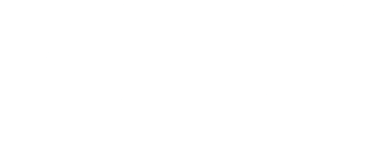
- Center on Health Equity and Access
- Health Care Cost
- Health Care Delivery
- Value-Based Care
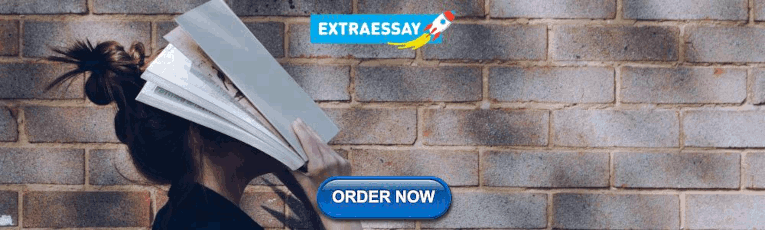
Cost-effectiveness of Case Management: A Systematic Review
- Felix Freigang, MA
- Matthias Arnold, Dr Oec Publ
This systematic review found that studies of case management interventions have adequate quality and, in many cases, show cost-effective or even cost-saving results.
Objectives: In this time of aging and increasingly multimorbid populations, effective and efficient case management approaches play a crucial role in supporting patients who are navigating complex health care systems. Until now, no rigorous systematic review has synthesized studies about the cost-effectiveness of case management.
Study Design: A systematic review was performed.
Methods: The bibliographic databases PubMed and CINAHL Plus were systematically searched using key blocks and synonyms of the terms case management , effectiveness , and costs . The methodological quality of the studies was assessed using the Consensus Health Economic Criteria list.
Results: A total of 29 studies were included. In 3 studies, the intervention was less effective and more costly than the control group and can therefore be considered not cost-effective. Two studies found that the intervention was less effective and less costly. A more effective and less costly intervention, and therefore a strong recommendation for case management, was found in 6 studies. In 17 studies, the intervention was more effective while being more costly. Nearly half of the studies met most of the quality criteria, with 16 or more points out of 19.
Conclusions: Existing studies often have adequate quality and, in many cases, show cost-effective or even cost-saving results. Case management appears to be a promising method to support patients facing complex care situations. However, variation among case management approaches is very high, and the topic needs further study to determine the most cost-effective way of providing such care coordination.
Am J Manag Care. 2022;28(7):e271-e279. https://doi.org/10.37765/ajmc.2022.89186
Takeaway Points
- Case management approaches play a crucial role in supporting patients who are navigating complex health care systems.
- Case management intervention studies often have adequate quality and, in many cases, show cost-effective or even cost-saving results.
- Variation among case management approaches is very high, and the topic needs further study to determine the most cost-effective way of providing such care coordination.
Health systems around the world are getting more complex. This increasing complexity may affect patients’ ability to access the right health services at the right time. This struggle to navigate the system has individual implications for the care seeker’s well-being and economic implications when it results in wasting the health system’s scarce resources and delaying the provision of the right treatment to the right patient or providing unnecessary care. Case management programs intend to guide individuals with complex medical needs through the health system to improve health service effectiveness and the efficiency of service provision. The concept of case management is not new; it has been practiced in the United States for more than a century, primarily in the disciplines of nursing and social services. 1 Case management programs are generally designed to tackle the challenges of episodic care, which are often fraught with inadequate transitions between care services and health care settings. The programs aim to coordinate fragmented services by providing guidance to individuals, attempting to improve health service effectiveness and reduce cost. Ideally, a case management program facilitates communication and the coordination of care, and its collaborative practice includes patients, caregivers, nurses, social workers, physicians, payers, support staff, other practitioners, and the community. 2
The oldest and largest case management membership organization in the world, the Case Management Society of America, which facilitates the growth and development of case management, defines case management as “a collaborative process of assessment, planning, facilitation, care coordination, evaluation, and advocacy for options and services to meet an individual’s and family’s comprehensive health needs through communication and available resources to promote patient safety, quality of care, and cost-effective outcomes.” 3 As defined by the UK-based Medical Research Council as well, case management is quite complex. 4 The complexity of case management interventions arises from, among other factors, the number of groups or organizational levels targeted by the intervention, the number and variability of outcomes, the number and difficulty of behaviors required by those delivering or receiving the intervention, and the degree of flexibility or tailoring of the intervention. Furthermore, there is complexity in the intervention components, among them case finding and assessment, case planning, navigation and coordination, monitoring, and reviewing of the case plan. These components aim to improve continuity of care and to enhance patients’ self-management skills and hence are intended to increase efficiency within the health care system.
Especially in regard to the aging multimorbid population, case management may play an important role in the support of patients facing complex care situations. With better coordination, it is posited, the health system’s ability to provide high-quality care and maintain resource requirements can improve. One recent analysis of case management’s effectiveness is the RubiN project (funded by the Federal Joint Committee’s German Innovations Fund), which is evaluating the implementation of case management for geriatric patients. The goal of RubiN is to develop a form of care throughout Germany that enables older people to remain in their homes for as long as possible. It is hoped that by case managers informing and guiding patients and their (caretaking) relatives, the quality of treatment will rise—by closing gaps in care—and support will be provided to physicians—by conserving scarce personnel resources.
Here, we set out to provide an overview of the evidence regarding cost-effectiveness of case management; until now, no systematic review has been conducted on this topic. Yet systematic reviews that have been done on case management’s overall effectiveness are promising: They have found that case management can effectively reduce hospital use and improve satisfaction with care when chronic illnesses are present. 5-7 Furthermore, a systematic review of reviews has found evidence that case management interventions reduce health care utilization in patients with chronic illnesses. 8
However, the question of whether case management is cost- effective has so far not been adequately addressed. Further, it is unclear whether cost-effective case management interventions have certain characteristics in common. The aim of this systematic review is therefore to investigate the cost-effectiveness of case management.
Objectives and Study Design
The objective of this systematic review was to synthesize the evidence for cost-effectiveness of case management.We conducted a systematic review of the literature following the Preferred Reporting Items for Systematic reviews and Meta-Analyses (PRISMA) guidelines. 9 Also, this review reported according to the PICOS (Population, Intervention, Comparison, Outcomes, Setting) Framework. 10 A protocol was developed before searching electronic databases.
Eligibility Criteria
Inclusion and exclusion criteria are outlined in Table 1 . Briefly, the review included cost-effectiveness studies that compare case management interventions with usual care. Model-based studies were excluded. No limits were applied to language and publication date.
Electronic Bibliographic Database Searches
The bibliographic databases PubMed and CINAHL Plus were systematically searched using key blocks of the terms case management , effectiveness , and costs and their synonyms. A complete search strategy list is provided in the eAppendix ( available at ajmc.com ).
Study Selection
Two authors (A.K.K. and J.J.) independently screened titles and abstracts from unduplicated references. The full text was reviewed when a decision was not possible from reading the abstract. Any discrepancies were resolved by discussion.
Data Collection and Synthesis
Data were collected using an extraction form developed to retrieve relevant information. This included study characteristics (nation, setting, patient group and sample size, comparison group, study design, type of economic evaluation, study duration), case management characteristics (case management model [with description], intensity of intervention, team or single case manager, training received, supervision, 24-hour availability of case manager, caseload per manager/team), and outcome characteristics (outcome measures, costs included, cost perspective, time horizon, cost analysis method, findings, sensitivity analysis/uncertainty assessment). The studies were summarized and synthesized by the first author independently. The extraction table is provided in the eAppendix.
Quality Assessment
The methodological quality of the cost-effectiveness analyses was assessed by the Consensus Health Economic Criteria (CHEC) list. 11 If a study qualified in a criterion, it scored 1; otherwise, it scored 0. Thus, this tool’s range was 0 to 19. In cases in which criteria were not applicable (eg, the question about the appropriate discount rate in a year-long study), the overall achievable score was reduced. Quality appraisal was verified by a second reviewer.
A total of 2388 unduplicated studies were retrieved from the database searches. After reading titles and abstracts, 61 full texts were analyzed, and inclusion and exclusion criteria were applied. From these, 32 studies were excluded. The remaining 29 studies were included in the qualitative analysis of the review. A flow diagram of this process, according to PRISMA guidelines, is presented in Figure 1 . 9
The results of the CHEC list show that nearly half of the studies (n = 13) met most of the quality criteria (≥ 16 of 19). 12-24 The main limitations were the narrow perspective chosen, as only about a quarter (n = 7) of all studies chose a broad societal perspective, 12,16,17,20,23,25,26 and the chosen short time horizon, which was only 1 year in about half the studies (n = 14). 13,16,19,26-36
Study Characteristics
Studies were from the United States (n = 12) 13-16,18,28,29,34,35,37-39 more than from any other nation, followed by studies from Germany (n = 8), 12,20,21,24,26,30,31,33 the Netherlands (n = 4), 17,19,22,23 the United Kingdom (n = 2), 32,40 Sweden (n = 1), 25 Denmark (n = 1), 36 and Canada (n = 1). 27 Except for one, 33 all studies were trial-based economic evaluations, assessing the cost-effectiveness of case management compared with usual care. Twenty-two of the economic evaluations were based on randomized controlled trials (RCTs) 12-16,18,20-30,32,34,36,39,40 ; the rest used non-RCT designs, such as nonrandomized controlled observational studies. Twenty of the studies adopted a health care system perspective in the analysis. 13-15,19,21,24,27-40 A societal perspective was adopted by 7 studies. 12,16,17,20,23,25,26 One study took the employers’ perspective. 18 One study adopted a health care perspective, a social care perspective, and a societal perspective. 22
Patient Groups
The patient group represented more than any other (see Table 2 12-40 ) were those with psychiatric disorders (n = 9), such as depressive disorders, anxiety, and/or posttraumatic stress disorder 12,15,16,18,22,30,31,35,39 ; they were followed by older patients (n = 4), 19,25,29,38 patients with dementia (n = 3), 17,24,33 and patients with diabetes (n = 2). 13,37 Further, several studies included patients belonging to more than 1 patient group, such as patients with diabetes and depression, 14 older patients with depression, 32,40 and older patients with myocardial infarction. 20,26 The rest of the studies included patients with HIV, 23 chronic obstructive pulmonary disease, 27,36 elevated blood pressure, 28 hypercholesterolemia, 34 and a long-term indication for oral anticoagulation therapy. 21
Case Management Model
In most studies, the case management interventions were described in enough detail to identify the program components. These components are case finding and assessment, case planning, navigation and coordination, monitoring, and reviewing of the case plan (Table 2 12-40 ).
The component of monitoring could be found in most descriptions of the case management intervention: Symptom monitoring and regular visits or telephone calls were described in 24 studies. Furthermore, the case management models often included navigation and coordination (n = 19) and health education (n = 17) components, such as informing the patient about the disease, counseling on general health behavior, emphasizing lifestyle changes, and promoting treatment adherence, self-care, and autonomy.
A combination of the components of monitoring and health education was often described, 13,15,21,23,27 as was the combination of monitoring and navigation/coordination. 14,32,37,39,40
A case management model with all components (assessment, case planning, navigation and coordination, monitoring, and health education) was described in 5 studies. 22,25,28,29,36
Case Managers
Case managers were nurses, health care assistants, social workers, physiotherapists, clinical therapists, pharmacists, and mental health workers. About half the studies (n = 14) stated that the case managers received training beforehand. The scope of the training received was heterogenous, with a duration of several hours, 2 days, or even 2 weeks. Case managers worked alone, although they frequently collaborated closely with the patient’s physician. Caseloads ranged between 10 and 76 patients, although 1 study analyzing a telecommunication-supported case management model stated a caseload of up to 120 less-active cases. 35
Outcomes and Costs
Highly heterogeneous among the studies were the outcomes. They included patient utility measures (eg, quality of life with EuroQol 5-dimension instrument, Short Form-36 questionnaire, World Health Organization Quality of Life), patient health effect measures (eg, mortality, symptoms, functioning in activities of daily living), other patient-relevant measures or system measures (eg, outpatient contacts, time in patients’ home environment, absenteeism), and situational program measures (eg, quality of parenting, abstinence).
Depending on the perspective chosen, intervention costs, direct medical costs (eg, inpatient and outpatient costs, emergency department costs, medication costs), direct nonmedical costs (costs for social support services [eg, community care such as nurse care and family support]), and indirect costs (eg, informal care costs and productivity losses) were included in the analyses of the studies. A table of perspectives chosen and costs included is provided in the eAppendix.
Economic Analyses
Findings regarding the economic analyses, the classification within the cost-effectiveness plane, and the results of the quality assessment using the CHEC list are listed in the results grid ( Table 3 [ part A and part B ] 12-40 ).
All except 2 studies 20,25 included an incremental analysis of costs and outcomes; most calculated an incremental cost-effectiveness ratio (n = 24) and conducted a sensitivity analysis (n = 24).
In Figure 2 , results are visualized in a cost-effectiveness plane, which is used to visually represent the differences in costs and health outcomes (effects) between treatment alternatives in 2 dimensions by plotting the costs against effects on a graph. Effects and costs are plotted on the x-axis and y-axis, respectively. The cost-effectiveness plane includes 4 quadrants: northwest (NW), southwest (SW), northeast (NE), and southeast (SE).
In 3 studies, the intervention was less effective and more costly than the control group (NW quadrant) and can therefore be considered not cost-effective. 19,30,35 The intervention is dominated by usual care.
Two studies found that the intervention was less effective and less costly (SW quadrant). One of these studies found that both costs (–€17.61) and effects (–0.0163 quality-adjusted life-years [QALYs]) were lower in the intervention group; therefore, the incremental cost-effectiveness ratio (€1080/QALY) represents the savings per additional QALY lost. 26 A study from the Netherlands, 17 which analyzed the cost-effectiveness of case management for patients with diagnosed dementia and their informal caregivers, found that the intervention saves costs and there is an approximately 45% chance that the intervention also has positive effects.
A more effective and less costly intervention (SE quadrant), and therefore evidence for cost-effectiveness, was provided in 6 studies. 12,20,24,27-29
The majority of studies (n = 18) found that the intervention was more effective while being more costly (NE quadrant). Of these, 7 studies reported incremental cost-effectiveness ratios below a willingness-to-pay threshold of US$50,000 for the gain of 1 QALY. 14,16,21,23,32,36,40 Only 1 study used QALYs and found that case management is not cost effective at US$50,000. 13 The remaining studies either used different outcome measures or did not provide a recommendation.
Case management interventions across all studies varied considerably. In cost-effective case management interventions, no patterns of common characteristics, such as case management model, type of case manager, or patient group, could be identified. No correlation of cost-effectiveness with a certain kind of health care system, study design, or time horizon could be observed either. Therefore, it remains unclear what makes some case management interventions cost-effective.
To our knowledge, this is the first systematic review that systematically synthesized studies to identify the cost-effectiveness of case management interventions. We identified 29 studies, which were published between 2000 and 2019. All studies compared case management to usual care without case management.
The results of the quality assessment of economic evaluations show that the quality of the included studies is good, although most studies chose a payer’s perspective and therefore did not include indirect costs such as productivity losses. In addition, in about half of all studies, the chosen time horizon was only 1 year. This is a short observation period, not appropriate to capture all relevant outcomes, because case management effects might be visible only after longer periods of time. In addition, considering that at the beginning of an intervention, costs of case management can be considerably higher because of up-front training costs, a relatively short study period of only 1 year might distort results. Results of the KORINNA studies illustrate this: After 1 year the case management for elderly patients with myocardial infarction was deemed less effective and less costly than usual care, 26 but a follow-up after 3 years 20 showed higher QALYs, significantly better quality of life, and lower costs (although not significantly lower). Hence, longer study durations are strongly recommended.
To provide successful case management, case managers require specialized training. However, only half of the studies stated that the case managers received training. A detailed description of the scope and content of training was scarce. The same applies for data on caseloads and descriptions of the intensity of case management—in other words, the patient contacts. We therefore recommend that studies provide detailed intervention protocols.
Limitations
The studies included conducted their interventions in 7 nations in which transferability of the data and conclusions to the German context was possible. Evidence from low- and middle-income countries was not included in this systematic review, and therefore its results may not be broadly applicable.
CONCLUSIONS
This systematic review found that because of a large variation in case management programs, the evidence for cost-effectiveness is not yet fully conclusive for case management in general. More definitive studies with a defined protocol of case management are needed to determine cost-effectiveness. However, the existing studies often have adequate quality and, in most cases, produce recommendable conclusions. The confluence of highly developed health systems, fragmented health care services, and aging populations with multimorbidity is a situation that calls out for individualized coordination and support. Case management appears to be a promising method to support patients facing complex care situations. We therefore advise policy makers to establish case management programs as core components of effective, patient-oriented health care systems, and to support rigorous evaluation of each program.
Author Affiliations: inav – Institute for Applied Health Services Research (AKK, JJ, FF, MA), Berlin, Germany.
Source of Funding: This study was conducted in the context of the research project RubiN, funded by the Federal Joint Committee’s German Innovations Fund.
Author Disclosures: The authors report no relationship or financial interest with any entity that would pose a conflict of interest with the subject matter of this article.
Authorship Information: Concept and design (AKK, JJ, FF, MA); acquisition of data (AKK, JJ); analysis and interpretation of data (AKK, MA); drafting of the manuscript (AKK); critical revision of the manuscript for important intellectual content (JJ, FF, MA); administrative, technical, or logistic support (AKK, FF); and supervision (MA).
Address Correspondence to: Ann-Kathrin Klaehn, MSc, inav – Institute for Applied Health Services Research, Schiffbauerdamm 12, 10117 Berlin, Germany. Email: [email protected].
1. Kersbergen AL. Case management: a rich history of coordinating care to control costs. Nurs Outlook . 1996;44(4):169-172. doi:10.1016/s0029-6554(96)80037-6
2. About ACMA: definition of case management. American Case Management Association. September 9, 2020. Accessed September 21, 2021. http://www.acmaweb.org/section.aspx?sID=4
3. What is a case manager? definition of case management. Case Management Society of America. September 9, 2020. Accessed September 21, 2021. https://www.cmsa.org/who-we-are/what-is-a-case-manager/
4. Craig P, Dieppe P, Macintyre S, Michie S, Nazareth I, Petticrew M; Medical Research Council Guidance. Developing and evaluating complex interventions: the new Medical Research Council guidance. BMJ . 2008;337:a1655. doi:10.1136/bmj.a1655
5. Huntley AL, Johnson R, King A, Morris RW, Purdy S. Does case management for patients with heart failure based in the community reduce unplanned hospital admissions? a systematic review and meta-analysis. BMJ Open . 2016;6(5):e010933. doi:10.1136/bmjopen-2015-010933
6. Joo JY, Liu MF. Case management effectiveness in reducing hospital use: a systematic review. Int Nurs Rev . 2017;64(2):296-308. doi:10.1111/inr.12335
7. Stokes J, Panagioti M, Alam R, Checkland K, Cheraghi-Sogi S, Bower P. Effectiveness of case management for ‘at risk’ patients in primary care: a systematic review and meta-analysis. PLoS One . 2015;10(7):e0132340. doi:10.1371/journal.pone.0132340
8. Joo JY, Huber DL. Case management effectiveness on health care utilization outcomes: a systematic review of reviews. West J Nurs Res . 2019;41(1):111-133. doi:10.1177/0193945918762135
9. Moher D, Liberati A, Tetzlaff J, Altman DG; PRISMA Group. Preferred reporting items for systematic reviews and meta-analyses: the PRISMA statement. PLoS Med . 2009;6(7):e1000097. doi:10.1371/journal.pmed.1000097
10. Robinson KA, Saldanha IJ, Mckoy NA. Frameworks for Determining Research Gaps During Systematic Reviews. Agency for Healthcare Research and Quality; 2011.
11. Evers S, Goossens M, de Vet H, van Tulder M, Ament A. Criteria list for assessment of methodological quality of economic evaluations: consensus on Health Economic Criteria. Int J Technol Assess Health Care . 2005;21(2):240-245.
12. Gensichen J, Petersen JJ, Von Korff M, et al. Cost-effectiveness of depression case management in small practices. Br J Psychiatry . 2013;202:441-446. doi:10.1192/bjp.bp.112.118257
13. Handley MA, Shumway M, Schillinger D. Cost-effectiveness of automated telephone self-management support with nurse care management among patients with diabetes. Ann Fam Med . 2008;6(6):512-518. doi:10.1370/afm.889
14. Hay JW, Katon WJ, Ell K, Lee PJ, Guterman JJ. Cost-effectiveness analysis of collaborative care management of major depression among low-income, predominantly Hispanics with diabetes. Value Health . 2012;15(2):249-254. doi:10.1016/j.jval.2011.09.008
15. Joesch JM, Sherbourne CD, Sullivan G, Stein MB, Craske MG, Roy-Byrne P. Incremental benefits and cost of coordinated anxiety learning and management for anxiety treatment in primary care. Psychol Med . 2012;42(9):1937-1948. doi:10.1017/S0033291711002893
16. Lavelle TA, Kommareddi M, Jaycox LH, Belsher B, Freed MC, Engel CC. Cost-effectiveness of collaborative care for depression and PTSD in military personnel. Am J Manag Care . 2018;24(2):91-98.
17. MacNeil Vroomen J, Bosmans JE, Eekhout I, et al. The cost-effectiveness of two forms of case management compared to a control group for persons with dementia and their informal caregivers from a societal perspective. PLoS One . 2016;11(9):e0160908. doi:10.1371/journal.pone.0160908
18. Rost K, Smith JL, Dickinson M. The effect of improving primary care depression management on employee absenteeism and productivity. a randomized trial. Med Care . 2004;42(12):1202-1210. doi:10.1097/00005650-200412000-00007
19. Ruikes FGH, Adang EM, Assendelft WJJ, Schers HJ, Koopmans RTCM, Zuidema SU. Cost-effectiveness of a multicomponent primary care program targeting frail elderly people. BMC Fam Pract . 2018;19(1):62. doi:10.1186/s12875-018-0735-4
20. Seidl H, Hunger M, Meisinger C, et al. The 3-year cost-effectiveness of a nurse-based case management versus usual care for elderly patients with myocardial infarction: results from the KORINNA follow-up study. Value Health . 2017;20(3):441-450. doi:10.1016/j.jval.2016.10.001
21. Ulrich LR, Petersen JJ, Mergenthal K, et al. Cost-effectiveness analysis of case management for optimized antithrombotic treatment in German general practices compared to usual care – results from the PICANT trial. Health Econ Rev . 2019;9(1):4. doi:10.1186/s13561-019-0221-2
22. Wansink HJ, Drost RMWA, Paulus ATG, et al. Cost-effectiveness of preventive case management for parents with a mental illness: a randomized controlled trial from three economic perspectives. BMC Health Serv Res . 2016;16:228. doi:10.1186/s12913-016-1498-z
23. Wijnen BFM, Oberjé EJM, Evers SMAA, et al. Cost-effectiveness and cost-utility of the adherence improving self-management strategy in human immunodeficiency virus care: a trial-based economic evaluation. Clin Infect Dis . 2019;68(4):658-667. doi:10.1093/cid/ciy553
24. Michalowsky B, Xie F, Eichler T, et al. Cost-effectiveness of a collaborative dementia care management—results of a cluster-randomized controlled trial. Alzheimers Dement . 2019;15(10):1296-1308. doi:10.1016/j.jalz.2019.05.008
25. Sandberg M, Jakobsson U, Midlöv P, Kristensson J. Cost-utility analysis of case management for frail older people: effects of a randomised controlled trial. Health Econ Rev . 2015;5(1):51. doi:10.1186/s13561-015-0051-9
26. Seidl H, Hunger M, Leidl R, et al. Cost-effectiveness of nurse-based case management versus usual care for elderly patients with myocardial infarction: results from the KORINNA study. Eur J Health Econ . 2015;16(6):671-681. doi:10.1007/s10198-014-0623-3
27. Bourbeau J, Collet JP, Schwartzman K, Ducruet T, Nault D, Bradley C. Economic benefits of self-management education in COPD. Chest . 2006;130(6):1704-1711. doi:10.1378/chest.130.6.1704
28. Dehmer SP, Maciosek MV, Trower NK, et al. Economic evaluation of the Home Blood Pressure Telemonitoring and Pharmacist Case Management to Control Hypertension (Hyperlink) trial. J Am Coll Clin Pharm . 2018;1(1):21-30. doi:10.1002/jac5.1001
29. Dorman Marek K, Stetzer F, Adams SJ, Kelly L. Cost utility analysis of a home-based nurse care coordination program. Nurs Econ . 2018;36(2):83-96.
30. Grochtdreis T, Zimmermann T, Puschmann E, et al. Cost-utility of collaborative nurse-led Self-Management support for primary care patients with Anxiety, Depressive or Somatic symptoms: a cluster-randomized controlled trial (the SMADS trial). Int J Nurs Stud . 2018;80:67-75. doi:10.1016/j.ijnurstu.2017.12.010
31. Jacke CO, Salize HJ. Cost effectiveness of a health insurance based case management programme for patients with affective disorders. Article in German. Neuropsychiatr . 2014;28(3):130-141. doi:10.1007/s40211-014-0109-7
32. Lewis H, Adamson J, Atherton K, et al. CollAborative care and active surveillance for Screen-Positive EldeRs with subthreshold depression (CASPER): a multicentred randomised controlled trial of clinical effectiveness and cost-effectiveness. Health Technol Assess . 2017;21(8):1-196. doi:10.3310/hta21080
33. Mostardt S, Matusiewicz D, Schröer W, Wasem J, Neumann A. Efficacy and cost effectiveness of case management in patients with dementia. Article in German. Z Gerontol Geriatr . 2012;45(7):642-646. doi:10.1007/s00391-012-0298-2
34. Paez KA, Allen JK. Cost-effectiveness of nurse practitioner management of hypercholesterolemia following coronary revascularization. J Am Acad Nurse Pract . 2006;18(9):436-444. doi:10.1111/j.1745-7599.2006.00159.x
35. Saleh SS, Vaughn T, Levey S, Fuortes L, Uden-Holmen T, Hall JA. Cost-effectiveness of case management in substance abuse treatment. Res Soc Work Pract . 2016;16(1):38-47. doi:10.1177/1049731505276408
36. Sørensen SS, Pedersen KM, Weinreich UM, Ehlers L. Economic evaluation of community-based case management of patients suffering from chronic obstructive pulmonary disease. Appl Health Econ Health Policy . 2017;15(3):413-424. doi:10.1007/s40258-016-0298-2
37. Hay JW, Lee PJ, Jin H, et al. Cost-effectiveness of a technology-facilitated depression care management adoption model in safety-net primary care patients with type 2 diabetes. Value Health . 2018;21(5):561-568. doi:10.1016/j.jval.2017.11.005
38. Long MJ, Marshall BS. What price an additional day of life? a cost-effectiveness study of case management. Am J Manag Care . 2000;6(8):881-886.
39. Simon GE, Ludman EJ, Rutter CM. Incremental benefit and cost of telephone care management and telephone psychotherapy for depression in primary care. Arch Gen Psychiatry . 2009;66(10):1081-1089. doi:10.1001/archgenpsychiatry.2009.123
40. Bosanquet K, Adamson J, Atherton K, et al. CollAborative care for Screen-Positive EldeRs with major depression (CASPER plus): a multicentred randomised controlled trial of clinical effectiveness and cost-effectiveness. Health Technol Assess . 2017;21(67):1-252. doi:10.3310/hta21670
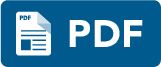
Immunotherapy Before Noon Linked to Improved Survival in mRCC
Results are consistent with the authors' prior findings on the possible effects of circadian rhythm on outcomes after immunotherapy.
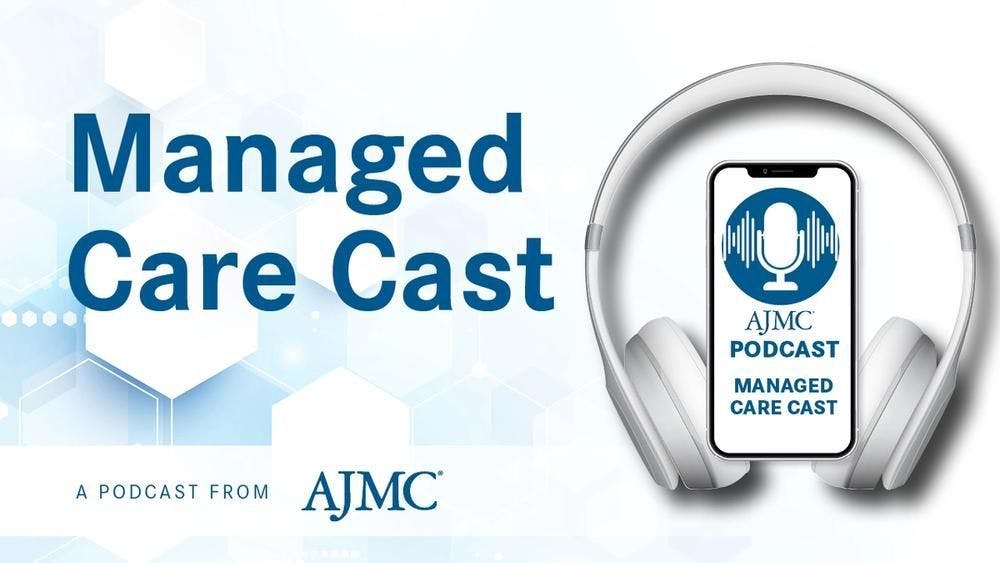
Updates on Adagrasib in CRC and the Importance of Genomic Testing With Dr Jun Gong
Jun Gong, MD, of Cedars-Sinai Medical Center, discusses the latest data on adagrasib in colorectal cancer, the importance of conducting genomic testing, and more.
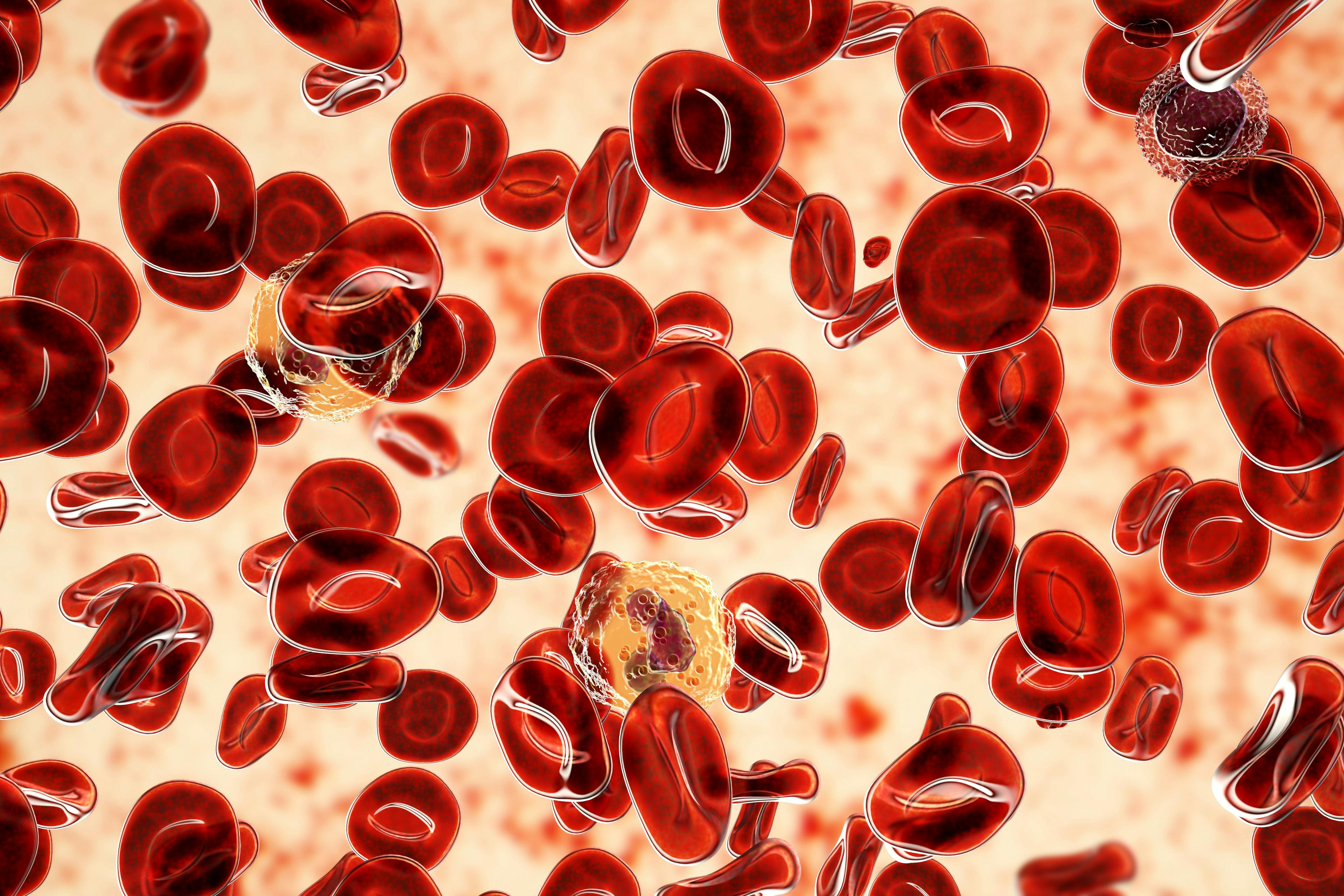
Younger Patients With PV May Benefit From Earlier Treatment With Cytoreductive Therapies
For most patients younger than age 60 with polycythemia vera (PV) who are not considered high risk, cytoreductive therapies are withheld despite being highly effective.
Managed Care Cast Presents: Insights Into Precision Medicine in NSCLC
In this podcast, a trio of experts discuss precision medicine in non–small cell lung cancer (NSCLC), current immunotherapies, and more.
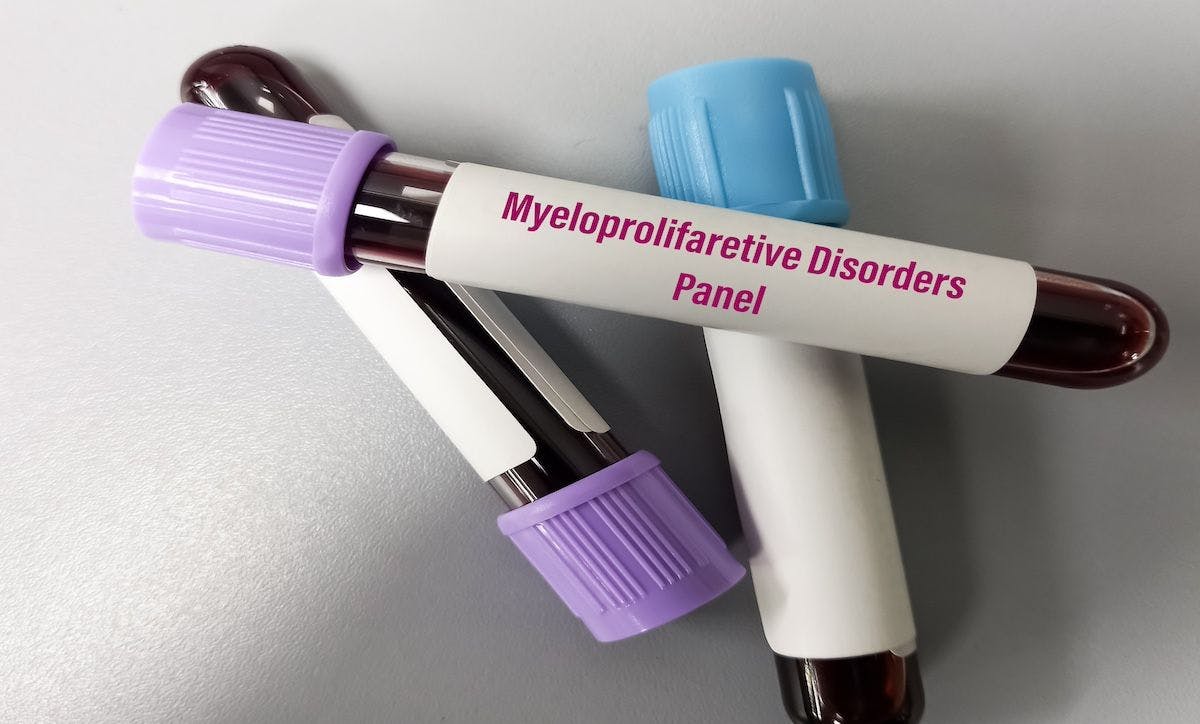
Pegylated Interferons Have Promise but Also Unmet Potential in MPNs
Despite potential benefits, the therapy remains in limited use for myeloproliferative neoplasms (MPNs).
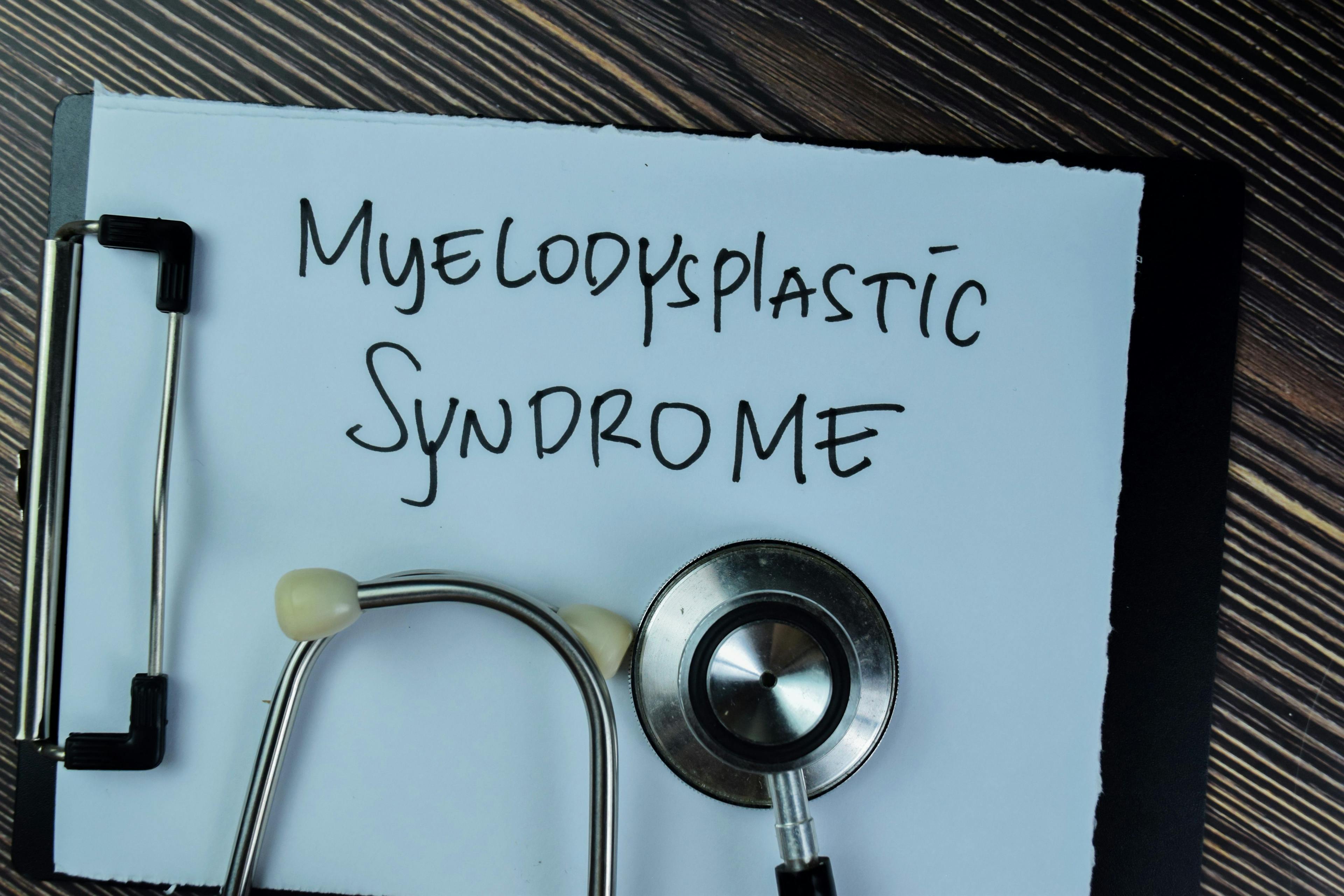
Venetoclax Resistance in Patients With MDS Explained in New Study
The report offers new insights into the limits of the BCL2 inhibitor among patients who have myelodysplastic syndromes.
