or similar stories (Zhang and Ghorbani 2020 ).
Social context-based aspects can be classified into two subcategories, user-based and network-based, and they can be used for context analysis and training tasks in the case of AI- and ML-based approaches. User-based aspects refer to information captured from OSN users such as user profile information (Shu et al. 2019b ; Wang et al. 2019c ; Hamdi et al. 2020 ; Nyow and Chua 2019 ; Jiang et al. 2019 ) and user behavior (Cardaioli et al. 2020 ) such as user engagement (Uppada et al. 2022 ; Jiang et al. 2019 ; Shu et al. 2018b ; Nyow and Chua 2019 ) and response (Zhang et al. 2019a ; Qian et al. 2018 ). Meanwhile, network-based aspects refer to information captured from the properties of the social network where the fake content is shared and disseminated such as news propagation path (Liu and Wu 2018 ; Wu and Liu 2018 ) (e.g., propagation times and temporal characteristics of propagation), diffusion patterns (Shu et al. 2019a ) (e.g., number of retweets, shares), as well as user relationships (Mishra 2020 ; Hamdi et al. 2020 ; Jiang et al. 2019 ) (e.g., friendship status among users).
Figure 7 summarizes some of the most widely adopted social context representations, as well as the most used detection techniques (i.e., AI, ML, DL, fact-checking and blockchain), in the social context-based category of approaches.
Table 7 lists the distinct features and metadata, the adopted detection cues, as well as the used datasets, in the context-based category of fake news detection approaches.
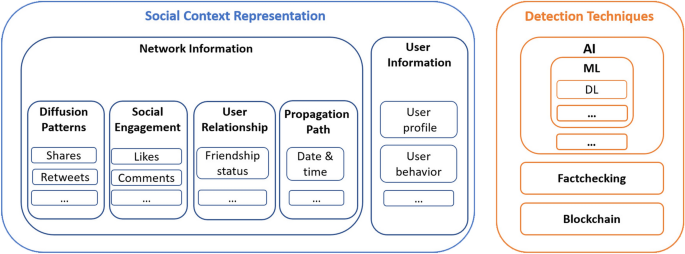
Social context-based category: social context representation and detection techniques
6.1.3 Hybrid approaches
Most researchers are focusing on employing a specific method rather than a combination of both content- and context-based methods. This is because some of them (Wu and Rao 2020 ) believe that there still some challenging limitations in the traditional fusion strategies due to existing feature correlations and semantic conflicts. For this reason, some researchers focus on extracting content-based information, while others are capturing some social context-based information for their proposed approaches.
However, it has proven challenging to successfully automate fake news detection based on just a single type of feature (Ruchansky et al. 2017 ). Therefore, recent directions tend to do a mixture by using both news content-based and social context-based approaches for fake news detection.
Table 8 lists the distinct features and metadata, as well as the used datasets, in the hybrid category of fake news detection approaches.
6.2 Fake news detection techniques
Another vision for classifying automatic fake news detection is to look at techniques used in the literature. Hence, we classify the detection methods based on the techniques into three groups:
Human-based techniques: This category mainly includes the use of crowdsourcing and fact-checking techniques, which rely on human knowledge to check and validate the veracity of news content.
Artificial Intelligence-based techniques: This category includes the most used AI approaches for fake news detection in the literature. Specifically, these are the approaches in which researchers use classical ML, deep learning techniques such as convolutional neural network (CNN), recurrent neural network (RNN), as well as natural language processing (NLP).
Blockchain-based techniques: This category includes solutions using blockchain technology to detect and mitigate fake news in social media by checking source reliability and establishing the traceability of the news content.
6.2.1 Human-based Techniques
One specific research direction for fake news detection consists of using human-based techniques such as crowdsourcing (Pennycook and Rand 2019 ; Micallef et al. 2020 ) and fact-checking (Vlachos and Riedel 2014 ; Chung and Kim 2021 ; Nyhan et al. 2020 ) techniques.
These approaches can be considered as low computational requirement techniques since both rely on human knowledge and expertise for fake news detection. However, fake news identification cannot be addressed solely through human force since it demands a lot of effort in terms of time and cost, and it is ineffective in terms of preventing the fast spread of fake content.
Crowdsourcing. Crowdsourcing approaches (Kim et al. 2018 ) are based on the “wisdom of the crowds” (Collins et al. 2020 ) for fake content detection. These approaches rely on the collective contributions and crowd signals (Tschiatschek et al. 2018 ) of a group of people for the aggregation of crowd intelligence to detect fake news (Tchakounté et al. 2020 ) and to reduce the spread of misinformation on social media (Pennycook and Rand 2019 ; Micallef et al. 2020 ).
Micallef et al. ( 2020 ) highlight the role of the crowd in countering misinformation. They suspect that concerned citizens (i.e., the crowd), who use platforms where disinformation appears, can play a crucial role in spreading fact-checking information and in combating the spread of misinformation.
Recently Tchakounté et al. ( 2020 ) proposed a voting system as a new method of binary aggregation of opinions of the crowd and the knowledge of a third-party expert. The aggregator is based on majority voting on the crowd side and weighted averaging on the third-party site.
Similarly, Huffaker et al. ( 2020 ) propose a crowdsourced detection of emotionally manipulative language. They introduce an approach that transforms classification problems into a comparison task to mitigate conflation content by allowing the crowd to detect text that uses manipulative emotional language to sway users toward positions or actions. The proposed system leverages anchor comparison to distinguish between intrinsically emotional content and emotionally manipulative language.
La Barbera et al. ( 2020 ) try to understand how people perceive the truthfulness of information presented to them. They collect data from US-based crowd workers, build a dataset of crowdsourced truthfulness judgments for political statements, and compare it with expert annotation data generated by fact-checkers such as PolitiFact.
Coscia and Rossi ( 2020 ) introduce a crowdsourced flagging system that consists of online news flagging. The bipolar model of news flagging attempts to capture the main ingredients that they observe in empirical research on fake news and disinformation.
Unlike the previously mentioned researchers who focus on news content in their approaches, Pennycook and Rand ( 2019 ) focus on using crowdsourced judgments of the quality of news sources to combat social media disinformation.
Fact-Checking. The fact-checking task is commonly manually performed by journalists to verify the truthfulness of a given claim. Indeed, fact-checking features are being adopted by multiple online social network platforms. For instance, Facebook Footnote 34 started addressing false information through independent fact-checkers in 2017, followed by Google Footnote 35 the same year. Two years later, Instagram Footnote 36 followed suit. However, the usefulness of fact-checking initiatives is questioned by journalists Footnote 37 , as well as by researchers such as Andersen and Søe ( 2020 ). On the other hand, work is being conducted to boost the effectiveness of these initiatives to reduce misinformation (Chung and Kim 2021 ; Clayton et al. 2020 ; Nyhan et al. 2020 ).
Most researchers use fact-checking websites (e.g., politifact.com, Footnote 38 snopes.com, Footnote 39 Reuters, Footnote 40 , etc.) as data sources to build their datasets and train their models. Therefore, in the following, we specifically review examples of solutions that use fact-checking (Vlachos and Riedel 2014 ) to help build datasets that can be further used in the automatic detection of fake content.
Yang et al. ( 2019a ) use PolitiFact fact-checking website as a data source to train, tune, and evaluate their model named XFake, on political data. The XFake system is an explainable fake news detector that assists end users to identify news credibility. The fakeness of news items is detected and interpreted considering both content and contextual (e.g., statements) information (e.g., speaker).
Based on the idea that fact-checkers cannot clean all data, and it must be a selection of what “matters the most” to clean while checking a claim, Sintos et al. ( 2019 ) propose a solution to help fact-checkers combat problems related to data quality (where inaccurate data lead to incorrect conclusions) and data phishing. The proposed solution is a combination of data cleaning and perturbation analysis to avoid uncertainties and errors in data and the possibility that data can be phished.
Tchechmedjiev et al. ( 2019 ) propose a system named “ClaimsKG” as a knowledge graph of fact-checked claims aiming to facilitate structured queries about their truth values, authors, dates, journalistic reviews and other kinds of metadata. “ClaimsKG” designs the relationship between vocabularies. To gather vocabularies, a semi-automated pipeline periodically gathers data from popular fact-checking websites regularly.
6.2.2 AI-based Techniques
Previous work by Yaqub et al. ( 2020 ) has shown that people lack trust in automated solutions for fake news detection However, work is already being undertaken to increase this trust, for instance by von der Weth et al. ( 2020 ).
Most researchers consider fake news detection as a classification problem and use artificial intelligence techniques, as shown in Fig. 8 . The adopted AI techniques may include machine learning ML (e.g., Naïve Bayes, logistic regression, support vector machine SVM), deep learning DL (e.g., convolutional neural networks CNN, recurrent neural networks RNN, long short-term memory LSTM) and natural language processing NLP (e.g., Count vectorizer, TF-IDF Vectorizer). Most of them combine many AI techniques in their solutions rather than relying on one specific approach.
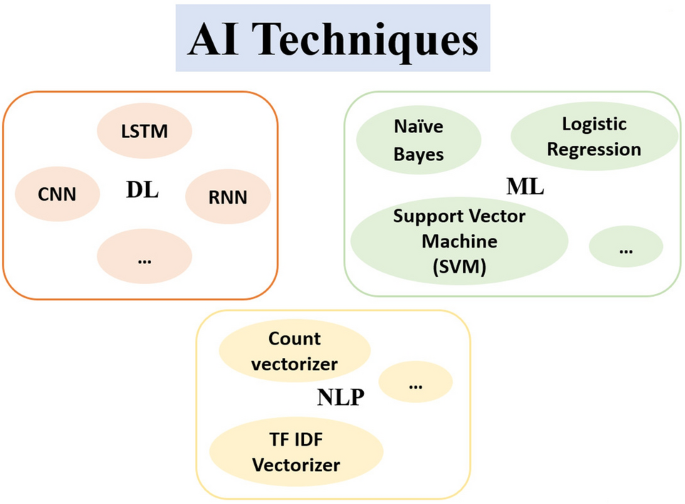
Examples of the most widely used AI techniques for fake news detection
Many researchers are developing machine learning models in their solutions for fake news detection. Recently, deep neural network techniques are also being employed as they are generating promising results (Islam et al. 2020 ). A neural network is a massively parallel distributed processor with simple units that can store important information and make it available for use (Hiriyannaiah et al. 2020 ). Moreover, it has been proven (Cardoso Durier da Silva et al. 2019 ) that the most widely used method for automatic detection of fake news is not simply a classical machine learning technique, but rather a fusion of classical techniques coordinated by a neural network.
Some researchers define purely machine learning models (Del Vicario et al. 2019 ; Elhadad et al. 2019 ; Aswani et al. 2017 ; Hakak et al. 2021 ; Singh et al. 2021 ) in their fake news detection approaches. The more commonly used machine learning algorithms (Abdullah-All-Tanvir et al. 2019 ) for classification problems are Naïve Bayes, logistic regression and SVM.
