
An official website of the United States government
The .gov means it’s official. Federal government websites often end in .gov or .mil. Before sharing sensitive information, make sure you’re on a federal government site.
The site is secure. The https:// ensures that you are connecting to the official website and that any information you provide is encrypted and transmitted securely.
- Publications
- Account settings
Preview improvements coming to the PMC website in October 2024. Learn More or Try it out now .
- Advanced Search
- Journal List
- Philos Trans R Soc Lond B Biol Sci
- v.372(1735); 2017 Dec 5
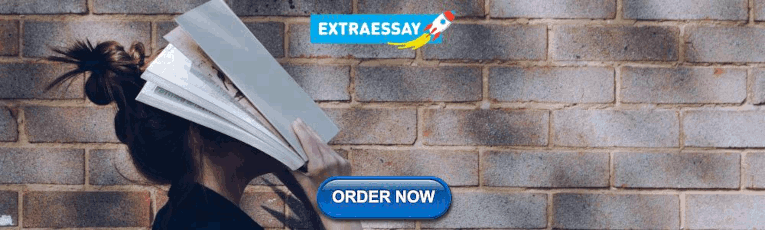
Innovation: an emerging focus from cells to societies
Michael e. hochberg.
1 Institut des Sciences de l'Evolution, Université de Montpellier, 34095 Montpellier, France
2 Santa Fe Institute, Santa Fe, NM 87501, USA
3 Institute for Advanced Study in Toulouse, 31015 Toulouse, France
Pablo A. Marquet
4 Departamento de Ecología, Facultad de Ciencias Biológicas, Pontificia Universidad Católica de Chile, Alameda 340, Santiago, Chile
5 Instituto de Ecología y Biodiversidad (IEB), Casilla 653, Santiago, Chile
6 Instituto de Sistemas Complejos de Valparaíso (ISCV), Artillería 4780, Valparaíso, Chile
Robert Boyd
7 School of Human Evolution and Social Change, Arizona State University, Tempe, AZ 85287, USA
Andreas Wagner
8 Department of Evolutionary Biology and Environmental Studies, University of Zurich, Winterthurerstrasse 190, 8057 Zurich, Switzerland
Innovations are generally unexpected, often spectacular changes in phenotypes and ecological functions. The contributions to this theme issue are the latest conceptual, theoretical and experimental developments, addressing how ecology, environment, ontogeny and evolution are central to understanding the complexity of the processes underlying innovations. Here, we set the stage by introducing and defining key terms relating to innovation and discuss their relevance to biological, cultural and technological change. Discovering how the generation and transmission of novel biological information, environmental interactions and selective evolutionary processes contribute to innovation as an ecosystem will shed light on how the dominant features across life come to be, generalize to social, cultural and technological evolution, and have applications in the health sciences and sustainability.
This article is part of the theme issue ‘Process and pattern in innovations from cells to societies’.
1. Introduction
A central feature of life is change. Change may be rapid, such as a population crash during a disease epidemic or the evolution of antibiotic resistance. Change may also be slow, a prime example being the evolution of novel biochemical pathways leading to altered phenotypic traits , possibly generating new species. Both rapid and slow change can occur at the level of the genotype and the phenotype, as well as in ecological aggregates such as populations and communities. Its magnitude ranges anywhere from imperceptible and difficult to quantify, to highly visible and transformational, impacting not only individuals and their interactions but also their biotic and abiotic environments. Innovations comprise a class of changes that signal an often a priori unexpected departure from a previous state (see glossary for definitions of this and other terms). Many of the conceptual foundations of innovation can be traced at least back to Darwin [ 1 , 2 ], who suggested that novelties could emerge through either natural selection or the co-opting of traits originally used for other functions. His ideas presaged some current concepts of innovation.
Innovations are important to understand because they are distinctive, apparently improbable changes to the phenotype, and yet pervade biology, culture and technology. Innovations are central to the evolution of complexity, to phenotypic diversity, and to the emergence and filling of new niches. Insights into the processes generating innovations are not only central to understanding life, but also for predicting the future of human society and natural ecosystems. The growth of innovation research is evidenced by a keyword search of articles published in ecology or evolution between 1997 and 2017, 1 which yielded an approximate fourfold increase in the fraction of articles using the keyword ‘innovation’ (currently about 1%).
The aim of this theme issue is to present our current understanding of innovations and the innovation process across a spectrum of systems and organizational levels, with the ultimate objective of advancing a theory of innovations. Each of the papers of this theme issue articulates with one or more of the steps in the innovation process depicted in figure 1 or the patterns they produce. Although the focus of most of the contributions in this theme issue is biological, given that ecological and evolutionary processes influence human culture, society, economics and technology, the insights emerging could contribute to a theory that encompasses complex behavioural systems, and be transferrable to applications in the biological and social sciences, ranging from conserving biodiversity to managing pathogens, pests and invasive species, to understanding the impacts of medical breakthroughs on human demography and the effects of global change on resource sustainability, and to forecasting the growth of cities, economies and technological breakthroughs. Therefore an important objective of this theme issue and the discussion below is to better understand the commonalities and contrasts of innovation in biology, culture and technology.

A simplified diagram of processes leading to innovation. An innovation is the result of inventions and their recombination that generates a novelty that spreads and evolves in conjunction with selective and stochastic effects. As emphasized by papers in this theme issue, the environment influences the emergence of innovations, which, in turn, impact the environment. Note that the conventional notion of biological innovation (as opposed to cultural and technological) downplays the role of sensory and cognitive processes in the generation, filtering and horizontal spread of information (and generation of possible novelties) among individuals. See main text for discussion.
2. What is an innovation?
Innovation and the related concepts of invention and novelty have been extensively discussed in the biological [ 3 ] and social sciences [ 4 ]. Given the many disciplines and systems to which innovations matter, a diversity of definitions is not surprising [ 5 ]. Even within disciplines, such as in biology, concepts such as evolutionary novelty are difficult to define rigorously [ 6 , 7 ]. In our opinion, synthesizing this vast literature (for discussion and reviews, see e.g. [ 8 – 18 ]) with the aim of finding a simple, one-size-fits-all definition of innovation may be futile, because the development of innovation as a concept has largely occurred at the discipline level with limited cross-talk, and different aspects of innovations are emphasized in different disciplines. Thus, regardless of how innovation is defined—mathematically, statistically or verbally—it invariably encompasses some degree of discipline- or system-specific subjectivity. Nevertheless, certain basic features common to most innovation concepts are worth highlighting.
In biology, although all innovations involve adaptive change, not all adaptations are innovations ( figure 2 ). This is because an innovation is a qualitatively new phenotypic trait that is associated with a step departure from an evolutionary trend ( figure 3 ). The terms innovation and novelty are often used interchangeably in the literature, but here we make the distinction that a novelty is an individual-level concept of qualitative phenotypic change, whereas only a subset of novelties become innovations, the latter having population, community, ecosystem and evolutionary consequences. Determining what constitutes a sufficient change in the phenotype to qualify as a novelty or an innovation is a major challenge ( box 1 ), as is quantifying (and possibly statistically evaluating) innovation ( box 2 ).

A classification of change. Most adaptations are not innovations (lower left), because the necessary conditions for an innovation (upper left) include both a qualitative change in a phenotypic trait (a novelty) and that the novelty has positive fitness; that is, it either spreads through the population (positive relative fitness and competitive elimination of the ancestor) or expands into a new niche (positive absolute fitness). Some qualitative trait changes have nearly neutral or even negative fitness effects, and either persist at low frequencies (latent innovations; upper right), or go extinct and may only return if specific mutation or recombination events occur, or (in the realm of human technology) if an invention is rediscovered and integrated into an existing process or product. See main text for discussion of additional conditions for innovation.

Illustration of how innovations may impact the state of a population through time. In (A), the population adapts, but does not innovate. It is also possible that no adaptation occurs during certain periods (zero slope) or that the population maladapts (negative slope; not shown). In (B), the population innovates without appreciably altering its ecological niche. Innovations (dotted segments) are infrequent step departures from the prevailing adaptive trend (solid segments). Innovations become increasingly frequent as the population grows in size (due to, for example, more mutations or more recombination events) and as the population accumulates or acquires more information (e.g. trait complexity and variants; cumulative culture). In (C), the population innovates (dotted segments) and in so doing invades and subsequently radiates in a new ecological niche. Four such populations (or species) are illustrated here (ancestor line A, the initial innovating lineage, and two subsequent derived populations/species, each innovation represented by a dashed black line followed by a solid line, the latter representing periods of adaptation). Note that ‘population state’ could be represented as any of a number of possible correlates of evolutionary adaptation, including population size, absolute fitness, relative fitness (selection), phenotypic complexity or cumulative culture. The trajectories in this figure therefore represent a hypothetical index that increases with adaptive (continuous) or innovative (step) change.
Sorites paradox.
Much of our understanding of innovations is based on descriptive, conceptual frameworks. An important problem of many conceptual frameworks is encapsulated in the Sorites paradox, where the categorical labelling of a state or function is subjective, yet different grades of the (subjectively) labelled state are quantitative measures themselves (e.g. [ 19 ]). For example, the determination of baldness in humans has no objective threshold as measured by the number of hairs on the scalp; however, a male with fewer hairs per unit scalp area could be said to be balder than someone with more hairs. The Sorites paradox highlights why some qualitatively new phenotypic traits are deemed ‘innovations’, and it highlights the role of consensus and subjective judgement in this labelling. It raises the unsolved question of how or whether relative levels or magnitudes of innovation (marginal to transformational) can be scientifically distinguished from one another (see box 2 ).
Statistical detection of innovation?
The very definition of new, novel, never before seen, makes most (if not all) innovations unknowable before they occur, or increasingly knowable (but still probabilistic) as candidate inventions emerge and novelties begin to spread. Relevant notions of feasibility and probability have been conceptualized as the ‘actual and the possible’ [ 20 ] and the ‘adjacent possible’ [ 21 ].
Recent computational and theoretical modelling work has addressed numerous themes relating to innovation, including patterns generated by the process of technological evolution [ 22 ], distinguishing novelty [ 23 ], quantifying the magnitude of innovation [ 24 ], measuring the time to attain an innovation [ 25 ], detecting innovation as revealed by power-law distributions of activities as proxies for inventions [ 26 ], and predicting innovations from the adjacent possible [ 27 ]. In the context of macroevolution, several interrelated approaches to assessing innovations have been proposed (reviewed in [ 28 ]; for adaptive radiations, see reviews in e.g. [ 14 , 29 ]). For example, Wagner [ 16 ] has established a rigorous definition of morphological novelty based on mechanisms leading to departures from homologous phenotypic characters (see also [ 30 ]). Heard & Hauser [ 31 ] proposed a two part test for key innovations, whereby (i) an ecological/functional change in a taxon is associated with increased speciation or decreased extinction rates compared with a sister taxon and (ii) a number of innovative and sister clades are treated as independent observations. Similarly, Bond & Opell [ 32 ] proposed three criteria for a key innovation: the trait must (i) be present in two or more organisms and inherited exclusively from their common ancestor, (ii) be functionally advantageous, and (iii) be capable of contributing to adaptive change. Rabosky [ 28 ] argues that components of these and other approaches are fraught with conceptual issues and/or methodological problems (see also [ 11 ]).
Based on the discussion above and on some of the contributions in this volume, we propose the following sequential criteria to identify innovations. Criterion 1 : The new trait is a qualitative departure from the ancestral trait, as revealed by a change in phenotypic information or complexity. The change can in principle be quantified using information theory [ 33 ] (see also [ 34 ]). This criterion aims to exclude adaptations that result from a loss of traits [ 10 ]. Criterion 2 : The qualitatively new phenotypic trait is associated with one or more measures of increased performance and/or utilization of a novel niche. Criterion 3 : The novel trait confers positive relative fitness (if in same niche) or positive absolute fitness (if entering a new niche). Criterion 4 : The growth of the population harbouring the novelty is associated with significant community and ecosystem impacts, and in the longer term (particularly for niche innovations), adaptive radiation of the innovated lineage. In the spirit of figure 3 , we suggest that these four criteria can each be assessed statistically as (significant) deviations from prevailing trends. Given difficulties in obtaining sufficiently rich data to evaluate these criteria (e.g. [ 34 ]), we expect that their application will be difficult if not impossible for many systems.
A novelty stems from a series of inventions (mutations in biology; discoveries, ideas, or new devices in culture and technology) and/or recombination events to a blueprint , buttressed by facilitating phenotypic traits, such as standards . A novelty may affect structure (e.g. biochemical pathways, tissue architecture, appendages) and/or function , and particularly for the latter be associated, for example, with the ways organisms acquire resources, evade predators, interact with other organisms and modify their environments through niche construction or technologies.
Novelties may derive either from de novo constructs, or from an existing phenotypic trait that is co-opted as an exaptation , that is, from a trait originally associated with other functions or no function at all [ 35 – 37 ]. Although rarely investigated in detail, because a novelty balances benefits to survival and reproduction with costs due to trade-offs and initial maladaptation, the invasion fitness of a novelty may be neutral or even negative ( figure 4 ; e.g. horizontal gene transfer [ 38 ]). This means that some inventions never become competitive novelties (a fascinating example in technology being the Einstein–Szilard refrigerators [ 39 ]), whereas others do, but only emerge as innovations at some later time (‘latent innovations’; figure 2 ), either when additional mutations or recombination events occur, or when the environment becomes favourable. By contrast to the hypothesized roles of selection in innovation, the implications of drift are more contentious [ 40 , 41 ] and require further study (see also [ 42 ]).

Simplified representation of the fitness of an innovated lineage through time. Inventions (e.g. mutations) and recombinations lead to a novelty, which may show an increase in fitness of the novel phenotype relative to the ancestral phenotype (dashed line), no change (not shown) or a net cost (solid line). The latter may occur if major rearrangements underlie the novelty, or if environmental conditions are not favourable. To potentially become an innovation, the novelty (or states leading up to it) must spread in the population, or promote population invasion into new niches, and either of these may require some combination of refinements (e.g. DNA mutations that compensate costs; ameliorations that make a technology more efficient or productive), adaptations in other phenotypic traits and conducive environmental conditions.
It is useful to distinguish two basic types of novel traits leading to innovation.
In performance innovations , the novel phenotypic trait is associated with increases in performance , without fundamental changes to the organism's ecological niche (figures (figures3B, 3 B, B,5B). 5 B). To become an innovation, such a trait needs to confer a fitness advantage and spread through the population 2 ( figure 4 ), and in so doing impact the surrounding community or ecosystem, and possibly create longer-term opportunities for further adaptation and innovation. In the economics and technological spheres, such innovations are often associated with the replacement or ‘creative destruction’ of existing processes or products, akin to some scenarios of competitive exclusion in ecology. The novelty underlying this type of innovation is typically found at lower phenotypic levels, such as, for example, a biochemical pathway permitting more efficient metabolism or tissue repair, or processes enabling the use of low-grade (less expensive) silicon for producing solar cells.

Effect of innovation on the niche. Niche breadth before a hypothetical innovation is shown by the solid curve. In (A), the innovation is associated with simultaneous expansion (right extreme) and contraction (left extreme) of the niche. In (B), the innovation permits increased effectiveness, efficiency and/or yield, resulting in greater domination of part of the same niche. In (C), the innovation is associated with entering a new niche.
In niche innovations , the novel phenotypic trait is associated with the utilization of a new ecological niche (figures (figures3C 3 C and and5C). 5 C). These are sometimes referred to in biology as key innovations (e.g. [ 14 , 28 , 31 , 43 ]). Entering a novel niche or ecological space may or may not result in higher fitness relative to the source population, because competition with the source population may have little or nothing to do with the success of the novelty in the new ecological niche. Similar to performance innovations, this type of novelty becomes an innovation if invasion fitness into the new niche is positive and the emerging population impacts the surrounding environment (i.e. the community and ecosystem). Niche innovations notably create opportunities for adaptive radiation. Novelties underlying niche innovations typically introduce a new function, such as homeothermy in mammals enabling survival over a wider range of temperature conditions, or radically alter an existing function, for example, the microprocessor enabling personalization of computers and telephones.
Importantly, these two types of novelty/innovation are not mutually exclusive: a novelty may affect both performance and the niche ( figure 5 A), as opposed to only intensifying domination of an existing niche ( figure 5 B), or only creating a new niche without changes in performance ( figure 5 C). Mirroring their effects on niches, innovations also produce phylogenetic pattern. By increasing in frequency and eventually becoming common and refined, innovations can produce some combination of replacing previous phenotypes, or entering a new niche and fostering adaptive radiations.
3. The scope of innovation and the innovation ecosystem
Historically, the study of innovation as a stand-alone concept is often associated with human society, culture and technology. Research topics range from the evolution of important technologies like hafting and fire, to the social transition of hunter–gatherers and the more sedentary agriculturalists who domesticated animals and plants [ 44 ], to the growth of cities and states, and the development of an immense array of technologies [ 45 – 49 ]. Indeed, niche construction, the manipulation of the environment resulting in increased prospects for survival and reproduction, is a recurring theme that bridges innovative phenomena in biology and social sciences [ 50 , 51 ]. Whereas most if not all innovations stemming from human activity can be interpreted as forms of niche construction, only a subset of those in biology fit this definition.
Although innovation has been (arguably) a less dominant theme in biology compared with the social sciences, the related concept of novelty was an important topic of discussion during the Modern Synthesis, and both innovation and novelty have garnered increased attention over the past few decades. Innovations in biology are often gauged in terms of their transformative effects on form and function. The most revolutionary include transitions in individuality [ 52 ] and fundamental adaptations to the environment [ 53 ]. Some innovations are polyphyletic (e.g. the ‘minor–major’ transition of multicellularity [ 54 ]), others are monophyletic (e.g. photosynthesis), whereas others—particularly in the human sphere—are more challenging to accurately determine in terms of spatial and temporal origins [ 55 ]. Cultural innovations in humans and in other animals include the ability to use (tools) or modify the environment [ 56 ], specific (social or asocial) behaviours [ 57 ] and adornment that influences mating success [ 58 ]. Moreover, several studies have investigated the tendency to innovate itself as a trait (with little supporting evidence, [ 59 , 60 ]), or as a correlate of either life-history traits such as maximum lifespan in birds [ 61 ], or cognitive traits such as brain size in birds and primates [ 62 , 63 ].
These and other general phenomena hint at an important feature of innovations: they usually involve multiple levels of organization [ 16 , 30 , 64 , 65 ]. Examples include an enzyme's newly acquired ability to catalyse a chemical reaction that allows an organism to survive in a new environment [ 15 ], gene expression changes that help generate novel tissues which provide a quantitative advantage for development [ 66 ], or the metabolism and musculature supporting the function of a new and advantageous body structure, such as a limb or wing, which may help in locomotion [ 67 ]. Moreover, in being a qualitative departure from a previous state, an emerging novelty will have consequences for the functioning and fitness contributions of existing traits, meaning that organisms harbouring such a novelty will be under selection at the loci of other traits to accommodate the novelty as it spreads and becomes an innovation ( figure 4 ).
A useful way to understand these multiple levels of organization and their environmental interactions is as composing an innovation ecosystem . Table 1 presents a highly simplified representation of the biological levels at which inventions, novelties and innovations operate in the innovation ecosystem of winged flight in birds. Winged flight in birds emerged as an innovation in their reptile ancestors, ultimately contributing to taxonomic diversification in the Aves [ 81 , 82 ]. For flying animals such as bats and birds, wings were derived from forelimbs, and thus the innovation is not the de novo emergence of a trait, but rather the radical modification (exaptation) of an existing trait. For wings to be an innovation supporting flight, it is necessary that (i) wings specifically fulfil the proximal function of generating lift for flight, (ii) flying individuals have the scaffolding (e.g. musculature, energy allocation, cognition) required for flight and (iii) the trait complex is maintained by selection. Thus, gliding species such as certain squirrels and snakes do not fit this definition because they do not possess appendages that enable uplift, but neither do kiwi birds, which have vestigial wings. Ostriches are flightless, but can use their wings for balance and direction changes while running. Albatrosses, on the other hand, have built on the innovation of winged flight through adaptations permitting air travel over thousands of kilometres without landing, in great part due to behavioural aerodynamics (using wind updrafts). Determining whether a phenotypic trait is an innovation based on the presence/absence or quantitative criteria alone is difficult, because the trait itself may not be specific to the function being innovated and vice versa [ 17 ]. Thus, as alluded to above, birds may use their wings for one or more of several functions in addition to flight, including flap-running, paddle-swimming, thermoregulation, brooding and displays [ 67 ].
Table 1.
Winged flight as an innovation ecosystem. This table shows multiple (non-exhaustive) biological levels of organization, how they are associated with invention, novelty and innovation, and how these associations manifest in the example of winged flight in birds, which originated in dinosaurs and persists to this day in their avian descendants with numerous adaptive and novel modifications [ 68 , 69 ], and loss of flight in certain lineages. ‘Winged flight’ is a convenient label for an innovation, but the underlying adaptations and novelties form a trait complex, and together with environmental interactions, compose an innovation ecosystem. All components of this innovation ecosystem (e.g. developmental pathways, tissue architectures, and behaviours) contribute to winged flight, and generic examples given (e.g. in the ‘molecules, cells, tissues’ level) are non-exhaustive. Components may themselves be novelties or innovations, may be singular to wings and flight, and may have been co-opted or modified from other trait complexes (see e.g. [ 70 ] for the origins of feathers). Symbol x ( ? ) indicates that the entity does (may) affect the associated biological level.
4. The roles of evolution and ecology in innovation
Whereas the basis of evolution and its importance in biology can be traced back more than 150 years [ 1 ], foundations in culture [ 83 , 84 ], economics [ 85 ] and technological change [ 48 , 86 , 87 ] are much more recent. Although there is a discipline-specific, historical component to the integration of evolutionary thought in studying innovations, we claim that our limited understanding of innovation ultimately derives from the complexity of the underlying processes ( figure 1 ).
At a coarse scale, biological evolution (heritability, mutation, recombination, drift and selection) has analogues in culture and technology. But even if operational in broadly similar ways across these disciplines, there are contrasts at finer scales in how different steps of the evolutionary process operate. This system/process-level specificity is associated with substantial scientific challenges, such as, for example, the identification of heritable information [ 88 ], understanding the roles of simple and complex cognitive and social processes [ 18 ] in cultural and technological systems, and assessing the extent to which biological evolution is in any way ‘goal-oriented’. Regarding the latter, insofar as some sexually reproducing organisms exhibit mate choice, they influence the identity of their mate and thus indirectly, which genetic material is given an opportunity to recombine and help produce their offspring. Although this process may generate novelty in sexually selected characters (e.g. peacock's tails), evidence is limited as to whether it accelerates natural selection in the short term [ 89 ], or facilitates phenotypic novelty in the longer term [ 90 ].
Evolution is the exploration of the possible, which leads to the to-be possible. This exploration, however, occurs in a space subject to constraints, which affect the emergence of novelties and the speed at which they may become innovations ( box 3 ). Obtaining a novelty may require multiple iterations in the generation of inventions and recombinants (see e.g. [ 93 ] for why single mutations are unlikely to produce complex adaptations), whereby intermediates either initially persist without changing appreciably in frequency (e.g. the Dykhuizen–Hartl effect [ 94 ]), or are positively selected and increase in frequency until the novel trait is fully formed and spreads through the population [ 95 – 97 ]. For example, Blount et al . [ 95 ] experimentally demonstrated how multiple clades of Escherichia coli persisted for over 10 000 generations before a citrate metabolism trait arose in one lineage through the capture of a promoter exapting a previously silent duplication of the citrate transporter. Wallbank et al . [ 97 ] showed that introgression between lineages of Heliconius butterflies can produce novel colour patterns based on the recombinatory shuffling of cis -regulatory modules.
The limits to innovation.
