Subscribe to the PwC Newsletter
Join the community, trending research, evolutionary optimization of model merging recipes.

Surprisingly, our Japanese Math LLM achieved state-of-the-art performance on a variety of established Japanese LLM benchmarks, even surpassing models with significantly more parameters, despite not being explicitly trained for such tasks.
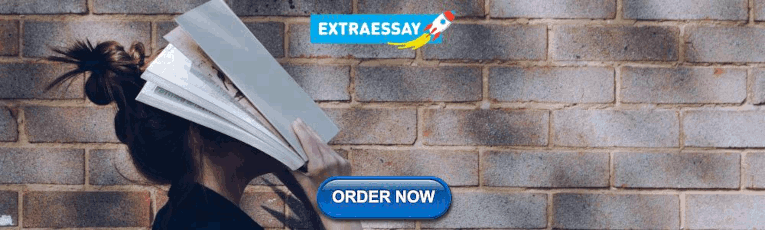
Mora: Enabling Generalist Video Generation via A Multi-Agent Framework
lichao-sun/mora • 20 Mar 2024
Sora is the first large-scale generalist video generation model that garnered significant attention across society.
FRESCO: Spatial-Temporal Correspondence for Zero-Shot Video Translation
In this paper, we introduce FRESCO, intra-frame correspondence alongside inter-frame correspondence to establish a more robust spatial-temporal constraint.
One-Step Image Translation with Text-to-Image Models
In this work, we address two limitations of existing conditional diffusion models: their slow inference speed due to the iterative denoising process and their reliance on paired data for model fine-tuning.

MVSplat: Efficient 3D Gaussian Splatting from Sparse Multi-View Images

We propose MVSplat, an efficient feed-forward 3D Gaussian Splatting model learned from sparse multi-view images.

GRM: Large Gaussian Reconstruction Model for Efficient 3D Reconstruction and Generation
justimyhxu/grm • 21 Mar 2024
We introduce GRM, a large-scale reconstructor capable of recovering a 3D asset from sparse-view images in around 0. 1s.
FeatUp: A Model-Agnostic Framework for Features at Any Resolution
Deep features are a cornerstone of computer vision research, capturing image semantics and enabling the community to solve downstream tasks even in the zero- or few-shot regime.

T-Rex2: Towards Generic Object Detection via Text-Visual Prompt Synergy
idea-research/t-rex • 21 Mar 2024
Recognizing the complementary strengths and weaknesses of both text and visual prompts, we introduce T-Rex2 that synergizes both prompts within a single model through contrastive learning.

Aggregated Contextual Transformations for High-Resolution Image Inpainting
For improving texture synthesis, we enhance the discriminator of AOT-GAN by training it with a tailored mask-prediction task.

StreamMultiDiffusion: Real-Time Interactive Generation with Region-Based Semantic Control
The enormous success of diffusion models in text-to-image synthesis has made them promising candidates for the next generation of end-user applications for image generation and editing.

- Search Menu
- Editor's Choice
- Author Guidelines
- Submission Site
- Open Access
- About Journal of Cybersecurity
- Editorial Board
- Advertising and Corporate Services
- Journals Career Network
- Self-Archiving Policy
- Journals on Oxford Academic
- Books on Oxford Academic

Editors-in-Chief
Tyler Moore
About the journal
Journal of Cybersecurity publishes accessible articles describing original research in the inherently interdisciplinary world of computer, systems, and information security …
Latest articles
Call for Papers
Journal of Cybersecurity is soliciting papers for a special collection on the philosophy of information security. This collection will explore research at the intersection of philosophy, information security, and philosophy of science.
Find out more

High-Impact Research Collection
Explore a collection of freely available high-impact research from 2020 and 2021 published in the Journal of Cybersecurity .
Browse the collection here

Submit your paper
Join the conversation moving the science of security forward. Visit our Instructions to Authors for more information about how to submit your manuscript.

Read and Publish deals
Authors interested in publishing in Journal of Cybersecurity may be able to publish their paper Open Access using funds available through their institution’s agreement with OUP.
Find out if your institution is participating
Related Titles