Other researchers (Wang et al. 2019c ; Wang 2017 ; Liu and Wu 2018 ; Mishra 2020 ; Qian et al. 2018 ; Zhang et al. 2020 ; Goldani et al. 2021 ) prefer to do a mixture of different deep learning models, without combining them with classical machine learning techniques. Some even prove that deep learning techniques outperform traditional machine learning techniques (Mishra et al. 2022 ). Deep learning is one of the most widely popular research topics in machine learning. Unlike traditional machine learning approaches, which are based on manually crafted features, deep learning approaches can learn hidden representations from simpler inputs both in context and content variations (Bondielli and Marcelloni 2019 ). Moreover, traditional machine learning algorithms almost always require structured data and are designed to “learn” to act by understanding labeled data and then use it to produce new results with more datasets, which requires human intervention to “teach them” when the result is incorrect (Parrish 2018 ), while deep learning networks rely on layers of artificial neural networks (ANN) and do not require human intervention, as multilevel layers in neural networks place data in a hierarchy of different concepts, which ultimately learn from their own mistakes (Parrish 2018 ). The two most widely implemented paradigms in deep neural networks are recurrent neural networks (RNN) and convolutional neural networks (CNN).
Still other researchers (Abdullah-All-Tanvir et al. 2019 ; Kaliyar et al. 2020 ; Zhang et al. 2019a ; Deepak and Chitturi 2020 ; Shu et al. 2018a ; Wang et al. 2019c ) prefer to combine traditional machine learning and deep learning classification, models. Others combine machine learning and natural language processing techniques. A few combine deep learning models with natural language processing (Vereshchaka et al. 2020 ). Some other researchers (Kapusta et al. 2019 ; Ozbay and Alatas 2020 ; Ahmed et al. 2020 ) combine natural language processing with machine learning models. Furthermore, others (Abdullah-All-Tanvir et al. 2019 ; Kaur et al. 2020 ; Kaliyar 2018 ; Abdullah-All-Tanvir et al. 2020 ; Bahad et al. 2019 ) prefer to combine all the previously mentioned techniques (i.e., ML, DL and NLP) in their approaches.
Table 11 , which is relegated to the Appendix (after the bibliography) because of its size, shows a comparison of the fake news detection solutions that we have reviewed based on their main approaches, the methodology that was used and the models.
6.2.3 Blockchain-based Techniques for Source Reliability and Traceability
Another research direction for detecting and mitigating fake news in social media focuses on using blockchain solutions. Blockchain technology is recently attracting researchers’ attention due to the interesting features it offers. Immutability, decentralization, tamperproof, consensus, record keeping and non-repudiation of transactions are some of the key features that make blockchain technology exploitable, not just for cryptocurrencies, but also to prove the authenticity and integrity of digital assets.
However, the proposed blockchain approaches are few in number and they are fundamental and theoretical approaches. Specifically, the solutions that are currently available are still in research, prototype, and beta testing stages (DiCicco and Agarwal 2020 ; Tchechmedjiev et al. 2019 ). Furthermore, most researchers (Ochoa et al. 2019 ; Song et al. 2019 ; Shang et al. 2018 ; Qayyum et al. 2019 ; Jing and Murugesan 2018 ; Buccafurri et al. 2017 ; Chen et al. 2018 ) do not specify which fake news type they are mitigating in their studies. They mention news content in general, which is not adequate for innovative solutions. For that, serious implementations should be provided to prove the usefulness and feasibility of this newly developing research vision.
Table 9 shows a classification of the reviewed blockchain-based approaches. In the classification, we listed the following:
The type of fake news that authors are trying to mitigate, which can be multimedia-based or text-based fake news.
The techniques used for fake news mitigation, which can be either blockchain only, or blockchain combined with other techniques such as AI, Data mining, Truth-discovery, Preservation metadata, Semantic similarity, Crowdsourcing, Graph theory and SIR model (Susceptible, Infected, Recovered).
The feature that is offered as an advantage of the given solution (e.g., Reliability, Authenticity and Traceability). Reliability is the credibility and truthfulness of the news content, which consists of proving the trustworthiness of the content. Traceability aims to trace and archive the contents. Authenticity consists of checking whether the content is real and authentic.
A checkmark ( \(\checkmark \) ) in Table 9 denotes that the mentioned criterion is explicitly mentioned in the proposed solution, while the empty dash (–) cell for fake news type denotes that it depends on the case: The criterion was either not explicitly mentioned (e.g., fake news type) in the work or the classification does not apply (e.g., techniques/other).
7 Discussion
After reviewing the most relevant state of the art for automatic fake news detection, we classify them as shown in Table 10 based on the detection aspects (i.e., content-based, contextual, or hybrid aspects) and the techniques used (i.e., AI, crowdsourcing, fact-checking, blockchain or hybrid techniques). Hybrid techniques refer to solutions that simultaneously combine different techniques from previously mentioned categories (i.e., inter-hybrid methods), as well as techniques within the same class of methods (i.e., intra-hybrid methods), in order to define innovative solutions for fake news detection. A hybrid method should bring the best of both worlds. Then, we provide a discussion based on different axes.
7.1 News content-based methods
Most of the news content-based approaches consider fake news detection as a classification problem and they use AI techniques such as classical machine learning (e.g., regression, Bayesian) as well as deep learning (i.e., neural methods such as CNN and RNN). More specifically, classification of social media content is a fundamental task for social media mining, so that most existing methods regard it as a text categorization problem and mainly focus on using content features, such as words and hashtags (Wu and Liu 2018 ). The main challenge facing these approaches is how to extract features in a way to reduce the data used to train their models and what features are the most suitable for accurate results.
Researchers using such approaches are motivated by the fact that the news content is the main entity in the deception process, and it is a straightforward factor to analyze and use while looking for predictive clues of deception. However, detecting fake news only from the content of the news is not enough because the news is created in a strategic intentional way to mimic the truth (i.e., the content can be intentionally manipulated by the spreader to make it look like real news). Therefore, it is considered to be challenging, if not impossible, to identify useful features (Wu and Liu 2018 ) and consequently tell the nature of such news solely from the content.
Moreover, works that utilize only the news content for fake news detection ignore the rich information and latent user intelligence (Qian et al. 2018 ) stored in user responses toward previously disseminated articles. Therefore, the auxiliary information is deemed crucial for an effective fake news detection approach.
7.2 Social context-based methods
The context-based approaches explore the surrounding data outside of the news content, which can be an effective direction and has some advantages in areas where the content approaches based on text classification can run into issues. However, most existing studies implementing contextual methods mainly focus on additional information coming from users and network diffusion patterns. Moreover, from a technical perspective, they are limited to the use of sophisticated machine learning techniques for feature extraction, and they ignore the usefulness of results coming from techniques such as web search and crowdsourcing which may save much time and help in the early detection and identification of fake content.
7.3 Hybrid approaches
Hybrid approaches can simultaneously model different aspects of fake news such as the content-based aspects, as well as the contextual aspect based on both the OSN user and the OSN network patterns. However, these approaches are deemed more complex in terms of models (Bondielli and Marcelloni 2019 ), data availability, and the number of features. Furthermore, it remains difficult to decide which information among each category (i.e., content-based and context-based information) is most suitable and appropriate to be used to achieve accurate and precise results. Therefore, there are still very few studies belonging to this category of hybrid approaches.
7.4 Early detection
As fake news usually evolves and spreads very fast on social media, it is critical and urgent to consider early detection directions. Yet, this is a challenging task to do especially in highly dynamic platforms such as social networks. Both news content- and social context-based approaches suffer from this challenging early detection of fake news.
Although approaches that detect fake news based on content analysis face this issue less, they are still limited by the lack of information required for verification when the news is in its early stage of spread. However, approaches that detect fake news based on contextual analysis are most likely to suffer from the lack of early detection since most of them rely on information that is mostly available after the spread of fake content such as social engagement, user response, and propagation patterns. Therefore, it is crucial to consider both trusted human verification and historical data as an attempt to detect fake content during its early stage of propagation.
8 Conclusion and future directions
In this paper, we introduced the general context of the fake news problem as one of the major issues of the online deception problem in online social networks. Based on reviewing the most relevant state of the art, we summarized and classified existing definitions of fake news, as well as its related terms. We also listed various typologies and existing categorizations of fake news such as intent-based fake news including clickbait, hoax, rumor, satire, propaganda, conspiracy theories, framing as well as content-based fake news including text and multimedia-based fake news, and in the latter, we can tackle deepfake videos and GAN-generated fake images. We discussed the major challenges related to fake news detection and mitigation in social media including the deceptiveness nature of the fabricated content, the lack of human awareness in the field of fake news, the non-human spreaders issue (e.g., social bots), the dynamicity of such online platforms, which results in a fast propagation of fake content and the quality of existing datasets, which still limits the efficiency of the proposed solutions. We reviewed existing researchers’ visions regarding the automatic detection of fake news based on the adopted approaches (i.e., news content-based approaches, social context-based approaches, or hybrid approaches) and the techniques that are used (i.e., artificial intelligence-based methods; crowdsourcing, fact-checking, and blockchain-based methods; and hybrid methods), then we showed a comparative study between the reviewed works. We also provided a critical discussion of the reviewed approaches based on different axes such as the adopted aspect for fake news detection (i.e., content-based, contextual, and hybrid aspects) and the early detection perspective.
To conclude, we present the main issues for combating the fake news problem that needs to be further investigated while proposing new detection approaches. We believe that to define an efficient fake news detection approach, we need to consider the following:
Our choice of sources of information and search criteria may have introduced biases in our research. If so, it would be desirable to identify those biases and mitigate them.
News content is the fundamental source to find clues to distinguish fake from real content. However, contextual information derived from social media users and from the network can provide useful auxiliary information to increase detection accuracy. Specifically, capturing users’ characteristics and users’ behavior toward shared content can be a key task for fake news detection.
Moreover, capturing users’ historical behavior, including their emotions and/or opinions toward news content, can help in the early detection and mitigation of fake news.
Furthermore, adversarial learning techniques (e.g., GAN, SeqGAN) can be considered as a promising direction for mitigating the lack and scarcity of available datasets by providing machine-generated data that can be used to train and build robust systems to detect the fake examples from the real ones.
Lastly, analyzing how sources and promoters of fake news operate over the web through multiple online platforms is crucial; Zannettou et al. ( 2019 ) discovered that false information is more likely to spread across platforms (18% appearing on multiple platforms) compared to valid information (11%).