Scaling theory in biology and technology offers several examples of contrasts between different organisms or products that—although apparently substantially different—are nevertheless variations on the same theme. In the accompanying figure, we show two cases. The first involves metabolic rate. Even though a unicellular organism is clearly different than an elephant, both actually satisfy the same statistical scaling law between mass and metabolic rate. Metabolic innovations, however, are strongly suggested by the visible discontinuities (changes in the intercept) that define qualitatively different groups (poikilotherms and homeotherms) (cf . discontinuities portrayed in figure 3 ). The second example involves motors, both biological and engineered, and their force output. Here, the constraints appear more severe, resulting in that the maximum force output generated by a fly's muscles satisfies the same constraint as the force output of a jet engine. Thus, the ‘space of the possible’ in this relationship is constrained by simple, general rules stemming from the fundamental laws of chemistry, physics and biology.
Ecological interactions and the environment impact survival, reproduction and dispersal, and may play a key role in the transition from novelty to innovation. A good example is the competition among technologies that serve the same purpose, such as transportation. Invented by 1834, electric cabs looked to outcompete horse-drawn cabs at the turn of the twentieth century, but after a period of national and international expansion they failed (and so did the whole electric car industry) and by 1930 were outcompeted by internal combustion engine vehicles. This event was due not only to the quality of the technology itself, but also to insufficient environmental conditions, and in particular to battery limitations and the lack of electric infrastructure in the form of central stations [ 98 ]. Interestingly, the same technology re-emerged in California during the 1990s owing to an environmental factor (air pollution), as the Zero Emission Vehicle Mandate required major car companies to make available zero emissions vehicles [ 99 ]. But again electric vehicles failed, this time owing to reasons including lack of customer interest, competition with other industries, and insufficient cooperation with key technological developers in the battery sector. More recently, the environmental context has become favourable for electric vehicles, owing to both concerns about climate warming and the longer charge period and lifespan of electric batteries.
5. Overview of the theme issue
The idea for this collection of reviews, perspectives and original research stems from an interdisciplinary workshop entitled ‘Origins of novelty in biological, social and technological systems: towards a general theory of innovation’, held at the Santa Fe Institute in October 2014. It was clear from the workshop that the field is ready for synthesis, and that a reasonable step forward is to centre this synthesis in the biological sciences, with bridges to culture and technology. Box 4 and the discussion below summarize important topics addressed by contributions to this theme issue, and their promise for future research.
The importance of innovation.
A deeper understanding of innovative change will be built on a research programme integrating behaviour, ecology, evolution, environment, network theory and information theory. This programme is important for several reasons and generates numerous challenges.
- — Understanding innovation contributes to crystallizing notions of the predictability of evolution . How predictable (or inevitable) are innovations in the biological and in the cultural and technological realms? This is important because a major distinction between biology and culture/technology is the latter's (apparent) goal-oriented, rapid generation and filtering of ideas and inventions (the sensory and cognition box in figure 1 ; see also [ 100 ]). Indeed, there is evidence that some innovations in culture/technology stem from serendipitous events [ 101 , 102 ]. In biology, novelties are similarly difficult if not impossible to predict [ 103 , 104 ].
- — Innovations and population growth are expected to be mutually reinforcing . Features of a population including its size, social interactions and established standards [ 102 , 105 , 106 ] influence the probability of innovation. Innovation may foster ecological opportunity, which in turn promotes population expansion and adaptive radiation [ 14 , 107 ]. In cultural evolution where this phenomenon is also reasonably well studied, population growth may promote cultural evolution [ 108 ] and demographic change [ 109 ]. Therefore, population growth and innovation are expected to be mutually reinforcing [ 26 , 110 – 113 ].
- — Innovation is often associated with solving problems and/or realizing opportunities . Problems or challenges occur within the current niche and include unfavourable abiotic conditions and difficulties in obtaining resources. Realizing opportunities may involve operating in other niches, and include access to more favourable abiotic conditions and the availability of novel resources (for biology and technology, see [ 114 ]; for behavioural innovations, see [ 115 , 116 ]). To the extent that unsolved problems severely limit population size (and the associated likelihood of inventions), does this also stymie innovation (e.g. [ 117 ])?
- — Studying innovation is central to understanding the diversity and complexity of life . Do innovations eventually saturate or become progressively smaller in magnitude? Or rather, is innovation open-ended [ 41 ]? Are environmental changes, disturbances or population extinctions necessary to ‘reset the clock’, providing adaptive space for new innovations? Does innovation simplify system structure in some contexts and complexify it in others? Under what conditions does organism complexity [ 118 ] or system robustness [ 119 ] affect innovation?
- — Innovation instructs on associations between adaptation and fitness . Mean population fitness is expected to increase for adaptations to the current ecological niche, but mean fitness may increase, decrease or remain unchanged when adapting to a new habitat (for discussion and examples, see [ 120 ]). In producing a (considerable) rewiring of phenotypic traits and being constrained by trade-offs, do niche innovations decrease certain measures of fitness? Headway in understanding the fitness dynamics of innovation has recently been made using an experimental evolution approach [ 121 ].
- — Innovations influence existing trade-offs and may create new ones . Trade-offs occur when there is competition involving phenotypic traits, gene expression, hormones and signalling molecules, and resource allocation [ 122 ]. In rewiring the phenotypic traits of an organism, or influencing levels of selection [ 123 ], will the emergence of a novelty also shift traits along existing trade-off surfaces or change the shapes of trade-offs themselves? This can be viewed metaphorically as altering the ruggedness and shifting the fitness peaks on a fitness landscape, or adding new trait dimensions to a multidimensional landscape [ 124 ].
- — Innovations may have negative externalities and evolve in terms of their function and impact on the environment . The impacts of innovations on the surrounding environment can be complex, a prime example being domestication [ 125 ]. Innovations can have net benefits, but also negative externalities, such as, for example, the effects of domestication on farm animal well-being and associated land use changes (see e.g. [ 126 ], and theoretical development in [ 110 ]). In addition, the same innovation can have different functions or uses, such as the exaptation of fertilizer components for making explosives [ 127 ]. Vermeij & Leigh [ 114 ] argue that human-driven innovative systems are more prone to negative externalities than are natural systems.
- — A general theory of innovation will identify processes that unite biological, cultural and technological spheres . Many authors have compared and contrasted evolutionary frameworks for innovation between biology, culture and technology (e.g. [ 48 , 51 , 106 , 114 ]). Is there a level of resolution at which all innovations follow the same basic rules? Or rather, are the apparent differences between innovations in generational (slow evolutionary) systems and intra-generational (fast) systems actually just different points along a continuum?
(a) Searching for and discovering innovation
A central theme linking the contributions to this theme issue is how innovations, which appear a priori unlikely or infeasible, can originate. Wagner [ 34 ] aims to address this problem by connecting evolutionary biology to information theory. Classical population genetics focuses on genotypes and the information encoded therein, but more recent work highlights the importance of phenotypes as gatherers and processors of environmental information [ 128 ]. Wagner employs information theory to quantify the information content of a phenotype and to investigate the feasibility of acquiring or ‘discovering’ novel and beneficial phenotypes. He develops a metric of the information change associated with a new phenotype (see also box 2 ), and illustrates its use in the context of DNA duplication (one route to more evolvable genotypes and a mechanism for exaptation), new transcription factor binding sites on DNA (resulting in new gene regulation and new phenotypic traits), and genes encoding biochemical reaction networks that transform environmental nutrients into biomass (e.g. amino acids and nucleotides). A limitation of this framework is that very large numbers of individuals may need to be sampled in order to estimate phenotypic information content and its change associated with novel phenotypes. Wagner proposes to mitigate this problem by using sequence data sampled from evolution experiments to quantify information differences between phenotypes. Although this approach also has limitations, for example, if a novel phenotype is highly information-rich, or requires many mutational steps to be ‘discovered’, Wagner shows that current technology suffices to quantify phenotypic information gain if the number of individuals sequenced from a population is sufficiently large relative to the amount of genetic variation in the population. In addition, Wagner suggests that the information-theoretic framework can address the broad question of what makes a system evolvable, by positing that evolvable phenotypes have low information content. Quantifying evolvability can help answer important questions about the rates at which species diversify (see also [ 28 ]), and about the related concept of the open-endedness of evolution in exploring spaces of possible adaptations.
With the exception of a handful of well-studied model systems, most involving microbes (e.g. [ 129 ]), and inferences linking major changes in phenotypic traits and phylogenetic structure (reviewed in [ 14 ]), we know little about how evolution brings about structural/functional novelty and innovation. The question is whether the evolutionary searches of the adjacent possible described by Wagner [ 34 ] typically describe more attainable, but less revolutionary innovations than many of those observed over macroevolutionary time, which could involve families of adaptations and innovations bundled into a single phenotypic feature (e.g. bird wings, table 1 ). Erwin [ 103 ] discusses this important topic, starting with an overview of the intellectual history of ‘spaces’, two of the most intuitive being how the states of different genes or different phenotypic traits in an individual are associated with fitness. But the metaphor of spaces extends to ecological strategies or ‘ecospaces’, and relationships between phenotype and function. Erwin develops the idea that a static predetermined landscape of how evolution could proceed is a considerable oversimplification of how many novelties and innovations occur. He argues that over macroevolutionary time scales, adaptive multidimensional surfaces change in topology and new dimensions are added and (these or others already existing) potentially lost. Moreover, the topology of many spaces is likely to be non-Euclidian, meaning that probabilities of shifting from A to B some topological distance away could be complex, and even change during the macroevolutionary process. Changes to topology are a form of a priori blind ‘construction’, in that contingency in population states and environments provides the fuel for generally unpredictable changes in fitness landscape topology, including trade-offs between phenotypic traits. Erwin summarizes the importance of construction versus search in three points. First, topologies are complex, such that, locally, they are knowable and searchable, but as the space becomes more distant and less defined it is unlikely that search algorithms apply. Second, spaces evolve. Third, novelty and innovation alter spaces and generate new spaces. Erwin wonders whether novelty and innovation are space-topology specific, but notes that the pervasive observation of phenotypic convergence between otherwise independent taxa suggests that topological constraints exist and, as such, innovation is not (entirely) open-ended. More research is needed to investigate the implications of topological construction.
There are considerable challenges in evaluating competing hypotheses to explain macroevolutionary patterns in innovation. Rabosky [ 28 ] addresses some of the main issues in statistically evaluating the impact of key innovations on adaptive radiation. Key innovations are important testing grounds for innovations more generally, because in opening a new ecological space, the former are a bellwether for the rates and extent of adaptive radiation (e.g. [ 29 ]). The expectation is that ecological opportunity is created when a new adaptive zone is opened, and innovations (e.g. functional novelties) are one possible source of that opportunity [ 14 ]. Rabosky evaluates innovations on phylogenetic trees, and begins by briefly reviewing the large literature on phylogenetic inference and the interpretation of innovations. A persistent problem is the circularity of the concept (key innovations occur when traits permitting invasion are obtained) and consequential difficulties in testing it. An alternative is to view the key innovation as an evolutionarily successful trait that results in increased diversification rates. Rabosky argues on the contrary that differential rates of lineage diversification should not be used to evaluate the existence of key innovations. If species richness is largely regulated by diversity-dependent (equilibrial) mechanisms, then there is little theoretical justification for linking innovations to faster diversification. This harkens to the idea that speciation generally entails both reproductive isolation and changes to the ecological niche (e.g. [ 130 ]); opening up a new niche (a key innovation) could facilitate the total number of new species, but does not necessarily increase the rates at which the species are formed. Rabosky argues that most tests of the predictions of key innovation have serious limitations, a central one being the low information content of many time-calibrated phylogenetic trees. He concludes that future work should focus on ecological and evolutionary mechanisms in generating macroevolutionary patterns and put less weight on oversimplified hypotheses.

Scaling in biology and technology. Left panel shows relationship between temperature-corrected metabolic rate, ln ( I exp( E / kT )), measured in watts, and body mass, ln M , measured in grams. Variables are M , body size; I , individual metabolic rate; k , Boltzmann's constant; T , absolute temperature (in K). E is the activation energy (after [ 91 ]). Right panel shows the scaling of force output for different biological and engineered motors (after [ 92 ]).
(b) Challenge and opportunity
The contributions to this theme issue highlight the fundamental importance of scale in innovation. Scale in evolution is evidently spatial and temporal, but more generally it characterizes the information stored and transmitted over networks. Information is in the genotype and in the phenotype, but also, as Wagner [ 34 ] relates, in the environment. The concept of information is a calculus. Information is differentiated in microevolutionary time through mutation, recombination and selection, and integrated in macroevolutionary time when living systems change in size and complexity. This raises the intriguing question of how the rate, magnitude and nature of innovations change as a system evolves from inception to maturity, and then possibly to senescence, decline and death [ 110 ]. West et al . [ 131 ] consider the inception of what is arguably the first major transition in life—heredity—and more specifically how membrane replication in protocells is an important step towards the emergence of nucleic acid blueprints and more complex cellular structures. Most theory regards RNA as the key initiator of heredity in biology. But as West et al . argue, there are some practical difficulties in heredity ‘jump-starting’ with RNA. Rather, innovations associated with the emergence of nucleic acids were possibly preceded by an innovation of membranes themselves as the blueprint of protocellular reproduction and inheritance. Here, the genotype is in many respects also the phenotype. The authors present an evolutionary, computational model based on the biochemistry of some of the earliest cells [ 132 ]. Leaky fatty-acid vesicles grow and reproduce based on positive feedbacks emerging from the chemical dynamics of proton gradients across the membrane, in interaction with mineral catalysts (FeS crystals), amino acids and fatty acids in the outer and inner environments. Even this conceptually simple, empirically based model is complex, and although data for parameter value estimates are either rudimentary or lacking, the canonical values used are plausible: when sufficiently high catalytic rates and tight amino acid binding constants are assumed, the protocells grow and reproduce. As the system evolves based on selection for larger quantities of organics inside the protocell, the likelihood of the next novelty—RNA heredity—becomes feasible. Once RNA and polymerase enzymes emerge, selection shifts in favour of a trade-off between replication speed and growth, avoiding parasitic collapse. This study highlights the pervasive observation that more complex, sophisticated levels of individuality are more likely to obtain and (generally) perform better if cheaters are prevented, kept at bay or eliminated.
Arguably, one of the most fundamental forms of innovation is the transition from autonomous individuals to the coordinated cooperating collective and to the new integrated individual [ 133 ]. Ratcliff et al . [ 123 ] consider how the evolution of collective life cycles could be associated with transitions to higher-level individuality. A major obstacle to transitions to higher-level individuality is the difficulty with which lower-level autonomy is lost, reflected by defection and freeloading. Interestingly, even when a transition is achieved, lower-level autonomy is never completely relinquished, as evidenced for example, in cancers [ 134 ]. Key to how life cycles select for higher-level dominance is the extent to which life cycle behaviours create variability within versus between collectives. Greater heritable variation between groups compared with within groups favours cooperation and transitions to higher-level individuals [ 135 ]. Life cycles that reproduce through a single-cell bottleneck and subsequently develop clonally are thus the most likely route to multicellularity. Ratcliff et al . review the life cycles of key systems, including Pseudomonas fluorescens biofilms, snowflake yeast, volvocine algae, and choanoflagellates as examples of different stages of transitions in individuality. The authors use mathematical models to compare and contrast the propensity for different life cycles to produce a transition by assessing the likelihood that a beneficial mutation affecting lower- and higher-level fitness would spread in the population. Previous work identified ‘ratcheting mutations’ (beneficial to collectives but deleterious to single cells) as those most likely to transfer command to the higher-level individual; here the authors show that these mutations should spread most quickly when multicellular individuals develop clonally and the life cycle lacks a persistent unicellular phase. Interestingly, the transition in the cases of contingent multicellularity mentioned above each require only a single mutation, suggesting the ease with which the initial novelty can emerge, which would most likely be built-upon by a battery of further genetic changes to achieve the obligate multicellular individual.
The study by Ratcliff et al . shows the complexity of innovations leading to multicellular emergence in the face of cheats. Cheating can take a number of non-mutually exclusive forms including: not executing a cooperative function, not contributing to the public good or abandoning the group. But, in leading to what amounts to competition or parasitism, cheating separates a population into two or more populations, one of which is coordinated and cooperative, and the other(s) that may form a single population of autonomous individuals, or break up into a heterogeneous ensemble of lineages or quasi-species. A key expectation is that both the cooperative ‘host’ and the cheating ‘parasite’ will evolve (and possibly coevolve). This opens the possibility that some adaptations in cooperators and cheaters will entail qualitative novelty and ultimately emerge as innovations. Aktipis & Maley [ 134 ] explore the routes to and maintenance of multicellularity, and draw parallels between innovations in cellular systems and those in human society. Although the analogies remain to be investigated in detail, there is appeal in the basic insight that similarities exist between relinquishing autonomy to form complex, cooperative structures such as multicellular organisms, and in complying with norms, morals, rules and laws in human society. Aktipis & Maley discuss how the tension between cooperation and cheating in multicellular organisms leads to innovations in the host, such as programmed cell death and immune systems that protect against disease from within and disease caused by other organisms. Moreover, innovations may emerge in cellular cheaters, such as invasive neoplasms (cancer), examples including evading the immune system, adapting to and changing the microenvironment, and dispersing to colonize new, hospitable tissues and organs [ 136 ]. Similar reasoning applies to visitors or residents in the organism, such as beneficial, neutral or pathogenic species and strains in the microbiota [ 137 ]. The authors conclude that insights into how cellular societies innovate so as to protect themselves could be translated into medical approaches that improve human health.
Social cheating as a form of parasitism is an example of a more general manifestation of ecological abundance: where there are resources to consume, there will be consumers; where there are living organisms to predate or parasitize, there will be predators or parasites. The natural question is to know how hosts avoid the negative fitness effects of parasites, and how parasites can counter any such adaptations in the host. There is a considerable empirical and theoretical literature on antagonistic coevolution (e.g. [ 138 , 139 ]), but the origins of new adaptive strategies still remain unclear. Fortuna and coworkers [ 140 ] investigate this question using self-replicating computer programs (i.e. digital organisms) that interact and evolve in a user-defined computational environment. This approach has proved very useful for understanding evolutionary and coevolutionary processes [ 141 , 142 ]. The authors find that when host resistance traits emerge additionally from non-adaptive origins (i.e. exaptations), coevolution leads to complex and phenotypically diverse networks of interacting hosts and parasites. The resulting coevolutionary outcome not only is predicted to increase population size and thereby facilitate additional adaptations and innovations, but also increases the persistence of entire communities. Interestingly, innovation mediated through exaptations may scale up to generate novel network structures that promote community persistence. These novel structures can be interpreted as forms of niche construction, and in a sense are innovations themselves.
(c) Transmission, selection and construction of novelty
The two most discussed routes to innovation are de novo construction of a qualitatively new phenotypic trait and exaptation of such a trait through gene duplication or the modification of single non-functional or functional genes [ 15 ]. There is however another, oft neglected, mechanism in biology, and in culture and technology: the introduction of novelty from another population or another species, and the diffusion of novelty between individuals within a population. The importance of horizontal gene transfer or HGT has been recognized since the 1950s, stemming from its role in the transfer of antibiotic resistance, but it is only recently that an appreciation for its diversity, complexity and importance has emerged. A defining characteristic of many prokaryotes is the fuzziness of the species concept. This is largely due to their ability to transfer and accept genetic material between closely and distantly related individuals, but also between what we would consider to be different species [ 143 ]. Accepting genetic material implies that—at least sometimes—there is a fitness benefit that maintains the character in the lineage, but also that other life forms or mobile genetic elements such as phages and plasmids benefit from this permissivity on the part of their hosts. Hall and colleagues [ 129 ] explore how the diverse battery of HGT mechanisms contributes to the introduction and diffusion of novelties in bacteria. Hall and coworkers take significant strides towards an evolutionary framework for how HGT has been so successful and effectively conquered many conceivable routes towards expression and carriage in the recipient individual. Unique here is that the would-be innovation is likely to have been of adaptive significance (and possibly an innovation) in the donor species or lineage. The authors make the important point that owing to the multitude of traits under selection in HGT, notions of the boundaries of what is an organism can become complex. In the context of innovation, the question is whether the novelty is shared between the transferred element and the donor, a sort of mutualism, which under certain environmental conditions can turn the relationship into conflict, more resembling parasitism. In either mutualistic or parasitic scenarios, there will be selection on the interacting entities to enhance or restrict subsequent transfer events, opening a world of possibilities of how HGT impacts other life-history traits, creates ecological opportunity resulting in adaptive radiations, and more generally how we view parasitism and mutualism as engines of novelty and innovation.
Horizontal information transfer such as that occurring in HGT is akin to the social transfer of information in multicellular animals. Social species span the gamut from microbes to men, but it is in the latter and in non-human animals where the transmission of novel information is best understood [ 18 , 126 , 144 ]. Specifically, a defining behaviour in many animal species is their ability to learn from others (social learning) and in so doing, incorporate the novelty (and the diffusion of the innovation through the population). However, the importance of network structure and social learning in the diffusion of innovations is difficult to assess. This is in part because agents may also learn asocially through trial and error [ 145 ]. Hoppitt [ 146 ] assesses methods for characterizing how an innovation diffuses through a social network of non-human animals when individuals can learn from others or, rather, learn through trial and error. The problem is that most past analyses have assumed basic one-on-one interactions, and have not developed more sophisticated tools for analysing more realistic social networks. Hoppitt evaluates an approach called network based diffusion analysis (NBDA) which can be used to (a) detect the action and strength of social transmission relative to asocial transmission, and (b) determine the typical pathways for diffusion of innovations in populations of animals. Hoppitt assesses what types of network should be used in an NBDA in order to accomplish each of these two goals. One problem encountered in such analyses is that the data may contain biases and noise. Hoppitt investigates different network types, among them observation networks, where social transmission can be inferred if the order of individual observation predicts the order of diffusion, and association networks where the probability of transmission is expected to correlate with the association time. He finds that these two network types are robust to bias and error in parameters. However, numerous other challenges remain, important among them being network-specific interpretations of social learning strength in the diffusion of innovations.
Many cultural variants achieve appreciable population frequencies without any apparent selective effect [ 147 , 148 ]. Examples in present-day humans include fads, fashions, adornment and first names. Defining characteristics of such cultural variants include their rapid emergence, spread and decline, coexistence with alternative variants, and their promotion via the copying or the emulation of prestigious individuals [ 149 ]. One hypothesis to explain their near-neutrality, is that the present-day manifestations of these behaviours reflect evolutionary mismatches with what were more important phenomena in our distant past, and that the background behaviours promoting them have persisted. This does not preclude that the mechanisms responsible for their dynamics function the same way today as they did in the distant past. O'Dwyer & Kandler [ 42 ] explore the dynamics of what would appear to be a trait with no effect on survival or reproduction—baby's names—with the objective of determining the extent to which transmission might follow neutral or non-neutral processes. Bentley et al . [ 150 ] previously showed a power-law distribution of newborn names, with an exponent that depends on invention rate and total population size. O'Dwyer & Kandler derive an analytical approximation for this progeny distribution under neutrality and show that the distribution of names over an interval of intermediate abundances shows an exponent −3/2, but this exponentially declines at and beyond sufficiently abundant names. Maximum-likelihood estimates of the neutral parameter allow direct application of the theory to data. Moreover, the authors show how selective differences between names, in the form of pro- and anti-novelty bias, can be modelled and applied to a data set of newborn names in Australia. They find that the empirical patterns are best described by anti-novelty selection against inventions before they reach appreciable frequencies, but once they do, selection is relaxed and names interact in a neutral manner. These results have potential implications for how marker information (i.e. neither too common, nor too unique) is achieved in the human population. Furthermore, the authors show that analyses based on only the most common cultural variants can lead to misleading inferences about underlying transmission processes.