2 Commerce Drive Cranbury, NJ 08512
609-716-7777

- Open access
- Published: 17 August 2016
Use of cost-effectiveness analysis to compare the efficiency of study identification methods in systematic reviews
- Ian Shemilt 1 ,
- Nada Khan 2 ,
- Sophie Park 2 &
- James Thomas 1
Systematic Reviews volume 5 , Article number: 140 ( 2016 ) Cite this article
10k Accesses
73 Citations
75 Altmetric
Metrics details
Meta-research studies investigating methods, systems, and processes designed to improve the efficiency of systematic review workflows can contribute to building an evidence base that can help to increase value and reduce waste in research. This study demonstrates the use of an economic evaluation framework to compare the costs and effects of four variant approaches to identifying eligible studies for consideration in systematic reviews.
A cost-effectiveness analysis was conducted using a basic decision-analytic model, to compare the relative efficiency of ‘safety first’, ‘double screening’, ‘single screening’ and ‘single screening with text mining’ approaches in the title-abstract screening stage of a ‘case study’ systematic review about undergraduate medical education in UK general practice settings. Incremental cost-effectiveness ratios (ICERs) were calculated as the ‘incremental cost per citation ‘saved’ from inappropriate exclusion’ from the review. Resource use and effect parameters were estimated based on retrospective analysis of ‘review process’ meta-data curated alongside the ‘case study’ review, in conjunction with retrospective simulation studies to model the integrated use of text mining. Unit cost parameters were estimated based on the ‘case study’ review’s project budget. A base case analysis was conducted, with deterministic sensitivity analyses to investigate the impact of variations in values of key parameters.
Use of ‘single screening with text mining’ would have resulted in title-abstract screening workload reductions (base case analysis) of >60 % compared with other approaches. Across modelled scenarios, the ‘safety first’ approach was, consistently, equally effective and less costly than conventional ‘double screening’. Compared with ‘single screening with text mining’, estimated ICERs for the two non-dominated approaches (base case analyses) ranged from £1975 (‘single screening’ without a ‘provisionally included’ code) to £4427 (‘safety first’ with a ‘provisionally included’ code) per citation ‘saved’. Patterns of results were consistent between base case and sensitivity analyses.
Conclusions
Alternatives to the conventional ‘double screening’ approach, integrating text mining, warrant further consideration as potentially more efficient approaches to identifying eligible studies for systematic reviews. Comparable economic evaluations conducted using other systematic review datasets are needed to determine the generalisability of these findings and to build an evidence base to inform guidance for review authors.
Peer Review reports
A series of recent journal articles highlighted the urgent need for more efficient prioritisation, design, conduct, analysis, management and regulation of research in order to increase its value and reduce waste, with the goal of improving the ways study data are curated, synthesised, used and re-used to inform decision-making about health and well-being [ 1 – 5 ]. It is therefore important to evaluate the costs and effects of methods, systems and processes designed to improve the efficiency of systematic review and evidence synthesis production workflows.
Economic evaluations are comparative analyses that assess alternative courses of action in terms of both their costs and effects and can be used to evaluate alternative methods, systems and processes. Study data compiled from economic evaluations conducted as ‘meta-research’ (‘research on research’) [ 6 , 7 ] can build into an evidence base for use to inform, for example: (i) decisions about the adoption of new methods proposed as adjuncts to, or replacements for, those commonly applied to achieve a given output at a given procedural stage of a systematic review or evidence synthesis workflow and/or (ii) choices between existing methods that could, in principle, each be applied to achieve the same output at a given stage of such workflows. With evidence from well-conducted economic evaluations in hand, decisions and choices about methods can be made on grounds of efficiency.
In this article, we aim to demonstrate the application of an economic evaluation framework to compare the costs and effects of four ( x 2) variant approaches to identifying studies for inclusion in systematic reviews. This evaluation framework is transferable and can be flexibly implemented by other systematic review authors as a ‘ S tudy W ithin A R eview’ (SWAR) [ 8 ], in order to help build an evidence base to underpin updated guidance for systematic review authors on study identification methods (for example, [ 9 – 11 ]). In the context of this evidence base, the current ‘case study’ can be viewed as an ‘ n of 1’ study that contributes a single SWAR dataset for potential incorporation into a methodology review on this topic [ 6 , 12 ].
This cost-effectiveness analysis is reported in line with the Consolidated Health Economic Evaluation Reporting Standards (CHEERS) statement [ 13 ]. Its aim was to compare the costs and effects of using each of four variant approaches, or ‘process models’ (i.e. workflows comprising a series of procedural stages, with underlying methods), to identify studies eligible for inclusion in a systematic review of the effects of undergraduate medical education in UK general practice settings. Methods and results of the ‘case study’ systematic review are reported elsewhere [ 14 ]. A brief summary of its search methods and study eligibility criteria is provided in Table 1 .
The cost-effectiveness analysis was conducted using a basic decision-analytic modelling framework. This involved the use of prospectively collected meta-data, on time use and eligibility (screening) decisions made by the ‘case study’ review team, to model the changes in flows of eligible and ineligible study records and full-text reports through each stage of the screening process that would have resulted from a decision to implement each process model, and thereby, to investigate differences in costs (resource use) and effects (recall) between the variant approaches (process models).
The structure of the decision-analytic model is a basic decision tree, as illustrated in Fig. 1 . The decision node (i.e. a node representing a decision between the four variant approaches) is shown at the top of Fig. 1 , and arrows represent the flow of title-abstract records and corresponding full-text study reports through the screening process in each process model. The four process models are described below. They differ only in those procedural steps highlighted in the upper portion (light blue-shaded area) of Fig. 1 , which concern the management, screening and coding (against the review’s eligibility criteria) of title-abstract records—as described below. All procedural steps in the full-text screening stage (lower portion, dark blue-shaded area of Fig. 1 ) are identical between the four process models: once title-abstract screening is completed, those records classified as ‘included’ or ‘provisionally included’ are retained, corresponding full-text study reports are retrieved and all of these full-texts are manually screened by two reviewers working independently, who then meet to resolve disagreements in their application of study eligibility criteria and to link together multiple full-text reports of the same eligible study. In all modelled scenarios, full-text reports are coded as either ‘included’ or ‘excluded’. In the ‘case study’ review, reviewers in practice recorded one of eight hierarchical ‘excluded’ codes for each full-text report, each denoting a specific exclusion criterion (for example ‘excluded—not in the UK’, and ‘excluded—learning not in general practice’—see [ 14 ] for further details).
Four screening methods compared in the analysis
Since the objective of the study identification process in systematic reviews is to identify all those studies that would meet their pre-specified eligibility criteria, we operationalised the analytic unit of effect as ‘a citation saved from inappropriate exclusion’ (i.e. to reflect our strong aversion to excluding a record of a study that in fact meets eligibility criteria: a ‘false negative’), compared with the least effective model in terms of its recall. This analytic unit of effect can be viewed as a measure of the performance of each ‘process model’ (approach) in identifying eligible studies: its effectiveness.
The ‘double screening’ model was selected for investigation because it represents a set of recommended and commonly used procedures to identify and select eligible studies in Cochrane and other systematic reviews [ 9 , 10 ]. However, the procedures applied in this approach are also ‘resource-hungry’ and, if there is high agreement between reviewers in their application of eligibility criteria, the cost per citation ‘saved’ from inappropriate exclusion—which can be viewed as a composite measure of the cost-effectiveness of each ‘process model’—may be high. Two of the other three ‘process models’ were selected for investigation because they are commonly used variants on a conventional ‘double screening’ approach. These can be viewed, respectively, as representing more (‘safety first’) and less (‘single screening’) cautious approaches to the title-abstract screening stage (see below). Finally, the ‘single screening with text mining’ model was selected because text mining has, in recent years, been advanced as a tool that can substantively reduce screening workload in systematic reviews; however, further evaluation is needed before it can be considered a reliable and widely accepted approach [ 15 – 17 ]. ‘Safety first’ was the method actually applied in the ‘case study’ systematic review. Each of the four variant approaches (process models) is described below.
Safety first
The first step in the ‘safety first’ process model (as in all four approaches) is that all title-abstract records retrieved by electronic searches and other search methods are uploaded to a screening platform [ 18 ] and de-duplicated, with unique records entering the title-abstract screening stage. Next, two reviewers (R1 and R2) are allocated sequential batches of the same 100–200 title-abstract records for independent manual screening. In this preliminary stage of the process, screening of each batch is followed by a teleconference between the reviewers to discuss disagreements in their application of study eligibility criteria, with the aim of establishing a high level of inter-rater reliability in advance of the main tranche of title-abstract screening.
In the main tranche of title-abstract screening that follows, the two reviewers independently screen and assign one of three mutually exclusive codes to each of the remaining title-abstract records: ‘included’ (i.e. records clearly relevant to the review); ‘provisionally included’ (i.e. records of unclear relevance based on the title-abstract, including ‘title-only’ records with no abstract) or ‘excluded’ (i.e. records clearly irrelevant to the review, to be discarded). In the ‘case study’ review, reviewers in practice recorded one of eight hierarchical ‘excluded’ codes, each denoting a specific exclusion criterion (see [ 14 ] for further details).
The key feature of the ‘safety first’ approach is that a decision by either reviewer (R1 or R2) to assign an ‘included’ or ‘provisionally included’ code to a title-abstract record is taken as sufficient for that record to proceed into the full-text screening stage. In line with the ‘safety first’ process model implemented in the ‘case study’ systematic review, a decision by either reviewer to assign an ‘included’ or ‘provisionally included’ code to a title-abstract record also triggers immediate retrieval of the corresponding full-text study report (i.e. even if the other reviewer’s decision is to assign the ‘excluded’ code). One reviewer (R1) is assigned to obtain the corresponding full-text study report for each ‘included’ or ‘provisionally included’ record. Full-texts are retrieved in electronic copy, either online or from university library resources, or alternatively in hard copy via the university library or an inter-library loan. Next, the two reviewers (R1 and R2) again work independently to screen each full-text against eligibility criteria; however, in the full-text screening stage, eligibility (coding) disagreements are flagged in real time for immediate discussion and resolution between the two reviewers. This means that title-abstract and full-text screening stages are effectively conducted in parallel, with full-texts retrieved—and final eligibility decisions made and recorded—as soon as possible after either reviewer has coded a title-abstract record as ‘included’ or ‘provisionally included’. The latter represents a variation on common practice in systematic reviews, which conventionally involves conducting title-abstract and full-text screening stages in linear sequence (i.e. fully completing title-abstract screening before commencing full-text screening—see, for example, the ‘double screening’ process model, below).
‘Safety first’ can be viewed as a more cautious approach to title-abstract screening than conventional ‘double screening’ (described below) because it eliminates the possibility that reviewers might reach an incorrect consensus decision to exclude a title-abstract record of an eligible study prior to examining the corresponding full-text. However, it could also increase the forward flow of ‘false positive’ records (i.e. records of ultimately ineligible studies coded as ‘included’ or ‘provisionally included’ by one or both reviewers) into the full-text screening stage. As such, the net impacts of this approach on overall screening workload and associated costs are unclear. We note that some methods guidance suggests study eligibility should also be checked with the authors of each primary study [ 11 ], but we have not modelled this step in the current analysis.
Double screening
‘Double screening’ was modelled as an identical set of procedures to those implemented in ‘safety first’, except that in this approach, both reviewers are required to agree to assign an ‘included’ or ‘provisionally included’ code to a title-abstract record before it is allowed to proceed to enter the full-text screening stage. The two reviewers (R1 and R2) therefore meet to discuss and resolve any disagreements between their independent title-abstract screening (coding) decisions, and make final consensus decisions on the eligibility of these title-abstracts, before corresponding full-texts are retrieved for examination. We modelled the latter procedural step using all those title-abstract records the two reviewers’ title-abstract coding decisions had disagreed about when using a ‘safety first’ approach in the ‘case study’ review.
Single screening
‘Single screening’ was again modelled as an identical set of procedures to those implemented in ‘safety first’, except that only one reviewer (R1) is assigned to manually screen all retrieved title-abstract records against eligibility criteria, instead of two reviewers (R1 and R2) working independently. For costing, R1 was modelled as a research officer and R2 as a clinical academic (see below for details of costing methods); in practice, the individuals concerned are experienced systematic reviewers (see also the ‘ Discussion ’ section).
As well as corollary reductions in research staff time invested in title-abstract screening, the ‘single screening’ process model (as with the ‘safety first’ approach) eliminates the need for meetings to discuss and resolve coding disagreements. However, ‘single screening’ is also widely perceived as a less conservative approach compared with ‘safety first’ and ‘double screening’, because it relies on the judgement of a single person to apply eligibility criteria accurately and consistently, and therefore has the potential to increase the frequency of ‘false negative’ eligibility decisions (i.e. to reduce recall) [ 19 ], which could lead to syntheses based on incomplete sets of study data, with corollary risk of introducing study selection bias into the systematic review process and its findings.
Single screening with text mining
The ‘single screening with text mining’ approach was modelled as an identical set of procedures to those implemented in the ‘single screening’ ‘process model’, except that text mining is used to prioritise title-abstract records for duplicate manual screening, and the screening process is truncated before all title-abstract records have been screened, with the remainder being automatically excluded from the review and discarded. In the current analysis, we modelled an ‘active learning’ scenario in which one reviewer (R1) commences title-abstract screening as usual and initially small sets of title-abstract records coded as ‘included or provisionally included’ or ‘excluded’ are used to train a classifier (a machine learning algorithm), which then automatically classifies all remaining (unscreened) records and returns an ordered list, with those records most likely to be eligible placed higher. The ‘active learning’ process continues in the simulation until all studies have been screened ‘manually’. We ran this simulation ten times, beginning with a random sample each time. We then assessed the consistency of results graphically and by examining the relative rank-order placement of citations across different ‘runs’ of the simulation. In the modelled scenario, the reviewer continues to screen records in prioritised order, the ‘active learning’ sequence is repeated (i.e. the classifier is re-trained and a new, re-ordered list is created) after every 25 title-abstract records have been screened, and title-abstract screening is truncated after a certain proportion of all title-records have been screened and coded, with all remaining records automatically excluded. Use of a ‘single screening with text mining’ approach can substantively reduce title-abstract screening workload, with corollary reductions in research staff time, needed to complete this stage. Current evaluations suggest that between 30 % and more than 90 % of workload might be reduced using this approach [ 16 ]; however—in addition to potential adverse effects of the ‘single screening’ approach, described above—adjunctive use of text mining could, when applied in this way, further reduce recall if the set of automatically excluded records includes ‘false negatives’ (i.e. records of eligible studies).
In order to determine a threshold recall rate to be modelled in the cost-effectiveness analysis, we conducted a retrospective simulation study to evaluate the performance of the ‘single screening with text mining’ approach, had this been implemented in the ‘case-study’ systematic review. Because simulation results showed that the use of text mining would invariably not have achieved 100 % recall in the ‘case study’ review until after the large majority of prioritised title-abstract records had been manually screened, a decision to deploy text mining in this review would in practice (and, as is typical [ 16 ]) have represented a trade-off between recall and workload. For the cost-effectiveness analysis, we therefore modelled a scenario in which the adjunctive use of text mining achieved 95 % recall, which (on average) occurred in simulations after 36 % of retrieved records had been manually screened. Our decision to model this scenario effectively meant we set ‘single screening with text mining’ to be the least effective among the four compared process models (i.e. at 95 %, it was set to achieve the lowest recall, which is used to calculate the number of citations ‘saved’ from inappropriate exclusion in the denominator of the cost-effectiveness equation).
We additionally investigated a further variant of each of the above four ‘process models’, in which the procedural step of classifying each title-abstract record does not incorporate the option of assigning a ‘provisionally included’ code instead of an ‘included’ or ‘excluded’ code. Many systematic reviews include a ‘provisionally included’ code option at the title-abstract screening stage for use to mark ‘tricky’ and/or ‘title-only’ records (i.e. those without an abstract) for later full-text assessment. While incorporating this code option provides a ‘safety net’ for reviewers when they are unsure about whether a record meets all eligibility criteria, it could increase overall screening workload by increasing the forward flow of ‘false positive records’ into the full-text screening stage (i.e. causing more corresponding full-text reports that do not ultimately meet eligibility criteria to be retrieved and unnecessarily examined). To simulate the impact of excluding this code option in each of the four variant ‘process models’ under investigation, we calculated the incremental costs associated with identifying each eligible study in each model based on the assumption that, in the absence of a ‘provisionally included’ code option, 50 % of those title-abstract records assigned this code that had an abstract would instead have been coded as ‘excluded’ and discarded, whereas the all title-only records would instead have been coded as ‘included’ (based on a ‘precautionary principle’). We also modelled a pair of simple, deterministic univariate analyses (5a and 5b in Table 5 ) in which the 50 % assumption concerning ‘provisionally included’ records with an abstract was varied +/− 25 % (i.e. 25 and 75 %).
Overall, this provided eight (4 × 2) variant process models for investigation in the cost-effectiveness analysis, each comprising variant sets of sequential procedural stages (see Fig. 1 and descriptions above). For the ‘single screening with text mining’ process model with a ‘provisionally included’ code option, simulations showed that, on average, this approach achieved 95 % recall after 39 % records had been manually screened.
The specific research objectives addressed by the cost-effectiveness analysis reported here were as follows:
To estimate the incremental costs (resource use) and effects (recall of studies included in the review) associated with the use of four variant approaches to title-abstract screening in the ‘case study’ systematic review; and
To estimate the incremental cost-effectiveness of using each approach, by combining estimates of incremental costs and effects.
The analytic perspective of the cost-effectiveness analysis was that of the systematic review author team’s research institution (a ‘single provider’ perspective). It therefore included the costs of those items of resource use expected to be the main drivers of differences between process models in costs—namely, differences in the quantities of research staff (reviewer) time allocated to identifying eligible studies, comprising time spent on manually screening title-abstract records and retrieving and examining full-text reports, and time spent in discussion to reach consensus on eligibility decisions, resulting from the different flows of study records and reports through each variant process model. The research team conducting the ‘case study’ systematic review had access to the large majority of full-text study reports via electronic library resources (online databases) provided by university subscription at no marginal cost per study report, so this item of resource use was not included in the costing.
To measure resource use in the ‘safety first’ process model (i.e. the method applied in the review), members of the ‘case study’ review team prospectively recorded the time allocated by each member of research staff to the completion of title-abstract and full-text screening, as well as the time allocated to full-text retrieval, and to discuss and resolve disagreements about the eligibility (coding) of full-text study reports. We then used these 'time use' data to estimate quantities of resource use associated with the procedural steps included within each process model (expressed in natural units, namely minutes of research staff time).
We next valued quantities of resource use by applying local unit costs obtained from university administrative database records that included details of the budget for this specific review project (this step involved simple multiplication of the relevant unit cost by the number of units of each included item of resource use: minutes of research staff time). Estimated unit costs of research staff time incorporated salaries, direct salary costs (such as national insurance and pension contributions) and university ‘indirect’ and ‘estates’ costs and were estimated separately for each of two categories of research staff involved in conducting the screening.
All costs are reported in 2013 UK GBP (£s)—the same price year and currency in which the reported costs were incurred. Estimated costs may therefore be considered specific to the UK higher education setting but, notably, they also incorporate ‘London weighting’ (i.e. an effective uplift in direct salary and university ‘indirect’ and ‘estates’ costs compared with universities located in other areas of the UK). For the ‘double screening’ model, the unit cost of resolving each disagreement about the eligibility (coding) of a title-abstract record by teleconference after the main tranche of title-abstract screening had been completed (see Fig. 1 ) was assumed to be the same as that of the same task undertaken for the purpose of establishing inter-rater reliability (see, for example, ‘safety first’, above).
All costs and effects incorporated into the cost-effectiveness analysis occurred within the time horizon of the screening process (i.e. from the start of the title-abstract screening stage to the end of the full-text screening stage) which was completed over a 19-week period during 2013 (and therefore no discount rate was applied). Cost-effectiveness was assessed in terms of the ‘incremental cost per citation saved from inappropriate exclusion’ (i.e. the incremental cost-effectiveness ratio, or ICER [ 20 ]) as a result of implementing each of the four variant study identification procedures (process models), compared with the least effective method in terms of its recall. This involved combining estimates of the incremental cost (resource use) with estimates of the incremental effect (the number of citations ‘saved’ from inappropriate exclusion) of using each of the variant process models, compared with the least effective model. Our decision to conduct a cost-effectiveness analysis reflects our interest in achieving a specified unit of output (i.e. a citation ‘saved’ from inappropriate exclusion) at the lowest cost in terms of resource use associated with this unit of output (effect).
We next conducted a series of simple, deterministic univariate sensitivity analyses to assess the resilience of our estimates of cost-effectiveness to plausible variations in the values of selected key input parameters, namely: time to screen a title-abstract record (+/− 50 %; sensitivity analysis 1a and 1b in Tables 4 and 5 ); time to screen a full-text study report (+/− 50 %; sensitivity analysis 2a and 2b in Tables 4 and 5 ); time to discuss and resolve a disagreement about the eligibility (coding) of a full-text study report (+/− 50 %; sensitivity analysis 3a and 3b in Tables 4 and 5 ); and unit costs (+/− 50 %; sensitivity analysis 4a and 4b in Tables 4 and 5 ). Finally, we investigated the impact of reduced recall on findings and conclusions of the case study review by qualitatively assessing the contribution to the ‘case study’ review of those studies that would have been excluded from consideration as a consequence of using each variant approach, if applicable.
Overall impacts on workflows
Figures 2 and 3 illustrate modelled flows of study records and corresponding full-text reports from the title-abstract screening stage into the full-text screening stage, culminating in studies being accepted into the review, and how these differ between each of the 4 × 2 variant process models, using PRISMA-style flow diagrams [ 21 ]. These figures illustrate differences in workload between the four approaches, as well as trade-offs between workload and recall. In particular, they illustrate the large modelled reduction in title-abstract screening workload of 64 % ( with a ‘provisionally included’ code option) or 61 % ( without a ‘provisionally included’ code option) associated with the use of the ‘single screening with text mining’—and corollary reductions in full-text screening workload—compared with each of the other three approaches (in which all title-abstract records are screened), set against the reduced recall of this approach (95 % compared with 99–100 %).
Modelled flows of records and study reports through screening, with a ‘provisionally included’ code
Modelled flows of records and study reports through screening, without a ‘provisionally included’ code
Impacts on resource use, costs and cost-effectiveness
Table 2 shows estimated resource use per unit (as measured in the ‘case study’ systematic review), and Table 3 shows unit costs incorporated as data inputs into the cost-effectiveness analysis.
With ‘provisionally included’ code option
Table 4 presents main results, including estimates of incremental resource use, costs, effects and cost-effectiveness associated with each process model for the four variants with a ‘provisionally included’ code option. ‘Single screening with text mining’ was set to be the least effective approach, in terms of recall, identifying 95 % of eligible study reports. Incremental results in Table 4 (and Table 5 ) are therefore presented in comparison to the ‘single screening with text mining’ approach.
Compared with ‘single screening with text mining’, the ‘single screening’ approach ‘saved’ seven citations (study records/reports) from inappropriate exclusion (99 % recall), while ‘safety first’ and ‘double screening’ each ‘saved’ eight citations (100 % recall)—these were the two most effective approaches. However, in the base case analysis, the ‘single screening with text mining’ approach was also the least costly to implement (Table 4 ; modelled with a ‘provisionally included’ code option), at an estimated total cost of £37,860 (i.e. adding together costs incurred in both the title-abstract and full-text screening stages), with a higher total cost associated with implementation of ‘single screening’ (40 % higher), ‘safety first’ (94 % higher) and ‘double screening’ (98 % higher), the latter being the most costly approach at a total cost of £75,139.
Compared with ‘single screening with text mining’ (set to 95 % recall), estimated incremental cost-effectiveness ratios (ICERs) (i.e. incremental cost per citation ‘saved’ from inappropriate exclusion) for ‘double screening’ (100 % recall) and ‘safety first’ (100 % recall) were £4660 and £4427, respectively (base case analysis). As such, the ‘double screening’ approach was dominated by ‘safety first’ in terms of cost-effectiveness (i.e. ‘double screening’ and ‘safety first’ were equally effective but ‘double screening’ was more costly). Compared with ‘single screening with text-mining’, the ICER for ‘single screening’ (99 % recall) was £2165 per citation ‘saved’ from inappropriate exclusion (base case analysis).
In sensitivity analyses, ranges of estimated ICERs (compared with ‘single screening with text mining’) were £2213 to £5986 per inappropriate exclusion avoided for ‘safety first’ approach, £2330 to £6219 for ‘double screening’ and £832 to £2718 for ‘single screening’. Within each sensitivity analysis, patterns of results for incremental costs and effects between approaches were almost invariably consistent with the base case analysis. The exception was that, in the sensitivity analysis in which the resource input (staff time) allocated to meetings held to resolve ‘coding’ disagreements was reduced by 50 %, the ‘double screening’ approach dominated ‘safety first’; ‘double screening’ was equally effective but, in this case only, cost less than ‘safety first’. This result was observed because meetings to discuss and resolve disagreements about title-abstract records are required by the ‘double screening’ approach, but not by the ‘safety first’ approach. This result implies that the incremental costs of these two approaches are likely to be sensitive to amounts of time spent discussing and resolving coding disagreements.
Without a ‘provisionally included’ code option
Table 5 presents comparable results for the four process model variants without a ‘provisionally included’ code option. In the base case analysis, estimates of the incremental costs and cost-effectiveness of ‘double screening’, ‘safety first’ and ‘single screening’ (compared with ‘single screening with text mining’) were invariably lower than was found with a ‘provisionally included’ code option, driven largely by a marginal improvement in the simulated performance of text mining, which can be attributed to lower numbers of title-abstract records of ineligible studies being present among the set of ‘included or provisionally included’ records on which the classifier is iteratively trained when a ‘provisionally included’ code is not available.
In sensitivity analyses, ranges of estimated ICERs (compared with ‘single screening with text mining’) were £2128 to £6384 per inappropriate exclusion avoided for ‘safety first’ approach, £2236 to £6709 for ‘double screening’ and £987 to £2962 for ‘single screening’. Within each sensitivity analysis, patterns of results for incremental costs and effects between approaches were entirely consistent with those reported above from sensitivity analyses for variants of process models with the provisional include option. Results of the two additional sensitivity analyses conducted for variants of process models without the provisional include option (5a and 5b in Table 5 ), concerning our base case assumption of a 50 % exclusion rate among title-abstract records coded in practice as ‘provisionally included’ with abstracts (see the ‘ Methods ’ section), showed estimates of incremental cost-effectiveness were insensitive to a +/− 25 % variation in the exclusion rate among those records.
Impact of reduced recall on the ‘case study’ review
As shown in Figs. 2 and 3 (and in Tables 4 and 5 ) above, the use of a ‘single screening’ approach would have resulted in the exclusion of one eligible study [ 22 ] from the ‘case study’ systematic review, while use of the ‘single screening with text mining’ approach would have resulted in the exclusion of eight other eligible studies [ 23 – 30 ]. Analysis of the contributions made by these nine ‘false negative’ studies to the ‘case study’ review found that all nine contributed only to the descriptive component of the review (i.e. were used to inform a descriptive summary of the included studies) but none were cited in relation to specific points of analysis within this component. None of these ‘false negative’ studies were among the set of studies incorporated into either the quantitative in-depth analysis, nor among the set incorporated into the in-depth qualitative synthesis (meta-ethnography). While one of the ‘false negative’ studies did provide a distinctive perspective concerning the influence of workplace-based learning in general practice on patient care [ 26 ], we believe this study would have been identified by one of the two complementary search methods deployed in the ‘case study’ review (namely, stakeholder consultation; the other complementary search method used, namely backward citation tracking [ 31 , 32 ], would not have identified this study as it was not cited in reference lists of studies incorporated into the in-depth syntheses). These results indicate that there would have been negligible impact on the findings or conclusions of this ‘case study’ review as a consequence of reduced recall associated with use of ‘single screening’, or ‘single screening with text mining’, rather than the ‘safety first’ approach implemented in practice or conventional ‘double screening’.
Summary of main findings
A first key finding from this analysis was that, in a systematic review of the effects of undergraduate medical education in UK general practice settings, the use of a ‘safety first’ approach to title-abstract screening—in which a record marked as ‘included’ (or ‘included or provisionally included’) by any reviewer ‘automatically’ proceeds to the full-text screening stage—was almost invariably equally effective and less costly than conventional ‘double screening’ (i.e. ‘safety first’ dominated ‘double screening’ in terms of cost-effectiveness). If this key finding was replicated in similar analyses of other systematic review datasets, conducted using a comparable modelling framework, this would justify the adoption of a ‘safety first’ approach for title-abstract screening in reviews that require broad and/or highly sensitive searches, on efficiency grounds. However, the results of the current study also highlighted that the relative efficiency of these two (and other) approaches is likely to vary between systematic reviews, contingent not only on the amount of time spent discussing and resolving coding disagreements in the title-abstract screening stage (as implied by the results of sensitivity analyses) but also on factors such as search yield (i.e. the total number of title-abstract records retrieved by searches), the inclusion rate among retrieved records, levels of topic expertise and experience among the reviewers and inter-reviewer reliability. For example, further investigation of ‘case study’ review data indicated that marginal efficiency gains from using a ‘safety first’ (compared with ‘double screening’) would have increased if larger numbers of title-abstract records had needed to be screened. Similarly, in the current ‘case study’ systematic review of undergraduate medical education in UK general practice settings, screening was completed by a medical student and GP academics, reflecting levels of expertise and familiarity with the topic that may not pertain in other reviews. Further research could therefore usefully include a focus on developing a better understanding of how variation in these factors may drive the incremental costs and effects of using ‘safety first’, compared with ‘double screening’ (or other approaches).
A second key finding was that, with recall set to 95 %, the use of ‘single screening with text mining’ would have resulted in overall title-abstract screening workload reductions (base case analysis) of 64 % ( with a ‘provisionally included’ code option) or 61 % ( without a ‘provisionally included’ code option), compared with each of the other approaches, and would therefore have incurred around half of the total cost of ‘safety first’ and ‘double screening’ (with these incremental costs being lower when comparisons were modelled without the ‘provisionally included’ code option, due to the improved performance of text mining in this scenario). This finding suggests that conducting electronic searches, then using text mining as an adjunct to a ‘single screening’ approach and applying a reasonable ‘stopping rule’ to truncate title-abstract screening, combined with complementary search methods, may represent a pragmatic and efficient approach to identifying eligible studies in large-scale, complex systematic reviews. However, this finding also highlights that decisions to use text mining as an adjunct to a ‘single screening’, ‘safety first’ or conventional ‘double screening’ approach, to prioritise records for manual screening, will be contingent on contextual factors, including the resources available to be allocated to title-abstract screening and the willingness of review teams and funders to sacrifice recall in order to substantively reduce the overall workload and total costs of systematic review production. The estimated ICERs from base case analyses of the two most conservative scenarios, ranging from £3158 (‘safety first’ with a ‘provisionally included’ code) to £4457 (‘double screening’ without a ‘provisionally included’ code) per citation ‘saved’ from inappropriate exclusion, further illustrate this trade-off. We further note that a similar trade-off would have applied in the current ‘case study’ review to a choice between the ‘single screening’ model and either of the two more costly, but also slightly more effective, approaches to title-abstract screening.
A third key finding was that incorporating a ‘provisionally included’ code option incurred higher resource use and associated costs in all four process models, due to consequent increases in the forward flow of ultimately ineligible (i.e. false positive) study records and reports into to the full-text screening stage, and would therefore have represented a less efficient strategy compared with excluding this code option.
Limitations of the cost-effectiveness analysis
This cost-effectiveness analysis contributes a single study dataset to an emerging evidence base for the relative efficiency of variant approaches to title-abstract screening in systematic reviews. As described above, it was based on data prospectively collected alongside a ‘case study’ systematic review of the effects of undergraduate medical education in UK general practice settings, conducted by an experienced team of systematic reviewers with substantial experience in primary care and medical education research, and access to UK university infrastructure (e.g. extensive electronic library resources, and systematic review software that enabled concurrent, multi-user workflows to be implemented in the study identification stage of the ‘case study’ review). It is important to highlight that contextual factors such as these determine absolute levels of resource use associated with each of the four modelled approaches. Estimates of resource use (researcher time) and costs of study identification are also specific to design features of the ‘case study’ systematic review, for example, the number and complexity of criteria that needed to be applied to reach eligibility decisions and the complexity of the topic under review. As such, the generalisability of the findings of this cost-effectiveness analysis beyond the current ‘case study’ review, and to research settings other than experienced teams based in UK higher education institutions located in London, remains to be established. This empirical question can be addressed by conducting similar cost-effectiveness analyses using the simple modelling framework demonstrated in this article, in order to contribute to building an evidence base to help inform guidance on study data identification methods in systematic reviews.
The ideal primary study design for use as a framework for an economic evaluation to assess the cost-effectiveness of variant approaches to title-abstract screening would be an adequately powered cluster randomised controlled trial, in which a sample of review teams were randomly assigned to undertake screening for the same systematic review using each variant approach (‘process model’). While such studies are in principle possible, they are unlikely in practice due to the duplication of effort such a study design would entail and the corollary impact on costs of the research. In these circumstances, simple, model-based economic evaluations using single systematic review datasets offer a feasible, low-cost alternative that can help to build the evidence base. With improved electronic curation of systematic review meta-data, coupled with prospective recording of time use among review teams, we can amass the datasets needed for such analyses relatively quickly. This includes new and existing datasets produced as a by-product of the increasing number of reviews that use text mining in their screening workflows to support study identification [ 16 ]; such datasets need to be analysed to inform the further diffusion and use of this technology [ 17 ].
It is also important to highlight that the retrospective simulations of text mining performance used as the basis for modelling the flow of study records and reports through the ‘single screening with text mining’ process model cannot, by definition, be conducted until after the screening and study selection process has been completed. As such, these data are not available to review teams in advance, to inform a decision about whether or not to use text mining, which needs to be taken at the protocol stage. This consideration highlights that, in practice, decisions to deploy text mining in the way described (i.e. to prioritise records for manual screening) are currently made on pragmatic grounds (for example, the resource available to be allocated to screening in relation to the total number of records that need to be screened) but also that such decisions should be made cognisant of evidence for the potential trade-off between reduced screening workload (cost) and reduced recall (effectiveness). Similarly, the analysis presented in this article assumes that those studies identified for inclusion in the ‘case study’ systematic review represent a complete, ‘gold standard’ reference set of all eligible studies (and recall is measured against this standard); however, in practice, this is not known at either the outset or end of any review, so authors cannot base pre-specified ‘stopping rules’ for truncating title-abstract screening on such data. Instead, pre-specified ‘stopping rules’ currently need to be formulated based on estimates of the predicted number of eligible title-abstract records (‘baseline inclusion rate’) among retrieved title-abstract records, based on preliminary screening of a random sample of those records [ 33 ]. The results of this analysis can, in conjunction with those of similar retrospective simulations of text mining performance in other systematic review datasets, be used to inform evidence-based guidance on ‘stopping rules’ for truncating title-abstract screening once a ‘sufficient’ proportion of prioritised title-abstract records have been manually screened, in order to provide ‘adequate’ insurance against the risk of ‘distorted assembly of data’ due to reviews potentially being based on less-than-complete sets of study data. The latter risk can also be mitigated in systematic reviews by the use of complementary search methods, such as backward and forward citation tracking, grey literature searches [ 10 , 31 , 32 ] and stakeholder consultation, in conjunction with electronic searches of the kind modelled in the current study.
This study has demonstrated the application of a simple, model-based economic evaluation framework to assess the incremental costs, effects and cost-effectiveness of variant approaches to study identification in systematic reviews. Its key findings suggest that alternatives to the conventional ‘double screening’ approach, implemented without a ‘provisional include’ code option and integrating text mining, may warrant further consideration as promising, potentially more efficient approaches to identifying eligible studies for systematic reviews. Further, comparable economic evaluations of other systematic review datasets are needed to determine the generalisabiity of these findings to other systematic reviews and research settings, and also to help build an evidence base to inform updated guidance for review authors on study identification methods.
Macleod MR, Michie S, Roberts I, Dirnagl U, Chalmers I, Ioannidis JPA, et al. Biomedical research: increasing value, reducing waste [Editorial]. Lancet. 2014;383(9912):101–4.
Article PubMed Google Scholar
Ioannidis JPA, Greenland S, Hlatky MA, Khoury MJ, Macleod MR, Moher D, et al. Increasing value and reducing waste in research design, conduct, and analysis. Lancet. 2014;383(9912):166–75.
Article PubMed PubMed Central Google Scholar
Salman RA-S, Beller E, Kagan J, Hemminki E, Phillips RS, Savulescu J, et al. Increasing value and reducing waste in biomedical research regulation and management. Lancet. 2014;383(9912):176–85.
Article PubMed Central Google Scholar
Chan A-W, Song F, Vickers A, Jefferson T, Dickersin K, Gøtzsche PC, et al. Increasing value and reducing waste: addressing inaccessible research. Lancet. 2014;383(9913):257–66.
Glasziou P, Altman DG, Bossuyt P, Boutron I, Clarke M, Julious S, et al. Reducing waste from incomplete or unusable reports of biomedical research. Lancet. 2014;383(9913):267–76.
Ioannidis JPA, Fanelli D, Dunne DD, Goodman SN. Meta-research: evaluation and improvement of research methods and practices. PLoS Biol. 2015;13(10):e1002264.
McKenzie JE, Clarke MJ, Chandler J. Why do we need evidence-based methods in Cochrane? [Editorial]. Cochrane Database Syst Rev. 2015. doi: 10.1002/14651858.ED000102 .
PubMed Google Scholar
Anonymous. Education section—studies within a review (SWAR). J Evid Based Med. 2012;5(3):188–89
Brunton G, Stansfield C, Thomas J. Finding Relevant Studies. In: Gough D, Oliver S, Thomas J, editors. An introduction to systematic reviews. London: Sage; 2012. p. 107–34.
Google Scholar
Lefebvre C, Manheimer E, Glanville J: Chapter 6: Searching for studies. In: Higgins JPT, Green S, editors. Cochrane Handbook for Systematic Reviews of Interventions, Version 5.1.0 [updated March 2011]. http://handbook.cochrane.org . Accessed 1 July 2016.
Higgins JPT, Deeks JJ, editors. Chapter 7: Selecting studies and collecting data. In: Higgins JPT, Green S, editors. Cochrane Handbook for Systematic Reviews of Interventions, Version 5.1.0 [updated March 2011]. http://handbook.cochrane.org . Accessed 1 July 2016.
Cochrane methodology reviews. Cochrane Methodology Review Group. 2016. http://methodology.cochrane.org . Accessed 1 July 2016.
Husereau D, Drummond M, Petrou S, Carswell C, Moher D, Greenberg D, et al. Consolidated health economic evaluation reporting standards (CHEERS) statement. BMJ. 2013;346:f1049.
Park S, Khan NF, Hampshire M, Knox R, Malpass A, Thomas J, et al. A BEME systematic review of UK undergraduate medical education in the general practice setting: BEME Guide No. 32. Med Teach. 2015;37(7):611–30.
Article Google Scholar
Wallace B, Trikalinos T, Lau J, Brodley C, Schmid C. Semi-automated screening of biomedical citations for systematic reviews. BMC Bioinformatics. 2010;11:55.
O'Mara-Eves A, Thomas J, McNaught J, Miwa M, Ananiadou S. Using text mining for study identification in systematic reviews: a systematic review of current approaches. Syst Rev. 2015;4(1):5.
Thomas J. Diffusion of innovation in systematic review methodology: why is study selection not yet assisted by automation? Evid Based Med. 2013;1(2):12.
Thomas J, Brunton J, Graziosi S. EPPI-Reviewer 4.0: software for research synthesis. London: EPPI-Centre Software, Social Science Research Unit, Institute of Education; 2010.
Buscemi N, Hartling L, Vandermeer B, Tjosvold L, Klassen TP. Single data extraction generated more errors than double data extraction in systematic reviews. J Clin Epidemiol. 2006;59(7):697–703.
Drummond MF, Sculpher MJ, Claxton K, Stoddart GL, Torrance GW. Methods for the economic evaluation of health care programmes. 4th ed. Oxford: Oxford University Press; 2015.
Moher D, Liberati A, Tetzlaff J, Altman DG, The PRISMA Group. Preferred reporting items for systematic reviews and meta-analyses: the PRISMA statement. PLoS Med. 2009;6(7):e1000097.
