6.894 : Interactive Data Visualization
Assignment 2: exploratory data analysis.
In this assignment, you will identify a dataset of interest and perform an exploratory analysis to better understand the shape & structure of the data, investigate initial questions, and develop preliminary insights & hypotheses. Your final submission will take the form of a report consisting of captioned visualizations that convey key insights gained during your analysis.
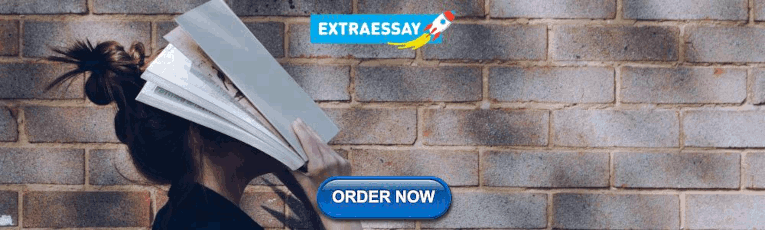
Step 1: Data Selection
First, you will pick a topic area of interest to you and find a dataset that can provide insights into that topic. To streamline the assignment, we've pre-selected a number of datasets for you to choose from.
However, if you would like to investigate a different topic and dataset, you are free to do so. If working with a self-selected dataset, please check with the course staff to ensure it is appropriate for the course. Be advised that data collection and preparation (also known as data wrangling ) can be a very tedious and time-consuming process. Be sure you have sufficient time to conduct exploratory analysis, after preparing the data.
After selecting a topic and dataset – but prior to analysis – you should write down an initial set of at least three questions you'd like to investigate.
Part 2: Exploratory Visual Analysis
Next, you will perform an exploratory analysis of your dataset using a visualization tool such as Tableau. You should consider two different phases of exploration.
In the first phase, you should seek to gain an overview of the shape & stucture of your dataset. What variables does the dataset contain? How are they distributed? Are there any notable data quality issues? Are there any surprising relationships among the variables? Be sure to also perform "sanity checks" for patterns you expect to see!
In the second phase, you should investigate your initial questions, as well as any new questions that arise during your exploration. For each question, start by creating a visualization that might provide a useful answer. Then refine the visualization (by adding additional variables, changing sorting or axis scales, filtering or subsetting data, etc. ) to develop better perspectives, explore unexpected observations, or sanity check your assumptions. You should repeat this process for each of your questions, but feel free to revise your questions or branch off to explore new questions if the data warrants.
- Final Deliverable
Your final submission should take the form of a Google Docs report – similar to a slide show or comic book – that consists of 10 or more captioned visualizations detailing your most important insights. Your "insights" can include important surprises or issues (such as data quality problems affecting your analysis) as well as responses to your analysis questions. To help you gauge the scope of this assignment, see this example report analyzing data about motion pictures . We've annotated and graded this example to help you calibrate for the breadth and depth of exploration we're looking for.
Each visualization image should be a screenshot exported from a visualization tool, accompanied with a title and descriptive caption (1-4 sentences long) describing the insight(s) learned from that view. Provide sufficient detail for each caption such that anyone could read through your report and understand what you've learned. You are free, but not required, to annotate your images to draw attention to specific features of the data. You may perform highlighting within the visualization tool itself, or draw annotations on the exported image. To easily export images from Tableau, use the Worksheet > Export > Image... menu item.
The end of your report should include a brief summary of main lessons learned.
Recommended Data Sources
To get up and running quickly with this assignment, we recommend exploring one of the following provided datasets:
World Bank Indicators, 1960–2017 . The World Bank has tracked global human developed by indicators such as climate change, economy, education, environment, gender equality, health, and science and technology since 1960. The linked repository contains indicators that have been formatted to facilitate use with Tableau and other data visualization tools. However, you're also welcome to browse and use the original data by indicator or by country . Click on an indicator category or country to download the CSV file.
Chicago Crimes, 2001–present (click Export to download a CSV file). This dataset reflects reported incidents of crime (with the exception of murders where data exists for each victim) that occurred in the City of Chicago from 2001 to present, minus the most recent seven days. Data is extracted from the Chicago Police Department's CLEAR (Citizen Law Enforcement Analysis and Reporting) system.
Daily Weather in the U.S., 2017 . This dataset contains daily U.S. weather measurements in 2017, provided by the NOAA Daily Global Historical Climatology Network . This data has been transformed: some weather stations with only sparse measurements have been filtered out. See the accompanying weather.txt for descriptions of each column .
Social mobility in the U.S. . Raj Chetty's group at Harvard studies the factors that contribute to (or hinder) upward mobility in the United States (i.e., will our children earn more than we will). Their work has been extensively featured in The New York Times. This page lists data from all of their papers, broken down by geographic level or by topic. We recommend downloading data in the CSV/Excel format, and encourage you to consider joining multiple datasets from the same paper (under the same heading on the page) for a sufficiently rich exploratory process.
The Yelp Open Dataset provides information about businesses, user reviews, and more from Yelp's database. The data is split into separate files ( business , checkin , photos , review , tip , and user ), and is available in either JSON or SQL format. You might use this to investigate the distributions of scores on Yelp, look at how many reviews users typically leave, or look for regional trends about restaurants. Note that this is a large, structured dataset and you don't need to look at all of the data to answer interesting questions. In order to download the data you will need to enter your email and agree to Yelp's Dataset License .
Additional Data Sources
If you want to investigate datasets other than those recommended above, here are some possible sources to consider. You are also free to use data from a source different from those included here. If you have any questions on whether your dataset is appropriate, please ask the course staff ASAP!
- data.boston.gov - City of Boston Open Data
- MassData - State of Masachussets Open Data
- data.gov - U.S. Government Open Datasets
- U.S. Census Bureau - Census Datasets
- IPUMS.org - Integrated Census & Survey Data from around the World
- Federal Elections Commission - Campaign Finance & Expenditures
- Federal Aviation Administration - FAA Data & Research
- fivethirtyeight.com - Data and Code behind the Stories and Interactives
- Buzzfeed News
- Socrata Open Data
- 17 places to find datasets for data science projects
Visualization Tools
You are free to use one or more visualization tools in this assignment. However, in the interest of time and for a friendlier learning curve, we strongly encourage you to use Tableau . Tableau provides a graphical interface focused on the task of visual data exploration. You will (with rare exceptions) be able to complete an initial data exploration more quickly and comprehensively than with a programming-based tool.
- Tableau - Desktop visual analysis software . Available for both Windows and MacOS; register for a free student license.