Availability of data and material
All the data and material are available in the papers cited in the references.
https://www.nationalacademies.org/news/2021/07/as-surgeon-general-urges-whole-of-society-effort-to-fight-health-misinformation-the-work-of-the-national-academies-helps-foster-an-evidence-based-information-environment , last access date: 26-12-2022.
https://time.com/4897819/elvis-presley-alive-conspiracy-theories/ , last access date: 26-12-2022.
https://www.therichest.com/shocking/the-evidence-15-reasons-people-think-the-earth-is-flat/ , last access date: 26-12-2022.
https://www.grunge.com/657584/the-truth-about-1952s-alien-invasion-of-washington-dc/ , last access date: 26-12-2022.
https://www.journalism.org/2021/01/12/news-use-across-social-media-platforms-in-2020/ , last access date: 26-12-2022.
https://www.pewresearch.org/fact-tank/2018/12/10/social-media-outpaces-print-newspapers-in-the-u-s-as-a-news-source/ , last access date: 26-12-2022.
https://www.buzzfeednews.com/article/janelytvynenko/coronavirus-fake-news-disinformation-rumors-hoaxes , last access date: 26-12-2022.
https://www.factcheck.org/2020/03/viral-social-media-posts-offer-false-coronavirus-tips/ , last access date: 26-12-2022.
https://www.factcheck.org/2020/02/fake-coronavirus-cures-part-2-garlic-isnt-a-cure/ , last access date: 26-12-2022.
https://www.bbc.com/news/uk-36528256 , last access date: 26-12-2022.
https://en.wikipedia.org/wiki/Pizzagate_conspiracy_theory , last access date: 26-12-2022.
https://www.theguardian.com/world/2017/jan/09/germany-investigating-spread-fake-news-online-russia-election , last access date: 26-12-2022.
https://www.macquariedictionary.com.au/resources/view/word/of/the/year/2016 , last access date: 26-12-2022.
https://www.macquariedictionary.com.au/resources/view/word/of/the/year/2018 , last access date: 26-12-2022.
https://apnews.com/article/47466c5e260149b1a23641b9e319fda6 , last access date: 26-12-2022.
https://blog.collinsdictionary.com/language-lovers/collins-2017-word-of-the-year-shortlist/ , last access date: 26-12-2022.
https://www.gartner.com/smarterwithgartner/gartner-top-strategic-predictions-for-2018-and-beyond/ , last access date: 26-12-2022.
https://www.technologyreview.com/s/612236/even-the-best-ai-for-spotting-fake-news-is-still-terrible/ , last access date: 26-12-2022.
https://scholar.google.ca/ , last access date: 26-12-2022.
https://ieeexplore.ieee.org/ , last access date: 26-12-2022.
https://link.springer.com/ , last access date: 26-12-2022.
https://www.sciencedirect.com/ , last access date: 26-12-2022.
https://www.scopus.com/ , last access date: 26-12-2022.
https://www.acm.org/digital-library , last access date: 26-12-2022.
https://www.politico.com/magazine/story/2016/12/fake-news-history-long-violent-214535 , last access date: 26-12-2022.
https://en.wikipedia.org/wiki/Trial_of_Socrates , last access date: 26-12-2022.
https://trends.google.com/trends/explore?hl=en-US &tz=-180 &date=2013-12-06+2018-01-06 &geo=US &q=fake+news &sni=3 , last access date: 26-12-2022.
https://ec.europa.eu/digital-single-market/en/tackling-online-disinformation , last access date: 26-12-2022.
https://www.nato.int/cps/en/natohq/177273.htm , last access date: 26-12-2022.
https://www.collinsdictionary.com/dictionary/english/fake-news , last access date: 26-12-2022.
https://www.statista.com/statistics/657111/fake-news-sharing-online/ , last access date: 26-12-2022.
https://www.statista.com/statistics/657090/fake-news-recogition-confidence/ , last access date: 26-12-2022.
https://www.nbcnews.com/tech/social-media/now-available-more-200-000-deleted-russian-troll-tweets-n844731 , last access date: 26-12-2022.
https://www.theguardian.com/technology/2017/mar/22/facebook-fact-checking-tool-fake-news , last access date: 26-12-2022.
https://www.theguardian.com/technology/2017/apr/07/google-to-display-fact-checking-labels-to-show-if-news-is-true-or-false , last access date: 26-12-2022.
https://about.instagram.com/blog/announcements/combatting-misinformation-on-instagram , last access date: 26-12-2022.
https://www.wired.com/story/instagram-fact-checks-who-will-do-checking/ , last access date: 26-12-2022.
https://www.politifact.com/ , last access date: 26-12-2022.
https://www.snopes.com/ , last access date: 26-12-2022.
https://www.reutersagency.com/en/ , last access date: 26-12-2022.
Abdullah-All-Tanvir, Mahir EM, Akhter S, Huq MR (2019) Detecting fake news using machine learning and deep learning algorithms. In: 7th international conference on smart computing and communications (ICSCC), IEEE, pp 1–5 https://doi.org/10.1109/ICSCC.2019.8843612
Abdullah-All-Tanvir, Mahir EM, Huda SMA, Barua S (2020) A hybrid approach for identifying authentic news using deep learning methods on popular Twitter threads. In: International conference on artificial intelligence and signal processing (AISP), IEEE, pp 1–6 https://doi.org/10.1109/AISP48273.2020.9073583
Abu Arqoub O, Abdulateef Elega A, Efe Özad B, Dwikat H, Adedamola Oloyede F (2022) Mapping the scholarship of fake news research: a systematic review. J Pract 16(1):56–86. https://doi.org/10.1080/17512786.2020.1805791
Article Google Scholar
Ahmed S, Hinkelmann K, Corradini F (2020) Development of fake news model using machine learning through natural language processing. Int J Comput Inf Eng 14(12):454–460
Google Scholar
Aïmeur E, Brassard G, Rioux J (2013) Data privacy: an end-user perspective. Int J Comput Netw Commun Secur 1(6):237–250
Aïmeur E, Hage H, Amri S (2018) The scourge of online deception in social networks. In: 2018 international conference on computational science and computational intelligence (CSCI), IEEE, pp 1266–1271 https://doi.org/10.1109/CSCI46756.2018.00244
Alemanno A (2018) How to counter fake news? A taxonomy of anti-fake news approaches. Eur J Risk Regul 9(1):1–5. https://doi.org/10.1017/err.2018.12
Allcott H, Gentzkow M (2017) Social media and fake news in the 2016 election. J Econ Perspect 31(2):211–36. https://doi.org/10.1257/jep.31.2.211
Allen J, Howland B, Mobius M, Rothschild D, Watts DJ (2020) Evaluating the fake news problem at the scale of the information ecosystem. Sci Adv. https://doi.org/10.1126/sciadv.aay3539
Allington D, Duffy B, Wessely S, Dhavan N, Rubin J (2020) Health-protective behaviour, social media usage and conspiracy belief during the Covid-19 public health emergency. Psychol Med. https://doi.org/10.1017/S003329172000224X
Alonso-Galbán P, Alemañy-Castilla C (2022) Curbing misinformation and disinformation in the Covid-19 era: a view from cuba. MEDICC Rev 22:45–46 https://doi.org/10.37757/MR2020.V22.N2.12
Altay S, Hacquin AS, Mercier H (2022) Why do so few people share fake news? It hurts their reputation. New Media Soc 24(6):1303–1324. https://doi.org/10.1177/1461444820969893
Amri S, Sallami D, Aïmeur E (2022) Exmulf: an explainable multimodal content-based fake news detection system. In: International symposium on foundations and practice of security. Springer, Berlin, pp 177–187. https://doi.org/10.1109/IJCNN48605.2020.9206973
Andersen J, Søe SO (2020) Communicative actions we live by: the problem with fact-checking, tagging or flagging fake news-the case of Facebook. Eur J Commun 35(2):126–139. https://doi.org/10.1177/0267323119894489
Apuke OD, Omar B (2021) Fake news and Covid-19: modelling the predictors of fake news sharing among social media users. Telematics Inform 56:101475. https://doi.org/10.1016/j.tele.2020.101475
Apuke OD, Omar B, Tunca EA, Gever CV (2022) The effect of visual multimedia instructions against fake news spread: a quasi-experimental study with Nigerian students. J Librariansh Inf Sci. https://doi.org/10.1177/09610006221096477
Aswani R, Ghrera S, Kar AK, Chandra S (2017) Identifying buzz in social media: a hybrid approach using artificial bee colony and k-nearest neighbors for outlier detection. Soc Netw Anal Min 7(1):1–10. https://doi.org/10.1007/s13278-017-0461-2
Avram M, Micallef N, Patil S, Menczer F (2020) Exposure to social engagement metrics increases vulnerability to misinformation. arXiv preprint arxiv:2005.04682 , https://doi.org/10.37016/mr-2020-033
Badawy A, Lerman K, Ferrara E (2019) Who falls for online political manipulation? In: Companion proceedings of the 2019 world wide web conference, pp 162–168 https://doi.org/10.1145/3308560.3316494
Bahad P, Saxena P, Kamal R (2019) Fake news detection using bi-directional LSTM-recurrent neural network. Procedia Comput Sci 165:74–82. https://doi.org/10.1016/j.procs.2020.01.072
Bakdash J, Sample C, Rankin M, Kantarcioglu M, Holmes J, Kase S, Zaroukian E, Szymanski B (2018) The future of deception: machine-generated and manipulated images, video, and audio? In: 2018 international workshop on social sensing (SocialSens), IEEE, pp 2–2 https://doi.org/10.1109/SocialSens.2018.00009
Balmas M (2014) When fake news becomes real: combined exposure to multiple news sources and political attitudes of inefficacy, alienation, and cynicism. Commun Res 41(3):430–454. https://doi.org/10.1177/0093650212453600
Baptista JP, Gradim A (2020) Understanding fake news consumption: a review. Soc Sci. https://doi.org/10.3390/socsci9100185
Baptista JP, Gradim A (2022) A working definition of fake news. Encyclopedia 2(1):632–645. https://doi.org/10.3390/encyclopedia2010043
Bastick Z (2021) Would you notice if fake news changed your behavior? An experiment on the unconscious effects of disinformation. Comput Hum Behav 116:106633. https://doi.org/10.1016/j.chb.2020.106633
Batailler C, Brannon SM, Teas PE, Gawronski B (2022) A signal detection approach to understanding the identification of fake news. Perspect Psychol Sci 17(1):78–98. https://doi.org/10.1177/1745691620986135
Bessi A, Ferrara E (2016) Social bots distort the 2016 US presidential election online discussion. First Monday 21(11-7). https://doi.org/10.5210/fm.v21i11.7090
Bhattacharjee A, Shu K, Gao M, Liu H (2020) Disinformation in the online information ecosystem: detection, mitigation and challenges. arXiv preprint arXiv:2010.09113
Bhuiyan MM, Zhang AX, Sehat CM, Mitra T (2020) Investigating differences in crowdsourced news credibility assessment: raters, tasks, and expert criteria. Proc ACM Hum Comput Interact 4(CSCW2):1–26. https://doi.org/10.1145/3415164
Bode L, Vraga EK (2015) In related news, that was wrong: the correction of misinformation through related stories functionality in social media. J Commun 65(4):619–638. https://doi.org/10.1111/jcom.12166
Bondielli A, Marcelloni F (2019) A survey on fake news and rumour detection techniques. Inf Sci 497:38–55. https://doi.org/10.1016/j.ins.2019.05.035
Bovet A, Makse HA (2019) Influence of fake news in Twitter during the 2016 US presidential election. Nat Commun 10(1):1–14. https://doi.org/10.1038/s41467-018-07761-2
Brashier NM, Pennycook G, Berinsky AJ, Rand DG (2021) Timing matters when correcting fake news. Proc Natl Acad Sci. https://doi.org/10.1073/pnas.2020043118
Brewer PR, Young DG, Morreale M (2013) The impact of real news about “fake news’’: intertextual processes and political satire. Int J Public Opin Res 25(3):323–343. https://doi.org/10.1093/ijpor/edt015
Bringula RP, Catacutan-Bangit AE, Garcia MB, Gonzales JPS, Valderama AMC (2022) “Who is gullible to political disinformation?’’ Predicting susceptibility of university students to fake news. J Inf Technol Polit 19(2):165–179. https://doi.org/10.1080/19331681.2021.1945988