Two defining characteristics of humans are the complexity of their innovations and the remarkable diversity of their innovation toolbox. Innovation complexity and diversity reflect cumulative culture, that is, building culture over lifetimes and through generations. Whereas models and data indicate the probable routes of cumulative culture [ 151 ], less is known about how culture forms within the individual. Building culture during a lifetime requires repeated cycles of individual learning (a form of invention) and copying others (e.g. parents, peers, and prestigious individuals). A critical period in endogenizing is childhood. McGuigan and colleagues [ 152 ] review the growing literature on the significance of childhood in cumulative culture, and identify the underlying ‘dual engines’ as invention and copying/transmission. The authors then present a study of children in which the subjects were free to invent, rather than have adult models introduce inventions. The authors focus on the scenario where the origin of certain inventions that contribute to cumulative culture occur during childhood [ 153 ]. The experimental design is complex, similar in spirit to an ethological field study. Despite inherent limitations in interpreting these kinds of controlled but complex experiments, the study reveals that social learning can facilitate more cumulative innovation than asocial learning, that higher-level problem solving was more likely following success on a simple problem in a series, and that cumulative success could be driven both by the challenges of ecological loss of existing solutions and by the involvement of occasional superior innovators (older children). The broader picture is that humans, and ostensibly certain other primates, may be adapted to experiment and selectively transmit successful outcomes, providing a basis for the exaptation or amelioration of existing behaviours and the emergence and spread of novelties that become cumulative cultural innovations.
(d) Interacting with, constructing and destructing the environment
The abilities of life forms to generate inventions, refine novel phenotypic traits, and diffuse some of these to become innovations are, sometimes, innovations themselves. Cognition and information processing is a vast space for such innovations. Dukas [ 154 ] provides an overview of the foundations of cognition and associated innovations leading to ever-greater complexity, particularly in mammals. An important theme in the development of cognition is the perception of danger (e.g. temperature, predators) and opportunity (e.g. resources), in particular information gathering and transmission in a social environment. A basic form of information gathering found in single cells, from bacteria to vertebrate immune systems, relies on receptors and responses, both expressed via gene networks. These mechanisms, although rapid, are limited in the range of environmental responses they can accommodate. A major innovation in animals was the advent of the nervous system, which promoted more effective environmental sensing and inter-individual communication, and further innovation enabled capacities for information storage and retrieval. The advent of learning and long-term memory required the employment of existing standards, and in particular, biochemical chains and changes in gene expression. Learning and memory, in turn, provided the basis for further behavioural innovations and in particular, social learning. Thus, each successive innovation has either incorporated or complemented previous cognitive innovations. Dukas relates how social learning would have been selected in taxa with repeated interactions between individuals and long life-spans. This highlights a unique aspect of social and individual learning: expertise. Expertise is how experience (through improved skills and increased knowledge) improves the performance of complex tasks. Once social learning was established, there would have been significant selection pressure to adapt it to complex social and asocial environments. Language fills this gap, and produces new opportunities and challenges that result in further adaptations for improved cognitive ability (e.g. [ 155 ]) and (its correlate) further innovation [ 61 ]. More research is necessary, particularly in understanding how evolution underpins innovation epochs in cognition and expertise, and how these employ automated and plastic responses.
The evolution of ever more sophisticated cognition in response to environmental opportunities and challenges is a prime example of how phenotypic traits diversify and complexify in form and function. Signalling (including language) and social learning would have had major consequences for how well organisms could cope with and exploit changing environments. One of the most fundamental ways in which this is accomplished is through altering the environment: niche construction. Niche construction is a powerful conduit of adaptation and innovation (e.g. [ 156 ]). However, niche construction is not limited to the extra-organismal environment—it can involve the organism's phenotype itself, through for example, ‘phenotypic accommodation’ [ 156 , 157 ]. Arguably, the main distinction between the (classic conception of) the organism and its environment is that the former is an autonomous, reproducing entity. Beyond this, there may or may not be a disjunct in how information is stored and transmitted between an organism and its environment [ 158 ]. Niche construction is therefore an extension of the phenotype, subjected to selection, and manifests in gene–culture coevolution [ 159 ]. Allaby et al . [ 44 ] review one of the most fascinating forms of niche construction: domestication. Briefly, one species selects for sought-after traits in another, especially predictability, value and yield, and in so doing the former evolves through the development of management techniques and technologies. Although domestication and agriculture in particular occur across a broad range of taxa (slime moulds, snails, beetles, termites, ants), nowhere are they more diverse and complex than in the human population. Allaby et al . relate how true domestication results from directional selection on major traits, whereas the specific forms of agricultural traits in crop improvement or selection for particular varieties are often idiosyncratic to specific needs or environments. The authors discuss the well documented example of cereals, such as wheat and barley, where archaeobotanical evidence suggests that morphological change occurred over thousands rather than hundreds of years, indicative of generally weak selection, but not incompatible with periods of strong selection due to environmental influences, social conditions, and prevailing technology. Allaby et al . evaluate this hypothesis using a combined model–data analysis of the fossil record of crop domestication to predict periods when human-driven selection in several cereals was particularly strong, and estimate when the earliest selection for domestication began. They find that selection is indeed generally weak, and that periods of stronger selection may occur in parallel with technological innovations such as sickle technologies. Additionally, when many traits are under selection and no two are necessary for crop improvement, selection tends to be diluted on most traits and over most time periods. The authors relate how environmental and cultural contingencies were crucial to domestication rates and particular sought-after traits. Similar to other cultural and technological innovations (e.g. [ 105 , 158 , 160 ]), it appears that sufficient human populations sizes would have been necessary for cereal domestication and production innovations to obtain.
The potential for biological evolution ultimately derived from mutation. Because many if not most mutations have negative impacts on fitness, genomes have adaptations to control mutation. The stochastic nature of mutation means that their appearance (i.e. nearly neutral or beneficial mutation) depends on population size. Although controversial, this same dependence—large population size—has been hypothesized to favour cumulative cultural evolution [ 161 ]. Fogarty & Creanza [ 111 ] review the literature, which suggests that this process operates in food producing societies but not in food gathering societies, the latter of which depend more on environmental risk. The authors develop a model to investigate the independent and interactive influences of population size and environment on cultural accumulation in the form of tools and technologies. They assume that innovation is primarily driven by environmental challenge, but that innovations are to some extent environment-specific, meaning that innovations are potentially gained and lost when populations migrate or environments change [ 55 , 162 , 163 ]. However and importantly, those populations that construct their environments (e.g. building shelters, domesticating plants and animals and associated agriculture and husbandry) buffer themselves from environmental vagaries, and population size will dominate environmental contingency in driving innovative cultural accumulation. Taken together this harkens at predictions of evolutionary theory whereby adaptation to harsh or variable environmental conditions may be achieved by increased mutation rates or phenotypic plasticity, whereas adapting to long-term predictable environmental trends depends on occasional beneficial germ line changes (mutation), which become more probable with population size [ 164 ].
How novelty manifests itself in terms of phenotypic traits, functions and fitness depends on where we look in the innovation ecosystem, the time frame in the emergence and development of the innovation, and the prevailing environmental conditions. Innovations may be beneficial for one species and detrimental for other species, as for example, an innovation permitting a host to better resist its parasite, although as shown by Fortuna et al . [ 140 ] the precise effects can be complex. More generally, an innovation may be detrimental to individuals, populations or ecosystems in several non-mutually exclusive ways. First, an initially beneficial innovation may be expatiated or co-opted to become a detrimental one, as in the case of dynamite invented for mining but subsequently then used for artillery. Second, an innovation may outcompete existing phenotypes, resulting in their demise or extinction (‘creative destruction’ in economics and technology). Third, the innovation may have negative externalities, such as pollution produced by combustion engines. And fourth, in favouring population growth, the innovation may result in overexploitation of resources and the tragedy of the commons. Weinberger et al . [ 110 ] consider innovation in the human population and how it can sustain growth or rather result in population collapse or even extinction. They model how innovation increases the flux of ecosystem services, which boosts population size and results in further innovation and population growth via cumulative cultural evolution. A key point underscored by the authors is that innovations can also have social and environmental costs, which limit population growth [ 165 ]. The authors find that continued innovation and population growth is only possible if the positive externalities of the innovations are sufficient, which allows a large stock of technologies. When externalities are sufficiently negative, the population can only persist, albeit at lower numbers, if the stock is reduced to a minimum. Finally, the model shows that population collapses are possible in between these two extremes, and these further depend on the minimum standard of technology humans are willing to accept. This study suggests that the coevolutionary dynamics between population and innovation critically depend on the positive or negative externalities of innovations and on the changing minimum technological requirements of populations.
6. Concluding remarks
Innovation research encompasses a vast spectrum of phenomena, spanning the biological, cultural and technological sciences. This theme issue takes modest steps in surveying and investigating the scope of interesting problems surrounding innovation, and yields a number of insights that will guide future directions. The conceptual frameworks, mathematical and computational models and experiments in this theme issue support the view that only a small number of key mechanisms may be necessary to understand innovations. Nevertheless, robust assessments of putative driving processes are elusive, particularly for innovations manifesting over long time periods. In this regard sufficiently rich data can reveal macroevolutionary patterns through, for example, ancient DNA analysis [ 166 ]. At the other extreme, the recent explosion of experimental evolution [ 104 , 167 , 168 ] and ever-increasing sophistication in behavioural studies [ 18 ] have important roles to play in scientifically evaluating competing hypotheses—depending on the system—over time scales amenable to many research programmes. Mathematical and computational models will be central in evaluating candidate processes driving innovation, regardless of time scales.
Despite our suggestion that contributors to this theme issue adhere to a common definition of the term ‘innovation’, there were notable contrasts. These spanned the gamut from single quantitative changes to the phenotype through to qualitative novelties requiring multiple mutations and recombination events. All had in common either some form of phenotypic novelty, or a major step in the performance of a function. This state of affairs reflects both contrasts in perspective of where adaptation stops and innovation begins, and differences in the complexity of the topology of evolutionary spaces on the path to innovation. We view this as an enriching, challenging reality of the current state of the field.
The contributions to this theme issue highlight the intricacy of the innovation ecosystem, which consists of networks of phenotypic traits on different scales, associated changes in behaviour and function, performance and fitness, and current and future impacts on the surrounding community and ecosystem. Clearly, developing a constellation of theories for such multi-layered systems will be a considerable undertaking, and insights into how the complexity of novel traits limits their occurrence and influences their evolvability [ 169 ] will be key to theoretical developments. Given current lacunae, it is not surprising that innovation largely remains an a posteriori assessment of change and its impact. Future study should identify the milestones that precede and predict innovations.
In closing, we believe that innovation processes in biology, culture and technology have a small set of key features in common. The main defining contrast appears to be the dynamics and complexity of sensory–cognition–communication interactions ( figure 1 ). In this regard, we claim that these disciplines are not as distinct as they may appear, but rather they form an overlapping continuum. The moon-shot is to understand the central features that drive innovation in all living systems, paving the way for a general theory.
Acknowledgements
We thank the authors for their hard work and the quality of their contributions to this theme issue, and the many external reviewers who provided constructive feedback. We would also like to thank Jennifer Dunne, Doug Erwin, Stuart Kauffman, Mimi Koehl, Hanna Kokko, Manfred Laubichler, Jose Lobo, Doyle McKey, John Miller, Rolando Rebolledo, Deborah Strumsky, Peter Turchin, Jorge Velasco-Hernandez and Andrew Whiten for their support and discussions, Helen Eaton for advice, the contributors to this theme issue for their comments on this introductory paper, and to the Santa Fe Institute for facilitating our discussions over the past several years.
Editor profiles
1 Conducted on 15 August 2017 using the ISI ‘All Databases’. Search term used for each year between 2000 and 2017 was (‘innovation*’ and (‘ecolog*’ or ‘evolut*’))/(‘ecolog*’ or ‘evolut*’). The annual fraction has increased at a slightly greater than linear rate between 1997 and 2017.
2 ‘Spread’ as employed here includes scenarios where phenotypic variants contributing to the full novelty attain appreciable population frequencies prior to the emergence and spread of the full novelty itself.
Authors' contributions
M.E.H. wrote the manuscript. All authors contributed significantly to writing and revisions.
Competing interests
We have no competing interests.
M.E.H. acknowledges the James S. McDonnell Foundation Studying Complex Systems Research Award no. 220020294, the CNRS PICS Award no. 06313, the Agence National de la Recherche (EvoCan ANR-13-BSV7-0003-01), and the Institut National du Cancer (2014-1-PL-BIO-12-IGR-1), for funding. P.A.M. acknowledges support from Grants Programa de Investigación Asociativa (PIA), Anillo SOC1405, ICM-MINECON P05-001 and PFB-CONICYT P-053. R.B. was supported by grant no. 48952 from the John Templeton Foundation to the Institute of Human Origins at Arizona State University. The opinions expressed in this publication are those of the authors and do not necessarily reflect the views of the John Templeton Foundation. A.W. acknowledges support by ERC Advanced grant no. 739874, by Swiss National Science Foundation grant no. 31003A_146137, by an EpiphysX RTD grant from SystemsX.ch, as well as by the University Priority Research Program in Evolutionary Biology at the University of Zurich.
- Tools and Resources
- Customer Services
- Business Education
- Business Law
- Business Policy and Strategy
- Entrepreneurship
- Human Resource Management
- Information Systems
- International Business
- Negotiations and Bargaining
- Operations Management
- Organization Theory
- Organizational Behavior
- Problem Solving and Creativity
- Research Methods
- Social Issues
- Technology and Innovation Management
- Share This Facebook LinkedIn Twitter
Article contents
Organizational innovation.
- Fariborz Damanpour Fariborz Damanpour Rutgers Business School, Newark and New Brunswick
- https://doi.org/10.1093/acrefore/9780190224851.013.19
- Published online: 22 August 2017
Innovation is a complex construct and overlaps with a few other prevalent concepts such as technology, creativity, and change. Research on innovation spans many fields of inquiry including business, economics, engineering, and public administration. Scholars have studied innovation at different levels of analysis such as individual, group, organization, industry, and economy. The term organizational innovation refers to the studies of innovation in business and public organizations.
Studies of innovations in organizations are multidimensional, multilevel, and context-dependent. They investigate what external and internal conditions induce innovation, how organizations manage innovation process, and in what ways innovation changes organizational conduct and outcome. Indiscreet application of findings from one discipline or context to another, lack of distinction between generating (creating) and adopting (using) innovations, and likening organizational innovation with technological innovation have clouded the understanding of this important concept, hampering its advancement. This article organizes studies of organizational innovation to make them more accessible to interested scholars and combines insights from various strands of innovation research to help them design and conduct new studies to advance the field.
The perspectives of organizational competition and performance and organizational adaptation and progression are introduced to serve as platforms to position organizational innovation in the midst of innovation concepts, elaborate differences between innovating and innovativeness, and decipher key typologies, primary sets of antecedents, and performance consequences of generating and adopting innovations. The antecedents of organizational innovation are organized into three dimensions of environmental (external, contextual), organizational (structure, culture), and managerial (leadership, human capital). A five-step heuristic based on innovation type and process is proposed to ease understanding of the existing studies and select suitable dimensions and factors for conducting new studies. The rationale for the innovation–performance relationship in strands of organizational innovation research, and the employment of types of innovation and performance indicators, is articulated by first-mover advantage and performance gap theory, in conjunction with the perspectives of competition and performance and of adaptation and progression. Differences between effects of technological and nontechnological innovation and stand-alone and synchronous innovations are discussed to articulate how and to what extent patterns of the introduction of different types of innovation could contribute to organizational performance or effectiveness. In conclusion, ideas are proposed to demystify organizational innovation to allure new researches, facilitate their learning, and provide opportunities for the development of new studies to advance the state of knowledge on organizational innovation.
- innovation and organization
- and innovation
- process of innovation
- typologies of innovation
- antecedents of innovation
- innovation and firm performance
- technological innovation
- managerial innovation
Introduction
Research on innovation spans many fields of inquiry including science and engineering, humanities and art, and social sciences. In academia, innovation has been probed at different levels of analysis: individual, group, organization, industry, economy. The term organizational innovation refers to the studies of innovation in organizations, including both business and public organizations. Organizational innovation research examines what external and internal conditions induce innovation, how organizations manage innovation process, and in what ways innovation changes organizational conduct and outcome.
Innovation in organizations is conceived both as process and outcome. Research on innovation as outcome aims to identify the contextual, organizational, and managerial conditions under which organizations innovate. Research on innovation as process aims to identify how organizations create, develop, adopt, implement, and use innovation. Outcome and process research are denoted as studies of innovativeness and innovating , respectively. The studies of innovativeness are primarily large-sample studies of multiple innovations in organizations. The studies of innovating are mainly case studies of one or few innovations in organizations.
The term organizational innovation is simple and easily understandable, but research on organizational innovation is complex—multilevel, multidimensional, and context-dependent. First, innovation overlaps with several other concepts—creativity, invention, imitation, organizational and technological change—and is often used as an umbrella concept covering all. Conceptual diversity and indiscriminate use confounds the antecedents and outcomes of organizational innovation. Second, organizations can both generate (create) and adopt (use) innovations. Generation and adoption are distinct processes that occur typically at different parts or units of organizations. They are not necessarily induced in similar environmental contexts or organizational conditions, and are not necessarily affected by the same sets of antecedents. Third, organizations generate and adopt different types of innovation—product, process, technological, and managerial, major or minor. Many authors do not distinguish between innovation types, and use the term innovation while studying only one type (especially technological and product). The role and importance of innovation types differ along the value chain (Porter, 1985 ), suggesting that the contextual and organizational conditions that could motivate their adoption are not similar. Fourth, myriad theoretical perspectives and approaches (rational, institutional, political, cultural, learning, interpretive, interactional), each constrained by its disciplinary discourse and methodological disposition, are applied to ground studies of organizational innovations (Crossan & Apaydin, 2010 ; Damanpour & Gopalakrishnan, 1998 ; Sturdy, 2004 ; Van de Ven & Rogers, 1988 ). They offer competing explanations of motivation for and consequences of the generation and adoption of innovation in organizations.
However, despite the complexity of the construct and diversity of research on it, the term innovation is applied broadly and the research findings are interpreted generally. Lack of due attention to the differences emanating from disciplinary approaches, levels of analysis, generation or adoption, innovation types, and external and internal contexts challenge a common understanding of organizational innovation. Accordingly, basic questions of importance to practice such as “what are the characteristics of innovative organizations” and “how do innovations affect organizational conduct or outcome” remain unanswered. The goal of this article is to carve out the key facets and dimensions of organizational innovation, coalesce its elements, and combine insights from existing research to inform and help guide future research on its dimensions, antecedents, and outcomes. While the article draws insights from innovation research in economics, psychology, and sociology, its primary disciplinary focus is organization studies, and in particular management of innovation in organizations. 1
The rest of this article is organized as follows. First, two general perspectives for studying innovations in organizations are offered. The definition of innovation and its distinction from the related concepts are presented next. This is followed with an overview of generation, adoption, and typologies of innovation in organizations, and of their salient antecedents. Finally, consequences of innovation for organizational conduct and outcome, and ideas for future research on organizational innovation are discussed.
Perspectives of Organizational Innovation
Academic research on innovation in social sciences has markedly increased since the 1950s (Crossan & Apaydin, 2010 ; Fagerberg, 2005 ). Innovation, however, is a much older term and its meaning, understanding, and terminology have changed over time. Godin ( 2015a ) chronicles emergence, evolvement, conceptualization, and application of innovation leading to its contemporary understanding. Innovation has a positive connotation and is viewed as a practical construct with beneficial outcome for its generators and adopters. Organizations generate and adopt different types of innovations that are deemed to be of value to meeting their short-term and long-terms goals and making their operation efficient and effective. Accordingly, the domain of research on organizational innovation encompasses organizational activities and mechanisms for the creation (generation) and application (adoption) of new technological or nontechnological ideas and practices across their value chain.
Two primary perspectives of innovations in organizations were introduced in the 20th century . The first perspective focused mainly on the generation of new commercialized, technology-based products and processes (Damanpour & Wischnevsky, 2006 ; Fagerberg, Mowery, & Nelson, 2005 ; Godin, 2008 ). Organizations innovate to improve efficiency and productivity, increase market share and profitability and to generate economic wealth for their owners. The second perspective was introduced in sociology and flourished in organization management in the second half of the 20th century alongside the advent of organizations as open systems. Organizations introduce innovations to adapt to environmental change and achieve strategic intents for maintaining and improving performance (Hage & Aiken, 1970 ; Becker & Whisler, 1967 ; Mohr, 1969 ; Zaltman, Duncan, & Holbek, 1973 ). Whereas both perspectives consider organization as a vehicle for innovation, from the first perspective innovation is mainly to increase productivity and serve product, service, and performance outcomes, and from the second perspective innovation is mainly a means of organizational change and improvement to stay in business and thrive. The two perspectives are viewed here as conjoining, not competing, and are termed as “competition and performance” and “adaptation and progression” perspectives of organizational innovation.
Organization Competition and Performance
This perspective is rooted in Schumpeter’s work and focuses mainly on the development and launch of new products and technological processes by organizations, where the newness is gauged at the level of product class or market. Schumpeter ( 1934 , 1983 ) defined innovation (new combination) as a novel output and distinguished among five types of innovation: new products (new goods and new quality of goods), new methods of production, new markets, new sources of supply, and new ways to organize business (Fagerberg, 2005 ; Schumpeter, 1983 ). 2 These typologies were introduced in the context of economic development and technological change, where innovation concerns radical, discontinuous change due to the occurrence of productive revolutions driven by new firms through technology push (Damanpour & Wischnevsky, 2006 ; Schumpeter, 1983 ). This work is known as Schumpeter’s entrepreneurial model of innovation (or Mark I), which champions entrepreneurial start-ups and their contributions to economic growth (Barras, 1986 ; Fagerberg, 2005 ). Innovation is the essence of new, independent companies creating new industries or acting as major agents of change in established industries (Barras, 1990 ; Sanidas, 2005 ).
In his later work, Schumpeter also noted the role of incumbents—established firms—as a source of innovation for economic development (Barras, 1986 ; Fagerberg, 2005 ; Schumpeter, 1950 ). This work is referred to as Schumpeter’s corporate model of innovation (or Mark II), where established firms are the vehicles for innovation because they possess scientific knowledge and management expertise, production means and other complementary assets, better access to capital, and often some degree of monopoly power, which increase the likelihood of investing in innovation (Barras, 1990 ; Damanpour & Wischnevsky, 2006 ; Sanidas, 2005 ). According to this view, established organizations (like start-ups) drive efficiency and effectiveness through innovation and create economic wealth for owners/investors, and eventually the society at large.
Schumpeter’s innovation models have mostly been applied to the generation of new technology-based products and processes. When entrepreneurial opportunities—situations in which new businesses, products, processes, and services can be introduced (Shane & Venkataraman, 2000 )—exist, individuals and organizations alike engage in innovation (Damanpour & Wischnevsky, 2006 ). Entrepreneurs (members of a large and dynamic population of innovators) pursue these opportunities by starting new organizations; incumbents (members of a small and stable population of innovators) pursue them by forming new businesses, alliances, and joint ventures. The primary motivation for seizing new opportunities for both individuals and organizations is to increase productivity and profitability and to create economic wealth and growth (Drucker, 1985 ).
In organization management, the competition and performance perspective of innovation is prominent in business policy and strategy, global (international) business, and technological and strategic entrepreneurship (Grant, 1996 ; Teece, Pisano, & Shuen, 1997 ; Hitt, Ireland, Camp, & Sexton, 2001 ; Lengnick-Hall, 1992 ). 3 However, the focus of this perspective on innovation as technological advancement in the industrial (commercial) entities restricts its application to other types of innovation and broader types of organizations. Hence, it alone is not sufficient to fully comprehend organizational innovation.