- Data Transforms in Vega-Lite . A tutorial on the various built-in data transformation operators available in Vega-Lite.
- Data Voyager , a research prototype from the UW Interactive Data Lab, combines a Tableau-style interface with visualization recommendations. Use at your own risk!
- R , using the ggplot2 library or with R's built-in plotting functions.
- Jupyter Notebooks (Python) , using libraries such as Altair or Matplotlib .
Data Wrangling Tools
The data you choose may require reformatting, transformation or cleaning prior to visualization. Here are tools you can use for data preparation. We recommend first trying to import and process your data in the same tool you intend to use for visualization. If that fails, pick the most appropriate option among the tools below. Contact the course staff if you are unsure what might be the best option for your data!
Graphical Tools
- Tableau Prep - Tableau provides basic facilities for data import, transformation & blending. Tableau prep is a more sophisticated data preparation tool
- Trifacta Wrangler - Interactive tool for data transformation & visual profiling.
- OpenRefine - A free, open source tool for working with messy data.
Programming Tools
- JavaScript data utilities and/or the Datalib JS library .
- Pandas - Data table and manipulation utilites for Python.
- dplyr - A library for data manipulation in R.
- Or, the programming language and tools of your choice...
The assignment score is out of a maximum of 10 points. Submissions that squarely meet the requirements will receive a score of 8. We will determine scores by judging the breadth and depth of your analysis, whether visualizations meet the expressivenes and effectiveness principles, and how well-written and synthesized your insights are.
We will use the following rubric to grade your assignment. Note, rubric cells may not map exactly to specific point scores.
Submission Details
This is an individual assignment. You may not work in groups.
Your completed exploratory analysis report is due by noon on Wednesday 2/19 . Submit a link to your Google Doc report using this submission form . Please double check your link to ensure it is viewable by others (e.g., try it in an incognito window).
Resubmissions. Resubmissions will be regraded by teaching staff, and you may earn back up to 50% of the points lost in the original submission. To resubmit this assignment, please use this form and follow the same submission process described above. Include a short 1 paragraph description summarizing the changes from the initial submission. Resubmissions without this summary will not be regraded. Resubmissions will be due by 11:59pm on Saturday, 3/14. Slack days may not be applied to extend the resubmission deadline. The teaching staff will only begin to regrade assignments once the Final Project phase begins, so please be patient.
- Due: 12pm, Wed 2/19
- Recommended Datasets
- Example Report
- Visualization & Data Wrangling Tools
- Submission form
A Step-by-Step Guide to the Data Analysis Process
Like any scientific discipline, data analysis follows a rigorous step-by-step process. Each stage requires different skills and know-how. To get meaningful insights, though, it’s important to understand the process as a whole. An underlying framework is invaluable for producing results that stand up to scrutiny.
In this post, we’ll explore the main steps in the data analysis process. This will cover how to define your goal, collect data, and carry out an analysis. Where applicable, we’ll also use examples and highlight a few tools to make the journey easier. When you’re done, you’ll have a much better understanding of the basics. This will help you tweak the process to fit your own needs.
Here are the steps we’ll take you through:
- Defining the question
- Collecting the data
- Cleaning the data
- Analyzing the data
- Sharing your results
- Embracing failure
On popular request, we’ve also developed a video based on this article. Scroll further along this article to watch that.
Ready? Let’s get started with step one.
1. Step one: Defining the question
The first step in any data analysis process is to define your objective. In data analytics jargon, this is sometimes called the ‘problem statement’.
Defining your objective means coming up with a hypothesis and figuring how to test it. Start by asking: What business problem am I trying to solve? While this might sound straightforward, it can be trickier than it seems. For instance, your organization’s senior management might pose an issue, such as: “Why are we losing customers?” It’s possible, though, that this doesn’t get to the core of the problem. A data analyst’s job is to understand the business and its goals in enough depth that they can frame the problem the right way.
Let’s say you work for a fictional company called TopNotch Learning. TopNotch creates custom training software for its clients. While it is excellent at securing new clients, it has much lower repeat business. As such, your question might not be, “Why are we losing customers?” but, “Which factors are negatively impacting the customer experience?” or better yet: “How can we boost customer retention while minimizing costs?”
Now you’ve defined a problem, you need to determine which sources of data will best help you solve it. This is where your business acumen comes in again. For instance, perhaps you’ve noticed that the sales process for new clients is very slick, but that the production team is inefficient. Knowing this, you could hypothesize that the sales process wins lots of new clients, but the subsequent customer experience is lacking. Could this be why customers don’t come back? Which sources of data will help you answer this question?
Tools to help define your objective
Defining your objective is mostly about soft skills, business knowledge, and lateral thinking. But you’ll also need to keep track of business metrics and key performance indicators (KPIs). Monthly reports can allow you to track problem points in the business. Some KPI dashboards come with a fee, like Databox and DashThis . However, you’ll also find open-source software like Grafana , Freeboard , and Dashbuilder . These are great for producing simple dashboards, both at the beginning and the end of the data analysis process.
2. Step two: Collecting the data
Once you’ve established your objective, you’ll need to create a strategy for collecting and aggregating the appropriate data. A key part of this is determining which data you need. This might be quantitative (numeric) data, e.g. sales figures, or qualitative (descriptive) data, such as customer reviews. All data fit into one of three categories: first-party, second-party, and third-party data. Let’s explore each one.
What is first-party data?
First-party data are data that you, or your company, have directly collected from customers. It might come in the form of transactional tracking data or information from your company’s customer relationship management (CRM) system. Whatever its source, first-party data is usually structured and organized in a clear, defined way. Other sources of first-party data might include customer satisfaction surveys, focus groups, interviews, or direct observation.
What is second-party data?
To enrich your analysis, you might want to secure a secondary data source. Second-party data is the first-party data of other organizations. This might be available directly from the company or through a private marketplace. The main benefit of second-party data is that they are usually structured, and although they will be less relevant than first-party data, they also tend to be quite reliable. Examples of second-party data include website, app or social media activity, like online purchase histories, or shipping data.
What is third-party data?
Third-party data is data that has been collected and aggregated from numerous sources by a third-party organization. Often (though not always) third-party data contains a vast amount of unstructured data points (big data). Many organizations collect big data to create industry reports or to conduct market research. The research and advisory firm Gartner is a good real-world example of an organization that collects big data and sells it on to other companies. Open data repositories and government portals are also sources of third-party data .