Buccafurri F, Lax G, Nicolazzo S, Nocera A (2017) Tweetchain: an alternative to blockchain for crowd-based applications. In: International conference on web engineering, Springer, Berlin, pp 386–393. https://doi.org/10.1007/978-3-319-60131-1_24
Burshtein S (2017) The true story on fake news. Intell Prop J 29(3):397–446
Cardaioli M, Cecconello S, Conti M, Pajola L, Turrin F (2020) Fake news spreaders profiling through behavioural analysis. In: CLEF (working notes)
Cardoso Durier da Silva F, Vieira R, Garcia AC (2019) Can machines learn to detect fake news? A survey focused on social media. In: Proceedings of the 52nd Hawaii international conference on system sciences. https://doi.org/10.24251/HICSS.2019.332
Carmi E, Yates SJ, Lockley E, Pawluczuk A (2020) Data citizenship: rethinking data literacy in the age of disinformation, misinformation, and malinformation. Intern Policy Rev 9(2):1–22 https://doi.org/10.14763/2020.2.1481
Celliers M, Hattingh M (2020) A systematic review on fake news themes reported in literature. In: Conference on e-Business, e-Services and e-Society. Springer, Berlin, pp 223–234. https://doi.org/10.1007/978-3-030-45002-1_19
Chen Y, Li Q, Wang H (2018) Towards trusted social networks with blockchain technology. arXiv preprint arXiv:1801.02796
Cheng L, Guo R, Shu K, Liu H (2020) Towards causal understanding of fake news dissemination. arXiv preprint arXiv:2010.10580
Chiu MM, Oh YW (2021) How fake news differs from personal lies. Am Behav Sci 65(2):243–258. https://doi.org/10.1177/0002764220910243
Chung M, Kim N (2021) When I learn the news is false: how fact-checking information stems the spread of fake news via third-person perception. Hum Commun Res 47(1):1–24. https://doi.org/10.1093/hcr/hqaa010
Clarke J, Chen H, Du D, Hu YJ (2020) Fake news, investor attention, and market reaction. Inf Syst Res. https://doi.org/10.1287/isre.2019.0910
Clayton K, Blair S, Busam JA, Forstner S, Glance J, Green G, Kawata A, Kovvuri A, Martin J, Morgan E et al (2020) Real solutions for fake news? Measuring the effectiveness of general warnings and fact-check tags in reducing belief in false stories on social media. Polit Behav 42(4):1073–1095. https://doi.org/10.1007/s11109-019-09533-0
Collins B, Hoang DT, Nguyen NT, Hwang D (2020) Fake news types and detection models on social media a state-of-the-art survey. In: Asian conference on intelligent information and database systems. Springer, Berlin, pp 562–573 https://doi.org/10.1007/978-981-15-3380-8_49
Conroy NK, Rubin VL, Chen Y (2015) Automatic deception detection: methods for finding fake news. Proc Assoc Inf Sci Technol 52(1):1–4. https://doi.org/10.1002/pra2.2015.145052010082
Cooke NA (2017) Posttruth, truthiness, and alternative facts: Information behavior and critical information consumption for a new age. Libr Q 87(3):211–221. https://doi.org/10.1086/692298
Article MathSciNet Google Scholar
Coscia M, Rossi L (2020) Distortions of political bias in crowdsourced misinformation flagging. J R Soc Interface 17(167):20200020. https://doi.org/10.1098/rsif.2020.0020
Dame Adjin-Tettey T (2022) Combating fake news, disinformation, and misinformation: experimental evidence for media literacy education. Cogent Arts Human 9(1):2037229. https://doi.org/10.1080/23311983.2022.2037229
Deepak S, Chitturi B (2020) Deep neural approach to fake-news identification. Procedia Comput Sci 167:2236–2243. https://doi.org/10.1016/j.procs.2020.03.276
de Cock Buning M (2018) A multi-dimensional approach to disinformation: report of the independent high level group on fake news and online disinformation. Publications Office of the European Union
Del Vicario M, Quattrociocchi W, Scala A, Zollo F (2019) Polarization and fake news: early warning of potential misinformation targets. ACM Trans Web (TWEB) 13(2):1–22. https://doi.org/10.1145/3316809
Demuyakor J, Opata EM (2022) Fake news on social media: predicting which media format influences fake news most on facebook. J Intell Commun. https://doi.org/10.54963/jic.v2i1.56
Derakhshan H, Wardle C (2017) Information disorder: definitions. In: Understanding and addressing the disinformation ecosystem, pp 5–12
Desai AN, Ruidera D, Steinbrink JM, Granwehr B, Lee DH (2022) Misinformation and disinformation: the potential disadvantages of social media in infectious disease and how to combat them. Clin Infect Dis 74(Supplement–3):e34–e39. https://doi.org/10.1093/cid/ciac109
Di Domenico G, Sit J, Ishizaka A, Nunan D (2021) Fake news, social media and marketing: a systematic review. J Bus Res 124:329–341. https://doi.org/10.1016/j.jbusres.2020.11.037
Dias N, Pennycook G, Rand DG (2020) Emphasizing publishers does not effectively reduce susceptibility to misinformation on social media. Harv Kennedy School Misinform Rev. https://doi.org/10.37016/mr-2020-001
DiCicco KW, Agarwal N (2020) Blockchain technology-based solutions to fight misinformation: a survey. In: Disinformation, misinformation, and fake news in social media. Springer, Berlin, pp 267–281, https://doi.org/10.1007/978-3-030-42699-6_14
Douglas KM, Uscinski JE, Sutton RM, Cichocka A, Nefes T, Ang CS, Deravi F (2019) Understanding conspiracy theories. Polit Psychol 40:3–35. https://doi.org/10.1111/pops.12568
Edgerly S, Mourão RR, Thorson E, Tham SM (2020) When do audiences verify? How perceptions about message and source influence audience verification of news headlines. J Mass Commun Q 97(1):52–71. https://doi.org/10.1177/1077699019864680
Egelhofer JL, Lecheler S (2019) Fake news as a two-dimensional phenomenon: a framework and research agenda. Ann Int Commun Assoc 43(2):97–116. https://doi.org/10.1080/23808985.2019.1602782
Elhadad MK, Li KF, Gebali F (2019) A novel approach for selecting hybrid features from online news textual metadata for fake news detection. In: International conference on p2p, parallel, grid, cloud and internet computing. Springer, Berlin, pp 914–925, https://doi.org/10.1007/978-3-030-33509-0_86
ERGA (2018) Fake news, and the information disorder. European Broadcasting Union (EBU)
ERGA (2021) Notions of disinformation and related concepts. European Regulators Group for Audiovisual Media Services (ERGA)
Escolà-Gascón Á (2021) New techniques to measure lie detection using Covid-19 fake news and the Multivariable Multiaxial Suggestibility Inventory-2 (MMSI-2). Comput Hum Behav Rep 3:100049. https://doi.org/10.1016/j.chbr.2020.100049
Fazio L (2020) Pausing to consider why a headline is true or false can help reduce the sharing of false news. Harv Kennedy School Misinformation Rev. https://doi.org/10.37016/mr-2020-009
Ferrara E, Varol O, Davis C, Menczer F, Flammini A (2016) The rise of social bots. Commun ACM 59(7):96–104. https://doi.org/10.1145/2818717
Flynn D, Nyhan B, Reifler J (2017) The nature and origins of misperceptions: understanding false and unsupported beliefs about politics. Polit Psychol 38:127–150. https://doi.org/10.1111/pops.12394
Fraga-Lamas P, Fernández-Caramés TM (2020) Fake news, disinformation, and deepfakes: leveraging distributed ledger technologies and blockchain to combat digital deception and counterfeit reality. IT Prof 22(2):53–59. https://doi.org/10.1109/MITP.2020.2977589
Freeman D, Waite F, Rosebrock L, Petit A, Causier C, East A, Jenner L, Teale AL, Carr L, Mulhall S et al (2020) Coronavirus conspiracy beliefs, mistrust, and compliance with government guidelines in England. Psychol Med. https://doi.org/10.1017/S0033291720001890
Friggeri A, Adamic L, Eckles D, Cheng J (2014) Rumor cascades. In: Proceedings of the international AAAI conference on web and social media
García SA, García GG, Prieto MS, Moreno Guerrero AJ, Rodríguez Jiménez C (2020) The impact of term fake news on the scientific community. Scientific performance and mapping in web of science. Soc Sci. https://doi.org/10.3390/socsci9050073
Garrett RK, Bond RM (2021) Conservatives’ susceptibility to political misperceptions. Sci Adv. https://doi.org/10.1126/sciadv.abf1234
Giachanou A, Ríssola EA, Ghanem B, Crestani F, Rosso P (2020) The role of personality and linguistic patterns in discriminating between fake news spreaders and fact checkers. In: International conference on applications of natural language to information systems. Springer, Berlin, pp 181–192 https://doi.org/10.1007/978-3-030-51310-8_17
Golbeck J, Mauriello M, Auxier B, Bhanushali KH, Bonk C, Bouzaghrane MA, Buntain C, Chanduka R, Cheakalos P, Everett JB et al (2018) Fake news vs satire: a dataset and analysis. In: Proceedings of the 10th ACM conference on web science, pp 17–21, https://doi.org/10.1145/3201064.3201100
Goldani MH, Momtazi S, Safabakhsh R (2021) Detecting fake news with capsule neural networks. Appl Soft Comput 101:106991. https://doi.org/10.1016/j.asoc.2020.106991
Goldstein I, Yang L (2019) Good disclosure, bad disclosure. J Financ Econ 131(1):118–138. https://doi.org/10.1016/j.jfineco.2018.08.004
Grinberg N, Joseph K, Friedland L, Swire-Thompson B, Lazer D (2019) Fake news on Twitter during the 2016 US presidential election. Science 363(6425):374–378. https://doi.org/10.1126/science.aau2706
Guadagno RE, Guttieri K (2021) Fake news and information warfare: an examination of the political and psychological processes from the digital sphere to the real world. In: Research anthology on fake news, political warfare, and combatting the spread of misinformation. IGI Global, pp 218–242 https://doi.org/10.4018/978-1-7998-7291-7.ch013
Guess A, Nagler J, Tucker J (2019) Less than you think: prevalence and predictors of fake news dissemination on Facebook. Sci Adv. https://doi.org/10.1126/sciadv.aau4586
Guo C, Cao J, Zhang X, Shu K, Yu M (2019) Exploiting emotions for fake news detection on social media. arXiv preprint arXiv:1903.01728
Guo B, Ding Y, Yao L, Liang Y, Yu Z (2020) The future of false information detection on social media: new perspectives and trends. ACM Comput Surv (CSUR) 53(4):1–36. https://doi.org/10.1145/3393880
Gupta A, Li H, Farnoush A, Jiang W (2022) Understanding patterns of covid infodemic: a systematic and pragmatic approach to curb fake news. J Bus Res 140:670–683. https://doi.org/10.1016/j.jbusres.2021.11.032
Ha L, Andreu Perez L, Ray R (2021) Mapping recent development in scholarship on fake news and misinformation, 2008 to 2017: disciplinary contribution, topics, and impact. Am Behav Sci 65(2):290–315. https://doi.org/10.1177/0002764219869402
Habib A, Asghar MZ, Khan A, Habib A, Khan A (2019) False information detection in online content and its role in decision making: a systematic literature review. Soc Netw Anal Min 9(1):1–20. https://doi.org/10.1007/s13278-019-0595-5
Hage H, Aïmeur E, Guedidi A (2021) Understanding the landscape of online deception. In: Research anthology on fake news, political warfare, and combatting the spread of misinformation. IGI Global, pp 39–66. https://doi.org/10.4018/978-1-7998-2543-2.ch014
Hakak S, Alazab M, Khan S, Gadekallu TR, Maddikunta PKR, Khan WZ (2021) An ensemble machine learning approach through effective feature extraction to classify fake news. Futur Gener Comput Syst 117:47–58. https://doi.org/10.1016/j.future.2020.11.022
Hamdi T, Slimi H, Bounhas I, Slimani Y (2020) A hybrid approach for fake news detection in Twitter based on user features and graph embedding. In: International conference on distributed computing and internet technology. Springer, Berlin, pp 266–280. https://doi.org/10.1007/978-3-030-36987-3_17