Wainwright JR, Sullivan FM, Morrison JM, MacNaughton RJ, McConnachie A. Audit encourages an evidence-based approach to medical practice. Med Educ. 1999;33(12):907–14.
Article CAS PubMed Google Scholar
Hawthorne K, Wood F, Hood K, Cannings-John R, Houston H. Learning to mark: a qualitative study of the experiences and concerns of medical markers. BMC Med Educ. 2006;6:25.
Cannings R, Hawthorne K, Hood K, Houston H. Putting double marking to the test: a framework to assess if it is worth the trouble. Med Educ. 2005;39(3):299–308.
Duncan P, Cribb A, Stephenson A. Developing ‘the good healthcare practitioner’: clues from a study in medical education. Learn Health Soc Care. 2003;2(4):181–90.
McKinley RK, Fraser RC, Baker RH, Riley RD. The relationship between measures of patient satisfaction and enablement and professional assessments of consultation competence. Med Teach. 2004;26(3):223–8.
Nagel C, Kirby J, Rushforth B, Pearson D. Foundation programme doctors as teachers. Clinical Teach. 2011;8(4):249–53.
Himmel W, Kochen MM. How do academic heads of departments of general practice organize patient care? A European survey. Br J Gen Pract. 1995;45(394):231–4.
CAS PubMed PubMed Central Google Scholar
Wilson M, Cleland J. Evidence for the acceptability and academic success of an innovative remote and rural extended placement. Rural Remote Health. 2008;8(3):960.
Macfarlane F, Gantley M, Murray E. The CeMENT project: a case study in change management. Med Teach. 2002;24(3):320–6.
Papaioannou D, Sutton A, Carroll C, Booth A, Wong R. Literature searching for social science systematic reviews: consideration of a range of search techniques. Health Info Libr J. 2010;27(2):114–22.
Greenhalgh T, Peacock R. Effectiveness and efficiency of search methods in systematic reviews of complex evidence: audit of primary sources. BMJ. 2005;331(7524):1064–5.
Shemilt I, Simon A, Hollands GJ, Marteau TM, Ogilvie D, O’Mara-Eves A, et al. Pinpointing needles in giant haystacks: use of text mining to reduce impractical screening workload in extremely large scoping reviews. Res Synth Methods. 2014;5(1):31–9.
Download references
Acknowledgements
We are grateful to members of the wider research team and steering committee that supported completion of the ‘case study’ systematic review investigated in this study. We also thank Phil Rose (EPPI-Centre, UCL Institute of Education) for providing technical assistance to develop Fig. 1 .
Funding for the ‘case study’ systematic review investigated in this study was provided by the National Institute for Health Research School for Primary Care Research (NIHR SPCR) (PI: Sophie Park). The views expressed in this article are those of the authors and not necessarily those of the NIHR or the Department of Health. Retrospective simulation analyses were conducted as part of a UK Medical Research Council funded grant investigating the use of text mining in systematic reviews (Grant No. MR/J005037/1) (PI: James Thomas).
Availability of data and materials
The datasets analysed during the current study are available from the corresponding author on reasonable request.
Authors’ contributions
IS analysed and interpreted the study data and drafted the manuscript. NK and SP contributed to generating the study data and revised the manuscript critically for important intellectual content. JT contributed to generating, analysing and interpreting the study data and revised the manuscript critically for important intellectual content. All authors read and approved the final manuscript.
Competing interests
Ian Shemilt is an Associate Editor for Systematic Reviews.
The authors declare that they have no other competing interests.
Consent for publication
Not applicable.
Ethics approval and consent to participate
Author information, authors and affiliations.
Social Sciences Research Unit, UCL Institute of Education, London, UK
Ian Shemilt & James Thomas
Department of Primary Care and Population Health, University College London, London, UK
Nada Khan & Sophie Park
You can also search for this author in PubMed Google Scholar
Corresponding author
Correspondence to Ian Shemilt .
Rights and permissions
Open Access This article is distributed under the terms of the Creative Commons Attribution 4.0 International License ( http://creativecommons.org/licenses/by/4.0/ ), which permits unrestricted use, distribution, and reproduction in any medium, provided you give appropriate credit to the original author(s) and the source, provide a link to the Creative Commons license, and indicate if changes were made. The Creative Commons Public Domain Dedication waiver ( http://creativecommons.org/publicdomain/zero/1.0/ ) applies to the data made available in this article, unless otherwise stated.
Reprints and permissions
About this article
Cite this article.
Shemilt, I., Khan, N., Park, S. et al. Use of cost-effectiveness analysis to compare the efficiency of study identification methods in systematic reviews. Syst Rev 5 , 140 (2016). https://doi.org/10.1186/s13643-016-0315-4
Download citation
Received : 22 May 2015
Accepted : 04 August 2016
Published : 17 August 2016
DOI : https://doi.org/10.1186/s13643-016-0315-4
Share this article
Anyone you share the following link with will be able to read this content:
Sorry, a shareable link is not currently available for this article.
Provided by the Springer Nature SharedIt content-sharing initiative
- Incremental Cost
- Text Mining
- Base Case Analysis
- Undergraduate Medical Education
- Code Option
Systematic Reviews
ISSN: 2046-4053
- Submission enquiries: Access here and click Contact Us
- General enquiries: [email protected]
- Open access
- Published: 07 February 2019
Cost-effectiveness analysis of case management for optimized antithrombotic treatment in German general practices compared to usual care – results from the PICANT trial
- Lisa R. Ulrich 1 ,
- Juliana J. Petersen 1 ,
- Karola Mergenthal 1 ,
- Andrea Berghold 2 ,
- Gudrun Pregartner 2 ,
- Rolf Holle 3 , 4 &
- Andrea Siebenhofer 1 , 5
Health Economics Review volume 9 , Article number: 4 ( 2019 ) Cite this article
4301 Accesses
2 Citations
11 Altmetric
Metrics details
By performing case management, general practitioners and health care assistants can provide additional benefits to their chronically ill patients. However, the economic effects of such case management interventions often remain unclear although how to manage the burden of chronic disease is a key question for policy-makers. This analysis aimed to compare the cost-effectiveness of 24 months of primary care case management for patients with a long-term indication for oral anticoagulation therapy with usual care.
This analysis is part of the cluster-randomized controlled Primary Care Management for Optimized Antithrombotic Treatment (PICANT) trial. A sample of 680 patients with German statutory health insurance was initially considered for the cost analysis (92% of all participants at baseline). Costs included all disease-related direct health care costs from the payer’s perspective (German statutory health insurers) plus case management costs for the intervention group. A-Quality Adjusted Life Year (QALY) measurement (EQ-5D-3 L instrument) was used to evaluate utility, and incremental cost-effectiveness ratio (ICER) to assess cost-effectiveness. Mean differences were calculated and displayed with 95%-confidence intervals (CI) from non-parametric bootstrapping (1000 replicates).
N = 505 patients (505/680, 74%) were included in the cost analysis (complete case analysis with a follow-up after 12 and 24 months as well as information on cost and QALY). After two years, the mean difference of direct health care costs per patient (€115, 95% CI [− 201; 406]) and QALYs (0.03, 95% CI [− 0.04; 0.11]) in the two groups was small and not significant. The costs of case management in the intervention group caused mean total costs per patient in this group to rise significantly (mean difference €503, 95% CI [188; 794]). The ICER was €16,767 per QALY. Regardless of the willingness of insurers to pay per QALY, the probability of the intervention being cost-effective never rose above 70%.
Conclusions
A primary care case management for patients with a long-term indication for oral anticoagulation therapy improved QALYs compared to usual care, but was more costly. However, the results may help professionals and policy-makers allocate scarce health care resources in such a way that the overall quality of care is improved at moderate costs, particularly for chronically ill patients.
Trial registration
Current Controlled Trials ISRCTN41847489 .
In Germany, general practitioners (GPs) are responsible for managing lifelong oral anticoagulation (OAC) therapy for the majority of patients [ 1 ]. Most such patients suffer from chronic conditions such as atrial fibrillation / flutter, recurrent venous and / or pulmonary thromboembolisms, or have mechanical heart prostheses [ 2 ]. They are generally treated with coumarins, or the direct oral anticoagulants (DOACs) dabigatran, rivaroxaban, apixaban, and edoxaban that have been shown to be effective in preventing thromboembolic complications [ 3 ] and reducing the risk of stroke [ 4 ]. Care for patients with (multiple) chronic conditions is quickly becoming a dominant health and economic burden for almost all health care systems [ 5 ] and effective interventions are necessary to meet their needs [ 6 ]. Patients with complex and chronic conditions can benefit considerably from the provision of care by team-based and inter-professional collaborative health care management [ 7 , 8 ], in which different health care professions such as medical doctors, health care assistants (HCAs), nurse practitioners, and physician assistants cooperate [ 9 ] at modest incremental costs [ 10 ]. In Germany, general practices generally employ one or more HCAs. They receive 2 years of basic vocational training and usually perform administrative tasks and deliver basic medical care. Even though health care assistants do not have similar academic qualifications to physician assistants and nurse practitioners [ 11 ], they increasingly perform case management and other delegated tasks [ 12 ]. Tasks in primary care case management that are typically performed by HCAs are regular patient care planning and monitoring, as well as patient education to support self-management [ 13 ]. Several randomized controlled trials (RCT) in general practices have indicated that a complex intervention that includes components of primary care case management can improve patient-relevant outcomes compared to usual care, e.g. in patients with major depression [ 14 ], with chronic heart failure [ 15 ], and at high risk [ 16 ]. A systematic review by Panagioti et al. [ 17 ] showed that patient self-management support was associated with small but significant improvements in health outcomes and a reduction in health service utilization. However, the costs and cost-effectiveness of case management interventions alongside RCTs often remain unclear [ 18 ] although how to manage the burden of chronic disease is a key question for policy-makers. They are actively seeking interventions leading to better health outcomes but the evidence on cost-effectiveness of case management interventions is still scarce, perhaps as a result of methodological challenges [ 19 , 20 ].
The objective of this analysis was to evaluate the cost-effectiveness of 24 months of primary care case management for patients with a long-term indication for oral anticoagulation therapy in general practices in the federal states of Hesse and Rhineland-Palatinate, Germany. The manuscript adheres to the Consolidated Health Economic Evaluation Reporting Standards (CHEERS) statement/checklist [ 21 ].
The analysis is part of the cluster-randomized controlled PICANT trial (Primary Care Management for Optimized Antithrombotic Treatment) that was conducted by the Institute of General Practice, Goethe-University Frankfurt am Main, Germany, between June 2012 and March 2015. The aim of the PICANT study was to investigate whether a complex intervention can improve antithrombotic management in primary health care by reducing major thromboembolic and bleeding events compared to usual care. The study protocol reporting the primary and secondary outcomes of the PICANT trial has been published elsewhere [ 22 ], as are details of the practice and patient-recruiting process and the results of the screening [ 23 ]. In brief, 52 general practices and 736 patients of ≥18 years of age, with a long-term (lifelong) indication for oral anticoagulation, and who were receiving an OAC therapy (e.g. coumarin, dabigatran, rivaroxaban), participated in the PICANT trial. At baseline, 680 (92.4%) had German statutory health insurance (SHI), compared with approximately 90% in the German population as a whole. These patients were considered for the economic analysis because costs were assessed from the perspective of statutory health funds.
Intervention
The complex intervention in the PICANT trial consisted of a best practice model that included major elements of case management, and patient education tools (e.g. information brochures and a video developed by Hua et al. [ 24 ]) for patients with a long-term indication for OAC [ 25 ]. We trained HCAs and GPs in case management and regularly monitored patients using the Coagulation-Monitoring-List to improve practice routines [ 26 ]. The main elements of the monitoring sessions were to inform patients about their disease and treatment conditions, to encourage patients to perform self-management of oral anticoagulation if they were taking coumarins, and to monitor symptoms and adherence to antithrombotic treatment. HCAs were also trained to detect complications early and to assess adverse events, such as major or minor thromboembolisms or bleeding complications, as well as drug-related side effects and interactions. The HCAs reported the monitoring results to the GP, who decided whether any further action was necessary.
Data collection and calculation
Cost data was collected using the case report form (CRF), the patient questionnaire, and an additional case management questionnaire for GPs and HCAs for the intervention group only (see Table 1 ). Data collection started at baseline (T0) and follow-up appraisals were carried out after 12 (T1) and 24 (T2) months. Utility was based on Quality-Adjusted Life Years (QALY) [ 27 ] assessed using the generic EuroQol five-dimensional questionnaire (EQ-5D-3 L) [ 28 ] included in the patient questionnaire. QALYs were calculated by converting the EQ-5D-3 L health states into utility scores using the German time trade-off scoring algorithm [ 29 ]. We used constant price weights to value medical services used and therefore neither cost nor effectiveness outcomes were discounted or adjusted for inflation [ 30 ]. Costs and utility were only calculated for non-dropouts with complete data (complete case analysis) [ 31 ].
Cost determinants by resource category
To perform the economic analysis from the perspective of statutory health funds, we assessed resource usage using cost determinants recommended by Krauth [ 32 ], as shown in Table 1 .
Only disease-related costs associated with the patients’ main indication for OAC therapy were evaluated and all costs were calculated in Euros (€). Unit prices were taken from official lists and public sources (see Table 1 ). All unit prices included rebates and patient co-payments to determine the level of reimbursement relevant for the health funds [ 33 ]. For the intervention group, we assessed the resource usage based on the cost determinants applied by Baron et al. [ 34 ].
Statistical analyses
To take into account the skewed distribution of the cost data, 95% confidence intervals (CI) for the mean differences between intervention and control group costs were calculated using the 2.5% and 97.5% percentiles from the non-parametric bootstrapping with 1000 replicates [ 35 ]. To adjust for the clustered structure of the data, we drew 26 general practices with replacement per group and calculated unweighted means of costs and QALYs for all patients within those practices in each bootstrap sample. The incremental cost-effectiveness ratio (ICER) was calculated as the ratio of differences in mean total costs and mean number of QALYs between the intervention and the control group [ 31 ]. For the bootstrapped data, mean differences between groups were plotted on a cost-effectiveness plane. Furthermore, we calculated the cost-effectiveness acceptability curve (CEAC), which indicates the probability that the intervention was cost-effectiveness at different thresholds of “willingness-to-pay” for an additional QALY [ 31 ].
We conducted sensitivity analyses following the example of Hernández et al. [ 36 ], who explored the extent to which participants with very high costs influence the cost-effectiveness. We therefore excluded patients with total costs above the 95th and 90th percentile in each study group, respectively, and repeated the analyses. All statistical analyses were performed using IBM SPSS Statistics (version 20 or higher) and R (version 3.4.2).
N = 505 patients (505/680, 74%) were included in the cost analysis because they had SHI, did not drop out of the trial, and could provide cost and QALY data. Their baseline characteristics are presented in Table 2 . Participants in the intervention and control groups were comparable in terms of sex, age, indication for oral anticoagulation therapy, type of antithrombotic medication, and EQ-5D score.
Costs and effects
After 24 months, there was no statistically significant difference between the intervention and control, either in terms of mean direct health care costs (mean difference €115, 95% CI [− 201; 406]), or with regard to the various categories of direct health care costs. The mean difference in QALYs between the groups was small and not significant (0.03, 95% CI [− 0.04; 0.11]). The mean difference in total costs was statistically significant (€503, 95% CI [188; 794]) due to the costs of case management that only applied to the intervention group. These results are shown in Table 3 .
Cost drivers in both groups were costs for hospital care (≥ 40%), for physician outpatient care (≥ 25%), and for oral anticoagulation medication (≥ 23%). The intervention costs per patient were approximately €388 after 24 months, comprising higher costs in the first year (€215) and lower costs in the second year (€175). Although relevant for statutory health insurers, costs for rehabilitation services (outpatient and inpatient), sick pay (transfer payments) for employees, and loss of patients’ contributions to SHI and other statutory insurance programs were not assessed in the economic analysis because the amounts concerned were negligible in this study population, ≥ 81% of whom were retirees.
Cost-effectiveness
The ICER was €16,767 per QALY. Figure 1 presents the bootstrapped results in the intervention and control groups displayed in a cost-effectiveness plane.
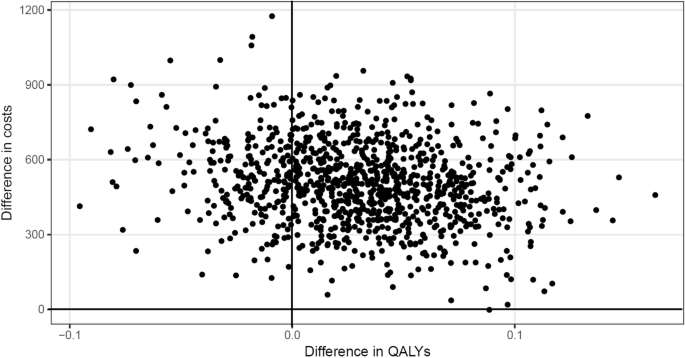
Distribution of bootstrapped incremental total costs and QALYs
It shows that the intervention was more effective regarding QALYs than usual care, but was also more costly. Of the bootstrapped ICERs, the majority (more than 75%) indicated an increase in QALYs at an incremental cost, whereas only around 25% indicated a decrease in QALYs at an incremental cost. The resulting CEAC (see Fig. 2 ) shows that the probability of the intervention being cost-effective never rose above 70%, regardless of health insurer’s willingness to pay per QALY. If the health insurer was willing to pay €15,000 per additional QALY, the probability of cost-effectiveness was 50%.
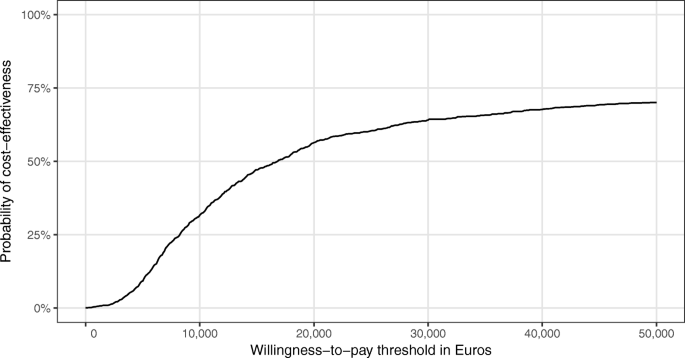
Cost-effectiveness acceptability curve (CEAC)
Sensitivity analyses
The results of the sensitivity analyses are presented in Table 4 .
When the 5% of participants that generated the highest costs were excluded, no statistically significant differences existed between the groups in terms of either direct health care costs, or QALYs. The results remained similar when the 10% of participants that were responsible for the highest cost were excluded. In terms of total costs, the results were only statistically significant because of the additional case management costs relating to the intervention group. However, the sensitivity analyses had only minimal effects on the incremental cost-effectiveness ratio.
In this analysis, we compared cost-effectiveness after 24 months of primary care case management in German general practices for patients with a lifelong indication for OAC therapy with usual care. The mean difference in direct health care costs and QALYs between the two groups was small and not significant. The difference in mean total costs per patient was statistically significant as the costs of case management were only relevant in the intervention group. The ICER was €16,767 per QALY, and the probability of the intervention being cost-effective never rose above 70%, regardless of payer willingness to pay for each QALY.
Several studies have indicated that case management interventions can improve patient-relevant outcomes [ 7 , 9 ]. This holds also true for the PICANT trial. As a secondary objective, we investigated whether the complex intervention leads to an increase in patient knowledge about anticoagulation therapy compared to usual care [ 22 ]. After 12 months, the improvement in patients’ knowledge (compared to baseline) was significantly greater in the intervention than in the control group, and the difference between both groups remained statistically significant after 24 months [ 37 ]. However, little is known about the economic effects of such complex interventions tested alongside studies with an adequate study design like RCTs [ 18 ]. In the SPRING trial, Tiessen et al. [ 38 ] assessed the costs and cost-effectiveness of cardiovascular prevention when conducted in patients with an elevated cardiovascular risk by practice nurses in general practice. The results are similar to those of the PICANT trial, as the total costs were higher in the intervention group (mean difference €175 [SPRING trial] vs. €503 [PICANT trial]), and 65% vs. 75% of the bootstrapped ICERs were located in the northeast quadrant. Regardless of a decision maker’s willingness to pay, the probability that the SPRING intervention would be cost effective compared to usual care never rose above 60% (vs. 70% in the PICANT trial). A cost-effectiveness analysis of a HCA-based case management for patients with depression showed no significant differences in QALYs and total costs between intervention and control groups after 24 months [ 39 ]. Oksman et al. [ 40 ] performed a cost-effectiveness analysis of a tele-based health-coaching program for patients with chronic diseases (type 2 diabetes, coronary artery diseases, and congestive heart failure). Similar to the results of the PICANT trial, the intervention was more effective regarding QALYs than usual care but also more costly. Compared to a HCA-based case management for high-risk patients [ 16 ], the cost of training and performing case management in the intervention group was slightly higher in the PICANT trial (€388 vs. US$247, or €211.80 based on the exchange of €1 = US$1.16622 rate on November 13, 2017). However, in both RCTs the costs of case management decreased in the second year because training costs were only relevant at the beginning of the intervention. Kaier et al. [ 41 ] performed a budget impact analysis of a telemedically supported case management (intervention) for patients with donor kidney transplantation and the intervention group showed a lower utilization of medical services as well as better medical outcomes. Other economic assessments failed to show that a nurse-based case management was either effective or cost-effective compared to usual care, e.g. for patients recently discharged from intensive care units [ 36 ], and for elderly patients with myocardial infarction after 1 year [ 42 ]. However, the latter results was revised after 3 years [ 43 ].
Several methodological challenges must be confronted when conducting economic evaluations in parallel to RCTs:
the study duration may be too short to capture relevant economic outcomes [ 19 ];
resources can be consumed for trial purposes only and therefore costs can be overestimated (“protocol driven care”) [ 19 ];
the limited follow-up may alter estimated clinical effectiveness [ 44 ];
when calculated to detect differences in clinical outcomes, the sample size may be too small (“underpowered”) to detect differences in economic indicators [ 45 ];
the generalizability of cost-effectiveness analysis can be threated “[…] when the comparison therapy is not the most relevant for the policy question being addressed.” [ 31 , p., 248];
the additional cost data collection in RCTs can increase both the costs of clinical trials and the burden on study participants [ 30 ].
Based on an analysis of registry data, Reinhold et al. [ 46 ] calculated that the direct health care costs covered by SHI of patients with atrial fibrillation in Germany amounted to at least €3274 annually. Although we chose the same perspective, the direct health care costs in the PICANT trial were much lower, possibly, because we only took the costs of oral anticoagulation and not antiplatelet therapies into account. Similar to the PICANT trial, direct health care costs were mainly driven by hospital care [ 46 ]. Other studies from Finland [ 47 ], USA [ 48 ], and Canada [ 49 ], reported direct health care costs for patients with atrial fibrillation of between €500 and €600 annually (at the current Euro exchange rates). Nevertheless, these studies only included patients who were taking warfarin. In the PICANT trial, we also included patients who were taking DOACs such as dabigatran, rivaroxaban or apixaban, which are more costly. In Germany, the mean net cost of coumarins is €0.18 per daily defined dose (DDD), compared to €3.75 for dabigatran, and €3.45 for factor Xa antagonists (e.g. rivaroxaban, apixaban) [ 50 ]. A recently published health technology assessment from UK [ 51 ] aimed to identify the most effective, safe and cost-effective anticoagulant for stroke prevention in patients with atrial fibrillation, and for primary prevention, treatment and secondary prevention of venous thromboembolisms. The results suggested that DOACs have efficacy and safety advantages over warfarin in patients with atrial fibrillation, but no more efficacious when used to treat acute venous thromboembolisms [ 51 ]. Of the available DOACs, apixaban had the highest probability of being cost-effective compared to warfarin, with a willingness-to-pay threshold of > £5000 (which corresponds to €5575.50 based on the exchange rate of €1 = £0.8970 on November 15, 2017) [ 51 ].
Strengths and limitations
Although economic evaluations are mostly performed from a societal perspective, this analysis chose the perspective of statutory health insurers. As these sickness funds cover most of the cost of health care in Germany, the results may help health care professionals decide how best to allocate resources, especially for chronically ill patients. Unrelated health care costs did not bias the results of our economic analysis as only disease related health care costs were included. One limitation of our analysis is that utilization and costs are more likely to have been underestimated than overestimated because we used unit prices from official lists and public sources. Furthermore, costs were partly calculated based on patient’s self-reported data on service use. A recall bias may therefore have led to an underestimation of costs. Although the study included a 24-month follow-up, we never used conservative methods to deal with missing data (e.g., data imputation using the last observation carried forward method). Instead of this, we used a complete case analysis. This is a more naive and simple approach to deal with missing data. However, when complete case analyses are used, (mean) cost estimates are always less precise than would be desirable. No adjustment besides the sampling strategy for the bootstrap was made to take the effects of clustering into account. With respect to the calculation of QALYs, alternative utility measurements could also have been considered, such as the 36-item short-form health survey (SF-36) [ 52 ] or the Health Utilities Index Mark 2 (HUI2) [ 53 ] or Mark 3 (HUI3) [ 54 ] but no German-specific utility weights for these measurements yet exist. However, our study is one of very few cost-effectiveness analyses of primary care case management programs for chronically ill patients that have been carried out in a real-life primary care setting as part of an RCT.
The PICANT trial indicated that primary care case management for patients with a long-term indication for oral anticoagulation therapy improved QALYs compared to usual care, but was also more costly. However, case management did not result in a statistically significant improvement in QALYs or direct health care costs compared to usual care over a period of 24 months. This RCT was conducted under real-life conditions in primary care and may help professionals and policy-makers allocate scarce health care resources in such a way that the overall quality of care is improved at moderate costs, particularly for chronically ill patients, such as those with a long-term indication for OAC therapy. Our study could help to inform the policy debate about whether an effective therapy provides sufficient value for its cost to be adopted for use and to facilitate judgments about health care interventions.
Abbreviations
Cost-effectiveness acceptability curve
Confidence interval
Case report form
Daily defined dose
Direct oral anticoagulant
Diagnosis related groups
EuroQol five-dimensional questionnaire
General practitioner
Health care assistant
Health Utilities Index Mark
Incremental cost-effectiveness ratio
Oral anticoagulant
Primary Care Management for Optimized Antithrombotic Treatment
Quality adjusted life year
Randomized controlled trial
Statutory health insurance
Chenot J-F, Hua TD, Abu Abed M, Schneider-Rudt H, Friede T, Schneider S, Vormfelde SV. Safety relevant knowledge of orally anticoagulated patients without self-monitoring: a baseline survey in primary care. BMC Fam Pract. 2014;15:104. https://doi.org/10.1186/1471-2296-15-104 .
Hua TD, Vormfelde SV, Abded MA, Schneider-Rudt H, Sobotta P, Chenot J-F. Oral anticoagulation therapy in family medicine. Z Allg Med. 2010;86:382–9.
Google Scholar
Guyatt GH. Methodology for the development of antithrombotic therapy and prevention of thrombosis guidelines: antithrombotic therapy and prevention of thrombosis, 9th ed: American College of Chest Physicians Evidence-Based Clinical Practice Guidelines. Chest. 2012;141(53). https://doi.org/10.1378/chest.11-2288 .
Camm AJ, Lip GYH. Caterina R de, Savelieva I, Atar D, Hohnloser SH, et al. 2012 focused update of the ESC guidelines for the management of atrial fibrillation: an update of the 2010 ESC guidelines for the management of atrial fibrillation * developed with the special contribution of the European heart rhythm association. Eur Heart J. 2012;33:2719–47. https://doi.org/10.1093/eurheartj/ehs253 .
Article PubMed Google Scholar
Lehnert T, Heider D, Leicht H, Heinrich S, Corrieri S, Luppa M, et al. Review: health care utilization and costs of elderly persons with multiple chronic conditions. Med Care Res Rev. 2011;68:387–420. https://doi.org/10.1177/1077558711399580 .
Wagner EH, Austin BT, von Korff M. Organizing care for patients with chronic illness. Milbank Q. 1996;74:511–44.
Article CAS Google Scholar
Wagner EH. The role of patient care teams in chronic disease management. BMJ. 2000;320:569–72.
Hudon C, Chouinard M-C, Lambert M, Diadiou F, Bouliane D, Beaudin J. Key factors of case management interventions for frequent users of healthcare services: a thematic analysis review. BMJ Open. 2017;7:e017762. https://doi.org/10.1136/bmjopen-2017-017762 .
Article PubMed PubMed Central Google Scholar
Morgan S, Pullon S, McKinlay E. Observation of interprofessional collaborative practice in primary care teams: an integrative literature review. Int J Nurs Stud. 2015;52:1217–30. https://doi.org/10.1016/j.ijnurstu.2015.03.008 .
Litaker D, Mion L, Planavsky L, Kippes C, Mehta N, Frolkis J. Physician - nurse practitioner teams in chronic disease management: the impact on costs, clinical effectiveness, and patients' perception of care. J Interprof Care. 2003;17:223–37. https://doi.org/10.1080/1356182031000122852 .
Lovink MH, Persoon A, van Vught AJAH, Koopmans RTCM, Schoonhoven L, Laurant MGH. Physician substitution by mid-level providers in primary healthcare for older people and long-term care facilities: protocol for a systematic literature review. J Adv Nurs. 2015;71:2998–3005. https://doi.org/10.1111/jan.12759 .
Bosley S, Dale J. Healthcare assistants in general practice: Practical and conceptual issues of skill-mix change. Br J Gen Pract. 2008;58:118–24. https://doi.org/10.3399/bjgp08X277032 .
von Korff M, Glasgow RE, Sharpe M. Organising care for chronic illness. BMJ. 2002;325:92–4.
Article Google Scholar
Gensichen J, von Korff M, Peitz M, Muth C, Beyer M, Güthlin C, et al. Case management for depression by health care assistants in small primary care practices: a cluster randomized trial. Ann Intern Med. 2009;151:369–78.
Peters-Klimm F, Campbell S, Hermann K, Kunz CU, Muller-Tasch T, Szecsenyi J, Failure CNH. Case management for patients with chronic systolic heart failure in primary care: the HICMan exploratory randomised controlled trial. Trials. 2010;11:56. https://doi.org/10.1186/1745-6215-11-56 .
Freund T, Peters-Klimm F, Boyd CM, Mahler C, Gensichen J, Erler A, et al. Medical assistant-based Care Management for High-Risk Patients in Small primary care practices: a cluster randomized clinical trial. Ann Intern Med. 2016;164:323–30. https://doi.org/10.7326/M14-2403 .
Panagioti M, Richardson G, Small N, Murray E, Rogers A, Kennedy A, et al. Self-management support interventions to reduce health care utilisation without compromising outcomes: a systematic review and meta-analysis. BMC Health Serv Res. 2014;14:356. https://doi.org/10.1186/1472-6963-14-356 .
Hudon C, Chouinard M-C, Lambert M, Dufour I, Krieg C. Effectiveness of case management interventions for frequent users of healthcare services: a scoping review. BMJ Open. 2016;6:e012353. https://doi.org/10.1136/bmjopen-2016-012353 .
O'Sullivan AK, Thompson D, Drummond MF. Collection of health-economic data alongside clinical trials: is there a future for piggyback evaluations? Value Health. 2005;8:67–79. https://doi.org/10.1111/j.1524-4733.2005.03065.x .
Seidl H, Meisinger C, Wende R, Holle R. Empirical analysis shows reduced cost data collection may be an efficient method in economic clinical trials. BMC Health Serv Res. 2012;12:318. https://doi.org/10.1186/1472-6963-12-318 .
Husereau D, Drummond M, Petrou S, Carswell C, Moher D, Greenberg D, et al. Consolidated health economic evaluation reporting standards (CHEERS)--explanation and elaboration: a report of the ISPOR health economic evaluation publication guidelines good reporting practices task force. Value Health. 2013;16:231–50. https://doi.org/10.1016/j.jval.2013.02.002 .
Siebenhofer A, Ulrich LR, Mergenthal K, Roehl I, Rauck S, Berghold A, et al. Primary care management for optimized antithrombotic treatment [PICANT]: study protocol for a cluster-randomized controlled trial. Implement Sci. 2012;7:79. https://doi.org/10.1186/1748-5908-7-79 .
Ulrich L-R, Mergenthal K, Petersen JJ, Roehl I, Rauck S, Kemperdick B, et al. Anticoagulant treatment in German family practices ¿ screening results from a cluster randomized controlled trial. BMC Fam Pract. 2014;15:170. https://doi.org/10.1186/s12875-014-0170-0 .
Hua TD, Vormfelde SV, Abu AM, Schneider-Rudt H, Sobotta P, Friede T, Chenot J-F. Practice nursed-based, individual and video-assisted patient education in oral anticoagulation--protocol of a cluster-randomized controlled trial. BMC Fam Pract. 2011;12:17. https://doi.org/10.1186/1471-2296-12-17 .
Siebenhofer A, Hemkens LG, Rakovac I, Spat S, Didjurgeit U. Self-management of oral anticoagulation in elderly patients - effects on treatment-related quality of life. Thromb Res. 2012;130(6). https://doi.org/10.1016/j.thromres.2012.06.012 .
Ulrich L-R, Petersen JJ, Mergenthal K, Roehl I, Rauck S, Erler A, et al. A monitoring list of Oral anticoagulation case management in primary care. Z Allg Med. 2013;89:165–71.
Testa MA, Simonson DC. Assessment of quality-of-life outcomes. N Engl J Med. 1996;334:835–40. https://doi.org/10.1056/NEJM199603283341306 .
Article CAS PubMed Google Scholar
The EuroQol Group. EuroQol--a new facility for the measurement of health-related quality of life. The EuroQol Group Health Policy. 1990;16:199–208.
Greiner W, Claes C, Busschbach JJV. Schulenburg J-MG von der. Validating the EQ-5D with time trade off for the German population. Eur J Health Econ. 2005;6:124–30.
Glick HA, Doshi JA, Sonnad SS, Polsky D. Economic evaluation in clinical trials. Oxford [u.a.]: Oxford University Press; 2010.
Drummond MF, Sculpher MJ, Torrance GW, O'Brien BJ, Stoddart GL. Methods for the economic evaluation of health care programmes. 3rd ed. Oxford. New York: Oxford University Press; 2005.
Krauth C. Estimation methods in health economic evaluation. Gesundh ökon Qual manag. 2010;15:251–9.
Braun S, Prenzler A, Mittendorf T, JMvd S. Appraisal of resource use in the German health-care system from perspective oft he statutory health insurance. Gesundheitswesen. 2009;71:19–23. https://doi.org/10.1055/s-0028-1102930 .
Baron S, Heider D, Gensichen J, Petersen JJ, Gerlach FM, Krauth C, et al. Cost Structure of a Telephone-Based Case Management in Primary Care Depression Therapy. Psychiatr Prax 2011;38:342–344. doi: https://doi.org/10.1055/s-0030-1266091 .
Barber JA, Thompson SG. Analysis of cost data in randomized trials: an application of the non-parametric bootstrap. Stat Med. 2000;19:3219–36.
Hernández RA, Jenkinson D, Vale L, Cuthbertson BH. Economic evaluation of nurse-led intensive care follow-up programmes compared with standard care: the PRaCTICaL trial. Eur J Health Econ. 2014;15:243–52. https://doi.org/10.1007/s10198-013-0470-7 .
Maikranz V, Siebenhofer A, Ulrich L-R, Mergenthal K, Schulz-Rothe S, Kemperdick B, et al. Does a complex intervention increase patient knowledge about oral anticoagulation? - a cluster-randomised controlled trial. BMC Fam Pract. 2017;18:15. https://doi.org/10.1186/s12875-017-0588-2 .
Tiessen AH, Vermeulen KM, Broer J, Smit AJ, van der Meer, Klaas. Cost-effectiveness of cardiovascular risk management by practice nurses in primary care. BMC Public Health 2013;13:148. https://doi.org/10.1186/1471-2458-13-148 .
Gensichen J, Petersen JJ, von Korff M, Heider D, Baron S, König J, et al. Cost-effectiveness of depression case management in small practices. Br J Psychiatry. 2013. https://doi.org/10.1192/bjp.bp.112.118257 .
Oksman E, Linna M, Hörhammer I, Lammintakanen J, Talja M. Cost-effectiveness analysis for a tele-based health coaching program for chronic disease in primary care. BMC Health Serv Res. 2017;17:138. https://doi.org/10.1186/s12913-017-2088-4 .
Kaier K, Hils S, Fetzer S, Hehn P, Schmid A, Hauschke D, et al. Results of a randomized controlled trial analyzing telemedically supported case management in the first year after living donor kidney transplantation - a budget impact analysis from the healthcare perspective. Health Econ Rev. 2017;7(1). https://doi.org/10.1186/s13561-016-0141-3 .
Seidl H, Hunger M, Leidl R, Meisinger C, Wende R, Kuch B, Holle R. Cost-effectiveness of nurse-based case management versus usual care for elderly patients with myocardial infarction: results from the KORINNA study. Eur J Health Econ. 2014. https://doi.org/10.1007/s10198-014-0623-3 .
Seidl H, Hunger M, Meisinger C, Kirchberger I, Kuch B, Leidl R, Holle R. The 3-year cost-effectiveness of a nurse-based case management versus usual Care for Elderly Patients with myocardial infarction: results from the KORINNA follow-up study. Value Health. 2017;20:441–50. https://doi.org/10.1016/j.jval.2016.10.001 .
Hlatky MA, Owens DK, Sanders GD. Cost-effectiveness as an outcome in randomized clinical trials. Clin Trials. 2006;3:543–51. https://doi.org/10.1177/1740774506073105 .
Briggs A. Economic evaluation and clinical trials: size matters. BMJ. 2000;321:1362–3.
Reinhold T, Rosenfeld S, Müller-Riemenschneider F, Willich SN, Meinertz T, Kirchhof P, Brüggenjürgen B. Patients suffering from atrial fibrillation in Germany. Characteristics, resource consumption and costs Herz. 2012;37:534–42. https://doi.org/10.1007/s00059-011-3575-8 .
Hallinen T, Martikainen JA, Soini EJO, Suominen L, Aronkytö T. Direct costs of warfarin treatment among patients with atrial fibrillation in a Finnish health care setting. Curr Med Res Opin. 2006;22:683–92. https://doi.org/10.1185/030079906X100014 .
Anderson RJ. Cost analysis of a managed care decentralized outpatient pharmacy anticoagulation service. J Manag Care Pharm. 2004;10:159–65.
PubMed Google Scholar
SCHULMAN S, ANDERSON DR, Bungard TJ, Jaeger T, Kahn SR, Wells P, Wilson SJ. Direct and indirect costs of management of long-term warfarin therapy in Canada. J Thromb Haemost. 2010;8:2192–200. https://doi.org/10.1111/j.1538-7836.2010.03989.x .
Schwabe U, Arzneiverordnungs-Report PD. Berlin. Heidelberg: Springer Berlin Heidelberg; 2016. p. 2016.
Sterne JA, Bodalia PN, Bryden PA, Davies PA, López-López JA, Okoli GN, et al. Oral anticoagulants for primary prevention, treatment and secondary prevention of venous thromboembolic disease, and for prevention of stroke in atrial fibrillation: systematic review, network meta-analysis and cost-effectiveness analysis. Health Technol Assess. 2017;21:1–386. https://doi.org/10.3310/hta21090 .
Brazier J, Roberts J, Deverill M. The estimation of a preference-based measure of health from the SF-36. J Health Econ. 2002;21:271–92.
Torrance GW, Feeny DH, Furlong WJ, Barr RD, Zhang Y, Wang Q. Multiattribute utility function for a comprehensive health status classification system. Health utilities index mark 2. Med Care. 1996;34:702–22.
Feeny D, Furlong W, Torrance GW, Goldsmith CH, Zhu Z, DePauw S, et al. Multiattribute and single-attribute utility functions for the health utilities index mark 3 system. Med Care. 2002;40:113–28.
Kassenärztliche Bundesvereinigung (KBV). Einheitlicher Bewertungsmaßstab (EBM): Arztgruppen-EBM, Hausarzt; 1. Quartal 2014. https://www.kbv.de/media/EBM-2009-Archiv_2.zip . Accessed 27 Feb 2014.
Kassenärztliche Bundesvereinigung (KBV). Arztgruppen-EBM. https://www.kbv.de/html/arztgruppen_ebm.php . Accessed 11 Aug 2016.