Tools to help you collect data
Once you’ve devised a data strategy (i.e. you’ve identified which data you need, and how best to go about collecting them) there are many tools you can use to help you. One thing you’ll need, regardless of industry or area of expertise, is a data management platform (DMP). A DMP is a piece of software that allows you to identify and aggregate data from numerous sources, before manipulating them, segmenting them, and so on. There are many DMPs available. Some well-known enterprise DMPs include Salesforce DMP , SAS , and the data integration platform, Xplenty . If you want to play around, you can also try some open-source platforms like Pimcore or D:Swarm .
Want to learn more about what data analytics is and the process a data analyst follows? We cover this topic (and more) in our free introductory short course for beginners. Check out tutorial one: An introduction to data analytics .
3. Step three: Cleaning the data
Once you’ve collected your data, the next step is to get it ready for analysis. This means cleaning, or ‘scrubbing’ it, and is crucial in making sure that you’re working with high-quality data . Key data cleaning tasks include:
- Removing major errors, duplicates, and outliers —all of which are inevitable problems when aggregating data from numerous sources.
- Removing unwanted data points —extracting irrelevant observations that have no bearing on your intended analysis.
- Bringing structure to your data —general ‘housekeeping’, i.e. fixing typos or layout issues, which will help you map and manipulate your data more easily.
- Filling in major gaps —as you’re tidying up, you might notice that important data are missing. Once you’ve identified gaps, you can go about filling them.
A good data analyst will spend around 70-90% of their time cleaning their data. This might sound excessive. But focusing on the wrong data points (or analyzing erroneous data) will severely impact your results. It might even send you back to square one…so don’t rush it! You’ll find a step-by-step guide to data cleaning here . You may be interested in this introductory tutorial to data cleaning, hosted by Dr. Humera Noor Minhas.
Carrying out an exploratory analysis
Another thing many data analysts do (alongside cleaning data) is to carry out an exploratory analysis. This helps identify initial trends and characteristics, and can even refine your hypothesis. Let’s use our fictional learning company as an example again. Carrying out an exploratory analysis, perhaps you notice a correlation between how much TopNotch Learning’s clients pay and how quickly they move on to new suppliers. This might suggest that a low-quality customer experience (the assumption in your initial hypothesis) is actually less of an issue than cost. You might, therefore, take this into account.
Tools to help you clean your data
Cleaning datasets manually—especially large ones—can be daunting. Luckily, there are many tools available to streamline the process. Open-source tools, such as OpenRefine , are excellent for basic data cleaning, as well as high-level exploration. However, free tools offer limited functionality for very large datasets. Python libraries (e.g. Pandas) and some R packages are better suited for heavy data scrubbing. You will, of course, need to be familiar with the languages. Alternatively, enterprise tools are also available. For example, Data Ladder , which is one of the highest-rated data-matching tools in the industry. There are many more. Why not see which free data cleaning tools you can find to play around with?
4. Step four: Analyzing the data
Finally, you’ve cleaned your data. Now comes the fun bit—analyzing it! The type of data analysis you carry out largely depends on what your goal is. But there are many techniques available. Univariate or bivariate analysis, time-series analysis, and regression analysis are just a few you might have heard of. More important than the different types, though, is how you apply them. This depends on what insights you’re hoping to gain. Broadly speaking, all types of data analysis fit into one of the following four categories.
Descriptive analysis
Descriptive analysis identifies what has already happened . It is a common first step that companies carry out before proceeding with deeper explorations. As an example, let’s refer back to our fictional learning provider once more. TopNotch Learning might use descriptive analytics to analyze course completion rates for their customers. Or they might identify how many users access their products during a particular period. Perhaps they’ll use it to measure sales figures over the last five years. While the company might not draw firm conclusions from any of these insights, summarizing and describing the data will help them to determine how to proceed.
Learn more: What is descriptive analytics?
Diagnostic analysis
Diagnostic analytics focuses on understanding why something has happened . It is literally the diagnosis of a problem, just as a doctor uses a patient’s symptoms to diagnose a disease. Remember TopNotch Learning’s business problem? ‘Which factors are negatively impacting the customer experience?’ A diagnostic analysis would help answer this. For instance, it could help the company draw correlations between the issue (struggling to gain repeat business) and factors that might be causing it (e.g. project costs, speed of delivery, customer sector, etc.) Let’s imagine that, using diagnostic analytics, TopNotch realizes its clients in the retail sector are departing at a faster rate than other clients. This might suggest that they’re losing customers because they lack expertise in this sector. And that’s a useful insight!
Predictive analysis
Predictive analysis allows you to identify future trends based on historical data . In business, predictive analysis is commonly used to forecast future growth, for example. But it doesn’t stop there. Predictive analysis has grown increasingly sophisticated in recent years. The speedy evolution of machine learning allows organizations to make surprisingly accurate forecasts. Take the insurance industry. Insurance providers commonly use past data to predict which customer groups are more likely to get into accidents. As a result, they’ll hike up customer insurance premiums for those groups. Likewise, the retail industry often uses transaction data to predict where future trends lie, or to determine seasonal buying habits to inform their strategies. These are just a few simple examples, but the untapped potential of predictive analysis is pretty compelling.
Prescriptive analysis
Prescriptive analysis allows you to make recommendations for the future. This is the final step in the analytics part of the process. It’s also the most complex. This is because it incorporates aspects of all the other analyses we’ve described. A great example of prescriptive analytics is the algorithms that guide Google’s self-driving cars. Every second, these algorithms make countless decisions based on past and present data, ensuring a smooth, safe ride. Prescriptive analytics also helps companies decide on new products or areas of business to invest in.
Learn more: What are the different types of data analysis?
5. Step five: Sharing your results
You’ve finished carrying out your analyses. You have your insights. The final step of the data analytics process is to share these insights with the wider world (or at least with your organization’s stakeholders!) This is more complex than simply sharing the raw results of your work—it involves interpreting the outcomes, and presenting them in a manner that’s digestible for all types of audiences. Since you’ll often present information to decision-makers, it’s very important that the insights you present are 100% clear and unambiguous. For this reason, data analysts commonly use reports, dashboards, and interactive visualizations to support their findings.
How you interpret and present results will often influence the direction of a business. Depending on what you share, your organization might decide to restructure, to launch a high-risk product, or even to close an entire division. That’s why it’s very important to provide all the evidence that you’ve gathered, and not to cherry-pick data. Ensuring that you cover everything in a clear, concise way will prove that your conclusions are scientifically sound and based on the facts. On the flip side, it’s important to highlight any gaps in the data or to flag any insights that might be open to interpretation. Honest communication is the most important part of the process. It will help the business, while also helping you to excel at your job!