Hameleers M (2022) Separating truth from lies: comparing the effects of news media literacy interventions and fact-checkers in response to political misinformation in the us and netherlands. Inf Commun Soc 25(1):110–126. https://doi.org/10.1080/1369118X.2020.1764603
Hameleers M, Powell TE, Van Der Meer TG, Bos L (2020) A picture paints a thousand lies? The effects and mechanisms of multimodal disinformation and rebuttals disseminated via social media. Polit Commun 37(2):281–301. https://doi.org/10.1080/10584609.2019.1674979
Hameleers M, Brosius A, de Vreese CH (2022) Whom to trust? media exposure patterns of citizens with perceptions of misinformation and disinformation related to the news media. Eur J Commun. https://doi.org/10.1177/02673231211072667
Hartley K, Vu MK (2020) Fighting fake news in the Covid-19 era: policy insights from an equilibrium model. Policy Sci 53(4):735–758. https://doi.org/10.1007/s11077-020-09405-z
Hasan HR, Salah K (2019) Combating deepfake videos using blockchain and smart contracts. IEEE Access 7:41596–41606. https://doi.org/10.1109/ACCESS.2019.2905689
Hiriyannaiah S, Srinivas A, Shetty GK, Siddesh G, Srinivasa K (2020) A computationally intelligent agent for detecting fake news using generative adversarial networks. Hybrid computational intelligence: challenges and applications. pp 69–96 https://doi.org/10.1016/B978-0-12-818699-2.00004-4
Hosseinimotlagh S, Papalexakis EE (2018) Unsupervised content-based identification of fake news articles with tensor decomposition ensembles. In: Proceedings of the workshop on misinformation and misbehavior mining on the web (MIS2)
Huckle S, White M (2017) Fake news: a technological approach to proving the origins of content, using blockchains. Big Data 5(4):356–371. https://doi.org/10.1089/big.2017.0071
Huffaker JS, Kummerfeld JK, Lasecki WS, Ackerman MS (2020) Crowdsourced detection of emotionally manipulative language. In: Proceedings of the 2020 CHI conference on human factors in computing systems. pp 1–14 https://doi.org/10.1145/3313831.3376375
Ireton C, Posetti J (2018) Journalism, fake news & disinformation: handbook for journalism education and training. UNESCO Publishing, Paris
Islam MR, Liu S, Wang X, Xu G (2020) Deep learning for misinformation detection on online social networks: a survey and new perspectives. Soc Netw Anal Min 10(1):1–20. https://doi.org/10.1007/s13278-020-00696-x
Ismailov M, Tsikerdekis M, Zeadally S (2020) Vulnerabilities to online social network identity deception detection research and recommendations for mitigation. Fut Internet 12(9):148. https://doi.org/10.3390/fi12090148
Jakesch M, Koren M, Evtushenko A, Naaman M (2019) The role of source and expressive responding in political news evaluation. In: Computation and journalism symposium
Jamieson KH (2020) Cyberwar: how Russian hackers and trolls helped elect a president: what we don’t, can’t, and do know. Oxford University Press, Oxford. https://doi.org/10.1093/poq/nfy049
Book Google Scholar
Jiang S, Chen X, Zhang L, Chen S, Liu H (2019) User-characteristic enhanced model for fake news detection in social media. In: CCF International conference on natural language processing and Chinese computing, Springer, Berlin, pp 634–646. https://doi.org/10.1007/978-3-030-32233-5_49
Jin Z, Cao J, Zhang Y, Luo J (2016) News verification by exploiting conflicting social viewpoints in microblogs. In: Proceedings of the AAAI conference on artificial intelligence
Jing TW, Murugesan RK (2018) A theoretical framework to build trust and prevent fake news in social media using blockchain. In: International conference of reliable information and communication technology. Springer, Berlin, pp 955–962, https://doi.org/10.1007/978-3-319-99007-1_88
Jones-Jang SM, Mortensen T, Liu J (2021) Does media literacy help identification of fake news? Information literacy helps, but other literacies don’t. Am Behav Sci 65(2):371–388. https://doi.org/10.1177/0002764219869406
Jungherr A, Schroeder R (2021) Disinformation and the structural transformations of the public arena: addressing the actual challenges to democracy. Soc Media Soc. https://doi.org/10.1177/2056305121988928
Kaliyar RK (2018) Fake news detection using a deep neural network. In: 2018 4th international conference on computing communication and automation (ICCCA), IEEE, pp 1–7 https://doi.org/10.1109/CCAA.2018.8777343
Kaliyar RK, Goswami A, Narang P, Sinha S (2020) Fndnet—a deep convolutional neural network for fake news detection. Cogn Syst Res 61:32–44. https://doi.org/10.1016/j.cogsys.2019.12.005
Kapantai E, Christopoulou A, Berberidis C, Peristeras V (2021) A systematic literature review on disinformation: toward a unified taxonomical framework. New Media Soc 23(5):1301–1326. https://doi.org/10.1177/1461444820959296
Kapusta J, Benko L, Munk M (2019) Fake news identification based on sentiment and frequency analysis. In: International conference Europe middle east and North Africa information systems and technologies to support learning. Springer, Berlin, pp 400–409, 10.1007/978-3-030-36778-7_44
Kaur S, Kumar P, Kumaraguru P (2020) Automating fake news detection system using multi-level voting model. Soft Comput 24(12):9049–9069. https://doi.org/10.1007/s00500-019-04436-y
Khan SA, Alkawaz MH, Zangana HM (2019) The use and abuse of social media for spreading fake news. In: 2019 IEEE international conference on automatic control and intelligent systems (I2CACIS), IEEE, pp 145–148. https://doi.org/10.1109/I2CACIS.2019.8825029
Kim J, Tabibian B, Oh A, Schölkopf B, Gomez-Rodriguez M (2018) Leveraging the crowd to detect and reduce the spread of fake news and misinformation. In: Proceedings of the eleventh ACM international conference on web search and data mining, pp 324–332. https://doi.org/10.1145/3159652.3159734
Klein D, Wueller J (2017) Fake news: a legal perspective. J Internet Law 20(10):5–13
Kogan S, Moskowitz TJ, Niessner M (2019) Fake news: evidence from financial markets. Available at SSRN 3237763
Kuklinski JH, Quirk PJ, Jerit J, Schwieder D, Rich RF (2000) Misinformation and the currency of democratic citizenship. J Polit 62(3):790–816. https://doi.org/10.1111/0022-3816.00033
Kumar S, Shah N (2018) False information on web and social media: a survey. arXiv preprint arXiv:1804.08559
Kumar S, West R, Leskovec J (2016) Disinformation on the web: impact, characteristics, and detection of Wikipedia hoaxes. In: Proceedings of the 25th international conference on world wide web, pp 591–602. https://doi.org/10.1145/2872427.2883085
La Barbera D, Roitero K, Demartini G, Mizzaro S, Spina D (2020) Crowdsourcing truthfulness: the impact of judgment scale and assessor bias. In: European conference on information retrieval. Springer, Berlin, pp 207–214. https://doi.org/10.1007/978-3-030-45442-5_26
Lanius C, Weber R, MacKenzie WI (2021) Use of bot and content flags to limit the spread of misinformation among social networks: a behavior and attitude survey. Soc Netw Anal Min 11(1):1–15. https://doi.org/10.1007/s13278-021-00739-x
Lazer DM, Baum MA, Benkler Y, Berinsky AJ, Greenhill KM, Menczer F, Metzger MJ, Nyhan B, Pennycook G, Rothschild D et al (2018) The science of fake news. Science 359(6380):1094–1096. https://doi.org/10.1126/science.aao2998
Le T, Shu K, Molina MD, Lee D, Sundar SS, Liu H (2019) 5 sources of clickbaits you should know! Using synthetic clickbaits to improve prediction and distinguish between bot-generated and human-written headlines. In: 2019 IEEE/ACM international conference on advances in social networks analysis and mining (ASONAM). IEEE, pp 33–40. https://doi.org/10.1145/3341161.3342875
Lewandowsky S (2020) Climate change, disinformation, and how to combat it. In: Annual Review of Public Health 42. https://doi.org/10.1146/annurev-publhealth-090419-102409
Liu Y, Wu YF (2018) Early detection of fake news on social media through propagation path classification with recurrent and convolutional networks. In: Proceedings of the AAAI conference on artificial intelligence, pp 354–361
Luo M, Hancock JT, Markowitz DM (2022) Credibility perceptions and detection accuracy of fake news headlines on social media: effects of truth-bias and endorsement cues. Commun Res 49(2):171–195. https://doi.org/10.1177/0093650220921321
Lutzke L, Drummond C, Slovic P, Árvai J (2019) Priming critical thinking: simple interventions limit the influence of fake news about climate change on Facebook. Glob Environ Chang 58:101964. https://doi.org/10.1016/j.gloenvcha.2019.101964
Maertens R, Anseel F, van der Linden S (2020) Combatting climate change misinformation: evidence for longevity of inoculation and consensus messaging effects. J Environ Psychol 70:101455. https://doi.org/10.1016/j.jenvp.2020.101455
Mahabub A (2020) A robust technique of fake news detection using ensemble voting classifier and comparison with other classifiers. SN Applied Sciences 2(4):1–9. https://doi.org/10.1007/s42452-020-2326-y
Mahbub S, Pardede E, Kayes A, Rahayu W (2019) Controlling astroturfing on the internet: a survey on detection techniques and research challenges. Int J Web Grid Serv 15(2):139–158. https://doi.org/10.1504/IJWGS.2019.099561
Marsden C, Meyer T, Brown I (2020) Platform values and democratic elections: how can the law regulate digital disinformation? Comput Law Secur Rev 36:105373. https://doi.org/10.1016/j.clsr.2019.105373
Masciari E, Moscato V, Picariello A, Sperlí G (2020) Detecting fake news by image analysis. In: Proceedings of the 24th symposium on international database engineering and applications, pp 1–5. https://doi.org/10.1145/3410566.3410599
Mazzeo V, Rapisarda A (2022) Investigating fake and reliable news sources using complex networks analysis. Front Phys 10:886544. https://doi.org/10.3389/fphy.2022.886544
McGrew S (2020) Learning to evaluate: an intervention in civic online reasoning. Comput Educ 145:103711. https://doi.org/10.1016/j.compedu.2019.103711
McGrew S, Breakstone J, Ortega T, Smith M, Wineburg S (2018) Can students evaluate online sources? Learning from assessments of civic online reasoning. Theory Res Soc Educ 46(2):165–193. https://doi.org/10.1080/00933104.2017.1416320
Meel P, Vishwakarma DK (2020) Fake news, rumor, information pollution in social media and web: a contemporary survey of state-of-the-arts, challenges and opportunities. Expert Syst Appl 153:112986. https://doi.org/10.1016/j.eswa.2019.112986
Meese J, Frith J, Wilken R (2020) Covid-19, 5G conspiracies and infrastructural futures. Media Int Aust 177(1):30–46. https://doi.org/10.1177/1329878X20952165
Metzger MJ, Hartsell EH, Flanagin AJ (2020) Cognitive dissonance or credibility? A comparison of two theoretical explanations for selective exposure to partisan news. Commun Res 47(1):3–28. https://doi.org/10.1177/0093650215613136
Micallef N, He B, Kumar S, Ahamad M, Memon N (2020) The role of the crowd in countering misinformation: a case study of the Covid-19 infodemic. arXiv preprint arXiv:2011.05773
Mihailidis P, Viotty S (2017) Spreadable spectacle in digital culture: civic expression, fake news, and the role of media literacies in “post-fact society. Am Behav Sci 61(4):441–454. https://doi.org/10.1177/0002764217701217
Mishra R (2020) Fake news detection using higher-order user to user mutual-attention progression in propagation paths. In: Proceedings of the IEEE/CVF conference on computer vision and pattern recognition workshops, pp 652–653