Lauer-Fischer. WEBAPO®InfoSystem. https://www.cgm.com/lauer-fischer/loesungen_lf/lauer_taxe_lf/webapo_infosystem_lf/webapo_infosystem.de.jsp . Accessed 10 June 2014.
Institut für das Entgeltsystem im Krankenhaus (InEK). Fallpauschalenkatalog 2013; 2013. https://www.g-drg.de/Aktuelles/Abrechnungsbestimmungen_und_Fallpauschalen-Katalog_2013/(language)/ger-DE . Accessed 20 May 2014.
Bock J-O, Brettschneider C, Seidl H, Bowles D, Holle R, Greiner W, König HH. Calculation of standardised unit costs from societal perspective for health economic evaluation. Gesundheitswesen. 2014. https://doi.org/10.1055/s-0034-1374621 .
Statistisches Bundesamt. Verdienste und Arbeitskosten: Verdienststrukturerhebung 2010. Wiesbaden; 2010. https://www.destatis.de/DE/Publikationen/Thematisch/VerdiensteArbeitskosten/VerdiensteBerufe/VerdienststrukturerhebungHeft1_2162001109004.pdf?__blob=publicationFile . Accessed 2 Apr 2014.
Download references
Acknowledgments
The authors would like to thank Phillip Elliott for the final editing of the manuscript, as well as the practice teams and patients of the 52 general practices that participated in the PICANT trial.
The PICANT trial was funded by the German Federal Ministry of Education and Research (grant number 01GY1145). The funding body was not involved in the design of the study, the collection, analysis, and interpretation of data, and writing the manuscript.
Availability of data and materials
All data generated or analyzed for the economic part during the PICANT trial are included in this published article.
Author information
Authors and affiliations.
Institute of General Practice, Goethe-University Frankfurt am Main, Frankfurt, Germany
Lisa R. Ulrich, Juliana J. Petersen, Karola Mergenthal & Andrea Siebenhofer
Institute for Medical Informatics, Statistics and Documentation, Medical University of Graz, Graz, Austria
Andrea Berghold & Gudrun Pregartner
Helmholtz Zentrum München - German Research Center for Environmental Health, Institute of Health Economics and Health Care Management, Neuherberg, Germany
German Center for Diabetes Research, Neuherberg, Germany
Institute of General Practice and Evidence-Based Health Services Research, Medical University of Graz, Graz, Austria
Andrea Siebenhofer
You can also search for this author in PubMed Google Scholar
Contributions
LRU was the lead author responsible for the initial draft of the manuscript that was critically revised by all authors. RH was involved in the study design for the economic analysis. AB, GP and LRU performed the statistical analyses. JJP, KM and AS participated in the whole of the study design and applied for funding. All authors read and approved the final manuscript.
Corresponding author
Correspondence to Andrea Siebenhofer .
Ethics declarations
Ethics approval and consent to participate.
The ethical review committee of the University Hospital, Goethe-University Frankfurt, Germany, approved the study June 26, 2012. All participants had to give written informed consent.
Consent for publication
Not applicable.
Competing interests
The authors declare that they have no competing interests.
Publisher’s Note
Springer Nature remains neutral with regard to jurisdictional claims in published maps and institutional affiliations.
Rights and permissions
Open Access This article is distributed under the terms of the Creative Commons Attribution 4.0 International License ( http://creativecommons.org/licenses/by/4.0/ ), which permits unrestricted use, distribution, and reproduction in any medium, provided you give appropriate credit to the original author(s) and the source, provide a link to the Creative Commons license, and indicate if changes were made.
Reprints and permissions
About this article
Cite this article.
Ulrich, L.R., Petersen, J.J., Mergenthal, K. et al. Cost-effectiveness analysis of case management for optimized antithrombotic treatment in German general practices compared to usual care – results from the PICANT trial. Health Econ Rev 9 , 4 (2019). https://doi.org/10.1186/s13561-019-0221-2
Download citation
Received : 27 July 2018
Accepted : 27 January 2019
Published : 07 February 2019
DOI : https://doi.org/10.1186/s13561-019-0221-2
Share this article
Anyone you share the following link with will be able to read this content:
Sorry, a shareable link is not currently available for this article.
Provided by the Springer Nature SharedIt content-sharing initiative
- Anticoagulants [MeSH]
- Chronic disease [MeSH]
- Cost-effectiveness analysis
- Primary health care [MeSH]
- Case management [MeSH]
- Health services research [MeSH]
Health Economics Review
ISSN: 2191-1991
- Submission enquiries: [email protected]
- General enquiries: [email protected]

An official website of the United States government
The .gov means it’s official. Federal government websites often end in .gov or .mil. Before sharing sensitive information, make sure you’re on a federal government site.
The site is secure. The https:// ensures that you are connecting to the official website and that any information you provide is encrypted and transmitted securely.
- Publications
- Account settings
Preview improvements coming to the PMC website in October 2024. Learn More or Try it out now .
- Advanced Search
- Journal List

The Impact of Hospital Costing Methods on Cost-Effectiveness Analysis: A Case Study
José leal.
1 Health Economics Research Centre, Nuffield Department of Population Health, University of Oxford, Old Road Campus, Headington, Oxford OX3 7LF UK
Stefania Manetti
2 Institute of Management, Scuola Superiore Sant’Anna, Pisa, Italy
James Buchanan
Associated data.
The data underlying the analyses in this paper cannot be made publicly available as this is not permitted by the data supplier, NHS Digital.
Several methods exist to cost hospital contacts when estimating the cost effectiveness of a new intervention. However, the implications of choosing a particular approach remain unclear. We compare the use of the three main diagnosis-related group (DRG)-based national unit costs in England to determine whether choice of approach can impact on economic evaluation results.
A cost-utility model was developed to compare secondary fracture prevention models of care for hip fracture patients, using data from large primary and hospital care administrative datasets in England. A healthcare and personal social services payer perspective was adopted, and utilities were informed by a meta-regression. Hospital resource use was valued using three DRG-based unit costs, and regression-based costing models were developed using data from 13,906 patients to inform the model health states.
Finished consultant episode (FCE)-level reference costs resulted in the highest costs on admission (£9075) and in the year of the fracture (£14,440). Relative to FCE-level costs, spell-level tariffs led to the lowest total hospital care costs per patient within 1 year of fracture (− £3691) compared with spell-level reference costs (− £2106). At a £20,000/quality-adjusted life-year threshold, using spell-level reference costs or spell-level tariffs, the introduction of a nurse-led fracture liaison service model of care was the cost-effective alternative. However, using FCE-level reference costs, usual care was the cost-effective option.
Conclusions
Our results show that, conditional on the set of national unit costs adopted, the costs of hip fracture may vary considerably and different decisions may be reached regarding the introduction of new healthcare interventions.
Electronic supplementary material
The online version of this article (10.1007/s40273-018-0673-y) contains supplementary material, which is available to authorized users.
Key Points for Decision Makers
Introduction.
There are several methods to cost hospital contacts when estimating the cost effectiveness of a new intervention. These can range from local micro-costing approaches to the use of diagnosis-related group (DRG)-based costs, which group patients according to their diagnosis and procedure codes as recorded in healthcare administrative records. In England, DRGs are called healthcare resource groups (HRGs) and the National Institute for Health and Care Excellence (NICE) recommends their use to cost hospital resource utilisation and inform economic evaluations [ 1 ]. However, analysts must choose between three main sources of HRG unit costs: (1) spell-level tariffs (commonly used to reimburse National Health Service [NHS] providers); (2) finished consultant episode (FCE)-level reference costs; and (3) spell-level reference costs.
A hospital spell, or hospital admission, comprises the total continuous stay of a patient using a hospital bed in the same hospital. During a hospital spell, a patient may receive medical care from one or more consultants. Time spent in the care of one consultant is called an FCE, and a hospital spell may contain one or more FCEs. Since 1997-98, reference cost data has been collected in England for all public-funded healthcare services (i.e. the NHS). Reference costs represent the cost of providing one unit of care in a given financial year and allow comparisons across hospital providers at the level of diagnosis, treatment and procedures [ 2 , 3 ]. Reference costs reflect the direct, indirect and overhead costs associated with providing patient care and are collected from all NHS organisations at the FCE, spell and HRG level. The process is as follows: hospital-specific cost and activity data from a given financial year (e.g. 2014/2015) are collected in the following year (2015/2016) and analysed in the third year (2016/2017) to produce a set of national reference costs (2014/2015). In contrast, national tariffs are based on historical reference costs, filtered for services relevant to the tariff, inflated to tariff year prices, adjusted for unavoidable cost differences across region, and in some cases further adjusted downwards to incentivise the efficient delivery of medical care. Tariffs serve as national prices for healthcare services [ 4 ] and are a key source of acute provider income [ 3 ].
Tariffs for admitted patient activity are paid at spell level not FCE level as the Department of Health in England considers spells to be a more robust measure of hospital activity than FCEs. Spell-level costs were first collected in 2011–2012 alongside FCE-level costs [ 3 ]. These should ideally be based on patient-level costs or FCE mean costs if the former is not possible.
The analyst is therefore faced with three potential sources of unit costs for a given financial year to apply in an economic evaluation or cost-of-illness study. The implications of choosing a particular source of HRG-based unit costs when conducting costing studies and economic evaluations remain unclear. For example, the costs of a disease may be considerably underestimated or overestimated depending on which source of unit costs is used. Also, an intervention may be judged to be cost effective at a given willingness-to-pay threshold when a particular set of unit costs is used but not another.
We aim to address this gap in knowledge using hip fractures as a case study. Hip fractures are a major public health problem with significant patient morbidity and mortality and were estimated to cost £2–3 billion annually in health and social care costs in the UK [ 5 , 6 ]. We estimated the cost variation of a hip fracture conditional on the source of HRG costs used and updated a cost-utility model developed to compare three secondary fracture prevention models of care for hip fracture patients [ 7 ]. The costs informing the cost-utility model were originally derived from the analysis of a large national hospital administrative dataset and we revisited these calculations using the three different HRG-based sets of unit costs for the financial year 2014–2015. For each source of HRG-based costs, we report the hospital costs of hip fracture as well as the absolute and incremental costs and incremental cost-effectiveness ratios (ICERs) associated with three models of secondary fracture prevention care.
We developed a cohort transition model (Markov model) to estimate the lifetime costs, quality-adjusted life-years (QALYs) and cost effectiveness of three models of care for patients with a hip fracture admitted to an NHS hospital in England: (1) introduction of an orthogeriatrician (OG)-led service which focuses on achieving optimal recovery after hip fracture; (2) introduction of a nurse-led fracture liaison service (FLS) which focuses on secondary fracture prevention; and (3) standard post-hip fracture care (without the introduction and/or expansion of the OG and FLS models of care).
The cost-utility model is described in detail elsewhere [ 7 ]. Briefly, we developed the model in Microsoft Excel ® (Microsoft Corp., Redmond, WA, USA) to simulate the natural history, quality of life and costs of individuals with an index hip fracture across health states representing history of index hip fracture, second hip fracture, major non-hip fracture(s) (pelvic, spine, wrist, humerus and rib) requiring hospitalisation, living in patient’s own home or in a care home, and death (within 30 days post-hip fracture or within a year) (Electronic Supplementary Material, Figures A1 and A2). We used an iterative process to define the model involving discussions with clinical experts and epidemiologists, supplemented by a literature review of economic models in the area. An annual cycle length was adopted, the model was run until all individuals were dead (lifetime) and half-cycle correction was performed [ 8 ]. All costs from the original model were updated to 2014/2015 values and, together with outcomes, discounted at an annual rate of 3.5%. Hospital costs were updated after re-analysis of the data used to inform the original model (see Sect. 2.3 ).
Model inputs were derived from two main sources: Hospital Episode Statistics (HES) records and Clinical Practice Research Datalink (CPRD) records. The HES dataset comprised hospital records for 33,152 patients older than 60 years who had had an emergency hospital admission with a primary International Classification of Diseases, 10th revision (ICD-10) [ 24 ] diagnosis code for hip fracture (S72.0, S72.1, S72.2 and S72.9) between April 2003 and March 2013 for a representative region of the UK [ 9 ]. This dataset was used to estimate risk equations for the following events: time to second hip fracture, time to major non-hip fragility fracture requiring hospitalisation, discharge to care home (nursing or residential) after hip fracture, and time to death [ 7 ]. HES data between April 2009 and March 2013 were used to estimate the hospital hip fracture costs in the first year following fracture and the annual hospitalisation costs for each health state of the model (inpatient, outpatient, emergency and critical care costs as described in Sects. 2.2 – 2.5 ). This time period was chosen as adult critical care records have been available as a separate HES dataset since April 2008, allowing more precise costs to be estimated for each critical episode from this date onwards. The CPRD dataset comprised all primary care contacts, laboratory tests and prescribed drugs for 4063 patients registered in the CPRD GOLD database between 1 April 2003 and 31 March 2012, who had linked hospital records indicating a hip fracture. This dataset was used to estimate the annual primary care costs for each health state of the model.
Quality-of-life estimates were derived from a meta-regression, using a linear mixed-effects model, of 32 studies (21,085 patients) reporting preference-based quality of life [ 7 ]. All model input values and sources are described in detail in the Electronic Supplementary Material.
Converting Hospital Data to Healthcare Resource Groups (HRGs)
HES data captures all hospital NHS patient care, as well as care for private patients treated in NHS hospitals and care delivered by treatment centres (including private providers) funded by the NHS. For each FCE, it contains anonymised patient administrative information (such as date of admission and discharge, admission method, age, sex and length of stay), diagnosis (ICD-10) and procedure codes (Office of Population Censuses and Surveys, 4th Revision [OPCS-4]). For the hospital cost analysis, we used HES data from April 2009 to March 2013 (4 years of data) comprising admitted patient care records, hospital outpatient activity (available from April 2003), adult critical care data (available from April 2008), and accident and emergency (A&E) attendances (available from April 2007). For each HES dataset, we derived two sets of 2014/2015 HRGs, one corresponding to the tariffs and one corresponding to the reference costs using specific Grouper software ( HRG4 2014 - 15 Payment Grouper and HRG4 + 2014 - 15 Reference Costs Grouper ) [ 10 , 11 ]. The basis of reference costs in 2014/2015 was the HRG4 + , whereas the basis for payment tariffs for the same period was the HRG4. The number of HRGs increased from 1657 in the HRG4 to 2100 in the HRG4 + [ 12 ]. The Grouper software reads patient-level data at the FCE level to produce one HRG at FCE level and one HRG at spell level (which may differ). Furthermore, if there are additional high-cost elements related to the hospital episode and spell then additional HRGs are reported (called unbundled HRGs) so that these can be fully captured.
Valuing HRGs
We matched the derived sets of HRGs at FCE and spell level to three sets of unit costs to convert hospital resource utilisation into 2014–2015 tariffs and costs:
- Spell-level tariffs 2014/2015 [ 13 ]
- FCE-level reference costs 2014/2015 [ 14 ]
- Spell-level reference costs 2014/2015 [ 14 ].
When the Grouper software produced an error code for the HRG at FCE or spell level (e.g. UZ01Z), we valued these HRGs using the weighted average of all HRGs (weights from FCE and spell activity as reported in the unit cost databases) by type of admission (elective, non-elective, day case, and regular day and night). If the length of stay exceeded the defined trimpoints for a given HRG, the cost of each additional bed day was added to the FCE-level costs or spell-level tariffs. Excess bed days were already included in the spell-level costs.
Spell-level reference costs are only collected for admitted patient care data (day cases, elective and non-elective inpatient stay) so we valued other types of hospital activity (critical care, outpatient visits/procedures, A&E attendance, unbundled HRGs) using the FCE-level reference costs dataset [ 14 ]. In the tariffs database of unit costs, some HRGs do not have national prices (e.g. critical care, dialysis for acute kidney injury, etc.) as they are subject to local prices to be contracted between commissioners and providers [ 13 ]. Furthermore, there are no data on the agreed local prices. Hence, we assumed that reference costs for the same period were proxies for the tariffs of the missing HRGs and cross-matched the HRG4 to HRG4+ codes, where required, using the patient-level diagnosis and procedure codes and the Reference Grouper software [ 10 , 11 ]. This assumption reflects the calculation of tariffs where the reference costs of all NHS providers predates and informs the introduction of the tariff [ 3 ]. Figure 1 illustrates the potential impact of the decision to use one of the three sources of unit costs to value a single hospital stay.

Valuing spell and finished consultant episode healthcare resource groups using reference costs and tariffs. This figure illustrates the potential impact of the decision to use one of the three sources of unit costs to value a single hospital stay. Using a hypothetical example of a patient being admitted with a hip fracture and having two finished consultant episodes during the hospital stay, the costs could vary between £6321 (using spell-level tariffs) and £11,741 (using finished consultant episode-level reference costs) based on the same patient and set of diagnosis, procedures and length of stay. In this example, spell-level tariffs for 2014/2015 were informed by HRG4, while reference costs for 2014/2015 were informed by HRG4+. CC complication or comorbidity, FCE finished consultant episode, HRG healthcare resource group
Statistical Analysis of Hospital Costs
Total hospital costs per patient were aggregated into annual amounts for the purposes of the analysis. We estimated the hospital costs of index fracture, hospital costs in the year of fracture and total annual hospital costs of incident hip fractures for the UK. The latter was estimated by multiplying the hospital costs in the year of fracture by the incidence of hip fracture in the UK (79,243 cases) [ 15 ]. The HES database was censored in 31 March 2013, and complete follow-up was not available for all cases. Adjusting for censoring using the methodology developed by Lin [ 16 ], we found the costs in the first 2 years of analysis to be very similar to a complete-case analysis [ 9 ]. Hence, we used complete cases to estimate the hospital costs in the first year following hip fracture as well as the annual costs associated with each health state.
Generalised linear models (GLMs) were used to predict annual hospital costs by health state. We estimated separate models for hospitalisation costs (inpatient and critical care) and non-hospitalisation costs (A&E and outpatient consultations). The following covariates were examined: sex, current age, age at hip fracture (first and second), living in a care home (nursing or residential), 30-day mortality following hip fracture, 1-year mortality following hip fracture, second hip fracture, major non-hip fracture requiring hospitalisation, history of second hip fracture, and history of major non-hip fracture. Covariates had to have a frequency of at least 100 patients to be considered for analysis, and were included in the final model if they were found to be statistically significant ( p < 0.05). Model fit was assessed using Pregibon’s Link test and different family and link functions were compared using Akaike’s information criterion.
The distributions for the regression coefficients informing the models described above were obtained by bootstrapping the sample and re-estimating the regression models. This ensured that the correlation between coefficients and regressions was fully captured.
The impact of the three HRG-based sets of national unit costs was assessed in terms of absolute hospital costs and the relative cost effectiveness of the models of care. A hypothetical cohort of 1000 identical men was used to simulate the costs and QALYs of a representative patient with a hip fracture who is living in their own home before the fracture. The model was run three times using hospital costs based on the different HRG-based sets of costs. A model of care was deemed to be cost effective if the ICER was below £20,000 per QALY gained [ 1 ] The ICER was estimated by dividing the difference in mean costs by the difference in mean effects (life-years and QALYs) for a given model of care compared with its next best alternative. The internal validity of the model was checked using sensitivity analysis (extreme values) and by comparing the model outputs with the data used to build the model. Parameter uncertainty was evaluated using probabilistic sensitivity analysis and quantified using a cost-effectiveness acceptability curve (CEAC) [ 17 ].
Using the Patient Sample to Estimate Hospital Costs
Between 1 April 2009 and 31 March 2013, 13,906 patients were identified as having had a hip fracture (Table 1 ). The mean age of the sample was 83 years and 73% were female. Most patients were of white ethnicity. The average follow-up of the cohort was 1.4 years. For cases with complete follow-up in the first year, the mean length of stay was 20.1 days in the index admission and 35.7 days in the year of the fracture.
Table 1
Characteristics of individuals with hip fracture between 2009 and 2013
SD standard deviation
a 346 missing
b Cases with complete follow-up during the 30 days following index fracture ( n = 13,743)
c Cases with complete follow-up, including those who died in that year ( n = 11,184)
Absolute Costs of Hip Fracture
Table 2 reports the mean hospital costs associated with hip fracture, estimated using the three different sets of national unit costs. Use of the FCE-level reference costs database resulted in the highest costs at the index admission (£9075) and in the year of the fracture (£14,440). Relative to FCE-level costs, use of spell-level tariffs led to the lowest total hospital care costs per patient within 1 year of fracture (a difference of –£3691, 95% confidence interval [CI] £3597 to − £3785) compared with spell-level reference costs (− £2106, 95% CI − £1987 to − £2226). Across all HRG-based sets of national unit costs, 96% of costs in the year of the fracture were due to inpatient stay and critical care.
Table 2
Hospital costs in the year of hip fracture, by source of unit costs
A&E accident and emergency, FCE finished consultant episode
a Individuals with at least 1 year of follow-up, alive or dead ( n = 11,184)
Table 3 reports the index hospital costs conditional on the number of FCEs. As the number of FCEs in a spell increases, the difference between using FCE-level reference costs and the other two national databases increases. For index admissions with only one FCE, the highest costs resulted from using the spell-level reference costs followed by FCE-level reference costs (− £694; p < 0.001) and spell-level tariffs (− £1738; p < 0.001). In contrast, index admissions with two FCEs were valued £2850 ( p < 0.001) and £3922 ( p < 0.001) higher using FCE-level reference costs than using spell-level reference costs and spell-level tariffs, respectively. Index admissions with three or more FCEs were £7308 ( p < 0.001) and £7778 ( p < 0.001) higher if valued using FCE-level reference costs than using spell-level reference costs and spell-level tariffs, respectively. Using spell-level tariffs resulted in the lowest costs of index admissions. Table A15 in the Electronic Supplementary Material reports the top five HRGs in our dataset for index admissions with one FCE according to the three sets of unit costs.
Table 3
Hospital costs in the index admission, by source of unit costs and number of finished consultant episodes per admission
FCE finished consultant episode
The total annual hospital costs associated with all incident hip fractures in the UK were estimated to vary between £813 million (spell-level tariffs) and £1099 million (FCE-level reference cost) per year depending on the source of unit costs used (Fig. 2 ).

Total annual hospital costs associated with incident hip fracture in the UK conditional on source of unit costs
Between 2009 and 2013, there were 19,644 patient-years of data available in the HES dataset to estimate the hospital cost equations. Tables A5–A14 in the Electronic Supplementary Material report the regression models for hospitalisation (admitted patient care and critical care) and non-hospitalisation costs (A&E and outpatient) based on the three sets of national unit costs. Overall, the direction of the coefficients was consistent across the three sets of unit costs. However, the magnitude and statistical significance of the covariates varied depending on the unit costs used.
For example, hospitalisation costs in year of second hip fracture were significantly associated with death within 30 days of hip fracture using FCE-level reference costs and spell-level tariffs but not with spell-level reference costs (Electronic Supplementary Material Table A6). Conditional on hospitalisation and death, living in a care home was significantly associated with higher hospitalisation costs if HRGs were valued using reference costs at FCE-level or national tariffs, but the association was no longer significant using reference costs at spell level (Electronic Supplementary Material Table A9).
Representative Male Patient
The average age of a male patient with a hip fracture, not living in a care home, was 81.4 years with a Charlson co-morbidity index (CCI) score of 1.9. Table 4 reports the total QALYs and costs (healthcare and social care) associated with usual care of a representative male patient. Using FCE-level reference costs, over the lifetime of the patient, we would expect usual care to cost £39,906 and result in 2.57 life-years (discounted). Relative to FCE-level reference costs, the mean discounted total costs were estimated to be £4057 and £5999 lower when based on spell-level reference costs and spell-level tariffs, respectively. Care home costs accounted for 32–35% of total discounted costs, depending on the set of unit costs used.
Table 4
Lifetime costs and quality-adjusted life-years, by source of unit costs a
Data are given as mean (95% confidence interval)
FCE finished consultant episode, FLS nurse-led fracture liaison services, QALYs quality-adjusted life-years
a Discounted at 3.5%
For our male cohort, the most effective model of care was the introduction of an OG, followed by the introduction of an FLS and then usual care. On average, when compared with usual care, FLS and OG-led models of care resulted in an additional 0.10 and 0.13 QALYs gained (discounted), respectively. At a £20,000/QALY threshold, using spell-level reference costs or spell-level tariffs, the introduction of a nurse-led FLS model of care was the cost-effective alternative. The probability that this was the cost-effective option was estimated at 53% (Table 5 and Electronic Supplementary Material Figure A3). However, using FCE-level reference costs, usual care was the cost-effective option at the £20,000/QALY threshold.
Table 5
Cost-effectiveness analysis results, by source of unit cost
FCE finished consultant episode, FLS nurse-led fracture liaison services, ICER incremental cost-effectiveness ratio
a Intervention judged to be cost effective if ICER was below £20,000 per quality-adjusted life-year
Our study illustrates the implications of choosing a particular source of HRG-based national unit costs when conducting costing studies and economic evaluations. To demonstrate this, we used a large dataset of hospital administrative records to estimate the costs of hip fracture and the inputs of a decision model based on three national unit cost datasets. We found that the hospital costs of hip fracture in the year of the event varied between £10,749 and £14,440 per fracture depending on the set of unit costs used. These differences impacted on the lifetime costs of individuals as well as the total hospital costs of incident hip fracture in the UK, which varied between £813 million and £1099 million per year. In addition to the impact on absolute costs, the methodological uncertainty of the cost models was considerable. Some of the predictors of costs that were significant with one dataset of costs were no longer significant using another dataset of costs.
In the reference case for technology appraisal [ 1 ], NICE recognises HRGs as a valuable source of hospital resource use and recommends the use of national unit costs collected in the form of reference costs. However, the reference case does not explicitly exclude the use of national tariffs nor sets out the type of reference cost database to use. In our case study and at a threshold of £20,000/QALY, we found that there was a notable impact on the recommendation of which intervention to implement conditional on the source of unit costs adopted. Using reference costs at spell level and tariffs resulted in FLS-led services being the most cost-effective option, whereas usual care was found to be the most cost-effective option using reference costs at FCE-level. The impact of the source of unit costs on incremental costs and incremental cost effectiveness is smaller than on absolute costs, with the ICER varying from £18,982/QALY to £20,605/QALY for FLS compared with usual care. However, in specific cases where the ICER is close to the maximum willingness-to-pay value, this is likely to impact on the suggested adoption decision. Policy makers, researchers and analysts should therefore be aware of this issue.
Reference costs were initially collected to facilitate comparisons between hospitals. However, their use has been further expanded to inform local payments, academic research and national decisions concerning the implementation of novel interventions based on economic evaluations of novel interventions. Hence, every year the Department of Health in England collects data from NHS providers and commissioners on all running costs of providing services at FCE level and, recently, at spell level for admitted patient care services. In 2014–2015, FCE-based reference costs captured £61 billion of NHS expenditure (55% of total expenditure), of which £25 billion concerned admitted patient care. Spell-based reference costs captured £25 billion in the same period [ 2 ]. Our results highlight concerns about the quality of reference cost data [ 18 , 19 ]. The reason for the significant differences in costs of hospital admissions using FCE-based and spell-based reference costs is unclear. For example, for index hospital admissions comprising two or more FCEs, we would expect hospital admission costs to be lower when based on spell-level unit costs due to potential savings on hospital entry or consultant transfer costs. However, we did not expect these differences to be as high as those observed (£2850 and £7308 for two or more FCEs, respectively), raising questions about the spell-based HRG allocation algorithms and the accuracy of the validation checks of spell and FCE costs submitted by each hospital provider. Furthermore, for index admissions with a single FCE, using spell-based unit costs resulted in higher hospital admission costs than using FCE-based unit costs. In these cases, the estimated costs using spell-based unit costs were consistently higher across all HRGs than using FCE-based unit costs, perhaps reflecting an FCE to spell ratio > 1 for index admissions. We also found that the spell-level and FCE-level HRGs did not always agree for the same patient. There is clearly a need for hospital providers to record and report cost data more accurately as well as for more transparently calculated costs and tariffs.
This is the first study to evaluate the implications of choosing different HRG-based national unit costs when conducting costing studies and to then use the resulting cost estimates within economic evaluations. A key strength of our study is that we were able to utilise a large linked dataset of hospital and clinical practice records that allowed us to ensure that observed differences were precisely estimated. Our work builds on two earlier related studies. The first study, by Geue et al. [ 20 ], compared five methods of costing HES using data from Scotland on acute hospital admissions, applying HRG version 3.5 Grouper software. The different approaches were based on HRG codes, used information on per diem costs, or derived specialty specific costs using information on length of stay. Substantial differences in cost estimates were observed, with approaches tied to length of stay yielding higher costs. The study concludes by recommending the use of a specific HRG costing method.
The second study, by Thorn et al. [ 21 ], evaluated the inpatient costs of 292 men with prostate cancer using two approaches: HES data combined with NHS reference costs; and costs derived from a review of medical records. Again, HRG version 3.5 Grouper software was used. The key finding was that the costs estimated using the HES approach were 8% lower than those estimated via medical record review. However, this was not a significant difference.
Our work moves this literature forward by using linked data on a variety of hospital activities (not just acute care) over a long time period for a large sample to investigate the impact of choice of costing approach on economic evaluation results. For this reason, we believe that our findings are broadly generalisable to other costing studies and economic evaluations in an English NHS context. Several limitations of our study should, however, be noted. It would have been useful to contrast the results against those obtained using a micro-costing approach. However, given the scale of the exercise and the available dataset, this was not judged to be appropriate or feasible. Furthermore, our aim was to replicate what is most likely to occur in practice when a researcher needs to cost a disease or perform an economic evaluation. Spell-based reference costs only concern admitted patient care and, as a result, non-admitted patient care (A&E, outpatient visits and procedures) were valued using the FCE-based reference costs. Spell-based tariffs were missing for some HRGs as they are subject to local prices to be contracted between commissioners and providers [ 13 ]; we used as a proxy the FCE-based reference costs for the same year. These assumptions likely reduced the estimated differences in costs in the three analyses. However, the impact of this effect is likely to be small as the contribution of the non-admitted patient care costs to total costs is small and the proportion of hospitalisation data with missing spell-based tariffs was limited. A final point to note is that 2014/2015 reference costs were based on HRG4+, whereas in the same period payment tariffs were based on HRG4 (which contained fewer HRGs). However, the impact of this change on our results is unclear.
We hope that the results of our study will be informative for analysts who need to select a set of HRG-based unit costs for a future costing study or economic evaluation. The appropriate set of unit costs to use will vary depending on the analytical perspective that is adopted; hence, it is not possible to make an overall recommendation regarding this analytical decision. If the broad aim of a study is to inform resource allocation, analysts may prefer to apply reference costs as these are likely to be the best proxy for opportunity costs. Alternatively, if a study is being conducted from the perspective of a hospital provider, tariffs may be the more appropriate choice. It should also be noted that the Department of Health favour the use of FCE-based reference costs rather than spell costs [ 14 ]. Health technology assessment agencies in other countries, such as Canada and Australia, have been more ambiguous about the appropriate approach to use in different circumstances [ 22 , 23 ]. Finally, regardless of the approach selected in the base-case analysis of a study, if the ICERs generated in an economic evaluation fall close to the threshold of the relevant decision maker, and/or considerable uncertainty is observed in the CEACs, it would be good practice for analysts to conduct sensitivity analyses in which alternative sets of unit costs are applied.
As the availability of large administrative records increases it becomes important to ensure that such data are analysed appropriately and the methods used are documented fully. Our results show that, conditional on the set of national unit costs adopted, the cost of diseases may vary considerably and different policy decisions may be made regarding the introduction of new healthcare interventions. The variability in cost estimates may impair healthcare planning and any misallocation of scarce healthcare resources may ultimately lead to suboptimal patient health outcomes, reducing population health.
Data Availability Statement
Below is the link to the electronic supplementary material.
Acknowledgements
This project was funded by the National Institute for Health Research (NIHR) Health Services and Delivery Research programme (project number 11/1023/01). In addition, Stefania Manetti was awarded a traineeship to undertake work related to this project by the “Talent at Work” Erasmus + Mobility Consortium, coordinated by Scuola Superiore Sant’Anna in Pisa, Italy. The interpretation and conclusions contained in this study are those of the authors alone.
Author contributions
José Leal initiated, designed and led the study, contributed to the analysis and interpretation of the results, and drafted the manuscript. Stefania Manetti conducted the analysis and contributed to the interpretation of the results. James Buchanan contributed to the design of the study and the interpretation of the results, and drafted the manuscript. José Leal acts as the overall guarantor for this work.
Compliance with Ethical Standards
José Leal, Stefania Manetti and James Buchanan declare that they have no conflicts of interest.
This project was funded by the NIHR Health Services and Delivery Research programme (project number 11/1023/01).
Ethical approval was not required for this study.
The original version of this article was revised due to Open Access License conversion from CC-BY-NC TO CC-BY.
Change history
The Open Access license, which previously read.