Tools for interpreting and sharing your findings
There are tons of data visualization tools available, suited to different experience levels. Popular tools requiring little or no coding skills include Google Charts , Tableau , Datawrapper , and Infogram . If you’re familiar with Python and R, there are also many data visualization libraries and packages available. For instance, check out the Python libraries Plotly , Seaborn , and Matplotlib . Whichever data visualization tools you use, make sure you polish up your presentation skills, too. Remember: Visualization is great, but communication is key!
You can learn more about storytelling with data in this free, hands-on tutorial . We show you how to craft a compelling narrative for a real dataset, resulting in a presentation to share with key stakeholders. This is an excellent insight into what it’s really like to work as a data analyst!
6. Step six: Embrace your failures
The last ‘step’ in the data analytics process is to embrace your failures. The path we’ve described above is more of an iterative process than a one-way street. Data analytics is inherently messy, and the process you follow will be different for every project. For instance, while cleaning data, you might spot patterns that spark a whole new set of questions. This could send you back to step one (to redefine your objective). Equally, an exploratory analysis might highlight a set of data points you’d never considered using before. Or maybe you find that the results of your core analyses are misleading or erroneous. This might be caused by mistakes in the data, or human error earlier in the process.
While these pitfalls can feel like failures, don’t be disheartened if they happen. Data analysis is inherently chaotic, and mistakes occur. What’s important is to hone your ability to spot and rectify errors. If data analytics was straightforward, it might be easier, but it certainly wouldn’t be as interesting. Use the steps we’ve outlined as a framework, stay open-minded, and be creative. If you lose your way, you can refer back to the process to keep yourself on track.
In this post, we’ve covered the main steps of the data analytics process. These core steps can be amended, re-ordered and re-used as you deem fit, but they underpin every data analyst’s work:
- Define the question —What business problem are you trying to solve? Frame it as a question to help you focus on finding a clear answer.
- Collect data —Create a strategy for collecting data. Which data sources are most likely to help you solve your business problem?
- Clean the data —Explore, scrub, tidy, de-dupe, and structure your data as needed. Do whatever you have to! But don’t rush…take your time!
- Analyze the data —Carry out various analyses to obtain insights. Focus on the four types of data analysis: descriptive, diagnostic, predictive, and prescriptive.
- Share your results —How best can you share your insights and recommendations? A combination of visualization tools and communication is key.
- Embrace your mistakes —Mistakes happen. Learn from them. This is what transforms a good data analyst into a great one.
What next? From here, we strongly encourage you to explore the topic on your own. Get creative with the steps in the data analysis process, and see what tools you can find. As long as you stick to the core principles we’ve described, you can create a tailored technique that works for you.
To learn more, check out our free, 5-day data analytics short course . You might also be interested in the following:
- These are the top 9 data analytics tools
- 10 great places to find free datasets for your next project
- How to build a data analytics portfolio
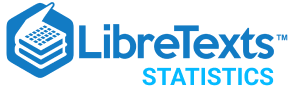
- school Campus Bookshelves
- menu_book Bookshelves
- perm_media Learning Objects
- login Login
- how_to_reg Request Instructor Account
- hub Instructor Commons
- Download Page (PDF)
- Download Full Book (PDF)
- Periodic Table
- Physics Constants
- Scientific Calculator
- Reference & Cite
- Tools expand_more
- Readability
selected template will load here
This action is not available.
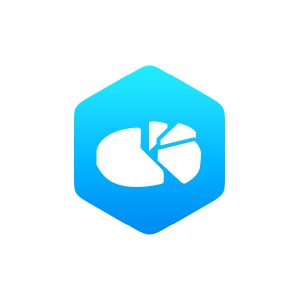
Unit 1: Exploratory Data Analysis
- Last updated
- Save as PDF
- Page ID 31260
CO-1: Describe the roles biostatistics serves in the discipline of public health.
CO-6: Apply basic concepts of probability, random variation, and commonly used statistical probability distributions.
Exploratory Data Analysis Introduction (2 videos, 7:04 total)
The Big Picture
Learning objectives.
LO 1.3: Identify and differentiate between the components of the Big Picture of Statistics
Recall “The Big Picture,” the four-step process that encompasses statistics (as it is presented in this course):
1. Producing Data — Choosing a sample from the population of interest and collecting data.
2. Exploratory Data Analysis (EDA) {Descriptive Statistics} — Summarizing the data we’ve collected.
3. and 4. Probability and Inference — Drawing conclusions about the entire population based on the data collected from the sample.
Even though in practice it is the second step in the process, we are going to look at Exploratory Data Analysis (EDA) first. (If you have forgotten why, review the course structure information at the end of the page on The Big Picture and in the video covering The Big Picture .)
Exploratory Data Analysis
LO 1.5: Explain the uses and important features of exploratory data analysis.
As you can tell from the examples of datasets we have seen, raw data are not very informative. Exploratory Data Analysis (EDA) is how we make sense of the data by converting them from their raw form to a more informative one.
In particular, EDA consists of:
- organizing and summarizing the raw data,
- discovering important features and patterns in the data and any striking deviations from those patterns, and then
- interpreting our findings in the context of the problem
And can be useful for:
- describing the distribution of a single variable (center, spread, shape, outliers)
- checking data (for errors or other problems)
- checking assumptions to more complex statistical analyses
- investigating relationships between variables
Exploratory data analysis (EDA) methods are often called Descriptive Statistics due to the fact that they simply describe, or provide estimates based on, the data at hand.
In Unit 4 we will cover methods of Inferential Statistics which use the results of a sample to make inferences about the population under study.
Comparisons can be visualized and values of interest estimated using EDA but descriptive statistics alone will provide no information about the certainty of our conclusions.
Important Features of Exploratory Data Analysis
There are two important features to the structure of the EDA unit in this course:
- The material in this unit covers two broad topics:
Examining Distributions — exploring data one variable at a time .
Examining Relationships — exploring data two variables at a time .
- In Exploratory Data Analysis, our exploration of data will always consist of the following two elements:
visual displays , supplemented by
numerical measures .
Try to remember these structural themes, as they will help you orient yourself along the path of this unit.
Examining Distributions
LO 6.1: Explain the meaning of the term distribution in statistics.
We will begin the EDA part of the course by exploring (or looking at) one variable at a time .
As we have seen, the data for each variable consist of a long list of values (whether numerical or not), and are not very informative in that form.
In order to convert these raw data into useful information, we need to summarize and then examine the distribution of the variable.
By distribution of a variable, we mean:
- what values the variable takes, and
- how often the variable takes those values.
We will first learn how to summarize and examine the distribution of a single categorical variable, and then do the same for a single quantitative variable.