Affiliations
- Online ISSN 2057-2093
- Print ISSN 2057-2085
- Copyright © 2024 Oxford University Press
- About Oxford Academic
- Publish journals with us
- University press partners
- What we publish
- New features
- Open access
- Institutional account management
- Rights and permissions
- Get help with access
- Accessibility
- Advertising
- Media enquiries
- Oxford University Press
- Oxford Languages
- University of Oxford
Oxford University Press is a department of the University of Oxford. It furthers the University's objective of excellence in research, scholarship, and education by publishing worldwide
- Copyright © 2024 Oxford University Press
- Cookie settings
- Cookie policy
- Privacy policy
- Legal notice
This Feature Is Available To Subscribers Only
Sign In or Create an Account
This PDF is available to Subscribers Only
For full access to this pdf, sign in to an existing account, or purchase an annual subscription.
Advertisement
Machine Learning: Algorithms, Real-World Applications and Research Directions
- Review Article
- Published: 22 March 2021
- Volume 2 , article number 160 , ( 2021 )
Cite this article
- Iqbal H. Sarker ORCID: orcid.org/0000-0003-1740-5517 1 , 2
455k Accesses
1276 Citations
21 Altmetric
Explore all metrics
In the current age of the Fourth Industrial Revolution (4 IR or Industry 4.0), the digital world has a wealth of data, such as Internet of Things (IoT) data, cybersecurity data, mobile data, business data, social media data, health data, etc. To intelligently analyze these data and develop the corresponding smart and automated applications, the knowledge of artificial intelligence (AI), particularly, machine learning (ML) is the key. Various types of machine learning algorithms such as supervised, unsupervised, semi-supervised, and reinforcement learning exist in the area. Besides, the deep learning , which is part of a broader family of machine learning methods, can intelligently analyze the data on a large scale. In this paper, we present a comprehensive view on these machine learning algorithms that can be applied to enhance the intelligence and the capabilities of an application. Thus, this study’s key contribution is explaining the principles of different machine learning techniques and their applicability in various real-world application domains, such as cybersecurity systems, smart cities, healthcare, e-commerce, agriculture, and many more. We also highlight the challenges and potential research directions based on our study. Overall, this paper aims to serve as a reference point for both academia and industry professionals as well as for decision-makers in various real-world situations and application areas, particularly from the technical point of view.
Similar content being viewed by others
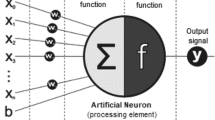
Deep Learning: A Comprehensive Overview on Techniques, Taxonomy, Applications and Research Directions
Iqbal H. Sarker
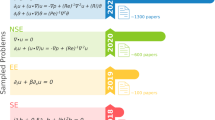
Scientific Machine Learning Through Physics–Informed Neural Networks: Where we are and What’s Next
Salvatore Cuomo, Vincenzo Schiano Di Cola, … Francesco Piccialli
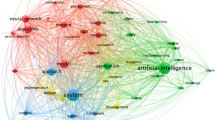
Quo vadis artificial intelligence?
Yuchen Jiang, Xiang Li, … Okyay Kaynak
Avoid common mistakes on your manuscript.
Introduction
We live in the age of data, where everything around us is connected to a data source, and everything in our lives is digitally recorded [ 21 , 103 ]. For instance, the current electronic world has a wealth of various kinds of data, such as the Internet of Things (IoT) data, cybersecurity data, smart city data, business data, smartphone data, social media data, health data, COVID-19 data, and many more. The data can be structured, semi-structured, or unstructured, discussed briefly in Sect. “ Types of Real-World Data and Machine Learning Techniques ”, which is increasing day-by-day. Extracting insights from these data can be used to build various intelligent applications in the relevant domains. For instance, to build a data-driven automated and intelligent cybersecurity system, the relevant cybersecurity data can be used [ 105 ]; to build personalized context-aware smart mobile applications, the relevant mobile data can be used [ 103 ], and so on. Thus, the data management tools and techniques having the capability of extracting insights or useful knowledge from the data in a timely and intelligent way is urgently needed, on which the real-world applications are based.
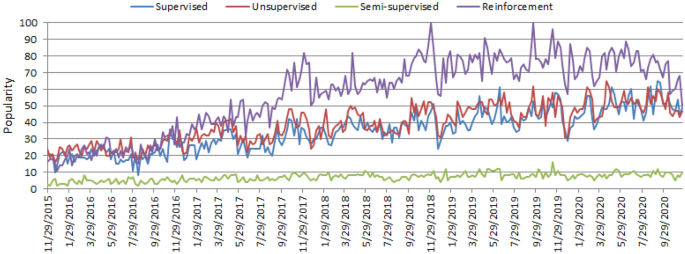
The worldwide popularity score of various types of ML algorithms (supervised, unsupervised, semi-supervised, and reinforcement) in a range of 0 (min) to 100 (max) over time where x-axis represents the timestamp information and y-axis represents the corresponding score
Artificial intelligence (AI), particularly, machine learning (ML) have grown rapidly in recent years in the context of data analysis and computing that typically allows the applications to function in an intelligent manner [ 95 ]. ML usually provides systems with the ability to learn and enhance from experience automatically without being specifically programmed and is generally referred to as the most popular latest technologies in the fourth industrial revolution (4 IR or Industry 4.0) [ 103 , 105 ]. “Industry 4.0” [ 114 ] is typically the ongoing automation of conventional manufacturing and industrial practices, including exploratory data processing, using new smart technologies such as machine learning automation. Thus, to intelligently analyze these data and to develop the corresponding real-world applications, machine learning algorithms is the key. The learning algorithms can be categorized into four major types, such as supervised, unsupervised, semi-supervised, and reinforcement learning in the area [ 75 ], discussed briefly in Sect. “ Types of Real-World Data and Machine Learning Techniques ”. The popularity of these approaches to learning is increasing day-by-day, which is shown in Fig. 1 , based on data collected from Google Trends [ 4 ] over the last five years. The x - axis of the figure indicates the specific dates and the corresponding popularity score within the range of \(0 \; (minimum)\) to \(100 \; (maximum)\) has been shown in y - axis . According to Fig. 1 , the popularity indication values for these learning types are low in 2015 and are increasing day by day. These statistics motivate us to study on machine learning in this paper, which can play an important role in the real-world through Industry 4.0 automation.
In general, the effectiveness and the efficiency of a machine learning solution depend on the nature and characteristics of data and the performance of the learning algorithms . In the area of machine learning algorithms, classification analysis, regression, data clustering, feature engineering and dimensionality reduction, association rule learning, or reinforcement learning techniques exist to effectively build data-driven systems [ 41 , 125 ]. Besides, deep learning originated from the artificial neural network that can be used to intelligently analyze data, which is known as part of a wider family of machine learning approaches [ 96 ]. Thus, selecting a proper learning algorithm that is suitable for the target application in a particular domain is challenging. The reason is that the purpose of different learning algorithms is different, even the outcome of different learning algorithms in a similar category may vary depending on the data characteristics [ 106 ]. Thus, it is important to understand the principles of various machine learning algorithms and their applicability to apply in various real-world application areas, such as IoT systems, cybersecurity services, business and recommendation systems, smart cities, healthcare and COVID-19, context-aware systems, sustainable agriculture, and many more that are explained briefly in Sect. “ Applications of Machine Learning ”.
Based on the importance and potentiality of “Machine Learning” to analyze the data mentioned above, in this paper, we provide a comprehensive view on various types of machine learning algorithms that can be applied to enhance the intelligence and the capabilities of an application. Thus, the key contribution of this study is explaining the principles and potentiality of different machine learning techniques, and their applicability in various real-world application areas mentioned earlier. The purpose of this paper is, therefore, to provide a basic guide for those academia and industry people who want to study, research, and develop data-driven automated and intelligent systems in the relevant areas based on machine learning techniques.
The key contributions of this paper are listed as follows:
To define the scope of our study by taking into account the nature and characteristics of various types of real-world data and the capabilities of various learning techniques.
To provide a comprehensive view on machine learning algorithms that can be applied to enhance the intelligence and capabilities of a data-driven application.
To discuss the applicability of machine learning-based solutions in various real-world application domains.
To highlight and summarize the potential research directions within the scope of our study for intelligent data analysis and services.
The rest of the paper is organized as follows. The next section presents the types of data and machine learning algorithms in a broader sense and defines the scope of our study. We briefly discuss and explain different machine learning algorithms in the subsequent section followed by which various real-world application areas based on machine learning algorithms are discussed and summarized. In the penultimate section, we highlight several research issues and potential future directions, and the final section concludes this paper.
Types of Real-World Data and Machine Learning Techniques
Machine learning algorithms typically consume and process data to learn the related patterns about individuals, business processes, transactions, events, and so on. In the following, we discuss various types of real-world data as well as categories of machine learning algorithms.
Types of Real-World Data
Usually, the availability of data is considered as the key to construct a machine learning model or data-driven real-world systems [ 103 , 105 ]. Data can be of various forms, such as structured, semi-structured, or unstructured [ 41 , 72 ]. Besides, the “metadata” is another type that typically represents data about the data. In the following, we briefly discuss these types of data.
Structured: It has a well-defined structure, conforms to a data model following a standard order, which is highly organized and easily accessed, and used by an entity or a computer program. In well-defined schemes, such as relational databases, structured data are typically stored, i.e., in a tabular format. For instance, names, dates, addresses, credit card numbers, stock information, geolocation, etc. are examples of structured data.
Unstructured: On the other hand, there is no pre-defined format or organization for unstructured data, making it much more difficult to capture, process, and analyze, mostly containing text and multimedia material. For example, sensor data, emails, blog entries, wikis, and word processing documents, PDF files, audio files, videos, images, presentations, web pages, and many other types of business documents can be considered as unstructured data.
Semi-structured: Semi-structured data are not stored in a relational database like the structured data mentioned above, but it does have certain organizational properties that make it easier to analyze. HTML, XML, JSON documents, NoSQL databases, etc., are some examples of semi-structured data.
Metadata: It is not the normal form of data, but “data about data”. The primary difference between “data” and “metadata” is that data are simply the material that can classify, measure, or even document something relative to an organization’s data properties. On the other hand, metadata describes the relevant data information, giving it more significance for data users. A basic example of a document’s metadata might be the author, file size, date generated by the document, keywords to define the document, etc.
In the area of machine learning and data science, researchers use various widely used datasets for different purposes. These are, for example, cybersecurity datasets such as NSL-KDD [ 119 ], UNSW-NB15 [ 76 ], ISCX’12 [ 1 ], CIC-DDoS2019 [ 2 ], Bot-IoT [ 59 ], etc., smartphone datasets such as phone call logs [ 84 , 101 ], SMS Log [ 29 ], mobile application usages logs [ 137 ] [ 117 ], mobile phone notification logs [ 73 ] etc., IoT data [ 16 , 57 , 62 ], agriculture and e-commerce data [ 120 , 138 ], health data such as heart disease [ 92 ], diabetes mellitus [ 83 , 134 ], COVID-19 [ 43 , 74 ], etc., and many more in various application domains. The data can be in different types discussed above, which may vary from application to application in the real world. To analyze such data in a particular problem domain, and to extract the insights or useful knowledge from the data for building the real-world intelligent applications, different types of machine learning techniques can be used according to their learning capabilities, which is discussed in the following.
Types of Machine Learning Techniques
Machine Learning algorithms are mainly divided into four categories: Supervised learning, Unsupervised learning, Semi-supervised learning, and Reinforcement learning [ 75 ], as shown in Fig. 2 . In the following, we briefly discuss each type of learning technique with the scope of their applicability to solve real-world problems.
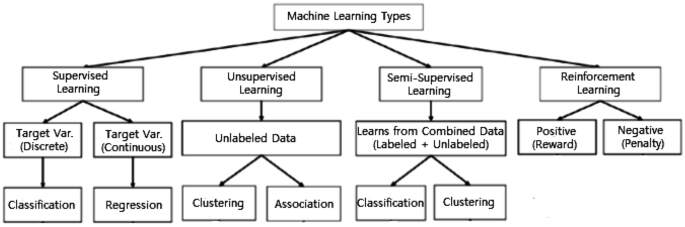
Various types of machine learning techniques
Supervised: Supervised learning is typically the task of machine learning to learn a function that maps an input to an output based on sample input-output pairs [ 41 ]. It uses labeled training data and a collection of training examples to infer a function. Supervised learning is carried out when certain goals are identified to be accomplished from a certain set of inputs [ 105 ], i.e., a task-driven approach . The most common supervised tasks are “classification” that separates the data, and “regression” that fits the data. For instance, predicting the class label or sentiment of a piece of text, like a tweet or a product review, i.e., text classification, is an example of supervised learning.
Unsupervised: Unsupervised learning analyzes unlabeled datasets without the need for human interference, i.e., a data-driven process [ 41 ]. This is widely used for extracting generative features, identifying meaningful trends and structures, groupings in results, and exploratory purposes. The most common unsupervised learning tasks are clustering, density estimation, feature learning, dimensionality reduction, finding association rules, anomaly detection, etc.
Semi-supervised: Semi-supervised learning can be defined as a hybridization of the above-mentioned supervised and unsupervised methods, as it operates on both labeled and unlabeled data [ 41 , 105 ]. Thus, it falls between learning “without supervision” and learning “with supervision”. In the real world, labeled data could be rare in several contexts, and unlabeled data are numerous, where semi-supervised learning is useful [ 75 ]. The ultimate goal of a semi-supervised learning model is to provide a better outcome for prediction than that produced using the labeled data alone from the model. Some application areas where semi-supervised learning is used include machine translation, fraud detection, labeling data and text classification.
Reinforcement: Reinforcement learning is a type of machine learning algorithm that enables software agents and machines to automatically evaluate the optimal behavior in a particular context or environment to improve its efficiency [ 52 ], i.e., an environment-driven approach . This type of learning is based on reward or penalty, and its ultimate goal is to use insights obtained from environmental activists to take action to increase the reward or minimize the risk [ 75 ]. It is a powerful tool for training AI models that can help increase automation or optimize the operational efficiency of sophisticated systems such as robotics, autonomous driving tasks, manufacturing and supply chain logistics, however, not preferable to use it for solving the basic or straightforward problems.
Thus, to build effective models in various application areas different types of machine learning techniques can play a significant role according to their learning capabilities, depending on the nature of the data discussed earlier, and the target outcome. In Table 1 , we summarize various types of machine learning techniques with examples. In the following, we provide a comprehensive view of machine learning algorithms that can be applied to enhance the intelligence and capabilities of a data-driven application.
Machine Learning Tasks and Algorithms
In this section, we discuss various machine learning algorithms that include classification analysis, regression analysis, data clustering, association rule learning, feature engineering for dimensionality reduction, as well as deep learning methods. A general structure of a machine learning-based predictive model has been shown in Fig. 3 , where the model is trained from historical data in phase 1 and the outcome is generated in phase 2 for the new test data.
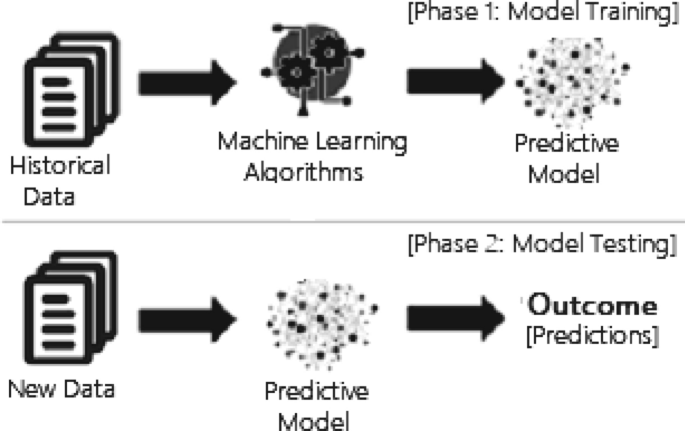
A general structure of a machine learning based predictive model considering both the training and testing phase
Classification Analysis
Classification is regarded as a supervised learning method in machine learning, referring to a problem of predictive modeling as well, where a class label is predicted for a given example [ 41 ]. Mathematically, it maps a function ( f ) from input variables ( X ) to output variables ( Y ) as target, label or categories. To predict the class of given data points, it can be carried out on structured or unstructured data. For example, spam detection such as “spam” and “not spam” in email service providers can be a classification problem. In the following, we summarize the common classification problems.
Binary classification: It refers to the classification tasks having two class labels such as “true and false” or “yes and no” [ 41 ]. In such binary classification tasks, one class could be the normal state, while the abnormal state could be another class. For instance, “cancer not detected” is the normal state of a task that involves a medical test, and “cancer detected” could be considered as the abnormal state. Similarly, “spam” and “not spam” in the above example of email service providers are considered as binary classification.
Multiclass classification: Traditionally, this refers to those classification tasks having more than two class labels [ 41 ]. The multiclass classification does not have the principle of normal and abnormal outcomes, unlike binary classification tasks. Instead, within a range of specified classes, examples are classified as belonging to one. For example, it can be a multiclass classification task to classify various types of network attacks in the NSL-KDD [ 119 ] dataset, where the attack categories are classified into four class labels, such as DoS (Denial of Service Attack), U2R (User to Root Attack), R2L (Root to Local Attack), and Probing Attack.
Multi-label classification: In machine learning, multi-label classification is an important consideration where an example is associated with several classes or labels. Thus, it is a generalization of multiclass classification, where the classes involved in the problem are hierarchically structured, and each example may simultaneously belong to more than one class in each hierarchical level, e.g., multi-level text classification. For instance, Google news can be presented under the categories of a “city name”, “technology”, or “latest news”, etc. Multi-label classification includes advanced machine learning algorithms that support predicting various mutually non-exclusive classes or labels, unlike traditional classification tasks where class labels are mutually exclusive [ 82 ].
Many classification algorithms have been proposed in the machine learning and data science literature [ 41 , 125 ]. In the following, we summarize the most common and popular methods that are used widely in various application areas.
Naive Bayes (NB): The naive Bayes algorithm is based on the Bayes’ theorem with the assumption of independence between each pair of features [ 51 ]. It works well and can be used for both binary and multi-class categories in many real-world situations, such as document or text classification, spam filtering, etc. To effectively classify the noisy instances in the data and to construct a robust prediction model, the NB classifier can be used [ 94 ]. The key benefit is that, compared to more sophisticated approaches, it needs a small amount of training data to estimate the necessary parameters and quickly [ 82 ]. However, its performance may affect due to its strong assumptions on features independence. Gaussian, Multinomial, Complement, Bernoulli, and Categorical are the common variants of NB classifier [ 82 ].
Linear Discriminant Analysis (LDA): Linear Discriminant Analysis (LDA) is a linear decision boundary classifier created by fitting class conditional densities to data and applying Bayes’ rule [ 51 , 82 ]. This method is also known as a generalization of Fisher’s linear discriminant, which projects a given dataset into a lower-dimensional space, i.e., a reduction of dimensionality that minimizes the complexity of the model or reduces the resulting model’s computational costs. The standard LDA model usually suits each class with a Gaussian density, assuming that all classes share the same covariance matrix [ 82 ]. LDA is closely related to ANOVA (analysis of variance) and regression analysis, which seek to express one dependent variable as a linear combination of other features or measurements.
Logistic regression (LR): Another common probabilistic based statistical model used to solve classification issues in machine learning is Logistic Regression (LR) [ 64 ]. Logistic regression typically uses a logistic function to estimate the probabilities, which is also referred to as the mathematically defined sigmoid function in Eq. 1 . It can overfit high-dimensional datasets and works well when the dataset can be separated linearly. The regularization (L1 and L2) techniques [ 82 ] can be used to avoid over-fitting in such scenarios. The assumption of linearity between the dependent and independent variables is considered as a major drawback of Logistic Regression. It can be used for both classification and regression problems, but it is more commonly used for classification.
K-nearest neighbors (KNN): K-Nearest Neighbors (KNN) [ 9 ] is an “instance-based learning” or non-generalizing learning, also known as a “lazy learning” algorithm. It does not focus on constructing a general internal model; instead, it stores all instances corresponding to training data in n -dimensional space. KNN uses data and classifies new data points based on similarity measures (e.g., Euclidean distance function) [ 82 ]. Classification is computed from a simple majority vote of the k nearest neighbors of each point. It is quite robust to noisy training data, and accuracy depends on the data quality. The biggest issue with KNN is to choose the optimal number of neighbors to be considered. KNN can be used both for classification as well as regression.
Support vector machine (SVM): In machine learning, another common technique that can be used for classification, regression, or other tasks is a support vector machine (SVM) [ 56 ]. In high- or infinite-dimensional space, a support vector machine constructs a hyper-plane or set of hyper-planes. Intuitively, the hyper-plane, which has the greatest distance from the nearest training data points in any class, achieves a strong separation since, in general, the greater the margin, the lower the classifier’s generalization error. It is effective in high-dimensional spaces and can behave differently based on different mathematical functions known as the kernel. Linear, polynomial, radial basis function (RBF), sigmoid, etc., are the popular kernel functions used in SVM classifier [ 82 ]. However, when the data set contains more noise, such as overlapping target classes, SVM does not perform well.
Decision tree (DT): Decision tree (DT) [ 88 ] is a well-known non-parametric supervised learning method. DT learning methods are used for both the classification and regression tasks [ 82 ]. ID3 [ 87 ], C4.5 [ 88 ], and CART [ 20 ] are well known for DT algorithms. Moreover, recently proposed BehavDT [ 100 ], and IntrudTree [ 97 ] by Sarker et al. are effective in the relevant application domains, such as user behavior analytics and cybersecurity analytics, respectively. By sorting down the tree from the root to some leaf nodes, as shown in Fig. 4 , DT classifies the instances. Instances are classified by checking the attribute defined by that node, starting at the root node of the tree, and then moving down the tree branch corresponding to the attribute value. For splitting, the most popular criteria are “gini” for the Gini impurity and “entropy” for the information gain that can be expressed mathematically as [ 82 ].
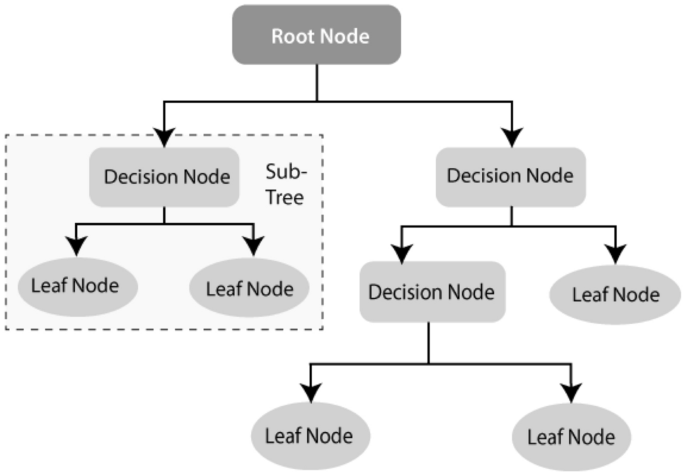
An example of a decision tree structure
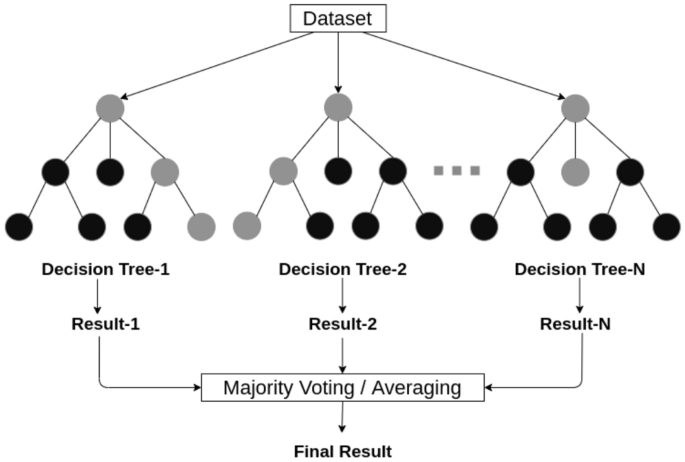
An example of a random forest structure considering multiple decision trees
Random forest (RF): A random forest classifier [ 19 ] is well known as an ensemble classification technique that is used in the field of machine learning and data science in various application areas. This method uses “parallel ensembling” which fits several decision tree classifiers in parallel, as shown in Fig. 5 , on different data set sub-samples and uses majority voting or averages for the outcome or final result. It thus minimizes the over-fitting problem and increases the prediction accuracy and control [ 82 ]. Therefore, the RF learning model with multiple decision trees is typically more accurate than a single decision tree based model [ 106 ]. To build a series of decision trees with controlled variation, it combines bootstrap aggregation (bagging) [ 18 ] and random feature selection [ 11 ]. It is adaptable to both classification and regression problems and fits well for both categorical and continuous values.
Adaptive Boosting (AdaBoost): Adaptive Boosting (AdaBoost) is an ensemble learning process that employs an iterative approach to improve poor classifiers by learning from their errors. This is developed by Yoav Freund et al. [ 35 ] and also known as “meta-learning”. Unlike the random forest that uses parallel ensembling, Adaboost uses “sequential ensembling”. It creates a powerful classifier by combining many poorly performing classifiers to obtain a good classifier of high accuracy. In that sense, AdaBoost is called an adaptive classifier by significantly improving the efficiency of the classifier, but in some instances, it can trigger overfits. AdaBoost is best used to boost the performance of decision trees, base estimator [ 82 ], on binary classification problems, however, is sensitive to noisy data and outliers.
Extreme gradient boosting (XGBoost): Gradient Boosting, like Random Forests [ 19 ] above, is an ensemble learning algorithm that generates a final model based on a series of individual models, typically decision trees. The gradient is used to minimize the loss function, similar to how neural networks [ 41 ] use gradient descent to optimize weights. Extreme Gradient Boosting (XGBoost) is a form of gradient boosting that takes more detailed approximations into account when determining the best model [ 82 ]. It computes second-order gradients of the loss function to minimize loss and advanced regularization (L1 and L2) [ 82 ], which reduces over-fitting, and improves model generalization and performance. XGBoost is fast to interpret and can handle large-sized datasets well.
Stochastic gradient descent (SGD): Stochastic gradient descent (SGD) [ 41 ] is an iterative method for optimizing an objective function with appropriate smoothness properties, where the word ‘stochastic’ refers to random probability. This reduces the computational burden, particularly in high-dimensional optimization problems, allowing for faster iterations in exchange for a lower convergence rate. A gradient is the slope of a function that calculates a variable’s degree of change in response to another variable’s changes. Mathematically, the Gradient Descent is a convex function whose output is a partial derivative of a set of its input parameters. Let, \(\alpha\) is the learning rate, and \(J_i\) is the training example cost of \(i \mathrm{th}\) , then Eq. ( 4 ) represents the stochastic gradient descent weight update method at the \(j^\mathrm{th}\) iteration. In large-scale and sparse machine learning, SGD has been successfully applied to problems often encountered in text classification and natural language processing [ 82 ]. However, SGD is sensitive to feature scaling and needs a range of hyperparameters, such as the regularization parameter and the number of iterations.
Rule-based classification : The term rule-based classification can be used to refer to any classification scheme that makes use of IF-THEN rules for class prediction. Several classification algorithms such as Zero-R [ 125 ], One-R [ 47 ], decision trees [ 87 , 88 ], DTNB [ 110 ], Ripple Down Rule learner (RIDOR) [ 125 ], Repeated Incremental Pruning to Produce Error Reduction (RIPPER) [ 126 ] exist with the ability of rule generation. The decision tree is one of the most common rule-based classification algorithms among these techniques because it has several advantages, such as being easier to interpret; the ability to handle high-dimensional data; simplicity and speed; good accuracy; and the capability to produce rules for human clear and understandable classification [ 127 ] [ 128 ]. The decision tree-based rules also provide significant accuracy in a prediction model for unseen test cases [ 106 ]. Since the rules are easily interpretable, these rule-based classifiers are often used to produce descriptive models that can describe a system including the entities and their relationships.
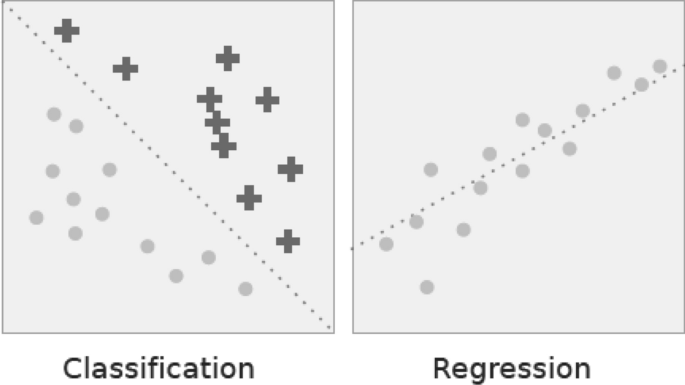
Classification vs. regression. In classification the dotted line represents a linear boundary that separates the two classes; in regression, the dotted line models the linear relationship between the two variables
Regression Analysis
Regression analysis includes several methods of machine learning that allow to predict a continuous ( y ) result variable based on the value of one or more ( x ) predictor variables [ 41 ]. The most significant distinction between classification and regression is that classification predicts distinct class labels, while regression facilitates the prediction of a continuous quantity. Figure 6 shows an example of how classification is different with regression models. Some overlaps are often found between the two types of machine learning algorithms. Regression models are now widely used in a variety of fields, including financial forecasting or prediction, cost estimation, trend analysis, marketing, time series estimation, drug response modeling, and many more. Some of the familiar types of regression algorithms are linear, polynomial, lasso and ridge regression, etc., which are explained briefly in the following.
Simple and multiple linear regression: This is one of the most popular ML modeling techniques as well as a well-known regression technique. In this technique, the dependent variable is continuous, the independent variable(s) can be continuous or discrete, and the form of the regression line is linear. Linear regression creates a relationship between the dependent variable ( Y ) and one or more independent variables ( X ) (also known as regression line) using the best fit straight line [ 41 ]. It is defined by the following equations:
where a is the intercept, b is the slope of the line, and e is the error term. This equation can be used to predict the value of the target variable based on the given predictor variable(s). Multiple linear regression is an extension of simple linear regression that allows two or more predictor variables to model a response variable, y, as a linear function [ 41 ] defined in Eq. 6 , whereas simple linear regression has only 1 independent variable, defined in Eq. 5 .
Polynomial regression: Polynomial regression is a form of regression analysis in which the relationship between the independent variable x and the dependent variable y is not linear, but is the polynomial degree of \(n^\mathrm{th}\) in x [ 82 ]. The equation for polynomial regression is also derived from linear regression (polynomial regression of degree 1) equation, which is defined as below:
Here, y is the predicted/target output, \(b_0, b_1,... b_n\) are the regression coefficients, x is an independent/ input variable. In simple words, we can say that if data are not distributed linearly, instead it is \(n^\mathrm{th}\) degree of polynomial then we use polynomial regression to get desired output.
LASSO and ridge regression: LASSO and Ridge regression are well known as powerful techniques which are typically used for building learning models in presence of a large number of features, due to their capability to preventing over-fitting and reducing the complexity of the model. The LASSO (least absolute shrinkage and selection operator) regression model uses L 1 regularization technique [ 82 ] that uses shrinkage, which penalizes “absolute value of magnitude of coefficients” ( L 1 penalty). As a result, LASSO appears to render coefficients to absolute zero. Thus, LASSO regression aims to find the subset of predictors that minimizes the prediction error for a quantitative response variable. On the other hand, ridge regression uses L 2 regularization [ 82 ], which is the “squared magnitude of coefficients” ( L 2 penalty). Thus, ridge regression forces the weights to be small but never sets the coefficient value to zero, and does a non-sparse solution. Overall, LASSO regression is useful to obtain a subset of predictors by eliminating less important features, and ridge regression is useful when a data set has “multicollinearity” which refers to the predictors that are correlated with other predictors.
Cluster Analysis
Cluster analysis, also known as clustering, is an unsupervised machine learning technique for identifying and grouping related data points in large datasets without concern for the specific outcome. It does grouping a collection of objects in such a way that objects in the same category, called a cluster, are in some sense more similar to each other than objects in other groups [ 41 ]. It is often used as a data analysis technique to discover interesting trends or patterns in data, e.g., groups of consumers based on their behavior. In a broad range of application areas, such as cybersecurity, e-commerce, mobile data processing, health analytics, user modeling and behavioral analytics, clustering can be used. In the following, we briefly discuss and summarize various types of clustering methods.
Partitioning methods: Based on the features and similarities in the data, this clustering approach categorizes the data into multiple groups or clusters. The data scientists or analysts typically determine the number of clusters either dynamically or statically depending on the nature of the target applications, to produce for the methods of clustering. The most common clustering algorithms based on partitioning methods are K-means [ 69 ], K-Mediods [ 80 ], CLARA [ 55 ] etc.
Density-based methods: To identify distinct groups or clusters, it uses the concept that a cluster in the data space is a contiguous region of high point density isolated from other such clusters by contiguous regions of low point density. Points that are not part of a cluster are considered as noise. The typical clustering algorithms based on density are DBSCAN [ 32 ], OPTICS [ 12 ] etc. The density-based methods typically struggle with clusters of similar density and high dimensionality data.
Hierarchical-based methods: Hierarchical clustering typically seeks to construct a hierarchy of clusters, i.e., the tree structure. Strategies for hierarchical clustering generally fall into two types: (i) Agglomerative—a “bottom-up” approach in which each observation begins in its cluster and pairs of clusters are combined as one, moves up the hierarchy, and (ii) Divisive—a “top-down” approach in which all observations begin in one cluster and splits are performed recursively, moves down the hierarchy, as shown in Fig 7 . Our earlier proposed BOTS technique, Sarker et al. [ 102 ] is an example of a hierarchical, particularly, bottom-up clustering algorithm.
Grid-based methods: To deal with massive datasets, grid-based clustering is especially suitable. To obtain clusters, the principle is first to summarize the dataset with a grid representation and then to combine grid cells. STING [ 122 ], CLIQUE [ 6 ], etc. are the standard algorithms of grid-based clustering.
Model-based methods: There are mainly two types of model-based clustering algorithms: one that uses statistical learning, and the other based on a method of neural network learning [ 130 ]. For instance, GMM [ 89 ] is an example of a statistical learning method, and SOM [ 22 ] [ 96 ] is an example of a neural network learning method.
Constraint-based methods: Constrained-based clustering is a semi-supervised approach to data clustering that uses constraints to incorporate domain knowledge. Application or user-oriented constraints are incorporated to perform the clustering. The typical algorithms of this kind of clustering are COP K-means [ 121 ], CMWK-Means [ 27 ], etc.
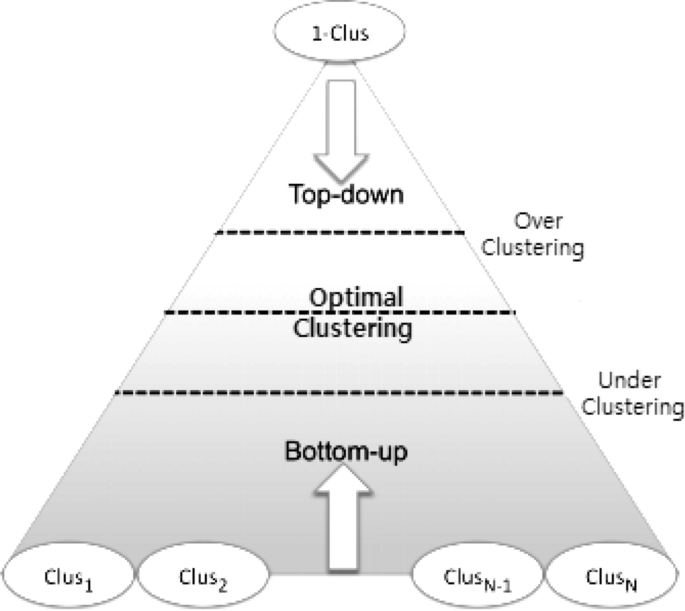
A graphical interpretation of the widely-used hierarchical clustering (Bottom-up and top-down) technique
Many clustering algorithms have been proposed with the ability to grouping data in machine learning and data science literature [ 41 , 125 ]. In the following, we summarize the popular methods that are used widely in various application areas.
K-means clustering: K-means clustering [ 69 ] is a fast, robust, and simple algorithm that provides reliable results when data sets are well-separated from each other. The data points are allocated to a cluster in this algorithm in such a way that the amount of the squared distance between the data points and the centroid is as small as possible. In other words, the K-means algorithm identifies the k number of centroids and then assigns each data point to the nearest cluster while keeping the centroids as small as possible. Since it begins with a random selection of cluster centers, the results can be inconsistent. Since extreme values can easily affect a mean, the K-means clustering algorithm is sensitive to outliers. K-medoids clustering [ 91 ] is a variant of K-means that is more robust to noises and outliers.
Mean-shift clustering: Mean-shift clustering [ 37 ] is a nonparametric clustering technique that does not require prior knowledge of the number of clusters or constraints on cluster shape. Mean-shift clustering aims to discover “blobs” in a smooth distribution or density of samples [ 82 ]. It is a centroid-based algorithm that works by updating centroid candidates to be the mean of the points in a given region. To form the final set of centroids, these candidates are filtered in a post-processing stage to remove near-duplicates. Cluster analysis in computer vision and image processing are examples of application domains. Mean Shift has the disadvantage of being computationally expensive. Moreover, in cases of high dimension, where the number of clusters shifts abruptly, the mean-shift algorithm does not work well.
DBSCAN: Density-based spatial clustering of applications with noise (DBSCAN) [ 32 ] is a base algorithm for density-based clustering which is widely used in data mining and machine learning. This is known as a non-parametric density-based clustering technique for separating high-density clusters from low-density clusters that are used in model building. DBSCAN’s main idea is that a point belongs to a cluster if it is close to many points from that cluster. It can find clusters of various shapes and sizes in a vast volume of data that is noisy and contains outliers. DBSCAN, unlike k-means, does not require a priori specification of the number of clusters in the data and can find arbitrarily shaped clusters. Although k-means is much faster than DBSCAN, it is efficient at finding high-density regions and outliers, i.e., is robust to outliers.
GMM clustering: Gaussian mixture models (GMMs) are often used for data clustering, which is a distribution-based clustering algorithm. A Gaussian mixture model is a probabilistic model in which all the data points are produced by a mixture of a finite number of Gaussian distributions with unknown parameters [ 82 ]. To find the Gaussian parameters for each cluster, an optimization algorithm called expectation-maximization (EM) [ 82 ] can be used. EM is an iterative method that uses a statistical model to estimate the parameters. In contrast to k-means, Gaussian mixture models account for uncertainty and return the likelihood that a data point belongs to one of the k clusters. GMM clustering is more robust than k-means and works well even with non-linear data distributions.
Agglomerative hierarchical clustering: The most common method of hierarchical clustering used to group objects in clusters based on their similarity is agglomerative clustering. This technique uses a bottom-up approach, where each object is first treated as a singleton cluster by the algorithm. Following that, pairs of clusters are merged one by one until all clusters have been merged into a single large cluster containing all objects. The result is a dendrogram, which is a tree-based representation of the elements. Single linkage [ 115 ], Complete linkage [ 116 ], BOTS [ 102 ] etc. are some examples of such techniques. The main advantage of agglomerative hierarchical clustering over k-means is that the tree-structure hierarchy generated by agglomerative clustering is more informative than the unstructured collection of flat clusters returned by k-means, which can help to make better decisions in the relevant application areas.
Dimensionality Reduction and Feature Learning
In machine learning and data science, high-dimensional data processing is a challenging task for both researchers and application developers. Thus, dimensionality reduction which is an unsupervised learning technique, is important because it leads to better human interpretations, lower computational costs, and avoids overfitting and redundancy by simplifying models. Both the process of feature selection and feature extraction can be used for dimensionality reduction. The primary distinction between the selection and extraction of features is that the “feature selection” keeps a subset of the original features [ 97 ], while “feature extraction” creates brand new ones [ 98 ]. In the following, we briefly discuss these techniques.
Feature selection: The selection of features, also known as the selection of variables or attributes in the data, is the process of choosing a subset of unique features (variables, predictors) to use in building machine learning and data science model. It decreases a model’s complexity by eliminating the irrelevant or less important features and allows for faster training of machine learning algorithms. A right and optimal subset of the selected features in a problem domain is capable to minimize the overfitting problem through simplifying and generalizing the model as well as increases the model’s accuracy [ 97 ]. Thus, “feature selection” [ 66 , 99 ] is considered as one of the primary concepts in machine learning that greatly affects the effectiveness and efficiency of the target machine learning model. Chi-squared test, Analysis of variance (ANOVA) test, Pearson’s correlation coefficient, recursive feature elimination, are some popular techniques that can be used for feature selection.