- Search Menu
- Browse content in Arts and Humanities
- Browse content in Archaeology
- Anglo-Saxon and Medieval Archaeology
- Archaeological Methodology and Techniques
- Archaeology by Region
- Archaeology of Religion
- Archaeology of Trade and Exchange
- Biblical Archaeology
- Contemporary and Public Archaeology
- Environmental Archaeology
- Historical Archaeology
- History and Theory of Archaeology
- Industrial Archaeology
- Landscape Archaeology
- Mortuary Archaeology
- Prehistoric Archaeology
- Underwater Archaeology
- Urban Archaeology
- Zooarchaeology
- Browse content in Architecture
- Architectural Structure and Design
- History of Architecture
- Residential and Domestic Buildings
- Theory of Architecture
- Browse content in Art
- Art Subjects and Themes
- History of Art
- Industrial and Commercial Art
- Theory of Art
- Biographical Studies
- Byzantine Studies
- Browse content in Classical Studies
- Classical Literature
- Classical Reception
- Classical History
- Classical Philosophy
- Classical Mythology
- Classical Art and Architecture
- Classical Oratory and Rhetoric
- Greek and Roman Papyrology
- Greek and Roman Archaeology
- Greek and Roman Epigraphy
- Greek and Roman Law
- Late Antiquity
- Religion in the Ancient World
- Digital Humanities
- Browse content in History
- Colonialism and Imperialism
- Diplomatic History
- Environmental History
- Genealogy, Heraldry, Names, and Honours
- Genocide and Ethnic Cleansing
- Historical Geography
- History by Period
- History of Emotions
- History of Agriculture
- History of Education
- History of Gender and Sexuality
- Industrial History
- Intellectual History
- International History
- Labour History
- Legal and Constitutional History
- Local and Family History
- Maritime History
- Military History
- National Liberation and Post-Colonialism
- Oral History
- Political History
- Public History
- Regional and National History
- Revolutions and Rebellions
- Slavery and Abolition of Slavery
- Social and Cultural History
- Theory, Methods, and Historiography
- Urban History
- World History
- Browse content in Language Teaching and Learning
- Language Learning (Specific Skills)
- Language Teaching Theory and Methods
- Browse content in Linguistics
- Applied Linguistics
- Cognitive Linguistics
- Computational Linguistics
- Forensic Linguistics
- Grammar, Syntax and Morphology
- Historical and Diachronic Linguistics
- History of English
- Language Evolution
- Language Reference
- Language Variation
- Language Families
- Language Acquisition
- Lexicography
- Linguistic Anthropology
- Linguistic Theories
- Linguistic Typology
- Phonetics and Phonology
- Psycholinguistics
- Sociolinguistics
- Translation and Interpretation
- Writing Systems
- Browse content in Literature
- Bibliography
- Children's Literature Studies
- Literary Studies (Romanticism)
- Literary Studies (American)
- Literary Studies (Modernism)
- Literary Studies (Asian)
- Literary Studies (European)
- Literary Studies (Eco-criticism)
- Literary Studies - World
- Literary Studies (1500 to 1800)
- Literary Studies (19th Century)
- Literary Studies (20th Century onwards)
- Literary Studies (African American Literature)
- Literary Studies (British and Irish)
- Literary Studies (Early and Medieval)
- Literary Studies (Fiction, Novelists, and Prose Writers)
- Literary Studies (Gender Studies)
- Literary Studies (Graphic Novels)
- Literary Studies (History of the Book)
- Literary Studies (Plays and Playwrights)
- Literary Studies (Poetry and Poets)
- Literary Studies (Postcolonial Literature)
- Literary Studies (Queer Studies)
- Literary Studies (Science Fiction)
- Literary Studies (Travel Literature)
- Literary Studies (War Literature)
- Literary Studies (Women's Writing)
- Literary Theory and Cultural Studies
- Mythology and Folklore
- Shakespeare Studies and Criticism
- Browse content in Media Studies
- Browse content in Music
- Applied Music
- Dance and Music
- Ethics in Music
- Ethnomusicology
- Gender and Sexuality in Music
- Medicine and Music
- Music Cultures
- Music and Media
- Music and Culture
- Music and Religion
- Music Education and Pedagogy
- Music Theory and Analysis
- Musical Scores, Lyrics, and Libretti
- Musical Structures, Styles, and Techniques
- Musicology and Music History
- Performance Practice and Studies
- Race and Ethnicity in Music
- Sound Studies
- Browse content in Performing Arts
- Browse content in Philosophy
- Aesthetics and Philosophy of Art
- Epistemology
- Feminist Philosophy
- History of Western Philosophy
- Metaphysics
- Moral Philosophy
- Non-Western Philosophy
- Philosophy of Language
- Philosophy of Mind
- Philosophy of Perception
- Philosophy of Action
- Philosophy of Law
- Philosophy of Religion
- Philosophy of Science
- Philosophy of Mathematics and Logic
- Practical Ethics
- Social and Political Philosophy
- Browse content in Religion
- Biblical Studies
- Christianity
- East Asian Religions
- History of Religion
- Judaism and Jewish Studies
- Qumran Studies
- Religion and Education
- Religion and Health
- Religion and Politics
- Religion and Science
- Religion and Law
- Religion and Art, Literature, and Music
- Religious Studies
- Browse content in Society and Culture
- Cookery, Food, and Drink
- Cultural Studies
- Customs and Traditions
- Ethical Issues and Debates
- Hobbies, Games, Arts and Crafts
- Lifestyle, Home, and Garden
- Natural world, Country Life, and Pets
- Popular Beliefs and Controversial Knowledge
- Sports and Outdoor Recreation
- Technology and Society
- Travel and Holiday
- Visual Culture
- Browse content in Law
- Arbitration
- Browse content in Company and Commercial Law
- Commercial Law
- Company Law
- Browse content in Comparative Law
- Systems of Law
- Competition Law
- Browse content in Constitutional and Administrative Law
- Government Powers
- Judicial Review
- Local Government Law
- Military and Defence Law
- Parliamentary and Legislative Practice
- Construction Law
- Contract Law
- Browse content in Criminal Law
- Criminal Procedure
- Criminal Evidence Law
- Sentencing and Punishment
- Employment and Labour Law
- Environment and Energy Law
- Browse content in Financial Law
- Banking Law
- Insolvency Law
- History of Law
- Human Rights and Immigration
- Intellectual Property Law
- Browse content in International Law
- Private International Law and Conflict of Laws
- Public International Law
- IT and Communications Law
- Jurisprudence and Philosophy of Law
- Law and Society
- Law and Politics
- Browse content in Legal System and Practice
- Courts and Procedure
- Legal Skills and Practice
- Primary Sources of Law
- Regulation of Legal Profession
- Medical and Healthcare Law
- Browse content in Policing
- Criminal Investigation and Detection
- Police and Security Services
- Police Procedure and Law
- Police Regional Planning
- Browse content in Property Law
- Personal Property Law
- Study and Revision
- Terrorism and National Security Law
- Browse content in Trusts Law
- Wills and Probate or Succession
- Browse content in Medicine and Health
- Browse content in Allied Health Professions
- Arts Therapies
- Clinical Science
- Dietetics and Nutrition
- Occupational Therapy
- Operating Department Practice
- Physiotherapy
- Radiography
- Speech and Language Therapy
- Browse content in Anaesthetics
- General Anaesthesia
- Neuroanaesthesia
- Clinical Neuroscience
- Browse content in Clinical Medicine
- Acute Medicine
- Cardiovascular Medicine
- Clinical Genetics
- Clinical Pharmacology and Therapeutics
- Dermatology
- Endocrinology and Diabetes
- Gastroenterology
- Genito-urinary Medicine
- Geriatric Medicine
- Infectious Diseases
- Medical Toxicology
- Medical Oncology
- Pain Medicine
- Palliative Medicine
- Rehabilitation Medicine
- Respiratory Medicine and Pulmonology
- Rheumatology
- Sleep Medicine
- Sports and Exercise Medicine
- Community Medical Services
- Critical Care
- Emergency Medicine
- Forensic Medicine
- Haematology
- History of Medicine
- Browse content in Medical Skills
- Clinical Skills
- Communication Skills
- Nursing Skills
- Surgical Skills
- Medical Ethics
- Browse content in Medical Dentistry
- Oral and Maxillofacial Surgery
- Paediatric Dentistry
- Restorative Dentistry and Orthodontics
- Surgical Dentistry
- Medical Statistics and Methodology
- Browse content in Neurology
- Clinical Neurophysiology
- Neuropathology
- Nursing Studies
- Browse content in Obstetrics and Gynaecology
- Gynaecology
- Occupational Medicine
- Ophthalmology
- Otolaryngology (ENT)
- Browse content in Paediatrics
- Neonatology
- Browse content in Pathology
- Chemical Pathology
- Clinical Cytogenetics and Molecular Genetics
- Histopathology
- Medical Microbiology and Virology
- Patient Education and Information
- Browse content in Pharmacology
- Psychopharmacology
- Browse content in Popular Health
- Caring for Others
- Complementary and Alternative Medicine
- Self-help and Personal Development
- Browse content in Preclinical Medicine
- Cell Biology
- Molecular Biology and Genetics
- Reproduction, Growth and Development
- Primary Care
- Professional Development in Medicine
- Browse content in Psychiatry
- Addiction Medicine
- Child and Adolescent Psychiatry
- Forensic Psychiatry
- Learning Disabilities
- Old Age Psychiatry
- Psychotherapy
- Browse content in Public Health and Epidemiology
- Epidemiology
- Public Health
- Browse content in Radiology
- Clinical Radiology
- Interventional Radiology
- Nuclear Medicine
- Radiation Oncology
- Reproductive Medicine
- Browse content in Surgery
- Cardiothoracic Surgery
- Gastro-intestinal and Colorectal Surgery
- General Surgery
- Neurosurgery
- Paediatric Surgery
- Peri-operative Care
- Plastic and Reconstructive Surgery
- Surgical Oncology
- Transplant Surgery
- Trauma and Orthopaedic Surgery
- Vascular Surgery
- Browse content in Science and Mathematics
- Browse content in Biological Sciences
- Aquatic Biology
- Biochemistry
- Bioinformatics and Computational Biology
- Developmental Biology
- Ecology and Conservation
- Evolutionary Biology
- Genetics and Genomics
- Microbiology
- Molecular and Cell Biology
- Natural History
- Plant Sciences and Forestry
- Research Methods in Life Sciences
- Structural Biology
- Systems Biology
- Zoology and Animal Sciences
- Browse content in Chemistry
- Analytical Chemistry
- Computational Chemistry
- Crystallography
- Environmental Chemistry
- Industrial Chemistry
- Inorganic Chemistry
- Materials Chemistry
- Medicinal Chemistry
- Mineralogy and Gems
- Organic Chemistry
- Physical Chemistry
- Polymer Chemistry
- Study and Communication Skills in Chemistry
- Theoretical Chemistry
- Browse content in Computer Science
- Artificial Intelligence
- Computer Architecture and Logic Design
- Game Studies
- Human-Computer Interaction
- Mathematical Theory of Computation
- Programming Languages
- Software Engineering
- Systems Analysis and Design
- Virtual Reality
- Browse content in Computing
- Business Applications
- Computer Games
- Computer Security
- Computer Networking and Communications
- Digital Lifestyle
- Graphical and Digital Media Applications
- Operating Systems
- Browse content in Earth Sciences and Geography
- Atmospheric Sciences
- Environmental Geography
- Geology and the Lithosphere
- Maps and Map-making
- Meteorology and Climatology
- Oceanography and Hydrology
- Palaeontology
- Physical Geography and Topography
- Regional Geography
- Soil Science
- Urban Geography
- Browse content in Engineering and Technology
- Agriculture and Farming
- Biological Engineering
- Civil Engineering, Surveying, and Building
- Electronics and Communications Engineering
- Energy Technology
- Engineering (General)
- Environmental Science, Engineering, and Technology
- History of Engineering and Technology
- Mechanical Engineering and Materials
- Technology of Industrial Chemistry
- Transport Technology and Trades
- Browse content in Environmental Science
- Applied Ecology (Environmental Science)
- Conservation of the Environment (Environmental Science)
- Environmental Sustainability
- Environmentalist Thought and Ideology (Environmental Science)
- Management of Land and Natural Resources (Environmental Science)
- Natural Disasters (Environmental Science)
- Nuclear Issues (Environmental Science)
- Pollution and Threats to the Environment (Environmental Science)
- Social Impact of Environmental Issues (Environmental Science)
- History of Science and Technology
- Browse content in Materials Science
- Ceramics and Glasses
- Composite Materials
- Metals, Alloying, and Corrosion
- Nanotechnology
- Browse content in Mathematics
- Applied Mathematics
- Biomathematics and Statistics
- History of Mathematics
- Mathematical Education
- Mathematical Finance
- Mathematical Analysis
- Numerical and Computational Mathematics
- Probability and Statistics
- Pure Mathematics
- Browse content in Neuroscience
- Cognition and Behavioural Neuroscience
- Development of the Nervous System
- Disorders of the Nervous System
- History of Neuroscience
- Invertebrate Neurobiology
- Molecular and Cellular Systems
- Neuroendocrinology and Autonomic Nervous System
- Neuroscientific Techniques
- Sensory and Motor Systems
- Browse content in Physics
- Astronomy and Astrophysics
- Atomic, Molecular, and Optical Physics
- Biological and Medical Physics
- Classical Mechanics
- Computational Physics
- Condensed Matter Physics
- Electromagnetism, Optics, and Acoustics
- History of Physics
- Mathematical and Statistical Physics
- Measurement Science
- Nuclear Physics
- Particles and Fields
- Plasma Physics
- Quantum Physics
- Relativity and Gravitation
- Semiconductor and Mesoscopic Physics
- Browse content in Psychology
- Affective Sciences
- Clinical Psychology
- Cognitive Psychology
- Cognitive Neuroscience
- Criminal and Forensic Psychology
- Developmental Psychology
- Educational Psychology
- Evolutionary Psychology
- Health Psychology
- History and Systems in Psychology
- Music Psychology
- Neuropsychology
- Organizational Psychology
- Psychological Assessment and Testing
- Psychology of Human-Technology Interaction
- Psychology Professional Development and Training
- Research Methods in Psychology
- Social Psychology
- Browse content in Social Sciences
- Browse content in Anthropology
- Anthropology of Religion
- Human Evolution
- Medical Anthropology
- Physical Anthropology
- Regional Anthropology
- Social and Cultural Anthropology
- Theory and Practice of Anthropology
- Browse content in Business and Management
- Business Ethics
- Business History
- Business Strategy
- Business and Technology
- Business and Government
- Business and the Environment
- Comparative Management
- Corporate Governance
- Corporate Social Responsibility
- Entrepreneurship
- Health Management
- Human Resource Management
- Industrial and Employment Relations
- Industry Studies
- Information and Communication Technologies
- International Business
- Knowledge Management
- Management and Management Techniques
- Operations Management
- Organizational Theory and Behaviour
- Pensions and Pension Management
- Public and Nonprofit Management
- Strategic Management
- Supply Chain Management
- Browse content in Criminology and Criminal Justice
- Criminal Justice
- Criminology
- Forms of Crime
- International and Comparative Criminology
- Youth Violence and Juvenile Justice
- Development Studies
- Browse content in Economics
- Agricultural, Environmental, and Natural Resource Economics
- Asian Economics
- Behavioural Finance
- Behavioural Economics and Neuroeconomics
- Econometrics and Mathematical Economics
- Economic History
- Economic Methodology
- Economic Systems
- Economic Development and Growth
- Financial Markets
- Financial Institutions and Services
- General Economics and Teaching
- Health, Education, and Welfare
- History of Economic Thought
- International Economics
- Labour and Demographic Economics
- Law and Economics
- Macroeconomics and Monetary Economics
- Microeconomics
- Public Economics
- Urban, Rural, and Regional Economics
- Welfare Economics
- Browse content in Education
- Adult Education and Continuous Learning
- Care and Counselling of Students
- Early Childhood and Elementary Education
- Educational Equipment and Technology
- Educational Strategies and Policy
- Higher and Further Education
- Organization and Management of Education
- Philosophy and Theory of Education
- Schools Studies
- Secondary Education
- Teaching of a Specific Subject
- Teaching of Specific Groups and Special Educational Needs
- Teaching Skills and Techniques
- Browse content in Environment
- Applied Ecology (Social Science)
- Climate Change
- Conservation of the Environment (Social Science)
- Environmentalist Thought and Ideology (Social Science)
- Natural Disasters (Environment)
- Social Impact of Environmental Issues (Social Science)
- Browse content in Human Geography
- Cultural Geography
- Economic Geography
- Political Geography
- Browse content in Interdisciplinary Studies
- Communication Studies
- Museums, Libraries, and Information Sciences
- Browse content in Politics
- African Politics
- Asian Politics
- Chinese Politics
- Comparative Politics
- Conflict Politics
- Elections and Electoral Studies
- Environmental Politics
- European Union
- Foreign Policy
- Gender and Politics
- Human Rights and Politics
- Indian Politics
- International Relations
- International Organization (Politics)
- International Political Economy
- Irish Politics
- Latin American Politics
- Middle Eastern Politics
- Political Behaviour
- Political Economy
- Political Institutions
- Political Theory
- Political Methodology
- Political Communication
- Political Philosophy
- Political Sociology
- Politics and Law
- Public Policy
- Public Administration
- Quantitative Political Methodology
- Regional Political Studies
- Russian Politics
- Security Studies
- State and Local Government
- UK Politics
- US Politics
- Browse content in Regional and Area Studies
- African Studies
- Asian Studies
- East Asian Studies
- Japanese Studies
- Latin American Studies
- Middle Eastern Studies
- Native American Studies
- Scottish Studies
- Browse content in Research and Information
- Research Methods
- Browse content in Social Work
- Addictions and Substance Misuse
- Adoption and Fostering
- Care of the Elderly
- Child and Adolescent Social Work
- Couple and Family Social Work
- Developmental and Physical Disabilities Social Work
- Direct Practice and Clinical Social Work
- Emergency Services
- Human Behaviour and the Social Environment
- International and Global Issues in Social Work
- Mental and Behavioural Health
- Social Justice and Human Rights
- Social Policy and Advocacy
- Social Work and Crime and Justice
- Social Work Macro Practice
- Social Work Practice Settings
- Social Work Research and Evidence-based Practice
- Welfare and Benefit Systems
- Browse content in Sociology
- Childhood Studies
- Community Development
- Comparative and Historical Sociology
- Economic Sociology
- Gender and Sexuality
- Gerontology and Ageing
- Health, Illness, and Medicine
- Marriage and the Family
- Migration Studies
- Occupations, Professions, and Work
- Organizations
- Population and Demography
- Race and Ethnicity
- Social Theory
- Social Movements and Social Change
- Social Research and Statistics
- Social Stratification, Inequality, and Mobility
- Sociology of Religion
- Sociology of Education
- Sport and Leisure
- Urban and Rural Studies
- Browse content in Warfare and Defence
- Defence Strategy, Planning, and Research
- Land Forces and Warfare
- Military Administration
- Military Life and Institutions
- Naval Forces and Warfare
- Other Warfare and Defence Issues
- Peace Studies and Conflict Resolution
- Weapons and Equipment

- < Previous chapter
- Next chapter >

4 Designing a Cost-Effectiveness Analysis
- Published: November 2016
- Cite Icon Cite
- Permissions Icon Permissions
This chapter provides an overview of how to design a cost-effectiveness analysis (CEA). The chapter highlights the importance of early conceptualization and planning steps to define the objectives, the research question, the perspective(s), the intervention(s), the target population, the comparators, the scope, the time horizon, and the analysis plan for a cost-effectiveness study. The chapter recommends that analysts conduct Reference Case analyses from both the healthcare sector perspective and the societal perspective. We also recommend that analysts use an Impact Inventory, which lists the consequences across sectors (e.g., healthcare, education, criminal justice system) affected by an intervention, and that they develop a written protocol that details key aspects of the CEA’s design and conduct.
Signed in as
Institutional accounts.
- GoogleCrawler [DO NOT DELETE]
- Google Scholar Indexing
Personal account
- Sign in with email/username & password
- Get email alerts
- Save searches
- Purchase content
- Activate your purchase/trial code
Institutional access
- Sign in with a library card Sign in with username/password Recommend to your librarian
- Institutional account management
- Get help with access
Access to content on Oxford Academic is often provided through institutional subscriptions and purchases. If you are a member of an institution with an active account, you may be able to access content in one of the following ways:
IP based access
Typically, access is provided across an institutional network to a range of IP addresses. This authentication occurs automatically, and it is not possible to sign out of an IP authenticated account.
Sign in through your institution
Choose this option to get remote access when outside your institution. Shibboleth/Open Athens technology is used to provide single sign-on between your institution’s website and Oxford Academic.
- Click Sign in through your institution.
- Select your institution from the list provided, which will take you to your institution's website to sign in.
- When on the institution site, please use the credentials provided by your institution. Do not use an Oxford Academic personal account.
- Following successful sign in, you will be returned to Oxford Academic.
If your institution is not listed or you cannot sign in to your institution’s website, please contact your librarian or administrator.
Sign in with a library card
Enter your library card number to sign in. If you cannot sign in, please contact your librarian.
Society Members
Society member access to a journal is achieved in one of the following ways:
Sign in through society site
Many societies offer single sign-on between the society website and Oxford Academic. If you see ‘Sign in through society site’ in the sign in pane within a journal:
- Click Sign in through society site.
- When on the society site, please use the credentials provided by that society. Do not use an Oxford Academic personal account.
If you do not have a society account or have forgotten your username or password, please contact your society.
Sign in using a personal account
Some societies use Oxford Academic personal accounts to provide access to their members. See below.
A personal account can be used to get email alerts, save searches, purchase content, and activate subscriptions.
Some societies use Oxford Academic personal accounts to provide access to their members.
Viewing your signed in accounts
Click the account icon in the top right to:
- View your signed in personal account and access account management features.
- View the institutional accounts that are providing access.
Signed in but can't access content
Oxford Academic is home to a wide variety of products. The institutional subscription may not cover the content that you are trying to access. If you believe you should have access to that content, please contact your librarian.
For librarians and administrators, your personal account also provides access to institutional account management. Here you will find options to view and activate subscriptions, manage institutional settings and access options, access usage statistics, and more.
Our books are available by subscription or purchase to libraries and institutions.
- About Oxford Academic
- Publish journals with us
- University press partners
- What we publish
- New features
- Open access
- Rights and permissions
- Accessibility
- Advertising
- Media enquiries
- Oxford University Press
- Oxford Languages
- University of Oxford
Oxford University Press is a department of the University of Oxford. It furthers the University's objective of excellence in research, scholarship, and education by publishing worldwide
- Copyright © 2024 Oxford University Press
- Cookie settings
- Cookie policy
- Privacy policy
- Legal notice
This Feature Is Available To Subscribers Only
Sign In or Create an Account
This PDF is available to Subscribers Only
For full access to this pdf, sign in to an existing account, or purchase an annual subscription.
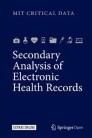
Secondary Analysis of Electronic Health Records pp 351–367 Cite as
Markov Models and Cost Effectiveness Analysis: Applications in Medical Research
- Matthieu Komorowski 2 &
- Jesse Raffa 2
- Open Access
- First Online: 10 September 2016
83k Accesses
16 Citations
This case study describes common Markov models, their specific application in medical research, health economics and cost-effectiveness analysis.
- Markov chain
- Clinical decision making
- Health economics
- Cost-effectiveness analysis
Download chapter PDF
Understand how Markov models can be used to analyze medical decisions and perform cost-effectiveness analysis.
This case study introduces concepts that should improve understanding of the following:
Markov models and their use in medical research.
Basics of health economics.
Replicating the results of a large prospective randomized controlled trial using a Markov Chain and Monte Carlo simulations, and
Relating quality-adjusted life years (QALYs) and cost of interventions to each state of a Markov Chain, in order to conduct a simple cost-effectiveness analysis.
1 Introduction
Markov models were initially theroreticized at the beginning of the 20th century by Russian mathematician Andrey Markov [ 1 ]. They are stochastic processes that undergo transitions from one state to another. Over the years, they have found countless applications, especially for modeling processes and informing decision making, in the fields of physics, queuing theory, finance, social sciences, statistics and of course medicine. Markov models are useful to model environments and problems involving sequential, stochastic decisions over time . Representing such environments with decision trees would be confusing or intractable, if at all possible, and would require major simplifying assumptions [ 2 ]. Markov models can be examined by an array of tools including linear algebra (brute force), cohort simulations, Monte Carlo simulations and, for Markov Decision Processes, dynamic programming and reinforcement learning [ 3 , 4 ].
A fundamental property of all Markov models is their memorylessness . They satisfy a first-order Markov property if the probability to move a new state to s t +1 only depends on the current state \( s_{t} \) , and not on any previous state, where t is the current time. Said otherwise, given the present state, the future and past states are independent. Formally, a stochastic process has the first order Markov property if the conditional probability distribution of future states of the process (conditional on both past and present values) depends only upon the present state:
This chapter will provide a brief introduction to the most common Markov models, and outline some potential applications in medical research and health economics. The last section will discuss a practical example inspired from the medical literature, in which a Markov chain will be used to conduct the cost-effectiveness analysis of a particular medical intervention. In general, the crude results of a study are unable to provide the necessary information to fully implement cost-effectiveness analysis, thus demonstrating the value of expressing the problem as a Markov Chain.
2 Formalization of Common Markov Models
The four most common Markov models are shown in Table 24.1 . They can be classified into two categories depending or not whether the entire sequential state is observable [ 5 ]. Additionally, in Markov Decision Processes, the transitions between states are under the command of a control system called the agent, which selects actions that may lead to a particular subsequent state. By contrast, in Markov chains and hidden Markov models, the transition between states is autonomous. All Markov models can be finite (discrete) or continuous, depending on the definition of their state space.
2.1 The Markov Chain
The discrete time Markov chain, defined by the tuple \( \{ S, T\} \) is the simplest Markov model, where S is a finite set of states and T is a state transition probability matrix, \( T\left( {s^{{\prime }} , s} \right) = P\left( {s_{t + 1} = s^{{\prime }} |s_{t} = s} \right) \) . A Markov chain can be ergodic , if it is possible to go from any state to every other state in finitely many moves. Figure 24.1 shows a simple example of a Markov Chain.
Example of a Markov chain, defined by a set S of finite states {Healthy, Ill} and a transition matrix, containing the probabilities to move from current state s to next state s′ at each iteration
In the transition matrix, the entries in each column are between 0 and 1 (inclusive) and their sum is 1. Such vectors are called probability vectors . The Table 24.2 shows the transition matrix corresponding to Fig. 24.1 . A state is said to be absorbing if it is impossible to leave it (e.g. death).
2.2 Exploring Markov Chains with Monte Carlo Simulations
Monte Carlo (MC) simulations are a useful technique to explore and understand phenomena and systems modeled under a Markov model. MC simulation generates pseudorandom variables on a computer in order to approximate difficult to estimate quantities. It has wide use in numerous fields and applications [ 6 ]. Our focus is on the MC simulation of a Markov chain, and it is straightforward once a transition probability matrix, \( T\left( {s^{{\prime }} , s} \right) \) , and final time t * have been defined. We will assume at the index time ( t = 0), the state is known, and call it s 0 . At t = 1, we simulate a categorical random variable using the s 0 th row of the transition probability matrix \( T\left( {s^{{\prime }} , s} \right) \) . We repeat this \( t = 1,2, \ldots ,t^{*} - 1,t^{*} \) to simulate one simulated instance of the Markov chain we are studying. One simulated instance only tells us about one possible sequence of transitions out of very many for this Markov chain, and we need to repeat this many ( N ) times, recording the sequence of states for each of the simulated instances. Repeating this process many times, allows us to estimate quantities such as: the probability at t = 5, that the chain is in state 1; the average proportion of time spent in state 1 over the first 10 time points; or the average length of the longest consecutive streak in state 1 in the first t * time points.
Using the example shown in Fig. 24.1 , we will estimate the probability for someone to be healthy or ill in 5 days, knowing that he is healthy today. MC methods will simulate a large number of samples (say 10,000), starting in s 0 = Healthy and following the transition matrix \( T\left( {s^{{\prime }} , s} \right) \) for 5 steps, sequentially picking transitions to s′ according to their probability. The output variable (the value of the final state) is recorded for each sample, and we conclude by analyzing the characteristics of the distribution of this output variable (Table 24.3 ).
The distribution of the final state at day + 5 for 10,000 simulated instances is represented on Fig. 24.2 .
Distribution of the health on day 5, for 10,000 instances
Table 24.4 reports some sample characteristics for “healthy” state on day 5 for 100 and 10,000 simulated instances, which illustrates why it is important to simulate a very large number of samples.
By increasing the number of simulated instances, we drastically increase our confidence that the true sample mean falls within a very narrow window (0.83–0.84 in this example). The true mean calculated analytically is 0.838, which is very close to the estimate generated from MC simulation.
2.3 Markov Decision Process and Hidden Markov Models
Markov Decision Processes (MDPs) provide a framework for running reinforcement learning methods. MDPs are an extension of Markov chains, which include a control process. MDPs are a powerful and appropriate technique for modeling medical decision [ 3 ]. MDPs are most useful in classes of problems involving complex, stochastic and dynamic decisions like medical treatment decisions , for which they can find optimal solutions [ 3 ]. Physicians will always need to make subjective judgments about treatment strategies, but mathematical decision models can provide insight into the nature of optimal choices and guide treatment decisions.
In Hidden Markov models (HMMs), the state space is only partially observable [ 7 ]. It is formed by two dependent stochastic processes (Fig. 24.3 ). The first is a classical Markov chain, whose states are not directly observable externally, therefore “hidden.” The second stochastic process generates observable emissions, conditional on the hidden process. Methodology has been developed to decode the hidden states from the observed data and has applications in a multitude of areas [ 7 ].
Example of a hidden Markov model (HMM)
2.4 Medical Applications of Markov Models
MDPs have been praised by authors as being a powerful and appropriate approach for modeling sequences of medical decisions [ 3 ]. Controlled Markov models can be solved by algorithms such as dynamic programming or reinforcement learning, which intends to identify or approximate the optimal policy (set of rules that maximizes the expected sum of discounted rewards).
In the medical literature, Markov models have explored very diverse problems such as timing of liver transplant [ 8 ], HIV therapy [ 9 ], breast cancer [ 10 ], Hepatitis C [ 11 ], statin therapy [ 12 ] or hospital discharge management [ 5 , 13 ]. Markov models can be used to describe various health states in a population of interest, and to detect the effects of various policies or therapeutic choices. For example, Scott et al. has used a HMM to classify patients into 7 health states corresponding to side effects of 2 psychotropic drugs [ 14 ]. The transitions were analyzed to specify which drug was associated with the least side-effects. Very recently, a Markov chain model was proposed to model the progression of diabetic retinopathy, using 5 pre-defined states, from mild retinopathy to blindness [ 15 ]. MDPs have also been exploited in medical imaging applications. Alterovitz has used very large MDPs (800,000 states) for motion planning in image-guided needle steering [ 16 ].
Besides those medical applications, Markov models are extensively used in health economics research, which is the focus of the next section of this chapter.
3 Basics of Health Economics
3.1 the goal of health economics: maximizing cost-effectiveness.
This section provides the reader with a minimal background about health economics, followed by a worked example. Health economics intends to maximize “value for money” in healthcare, by optimizing not only clinical effectiveness, but also cost-effectiveness of medical interventions. As explained by Morris: “ Achieving ‘value for money’ implies either a desire to achieve a predetermined objective at least cost or a desire to maximise [sic] the benefit to the population of patients served from a limited amount of resources ” [ 17 ].
Two main approaches can be outlined in health economics: cost-minimization and cost-effectiveness analysis (CEA). In both cases, the purpose is identical: to identify which treatment option is the most cost-effective. Cost minimization deals with the simple case where the several treatment options available have the same effectiveness but different costs. Quite logically, cost-minimization will favor the cheapest option. CEA represents a more likely scenario and is more widely used. In CEA, several options with different costs and different effectiveness are compared. The analysis will compute the relative cost of an improvement in health, and metrics to optimally inform decision makers.
3.2 Definitions
Measuring Outcome: Survival, Quality of Life (QoL), Quality-Adjusted Life-Years (QALY)
Outcomes are assessed in terms of enhanced survival (“ adding years to life ”) and enhanced quality of life (QoL) (“ adding life to years ”) [ 17 ]. Although sometimes criticized, the concept of Quality-adjusted life-years (QALY) remains of central importance in cost-utility analysis [ 18 ]. QALYs apply weights that reflect the QoL being experienced by the patient. One QALY equates to one year in perfect health. Perfect health is equivalent to 1 while death is equivalent to 0. QALYs are estimated by various methods including scales and questionnaires filled by patients or external examiners [ 19 ]. As an example, the EuroQoL EQ 5D questionnaire assesses health in 5 dimensions: mobility, self-care, usual activities, pain/discomfort and anxiety/depression.
Cost-Effectiveness Ratio (CER)
The cost-effectiveness ratio (CER) will inform the decision makers about the cost of an intervention, relative to the health benefits this intervention generates. For example, an intervention costing $20,000 per patient and providing 5 QALYs (5 years of perfect health) has a CER of $20,000/5 = $4000 per QALY. This measure allows a direct comparison of cost-effectiveness between interventions.
Incremental Cost-Effectiveness Ratio (ICER)
The incremental cost-effectiveness ratio (ICER) is a measure very commonly reported in the health economics literature and allows comparing two different interventions in terms of “cost of gained effectiveness.” It is computed by dividing the difference in cost of 2 interventions by the difference of their effectiveness [ 20 ].
As an example, if treatment A costs $5000 per patient and provides 2 QALYs, and treatment B costs $8000 while providing 3 QALYS, the ICER of treatment B will be:
Said otherwise, it will cost $3000 more to gain one more QALY with treatment B, for this particular medical condition. ICER can inform decision makers about the need to adopt or fund a new medical intervention. Schematically, if the ICER of a new medical intervention lies below a certain threshold, it means that health benefits can be achieved with an acceptable level of spending.
The Cost Effectiveness Plane
The cost-effectiveness plane (CE plane) is an important tool used in CEA (Fig. 24.4 ). It aims to clearly illustrate differences in costs and effects between different strategies, whether they comprise medical interventions, treatments, or even a combination of the two.
The cost-effectiveness plane, comparing treatment A with treatment B
The CE plane consists of a four-quadrant diagram where the X-axis represents the incremental level of effectiveness of an outcome and the Y-axis represents the additional total cost of implementing this outcome. For example, the further right you move on the X-axis, the more effective the outcome. In the upper-right quadrant, a treatment may receive funding if its ICER lies below the maximum acceptable ICER threshold.
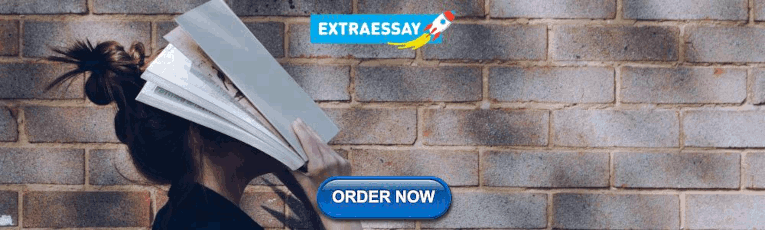
4 Case Study: Monte Carlo Simulations of a Markov Chain for Daily Sedation Holds in Intensive Care, with Cost-Effectiveness Analysis
This example is inspired by the publication by Girard et al. [ 21 ], and will allow us to illustrate how to construct and examine a simple Markov Chain to represent a medical intervention, how to relate QALYs and cost of interventions to each state of the Markov Chain, in order to carry out a cost-effectiveness analysis. In this prospective randomized controlled trial, the authors evaluated the impact of daily sedation holds in intensive care on various outcomes such as the number of ventilator-free days, delirium and 28-day mortality. In the ICU, patients frequently undergo mechanical ventilation in the setting of severely impaired consciousness, after heavy surgical procedures, and when suffering from severe respiratory failure. Therapeutically, patients are sedated to maximize their comfort. A growing body of literature, however, has identified the risks of continuous sedation in the ICU, as it is associated with increased mortality, delirium, duration of mechanical ventilation and length of ICU and hospital stay [ 22 ]. To strike the right balance between maintaining sedation and mechanical ventilator support as long as the patient needs it, but also moving to extubation as soon as possible, Girard and colleagues proposed actively waking up the patients daily to assess their readiness to come off of the ventilator. The main results are shown in Table 24.5 .
In this case study example, we will attempt to approximate those results using a very simple 3-state Markov Chain examined by MC simulation. As an exercise, we will extend the study to CEA. This tutorial will provide the reader with all the tools necessary to implement in other contexts Markov Chain MC simulation methods and simple cost-effectiveness studies.
Most of the study results can be approximated using a very crude 3-state Markov chain (Fig. 24.5 ), with the following state space: {Intubated, Extubated, Dead}. In this simplistic model, only 7 transitions are possible, and the state ‘dead’ is absorbing.
The 3-state Markov chain used in this example
Two different transition matrices can be built by trial-and-error, corresponding to the intervention and control arms of the study (Table 24.6 ). They correspond to the daily probabilities of transitioning from one state to another. The initial values were selected using a few simple assumptions: the state ‘death’ is absorbing, the probability to remain intubated or extubated is larger than the probability to change state, the risk of dying while intubated is larger than when extubated, and the total of each row in the transition matrix is one. Another assumption is that the intervention (daily sedation hold) will change the probability of successful extubation and mortality, hence the transition matrix. After each modification, the number of patients in each state was computed for 28 days (results in Table 24.8 ), so as to try to match the initial study’s results as closely as possible.
We can check to see if our code is running correctly by comparing important aspects of the simulation to known theoretical properties of probability theory and Markov Chains. For example, in our example all patients are assumed to be intubated at t = 0. Under our Markov model, the waiting time until extubation or death can be determined theoretically, but how to determine this is beyond the scope of this chapter. This waiting time, W * , is a discrete random variable with a geometric distribution. Geometric distributions have probability mass functions, for a given waiting time, w of \( p(w) = (1 - p) p ^ {(w - 1)} \) , where p is the probability of remaining intubated. In Fig. 24.6 , we compare the number of times we observed different values of w to what we would expect under the true theoretical distribution of W * , by computing Np ( w ), where N is the number of simulated instances we computed. We can see that our simulation follows very closely to what is theoretically known to be true.
Example of the life expectancy in state “I” in the control group, with fitted geometric distribution. The bar chart represents the distribution of the time spent in the state “intubated” of the Markov chain, before transitioning to another state, for 5000 samples
In order to perform CEA, each state must be assigned a value for QALYs and cost. For the purpose of this example, let’s also assume the values for QALYs and daily costs shown in Table 24.7 .
Table 24.8 shows the results of the first iterations for the control group, when starting with 100 patients intubated ( function IED_transition.m ). At each time step, the number of patients still intubated corresponds to the patients who stayed intubated, minus the patients who became extubated (daily probability of 10 %) and those who died (probability of 2.2 %), plus the extubated patients who had to be re-intubated (probability 1 %). After 28 days, the cumulated mortality reaches 35.6 %, and the ratio of patients extubated among the patients still alive is 88.8 %, hence matching quite closely the results of the initial study. At each time step, the sum of the QALYs and costs for all the patients is computed, as well as their cumulative values. The number of QALYs initially increases as more patients become extubated, then decreases as a consequence the number of patients dying.
The following figure represents the ratio of number of patients extubated over number of patients alive, over time and for both strategies (Fig. 24.7 ). It can be compared to the original figure in the source article.
Modelled primary outcome of the study using a Markov chain
By simulating the distribution of the average number of ventilator-free days, and its characteristics, can be computed for both strategies ( function MCMC_solver.m ). The following Table 24.9 shows examples of patients’ states computed using the transition matrix of the control group.
The distribution of ventilator-free days in our 10,000 samples is plotted shown in Fig. 24.8 .
Ventilator-free days for 10,000 samples, for the intervention and control group
The mean and median number of ventilator-free days for both groups is shown in Table 24.10 .
The cost-effectiveness ratio at 28 day of the both strategies can be computed by dividing the final cumulative cost by the cumulative QALYs (Table 24.11 ).
The intervention is more expensive but is also associated with health benefits (significantly more QALYs). It belongs to the upper-right quadrant of the CE plane, where the ICER is used to determine the cost-effectiveness of an intervention. The ICER of this intervention is shown below:
According to this crude analysis, Sedation holds appear to be a very cost-effective strategy, costing only $177 more per additional QALY, relative to the control strategy. Reducing the value (QALY) of the state E from 1 to 0.6 significantly increases the ICER to $1918 per QALY gained, demonstrating the huge impact that the definition of our health states has on the results of the CEA. Likewise, increasing the daily cost of state E from $1000 to $1900 (now only slightly cheaper than state I) leads to a much more expensive ICER of $2041 per QALY gained. Some medical interventions may or may not be funded depending on the assumptions of the model!
5 Model Validation and Sensitivity Analysis for Cost-Effectiveness Analysis
An important component to any CEA is to assess whether the model is appropriate for the phenomena being examined, which is the purpose of model validation and sensitivity analyses. In the previous section, we model daily sedation hold as a Markov chain with a known transition probability matrix and costs. Deviations from this model can come in at least two types.
First, the use of a Markov Chain may be inappropriate to describe how subjects transition from the intubation, extubation and death states. It was presumed that this process follows a first-order Markov chain. Given enough real clinical data we can test to see if this assumption is reasonable. For example, given the transition probability matrices above, we can calculate quantities via MC simulation and compare them to values reported in the real data. For instance, the authors report a 28-day mortality rate of 29 and 35 % in the intervention and control groups, respectively. From our simulation study, we estimate these quantities to be 27 and 35 %, which is reasonably close. One can perform formal goodness-of-fit testing as well to better assess if any differences noted provide any evidence that the model may be mis-specified. This process can also be repeated for other quantities, for example, the mean number of ventilator-free days.
In addition to validating the Markov model used to simulate the states and transitions for the system of interest, it is also important to perform a sensitivity analysis on the assumptions and parameters used in the simulation. Performing this step allows one to see how sensitive the results are to slight changes to parameter values. Choosing which parameters values to use in sensitivity analyses can be difficult, but some good practices are to find other parameters (e.g., transition probability matrices) reported in other studies of a similar type. For cost estimates, one may want to try costs reported in other countries, or incorporate important economic parameters like inflation. If using these other scenarios drastically affects the conclusions drawn from the simulation study, this does not necessarily mean that the study was a failure, but rather that there are limits to the generalizability of the simulation study’s results. If particular parameters cause great fluctuations this may warrant further investigation into why this is the case. In addition to changing the parameters, one may try to alter the model significantly, by for example, using a higher order Markov model or semi-Markov model in place of a simple first order assumption, but these are advanced topic beyond the scope of this chapter.
The theoretical concepts introduced in the first sections of this chapter were applied to a concrete example coming from the medical literature. We demonstrated how clinical states and transition probabilities could be defined ad hoc, and how the stationary distribution of the chain could be estimated using Monte Carlo methods. The methodology outlined in this chapter will allow the reader to expand the results of other interventional studies to CEA, but countless other applications of Markov models exist, in particular in the domain of decision support systems.
6 Conclusion
Markov models have been used extensively in the medical literature, and offer an appealing framework for modeling medical decision making, with potential powerful applications in decision support systems and health economics analysis. They represent relatively simple mathematical models that are easy to grasp by non-data scientists or non-statisticians. Very careful attention must be paid to the verification of a fundamental assumption which is the Markov property, without which no further analysis should be carried out.
7 Next Steps
This tutorial hopefully provided basic tools to understand or develop CEA and Markov chains to model the effect of medical interventions. For more information on health economics, the reader is directed towards external references, such as the work by Morris and colleagues [ 17 ]. Guidance regarding the use of more advanced Markov models such as MDPs and HMMs is beyond the scope of this book, but numerous sources are available, such as the excellent Sutton and Barto, freely available online [ 4 ].
Basharin GP, Langville AN, Naumov VA (2004) The life and work of A.A. Markov. Linear Algebra Appl 386:3–26
Article Google Scholar
Sonnenberg FA, Beck JR (1993) Markov models in medical decision making: a practical guide. Med Decis Mak Int J Soc Med Decis Mak 13(4):322–338
Article CAS Google Scholar
Schaefer AJ, Bailey MD, Shechter SM, Roberts MS (2005) Modeling medical treatment using Markov decision processes. In: Brandeau ML, Sainfort F, Pierskalla WP (eds) Operations research and health care. Springer, US, pp 593–612
Chapter Google Scholar
Sutton RS, Barto AG (1998) Reinforcement learning: an introduction. A Bradford Book, Cambridge, Mass
Google Scholar
Kreke JE (2007) Modeling disease management decisions for patients with pneumonia-related sepsis [Online]. Available: http://d-scholarship.pitt.edu/8143/
Liu JS (2004) Monte Carlo strategies in scientific computing. Springer, New York
Book Google Scholar
Zucchini W, MacDonald IL (2009) Hidden Markov models for time series: an introduction using R. Chapman and Hall/CRC, Boca Raton (2Rev Ed edition)
Alagoz O, Maillart LM, Schaefer AJ, Roberts MS (2004) The optimal timing of living-donor liver transplantation. Manag Sci 50(10):1420–1430
Shechter SM, Bailey MD, Schaefer AJ, Roberts MS (2008) The optimal time to initiate HIV therapy under ordered health states. Oper Res 56(1):20–33
Maillart LM, Ivy JS, Ransom S, Diehl K (2008) Assessing dynamic breast cancer screening policies. Oper Res 56(6):1411–1427
Daniel PMG, Faissol M (2007) Timing of testing and treatment of hepatitis C and other diseases. Inf J Comput Inf
Denton BT, Kurt M, Shah ND, Bryant SC, Smith SA (2009) Optimizing the start time of statin therapy for patients with diabetes. Med Decis Mak Int J Soc Med Decis Mak 29(3):351–367
Raffa JD, Dubin JA (2015) Multivariate longitudinal data analysis with mixed effects hidden Markov models. Biometrics 71(3):821–831
Article PubMed Google Scholar
Scott SL, James GM, Sugar CA (2005) Hidden Markov models for longitudinal comparisons. J Am Stat Assoc 100:359–369
Srikanth P (2015) Using Markov chains to predict the natural progression of diabetic retinopathy. Int J Ophthalmol 8(1):132–137
PubMed PubMed Central Google Scholar
Alterovitz R, Branicky M, Goldberg K (2008) Motion planning under uncertainty for image-guided medical needle steering. Int J Robot Res 27(11–12):1361–1374
Morris S, Devlin N, Parkin D, Spencer A (2012) Economic analysis in healthcare, 2nd edn. Wiley, Chichester
Nord E, Daniels N, Kamlet M (2009) QALYs: some challenges. Value Health 12(Supplement 1):S10–S15
Torrance GW (1986) Measurement of health state utilities for economic appraisal. J Health Econ 5(1):1–30
Article CAS PubMed Google Scholar
Drummond M, Sculpher M (2005) Common methodological flaws in economic evaluations. Med Care 43(7 Suppl):5–14
PubMed Google Scholar
Girard TD, Kress JP, Fuchs BD, Thomason JWW, Schweickert WD, Pun BT, Taichman DB, Dunn JG, Pohlman AS, Kinniry PA, Jackson JC, Canonico AE, Light RW, Shintani AK, Thompson JL, Gordon SM, Hall JB, Dittus RS, Bernard GR, Ely EW (2008) Efficacy and safety of a paired sedation and ventilator weaning protocol for mechanically ventilated patients in intensive care (awakening and breathing controlled trial): a randomised controlled trial. Lancet Lond Engl 371(9607):126–134
Roberts DJ, Haroon B, Hall RI (2012) Sedation for critically ill or injured adults in the intensive care unit: a shifting paradigm. Drugs 72(14):1881–1916
Download references
Author information
Authors and affiliations.