- One Categorical Variable
- One Quantitative Variable: Introduction
- Role-Type Classification
- Summary (Unit 1)
DATA 275 Introduction to Data Analytics
- Getting Started with SPSS
- Variable View
- Option Suggestions
- SPSS Viewer
- Entering Data
- Cleaning & Checking Your SPSS Database
- Recoding Data: Collapsing Continuous Data
- Constructing Scales and Checking Their Reliability
- Formatting Tables in APA style
- Creating a syntax
- Public Data Sources
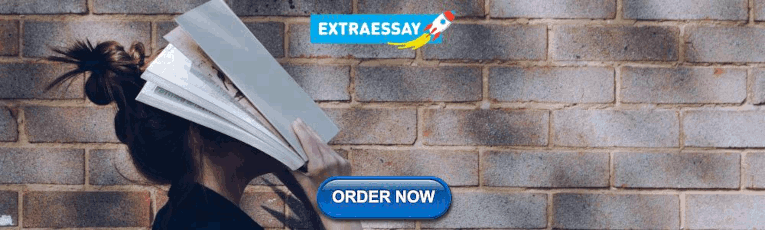
Data Analytics Project Assignment
- Literature Review This link opens in a new window
For your research project you will conduct data analysis and right a report summarizing your analysis and the findings from your analysis. You will accomplish this by completing a series of assignments.
Data 275 Research Project Assignment
In this week’s assignment, you are required accomplish the following tasks:
1. Propose a topic for you project
The topic you select for your capstone depends on your interest and the data problem you want to address. Try to pick a topic that you would enjoy researching and writing about.
Your topic selection will also be influenced by data availability. Because, this is a data analytics project, you will need to have access to data. If you have access to your organization’s data, you are free to use it. If you choose to do so, all information presented must be in secure form because Davenport University does not assume any responsibility for the security of corporate data. Otherwise, you can select a topic that is amenable to publicly available data.
Click the link for some useful suggestions: Project Proposal Suggestions
2. Find a data set of your interest and download it
There are many publicly available data sets that you can use for your project. The library has compiled a list of many possible sources of data. Click on the link below to explore these sources.
Public Data Sources
The data set you select must have:
At least 50 observations (50 rows) and at least 4 variables (columns) excluding identification variables At least one dependent variable
You must provide:
A proper citation of the data source using APA style format A discussion on how the data was collected and by whom The number of variables in the data set The number of observations/subjects in the data set A description of each variable together with an explanation of how it is measured (e.g. the unit of measurement).
Deliverable
A minimum of one page description of your data analytics project which must include the following:
A title for your project A brief description of the project Major stakeholders who would use the information that would be generated from your analysis and how they would use/benefit from that information A description of the dataset you will use for your project
- << Previous: Public Data Sources
- Next: Literature Review >>
- Last Updated: Mar 15, 2024 10:33 AM
- URL: https://davenport.libguides.com/data275
- Data Analysis Tutorial
- Python-Data visualization tutorial
- Machine Learning Tutorial
- Machine Learning Projects
- Machine Learning Interview Questions
- Machine Learning Mathematics
- Deep Learning Tutorial
- Deep Learning Project
- Deep Learning Interview Questions
- Computer Vision Tutorial
- Computer Vision Projects
- NLP Project
- NLP Interview Questions
- Statistics with Python
- S&P 500 Companies Data Analysis Tutorial using R
- TensorFlow Tutorial
- Python for Data Science - Learn the Uses of Python in Data Science
- What is Data Analytics?
- Data Analysis and Visualization with Jupyter Notebook
- Advanced Audio Processing and Recognition with Transformer
- Artificial Intelligence (AI) Algorithms
- What is H - Index?
- Reasoning Ability For IBPS Clerk - Prelims & Mains
- Data Archiving Strategy
- What is SEO Optimization or Search Engine Optimization?
- List of File Formats with Types and Extensions
- Learn Product Management | Beginner to Advanced Tutorial
- Indian Politics - General Knowledge Questions and Answers
- Data Analyst Roadmap - A Complete Guide
- Web Browser - A Complete Overview
30+ Top Data Analytics Projects in 2024 [With Source Codes]
Are you an aspiring data analyst? Dive into 40+ FREE Data Analytics Projects packed with the hottest 2024 tech. Data Analytics Projects for beginners , final-year students , and experienced professionals to Master essential data analytical skills.
These top data analytics projects serve as a simple yet powerful gateway for beginners. Learn with free source code , mastering the art of data analytics. Make informed choices, reduce costs, and innovate for business success.
Building these data analytics projects helps you incorporate your theoretical knowledge with practical applications. These are the best data analytics projects for resumes , as they focus on real-world problems.
Let’s understand the need to build data analytics projects, and how they can help you in building your career.
Why Build Data Analytics Projects?
There are many applications of data analytics, and building data analytics projects helps you learn these applications and build a strong fundamental understanding of the subject.
Apart from adding value to your resume, data science projects also help you in building skills and solve real-world problems. Some benefits of building data analytics projects:
- Smart Decisions: Data analytics helps you make smart choices by turning data into actionable insights.
- Identify Trends: It gives you an edge by spotting trends and opportunities before others.
- Cost Analysis: Identifies areas to cut costs and make operations more efficient.
- Customer Insights: Reveals customer habits and preferences for better service and loyalty.
- Business Growth: Pinpoints where and how your business can grow successfully.
- Risk Management: Helps in foreseeing and managing potential risks effectively.
- Performance Tracking: Keeps you updated on how well your business is doing in real time.
- Personalized Marketing: Allows tailored marketing for better customer engagement.
- Work Efficiency: Streamlines processes for overall operational efficiency.
- Innovation: Fosters a culture of innovation through data-driven insights.
Big Data Analytics Projects with Source Codes
We have shortlisted some of the big data analytics Projects and categorized them into 3 categories. You can choose a single category to build projects or multiple categories to diversify your knowledge of data analytics.
We have provided multiple data analytics projects in each category. Combined there are over 30 projects to choose from.
Let’s look at these categories below, and the fun projects in them.
Data Analytics Project Categories
WebScraping Data Analytics Projects
Data analysis and visualization projects.
- Time Series Data Analysis Projects
Explore these top web scraping projects with source code.
- Movies Review Scraping And Analysis
- Product Price Scraping and Analysis
- News Scraping and Analysis
- Real-time Share Price scrapping and analysis
Here are the top Data Analysis and Visualization projects with source code.