Mishra S, Shukla P, Agarwal R (2022) Analyzing machine learning enabled fake news detection techniques for diversified datasets. Wirel Commun Mobile Comput. https://doi.org/10.1155/2022/1575365
Molina MD, Sundar SS, Le T, Lee D (2021) “Fake news’’ is not simply false information: a concept explication and taxonomy of online content. Am Behav Sci 65(2):180–212. https://doi.org/10.1177/0002764219878224
Moro C, Birt JR (2022) Review bombing is a dirty practice, but research shows games do benefit from online feedback. Conversation. https://research.bond.edu.au/en/publications/review-bombing-is-a-dirty-practice-but-research-shows-games-do-be
Mustafaraj E, Metaxas PT (2017) The fake news spreading plague: was it preventable? In: Proceedings of the 2017 ACM on web science conference, pp 235–239. https://doi.org/10.1145/3091478.3091523
Nagel TW (2022) Measuring fake news acumen using a news media literacy instrument. J Media Liter Educ 14(1):29–42. https://doi.org/10.23860/JMLE-2022-14-1-3
Nakov P (2020) Can we spot the “fake news” before it was even written? arXiv preprint arXiv:2008.04374
Nekmat E (2020) Nudge effect of fact-check alerts: source influence and media skepticism on sharing of news misinformation in social media. Soc Media Soc. https://doi.org/10.1177/2056305119897322
Nygren T, Brounéus F, Svensson G (2019) Diversity and credibility in young people’s news feeds: a foundation for teaching and learning citizenship in a digital era. J Soc Sci Educ 18(2):87–109. https://doi.org/10.4119/jsse-917
Nyhan B, Reifler J (2015) Displacing misinformation about events: an experimental test of causal corrections. J Exp Polit Sci 2(1):81–93. https://doi.org/10.1017/XPS.2014.22
Nyhan B, Porter E, Reifler J, Wood TJ (2020) Taking fact-checks literally but not seriously? The effects of journalistic fact-checking on factual beliefs and candidate favorability. Polit Behav 42(3):939–960. https://doi.org/10.1007/s11109-019-09528-x
Nyow NX, Chua HN (2019) Detecting fake news with tweets’ properties. In: 2019 IEEE conference on application, information and network security (AINS), IEEE, pp 24–29. https://doi.org/10.1109/AINS47559.2019.8968706
Ochoa IS, de Mello G, Silva LA, Gomes AJ, Fernandes AM, Leithardt VRQ (2019) Fakechain: a blockchain architecture to ensure trust in social media networks. In: International conference on the quality of information and communications technology. Springer, Berlin, pp 105–118. https://doi.org/10.1007/978-3-030-29238-6_8
Ozbay FA, Alatas B (2020) Fake news detection within online social media using supervised artificial intelligence algorithms. Physica A 540:123174. https://doi.org/10.1016/j.physa.2019.123174
Ozturk P, Li H, Sakamoto Y (2015) Combating rumor spread on social media: the effectiveness of refutation and warning. In: 2015 48th Hawaii international conference on system sciences, IEEE, pp 2406–2414. https://doi.org/10.1109/HICSS.2015.288
Parikh SB, Atrey PK (2018) Media-rich fake news detection: a survey. In: 2018 IEEE conference on multimedia information processing and retrieval (MIPR), IEEE, pp 436–441. https://doi.org/10.1109/MIPR.2018.00093
Parrish K (2018) Deep learning & machine learning: what’s the difference? Online: https://parsers.me/deep-learning-machine-learning-whats-the-difference/ . Accessed 20 May 2020
Paschen J (2019) Investigating the emotional appeal of fake news using artificial intelligence and human contributions. J Prod Brand Manag 29(2):223–233. https://doi.org/10.1108/JPBM-12-2018-2179
Pathak A, Srihari RK (2019) Breaking! Presenting fake news corpus for automated fact checking. In: Proceedings of the 57th annual meeting of the association for computational linguistics: student research workshop, pp 357–362
Peng J, Detchon S, Choo KKR, Ashman H (2017) Astroturfing detection in social media: a binary n-gram-based approach. Concurr Comput: Pract Exp 29(17):e4013. https://doi.org/10.1002/cpe.4013
Pennycook G, Rand DG (2019) Fighting misinformation on social media using crowdsourced judgments of news source quality. Proc Natl Acad Sci 116(7):2521–2526. https://doi.org/10.1073/pnas.1806781116
Pennycook G, Rand DG (2020) Who falls for fake news? The roles of bullshit receptivity, overclaiming, familiarity, and analytic thinking. J Pers 88(2):185–200. https://doi.org/10.1111/jopy.12476
Pennycook G, Bear A, Collins ET, Rand DG (2020a) The implied truth effect: attaching warnings to a subset of fake news headlines increases perceived accuracy of headlines without warnings. Manag Sci 66(11):4944–4957. https://doi.org/10.1287/mnsc.2019.3478
Pennycook G, McPhetres J, Zhang Y, Lu JG, Rand DG (2020b) Fighting Covid-19 misinformation on social media: experimental evidence for a scalable accuracy-nudge intervention. Psychol Sci 31(7):770–780. https://doi.org/10.1177/0956797620939054
Potthast M, Kiesel J, Reinartz K, Bevendorff J, Stein B (2017) A stylometric inquiry into hyperpartisan and fake news. arXiv preprint arXiv:1702.05638
Previti M, Rodriguez-Fernandez V, Camacho D, Carchiolo V, Malgeri M (2020) Fake news detection using time series and user features classification. In: International conference on the applications of evolutionary computation (Part of EvoStar), Springer, Berlin, pp 339–353. https://doi.org/10.1007/978-3-030-43722-0_22
Przybyla P (2020) Capturing the style of fake news. In: Proceedings of the AAAI conference on artificial intelligence, pp 490–497. https://doi.org/10.1609/aaai.v34i01.5386
Qayyum A, Qadir J, Janjua MU, Sher F (2019) Using blockchain to rein in the new post-truth world and check the spread of fake news. IT Prof 21(4):16–24. https://doi.org/10.1109/MITP.2019.2910503
Qian F, Gong C, Sharma K, Liu Y (2018) Neural user response generator: fake news detection with collective user intelligence. In: IJCAI, vol 18, pp 3834–3840. https://doi.org/10.24963/ijcai.2018/533
Raza S, Ding C (2022) Fake news detection based on news content and social contexts: a transformer-based approach. Int J Data Sci Anal 13(4):335–362. https://doi.org/10.1007/s41060-021-00302-z
Ricard J, Medeiros J (2020) Using misinformation as a political weapon: Covid-19 and Bolsonaro in Brazil. Harv Kennedy School misinformation Rev 1(3). https://misinforeview.hks.harvard.edu/article/using-misinformation-as-a-political-weapon-covid-19-and-bolsonaro-in-brazil/
Roozenbeek J, van der Linden S (2019) Fake news game confers psychological resistance against online misinformation. Palgrave Commun 5(1):1–10. https://doi.org/10.1057/s41599-019-0279-9
Roozenbeek J, van der Linden S, Nygren T (2020a) Prebunking interventions based on the psychological theory of “inoculation’’ can reduce susceptibility to misinformation across cultures. Harv Kennedy School Misinformation Rev. https://doi.org/10.37016//mr-2020-008
Roozenbeek J, Schneider CR, Dryhurst S, Kerr J, Freeman AL, Recchia G, Van Der Bles AM, Van Der Linden S (2020b) Susceptibility to misinformation about Covid-19 around the world. R Soc Open Sci 7(10):201199. https://doi.org/10.1098/rsos.201199
Rubin VL, Conroy N, Chen Y, Cornwell S (2016) Fake news or truth? Using satirical cues to detect potentially misleading news. In: Proceedings of the second workshop on computational approaches to deception detection, pp 7–17
Ruchansky N, Seo S, Liu Y (2017) Csi: a hybrid deep model for fake news detection. In: Proceedings of the 2017 ACM on conference on information and knowledge management, pp 797–806. https://doi.org/10.1145/3132847.3132877
Schuyler AJ (2019) Regulating facts: a procedural framework for identifying, excluding, and deterring the intentional or knowing proliferation of fake news online. Univ Ill JL Technol Pol’y, vol 2019, pp 211–240
Shae Z, Tsai J (2019) AI blockchain platform for trusting news. In: 2019 IEEE 39th international conference on distributed computing systems (ICDCS), IEEE, pp 1610–1619. https://doi.org/10.1109/ICDCS.2019.00160
Shang W, Liu M, Lin W, Jia M (2018) Tracing the source of news based on blockchain. In: 2018 IEEE/ACIS 17th international conference on computer and information science (ICIS), IEEE, pp 377–381. https://doi.org/10.1109/ICIS.2018.8466516
Shao C, Ciampaglia GL, Flammini A, Menczer F (2016) Hoaxy: A platform for tracking online misinformation. In: Proceedings of the 25th international conference companion on world wide web, pp 745–750. https://doi.org/10.1145/2872518.2890098
Shao C, Ciampaglia GL, Varol O, Yang KC, Flammini A, Menczer F (2018) The spread of low-credibility content by social bots. Nat Commun 9(1):1–9. https://doi.org/10.1038/s41467-018-06930-7
Shao C, Hui PM, Wang L, Jiang X, Flammini A, Menczer F, Ciampaglia GL (2018) Anatomy of an online misinformation network. PLoS ONE 13(4):e0196087. https://doi.org/10.1371/journal.pone.0196087
Sharma K, Qian F, Jiang H, Ruchansky N, Zhang M, Liu Y (2019) Combating fake news: a survey on identification and mitigation techniques. ACM Trans Intell Syst Technol (TIST) 10(3):1–42. https://doi.org/10.1145/3305260
Sharma K, Seo S, Meng C, Rambhatla S, Liu Y (2020) Covid-19 on social media: analyzing misinformation in Twitter conversations. arXiv preprint arXiv:2003.12309
Shen C, Kasra M, Pan W, Bassett GA, Malloch Y, O’Brien JF (2019) Fake images: the effects of source, intermediary, and digital media literacy on contextual assessment of image credibility online. New Media Soc 21(2):438–463. https://doi.org/10.1177/1461444818799526
Sherman IN, Redmiles EM, Stokes JW (2020) Designing indicators to combat fake media. arXiv preprint arXiv:2010.00544
Shi P, Zhang Z, Choo KKR (2019) Detecting malicious social bots based on clickstream sequences. IEEE Access 7:28855–28862. https://doi.org/10.1109/ACCESS.2019.2901864
Shu K, Sliva A, Wang S, Tang J, Liu H (2017) Fake news detection on social media: a data mining perspective. ACM SIGKDD Explor Newsl 19(1):22–36. https://doi.org/10.1145/3137597.3137600
Shu K, Mahudeswaran D, Wang S, Lee D, Liu H (2018a) Fakenewsnet: a data repository with news content, social context and spatialtemporal information for studying fake news on social media. arXiv preprint arXiv:1809.01286 , https://doi.org/10.1089/big.2020.0062
Shu K, Wang S, Liu H (2018b) Understanding user profiles on social media for fake news detection. In: 2018 IEEE conference on multimedia information processing and retrieval (MIPR), IEEE, pp 430–435. https://doi.org/10.1109/MIPR.2018.00092
Shu K, Wang S, Liu H (2019a) Beyond news contents: the role of social context for fake news detection. In: Proceedings of the twelfth ACM international conference on web search and data mining, pp 312–320. https://doi.org/10.1145/3289600.3290994
Shu K, Zhou X, Wang S, Zafarani R, Liu H (2019b) The role of user profiles for fake news detection. In: Proceedings of the 2019 IEEE/ACM international conference on advances in social networks analysis and mining, pp 436–439. https://doi.org/10.1145/3341161.3342927
Shu K, Bhattacharjee A, Alatawi F, Nazer TH, Ding K, Karami M, Liu H (2020a) Combating disinformation in a social media age. Wiley Interdiscip Rev: Data Min Knowl Discov 10(6):e1385. https://doi.org/10.1002/widm.1385
Shu K, Mahudeswaran D, Wang S, Liu H (2020b) Hierarchical propagation networks for fake news detection: investigation and exploitation. Proc Int AAAI Conf Web Soc Media AAAI Press 14:626–637