Organization Adaptation and Progression
Organizations in all sectors, whether commercial or noncommercial, private or public, innovate to operate efficiently and perform effectively. Organizations introduce all types of innovations, whether technological or nontechnological, product or process, radical or incremental. Innovation is not only to gain competitive advantage over rivals, it is also a means of organizational adaptation and progression. Sustained performance or effectiveness can be gained not only by generating innovation (new to market or industry) but also by adopting innovation (new to the adopting organization). While organizations can develop competencies to generate one or few types of innovation, they can adopt all kinds of innovations along their value chain (Baldridge & Burnham, 1975 ; Hage & Aiken, 1970 ; Ross, 1974 ).
The adaptation and progression perspective assumes that organizations innovate to respond to environmental change, renew business portfolios, and serve their customers or clients effectively in order to achieve strategic positions and boost long-term performance (Damanpour & Gopalakrishnan, 1998 ; Roberts & Amit, 2003 ). It gained currency in the second half of the 20th century after the importation of system theory to organization studies (Ackoff & Emery, 1972 ; Churchman, 1968 ; von Bertalanfy, 1951 , 1968 ), advancement of behavioral theory of the firm and contingency theory (Burns & Stalker, 1961 ; Cyert & March, 1963 ; Lawrence & Lorsch, 1967 ), and the advent of the long-term planning and business strategy (Ackoff, 1970 ; Ansoff, 1968 ; Schendel, Ansoff, & Channon, 1980 ). Organization is defined as an open system that is composed of interdependent parts (subsystems) and is embedded in an environment with which it exchanges and interacts (Ackoff, 1981 ; Emery & Trist, 1960 ; Scott, 1992 ). The environment is also a system, albeit larger and more complex than the organization, with its own subsystems and environment. It is usually divided into two levels: general (macro) environment, and transactional (micro, operating, competitive) environment (Daft, 2001 ; Mintzberg, 1979 ). Changes in either environment prompt organizational actions to maintain external fit (balance with environmental components) and internal fit (harmony among internal subsystems). Effectiveness of the organization requires carrying out the systemic processes of maintaining, adapting, and progressing (Evan, 1976 ). Organizations can adapt to environmental changes, shifts, or jolts via developmental, transitional, or transformational change (Burke, 2002 ; Jick, 1993 ). They may even choose to preempt changes in their competitive environment by investing in the state-of-the-art technologies, processes, and services to gain competitive advantage. Independent of the type and extent of change, innovation is viewed as a means of coping with and influencing the environment.
The adaptation and progression perspective offers that organizations are motivated to innovate because of (1) pressures from the external environment due to competition, deregulation, isomorphism, resource scarcity, and customer demands, and (2) internal organizational choices for gaining distinctive competencies, reaching a higher level of aspiration, and increasing the extent and quality of their products and services. Innovations are instruments of organizational change for effective performance. The adaptation and progression perspective partially overlaps with a few other theoretical perspectives of innovation in organizations. 4 However, it provides a unique platform to coalesce insights from several strands of organization and innovation management literatures to explain innovation as a process and an outcome in organizations.
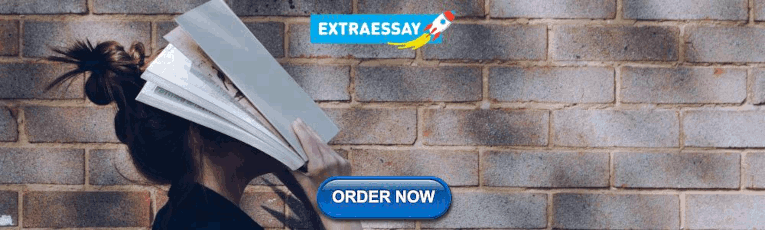
Conceptions of Innovation in Organizations
Godin ( 2008 ) reviewed the history of innovation as a category and identified over ten concepts (discovery, invention, imitation, technology, creativity, change, etc.) that have been used to portray innovation over time. Among them, I have selected three that are closely associated with or taken for organizational innovation: technological innovation, organizational creativity, and organizational change.
I discuss the peripheries of innovation with technology, creativity, and change and offer a pathway to help determine how innovation can be distinguished from the overlapping concepts. I rely on the notion of low- and high-order concepts, which is derived from the classification of systems into levels (Boulding, 1956 ), where the complexity of the system increases from a lower-level system to a higher-level system. A high-order concept embodies a low-order concept similar to a system (organization) including its subsystems (units or parts). The low–high order portrays the hierarchy of goals of systems, the means–end relationship where the actions of a lower-order system affect the behavior of a higher-order system (like the effect of a part on the whole).
Innovation and Technology
Public perception of innovation equates innovation with new technology or technical invention, and understands innovation in organizations as technological innovation. Innovation researchers have exacerbated this misunderstanding by using the term innovation to portray technology-based product and process innovations. 5 While the importance of technology and technological innovation for organization adaptation, competition, and performance is undeniable, taking technological innovation for innovation in general is simply wrong. The concept of innovation is broader than technical invention, and technological innovation is only one type of innovation that organizations generate or adopt. 6
Tushman and Anderson ( 1986 , p. 440) define technology as tools, devices, and knowledge that create new products or services (product technology) and mediate between inputs and outputs (process technology). Technology affects organizational efficiency, facilitates the conversion of inputs into outputs, and reduces inefficiencies in the development, production, and delivery of products and services. The product and process technologies represent physical technologies. New physical technologies may drive the introduction of technology-based product or process innovations. In this vein, technology (technical invention) is a lower-order concept than technological innovation. Similarly, technological innovation can be viewed as a lower-order concept than technological change.
The definition of technological change in business and management is diverse. For instance, technological change reflects significant advances in technological performance within a technological regime (Lawless & Anderson, 1996 ); changes within uniform and differentiated technological systems leading to technological development of industries (Barnett, 1990 ); and technological breakthroughs or discontinuities leading to a dominant design (Wade, 1996 ). Godin’s ( 2015b ) study of the conceptual history of technological change offered two general meanings for it: (1) a narrower meaning—change in the methods and techniques of production; and (2) a broader meaning—change in the society due to technology. I adopt the broader meaning and posit that technological change occurs due to cumulative effects of multiple technologies and technological innovations over time. In this vein, technological change is a concept suitable to the level of product class, industry, and economy, not the organization. At the macro level, technological change can impact development of new industries, economic growth, level of employment, and societal prosperity (Ahlstrom, 2010 ; Edquist, Hommen, & McKelvey, 2001 ; Nelson & Winter, 1982 ).
In summary, I view technology as a lower-order concept than technological innovation, itself one among several types of innovations organizations generate or adopt. Technological change is a higher concept than organizational innovation, is the outcome of a series of innovations in contexts such as industry, product class, region, and economy, and is not discussed in this article.
Innovation and Creativity
Creativity is a concept that is imported to organization management from psychology, where it has been studied primarily at the individual level. Ford ( 1996 ) compared creativity with conformity and proposed a theory of creative individual actions as opposed to habitual individual actions. He defined creativity as the outcome of a particular individual action that is judged novel and valuable (Ford, 1996 ). Amabile ( 1988 ) states that innovation is “creativity plus implementation.” She distinguishes creativity from innovation by relating creativity to the production of novel and useful ideas by individuals and small groups and innovation to the successful implementation of those ideas (Amabile, 1988 ). This view suggests that creativity is a subprocess of innovation process, associates creativity more closely to the generation than the adoption of innovation, and has prevailed among the studies of creativity in organizations (Anderson, Potočnik, & Zhou, 2014 ; Baer, 2012 ; Woodman, Sawyer, & Griffin, 1993 ).
Research on technological innovation has related creativity to technical invention. For instance, in the 25th anniversary publication of R&D Management , Roberts ( 1988 ) characterizes innovation as “invention plus exploitation,” where invention is linked with the creative work of scientists and technologists. OECD’s definition of R&D also links it with technical invention, associating the connotation of creativity to innovation through invention (Godin, 2014 ). The oversized role of R&D on innovation prompted organizational psychologists to study scientists and engineers in R&D functions of organizations (Andrews & Farris, 1967 ; Pelz & Andrews, 1966 ; Pelz, 1956 ). Studies of creativity at work and in organizations gained currency (West & Farr, 1990 ; King, 1990 ; Scott & Bruce, 1994 ), eventually adding the term organizational creativity to innovation vocabulary in business and management. For instance, Woodman et al. ( 1993 ) define organizational creativity as the creation of useful and valuable new ideas, products, services, and processes by individuals working together in an organization, and conceive organizational creativity as a subset of the broader concept of organizational innovation. According to these authors, the difference between organizational creativity and organizational innovation is that the former includes creating new ideas and practices, but the latter can also include the adaptation of preexisting ideas and practices (Woodman et al., 1993 ). This distinction associates newness to creativity, but not to innovation. 7
However, novelty or newness is a commonly accepted component of the definition of innovation across disciplinary fields. Moreover, organizational creativity cannot be distinguished from organizational innovation by stressing the importance of cooperative actions of individuals, because organizational members’ interactions are intrinsic to nearly all organizational activities. By definition organizations are social systems, and their activities depend on human actions. The creative behavior of individuals and small groups is necessary for every organizational action, including innovation.
Overall, in the context of organizations, I posit that creativity is a subset or a subprocess of innovation. Creative ideas, behaviors, and outcomes of individuals and small groups can help solve problems that arise throughout the innovation process. That is, they influence both the generation and adoption of innovations in organizations.
Innovation and Change
Change is a shift or transfer from one state (prior to change) to another state (after the change) (Nadler & Tushman, 1997 ). It is an observation of differences in time in any dimension of organization (Van de Ven & Rogers, 1988 ). Organizational change is the introduction of activities that are different from those currently in use (Burke, 2002 ; Daft & Becker, 1978 ; Wischnevsky, Damanpour, & Mendez, 2011 ). It occurs when organizations evolve from old behaviors and methods of operation to new ones. Thus, by definition change and innovation are distinguished primarily by the newness or novelty of the idea or actions to the focal organization.
Newness is a term relative to the unit of adoption (Rogers, 1995 ), and its notion varies in different streams of innovation research (Gopalakrishnan & Damanpour, 1997 ; Crossan & Apaydin, 2010 ). Traditionally, in innovation diffusion research, newness is perceived in relation to the individual adopter (Rogers, 1995 ); in technology and strategic management, it is newness to a product class or an industry (Roberts, 1988 ; Tornatzky & Fleischer, 1990 ); and in innovation management, it is newness to an organizational unit (plant, business, division, department) or the entire organization (Kimberly & Evanisko, 1981 ; Ettlie, 1988 ). In most empirical studies, what constitutes newness is left as an empirical question, an issue for managers (respondents to surveys) or a panel of experts (academics and experts) to resolve. Thus, newness is determined subjectively through judgment of adopters or professional elites, exacerbating the distinction between innovation and change.
The processes of innovation and change are considerably similar. Organizations adopt both innovation and change, and each can be grouped as major or minor. The difference between the two concepts would need to be established conceptually. I thus view innovation as a subset or a subprocess of organizational change, mirroring the distinction that was made between creativity and innovation.
Innovation in Organizations
Technology, creativity, and change intersect with innovation but are different concepts. In the context of organizations, creativity is a lower-order concept to innovation and innovation is a lower-order concept to organizational change. Innovation is a means to organizational change, although change can occur without innovation. Whereas the intersection of innovation and creativity associates more closely with the generation of innovation, the intersection of innovation and organizational change associates more closely with the adoption of innovation (details below). 8 Technology is an element or component of innovation: Some innovations are technology-based; others are not. Technological innovation is only a type of organizational innovation and should not be mistaken for it.
The locus of innovation and its related concepts in organizations differs. Creativity is a concept associated with individuals and small teams, innovation and change with units and organizations, and technological change with product class and industry. Loose application of these concepts and indiscriminate interpretation of research findings of one to the others causes confusion and could impede an understanding of innovating and innovativeness in organizations.
Innovation in organizations is a systematic (focused, purposeful, and organized) activity (Drucker, 1985 ). The creative ideas and actions of individuals and small groups, as well as the organizational capabilities to manage the innovation process, influence organizational innovations. Moreover, both physical (hard, tangible) and social (soft, intangible) technologies can advance organizational capacity for innovations (Tether & Tajar, 2008 ). In terms of the means-end relationship, therefore:
Technology (physical) ➔ technological innovation ➔ technological change 9 Creativity (individual and group) ➔ organizational innovation ➔ organizational change
The Process of Generation and Adoption of Innovation
The innovation process is usually conceptualized as a sequential process, including recognition of problem/opportunity, development, production, commercialization, adoption, and implementation (Rogers, 1995 ; Angle & Van de Ven, 1989 ). 10 The wide-scope view of the process of innovation in organizations assumes that innovation is developed and implemented in the same organization. But innovations can be developed and commercialized by one organization, and adopted and used by others (Damanpour & Wischnevsky, 2006 ). In this vein, Tornatzky and Fleischer ( 1990 ) grouped the innovation process into two processes of “developing” and “using.” Klein and Sorra ( 1996 ) also distinguished between “source-based” and “user-based” process, where innovation in the former is a new product or practice an organization (or a unit of it) produces, and in the latter it is the first-time adoption of a product or practice by an organization (or a unit of it). 11
Most studies of innovation in organizations do not discern between generation and adoption, and refer to both as innovation process. However, generation and adoption are distinct processes, with different phases and characteristics (Damanpour & Gopalakrishnan, 1998 ). 12 Generation is a process that results in the introduction of a new product, service, process, or practice to the market. It covers all organizational activities related to creating new ideas, getting them to work, and supplying them to the market for use by individuals and/or organizations (Roberts, 1988 ). The generation process includes recognition of opportunity, research, design, piloting and testing, commercial development, production, marketing, and distribution (Tornatzky & Fleischer, 1990 ; Roberts, 1988 ). Adoption is the process of choosing and using a product, service, process, or practice that is new to the adopting organization. Adoption basically means that the innovation is developed elsewhere, not in the adopting organization (Angle & Van de Ven, 1989 ). The adoption process includes problem perception, searching for solutions, evaluating and selecting one solution, initial implementation, sustained implementation, and eventually termination (Angle & Van de Ven, 1989 ; Hage & Aiken, 1970 ; Tornatzky & Fleischer, 1990 ). While in generation new and existing ideas are combined in a novel way to produce a configuration that was previously unknown, in adoption ideas new to the adopting organization are identified, acquired, and adapted to fulfill recognized needs or solve existing problems (Damanpour & Wischnevsky, 2006 ). The adoption is complete when organizational members or clients use the innovation regularly.
Research on the generation process has typically focused on the generation of technological innovations. For instance, in Roberts’ ( 1988 ) characterization of innovation (invention plus exploitation), invention is marked by a new discovery (usually at the laboratory) and exploitation consists of the commercial development and conversion of that discovery into a useful application. Prominent in business policy, technology management, and economics, this view assumes that innovations are driven by technical invention (Godin, 2008 ). However, as Brozen ( 1951 ) points out, even the generation of technological innovation (change in the productive methods of technological possibilities) need not be the result of technical invention (change in technological possibilities). Moreover, organizational innovation includes nontechnological innovation, whose generation has not been scrutinized in organization studies. A notable exception is Birkinshaw, Hamel, and Mol’s ( 2008 ) four-stage process framework (motivation, invention, implementation, theorization, and labeling) for the generation of management innovations.
The generation of innovation requires more in-depth specialized knowledge than adoption. Thus, organizations can obtain expertise in generating a certain type of innovation. However, they can adopt a greater variety of innovation types, making innovation adoption a more commonly researched subject in innovation management. The adoption process has been grouped into two general stages of initiation and implementation, which are separated by the adoption decision (Rogers, 1995 ; Zaltman et al., 1973 ). 13 Initiation consists of activities that pertain to recognizing a need, searching for solutions, becoming aware of existing innovations, identifying suitable innovations, and proposing a few for adoption (Duncan, 1976 ; Rogers, 1995 ). In this phase organizations learn of the innovation’s existence, evaluate its suitability, solicit advice from internal and external constituents, and make the adoption decision (Birkinshaw et al., 2008 ; Meyer & Goes, 1988 ). Implementation consists of activities that pertain to modifying the innovation, preparing the organization for its use, trial use, acceptance of the innovation by the users, and continued use until the innovation’s use is routinized (Duncan, 1976 ; Rogers, 1995 ).
In summary, organizations can both generate and adopt innovations. In generation, the newness of innovation relates to an organizational population; in adoption, it relates to an organization. The process of generation and adoption are not alike. The generation process can be characterized more like a creative process, the adoption process more like a problem-solving process. The generation process is relatively disorderly, more like a random process of chance or chaotic events; the adoption process is relatively orderly, more like a periodic and sequential progression of phases (Cheng & Van de Ven, 1996 ; Damanpour & Wischnevsky, 2006 ). The generation process is usually slower and takes longer to complete than the adoption process. Since the stages and the characteristics of generation and adoption differ, the distinction between them is necessary to understand how organizations can innovate and what factors motivate innovating.
Typologies of Organizational Innovation
The primary approach for reducing the complexity of innovation to study its antecedents and consequences has been to develop typologies. For instance, Schumpeter ( 1934 ) grouped innovation into five types, and Zaltman et al. ( 1973 ) listed approximately 20 types. Since then, more innovation types have been introduced, including architectural, business model, exploratory, exploitative, open, green, and so on. Among the typologies of innovation, three have been most widely studied: product–process, technical (technological)-managerial (administrative), and radical–incremental (Damanpour & Aravind, 2012b ). Meeus and Edquist ( 2006 ) offered a taxonomy by juxtaposing the first two typologies. These authors distinguished between two types of product innovations—product (innovation in goods) and service (innovation in services)—and two types of process innovations—technological process (technical) and organizational (managerial). 14 Meeus and Edquist’s taxonomy does not account for the openness of innovation. Tether and Tajar’s ( 2008 ) model of firm-based innovation does. Tether and Tajar’s model is based on three dimensions of change—changes to what the firm produces (product) versus changes to how the firm operates (process), changes to physical technologies (technical) versus changes to social technologies (administrative), and the locus of change, intrafirm (organic) versus interfirm (open).
I organize the discussion of innovation types into four pairs: product–process, technical–managerial, radical–incremental, and organic–open. The resulting eight types of innovation provide a general framework for studying the majority of innovations organizations generate and adopt.
Product and Process Innovation
Product and process innovations are the most commonly studied innovation types. Academic research on this typology has generally focused on industrial innovations, specifically on R&D-based innovations (Damanpour, 2010 ; Tether & Tajar, 2008 ). This orientation has resulted in the understanding of product and process innovations as two types of technological innovations.
Product innovation is defined as the introduction of a new product or service to meet an external user need, and process innovation as the introduction of new elements in a firm’s production or service operation in order to produce a product or render a service (Damanpour, 2010 ; Schilling, 2013 ; Utterback, 1994 ). Product innovations have an external focus and are primarily market-driven; process innovations have an internal focus and are mainly techniques of producing and marketing goods or services. The drivers of product innovations are customer need and demand, and firms’ aspiration to compete and grow. The drivers of process innovations are reduction in delivery time, increase in operational flexibility, and lowering of production costs. Hence, while product innovations are embodied in the outputs of an organization and may result in product differentiation and market expansion, process innovations are oriented toward the efficiency or effectiveness of production and may decrease production costs or increase product quality (Damanpour, 2010 ; Schilling, 2013 ; Utterback, 1994 ).
Henderson and Clark ( 1990 ) expanded the product–process typology based on two dimensions of “core concept” and “linkage between core concepts and components,” and introduced architectural innovation in contrast to component innovation. Component innovation entails changes to one or more components of a product system without significantly changing the overall design. Architectural innovation entails changing the overall design of the system or the way components interact (Henderson & Clark, 1990 ; Schilling, 2013 ). Architectural innovations may require changes in the underlying components. In introducing architectural innovations, Henderson and Clark ( 1990 , p. 12) portray their conceptual model as “a framework for defining innovation.” However, architectural innovation is merely a subset or a subtype of technological product innovation and has rarely been applied to other types of innovation organizations generate or adopt.
Most studies of innovation in organizations do not distinguish service innovations from product innovations. Generally, services offered by organizations in the service sector are conceptualized to be similar to products introduced by organizations in the manufacturing sector (Damanpour & Aravind, 2012b ; Miles, 2005 ; Meeus & Edquist, 2006 ). In this vein, like product innovations, the drivers of service innovations are clients’ demand for new services and executives’ desire to create new services for existing markets or to find new market niches for existing services (Miles, 2005 ). However, service innovations are not necessarily technology-based (Tether & Tajar, 2008 ), and firms in both goods and service industries can introduce them. Accordingly, service innovation is defined as the introduction of a new service to increase the effectiveness and quality of the organization’s output, whether a product or a service, to the customers or clients (Damanpour & Aravind, 2012b ).
Technical and Managerial Innovation
The technical–managerial typology was introduced in organization management in contrast to the product–process typology that dominated the studies of innovation in economics and technology management. The distinction between technical (technological) and managerial (administrative) innovations relates to a more general distinction between technology and social structure (Evan, 1966 ). Technical and managerial innovations are respectively associated with the organizations’ technical and social systems (Damanpour & Evan, 1984 ) and technical and administrative cores (Daft, 1978 ). Evan ( 1966 , p. 51) defined technical and administrative innovations as ideas for new product, process, and service, and ideas for new personnel policy, reward system, resource allocation, and structuring, respectively. Technical innovations are directly related to the primary work activity of the organization and produce changes mainly in its operating systems. Administrative innovations are indirectly related to the organization’s primary work activity and affect mainly its management systems (Daft, 1978 ; Damanpour & Evan, 1984 ; Kimberly, 1981 ). Recently, the term management innovation has replaced the term administrative innovation. Management innovations are departures from management principles, processes, and practices that alter the way the work of management is performed, change how managers do what they do, and constitute the rules and routines by which work gets done inside organizations (Birkinshaw et al., 2008 ; Hamel, 2006 ). They reflect approaches to devising strategy, structure, and processes that are new to the organization (Kimberly, 1981 ; Vaccaro, Jansen, Van Den Bosch, & Volberda, 2012 ; Walker et al., 2011 ). 15
While this new term has renewed interest in research on managerial innovation, the state of knowledge on this innovation type is in its infancy. The domain of managerial innovation is wide; the concept is complex, ambiguous, and difficult to measure; and rival theoretical arguments on motivation for its generation, adoption, and performance consequences exist (Birkinshaw et al., 2008 ; Damanpour & Aravind, 2012a ; Sturdy, 2004 ). A variety of terms have been used to describe managerial innovations. In a literature review, Černe, Kaše, & Škerlavaj ( 2016 ) identified ten nontechnological innovations, the majority of which represent managerial innovation. The definitions and characteristics of the terms for managerial innovations show that they overlap markedly (Černe et al., 2016 , pp. 71, 79). Černe et al. ( 2016 ) also conducted a cocitation analysis of nontechnological innovations and found considerable similarity in their intellectual structure. Multiplicity of terminology, combined with lack of established typologies and measurements of managerial innovations, has constrained the advancement of this important type of innovation (Armbruster, Bikfalvi, Kinkel, & Lay, 2008 ; Damanpour, 2014 ). For technological innovations, for instance, product and process innovations have been commonly accepted as subtypes, indicators for their measurement are established (patents, R&D expenditure, scientific publications, etc.), and historical data sets for their measurement exist. For the development of data sets of managerial innovations at par with those for technological product and process innovations, commonly accepted terminology, typology, and measurement indicators should be selected and followed.