- Zomato Data Analysis Using Python
- IPL Data Analysis
- Airbnb Data Analysis
- Global Covid-19 Data Analysis and Visualizations
- Housing Price Analysis & Predictions
- Market Basket Analysis
- Titanic Dataset Analysis and Survival Predictions
- Iris Flower Dataset Analysis and Predictions
- Customer Churn Analysis
- Car Price Prediction Analysis
- Indian Election Data Analysis
- HR Analytics to Track Employee Performance
- Product Recommendation Analysis
- Credit Card Approvals Analysis & Predictions
- Uber Trips Data Analysis
- iPhone Sales Analysis
- Google Search Analysis
Time Series Data Analytics Projects
Here are the top 10 Data Analytics Projects with source code based on Time Series
- Time Series Analysis with Stock Price Data
- Weather Data Analysis
- Time Series Analysis with Cryptocurrency Data
- Climate Change Data Analysis
- Anomaly Detection in Time Series Data
- Predictive Modeling for Sales or Demand Forecasting
- Air Quality Data Analysis and Dynamic Visualizations
- Gold Price Analysis and Forcasting Over Time
- Food Price Forecasting
- Time wise Unemployement Data Analysis
Now that you’ve decided on the project that you will be building, let’s look at some platforms that will help you in building projects.
Best Platforms to Build Data Analyst Projects
Here are some best platforms for making data analysis projects:
- Microsoft Excel : Widely used for data manipulation and analysis, particularly suitable for beginners.
- Python ( Pandas and NumPy ): A versatile coding environment for advanced analytics and machine learning.
- RStudio : Ideal for statistical analysis, offering a comprehensive platform for data exploration.
- Tableau : Renowned for its data visualization capabilities, making complex datasets more accessible.
- Jupyter Notebooks : An interactive and collaborative environment, facilitating code execution and documentation.
- Google Colab : A cloud-based solution offering scalable computing resources for efficient data processing.
- Microsoft Azure : Another cloud-based option providing extensive computing power, especially beneficial for handling large datasets.
Choose a platform based on your project’s specific needs, your familiarity with the tools, and the desired level of collaboration and visualization.
Also Explore:
Data Analyst Salary In India 2024 Data Scientist Salary in India 2024 Business Analyst Salary in India 2024: Fresher & Experienced
In conclusion, our collection of top data analytics projects offers a hands-on journey for beginners and experienced individuals into the realm of data analytics. With free source code on project problems, you can learn to master data analytics and begin your journey to be a data analyst.
These projects cover a variety of areas, from web scraping to predictive modeling, enabling you to understand and implement data analytics straightforwardly. Elevate your skills, dive into these projects, and unlock the potential of data analytics to drive your career forward.
Data Analytics Projects – FAQs
What is a data analytics project.
A data analytics project involves analyzing data to extract insights for informed decision-making, often addressing specific business challenges or questions.
What are the types of data analytics?
There are 4 Types of Data Analytics: Descriptive: Summarizes past data. Diagnostic : Examines why past events occurred. Predictive : Forecasts future trends. Prescriptive : Recommends actions based on analysis.
How do you build a data analytics project?
To build a data analytics project, you need to: Understand Problem Gather data Preprocess and clean data Analyze data Conclude findings
How do you present a data analytics project?
Share findings through clear visuals, like charts or graphs. Explain insights in simple language, emphasizing key takeaways for easy understanding.
Please Login to comment...
Similar reads.
- Data Analytics
- Data Analysis
- Data Science
- Google Releases ‘Prompting Guide’ With Tips For Gemini In Workspace
- Google Cloud Next 24 | Gmail Voice Input, Gemini for Google Chat, Meet ‘Translate for me,’ & More
- 10 Best Viber Alternatives for Better Communication
- 12 Best Database Management Software in 2024
- 30 OOPs Interview Questions and Answers (2024)
Improve your Coding Skills with Practice
What kind of Experience do you want to share?
Homework 8: Final project: Design your own data analysis
So far, you have performed data analysis on a variety of data sources, to solve realistic problems from science, engineering, and business. Now it's your turn to choose and analyze a problem. This is good practice for how you will use Python in the remainder of your career.
There are two parts to this assignment, due separately. Part I is due on Thursday, August 9. Part II is due on Thursday, August 16.
For this assignment, you are permitted to work with a partner ; the two of you will submit just one solution. You are not required to work with a partner, and groups may not be larger than two people. Only one of you will submit the assignment — do not submit duplicates. If you work with a partner, we will expect your project to be twice as substantial as a project done individually.
Part I: Propose an analysis and locate data
Propose a data analysis project to the course staff. This can be almost anything that you choose. You might select a project from your field of study, from your extracurricular interests, from open government or public policy, or from elsewhere. We are just looking for you to show that you have absorbed the lessons of CSE 190p. Impress us!
Think of your proposal as a pitch to a venture capitalist, a foundation, or a scientific review panel. Your data analysis proposal must clearly state the problem, in the form of one or more questions that you will seek to answer. It must explain your algorithm or other analysis, and you must have already located a pre-existing dataset that your code will analyze.
More specifically, you should turn in a draft report, in the format required in Part II of this assignment. Your report should include all of the parts required in the final report, except for the results in part 1, part 5 (results), and part 6 (reproducing results).
Your proposal will probably be about 2 pages long. Submit your proposal in PDF (not in plain text nor in proprietary binary formats like Microsoft Word or Rich Text Format).
The course staff will review your proposal and will either approve it or will require you to make changes. They will base their assessment on:
- whether your proposal conforms to the above requirements, and
- scoping (we don't want you to proceed with a project that is too hard nor one that is too easy).
Hints about datasets: A good approach is to start with a problem of interest and then look for data. Alternately, you can start with a dataset. Here are some possible data sources, but many more exist:
- Baron Schwartz's list of datasets . Some of these are themselves rich lists of datasets, such as the Amazon AWS public data sets
- Data.gov , U.S. open government data data.wa.gov , Washington state open government data
- SQLShare : public scientific datasets. Some require considerable knowledge to interpret, others are easier to understand. You can select "All datasets" and then filter by keyword, or you can select a tag from among those in the left column.
Part II: Perform the analysis and report the results
Implement your analysis, process your data, and interpret the results. Then, submit a report that describes the results and conclusions of your analysis. It might include graphs, tables of numbers, or just a few key computations. Remember that plots and other visual representations of data are very useful in conveying your conclusions.
Submit your report in PDF (not in plain text nor in proprietary binary formats like Microsoft Word or Rich Text Format). Your report will probably be about 4-6 pages of text long, but there are no fixed upper or lower bounds on its size. You should write at an appropriate length: neither so briefly that you omit information, nor so verbosely that you pad your report or bury the important information under irrelevant details.