Shu K, Wang S, Lee D, Liu H (2020c) Mining disinformation and fake news: concepts, methods, and recent advancements. In: Disinformation, misinformation, and fake news in social media. Springer, Berlin, pp 1–19 https://doi.org/10.1007/978-3-030-42699-6_1
Shu K, Zheng G, Li Y, Mukherjee S, Awadallah AH, Ruston S, Liu H (2020d) Early detection of fake news with multi-source weak social supervision. In: ECML/PKDD (3), pp 650–666
Singh VK, Ghosh I, Sonagara D (2021) Detecting fake news stories via multimodal analysis. J Am Soc Inf Sci 72(1):3–17. https://doi.org/10.1002/asi.24359
Sintos S, Agarwal PK, Yang J (2019) Selecting data to clean for fact checking: minimizing uncertainty vs. maximizing surprise. Proc VLDB Endowm 12(13), 2408–2421. https://doi.org/10.14778/3358701.3358708
Snow J (2017) Can AI win the war against fake news? MIT Technology Review Online: https://www.technologyreview.com/s/609717/can-ai-win-the-war-against-fake-news/ . Accessed 3 Oct. 2020
Song G, Kim S, Hwang H, Lee K (2019) Blockchain-based notarization for social media. In: 2019 IEEE international conference on consumer clectronics (ICCE), IEEE, pp 1–2 https://doi.org/10.1109/ICCE.2019.8661978
Starbird K, Arif A, Wilson T (2019) Disinformation as collaborative work: Surfacing the participatory nature of strategic information operations. In: Proceedings of the ACM on human–computer interaction, vol 3(CSCW), pp 1–26 https://doi.org/10.1145/3359229
Sterret D, Malato D, Benz J, Kantor L, Tompson T, Rosenstiel T, Sonderman J, Loker K, Swanson E (2018) Who shared it? How Americans decide what news to trust on social media. Technical report, Norc Working Paper Series, WP-2018-001, pp 1–24
Sutton RM, Douglas KM (2020) Conspiracy theories and the conspiracy mindset: implications for political ideology. Curr Opin Behav Sci 34:118–122. https://doi.org/10.1016/j.cobeha.2020.02.015
Tandoc EC Jr, Thomas RJ, Bishop L (2021) What is (fake) news? Analyzing news values (and more) in fake stories. Media Commun 9(1):110–119. https://doi.org/10.17645/mac.v9i1.3331
Tchakounté F, Faissal A, Atemkeng M, Ntyam A (2020) A reliable weighting scheme for the aggregation of crowd intelligence to detect fake news. Information 11(6):319. https://doi.org/10.3390/info11060319
Tchechmedjiev A, Fafalios P, Boland K, Gasquet M, Zloch M, Zapilko B, Dietze S, Todorov K (2019) Claimskg: a knowledge graph of fact-checked claims. In: International semantic web conference. Springer, Berlin, pp 309–324 https://doi.org/10.1007/978-3-030-30796-7_20
Treen KMd, Williams HT, O’Neill SJ (2020) Online misinformation about climate change. Wiley Interdiscip Rev Clim Change 11(5):e665. https://doi.org/10.1002/wcc.665
Tsang SJ (2020) Motivated fake news perception: the impact of news sources and policy support on audiences’ assessment of news fakeness. J Mass Commun Q. https://doi.org/10.1177/1077699020952129
Tschiatschek S, Singla A, Gomez Rodriguez M, Merchant A, Krause A (2018) Fake news detection in social networks via crowd signals. In: Companion proceedings of the the web conference 2018, pp 517–524. https://doi.org/10.1145/3184558.3188722
Uppada SK, Manasa K, Vidhathri B, Harini R, Sivaselvan B (2022) Novel approaches to fake news and fake account detection in OSNS: user social engagement and visual content centric model. Soc Netw Anal Min 12(1):1–19. https://doi.org/10.1007/s13278-022-00878-9
Van der Linden S, Roozenbeek J (2020) Psychological inoculation against fake news. In: Accepting, sharing, and correcting misinformation, the psychology of fake news. https://doi.org/10.4324/9780429295379-11
Van der Linden S, Panagopoulos C, Roozenbeek J (2020) You are fake news: political bias in perceptions of fake news. Media Cult Soc 42(3):460–470. https://doi.org/10.1177/0163443720906992
Valenzuela S, Muñiz C, Santos M (2022) Social media and belief in misinformation in mexico: a case of maximal panic, minimal effects? Int J Press Polit. https://doi.org/10.1177/19401612221088988
Vasu N, Ang B, Teo TA, Jayakumar S, Raizal M, Ahuja J (2018) Fake news: national security in the post-truth era. RSIS
Vereshchaka A, Cosimini S, Dong W (2020) Analyzing and distinguishing fake and real news to mitigate the problem of disinformation. In: Computational and mathematical organization theory, pp 1–15. https://doi.org/10.1007/s10588-020-09307-8
Verstraete M, Bambauer DE, Bambauer JR (2017) Identifying and countering fake news. Arizona legal studies discussion paper 73(17-15). https://doi.org/10.2139/ssrn.3007971
Vilmer J, Escorcia A, Guillaume M, Herrera J (2018) Information manipulation: a challenge for our democracies. In: Report by the Policy Planning Staff (CAPS) of the ministry for europe and foreign affairs, and the institute for strategic research (RSEM) of the Ministry for the Armed Forces
Vishwakarma DK, Varshney D, Yadav A (2019) Detection and veracity analysis of fake news via scrapping and authenticating the web search. Cogn Syst Res 58:217–229. https://doi.org/10.1016/j.cogsys.2019.07.004
Vlachos A, Riedel S (2014) Fact checking: task definition and dataset construction. In: Proceedings of the ACL 2014 workshop on language technologies and computational social science, pp 18–22. https://doi.org/10.3115/v1/W14-2508
von der Weth C, Abdul A, Fan S, Kankanhalli M (2020) Helping users tackle algorithmic threats on social media: a multimedia research agenda. In: Proceedings of the 28th ACM international conference on multimedia, pp 4425–4434. https://doi.org/10.1145/3394171.3414692
Vosoughi S, Roy D, Aral S (2018) The spread of true and false news online. Science 359(6380):1146–1151. https://doi.org/10.1126/science.aap9559
Vraga EK, Bode L (2017) Using expert sources to correct health misinformation in social media. Sci Commun 39(5):621–645. https://doi.org/10.1177/1075547017731776
Waldman AE (2017) The marketplace of fake news. Univ Pa J Const Law 20:845
Wang WY (2017) “Liar, liar pants on fire”: a new benchmark dataset for fake news detection. arXiv preprint arXiv:1705.00648
Wang L, Wang Y, de Melo G, Weikum G (2019a) Understanding archetypes of fake news via fine-grained classification. Soc Netw Anal Min 9(1):1–17. https://doi.org/10.1007/s13278-019-0580-z
Wang Y, Han H, Ding Y, Wang X, Liao Q (2019b) Learning contextual features with multi-head self-attention for fake news detection. In: International conference on cognitive computing. Springer, Berlin, pp 132–142. https://doi.org/10.1007/978-3-030-23407-2_11
Wang Y, McKee M, Torbica A, Stuckler D (2019c) Systematic literature review on the spread of health-related misinformation on social media. Soc Sci Med 240:112552. https://doi.org/10.1016/j.socscimed.2019.112552
Wang Y, Yang W, Ma F, Xu J, Zhong B, Deng Q, Gao J (2020) Weak supervision for fake news detection via reinforcement learning. In: Proceedings of the AAAI conference on artificial intelligence, pp 516–523. https://doi.org/10.1609/aaai.v34i01.5389
Wardle C (2017) Fake news. It’s complicated. Online: https://medium.com/1st-draft/fake-news-its-complicated-d0f773766c79 . Accessed 3 Oct 2020
Wardle C (2018) The need for smarter definitions and practical, timely empirical research on information disorder. Digit J 6(8):951–963. https://doi.org/10.1080/21670811.2018.1502047
Wardle C, Derakhshan H (2017) Information disorder: toward an interdisciplinary framework for research and policy making. Council Eur Rep 27:1–107
Weiss AP, Alwan A, Garcia EP, Garcia J (2020) Surveying fake news: assessing university faculty’s fragmented definition of fake news and its impact on teaching critical thinking. Int J Educ Integr 16(1):1–30. https://doi.org/10.1007/s40979-019-0049-x
Wu L, Liu H (2018) Tracing fake-news footprints: characterizing social media messages by how they propagate. In: Proceedings of the eleventh ACM international conference on web search and data mining, pp 637–645. https://doi.org/10.1145/3159652.3159677
Wu L, Rao Y (2020) Adaptive interaction fusion networks for fake news detection. arXiv preprint arXiv:2004.10009
Wu L, Morstatter F, Carley KM, Liu H (2019) Misinformation in social media: definition, manipulation, and detection. ACM SIGKDD Explor Newsl 21(2):80–90. https://doi.org/10.1145/3373464.3373475
Wu Y, Ngai EW, Wu P, Wu C (2022) Fake news on the internet: a literature review, synthesis and directions for future research. Intern Res. https://doi.org/10.1108/INTR-05-2021-0294
Xu K, Wang F, Wang H, Yang B (2019) Detecting fake news over online social media via domain reputations and content understanding. Tsinghua Sci Technol 25(1):20–27. https://doi.org/10.26599/TST.2018.9010139
Yang F, Pentyala SK, Mohseni S, Du M, Yuan H, Linder R, Ragan ED, Ji S, Hu X (2019a) Xfake: explainable fake news detector with visualizations. In: The world wide web conference, pp 3600–3604. https://doi.org/10.1145/3308558.3314119
Yang X, Li Y, Lyu S (2019b) Exposing deep fakes using inconsistent head poses. In: ICASSP 2019-2019 IEEE international conference on acoustics, speech and signal processing (ICASSP), IEEE, pp 8261–8265. https://doi.org/10.1109/ICASSP.2019.8683164
Yaqub W, Kakhidze O, Brockman ML, Memon N, Patil S (2020) Effects of credibility indicators on social media news sharing intent. In: Proceedings of the 2020 CHI conference on human factors in computing systems, pp 1–14. https://doi.org/10.1145/3313831.3376213
Yavary A, Sajedi H, Abadeh MS (2020) Information verification in social networks based on user feedback and news agencies. Soc Netw Anal Min 10(1):1–8. https://doi.org/10.1007/s13278-019-0616-4
Yazdi KM, Yazdi AM, Khodayi S, Hou J, Zhou W, Saedy S (2020) Improving fake news detection using k-means and support vector machine approaches. Int J Electron Commun Eng 14(2):38–42. https://doi.org/10.5281/zenodo.3669287
Zannettou S, Sirivianos M, Blackburn J, Kourtellis N (2019) The web of false information: rumors, fake news, hoaxes, clickbait, and various other shenanigans. J Data Inf Qual (JDIQ) 11(3):1–37. https://doi.org/10.1145/3309699
Zellers R, Holtzman A, Rashkin H, Bisk Y, Farhadi A, Roesner F, Choi Y (2019) Defending against neural fake news. arXiv preprint arXiv:1905.12616
Zhang X, Ghorbani AA (2020) An overview of online fake news: characterization, detection, and discussion. Inf Process Manag 57(2):102025. https://doi.org/10.1016/j.ipm.2019.03.004
Zhang J, Dong B, Philip SY (2020) Fakedetector: effective fake news detection with deep diffusive neural network. In: 2020 IEEE 36th international conference on data engineering (ICDE), IEEE, pp 1826–1829. 10.1109/ICDE48307.2020.00180
Zhang Q, Lipani A, Liang S, Yilmaz E (2019a) Reply-aided detection of misinformation via Bayesian deep learning. In: The world wide web conference, pp 2333–2343. https://doi.org/10.1145/3308558.3313718
Zhang X, Karaman S, Chang SF (2019b) Detecting and simulating artifacts in GAN fake images. In: 2019 IEEE international workshop on information forensics and security (WIFS), IEEE, pp 1–6 https://doi.org/10.1109/WIFS47025.2019.9035107
Zhou X, Zafarani R (2020) A survey of fake news: fundamental theories, detection methods, and opportunities. ACM Comput Surv (CSUR) 53(5):1–40. https://doi.org/10.1145/3395046
Zubiaga A, Aker A, Bontcheva K, Liakata M, Procter R (2018) Detection and resolution of rumours in social media: a survey. ACM Comput Surv (CSUR) 51(2):1–36. https://doi.org/10.1145/3161603
Download references
This work is supported in part by Canada’s Natural Sciences and Engineering Research Council.