Radical and Incremental Innovation
The radical–incremental typology is primarily applied to technological product and process innovations at both industry/product class and organizational level. At the level of industry, technology can be disruptive or sustaining depending on whether it is based on entirely new knowledge and obsoletes the existing products and processes or it improves the performance of products and processes along the existing dimensions of performance (Christensen, 1997 ). Innovation can be competence-destroying , when it departs from the organization’s existing competencies, or competence-enhancing , when it builds on and improves existing competencies (Tushman & Anderson, 1986 ). At the organizational level, radical and incremental innovations are distinguished by the extent to which they change internal activities or outputs of the organization. As such, radical innovations are those that cause fundamental changes in organizational activities and result in a clear departure from existing products, processes, and practices, and incremental innovations are those that result in minor changes in the existing activities, products, processes, and practices (Damanpour, 1991 ; Dewar & Dutton, 1986 ; Ettlie, Bridges, & O’Keefe, 1984 ).
More recently, the radical–incremental typology has been augmented by the exploratory–exploitative typology. The new typology is based on the exploration-exploitation in organizational learning (March, 1991 ). Exploration refers to the application of learning to produce new products and technologies, and exploitation refers to the application of learning to refine the organization’s existing products and improve its processes (March, 1991 ). The essence of exploration is experimentation with new ideas; it is associated with divergent thinking and flexibility. The essence of exploitation is the refinement of existing ideas; it is associated with convergent thinking and focus (March, 1991 ). Exploratory and exploitative innovations reflect the results of exploration and exploitation for an organization’s innovative actions (Bierly, Damanpour, & Santoro, 2009 ; Jansen, Van Den Bosch, & Volberda, 2006 ).
Whereas the radical–incremental and the exploration–exploitation typologies have been applied mainly to technological innovations, they are also applicable to other innovation types. Both typologies are based on innovation radicalness , an attribute of innovation defined as (1) the extent to which the innovation departs from existing knowledge, or (2) the degree of change the innovation creates in organizational conduct or outcome (Damanpour & Wischnevsky, 2006 ). At the organization level, change can be a result of the introduction of technological or nontechnological innovations and the nature of knowledge can be technological or nontechnological. The dimension of radicalness can also assist in separating generation (more radical) from adoption (more incremental), and innovation (more radical) from change (more incremental). In particular, the application of radicalness to nontechnological innovation will be helpful in screening its subtypes and identifying a few at par with product and process innovations (subtypes of technological innovation) to further theory and measurement of nontechnological innovations.
Organic and Open Innovation
Organic innovation refers to in-house development of a new product, process, or service, when the focal organization invents, develops, and commercializes the innovation. Chesbrough ( 2003 ) called a company’s full control of the innovation process “closed innovation,” and introduced open innovation , an approach where the tight control is relaxed and the company brings in partners through various means of interorganizational cooperation (strategic alliances, joint ventures, consortia) in one or more aspect of the generation of innovation (Chesbrough, 2003 ). The flexibility in developing and commercializing internal and external ideas in cooperation with other firms expedites the generation of new products and processes for the current market and facilitates entering new markets (Chesbrough, 2003 ). Open innovation is an important concept that has captured a timely management practice that has been induced by the demise of large in-house R&D organizations (Economist, 2007 ), globalization of business operations and services, and the advance in information technology.
The concept of open innovation has been embraced in strategy and technology management. While early writings focused mainly on the ideation aspect of innovation generation (e.g., emphasis on crowdsourcing as a key means of open innovation), research on open innovation has been expanded to include the development, utilization, and retention of knowledge inside and outside of an organization’s boundary throughout the innovation generation process (Chesbrough & Appleyard, 2007 ; Lichtenthaler, 2011 ). Theoretically, open innovation has followed the concept of absorptive capacity, which highlighted the importance of external sources of knowledge for the generation of technological innovations (Cohen & Levinthal, 1990 ). Strategy scholars have probed the impact of the breadth and depth of external sources for innovation and its performance outcomes (Laursen & Salter, 2006 ; Leiponen & Helfat, 2010 ). This research has focused mainly on the generation of technological innovations along the perspective of organization competition and performance.
To enrich open innovation’s theoretical domain and integrate it with the extant literature on organizational innovation, the concept should be augmented to enable its application along the perspective of organization adaptation and progression. That is, the application of the concept should include the generation of nontechnological innovation, as well as the adoption of innovations. For instance, Birkinshaw et al. ( 2008 ) developed a conceptual model for the generation of management innovations and discussed the dual role of internal and external sources of information. Mol and Birkinshaw ( 2014 ) examined the forms of external involvement on the generation of management innovation. Damanpour, Sanchez, and Chiu ( 2017 ) discussed the dual role of internal and external knowledge sources on the adoption of management innovations. These studies suggest that there is room to extend and expand open innovation to generation and adoption of all types of innovations, in goods and services, and in business and public organizations.
Whereas comparative studies of antecedents and consequences of product and process, technical and managerial, and radical and incremental innovations have been conducted, research on motivators and outcomes of organic versus open innovations are scarce. Large-sample comparative studies of this pair of innovation type are needed to develop a stronger theoretical foundation for the role of innovation openness on organizational conduct and outcome. From an adaptation and progression perspective, organizations as social systems are inherently open systems. The openness property of an organization is crucial to innovation activities because innovation in essence cannot occur in isolation inside a firm’s boundary. Some form of interdependencies with suppliers, customers, research institutions, and even competitors are needed because individual companies are unable to keep up with the pace of the development of technical and managerial knowledge, even in modestly complex and dynamic environments. In general, the more complex the physical or social technologies that constitute the innovation and the more dynamic the external environment of the organization, the more porous the organization–environment boundary and the greater the need for sourcing knowledge through different means of organization–environment relations. 16 Future research should ground innovation openness in the behavioral theories of organization in order to clarify the dynamic of internal and external sources, and develop mechanisms for facilitating cooperation, preventing conflicts, and managing the diversity of knowledge sources (Damanpour et al., 2017 ).
Antecedents of Organizational Innovation
Business and public managers are keen to understand conditions under which their organization can successfully innovate. Consequently, studies of the antecedents of organizational innovation constitute the largest body of this research. These studies have focused more on innovativeness than innovating, and on innovation adoption than generation. The majority have also examined organizational innovation as a single construct, although a considerable minority have distinguished between factors that predict pairs of innovation types. While innovation is recognized as a multilevel and multidimensional construct (Baldridge & Burnham, 1975 ; Damanpour & Schneider, 2006 ; Kimberly & Evanisko, 1981 ; Sears & Baba, 2011 ), most studies have examined a set of factors associated with one level (individual, group, organization) and one dimension (industry, internal structure, personal attributes).
I focus on three dimensions that embody the majority of organizational innovation antecedents: environmental (external, contextual), organizational (structure, culture), and managerial (leadership, human capital). 17 Myriad number of variables have been associated with each dimension, and qualitative and quantitative reviews to identify salient antecedents have been conducted. For parsimony, I rely mainly on the review studies to discuss factors within each dimension.
Environmental Antecedents
The review studies of environmental antecedents of innovation have identified different sets of factors. For instance, in a systematic review of publications on organizational innovation during 1981–2008 , Crossan and Apaydin ( 2010 , p. 1182) identified organization, technology, market, and innovation types as environmental antecedents. In another systematic review of publications during 1983–2003 , Damanpour and Aravind ( 2006 , p. 58) identified competition, concertation, technological opportunity, appropriability conditions, and growth of demand as contextual antecedents of innovation. The difference between the two reviews is threefold. First, Crossan and Apaydin’s review is based on articles published mainly in management journals, whereas Damanpour and Aravind’s review is based on publications mainly in economic journals. Second, Damanpour and Aravind’s review includes only technological innovations; Crossan and Apaydin’s review includes both technological and nontechnological innovations. Third, the focus of studies in Damanpour and Aravind’s review is mainly the generation of innovations; in Crossan and Apaydin’s review it is the adoption of innovations. As such, the original publications in the two reviews come from different disciplinary fields, resulting in dissimilar sets of variables as salient environmental antecedents of innovation.
Context dependency has also been shown in two recent systematic reviews that focused on innovation adoption. Černe et al. ( 2016 ) reviewed nontechnological innovations within 1975–2011 in the business context, and identified market orientation, dynamism, and competitiveness as typical antecedents. De Vries, Bekkers, and Tummers ( 2016 ) reviewed innovation in the public context during 1990–2014 and reported environmental pressures (institutional, political, public, media), participation in networks, and extent of regulation as usual environmental antecedents. In general, while typical environmental variables in public organizations are urbanization, deprivation, ethnicity, political orientation, and community affluence (Boyne, 2002 ; Damanpour & Schneider, 2009 ; Walker, 2008 ), in business organizations they are market competition, industry structure, governmental regulation, technological intensity, supplier power, and customer demand (Cohen & Levin, 1989 ; Roberts & Amit, 2003 ; Schilling, 2013 ).
The differences between environmental antecedents in these four reviews illustrate the crucial role of academic discipline, generation versus adoption, and innovation type in predicting organizational innovation. One way to partially bridge such differences toward coalescing environmental factors that affect organizational innovation is to rely on more general constructs (e.g., environmental uncertainty) and examine the influence of its components (e.g., complexity, dynamism, and diversity) on organizational innovation (Daft, 2001 ; Damanpour & Gopalakrishnan, 1998 ; Mintzberg, 1979 ; Tidd, 2001 ). Reliance on general constructs, however, could cloud the specificity of the findings.
Organizational Antecedents
Although environmental conditions and events motivate and influence organizations to engage in innovation, internal organizational conditions reflect their intent and capacity to do so. Organizations are managed entities, setting goals and priorities, and designing structure and processes to conduct their activities. Innovation is also a managed activity. It is a choice that requires financial and human resources, supportive climate and culture, and enabling structure, processes, and systems. Hence, organizational determinants of innovation have been examined more than environmental and managerial antecedents, especially in organization management.
Damanpour and Aravind ( 2012b ) conducted a systematic review of the antecedents of organizational innovation in 1990–2009 , compared their results with those reported in an earlier meta-analysis of publications in 1971–1988 , and found the findings from the two reviews are generally consistent. They identified seven salient antecedents supported in the empirical studies in both periods: professionalism, specialization, technical knowledge resources, functional differentiation, management attitude toward change, and internal and external communication (p. 502). Crossan and Apaydin’s ( 2010 ) review added organizational culture, learning, and strategy (mission, goals, resource allocation) to these structural variables (p. 1182). However, a systematic review of publications in strategic management of innovation ( 1992–2010 ) offered a different set of antecedents. Keupp, Palmie, & Gassmann ( 2012 ) grouped organizational antecedents into intended/emergent initiatives (R&D investment, technology sourcing, competitive strategy), internal organization (size, culture, structural integration), managerial/ownership issues (human resources, ownership, process management), and resources (prior performance, knowledge and capabilities, slack) (p. 374). With the exception of internal organization, the set of variables from Keupp et al.’s review corresponds closely with organizational antecedents of the generation of technological product and process innovations in Damanpour and Aravind’s ( 2006 , p. 58) review (firm size, profit, capital intensity, diversification, ownership, and technical knowledge resources). Thus, similar to environmental antecedents, organizational predictors of innovation are disciplinary-based, and are contingent on generation and adoption and innovation types. Organizational size is an exception, however.
Firm size is the most widely researched antecedent of innovation across disciplinary fields. The size–innovation association is governed by two sets of compelling arguments. On the one hand, small organizations are more innovative because they can make quicker decisions to go ahead with new and ambitious projects, and have less bureaucratic and more flexible structure, greater ability to adapt and improve, and less difficulty in accepting and implementing change (Damanpour, 2010 ; Nord & Tucker, 1987 ; Stevenson & Jarillo, 1990 ). On the other hand, large organizations are more innovative because they can risk failure and absorb the costs, have diverse professional skills allowing cross-fertilization of ideas, higher technical potential and knowledge, and better scale economies for raising capital and marketing new products and processes (Damanpour, 2010 ; Hitt, Hoskisson, & Ireland, 1990 ; Nord & Tucker, 1987 ). While empirical results from single studies remain inconsistent (Cohen & Levin, 1989 ), the findings from systematic reviews report a positive relationship between size and innovation. For instance, Damanpour ( 2010 ) found that size positively affects both product and process innovations. Similarly, in a meta-analytic review that included all types of innovation, Camisón, Lapiedra, Segarra, and Boronat ( 2004 , p. 331) found a positive association between organizational size and innovation (r=.15, p<.05). 18
Chandy and Tellis’s ( 2000 ) study on the influence of firm size on the introduction of 64 radical product innovations in consumer durables and office products from 1851 to 1998 provides historical evidence regarding the two competing arguments on the size–innovation relationship. These authors found that while 73% of radical product innovations were generated by nonincumbents before World War II, the incumbents significantly outnumbered nonincumbents (74% to 26%) for the innovations generated after the war (Chandy & Tellis, 2000 , p. 8). The results from this longitudinal analysis suggest that by the middle of the 20th century the share of innovations introduced by larger firms surpassed those introduced by smaller firms. More recent cumulative evidence on the size-innovation relationship that suggest otherwise has not been reported.
Managerial Characteristics
Top managers or strategic leaders influence innovation because they modulate the process of scanning the environment for threats and opportunities, formulate policy to respond to environmental change, control resources, and shape capabilities to enable innovation activity (Bantel & Jackson, 1989 ; Damanpour & Schneider, 2006 ; Elenkov, Judge, & Wright, 2005 ). They are also responsible for instituting values supportive of innovation, empowering middle and line managers, motivating members and improving their morale, and encouraging innovation actions and establishing rewards for them (Damanpour & Schneider, 2006 ; Hoffman & Hegarty, 1993 ; West & Anderson, 1996 ).
Prior research has explored three sets of managerial characteristics on innovations in organizations: demographic (age, gender, education, experience), personality (agreeableness, authoritarianism, openness to experience), and behavioral (inspirational motivation, championing innovation, contingent rewards). Studies in business and public management have generally identified transformational leadership, change-oriented behavior, favorable attitude and disposition toward change, and skills and ability to create a climate supportive of innovation as key managerial characteristics (Crossan & Apaydin, 2010 ; de Vries et al., 2016 ; Ekvall & Arvonen, 1991 ). Managers with these attributes build feelings of confidence among organization members, promote the generation of new ideas, and facilitate replacing existing practices with new ones (Damanpour & Schneider, 2006 ; Madjar, Oldham, & Pratt, 2002 ; Mumford, 2000 ). They also promote the implementation of innovation by allocating resources, laying the social and technical groundwork, building coalitions among different constituencies, and assisting coordination and conflict resolution among units and members (Damanpour & Schneider, 2006 ; Dewar & Dutton, 1986 ; Mumford, 2000 ).
Quantitative reviews of the influence of managerial characteristics on organizational innovation have not been conducted. However, the articles in a two-part special issue of Leadership Quarterly on Leading for Innovation point out that leadership makes a major difference in the generation of ideas for new products and practices, and highlights how leaders could manage creative people to conduct creative work in creative ventures (Mumford, Scott, Gaddis, & Strange, 2002 ; Mumford & Licuanan, 2004 ). However, effective leadership of creative efforts of individuals and small teams is necessary but not sufficient for organizational innovation. In organizational settings, the selection of good ideas could be more crucial than the mere generation of new ideas (Grant, 2016 ). Hence, in addition to the generation of ideas, research on leadership for innovation should probe the process of selecting an idea from the portfolio of ideas, account for the effect of environmental and organizational factors on idea creation and selection, and explore whether the role of organizational leader differs in the process of generation of innovations of different types.
To make sense of multiple dimensions and numerous factors that could affect innovation in organizations, I suggest a sequence of decisions in selecting the dimensions and deciphering the antecedents. The first and perhaps most important decision is to identify organizational type, whether goods or services, business or public, low-tech or high-tech, and so on. Meta-analytical studies have reported significant differences between the antecedent–innovation relationships in different types of organizations (Camisón et al., 2004 ; Damanpour, 1991 ). Second, factors that influence the generation of innovation may not be compatible with those that influence the adoption of innovation. As such, a distinction between innovation-generating and innovation-adopting organizations is necessary. Third, while a set of antecedents may predict the process of innovation (innovating), a different set may predict organizational ability to innovate continually (innovativeness). Fourth is the distinction between innovation types, especially the technological–nontechnological and the radical–incremental, for the identification of salient antecedents of each type. Fifth, the relative importance of the environmental, organizational, and managerial characteristics in different types of organizations may differ. Current studies have not tested such differences, future studies should. Finally, the complexity of innovation constrains offering a common theory of organizational innovation. A possible approach researchers may pursue could be to focus on the antecedents of pairs of innovation types along firms’ value chains (Porter, 1985 ; Schilling, 2013 ). For example, antecedents of: (1) product–process pair for inbound versus outbound logistics; (2) technical–managerial pair in firm infrastructure versus human resource management; and (3) radical–incremental pair in technology development versus operations.
Organizational Innovation and Firm Performance
The widespread popularity of innovation stems from the assumption that its introduction results in positive (intended, expected, desired) outcomes. Rogers ( 1995 ) referred to this view as “pro-innovation bias.” While innovation is risky and its success is not certain, scholars and practitioners alike postulate that innovation strategies and activities boost firm performance. Empirical studies of the generation and adoption of innovations in organizations have usually supported this expectation (Bowen, Rostami, & Steel, 2010 ; Calantone, Harmancioglu, & Droge, 2010 ; Rosenbusch, Brinckmann, & Bausch, 2011 ; Walker, Chen, & Aravind, 2015 ). Studies of innovation failure are scarce.
The rationale for the favorable influence of innovation on firm conduct and outcome is offered by the first-mover advantage and performance gap theory (Damanpour, Walker, & Avellaneda, 2009 ; Keupp et al., 2012 ; Lam, 2005 ). The first-mover advantage imbedded in strategic management stresses the importance of generating new products and services for firm competitiveness and growth (Lieberman & Montgomery, 1988 ). Firms adopt first-mover strategy to become dominant in a product class or market and gain superior performance over time (Cohen & Levinthal, 1990 ; Roberts & Amit, 2003 ). First-mover strategy prompts organizations to engage in innovation activity, enables them to be aware of the latest developments, absorbs new and related knowledge, and increases the likelihood of benefiting from innovation activities in the long term (Bierly et al., 2009 ; Lieberman & Montgomery, 1988 ; Roberts & Amit, 2003 ).
Performance gap is defined as the perceived difference between an organization’s potential and actual accomplishments (Damanpour et al., 2009 ; Zaltman et al., 1973 ). Performance gap creates a need for organizational change, which in turn provides motivation to introduce innovation to produce change and reduce the perceived gap. The domain of performance gap theory is broader than that of first-mover advantage. While first-mover advantage applies to business organizations, performance gap applies to all types of organizations, whether business or public, service or manufacturing, low- or high-performance (Damanpour et al., 2009 ). The first-mover theory suits the generation of new products and services; performance gap theory is applicable to the adoption of any type of innovation, although it can also induce the generation of innovation.
Technological and Nontechnological Innovations and Performance
The conceptual confusion surrounding innovation and technology and misrepresentation of innovation as solely technology-based new products and processes has resulted in the perception that firm performance is affected by technological, but not necessarily by nontechnological, innovations. Studies of technological innovations in organizations are often espoused by the theories of economies of organization, which in management is referred to as rational (technical-efficiency) approach. The studies of nontechnological innovations, however, are governed by multiple theoretical approaches. Sturdy ( 2004 ) identified five such approaches (political, cultural, institutional, dynamic, and dramaturgical or rhetorical) for managerial innovations and compared them with the rational approach. He argued that the alternative approaches marginalize managerial rationality, might lead to empirical neglect, and portray rational management as bounded and emotional (Sturdy, 2004 ). In organization studies, the main alternative to the rational approach has been the institutional approach, often under the label of management fad and fashion (Damanpour, 2014 ).
To induce innovation, the rational perspective emphasizes on the influence of market dynamism and competition; the institutional perspective emphasizes pressures from regulators, parent organizations, and network members (Ashworth, Boyne, & Delbridge, 2009 ; Sturdy, 2004 ). Institutional pressures impel organizations toward conformity with rules and norms of their fields and heighten the importance of pursuit of legitimacy in organizational actions (Ansari, Fiss, & Zajac, 2010 ; Ang & Cummings, 1997 ). These pressures would more strongly affect nontechnological than technological innovations. For instance, organizational leaders are uncertain about technical efficiency of managerial innovations and rely on their currency in the population (Abrahamson, 1991 ; Burns & Wholey, 1993 ). Hence, the adoption of managerial innovations would result in social approval and reputation (social gain) rather than performance outcome (economic gain) (Abrahamson, 1991 ; Greve, 1995 ). Staw and Epstein ( 2000 ) provided empirical evidence for this view. They studied three administrative practices (quality, empowerment, and teams) and the implementation of TQM, and found that organizations that adopted them did not show higher economic performance (returns on asset, equity, and sales) but were more admired, perceived to be more innovative, and rated higher in management quality in their population (Staw & Epstein, 2000 , p. 523).
However, a recent quantitative review of the relationship between managerial innovation and firm performance provided evidence for a positive effect. Walker et al. ( 2015 ) integrated the empirical findings from 44 articles published in peer-reviewed journals via two different quantitative procedures, examined moderating effects of several factors, and found that the adoption of managerial innovations positively affects organizational performance. Further, using data from a subsample of 22 articles, Walker et al. ( 2015 ) integrated the empirical findings for the technological innovation–performance association and found a positive relationship also. 19 A comparison of a matched sample of associations of technological and managerial innovations with organizational performance showed that the two types of innovations affect performance similarly (Walker et al., 2015 ).
Overall, while managerial (nontechnological process) innovations are considered to be economically and socially important (Arrow, 1962 ; Edquist et al., 2001 ; Sanidas, 2005 ), and their introduction is deemed necessary to rejuvenate organizational strategy, structure, and systems (Birkinshaw et al., 2008 ; Stata, 1989 ; Volberda, Van Den Bosch, & Heij, 2013 ), research on this type of innovation lags behind technological innovation, and its influence on performance is deemed to be less predictable. Managerial innovations are operationally complex (difficult to implement and use), pervasive (changing administrative structure, authority, and power), and adaptable (modified during the adoption process) (Ansari et al., 2010 ; Damanpour, 2014 ). 20 Tidd ( 2001 ) argued that establishing a strong empirical relationship between innovation and performance is difficult because of technological and market contingencies, and methodological shortcomings (measurement of both constructs). For technological innovations surrogate measures such as patents and R&D expenditure are available and accepted; for nontechnological innovations easily quantifiable surrogate measures have not yet been developed (Armbruster et al., 2008 ; Damanpour & Aravind, 2012a ; Evangelista & Vezzani, 2010 ). Convincing empirical evidence on the stronger effect of one type above the other type has not yet emerged. Theoretical arguments, however, point to their combinative rather than stand-alone effects on performance outcomes.
Combinative Effects of Innovation Types
On the one hand, according to the first-mover advantage theory and based on the logic of organization competition and performance, superior performance occurs when a (technological) product or process new to a product class is introduced in the market and is received well by customers. Positive performance outcomes induce organizations to invest in excelling at the type of innovation for which they have been successful. Prior experience with a certain body of knowledge encourages further absorption of the same type of knowledge because organizations can more easily integrate, explore, and exploit the absorbed knowledge to create new opportunities that would further result in performance advantages (Bierly et al., 2009 ; Cohen & Levinthal, 1990 ; Roberts & Amit, 2003 ). Most studies of performance consequences of innovation follow this logic and focus on one type of innovation, often product or technological.
On the other hand, according to the performance gap theory and based on the logic of organizational adaptation and progression, sustained performance requires the introduction of different types of innovations over time to help adapt organizations to the external and internal changes (Damanpour et al., 2009 ; Roberts & Amit, 2003 ). Innovation types are interdependent, the introduction of one type could prompt the introduction of another type, and an understanding of contributions of each type requires an understanding of its relations with the other types. Performance consequences of innovation could best be captured by longitudinal studies that include the introduction of compatible sets of innovation types (product and process, technological and nontechnological, radical and incremental) across organizational parts or subsystems (Damanpour, 2014 ).