Feature extraction: In a machine learning-based model or system, feature extraction techniques usually provide a better understanding of the data, a way to improve prediction accuracy, and to reduce computational cost or training time. The aim of “feature extraction” [ 66 , 99 ] is to reduce the number of features in a dataset by generating new ones from the existing ones and then discarding the original features. The majority of the information found in the original set of features can then be summarized using this new reduced set of features. For instance, principal components analysis (PCA) is often used as a dimensionality-reduction technique to extract a lower-dimensional space creating new brand components from the existing features in a dataset [ 98 ].
Many algorithms have been proposed to reduce data dimensions in the machine learning and data science literature [ 41 , 125 ]. In the following, we summarize the popular methods that are used widely in various application areas.
Variance threshold: A simple basic approach to feature selection is the variance threshold [ 82 ]. This excludes all features of low variance, i.e., all features whose variance does not exceed the threshold. It eliminates all zero-variance characteristics by default, i.e., characteristics that have the same value in all samples. This feature selection algorithm looks only at the ( X ) features, not the ( y ) outputs needed, and can, therefore, be used for unsupervised learning.
Pearson correlation: Pearson’s correlation is another method to understand a feature’s relation to the response variable and can be used for feature selection [ 99 ]. This method is also used for finding the association between the features in a dataset. The resulting value is \([-1, 1]\) , where \(-1\) means perfect negative correlation, \(+1\) means perfect positive correlation, and 0 means that the two variables do not have a linear correlation. If two random variables represent X and Y , then the correlation coefficient between X and Y is defined as [ 41 ]
ANOVA: Analysis of variance (ANOVA) is a statistical tool used to verify the mean values of two or more groups that differ significantly from each other. ANOVA assumes a linear relationship between the variables and the target and the variables’ normal distribution. To statistically test the equality of means, the ANOVA method utilizes F tests. For feature selection, the results ‘ANOVA F value’ [ 82 ] of this test can be used where certain features independent of the goal variable can be omitted.
Chi square: The chi-square \({\chi }^2\) [ 82 ] statistic is an estimate of the difference between the effects of a series of events or variables observed and expected frequencies. The magnitude of the difference between the real and observed values, the degrees of freedom, and the sample size depends on \({\chi }^2\) . The chi-square \({\chi }^2\) is commonly used for testing relationships between categorical variables. If \(O_i\) represents observed value and \(E_i\) represents expected value, then
Recursive feature elimination (RFE): Recursive Feature Elimination (RFE) is a brute force approach to feature selection. RFE [ 82 ] fits the model and removes the weakest feature before it meets the specified number of features. Features are ranked by the coefficients or feature significance of the model. RFE aims to remove dependencies and collinearity in the model by recursively removing a small number of features per iteration.
Model-based selection: To reduce the dimensionality of the data, linear models penalized with the L 1 regularization can be used. Least absolute shrinkage and selection operator (Lasso) regression is a type of linear regression that has the property of shrinking some of the coefficients to zero [ 82 ]. Therefore, that feature can be removed from the model. Thus, the penalized lasso regression method, often used in machine learning to select the subset of variables. Extra Trees Classifier [ 82 ] is an example of a tree-based estimator that can be used to compute impurity-based function importance, which can then be used to discard irrelevant features.
Principal component analysis (PCA): Principal component analysis (PCA) is a well-known unsupervised learning approach in the field of machine learning and data science. PCA is a mathematical technique that transforms a set of correlated variables into a set of uncorrelated variables known as principal components [ 48 , 81 ]. Figure 8 shows an example of the effect of PCA on various dimensions space, where Fig. 8 a shows the original features in 3D space, and Fig. 8 b shows the created principal components PC1 and PC2 onto a 2D plane, and 1D line with the principal component PC1 respectively. Thus, PCA can be used as a feature extraction technique that reduces the dimensionality of the datasets, and to build an effective machine learning model [ 98 ]. Technically, PCA identifies the completely transformed with the highest eigenvalues of a covariance matrix and then uses those to project the data into a new subspace of equal or fewer dimensions [ 82 ].
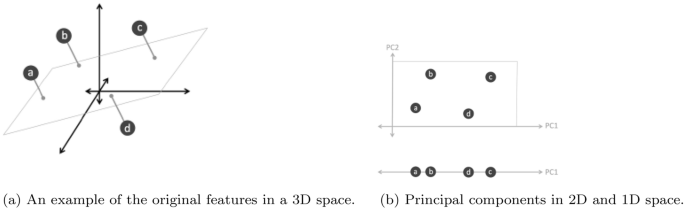
An example of a principal component analysis (PCA) and created principal components PC1 and PC2 in different dimension space
Association Rule Learning
Association rule learning is a rule-based machine learning approach to discover interesting relationships, “IF-THEN” statements, in large datasets between variables [ 7 ]. One example is that “if a customer buys a computer or laptop (an item), s/he is likely to also buy anti-virus software (another item) at the same time”. Association rules are employed today in many application areas, including IoT services, medical diagnosis, usage behavior analytics, web usage mining, smartphone applications, cybersecurity applications, and bioinformatics. In comparison to sequence mining, association rule learning does not usually take into account the order of things within or across transactions. A common way of measuring the usefulness of association rules is to use its parameter, the ‘support’ and ‘confidence’, which is introduced in [ 7 ].
In the data mining literature, many association rule learning methods have been proposed, such as logic dependent [ 34 ], frequent pattern based [ 8 , 49 , 68 ], and tree-based [ 42 ]. The most popular association rule learning algorithms are summarized below.
AIS and SETM: AIS is the first algorithm proposed by Agrawal et al. [ 7 ] for association rule mining. The AIS algorithm’s main downside is that too many candidate itemsets are generated, requiring more space and wasting a lot of effort. This algorithm calls for too many passes over the entire dataset to produce the rules. Another approach SETM [ 49 ] exhibits good performance and stable behavior with execution time; however, it suffers from the same flaw as the AIS algorithm.
Apriori: For generating association rules for a given dataset, Agrawal et al. [ 8 ] proposed the Apriori, Apriori-TID, and Apriori-Hybrid algorithms. These later algorithms outperform the AIS and SETM mentioned above due to the Apriori property of frequent itemset [ 8 ]. The term ‘Apriori’ usually refers to having prior knowledge of frequent itemset properties. Apriori uses a “bottom-up” approach, where it generates the candidate itemsets. To reduce the search space, Apriori uses the property “all subsets of a frequent itemset must be frequent; and if an itemset is infrequent, then all its supersets must also be infrequent”. Another approach predictive Apriori [ 108 ] can also generate rules; however, it receives unexpected results as it combines both the support and confidence. The Apriori [ 8 ] is the widely applicable techniques in mining association rules.
ECLAT: This technique was proposed by Zaki et al. [ 131 ] and stands for Equivalence Class Clustering and bottom-up Lattice Traversal. ECLAT uses a depth-first search to find frequent itemsets. In contrast to the Apriori [ 8 ] algorithm, which represents data in a horizontal pattern, it represents data vertically. Hence, the ECLAT algorithm is more efficient and scalable in the area of association rule learning. This algorithm is better suited for small and medium datasets whereas the Apriori algorithm is used for large datasets.
FP-Growth: Another common association rule learning technique based on the frequent-pattern tree (FP-tree) proposed by Han et al. [ 42 ] is Frequent Pattern Growth, known as FP-Growth. The key difference with Apriori is that while generating rules, the Apriori algorithm [ 8 ] generates frequent candidate itemsets; on the other hand, the FP-growth algorithm [ 42 ] prevents candidate generation and thus produces a tree by the successful strategy of ‘divide and conquer’ approach. Due to its sophistication, however, FP-Tree is challenging to use in an interactive mining environment [ 133 ]. Thus, the FP-Tree would not fit into memory for massive data sets, making it challenging to process big data as well. Another solution is RARM (Rapid Association Rule Mining) proposed by Das et al. [ 26 ] but faces a related FP-tree issue [ 133 ].
ABC-RuleMiner: A rule-based machine learning method, recently proposed in our earlier paper, by Sarker et al. [ 104 ], to discover the interesting non-redundant rules to provide real-world intelligent services. This algorithm effectively identifies the redundancy in associations by taking into account the impact or precedence of the related contextual features and discovers a set of non-redundant association rules. This algorithm first constructs an association generation tree (AGT), a top-down approach, and then extracts the association rules through traversing the tree. Thus, ABC-RuleMiner is more potent than traditional rule-based methods in terms of both non-redundant rule generation and intelligent decision-making, particularly in a context-aware smart computing environment, where human or user preferences are involved.
Among the association rule learning techniques discussed above, Apriori [ 8 ] is the most widely used algorithm for discovering association rules from a given dataset [ 133 ]. The main strength of the association learning technique is its comprehensiveness, as it generates all associations that satisfy the user-specified constraints, such as minimum support and confidence value. The ABC-RuleMiner approach [ 104 ] discussed earlier could give significant results in terms of non-redundant rule generation and intelligent decision-making for the relevant application areas in the real world.
Reinforcement Learning
Reinforcement learning (RL) is a machine learning technique that allows an agent to learn by trial and error in an interactive environment using input from its actions and experiences. Unlike supervised learning, which is based on given sample data or examples, the RL method is based on interacting with the environment. The problem to be solved in reinforcement learning (RL) is defined as a Markov Decision Process (MDP) [ 86 ], i.e., all about sequentially making decisions. An RL problem typically includes four elements such as Agent, Environment, Rewards, and Policy.
RL can be split roughly into Model-based and Model-free techniques. Model-based RL is the process of inferring optimal behavior from a model of the environment by performing actions and observing the results, which include the next state and the immediate reward [ 85 ]. AlphaZero, AlphaGo [ 113 ] are examples of the model-based approaches. On the other hand, a model-free approach does not use the distribution of the transition probability and the reward function associated with MDP. Q-learning, Deep Q Network, Monte Carlo Control, SARSA (State–Action–Reward–State–Action), etc. are some examples of model-free algorithms [ 52 ]. The policy network, which is required for model-based RL but not for model-free, is the key difference between model-free and model-based learning. In the following, we discuss the popular RL algorithms.
Monte Carlo methods: Monte Carlo techniques, or Monte Carlo experiments, are a wide category of computational algorithms that rely on repeated random sampling to obtain numerical results [ 52 ]. The underlying concept is to use randomness to solve problems that are deterministic in principle. Optimization, numerical integration, and making drawings from the probability distribution are the three problem classes where Monte Carlo techniques are most commonly used.
Q-learning: Q-learning is a model-free reinforcement learning algorithm for learning the quality of behaviors that tell an agent what action to take under what conditions [ 52 ]. It does not need a model of the environment (hence the term “model-free”), and it can deal with stochastic transitions and rewards without the need for adaptations. The ‘Q’ in Q-learning usually stands for quality, as the algorithm calculates the maximum expected rewards for a given behavior in a given state.
Deep Q-learning: The basic working step in Deep Q-Learning [ 52 ] is that the initial state is fed into the neural network, which returns the Q-value of all possible actions as an output. Still, when we have a reasonably simple setting to overcome, Q-learning works well. However, when the number of states and actions becomes more complicated, deep learning can be used as a function approximator.
Reinforcement learning, along with supervised and unsupervised learning, is one of the basic machine learning paradigms. RL can be used to solve numerous real-world problems in various fields, such as game theory, control theory, operations analysis, information theory, simulation-based optimization, manufacturing, supply chain logistics, multi-agent systems, swarm intelligence, aircraft control, robot motion control, and many more.
Artificial Neural Network and Deep Learning
Deep learning is part of a wider family of artificial neural networks (ANN)-based machine learning approaches with representation learning. Deep learning provides a computational architecture by combining several processing layers, such as input, hidden, and output layers, to learn from data [ 41 ]. The main advantage of deep learning over traditional machine learning methods is its better performance in several cases, particularly learning from large datasets [ 105 , 129 ]. Figure 9 shows a general performance of deep learning over machine learning considering the increasing amount of data. However, it may vary depending on the data characteristics and experimental set up.
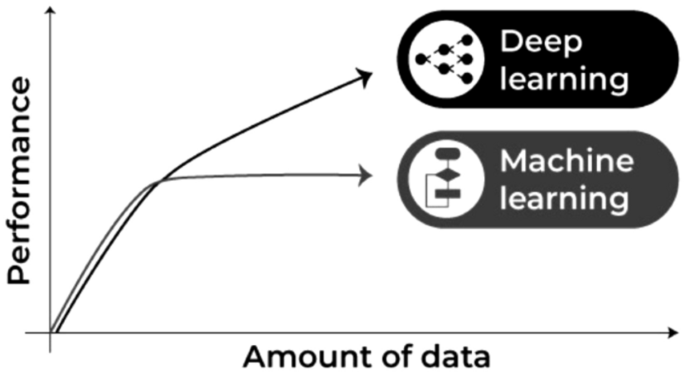
Machine learning and deep learning performance in general with the amount of data
The most common deep learning algorithms are: Multi-layer Perceptron (MLP), Convolutional Neural Network (CNN, or ConvNet), Long Short-Term Memory Recurrent Neural Network (LSTM-RNN) [ 96 ]. In the following, we discuss various types of deep learning methods that can be used to build effective data-driven models for various purposes.
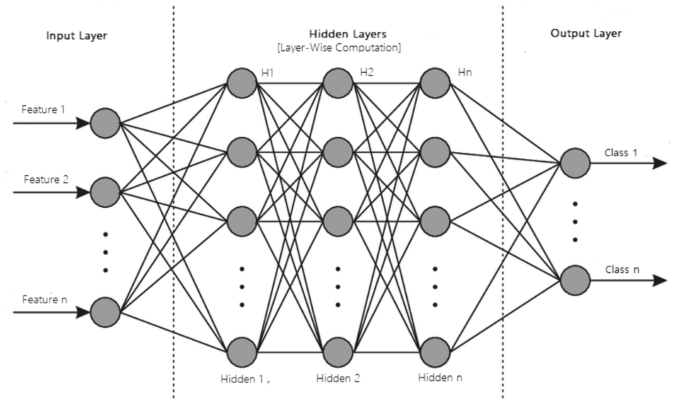
A structure of an artificial neural network modeling with multiple processing layers
MLP: The base architecture of deep learning, which is also known as the feed-forward artificial neural network, is called a multilayer perceptron (MLP) [ 82 ]. A typical MLP is a fully connected network consisting of an input layer, one or more hidden layers, and an output layer, as shown in Fig. 10 . Each node in one layer connects to each node in the following layer at a certain weight. MLP utilizes the “Backpropagation” technique [ 41 ], the most “fundamental building block” in a neural network, to adjust the weight values internally while building the model. MLP is sensitive to scaling features and allows a variety of hyperparameters to be tuned, such as the number of hidden layers, neurons, and iterations, which can result in a computationally costly model.
CNN or ConvNet: The convolution neural network (CNN) [ 65 ] enhances the design of the standard ANN, consisting of convolutional layers, pooling layers, as well as fully connected layers, as shown in Fig. 11 . As it takes the advantage of the two-dimensional (2D) structure of the input data, it is typically broadly used in several areas such as image and video recognition, image processing and classification, medical image analysis, natural language processing, etc. While CNN has a greater computational burden, without any manual intervention, it has the advantage of automatically detecting the important features, and hence CNN is considered to be more powerful than conventional ANN. A number of advanced deep learning models based on CNN can be used in the field, such as AlexNet [ 60 ], Xception [ 24 ], Inception [ 118 ], Visual Geometry Group (VGG) [ 44 ], ResNet [ 45 ], etc.
LSTM-RNN: Long short-term memory (LSTM) is an artificial recurrent neural network (RNN) architecture used in the area of deep learning [ 38 ]. LSTM has feedback links, unlike normal feed-forward neural networks. LSTM networks are well-suited for analyzing and learning sequential data, such as classifying, processing, and predicting data based on time series data, which differentiates it from other conventional networks. Thus, LSTM can be used when the data are in a sequential format, such as time, sentence, etc., and commonly applied in the area of time-series analysis, natural language processing, speech recognition, etc.
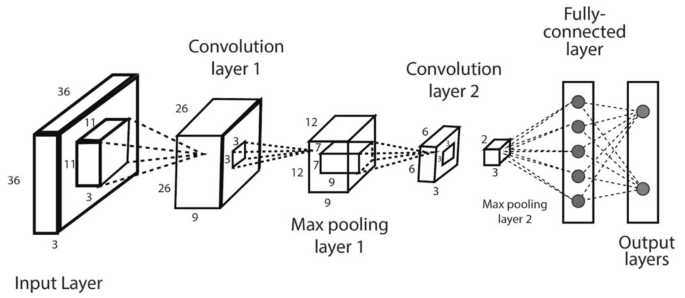
An example of a convolutional neural network (CNN or ConvNet) including multiple convolution and pooling layers
In addition to these most common deep learning methods discussed above, several other deep learning approaches [ 96 ] exist in the area for various purposes. For instance, the self-organizing map (SOM) [ 58 ] uses unsupervised learning to represent the high-dimensional data by a 2D grid map, thus achieving dimensionality reduction. The autoencoder (AE) [ 15 ] is another learning technique that is widely used for dimensionality reduction as well and feature extraction in unsupervised learning tasks. Restricted Boltzmann machines (RBM) [ 46 ] can be used for dimensionality reduction, classification, regression, collaborative filtering, feature learning, and topic modeling. A deep belief network (DBN) is typically composed of simple, unsupervised networks such as restricted Boltzmann machines (RBMs) or autoencoders, and a backpropagation neural network (BPNN) [ 123 ]. A generative adversarial network (GAN) [ 39 ] is a form of the network for deep learning that can generate data with characteristics close to the actual data input. Transfer learning is currently very common because it can train deep neural networks with comparatively low data, which is typically the re-use of a new problem with a pre-trained model [ 124 ]. A brief discussion of these artificial neural networks (ANN) and deep learning (DL) models are summarized in our earlier paper Sarker et al. [ 96 ].
Overall, based on the learning techniques discussed above, we can conclude that various types of machine learning techniques, such as classification analysis, regression, data clustering, feature selection and extraction, and dimensionality reduction, association rule learning, reinforcement learning, or deep learning techniques, can play a significant role for various purposes according to their capabilities. In the following section, we discuss several application areas based on machine learning algorithms.
Applications of Machine Learning
In the current age of the Fourth Industrial Revolution (4IR), machine learning becomes popular in various application areas, because of its learning capabilities from the past and making intelligent decisions. In the following, we summarize and discuss ten popular application areas of machine learning technology.
Predictive analytics and intelligent decision-making: A major application field of machine learning is intelligent decision-making by data-driven predictive analytics [ 21 , 70 ]. The basis of predictive analytics is capturing and exploiting relationships between explanatory variables and predicted variables from previous events to predict the unknown outcome [ 41 ]. For instance, identifying suspects or criminals after a crime has been committed, or detecting credit card fraud as it happens. Another application, where machine learning algorithms can assist retailers in better understanding consumer preferences and behavior, better manage inventory, avoiding out-of-stock situations, and optimizing logistics and warehousing in e-commerce. Various machine learning algorithms such as decision trees, support vector machines, artificial neural networks, etc. [ 106 , 125 ] are commonly used in the area. Since accurate predictions provide insight into the unknown, they can improve the decisions of industries, businesses, and almost any organization, including government agencies, e-commerce, telecommunications, banking and financial services, healthcare, sales and marketing, transportation, social networking, and many others.
Cybersecurity and threat intelligence: Cybersecurity is one of the most essential areas of Industry 4.0. [ 114 ], which is typically the practice of protecting networks, systems, hardware, and data from digital attacks [ 114 ]. Machine learning has become a crucial cybersecurity technology that constantly learns by analyzing data to identify patterns, better detect malware in encrypted traffic, find insider threats, predict where bad neighborhoods are online, keep people safe while browsing, or secure data in the cloud by uncovering suspicious activity. For instance, clustering techniques can be used to identify cyber-anomalies, policy violations, etc. To detect various types of cyber-attacks or intrusions machine learning classification models by taking into account the impact of security features are useful [ 97 ]. Various deep learning-based security models can also be used on the large scale of security datasets [ 96 , 129 ]. Moreover, security policy rules generated by association rule learning techniques can play a significant role to build a rule-based security system [ 105 ]. Thus, we can say that various learning techniques discussed in Sect. Machine Learning Tasks and Algorithms , can enable cybersecurity professionals to be more proactive inefficiently preventing threats and cyber-attacks.
Internet of things (IoT) and smart cities: Internet of Things (IoT) is another essential area of Industry 4.0. [ 114 ], which turns everyday objects into smart objects by allowing them to transmit data and automate tasks without the need for human interaction. IoT is, therefore, considered to be the big frontier that can enhance almost all activities in our lives, such as smart governance, smart home, education, communication, transportation, retail, agriculture, health care, business, and many more [ 70 ]. Smart city is one of IoT’s core fields of application, using technologies to enhance city services and residents’ living experiences [ 132 , 135 ]. As machine learning utilizes experience to recognize trends and create models that help predict future behavior and events, it has become a crucial technology for IoT applications [ 103 ]. For example, to predict traffic in smart cities, parking availability prediction, estimate the total usage of energy of the citizens for a particular period, make context-aware and timely decisions for the people, etc. are some tasks that can be solved using machine learning techniques according to the current needs of the people.
Traffic prediction and transportation: Transportation systems have become a crucial component of every country’s economic development. Nonetheless, several cities around the world are experiencing an excessive rise in traffic volume, resulting in serious issues such as delays, traffic congestion, higher fuel prices, increased CO \(_2\) pollution, accidents, emergencies, and a decline in modern society’s quality of life [ 40 ]. Thus, an intelligent transportation system through predicting future traffic is important, which is an indispensable part of a smart city. Accurate traffic prediction based on machine and deep learning modeling can help to minimize the issues [ 17 , 30 , 31 ]. For example, based on the travel history and trend of traveling through various routes, machine learning can assist transportation companies in predicting possible issues that may occur on specific routes and recommending their customers to take a different path. Ultimately, these learning-based data-driven models help improve traffic flow, increase the usage and efficiency of sustainable modes of transportation, and limit real-world disruption by modeling and visualizing future changes.
Healthcare and COVID-19 pandemic: Machine learning can help to solve diagnostic and prognostic problems in a variety of medical domains, such as disease prediction, medical knowledge extraction, detecting regularities in data, patient management, etc. [ 33 , 77 , 112 ]. Coronavirus disease (COVID-19) is an infectious disease caused by a newly discovered coronavirus, according to the World Health Organization (WHO) [ 3 ]. Recently, the learning techniques have become popular in the battle against COVID-19 [ 61 , 63 ]. For the COVID-19 pandemic, the learning techniques are used to classify patients at high risk, their mortality rate, and other anomalies [ 61 ]. It can also be used to better understand the virus’s origin, COVID-19 outbreak prediction, as well as for disease diagnosis and treatment [ 14 , 50 ]. With the help of machine learning, researchers can forecast where and when, the COVID-19 is likely to spread, and notify those regions to match the required arrangements. Deep learning also provides exciting solutions to the problems of medical image processing and is seen as a crucial technique for potential applications, particularly for COVID-19 pandemic [ 10 , 78 , 111 ]. Overall, machine and deep learning techniques can help to fight the COVID-19 virus and the pandemic as well as intelligent clinical decisions making in the domain of healthcare.
E-commerce and product recommendations: Product recommendation is one of the most well known and widely used applications of machine learning, and it is one of the most prominent features of almost any e-commerce website today. Machine learning technology can assist businesses in analyzing their consumers’ purchasing histories and making customized product suggestions for their next purchase based on their behavior and preferences. E-commerce companies, for example, can easily position product suggestions and offers by analyzing browsing trends and click-through rates of specific items. Using predictive modeling based on machine learning techniques, many online retailers, such as Amazon [ 71 ], can better manage inventory, prevent out-of-stock situations, and optimize logistics and warehousing. The future of sales and marketing is the ability to capture, evaluate, and use consumer data to provide a customized shopping experience. Furthermore, machine learning techniques enable companies to create packages and content that are tailored to the needs of their customers, allowing them to maintain existing customers while attracting new ones.
NLP and sentiment analysis: Natural language processing (NLP) involves the reading and understanding of spoken or written language through the medium of a computer [ 79 , 103 ]. Thus, NLP helps computers, for instance, to read a text, hear speech, interpret it, analyze sentiment, and decide which aspects are significant, where machine learning techniques can be used. Virtual personal assistant, chatbot, speech recognition, document description, language or machine translation, etc. are some examples of NLP-related tasks. Sentiment Analysis [ 90 ] (also referred to as opinion mining or emotion AI) is an NLP sub-field that seeks to identify and extract public mood and views within a given text through blogs, reviews, social media, forums, news, etc. For instance, businesses and brands use sentiment analysis to understand the social sentiment of their brand, product, or service through social media platforms or the web as a whole. Overall, sentiment analysis is considered as a machine learning task that analyzes texts for polarity, such as “positive”, “negative”, or “neutral” along with more intense emotions like very happy, happy, sad, very sad, angry, have interest, or not interested etc.
Image, speech and pattern recognition: Image recognition [ 36 ] is a well-known and widespread example of machine learning in the real world, which can identify an object as a digital image. For instance, to label an x-ray as cancerous or not, character recognition, or face detection in an image, tagging suggestions on social media, e.g., Facebook, are common examples of image recognition. Speech recognition [ 23 ] is also very popular that typically uses sound and linguistic models, e.g., Google Assistant, Cortana, Siri, Alexa, etc. [ 67 ], where machine learning methods are used. Pattern recognition [ 13 ] is defined as the automated recognition of patterns and regularities in data, e.g., image analysis. Several machine learning techniques such as classification, feature selection, clustering, or sequence labeling methods are used in the area.
Sustainable agriculture: Agriculture is essential to the survival of all human activities [ 109 ]. Sustainable agriculture practices help to improve agricultural productivity while also reducing negative impacts on the environment [ 5 , 25 , 109 ]. The sustainable agriculture supply chains are knowledge-intensive and based on information, skills, technologies, etc., where knowledge transfer encourages farmers to enhance their decisions to adopt sustainable agriculture practices utilizing the increasing amount of data captured by emerging technologies, e.g., the Internet of Things (IoT), mobile technologies and devices, etc. [ 5 , 53 , 54 ]. Machine learning can be applied in various phases of sustainable agriculture, such as in the pre-production phase - for the prediction of crop yield, soil properties, irrigation requirements, etc.; in the production phase—for weather prediction, disease detection, weed detection, soil nutrient management, livestock management, etc.; in processing phase—for demand estimation, production planning, etc. and in the distribution phase - the inventory management, consumer analysis, etc.
User behavior analytics and context-aware smartphone applications: Context-awareness is a system’s ability to capture knowledge about its surroundings at any moment and modify behaviors accordingly [ 28 , 93 ]. Context-aware computing uses software and hardware to automatically collect and interpret data for direct responses. The mobile app development environment has been changed greatly with the power of AI, particularly, machine learning techniques through their learning capabilities from contextual data [ 103 , 136 ]. Thus, the developers of mobile apps can rely on machine learning to create smart apps that can understand human behavior, support, and entertain users [ 107 , 137 , 140 ]. To build various personalized data-driven context-aware systems, such as smart interruption management, smart mobile recommendation, context-aware smart searching, decision-making that intelligently assist end mobile phone users in a pervasive computing environment, machine learning techniques are applicable. For example, context-aware association rules can be used to build an intelligent phone call application [ 104 ]. Clustering approaches are useful in capturing users’ diverse behavioral activities by taking into account data in time series [ 102 ]. To predict the future events in various contexts, the classification methods can be used [ 106 , 139 ]. Thus, various learning techniques discussed in Sect. “ Machine Learning Tasks and Algorithms ” can help to build context-aware adaptive and smart applications according to the preferences of the mobile phone users.
In addition to these application areas, machine learning-based models can also apply to several other domains such as bioinformatics, cheminformatics, computer networks, DNA sequence classification, economics and banking, robotics, advanced engineering, and many more.
Challenges and Research Directions
Our study on machine learning algorithms for intelligent data analysis and applications opens several research issues in the area. Thus, in this section, we summarize and discuss the challenges faced and the potential research opportunities and future directions.
In general, the effectiveness and the efficiency of a machine learning-based solution depend on the nature and characteristics of the data, and the performance of the learning algorithms. To collect the data in the relevant domain, such as cybersecurity, IoT, healthcare and agriculture discussed in Sect. “ Applications of Machine Learning ” is not straightforward, although the current cyberspace enables the production of a huge amount of data with very high frequency. Thus, collecting useful data for the target machine learning-based applications, e.g., smart city applications, and their management is important to further analysis. Therefore, a more in-depth investigation of data collection methods is needed while working on the real-world data. Moreover, the historical data may contain many ambiguous values, missing values, outliers, and meaningless data. The machine learning algorithms, discussed in Sect “ Machine Learning Tasks and Algorithms ” highly impact on data quality, and availability for training, and consequently on the resultant model. Thus, to accurately clean and pre-process the diverse data collected from diverse sources is a challenging task. Therefore, effectively modifying or enhance existing pre-processing methods, or proposing new data preparation techniques are required to effectively use the learning algorithms in the associated application domain.
To analyze the data and extract insights, there exist many machine learning algorithms, summarized in Sect. “ Machine Learning Tasks and Algorithms ”. Thus, selecting a proper learning algorithm that is suitable for the target application is challenging. The reason is that the outcome of different learning algorithms may vary depending on the data characteristics [ 106 ]. Selecting a wrong learning algorithm would result in producing unexpected outcomes that may lead to loss of effort, as well as the model’s effectiveness and accuracy. In terms of model building, the techniques discussed in Sect. “ Machine Learning Tasks and Algorithms ” can directly be used to solve many real-world issues in diverse domains, such as cybersecurity, smart cities and healthcare summarized in Sect. “ Applications of Machine Learning ”. However, the hybrid learning model, e.g., the ensemble of methods, modifying or enhancement of the existing learning techniques, or designing new learning methods, could be a potential future work in the area.
Thus, the ultimate success of a machine learning-based solution and corresponding applications mainly depends on both the data and the learning algorithms. If the data are bad to learn, such as non-representative, poor-quality, irrelevant features, or insufficient quantity for training, then the machine learning models may become useless or will produce lower accuracy. Therefore, effectively processing the data and handling the diverse learning algorithms are important, for a machine learning-based solution and eventually building intelligent applications.
In this paper, we have conducted a comprehensive overview of machine learning algorithms for intelligent data analysis and applications. According to our goal, we have briefly discussed how various types of machine learning methods can be used for making solutions to various real-world issues. A successful machine learning model depends on both the data and the performance of the learning algorithms. The sophisticated learning algorithms then need to be trained through the collected real-world data and knowledge related to the target application before the system can assist with intelligent decision-making. We also discussed several popular application areas based on machine learning techniques to highlight their applicability in various real-world issues. Finally, we have summarized and discussed the challenges faced and the potential research opportunities and future directions in the area. Therefore, the challenges that are identified create promising research opportunities in the field which must be addressed with effective solutions in various application areas. Overall, we believe that our study on machine learning-based solutions opens up a promising direction and can be used as a reference guide for potential research and applications for both academia and industry professionals as well as for decision-makers, from a technical point of view.
Canadian institute of cybersecurity, university of new brunswick, iscx dataset, http://www.unb.ca/cic/datasets/index.html/ (Accessed on 20 October 2019).
Cic-ddos2019 [online]. available: https://www.unb.ca/cic/datasets/ddos-2019.html/ (Accessed on 28 March 2020).
World health organization: WHO. http://www.who.int/ .
Google trends. In https://trends.google.com/trends/ , 2019.
Adnan N, Nordin Shahrina Md, Rahman I, Noor A. The effects of knowledge transfer on farmers decision making toward sustainable agriculture practices. World J Sci Technol Sustain Dev. 2018.
Agrawal R, Gehrke J, Gunopulos D, Raghavan P. Automatic subspace clustering of high dimensional data for data mining applications. In: Proceedings of the 1998 ACM SIGMOD international conference on Management of data. 1998; 94–105
Agrawal R, Imieliński T, Swami A. Mining association rules between sets of items in large databases. In: ACM SIGMOD Record. ACM. 1993;22: 207–216
Agrawal R, Gehrke J, Gunopulos D, Raghavan P. Fast algorithms for mining association rules. In: Proceedings of the International Joint Conference on Very Large Data Bases, Santiago Chile. 1994; 1215: 487–499.
Aha DW, Kibler D, Albert M. Instance-based learning algorithms. Mach Learn. 1991;6(1):37–66.
Article Google Scholar
Alakus TB, Turkoglu I. Comparison of deep learning approaches to predict covid-19 infection. Chaos Solit Fract. 2020;140:
Amit Y, Geman D. Shape quantization and recognition with randomized trees. Neural Comput. 1997;9(7):1545–88.
Ankerst M, Breunig MM, Kriegel H-P, Sander J. Optics: ordering points to identify the clustering structure. ACM Sigmod Record. 1999;28(2):49–60.
Anzai Y. Pattern recognition and machine learning. Elsevier; 2012.
MATH Google Scholar
Ardabili SF, Mosavi A, Ghamisi P, Ferdinand F, Varkonyi-Koczy AR, Reuter U, Rabczuk T, Atkinson PM. Covid-19 outbreak prediction with machine learning. Algorithms. 2020;13(10):249.
Article MathSciNet Google Scholar
Baldi P. Autoencoders, unsupervised learning, and deep architectures. In: Proceedings of ICML workshop on unsupervised and transfer learning, 2012; 37–49 .
Balducci F, Impedovo D, Pirlo G. Machine learning applications on agricultural datasets for smart farm enhancement. Machines. 2018;6(3):38.
Boukerche A, Wang J. Machine learning-based traffic prediction models for intelligent transportation systems. Comput Netw. 2020;181
Breiman L. Bagging predictors. Mach Learn. 1996;24(2):123–40.
Article MATH Google Scholar
Breiman L. Random forests. Mach Learn. 2001;45(1):5–32.
Breiman L, Friedman J, Stone CJ, Olshen RA. Classification and regression trees. CRC Press; 1984.
Cao L. Data science: a comprehensive overview. ACM Comput Surv (CSUR). 2017;50(3):43.
Google Scholar
Carpenter GA, Grossberg S. A massively parallel architecture for a self-organizing neural pattern recognition machine. Comput Vis Graph Image Process. 1987;37(1):54–115.
Chiu C-C, Sainath TN, Wu Y, Prabhavalkar R, Nguyen P, Chen Z, Kannan A, Weiss RJ, Rao K, Gonina E, et al. State-of-the-art speech recognition with sequence-to-sequence models. In: 2018 IEEE International Conference on Acoustics, Speech and Signal Processing (ICASSP), 2018 pages 4774–4778. IEEE .
Chollet F. Xception: deep learning with depthwise separable convolutions. In: Proceedings of the IEEE conference on computer vision and pattern recognition, pages 1251–1258, 2017.
Cobuloglu H, Büyüktahtakın IE. A stochastic multi-criteria decision analysis for sustainable biomass crop selection. Expert Syst Appl. 2015;42(15–16):6065–74.
Das A, Ng W-K, Woon Y-K. Rapid association rule mining. In: Proceedings of the tenth international conference on Information and knowledge management, pages 474–481. ACM, 2001.
de Amorim RC. Constrained clustering with minkowski weighted k-means. In: 2012 IEEE 13th International Symposium on Computational Intelligence and Informatics (CINTI), pages 13–17. IEEE, 2012.
Dey AK. Understanding and using context. Person Ubiquit Comput. 2001;5(1):4–7.
Eagle N, Pentland AS. Reality mining: sensing complex social systems. Person Ubiquit Comput. 2006;10(4):255–68.
Essien A, Petrounias I, Sampaio P, Sampaio S. Improving urban traffic speed prediction using data source fusion and deep learning. In: 2019 IEEE International Conference on Big Data and Smart Computing (BigComp). IEEE. 2019: 1–8. .
Essien A, Petrounias I, Sampaio P, Sampaio S. A deep-learning model for urban traffic flow prediction with traffic events mined from twitter. In: World Wide Web, 2020: 1–24 .
Ester M, Kriegel H-P, Sander J, Xiaowei X, et al. A density-based algorithm for discovering clusters in large spatial databases with noise. Kdd. 1996;96:226–31.
Fatima M, Pasha M, et al. Survey of machine learning algorithms for disease diagnostic. J Intell Learn Syst Appl. 2017;9(01):1.
Flach PA, Lachiche N. Confirmation-guided discovery of first-order rules with tertius. Mach Learn. 2001;42(1–2):61–95.
Freund Y, Schapire RE, et al. Experiments with a new boosting algorithm. In: Icml, Citeseer. 1996; 96: 148–156
Fujiyoshi H, Hirakawa T, Yamashita T. Deep learning-based image recognition for autonomous driving. IATSS Res. 2019;43(4):244–52.
Fukunaga K, Hostetler L. The estimation of the gradient of a density function, with applications in pattern recognition. IEEE Trans Inform Theory. 1975;21(1):32–40.
Article MathSciNet MATH Google Scholar
Goodfellow I, Bengio Y, Courville A, Bengio Y. Deep learning. Cambridge: MIT Press; 2016.
Goodfellow I, Pouget-Abadie J, Mirza M, Xu B, Warde-Farley D, Ozair S, Courville A, Bengio Y. Generative adversarial nets. In: Advances in neural information processing systems. 2014: 2672–2680.
Guerrero-Ibáñez J, Zeadally S, Contreras-Castillo J. Sensor technologies for intelligent transportation systems. Sensors. 2018;18(4):1212.
Han J, Pei J, Kamber M. Data mining: concepts and techniques. Amsterdam: Elsevier; 2011.
Han J, Pei J, Yin Y. Mining frequent patterns without candidate generation. In: ACM Sigmod Record, ACM. 2000;29: 1–12.
Harmon SA, Sanford TH, Sheng X, Turkbey EB, Roth H, Ziyue X, Yang D, Myronenko A, Anderson V, Amalou A, et al. Artificial intelligence for the detection of covid-19 pneumonia on chest ct using multinational datasets. Nat Commun. 2020;11(1):1–7.
He K, Zhang X, Ren S, Sun J. Spatial pyramid pooling in deep convolutional networks for visual recognition. IEEE Trans Pattern Anal Mach Intell. 2015;37(9):1904–16.
He K, Zhang X, Ren S, Sun J. Deep residual learning for image recognition. In: Proceedings of the IEEE conference on computer vision and pattern recognition, 2016: 770–778.
Hinton GE. A practical guide to training restricted boltzmann machines. In: Neural networks: Tricks of the trade. Springer. 2012; 599-619
Holte RC. Very simple classification rules perform well on most commonly used datasets. Mach Learn. 1993;11(1):63–90.
Hotelling H. Analysis of a complex of statistical variables into principal components. J Edu Psychol. 1933;24(6):417.
Houtsma M, Swami A. Set-oriented mining for association rules in relational databases. In: Data Engineering, 1995. Proceedings of the Eleventh International Conference on, IEEE.1995:25–33.
Jamshidi M, Lalbakhsh A, Talla J, Peroutka Z, Hadjilooei F, Lalbakhsh P, Jamshidi M, La Spada L, Mirmozafari M, Dehghani M, et al. Artificial intelligence and covid-19: deep learning approaches for diagnosis and treatment. IEEE Access. 2020;8:109581–95.
John GH, Langley P. Estimating continuous distributions in bayesian classifiers. In: Proceedings of the Eleventh conference on Uncertainty in artificial intelligence, Morgan Kaufmann Publishers Inc. 1995; 338–345
Kaelbling LP, Littman ML, Moore AW. Reinforcement learning: a survey. J Artif Intell Res. 1996;4:237–85.
Kamble SS, Gunasekaran A, Gawankar SA. Sustainable industry 4.0 framework: a systematic literature review identifying the current trends and future perspectives. Process Saf Environ Protect. 2018;117:408–25.
Kamble SS, Gunasekaran A, Gawankar SA. Achieving sustainable performance in a data-driven agriculture supply chain: a review for research and applications. Int J Prod Econ. 2020;219:179–94.