Author information
Authors and affiliations.
Department of Computer Science and Operations Research (DIRO), University of Montreal, Montreal, Canada
Esma Aïmeur, Sabrine Amri & Gilles Brassard
You can also search for this author in PubMed Google Scholar
Contributions
The order of authors is alphabetic as is customary in the third author’s field. The lead author was Sabrine Amri, who collected and analyzed the data and wrote a first draft of the paper, all along under the supervision and tight guidance of Esma Aïmeur. Gilles Brassard reviewed, criticized and polished the work into its final form.
Corresponding author
Correspondence to Sabrine Amri .
Ethics declarations
Conflict of interest.
On behalf of all authors, the corresponding author states that there is no conflict of interest.
Additional information
Publisher's note.
Springer Nature remains neutral with regard to jurisdictional claims in published maps and institutional affiliations.
Appendix: A Comparison of AI-based fake news detection techniques
This Appendix consists only in the rather long Table 11 . It shows a comparison of the fake news detection solutions based on artificial intelligence that we have reviewed according to their main approaches, the methodology that was used, and the models, as explained in Sect. 6.2.2 .
Rights and permissions
Springer Nature or its licensor (e.g. a society or other partner) holds exclusive rights to this article under a publishing agreement with the author(s) or other rightsholder(s); author self-archiving of the accepted manuscript version of this article is solely governed by the terms of such publishing agreement and applicable law.
Reprints and permissions
About this article
Aïmeur, E., Amri, S. & Brassard, G. Fake news, disinformation and misinformation in social media: a review. Soc. Netw. Anal. Min. 13 , 30 (2023). https://doi.org/10.1007/s13278-023-01028-5
Download citation
Received : 20 October 2022
Revised : 07 January 2023
Accepted : 12 January 2023
Published : 09 February 2023
DOI : https://doi.org/10.1007/s13278-023-01028-5
Share this article
Anyone you share the following link with will be able to read this content:
Sorry, a shareable link is not currently available for this article.
Provided by the Springer Nature SharedIt content-sharing initiative
- Disinformation
- Misinformation
- Information disorder
- Online deception
- Online social networks
- Find a journal
- Publish with us
- Track your research
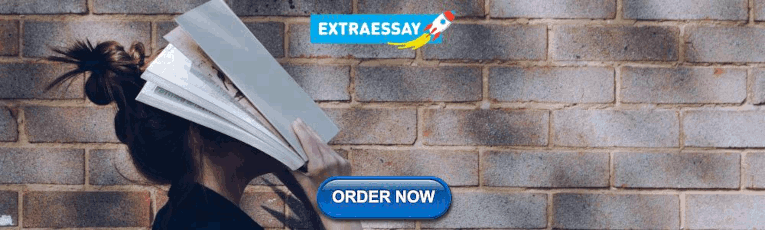
IMAGES
VIDEO
COMMENTS
the knowledge gaps and research needs brought to light by a critical review of the relevant literature and then ensuring that their research design, methods, results, and conclusions follow logically from these objectives (Maier, 2013). There exist a number of papers devoted to instruction on how to write a good review paper. Among the most
Scholarly literature can be of different types; some of which require that researchers conduct an original study, whereas others can be based on existing research. One of the most popular Q&As led us to conclude that of all the types of scholarly literature, researchers are most confused by the differences between a research paper and a review paper. This infographic explains the five main ...
The research paper will be based on the analysis and interpretation of this data. A review article or review paper is based on other published articles. It does not report original research. Review articles generally summarize the existing literature on a topic in an attempt to explain the current state of understanding on the topic.
How to review a paper. A good peer review requires disciplinary expertise, a keen and critical eye, and a diplomatic and constructive approach. Credit: dmark/iStockphoto. As junior scientists develop their expertise and make names for themselves, they are increasingly likely to receive invitations to review research manuscripts.
A literature review is a survey of credible sources on a topic, often used in dissertations, theses, and research papers. Literature reviews give an overview of knowledge on a subject, helping you identify relevant theories and methods, as well as gaps in existing research.
One of my favourite review-style articles 3 presents a plot bringing together data from multiple research papers (many of which directly contradict each other). This is then used to identify broad ...
Think about structuring your review like an inverted pyramid. Put the most important information at the top, followed by details and examples in the center, and any additional points at the very bottom. Here's how your outline might look: 1. Summary of the research and your overall impression. In your own words, summarize what the manuscript ...
The fundamental rationale of writing a review article is to make a readable synthesis of the best literature sources on an important research inquiry or a topic. This simple definition of a review article contains the following key elements: The question (s) to be dealt with.
Just like research papers, the most common and convenient practice is to write review papers in "introduction, methods, results, and discussion (IMRaD)" format accompanied by title, abstract, key words, and references. The title makes the first introductory and is the most important sentence of the review paper.
Many research disciplines feature high-impact journals that are dedicated outlets for review papers (or review-conceptual combinations) (e.g., Academy of Management Review, Psychology Bulletin, Medicinal Research Reviews).The rationale for such outlets is the premise that research integration and synthesis provides an important, and possibly even a required, step in the scientific process.
Time needed: 20 days and 7 hours. The systematic procedural steps to write the best review paper are as follows: Topic selection. Select a suitable area in your research field formulate clear objectives, and prepare the specific research hypotheses that are to be explored. Research design.
A review article can also be called a literature review, or a review of literature. It is a survey of previously published research on a topic. It should give an overview of current thinking on the topic. And, unlike an original research article, it will not present new experimental results. Writing a review of literature is to provide a ...
Like a well-baked cake, a good review has a number of telling features: it is worth the reader's time, timely, systematic, well written, focused, and critical. It also needs a good structure. With reviews, the usual subdivision of research papers into introduction, methods, results, and discussion does not work or is rarely used.
WHAT IS A REVIEW PAPER? The purpose of a review paper is to succinctly review recent progress in a particular topic. Overall, the paper summarizes the current state of knowledge of the topic. It creates an understanding of the topic for the reader by discussing the findings presented in recent research papers. A review paper is not a "term ...
With research accelerating at an unprecedented speed in recent years and more and more original papers being published, review articles have become increasingly important as a means to keep up to date with developments in a particular area of research. A good review article provides readers with an in-depth understanding of a field and ...
A literature review can be a part of a research paper or scholarly article, usually falling after the introduction and before the research methods sections. In these cases, the lit review just needs to cover scholarship that is important to the issue you are writing about; sometimes it will also cover key sources that informed your research ...
Format for a review paper Title page: Title-- reflecting topic of review Your Name Date Abstract: An abstract should be of approximately 200-300 words. Provide a brief ... In these sections, be sure to describe the research methods and evaluate how studies were conducted focusing on the study design and analysis e.g., intention to treat versus ...
A literature review is a surveys scholarly articles, books and other sources relevant to a particular. issue, area of research, or theory, and by so doing, providing a description, summary, and ...
A review article is a comprehensive summary of the current understanding of a specific research topic and is based on previously published research. Unlike research papers, it does not contain new results, but can propose new inferences based on the combined findings of previous research. Types of review articles
This paper discusses literature review as a methodology for conducting research and offers an overview of different types of reviews, as well as some guidelines to how to both conduct and evaluate a literature review paper. It also discusses common pitfalls and how to get literature reviews published. 1.
1. review papers in a field that you are an expert in. 2. always read well in terms of recent research conducted in the field of knowledge under review. 3. check if the title of the paper you are ...
As a revolutionary neural implicit field representation, NeRF has caused a continuous research boom in the academic community. Therefore, the purpose of this review is to provide an in-depth analysis of the research literature on NeRF within the past two years, to provide a comprehensive academic perspective for budding researchers.
Scoping reviews help provide an overview of the research available on a given area of interest where evidence is emerging [].While there are several accepted approaches to such reviews, this scoping review was undertaken using the Joanna Briggs Institute (JBI) Guideline for scoping reviews [].This approach was based on the Arksey and O'Malley methodological framework [], which was further ...
Given the uncertainty surrounding how existing explainability methods for autonomous vehicles (AVs) meet the diverse needs of stakeholders, a thorough investigation is imperative to determine the contexts requiring explanations and suitable interaction strategies. A comprehensive review becomes crucial to assess the alignment of current approaches with the varied interests and expectations ...
Methodology: A Systematic Review. This paper leverages from existing evidence by conducting a systematic review of existing studies. Systematic review method has been extensively employed in examining the linkage between climate change and violent conflict (Ide 2018; Nordqvist and Krampe 2018; Van Baalen and Mobjörk 2018; Tarif 2022 ...
Recent advances in single-cell biotechnologies have resulted in high-dimensional datasets with increased complexity, making feature selection an essential technique for single-cell data analysis. Here, we revisit feature selection techniques and summarise recent developments. We review their application to a range of single-cell data types generated from traditional cytometry and imaging ...
We focused our research over a period of ten years, but we made sure that about two-thirds of the research papers that we considered were published in or after 2019. Additionally, we defined a set of keywords to search the above-mentioned scientific databases since we concentrated on reviewing the current state of the art in addition to the ...
We review the ecological, morphological and genetic data for the well-studied 'resident' and Bigg's (aka 'transient') ecotypes in the eastern North Pacific and use quantitative taxonomic guidelines and standards to determine whether the taxonomic status of these killer whale ecotypes should be revised. ... This paper is dedicated to ...