Georgantzas and Shapiro ( 1993 ) defined synchronous innovation as the adoption of compatible technological and managerial innovations, examined the influence of four descriptive models of synchronous innovation (independent, moderating, mediating, and interactive) on organizational performance, and found that the independent effect of each innovation type on performance is negligible without synchronous innovation (p. 161). Roberts and Amit ( 2003 ) extended the notion of synchronous innovation to compositions of innovation types. These authors investigated the influence of three compositions (focus, commitment, and divergence) of three types of innovations (product, process, and distribution) on performance in retail banking organizations longitudinally and found that long-term performance depends on the history of innovation activity in organizations rather than occasional success of stand-alone innovations (Roberts & Amit, 2003 ). Damanpour et al. ( 2009 ) also investigated three compositions (focus, consistency, and divergence) of three different types of innovation (technological, administrative, and service) in public service organizations and confirmed Roberts and Amit’s conclusion. Longitudinal, empirical evidence from these studies challenges the notion that firm performance is enhanced by focus on excelling at a specific type of innovation, whether product, service, process, technical, or managerial. Instead, in line with adaptation and progression view they suggest that sustained performance requires harmonious modifications of various organizational subsystems via the introduction of complementary innovation types (Ballot, Fakhfakh, Galia, & Slater, 2015 ; Battisti & Iona, 2009 ; Hervas-Oliver & Sempere-Ripoll, 2014 ; Naranjo-Gil, 2009 ).
Theoretical support for complementarity of innovation types and their combinative performance effects can be found in organization and strategic management. The perspective of organizations as socio-technical systems is an early example. This perspective theorized that the relationship between organizational subsystems is not strictly a one-to-one relationship; rather, it is a correlative relationship representing a coupling of dissimilarities, where changes in one subsystem necessitate corresponding changes in the other subsystems (Emery & Trist, 1960 ). The social and technical systems interact continuously and are inclined toward a dynamic equilibrium in relation to the external environment (Boonstra & Vink, 1996 ; Trist & Murray, 1993 ). Any change in one system sets certain constraints and requirements, and necessitates a corresponding change in the other system. Considering technical and administrative innovations as means of changing the technical and social systems, organizational performance requires a balanced introduction of both types (Damanpour & Evan, 1984 ).
Theories and perspective in strategic management also allude to the complementary role of innovation types. For example, the resource-based and knowledge-based views underscore the roles of external and internal sources of knowledge and the firm’s capability to integrate them to gain distinctive competencies (Barney, 2001 ; Grant, 1996 ). Theories of operational and combinative capabilities also imply that innovating across organizational functions and systems could ensure renewal of competencies to build, reconfigure, and integrate internal and external experiences to cope with the dynamics of environmental change (Eisenhardt & Martin, 2000 ; Helfat & Winter, 2011 ; Van den Bosch, Volberda, & de Boer, 1999 ). The application of these views to innovation activity at the firm level underlines the synergistic use of organizations’ technological, operational, and managerial knowledge resources, motivating the synchronous introduction of innovation types across organizational parts to gain sustained performance outcomes (Damanpour, 2014 ).
The complexity of both innovation and performance constructs combined with myriad indicators for their measurement has prevented rigorous evidence on conditions and the extent to which the generation or adoption of innovation contributes to organizational performance. However, two important trends have emerged. First, since organizations generate and adopt innovation continually over time, an assessment of the true impact of innovation on performance requires longitudinal research. Second, research on performance consequences of innovation has shifted from the stand-alone to synchronous innovations. The synchronous view departs from the prevailing logic that espouses autonomous strategies of innovation types for competitive advantage and submits that innovation types, along with organizational subsystems, are interdependent and their complementary introduction could best influence organizational conduct and outcome (Damanpour, 2014 ). In this vein, the notion of internal fit , which espouses congruency in the behavior of organizational parts, also applies to the introduction of types of innovations in organizations to facilitate external fit , which espouses congruency in the behavior of organizations with their competitive and institutional environments.
Conclusions and Future Research
A student in a doctoral seminar on the management of innovation observed that each article he reads adds one more star to the innovation galaxy, but the new star, as bright as it might be, does not improve his understanding of innovation. This student’s predicament is not unique to him or to innovation studies. Researchers may face similar predicaments in organizational sciences, where theories are incompatible, findings inconsistent, and the body of knowledge indigestible (Zammuto & Connolly, 1984 , p. 32). Research in management commonly pursues a scattered pattern where empirical studies are rarely replicated and can differ greatly in terms of definitions of key constructs, the nature of the phenomenon studied, and measurement instruments (Tsang & Kwan, 1999 ). Organizational studies are diverse and fragmented and theoretical and methodological consensus are not in sight (Hambrick, 1994 ; Pfeffer, 1993 ).
Assuming that diversity in innovation management research is unavoidable and consensus rather impossible, this article has mapped this research, identified major dimensions and their key components, discussed differences among components, and offered ideas to avoid unsuitable inferences. This section concludes by proposing steps to scan organizational innovation research, identify issues in the existing studies, and develop new studies. The study of innovation in organizations is theoretically and practically important, and ample opportunities exist for additional research to help explain how organizations innovate and in which contexts innovation could contribute to their conduct and outcomes. To advance the state of knowledge, innovation scholars should set out to demystify the innovation galaxy to allure new scholars and facilitate their learning rather than confusing them in the name of generating new theories (Hambrick, 2007 ).
Disciplinary Differences in Conceptualization of Innovation
Research on innovation from the economic perspective treats organization as a black box often recognized by its small or large size, sector or industry. Organizational innovation research, however, requires opening the black box, observing operational and administrative activities occurring in it, and explaining what set of activities could lead to innovation and how. Whereas insights from multiple disciplinary fields enrich research on innovation in organizations, the differences in conceptualizations, levels of analysis, and methodological predispositions should be accounted for. The absorption and integration of theories and findings from another discipline require a deliberate effort to articulate relevance and applicability. Otherwise, disciplinary differences will result in fragmentation and confusion rather than contribution and understanding.
Intradisciplinary differences also exist among subfields of innovation management, but the absorption and application of knowledge from one subfield to another is more feasible. For instance, to bridge the differences between innovation research from micro and macro organizational behavior, Crossan and Apaydin ( 2010 ) propose a unifying approach at a meso level to link managerial actions with innovation conduct and outcome. In another example, Keupp et al. ( 2012 ) identify theoretical inconsistencies and knowledge gaps in the strategic management of innovation, encourage strategy scholars to take notice and use insights from other subfields of innovation management, and advise them to scrutinize commonalities and differences in the definition and operationalization of innovation. Innovation strategy research would need to move beyond its mere focus on technological innovations and their singular impact on firm performance. Research on other types of innovation and how different types can be introduced and managed strategically can provide valuable insights for understanding management of innovation in strategy and other subfields of organization management.
Using innovation as a sweeping word that crosses disciplines will enlarge and further complicate the innovation galaxy. Authors should be cognizant of innovation as understood and defined in various disciplines and refrain from irrelevant importation and loose generalization. Reviewers and editors of academic journals should be more cognizant of authors’ limitations, and their own, refrain from discouraging repetitions, seeking new theory in every single submission, and protecting their personal investments in certain theoretical perspectives and methodologies (Starbuck, 2016 ). For a start, the type of innovation a paper studies and the context of the study should be included in the paper’s title. This simple act mitigates the major conceptual confusion in innovation research: mistaking technology for innovation. The context of innovation studies in the subfields of organization studies differs. The primary purpose, innovation issue, and key actors in small and large, and in business and public, organizations differ. For example, the central actor in a small start-up firm is the entrepreneur, but in medium-size and large organizations individual actors’ influence is mitigated by organizational culture, structure, power, and politics. In a business organization the ultimate outcome is often market share or financial outcome, in a government organization it is the reach and quality of services to citizens. These differences make the distinction between organizational types and innovating and innovativeness necessary for understanding, interpreting, and learning from the vast body of knowledge on innovation in organizations.
Generation, Adoption, and Organizational Type
In addition to the assumption that innovation is merely technology-based, many innovation studies also assume that innovation is a unitary process, and thus bypass the differences between generating and adopting innovations. Organizations can be generator of innovation, adopter of innovation, or both. They may also generate innovation for their own use, for external markets, or both. A distinction of the type of relationship between innovation and organization is necessary for deciphering the existing research and associating the conditions that prompt innovation in a certain type of organization (Kimberly, 1986 ).
Research on structuring for innovation has proposed several dual or ambidextrous structures based on initiation and implementation stages of adoption (Duncan, 1976 ), technical and administrative types of innovation (Daft, 1978 ), and radical and incremental types of innovation (Tushman & O’Reilly, 2002 ). Damanpour and Wischnevsky ( 2006 ) distinguished between organizations for generating innovations and organizations for adopting innovations. The innovation-generating organization, whether a new firm created by an entrepreneur or a self-contained unit of a large organization, requires the ability to accumulate knowledge and diffuse it inside the organization, motivate individuals’ and teams’ creative actions, and overcome technological and organizational obstacles to generate innovations expeditiously. The innovation-adopting organization mainly exploits current knowledge to seize new strategic opportunities or to solve existing organizational problems. It adopts new technologies, products, and practices available in the market, and applies them to improve its products, services, practices, and systems (Damanpour & Wischnevsky, 2006 ). These authors concluded that the typical questions of how to innovate, what conditions induce or drive innovativeness, and how innovation impacts performance should be broken into two sets of questions: one for generating innovations, another for adopting innovations.
The distinction between organizational types in general, and ambidextrous structures in particular, can help organize the existing research. Recognition of the differences between innovation generating and adopting organizations, for instance, is useful for separating the conditions that drive generation versus adoption, for aligning the studies of innovation in strategy with those in organization management, and helping to distinguish innovation activities for gaining competitive advantage from those for sustaining competitiveness.
Emerging Field of Nontechnological Innovations
Research on managerial innovation dates back to the 1960s–1980s. Birkinshaw et al.’s ( 2008 ) article rejuvenated interest in this innovation type, resulting in a considerable number of new studies. For instance, European Academy of Management has thus far sponsored three thematic conferences on management innovation, and two special issues edited by Volberda and colleagues (Volberda et al., 2013 ; Volberda, Van Den Bosch, & Mihalache, 2014 ) have been published. Recent articles have focused on theoretical perspectives, creation, adoption, and antecedents of managerial innovation, and have articulated future research avenues (Birkinshaw et al., 2008 , pp. 839–842; Damanpour, 2014 , pp. 1276–1279; Volberda et al., 2013 , p. 8; Volberda et al., 2014 , pp. 1258–1260). However, lack of consensus on the definition and measurement of managerial innovations remains an obstacle to the advancement of this untapped area of innovation research.
The OECD ( 2005 ) Oslo Manual added two types of nontechnological (organizational and marketing) innovations to technological (product and process) innovations, which were surveyed since 1993 . OECD’s organizational innovation (synonymous with managerial innovation as defined in this paper) is grouped into three types: business practices for organizing procedures, methods of organizing work responsibilities and decision-making , and methods of organizing external relations . It groups marketing innovation into four types: aesthetic design or packaging of good or service, techniques for product promotion ; methods for product placement , and methods for pricing goods or services. Data on organizational and marketing innovations are collected by seven dichotomous questions via Community Innovation Survey (CIS) since 2004 . Empirical studies have selectively included OECD’s organizational and marketing innovations in their operationalization of nontechnological innovations (Černe et al., 2016 ; Damanpour, 2014 ).
Černe et al. ( 2016 ) recommends coalescing various types of nontechnological innovations under one umbrella category. The category includes ancillary, business model, marketing, and open innovations, in addition to managerial innovation. Marketing innovation is an established innovation type, and has its own relatively large literature. It overlaps with the studies of product innovations, and relates mainly to the generation of innovations. Marketing and management are also two separate functional areas within business schools and are viewed as different specialties in business and management. However, in-depth comparative review analyses of managerial and marketing innovations may show that they do have a similar theoretical foundation and intellectual structure. Future research can explore such ideas and issues on the composition of nontechnological innovations.
Open innovation has been applied in strategic management and can be more easily integrated with the studies of innovation in other subfields of management. However, since closed innovation is an anomaly and cannot exist by definition, open innovation, similar to radicalness of innovation, can be regarded as a continuum rather than a type. Innovation openness , the extent to which organizations involve external players in various stages of generation or adoption of innovation, is applicable to all types of innovation, as is innovation radicalness. Future research on innovation openness should go beyond technological innovations in the goods sector and examine forms of external involvement for nontechnological innovations in services and public organizations. External partners, whether individuals or organizations, can participate and influence the creation and utilization of all types of innovation. For example, Birkinshaw et al. ( 2008 ) discussed roles of internal and external players in the development of management innovations and argued that external players (academic and nonacademic experts) have a more prominent role than internal players. Future research can also investigate the consequences of innovation openness. Innovation openness via strategic alliances and joint ventures is not a panacea for success. Cooperative innovation mode should be compatible with organizational culture and strategy, and interfirm differences should be managed effectively (Lichtenthaler, 2011 ). The success of innovation openness is not just a function of strategy formulation; instead, it depends heavily on organizational competencies for strategy implementation. Boeing’s experience in the design and production of the 787 Dreamliner is a revealing example (HBS Case #9-305-101).
Innovation from Garage, Innovation from Office
In the late 19th century Gabriel Trade, a sociologist, portrayed innovation as imitation concerning social transformation; in the early 20th century Joseph Schumpeter, an economist, set the ground for portraying innovation (new combination) as technical invention concerning economic development (Godin, 2008 ). Schumpeter’s entrepreneurial model of innovation underlined the role of entrepreneurs for bringing technical and social change, and personal and economic prosperity. In North America, innovation as a function of entrepreneurs continues to fascinate researchers, practitioners, and the general public. Young people, often without formal education or college dropouts, come up with an innovative product or service, launch and manage a new business, and gain enormous success. A contemporary example of this idealized model of innovation is the story of Steve Jobs and Apple, Inc. The corporate model of innovation, along with continued growth and global dominance of large corporations in the second half of the 20th century , brought attention to “innovation from office,” but did not replace “innovation from garage” in the public imagination.
Research on innovation in organizations has been influenced by both views. However, in medium-size or large organizations where the entrepreneurial stage has passed, formal structure and processes have been devised, and the leaders are professional managers, not owner-managers, innovation from office is essential. Managing innovation in a new enterprise in the early stages of its life cycle is different from that of a medium-size or large organization in the later stages. In the postentrepreneurial stages, for instance, the central actor for innovation is not the owner-manager or the CEO only. Influences of individual actors in innovation activities are mitigated by the organization’s culture and structure. Hence, reliance on the competition and performance perspective to explain innovation in medium-size and large organizations is inadequate. This perspective would need to be augmented or replaced with the adaptation and progression perspective in accordance with types and contexts of organizations. In this vein, research on organizational innovation would need to move beyond the 20th-century paradigm—that sustained long-term performance of organizations is based on the introduction of commercialized technology-based new products and processes—to a 21st-century paradigm in which continuous high performance pivots on the complementary effects of sets of innovation types guided by environmental demands and managerial aspirations.
Early steps toward the new paradigm have been taken. For instance, research on the relationship between innovation types has moved from a sequential pattern (product leads process, technical leads managerial) to a synchronous pattern (product and process intersect, technical and managerial complement), and from a focus on the importance of technological innovations for organizational effectiveness to one that also includes nontechnological innovations. Better theory and more empirical evidence are needed, however. Future studies of organizational innovation can contribute by continuing and advancing these new research trends by developing theory and investigating the dynamics of innovation types and their combinative effects on organizational conduct and outcome in a variety of contexts. The studies should also inform practitioners how to design and manage organizations for innovation, create and maintain a proinnovation culture and climate across organizational parts, drive continuous improvement of operations, systems, and human knowledge, and ensure that innovation not only benefits the organization but also does not harm the people and the environment.
Acknowledgement
The author thanks Rachel Bocquet, Benoît Godin, and the reviewers and editors of the Oxford Research Encyclopedia for Business and Management for their helpful comments.
- Abernathy, W. J. , & Utterback, J. M. (1978). Patterns of industrial innovation. Technology Review , 80 , 40–47.
- Abrahamson, E. (1991). Managerial fads and fashion: The diffusion and rejection of innovation. Academy of Management Review , 16 , 586–612.
- Ackoff, R. L. (1970). A concept of corporate planning . New York: Wiley-Interscience.
- Ackoff, R. L. (1981). Creating the corporate future . New York: John Wiley.
- Ackoff, R. L. , & Emery, F. E. (1972). On purposeful systems: An interdisciplinary analysis of individual and social behavior as a system of purposeful events . Chicago: Aldine-Atherton.
- Ahlstrom, D. (2010). Innovation and growth: How business contributes to society. Academy of Management Perspective, August , 10–23.
- Aiken, M. , & Hage, J. (1971). The organic organization and innovation. Sociology , 5 , 63–82.
- Allan, T. A. , & Sosa, M. L. (2004). 50 years of engineering management through the lens of the IEEE Transactions. IEEE Transactions on Engineering Management , 51 (4), 391–395.
- Amabile, T. M. (1988). A model of creativity and innovation in organizations. In L. L. Cummings & B. M. Staw (Eds.), Research in organizational behavior (Vol. 10, pp. 123–167). Greenwich, CT: JAI Press.
- Anderson, N. , Potočnik, K. , & Zhou, J. (2014). Innovation and creativity in organizations: A state-of-the-science review, prospective commentary, and guiding framework. Journal of Management , 40 (5), 1297–1333.
- Andrews, F. M. , & Farris, G. F. (1967). Supervisory practices and innovation in scientific teams, Personnel Psychology , 20 (4), 497–515.
- Ang, S. , & Cummings, L. L. (1997). Strategic response to institutional influences on information systems outsourcing. Organization Science , 8 (3), 235–256.
- Angle, H. L. , & Van de Ven, A. H. (1989). Suggestions for managing the innovation journey. In A. H. Van de Ven , H. L. Angle , & M. S. Poole (Eds.), Research on the management of innovation: The Minnesota studies (pp. 663–697). New York: Oxford University Press.
- Ansari, S. M. , Fiss, P. C. , & Zajac, E. (2010). Made to fit: How practices vary as they diffuse. Academy of Management Review , 35 , 67–92.
- Ansoff, H. I. (1968). Corporate strategy: An analytic approach for business policy and growth . New York: McGraw-Hill.
- Armbruster, H. , Bikfalvi, A. , Kinkel, S. , & Lay, G. (2008). Organizational innovation: The challenge of measuring non-technical innovation in large-scale surveys. Technovation , 28 , 644–657.
- Arrow, K. J. (1962). The economic implications of learning by doing. The Review of Economic Studies , 29 (3), 155–173.
- Ashworth, R. , Boyne, G. , & Delbridge, R. (2009). Escape from the iron cage? Organizational change and isomorphic pressures in the public sector. Journal of Public Administration Research and Theory , 19 , 165–187.
- Baer, M. (2012). Putting creativity to work: The implementation of creative ideas in organizations. Academy of Management Journal , 55 , 1102–1119.
- Baldridge, J. V. , & Burnham, R. A. (1975). Organizational innovation, individual, organizational, and environmental impacts. Administrative Science Quarterly , 20 , 165–176.
- Ballot, G. , Fakhfakh, F. , Galia, F. , & Slater, A. (2015). The fateful triangle: Complementarities in performance between products, process and organizational innovation in France and the UK. Research Policy , 44 , 217–232.
- Bantel, K. A. , & Jackson, S. E. (1989). Top management and innovations in banking: Does the composition of the top management team make a difference. Strategic Management Journal , 10 (S), 107–124.
- Barnett, W. P. (1990). The organizational ecology of a technological system. Administrative Science Quarterly , 35 , 31–60.
- Barney, J. B. (2001). Is the resource-based view a useful perspective for strategic management research? Yes. Academy of Management Review , 26 , 41–56.
- Barras, R. (1986). Towards a theory of innovation in services. Research Policy , 15 , 161–173.
- Barras, R. (1990). Interactive innovation in financial and business services: The vanguard of the service revolution. Research Policy , 19 (3), 215–237.
- Battisti, G. , & Iona, A. (2009). The intra-firm diffusion of complementary innovations: Evidence from the adoption of management practices by British establishments. Research Policy , 38 , 1326–1339.
- Becker, S. W. , & Whisler, T. M. (1967). The innovative organization: A selective view of current theory and research. Journal of Business , 40 (4), 462–469.
- Bierly, P. , Damanpour, F. , & Santoro, M. (2009). The application of external knowledge: Organizational conditions for exploration and exploitation. Journal of Management Studies , 46 (3), 481–509.
- Birkinshaw, J. , Hamel, G. , & Mol, M. (2008). Management innovation. Academy of Management Review , 33 (4), 825–845.
- Boonstra, J. J. , & Vink, M. J. (1996). Technological and organizational innovation: A dilemma of fundamental change and participation. European Journal of Work and Organizational Psychology , 5 (3), 351–376.
- Boulding, K. (1956). General system theory: The skeleton of science. General Systems (Yearbook of the Society for the Advancement of General Systems Theory) , 1 , 11–17.
- Bowen, F. E. , Rostami, M. , & Steel, P. (2010). Timing is everything: A meta-analysis of the relationship between organizational performance and innovation. Journal of Business Research , 63 , 1179–1185.
- Boyne, G. A. (2002). Public and private management: What’s the difference? Journal of Management Studies , 39 , 97–129.
- Brozen, Y. (1951). Research, technology, and productivity. In L. R. Tripp (Ed.), Industrial productivity (pp. 25–49). Champaign, IL: Industrial Relations Research Association.
- Burke, W. W. (2002). Organization change: Theory and practice. Thousand Oaks, CA: SAGE.
- Burns, L. R. , & Wholey, D. R. (1993). Adoption and abandonment of matrix management programs: Effects of organizational characteristics and interorganizational networks. Academy of Management Journal , 36 (1), 106–138.
- Burns, T. , & Stalker, G. M. (1961). The management of innovation . London: Tavistock.
- Calantone, R. J. , Cavusgil, S. T. , & Zhao, Y. (2002). Learning orientation, firm innovation capability, and firm performance. Industrial Marketing Management , 31 (6), 515–524.
- Calantone, R. J. , Harmancioglu, N. , & Droge, C. (2010). Inconclusive innovation returns: A meta-analysis of research on innovation in new product development. Journal of Product Innovation Management , 27 , 1065–1081.
- Camisón, C. , Lapiedra, R. , Segarra, M. , & Boronat, M. (2004). A meta-analysis of innovation and organizational size. Organization Studies , 25 , 331–361.
- Černe, M. , Kaše, R. , & Škerlavaj, M. (2016). Non-technological innovation research: Evaluating the intellectual structure and prospects of an emerging field. Scandinavian Journal of Management , 32 , 69–85.
- Chandy, R. K. , & Tellis, G. L. (2000). The incumbent’s curse? Incumbency, size, and radical product innovation, Journal of Marketing , 64 , 1–17.
- Cheng, Y. , & Van de Ven, A. H. (1996). Learning the innovation journey: Order out of chaos. Organization Science , 7 , 593–614.
- Chesbrough, H. W. (2003). The era of open innovation. Sloan Management Review , 44 (3), 35–41.
- Chesbrough, H. W. , & Appleyard, M. M. (2007). Open innovation and strategy. California Management Review , 50 (1).
- Christensen, C. M. (1997). The innovation’s dilemma . Boston: Harvard Business School Press.
- Churchman, C. W. (1968). The systems approach . New York: Delacorte Press.
- CIS . (2010). The Community Innovation Survey . Luxembourg: Eurostat.
- Cohen, W. M. , & Levin, R. C. (1989). Empirical studies of innovation and market structure. In R. Schmalansee & R. D. Willing (Eds.), Handbook of industrial organization (Vol. 2, pp. 1059–1107). Oxford: Elsevier.