Kaufman L, Rousseeuw PJ. Finding groups in data: an introduction to cluster analysis, vol. 344. John Wiley & Sons; 2009.
Keerthi SS, Shevade SK, Bhattacharyya C, Radha Krishna MK. Improvements to platt’s smo algorithm for svm classifier design. Neural Comput. 2001;13(3):637–49.
Khadse V, Mahalle PN, Biraris SV. An empirical comparison of supervised machine learning algorithms for internet of things data. In: 2018 Fourth International Conference on Computing Communication Control and Automation (ICCUBEA), IEEE. 2018; 1–6
Kohonen T. The self-organizing map. Proc IEEE. 1990;78(9):1464–80.
Koroniotis N, Moustafa N, Sitnikova E, Turnbull B. Towards the development of realistic botnet dataset in the internet of things for network forensic analytics: bot-iot dataset. Fut Gen Comput Syst. 2019;100:779–96.
Krizhevsky A, Sutskever I, Hinton GE. Imagenet classification with deep convolutional neural networks. In: Advances in neural information processing systems, 2012: 1097–1105
Kushwaha S, Bahl S, Bagha AK, Parmar KS, Javaid M, Haleem A, Singh RP. Significant applications of machine learning for covid-19 pandemic. J Ind Integr Manag. 2020;5(4).
Lade P, Ghosh R, Srinivasan S. Manufacturing analytics and industrial internet of things. IEEE Intell Syst. 2017;32(3):74–9.
Lalmuanawma S, Hussain J, Chhakchhuak L. Applications of machine learning and artificial intelligence for covid-19 (sars-cov-2) pandemic: a review. Chaos Sol Fract. 2020:110059 .
LeCessie S, Van Houwelingen JC. Ridge estimators in logistic regression. J R Stat Soc Ser C (Appl Stat). 1992;41(1):191–201.
LeCun Y, Bottou L, Bengio Y, Haffner P. Gradient-based learning applied to document recognition. Proc IEEE. 1998;86(11):2278–324.
Liu H, Motoda H. Feature extraction, construction and selection: A data mining perspective, vol. 453. Springer Science & Business Media; 1998.
López G, Quesada L, Guerrero LA. Alexa vs. siri vs. cortana vs. google assistant: a comparison of speech-based natural user interfaces. In: International Conference on Applied Human Factors and Ergonomics, Springer. 2017; 241–250.
Liu B, HsuW, Ma Y. Integrating classification and association rule mining. In: Proceedings of the fourth international conference on knowledge discovery and data mining, 1998.
MacQueen J, et al. Some methods for classification and analysis of multivariate observations. In: Proceedings of the fifth Berkeley symposium on mathematical statistics and probability, 1967;volume 1, pages 281–297. Oakland, CA, USA.
Mahdavinejad MS, Rezvan M, Barekatain M, Adibi P, Barnaghi P, Sheth AP. Machine learning for internet of things data analysis: a survey. Digit Commun Netw. 2018;4(3):161–75.
Marchand A, Marx P. Automated product recommendations with preference-based explanations. J Retail. 2020;96(3):328–43.
McCallum A. Information extraction: distilling structured data from unstructured text. Queue. 2005;3(9):48–57.
Mehrotra A, Hendley R, Musolesi M. Prefminer: mining user’s preferences for intelligent mobile notification management. In: Proceedings of the International Joint Conference on Pervasive and Ubiquitous Computing, Heidelberg, Germany, 12–16 September, 2016; pp. 1223–1234. ACM, New York, USA. .
Mohamadou Y, Halidou A, Kapen PT. A review of mathematical modeling, artificial intelligence and datasets used in the study, prediction and management of covid-19. Appl Intell. 2020;50(11):3913–25.
Mohammed M, Khan MB, Bashier Mohammed BE. Machine learning: algorithms and applications. CRC Press; 2016.
Book Google Scholar
Moustafa N, Slay J. Unsw-nb15: a comprehensive data set for network intrusion detection systems (unsw-nb15 network data set). In: 2015 military communications and information systems conference (MilCIS), 2015;pages 1–6. IEEE .
Nilashi M, Ibrahim OB, Ahmadi H, Shahmoradi L. An analytical method for diseases prediction using machine learning techniques. Comput Chem Eng. 2017;106:212–23.
Yujin O, Park S, Ye JC. Deep learning covid-19 features on cxr using limited training data sets. IEEE Trans Med Imaging. 2020;39(8):2688–700.
Otter DW, Medina JR , Kalita JK. A survey of the usages of deep learning for natural language processing. IEEE Trans Neural Netw Learn Syst. 2020.
Park H-S, Jun C-H. A simple and fast algorithm for k-medoids clustering. Expert Syst Appl. 2009;36(2):3336–41.
Liii Pearson K. on lines and planes of closest fit to systems of points in space. Lond Edinb Dublin Philos Mag J Sci. 1901;2(11):559–72.
Pedregosa F, Varoquaux G, Gramfort A, Michel V, Thirion B, Grisel O, Blondel M, Prettenhofer P, Weiss R, Dubourg V, et al. Scikit-learn: machine learning in python. J Mach Learn Res. 2011;12:2825–30.
MathSciNet MATH Google Scholar
Perveen S, Shahbaz M, Keshavjee K, Guergachi A. Metabolic syndrome and development of diabetes mellitus: predictive modeling based on machine learning techniques. IEEE Access. 2018;7:1365–75.
Santi P, Ram D, Rob C, Nathan E. Behavior-based adaptive call predictor. ACM Trans Auton Adapt Syst. 2011;6(3):21:1–21:28.
Polydoros AS, Nalpantidis L. Survey of model-based reinforcement learning: applications on robotics. J Intell Robot Syst. 2017;86(2):153–73.
Puterman ML. Markov decision processes: discrete stochastic dynamic programming. John Wiley & Sons; 2014.
Quinlan JR. Induction of decision trees. Mach Learn. 1986;1:81–106.
Quinlan JR. C4.5: programs for machine learning. Mach Learn. 1993.
Rasmussen C. The infinite gaussian mixture model. Adv Neural Inform Process Syst. 1999;12:554–60.
Ravi K, Ravi V. A survey on opinion mining and sentiment analysis: tasks, approaches and applications. Knowl Syst. 2015;89:14–46.
Rokach L. A survey of clustering algorithms. In: Data mining and knowledge discovery handbook, pages 269–298. Springer, 2010.
Safdar S, Zafar S, Zafar N, Khan NF. Machine learning based decision support systems (dss) for heart disease diagnosis: a review. Artif Intell Rev. 2018;50(4):597–623.
Sarker IH. Context-aware rule learning from smartphone data: survey, challenges and future directions. J Big Data. 2019;6(1):1–25.
Sarker IH. A machine learning based robust prediction model for real-life mobile phone data. Internet Things. 2019;5:180–93.
Sarker IH. Ai-driven cybersecurity: an overview, security intelligence modeling and research directions. SN Comput Sci. 2021.
Sarker IH. Deep cybersecurity: a comprehensive overview from neural network and deep learning perspective. SN Comput Sci. 2021.
Sarker IH, Abushark YB, Alsolami F, Khan A. Intrudtree: a machine learning based cyber security intrusion detection model. Symmetry. 2020;12(5):754.
Sarker IH, Abushark YB, Khan A. Contextpca: predicting context-aware smartphone apps usage based on machine learning techniques. Symmetry. 2020;12(4):499.
Sarker IH, Alqahtani H, Alsolami F, Khan A, Abushark YB, Siddiqui MK. Context pre-modeling: an empirical analysis for classification based user-centric context-aware predictive modeling. J Big Data. 2020;7(1):1–23.
Sarker IH, Alan C, Jun H, Khan AI, Abushark YB, Khaled S. Behavdt: a behavioral decision tree learning to build user-centric context-aware predictive model. Mob Netw Appl. 2019; 1–11.
Sarker IH, Colman A, Kabir MA, Han J. Phone call log as a context source to modeling individual user behavior. In: Proceedings of the 2016 ACM International Joint Conference on Pervasive and Ubiquitous Computing (Ubicomp): Adjunct, Germany, pages 630–634. ACM, 2016.
Sarker IH, Colman A, Kabir MA, Han J. Individualized time-series segmentation for mining mobile phone user behavior. Comput J Oxf Univ UK. 2018;61(3):349–68.
Sarker IH, Hoque MM, MdK Uddin, Tawfeeq A. Mobile data science and intelligent apps: concepts, ai-based modeling and research directions. Mob Netw Appl, pages 1–19, 2020.
Sarker IH, Kayes ASM. Abc-ruleminer: user behavioral rule-based machine learning method for context-aware intelligent services. J Netw Comput Appl. 2020; page 102762
Sarker IH, Kayes ASM, Badsha S, Alqahtani H, Watters P, Ng A. Cybersecurity data science: an overview from machine learning perspective. J Big Data. 2020;7(1):1–29.
Sarker IH, Watters P, Kayes ASM. Effectiveness analysis of machine learning classification models for predicting personalized context-aware smartphone usage. J Big Data. 2019;6(1):1–28.
Sarker IH, Salah K. Appspred: predicting context-aware smartphone apps using random forest learning. Internet Things. 2019;8:
Scheffer T. Finding association rules that trade support optimally against confidence. Intell Data Anal. 2005;9(4):381–95.
Sharma R, Kamble SS, Gunasekaran A, Kumar V, Kumar A. A systematic literature review on machine learning applications for sustainable agriculture supply chain performance. Comput Oper Res. 2020;119:
Shengli S, Ling CX. Hybrid cost-sensitive decision tree, knowledge discovery in databases. In: PKDD 2005, Proceedings of 9th European Conference on Principles and Practice of Knowledge Discovery in Databases. Lecture Notes in Computer Science, volume 3721, 2005.
Shorten C, Khoshgoftaar TM, Furht B. Deep learning applications for covid-19. J Big Data. 2021;8(1):1–54.
Gökhan S, Nevin Y. Data analysis in health and big data: a machine learning medical diagnosis model based on patients’ complaints. Commun Stat Theory Methods. 2019;1–10
Silver D, Huang A, Maddison CJ, Guez A, Sifre L, Van Den Driessche G, Schrittwieser J, Antonoglou I, Panneershelvam V, Lanctot M, et al. Mastering the game of go with deep neural networks and tree search. nature. 2016;529(7587):484–9.
Ślusarczyk B. Industry 4.0: Are we ready? Polish J Manag Stud. 17, 2018.
Sneath Peter HA. The application of computers to taxonomy. J Gen Microbiol. 1957;17(1).
Sorensen T. Method of establishing groups of equal amplitude in plant sociology based on similarity of species. Biol Skr. 1948; 5.
Srinivasan V, Moghaddam S, Mukherji A. Mobileminer: mining your frequent patterns on your phone. In: Proceedings of the International Joint Conference on Pervasive and Ubiquitous Computing, Seattle, WA, USA, 13-17 September, pp. 389–400. ACM, New York, USA. 2014.
Szegedy C, Liu W, Jia Y, Sermanet P, Reed S, Anguelov D, Erhan D, Vanhoucke V, Rabinovich A. Going deeper with convolutions. In: Proceedings of the IEEE conference on computer vision and pattern recognition. 2015; pages 1–9.
Tavallaee M, Bagheri E, Lu W, Ghorbani AA. A detailed analysis of the kdd cup 99 data set. In. IEEE symposium on computational intelligence for security and defense applications. IEEE. 2009;2009:1–6.
Tsagkias M. Tracy HK, Surya K, Vanessa M, de Rijke M. Challenges and research opportunities in ecommerce search and recommendations. In: ACM SIGIR Forum. volume 54. NY, USA: ACM New York; 2021. p. 1–23.
Wagstaff K, Cardie C, Rogers S, Schrödl S, et al. Constrained k-means clustering with background knowledge. Icml. 2001;1:577–84.
Wang W, Yang J, Muntz R, et al. Sting: a statistical information grid approach to spatial data mining. VLDB. 1997;97:186–95.
Wei P, Li Y, Zhang Z, Tao H, Li Z, Liu D. An optimization method for intrusion detection classification model based on deep belief network. IEEE Access. 2019;7:87593–605.
Weiss K, Khoshgoftaar TM, Wang DD. A survey of transfer learning. J Big data. 2016;3(1):9.
Witten IH, Frank E. Data Mining: Practical machine learning tools and techniques. Morgan Kaufmann; 2005.
Witten IH, Frank E, Trigg LE, Hall MA, Holmes G, Cunningham SJ. Weka: practical machine learning tools and techniques with java implementations. 1999.
Wu C-C, Yen-Liang C, Yi-Hung L, Xiang-Yu Y. Decision tree induction with a constrained number of leaf nodes. Appl Intell. 2016;45(3):673–85.
Wu X, Kumar V, Quinlan JR, Ghosh J, Yang Q, Motoda H, McLachlan GJ, Ng A, Liu B, Philip SY, et al. Top 10 algorithms in data mining. Knowl Inform Syst. 2008;14(1):1–37.
Xin Y, Kong L, Liu Z, Chen Y, Li Y, Zhu H, Gao M, Hou H, Wang C. Machine learning and deep learning methods for cybersecurity. IEEE Access. 2018;6:35365–81.
Xu D, Yingjie T. A comprehensive survey of clustering algorithms. Ann Data Sci. 2015;2(2):165–93.
Zaki MJ. Scalable algorithms for association mining. IEEE Trans Knowl Data Eng. 2000;12(3):372–90.
Zanella A, Bui N, Castellani A, Vangelista L, Zorzi M. Internet of things for smart cities. IEEE Internet Things J. 2014;1(1):22–32.
Zhao Q, Bhowmick SS. Association rule mining: a survey. Singapore: Nanyang Technological University; 2003.
Zheng T, Xie W, Xu L, He X, Zhang Y, You M, Yang G, Chen Y. A machine learning-based framework to identify type 2 diabetes through electronic health records. Int J Med Inform. 2017;97:120–7.
Zheng Y, Rajasegarar S, Leckie C. Parking availability prediction for sensor-enabled car parks in smart cities. In: Intelligent Sensors, Sensor Networks and Information Processing (ISSNIP), 2015 IEEE Tenth International Conference on. IEEE, 2015; pages 1–6.
Zhu H, Cao H, Chen E, Xiong H, Tian J. Exploiting enriched contextual information for mobile app classification. In: Proceedings of the 21st ACM international conference on Information and knowledge management. ACM, 2012; pages 1617–1621
Zhu H, Chen E, Xiong H, Kuifei Y, Cao H, Tian J. Mining mobile user preferences for personalized context-aware recommendation. ACM Trans Intell Syst Technol (TIST). 2014;5(4):58.
Zikang H, Yong Y, Guofeng Y, Xinyu Z. Sentiment analysis of agricultural product ecommerce review data based on deep learning. In: 2020 International Conference on Internet of Things and Intelligent Applications (ITIA), IEEE, 2020; pages 1–7
Zulkernain S, Madiraju P, Ahamed SI. A context aware interruption management system for mobile devices. In: Mobile Wireless Middleware, Operating Systems, and Applications. Springer. 2010; pages 221–234
Zulkernain S, Madiraju P, Ahamed S, Stamm K. A mobile intelligent interruption management system. J UCS. 2010;16(15):2060–80.
Download references
Author information
Authors and affiliations.
Swinburne University of Technology, Melbourne, VIC, 3122, Australia
Department of Computer Science and Engineering, Chittagong University of Engineering & Technology, 4349, Chattogram, Bangladesh
You can also search for this author in PubMed Google Scholar
Corresponding author
Correspondence to Iqbal H. Sarker .
Ethics declarations
Conflict of interest.
The author declares no conflict of interest.
Additional information
Publisher's note.
Springer Nature remains neutral with regard to jurisdictional claims in published maps and institutional affiliations.
This article is part of the topical collection “Advances in Computational Approaches for Artificial Intelligence, Image Processing, IoT and Cloud Applications” guest edited by Bhanu Prakash K N and M. Shivakumar.
Rights and permissions
Reprints and permissions
About this article
Sarker, I.H. Machine Learning: Algorithms, Real-World Applications and Research Directions. SN COMPUT. SCI. 2 , 160 (2021). https://doi.org/10.1007/s42979-021-00592-x
Download citation
Received : 27 January 2021
Accepted : 12 March 2021
Published : 22 March 2021
DOI : https://doi.org/10.1007/s42979-021-00592-x
Share this article
Anyone you share the following link with will be able to read this content:
Sorry, a shareable link is not currently available for this article.
Provided by the Springer Nature SharedIt content-sharing initiative
- Machine learning
- Deep learning
- Artificial intelligence
- Data science
- Data-driven decision-making
- Predictive analytics
- Intelligent applications
- Find a journal
- Publish with us
- Track your research
Internet of Things (IoT) for Next-Generation Smart Systems: A Review of Current Challenges, Future Trends and Prospects for Emerging 5G-IoT Scenarios
Ieee account.
- Change Username/Password
- Update Address
Purchase Details
- Payment Options
- Order History
- View Purchased Documents
Profile Information
- Communications Preferences
- Profession and Education
- Technical Interests
- US & Canada: +1 800 678 4333
- Worldwide: +1 732 981 0060
- Contact & Support
- About IEEE Xplore
- Accessibility
- Terms of Use
- Nondiscrimination Policy
- Privacy & Opting Out of Cookies
A not-for-profit organization, IEEE is the world's largest technical professional organization dedicated to advancing technology for the benefit of humanity. © Copyright 2024 IEEE - All rights reserved. Use of this web site signifies your agreement to the terms and conditions.
- Browse All Articles
- Newsletter Sign-Up
TechnologicalInnovation →
No results found in working knowledge.
- Were any results found in one of the other content buckets on the left?
- Try removing some search filters.
- Use different search filters.
Advances, Systems and Applications
- Open access
- Published: 12 July 2021
Survey on serverless computing
- Hassan B. Hassan 1 ,
- Saman A. Barakat 2 &
- Qusay I. Sarhan 2
Journal of Cloud Computing volume 10 , Article number: 39 ( 2021 ) Cite this article
28k Accesses
39 Citations
1 Altmetric
Metrics details
Serverless computing has gained importance over the last decade as an exciting new field, owing to its large influence in reducing costs, decreasing latency, improving scalability, and eliminating server-side management, to name a few. However, to date there is a lack of in-depth survey that would help developers and researchers better understand the significance of serverless computing in different contexts. Thus, it is essential to present research evidence that has been published in this area. In this systematic survey, 275 research papers that examined serverless computing from well-known literature databases were extensively reviewed to extract useful data. Then, the obtained data were analyzed to answer several research questions regarding state-of-the-art contributions of serverless computing, its concepts, its platforms, its usage, etc. We moreover discuss the challenges that serverless computing faces nowadays and how future research could enable its implementation and usage.
Introduction
Cloud computing emerged after the appearance of virtualization in software and hardware infrastructures; hence cloud providers increasingly adopted it to offer their services to customers [ 1 , 2 ]. Customers can access these cloud services via the Internet. Software developers have been using cloud technologies in their software solutions owing to their benefits including scalability, availability, and flexibility [ 3 ].
In general, cloud computing is divided into three main categories based on the provision of services, which are software as a service (SaaS), platform as a service (PaaS), and infrastructure as a service (IaaS). In the SaaS category, cloud providers offer different types of software as services to the users. For example, Google provides many applications as a service (e.g., Gmail, Google docs, Google sheets, and Google forms). In this type of cloud, the user is not responsible for the services development, deployment, and management. The user here only uses them without worrying about their settings, configurations, etc. Meanwhile, in the PaaS, cloud companies provide services such as network access, storage, servers, and operating systems to be purchased by developers. The developers access these services to deploy, run, and manage their applications. In this kind of cloud, the developer is responsible for the deployment and management (settings and configurations) of their software to ensure that the application is running, while they do not control the services. Finally, in the IaaS category, the cloud consumers control and manage services such as network access, servers, operating systems, and storage.
Managing cloud services is not an easy task at all. The authors in [ 4 ] have addressed several challenges while managing a cloud environment by a user such as availability, load balancing, auto-scaling, security, monitoring, etc. For example, the cloud user has to ensure the availability of the services in which if a single machine failure occurs, it does not affect the whole services. Also, he/she has to consider distributing copies of the services geographically to protect them when disasters happen. Another challenge is load balancing. In this case, the cloud user has to ensure that requests to the services are balanced to utilize all resources efficiently.
These challenges have led to introduce another cloud computing model, which is called serverless cloud computing [ 4 ]. Serverless cloud computing offers backend as a service (BaaS) and function as a service (FaaS), as shown in Fig. 1 . The BaaS includes services like storage, messaging, user management, etc. While, the FaaS enables developers to deploy and execute their code on computing platforms. The FaaS relies on the services provided by the BaaS such as a database, messaging, user authentications, etc. The FaaS is considered as the most dominant model of serverless, and it is also known as “event-driven functions” [ 5 , 6 ].
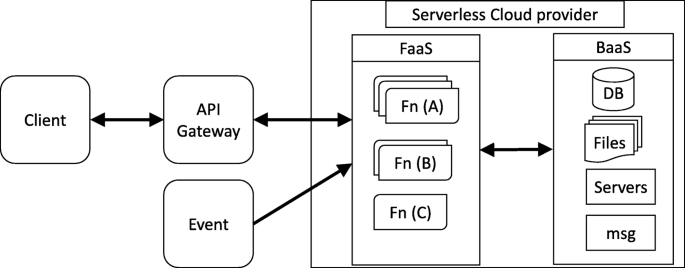
Serverless architecture
Serverless cloud model was for the first time introduced by Amazon Lambda in 2014, after which cloud companies like Google and Microsoft adopted it in 2016. Serverless cloud computing adds an additional abstraction layer to the existing cloud computing paradigms, while it abstracts away the server-side management from the developers [ 7 ]. Serverless model lets the developers focus on the application logic rather than the server-side management and configurations. For example, the developers deploy their applications to the serverless cloud as functions see Fig. 1 . Then, the cloud provider takes responsibility for managing, scaling, and providing different resources to ensure the smooth running of these functions [ 8 , 9 ].
However, FaaS and the term “serverless” could be used interchangeably, as the FaaS platform automatically configures and maintains the execution context of functions and connects them to cloud services without requiring server provision by developers [ 10 , 11 ]. We refer to the FaaS when we use the term serverless computing.
Serverless cloud computing has many good characteristics [ 12 , 13 ], one of which is scalability. Scaling could be vertical or horizontal; vertical scaling adds or removes cores from the running container, while horizontal scaling creates new containers or eliminates running ones without affecting the current resource allocations [ 14 ]. In serverless computing, the applications automatically scale up and down on demand, and the developer does not have to concern themselves about the scaling issues. For example, when an application runs on a serverless cloud, it will scale up automatically when the application requests increase. Another characteristic of serverless computing is the payment per resource usage. This paradigm of cloud computing charges developers based on the actual resource usage. For example, deploying an application will not cost the developer in the case where the application is idle, and the serverless provider will only charge whenever the application has started using resources.
However, any new technology will face numerous technical and operational issues and obstacles at the beginning. Since the recent introduction of serverless cloud computing, several drawbacks have been identified [ 7 ]. Serverless cloud computing lacks tools that help managing and monitoring serverless applications. Moreover, it might comprise security concerns. Further, the serverless providers have a vendor lock-in problem. Nevertheless, serverless cloud computing has gained positive attention in the industry, despite that it has not been studied extensively in academic research [ 7 ].
Therefore, the aim of this research is to answer some crucial research questions related to serverless cloud computing and thereby help researchers as well as developers to better understand serverless cloud computing and contribute to its development.
The rest of this paper is structured as follows: “ Related works ” section presents the related works for this study. “ Research methodology ” section describes in detail the research methodology used to conduct this survey study. “ Results ” section presents the results and outcomes of the study. “ Threats to validity ” section presents the threats to validity of this study. Finally, the conclusions of the study are provided in “ Conclusions ” section.
Related works
The most relevant studies published on the topic are briefly presented here. The authors in [ 15 ] and [ 16 ] discussed some important background to the origin and evolution of serverless computing and the long road that serverless computing has taken over the years. The authors in [ 9 ] thoroughly discussed the true meaning of serverless architectures and how they are changing the way in which applications are built, deployed, and distributed.
Numerous studies focused on technical interpretations of serverless computing, while other more recent research suggested various benefits that it brings to developers. Nowadays, this type of computing is being used in several ways. In an empirical study, the authors in [ 17 ] aimed to investigate the development practices of serverless computing in the industry. They concluded that for developers, it remains a barrier to adopt the right mindset to best utilize the tools inherent to serverless architecture. More documentation and easier access to such resources would help developers to embrace the possibilities that serverless computing has to offer.
The concept of serverless computing within the scope of the IT industry has the great potential of progressively increasing its capabilities to involve a wider set of domains. Thus, the implementation of serverless computing is not restricted only to the enhancement of infrastructure, and it can be employed for many different purposes, e.g., serverless messaging, neural network training [ 18 ], video processing [ 19 ], and big data [ 20 ]. Undeniably, their contributions are valuable to the general public and researchers in the field, as it is of primarily importance to comprehend how this technology works.
However, it is presently crucial to provide more than only theories and concepts: it is time to weigh the benefits and drawbacks of serverless computing and to analyze how far the field has progressed, to assess what remains to be done and improved. As an example, the authors in [ 21 ] discussed some possible new abstraction levels to reduce processing limitations. The authors in [ 22 ] discussed the results from an open-source framework to achieve on-premises serverless computing that can process big workloads with a scalable and sensible usage of resources. We can infer from these related publications that researchers everywhere are working to determine how to best exploit the potential that serverless computing frameworks could introduce to software development.
In [ 23 ], the authors described how serverless computing is becoming the next step in the evolution of cloud computing and its platforms. In our paper, we focus on the ongoing challenges, benefits, and drawbacks of using it.
The authors in [ 24 ] have conducted a systematic exploration of serverless computing-related research papers. As they mentioned, their work is not a survey, but it is a supporting source for future research papers. They proposed an open dataset for serverless computing papers. The dataset includes 60 papers for the period (2016-July 2018). Also, they have analyzed the dataset according to bibliometric, content, technology, and produced statistics about each section. In contrast, our paper aims to conduct a systematic survey. In this survey, we try to find answers to several critical questions related to serverless computing. In addition to that, our study covered the duration (2016–2020) and thus 275 papers have been considered.
The authors in [ 25 ] mainly focused on scheduling tasks in the cloud. They described the various techniques in scheduling workflows to reduce the execution time, cost, or both. Moreover, they proposed a hybrid method by both FaaS and IaaS. The small tasks could be executed remotely using the FaaS, which reduces the execution cost; hence, the number of virtual machines will be decreased as well. Therefore, the whole focus would be on the long-running tasks on IaaS.
The authors in [ 26 ] covered only 24 research papers during 2017–2019. In their paper, they considered both the white and grey literatures. Besides, they identified 32 characteristics of serverless and the possible issues related to them, only eight of them were stated by both white and grey literatures while the remaining are from grey literature only. All the characteristics are explained and presented briefly. In our paper, 275 research papers from 2016–2020 have been covered and more research questions have been answered. Besides, a well-defined systematic literature study process has been employed. Thus, the grey literature has been excluded in our paper and, our results are reproducible compared to their results.
The authors in [ 27 ] mainly concentrated on difficulties and gaps in data-centric and distributed computing using FaaS. Additionally, they evaluated the severity of these challenges via taking three case studies from big data and distributed computing settings: model training, low-latency prediction serving using the batch and, distributed computing. While our paper is a broad and comprehensive study on FaaS, 275 research papers are taken from the white literature during 2016–2020.
The paper [ 28 ] presented only four use cases of FaaS: event-triggered computing, video broadcasting, Internet of Things (IoT) data processing, and shared delivery system. Additionally, the paper only compared three platforms namely, Amazon web services (AWS) Lambda, Google Cloud Function, and Microsoft Azure Function. On the other hand, our paper presents a comprehensive study about FaaS. We identified in detail eight use cases: chatbot, information retrieval, file processing, smart grid, security, networks and, mobile and IoT. Moreover, our paper compared ten FaaS platforms namely, AWS Lambda, Apache OpenWhisk, Microsoft Azure functions, Google Cloud functions, OpenLambda, IBM Cloud functions, OpenFaaS, Knative, FunctionStage, Huawei Cloud, and Nuclio.
The authors in [ 29 ] covered only 15 papers during 2016–2018. They took both the white and grey literatures into account. On the other hand, our paper includes 275 research papers published in the period 2016–2020; they are taken from the white literature only. Moreover, our paper has formulated and answered eight clear and well-defined research questions.
The authors in [ 30 ] focused on the FaaS performance evaluation and their publication trends during 2016–2019. They identified the most commonly evaluated FaaS platforms. Additionally, they evaluated the performance features for benchmark types, micro-benchmarks, and common features across FaaS platforms. Moreover, they presented the most common platform configurations in FaaS, namely language runtimes, function triggers, and external services. This paper presents a survey of the most important and state of the art aspects of FaaS. Besides, comprehensive theoretical aspects of FaaS are covered taking from the white literature during 2016–2020.
The authors in [ 11 ] have conducted a systematic mapping study on serverless cloud computing. The main aim of their study is to concentrate on FaaS engineering. They raised two main concerns: (a) identifying publication research that considers developing or modifying serverless platforms and tools. (b) identifying the challenges and drivers related to these publications. On the other hand, our study extends the challenges and issues related to serverless computing. Moreover, we provide more details about serverless computing platforms and the use of these platforms in the literature. Also, it provides a detailed comparison among the most widely used serverless platforms. Besides, it addresses more aspects of serverless cloud computing such as application areas of serverless computing, future directions of serverless computing, etc.
The authors in [ 7 ] provided useful observations about serverless computing, its architecture, and use cases. Also, they discussed the challenges and benefits of moving forward from monolithic applications and the differences between traditional cloud services and serverless computing. Our work has extended the details of their work regarding the benefits and drawbacks of using serverless computing. It has also included more use cases and workloads to deepen the findings of previous studies.
The authors in [ 4 ] presented a technical report on serverless computing. They covered the serverless emergence with its limitations, including limited storage for fine-grained tasks, lack of coordination among functions, inadequate performance for standard communication patterns, and functions’ performance. Also, they compared AWS serverful with AWS serverless. Moreover, they also explained the challenges of architecture, networking, security, and abstractions of serverless computing. They identified five application areas including, video encoding in real-time, MapReduce, linear algebra, machine learning training, and databases. While our paper has covered 275 research papers from 2016–2020 forming a well-defined systematic literature study. We also identified 21 serverless challenges and issues. Besides, we compared serverless with the traditional cloud computing paradigm. We identified more application areas including, chatbot, information retrieval, file processing, smart grid, security, networks, IoT, and edge computing.
The authors in [ 31 ] presented a white paper based on published research papers during 2015–2017. They outlined the serverless definition alongside its advantages and disadvantages. Also, they classified serverless use-cases into six categories, namely, backends, web applications, chatbots, big data, IT automation, and Amazon Alexa. Moreover, they addressed a few research questions including, the need for the stateless feature in serverless, whether serverless could execute long-running tasks, programming models, serverless standards, and the importance of serverless for scientific research. While our paper is a comprehensive study on FaaS; we covered 275 research papers which are taken from the grey literature during 2016–2020. In our paper, eight application areas have been identified as mentioned earlier. We have identified and answered ten research questions that cover many aspects of the topic in detail compared to the aforementioned study.
We are in fact addressing with this paper ten important research questions about the topic, potentially making it a more complete guide to the development and use of serverless computing. Our work contributes to the analysis of the serverless paradigm in the context of similar applications and how could they better fit specific computing needs. Moreover, information about the current state of serverless platforms, tools, and frameworks has been updated for this survey. This due to the importance of the topic and its potential to change how both the industry and academia have managed the deployment of cloud applications until now. Updated information about the area could benefit future studies focused on the serverless computing paradigm as they make researchers aware of the latest resources and opportunities in the area.
Research methodology
Research questions.
In this study, a number of research questions (RQs) have been identified and answered. Each RQ addresses a particular aspect of serverless computing as follows.
RQ1. What is the number and distribution of studies published on serverless computing in the period (2016–2020)?
RQ2. Which researchers, organizations, and countries are active in serverless computing research?
RQ3. What are the differences between serverless computing and traditional cloud computing?
RQ4. What are the benefits of using serverless computing?
RQ5. What are the most used software platforms that enable serverless computing in the literature?
RQ6. What are the application areas of serverless computing in the literature?
RQ7. What are the challenges and issues of using serverless computing?
RQ8. What tools are available for serverless computing? (serverless tools)
RQ9. What are the available research approaches to analyze the migration of monolithic applications to serverless computing?
RQ10. What are the potential future directions of research on serverless computing?
Search strategy
Literature sources.
In this study, five standard online databases have been selected as sources that index the literature of software engineering and computer science. These sources are presented in Table 1 .
Search string
To find the publications relevant to this study, the following extensive search string has been applied on the database sources of literature:
(serverless OR FaaS OR “function as a service” OR “function-as-a-service”) AND (computing OR paradigm OR architecture OR model OR application OR function OR service OR platform OR programming)
To obtain the best publication list, a generic search string is created. It contains serverless cloud computing-related keywords. The string with duration (2016 - 2020) have been applied to all libraries. Because the Springer Link library covers many fields, the result of search was greater than other libraries. This because the keyword FaaS is used in many research areas for different purposes. For instance, fish as a service (FaaS) and FPGA as a fervice (FaaS). Therefore, we used Computer Science subject filter with Springer Link, ScienceDirect, and Scopus to reduce the number of incorrect papers. The results of the initial search are shown in Fig. 2 . Additionally, some inaccurate results have been obtained due to the partial similarity to FaaS, such as the federal aviation administration (FAA). The results of the initial search were 5,021 papers in total.
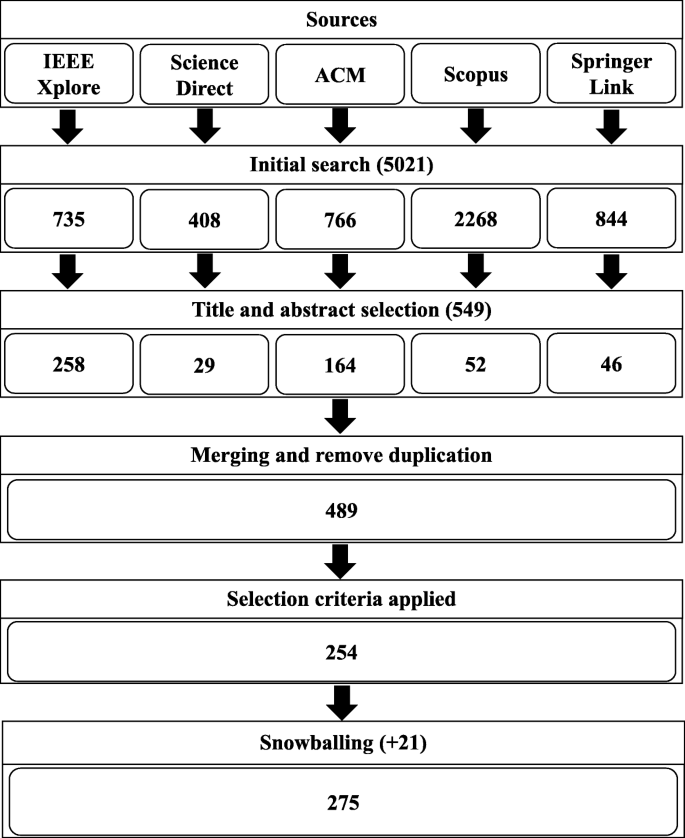
Results of papers selection process
After obtaining the initial list of publications, some filters have been applied to reduce the number of incorrect results based on their relation to the serverless computing and FaaS topics. Most of the papers have been analyzed based on the title and abstract. However, when we were unable to make a decision based on the title and the abstract, we read the content of the paper to ascertain whether to include or exclude. As a result, the list of papers which are related to serverless computing has been decreased to 549 papers.
After filtering the papers based on the title and abstract, we merged all the papers that were relevant to serverless cloud computing, which was 549 papers into a single dataset. Then we removed the duplicated papers based on the combination of a title, author names, publication year, and venue. Thus, the number of publications has been reduced to 489 papers.
Then, the publications have been selected based on the content of the paper and based on a set of inclusion/exclusion criteria (see the following section) that have been selected carefully. Eventually, we could obtain 254 papers that are related to serverless cloud computing. In the next step, we applied backward snowballing to increase the set of relevant papers to serverless cloud computing. In this phase, we could add 21 more papers to our list of papers. As a result, the total numbers of relevant papers become 275 papers. The list of these papers and its meta-data have been published in Zenodo website as a dataset [ 32 ].
Paper inclusion/exclusion criteria
To decide whether a publication is relevant to the scope of this research, a set of inclusion and exclusion criteria have been established and employed as follows:
Inclusion criteria:
Publications in the field of software engineering and computer science.
Publications published online from 2016 – 2020.
Publications related directly to serverless computing.
Exclusion criteria:
Publications not published in English.
Publications without accessible full text.
Publications not formally peer reviewed (e.g., gray literature).
Publications not published electronically.
Publications that are duplicates of other previous publications.
The selected publications were carefully read to answer the raised RQs. Here, a short title is used to represent each RQ. The following subsections present and discuss the results based on each RQ.
Distribution of publications (RQ1)
Publication frequency.
All the selected papers of this study were analyzed to determine their frequency and evolution. Figure 3 shows the results of this analysis. The results show that the average number of publications per year is approximately 55 papers.
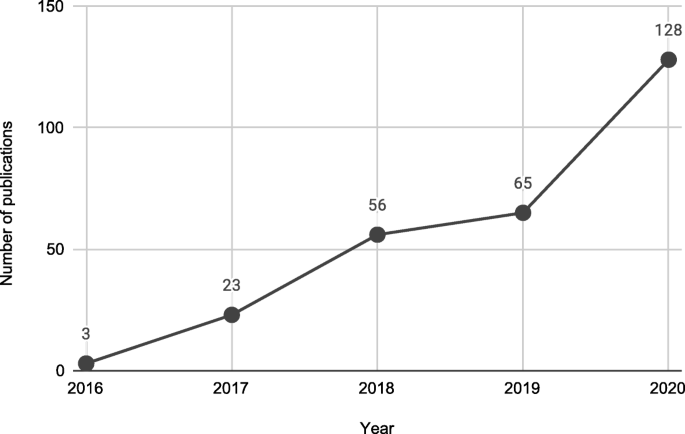
Published papers per year
Serverless computing has trended a significant engagement over the past two years. This boost has been caused by industry, academia, and developers for several reasons. The first important reason is the attractive engagement opportunities that serverless offers cloud providers. Serverless nature equipped cloud providers with more convenient and efficient methods to manage and utilize idle computing resources. Another reason is that the billing is only on the basis of function execution time and resource allocation. Also, the developers are not required to be aware of the underlying infrastructure and workflows. Hence, this attracts cloud providers and businesses to migrate and support serverless alongside many directions. At the same time, researchers are paying more attention to serverless as it is becoming the future paradigm for cloud computing. Moreover, current challenges and limits in serverless computing draw attention to more academics to address the issues and enhance the currently available features. For the aforementioned reasons, developers and customers are well encouraged and satisfied to select serverless computing for developing applications and services.
Publication venue
The distribution of the selected papers in various publication venues is shown in Fig. 4 . The percentages of publications in conference papers, workshop papers, symposium papers, and journal papers are approximately 62%, 11%, 14%, and 13%, respectively. However, almost 13% of the studies have been published in journals, which indicates the immaturity of research in serverless computing [ 33 , 34 ]. It is worth mentioning that some conference papers were published as book chapters. Thus, the original venues, which are conferences, of such papers were considered.
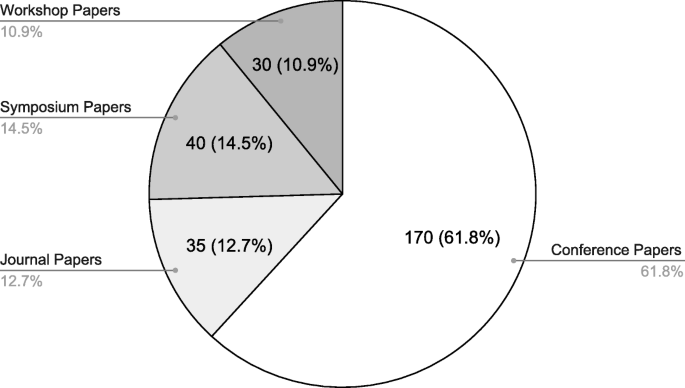
Published papers ratio per each venue
Following the interpretation of publications, the most productive and primary journals, symposiums, conferences, and workshops venues related to serverless computing can be clarified. Due to their long names, abbreviations are used in this paper. The active journals are shown in Fig. 5 and their full names can be found in Table 2 . It can be observed from the figure that the top and vital three journals are “FGCS”, “IoT”, and “JSS”. Also, it can be noticed that the top three journals contain almost 34% of the published journal papers, while the others own approximately 66%.
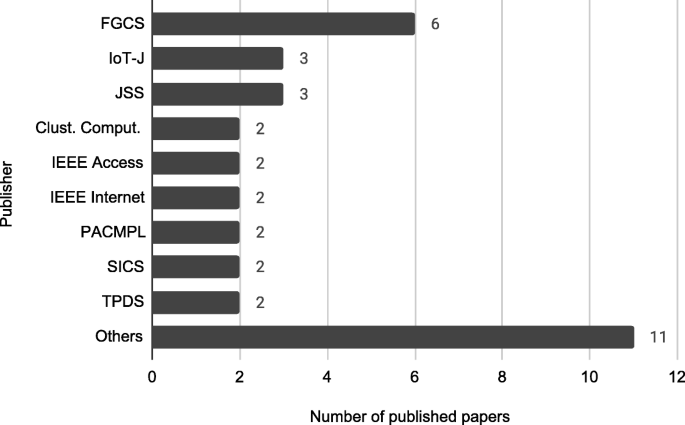
Published papers vs. journal name
The active conferences are shown in Fig. 6 and their full names are presented in Table 3 . The “WOSC”, “Cloud”, “UCC”, “SoCC”, and “Middleware” are considered the most active conferences that hold approximately 28% of the published conference papers. By including other conferences with three published papers or more, then approximately 23% of the conference papers are published in annual conferences. The majority (almost 49%) of the conference papers were published at individual conferences, which are denoted as “Others” in Fig. 6 .
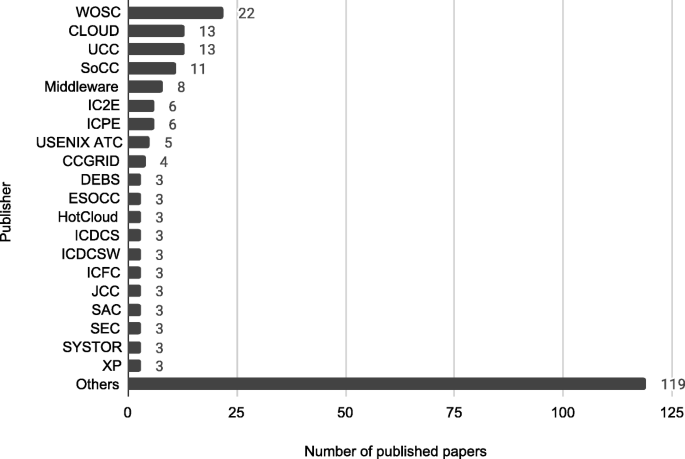
Published papers vs. conference name
Active researchers (RQ2)
Serverless computing is a vital research area through the contribution of several scholars. Yet, the researchers are counted active if they contributed to more than two research studies, as presented in Fig. 7 . The figure shows that the top six active researchers are “Pedro Garcáa López”, “Erwin Van Eyk”, “Alexandru Iosup”, “Marc Sánchez-Artigas”, “Sebastian Werner”, and “Wes Lloyd”. Table 4 presents the active nations, research institutions, researchers, references to the published papers, and the total number of publications.
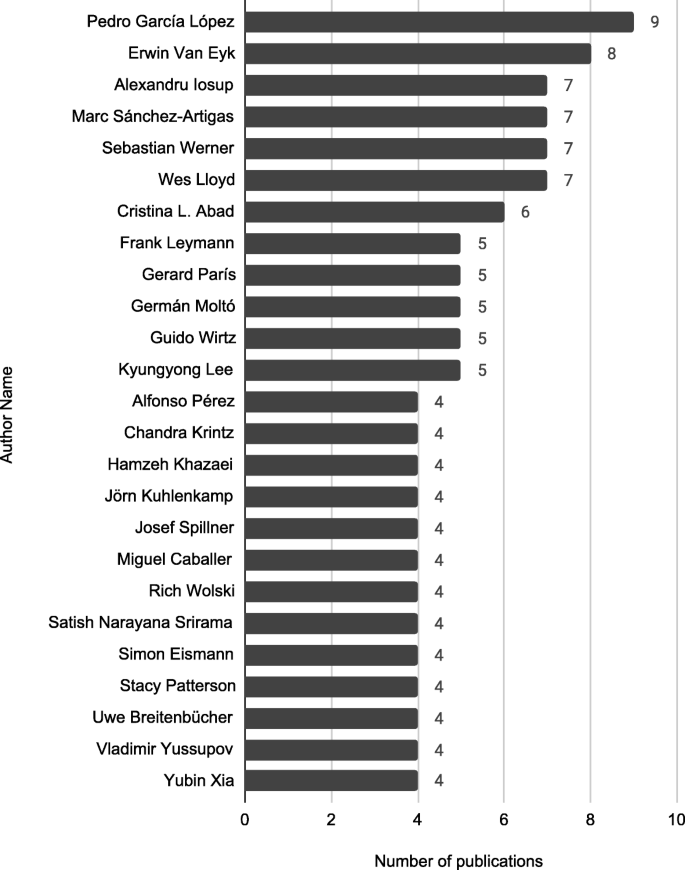
Active researchers based on the published papers
The active nations in the number of papers are obtained from the information presented in Table 4 by extracting the institutional affiliation of the authors and co-authors. An overview of the most active nations and the total number of publications is shown in Fig. 8 . It is observable that the United States and Germany are the largest contributors to papers published on serverless computing with 104 and 39 published papers, respectively.