Massachusetts Institute of Technology, Cambridge, MA, USA
Matthieu Komorowski & Jesse Raffa
You can also search for this author in PubMed Google Scholar
Code Appendix
The code used in this case study is available from the GitHub repository accompanying this book: https://github.com/MIT-LCP/critical-data-book . Further information on the code is available from this website. The following functions are provided:
health_forecast.m : This function computes 100 Monte-Carlo simulations of a 5-day health forecast and displays the results.
IED_transition.m : This function computes and displays the proportion of patients in each state (Intubated, Extubated, or Dead), following the transition matrix in the intervention group.
MCMC_solver.m : This function computes 10,000 Monte Carlo simulations for both the control and intervention group, and computes the distribution of ventilator-free days.
Rights and permissions
Open Access This chapter is distributed under the terms of the Creative Commons Attribution-NonCommercial 4.0 International License ( http://creativecommons.org/licenses/by-nc/4.0/ ), which permits any noncommercial use, duplication, adaptation, distribution and reproduction in any medium or format, as long as you give appropriate credit to the original author(s) and the source, a link is provided to the Creative Commons license and any changes made are indicated.
The images or other third party material in this chapter are included in the work’s Creative Commons license, unless indicated otherwise in the credit line; if such material is not included in the work’s Creative Commons license and the respective action is not permitted by statutory regulation, users will need to obtain permission from the license holder to duplicate, adapt or reproduce the material.
Reprints and permissions
Copyright information
© 2016 The Author(s)
About this chapter
Cite this chapter.
Komorowski, M., Raffa, J. (2016). Markov Models and Cost Effectiveness Analysis: Applications in Medical Research. In: Secondary Analysis of Electronic Health Records. Springer, Cham. https://doi.org/10.1007/978-3-319-43742-2_24
Download citation
DOI : https://doi.org/10.1007/978-3-319-43742-2_24
Published : 10 September 2016
Publisher Name : Springer, Cham
Print ISBN : 978-3-319-43740-8
Online ISBN : 978-3-319-43742-2
eBook Packages : Medicine Medicine (R0)
Share this chapter
Anyone you share the following link with will be able to read this content:
Sorry, a shareable link is not currently available for this article.
Provided by the Springer Nature SharedIt content-sharing initiative
- Publish with us
Policies and ethics
- Find a journal
- Track your research
Cost-Effectiveness Analysis
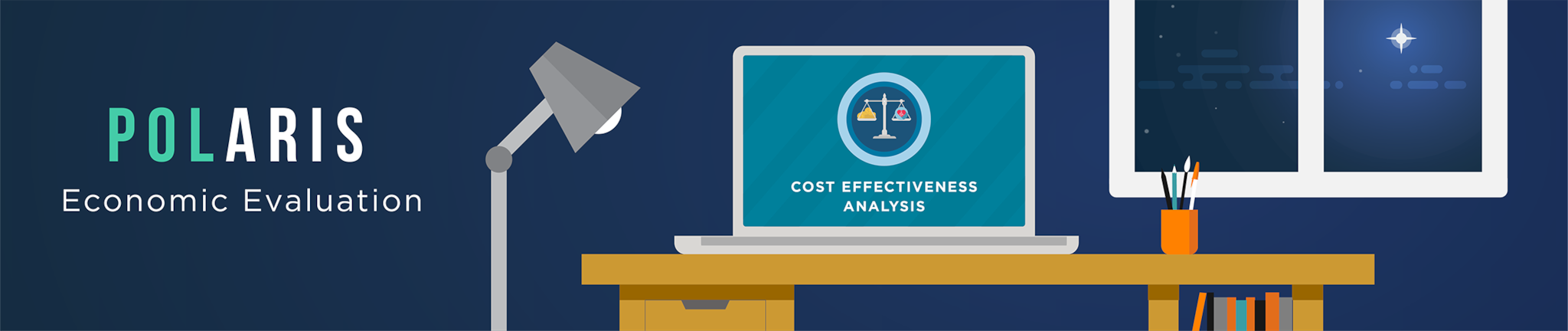
What is cost-effectiveness analysis?
Cost-effectiveness analysis is a way to examine both the costs and health outcomes of one or more interventions. It compares an intervention to another intervention (or the status quo) by estimating how much it costs to gain a unit of a health outcome, like a life year gained or a death prevented.
Because CEA is comparative, an intervention can only be considered cost effective compared to something else.
What inputs are included?
- Net cost is the intervention costs minus averted medical and productivity costs.
- Changes in health outcomes are outcomes with the intervention in place minus outcomes without the intervention in place.
- Examples of health outcomes include heart attacks and deaths from heart disease.
What output does a cost-effectiveness analysis provide?
CEA provides information on health and cost impacts of an intervention compared to an alternative intervention (or the status quo). If the net costs of an intervention are positive (which means a more effective intervention is more costly), the results are presented as a cost-effectiveness ratio. A cost-effectiveness ratio is the net cost divided by changes in health outcomes. Examples include cost per case of disease prevented or cost per death averted. However, if the net costs are negative (which means a more effective intervention is less costly), the results are reported as net cost savings.
CEA Example (Intervention is More Effective and More Costly):
The example below presents the results from a cost-effectiveness analysis of a screening intervention for preventing chlamydia infections among high risk women (compared to the status quo of no screening). The results are presented as a cost-effectiveness ratio. This cost-effectiveness ratio can be compared to another intervention to determine which is more cost-effective.
Sexually Transmitted Diseases
This analysis modeled the intervention as applied to 10000 women
CEA Example (Intervention is More Effective and Less Costly):
In the example below, we compare the childhood vaccination program to the status quo of no vaccination program. We can see that the costs of implementing the program are less than the medical and productivity costs averted. Because the intervention is cost saving, the results are not presented as a cost-effectiveness ratio. Instead, they are presented as net cost savings.
Childhood Vaccination Program
For additional information, please see the example as used in the CDC Introduction to Economic Evaluation in Public Health online training, as well as the original study.
How can information from a CEA be useful for decision makers?
CEA can be useful in comparing the health and cost impacts of different interventions affecting the same health outcome. It can also be useful for understanding how much an intervention may cost (per unit of health gained) compared to an alternative intervention. For example, a decision maker might find it useful to know if an intervention is cost saving, and if not how much more would it cost to implement it compared to a less effective intervention.
CDC Introduction to Economic Evaluation
Course providing a broad overview of economic evaluation methods with illustrative examples from public health
Tufts Medical Center Cost-Effectiveness Analysis Registry
Comprehensive database of cost-effectiveness analyses on a wide variety of diseases and treatment
Exit Notification / Disclaimer Policy
- The Centers for Disease Control and Prevention (CDC) cannot attest to the accuracy of a non-federal website.
- Linking to a non-federal website does not constitute an endorsement by CDC or any of its employees of the sponsors or the information and products presented on the website.
- You will be subject to the destination website's privacy policy when you follow the link.
- CDC is not responsible for Section 508 compliance (accessibility) on other federal or private website.
- Open access
- Published: 29 March 2024
Cost-effectiveness of severe acute malnutrition treatment delivered by community health workers in the district of Mayahi, Niger
- Elisa M. Molanes-López ORCID: orcid.org/0000-0003-3217-8551 1 ,
- José M. Ferrer ORCID: orcid.org/0000-0002-1021-1157 2 ,
- Abdias Ogobara Dougnon 3 ,
- Abdoul Aziz Gado 4 ,
- Atté Sanoussi 5 ,
- Nassirou Ousmane 5 ,
- Ramatoulaye Hamidou Lazoumar 6 &
- Pilar Charle-Cuéllar ORCID: orcid.org/0000-0003-4784-5003 7
Human Resources for Health volume 22 , Article number: 22 ( 2024 ) Cite this article
56 Accesses
1 Altmetric
Metrics details
A non-randomized controlled trial, conducted from June 2018 to March 2019 in two rural communes in the health district of Mayahi in Niger, showed that including community health workers (CHWs) in the treatment of severe acute malnutrition (SAM) resulted in a better recovery rate (77.2% vs. 72.1%) compared with the standard treatment provided solely at the health centers. The present study aims to assess the cost and cost-effectiveness of the CHWs led treatment of uncomplicated SAM in children 6–59 months compared to the standard national protocol.
To account for all relevant costs, the cost analysis included activity-based costing and bottom-up approaches from a societal perspective and on a within-trial time horizon. The cost-effectiveness analysis was conducted through a decision analysis network built with OpenMarkov and evaluated under two approaches: (1) with recovery rate and cost per child admitted for treatment as measures of effectiveness and cost, respectively; and (2) assessing the total number of children recovered and the total cost incurred. In addition, a multivariate probabilistic sensitivity analysis was carried out to evaluate the effect of uncertainty around the base case input data.
For the base case data, the average cost per child recovered was 116.52 USD in the standard treatment and 107.22 USD in the CHWs-led treatment. Based on the first approach, the CHWs-led treatment was more cost-effective than the standard treatment with an average cost per child admitted for treatment of 82.81 USD vs. 84.01 USD. Based on the second approach, the incremental cost-effectiveness ratio of the transition from the standard to the CHWs-led treatment amounted to 98.01 USD per additional SAM case recovered.
Conclusions
In the district of Mayahi in Niger, the CHWs-led SAM treatment was found to be cost-effective when compared to the standard protocol and provided additional advantages such as the reduction of costs for households.
Trial registration : ISRCTN with ID 31143316. https://doi.org/10.1186/ISRCTN31143316
Peer Review reports
Introduction
Acute malnutrition is one of the major public health issues in the Sahel region. According to the World Health Organization (WHO), 38.4 million children under 5 years of age were affected by global acute malnutrition (GAM) in 2020 and of those 8 million had severe acute malnutrition (SAM) [ 1 ]. Children affected by this condition are 11 times more likely to die compared to well-nourished children [ 2 , 3 ]. The Standardized Monitoring and Assessment of Relief and Transition (SMART) survey conducted in Niger in 2022 showed a GAM prevalence of 13.6% (95% CI 11.2–16.4) in the Maradi region of which 3.9% (95% CI 2.5–6.1) SAM and 9.7% (95% CI 7.6–12.3) moderate acute malnutrition (MAM) [ 4 ]. These figures mean that 457 200 children aged 6–59 months suffered from SAM in 2021 [ 5 ].
According to the Community management of acute malnutrition (CMAM) protocol, children suffering from uncomplicated SAM are treated at health centers (HCs), where they receive outpatient treatment with ready-to-use therapeutic food (RUTF) and systemic treatment with amoxicillin (50–100 mg/kg/day twice a day for 5 days) and one single dose of 500 mg of mebendazole at the first visit for deworming. In addition, they receive RUTF every visit throughout the next 6–8 consecutive weeks of follow-up. The Simplified Lot Quality Assurance Sampling Evaluation of Access and Coverage (SLEAC) survey conducted in 2016 showed a treatment coverage of 41.5% in the Maradi region. This assessment outlined several geographical barriers, especially during the hunger gap, when families deplete their food reserves and new crops have not yet been harvested. The challenges include the significant time caregivers spend traveling to or waiting at HCs, misunderstandings about malnutrition, and a lack of funds for transportation. These factors are identified as the primary obstacles contributing to low access to health services [ 6 ]. To address this issue, between 2018 and 2019, a research study was conducted to assess the effectiveness and treatment coverage by incorporating community health workers (CHWs) into health posts (HPs) in addition to the standard SAM treatment provided solely at HCs. The control group received outpatient treatment for uncomplicated SAM by nurses at HCs, while the intervention group received outpatient treatment for uncomplicated SAM by nurses at HCs or by CHWs at HPs. The primary treatment outcome was recovery defined as the absence of bilateral pitting edema (fluid build-up in feet, legs, hands and arms) for 14 days and weight-for-height z -score (WHZ) ≥ −2 and/or mid upper arm circumference (MUAC) ≥ 125 mm, during two consecutive follow-up visits. The results showed a statistically significant difference in recovery rates with 77.2% children recovered in the intervention group (73.1% at HCs and 83.7% at HPs) vs. 72.1% in the control group ( p < 0.001); and a treatment coverage of 61.2% in the intervention group compared to 43.6% in the standard treatment group [ 7 ]. The CHWs-led treatment approach, part of the simplified approaches supported by UNICEF [ 8 ], has also shown its effectiveness and positive impact on coverage in other contexts such as Mali, Mauritania and Tanzania [ 9 , 10 , 11 ].
To plan and implement at scale, policymakers need stronger evidence to support the promising cost-effectiveness of using CHWs in child health-related settings, such as in the case of SAM treatment [ 12 ]. Bringing healthcare delivery closer to families through CHWs directly reduces the time and cost of every medical visit for the household and it is expected that it will also cause children to begin to be treated in better conditions, increasing the probability of recovery and/or reducing the duration of treatment. A study in Mali showed a recovery rate of 94.2% in the intervention group vs. 88.2% in the standard protocol highlighting that the cost per child recovered from SAM with the CHWs-led approach was 259 USD vs. 501 USD of the standard HCs-based treatment protocol (2016 USD). Each week of treatment, households under the CHWs-led approach spent half of the time receiving treatment and three times less money compared to those receiving treatment solely at the health center [ 13 ]. In Pakistan, the centralization of acute malnutrition treatment with lady health workers (CHWs in the country) did not show evidence of being a cost-effective intervention. The recovery rate was 76.0% and 83.0% in the intervention and control group, respectively and the cost per child recovered by implementing lady health workers was similar to cost at HCs (382 vs. 363, in 2016 USD). However, the cost for households receiving SAM treatment at HCs was double than the cost of care provided by lady health workers [ 14 ]. This wide variation in results suggests that cost-effectiveness may be influenced not only by the service delivery model of treating acute malnutrition in the community, but also by other factors such as the burden of acute malnutrition and the expected number of children suffering from the disease; and the quality of care and number of children recovered due to treatment delivered by these CHWs [ 15 ]. According to the Global Action Plan against Child Wasting, it is crucial to further analyze the cost-effectiveness of interventions to increase treatment coverage and achieve a reduction of the prevalence of wasting to less than 5% by 2025 [ 16 ].
The present study aims to analyze the costs and cost-effectiveness of SAM treatment delivered by CHWs compared to the standard protocol from a societal perspective in the Mayahi district of the Maradi region in Niger. This economic evaluation will be conducted on a within-trial time horizon for both cost and effectiveness results, and following the Consolidated Health Economic Evaluation Reporting Standards (CHEERS) guidelines [ 17 ] (see Additional file 1 ).
Methodology
Description of the context and intervention.
A non-randomized controlled trial was conducted from June 2018 to March 2019 in the health district of Mayahi, of the region of Maradi in Niger. It included two rural communes, Maireyrey (the control area) and Guidan Amoumoune (the intervention area). According to the 2012 population census [ 18 ], Maireyrey and Guidan Amoumoune had, respectively, 64 183 and 88 199 inhabitants. Figure 1 shows a map of the two study areas with the location of the HCs and HPs. Socio-demographic characteristics in both areas were similar, for example family sample size, proportion of women, source of water in the household, HCs as the first option for caring children, among others. The intervention group appeared to have houses with better roofing and reported distance to HCs as the main barrier to health access [ 7 ].
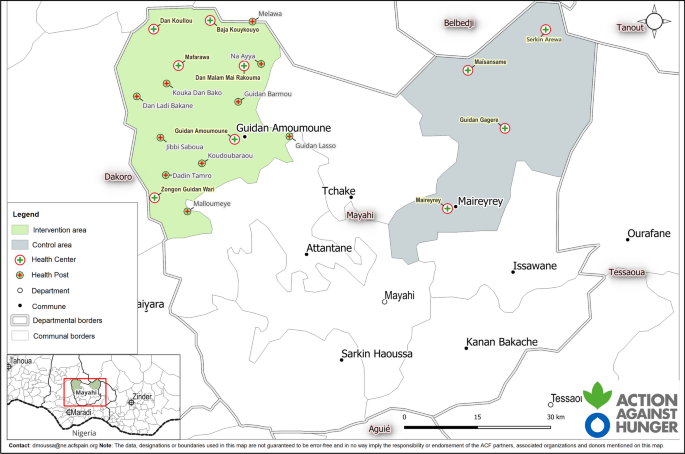
Sanitary map of the two study areas
Prior to the start of the study, treatment of acute malnutrition was carried out by nurses at HCs, decentralized treatment at HPs with CHWs not being allowed by the administrators of the country’s SAM policy. All children 6–59 months who attended HCs or HPs and met the inclusion criteria were recruited in the study. The inclusion criteria were the presence of mild (+) or moderate (++) edema and/or a WHZ less than − 3 and/or a MUAC less than 115 mm [ 19 ]. Cases with severe edema, medical complications, or failed appetite tests were excluded from the study and referred for inpatient treatment. Outpatient treatment for uncomplicated SAM was provided for a maximum of 8 weeks (initial visit plus seven follow-up weekly visits) by nurses at the 4 existing HCs in the control group (standard treatment), and by nurses at the 6 existing HCs and 10 additional CHWs located at HPs in the intervention group (CHWs-led treatment). At the end of the treatment, the final nutritional recovery status of the children is assessed. The Ministry of Health provided treatment, UNICEF supplied RUTF while Action against Hunger (AAH) supervised activities.
Data collection
Treatment outcome data were obtained from the primary study [ 7 ]. Field data collection for the economic component was conducted between June 2019 in Niamey and August 2019 in Mayahi. Data on cost and resource usage were collected from (1) nurses providing SAM treatment at HCs; (2) CHWs at HPs; (3) AAH staff and partners involved in support, supervision, management and logistics; (4) caregivers of SAM children.
Financial and accounting costs of AAH Niger and study financial records were used as primary data sources. HCs staff, CHWs and project key informants including AAH staff and relevant partners were selected through deliberate sampling and interviewed using semi-structured interviews to map activities and to allocate the time for their implementation.
A total of 18 semi-structured interviews were carried out which included five CHWs, five nurses responsible for the HCs, one regional nutrition focal point, one Chief District Medical Officer, one financial District Officer, one doctor from the Ministry of Health responsible for inpatient treatment of SAM, and four AAH staff comprising a supervisor and the heads of the finance, logistics and human resources (HR) departments in Niamey and Maradi. These interviews allowed us to identify the resources used for the treatment of SAM at the HCs and HPs including but not limited to RUTF, drugs, medical equipment and consumables. Moreover, 16 focus group discussions were conducted: seven with community volunteers and nine involving children’s caregivers, who were enabled to gather data on the costs incurred by households for seeking care, and on the associated opportunity costs resulting from loss of income.
The tasks and activities linked to the provision of treatment such as consultations, training, and supervision of CHWs were mapped out and the corresponding time allocation for each activity was collected. Costs were calculated triangulating both accounting records and information obtained through key informant interviews.
Data analysis
The cost analysis is conducted using a combination of activity-based costing and a bottom-up approach, in line with the classification proposed by Njuguna et al. [ 20 ] on a within-trial time horizon. Besides, a societal perspective is employed to assess the impact of incorporating CHWs on household costs. Consequently, since opportunity costs associated with family income losses are included, our analysis considers economic and not just financial costs [ 21 ].
The allocation of fixed costs to activities is determined using activity-based costing, and the specific details are provided in Table 1 . Fixed costs include the costs independent of the number of children admitted for treatment and comprise activities grouped in the following categories: Supervision, Staff support and HPs implementation. In addition, HPs implementation category includes the following subcategories: Management and coordination, Training, HPs procurement and RUTF logistics. On the one hand, Supervision and Staff support categories’ costs are common to the entire program, and they must be distributed between control and intervention groups according to the population size of the areas. An exception was considered in the Monthly monitoring activity of the Supervision category as the number of supervisors involved differed. In specific, the control group had one supervisor while the intervention group had two, resulting in a double cost for the intervention group in this activity. On the other hand, all costs in the HPs implementation category are allocated entirely to the intervention group.
The bottom-up approach is used to compute variable costs, which are those dependent on the number of children admitted for treatment and/or the number of medical visits attended. Variable costs include the following categories: Transport, Opportunity costs, RUTF procurement, Healthcare delivery HR and Hospital referral. In each of these categories, the unit cost is multiplied by the number of medical visits attended, except for the Hospital referral category. In this case, the unit cost (which includes transport, care during inpatient treatment and opportunity cost for families) is multiplied by the number of children admitted for treatment but later transferred to the hospital due to medical complications developed during the follow-up. To determine the total cost of transport, opportunity and healthcare delivery HR for each group, the initial and final visits are added to the follow-up visits per each child. RUTF procurement total costs were calculated considering the initial and follow-up visits but excluding the final visit. Table 1 also outlines which parties are responsible for the costs associated with each activity and/or category.
Research costs related to investigator salaries and study registration are not included. All costs are reported in CFA Francs and converted to US Dollars using the January 2019 exchange rate (1 US Dollar = 575 CFA Francs). Since all costs were measured within a 1-year period, no discounting or inflation adjustments are applied, and it is assumed that no capitalization has occurred.
- Cost-effectiveness
To carry out the cost-effectiveness analysis of our data, the decision analysis network (DAN) presented in Figure 2 was developed in OpenMarkov (version 0.4.0), an open-source software package for probabilistic graphical models (PGMs), developed by the Research Centre for Intelligent Decision-Support Systems (CISIAD) at the Universidad Nacional de Educación a Distancia (UNED) in Madrid, Spain [ 22 , 23 , 24 ]. OpenMarkov has recently been applied in several medical cost-effectiveness analyses [ 25 , 26 , 27 ]. More specific details are given in Additional file 2 .
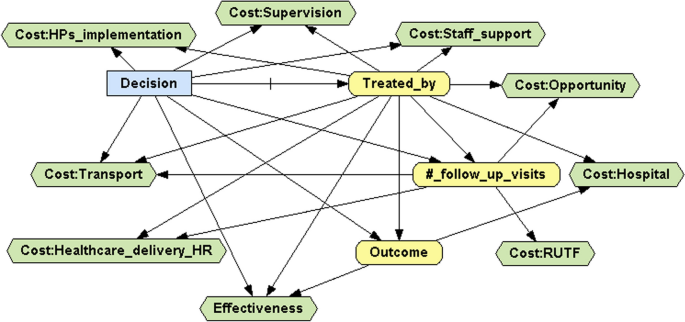
Decision analysis network
Based on this DAN, two approaches were considered. In the first approach, the measure of effectiveness was the recovery rate and the cost was measured per child admitted for treatment, as in the works of Rogers et al. [ 13 , 14 ]. Note that both cost and effectiveness measures are normalized to a per-patient basis. In the second approach, the measures of effectiveness and cost were, respectively, the number of children recovered and the total cost, as in the works of Johns et al. [ 28 ] and Wilunda et al. [ 11 ]. An advantage of this approach is that it considers the increased coverage attained when the treatment is delivered by CHWs at HPs, thus effectively addressing barriers to accessing health care. To ensure the fairness of the comparison, the total cost and the number of children recovered from the intervention area were rescaled to the population size of the control area, as was done previously by Zeng et al. [ 29 , 30 ]. To compare the control and intervention treatments in terms of cost-effectiveness, the average cost-effectiveness ratio (ACER) of each treatment and the incremental cost-effectiveness ratio (ICER) were calculated [ 31 ].
Sensitivity analysis
A multivariate probabilistic sensitivity analysis based on 1000 Monte Carlo simulations was performed under each approach to assess how changes in the input data affected the base case results. Dirichlet distributions were used for probabilities (three yellow nodes in Fig. 2 ), assuming standard deviations less than 0.1. Triangular symmetric distributions were used for those costs that were independent of the number of follow-up visits (Cost:Supervision, Cost:Staff_support, Cost:HPs_implementation and Cost:Hospital nodes), with the interval endpoints set at 10% from the mode. In contrast, normal distributions were used for those variable costs that were dependent on the number of follow-up visits (Cost:Transport, Cost:Opportunity, Cost:RUTF and Cost:Health_delivery_HR nodes), with standard deviations equal to 10% of the corresponding means. Illustrative examples of how uncertainty was introduced into the DAN presented in Fig. 2 , depending on the nature of each node, are given in Additional file 3 .
Table 1 presents the input costs for the base case, along with the relative percentage of each cost category and subcategory by group.
The transport cost per visit was 3.48 USD (2 h round trip on average) in the control group while in the intervention group it varied from 1.74 USD (1 hour round trip on average) for children treated at HCs to 1.30 USD (45-min round trip on average) for children treated at HPs. The opportunity cost per visit for children treated at HCs was 0.65 USD in both the control group and the intervention group, while for children treated at HPs this opportunity cost was reported by focus group participants to be null. The cost of the healthcare delivery HR was 0.65 USD per visit for children treated by nurses at HCs and only 0.18 USD for children treated by CHWs at HPs. To obtain these values, we have taken into account that visits lasted on average 20 min and that the monthly salaries of nurses and CHWs were, respectively, 313.04 USD and 86.96 USD. The RUTF procurement cost was 4.96 USD per visit across all groups. The unit cost per child transferred to the hospital is 131.95 USD in both groups. In the control group, 14 children were transferred, while in the intervention group, 27 children were transferred, with 20 treated at HCs and 7 at HPs.
The group frequencies and percentages of the follow-up visits are presented in Table 2 . Note that children with 0 follow-up visits are those who were admitted for treatment in the initial visit but did not attend any follow-up visits for some reason.
Table 3 presents the base case cost-effectiveness results. According to the first approach, the recovery rate was 72.1% in the control group and 77.2% in the intervention group [ 7 ]. The average cost per child admitted for treatment was 84.01 USD in the control group and 82.81 USD in the intervention group. These results showed that the CHWs-led treatment dominates the standard treatment, since it provided better outcomes according to both indicators. However, according to the second approach, the CHWs-led treatment was not only more effective than the standard treatment but also more expensive. The ACER, calculated following either approach, was 116.52 USD per child recovered in the control group and 107.22 USD in the intervention group.
As part of the second approach, the ICER was calculated at 98.01 USD, implying that having one additional child recovered in the rescaled intervention group required an additional cost of 98.01 USD compared to the control group. The rescaling factor was 0.7277 (64,183/88,199) while the ICER value of 98.01 USD was obtained from the results collected in Table 3 :
where \(\mathrm{119,141.38}=0.7277\times \mathrm{163,721.71}\) and \(\mathrm{1,111.21}=0.7277\times 1527.\) This result signifies that, compared to the control group, approximately 558 more children recovered in the rescaled intervention group at an additional cost of 54,708.33 USD.
Figure 3 shows the results of the multivariate probabilistic sensitivity analysis carried out under each approach. In the cost-effectiveness plane, each pair of blue and red points represents the cost and the effectiveness corresponding to the control group (in blue) and the intervention group (in red) of one Monte Carlo simulation. This cost-effectiveness plane provides a clear image of the uncertainty introduced in the input data. Greater concentration of points indicates reduced uncertainty, whereas increased scattering of points indicates greater uncertainty. The cost-effectiveness plane allows the calculation of the percentage of simulations where one treatment is cost-effective compared to the other based on a specific willingness to pay (WTP) value. Interestingly, under the first approach, the probability of being cost-effective is always higher for the intervention group than the control group, independently of the WTP value. However, this changes under the second approach, where (1) for a WTP value smaller than 98.01 USD, which coincides with the ICER of the base case, the standard treatment has more probability of being cost-effective than the CHWs-led treatment; (2) for values above 98.01 USD the probability increases for the CHWs-led treatment; and (3) when the WTP value was 98.01 USD, both treatments have the same probability of being cost-effective.
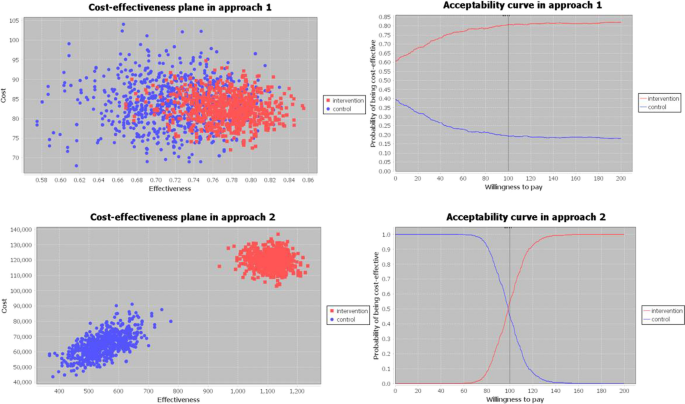
Sensitivity analysis graphs
This analysis showed that CHWs-led treatment in Niger is a cost-effective intervention, compared to the standard protocol delivered solely at HCs, which is consistent with the findings from previous studies in other contexts. In terms of costs, RUTF procurement was the category with the highest cost, representing 34.7% of the total cost in the control group and 31.7% in the intervention group. This proportion was similar to the one obtained in Malawi [ 32 ], lower than in Tanzania [ 11 ], higher than Pakistan, where cost related to RUTF represents 15.2% in the control and 15.7% in the intervention group [ 14 ], and much higher than Mali, where the cost is 6.0% and 11.8% in the control and intervention group, respectively [ 13 ]. Transport was the second highest cost category for the control group reaching 28.5% but represented only 11.9% of the total cost for the intervention group. This difference can be explained by three main factors. First, the location of HPs and therefore the reduced distance to health services in the intervention area compared to the control area. Second, the fixed costs being much higher in the intervention group (47.6%) compared to the control group (23.3%) due to the implementation of the HPs, and consequently, the variable costs categories, such as Transport, having less relative relevance for the intervention group than for the control group. Third, as presented in Table 2 , children in the control group required more follow-up visits to reach recovery than those in the intervention group (4.88 vs. 4.30 on average). In specific, the study found a higher number of children in the control group who needed to attend at least 6 follow-up visits to be discharged as recovered. The most plausible explanation for the difference in the number of visits among groups is that children from the control group accessed treatment later and in a worse clinical condition [ 7 ]. This finding supports the hypothesis that CHWs facilitate early identification and treatment of children leading to a shorter average length of stay and, consequently, reducing the variable costs, including the transport cost [ 33 ]. Regarding the costs of the healthcare delivery HR, they constitute less than 6% of total costs in both groups. However, these costs represent a lower percentage in the intervention group (3.6%) than in the control group (5.3%).
The costs were distributed among the different payers as follows: 11.9% by the Ministry of Health, 53.8% by NGOs and 34.3% by the households in the control group; 7.7% by the Ministry of Health, 77.0% by NGOs and 15.3% by the households in the intervention group. NGOs incurred the highest cost in both groups while communities incurred the lowest costs, which aligns with the findings of other studies conducted previously [ 13 , 14 ]. The cost to implement the intervention increased the NGOs’ cost percentage in the intervention group compared to the control group.
In our study, the costs per child admitted to treatment (82.81 USD) and recovered (107.22 USD) in the intervention group are among the lowest reported in programs where CHWs support the treatment of SAM in Africa. For example, these costs were 166.31 USD and 179.40 USD in Ethiopia [ 34 ], 146.50 USD and 161.77 USD in Tanzania [ 11 ] and 259.91 USD and 275.89 USD in Mali [ 13 ]. This lower cost in our study could be influenced by the higher number of children admitted in Niger, which is 1,977, with fixed costs being shared. In the three other studies, less than 400 children under five were admitted in the intervention group. However, although we have expressed all these costs in 2019 US dollars, comparing CMAM programs can be challenging due to the differences in methodologies, timelines, ways of implementation and data collection.
In Niger, the treatment of acute malnutrition is free of charge for communities. However, during the treatment community members incur expenses linked to transport costs to reach health services and the corresponding opportunity costs associated with seeking treatment. Our study showed that a CHWs-led treatment decreased these expenses. The cost per child admitted for treatment in the control group amounted to 28.74 USD for the households, whereas in the intervention group it was 12.62 USD, less than half of the control group's cost. This difference slightly increases when comparing the cost for the households per child recovered (39.86 USD in the control group vs 16.34 USD in the intervention group). Regarding the cost per visit, in the control group the households that received treatment at HCs spent an average of 4.13 USD. In contrast, within the intervention group, households spent 2.39 USD per visit at HCs and 1.30 USD at HPs. These differences may be explained by the greater proximity to health services in the intervention area, which is one of the main arguments in favor of the CHWs-led treatment approach. Similar findings were presented in Mali [ 13 ], where households whose children received treatment from CHWs spent on average three times less money. In the case of Pakistan, the treatment with the lady health workers did not lead to cost savings for households [ 14 ]. This significant reduction in cost reported in our study could enable not only an increase in provision and access to health services, but also, from a societal perspective, cost savings that could free resources for other purposes, and time savings from reduced treatment, meaning that caregivers and patients can use the time for other activities.
Our findings indicate that SAM treatment delivered by CHWs is a cost-effective intervention compared to the standard treatment, with an additional cost of 98.01 USD per each additional child recovered. Implementing this program over a longer period of time could enhance its cost-effectiveness since some of the fixed costs would be diluted over time. For example, if the program had been continued long enough for the number of children admitted to double, and assuming that fixed costs had not increased, the projected cost per child admitted for treatment in the intervention (control) group would be 63.11 USD (74.23 USD), and the projected cost per child recovered would be 81.71 USD (102.96 USD). In addition, the projected ICER under the second approach would be 60.66 USD, which is 38% less than the ICER calculated in the base case. In the same way, if the program had been continued long enough for the number of children admitted to quintuple, the projected ICER would be 38.26 USD, 61% less than in the base case.
According to our first approach, in contrast with the findings reported by Rogers et al. in the Sindh Province of Pakistan [ 14 ], our intervention group had better outcomes in terms of both recovery rates and cost per child admitted for treatment compared to the control group, which was also reflected by the smaller ACER in the intervention group.
The second approach proved particularly relevant in scenarios where the new intervention effectively tackles barriers of access to healthcare, enabling a greater number of children to be admitted for treatment at earlier stages of severity, resulting in shorter recovery times and reduction in variable costs. According to this second approach, rescaling the measures of effectiveness and cost, namely the total number of children recovered and total cost incurred, based on the population sizes, ensured a fairer comparison and should be considered in similar cost-effectiveness studies. However, this aspect has been at times overlooked in existing literature.
The second approach also provided an additional advantage over the first approach by conveying a clear message, particularly valuable for policymakers and donors, regarding the additional cost necessary to achieve the recovery of an additional child through the CHWs-led treatment compared to the standard treatment. This approach considered the increased number of children admitted for treatment as well as the higher recovery rate in the CHWs-led treatment while the first approach only took into account the recovery rate, independently of the number of children admitted for treatment.
This research presents several strengths. Concerning costs, the most important is the use of a societal perspective, which incorporates household costs, emphasizing the cost savings for families resulting from the inclusion of CHWs. Besides, the combination of activity-based costing and a bottom-up approach has allowed us to calculate variable costs according to the number of children admitted for treatment and the number of follow-up visits made. Regarding the cost-effectiveness analysis, the use of two different methodological approaches has made it possible for our study to be comparable across a wider group of studies and to yield significant results that would remain hidden if only the first approach had been used.
The study also presents two important limitations. First, the data come from a non-randomized control trial, which does not allow us to assume comparability between the two groups. In addition, the potential impact of the difference in the population size between the groups has been minimized by rescaling the data to calculate the ICER. Second, some costs such as RUTF, transportation costs, community volunteer salaries and material for medical appointments were considered for HPs but not for HCs in both control and intervention groups. The absence of these costs, for which data were not available, may have slightly biased the results in favor of the control group.
Considering the substantial number of children affected by acute malnutrition every year, and the geographical, economic and social barriers to health service delivery, new approaches are necessary to increase treatment coverage. Given the limited availability of resources, it is crucial for the Niger Ministry of Health and international stakeholders to prioritize their interventions. The present study aligns with the available evidence regarding the effectiveness of the CHWs-led treatment as one of the proposed simplified approaches also showing its ability to reduce expenses for families. Policymakers can consider these results when making decisions about the implementation of this new approach to tackle the impact of acute malnutrition.
Availability of data and materials
The datasets used and/or analyzed during the current study are available from the corresponding author on reasonable request.
World Health Organization, United Nations Children’s Fund (UNICEF), World Bank. Levels and trends in child malnutrition: UNICEF/WHO/The World Bank Group joint child malnutrition estimates: key findings of the 2021 edition [Internet]. World Health Organization; 2021 [cited 2024 Jan 27]. Available from: https://iris.who.int/handle/10665/341135
Black RE, Victora CG, Walker SP, Bhutta ZA, Christian P, de Onis M, et al. Maternal and child undernutrition and overweight in low-income and middle-income countries. The Lancet. 2013;382:427–51.
Article Google Scholar
Olofin I, McDonald CM, Ezzati M, Flaxman S, Black RE, Fawzi WW, et al. Associations of suboptimal growth with all-cause and cause-specific mortality in children under five years: a pooled analysis of ten prospective studies. PLoS ONE. 2013;8: e64636.
Article CAS PubMed PubMed Central Google Scholar
SMART. Enquête nutritionnelle et de mortalité retrospective au Niger [Internet]. 2022. Available from: https://www.stat-niger.org/wp-content/uploads/nutrition/RAPPORT_SMART_NUTRITION_Niger_2022_INS.pdf
United Nations Office for the Coordination of Humanitarian Affairs. Niger [Internet]. OCHA. 2022 [cited 2024 Jan 27]. Available from: https://www.unocha.org/niger
Simplified Lot Quality Assurance Sampling Evaluation of Access and Coverage survey [Internet]. 2022. Available from: https://acutemalnutrition.org/en/coverage
Ogobara Dougnon A, Charle-Cuéllar P, Toure F, Aziz Gado A, Sanoussi A, Lazoumar RH, et al. Impact of integration of severe acute malnutrition treatment in primary health care provided by community health workers in rural Niger. Nutrients. 2021;13:4067.
Article PubMed PubMed Central Google Scholar
UNICEF. Simplified Approaches [Internet]. 2020. Available from: https://www.simplifiedapproaches.org/es/what-are-simplified-approaches
Alvarez Morán JL, Alé GBF, Charle P, Sessions N, Doumbia S, Guerrero S. The effectiveness of treatment for Severe Acute Malnutrition (SAM) delivered by community health workers compared to a traditional facility based model. BMC Health Serv Res. 2018;18:207.
Charle-Cuéllar P, Lopez-Ejeda N, Toukou Souleymane H, Yacouba D, Diagana M, Dougnon AO, et al. Effectiveness and coverage of treatment for severe acute malnutrition delivered by community health workers in the Guidimakha Region. Mauritania Children. 2021;8:1132.
Article PubMed Google Scholar
Wilunda C, Mumba FG, Putoto G, Maya G, Musa E, Lorusso V, et al. Effectiveness of screening and treatment of children with severe acute malnutrition by community health workers in Simiyu region, Tanzania: a quasi-experimental pilot study. Sci Rep. 2021;11:2342.
Vaughan K, Kok MC, Witter S, Dieleman M. Costs and cost-effectiveness of community health workers: evidence from a literature review. Hum Resour Health. 2015;13:71.
Rogers E, Martínez K, Morán JLA, Alé FGB, Charle P, Guerrero S, et al. Cost-effectiveness of the treatment of uncomplicated severe acute malnutrition by community health workers compared to treatment provided at an outpatient facility in rural Mali. Hum Resour Health. 2018;16:12.
Rogers E, Guerrero S, Kumar D, Soofi S, Fazal S, Martínez K, et al. Evaluation of the cost-effectiveness of the treatment of uncomplicated severe acute malnutrition by lady health workers as compared to an outpatient therapeutic feeding programme in Sindh Province, Pakistan. BMC Public Health. 2019;19:84.
Action against Hunger, Save the Children. The Cost-efficiency and Cost-effectiveness of the Management of Wasting in Children: A review of the evidence, approaches, and lessons [Internet]. Save Child. Resour. Cent. 2020 [cited 2024 Jan 27]. Available from: https://resourcecentre.savethechildren.net/document/cost-efficiency-and-cost-effectiveness-management-wasting-children-review-evidence/
World Health Organization. Global action plan on child wasting: a framework for action to accelerate progress in preventing and managing child wasting and the achievement of the Sustainable Development Goals [Internet]. 2020. Available from: https://www.who.int/publications/m/item/global-action-plan-on-child-wasting-a-framework-for-action
Husereau D, Drummond M, Augustovski F, De Bekker-Grob E, Briggs AH, Carswell C, et al. Consolidated Health Economic Evaluation Reporting Standards (CHEERS) 2022 Explanation and Elaboration: a report of the ISPOR CHEERS II Good Practices Task Force. Value Health. 2022;25:10–31.
Brinkhoff T. City Population [Internet]. [cited 2024 Jan 27]. Available from: https://www.citypopulation.de/en/niger/admin/
World Health Organization. WHO Child Growth Standards—Length/Height-for-age, Weight-for-age, Weight-for-length, Weight-for-height and Body Mass Index-for age: Methods and Development [Internet]. Geneva: World Health Organization; 2006. Available from: https://www.who.int/publications-detail-redirect/924154693X
Njuguna RG, Berkley JA, Jemutai J. Cost and cost-effectiveness analysis of treatment for child undernutrition in low- and middle-income countries: a systematic review. Wellcome Open Res. 2020;5:62.