Your report should contain at least the following parts. You are permitted to write additional sections as well.
- Title and author(s). The title should be descriptive of your research questions. It should not be something like "CSE 190p homework 8" (though you could use that as a subtitle if you wish).
- Overview of research questions and results. In 1-3 sentences, state each research question — that is, each problem you investigated. What are you trying to compute, and why? After each research question, clearly state the answer you determined. You don't have to give details or justifications yet — just the answer.
- Motivation and background. State, explain, and motivate the problem. In other words, explain the context and why the problem matters. This expands on the research questions that you already stated. Why are they worth computing? What difference would knowing the answers make? We require a problem with some kind of real-world motivation.
- Dataset. Describe the real, pre-existing dataset that you used, including exact URLs. You may not use a dataset that has been used in a previous CSE 190p assignment or demo. You do not have to turn in the dataset itself (it may be quite large, or it might be available only via the web rather than as a single download). If the dataset needs to be downloaded, then ideally, when your program is run, it should automatically check whether the dataset has already been downloaded &mdash if not, your program should download it before doing any additional work. If that is not the case, then your report must include clear, unambiguous instructions that anyone can follow to download it themselves.
- Methodology (algorithm or analysis). A complete, clear, unambiguous English description of the analysis you performed. This should be sufficient for someone else to reproduce your results, even without access to your source code, and without having to guess nor to make significant design choices. This description is also likely to be helpful to people who read your code.
- Results. Present and discuss your research results. Focus in particular on the parts that are most interesting, surprising, or important. Discuss the consequences or implications.
- Reproducing your results. Give clear and explicit instructions for obtaining the data and for running the analysis, and for interpreting the results or finding the interesting parts in the output. It must be possible for the course staff to run the code, without any additional data entry or interaction, to re-create every number or figure that appears in your report (and also any other results that support your argument but that you did not include in your report).
- Collaboration and reflection. What did you learn from this assignment? What do you wish you had known before you started? What would you do differently? What advice would you offer to future students embarking on this project? Also, state which students or other people (besides the course staff) helped you with the assignment, or that no one did. State how many hours you spent on Part I and Part II of the assignment. Also state what you or the staff could have done to help you with the assignment.
Also, submit your commented source code. Your source code should be clear enough for another programmer, such as the course staff, to understand and modify if needed. Your source code documentation may assume that the programmer has already read your report.
Submit your work
Submit your files via Catalyst CollectIt (a.k.a. Dropbox) .
In-class presentation
You will present your work to the rest of the class on Friday, August 17.
It is recommended that you use slides (which you can prepare using PowerPoint, KeyNote, Impress, etc.). Your presentation will be strictly limited to 5 minutes. It is strongly recommended that you practice your presentation ahead of time. You will only have time to present the most important results from your project. Be sure to clearly state the research questions and your answers to them.
- Study Guides
- Homework Questions
Assignment #1 Descriptive Statistics Data Analysis Plan Template REVIEW
Data Analysis
Data analysis (DA) is the science of examining raw data with the purpose of drawing conclusions about that information. It refers to qualitative and quantitative techniques and processes used to enhance productivity and business gain. Data analysis is distinguished from data mining by the scope, purpose and focus of the analytics. This is also focuses on inference, the process of deriving a conclusion based solely on what is already known by the researcher.
Authorization
Know about database management, benefits of the wireless microphone, classification of servers, application monitoring tool, long-range and ultra-secure communication using a nanoantenna, bringing life to the stars by interstellar travel, inferior good, after a bombing steam review, war thunder adjusts its economic policy, researchers build “time machine” simulations to examine the lifecycle of galaxy “cities” in the ancestors, latest post, mid-ocean ridge (mor), harnessing hydrogen at the genesis of life, ngc 5728’s faint characteristics are exposed, astronomers discover the oldest black hole ever observed, atomic hydrogen welding, variable-frequency transformer (vft).
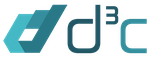
- Advancing the science of adaptive interventions
- Advancing mobile health interventions
- Integrating human-delivered and digital interventions
- Solving implementation challenges
- Intervention Designs
- Trial Designs
- Software Tools
- Training Programs
- Online Courses
- Publications
- CATIE Training for Education Researchers
- Pilot Grant Program
- Postdoctoral Fellowships
Data Analysis for Hybrid Experimental Designs
Home / Code Library
About this code
On this page, we provide example datasets, analysis code in SAS and R, and outputs, for the three kinds of hybrid experimental designs considered in “ Design of Experiments with Sequential Randomizations at Multiple Time Scales: The Hybrid Experimental Design .”
The specific hybrids considered combine: a classic factorial experiment with a sequential multiple assignment randomized trial (SMART), a classic factorial experiment with a micro-randomized trial (MRT), a SMART with an MRT.
How can a behavioral scientist use this code?
A behavioral scientist can use this code to learn how to analyze data for three different types of Hybrid Experimental Designs (HED). They may then repurpose the code to analyze data from their own hybrid design.
Related References
Nahum-Shani, I., Dziak, J. J., Venera, H., Spring, B., & Dempsey W. (2023). Design of Experiments with Sequential Randomizations at Multiple Time Scales: The Hybrid Experimental Design. Behavior Research Methods, doi:10.3758/s13428-023-02119-z.
April 12, 2024, 3 p.m.
Jillian C. Strayhorn
d3center Seminar Series
April 17, 2024, 1 p.m. EDT
Hybrid experimental designs for optimizing digital interventions that adapt on multiple timescales
cadio Webinar Series
April 18-19, 2024
P50 Annual Meetings
April 19, 2024, 3 p.m.
April 30, 2024
Hybrid Experimental Designs
Center for Healthy Minds, UW-Madison
May 3rd, 2024, 3 p.m.
Timothy Lycurgus
May 6, 2024, 11 a.m. EDT
Experimental Designs for Adapting Digital Interventions
mHealth Training Institute 2024
June 3-5, 2024, Day & Time TBA
Missing Data in Micro-Randomized Trials: Challenges and Opportunities
Society for Ambulatory Assessment 2024
June 15, 2024
Innovations in Digital Interventions for Substance Use
College on Problems of Drug Dependence Conference
Join the d3center Mailing List
Keep up to date with the latest news, events, software releases, learning modules, and resources from the d3center.