Active countries
Serverless computing vs. traditional cloud
computing (RQ3) There are several differences between serverless and traditional cloud computing. In the traditional cloud architecture, the server acts as a monolithic system containing all business logic. Meanwhile, the serverless architecture is modeled into smaller, event-driven, and stateless ‘triggers’ (events) and ‘actions’ (functions) [ 175 ]. Each component handles different pieces of data and runs independently [ 176 ]. Spreading business logic into smaller functions increase the development efficiency [ 77 , 177 ] and also decreases the chance of a single point of failure [ 77 ]. On the other hand, the component dependency within monolithic applications affects the availability of other services adversely.
In a serverless architecture, the developers are unable to take control of listening to the TCP socket, managing load balancers, maintenance or configuration of the server, as well as provisioning and resource allocation. Therefore, there is no need for system administrators; the developers only focus on handling client requests and paying attention to deliver valuable services [ 8 ].
Serverless computing also differs from monolithic computing as the functions have shorter life cycles.
The traditional monitoring and debugging tools that are used in monolithic applications are not included in the serverless architecture; the developers are compelled to use built-in tools for debugging and monitoring. The computing power is no longer a concern for the developers in the serverless paradigm, as it could scale horizontally almost indefinitely [ 178 , 179 ]. Meanwhile, in the client-server architecture, it usually requires dedicating two server instances; the primary instance and a second in case if the former fails. This leads to higher costs in the monolith paradigm. Serverless architecture could be more economical for unsteady load conditions while the server-based is more suitable for steady loads [ 152 ]. As serverless applications scale up and down according to the requests, thus, unlike the traditional systems, it is unnecessary to keep the sessions in the memory [ 8 ]. Hence, it is difficult to keep track across requests.
FaaS boosts the security level as cloud providers continuously update their infrastructure with the latest security patches; this also removes the security burden on developers [ 17 ]. Directly accessing the backend resources in the traditional model is considered a critical security issue. Thus, any requests from the clients and internal functions in the serverless environment must go through a distributed request-level authorization mechanism that strengthens the security level [ 8 ]. Additionally, denial of service (DoS) attacks are controlled, as it is more difficult to attack distributed servers than a single server [ 175 ]. However, some security concerns remain due to the third-party API usage [ 9 ]. Besides, there is a lack of tools to identify vulnerabilities and access control risks. Table 5 summarizes the aforementioned differences.
Benefits of serverless computing (RQ4)
Serverless computing offers numerous benefits to its users, and Table 6 presents papers that states these benefits. This section summarizes those benefits as follows:
Cost effective
Serverless applications are abstracted from server infrastructure, which results in cost-based services depending on usage [ 180 ]. For example, applications run whenever a user makes a request to a service within the application. The cloud vendors charge only for the used space, and there is no cost while their applications are in an idle state.
Scalability
Serverless reasonably solved the resource allocation problem [ 191 ]. Therefore, developers do not have to concern themselves with the application scalability, because the application will scale automatically whenever user application requests are increased. If there are numerous requests to a function within the application, the serverless providers will start servers to handle these requests.
Server-side management
In serverless computing, developers do not need to concern themselves with the server-side and its management. Serverless cloud providers take care of managing and maintaining the hardware and software required to deploy applications. In addition to that, they handle all administration operations to let developers focus on different kinds of resources such as central processing unit (CPU), memory, and storage.
Easy to deploy
Serverless applications are easy to deploy. For example, to deploy an application, developers only need to upload some functions and release a new product. The serveless will take care of deployment management and infrastructure related concerns such as server provisioning and scaling.
Decrease latency
Serverless applications are not hosted on a specific server; the code can run from any server in any location. Therefore, cloud vendors can run the application on servers near the end user’ location. This reduces latency, because end user requests do not have to travel across the Internet to access the original server.
Serverless platforms in the literature (RQ5)
The software platforms are generally implemented to deal with resources from several clouds and ensure proper running of client applications. The heterogeneous nature of the cloud providers’ infrastructure (hardware and operating systems) led to the necessity to direct the developers’ focus to the functional part, rather than the underlying infrastructure [ 199 ].
With the emergence of the first serverless platform, AWS Lambda by Amazon in 2014 [ 8 ], cloud computing has evolved to a new generation referred to as serverless computing. However, serverless was not a brand-new paradigm; it emerged after the advancements in adopting virtual machines and container technologies [ 120 ]. By 2016, other competitors, namely Google, Microsoft, and IBM followed the trend. Several commercial and open-source platforms offer serverless computing. The well-known commercial systems are AWS Lambda, Google Cloud Functions, and Azure functions. Alternately, there are several open source platforms available including IBM Cloud Functions, and Apache OpenWhisk.
There are several criteria to help developers in selecting a serverless platform: cost, performance, supported programming languages and model, deployment easiness, easiness in composing functions from different providers, security, and monitoring and debugging tools [ 184 ].
Table 7 presents the serverless platforms used in the considered papers of this study. It can be noted that “AWS Lambda”, “Apache OpenWhisk”, and “Azure Functions” are the most used platforms with 78, 23, and 11 published papers, respectively. However, it is worth mentioning that each platform has its own set of features and differs from others.
The application areas of serverless computing in the literature (RQ6)
Serverless computing can be utilized in a number of application areas as follows:
A chatbot application is developed using serverless computing, which enables interaction with users via voice or text commands. Within this application, six functionalities have been implemented, namely the Date, News, Jokes, Weather, Music Tutor, and Alarm Service. For example, a user can ask for the current date using a voice or text command. The request is routed to a required serverless action on OpenWhisk for further processing. The Date action is activated via the issued command and retrieves the current date to the user in the form of text or voice [ 44 ].
Another example is the ticketing chatbot service developed using serverless computing and natural language processing (NLP). The architecture of the system consists of three parts: (1) the node.js Webhook, which works based on hypertext transfer protocol (HTTP) POST or GET requests (2) Wit.AI, which is a NLP service (3) Ticket.com, which is a ticketing order API. For example, when a user books a flight ticket; a specific function on Webhook will be activated, which routes the user query to the Wit.AI service. Wit.AI will process the query and extract useful parameters from the request such as destination, date, and time, then send it back to Webhook. After receiving the processed query from Wit.Ai; another action will be triggered and pass the processed query to Tickt.com API to retrieve flight information such as the flight number, airline name, departure time, and ticket price from several airline companies. Finally, Webhook will provide flight information to the user [ 44 , 179 , 248 ].
Information retrieval
A search engine web-based application is developed based on serverless architecture. Search engine functionalities are implemented as Amazon lambda functions. The search engine executes all the details of retrieval processing after receiving the user query (e.g., tokenization, stop-word removal, term weighting, similarity calculation, and ranking). Then, it sends back the results to the user as documents stored in the DynamoDB database to be accessed using the web application interface [ 173 ].
File processing
Serverless computing can been utilized in file processing applications [ 119 , 249 ]. For instance, in [ 119 ] a model for highly parallel file processing applications based on serverless architecture is proposed. This model provides users with different ways to process their files.
The first method is by using the API gateway. In this method, users submit files using the HTTP request employing the API gateway to a lambda function to process the file (e.g., medical images and video files).
The second method is by uploading/reading files to the Amazon simple storage service (Amazon S3) bucket. This method provides the user with three different ways to execute a lambda function using S3 buckets: (a) by uploading a file to S3 buckets. When the file is uploaded, S3 creates an event to invoke a lambda function; (b) by copying a file from another bucket to the bucket linked with the lambda function. This will cause the trigger of an event from S3 to invoke a lambda function as in the previous manner; (c) by specifying a bucket where the files to be processed are stored. Then, for each file found, the lambda function is invoked in parallel using an S3 bucket.
The third method is by specifying the output file. By this method, the user can set a chain of lambda functions to be invoked by S3 buckets. In this case, the user defines the input/output buckets for each of the lambda functions. Thus, the output bucket can be used as an input to another lambda function [ 119 ]. Here, serverless functions can handle different types of data (stored in files) such as sensory, textual, and biological data [ 200 ]. Also, many preprocessing operations using NLP may be applied to data files before processing, such as stemming and noise removal [ 78 ].
A MATLAB simulation scenario is created to illustrate the use of the smart grid with serverless cloud computing to control and manage electrical loads (devices). In this scenario, the Simulink tool is employed for simulation. A MATLAB program is developed to indicate the start and end of the simulated grid model via a batch file. The batch file is used to upload grid model data generated by the program to Amazon S3. Afterwards, a lambda function in the serverless side will be activated to process the uploaded data, and subsequently the result will be sent back to the batch file as a response. In return, the program will read continuously the response from the batch file and interpret its content to manage the electrical switch (loads) [ 201 ].
Also, An electrical overload warning system is implemented in the smart grid, based on serverless architecture. The system uses some Amazon web services, including S3, lambda functions, simple notification service (SNS), and CloudWatch. S3 is used as a storage service in the system. Lambda functions constitute a computing service that executes the code of the application. CloudWatch is a monitoring tool that monitors AWS resources and applications. The SNS is a notification service that sends and receives notifications.
The main sections of this warning system consist of data collection, data acquisition, data analysis, data mining, conclusion verification, and conclusion publishing. In this architecture, the AWS Lambda is used in data analysis and data mining. AWS CloudWatch is used for data conclusion verification. The SNS is used to generate alarms. For instance, the data is uploaded to S3, and subsequently, a lambda function is activated for data analysis and data mining. After the lambda function execution, its log data is stored in CloudWatch logs. CloudWatch is used for conclusion verification. CloudWatch defines an alarm size to a specific value, upon which it compares the value of log data with a predefined alarm size to check the current state. Then, the CloudWatch uses SNS for publishing conclusions. If the receiving data is greater than the alarm size, an alarm signal will be triggered and send an email via SNS [ 5 ].
An automated threat detection system is introduced using serverless cloud computing and Kubernetes. Kubernetes is an open source system to automatically deploy and manage application containers [ 243 , 250 ]. The system deals with threats (e.g., software vulnerabilities and insecure configurations) automatically based on user-defined policies. The system includes a vulnerability scanner (VS), which is a thread detection component. Whenever users deploy new application containers, the containers are registered with the VS, and a scanner agent is installed. When a thread is detected by the scanner, a notification is sent to the OpenWhisk component, which activates a serverless function that takes actions to reduce the threat. OpenWhisk will invoke a Kubernetes API extension and let the security enforcement operator (SEO) handle the operation [ 35 ].
Serverless cloud computing has been employed in different networking domains[ 175 , 188 , 251 , 252 ]. In [ 188 ], a variety of networking fields including software-defined networking (SDN) which can utilize advantages of serverless computing architecture have been discussed. The SDN is a network architecture approach that enables the network to be manageable and adaptive. This architecture separates the network control plane from the forwarding functions (the data plane). This decoupling enables network switches to become a simple forwarding device, and the network control is implemented as a network application that executed on a logically centralized controller. Serverless computing can be used in the SDN controllers. These controllers can be implemented as independent functions deployed on serverless platforms. For example, when a packet arrives to the SDN forwarding device, the device will parse the packet header and forward it to the SDN controller. The functions within the SDN controller will be activated then it will determine what action to be taken with the packet. After that, it will send the information to the forwarding device. The action might be modifying the header, dropping the packet, etc.
Serverless computing has been utilized in many IoT applications, as shown in Table 8 . For example, a camera can be installed to monitor a house, after which processing images captured by the camera can be performed by some serverless functions provided by the OpenWhisk platform. When a camera detects an interesting object such as a car or a human, the camera sends its pictures to the serverless platform for further processing. To extract features, a serverless function is called to perform feature extraction and then reports its status to the users [ 232 ].
Edge computing
Serverless cloud computing and edge computing have been used to build different kinds of applications, as presented in Table 8 . For instance, the authors of [ 217 ] have implemented an autonomous mobile robot (AMR) system based on serverless computing and edge computing. The system consists of three main components: an AMR with NVIDIA Jetson TX2 module for edge computing, a serverless architecture based on AWS, and a cross-platform mobile application developed using React Native. The main idea of the system is to deliver a package to a user. For example, the user will interact with the mobile application to send a package. Once the delivery request has been received from the user, the AWS IoT can activate related lambda functions, such as position coordinate. Then, the AMR would start its mission, sending the package to the receiver’s location. Also, facial images were regularly retrieved by AWS lambda to identify the receiver’s face. Finally, the task is completed when the receiver’s identification is confirmed [ 217 ].
Serverless computing challenges and issues (RQ7)
Studying the literature reveals a number of challenges and issues posed by employing serverless computing. These challenges cover the functional and non-functional aspects of serverless computing as follows:
Cost and pricing model
Cost is a fundamental challenge; therefore, serverless computing providers should reduce the usage of resources to the minimum, while functioning in both execution and idle states. Further, the pricing model is another challenge in serverless computing compared to other cloud computing approaches. For example, the CPU bound is cheap, whereas the input/output (I/O) bound functions may be more expensive from dedicated servers. Table 9 presents papers that investigate issues on cost and pricing models in serverless cloud computing.
Serverless computing can scale to zero while there is no request for functions and services. Scaling to zero leads to a problem called cold start. A cold start occurs when serverless functions remain idle for some time, and the next time these functions are invoked, a longer start time is required. Methods and techniques to reduce the cold start problem are crucial as a result, many papers have been studied this problem, as shown in Table 9 .
Resource limits
In serverless computing, resources are required to ensure that the platform can deal with load increasing. This includes CPU usage, memory, execution time, and bandwidth [ 94 , 202 , 210 , 235 , 280 ].
Security is the most challenging issue in serverless cloud computing. One of the security issues is isolation, because functions are running on a shared platform by many users. Therefore, strong isolation is required. Another security issue is trust when it comes to process-sensitive data. The serverless applications work with many system components, which must function correctly to maintain security properties. Table 9 presents several papers associated with serverless security.
Serverless computing must ensure function scalability and elasticity. For example, when many requests are issued to a serverless application, these requests should all be served and the used serverless cloud provider should provide the required resources to process all these requests and should scale up with the number of requests [ 210 , 280 , 281 ].
Long-running
Serverless computing runs function in a limited and short execution time, while there are some tasks might require long execution time. This does not support long execution running, since these functions are stateless, which means that if the function is paused it cannot be resumed again [ 11 , 202 , 234 , 280 ].
Programming & debugging
There is currently a lack of debugging tools. Further, monitoring tools are required, since developers need to monitor the application and observe how functions are working. More advanced integrated development environments (IDEs) are needed, so developers can perform refactoring functions, such as merging or splitting functions, and reverting functions to the previous version. Moreover, logs from serverless function invocations need to be sent to the developer and provide detailed stack traces. When an error occurs, a good method is required to report details on the occurrence to the developer. The equivalent of a stack trace for serverless computing is currently not available. Table 9 shows many papers that consider programming and debugging challenges and issues.
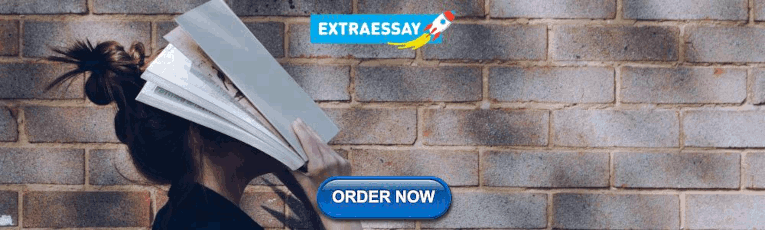
Vendor lock-in
The FaaS paradigm separates the code from the data, which leads the functions to depend strongly on the could provider’s ecosystem for storing, obtaining, and transferring data [ 282 ]. This issue makes the customers dependent on the serverless provider for products and services, and the customers cannot easily use different vendors in the future without substantial cost. Thus, customers have to wait on the serverless provider for additional services [ 9 , 130 , 202 ].
Performance
Serverless computing has many performance challenges and issues such as scheduling and service calling overhead. For instance, scheduling means when a serverless function is activated in response to an event this function should be mapped to a specific resource (e.g., container or VM) to be run. The resource can have a significant effect on performance based on available resources, location of input data and code, load balancing, etc. Table 9 shows papers related to serverless performance.
Fault tolerance
It refers to a system that continues working and provides its services despite the failure in some components. It mostly occurs when some containers fail. To overcome this challenge, a basic retry mechanism is used [ 11 , 210 , 235 ].
Function composition
Serverless cloud vendors provide users the ability to deploy small stateless functions to the cloud to handle a specific task. However, some complex tasks require multiple functions to work with each other collaboratively to be performed. Therefore, more research needs to be done on how function composition can be used effectively and efficiently in serverless cloud computing [ 11 , 38 , 235 ].
Resource sharing
Functions in serverless cloud computing share resources to achieve inexpensive cloud computing. Sharing resources among functions and other serverless components is a challenging task. Therefore, good techniques are required to be investigated to achieve this goal [ 98 , 210 , 283 ].
A serverless application consists of many small functions. These functions work together to accomplish the application’s functionality. Therefore, integration testing for these functions is a crucial issue to make sure that the application works properly [ 9 , 84 , 284 ].
Naming and addressing system
Users deploy functions to serverless cloud computing to solve problems. These functions cannot listen to network communications. The existing serverless cloud computing frameworks do not support this feature. Instead, they use third party services such as Amazon S3 to communicate with other functions. Therefore, finding the internet protocol (IP) address of a function by other functions and services is a challenging issue in serverless cloud computing [ 98 ].
Legacy systems
Legacy systems refer to old technologies, techniques, hardware, and software systems that are still in use. It should be possible to reach these systems from serverless cloud computing. Also, these systems might be required to be transferred to cloud computing. Therefore, more work needs to be done on the migration process and how the functions can be extracted from legacy systems to be deployed as serverless cloud functions [ 84 , 119 , 120 , 210 , 280 ].
Managing hybrid cloud
In a hybrid cloud, a developer may deploy an application to different clouds (hybrid cloud). For example, if some functions of an application are on a specific serverless cloud vendor and others are hosted on other public clouds; then, managing these functions and their interactions is a challenging issue [ 84 , 210 , 280 ].
Lack of quality of service (QoS) support
Existing serverless platforms and frameworks do not provide users the control over the QoS of serverless functions [ 235 ]. Cold starts, queuing, and orchestration are the main reasons affecting the QoS in serverless computing [ 8 ].
Architecture complexity
A serverless application may consist of several functions; the number of functions increases the complexity of the architecture. Managing these functions and services related to the application also leads to a complex architecture [ 9 ].
Interactions tracking
Stateful requests are usually used by real-life applications. It means deployed systems keep track of the state of users’ interactions and store them on the server-side for further uses. However, in stateless serverless functions, it is not obvious how these functions will handle and manage the states of each user [ 210 , 280 ].
Concurrency management
Concurrency means a function can handle any number of requests whenever a function is invoked. For example, if a request has been made to a serverless function, the function will process that request. However, if another request has been made to that function and the function is still processing the previous request, then the serverless should provide another instance of that function to serve the new request [ 210 , 280 ].
Support for heterogeneous hardware
Existing serverless platforms may not support some specialized hardware such as graphics processing unit (GPU) and field programmable gate arrays (FPGAs). This is a challenging issue for vendors to provide support for heterogeneous hardware [ 210 , 280 ].
Tools available for serverless computing (RQ8)
Nowadays, various providers strive to facilitate the adjustable use and allocation of machine resources on the cloud [ 9 ]. Likewise, plenty of supportive tools and services are aiding developers to more efficiently manage and deploy applications using serverless computing. Serverless computing is auto-scalable, reliable, and easily accessible [ 203 ]; for these reasons, big cloud providers such as Amazon, Microsoft, Google, IBM have realized the importance of offering frameworks, IDEs, software development kits (SDKs), function development kits (FDK), migrating mechanisms, logs, and monitoring tools to enhance and simplify the development, testing, deployment, and monitoring of serverless applications [ 17 ]. For instance, Amazon offers Cloud9 IDE for local deploying and testing [ 205 ].
Apart from the cloud providers’ specific tools, plenty of third-party tools exist for the developers. With the concept of these tools, developers can build and deploy applications on multi-cloud providers. Developers are also able to control platforms and resources by programming. The advantages of this are linking the applications with auto-scaling controllers and including advanced self-mechanisms into the code to automatically configure, secure, optimize, and recover the cloud applications. The core advantage of this feature is the acceleration in applying changes to the application environment [ 272 ].
There are several tools available to model serverless applications, which are based on deployment models as either imperative or declarative. The imperative model defines the execution steps to obtain a specific deployment task. While the declarative model describes the structure of a desired application deployment. However, to fully benefit from employing a serverless architecture, cloud providers should address issues that have arisen with the use of a serverless paradigm. For instance, debugging tools are unable to track and identify the exact reason behind errors [ 44 ], as most of them are limited to what cloud providers offer [ 179 ]. Although many powerful tools have been mentioned in this study and can be used in serverless computing in real scenarios, there is still a great opportunity to develop further tools and services.
Migration of monolithic applications to serverless computing (RQ9)
The nature of most existing applications is monolithic. Monolithic applications have several drawbacks; they are characterized by continuous growth in complexity and size over time.
The bigger size of the monolithic applications leads to slower startup time. Moreover, novice developers face difficulties in digesting the traditional programming paradigm. Economically, monolithic systems take more effort to be developed and debugged. Furthermore, integrating the latest technological development into monolithic systems is a tough and expensive process. Generally, monolithic applications are designed to be tightly coupled – the entire application will be unable to run or compile if one component is missing or fails [ 128 ]. It is also difficult to scale the application when multiple components have limited resources.
Another drawback is that updating any component will require redeployment of the entire project. The migration process to serverless computing involves transferring the legacy application code to serverless functions. This process could be more efficient and functional in applications with less size [ 76 ].
The key challenging aspect of migration is about extracting the serverless computing from the monolithic systems. There are several approaches to accomplish this task, one of them is Lift and shift [ 205 ]. This technique transfers the whole infrastructure to the cloud, however, this method also brings the already existing problems within the source to the destination. In [ 205 ] the authors proposed toLambda to automatically refactor, test, and deploy the monolithic applications (Java) into microservices (AWS Lambda Node.js). While rebuilding the legacy application from scratch is recommended for applications that no longer depend on the existing cloud services [ 130 ].
However, not all applications are suitable for migration to serverless computing [ 76 , 128 ]; therefore, the first important aspect to be considered before rebuilding the applications is whether it would save money [ 188 ]. For such cases, newly desired features could be implemented and added via serverless computing as an extension to the current systems [ 128 ].
The other approach is to refactor the entire legacy code into FaaS services. During the migration phase, it is crucial to address the coupling of the systems not only in the application logic but also in the databases, as more functions will call the same database. However, migrating the server-side while keeping the user interface could lead to problems. Moreover, the client cannot obtain integrated data by a single request. As the functions are decoupled into smaller entities, the server is unable to aggregate data from different entities. Thus, it is the client’s responsibility to call the necessary entities to achieve this task [ 76 ].
Future directions of research (RQ10)
As the evolution of serverless computing is relatively new, there are several research paths available to be focused on as follows:
Function startup
One of the major research opportunities is overcoming the cold start problem without affecting the primary feature of serverless which is scaling to zero [ 160 , 188 ]. The first call of functions needs initializing the required libraries, which will cause a cold start. To bypass this, the computing resources will be warm for a certain time. Hence, upcoming requests will be handled faster. This could be performed via enhancing scheduling policies and developing more accurate function performance measurements [ 86 ]. Serverless providers follow their approaches to keep the functions in the warm pool. However, most of them are based on the number of requests for a certain time. Thus, if a function is not called frequently, it will suffer again from the cold start.
Very few studies such as [ 272 ] suggested a periodic event scheduler for Kotless (a serverless framework for Kotlin) which will trigger a list of warm functions every few minutes. The authors of the study claimed that this will reduce the cold start without bringing extra costs. While in [ 233 ] argues that pre-warming methods are unnecessarily using resources with idle containers. The researchers are still working to avoid cold start by reducing high delayed function startups via optimizing compute resources [ 11 ].
Recycling and rebalancing minutes and hours of idle runtime is an expensive process for cloud providers. Therefore, reducing the cold start penalties will help cloud providers in the first place and hence customers. The authors in [ 202 ] proposed FaaStest an autonomous approach based on machine learning to capture the function call behavior and then dynamically select the optimal ones. This technique could reduce the cold start by 90%. They proposed a strategy to predict functions invoking time and warming the function using fine-grained regression method [ 285 ]. However, overcoming the issue of function startups is still considered as a research direction to be more investigated.
Keeping a guaranteed QoS level in the software level agreement (SLA) that describes the lower service level offered by the service providers [ 166 ] is a major obstacle for cloud providers to offer optimal performance metrics [ 167 , 207 ]. However, serverless frameworks should consider the objectives of both providers and users [ 242 ]; customers and developers have none or little QoS support over the functions [ 236 ]. In addition, the auto-scaling feature lacks QoS guarantees. This lack of QoS affects the performance of serverless applications. Increasing response time leads to decreasing the QoS level [ 207 ]. It also raises the cost of the service [ 236 ]. Therefore, achieving an ideal resource allocation management is a complicated and challenging task as several objectives should be fulfilled together [ 209 ]. Hence, providing more efficient QoS management of functions by the auto-scaling is essential to be considered without degrading the fault-tolerance features and increasing the cost.
Pricing is crucial for both customers and cloud providers. However, there is a shortage in pricing models, as there is an imbalance in needs between serverless providers, developers, and service end-users [ 236 ]. The pricing scheme for most cloud providers is based on the number of functions’ requests and execution time-the quantity of consumed resources [ 123 , 200 ]. Currently, FaaS is less expensive when functions are bound to I/O than CPU. Moreover, services that dynamically adjust resource consumption are unable to predict the optical computing technology. It is crucial to implement solutions that offer cost-effective computing resources. FaStest reduced the cost by 50% via learning the behavioral pattern of functions using machine learning [ 202 ]. Price estimation has a great impact on selecting the most optimal provider. Therefore there should be more researches on developing tools to predict the pricing in advance.
Since the serverless emergence, researchers are working on the open question of how to decompose legacy systems into FaaS without degrading performance [ 208 ]. Several works have been done on migrating to FaaS [ 76 , 130 , 286 ]. The currently available automated tools for migrating legacy code into FaaS are not fully practical due to the remaining manual work that needs to be done [ 17 ]. Therefore, finding optimal automatic migration solutions for existing legacy systems is an interesting research direction [ 130 ]. Moreover, research on tools for checking whether a legacy system will fit the serverless paradigm is a crucial line. Also, developing and enhancing automatic and semi-automatic analysis strategies based on artificial intelligence could be another future research field.
Debugging, testing, and benchmarking
The available tools for testing, debugging, and deployment are immature, this prevents some developers from entering the serverless environment. The shortage of tools in FaaS is a core problem, particularly the testing tools [ 17 ]. Moreover, most FaaS environments lack powerful local emulation platforms for testing. Therefore, developers are mostly depending on the server-side, which is expensive. Developers need to be ensured about the adequate testing tools before diving into the serverless world. A challenging aspect in benchmarking is the lack of information due to the heterogeneity of the cloud provider data center: hardware, software, and configurations [ 287 ]. Additionally, benchmarking FaaS platforms should take advantage of analyzing the cloud services, which lacks limited accessible measurements and hidden modification of services over time [ 55 ]. Thus, it is essential to have transparent, fair, and standardized benchmarking tools available for developers.
Threats to validity
Several threats might impact the validity of the literature mapping studies. In this paper, popular instructions and guidelines were taken into account to avoid threats to validity as follows:
Coverage of research questions: All up-to-date research aspects of serverless computing might not be included in this study. To overcome this threat, the brainstorming was conducted by all the authors in determining the most current research questions in the area.
Coverage of related papers: The process of obtaining all the related studies in serverless computing cannot be secured. In this study, various literature databases were employed; moreover, the method based on different terms and synonymous is followed by all the authors in determining the related questions.
Paper inclusion/exclusion criteria: The individual bias and interpretation could affect the implementation of the criteria. Therefore, to solve this problem, the agreements of all authors were considered in excluding or including a paper.
Accuracy of data extraction: The individual experience effects extracting the data, therefore online meetings were conducted after the data extraction process by each author. During the meetings, the outcomes from each author were compared with other findings to determine the differences and reach a final consensus.
Reproducibility of the study: Whether other researchers could obtain similar outcomes of this study is another threat. Thus, to address this, the research methodology contains the well-explained steps and actions conducted in this paper (as shown in “ Research methodology ” section).
Conclusions
The contributions of the work presented in this paper are threefold: (a) a methodical review of related literature on the topic of serverless computing, to address the issue of the lack of compiling information on the state-of-the-art of the field; (b) a comparison of the platforms and tools used in serverless computing; (c) an extensive analysis of the differences, benefits, and issues related to serverless computing, to provide a more complete understanding of the topic. Given the fast evolution and growing interest in the field, this survey focused on gathering the most outstanding trends and outcomes of serverless computing, as described by recent researchers. This survey could significantly reduce ambiguity and the entry barrier for novice developers to adapt to the serverless environment. Furthermore, the findings presented in this study could be of great value for future researchers interested in further investigating serverless computing. Finally, it is worth mentioning that the interest that both commercial and academic efforts fueled into studying, developing, and implementing serverless tools in forthcoming years could help maximize the potential that serverless computing could bring to the IT community.
Availability of data and materials
Not applicable.
Großmann M, Ioannidis C, Le DT (2019) Applicability of serverless computing in fog computing environments for iot scenarios In: Proceedings of the 12th IEEE/ACM International Conference on Utility and Cloud Computing Companion (UCC ‘19 Companion), 29–34.. Association for Computing Machinery, New York. https://doi.org/10.1145/3368235.3368834 .
Google Scholar
Boza EF, Abad CL, Villavicencio M, Quimba S, Plaza JA (2017) Reserved, on demand or serverless: Model-based simulations for cloud budget planning In: 2017 IEEE Second Ecuador Technical Chapters Meeting (ETCM), 1–6. https://doi.org/10.1109/ETCM.2017.8247460 .
Villamizar M, Garcés O, Ochoa L, Castro H, Salamanca L, Verano M, Casallas R, Gil S, Valencia C, Zambrano A, Lang M (2017) Cost comparison of running web applications in the cloud using monolithic, microservice, and aws lambda architectures. SOCA 11(2):233–247. https://doi.org/10.1007/s11761-017-0208-y .
Article Google Scholar
Jonas E, Schleier-Smith J, Sreekanti V, Tsai C-C, Khandelwal A, Pu Q, Shankar V, Carreira J, Krauth K, Yadwadkar N, Gonzalez JE, Popa RA, Stoica I, Patterson DA (2019) Cloud Programming Simplified: A Berkeley View on Serverless Computing. http://arxiv.org/abs/1902.03383. Accessed 6 Jan 2021.
Geng X, Ma Q, Pei Y, Xu Z, Zeng W, Zou J (2018) Research on early warning system of power network overloading under serverless architecture In: 2018 2nd IEEE Conference on Energy Internet and Energy System Integration (EI2), 1–6. https://doi.org/10.1109/EI2.2018.8582355 .
Kulkarni SG, Liu G, Ramakrishnan KK, Wood T (2019) Living on the edge: Serverless computing and the cost of failure resiliency In: 2019 IEEE International Symposium on Local and Metropolitan Area Networks (LANMAN), 1–6. https://doi.org/10.1109/LANMAN.2019.8846970 .
Baldini I, Castro P, Chang K, Cheng P, Fink S, Ishakian V, Mitchell N, Muthusamy V, Rabbah R, Slominski A, Suter P (2017) Serverless Computing: Current Trends and Open Problems In: Research Advances in Cloud Computing, 1–20.. Springer, Singapore. https://doi.org/10.1007/978-981-10-5026-8_1 .
Adzic G, Chatley R (2017) Serverless computing: Economic and architectural impact In: Proceedings of the 2017 11th Joint Meeting on Foundations of Software Engineering (ESEC/FSE 2017), 884–889.. Association for Computing Machinery, New York. https://doi.org/10.1145/3106237.3117767 .
Chapter Google Scholar
Jambunathan B, Yoganathan K (2018) Architecture decision on using microservices or serverless functions with containers In: 2018 International Conference on Current Trends Towards Converging Technologies (ICCTCT), 1–7. https://doi.org/10.1109/ICCTCT.2018.8551035 .
Wolski R, Krintz C, Bakir F, George G, Lin W-T (2019) Cspot: Portable, multi-scale functions-as-a-service for iot In: Proceedings of the 4th ACM/IEEE Symposium on Edge Computing (SEC ‘19), 236–249.. Association for Computing Machinery, New York. https://doi.org/10.1145/3318216.3363314 .
Yussupov V, Breitenbücher U, Leymann F, Wurster M (2019) A systematic mapping study on engineering function-as-a-service platforms and tools In: Proceedings of the 12th IEEE/ACM International Conference on Utility and Cloud Computing (UCC’19), 229–240.. Association for Computing Machinery, New York. https://doi.org/10.1145/3344341.3368803 .
Brenner S, Kapitza R (2019) Trust more, serverless In: Proceedings of the 12th ACM International Conference on Systems and Storage (SYSTOR ‘19), 33–43.. Association for Computing Machinery, New York. https://doi.org/10.1145/3319647.3325825 .
Kuhlenkamp J, Werner S (2018) Benchmarking faas platforms: Call for community participation In: 2018 IEEE/ACM International Conference on Utility and Cloud Computing Companion (UCC Companion), 189–194. https://doi.org/10.1109/UCC-Companion.2018.00055 .
Somma G, Ayimba C, Casari P, Romano SP, Mancuso V (2020) When less is more: Core-restricted container provisioning for serverless computing In: IEEE INFOCOM 2020 - IEEE Conference on Computer Communications Workshops (INFOCOM WKSHPS), 1153–1159. https://doi.org/10.1109/INFOCOMWKSHPS50562.2020.9162876 .
Sewak M, Singh S (2018) Winning in the era of serverless computing and function as a service In: 2018 3rd International Conference for Convergence in Technology (I2CT), 1–5. https://doi.org/10.1109/I2CT.2018.8529465 .
van Eyk E, Toader L, Talluri S, Versluis L, Ută̧ A, Iosup A (2018) Serverless is more: From paas to present cloud computing. IEEE Internet Comput 22(5):8–17. https://doi.org/10.1109/MIC.2018.053681358 .
Leitner P, Wittern E, Spillner J, Hummer W (2019) A mixed-method empirical study of function-as-a-service software development in industrial practice. J Syst Softw 149:340–359. https://doi.org/10.1016/j.jss.2018.12.013 .
Feng L, Kudva P, Da Silva D, Hu J (2018) Exploring serverless computing for neural network training In: 2018 IEEE 11th International Conference on Cloud Computing (CLOUD), 334–341. https://doi.org/10.1109/CLOUD.2018.00049 .
Ao L, Izhikevich L, Voelker GM, Porter G (2018) Sprocket: A serverless video processing framework In: Proceedings of the ACM Symposium on Cloud Computing (SoCC ‘18), 263–274.. Association for Computing Machinery, New York. https://doi.org/10.1145/3267809.3267815 .
Werner S, Kuhlenkamp J, Klems M, Müller J, Tai S (2018) Serverless big data processing using matrix multiplication as example In: 2018 IEEE International Conference on Big Data (Big Data), 358–365. https://doi.org/10.1109/BigData.2018.8622362 .
Al-Ali Z, Goodarzy S, Hunter E, Ha S, Han R, Keller E, Rozner E (2018) Making serverless computing more serverless In: 2018 IEEE 11th International Conference on Cloud Computing (CLOUD), 456–459. https://doi.org/10.1109/CLOUD.2018.00064 .
Pérez A, Risco S, Naranjo DM, Caballer M, Moltó G (2019) On-premises serverless computing for event-driven data processing applications In: 2019 IEEE 12th International Conference on Cloud Computing (CLOUD), 414–421. https://doi.org/10.1109/CLOUD.2019.00073 .
Glikson A, Nastic S, Dustdar S (2017) Deviceless edge computing: Extending serverless computing to the edge of the network In: Proceedings of the 10th ACM International Systems and Storage Conference (SYSTOR ‘17).. Association for Computing Machinery, New York. https://doi.org/10.1145/3078468.3078497 .
Al-Ameen M, Spillner J (2019) A systematic and open exploration of faas research In: Proceedings of the European Symposium on Serverless Computing and Applications (CEUR Workshop Proceedings ; 2330), 30–35.. CEUR-WS, Zurich. https://doi.org/10.21256/zhaw-3271 .
Alqaryouti O, Siyam N (2018) Serverless computing and scheduling tasks on cloud: A review. Am Sci Res J Eng Technol Sci (ASRJETS) 40(1):235–247.
Taibi D, El Ioini N, Pahl C, Niederkofler J (2020) Patterns for Serverless Functions (Function-as-a-Service): A Multivocal Literature Review In: Proceedings of the 10th International Conference on Cloud Computing and Services Science - Volume 1: CLOSER, 181–192. https://doi.org/10.5220/0009578501810192 .
Hellerstein JM, Faleiro J, Gonzalez JE, Schleier-Smith J, Sreekanti V, Tumanov A, Wu C (2018) Serverless Computing: One Step Forward, Two Steps Back. http://arxiv.org/abs/1812.03651. Accessed 4 Oct 2021.
Rajan AP (2020) A review on serverless architectures - function as a service (faas) in cloud computing. Telecommun Comput Electron Control 18(1):530–537. https://doi.org/10.12928/telkomnika.v18i1.12169 .