Turner HC, Sandmann FG, Downey LE, Orangi S, Teerawattananon Y, Vassall A, et al. What are economic costs and when should they be used in health economic studies? Cost Eff Resour Alloc. 2023;21:31.
Arias M, Pérez-Martín J, Luque M, Díez FJ. OpenMarkov, an Open-Source Tool for Probabilistic Graphical Models. Proc Twenty-Eighth Int Jt Conf Artif Intell [Internet]. Macao, China: International Joint Conferences on Artificial Intelligence Organization; 2019 [cited 2024 Jan 27]. p. 6485–7. Available from: https://www.ijcai.org/proceedings/2019/931
Díez FJ, Luque M, Bermejo I. Decision analysis networks. Int J Approx Reason. 2018;96:1–17.
Díez FJ, Luque M, Arias M, Pérez-Martín J. Cost-effectiveness analysis with unordered decisions. Artif Intell Med. 2021;117: 102064.
Pérez-Martín J, Artaso MA, Díez FJ. Cost-effectiveness of pediatric bilateral cochlear implantation in Spain: cost-effectiveness of pediatric BCI in Spain. Laryngoscope. 2017;127:2866–72.
Faccioli N, Santi E, Foti G, Mansueto G, Corain M. Cost-effectiveness of introducing cone-beam computed tomography (CBCT) in the management of complex phalangeal fractures: economic simulation. Musculoskelet Surg. 2022;106:169–77.
Article CAS PubMed Google Scholar
Faccioli N, Santi E, Foti G, D’Onofrio M. Cost-effectiveness analysis of including contrast-enhanced ultrasound in management of pancreatic cystic neoplasms. Radiol Med (Torino). 2022;127:349–59.
Johns B, Probandari A, Mahendradhata Y, Ahmad RA. An analysis of the costs and treatment success of collaborative arrangements among public and private providers for tuberculosis control in Indonesia. Health Policy. 2009;93:214–24.
Zeng W, Pradhan E, Khanna M, Fadeyibi O, Fritsche G, Odutolu O. Cost-effectiveness analysis of the decentralized facility financing and performance-based financing program in Nigeria. J Hosp Manag Health Policy. 2022;6:13–13.
Zeng W, Shepard DS, Nguyen H, Chansa C, Das AK, Qamruddin J, et al. Cost-effectiveness of results-based financing, Zambia: a cluster randomized trial. Bull World Health Organ. 2018;96:760–71.
Hoch JS, Dewa CS. A clinician’s guide to correct cost-effectiveness analysis: think incremental not average. Can J Psychiatry. 2008;53:267–74.
Wilford R, Golden K, Walker DG. Cost-effectiveness of community-based management of acute malnutrition in Malawi. Health Policy Plan. 2012;27:127–37.
López-Ejeda N, Charle-Cuellar P, Alé F, Álvarez JL, Vargas A, Guerrero S. Bringing severe acute malnutrition treatment close to households through community health workers can lead to early admissions and improved discharge outcomes. PloS One. 2020;15: e0227939.
Tekeste A, Wondafrash M, Azene G, Deribe K. Cost effectiveness of community-based and in-patient therapeutic feeding programs to treat severe acute malnutrition in Ethiopia. Cost Eff Resour Alloc. 2012;10:4.
Download references
Acknowledgements
The authors would like to thank all partners who contributed to this study, the Ministry of Health of Niger through the Directorate of Nutrition, Centre de Recherche Médicale et Sanitaire (CERMES) Niamey and all the organizations of the Technical Advisory Group. Our thanks to all the mothers of the children who participated in the study for their time, to the community health workers for their work, to the community leaders for their involvement, to the health staff of the Maradi regional public health directorate and the Mayahi health district for their support, to the entire Action Against Hunger team of Niger mission for their commitment with this intervention. A special mention must go to Dieynaba N'Diaye, who designed the study, conducted and supervised all the fieldwork. Finally, we would like to thank the two referees for their valuable comments that improved our manuscript.
All the actions in the field were supported by funds coming from the Office of U.S. foreign disaster assistance (OFDA/USAID) Award No. AID-OFDAG-17-00277, by the Government of Spain, Grant: PID2019-108679RBI00 13039/501100011033 and UNICEF for write up and publication.
Author information
Authors and affiliations.
Department of Statistics and Operational Research, Faculty of Medicine, Universidad Complutense de Madrid (UCM), 28040, Madrid, Spain
Elisa M. Molanes-López
Department of Statistics and Operational Research, Faculty of Medicine, Interdisciplinary Mathematics Institute, Universidad Complutense de Madrid (UCM), HUMLOG Research Group, 28040, Madrid, Spain
José M. Ferrer
Action Against Hunger. West and Central Africa Regional, 29621, Dakar, Senegal
Abdias Ogobara Dougnon
Action Against Hunger, BP 11491, Niamey, Niger
Abdoul Aziz Gado
Nutrition Direction, Ministry of Health, 623, Niamey, Niger
Atté Sanoussi & Nassirou Ousmane
Centre de Recherche Médicale et Sanitaire (CERMES), 10887, Niamey, Niger
Ramatoulaye Hamidou Lazoumar
Action Against Hunger, C/Duque de Sevilla No. 3., 28002, Madrid, Spain
Pilar Charle-Cuéllar
You can also search for this author in PubMed Google Scholar
Contributions
JMF and EMM-L interpreted and analyzed data, wrote the manuscript; PC-C identified and contributed to the design of the study and advised on the manuscript writing; AOD validated the field data information of the study; AAG supervised data collection during the implementation; AS, NO and RHL revised the manuscript draft. All authors have read and agreed to the published version of the manuscript.
Corresponding author
Correspondence to Pilar Charle-Cuéllar .
Ethics declarations
Ethical approval and consent to participate.
This study was conducted according to the guidelines laid down in the Declaration of Helsinki and all procedures involving research study participants were approved by The National Health Research Ethics Committee of Niger (007/201 8/CNERS).
Informed consent
Written informed consent was obtained from all subjects involved in the study.
Competing interests
The authors declare no competing interests.
Additional information
Publisher's note.
Springer Nature remains neutral with regard to jurisdictional claims in published maps and institutional affiliations.
Supplementary Information
Additional file 1. .
CHEERS 2022 Checklist.
Additional file 2.
Specific details of the DAN displayed in Figure 2 .
Additional file 3.
Illustrative examples of how uncertainty was incorporated in the DAN displayed in Figure 2 .
Rights and permissions
Open Access This article is licensed under a Creative Commons Attribution 4.0 International License, which permits use, sharing, adaptation, distribution and reproduction in any medium or format, as long as you give appropriate credit to the original author(s) and the source, provide a link to the Creative Commons licence, and indicate if changes were made. The images or other third party material in this article are included in the article's Creative Commons licence, unless indicated otherwise in a credit line to the material. If material is not included in the article's Creative Commons licence and your intended use is not permitted by statutory regulation or exceeds the permitted use, you will need to obtain permission directly from the copyright holder. To view a copy of this licence, visit http://creativecommons.org/licenses/by/4.0/ . The Creative Commons Public Domain Dedication waiver ( http://creativecommons.org/publicdomain/zero/1.0/ ) applies to the data made available in this article, unless otherwise stated in a credit line to the data.
Reprints and permissions
About this article
Cite this article.
Molanes-López, E.M., Ferrer, J.M., Dougnon, A.O. et al. Cost-effectiveness of severe acute malnutrition treatment delivered by community health workers in the district of Mayahi, Niger. Hum Resour Health 22 , 22 (2024). https://doi.org/10.1186/s12960-024-00904-1
Download citation
Received : 11 October 2023
Accepted : 29 February 2024
Published : 29 March 2024
DOI : https://doi.org/10.1186/s12960-024-00904-1
Share this article
Anyone you share the following link with will be able to read this content:
Sorry, a shareable link is not currently available for this article.
Provided by the Springer Nature SharedIt content-sharing initiative
- Severe acute malnutrition (SAM)
- Community health workers (CHWs)
- Community-based Management of Acute Malnutrition (CMAM)
- Decision analysis network (DAN)
Human Resources for Health
ISSN: 1478-4491
- Submission enquiries: Access here and click Contact Us
- General enquiries: [email protected]
Log in using your username and password
- Search More Search for this keyword Advanced search
- Latest content
- Supplements
- BMJ Journals More You are viewing from: Google Indexer
You are here
- Volume 9, Issue 3
- Midwife-led birthing centres in Bangladesh, Pakistan and Uganda: an economic evaluation of case study sites
- Article Text
- Article info
- Citation Tools
- Rapid Responses
- Article metrics

- http://orcid.org/0000-0001-7233-6804 Emily J Callander 1 ,
- Vanessa Scarf 1 ,
- Andrea Nove 2 ,
- http://orcid.org/0000-0002-7454-3011 Caroline Homer 3 ,
- http://orcid.org/0000-0002-5711-0417 Alayna Carrandi 4 ,
- Abu Sayeed Abdullah 5 ,
- Sheila Clow 6 ,
- Abdul Halim 5 ,
- Scovia Nalugo Mbalinda 7 ,
- Rose Chalo Nabirye 8 ,
- AKM Fazlur Rahman 5 ,
- Saad Ibrahim Rasheed 9 ,
- Arslan Munir Turk 9 ,
- Oliva Bazirete 2 , 10 ,
- Sabera Turkmani 1 , 3 ,
- Mandy Forrester 11 ,
- Shree Mandke 11 ,
- Sally Pairman 11 ,
- Martin Boyce 2
- 1 Faculty of Health , University of Technology Sydney , Sydney , New South Wales , Australia
- 2 Novametrics Ltd , Duffield , UK
- 3 Burnet Institute , Melbourne , Victoria , Australia
- 4 Monash University School of Public Health and Preventive Medicine , Melbourne , Victoria , Australia
- 5 Centre for Injury Prevention and Research , Dhaka , Bangladesh
- 6 University of Cape Town , Cape Town , South Africa
- 7 Makerere University , Kampala , Uganda
- 8 Busitema University , Tororo , Uganda
- 9 Research and Development Solutions , Islamabad , Pakistan
- 10 University of Rwanda , Kigali , Rwanda
- 11 International Confederation Of Midwives , The Hague , The Netherlands
- Correspondence to Professor Emily J Callander; Emily.callander{at}uts.edu.au
Introduction Achieving the Sustainable Development Goals to reduce maternal and neonatal mortality rates will require the expansion and strengthening of quality maternal health services. Midwife-led birth centres (MLBCs) are an alternative to hospital-based care for low-risk pregnancies where the lead professional at the time of birth is a trained midwife. These have been used in many countries to improve birth outcomes.
Methods The cost analysis used primary data collection from four MLBCs in Bangladesh, Pakistan and Uganda (n=12 MLBC sites). Modelled cost-effectiveness analysis was conducted to compare the incremental cost-effectiveness ratio (ICER), measured as incremental cost per disability-adjusted life-year (DALY) averted, of MLBCs to standard care in each country. Results were presented in 2022 US dollars.
Results Cost per birth in MLBCs varied greatly within and between countries, from US$21 per birth at site 3, Bangladesh to US$2374 at site 2, Uganda. Midwife salary and facility operation costs were the primary drivers of costs in most MLBCs. Six of the 12 MLBCs produced better health outcomes at a lower cost (dominated) compared with standard care; and three produced better health outcomes at a higher cost compared with standard care, with ICERs ranging from US$571/DALY averted to US$55 942/DALY averted.
Conclusion MLBCs appear to be able to produce better health outcomes at lower cost or be highly cost-effective compared with standard care. Costs do vary across sites and settings, and so further exploration of costs and cost-effectiveness as a part of implementation and establishment activities should be a priority.
- Maternal health
- Health economics
Data availability statement
No data are available. Ethics approval prohibits data sharing.
This is an open access article distributed in accordance with the Creative Commons Attribution 4.0 Unported (CC BY 4.0) license, which permits others to copy, redistribute, remix, transform and build upon this work for any purpose, provided the original work is properly cited, a link to the licence is given, and indication of whether changes were made. See: https://creativecommons.org/licenses/by/4.0/ .
https://doi.org/10.1136/bmjgh-2023-013643
Statistics from Altmetric.com
Request permissions.
If you wish to reuse any or all of this article please use the link below which will take you to the Copyright Clearance Center’s RightsLink service. You will be able to get a quick price and instant permission to reuse the content in many different ways.
WHAT IS ALREADY KNOWN ON THIS TOPIC
Midwife-led birth centres (MLBCs) have promising clinical evidence to support their implementation in low-income and middle-income countries, but there is an absence of evidence for costs and cost-effectiveness of implementing MLBCs relative to standard care.
WHAT THIS STUDY ADDS
This economic evaluation is the first study to quantify the real-word operation costs of MLBCs outside of high-income country settings. Our findings from Bangladesh, Pakistan and Uganda showed MLBCs can be cost-saving or cost-effective relative to standard care, and thus appear to be broadly consistent with results from other high-income country settings.
HOW THIS STUDY MIGHT AFFECT RESEARCH, PRACTICE OR POLICY
Our methodology, including a codesigned data collection tool with country researchers, highlighted the importance of close collaboration with local health service teams to identify the context of expenditure. The implementation of MLBCs in low-income and middle-income countries could be cost saving and cost-effective at small or larger scales, once contextual factors are considered.
Introduction
The United Nations has set targets within the Sustainable Development Goals (SDGs) to reduce maternal and neonatal mortality. 1 Also featured in the SDGs is universal access to healthcare—ensuring all people, regardless of location, have access to affordable and appropriate healthcare. 1 Achieving these dual goals is a challenge for all countries, particularly low-income and middle-income countries (LMICs), where maternal and neonatal mortality is highest, 2 3 as this will generally require improving service access and quality, alongside expanding services.
Increasing and promoting facility-based birth has been the main strategy for reducing maternal and neonatal mortality in many LMICs. 4 However, increased rates of births in a facility do not directly translate to reduced mortality if the facilities provide poor-quality care. 5 Regional and global disparities in maternity care across wealth quintiles and geographical locations, 5 alongside service challenges regarding funding and resources (including staffing and training), 6 pose significant hurdles to upscaling access to safe, high-quality maternity care.
High-income countries have increasingly taken a medicalised approach to maternity care. 7 8 While this approach sees low mortality rates, 8 there is a concern that the pendulum has swung too far. High rates of medical intervention during childbirth, such as caesarean birth and labour inductions, have led to short-term and long-term harms 9–12 and high and rapidly increasing costs per birth, 10 12 13 which may be becoming unaffordable even in high-income countries. 13 While many lessons can be learnt from models of care in high-income countries, 8 these may not represent the most effective and efficient path forward to achieving the SDGs in LMICs.
Midwife-led birth centres (MLBCs), where the lead healthcare professional at the time of birth is a midwife, are often seen as an alternative to hospital-based care for low-risk pregnancies and have been used in many countries. 14 This model of care been associated with increased rates of maternity service utilisation and reported satisfaction among women, strengthened networks of care and reduced rates of unnecessary interventions during childbirth. 14 As such, MLBCs may offer an appropriate option for providing maternity care in LMICs for women with uncomplicated pregnancies. There is, however, an absence of evidence about the costs associated with the establishment and operation of MLBCs and estimates around their cost-effectiveness relative to standard care in LMICs.
The objective of this study was to identify the costs of operating MLBCs in real-world LMIC settings, and to estimate their cost-effectiveness relative to standard care. We used a case study approach, with 4 MLBC sites in Bangladesh, Pakistan and Uganda (12 sites in total) to collect data on costs and outcomes of MLBCs and conduct a modelled cost-effectiveness analysis. The purpose of the study was to inform decision-making about the expansion of this model of care. The decision-making questions were as follows: (1) what would it cost to operate additional MLBCs in LMICs and (2) what would be the cost-effectiveness of additional MLBCs in LMICs?
Study setting and location
Bangladesh, Pakistan and Uganda were selected to participate in this study, based on the findings of a global literature review and survey 15 and consultation with the project’s advisory group. The advisory group consisted of experts in MLBCs from high-income, middle-income and low-income contexts and representatives of the International Confederation of Midwives, WHO, United Nations Population Fund, Bill & Melinda Gates Foundation and World Bank. The main inclusion criteria were as follows: (a) the country was classed by the World Bank in 2022 as low-income, lower-middle-income or upper-middle-income; (b) there was evidence from the literature and the survey that the country had at least four MLBCs that were either in the public sector or well integrated within the national health system; (c) good research capacity within the country and (d) data were expected to be available for this economic analysis. Each country that met the inclusion criteria was invited to participate through the national Ministry of Health and through the International Confederation of Midwives member association(s). National research teams were recruited by the International Confederation of Midwives, and these teams identified four MLBC sites per country for inclusion. Site selection was based on a combination of representativeness and feasibility and informed by a desk review of the literature and consultation with the national Ministry of Health, the International Confederation of Midwives member association, the national research team, the site manager(s) and other relevant stakeholders.
Study population
For the purposes of this study, we adopted the following definition of an MLBC: a dedicated space offering childbirth care, in which midwives take primary clinical responsibility for birthing care. Antenatal and postpartum care may also have been provided, but this was not essential for classification as an MLBC. Most of the 12 MLBC sites (n=10 sites), including all the Ugandan MLBCs, were freestanding, that is, on a site separate from a health facility to which the MLBC could refer women if needed. The remaining MLBCs (n=2 sites) were on the same site as a referral facility ( online supplemental appendix 1 ). Most MLBCs (n=8 sites) were in the private sector (including for-profit and not-for-profit), two were public–private partnerships (ie, public-sector facilities supported by non-governmental organisations) and two were in the public sector.
Supplemental material
The comparison was current ‘standard care’ in each country. This could have included a combination of hospital-based birth and home birth. As the decision-making question was concerned with expansion of MLBCs within the local setting, this heterogeneity in comparison was considered appropriate.
Study design
We conducted a cost analysis of MLBCs using primary data collection from routine data captured by the MLBCs in each of the four country sites within each of the three countries. Data were collected between October and December 2022. The data collection tool covered costs of operating the MLBC and outcomes of women and was codesigned with study teams from each country to ensure data availability. Data items related to costs of facility operation included utilities, staff salaries, staff training and equipment purchase and hire ( online supplemental appendix 2 ). The included costs represent the annual costs of operating an MLBC. Facility purchase costs were considered sunk costs and not included. Data related to health outcomes included transfer to other facilities, caesarean birth at other facilities, morbidity (eg, incidence of haemorrhage, third or fourth degree tears or other serious morbidities), maternal mortality, stillbirth, neonatal mortality and any costs paid by the women or their families.
A modelled cost-effectiveness analysis was then conducted, comparing MLBCs with standard care. This took the form of a decision analysis tree with 1000 hypothetical women ( online supplemental appendix 3 ). Women entered the model immediately prior to birth. In the MLBC arm, they then were either transferred or gave birth at the facility. All women who were transferred then had either a vaginal birth or caesarean birth and then had either no morbidities or morbidities. All women who gave birth in an MLBC had a vaginal birth and then had either no morbidities or morbidities. Data for the MLBC arm were collected from primary data from study sites. For current standard care within each country, rates of caesarean birth, stillbirth and neonatal death were obtained from the UNICEF Data Warehouse. 16 Rates of maternal mortality were obtained from WHO modelled estimates, 2 and maternal morbidity rates were obtained from the literature ( online supplemental appendix 4 ). 17 18
Per-woman costs for the operation of the MLBC were added to women in the MLBC arm, based on reported costs from the primary data collection. A cost for transfer was obtained from the primary data collection and applied to those who were transferred from the MLBC to another facility after onset of labour. For women in both arms who had a caesarean birth in a non-MLBC institution (MLBCs do not offer caesarean sections, because this procedure is not within the scope of practice of a midwife), costs per caesarean birth were sought for each country from the literature. Costs were separated into costs paid by the health service and out of pocket costs incurred by women ( online supplemental appendix 5 ).
Disability-adjusted life-years (DALYs) were allocated based on morbidity rates for the women and mortality rates for women and newborns. Categories were no maternal morbidity, maternal morbidity, maternal mortality and stillbirth or neonatal death ( online supplemental appendix 6 ). Disability weights for maternal morbidity were obtained from the average of the following conditions, identified from the Global Burden of Disease Study 19 and were calculated based on the average weight for maternal haemorrhage, pregnancy-related sepsis, hypertensive disorders, obstructed labour, rectal fistula and vesicovaginal fistula.
Patient and public involvement in research
The cost data collection tool was codesigned by the research team and the national researchers. The national researchers engaged with each of the MLBC sites to identify typical annual expenditures. After initial data collection, a series of meetings were held between the analysis team and the national research teams to validate the data provided.
Time horizon and discount rate
We adopted a health funder perspective. The time horizon for the cost and cost-effectiveness analysis was 1 year, and as such no discounting was required. The short 1-year time horizon is considered conservative and underestimates the value of health outcomes produced over a lifetime; however, due to the absence of primary data collection for health outcomes this was considered necessary to avoid introducing additional uncertainty.
Currency, price date and conversion
All costs are presented in 2022 US dollars. Costs were inflated to 2022 dollars based on published inflation rates and converted from original currency to US dollars based on the average exchange rates for the 2022 calendar year. 20
Reporting followed the Consolidated Health Economic Evaluation Reporting Standards 2022 ( online supplemental appendix 7 ). 21 A detailed reflexivity statement exploring the authorship of this piece is presented in online supplemental appendix 8 .
Data analysis
Data for each facility were presented separately based on primary data collected. Where ranges were reported, a midpoint was selected. Staff salary costs were calculated by multiplying reported full-time salary by the number of full-time equivalent staff. Based on the discussions between the research team and national researchers, the approximate average midwife salary of US$200 per month, identified by the country liaison researchers, was applied to Uganda due to the variability of costs reported by sites. Similarly, for site 3 in Pakistan, an average midwife salary from the other three sites was applied.
Costs of MLBCs were presented as total annual costs for the facility, and these were also divided by the number of births to present a cost per birth for each facility. For the cost analysis, the total health service and total user costs were identified and summed to present a total cost for each model of care. Total DALYs lost were also summed for each model of care. An incremental cost-effectiveness ratio (ICER) was identified by dividing the difference in the total costs of MLBCs and standard care by the difference in DALYs lost from MLBCs and standard care. All results were presented separately for each site and were designed to describe the costs and cost-effectiveness compared with standard care of MLBCs based on that site’s operation. All analyses were conducted using Microsoft Excel.
Uncertainty analysis
We conducted one-way sensitivity analysis based on cost data reported as zero in the study countries. This included facility, midwife salary, medical officer salary, recruitment and training, and transport costs.
The annual number of births for the four selected MLBC sites in Bangladesh ranged from 101 to 2189 per year. Total annual costs ranged from US$5068 (site 2; 101 births per year) to US$117 662 (site 4; 337 births per year) ( online supplemental appendix 9 ). Total costs per birth were highest at site 4—US$349 per birth; and lowest at site 3—US$21 per birth. Costs were mostly driven by staff salaries and facility operation costs. Facility operation costs per woman were generally higher in smaller facilities as were the midwife salary costs per woman. Site 2, which had 101 births per year, only reported midwife salary costs, with no costs for other staff.
In the modelled cost-effectiveness analysis of MLBCs compared with standard care in Bangladesh, total costs of care (including costs associated with transfers and caesarean births in other facilities) for MLBCs ranged from US$23 439 to US$469 100 ( table 1 ). Costs for standard care were US$314 754 for 1000 women. Sites 1, 2 and 3 had better health outcomes in the total number of DALYs lost than standard care. Sites 1, 2 and 3 produced better outcomes at a lower cost than standard care. Site 4 produced comparable health outcomes to standard care, at higher cost. These additional costs are largely due to the site being extremely remote and it being necessary to pay higher salaries to recruit and retain staff.
- View inline
Modelled cost-effectiveness of midwife-led birth centre sites compared with current standard care, Bangladesh, hypothetical cohort of 1000 women
The annual number of births for MLBC sites in Pakistan ranged from 95 to 5183 per year. Total annual costs ranged from US$4907 (site 3; 95 births per year) to US$288 649 (site 1; 544 births per year) ( online supplemental appendix 10 ). Total costs per birth were highest at site 1—US$531 per birth; and lowest at site 4—US$34 per birth. Costs were mostly driven facility operation, equipment purchase and other staff costs. Midwife staffing costs ranged from US$6 per woman at site 2 to US$42 per woman at site 1, however, this was less than the amount spent on other staff at sites 1, 2 and 4.
In the modelled cost-effectiveness analysis of MLBCs compared with standard care, total costs of care for MLBCs in Pakistan ranged from US$36 519 to US$693 521 ( table 2 ). Costs for standard care were US$176 057 for 1000 women. Sites 2 and 4 produced better outcomes at lower cost than standard care. Site 3 produced poorer outcomes and was more costly than standard care. Based on costs and outcomes of site 1, MLBCs would cost an additional US$7392 per DALY averted.
Modelled cost-effectiveness of midwife-led birth centre sites compared with current standard care, Pakistan, hypothetical cohort of 1000 women
The annual number of births for MLBC sites in Uganda ranged from 12 to 1242 per year. Total annual costs ranged from US$7922 (site 4; 64 births per year) to US$348 000 (site 3; 1242 births per year) ( online supplemental appendix 11 ). Total costs per birth were highest at site 2—US$2374 per birth, although this site cannot be considered typical. Site 2 is in a remote area and is supported by wealthy donors prepared to pay for equipment and four full-time midwives, even though there were only 12 births in the past twelve months. Total costs per birth were lowest at site 4—US$124 per birth. Costs were mostly driven by facility operations costs, midwife salaries and other staff salaries. Midwife staffing costs ranged from US$10 per woman at site 3 with 1242 births per annum to US$800 per woman at site 2 with just 12 births. Other staff salary costs ranged from US$232 per woman (site 3) to US$23 per woman (site 1). Sites 1 and 3 did not report any equipment costs.
In the modelled cost-effectiveness analysis for Uganda, total costs of care ranged from US$147 273 (site 4) to US$2 458 750 (site 2) ( table 3 ). Costs for standard care were US$277 012 for 1000 women. In terms of cost-effectiveness, sites 1, 2 and 3 MLBCs would lead to better health outcomes than standard care. Site 1 delivered better outcomes at a lower cost than standard care, site 3 had a small ICER of US$571 per DALY saved, and site 2 had a larger ICER of US$55 942 per DALY saved. Site 4 demonstrated lower costs, but poorer health outcomes compared with standard care.
Modelled cost-effectiveness of midwife-led birth centre sites compared with current standard care, Uganda, hypothetical cohort of 1000 women
Cross-country comparison
There was no discernible pattern between facility size and cost per birth, with both larger and smaller facilities reporting low costs per birth ( online supplemental appendix 12 ). Midwife salary costs and facility costs were generally the largest contributor to overall costs across each of the sites and countries ( figure 1 ). Sites 3 and 4 in Bangladesh and site 3 in Uganda were notable exceptions to this, with most costs being attributable to other staff salaries. From the modelled cost-effectiveness analysis, all public and public–private partnership MLBCs produced better health outcomes and were less costly than standard care ( figure 2 ). In total, 9 of the 12 (75%) of the sites produced better health outcomes than standard care, as measured by DALYs; and half (6 of the 12 MLBCs) produced better health outcomes and were cost saving.
- Download figure
- Open in new tab
- Download powerpoint
Proportion of total costs attributable to facility costs, midwife salaries, other staff salaries, recruitment and training for midwife-led birth centres in Bangladesh, Pakistan and Uganda in 2022 US dollars. BGD, Bangladesh; PAK, Pakistan; UGA, Uganda.
Incremental cost-effectiveness of midwife-led birth centres compared with standard care, Bangladesh, Pakistan, Uganda in 2022 US dollars. BGD, Bangladesh; PAK, Pakistan; UGA, Uganda.
Results of the sensitivity analysis are presented in online supplemental appendix 13 . Replacing data reported as having zero costs with country averages did not substantially change the ICERs produced.
Using a case study approach, this economic evaluation identified the range of reported costs of operating MLBCs in 12 sites in Bangladesh, Pakistan and Uganda, and estimated their cost-effectiveness relative to standard care. Costs of operating MLBCs within the countries varied greatly. Midwife salaries and annual facility operation costs were consistent cost drivers in all countries. In the modelled cost-effectiveness analysis, 6 of the 12 MLBCs were ‘dominant’, producing both better health outcomes and lower costs compared with standard care. Two of the remaining sites had an ICER of less than US$8000 per DALY averted, meaning it would cost less than US$8000 to prevent one additional DALY using MLBCs.
Our study is the first to quantify the costs of MLBCs outside of high-income country settings, making identification of comparable studies difficult due to differences in health systems characteristics. Nonetheless, our findings are consistent with a retrospective cohort study of more than 364 000 births in Australia between 2001 and 2012, which also found MLBCs resulted in lower costs than other models of care. 22 The Birthplace in England study also found similar results. 23 Other studies have also found that expanding access to midwife-led care can substantially reduce maternal and neonatal mortality and morbidity rates and improve maternal and newborn health and well-being. 24 25 Our findings show MLBCs can be cost-saving or cost-effective relative to other models of care, and thus appear to be broadly consistent with results from other settings. We did note that costs of operation and cost-effectiveness varied widely between and within countries, and cost-effectiveness does appear to be dependent on the unique local site characteristics as opposed to general characteristics such as size or rurality. Our methodology also highlighted the importance of close collaboration with local health service team to identify the context of expenditure. We identified MLBCs that demonstrated better health outcomes and cost savings in all three countries, private, public and public–private partnerships, rural and urban settings, and in freestanding as well as in those onsite with or alongside referral facilities.
MLBCs can help meet the growing demand for facility-based birth for low-risk women and might be particularly beneficial in LMICs where universal access to higher level facility-based care is limited. 26 Shifting the main strategy for reducing maternal and neonatal mortality in many LMICs from increasing the rate of deliveries within medical facilities 4 to focusing on the quality of healthcare may better translate to better maternal and neonatal health outcomes. 5 24 Clinical findings showing that care provided in MLBCs is as safe and effective as that in the obstetric units and results in less intervention justify the expansion of this model of care so that scarce resources can be used more effectively. 27 This study provides evidence for MLBCs in LMICs as an effective, evidence-based strategy to improve the quality, costs and experiences of maternity care. Further, there did not seem to be a clear scale efficiency effect, indicating that MLBCs could be cost-effective at small or larger scales in LMIC settings, once contextual factors are considered.
Strengths and limitations
A key strength of this study was that data were collected from a range of sites and countries in a real-world setting to identify variation in costs and outcomes. We codesigned our data collection tool with country researchers to comprehensively capture the range of operation costs. Nonetheless, our study was limited by the inability of some sites to identify some areas of expenditure—particularly equipment costs and midwife salaries. Furthermore, as all facilities were already established, we were unable to identify set-up costs. A key recommendation from this study is investment in prospective implementation analysis of the costs and outcomes produced when new MLBCs are established in LMICs, as well as investment in standardised data capture tools for identifying costs and outcomes. As such, the results of this study must be interpreted with caution as they are reliant on the accuracy of the reported data in a small number of sites.
Our analysis was also unable to capture some additional benefits of MLBCs beyond mortality and morbidity, particularly around women’s experiences and satisfaction with care—which are key to capturing the full value associated with midwife-led care. 28 A key component for all MLBCs and midwife-led care more broadly, is the woman-centred philosophy, continuity of care during pregnancy and after birth, and involvement of women in all decisions regarding perinatal care. 14 22 29–31 MLBCs seek to promote normal, physiological childbirth by recognising, respecting and safeguarding normal birth processes through individualised care, 32 as opposed to the typical hospital approach to labour which is much more time-oriented and standardised, and not infrequently, there is a pressure on midwives to accelerate the process by carrying out unnecessary medical intervention. 33 Consequently, women who give birth in an MLBC report feeling supported in their ability to participate in the decision-making process, greater autonomy, and, thus, greater acceptance of and satisfaction with perinatal care in this setting among pregnant women. 22 30–32 34 35 These additional benefits were unable to be captured in our study but are important to recognise when considering the value of MLBCs.
MLBCs offer a potentially cost-effective model of care for providing safe and high-quality care to women giving birth in LMICs. However, the cost of operating an MLBC varies greatly, and this does affect cost-effectiveness. Further research, including prospective evaluation of implementation of new MLBCs, is recommended to confirm the results produced in our study.
Ethics statements
Patient consent for publication.
Not applicable.
Ethics approval
This study involves human participants and ethical approval was obtained from the following ethics committees: Alfred Hospital Ethics Committee (Australia; Reference 381/22); the Centre for Injury Prevention and Research, Bangladesh (CIPRB) Institutional Review Board (Bangladesh; Reference CIPRB/ERC/2022/11); Research and Development Solutions (RADS) Institutional Review Board (Pakistan; Reference IRB00010843) and Mulago Hospital Research Ethics Committee (Uganda; Reference MHREC-2022-77). Participants gave informed consent to participate in the study before taking part.
- United Nations
- World Health Organization
- Blossom J , et al
- Puchalski Ritchie LM ,
- Moore JE , et al
- Johanson R ,
- Newburn M ,
- Macfarlane A
- Goldenberg RL ,
- Norman JE ,
- Humphrey T ,
- Abhyankar P ,
- Callander E ,
- Ellwood D , et al
- Tataj-Puzyna U ,
- Sys D , et al
- Bazirete O ,
- Hughes K , et al
- De Silva M ,
- Lindquist A , et al
- Nakimuli A ,
- Nakubulwa S ,
- Kakaire O , et al
- Center for the Evaluation of Value and Risk in Health
- Husereau D ,
- Drummond M ,
- Augustovski F , et al
- Fiebig DG ,
- Scarf V , et al
- Schroeder E ,
- Patel N , et al
- Renfrew MJ ,
- Friberg IK ,
- de Bernis L , et al
- Allanson ER ,
- Pontre J , et al
- Normand C , et al
- Callander EJ ,
- Edmonds JK ,
- Kafulafula U
- Christensen LF ,
- Overgaard C
- Macfarlane AJ ,
- Rocca-Ihenacho L ,
- Overgaard C ,
- Fenger-Grøn M ,
- Percival P , et al
Supplementary materials
Supplementary data.
This web only file has been produced by the BMJ Publishing Group from an electronic file supplied by the author(s) and has not been edited for content.
- Data supplement 1
Handling editor Lei Si
Twitter @VScarf, @LaneCarrandi, @ICM_CE
Contributors EJC led the overall study design, with input from VS, AN, CH and MB. Researchers from Bangladesh (ASA, AH and AKMFR) Uganda (SNM and RCN) and Pakistan (SIR and AMT) led the development of the data collection tools specifically designed for this study. These in-country researchers undertook all the data collection and assisted with data verification and analysis. EJC and VS undertook the analysis. All authors (EJC, VS, AN, CH, AC, ASA, SC, AH, SNM, RCN, AKMFR, SIR, AMT, OB, ST, MF, SM, SP and MB) contributed to the interpretation of data for the work. EJC led the drafting of the manuscript, with input from all authors. All authors (EJC, VS, AN, CH, AC, ASA, SC, AH, SNM, RCN, AKMFR, SIR, AMT, OB, ST, MF, SM, SP and MB) have read and approved the final manuscript version. All authors (EJC, VS, AN, CH, AC, ASA, SC, AH, SNM, RCN, AKMFR, SIR, AMT, OB, ST, MF, SM, SP and MB) agree to be accountable for all aspects of the work. EC acts as guarantor.
Funding The study was funded by a grant from the Bill & Melinda Gates Foundation (award number INV—033046).
Disclaimer The funding body was not involved in the study design or writing of this manuscript.
Competing interests None declared.
Patient and public involvement Patients and/or the public were involved in the design, or conduct, or reporting, or dissemination plans of this research. Refer to the Methods section for further details.
Provenance and peer review Not commissioned; internally peer reviewed.
Supplemental material This content has been supplied by the author(s). It has not been vetted by BMJ Publishing Group Limited (BMJ) and may not have been peer-reviewed. Any opinions or recommendations discussed are solely those of the author(s) and are not endorsed by BMJ. BMJ disclaims all liability and responsibility arising from any reliance placed on the content. Where the content includes any translated material, BMJ does not warrant the accuracy and reliability of the translations (including but not limited to local regulations, clinical guidelines, terminology, drug names and drug dosages), and is not responsible for any error and/or omissions arising from translation and adaptation or otherwise.
Read the full text or download the PDF:
Cost-effectiveness analysis of CTZ/TAZ for the treatment of ventilated hospital-acquired bacterial pneumonia and ventilator-associated bacterial pneumonia in Japan
Affiliations.
- 1 Graduate School of Public Health, St. Luke's International University, 10-1 Akashi-Cho, Chuo-City, Tokyo, 104-0044, Japan. [email protected].
- 2 St. Luke's International Hospital, Tokyo, Japan.
- 3 National Center for Global Health and Medicine, Tokyo, Japan.
- 4 Graduate School of Public Health, St. Luke's International University, 10-1 Akashi-Cho, Chuo-City, Tokyo, 104-0044, Japan.
- PMID: 38549158
- PMCID: PMC10976789
- DOI: 10.1186/s12913-024-10883-7
Background: Resistant bacterial infections, particularly those caused by gram-negative pathogens, are associated with high mortality and economic burdens. Ceftolozane/tazobactam demonstrated efficacy comparable to meropenem in patients with ventilated hospital-acquired bacterial pneumonia in the ASPECT-NP study. One cost-effectiveness analysis in the United States revealed that ceftolozane/tazobactam was cost effective, but no Japanese studies have been conducted. Therefore, the objective of this study was to assess the cost-effectiveness of ceftolozane/tazobactam compared to meropenem for patients with ventilated hospital-acquired bacterial pneumonia/ventilator-associated bacterial pneumonia from a health care payer perspective.
Methods: A hybrid decision-tree Markov decision-analytic model with a 5-year time horizon were developed to estimate costs and quality-adjusted life-years and to calculate the incremental cost-effectiveness ratio associated with ceftolozane/tazobactam and meropenem in the treatment of patients with ventilated hospital-acquired bacterial pneumonia/ventilator-associated bacterial pneumonia. Clinical outcomes were based on the ASPECT-NP study, costs were based on the national fee schedule of 2022, and utilities were based on published data. One-way sensitivity analysis and probabilistic sensitivity analysis were also conducted to assess the robustness of our modeled estimates.
Results: According to our base-case analysis, compared with meropenem, ceftolozane/tazobactam increased the total costs by 424,731.22 yen (£2,626.96) and increased the quality-adjusted life-years by 0.17, resulting in an incremental cost-effectiveness ratio of 2,548,738 yen (£15,763.94) per quality-adjusted life-year gained for ceftolozane/tazobactam compared with meropenem. One-way sensitivity analysis showed that although the incremental cost-effectiveness ratio remained below 5,000,000 yen (£30,925) for most of the parameters, the incremental net monetary benefit may have been less than 0 depending on the treatment efficacy outcome, especially the cure rate and mortality rate for MEPM and mortality rate for CTZ/TAZ. 53.4% of the PSA simulations demonstrated that CTZ/TAZ was more cost-effective than MEPM was.
Conclusion: Although incremental cost-effectiveness ratio was below ¥5,000,000 in base-case analysis, whether ceftolozane/tazobactam is a cost-effective alternative to meropenem for ventilated hospital-acquired bacterial pneumonia/ventilator-associated bacterial pneumonia in Japan remains uncertain. Future research should examine the unobserved heterogeneity across patient subgroups and decision-making settings, to characterise decision uncertainty and its consequences so as to assess whether additional research is required.
Keywords: Ceftolozane/tazobactam; Cost-effectiveness analysis; Meropenem; Ventilated-hospital-acquired bacterial pneumonia/ventilator-associated bacterial pneumonia.
© 2024. The Author(s).
Thank you for visiting nature.com. You are using a browser version with limited support for CSS. To obtain the best experience, we recommend you use a more up to date browser (or turn off compatibility mode in Internet Explorer). In the meantime, to ensure continued support, we are displaying the site without styles and JavaScript.