© 2024 • d3center • Institute for Social Research • University of Michigan
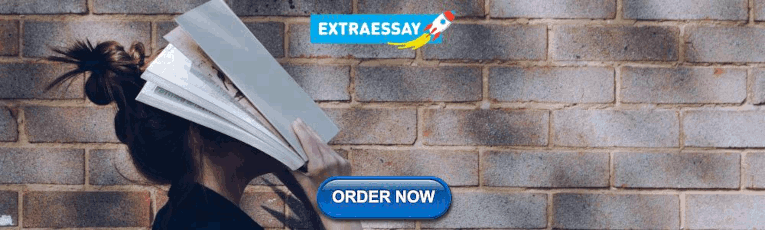
IMAGES
VIDEO
COMMENTS
Assignment 2: Exploratory Data Analysis. In this assignment, you will identify a dataset of interest and perform an exploratory analysis to better understand the shape & structure of the data, investigate initial questions, and develop preliminary insights & hypotheses. Your final submission will take the form of a report consisting of ...
3. Exploratory data analysis (EDA) Data analysis is all about answering questions with data. Exploratory data analysis, or EDA for short, helps you explore what questions to ask. This could be done separately from or in conjunction with data cleaning. Either way, you'll want to accomplish the following during these early investigations.
Call this method percent_change_bachelors_2000s and return the difference (the percent in 2010 minus the percent in 2000) as a float. For example, assuming we have parsed hw3-nces-ed-attainment.csv and stored it in a variable called data, then the call percent_change_bachelors_2000s(data) will return 2.599999999999998.
1. Step one: Defining the question. The first step in any data analysis process is to define your objective. In data analytics jargon, this is sometimes called the 'problem statement'. Defining your objective means coming up with a hypothesis and figuring how to test it.
This course presents you with a gentle introduction to Data Analysis, the role of a Data Analyst, and the tools used in this job. You will learn about the skills and responsibilities of a data analyst and hear from several data experts sharing their tips & advice to start a career. This course will help you to differentiate between the roles of ...
Written by Coursera Staff • Updated on Apr 1, 2024. Data analysis is the practice of working with data to glean useful information, which can then be used to make informed decisions. "It is a capital mistake to theorize before one has data. Insensibly one begins to twist facts to suit theories, instead of theories to suit facts," Sherlock ...
3.c BOX PLOT: Box plot is an alternative and more robust way to illustrate a continuous variable. The vertical lines in the box plot have a specific meaning. The centerline in the box is the 50th percentile of the data (median). Variability is represented by a box that is formed by marking the first and third quartile.
Exploratory data analysis (EDA) methods are often called Descriptive Statistics due to the fact that they simply describe, or provide estimates based on, the data at hand.. In Unit 4 we will cover methods of Inferential Statistics which use the results of a sample to make inferences about the population under study.. Comparisons can be visualized and values of interest estimated using EDA but ...
For your research project you will conduct data analysis and right a report summarizing your analysis and the findings from your analysis. You will accomplish this by completing a series of assignments. Data 275 Research Project Assignment. In this week's assignment, you are required accomplish the following tasks: 1. Propose a topic for you ...
Here are the top Data Analysis and Visualization projects with source code. Zomato Data Analysis Using Python. IPL Data Analysis. Airbnb Data Analysis. Global Covid-19 Data Analysis and Visualizations. Housing Price Analysis & Predictions. Market Basket Analysis. Titanic Dataset Analysis and Survival Predictions.
For this assignment, you are permitted to work with a partner; the two of you will submit just one solution. You are not required to work with a partner, and groups may not be larger than two people. ... Your data analysis proposal must clearly state the problem, in the form of one or more questions that you will seek to answer. It must explain ...
There are 5 modules in this course. Spreadsheet tools like Excel are an essential tool for working with data - whether for data analytics, business, marketing, or research. This course is designed to give you a basic working knowledge of Excel and how to use it for analyzing data. This course is suitable for those who are interested in pursuing ...
For this Mock Data Analysis Assignment, you'll analyze and visualize interview and quantitative survey data. Use the resources from Sessions 1-4 to support you with this assignment. Analyzing Interview Data Before you Start. Download this data set: MSSE Coaching Data and the End of Coaching Survey; Watch this video for a step-by-step approach ...
Assignment 2, FYC 6800 - Page 1 . Assignment 2: Sampling & Data Analysis (Group Assignment) Objectives: After completing this assignment, you will be able to • Explain the sampling and data analysis procedures used in research reports • Determine whether the researcher wants to generalize his/her specific findingsand/or
University of Maryland University College STAT200 - Assignment #1: Descriptive Statistics Data Analysis Plan Identifying Information Student (Full Name): Class: STATS 200 3150 INTRODUCTION TO STATISTIC Instructor: Date: November 3, 2019 Scenario: For this assignment, I will analyze the difference between the expenses of food and other expenses in single households vs. married households.
Data Analysis and Application Cristina Harrison Capella University. Analyzing Correlations Data Analysis Plan The four variables used for data analysis are Quiz 1, grade point average (GPA), Total, and Final. The data provided for this assessment were given through an SPSS data sample file through grades per the guidelines of this assignment.
Data Analysis Assignment # This is a continuation of Data Analysis Assignment #3. You can use the same data you used in Assignment #3 or you can choose 2 new columns of data. What I would like you to do in this next step is to create a linear regression model through the data.
Data analysis (DA) is the science of examining raw data with the purpose of drawing conclusions about that information. It refers to qualitative and quantitative techniques and processes used to enhance productivity and business gain. Data analysis is distinguished from data mining by the scope, purpose and focus of the analytics. This is also ...
This module will test your knowledge and the skills you've acquired so far. This module contains the graded final examination covering content from three courses: Introduction to Data Analytics, Excel Basics for Data Analysis, and Data Visualization and Dashboards with Excel and Cognos. What's included. 3 readings 1 assignment 3 plugins.
150/150 assignment correlations between multiple variables in course paige kusel capella university correlations between multiple variables in course the ... college year, division, course section, gpa, quiz scores, final scores, overall class grades, and many more. Data Analysis Plan I will be taking a deeper look into four specific variables ...
The published Guidelines on Trademark Assignment Procedures aim to help assignees follow the process of trademark registration. It is crucial for rights holders to understand these guidelines to facilitate smooth and cost-effective assignment. ... Data Analysis Data Hub Tools; Panoramic Rankings WTR 1000; Introduction ...
About this code. On this page, we provide example datasets, analysis code in SAS and R, and outputs, for the three kinds of hybrid experimental designs considered in " Design of Experiments with Sequential Randomizations at Multiple Time Scales: The Hybrid Experimental Design." The specific hybrids considered combine: a classic factorial experiment with a sequential multiple assignment ...