Sadaqat M, Colomo-Palacios R, Knudsen LES (2018) Serverless Computing: A Multivocal Literature Review. NOKOBIT - Norsk Konferanse for Organisasjoners Bruk Av Informasjonsteknologi 26(1):1–13.
Scheuner J, Leitner P (2020) Function-as-a-service performance evaluation: A multivocal literature review. J Syst Softw 170:110708. https://doi.org/10.1016/j.jss.2020.110708 .
Fox GC, Ishakian V, Muthusamy V, Slominski A (2017) Status of Serverless Computing and Function-as-a-Service(FaaS) in Industry and Research. arXiv e-prints:1708–08028. http://arxiv.org/abs/1708.08028. Accessed 6 Jan 2021.
Hassan HB, Barakat SA, Sarhan QI (2021) Serverless Literature Dataset. Zenodo. https://doi.org/10.5281/zenodo.4660553 .
Pedreira O, García F, Brisaboa N, Piattini M (2015) Gamification in software engineering - a systematic mapping. Inf Softw Technol 57:157–168.
Lopez-herrejon RE, Linsbauer L, Egyed A (2015) A systematic mapping study of search-based software engineering for software product lines. Inf Softw Technol 61:33–51.
Bila N, Dettori P, Kanso A, Watanabe Y, Youssef A (2017) Leveraging the serverless architecture for securing linux containers In: 2017 IEEE 37th International Conference on Distributed Computing Systems Workshops (ICDCSW), 401–404. https://doi.org/10.1109/ICDCSW.2017.66 .
Chang KS, Fink SJ (2017) Visualizing serverless cloud application logs for program understanding In: 2017 IEEE Symposium on Visual Languages and Human-Centric Computing (VL/HCC), 261–265. https://doi.org/10.1109/VLHCC.2017.8103476 .
Ishakian V, Muthusamy V, Slominski A (2018) Serving deep learning models in a serverless platform In: 2018 IEEE International Conference on Cloud Engineering (IC2E), 257–262. https://doi.org/10.1109/IC2E.2018.00052 .
Baldini I, Cheng P, Fink SJ, Mitchell N, Muthusamy V, Rabbah R, Suter P, Tardieu O (2017) The serverless trilemma: Function composition for serverless computing In: Proceedings of the 2017 ACM SIGPLAN International Symposium on New Ideas, New Paradigms, and Reflections on Programming and Software (Onward! 2017), 89–103.. Association for Computing Machinery, New York. https://doi.org/10.1145/3133850.3133855 .
Kanso A, Youssef A (2017) Serverless: Beyond the cloud In: Proceedings of the 2nd International Workshop on Serverless Computing (WoSC ‘17), 6–10.. Association for Computing Machinery, New York. https://doi.org/10.1145/3154847.3154854 .
Koller R, Williams D (2017) Will serverless end the dominance of linux in the cloud? In: Proceedings of the 16th Workshop on Hot Topics in Operating Systems (HotOS ‘17), 169–173.. Association for Computing Machinery, New York. https://doi.org/10.1145/3102980.3103008 .
Mukhi NK, Prabhu S, Slawson B (2017) Using a serverless framework for implementing a cognitive tutor: Experiences and issues In: Proceedings of the 2nd International Workshop on Serverless Computing (WoSC ‘17), 11–15.. Association for Computing Machinery, New York. https://doi.org/10.1145/3154847.3154852 .
Nadgowda S, Bila N, Isci C (2017) The less server architecture for cloud functions In: Proceedings of the 2nd International Workshop on Serverless Computing (WoSC ‘17), 22–27.. Association for Computing Machinery, New York. https://doi.org/10.1145/3154847.3154850 .
Klimovic A, Wang Y, Stuedi P, Trivedi A, Pfefferle J, Kozyrakis C (2018) Pocket: Elastic ephemeral storage for serverless analytics In: Proceedings of the 12th USENIX Conference on Operating Systems Design and Implementation (OSDI’18), 427–444.. USENIX Association, USA.
Yan M, Castro P, Cheng P, Ishakian V (2016) Building a chatbot with serverless computing In: Proceedings of the 1st International Workshop on Mashups of Things and APIs (MOTA ‘16), 1–4.. Association for Computing Machinery, New York. https://doi.org/10.1145/3007203.3007217 .
Barcelona-Pons D, García-López P, Ruiz A, Gómez-Gómez A, París G, Sánchez-Artigas M (2019) Faas orchestration of parallel workloads In: Proceedings of the 5th International Workshop on Serverless Computing (WOSC ‘19), 25–30.. Association for Computing Machinery, New York. https://doi.org/10.1145/3366623.3368137 .
Barcelona-Pons D, Sánchez-Artigas M, París G, Sutra P, García-López P (2019) On the faas track: Building stateful distributed applications with serverless architectures In: Proceedings of the 20th International Middleware Conference (Middleware ‘19), 41–54.. Association for Computing Machinery, New York. https://doi.org/10.1145/3361525.3361535 .
Kaviani N, Kalinin D, Maximilien M (2019) Towards serverless as commodity: A case of knative In: Proceedings of the 5th International Workshop on Serverless Computing (WOSC ‘19), 13–18.. Association for Computing Machinery, New York. https://doi.org/10.1145/3366623.3368135 .
Byrne A, Nadgowda S, Coskun AK (2020) Ace: Just-in-time serverless software component discovery through approximate concrete execution In: Proceedings of the 2020 Sixth International Workshop on Serverless Computing (WoSC’20), 37–42.. Association for Computing Machinery, New York. https://doi.org/10.1145/3429880.3430098 .
Sánchez-Artigas M, Eizaguirre GT, Vernik G, Stuart L, García-López P (2020) Primula: A Practical Shuffle/Sort Operator for Serverless Computing. Association for Computing Machinery, New York.
Book Google Scholar
Parás G, Garcáa-López P, Sánchez-Artigas M (2020) Serverless elastic exploration of unbalanced algorithms In: 2020 IEEE 13th International Conference on Cloud Computing (CLOUD), 149–157. https://doi.org/10.1109/CLOUD49709.2020.00033 .
López PG, Arjona A, Sampé J, Slominski A, Villard L (2020) Triggerflow: Trigger-based orchestration of serverless workflows In: Proceedings of the 14th ACM International Conference on Distributed and Event-Based Systems (DEBS ’20), 3–14.. Association for Computing Machinery, New York. https://doi.org/10.1145/3401025.3401731 .
Carver B, Zhang J, Wang A, Anwar A, Wu P, Cheng Y (2020) Wukong: A scalable and locality-enhanced framework for serverless parallel computing In: Proceedings of the 11th ACM Symposium on Cloud Computing (SoCC ’20), 1–15.. Association for Computing Machinery, New York. https://doi.org/10.1145/3419111.3421286 .
Klimovic A, Wang Y, Kozyrakis C, Stuedi P, Pfefferle J, Trivedi A (2018) Understanding ephemeral storage for serverless analytics In: Proceedings of the 2018 USENIX Conference on Usenix Annual Technical Conference (USENIX ATC ’18), 789–794.. USENIX Association, USA.
Wang A, Zhang J, Ma X, Anwar A, Rupprecht L, Skourtis D, Tarasov V, Yan F, Cheng Y (2020) Infinicache: Exploiting ephemeral serverless functions to build a cost-effective memory cache In: 18th USENIX Conference on File and Storage Technologies (FAST 20), 267–281.. USENIX Association, Santa Clara.
Kuhlenkamp J, Werner S, Borges MC, El Tal K, Tai S (2019) An evaluation of faas platforms as a foundation for serverless big data processing In: Proceedings of the 12th IEEE/ACM International Conference on Utility and Cloud Computing (UCC’19), 1–9.. Association for Computing Machinery, New York. https://doi.org/10.1145/3344341.3368796 .
Werner S, Girke R, Kuhlenkamp J (2020) An evaluation of serverless data processing frameworks In: Proceedings of the 2020 Sixth International Workshop on Serverless Computing (WoSC’20), 19–24.. Association for Computing Machinery, New York. https://doi.org/10.1145/3429880.3430095 .
Kuhlenkamp J, Werner S, Borges MC, Ernst D, Wenzel D (2020) Benchmarking elasticity of faas platforms as a foundation for objective-driven design of serverless applications In: Proceedings of the 35th Annual ACM Symposium on Applied Computing (SAC ’20), 1576–1585.. Association for Computing Machinery, New York. https://doi.org/10.1145/3341105.3373948 .
Werner S, Kuhlenkamp J, Pallas F, Anders N, Mucaj N, Tsaplina O, Schmidt C, Yildirim K (2020) Diminuendo! tactics in support of faas migrations. In: Paasivaara M Kruchten P (eds)Agile Processes in Software Engineering and Extreme Programming – Workshops, 125–132.. Springer, Cham.
Kuhlenkamp J, Werner S, Tai S (2020) The ifs and buts of less is more: A serverless computing reality check In: 2020 IEEE International Conference on Cloud Engineering (IC2E), 154–161. https://doi.org/10.1109/IC2E48712.2020.00023 .
Pfandzelter T, Bermbach D (2020) tinyfaas: A lightweight faas platform for edge environments In: 2020 IEEE International Conference on Fog Computing (ICFC), 17–24. https://doi.org/10.1109/ICFC49376.2020.00011 .
Bermbach D, Karakaya A-S, Buchholz S (2020) Using application knowledge to reduce cold starts in faas services In: Proceedings of the 35th Annual ACM Symposium on Applied Computing (SAC ’20), 134–143.. Association for Computing Machinery, New York. https://doi.org/10.1145/3341105.3373909 .
Bermbach D, Maghsudi S, Hasenburg J, Pfandzelter T (2020) Towards auction-based function placement in serverless fog platforms In: 2020 IEEE International Conference on Fog Computing (ICFC), 25–31. https://doi.org/10.1109/ICFC49376.2020.00012 .
Garcia Lopez P, Sanchez-Artigas M, Paris G, Barcelona Pons D, Ruiz Ollobarren A, Arroyo Pinto D2018. Comparison of faas orchestration systems. https://doi.org/10.1109/ucc-companion.2018.00049 .
Sampé J, Sánchez-Artigas M, García-López P, París G (2017) Data-driven serverless functions for object storage In: Proceedings of the 18th ACM/IFIP/USENIX Middleware Conference (Middleware ‘17), 121–133.. Association for Computing Machinery, New York. https://doi.org/10.1145/3135974.3135980 .
Sampé J, Vernik G, Sánchez-Artigas M, García-López P (2018) Serverless data analytics in the ibm cloud In: Proceedings of the 19th International Middleware Conference Industry (Middleware ‘18), 1–8.. Association for Computing Machinery, New York. https://doi.org/10.1145/3284028.3284029 .
Mirabelli ME, García-López P, Vernik G (2020) Bringing scaling transparency to proteomics applications with serverless computing In: Proceedings of the 2020 Sixth International Workshop on Serverless Computing (WoSC’20), 55–60.. Association for Computing Machinery, New York. https://doi.org/10.1145/3429880.3430101 .
Carreira J, Fonseca P, Tumanov A, Zhang A, Katz R (2019) Cirrus: A serverless framework for end-to-end ml workflows In: Proceedings of the ACM Symposium on Cloud Computing (SoCC ‘19), 13–24.. Association for Computing Machinery, New York. https://doi.org/10.1145/3357223.3362711 .
Sreekanti V, Wu C, Chhatrapati S, Gonzalez JE, Hellerstein JM, Faleiro JM (2020) A fault-tolerance shim for serverless computing In: Proceedings of the Fifteenth European Conference on Computer Systems (EuroSys ’20).. Association for Computing Machinery, New York. https://doi.org/10.1145/3342195.3387535 .
Ichnowski J, Lee W, Murta V, Paradis S, Alterovitz R, Gonzalez JE, Stoica I, Goldberg K (2020) Fog robotics algorithms for distributed motion planning using lambda serverless computing In: 2020 IEEE International Conference on Robotics and Automation (ICRA), 4232–4238. https://doi.org/10.1109/ICRA40945.2020.9196651 .
Zhang W, Fang V, Panda A, Shenker S (2020) Kappa: A programming framework for serverless computing In: Proceedings of the 11th ACM Symposium on Cloud Computing (SoCC ’20), 328–343.. Association for Computing Machinery, New York. https://doi.org/10.1145/3419111.3421277 .
Shankar V, Krauth K, Vodrahalli K, Pu Q, Recht B, Stoica I, Ragan-Kelley J, Jonas E, Venkataraman S (2020) Serverless linear algebra In: Proceedings of the 11th ACM Symposium on Cloud Computing (SoCC ’20), 281–295.. Association for Computing Machinery, New York. https://doi.org/10.1145/3419111.3421287 .
Gupta V, Carrano D, Yang Y, Shankar V, Courtade T, Ramchandran K (2020) Serverless straggler mitigation using error-correcting codes In: 2020 IEEE 40th International Conference on Distributed Computing Systems (ICDCS), 135–145. https://doi.org/10.1109/ICDCS47774.2020.00019 .
Wu C, Sreekanti V, Hellerstein JM (2020) Transactional causal consistency for serverless computing In: Proceedings of the 2020 ACM SIGMOD International Conference on Management of Data (SIGMOD ’20), 83–97.. Association for Computing Machinery, New York. https://doi.org/10.1145/3318464.3389710 .
Pu Q, Venkataraman S, Stoica I (2019) Shuffling, fast and slow: Scalable analytics on serverless infrastructure In: Proceedings of the 16th USENIX Conference on Networked Systems Design and Implementation (NSDI’19), 193–206.. USENIX Association, USA.
Lloyd W, Ramesh S, Chinthalapati S, Ly L, Pallickara S (2018) Serverless computing: An investigation of factors influencing microservice performance In: 2018 IEEE International Conference on Cloud Engineering (IC2E), 159–169. https://doi.org/10.1109/IC2E.2018.00039 .
Lloyd W, Vu M, Zhang B, David O, Leavesley G (2018) Improving application migration to serverless computing platforms: Latency mitigation with keep-alive workloads In: 2018 IEEE/ACM International Conference on Utility and Cloud Computing Companion (UCC Companion), 195–200. https://doi.org/10.1109/UCC-Companion.2018.00056 .
Al-Masri E, Diabate I, Jain R, Lam MHL, Nathala SR (2018) A serverless iot architecture for smart waste management systems In: 2018 IEEE International Conference on Industrial Internet (ICII), 179–180. https://doi.org/10.1109/ICII.2018.00034 .
Fotouhi M, Chen D, Lloyd WJ (2019) Function-as-a-service application service composition: Implications for a natural language processing application In: Proceedings of the 5th International Workshop on Serverless Computing (WOSC ‘19), 49–54.. Association for Computing Machinery, New York. https://doi.org/10.1145/3366623.3368141 .
Niu X, Kumanov D, Hung L-H, Lloyd W, Yeung KY (2019) Leveraging serverless computing to improve performance for sequence comparison In: Proceedings of the 10th ACM International Conference on Bioinformatics, Computational Biology and Health Informatics (BCB ‘19), 683–687.. Association for Computing Machinery, New York. https://doi.org/10.1145/3307339.3343465 .
Cordingly R, Yu H, Hoang V, Perez D, Foster D, Sadeghi Z, Hatchett R, Lloyd WJ (2020) Implications of programming language selection for serverless data processing pipelines In: 2020 IEEE Intl Conf on Dependable, Autonomic and Secure Computing, Intl Conf on Pervasive Intelligence and Computing, Intl Conf on Cloud and Big Data Computing, Intl Conf on Cyber Science and Technology Congress (DASC/PiCom/CBDCom/CyberSciTech), 704–711. https://doi.org/10.1109/DASC-PICom-CBDCom-CyberSciTech49142.2020.00120 .
Cordingly R, Shu W, Lloyd WJ (2020) Predicting performance and cost of serverless computing functions with saaf In: 2020 IEEE Intl Conf on Dependable, Autonomic and Secure Computing, Intl Conf on Pervasive Intelligence and Computing, Intl Conf on Cloud and Big Data Computing, Intl Conf on Cyber Science and Technology Congress (DASC/PiCom/CBDCom/CyberSciTech), 640–649. https://doi.org/10.1109/DASC-PICom-CBDCom-CyberSciTech49142.2020.00111 .
Cordingly R, Yu H, Hoang V, Sadeghi Z, Foster D, Perez D, Hatchett R, Lloyd W (2020) The serverless application analytics framework: Enabling design trade-off evaluation for serverless software In: Proceedings of the 2020 Sixth International Workshop on Serverless Computing (WoSC’20), 67–72.. Association for Computing Machinery, New York. https://doi.org/10.1145/3429880.3430103 .
Toader L, Uta A, Musaafir A, Iosup A (2019) Graphless: Toward serverless graph processing In: 2019 18th International Symposium on Parallel and Distributed Computing (ISPDC), 66–73. https://doi.org/10.1109/ISPDC.2019.00012 .
van Eyk E, Iosup A, Seif S, Thömmes M (2017) The spec cloud group’s research vision on faas and serverless architectures In: Proceedings of the 2nd International Workshop on Serverless Computing (WoSC ‘17), 1–4.. Association for Computing Machinery, New York. https://doi.org/10.1145/3154847.3154848 .
van Eyk E, Iosup A, Abad CL, Grohmann J, Eismann S (2018) A spec rg cloud group’s vision on the performance challenges of faas cloud architectures In: Companion of the 2018 ACM/SPEC International Conference on Performance Engineering (ICPE ‘18), 21–24.. Association for Computing Machinery, New York. https://doi.org/10.1145/3185768.3186308 .
van Eyk E, Grohmann J, Eismann S, Bauer A, Versluis L, Toader L, Schmitt N, Herbst N, Abad CL, Iosup A (2019) The spec-rg reference architecture for faas: From microservices and containers to serverless platforms. IEEE Internet Comput 23(6):7–18. https://doi.org/10.1109/MIC.2019.2952061 .
van Eyk E, Scheuner J, Eismann S, Abad CL, Iosup A (2020) Beyond microbenchmarks: The spec-rg vision for a comprehensive serverless benchmark In: Companion of the ACM/SPEC International Conference on Performance Engineering (ICPE ’20), 26–31.. Association for Computing Machinery, New York. https://doi.org/10.1145/3375555.3384381 .
Eismann S, Grohmann J, van Eyk E, Herbst N, Kounev S (2020) Predicting the costs of serverless workflows In: Proceedings of the ACM/SPEC International Conference on Performance Engineering (ICPE ’20), 265–276.. Association for Computing Machinery, New York. https://doi.org/10.1145/3358960.3379133 .
van Eyk E, Iosup A (2018) Addressing performance challenges in serverless computing In: ICT. OPEN.
Hendrickson S, Sturdevant S, Harter T, Venkataramani V, Arpaci-Dusseau AC, Arpaci-Dusseau RH (2016) Serverless computation with openlambda In: 8th USENIX Workshop on Hot Topics in Cloud Computing (HotCloud 16), 1–7.. USENIX Association, Denver.
Oakes E, Yang L, Zhou D, Houck K, Harter T, Arpaci-Dusseau AC, Arpaci-Dusseau RH (2018) Sock: Rapid task provisioning with serverless-optimized containers In: Proceedings of the 2018 USENIX Conference on Usenix Annual Technical Conference (USENIX ATC ‘18), 57–69.. USENIX Association, USA.
Oakes E, Yang L, Houck K, Harter T, Arpaci-Dusseau AC, Arpaci-Dusseau RH (2017) Pipsqueak: Lean lambdas with large libraries In: 2017 IEEE 37th International Conference on Distributed Computing Systems Workshops (ICDCSW), 395–400. https://doi.org/10.1109/ICDCSW.2017.32 .
Singhvi A, Khalid J, Akella A, Banerjee S (2020) Snf: Serverless network functions In: Proceedings of the 11th ACM Symposium on Cloud Computing (SoCC ’20), 296–310.. Association for Computing Machinery, New York. https://doi.org/10.1145/3419111.3421295 .
Wang L, Li M, Zhang Y, Ristenpart T, Swift M (2018) Peeking behind the curtains of serverless platforms In: Proceedings of the 2018 USENIX Conference on Usenix Annual Technical Conference (USENIX ATC ’18), 133–145.. USENIX Association, USA.
Abad CL, Boza EF, van Eyk E (2018) Package-aware scheduling of faas functions In: Companion of the 2018 ACM/SPEC International Conference on Performance Engineering (ICPE ‘18), 101–106.. Association for Computing Machinery, New York. https://doi.org/10.1145/3185768.3186294 .
Aumala G, Boza E, Ortiz-Avilés L, Totoy G, Abad C (2019) Beyond load balancing: Package-aware scheduling for serverless platforms In: 2019 19th IEEE/ACM International Symposium on Cluster, Cloud and Grid Computing (CCGRID), 282–291. https://doi.org/10.1109/CCGRID.2019.00042 .
Alpernas K, Flanagan C, Fouladi S, Ryzhyk L, Sagiv M, Schmitz T, Winstein K (2018) Secure serverless computing using dynamic information flow control. Proc ACM Program Lang 2(OOPSLA):1–26. https://doi.org/10.1145/3276488 .
Kaffes K, Yadwadkar NJ, Kozyrakis C (2019) Centralized core-granular scheduling for serverless functions In: Proceedings of the ACM Symposium on Cloud Computing (SoCC ‘19), 158–164.. Association for Computing Machinery, New York. https://doi.org/10.1145/3357223.3362709 .
Choi S, Shahbaz M, Prabhakar B, Rosenblum M (2019) λ -nic: Interactive serverless compute on smartnics In: Proceedings of the ACM SIGCOMM 2019 Conference Posters and Demos (SIGCOMM Posters and Demos ’19), 151–152.. Association for Computing Machinery, New York. https://doi.org/10.1145/3342280.3342341 .
Manner J, Endreß M, Heckel T, Wirtz G (2018) Cold start influencing factors in function as a service In: 2018 IEEE/ACM International Conference on Utility and Cloud Computing Companion (UCC Companion), 181–188. https://doi.org/10.1109/UCC-Companion.2018.00054 .
Manner J, Kolb S, Wirtz G (2019) Troubleshooting serverless functions: a combined monitoring and debugging approach. SICS Softw-Intensiv Cyber-Physical Syst 34(2):99–104. https://doi.org/10.1007/s00450-019-00398-6 .
Winzinger S, Wirtz G (2019) Model-based analysis of serverless applications In: 2019 IEEE/ACM 11th International Workshop on Modelling in Software Engineering (MiSE), 82–88. https://doi.org/10.1109/MiSE.2019.00020 .
Winzinger S, Wirtz G (2020) Applicability of coverage criteria for serverless applications In: 2020 IEEE International Conference on Service Oriented Systems Engineering (SOSE), 49–56. https://doi.org/10.1109/SOSE49046.2020.00013 .
Prechtl M, Lichtenthäler R, Wirtz G (2020) Investigating possibilites for protecting and hardening installable faas platforms. In: Dustdar S (ed)Service-Oriented Computing, 107–126.. Springer, Cham.
Gias AU, Casale G (2020) Cocoa: Cold start aware capacity planning for function-as-a-service platforms In: 2020 28th International Symposium on Modeling, Analysis, and Simulation of Computer and Telecommunication Systems (MASCOTS), 1–8. https://doi.org/10.1109/MASCOTS50786.2020.9285966 .
Chatley R, Allerton T (2020) Nimbus: Improving the developer experience for serverless applications In: 2020 IEEE/ACM 42nd International Conference on Software Engineering: Companion Proceedings (ICSE-Companion), 85–88.
Casale G, Artač M, van den Heuvel W-J, van Hoorn A, Jakovits P, Leymann F, Long M, Papanikolaou V, Presenza D, Russo A, Srirama SN, Tamburri DA, Wurster M, Zhu L (2020) Radon: rational decomposition and orchestration for serverless computing. SICS Softw-Intens Cyber-Phys Syst 35(1):77–87. https://doi.org/10.1007/s00450-019-00413-w .
Vandebon J, Coutinho JGF, Luk W, Nurvitadhi E, Naik M (2020) Slate: Managing heterogeneous cloud functions In: 2020 IEEE 31st International Conference on Application-specific Systems, Architectures and Processors (ASAP), 141–148. https://doi.org/10.1109/ASAP49362.2020.00032 .
Kim J, Lee K (2019) Functionbench: A suite of workloads for serverless cloud function service In: 2019 IEEE 12th International Conference on Cloud Computing (CLOUD), 502–504. https://doi.org/10.1109/CLOUD.2019.00091 .
Kim J, Park J, Lee K (2019) Network resource isolation in serverless cloud function service In: 2019 IEEE 4th International Workshops on Foundations and Applications of Self* Systems (FAS*W), 182–187. https://doi.org/10.1109/FAS-W.2019.00051 .
Park J, Kim H, Lee K (2020) Evaluating concurrent executions of multiple function-as-a-service runtimes with microvm In: 2020 IEEE 13th International Conference on Cloud Computing (CLOUD), 532–536. https://doi.org/10.1109/CLOUD49709.2020.00080 .
Choi J, Lee K (2020) Evaluation of network file system as a shared data storage in serverless computing In: Proceedings of the 2020 Sixth International Workshop on Serverless Computing (WoSC’20), 25–30.. Association for Computing Machinery, New York. https://doi.org/10.1145/3429880.3430096 .
Kim J, Lee K (2020) I/o resource isolation of public cloud serverless function runtimes for data-intensive applications. Clust Comput 23(3):2249–2259. https://doi.org/10.1007/s10586-020-03103-4 .
Wu M, Mi Z, Xia Y (2020) A survey on serverless computing and its implications for jointcloud computing In: 2020 IEEE International Conference on Joint Cloud Computing, 94–101. https://doi.org/10.1109/JCC49151.2020.00023 .
Li Z, Chen Q, Xue S, Ma T, Yang Y, Song Z, Guo M (2020) Amoeba: Qos-awareness and reduced resource usage of microservices with serverless computing In: 2020 IEEE International Parallel and Distributed Processing Symposium (IPDPS), 399–408. https://doi.org/10.1109/IPDPS47924.2020.00049 .
Du D, Yu T, Xia Y, Zang B, Yan G, Qin C, Wu Q, Chen H (2020) Catalyzer: Sub-millisecond startup for serverless computing with initialization-less booting In: Proceedings of the Twenty-Fifth International Conference on Architectural Support for Programming Languages and Operating Systems (ASPLOS ’20), 467–481.. Association for Computing Machinery, New York. https://doi.org/10.1145/3373376.3378512 .
Yu T, Liu Q, Du D, Xia Y, Zang B, Lu Z, Yang P, Qin C, Chen H (2020) Characterizing serverless platforms with serverlessbench In: Proceedings of the 11th ACM Symposium on Cloud Computing (SoCC ’20), 30–44.. Association for Computing Machinery, New York. https://doi.org/10.1145/3419111.3421280 .
Liu J, Mi Z, Huang Z, Hua Z, Xia Y (2020) Hcloud: A serverless platform for jointcloud computing In: 2020 IEEE International Conference on Joint Cloud Computing, 86–93. https://doi.org/10.1109/JCC49151.2020.00022 .
Pérez A, Moltó G, Caballer M, Calatrava A (2019) A programming model and middleware for high throughput serverless computing applications In: Proceedings of the 34th ACM/SIGAPP Symposium on Applied Computing (SAC ‘19), 106–113.. Association for Computing Machinery, New York. https://doi.org/10.1145/3297280.3297292 .
Pérez A, Moltó G, Caballer M, Calatrava A (2018) Serverless computing for container-based architectures. Future Generation Computer Systems 83:50–59. https://doi.org/10.1016/j.future.2018.01.022 .
Giménez-Alventosa V, Moltó G, Caballer M (2019) A framework and a performance assessment for serverless mapreduce on aws lambda. Future Generation Computer Systems 97:259–274. https://doi.org/10.1016/j.future.2019.02.057 .
Naranjo DM, Risco S, de Alfonso C, Pérez A, Blanquer I, Moltó G (2020) Accelerated serverless computing based on gpu virtualization. J Parallel Distrib Comput 139:32–42. https://doi.org/10.1016/j.jpdc.2020.01.004 .
Wang H, Niu D, Li B (2019) Distributed machine learning with a serverless architecture In: IEEE INFOCOM 2019 - IEEE Conference on Computer Communications, 1288–1296. https://doi.org/10.1109/INFOCOM.2019.8737391 .
Ghaemi S, Khazaei H, Musilek P (2020) Chainfaas: An open blockchain-based serverless platform. IEEE Access 8:131760–131778. https://doi.org/10.1109/ACCESS.2020.3010119 .
Goli A, Hajihassani O, Khazaei H, Ardakanian O, Rashidi M, Dauphinee T (2020) Migrating from monolithic to serverless: A fintech case study In: Companion of the ACM/SPEC International Conference on Performance Engineering (ICPE ’20), 20–25.. Association for Computing Machinery, New York. https://doi.org/10.1145/3375555.3384380 .
Mahmoudi N, Khazaei H (2020) Performance modeling of serverless computing platforms. IEEE Trans Cloud Comput:1–1. https://doi.org/10.1109/TCC.2020.3033373 .
Mahmoudi N, Khazaei H (2020) Temporal performance modelling of serverless computing platforms In: Proceedings of the 2020 Sixth International Workshop on Serverless Computing (WoSC’20), 1–6.. Association for Computing Machinery, New York. https://doi.org/10.1145/3429880.3430092 .
Wurster M, Breitenbücher U, Képes K, Leymann F, Yussupov V (2018) Modeling and automated deployment of serverless applications using tosca In: 2018 IEEE 11th Conference on Service-Oriented Computing and Applications (SOCA), 73–80. https://doi.org/10.1109/SOCA.2018.00017 .
Yussupov V, Breitenbücher U, Hahn M, Leymann F (2019) Serverless parachutes: Preparing chosen functionalities for exceptional workloads In: 2019 IEEE 23rd International Enterprise Distributed Object Computing Conference (EDOC), 226–235. https://doi.org/10.1109/EDOC.2019.00035 .
Yussupov V, Breitenbücher U, Leymann F, Müller C (2019) Facing the unplanned migration of serverless applications: A study on portability problems, solutions, and dead ends In: Proceedings of the 12th IEEE/ACM International Conference on Utility and Cloud Computing (UCC’19), 273–283.. Association for Computing Machinery, New York. https://doi.org/10.1145/3344341.3368813 .
Spillner J (2019) Serverless computing and cloud function-based applications In: Proceedings of the 12th IEEE/ACM International Conference on Utility and Cloud Computing Companion (UCC ‘19 Companion), 177–178.. Association for Computing Machinery, New York. https://doi.org/10.1145/3368235.3370269 .
Murphy S, Persaud L, Martini W, Bosshard B (2020) On the use of web assembly in a serverless context. In: Paasivaara M Kruchten P (eds)Agile Processes in Software Engineering and Extreme Programming – Workshops, 141–145.. Springer, Cham.
Spillner J (2020) Resource management for cloud functions with memory tracing, profiling and autotuning In: Proceedings of the 2020 Sixth International Workshop on Serverless Computing (WoSC’20), 13–18.. Association for Computing Machinery, New York. https://doi.org/10.1145/3429880.3430094 .
Spillner J, Mateos C, Monge DA (2018) Faaster, better, cheaper: The prospect of serverless scientific computing and hpc. In: Mocskos E Nesmachnow S (eds)High Performance Computing, 154–168.. Springer, Cham.
Alder F, Asokan N, Kurnikov A, Paverd A, Steiner M (2019) S-faas: Trustworthy and accountable function-as-a-service using intel sgx In: Proceedings of the 2019 ACM SIGSAC Conference on Cloud Computing Security Workshop (CCSW’19), 185–199.. Association for Computing Machinery, New York. https://doi.org/10.1145/3338466.3358916 .
Kuriata A, Illikkal RG (2020) Predictable performance for qos-sensitive, scalable, multi-tenant function-as-a-service deployments. In: Paasivaara M Kruchten P (eds)Agile Processes in Software Engineering and Extreme Programming – Workshops, 133–140.. Springer, Cham.
Mohan A, Sane H, Doshi K, Edupuganti S, Nayak N, Sukhomlinov V (2019) Agile cold starts for scalable serverless In: Proceedings of the 11th USENIX Conference on Hot Topics in Cloud Computing (HotCloud’19), 21.. USENIX Association, USA.
Shahrad M, Fonseca R, Goiri I, Chaudhry G, Batum P, Cooke J, Laureano E, Tresness C, Russinovich M, Bianchini R (2020) Serverless in the wild: Characterizing and optimizing the serverless workload at a large cloud provider In: 2020 USENIX Annual Technical Conference (USENIX ATC 20), 205–218.
Obetz M, Das A, Castiglia T, Patterson S, Milanova A (2020) Formalizing event-driven behavior of serverless applications. In: Brogi A, Zimmermann W, Kritikos K (eds)Service-Oriented and Cloud Computing, 19–29.. Springer, Cham.
Das A, Imai S, Patterson S, Wittie MP (2020) Performance optimization for edge-cloud serverless platforms via dynamic task placement In: 2020 20th IEEE/ACM International Symposium on Cluster, Cloud and Internet Computing (CCGRID), 41–50. https://doi.org/10.1109/CCGrid49817.2020.00-89 .
Das A, Leaf A, Varela CA, Patterson S (2020) Skedulix: Hybrid cloud scheduling for cost-efficient execution of serverless applications In: 2020 IEEE 13th International Conference on Cloud Computing (CLOUD), 609–618. https://doi.org/10.1109/CLOUD49709.2020.00090 .
Obetz M, Patterson S, Milanova A (2019) Static call graph construction in aws lambda serverless applications In: Proceedings of the 11th USENIX Conference on Hot Topics in Cloud Computing (HotCloud’19), 20.. USENIX Association, USA.
Lin W, Krintz C, Wolski R, Zhang M, Cai X, Li T, Xu W (2018) Tracking causal order in aws lambda applications In: 2018 IEEE International Conference on Cloud Engineering (IC2E), 50–60. https://doi.org/10.1109/IC2E.2018.00027 .
George G, Bakir F, Wolski R, Krintz C (2020) Nanolambda: Implementing functions as a service at all resource scales for the internet of things In: 2020 IEEE/ACM Symposium on Edge Computing (SEC), 220–231. https://doi.org/10.1109/SEC50012.2020.00035 .
Zhang M, Krintz C, Wolski R (2020) Stoic: Serverless teleoperable hybrid cloud for machine learning applications on edge device In: 2020 IEEE International Conference on Pervasive Computing and Communications Workshops (PerCom Workshops), 1–6. https://doi.org/10.1109/PerComWorkshops48775.2020.9156239 .
Datta P, Kumar P, Morris T, Grace M, Rahmati A, Bates A (2020) Valve: Securing function workflows on serverless computing platforms In: Proceedings of The Web Conference 2020 (WWW ’20), 939–950.. Association for Computing Machinery, New York. https://doi.org/10.1145/3366423.3380173 .
Sankaran A, Datta P, Bates A (2020) Workflow integration alleviates identity and access management in serverless computing In: Annual Computer Security Applications Conference (ACSAC ’20), 496–509.. Association for Computing Machinery, New York. https://doi.org/10.1145/3427228.3427665 .
Elgamal T (2018) Costless: Optimizing cost of serverless computing through function fusion and placement In: 2018 IEEE/ACM Symposium on Edge Computing (SEC), 300–312. https://doi.org/10.1109/SEC.2018.00029 .
Dehury CK, Srirama SN, Chhetri TR (2020) Ccodamic: A framework for coherent coordination of data migration and computation platforms. Futur Gener Comput Syst 109:1–16. https://doi.org/10.1016/j.future.2020.03.029 .
Dehury C, Jakovits P, Srirama SN, Tountopoulos V, Giotis G (2020) Data pipeline architecture for serverless platform. In: Muccini H, Avgeriou P, Buhnova B, Camara J, Caporuscio M, Franzago M, Koziolek A, Scandurra P, Trubiani C, Weyns D, Zdun U (eds)Software Architecture, 241–246.. Springer, Cham.
Sarkar S, Wankar R, Srirama SN, Suryadevara NK (2020) Serverless management of sensing systems for fog computing framework. IEEE Sensors J 20(3):1564–72. https://doi.org/10.1109/JSEN.2019.2939182 .
Malawski M, Gajek A, Zima A, Balis B, Figiela K (2017) Serverless execution of scientific workflows: Experiments with hyperflow, aws lambda and google cloud functions. Futur Gener Comput Syst:1–13. https://doi.org/10.1016/j.future.2017.10.029 .
Moczurad P, Malawski M (2018) Visual-textual framework for serverless computation: A luna language approach In: 2018 IEEE/ACM International Conference on Utility and Cloud Computing Companion (UCC Companion), 169–74. https://doi.org/10.1109/UCC-Companion.2018.00052 .
Pawlik M, Banach P, Malawski M (2020) Adaptation of workflow application scheduling algorithm to serverless infrastructure. In: Schwardmann U, Boehme C, B. Heras D, Cardellini V, Jeannot E, Salis A, Schifanella C, Manumachu RR, Schwamborn D, Ricci L, Sangyoon O, Gruber T, Antonelli L, Scott SL (eds)Euro-Par 2019: Parallel Processing Workshops, 345–356.. Springer, Cham.
Akhtar N, Raza A, Ishakian V, Matta I (2020) Cose: Configuring serverless functions using statistical learning In: IEEE INFOCOM 2020 - IEEE Conference on Computer Communications, 129–38. https://doi.org/10.1109/INFOCOM41043.2020.9155363 .
Cadden J, Unger T, Awad Y, Dong H, Krieger O, Appavoo J (2020) Seuss: Skip redundant paths to make serverless fast In: Proceedings of the Fifteenth European Conference on Computer Systems (EuroSys ’20).. Association for Computing Machinery, New York. https://doi.org/10.1145/3342195.3392698 .
Balla D, Maliosz M, Simon C (2020) Open source faas performance aspects In: 2020 43rd International Conference on Telecommunications and Signal Processing (TSP), 358–364. https://doi.org/10.1109/TSP49548.2020.9163456 .
Pelle I, Czentye J, Dóka J, Kern A, Gerő BP, Sonkoly B (2020) Operating latency sensitive applications on public serverless edge cloud platforms. IEEE Internet Things J:1–1. https://doi.org/10.1109/JIOT.2020.3042428 .
Balla D, Maliosz M, Simon C, Gehberger D (2020) Tuning runtimes in open source faas. In: Hsu C-H, Kallel S, Lan K-C, Zheng Z (eds)Internet of Vehicles. Technologies and Services Toward Smart Cities, 250–266.. Springer, Cham.
Carver B, Zhang J, Wang A, Cheng Y (2019) In search of a fast and efficient serverless dag engine In: 2019 IEEE/ACM Fourth International Parallel Data Systems Workshop (PDSW), 1–10. https://doi.org/10.1109/PDSW49588.2019.00005 .
Gadepalli PK, McBride S, Peach G, Cherkasova L, Parmer G (2020) Sledge: A Serverless-First, Light-Weight Wasm Runtime for the Edge. Association for Computing Machinery, New York.
Gadepalli PK, Peach G, Cherkasova L, Aitken R, Parmer G (2019) Challenges and opportunities for efficient serverless computing at the edge In: 2019 38th Symposium on Reliable Distributed Systems (SRDS), 261–2615. https://doi.org/10.1109/SRDS47363.2019.00036 .
Somu N, Daw N, Bellur U, Kulkarni P (2020) Panopticon: A comprehensive benchmarking tool for serverless applications In: 2020 International Conference on COMmunication Systems NETworkS (COMSNETS), 144–151. https://doi.org/10.1109/COMSNETS48256.2020.9027346 .
Bajaj D, Bharti U, Goel A, Gupta SC (2020) Partial migration for re-architecting a cloud native monolithic application into microservices and faas. In: Badica C, Liatsis P, Kharb L, Chahal D (eds)Information, Communication and Computing Technology, 111–124.. Springer, Singapore.
Daw N, Bellur U, Kulkarni P (2020) Xanadu: Mitigating Cascading Cold Starts in Serverless Function Chain Deployments. Association for Computing Machinery, New York.
HoseinyFarahabady M, Lee YC, Zomaya AY, Tari Z (2017) A qos-aware resource allocation controller for function as a service (faas) platform. In: Maximilien M, Vallecillo A, Wang J, Oriol M (eds)Service-Oriented Computing, 241–255.. Springer, Cham. https://doi.org/10.1007/978-3-319-69035-3_17 .
Kim YK, HoseinyFarahabady MR, Lee YC, Zomaya AY, Jurdak R (2018) Dynamic control of cpu usage in a lambda platform In: 2018 IEEE International Conference on Cluster Computing (CLUSTER), 234–244. https://doi.org/10.1109/CLUSTER.2018.00041 .
Kim YK, HoseinyFarahabady MR, Lee YC, Zomaya AY (2020) Automated fine-grained cpu cap control in serverless computing platform. IEEE Transactions on Parallel and Distributed Systems 31(10):2289–2301. https://doi.org/10.1109/TPDS.2020.2989771 .
Suresh A, Somashekar G, Varadarajan A, Kakarla VR, Upadhyay H, Gandhi A (2020) Ensure: Efficient scheduling and autonomous resource management in serverless environments In: 2020 IEEE International Conference on Autonomic Computing and Self-Organizing Systems (ACSOS), 1–10. https://doi.org/10.1109/ACSOS49614.2020.00020 .
Suresh A, Gandhi A (2019) Fnsched: An efficient scheduler for serverless functions In: Proceedings of the 5th International Workshop on Serverless Computing (WOSC ’19), 19–24.. Association for Computing Machinery, New York. https://doi.org/10.1145/3366623.3368136 .
Hunhoff E, Irshad S, Thurimella V, Tariq A, Rozner E (2020) Proactive serverless function resource management In: Proceedings of the 2020 Sixth International Workshop on Serverless Computing (WoSC’20), 61–66.. Association for Computing Machinery, New York. https://doi.org/10.1145/3429880.3430102 .
Tariq A, Pahl A, Nimmagadda S, Rozner E, Lanka S (2020) Sequoia: Enabling quality-of-service in serverless computing In: Proceedings of the 11th ACM Symposium on Cloud Computing (SoCC ’20), 311–327.. Association for Computing Machinery, New York. https://doi.org/10.1145/3419111.3421306 .
Crane M, Lin J (2017) An exploration of serverless architectures for information retrieval In: Proceedings of the ACM SIGIR International Conference on Theory of Information Retrieval (ICTIR ‘17), 241–244.. Association for Computing Machinery, New York. https://doi.org/10.1145/3121050.3121086 .
Kim Y, Lin J (2018) Serverless data analytics with flint In: 2018 IEEE 11th International Conference on Cloud Computing (CLOUD), 451–455. https://doi.org/10.1109/CLOUD.2018.00063 .
Król M, Psaras I (2017) Nfaas: Named function as a service In: Proceedings of the 4th ACM Conference on Information-Centric Networking (ICN ‘17), 134–144.. Association for Computing Machinery, New York. https://doi.org/10.1145/3125719.3125727 .
Parres-Peredo A, Piza-Davila I, Cervantes F (2019) Building and evaluating user network profiles for cybersecurity using serverless architecture In: 2019 42nd International Conference on Telecommunications and Signal Processing (TSP), 164–167. https://doi.org/10.1109/TSP.2019.8768825 .
Ivanov V, Smolander K (2018) Implementation of a devops pipeline for serverless applications. In: Kuhrmann M, Schneider K, Pfahl D, Amasaki S, Ciolkowski M, Hebig R, Tell P, Klünder J, Küpper S (eds)Product-Focused Software Process Improvement, 48–64.. Springer, Cham. https://doi.org/10.1007/978-3-030-03673-7_4 .
Chen H, Zhang L-J (2018) Fbaas: Functional blockchain as a service. In: Chen S, Wang H, Zhang L-J (eds)Blockchain – ICBC 2018, 243–250.. Springer, Cham. https://doi.org/10.1007/978-3-319-94478-4_17 .
Lehvä J, Mäkitalo N, Mikkonen T (2018) Case study: Building a serverless messenger chatbot. In: Garrigós I Wimmer M (eds)Current Trends in Web Engineering, 75–86.. Springer, Cham. https://doi.org/10.1007/978-3-319-74433-9_6 .
Poth A, Schubert N, Riel A (2020) Sustainability efficiency challenges of modern it architectures – a quality model for serverless energy footprint. In: Yilmaz M, Niemann J, Clarke P, Messnarz R (eds)Systems, Software and Services Process Improvement, 289–301.. Springer, Cham.
Deese A (2018) Implementation of unsupervised k-means clustering algorithm within amazon web services lambda In: 2018 18th IEEE/ACM International Symposium on Cluster, Cloud and Grid Computing (CCGRID), 626–632. https://doi.org/10.1109/CCGRID.2018.00093 .
Jangda A, Pinckney D, Brun Y, Guha A (2019) Formal foundations of serverless computing. Proc ACM Program Lang 3(OOPSLA):1–26. https://doi.org/10.1145/3360575 .
Back T, Andrikopoulos V (2018) Using a microbenchmark to compare function as a service solutions. In: Kritikos K, Plebani P, de Paoli F (eds)Service-Oriented and Cloud Computing, 146–160.. Springer, Cham. https://doi.org/10.1007/978-3-319-99819-0\_11 .
Kritikos K, Skrzypek P (2018) A review of serverless frameworks In: 2018 IEEE/ACM International Conference on Utility and Cloud Computing Companion (UCC Companion), 161–168. https://doi.org/10.1109/UCC-Companion.2018.00051 .
Kritikos K, Skrzypek P (2019) Simulation-as-a-service with serverless computing In: 2019 IEEE World Congress on Services (SERVICES), vol. 2642-939X, 200–205. https://doi.org/10.1109/SERVICES.2019.00056 .
Ast M, Gaedke M (2017) Self-contained web components through serverless computing In: Proceedings of the 2nd International Workshop on Serverless Computing (WoSC ‘17), 28–33.. Association for Computing Machinery, New York. https://doi.org/10.1145/3154847.3154849 .
Trach B, Oleksenko O, Gregor F, Bhatotia P, Fetzer C (2019) Clemmys: Towards secure remote execution in faas In: Proceedings of the 12th ACM International Conference on Systems and Storage (SYSTOR ‘19), 44–54.. Association for Computing Machinery, New York. https://doi.org/10.1145/3319647.3325835 .
Aditya P, Akkus IE, Beck A, Chen R, Hilt V, Rimac I, Satzke K, Stein M (2019) Will serverless computing revolutionize nfv?Proc IEEE 107(4):667–678. https://doi.org/10.1109/JPROC.2019.2898101 .
Baresi L, Filgueira Mendonça D, Garriga M (2017) Empowering low-latency applications through a serverless edge computing architecture. In: De Paoli F, Schulte S, Broch Johnsen E (eds)Service-Oriented and Cloud Computing, 196–210.. Springer, Cham.
Tang Y, Yang J (2020) Lambdata: Optimizing serverless computing by making data intents explicit In: 2020 IEEE 13th International Conference on Cloud Computing (CLOUD), 294–303. https://doi.org/10.1109/CLOUD49709.2020.00049 .
Ali A, Pinciroli R, Yan F, Smirni E (2020) Batch: Machine learning inference serving on serverless platforms with adaptive batching In: Proceedings of the International Conference for High Performance Computing, Networking, Storage and Analysis (SC ’20), 1–15.
Christidis A, Moschoyiannis S, Hsu C-H, Davies R (2020) Enabling serverless deployment of large-scale ai workloads. IEEE Access 8:70150–70161. https://doi.org/10.1109/ACCESS.2020.2985282 .
Aske A, Zhao X (2018) Supporting multi-provider serverless computing on the edge In: Proceedings of the 47th International Conference on Parallel Processing Companion (ICPP ‘18).. Association for Computing Machinery, New York. https://doi.org/10.1145/3229710.3229742 .
Gunasekaran JR, Thinakaran P, Nachiappan NC, Srivatsa Kannan R, Kandemir MT, Das CR (2020) Characterizing bottlenecks in scheduling microservices on serverless platforms In: 2020 IEEE 40th International Conference on Distributed Computing Systems (ICDCS), 1197–1198. https://doi.org/10.1109/ICDCS47774.2020.00195 .
Dziurzanski P, Swan J, Indrusiak LS (2018) Value-based manufacturing optimisation in serverless clouds for industry 4.0 In: Proceedings of the Genetic and Evolutionary Computation Conference (GECCO ‘18), 1222–1229.. Association for Computing Machinery, New York. https://doi.org/10.1145/3205455.3205501 .
Mujezinović A, Ljubović V (2019) Serverless architecture for workflow scheduling with unconstrained execution environment In: 2019 42nd International Convention on Information and Communication Technology, Electronics and Microelectronics (MIPRO), 242–246. https://doi.org/10.23919/MIPRO.2019.8756833 .
Tricomi G, Giosa D, Merlino G, Romeo O, Longo F (2020) Toward a function-as-a-service framework for genomic analysis In: 2020 IEEE International Conference on Smart Computing (SMARTCOMP), 314–319. https://doi.org/10.1109/SMARTCOMP50058.2020.00070 .
Fingler H, Akshintala A, Rossbach CJ (2019) Usetl: Unikernels for serverless extract transform and load why should you settle for less? In: Proceedings of the 10th ACM SIGOPS Asia-Pacific Workshop on Systems (APSys ’19), 23–30.. Association for Computing Machinery, New York. https://doi.org/10.1145/3343737.3343750 .
Soltani B, Ghenai A, Zeghib N (2018) Towards distributed containerized serverless architecture in multi cloud environment. Proc Comput Sci 134:121–128. https://doi.org/10.1016/j.procs.2018.07.152. The 15th International Conference on Mobile Systems and Pervasive Computing (MobiSPC 2018) / The 13th International Conference on Future Networks and Communications (FNC-2018) / Affiliated Workshops.
Crespo-Cepeda R, Agapito G, Vazquez-Poletti JL, Cannataro M (2019) Challenges and opportunities of amazon serverless lambda services in bioinformatics In: Proceedings of the 10th ACM International Conference on Bioinformatics, Computational Biology and Health Informatics (BCB ‘19), 663–668.. Association for Computing Machinery, New York. https://doi.org/10.1145/3307339.3343462 .
Dash S, Dash DK (2016) Serverless cloud computing framework for smart grid architecture In: 2016 IEEE 7th Power India International Conference (PIICON), 1–6. https://doi.org/10.1109/POWERI.2016.8077240 .
Horovitz S, Amos R, Baruch O, Cohen T, Oyar T, Deri A (2019) Faastest - machine learning based cost and performance faas optimization. In: Coppola M, Carlini E, D’Agostino D, Altmann J, Bañares JÁ (eds)Economics of Grids, Clouds, Systems, and Services, 171–186.. Springer, Cham. https://doi.org/10.1007/978-3-030-13342-9\_15 .
Bardsley D, Ryan L, Howard J (2018) Serverless performance and optimization strategies In: 2018 IEEE International Conference on Smart Cloud (SmartCloud), 19–26. https://doi.org/10.1109/SmartCloud.2018.00012 .
Jackson D, Clynch G (2018) An investigation of the impact of language runtime on the performance and cost of serverless functions In: 2018 IEEE/ACM International Conference on Utility and Cloud Computing Companion (UCC Companion), 154–160. https://doi.org/10.1109/UCC-Companion.2018.00050 .
Kaplunovich A (2019) Tolambda–automatic path to serverless architectures In: 2019 IEEE/ACM 3rd International Workshop on Refactoring (IWoR), 1–8. https://doi.org/10.1109/IWoR.2019.00008 .