- View all journals
- My Account Login
- Explore content
- About the journal
- Publish with us
- Sign up for alerts
- Brief Communication
- Open access
- Published: 25 March 2024
Assessing GPT-4 for cell type annotation in single-cell RNA-seq analysis
- Wenpin Hou ORCID: orcid.org/0000-0003-0972-2192 1 &
- Zhicheng Ji ORCID: orcid.org/0000-0002-9457-4704 2
Nature Methods ( 2024 ) Cite this article
30k Accesses
276 Altmetric
Metrics details
- Computational models
- Gene expression profiling
- Machine learning
- Transcriptomics
Here we demonstrate that the large language model GPT-4 can accurately annotate cell types using marker gene information in single-cell RNA sequencing analysis. When evaluated across hundreds of tissue and cell types, GPT-4 generates cell type annotations exhibiting strong concordance with manual annotations. This capability can considerably reduce the effort and expertise required for cell type annotation. Additionally, we have developed an R software package GPTCelltype for GPT-4’s automated cell type annotation.
Similar content being viewed by others
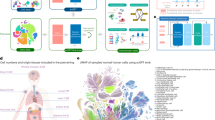
scGPT: toward building a foundation model for single-cell multi-omics using generative AI
Haotian Cui, Chloe Wang, … Bo Wang
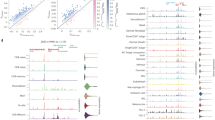
Single-cell multi-ome regression models identify functional and disease-associated enhancers and enable chromatin potential analysis
Sneha Mitra, Rohan Malik, … Christina S. Leslie
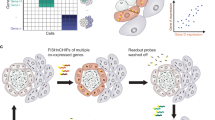
Highly sensitive spatial transcriptomics using FISHnCHIPs of multiple co-expressed genes
Xinrui Zhou, Wan Yi Seow, … Kok Hao Chen
Cell type annotation is a fundamental step in single-cell RNA sequencing (scRNA-seq) analysis. This process is often laborious and time-consuming, requiring a human expert to compare genes highly expressed in each cell cluster with canonical cell type marker genes. Although automated cell type annotation methods have been developed (Supplementary Table 1) , manual annotation using marker genes remains widely used.
Generative pre-trained transformers (GPT), including GPT-3.5 and GPT-4, are large language models designed for language understanding and generation. Recent studies have demonstrated their effectiveness in biomedical contexts 1 , 2 . In this Brief Communication, we hypothesize that GPT-4 can accurately annotate cell types, transitioning the annotation process from manual to a semi- or even fully automated procedure (Fig. 1a ). GPT-4 offers cost-efficiency and seamless integration into existing single-cell analysis pipelines such as Seurat 3 , avoiding the need for building additional pipelines and collecting high-quality reference datasets. The vast training data of GPT-4 enables broader applications across various tissues and cell types, and its chatbot nature allows for user-driven annotation refinement (Fig. 1a,b ).
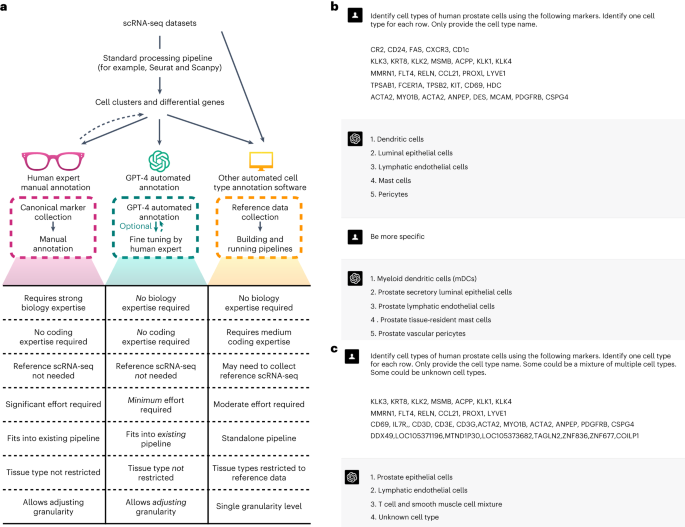
a , Comparison of cell type annotations by human experts, GPT-4, and other automated methods. b , Example of GPT-4 annotating human prostate cells with increasing granularity. c , Example of GPT-4 annotating single, mixed and new cell types.
We systematically assessed GPT-4’s cell type annotation performance across ten datasets 4 , 5 , 6 , 7 , 8 , 9 , 10 , 11 , 12 , covering five species and hundreds of tissue and cell types, and including both normal and cancer samples (Supplementary Table 2) . GPT-4 was queried using GPTCelltype, a software tool we developed ( Methods ). For competing methods, we evaluated GPT-3.5, a prior version of GPT-4, and CellMarker2.0 13 , SingleR 14 and ScType 15 , which are automatic cell type annotation methods that provide references applicable to a large number of tissues ( Methods and Supplementary Table 1) . Cell type annotations by GPT-4 or competing methods were evaluated based on their agreement with manual annotations provided by the original studies. The degree of agreement was measured using a numeric score ( Methods ). Supplementary Table 3 presents an example of evaluating GPT-4 cell type annotations in human prostate tissue, and details of all cell type annotations and their evaluation results are included in Supplementary Table 4 .
We first explored different factors that may affect the annotation accuracy of GPT-4 (Fig. 2a and Supplementary Table 5) . We found that GPT-4 performs best when using the top ten differential genes, and when differential genes are derived using the two-sided Wilcoxon test. GPT-4 exhibits similar accuracy across various prompt strategies, including a basic prompt strategy, a chain-of-thought 16 -inspired prompt strategy that includes reasoning steps, and a repeated prompt strategy ( Methods ). In subsequent analyses, both GPT-4 and GPT-3.5 used the basic prompt strategy with the top ten differential genes obtained from Wilcoxon test as inputs for applicable datasets.
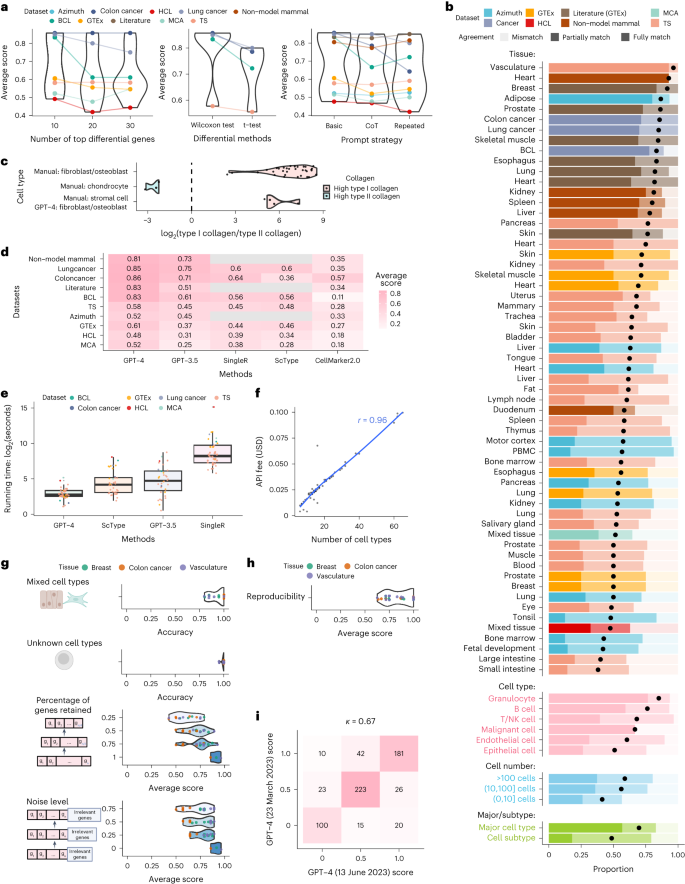
a , Average agreement scores for varying numbers of top differential genes, statistical tests for differential analysis, and prompt strategies. b , Proportion of cell types with varying agreement levels in each study and tissue, most abundant broad cell types, malignant cells, different cell population sizes, and major cell types versus cell subtypes. c , log 2 -transformed ratio of type I ( COL1A1 and COL1A2 ) and II ( COL2A1 ) collagen gene expression. d , e , Comparison of average agreement scores ( d ) and running times ( e ). In e , n = 59 for GPT-4 and GPT-3.5 and n = 36 for ScType and SingleR. Each boxplot shows the distribution (center: median; bounds of box: first and third quartiles; bounds of whiskers: data points within 1.5× interquartile range from the box; minima; maxima) of running time. f , Financial cost of querying GPT-4 API versus cell type numbers. g , GPT-4’s performance in identifying mixed/single cell types and known/unknown cell types, and under different subsampling and noise levels in multiple simulation rounds (dots). h , Reproducibility of GPT-4 annotations. i , Consistency of agreement scores between two versions of GPT-4.
GPT-4’s annotations fully or partially match manual annotations in over 75% of cell types in most studies and tissues (Fig. 2b ), demonstrating its competency in generating expert-comparable cell type annotations. This agreement is particularly high for marker genes from literature searches, with at least 70% fully match rate in most tissues. Though lower for genes identified by differential analysis, the agreement remains high. However, results from datasets published before September 2021 should be interpreted cautiously as they predate GPT-4’s training cutoff. GPT-4 performs better for immune cells like granulocytes compared to other cell types (Fig. 2b ). It identifies malignant cells in colon and lung cancer datasets but struggles with B lymphoma, potentially due to a lack of distinct gene sets. The identification of malignant cells could benefit from other approaches such as copy number variation 9 . Performance dips slightly in small cell populations comprising no more than ten cells (Fig. 2b ), possibly due to the limited available information. GPT-4 annotations fully match manual annotations more frequently in major cell types (for example, T cells) than in subtypes (for example, CD4 memory T cells), while over 75% of subtypes still achieve full or partial matches (Fig. 2b ).
The low agreement between GPT-4 and manual annotations in some cell types does not necessarily imply that GPT-4’s annotation is incorrect. For instance, cell types classified as stromal cells include fibroblasts and osteoblasts expressing type I collagen genes, and chondrocytes expressing type II collagen genes. For cells manually annotated as stromal cells, GPT-4 assigns cell type annotations with higher granularity (for example, fibroblasts and osteoblasts), resulting in partial matches and a lower agreement. For cell types that are manually annotated as stromal cells but identified by GPT-4 as fibroblasts or osteoblasts, type I collagen genes show substantially higher expression than type II collagen genes (Fig. 2c ). This agrees with the pattern observed in cells manually annotated as chondrocytes, fibroblasts, and osteoblasts (Fig. 2c ), suggesting that GPT-4 provides more accurate cell type annotations for stromal cells.
GPT-4 substantially outperforms other methods based on average agreement scores ( Methods and Fig. 2d ). Using GPTCelltype as the interface, GPT-4 is also notably faster (Fig. 2e ), partly due to its utilization of differential genes from the standard single-cell analysis pipelines such as Seurat 3 . Given the integral role of these pipelines, we regard the differential genes as immediately available for GPT-4. In contrast, other methods like SingleR and ScType require additional steps to reprocess the gene expression matrices. Compared to other methods that are free of charge, GPT-4 incurs a $20 monthly fee for using online web portal. Cost of GPT-4 API is linearly correlated with the number of queried cell types and does not exceed $0.1 for all queries in this study (Fig. 2f ).
We further assessed GPT-4’s robustness in complex real data scenarios (Fig. 1c ) with simulated datasets ( Methods ). GPT-4 can distinguish between pure and mixed cell types with 93% accuracy, and differentiate between known and unknown cell types with 99% accuracy (Fig. 2g ). When the input gene set includes fewer genes or is contaminated with noise, GPT-4’s performance decreases but remains high (Fig. 2g ). These results demonstrate GPT-4’s robustness in various scenarios.
Finally, we assessed the reproducibility of GPT-4’s annotations using prior simulation studies ( Methods ). GPT-4 generated identical annotations for the same marker genes in 85% of cases (Fig. 2h ), indicating high reproducibility. Annotations of two GPT-4 versions showed identical agreement scores in most cases, with a Cohen’s κ of 0.65, demonstrating substantial consistency (Fig. 2i ).
While GPT-4 excels in cell type annotation, which surpasses existing methods, there are limitations to consider. Firstly, the undisclosed nature of GPT-4’s training corpus makes verifying the basis of its annotations challenging, thus requiring human evaluation to ensure annotation quality and reliability. Secondly, human involvement in the optional fine-tuning of the model may affect reproducibility due to subjectivity and could limit the scalability of the model in large datasets. Thirdly, high noise levels in scRNA-seq data and unreliable differential genes can adversely affect GPT-4’s annotations. Lastly, over-reliance on GPT-4 risks artificial intelligence hallucination. We recommend validation of GPT-4’s cell type annotations by human experts before proceeding with downstream analyses.
While this study focuses on the standard version of GPT-4, fine-tuning GPT-4 with high-quality reference marker gene lists could further improve cell type annotation performance, utilizing services such ‘GPTs’ provided by OpenAI.
Dataset collection
For the HuBMAP Azimuth project, manually annotated cell types and their marker genes were downloaded from the Azimuth website ( https://azimuth.hubmapconsortium.org/ ). Azimuth provides cell type annotations for each tissue at different granularity levels. We selected the level of granularity with the fewest number of cell types, provided that there are more than ten cell types within that level. Details of how marker genes were generated are not reported by Azimuth.
For the GTEx 5 dataset, manually annotated cell types, differential gene lists and gene expression matrices were downloaded directly from the publication 5 . In the original study, gene expression raw counts were library-size-normalized and log-transformed after adding a pseudocount of 1 with SCANPY 17 . ComBat 18 was used to account for the protocol- and sex-specific effects with SCANPY 17 . Welch’s t -test was then performed to identify differential genes that compare one cell type against the rest. For each cell type, genes were ranked increasingly by P values, and genes with the same P values were further ranked decreasingly by t -statistics. Top 10, 20 and 30 differential genes were used in this study. Lists of marker genes through literature search and the corresponding cell types were downloaded from the same study 5 , and only cell types with at least five marker genes were used.
For the HCL 6 dataset, manually annotated cell types, differential gene lists and the gene expression matrix were downloaded directly from the publication 6 . In the original study, gene expression raw counts underwent a batch removal process to facilitate cross-tissue comparison and were subsequently normalized by library size and log-transformed after adding a pseudocount of 1. Two-sided Wilcoxon rank-sum test was then performed to identify differential genes comparing one cell type against the rest using Seurat 3 . Differential genes were further selected by log fold change larger than 0.25, Bonferroni-adjusted P value smaller than 0.1, and expressed in at least 15% of cells in either population. For each cell type, genes were ranked increasingly by P values, and genes with the same P values were further ranked decreasingly by two-sided Wilcoxon test statistics. Top 10, 20 and 30 differential genes were used in this study.
For the Mouse Cell Atlas (MCA) 7 dataset, manually annotated cell types, differential gene lists and gene expression matrix were downloaded directly from the publication 6 . In the original study, gene expression raw counts underwent a batch removal process to facilitate cross-tissue comparison, and Seurat 3 was used to perform preprocessing and differential analysis. For each cell type, genes were ranked increasingly by P values, and genes with the same P values were further ranked decreasingly by log fold change. Top 10, 20 and 30 differential genes were used in this study.
For non-model mammal dataset 12 , manually annotated cell types and lists of marker genes through literature search were downloaded directly from the original study.
For Tabula Sapiens (TS) 8 , B-cell lymphoma (BCL) 9 , lung cancer 11 and colon cancer 10 datasets, manually annotated cell types and raw gene expression count matrices were downloaded directly from original studies. Raw counts were normalized by library size and log-transformed after adding a pseudocount of 1. Seurat FindAllMarkers() function with default settings was used to obtain differential genes by comparing one cell type with the rest within each tissue. Briefly, genes with at least 0.25 log fold change between two cell populations and detected in at least 10% of cells in either cell population were retained. Two-sided Wilcoxon rank-sum test was then performed for differential analysis. In addition, two-sided two-sample t -test was also performed for differential analysis using the FindAllMarkers() function with default settings. For each cell type, genes were ranked increasingly by P values, and genes with the same P values were further ranked decreasingly by log fold changes. Top 10, 20 and 30 differential genes were used in this study.
Cell type annotation methods
Gpt-4 and gpt-3.5.
All GPT-4 (13 June 2023 version) and GPT-3.5 (13 June 2023 version) cell type annotations in this study were performed using GPTCelltype, an R software package we developed as an interface for GPT models. GPTCelltype takes marker genes or top differential genes as input, and automatically generates prompt message using the following template with the basic prompt strategy:
‘Identify cell types of TissueName cells using the following markers separately for each row. Only provide the cell type name. Do not show numbers before the name. Some can be a mixture of multiple cell types.\n GeneList’.
Here ‘TissueName’ is a variable that will be replaced with the actual name of the tissue (for example, human prostate), and ‘GeneList’ is a list of marker genes or top differential genes. Genes for the same cell population are joined by comma (,), and gene lists for different cell populations are separated by the newline character (\n). GPT-4 or GPT-3.5 was then queried using the generated prompt message through OpenAI API, and the returned information was parsed and converted to cell type annotations.
For chain-of-thought prompt strategy, the following sentence was added to the beginning of the message generated by the basic prompt strategy: ‘Because CD3 gene is a marker gene of T cells, if CD3 gene is included in the marker gene list of an unknown cell type, the cell type is likely to be T cells, a subtype of T cells, or a mixed cell type containing T cells’.
For repeated prompt strategy, GPT-4 was queried with the basic prompt strategy repeatedly for five times. The annotation result that appears most frequently among the five queries was selected as the final cell type annotation.
GPT-4 (23 March 2023 version) cell type annotations were performed by manually copying and pasting prompt messages to GPT-4 online web interface ( https://chat.openai.com/ ). The prompt message was constructed using the following template:
‘Identify cell types of TissueName cells using the following markers. Identify one cell type for each row. Only provide the cell type name. \n GeneList’.
Computationally identified differential genes in eight scRNA-seq datasets and canonical marker genes identified through literature search in two datasets were used as inputs to GPT-4 and GPT-3.5 (Supplementary Table 2) . Cell type annotation for HCL and MCA was performed and evaluated once by aggregating all tissues, similar to the original studies. In other studies, cell type annotation was performed and evaluated within each tissue.
SingleR 14 (version 1.4.1) R package was used to perform cell type annotations with default settings. For HCL and MCA datasets, the gene expression matrices after batch effect removal, library size normalization and log transformation across all tissues were used as input. For all other datasets, SingleR was performed separately within each tissue, and the input is the log-transformed and library-size normalized gene expression matrix. The built-in Human Primary Cell Atlas reference 19 was used as the reference dataset for all SingleR annotations. SingleR generates single-cell level cell type annotations by returning an assignment score matrix for each single cell and each cell type label in the reference. To convert single-cell level annotations to cell-cluster level annotations, for each manually annotated cell type, we assigned the reference label with assignment scores summed across all single cells in that manually annotated cell type as the predicted cell type annotation.
ScType 15 (version 1.0) R package was used to perform cell type annotations with default settings. To meet the need for computational efficiency when working with large datasets, we developed an in-house version of ScType. We utilized vectorization to optimize the most time-consuming steps, while still generating the same output of the original ScType software. The input gene expression matrices to ScType were the same as used in SingleR described above. The built-in cell type marker database was used as the reference for all ScType annotations. Manually annotated cell types were treated as cell clusters and given as inputs to ScType. ScType directly generates cluster-level cell type annotations.
CellMarker2.0
CellMarker2.0 (ref. 13 ) only provides an online user interface and does not have a software implementation. We used the exact same marker gene sets or top ten differential gene sets identified by two-sided Wilcoxon tests for GPT-4 and GPT-3.5 cell type annotations as inputs of CellMarker2.0.
Evaluations of cell type annotations
Cell type annotations by GPT-4 or competing methods were compared to manual annotations provided by the original studies. Each manually or automatically identified cell type annotation was assigned an unambiguous cell ontology (CL) name 20 and a broad cell type name when applicable. A pair of manually and automatically identified cell type annotations was classified as ‘fully match’ if they have the same annotation term or available CL cell ontology name, ‘partially match’ if they have the same or subordinate (for example, fibroblast and stromal cell) broad cell type name but different annotations and CL cell ontology names, and ‘mismatch’ if they have different broad cell type names, annotations and CL cell ontology names.
To facilitate comparison, we assigned agreement scores of 1, 0.5 and 0 to cases of ‘fully match’, ‘partially match’ and ‘mismatch’ respectively, and calculated average scores within each dataset across cell types and tissues.
Simulation studies and reproducibility
To generate simulation datasets, we used canonical cell type markers through GTEx literature search of human breast cells, the top ten differential genes from the human colon cancer dataset, and the top ten differential genes from the vasculature tissue of the TS dataset as templates. Simulation studies were performed separately for the three tissue types.
To generate simulation datasets of mixed cell types, marker genes for each mixed cell type were created by combining the marker gene lists of two randomly selected cell types. Ten mixed cell types were generated in each simulation iteration. Additionally, we incorporated the original cell type markers of ten randomly chosen cell types as negative controls of single cell types. This entire simulation process was repeated five times. Subsequently, GPT-4 was queried using these simulated marker gene lists, and its performance in differentiating between mixed and single cell types was assessed.
To generate simulation datasets of unknown cell types, we compiled a list of all human genes using the Bioconductor org.Hs.eg.db package 21 . In each simulation iteration, ten simulated unknown cell types were generated. The marker genes for each unknown cell type were produced by combining ten randomly selected human genes. Additionally, we included ten real cell types and their marker genes as negative controls of known cell types, similar to the previous simulation study. This entire simulation process was repeated five times. Subsequently, GPT-4 was queried using these simulated marker gene lists, and its performance in distinguishing between known and unknown cell types was assessed.
To generate simulation datasets with partial marker gene information, we randomly subsampled 25%, 50% or 75% of the original marker genes. The simulation process was repeated five times. Subsequently, GPT-4 was queried using these subsampled marker gene lists, and the performance was assessed by agreement scores.
To generate simulation datasets with contaminated information, we added randomly selected human genes to the original marker gene list. The numbers of randomly selected genes are 25%, 50% or 75% of the number of original marker genes. The simulation process was repeated five times. Subsequently, GPT-4 was queried using these subsampled marker gene lists, and the performance was assessed by agreement scores.
We assessed the reproducibility of GPT-4 responses by leveraging the repeated querying of GPT-4 with identical marker gene lists of the same negative control cell types in simulation studies. For each cell type, reproducibility is defined as the proportion of instances in which GPT-4 generates the most prevalent cell type annotation. For instance, in the case of vascular endothelial cells, GPT-4 produces ‘endothelial cells’ eight times and ‘blood vascular endothelial cells’ once. Consequently, the most prevalent cell type annotation is ‘endothelial cells’, and the reproducibility is calculated as \(\frac{8}{9}=0.89\) .
GPT-4 API financial cost
According to information provided by OpenAI, the application programming interface (API) cost for running GPT-4 13 June 2023 version is $0.03 for every thousand input tokens and $0.06 for every thousand output tokens. For each query, we obtained i and o , which represent the numbers of input tokens and output tokens respectively, through the OpenAI API. The total API financial cost is thus calculated as $(0.00003 i + 0.00006 o ).
Reporting summary
Further information on research design is available in the Nature Portfolio Reporting Summary linked to this article.
Data availability
The data used in this manuscript are all downloaded from publicly available data sources. Specifically, HubMAP Azimuth data were downloaded from the Azimuth website ( https://azimuth.hubmapconsortium.org/ ). GTEx manually annotated cell types and differential gene lists were downloaded from the supplementary materials of the original study 5 . GTEx gene expression matrix was downloaded from the GTEx website ( https://gtexportal.org/home/datasets ). Marker genes from literature search were downloaded from the supplementary materials of the original study 5 . HCL manually annotated cell types and differential gene lists were downloaded from the supplementary materials of the original study 6 . HCL gene expression matrix was downloaded from figshare ( https://figshare.com/articles/dataset/HCL_DGE_Data/7235471 ). MCA manually annotated cell types and differential gene lists were downloaded from the supplementary materials of the original study 7 . MCA gene expression matrix was downloaded from figshare ( https://figshare.com/s/865e694ad06d5857db4b ). BCL gene expression matrix and manually annotated cell types were downloaded from Zenodo ( https://zenodo.org/record/7813151 ). Colon cancer gene expression matrix and manually annotated cell types were downloaded from GEO under accession number GSE132465 . Lung cancer gene expression matrix and manually annotated cell types were downloaded from GEO under accession number GSE131907 . TS gene expression matrix and manually annotated cell types were downloaded from UCSC Cell Browser ( https://cells.ucsc.edu/?ds=tabula-sapiens ). Marker genes and cell type annotations for the non-model mammal dataset were downloaded from the supplementary materials of the original study 12 . All relevant information about data is described in Methods . All data generated in this study are included in the supplementary tables.
Code availability
The GPTCelltype package (v.1.0.0) is provided as an open-source software package with a detailed user manual available in the GitHub repository at https://github.com/Winnie09/GPTCelltype . The software is released in Zenodo under https://doi.org/10.5281/zenodo.8317406 for all versions (ref. 22 ). All codes to reproduce the presented analyses are publicly available in the GitHub repository at https://github.com/Winnie09/GPTCelltype_Paper and also in Zenodo under https://doi.org/10.5281/zenodo.8317410 ( https://zenodo.org/record/8317410 ) (ref. 23 ). R version 4.0.2 was used to perform the analyses in the manuscript.
Hou, W. et al. GeneTuring tests GPT models in genomics. Preprint at bioRxiv https://doi.org/10.1101/2023.03.11.532238 (2023).
Hou, W. et al. GPT-4V exhibits human-like performance in biomedical image classification. Preprint at bioRxiv https://doi.org/10.1101/2023.12.31.573796 (2024).
Hao, Y. et al. Integrated analysis of multimodal single-cell data. Cell 184 , 3573–3587 (2021).
Article PubMed PubMed Central CAS Google Scholar
HuBMAP Consortium. The human body at cellular resolution: the NIH Human Biomolecular Atlas Program. Nature 574 , 187–192 (2019).
Article ADS CAS Google Scholar
Eraslan, G. et al. Single-nucleus cross-tissue molecular reference maps toward understanding disease gene function. Science 376 , eabl4290 (2022).
Han, X. et al. Construction of a human cell landscape at single-cell level. Nature 581 , 303–309 (2020).
Article ADS PubMed CAS Google Scholar
Han, X. et al. Mapping the mouse cell atlas by microwell-seq. Cell 172 , 1091–1107 (2018).
Article PubMed CAS Google Scholar
The Tabula Sapiens Consortium. The Tabula Sapiens: a multiple-organ, single-cell transcriptomic atlas of humans. Science 376 , eabl4896 (2022).
Article PubMed Central Google Scholar
Liu, N. et al. Single-cell landscape of primary central nervous system diffuse large B-cell lymphoma. Cell Discov. 9 , 55 (2023).
Lee, H.-O. et al. Lineage-dependent gene expression programs influence the immune landscape of colorectal cancer. Nat. Genet. 52 , 594–603 (2020).
Kim, N. et al. Single-cell RNA sequencing demonstrates the molecular and cellular reprogramming of metastatic lung adenocarcinoma. Nat. Commun. 11 , 2285 (2020).
Article ADS PubMed PubMed Central CAS Google Scholar
Chen, D. et al. Single cell atlas for 11 non-model mammals, reptiles and birds. Nat. Commun. 12 , 7083 (2021).
Hu, C. et al. CellMarker 2.0: an updated database of manually curated cell markers in human/mouse and web tools based on scRNA-seq data. Nucleic Acids Res. 51 , D870–D876 (2023).
Aran, D. et al. Reference-based analysis of lung single-cell sequencing reveals a transitional profibrotic macrophage. Nat. Immunol. 20 , 163–172 (2019).
Ianevski, A. et al. Fully-automated and ultra-fast cell-type identification using specific marker combinations from single-cell transcriptomic data. Nat. Commun. 13 , 1246 (2022).
Wei, J. et al. Chain-of-thought prompting elicits reasoning in large language models. Adv. Neural Inf. Process. Syst. 35 , 24824–24837 (2022).
Google Scholar
Wolf, F. A. et al. SCANPY: large-scale single-cell gene expression data analysis. Genome Biol. 19 , 15 (2018).
Article PubMed PubMed Central Google Scholar
Leek, J. T. et al. The sva package for removing batch effects and other unwanted variation in high-throughput experiments. Bioinformatics 28 , 882–883 (2012).
Mabbott, N. A. et al. An expression atlas of human primary cells: inference of gene function from coexpression networks. BMC Genomics 14 , 632 (2013).
Côté, R. G. et al. A new Ontology Lookup Service at EMBL-EBI. BMC Bioinforma. 7 , 97 (2006).
Article Google Scholar
Gentleman, R. C. et al. Bioconductor: open software development for computational biology and bioinformatics. Genome Biol. 5 , R80 (2004).
Hou, W. et al. GPTCelltype R software package. Zenodo https://doi.org/10.5281/zenodo.8317406 (2023).
Hou, W. et al. Repository of code to reproduce the analysis in this study. Zenodo https://doi.org/10.5281/zenodo.8317410 (2023).
Download references
Acknowledgements
Z.J. was supported by the National Institutes of Health under award number U54AG075936 and by the Whitehead Scholars Program at Duke University School of Medicine. W.H. was partially supported by the National Institute Of General Medical Sciences of the National Institutes of Health under award number R35GM150887 and by the General Fund at Columbia University Department of Biostatistics. The content is solely the responsibility of the authors and does not necessarily represent the official views of the National Institutes of Health.
Author information
Authors and affiliations.
Department of Biostatistics, Columbia University Mailman School of Public Health, New York City, NY, USA
Department of Biostatistics and Bioinformatics, Duke University School of Medicine, Durham, NC, USA
Zhicheng Ji
You can also search for this author in PubMed Google Scholar
Contributions
W.H. and Z.J. conceived the study, conducted the analysis and wrote the manuscript.
Corresponding authors
Correspondence to Wenpin Hou or Zhicheng Ji .
Ethics declarations
Competing interests.
The authors declare no competing interests.
Peer review
Peer review information.
Nature Methods thanks Qin Ma and the other, anonymous, reviewer(s) for their contribution to the peer review of this work. Primary Handling Editor: Lin Tang, in collaboration with the Nature Methods team. Peer reviewer reports are available.
Additional information
Publisher’s note Springer Nature remains neutral with regard to jurisdictional claims in published maps and institutional affiliations.
Supplementary information
Reporting summary, peer review file, supplementary table.
Supplementary Tables 1–5.
Rights and permissions
Open Access This article is licensed under a Creative Commons Attribution 4.0 International License, which permits use, sharing, adaptation, distribution and reproduction in any medium or format, as long as you give appropriate credit to the original author(s) and the source, provide a link to the Creative Commons licence, and indicate if changes were made. The images or other third party material in this article are included in the article’s Creative Commons licence, unless indicated otherwise in a credit line to the material. If material is not included in the article’s Creative Commons licence and your intended use is not permitted by statutory regulation or exceeds the permitted use, you will need to obtain permission directly from the copyright holder. To view a copy of this licence, visit http://creativecommons.org/licenses/by/4.0/ .
Reprints and permissions
About this article
Cite this article.
Hou, W., Ji, Z. Assessing GPT-4 for cell type annotation in single-cell RNA-seq analysis. Nat Methods (2024). https://doi.org/10.1038/s41592-024-02235-4
Download citation
Received : 16 April 2023
Accepted : 05 March 2024
Published : 25 March 2024
DOI : https://doi.org/10.1038/s41592-024-02235-4
Share this article
Anyone you share the following link with will be able to read this content:
Sorry, a shareable link is not currently available for this article.
Provided by the Springer Nature SharedIt content-sharing initiative
Quick links
- Explore articles by subject
- Guide to authors
- Editorial policies
Sign up for the Nature Briefing newsletter — what matters in science, free to your inbox daily.

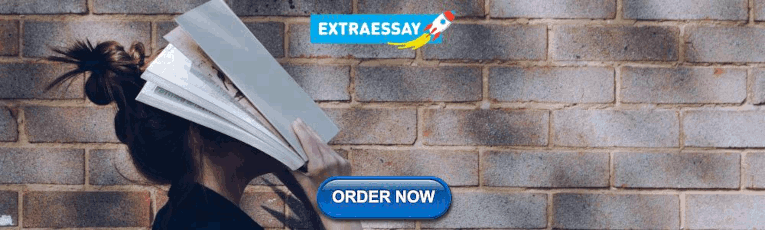
IMAGES
VIDEO
COMMENTS
Cost-effectiveness analysis is a way to understand the value of a health care intervention in terms of assessing the money spent to produce beneficial outcomes. Cost-effectiveness analyses are used by various stakeholders for such purposes because health care resources and financing may be scarce, depending on the economy, and certain ...
Economic Evaluation (EE) or cost-effectiveness analysis (CEA) is one of the important aspects of a health technology assessment. Classically, CEA is defined as a comparative assessment of two or more interventions, in terms of their costs and consequences. 1 As the definition suggests, any CEA would comprise two measurements - costs and consequences, which has to be carried out for both the ...
In 1996, the US Public Health Service's Panel on Cost-Effectiveness in Health and Medicine established a reference case analysis, a set of standard methodologies to improve the quality and comparability of CEAs that emphasized using QALY as a health outcome measure and applying a societal perspective. 2 A QALY measures the value of health ...
Of these, 7 studies reported incremental cost-effectiveness ratios below a willingness-to-pay threshold of US$50,000 for the gain of 1 QALY. 14,16,21,23,32,36,40 Only 1 study used QALYs and found ...
Cost-Effectiveness Analysis in Performance Assessments: A Case Study of the Objective Structured Clinical Examination Zhehan Jiang a Institute of Medical Education, Health Science Center, Peking University, Beijing, Peking, China;b National Center for Health Professions Education Development, Peking University, Beijing, Peking, China https ...
Cost-effectiveness analysis (CEA) ... The "e" in cost-effectiveness analyses. A case study of omalizumab efficacy and effectiveness for cost-effectiveness analysis evidence. Annals ATS. 2014;11:S105-S111. Google Scholar. Kreif N, Grieve R, Sadique MZ. Statistical methods for cost-effectiveness analyses that use observational data: a critical ...
The cost-effectiveness analysis was conducted using a basic decision-analytic modelling framework. This involved the use of prospectively collected meta-data, on time use and eligibility (screening) decisions made by the 'case study' review team, to model the changes in flows of eligible and ineligible study records and full-text reports through each stage of the screening process that ...
The Impact of Hospital Costing Methods on Cost-Effectiveness Analysis: A Case Study. Original Research Article; Open access; Published: 22 May 2018; Volume 36, pages 1263-1272, (2018) Cite this article
In this case, the measure that is ... The first shows the keywords most used in these studies, cost-effectiveness analysis, economic evaluation, and quality of life, through visualization by ...
Introduction. In 1996, the Original Panel on Cost-Effectiveness in Health and Medicine endorsed a societal perspective for reference case analyses, embracing the idea that analyses should reflect all relevant health and nonhealth consequences associated with the intervention. 1 Nevertheless, implementation of a societal perspective and choices about which nonhealth consequences should be ...
The "E" in Cost-Effectiveness Analyses. A Case Study of Omalizumab Efficacy and Effectiveness for Cost-Effectiveness Analysis Evidence ... Cost-effectiveness analysis (CEA) is a comparative method used to evaluate the costs and outcomes of interventions (7, 8). CEA offers a means of describing the tradeoffs associated with selecting one ...
There are 4 main types of economic evaluation, according to how effects are captured: cost-benefit analysis; cost-effectiveness analysis; cost-utility analysis (CUA; actually just a sub-set of cost-effectiveness analysis); and cost-minimisation analysis (Table (Table3). 3). . Each has its useful place, but CUA has the advantage of a common ...
By performing case management, general practitioners and health care assistants can provide additional benefits to their chronically ill patients. However, the economic effects of such case management interventions often remain unclear although how to manage the burden of chronic disease is a key question for policy-makers. This analysis aimed to compare the cost-effectiveness of 24 months of ...
This case study was used to produce an explicit comparison between Excel and R with Shiny interface in cost-effectiveness analysis. We have chosen a hypothetical case study using simulated data because the objective of this paper was to compare modelling platforms, rather than deriving a cost-effectiveness 'answer', and because the authors ...
The Impact of Hospital Costing Methods on Cost-Effectiveness Analysis: A Case Study. 2018 Oct;36 (10):1263-1272. doi: 10.1007/s40273-018-0673-y. Several methods exist to cost hospital contacts when estimating the cost effectiveness of a new intervention. However, the implications of choosing a particular approach remain unclear.
The Impact of Hospital Costing Methods on Cost-Effectiveness Analysis: A Case Study. José Leal, 1 Stefania Manetti, 2 and James Buchanan 1 ... However, the reference case does not explicitly exclude the use of national tariffs nor sets out the type of reference cost database to use. In our case study and at a threshold of £20,000/QALY, we ...
Abstract. Cost-effectiveness analysis is a way to understand the value of a health care intervention in terms of assessing the money spent to produce beneficial outcomes. Cost-effectiveness analyses are used by various stakeholders for such purposes because health care resources and financing may be scarce, depending on the economy, and certain ...
Abstract. This chapter provides an overview of how to design a cost-effectiveness analysis (CEA). The chapter highlights the importance of early conceptualization and planning steps to define the objectives, the research question, the perspective(s), the intervention(s), the target population, the comparators, the scope, the time horizon, and the analysis plan for a cost-effectiveness study.
Cost-Effectiveness Analysis in Performance Assessments: A Case Study of the Objective Structured Clinical Examination Med Educ Online. 2022 Dec;27 ... this brief report explores the real financial cost drivers associated with an assessment case in the context of medical education, presents the steps in bridging the effectiveness with its ...
This case study introduces concepts that should improve understanding of the following: 1. Markov models and their use in medical research. 2. Basics of health economics. 3. Replicating the results of a large prospective randomized controlled trial using a Markov Chain and Monte Carlo simulations, and. 4.
These final Reference Case Guidelines for Benefit-Cost Analysis in Global Health and Development. represent the conclusion of the ^ enefit‐ost Analysis Reference ase: Principles, Methods, and Standards _ project, initiated by the Bill & Melinda Gates Foundation in October 2016.
COST EFFECTIVENESS ANALYSIS - A CASE STUDY. NCJ Number. 48251. Journal. Bellringer Issue: 3 Dated: (NOV 1977) Pages: 6-8. Author(s) M P KIRBY; D CORUM. Date Published. ... A COST EFFECTIVENESS STUDY IS CONSIDERED TO BE RELATIVELY EASY TO COMPUTE, EASY TO UNDERSTAND, AND PERSUASIVE. (GLR) Additional Details Grant Number(s) 76-ED-99-0031 ...
Cost-effectiveness analysis is a way to examine both the costs and health outcomes of one or more interventions. It compares an intervention to another intervention (or the status quo) by estimating how much it costs to gain a unit of a health outcome, like a life year gained or a death prevented. Because CEA is comparative, an intervention can ...
For the base case data, the average cost per child recovered was 116.52 USD in the standard treatment and 107.22 USD in the CHWs-led treatment. Based on the first approach, the CHWs-led treatment was more cost-effective than the standard treatment with an average cost per child admitted for treatment of 82.81 USD vs. 84.01 USD.
Several studies have tried to manage the trade-offs among multiple policy objectives using multicriteria methods 20,21; however, there is a limited understanding of the overall cost-effectiveness ...
We used a case study approach, with 4 MLBC sites in Bangladesh, Pakistan and Uganda (12 sites in total) to collect data on costs and outcomes of MLBCs and conduct a modelled cost-effectiveness analysis. The purpose of the study was to inform decision-making about the expansion of this model of care.
One-way sensitivity analysis showed that although the incremental cost-effectiveness ratio remained below 5,000,000 yen (£30,925) for most of the parameters, the incremental net monetary benefit may have been less than 0 depending on the treatment efficacy outcome, especially the cure rate and mortality rate for MEPM and mortality rate for CTZ ...
Introduction The last COVID-19 vaccine offered to all adults in England became available from November 2021. The most recent booster programme commenced in September 2023. Bivalent BA.4-5 or monovalent XBB.1.5 boosters were given. During the study period, the JN.1 variant became dominant in England. Methods Vaccine effectiveness against hospitalisation was estimated throughout using the test ...
Recent studies have demonstrated their effectiveness in ... GPT-4 offers cost-efficiency and seamless integration ... W. et al. Repository of code to reproduce the analysis in this study.
Airborne magnetic particles may be harmful because of their composition, morphology, and association with potentially toxic elements that may be observed through relationships between magnetic parameters and pollution indices, such as the Tomlinson pollution load index (PLI). We present a fuzzy-based analysis of magnetic biomonitoring data from four Latin American cities, which allows us to ...