- Cohen, W. M. , & Levinthal, D. A. (1990). Absorptive capacity: A new perspective on learning and innovation. Administrative Science Quarterly , 35 , 128–152.
- Crossan, M. M. , & Apaydin, M. (2010). A multi-dimensional framework of organizational innovation: A systematic review of the literature, Journal of Management Studies , 47 (6), 1154–1191.
- Cyert, R. , & March, J. (1963). A behavioral theory of the firm . Englewood Cliffs, NJ: Prentice-Hall.
- Daft, R. L. (1978). A dual-core model of organizational innovation. Academy of Management Journal , 21 , 193–210.
- Daft, R. L. (2001). Organization theory and design . Cincinnati, OH: South-Western.
- Daft, R. L. , & Becker, S. W. (1978). The innovative organization . New York: Elsevier.
- Damanpour, F. (1987). The adoption of technological, administrative, and ancillary innovations: Impact of organizational factors, Journal of Management , 13 , 675–688.
- Damanpour, F. (1991). Organizational innovation: A meta-analysis of effects of determinants and moderators. Academy of Management Journal , 34 , 555–590.
- Damanpour, F. (1992). Organizational size and innovation. Organization Studies , 13 , 375–402.
- Damanpour, F. (2010). An integration of research findings of effects of firm size and market competition on product and process innovations. British Journal of Management , 21 , 996–1010.
- Damanpour, F. (2014). Footnotes to research on management innovation. Organization Studies , 39 (5), 1265–1285.
- Damanpour, F. , & Aravind, D. (2006). Product and process innovations: A review of organizational and environmental determinants. In J. Hage & M. Meeus (Eds.), Innovation, science, and institutional change: A research handbook (pp. 38–66). Oxford: Oxford University Press.
- Damanpour, F. , & Aravind, D. (2012a). Managerial innovation: Conceptions, processes, and antecedents. Management and Organization Review , 8 (2), 423–454.
- Damanpour, F. , & Aravind, D. (2012b). A review of research on organizational structure and innovation: From organic to ambidextrous structure. In M. D. Mumford (Ed.), Handbook of organizational creativity (pp. 483–513). Boston: Academic Press.
- Damanpour, F. , & Evan, W. M. (1984). Organizational innovation and performance: The problem of organizational lag. Administrative Science Quarterly , 29 , 392–409.
- Damanpour, F. , & Gopalakrishnan, S. (1998). Theories of organizational structure and innovation adoption: The role of environmental change. Journal of Engineering and Technology Management , 15 , 1–24.
- Damanpour, F. , Sanchez, F. , & Chiu, H. H. (2017). Management Innovativeness: Effects of internal and external involvement and implementation actions. Paper presented at the European Academy of Management, Glasgow.
- Damanpour F. , & Schneider, M. (2006). Phases of the adoption of innovation in organizations: Effects of environment, organization, and top managers. British Journal of Management , 17 , 215–236.
- Damanpour, F. , & Schneider, M. (2009). Characteristics of innovation and innovation adoption in public organizations: Assessing the role of management. Journal of Public Administration Research and Theory , 19 , 495–522.
- Damanpour, F. , Walker, R. M. , & Avellaneda, C. N. (2009). Combinative effects of innovation types and organizational performance: A longitudinal study of services organizations. Journal of Management Studies , 46 (4), 650–675.
- Damanpour, F. , & Wischnevsky, J. D. (2006). Research on innovation in organizations: Distinguishing innovation-generating from innovation-adopting organizations. Journal of Engineering and Technology Management , 23 , 269–291.
- De Vries, H. , Bekkers, V. , & Tummers, L. (2016). Innovation in the public sector: A systematic review and future research agenda. Public Administration , 94 (1), 146–166.
- Dewar, R. D. , & Dutton, J. E. (1986). The adoption of radical and incremental innovations: An empirical analysis. Management Science , 32 , 1422–1433.
- Drucker, P. F. (1985). The discipline of innovation. Harvard Business Review , 63 (3), 67–72.
- Duncan, R. B. (1976). The ambidextrous organization: Designing dual structures for innovation. In R. H. Kilmann , L. R. Pondy , & D. P. Slevin (Eds.), The management of organizational design: Strategy implementation , 1 , 167–188. New York: North-Holland.
- Economist . (2007, March 1). The rise and fall of corporate R&D.
- Edquist, C. , Hommen, C. L. , & McKelvey, M. (2001). Innovation and employment: Process versus product innovation . Cheltenham, U.K.: Edward Elgar.
- Eisenhardt K. , & Martin, J. A. (2000). Dynamic capabilities: What are they? Strategic Management Journal , 21 , 1105–1121.
- Ekvall, G. (1996). Organizational climate for creativity and innovation. European Journal of Work and Organizational Psychology , 5 , 105–123.
- Ekvall, G. , & Arvonen, J. (1991). Change-centered leadership: An extension of the two-dimensional model. Scandinavian Journal of Management , 7 , 17–26.
- Elenkov, D. S. , Judge, W. , & Wright, P. (2005). Strategic leadership and executive innovation influence: An international multi-cluster comparative study, Strategic Management Journal , 26 , 665–682.
- Emery, F. E. , & Trist, E. L. (1960). Socio-technical system. In C. W. Churchman & M. Verhulst (Eds.), Management science: Models and techniques (pp. 83–97). Oxford: Pergamon.
- Ettlie, J. E. (1988). Taking charge of manufacturing: How companies are combining technological and organizational innovations to compete successfully . San Francisco: Jossey-Bass.
- Ettlie, J. E. , Bridges, W. P. , & O’Keefe, R. D. (1984). Organization strategy and structural differences for radical versus incremental innovation. Management Science , 30 , 682–695.
- Evan, W. M. (1966). Organizational lag. Human Organization , 25 , 51–53.
- Evan, W. M. (1976). Organization theory and organizational effectiveness: An exploratory analysis. Organization and Administrative Science , 7 , 15–28.
- Evangelista, R. , & Vezzani, A. (2010). The economic impact of technological and organizational innovations: A firm level analysis. Research Policy , 39 (10), 1253–1263.
- Fagerberg, J. (2005). Innovation: A guide to the literature. In J. Fagerberg , D. C. Mowery , & R. R. Nelson (Eds.), The Oxford handbook of innovations (pp. 1–26). Oxford: Oxford University Press.
- Fagerberg, J. , Mowery, D. C. , & Nelson, R. R. (2005). The Oxford handbook of innovations . Oxford: Oxford University Press.
- Ford, C. M. (1995). Creativity is a mystery: Clues from the investigators’ notebooks. In C. M. Ford & D. A. Gioia (Eds.), Creative action in organizations (pp. 12–49). Thousand Oaks, CA: SAGE.
- Ford, C. M. (1996). A theory of individual creative action in multiple social domains. Academy of Management Review , 21 , 1112–1142.
- Georgantzas, N. C. , & Shapiro, J. H. (1993). Viable theoretical forms of synchronous product innovation. Journal of Operations Management , 11 , 161–183.
- Godin, B. (2008). Innovation: The history of a category. Project on the Intellectual History of Innovation, Working Paper No. 1. Montreal: INRS (Institut national de la recherche scientifique).
- Godin, B. (2014). Innovation and creativity: A slogan, nothing but a slogan. In C. Antonelli & A. N. Link (Eds.), Routledge handbook of the economics of knowledge (pp. 7–19). London: Routledge.
- Godin, B. (2015a). Innovation contested: The idea of innovation over the centuries. London: Routledge.
- Godin, B. (2015b). Technological change: What do technology and change stand for? Project on the Intellectual History of Innovation, Working Paper No. 24. Montreal: INRS.
- Gopalakrishnan, S. , & Damanpour, F. (1994). Patterns of generation and adoption of innovations in organizations: Contingency models of innovation attributes. Journal of Engineering and Technology Management , 11 , 95–116.
- Gopalakrishnan, S. , & Damanpour, F. (1997). A review of innovation research in economics, sociology, and technology management. Omega , 25 , 15–28.
- Gordon, R. (2016). The rise and fall of American growth . Princeton, NJ: Princeton University Press.
- Grant, A. (2016). Originals . New York: Viking.
- Grant, R. M. (1996). Toward a knowledge-based theory of the firm. Strategic Management Journal , 17 , 109–122.
- Greve, H. R. (1995). Jumping ship: The diffusion of strategy abandonment. Administrative Science Quarterly , 40 , 444–473.
- Hage, J. , & Aiken, M. (1970). Social change in complex organizations . New York: Random House.
- Hambrick, D. (1994). What if the academy actually mattered? Academy of Management Review , 19 , 11–16.
- Hambrick, D. C. (2007). The field of management’s devotion to theory: Too much of a good thing? Academy of Management Journal , 50 (6), 1346–1352.
- Hamel, G. (2006). The why, what and how of management innovation. Harvard Business Review , 84 (2), 72–84.
- Helfat, C. E. , & Winter, S. G. (2011). Untangling dynamic and operational capabilities: Strategy for the (n)ever-changing world. Strategic Management Journal , 29 , 79–81.
- Henderson, R. M. , & Clark, K. B. (1990). Architectural innovation: The reconfiguration of existing product technologies and the failure of established firms. Administrative Science Quarterly , 35 , 9–30.
- Hervas-Oliver, J.-L. , & Sempere-Ripoll, F. (2014). Disentangling the influence of technological process and product innovations. Journal of Business Research , 68 , 109–118.
- Hitt, M. A. , Hoskisson, R. E. , & Ireland, R. D. (1990). Mergers and acquisitions and managerial commitment to innovation in m-form firms. Strategic Management Journal , 11 (S), pp. 29–47.
- Hitt, M. A. , Ireland, R. D. , Camp, S. M. , & Sexton, D. L. (2001). Strategic entrepreneurship: Entrepreneurial strategies for wealth creation. Strategic Management Journal , 22 , 479–491.
- Hoffman, R. C. , & Hegarty, W. H. (1993). Top management influence on innovations: Effects of executive characteristics and social culture. Journal of Management , 19 , 549–574.
- Jansen, J. J. P. , Van Den Bosch, F. A. J. , & Volberda, H. W. (2006). Exploratory innovation, exploitative innovation, and performance: Effects of organizational antecedents and environmental moderators. Management Science , 52 (11), 1661–1674.
- Jick, T. (1993). Managing change . New York: McGraw-Hill.
- Keupp, M. M. , Palmie, M. , & Gassmann, O. (2012). The strategic management of innovation: A systematic review and paths for future research. International Journal of Management Review , 14 , 367–390.
- Kimberly, J. R. (1981). Managerial innovation. In P. C. Nystrom and W. H. Starbuck (Eds.), Handbook of organizational design (pp. 84–104). New York: Oxford University Press.
- Kimberly, J. R. (1986). The organization context of technological innovation. In D. D. Davis (Ed.), Managing technological innovation (pp. 23–43). San Francisco: Jossey-Bass.
- Kimberly, J. R. , & Evanisko, M. (1981). Organizational innovation: The influence of individual, organizational, and contextual factors on hospital adoption of technological and administrative innovations. Academy of Management Journal , 24 , 679–713.
- King, N. (1990). Innovation at work: The research literature. In M. A. West & J. L. Farr (Eds.), Innovation and creativity at work (pp. 15–59). New York: Wiley.
- Klein, K. J. , & Sorra, J. S. (1996). The challenge of innovation implementation. Academy of Management Journal , 21 , 1055–1080.
- Lam, A. (2005). Organizational innovation. In J. Fagerberg , D. C. Mowery , & R. R. Nelson (Eds.), The Oxford handbook of innovations (pp. 115–147). Oxford: Oxford University Press.
- Lawless, M. W. , & Anderson, P. C. (1996). Generational technological change: Effects of innovation and local rivalry on performance. Academy of Management Journal , 39 , 1185–1217.
- Lawrence, P. R. , & Lorsch, J. W. (1967). Organization and environment . Homewood, IL: Irwin.
- Laursen, K. , & Salter, A. (2006). Open for innovation: The role of openness in explaining innovation performance among U.K. manufacturing firms. Strategic Management Journal , 27 , 131–150.
- Leiponen, A. , & Helfat, C. E. (2010). Innovation objectives, knowledge sources, and the benefit of breadth. Strategic Management Journal , 31 , 224–236.
- Lengnick-Hall, C. A. (1992). Innovation and competitive advantage: What we know and what we need to learn. Journal of Management , 18 (2), 399–429.
- Lichtenthaler, U. (2011). Open innovation: Past research, current debates, and future direction. Academy of Management Perspectives , 25 (1), 75–93.
- Lieberman, M. B. , & Montgomery, D. B. (1988). First mover advantages. Strategic Management Journal , 9 (S1), 41–58.
- Madjar, N. , Oldham, G. R. , & Pratt, M. G. (2002). There’s no place like home? The contributions of work and non-work creativity support to employees’ creative performance. Academy of Management Journal , 45 , 757–767.
- March, J. G. (1991). Exploration and exploitation in organizational learning. Organization Science , 2 , 71–87.
- Meeus, M. T. H. , & Edquist, C. (2006). Introduction to Part I: Product and process innovation. In J. Hage & M. Meeus (Eds.), Innovation, science, and institutional change (pp. 23–37). Oxford: Oxford University Press.
- Meyer, A. D. , & Goes, J. B. (1988). Organizational assimilation of innovations: A multilevel contextual analysis. Academy of Management Journal , 31 , 897–923.
- Miles, Ian . (2005). Innovation in services. In J. Fagerberg , D. C. Mowery , & R. R. Nelson (Eds.), The Oxford handbook of innovations (pp. 433–458). Oxford: Oxford University Press.
- Mintzberg, H. (1979). The structuring of organizations . Englewood Cliffs, NJ: Prentice-Hall.
- Mohr, L. B. (1969). Determinants of innovation in organizations, American Political Science Review , 63 , 111–126.
- Mol, M. J. , & Birkinshaw, J. (2014). The role of external involvement in the creation of management innovations. Organization Studies , 35 , 1287–1312.
- Mumford, M. D. (2000). Managing creative people: Strategies and tactics for innovation. Human Resources Management Review , 10 , 313–355.
- Mumford, M. D. , & Licuanan, B. (2004). Leading for innovations: Conclusions, issues, and directions. Leadership Quarterly , 15 , 163–171.
- Mumford, M. D. , Scott, G. M. , Gaddis, B. , & Strange, J. M. (2002). Leading creative people: Orchestrating expertise and relationships. Leadership Quarterly , 13 , 705–750.
- Nadler, D. A. , & Tushman, M. L. (1997). Implementing new designs: Managing organizational change. In M. L. Tushman & P. Anderson (Eds.), Managing strategic innovation and change (pp. 595–606). New York: Oxford.
- Naranjo-Gil, D. (2009). The influence of environmental and organizational factors on innovation adoption: Consequences for performance in public sector organizations. Technovation , 29 (12), 810–818.
- Nelson, R. , & Winter, S. (1982). An evolutionary theory of economic change . Cambridge, MA: Harvard University Press.
- Nord, W. R. , & Tucker, S. (1987). Implementing routine and radical innovations . Lexington, MA: Lexington Books.
- OECD (2005). Oslo manual: The measurement of scientific and technological activities. Paris: OECD.
- Pelz, D. C. (1956). Some social factors related to performance in a research organization. Administrative Science Quarterly , 1 (3), 310–325.
- Pelz, D. C. , & Andrews, F. M. (1966). Scientists in organizations: Productive climate for research and development . New York: Wiley.
- Pfeffer, J. (1993). Barriers to the advance of organizational science: Paradigm development as a dependent variable. Academy of Management Review , 18 , 599–620.
- Piketty, T. (2014). Capital in the twenty-first century . Cambridge, MA: Harvard University Press.
- Poole, M. S. (1981). Decision development in small groups I: A comparison of two models. Communication Monographs , 48 , 1–24.
- Poole, M. S. (1983). Decision development in small groups II: A study of multiple sequences in decision-making. Communication Monographs , 50 , 206–232.
- Porter, M. E. (1985). Competitive advantage: Creating and sustaining superior performance. New York: Free Press.
- Roberts, E. B. (1988). Managing invention and innovation. Research Management , 31 , 11–29.
- Roberts, P. W. , & Amit, R. (2003). The dynamics of innovative activity and competitive advantage: The case of Australian retail banking, 1981 to 1995. Organization Science , 14 , 107–122.
- Rogers, E. M. (1995). Diffusion of innovations . New York: Free Press.
- Rosenberg, N. (1982). Inside the black box: Technology and economics . London: Cambridge University Press.
- Rosenbusch, N. , Brinckmann, J. , & Bausch, A. (2011). Is innovation always beneficial? A meta-analysis of the relationship between innovation and performance in SMEs. Journal of Business Venturing , 26 , 441–457.
- Ross, P. F. (1974). Innovation adoption by organizations. Personnel Psychology , 27 (1), 21–47.
- Sanidas, E. (2005). Organizational innovations and economic growth: Organosis and growth of firms, sectors, and countries . Cheltenham, U.K.: Edward Elgar.
- Sapprasert, K. , & Clausen, T. H. (2012). Organizational innovation and its effects. Industrial and Corporate Change , 21 (5), 1283–1305.
- Schendel, D. , Ansoff, I. , & Channon, D. (1980). Statement of editorial policy. Strategic Management Journal , 1 (1), 1–5.
- Schilling, M. A. (2013). Strategic management of technological innovation . New York: McGraw-Hill Irwin.
- Schroeder, R. G. , Linderman, K. , Liedtke, C. , & Choo, A. S. (2008). Six sigma: Definition and underlying theory. Journal of Operations Management , 26 , 536–554.
- Schumpeter, J. A. (1934). The theory of economic development . Cambridge, MA: Harvard University Press.
- Schumpeter, J. A. (1983). The theory of economic development . New Brunswick, NJ: Transaction Publishers.
- Schumpeter, J. A. (1950). Capitalism, socialism and democracy . New York: Harper & Row.
- Scott, W. R. (1992). Organizations: Rational, natural and open systems . Englewood Cliffs, NJ: Prentice-Hall.
- Scott, S. G. , & Bruce, R. A. (1994). Determinants of innovative behavior: A path model of individual innovation in the workplace. Academy of Management Journal , 37 , 580–607.
- Sears, G. J. , & Baba, V. V. (2011). Toward a multistage, multilevel theory of innovation. Canadian Journal of Administrative Sciences , 28 , 357–372.
- Shane, S. , & Venkataraman, S. (2000). The promise of entrepreneurship as a field of research. Academy of Management Review , 25 , 217–226.
- Starbuck, W. H. (2016). 60th anniversary essay: How journals could improve research practices in social sciences. Administrative Science Quarterly , 61 (2), 165–183.
- Stata, R. (1989). Organizational learning: The key to management innovation. Sloan Management Review , 30 , 63–74.
- Staw, B. , & Epstein, L. (2000). What bandwagons bring? Effects of popular management techniques on corporate performance, reputation, and CEO pay. Administrative Science Quarterly , 45 (3), 523–556.
- Stevenson, H. H. , & Jarillo, J. C. (1990). A paradigm of entrepreneurship: Entrepreneurial management. Strategic Management Journal , 11 (S): 17–27.
- Sturdy, A. (2004). The adoption of management ideas and practices. Management Learning , 35 (2), 155–179.
- Teece, D. J. , Pisano, G. , & Shuen, A. (1997). Dynamic capabilities and strategic management. Strategic Management Journal , 18 , 509–533.
- Tether, B. S. , & Tajar, A. (2008). The organizational-cooperation mode of innovation and its prominence amongst European service firms. Research Policy , 37 , 720–739.
- Tidd, J. (2001). Innovation management in context: Environment, organization and performance. International Journal of Management Review , 3 (3), 169–183.
- Tornatzky, L. G. , & Fleischer, M. (1990). The process of technological innovation . Lexington, MA: Lexington Books.
- Tornatzky, L. G. , & Klein, K. J. (1982). Innovation characteristics and innovation adoption–implementation: A meta-analysis of findings. Transactions on Engineering Management , 29 (1), 28–45.
- Trist, E. , & Murray, H. (1993). The social engagement of social science: A Tavistock anthology, Vol. 1: The social-psychological perspective . Philadelphia: University of Pennsylvania Press.
- Tsang, E. W. K. , & Kwan, K. (1999). Replication and theory development in organizational science: A critical realistic perspective. Academy of Management Review , 24 , 759–780.
- Tushman, M. L. , & Anderson, P. (1986). Technological discontinuities and organizational environments. Administrative Science Quarterly , 31 , 439–465.
- Tushman, M. L. , & O’Reilly, C. A. (2002). Winning through innovation . Boston: HBS Press.
- Utterback, J. M. (1994). Mastering the dynamics of innovation . Cambridge, MA: Harvard Business Press.
- Vaccaro, I. G. , Jansen, J. J. P. , Van Den Bosch, F. A. J. , & Volberda, H. (2012). Management innovation and leadership: The moderating role of organizational size. Journal of Management Studies , 49 (1), 28–51.
- Van de Ven, A. H. , Angle, H. L. , & Poole, M. S. (1989). Research on the management of innovation: The Minnesota studies . New York: Oxford University Press.
- Van de Ven, A. H. , & Rogers, E. M. (1988). Innovations and organizations. Communication Research , 15 , 632–651.
- Van Den Bosch, F. A. J. , Volberda, H. W. , & de Boer, M. (1999). Coevolution of firm absorptive capacity and knowledge environment: Organizational forms and combinative capabilities. Organization Science , 10 , 551–568.
- Volberda, H. W. , Van Den Bosch, F. A. , & Heij, C. V. (2013). Management innovation: Management as fertile ground for innovation. European Management Review , 10 (1), 1–15.
- Volberda, H. W. , Van Den Bosch, F. A. , & Mihalache, O. R. (2014). Advancing management innovation: Synthesizing processes, levels of analysis, and change agents. Organization Studies , 35 (9), 1245–1264.
- Von Bertalanfy, L. (1951). General system theory: A new approach to unity of science, Human Biology , 23 , 303–361.
- Von Bertalanfy, L. (1968). General system theory: Foundations, development, applications . New York: G. Braziller.
- Wade, J. (1996). A community-level analysis of sources and rates of technological variation in the microprocessor market. Academy of Management Journal , 39 (5), 1218–1244.
- Walker, R. M. (2008). Empirical evaluation of innovation types and organizational and environmental characteristics: Towards a configuration approach. Journal of Public Administration Research and Theory , 18 , 591–615.
- Walker, R. , Chen, J. , & Aravind, D. (2015). Management innovation and firm performance: An integration of research findings, European Management Journal , 33 (5), 407–422.
- Walker, R. M. , Damanpour, F. , & Devece, C. A. (2011). Management innovation and organizational performance: Mediating role of planning and control. Journal of Public Administration Research and Theory , 21 , 367–386.
- West, M. A. , & Anderson, N. R. (1996). Innovation in top management teams. Journal of Applied Psychology , 81 , 680–693.
- West, M. A. , & Farr, J. L. (1990). Innovation and creativity at work . New York: Wiley.
- Whittington, R. , Pettigrew, A. , Peck, S. , Fenton, E. , & Conyon, M. (1999). Change and complementarities in the new competitive landscape: A European panel study, 1992–1996. Organization Science , 10 , 583–600.
- Wischnevsky, J. D. , & Damanpour, F. (2006). Organizational transformation and performance: An examination of three perspectives. Journal of Managerial Issues , 18 , 104–128.
- Wischnevsky, J. D. , Damanpour, F. , & Mendez, F. (2011). Influence of environmental factors and prior changes on the organizational adoption of changes in products and in technological and administrative processes. British Journal of Management , 22 (1), 132–149.
- Wolfe, R. A. (1994). Organizational innovation: Review, critique, and suggested research directions. Journal of Management Studies , 31 , 405–431.
- Woodman, R. W. , Sawyer, J. E. , & Griffin, R. W. (1993). Toward a theory of organizational creativity. Academy of Management Review , 18 (2), 293–321.
- Zajac, E. J. , Kraatz, M. S. , & Bresser, R. K. F. (2000). Modeling the dynamics of strategic fit: A normative approach to strategic change. Strategic Management Journal , 21 , 429–453.
- Zaltman, G. , Duncan, R. , & Holbek, J. (1973). Innovations and organizations . New York: Wiley.
- Zammuto, R. F. , & Connolly, T. (1984). Coping with disciplinary fragmentation. Organizational Behavior and Teaching Review , 9 , 30–37.
1. As research on innovation in organizations has developed in the second half of the 20th century, this article focuses on a more recent understanding of innovation as a concept in business and management. For a historical evolution of innovation as a term, label, action, goal, and concept across multiple disciplinary fields see Godin ( 2008 , 2014 , 2015a ).
2. It should be noted that this is Schumpeter’s most commonly cited definition of innovation. He has offered other definitions such as new combinations of the means of production or change in the inputs or outputs of production. For details see Godin ( 2008 , pp. 35–36) and Godin ( 2014 , pp. 13–15). Also, Schumpeter’s fifth innovation type—defined as “the carrying out of the new organization of any industry, like the creation of a monopoly position . . . or the breaking up of a monopoly position” (Schumpeter, 1983 , p. 66)—is not synonymous with organizational innovation as defined in this article.
3. Since Schumpeter’s early work in the beginning of the 20th century, it is generally viewed that innovation is a positive force not only for its producers but for the society as well. Yet, productivity growth has been mainly an outcome of automation, and the extent to which economic wealth spreads beyond entrepreneurs and corporations to the society at large is uncertain. For example, two recent economic analyses question the continued validity of the ripple-down of economic wealth (Gordon, 2016 ; Piketty, 2014 ).
4. For example, Lam ( 2005 ) classifies three perspectives: organizational structure and design; organizational cognition and learning; and organizational change and adaptation. In the context of organizational transformation and performance, Wischnevsky and Damanpour ( 2006 ) also offer three perspectives: rational and performance gap; population ecology and the liability of newness; and institutionalism and the mimetic pressure. Crossan and Apaydin ( 2010 ) listed five sets of theoretical perspectives used in the highly cited articles in their review: institutional; economics and evolution; network; resource-based view and dynamic capabilities; and learning, knowledge management, adaptation, and change.
5. Many studies either do not clearly identify the type of innovation or focus on product and process innovations. For example, Crossan and Apaydin’s ( 2010 , p. 1162) review of 524 articles on innovation published in 10 business and economic journals found that 50% of the articles were unclear or did not identify the type of innovation that was studied, and 39% were related to technology, product/service, and process innovations.
6. Godin ( 2008 , p. 8) offers the conjunction of two primary factors for the prominence of technological innovations: (1) the culture of thing, and industrial development through technology; and (2) the influence of the (academic) conceptual frameworks of technology on policies for economic growth. For more detailed aggregation of these factors see Godin ( 2008 , pp. 19–22).
7. Indeed, Woodman et al.’s ( 1993 ) propositions regarding the effects of slack resources, internal and external communication, and organic structure on organizational creative performance (production of organizational creativity) are similar to the effects of these factors on innovation as found in the studies of innovations in organizations (see Damanpour & Aravind, 2012b ).
8. Similarly, Godin ( 2008 ) in his historical analysis of the emergence of innovation as a concept suggests that the view of innovation as a novel or new idea, artifact, or behavior has emerged in order to resolve the tension between invention —the process of coming up with new ideas (generation)—and imitation —the process of putting those ideas into positive practice (adoption).
9. A parallel order can be offered for social technologies: social technology → social innovation → social change. Social change , change at the level of society including both economic and social/cultural domains, could be the outcome or the results of both technological and nontechnological changes. Like technological change, it is a higher concept than innovation and is not a focus of this article.
10. The innovation process can be conceived to follow a unitary or a multiple sequence pattern (Poole, 1981 ). The unitary sequence pattern generally assumes that the process is orderly and occurs in a linear sequence; the multiple sequence pattern assumes that the process is more random and the stages and the sequence of their occurrence cannot be predicted (Gopalakrishnan & Damanpour, 1994 ). Both patterns have been found useful in describing the innovation process in organizations. However, the multiple sequence pattern is more applicable to studies of innovating ; the unitary sequence pattern to the studies of innovativeness .
11. When an organization develops innovations for its own use, usually one unit (R&D, product development, design) develops and another unit (manufacturing, marketing, human resources) uses the innovation.
12. At the level of innovation, the process includes three sequential phases: generation, diffusion, and adoption. Diffusion is a process in which an innovation is communicated through certain channels among the members of a social system (Rogers, 1995 ). Diffusion connects generation to adoption, is studied at the level of population, and is not viewed as an organizational process. Hence, it is not discussed in this article.
13. Damanpour and Schneider ( 2006 ) consider adoption decision as a separate phase that includes evaluating the proposed ideas from technical, financial and strategic perspectives, making the decision to accept an idea as the desired solution and allocating resources for its acquisition, alteration, and assimilation. It is the phase in which organizational leaders (managers, committees, boards) decide to adopt the innovation and allocate resources to it.
14. As is common in economics, Meeus and Edquist ( 2006 ) use the term organizational innovation to refer to nontechnological innovations, whether product or process. In this article, the term organizational innovation refers to both technological and nontechnological innovations that organizations generate or adopt.
15. In management, organizational innovation is understood in two ways. In a more specific way, it means nontechnological, managerial innovation (Damanpour & Aravind, 2012a ). In a broader way, as in this article, it refers to innovations in organizations, whether technological or nontechnological (Crossan & Apaydin, 2010 ; Damanpour, 1991 ; Lam, 2005 ).
16. Innovations in the organization–environment relations are referred to as ancillary innovations (Damanpour, 1987 ). Ancillary innovations pertain to working with and learning from partners (service providers, suppliers, clients, customers, public agencies, and professional and educational institutions) across organizational boundaries (Černe et al., 2016 ; Damanpour, 1987 ; Tether & Tajar, 2008 ). They are a type of nontechnological innovation (Černe et al., 2016 ), and resemble Tether and Tajar’s ( 2008 ) organization–cooperation mode of innovation.
17. A fourth dimension—attributes or characteristics of innovation—is also used in innovation research to predict the speed of diffusion and/or the rate of adoption of innovation in social systems. Wolfe ( 1994 ) provided definitions of 18 attributes and identified approximately 20 more with different names but similar definitions. Rogers ( 1995 ) identified five primary innovation attributes, of which four (relative advantage, compatibility, trialability, and observability) positively, and one (complexity) negatively affect the adoption of innovation. In a quantitative review of the innovation attribute–adoption relationship from 75 studies, Tornatzky and Klein ( 1982 ) found that compatibility, relative advantage, and complexity had the most consistent significant relationships with innovation adoption. The level of analysis of innovation attributes research is primarily the innovation, not the organization.
18. Camisón et al.’s review included 87 correlations from 53 studies. In an earlier and smaller meta-analysis (36 correlations, 20 original studies), Damanpour ( 1992 ) found a larger magnitude of mean correlation between size and innovation (r=.32, p<.05, p. 384). The low magnitude of the mean correlation in Camisón et al.’s ( 2004 ) analysis can be attributed to the greater diversity of measurements of size and innovation in the original studies.
19. This finding is similar to the finding from Rosenbusch et al.’s ( 2011 ) meta-analytical review of 42 articles on the innovation–performance relationship in SMEs. The original articles in the two reviews do not overlap because the selection procedure in the two reviews differs. Rosenbausch et al.’s original studies include merely technological innovations; innovation types in Walker et al.’s ( 2015 ) original studies are more varied. While Rosenbusch et al.’s review subscribes to the first-mover advantage view of innovation and performance, Walker et al.’s review falls mainly within the performance gap view.
20. For example, a TQM program has many elements such as service design, employee involvement, customer focus, and so on (Schroeder et al., 2008 , p. 547). An organization may choose to adopt a couple of these elements only or adopt some of them initially and others at a later time.
Related Articles
- Product and Innovation Portfolio Management
- Science, Technology, and Innovation Policy: Old Patterns and New Challenges
Printed from Oxford Research Encyclopedias, Business and Management. Under the terms of the licence agreement, an individual user may print out a single article for personal use (for details see Privacy Policy and Legal Notice).
date: 28 March 2024
- Cookie Policy
- Privacy Policy
- Legal Notice
- Accessibility
- [66.249.64.20|185.80.151.9]
- 185.80.151.9
Character limit 500 /500
Mustafa Suleyman, DeepMind and Inflection Co-founder, joins Microsoft to lead Copilot
Mar 19, 2024 | Microsoft Corporate Blogs
- Share on Facebook (opens new window)
- Share on Twitter (opens new window)
- Share on LinkedIn (opens new window)
Satya Nadella, Chief Executive Officer, shared the below communication today with Microsoft employees.
I want to share an exciting and important organizational update today. We are in Year 2 of the AI platform shift and must ensure we have the capability and capacity to boldly innovate.
There is no franchise value in our industry and the work and product innovation we drive at this moment will define the next decade and beyond. Let us use this opportunity to build world-class AI products, like Copilot, that are loved by end-users! This is about science, engineering, product, and design coming together and embracing a learning mindset to push our innovation culture and product building process forward in fundamental ways.
In that context, I’m very excited to announce that Mustafa Suleyman and Karén Simonyan are joining Microsoft to form a new organization called Microsoft AI, focused on advancing Copilot and our other consumer AI products and research.
Mustafa will be EVP and CEO, Microsoft AI, and joins the senior leadership team (SLT), reporting to me. Karén is joining this group as Chief Scientist, reporting to Mustafa. I’ve known Mustafa for several years and have greatly admired him as a founder of both DeepMind and Inflection, and as a visionary, product maker, and builder of pioneering teams that go after bold missions.
Karén, a Co-founder and Chief Scientist of Inflection, is a renowned AI researcher and thought leader, who has led the development of some of the biggest AI breakthroughs over the past decade including AlphaZero.
Several members of the Inflection team have chosen to join Mustafa and Karén at Microsoft. They include some of the most accomplished AI engineers, researchers, and builders in the world. They have designed, led, launched, and co-authored many of the most important contributions in advancing AI over the last five years. I am excited for them to contribute their knowledge, talent, and expertise to our consumer AI research and product making.
At our core, we have always been a platform and partner-led company, and we’ll continue to bring that sensibility to all we do. Our AI innovation continues to build on our most strategic and important partnership with OpenAI. We will continue to build AI infrastructure inclusive of custom systems and silicon work in support of OpenAI’s foundation model roadmap, and also innovate and build products on top of their foundation models. And today’s announcement further reinforces our partnership construct and principles.
As part of this transition, Mikhail Parakhin and his entire team, including Copilot, Bing, and Edge; and Misha Bilenko and the GenAI team will move to report to Mustafa. These teams are at the vanguard of innovation at Microsoft, bringing a new entrant energy and ethos, to a changing consumer product landscape driven by the AI platform shift. These organizational changes will help us double down on this innovation.
Kevin Scott continues as CTO and EVP of AI, responsible for all-up AI strategy, including all system architecture decisions, partnerships, and cross-company orchestration. Kevin was the first person I leaned on to help us manage our transformation to an AI-first company and I’ll continue to lean on him to ensure that our AI strategy and initiatives are coherent across the breadth of Microsoft.
Rajesh Jha continues as EVP of Experiences & Devices and I’m grateful for his leadership as he continues to build out Copilot for Microsoft 365, partnering closely with Mustafa and team.
There are no other changes to the senior leadership team or other organizations.
We have been operating with speed and intensity and this infusion of new talent will enable us to accelerate our pace yet again.
We have a real shot to build technology that was once thought impossible and that lives up to our mission to ensure the benefits of AI reach every person and organization on the planet, safely and responsibly. I’m looking forward to doing so with you.
- Check us out on RSS
- InterDigital-stock
- News for InterDigital InterDigital
InterDigital to Showcase Innovations in High Definition and Sustainable Video Streaming Alongside Partners at NAB 2024
WILMINGTON, Del., March 26, 2024 (GLOBE NEWSWIRE) -- InterDigital, Inc. (Nasdaq: IDCC), a mobile, video and AI technology research and development company, announced plans to showcase their contributions to video streaming and sustainability technologies alongside partners at NAB 2024. As critical contributor to Advanced HDR by Technicolor® solutions and longtime member of the Ultra HD Forum, InterDigital will showcase advancements in high dynamic range (HDR) production, distribution, and display solutions and AI-enhanced energy efficient streaming technologies at NAB 2024, taking place in Las Vegas from April 13 – 17.
Advanced HDR Streaming Solutions A collaboration between Philips, InterDigital and Technicolor, Advanced HDR by Technicolor® is a suite of HDR solutions that leverage machine learning to maximize image quality and enhance the consumer viewing experience. At NAB, partners InterDigital and Philips will highlight progress in industry adoption of Advanced HDR solutions that support the bandwidth-efficient distribution of HDR content for any type of video streaming service provider or live broadcast production workflow. The solution enables end-to-end HDR and SDR production with a single master workflow and ensures optimal source fidelity on both SDR-to-HDR and HDR-to-SDR conversions. Advanced HDR by Technicolor remains the only solution delivering HDR broadcasts of any content, any time, and anywhere over the ATSC 3.0 NextGen TV stations.
- Our contributions to Advanced HDR streaming solutions can be experienced at the ATSC booth at W3056 in West Hall, at the Ultra HD Forum booth at W4007 in West Hall, and by invitation at the Advanced HDR by Technicolor Meeting Room at W2476.
- In addition, InterDigital will deliver a presentation as part of NAB’s Broadcast Engineering and IT conference. The session, “ HDR-SDR conversion: Live HDR Single Master Production Conversion Interoperability Challenges ” will take place on Tuesday, April 16 from 10:00 AM - 10:20 AM PST in room W222-W223.
Sustainable Streaming Solutions At NAB booth W4007, members of the Ultra HD Forum will showcase current and future-looking solutions and strategies that support the sustainable delivery of Ultra HD content. As a member of the Ultra HD Forum, InterDigital leads and contributes to three of the demonstrations on display, including:
- “Pixel Value Reduction in HDR Content” and the ways we leverage InterDigital’s AI expertise to adjust pixel luminance to reduce energy consumption with minimal impact on visual quality.
- “ Energy Efficient Enhanced Media Streaming” alongside partner ATEME to display the impact of luminance adjustment on energy usage and the strategic insertion of video metadata to enhance content delivery and viewer experiences.
- “ Viewing Condition Impacts on Display Energy Consumption ” alongside collaborator CTOIC to illustrate the influence of ambient lighting on modern TV energy usage.
To register and learn more, please visit the NAB 2024 website here.
About Advanced HDR by Technicolor A collaboration between Philips, InterDigital and Technicolor, Advanced HDR by Technicolor® is a suite of High Dynamic Range (HDR) production, distribution and display solutions that leverages machine learning (ML) technology to maximize image quality and enhance the consumer viewing experience. There are two major components to Advanced HDR by Technicolor:
- The Intelligent Tone Management (ITM) tool provides a dynamic, tunable, real-time solution to up-convert SDR content to HDR with full freedom and flexibility to manage contrast, brightness and color saturation.
- The Single Layer HDR (SL-HDR) is a dynamic and tunable real-time tool that implements the ETSI SL-HDR standards to generate and deliver a single, consistent, high-quality broadcast stream starting from any mix of input content (such as live, movies, news) across a wide range of HDR formats (HDR10, HLG, S-LOG3). SL-HDR1 transforms HDR input streams into SDR-plus-metadata streams. SL-HDR compatible receivers provide consumers with high-quality HDR images that can be adapted to optimize the display capabilities of their devices. Thanks to the unique backward compatibility feature of SL-HDR1, consumers who do not have HDR devices can enjoy the highest quality SDR experience.
To learn more about Advanced HDR by Technicolor visit: https://advancedhdrbytechnicolor.com/
About Ultra HD Forum Founded in 2015, the Ultra HD Forum accelerates Ultra HD adoption by establishing best practices for the future of television. The Forum is a crucible for interoperability testing and standard synchronization.
For more information, please visit https://ultrahdforum.org or @UltraHDForum on LinkedIn.
About InterDigital ® InterDigital is a global research and development company focused primarily on wireless, video, artificial intelligence (“AI”), and related technologies. We design and develop foundational technologies that enable connected, immersive experiences in a broad range of communications and entertainment products and services. We license our innovations worldwide to companies providing such products and services, including makers of wireless communications devices, consumer electronics, IoT devices, cars and other motor vehicles, and providers of cloud-based services such as video streaming. As a leader in wireless technology, our engineers have designed and developed a wide range of innovations that are used in wireless products and networks, from the earliest digital cellular systems to 5G and today’s most advanced Wi-Fi technologies. We are also a leader in video processing and video encoding/decoding technology, with a significant AI research effort that intersects with both wireless and video technologies. Founded in 1972, InterDigital is listed on Nasdaq. InterDigital is a registered trademark of InterDigital, Inc.
For more information, visit: www.interdigital.com.
InterDigital Contact: Roya Stephens Email: [email protected] Phone: +1 (202) 349-1714

InterDigital News MORE
Related stocks.
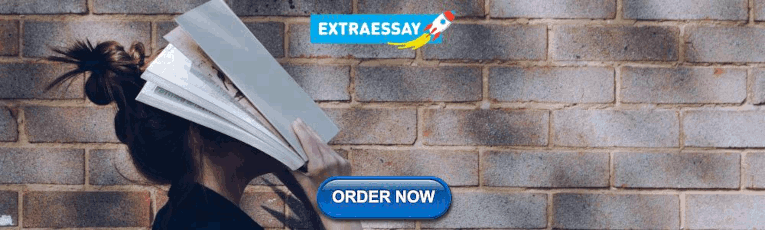
IMAGES
VIDEO
COMMENTS
This definition explicitly acknowledges the application of new ideas, rather than just the creative process itself (Acar, Tarakci, & van Knippenberg, 2019). Our focus is specifically on innovation in research methods, in other words, the introduction and application of (parts of) research methods that are new or seldom used in a research field.
Abstract. Innovative research is the medium required to drive the transformations we need for a better world. However, innovative research needs to be 'humanitarian' to birth products and services ...
In a business context, innovation is the ability to conceive, develop, deliver, and scale new products, services, processes, and business models for customers. Successful innovation delivers net new growth that is substantial. As McKinsey senior partner Laura Furstenthal notes in an episode of the Inside the Strategy Room podcast, "However ...
Innovation in qualitative research is a thorny issue. It first requires a shared understanding of what innovation actually means in this context. One definition is 'the intentional introduction and application of ideas, processes, products or procedures, new to the relevant unit of adoption, designed to significantly benefit' (Lê and ...
Carrying out research means being innovative, which requires novelty. Novelty is an important source of scientific breakthroughs and has great technological impact. Research institutions stand to benefit from fostering innovation. Here, we outline what academic institutions can do to help their scientists become more innovative.
Innovation is an approach organizations use to introduce changes to survive and thrive during uncertain and turbulent conditions. The word "organizations" connotes a variety of for-profit and non-profit oriented, private-, public-, third-sector, and hybrid firms (e.g., social enterprises).
Scholars keep a high interest in further exploring open innovation as also reflected by some of the more recent reviews on the topic: K. Randhawa, R. Wilden, and J. Hohberger, "A Bibliometric Review of Open Innovation: Setting a Research Agenda," Journal of Product Innovation Management, 33/6 (November 2016): 750-772; J. West and M. Bogers ...
This paper uses the more broad-encompassing concept of "innovation research," which we define, following Martin (2012), as the study of all the processes, explanatory factors, and economic and social consequences of innovation, regardless of the disciplines or perspectives involved. 2.2. Data-driven studies on the evolution of innovation ...
2 Innovation management - a few definitional issues. Innovation refers to the discovery, development, implementation and adoption of new products, services, or business models; new ways of organising the value chain; new production processes; and new forms of organisational structure (Tidd et al., 2013). As this definition suggests, the term ...
The starting point is a thorough analysis of the type of innovation, the phase in the innovation process, potential adopters and promoters of all kinds, barriers of implementation and a temporal and spatial diffusion path. The conceptual framework presented in this paper is designed to support this analysis.
Placing Developmental Psychology on the Stage of Innovation Research. There is a rich history, across academic disciplines, of applying the concepts, theories, and empirical advances of one field of study to another. ... Although this review ultimately provides an individual‐level definition of innovation, it is impossible to detach ...
Emerging innovative qualitative research methodologies in social sciences are briefly identified, and arguments for and against methodological innovation are explored. The stages of creativity and ...
Types of innovation. An innovation, by definition, is the process of developing or renewing a technique or product to create or improve value. ... It utilizes brainstorming, design thinking, and research and development to inspire innovations that drive revenue for the company. ... The innovation process describes all of the elements involved ...
The growth of innovation research is evidenced by a keyword search of articles published in ecology or evolution between 1997 and 2017, 1 which yielded an approximate fourfold increase in ... but that innovations are to some extent environment-specific, meaning that innovations are potentially gained and lost when populations migrate or ...
It's true that innovation relies, to an extent, on the vagaries of ingenuity. But according to McKinsey research, innovation—and, crucially, the type of outperformance that innovation can spark in organizations—is much more likely to happen when there is a rigorous process in place to bring ideas to fruition.
Within the science domain, we investigate a research field that should - by definition - be more enamoured of openness: innovation research, where researchers have strongly advocated the importance of openness for all types of innovation outcomes (e.g. Bogers et al. Citation 2017; von Hippel Citation 2017).
Innovation is defined as the process of bringing about new ideas, methods, products, services, or solutions that have a significant positive impact and value. It involves transforming creative concepts into tangible outcomes that improve efficiency, and effectiveness, or address unmet needs. Innovation is not limited to technological ...
Entrepreneurship and innovation. The definition of entrepreneurship has been increasingly reduced to innovative activities. ... and management research. Innovation plays a significant role in the development of Society 5.0, which is a vision of a future society guided by scientific and technological innovation, aiming to create a human-cantered ...
The research work stream provides a thoughtful reading and interpretation of the current and future state of humanitarian affairs, making space for reflection and a multiplicity of views and perspectives, This work carves out a space for research to not only to 'inform' and understand innovation better but also to be a place to experiment with methodologies, knowledge, disciplines and ideas.
Academic research on innovation in social sciences has markedly increased since the 1950s (Crossan & Apaydin, 2010; Fagerberg, 2005).Innovation, however, is a much older term and its meaning, understanding, and terminology have changed over time.
Innovation consists of the generation of a new idea and its implementation into a new product, process or service. .." In the opinion of Rowley J. et al. (2011 in Timur [5]), innovation includes ...
Some companies do succeed at innovation. Our research considered how proficient 183 companies were at innovation, and compared that assessment against a proprietary database of economic profit (the total profit minus the cost of capital). We found that companies that harness the essentials of innovation see a substantial performance edge that
RRI and its meaning. Within the framework of RRI, various approaches have emerged, such as the dimensions known as AIRR or AREA: Anticipation, Inclusion, Reflexibility, and Responsiveness (Stilgoe, Owen, and Macnaghten Citation 2013).According to these authors, these dimensions should be taken into account when defining the context of research processes: in the organizations that undertake ...
I am excited for them to contribute their knowledge, talent, and expertise to our consumer AI research and product making. At our core, we have always been a platform and partner-led company, and we'll continue to bring that sensibility to all we do. Our AI innovation continues to build on our most strategic and important partnership with OpenAI.
The research concludes that innovation can be identified with the creation of a new product or service or an improvement of an existing product or service. ... definition of innovation is the ...
WILMINGTON, Del., March 26, 2024 (GLOBE NEWSWIRE) -- InterDigital, Inc. (Nasdaq: IDCC), a mobile, video and AI technology research and development company, announced plans to showcase their ...