Lee H, Satyam K, Fox G (2018) Evaluation of production serverless computing environments In: 2018 IEEE 11th International Conference on Cloud Computing (CLOUD), 442–450. https://doi.org/10.1109/CLOUD.2018.00062 .
Asghar T, Rasool S, Iqbal MU, Qayyum Z, Mian AN, Ubakanma G (2018) Feasibility of serverless cloud services for disaster management information systems In: 2018 IEEE 20th International Conference on High Performance Computing and Communications; IEEE 16th International Conference on Smart City; IEEE 4th International Conference on Data Science and Systems (HPCC/SmartCity/DSS), 1054–1057. https://doi.org/10.1109/HPCC/SmartCity/DSS.2018.00175 .
McGrath G, Brenner PR (2017) Serverless computing: Design, implementation, and performance In: 2017 IEEE 37th International Conference on Distributed Computing Systems Workshops (ICDCSW), 405–410. https://doi.org/10.1109/ICDCSW.2017.36 .
Christoforou A, Andreou AS (2018) An effective resource management approach in a faas environment In: ESSCA@UCC, 2–8.
Rajan RAP (2018) Serverless architecture - a revolution in cloud computing In: 2018 Tenth International Conference on Advanced Computing (ICoAC), 88–93. https://doi.org/10.1109/ICoAC44903.2018.8939081 .
Rahman MM, Hasibul Hasan M (2019) Serverless architecture for big data analytics In: 2019 Global Conference for Advancement in Technology (GCAT), 1–5. https://doi.org/10.1109/GCAT47503.2019.8978443 .
Huber F, Körber N, Mock M (2019) Selena: A serverless energy management system In: Proceedings of the 5th International Workshop on Serverless Computing (WOSC ‘19), 7–12.. Association for Computing Machinery, New York. https://doi.org/10.1145/3366623.3368134 .
Aytekin A, Johansson M (2019) Exploiting serverless runtimes for large-scale optimization In: 2019 IEEE 12th International Conference on Cloud Computing (CLOUD), 499–501. https://doi.org/10.1109/CLOUD.2019.00090 .
Gabbrielli M, Giallorenzo S, Lanese I, Montesi F, Peressotti M, Zingaro SP (2019) No more, no less: A formal model for serverless computing. In: Riis Nielson H Tuosto E (eds)Coordination Models and Languages, 148–157.. Springer, Cham. https://doi.org/10.1007/978-3-030-22397-7_9 .
Fasogbon P, You Y, Aksu E (2020) 3d human model creation on a serverless environment In: 2020 IEEE International Symposium on Mixed and Augmented Reality Adjunct (ISMAR-Adjunct), 118–122. https://doi.org/10.1109/ISMAR-Adjunct51615.2020.00044 .
Dash S, Sodhi R, Sodhi B (2020) A serverless cloud computing framework for real-time appliance-usage recommendation In: 2020 21st National Power Systems Conference (NPSC), 1–6. https://doi.org/10.1109/NPSC49263.2020.9331847 .
Thong Tran T, Zhang Y-C, Liao W-T, Lin Y-J, Li M-C, Huang H-S (2020) An autonomous mobile robot system based on serverless computing and edge computing In: 2020 21st Asia-Pacific Network Operations and Management Symposium (APNOMS), 334–337. https://doi.org/10.23919/APNOMS50412.2020.9236976 .
Witte PA, Louboutin M, Modzelewski H, Jones C, Selvage J, Herrmann FJ (2020) An event-driven approach to serverless seismic imaging in the cloud. IEEE Trans Parallel Distributed Syst 31(9):2032–2049. https://doi.org/10.1109/TPDS.2020.2982626 .
Ghosh BC, Addya SK, Somy NB, Nath SB, Chakraborty S, Ghosh SK (2020) Caching techniques to improve latency in serverless architectures In: 2020 International Conference on COMmunication Systems NETworkS (COMSNETS), 666–669. https://doi.org/10.1109/COMSNETS48256.2020.9027427 .
Quaresma D, Fireman D, Pereira TE (2020) Controlling garbage collection and request admission to improve performance of faas applications In: 2020 IEEE 32nd International Symposium on Computer Architecture and High Performance Computing (SBAC-PAD), 175–182. https://doi.org/10.1109/SBAC-PAD49847.2020.00033 .
Birman Y, Hindi S, Katz G, Shabtai A (2020) Cost-effective malware detection as a service over serverless cloud using deep reinforcement learning In: 2020 20th IEEE/ACM International Symposium on Cluster, Cloud and Internet Computing (CCGRID), 420–429. https://doi.org/10.1109/CCGrid49817.2020.00-51 .
Quang T, Peng Y (2020) Device-driven on-demand deployment of serverless computing functions In: 2020 IEEE International Conference on Pervasive Computing and Communications Workshops (PerCom Workshops), 1–6. https://doi.org/10.1109/PerComWorkshops48775.2020.9156140 .
Gunasekaran JR, Thinakaran P, Nachiappan NC, Kandemir MT, Das CR (2020) Fifer: Tackling Resource Underutilization in the Serverless Era. Association for Computing Machinery, New York.
Müller I, Marroquín R, Alonso G (2020) Lambada: Interactive data analytics on cold data using serverless cloud infrastructure In: Proceedings of the 2020 ACM SIGMOD International Conference on Management of Data (SIGMOD ’20), 115–130.. Association for Computing Machinery, New York. https://doi.org/10.1145/3318464.3389758 .
Chahal D, Ojha R, Ramesh M, Singhal R (2020) Migrating large deep learning models to serverless architecture In: 2020 IEEE International Symposium on Software Reliability Engineering Workshops (ISSREW), 111–116. https://doi.org/10.1109/ISSREW51248.2020.00047 .
Jain P, Munjal Y, Gera J, Gupta P (2020) Performance analysis of various server hosting techniques. Proc Comput Sci 173:70–77. https://doi.org/10.1016/j.procs.2020.06.010. International Conference on Smart Sustainable Intelligent Computing and Applications under ICITETM2020.
Khatri D, Khatri SK, Mishra D (2020) Potential bottleneck and measuring performance of serverless computing: A literature study In: 2020 8th International Conference on Reliability, Infocom Technologies and Optimization (Trends and Future Directions) (ICRITO), 161–164. https://doi.org/10.1109/ICRITO48877.2020.9197837 .
Kehrer S, Zietlow D, Scheffold J, Blochinger W (2020) Self-tuning serverless task farming using proactive elasticity control. Clust Comput. https://doi.org/10.1007/s10586-020-03158-3 .
Kelly D, Glavin F, Barrett E (2020) Serverless computing: Behind the scenes of major platforms In: 2020 IEEE 13th International Conference on Cloud Computing (CLOUD), 304–312. https://doi.org/10.1109/CLOUD49709.2020.00050 .
Ivan C, Vasile R, Dadarlat V (2019) Serverless computing: An investigation of deployment environments for web apis. Computers 8(2). https://doi.org/10.3390/computers8020050 .
Baresi L, Filgueira Mendonça D (2019) Towards a serverless platform for edge computing In: 2019 IEEE International Conference on Fog Computing (ICFC), 1–10. https://doi.org/10.1109/ICFC.2019.00008 .
Hall A, Ramachandran U (2019) An execution model for serverless functions at the edge In: Proceedings of the International Conference on Internet of Things Design and Implementation (IoTDI ‘19), 225–236.. Association for Computing Machinery, New York. https://doi.org/10.1145/3302505.3310084 .
Akkus IE, Chen R, Rimac I, Stein M, Satzke K, Beck A, Aditya P, Hilt V (2018) Sand: Towards high-performance serverless computing In: Proceedings of the 2018 USENIX Conference on Usenix Annual Technical Conference (USENIX ATC ‘18), 923–935.. USENIX Association, USA.
Keshavarzian A, Sharifian S, Seyedin S (2019) Modified deep residual network architecture deployed on serverless framework of iot platform based on human activity recognition application. Futur Gener Comput Syst 101:14–28. https://doi.org/10.1016/j.future.2019.06.009 .
Palade A, Kazmi A, Clarke S (2019) An evaluation of open source serverless computing frameworks support at the edge In: 2019 IEEE World Congress on Services (SERVICES), vol. 2642-939X, 206–211. https://doi.org/10.1109/SERVICES.2019.00057 .
Shahrad M, Balkind J, Wentzlaff D (2019) Architectural implications of function-as-a-service computing In: Proceedings of the 52nd Annual IEEE/ACM International Symposium on Microarchitecture (MICRO ‘52), 1063–1075.. Association for Computing Machinery, New York. https://doi.org/10.1145/3352460.3358296 .
Cicconetti C, Conti M, Passarella A (2020) A decentralized framework for serverless edge computing in the internet of things. IEEE Trans Netw Serv Manag:1–1. https://doi.org/10.1109/TNSM.2020.3023305 .
De Palma G, Giallorenzo S, Mauro J, Zavattaro G (2020) Allocation priority policies for serverless function-execution scheduling optimisation. In: Kafeza E, Benatallah B, Martinelli F, Hacid H, Bouguettaya A, Motahari H (eds)Service-Oriented Computing, 416–430.. Springer, Cham.
Djemame K, Parker M, Datsev D (2020) Open-source serverless architectures: an evaluation of apache openwhisk In: 2020 IEEE/ACM 13th International Conference on Utility and Cloud Computing (UCC), 329–335. https://doi.org/10.1109/UCC48980.2020.00052 .
Chadha M, Jindal A, Gerndt M (2020) Towards federated learning using faas fabric In: Proceedings of the 2020 Sixth International Workshop on Serverless Computing (WoSC’20), 49–54.. Association for Computing Machinery, New York. https://doi.org/10.1145/3429880.3430100 .
Cheng B, Fuerst J, Solmaz G, Sanada T (2019) Fog function: Serverless fog computing for data intensive iot services In: 2019 IEEE International Conference on Services Computing (SCC), 28–35. https://doi.org/10.1109/SCC.2019.00018 .
Mohanty SK, Premsankar G, di Francesco M (2018) An evaluation of open source serverless computing frameworks In: 2018 IEEE International Conference on Cloud Computing Technology and Science (CloudCom), 115–120. https://doi.org/10.1109/CloudCom2018.2018.00033 .
Li J, Kulkarni SG, Ramakrishnan KK, Li D (2019) Understanding open source serverless platforms: Design considerations and performance In: Proceedings of the 5th International Workshop on Serverless Computing (WOSC ‘19), 37–42.. Association for Computing Machinery, New York. https://doi.org/10.1145/3366623.3368139 .
Nguyen HD, Zhang C, Xiao Z, Chien AA (2019) Real-time serverless: Enabling application performance guarantees In: Proceedings of the 5th International Workshop on Serverless Computing (WOSC ’19), 1–6.. Association for Computing Machinery, New York. https://doi.org/10.1145/3366623.3368133 .
Saha A, Jindal S (2018) Emars: Efficient management and allocation of resources in serverless In: 2018 IEEE 11th International Conference on Cloud Computing (CLOUD), 827–830. https://doi.org/10.1109/CLOUD.2018.00113 .
Solaiman K, Adnan MA (2020) Wlec: A not so cold architecture to mitigate cold start problem in serverless computing In: 2020 IEEE International Conference on Cloud Engineering (IC2E), 144–153. https://doi.org/10.1109/IC2E48712.2020.00022 .
Chan A, Wang K-TA, Kumar V (2019) BalloonJVM : Dynamically Resizable Heap for FaaS In: CLOUD COMPUTING 2019 : The Tenth International Conference on Cloud Computing, GRIDs, and Virtualization, 99–104.
Handoyo E, Arfan M, Soetrisno YAA, Somantri M, Sofwan A, Sinuraya EW (2018) Ticketing chatbot service using serverless nlp technology In: 2018 5th International Conference on Information Technology, Computer, and Electrical Engineering (ICITACEE), 325–330. https://doi.org/10.1109/ICITACEE.2018.8576921 .
Zhang M, Zhu Y, Zhang C, Liu J (2019) Video processing with serverless computing: A measurement study In: Proceedings of the 29th ACM Workshop on Network and Operating Systems Support for Digital Audio and Video (NOSSDAV ’19), 61–66.. Association for Computing Machinery, New York. https://doi.org/10.1145/3304112.3325608 .
Fan D, He D (2020) A scheduler for serverless framework base on kubernetes In: Proceedings of the 2020 4th High Performance Computing and Cluster Technologies Conference; 2020 3rd International Conference on Big Data and Artificial Intelligence (HPCCT; BDAI 2020), 229–232.. Association for Computing Machinery, New York. https://doi.org/10.1145/3409501.3409503 .
Thomas S, Ao L, Voelker GM, Porter G (2020) Particle: Ephemeral endpoints for serverless networking In: Proceedings of the 11th ACM Symposium on Cloud Computing (SoCC ’20), 16–29.. Association for Computing Machinery, New York. https://doi.org/10.1145/3419111.3421275 .
Gramaglia M, Serrano P, Banchs A, Garcia-Aviles G, Garcia-Saavedra A, Perez R (2020) The case for serverless mobile networking In: 2020 IFIP Networking Conference (Networking), 779–784.
Danayi A, Sharifian S (2018) Pess-mina: A proactive stochastic task allocation algorithm for faas edge-cloud environments In: 2018 4th Iranian Conference on Signal Processing and Intelligent Systems (ICSPIS), 27–31. https://doi.org/10.1109/ICSPIS.2018.8700543 .
Mendki P (2020) Evaluating webassembly enabled serverless approach for edge computing In: 2020 IEEE Cloud Summit, 161–166. https://doi.org/10.1109/IEEECloudSummit48914.2020.00031 .
Chaudhry SR, Palade A, Kazmi A, Clarke S (2020) Improved qos at the edge using serverless computing to deploy virtual network functions. IEEE Internet Things J 7(10):10673–10683. https://doi.org/10.1109/JIOT.2020.3011057 .
Benedict S (2020) Serverless blockchain-enabled architecture for iot societal applications. IEEE Trans Comput Soc Syst 7(5):1146–1158. https://doi.org/10.1109/TCSS.2020.3008995 .
Cicconetti C, Conti M, Passarella A (2020) Uncoordinated access to serverless computing in mec systems for iot. Comput Netw 172:107184. https://doi.org/10.1016/j.comnet.2020.107184 .
Qiang W, Dong Z, Jin H (2018) Se-lambda: Securing privacy-sensitive serverless applications using sgx enclave. In: Beyah R, Chang B, Li Y, Zhu S (eds)Security and Privacy in Communication Networks, 451–470.. Springer, Cham. https://doi.org/10.1007/978-3-030-01701-9\_25 .
Prasetyadi G, Hantoro UT, Mutiara AB, Muslim A, Refianti R (2019) Heresy: A serverless web application to store compressed and encrypted document in the form of url In: 2019 Fourth International Conference on Informatics and Computing (ICIC), 1–5. https://doi.org/10.1109/ICIC47613.2019.8985735 .
Kim B, Heo S, Lee J, Jeong S, Lee Y, Kim H (2020) Compiler-assisted semantic-aware encryption for efficient and secure serverless computing. IEEE Internet Things J:1–1. https://doi.org/10.1109/JIOT.2020.3031550 .
O’Meara W, Lennon RG (2020) Serverless computing security: Protecting application logic In: 2020 31st Irish Signals and Systems Conference (ISSC), 1–5. https://doi.org/10.1109/ISSC49989.2020.9180214 .
Agache A, Brooker M, Iordache A, Liguori A, Neugebauer R, Piwonka P, Popa D-M (2020) Firecracker: Lightweight virtualization for serverless applications In: 17th USENIX Symposium on Networked Systems Design and Implementation (NSDI 20), 419–434.. USENIX Association, Santa Clara.
Pinto D, Dias JP, Sereno Ferreira H2018. Dynamic allocation of serverless functions in iot environments. https://doi.org/10.1109/euc.2018.00008 .
Mejáa A, Marcillo D, Guaño M, Gualotuña T (2020) Serverless based control and monitoring for search and rescue robots In: 2020 15th Iberian Conference on Information Systems and Technologies (CISTI), 1–6. https://doi.org/10.23919/CISTI49556.2020.9140444 .
Meißner D, Erb B, Kargl F, Tichy M (2018) Retro- λ : An event-sourced platform for serverless applications with retroactive computing support In: Proceedings of the 12th ACM International Conference on Distributed and Event-Based Systems (DEBS ‘18), 76–87.. Association for Computing Machinery, New York. https://doi.org/10.1145/3210284.3210285 .
Zhang T, Xie D, Li F, Stutsman R (2019) Narrowing the gap between serverless and its state with storage functions In: Proceedings of the ACM Symposium on Cloud Computing (SoCC ‘19), 1–12.. Association for Computing Machinery, New York. https://doi.org/10.1145/3357223.3362723 .
Grogan J, Mulready C, McDermott J, Urbanavicius M, Yilmaz M, Abgaz Y, McCarren A, MacMahon ST, Garousi V, Elger P, Clarke P (2020) A multivocal literature review of function-as-a-service (faas) infrastructures and implications for software developers. In: Yilmaz M, Niemann J, Clarke P, Messnarz R (eds)Systems, Software and Services Process Improvement, 58–75.. Springer, Cham.
Qin S, Wu H, Wu Y, Yan B, Xu Y, Zhang W (2020) Nuka: A generic engine with millisecond initialization for serverless computing In: 2020 IEEE International Conference on Joint Cloud Computing, 78–85. https://doi.org/10.1109/JCC49151.2020.00021 .
Zuk P, Rzadca K (2020) Scheduling methods to reduce response latency of function as a service In: 2020 IEEE 32nd International Symposium on Computer Architecture and High Performance Computing (SBAC-PAD), 132–140. https://doi.org/10.1109/SBAC-PAD49847.2020.00028 .
Shen J, Yu H, Zheng Z, Sun C, Xu M, Wang J (2020) Serpens: A high-performance serverless platform for nfv In: 2020 IEEE/ACM 28th International Symposium on Quality of Service (IWQoS), 1–10. https://doi.org/10.1109/IWQoS49365.2020.9213030 .
Ginzburg S, Freedman MJ (2020) Serverless isn’t server-less: Measuring and exploiting resource variability on cloud faas platforms In: Proceedings of the 2020 Sixth International Workshop on Serverless Computing (WoSC’20), 43–48.. Association for Computing Machinery, New York. https://doi.org/10.1145/3429880.3430099 .
Tankov V, Golubev Y, Bryksin T (2019) Kotless: A serverless framework for kotlin In: 2019 34th IEEE/ACM International Conference on Automated Software Engineering (ASE), 1110–1113. https://doi.org/10.1109/ASE.2019.00114 .
Vahidinia P, Farahani B, Aliee FS (2020) Cold start in serverless computing: Current trends and mitigation strategies In: 2020 International Conference on Omni-layer Intelligent Systems (COINS), 1–7. https://doi.org/10.1109/COINS49042.2020.9191377 .
Silva P, Fireman D, Pereira TE (2020) Prebaking Functions to Warm the Serverless Cold Start. Association for Computing Machinery, New York.
Tan B, Liu H, Rao J, Liao X, Jin H, Zhang Y (2020) Towards lightweight serverless computing via unikernel as a function In: 2020 IEEE/ACM 28th International Symposium on Quality of Service (IWQoS), 1–10. https://doi.org/10.1109/IWQoS49365.2020.9213020 .
Cordasco G, D’Auria M, Negro A, Scarano V, Spagnuolo C (2020) Fly: A domain-specific language for scientific computing on faas. In: Schwardmann U, Boehme C, B. Heras D, Cardellini V, Jeannot E, Salis A, Schifanella C, Manumachu RR, Schwamborn D, Ricci L, Sangyoon O, Gruber T, Antonelli L, Scott SL (eds)Euro-Par 2019: Parallel Processing Workshops, 531–544.. Springer, Cham.
Nupponen J, Taibi D (2020) Serverless: What it is, what to do and what not to do In: 2020 IEEE International Conference on Software Architecture Companion (ICSA-C), 49–50. https://doi.org/10.1109/ICSA-C50368.2020.00016 .
Reuter A, Back T, Andrikopoulos V (2020) Cost efficiency under mixed serverless and serverful deployments In: 2020 46th Euromicro Conference on Software Engineering and Advanced Applications (SEAA), 242–245. https://doi.org/10.1109/SEAA51224.2020.00049 .
Mahajan K, Figueiredo D, Misra V, Rubenstein D (2019) Optimal pricing for serverless computing In: 2019 IEEE Global Communications Conference (GLOBECOM), 1–6. https://doi.org/10.1109/GLOBECOM38437.2019.9013156 .
Lynn T, Rosati P, Lejeune A, Emeakaroha V (2017) A preliminary review of enterprise serverless cloud computing (function-as-a-service) platforms In: 2017 IEEE International Conference on Cloud Computing Technology and Science (CloudCom), 162–169. https://doi.org/10.1109/CloudCom.2017.15 .
Enes J, Expósito RR, Touriño J (2020) Real-time resource scaling platform for big data workloads on serverless environments. Futur Gener Comput Syst 105:361–379. https://doi.org/10.1016/j.future.2019.11.037 .
Elsakhawy M, Bauer M (2020) Faas2f: A framework for defining execution-sla in serverless computing In: 2020 IEEE Cloud Summit, 58–65. https://doi.org/10.1109/IEEECloudSummit48914.2020.00015 .
Bhattacharjee A, Chhokra AD, Kang Z, Sun H, Gokhale A, Karsai G (2019) Barista: Efficient and scalable serverless serving system for deep learning prediction services In: 2019 IEEE International Conference on Cloud Engineering (IC2E), 23–33. https://doi.org/10.1109/IC2E.2019.00-10 .
Maissen P, Felber P, Kropf P, Schiavoni V (2020) Faasdom: A benchmark suite for serverless computing In: Proceedings of the 14th ACM International Conference on Distributed and Event-Based Systems (DEBS ’20), 73–84.. Association for Computing Machinery, New York. https://doi.org/10.1145/3401025.3401738 .
Xu Z, Zhang H, Geng X, Wu Q, Ma H (2019) Adaptive function launching acceleration in serverless computing platforms In: 2019 IEEE 25th International Conference on Parallel and Distributed Systems (ICPADS), 9–16. https://doi.org/10.1109/ICPADS47876.2019.00011 .
Soltani B, Ghenai A, Zeghib N (2018) A migration-based approach to execute long-duration multi-cloud serverless functions. In: Maamri R Belala F (eds)Proceedings of the 3rd International Conference on Advanced Aspects of Software Engineering, ICAASE 2018, Constantine, Algeria, December 1-2, 2018 (CEUR Workshop Proceedings), vol. 2326, 42–50.
Martins H, Araujo F, da Cunha PR (2020) Benchmarking serverless computing platforms. J Grid Comput 18(4):691–709. https://doi.org/10.1007/s10723-020-09523-1 .
Download references
Acknowledgements
Author information, authors and affiliations.
Software Engineering and Embedded Systems (SEES) Research Group, College of Medicine, University of Duhok, Duhok, Kurdistan Region, Iraq
Hassan B. Hassan
Software Engineering and Embedded Systems (SEES) Research Group, Department of Computer Science, College of Science, University of Duhok, Duhok, Kurdistan Region, Iraq
Saman A. Barakat & Qusay I. Sarhan
You can also search for this author in PubMed Google Scholar
Contributions
Authors’ contributions.
Conceptualization: HBH, SAB, and QIS; methodology: HBH, SAB, and QIS; validation: HBH, SAB, and QIS; formal analysis: HBH, SAB, and QIS; investigation: HBH, SAB, and QIS; resources: HBH; data curation, HBH and SAB; writing—original draft preparation: HBH, SAB, and QIS; writing—review and editing: HBH, SAB, and QIS; visualization: SAB; supervision: QIS; It is noted that all authors cooperated with each other to achieve suitable information flow across the entire paper. The authors read and approved the final manuscript.
Authors’ information
Hassan B. Hassan received the B.Sc. degree in Computer Science from University of Duhok, Iraq, in 2010. He completed the M.Sc. degree in Web Applications and Services, from Leicester University, UK, in 2015. He is currently working as an assistant lecturer at the college of medicine, University of Duhok, Iraq. His main areas of research interest are cloud computing, web programming, big data, and human computer interaction.
Saman A. Barakat received the B.Sc. degree in Computer Science from University of Duhok, Iraq, in 2008. He completed the M.Sc. degree in Advanced Computer Science, from Newcastle University, UK, in 2012. He is currently working as a lecturer at the college of science, University of Duhok, Iraq. His main areas of research interest are cloud computing, and software engineering.
Qusay I. Sarhan received the B.Sc. degree in Software Engineering from University of Mosul, Iraq, in 2007 and the M.Tech. degree in Software Engineering from Jawaharlal Nehru Technological University, India, in 2011. Currently, he is a lecturer and the leader of Software Engineering and Embedded Systems (SEES) research group at University of Duhok, Iraq. He has a couple of national and international publications and his research interests include software engineering, internet of things, and embedded systems.
Corresponding author
Correspondence to Qusay I. Sarhan .
Ethics declarations
Competing interests.
The authors declare that they have no competing interests.
Additional information
Publisher’s note.
Springer Nature remains neutral with regard to jurisdictional claims in published maps and institutional affiliations.
Rights and permissions
Open Access This article is licensed under a Creative Commons Attribution 4.0 International License, which permits use, sharing, adaptation, distribution and reproduction in any medium or format, as long as you give appropriate credit to the original author(s) and the source, provide a link to the Creative Commons licence, and indicate if changes were made. The images or other third party material in this article are included in the article’s Creative Commons licence, unless indicated otherwise in a credit line to the material. If material is not included in the article’s Creative Commons licence and your intended use is not permitted by statutory regulation or exceeds the permitted use, you will need to obtain permission directly from the copyright holder. To view a copy of this licence, visit http://creativecommons.org/licenses/by/4.0/ .
Reprints and permissions
About this article
Cite this article.
Hassan, H.B., Barakat, S.A. & Sarhan, Q.I. Survey on serverless computing. J Cloud Comp 10 , 39 (2021). https://doi.org/10.1186/s13677-021-00253-7
Download citation
Received : 01 July 2020
Accepted : 21 June 2021
Published : 12 July 2021
DOI : https://doi.org/10.1186/s13677-021-00253-7
Share this article
Anyone you share the following link with will be able to read this content:
Sorry, a shareable link is not currently available for this article.
Provided by the Springer Nature SharedIt content-sharing initiative
- Cloud computing
- Serverless computing
- Serverless platforms
- Serverless benefits
- Serverless challenges
computer science Recently Published Documents
Total documents.
- Latest Documents
- Most Cited Documents
- Contributed Authors
- Related Sources
- Related Keywords
Hiring CS Graduates: What We Learned from Employers
Computer science ( CS ) majors are in high demand and account for a large part of national computer and information technology job market applicants. Employment in this sector is projected to grow 12% between 2018 and 2028, which is faster than the average of all other occupations. Published data are available on traditional non-computer science-specific hiring processes. However, the hiring process for CS majors may be different. It is critical to have up-to-date information on questions such as “what positions are in high demand for CS majors?,” “what is a typical hiring process?,” and “what do employers say they look for when hiring CS graduates?” This article discusses the analysis of a survey of 218 recruiters hiring CS graduates in the United States. We used Atlas.ti to analyze qualitative survey data and report the results on what positions are in the highest demand, the hiring process, and the resume review process. Our study revealed that a software developer was the most common job the recruiters were looking to fill. We found that the hiring process steps for CS graduates are generally aligned with traditional hiring steps, with an additional emphasis on technical and coding tests. Recruiters reported that their hiring choices were based on reviewing resume’s experience, GPA, and projects sections. The results provide insights into the hiring process, decision making, resume analysis, and some discrepancies between current undergraduate CS program outcomes and employers’ expectations.
A Systematic Literature Review of Empiricism and Norms of Reporting in Computing Education Research Literature
Context. Computing Education Research (CER) is critical to help the computing education community and policy makers support the increasing population of students who need to learn computing skills for future careers. For a community to systematically advance knowledge about a topic, the members must be able to understand published work thoroughly enough to perform replications, conduct meta-analyses, and build theories. There is a need to understand whether published research allows the CER community to systematically advance knowledge and build theories. Objectives. The goal of this study is to characterize the reporting of empiricism in Computing Education Research literature by identifying whether publications include content necessary for researchers to perform replications, meta-analyses, and theory building. We answer three research questions related to this goal: (RQ1) What percentage of papers in CER venues have some form of empirical evaluation? (RQ2) Of the papers that have empirical evaluation, what are the characteristics of the empirical evaluation? (RQ3) Of the papers that have empirical evaluation, do they follow norms (both for inclusion and for labeling of information needed for replication, meta-analysis, and, eventually, theory-building) for reporting empirical work? Methods. We conducted a systematic literature review of the 2014 and 2015 proceedings or issues of five CER venues: Technical Symposium on Computer Science Education (SIGCSE TS), International Symposium on Computing Education Research (ICER), Conference on Innovation and Technology in Computer Science Education (ITiCSE), ACM Transactions on Computing Education (TOCE), and Computer Science Education (CSE). We developed and applied the CER Empiricism Assessment Rubric to the 427 papers accepted and published at these venues over 2014 and 2015. Two people evaluated each paper using the Base Rubric for characterizing the paper. An individual person applied the other rubrics to characterize the norms of reporting, as appropriate for the paper type. Any discrepancies or questions were discussed between multiple reviewers to resolve. Results. We found that over 80% of papers accepted across all five venues had some form of empirical evaluation. Quantitative evaluation methods were the most frequently reported. Papers most frequently reported results on interventions around pedagogical techniques, curriculum, community, or tools. There was a split in papers that had some type of comparison between an intervention and some other dataset or baseline. Most papers reported related work, following the expectations for doing so in the SIGCSE and CER community. However, many papers were lacking properly reported research objectives, goals, research questions, or hypotheses; description of participants; study design; data collection; and threats to validity. These results align with prior surveys of the CER literature. Conclusions. CER authors are contributing empirical results to the literature; however, not all norms for reporting are met. We encourage authors to provide clear, labeled details about their work so readers can use the study methodologies and results for replications and meta-analyses. As our community grows, our reporting of CER should mature to help establish computing education theory to support the next generation of computing learners.
Light Diacritic Restoration to Disambiguate Homographs in Modern Arabic Texts
Diacritic restoration (also known as diacritization or vowelization) is the process of inserting the correct diacritical markings into a text. Modern Arabic is typically written without diacritics, e.g., newspapers. This lack of diacritical markings often causes ambiguity, and though natives are adept at resolving, there are times they may fail. Diacritic restoration is a classical problem in computer science. Still, as most of the works tackle the full (heavy) diacritization of text, we, however, are interested in diacritizing the text using a fewer number of diacritics. Studies have shown that a fully diacritized text is visually displeasing and slows down the reading. This article proposes a system to diacritize homographs using the least number of diacritics, thus the name “light.” There is a large class of words that fall under the homograph category, and we will be dealing with the class of words that share the spelling but not the meaning. With fewer diacritics, we do not expect any effect on reading speed, while eye strain is reduced. The system contains morphological analyzer and context similarities. The morphological analyzer is used to generate all word candidates for diacritics. Then, through a statistical approach and context similarities, we resolve the homographs. Experimentally, the system shows very promising results, and our best accuracy is 85.6%.
A genre-based analysis of questions and comments in Q&A sessions after conference paper presentations in computer science
Gender diversity in computer science at a large public r1 research university: reporting on a self-study.
With the number of jobs in computer occupations on the rise, there is a greater need for computer science (CS) graduates than ever. At the same time, most CS departments across the country are only seeing 25–30% of women students in their classes, meaning that we are failing to draw interest from a large portion of the population. In this work, we explore the gender gap in CS at Rutgers University–New Brunswick, a large public R1 research university, using three data sets that span thousands of students across six academic years. Specifically, we combine these data sets to study the gender gaps in four core CS courses and explore the correlation of several factors with retention and the impact of these factors on changes to the gender gap as students proceed through the CS courses toward completing the CS major. For example, we find that a significant percentage of women students taking the introductory CS1 course for majors do not intend to major in CS, which may be a contributing factor to a large increase in the gender gap immediately after CS1. This finding implies that part of the retention task is attracting these women students to further explore the major. Results from our study include both novel findings and findings that are consistent with known challenges for increasing gender diversity in CS. In both cases, we provide extensive quantitative data in support of the findings.
Designing for Student-Directedness: How K–12 Teachers Utilize Peers to Support Projects
Student-directed projects—projects in which students have individual control over what they create and how to create it—are a promising practice for supporting the development of conceptual understanding and personal interest in K–12 computer science classrooms. In this article, we explore a central (and perhaps counterintuitive) design principle identified by a group of K–12 computer science teachers who support student-directed projects in their classrooms: in order for students to develop their own ideas and determine how to pursue them, students must have opportunities to engage with other students’ work. In this qualitative study, we investigated the instructional practices of 25 K–12 teachers using a series of in-depth, semi-structured interviews to develop understandings of how they used peer work to support student-directed projects in their classrooms. Teachers described supporting their students in navigating three stages of project development: generating ideas, pursuing ideas, and presenting ideas. For each of these three stages, teachers considered multiple factors to encourage engagement with peer work in their classrooms, including the quality and completeness of shared work and the modes of interaction with the work. We discuss how this pedagogical approach offers students new relationships to their own learning, to their peers, and to their teachers and communicates important messages to students about their own competence and agency, potentially contributing to aims within computer science for broadening participation.
Creativity in CS1: A Literature Review
Computer science is a fast-growing field in today’s digitized age, and working in this industry often requires creativity and innovative thought. An issue within computer science education, however, is that large introductory programming courses often involve little opportunity for creative thinking within coursework. The undergraduate introductory programming course (CS1) is notorious for its poor student performance and retention rates across multiple institutions. Integrating opportunities for creative thinking may help combat this issue by adding a personal touch to course content, which could allow beginner CS students to better relate to the abstract world of programming. Research on the role of creativity in computer science education (CSE) is an interesting area with a lot of room for exploration due to the complexity of the phenomenon of creativity as well as the CSE research field being fairly new compared to some other education fields where this topic has been more closely explored. To contribute to this area of research, this article provides a literature review exploring the concept of creativity as relevant to computer science education and CS1 in particular. Based on the review of the literature, we conclude creativity is an essential component to computer science, and the type of creativity that computer science requires is in fact, a teachable skill through the use of various tools and strategies. These strategies include the integration of open-ended assignments, large collaborative projects, learning by teaching, multimedia projects, small creative computational exercises, game development projects, digitally produced art, robotics, digital story-telling, music manipulation, and project-based learning. Research on each of these strategies and their effects on student experiences within CS1 is discussed in this review. Last, six main components of creativity-enhancing activities are identified based on the studies about incorporating creativity into CS1. These components are as follows: Collaboration, Relevance, Autonomy, Ownership, Hands-On Learning, and Visual Feedback. The purpose of this article is to contribute to computer science educators’ understanding of how creativity is best understood in the context of computer science education and explore practical applications of creativity theory in CS1 classrooms. This is an important collection of information for restructuring aspects of future introductory programming courses in creative, innovative ways that benefit student learning.
CATS: Customizable Abstractive Topic-based Summarization
Neural sequence-to-sequence models are the state-of-the-art approach used in abstractive summarization of textual documents, useful for producing condensed versions of source text narratives without being restricted to using only words from the original text. Despite the advances in abstractive summarization, custom generation of summaries (e.g., towards a user’s preference) remains unexplored. In this article, we present CATS, an abstractive neural summarization model that summarizes content in a sequence-to-sequence fashion while also introducing a new mechanism to control the underlying latent topic distribution of the produced summaries. We empirically illustrate the efficacy of our model in producing customized summaries and present findings that facilitate the design of such systems. We use the well-known CNN/DailyMail dataset to evaluate our model. Furthermore, we present a transfer-learning method and demonstrate the effectiveness of our approach in a low resource setting, i.e., abstractive summarization of meetings minutes, where combining the main available meetings’ transcripts datasets, AMI and International Computer Science Institute(ICSI) , results in merely a few hundred training documents.
Exploring students’ and lecturers’ views on collaboration and cooperation in computer science courses - a qualitative analysis
Factors affecting student educational choices regarding oer material in computer science, export citation format, share document.
Reference management. Clean and simple.
The top list of academic search engines
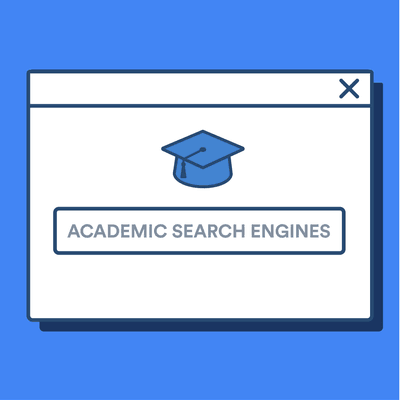
1. Google Scholar
4. science.gov, 5. semantic scholar, 6. baidu scholar, get the most out of academic search engines, frequently asked questions about academic search engines, related articles.
Academic search engines have become the number one resource to turn to in order to find research papers and other scholarly sources. While classic academic databases like Web of Science and Scopus are locked behind paywalls, Google Scholar and others can be accessed free of charge. In order to help you get your research done fast, we have compiled the top list of free academic search engines.
Google Scholar is the clear number one when it comes to academic search engines. It's the power of Google searches applied to research papers and patents. It not only lets you find research papers for all academic disciplines for free but also often provides links to full-text PDF files.
- Coverage: approx. 200 million articles
- Abstracts: only a snippet of the abstract is available
- Related articles: ✔
- References: ✔
- Cited by: ✔
- Links to full text: ✔
- Export formats: APA, MLA, Chicago, Harvard, Vancouver, RIS, BibTeX
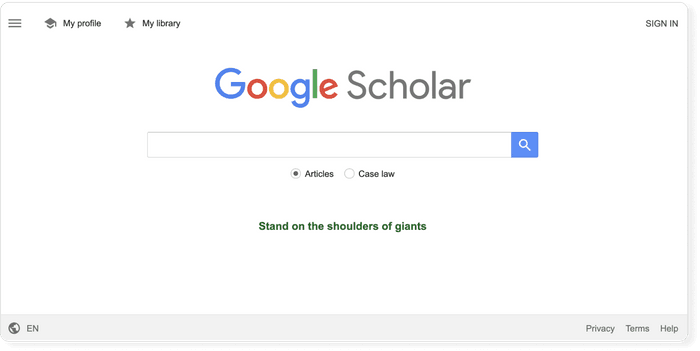
BASE is hosted at Bielefeld University in Germany. That is also where its name stems from (Bielefeld Academic Search Engine).
- Coverage: approx. 136 million articles (contains duplicates)
- Abstracts: ✔
- Related articles: ✘
- References: ✘
- Cited by: ✘
- Export formats: RIS, BibTeX
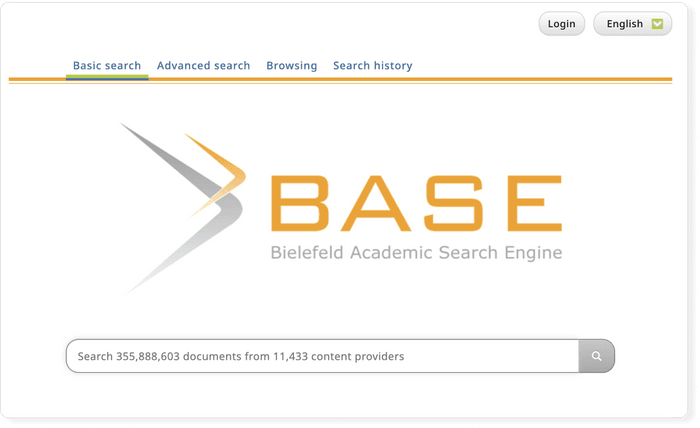
CORE is an academic search engine dedicated to open-access research papers. For each search result, a link to the full-text PDF or full-text web page is provided.
- Coverage: approx. 136 million articles
- Links to full text: ✔ (all articles in CORE are open access)
- Export formats: BibTeX
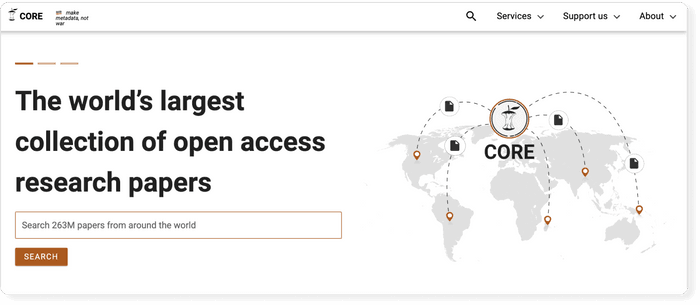
Science.gov is a fantastic resource as it bundles and offers free access to search results from more than 15 U.S. federal agencies. There is no need anymore to query all those resources separately!
- Coverage: approx. 200 million articles and reports
- Links to full text: ✔ (available for some databases)
- Export formats: APA, MLA, RIS, BibTeX (available for some databases)
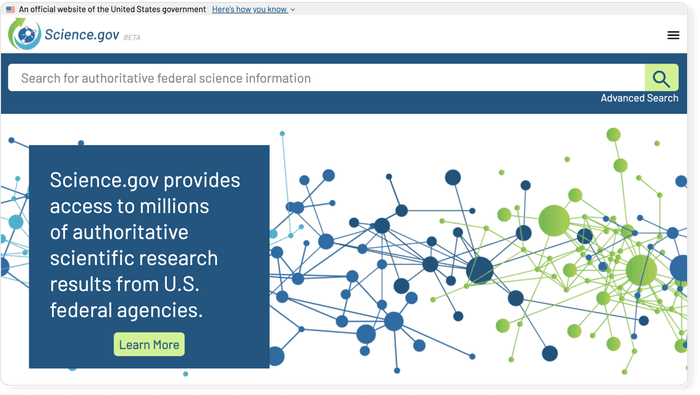
Semantic Scholar is the new kid on the block. Its mission is to provide more relevant and impactful search results using AI-powered algorithms that find hidden connections and links between research topics.
- Coverage: approx. 40 million articles
- Export formats: APA, MLA, Chicago, BibTeX
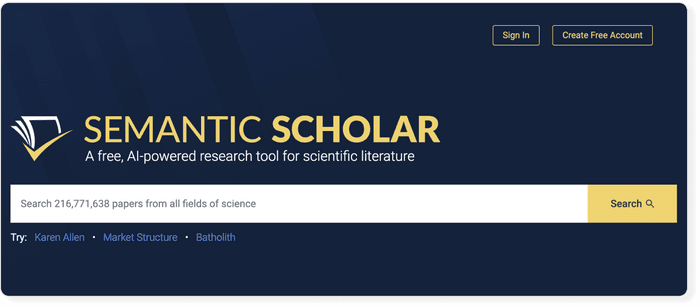
Although Baidu Scholar's interface is in Chinese, its index contains research papers in English as well as Chinese.
- Coverage: no detailed statistics available, approx. 100 million articles
- Abstracts: only snippets of the abstract are available
- Export formats: APA, MLA, RIS, BibTeX
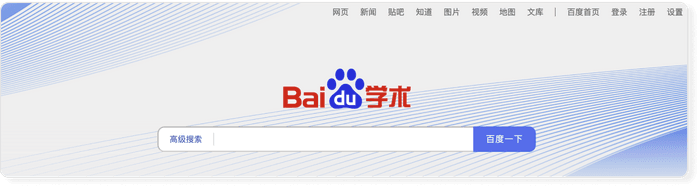
RefSeek searches more than one billion documents from academic and organizational websites. Its clean interface makes it especially easy to use for students and new researchers.
- Coverage: no detailed statistics available, approx. 1 billion documents
- Abstracts: only snippets of the article are available
- Export formats: not available
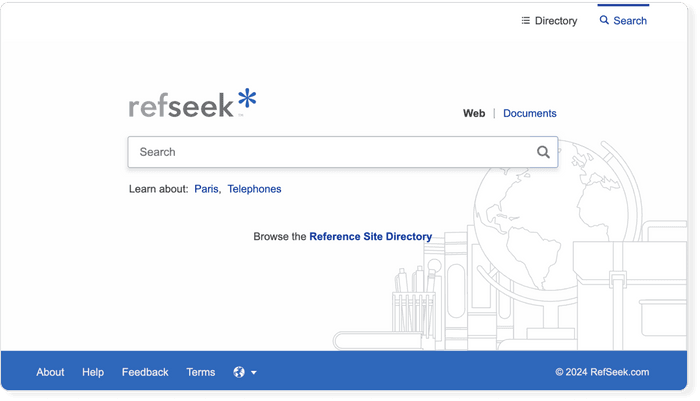
Consider using a reference manager like Paperpile to save, organize, and cite your references. Paperpile integrates with Google Scholar and many popular databases, so you can save references and PDFs directly to your library using the Paperpile buttons:
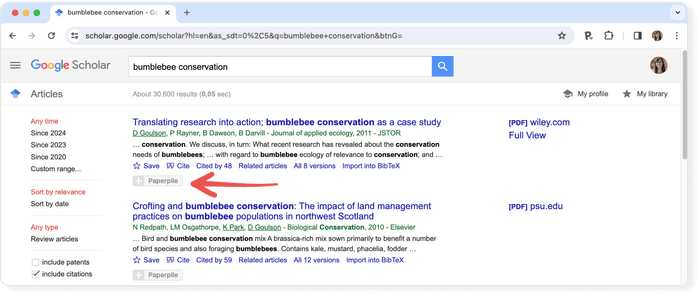
Google Scholar is an academic search engine, and it is the clear number one when it comes to academic search engines. It's the power of Google searches applied to research papers and patents. It not only let's you find research papers for all academic disciplines for free, but also often provides links to full text PDF file.
Semantic Scholar is a free, AI-powered research tool for scientific literature developed at the Allen Institute for AI. Sematic Scholar was publicly released in 2015 and uses advances in natural language processing to provide summaries for scholarly papers.
BASE , as its name suggest is an academic search engine. It is hosted at Bielefeld University in Germany and that's where it name stems from (Bielefeld Academic Search Engine).
CORE is an academic search engine dedicated to open access research papers. For each search result a link to the full text PDF or full text web page is provided.
Science.gov is a fantastic resource as it bundles and offers free access to search results from more than 15 U.S. federal agencies. There is no need any more to query all those resources separately!
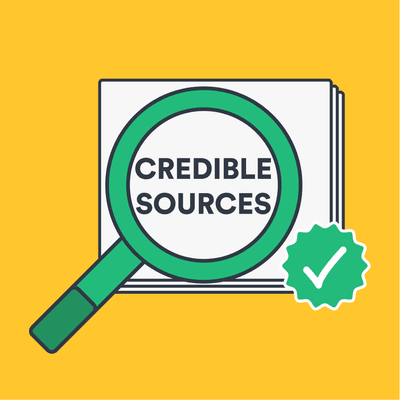
Navigating Higher Education Insurance: An Experimental Study on Demand and Adverse Selection
We conduct a survey-based experiment with 2,776 students at a non-profit university to analyze income insurance demand in education financing. We offered students a hypothetical choice: either a federal loan with income-driven repayment or an income-share agreement (ISA), with randomized framing of downside protections. Emphasizing income insurance increased ISA uptake by 43%. We observe that students are responsive to changes in contract terms and possible student loan cancellation, which is evidence of preference adjustment or adverse selection. Our results indicate that framing specific terms can increase demand for higher education insurance to potentially address risk for students with varying outcomes.
The authors appreciate the helpful comments and feedback of Neil Bhutta, Julia Cheney, Barry Cynamon, Andrew Hertzberg, Jerome Hodges, Caroline Hoxby, Rajeev Darolia, Robert Hunt, Jeff Larrimore, Joseph Marchand, Lois Miller, Kevin Mumford, Marshall Steinbaum, and Joshua Price. We are also grateful for participants at seminar and conference presentations including the American Economic Association, Appalachian State University, Association for Education Finance and Policy, Association for Policy Analysis and Management, Brigham Young University, National Bureau of Economic Research (Economics of Education), Providence College, Southern Economic Association, United States Air Force Academy, and University of Tennessee, Knoxville. The views expressed herein are those of the authors and do not necessarily reflect the views of the Board of Governors of the Federal Reserve, the Federal Reserve Bank of Philadelphia, the Federal Reserve System, or the National Bureau of Economic Research.
Before conducting this research, Michael Kofoed worked as a part time paid consultant with the research partner (called "The University" in the manuscript). Once the University allowed him to conduct the expirement for academic research, Michael Kofoed stopped the paid relationship and worked as a volunteer researcher.
MARC RIS BibTeΧ
Download Citation Data
- randomized controlled trials registry entry
More from NBER
In addition to working papers , the NBER disseminates affiliates’ latest findings through a range of free periodicals — the NBER Reporter , the NBER Digest , the Bulletin on Retirement and Disability , the Bulletin on Health , and the Bulletin on Entrepreneurship — as well as online conference reports , video lectures , and interviews .
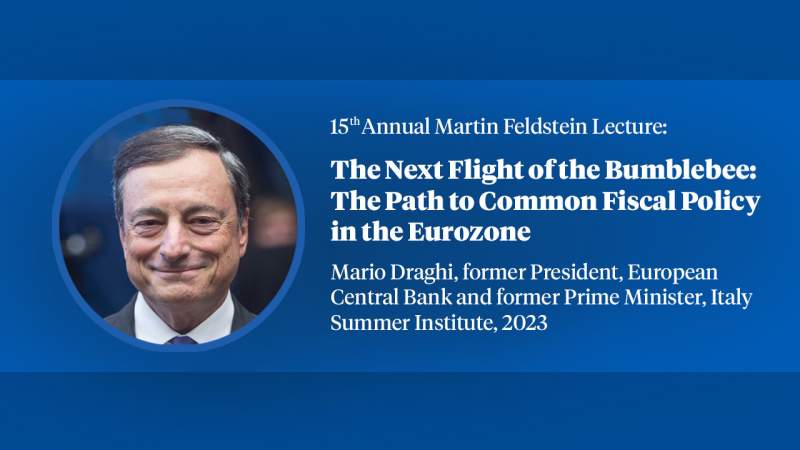
This paper is in the following e-collection/theme issue:
Published on 26.3.2024 in Vol 26 (2024)
The Political Economy of Digital Health Equity: Structural Analysis
Authors of this article:

- James Shaw 1, 2 , PhD ;
- Wiljeana Glover 3 , PhD
1 Department of Physical Therapy, Temerty Faculty of Medicine, University of Toronto, Toronto, ON, Canada
2 Joint Centre for Bioethics, Dalla Lana School of Public Health, University of Toronto, Toronto, ON, Canada
3 Technology, Operations, and Information Management Division, Babson College, Wellesley, MA, United States
Corresponding Author:
James Shaw, PhD
Department of Physical Therapy
Temerty Faculty of Medicine
University of Toronto
500 University Avenue
Toronto, ON, M5G1V7
Phone: 1 4169780315
Email: [email protected]
Digital technologies have produced many innovations in care delivery and enabled continuity of care for many people when in-person care was impossible. However, a growing body of research suggests that digital health can also exacerbate health inequities for those excluded from its benefits for reasons of cost, digital literacy, and structural discrimination related to characteristics such as age, race, ethnicity, and socioeconomic status. In this paper, we draw on a political economy perspective to examine structural barriers to progress in advancing digital health equity at the policy level. Considering the incentive structures and investments of powerful actors in the field, we outline how characteristics of neoliberal capitalism in Western contexts produce and sustain digital health inequities by describing 6 structural challenges to the effort to promote health equity through digital health, as follows: (1) the revenue-first incentives of technology corporations, (2) the influence of venture capital, (3) inequitable access to the internet and digital devices, (4) underinvestment in digital health literacy, (5) uncertainty about future reimbursement of digital health, and (6) justified mistrust of digital health. Building on these important challenges, we propose future immediate and long-term directions for work to support meaningful change for digital health equity.
Introduction
Digital health has become an integral component of health care delivery [ 1 - 3 ]. Although the use of digital technologies to deliver care has been significantly accelerated by the COVID-19 pandemic [ 3 ], the trend toward digitally enabled health care has been progressing for several years alongside the growing power of the technology industry and the widespread distribution of digital devices and infrastructure [ 4 - 6 ]. Digital technologies have produced many innovations in care delivery and enabled continuity of care for many populations when in-person care was challenging or impossible. However, a growing body of research suggests that digital health can also exacerbate health inequities for those excluded from its benefits for reasons of cost, digital literacy, and structural discrimination based on age, race, ethnicity, or socioeconomic status [ 7 , 8 ].
Often referred to as the “health-related digital divide,” the exacerbation of health inequities by digital health technologies has been widely discussed in health informatics and digital health literature [ 7 , 9 , 10 ]. Now that the digital divide has widened as a result of the COVID-19 pandemic, strategies to address its impacts on health have been more widely discussed at policy, health system leadership, clinical, and community levels [ 8 , 11 ]. Building on past research bringing critical perspectives to bear on health equity and digital innovation [ 12 ], we argue that these recommended practices and policies have not yet made meaningful progress on digital health equity in part because scholars, providers, policy makers, and advocates have not yet adequately confronted the social and structural influences that reinforce the status quo at the digital health and health care industry nexus.
In our paper, we draw on a political economy perspective that brings attention to the incentives and actions of powerful stakeholders in a given field [ 13 , 14 ], in this case primarily government, professional, and corporate actors who hold the power to shape the digital health landscape. Considering the incentive structures and investments of such powerful actors, we outline how characteristics of neoliberal capitalism in Western contexts produce and sustain digital health inequities by describing 6 structural challenges to the effort to promote health equity through digital health. Acknowledging that the concept of “digital health equity” has been defined in different ways [ 15 , 16 ], we focus here on digital health equity as an aspirational state in which all communities have access to digital health technologies that enable them to meet their health-related needs. Such a state can be defined at different scales, for example, within a patient population, a city, a province or state, an entire country, or worldwide. Achieving the goal of digital health equity requires commitments from diverse actors in various settings, which we explore in detail in our paper.
Instead of reproducing frameworks proposing multiple points of action to redress digital health inequities [ 10 , 15 , 17 ], in the concluding sections of our paper, we highlight what we understand to be a central tension inherent in efforts to enhance digital health equity. Specifically, we discuss the challenge of supporting commercial actors to invest in immediate actions that enhance digital health equity while acknowledging that the broader health care systems and business models through which they operate can be obstacles to the meaningful advancement of equity and justice for people’s health. We conclude by outlining the need for immediate and long-term directions for research, policy, and advocacy for digital health equity, one focused on foundational reform and the other on stronger collaboration to deepen the commitments of commercial organizations to equity in digital health.
Defining Digital Health
In its broadest sense, digital health refers to a diverse collection of technologies and approaches to care delivery that uses digital technologies or the data they generate to inform health care, self-management, and public health [ 4 , 18 , 19 ]. Digital health can be understood to include at least 4 distinct groups of applications of digital technology to achieve health-related aims, as outlined in Textbox 1 [ 20 , 21 ]. This broad sense of digital health introduces the wide variety of use cases that are considered when policy makers contemplate digital health strategies as solutions to problems in health care and public health.
Digital health refers to a diverse collection of technologies and their uses to achieve health-related goals. We identify the following 4 groups of digital health use cases [ 20 ]:
- The collection of data about health and health care into electronic patient records to inform service planning and care delivery
- Digital apps (usually used on smartphones) that support people to engage with their health and self-monitoring in an ongoing way
- Telemedicine and internet-based care strategies that enable health care to be delivered at a distance
- Analytics of health-related data from multiple sources, including Internet of Things devices, to generate new health-related insights and applications.
The hopes for what digital health can accomplish are high. One highly cited commentary on evaluation standards for digital health stated that “digital health interventions have enormous potential as scalable tools to improve health and health care delivery by improving effectiveness, efficiency, accessibility, safety, and personalization” [ 5 ]. The variety of goals embedded in this statement conveys a general sense that digital health strategies can help to achieve many of the most elusive goals of health care and public health. For example, the notion that digital health apps can lead patients to be more deeply engaged in their own health and well-being, becoming more active participants in their own wellness and care [ 22 , 23 ], or that electronic patient records can enhance collaboration between providers to produce more integrated health care experiences for patients [ 24 ]. If successfully implemented, these specific applications of digital health technologies would contribute to improving health care in profound ways.
Digital Health and Health Inequities
Despite these high ambitions, the positive impacts of digital health have not been evenly distributed. Over the past several years, researchers have documented the exclusion of particular communities from the benefits of digital health technologies in both high-income and low-income country settings [ 8 , 25 - 27 ]. Systematic reviews of this literature have consistently illustrated that communities excluded from the benefits of digital health are those communities facing forms of structural oppression, including economic exclusion, racism, housing injustice, ableism, ageism, and others [ 9 , 28 - 30 ]. For example, multiple systematic reviews have reported the exclusions of Indigenous peoples in North America and Australia from engaging with digital health technologies as a result of a lack of cultural safety in applications of digital health [ 17 , 31 ]. Communities speaking a first language other than English have also been excluded from the benefits of digital health, for example, in situations that demand people navigate a complex digital interface for mental health support [ 32 ]. Communities with lower levels of digital literacy or digital readiness as a result of a lack of educational opportunities also face added barriers to engaging in digital health; for example, older people with less exposure to digital technology and those living with lower incomes who lack the financial resources to afford such technologies in the first place [ 8 , 17 , 25 ]. The reliance on digital health during the COVID-19 pandemic has only exacerbated these realities, leading to deeper inequities in access to health care that stand to have important longer-term consequences [ 33 - 36 ]. For example, the accelerated adoption of internet-based care and other digital health technologies has led to a range of inequities in access to care [ 36 ], and although not enough time has passed to know the long-term impacts of these technologies, they are very likely to be a permanent element of care delivery.
A number of authors have proposed frameworks for understanding where, in the design, adoption, and use of digital health technologies, these exclusions occur in order to identify likely points of redress [ 9 , 17 , 26 ]. Such works have emphasized the importance of a multilevel approach to addressing digital health inequities, generally proposing interventions at the levels of policy, health system leadership, clinical practice, and patient or community engagement [ 9 , 11 , 16 ]. These strategies, some of which are summarized in Table 1 , constitute identifiable actions for specific stakeholders to engage in the effort to enhance health equity in the field of digital health. However, beyond a few notable examples [ 37 ], there is little indication that meaningful progress has been made in adopting these strategies across digital health stakeholders. In this paper, we explore why that is the case by pointing out structural obstacles to advancing digital health equity in the broader digital health ecosystem.
Political Economic Analysis: A Structural Approach
We approach our analysis from a political economy perspective on digital health inequities. The concept of political economy has been used in many ways in relation to health care and public health [ 38 - 40 ]. For our purposes, we describe political economy as a set of relationships and incentives that characterize the interconnections between state, corporate, and other actors with respect to a given domain of social activity, in our case, digital health. Central to analyses of political economies is the concept of power, understood in this context as the capacity of certain actors to exert influence over states of affairs in order to produce outcomes favorable to their interests [ 27 , 39 ]. Power is a complex and productive phenomenon embedded in systems of policy institutions, economic activity, and globalization that make such influence possible [ 41 ].
Analyses of political economy build on a clear understanding of the links between systems of policy or public administration and the economic regimes in place in a given jurisdiction. In North American and European contexts, the impacts of neoliberal capitalism on population health have garnered substantial attention [ 31 , 42 , 43 ]. In this sense, a core feature of neoliberal capitalism is the favoring of private enterprise and the reduction in capacity and responsibility of government or public services [ 42 ]. For example, Friel et al [ 41 ] showed how neoliberal policy approaches to health-related reforms in Australia led to the favoring of private enterprise and individualized strategies as opposed to structural- or population-level initiatives intended to improve health.
With respect to digital health, the policy framework and incentive structures of neoliberalism incentivize the development of products and technologies with a maximum capacity for scale because maximal scale generates maximal profits [ 42 , 43 ]. While neoliberalism has apparently led to a population-level decrease in poverty through economic growth, it has also increased financial and power inequities that have important impacts on health [ 44 ]. Given their capacity to explain whether and how large-scale change occurs in political and economic regimes, political economy approaches have been particularly useful in understanding why more profound action is not taken to address the social determinants of health by states, health care funders, and health systems [ 41 , 45 ].
In these ways, a political economy approach to digital health in the context of neoliberalism examines the influence held by powerful actors over investment and innovation in digital health and the capacity of the state or other actors to steer these processes in the public’s interest. Reflecting on the political economy of digital health, Storeng et al [ 27 ] exclaimed that “new actors, financial instruments, (absent) legal frameworks, and the power structures of capitalism drive the innovation, development, and applications of digital technologies, reshaping health systems, health care, and medicine.” We make observations in our analysis informed by these remarks about political economy, describing the impact of active investments by some actors and inaction by others on the possibilities of achieving digital health equity at scale.
In our analysis, we sought to take an innovation ecosystem perspective [ 46 ], beginning with a broader set of influences on digital health inequities and concluding with a narrower focus on health care delivery and patients. Specifically, we aimed to document the impacts of powerful political and economic actors that shape the broader ecosystem in which digital health innovation takes place. These actors include large corporations, venture capitalists, digital health innovators, and policy makers who set the terms of engagement in digital health. Although policy and investments in digital health are a central consideration, we also acknowledged that the influence of broader policy related to digital inclusion is essential to consider (eg, decisions regarding where high-speed internet is made available and how education regarding digital technologies will be provided). We then narrowed our perspective to focus on health care delivery systems and the patients with whom they engage, documenting decision-making and circumstances that impact digital health inequities at the interface between provider organizations and the communities they serve. Our observations were directly informed by the political economy perspective outlined here.
Structural Barriers to Digital Health Equity
The role of large technology corporations.
The first challenge we outline relates to the role of large technology corporations in the digital health innovation ecosystem. Large technology companies have come to enjoy immense influence over our everyday lives, and this influence extends deeply into health care [ 46 ]. The COVID-19 pandemic witnessed large technology corporations taking advantage of the need for rapid responses to issues such as contact tracing, with the Apple-Google contact tracing application programming interface becoming central to several countries’ contact tracing efforts [ 14 , 47 ]. However, investments from technology corporations extend well beyond contact tracing. Meta (Facebook), Apple, Microsoft, Google, and Amazon invested a combined US $6.8 billion in health care between January 2020 and June 2021 [ 48 ]. Investments ranged from Microsoft’s purchase of a health care chatbot company to Facebook’s development of a preventative health tool to promote regular health screening [ 48 ]. With the immense resources held by large technology corporations and their persistent investment in acquiring market share for digital technologies in health care, they stand to gain substantial influence over the structure and delivery of health care [ 49 ].
The influence of large technology companies may limit the progress that can be made in advancing digital health equity. The incentives of large corporations are fundamentally driven toward the scaling of technology offerings and the profit they generate [ 6 ]. Innovations developed in large corporate environments are those that can be marketed to a segment of the population (or some other payer) that is capable of paying market prices [ 8 , 14 ]. The disposable income or connection to other forms of financial capital (eg, generous health insurance plans) required to access these innovations as they arrive on the market excludes communities that are economically marginalized. This means that the incentives characterizing the activity of technology corporations are fundamentally different from those for achieving equitable access to and outcomes of digital health. Even applications that are free to end users, for example, contact-tracing apps, raise issues of privacy, security, access, and equity for cultural minority and other structurally marginalized patient populations because app rollout may not target these populations [ 50 ]. By supporting and incentivizing technologies that work well for the economically privileged and creating challenges for other actors aiming to provide free or low-cost access to technologies, the actions and incentives of large technology corporations risk widening the divide between those who benefit from access to digital health and those who do not.
Innovation Policy and the Venture Capital System
Beyond the power and impact of large technology corporations, smaller-scale digital health startups are constantly appearing in the digital health ecosystem [ 51 ]. These companies are established and developed through a variety of routes and seek out financial support to fund their activities progressing toward implementation and scale [ 52 ]. In some cases, health systems actively invest in digital health startups as a strategy to promote innovation that more directly addresses clinical and operational needs [ 53 ]. In these cases, an explicit focus on digital innovations that promote health equity is far more likely [ 16 ]. However, external venture capital investment in digital health startups is responsible for the largest portion of venture capital funding. Despite recent declines in new investments due to a market downturn, digital health startups worldwide attracted a combined US $30.7 billion of venture capital investments in 2021, with telemedicine, data analytics, and health-related mobile apps being the highest investment categories [ 54 ].
Importantly, venture capital investors have a deep influence over the development and direction of technologies and business models in health-focused start-ups [ 55 , 56 ]. In a series of papers reporting research on the role of venture capital in health care technology companies, Lehoux and colleagues [ 52 , 55 , 56 ] illustrated the ways that Canadian venture capital investors actively shape the activities of startups and influence the value proposition of the technology being developed. Investors retain a primary incentive to maximize future potential earnings and contribute to shaping activities and priorities in the earlier phases of a company’s development that situate the company to generate the highest potential profits. As was described with respect to large technology corporations, this incentive may close down possibilities for technology that is designed with health equity in mind. Parthasarathy [ 57 ] provided a detailed description of the ways in which a focus on economic gain detracts from the possibility of investing in technologies that meaningfully address the needs of structurally marginalized patients and health care systems. When considered at the scale of investments occurring in digital health around the world, this observation raises serious concerns regarding the likelihood of digital health making meaningful contributions to enhancing equity in access to and outcomes of health care.
Inequitable Access to High-Speed Internet and Digital Technologies
Where communities lack access to high-speed internet and digital devices, they will be unable to benefit from digital health in many ways [ 58 ]. Greene [ 59 ] explained that the reasons for a lack of internet access among marginalized populations relate directly to political and economic investments made during the period during which the internet was being established. The internet, relying on a collection of infrastructures, including physical cables, wireless signals, support personnel, and networks of information exchange, was established in 1983, during a time in the United States that led to a commercialized, free market approach to internet access [ 59 ]. Although there has been progress in expanding high-speed internet to rural areas and increasing free and affordable access in urban areas [ 60 ], a lack of access to high-speed internet and digital devices remains a major barrier to health equity in digital health [ 58 ]. As the centrality of high-speed internet access to health and social services becomes more widely understood, scholars have begun to call for high-speed internet access to be formally recognized as a social determinant of health [ 58 ].
Digital device availability also contributes to the digital divide. Roughly a quarter of American adults with household incomes below US $30,000 a year (24%) say they do not own a smartphone [ 61 ]. If digital health is going to remain a central feature of health care, then establishing sustainable approaches to making devices available to those without access is going to be as important as high-speed internet access [ 9 , 17 ]. A number of charitable programs arose during the COVID-19 pandemic to make digital devices available to those without access [ 62 ], but these have largely been temporary responses to the crisis. The question then becomes whether health care funders will fund digital devices for people without access in order to support their engagement with digital health. These 2 related issues of access to high-speed internet and digital devices raise important questions about perceptions of rightful investments by health care funders, health systems, and governments. Our contention is that without investments in these core infrastructures, digital health will exacerbate existing inequities in access to and outcomes of health care over the long term.
Lack of Investment in Digital Health Literacy
The availability of free or affordable technologies and the internet necessary to use them for health is a crucial starting point for promoting digital health equity. However, the skills and knowledge to use the technology in meaningful ways that promote the attainment of health-related goals are equally important. Digital health literacy has been discussed in different ways, and in this paper, we align with Azzopardi-Muscat and Sørensen [ 63 ], who define the concept broadly as “the ability of people to use emerging information and communications technologies to improve or enable health and health care.” In this way, digital health literacy is about possessing the skills and knowledge necessary to navigate digital technologies, digest health-related information, and engage with health care systems in ways that contribute to enhancing one’s health. Even though supporting access to technology for economically marginalized communities has been used in government strategy internationally [ 59 ], a lack of coherent investment in digital health literacy aligns with the neoliberal erosion of public services, public education, and coordinated approaches to government [ 64 ].
Although governments have progressively built policies on investments in technology as a strategy to promote upward economic mobility [ 59 ], digital health literacy initiatives have appeared to arise only sporadically. Government-sponsored initiatives exist internationally, such as the All of Us Research Program in the United States [ 65 ] and Health Education England’s collaboration with Libraries Connected [ 66 ]. However, the prospect of open-access, well-resourced, and scalable digital health literacy training programs remains elusive internationally. An effort to promote digital health equity at any meaningful scale would require coordinated multistakeholder investments in the delivery of digital health literacy initiatives across communities, health care organizations, and levels of government, and would necessarily include education across proprietary technology offerings.
Uncertainty About the Future of Equitable Reimbursement for Digital Health Care
In the context of the rapid virtualization of care experienced during the COVID-19 pandemic, the question of the sustainability of multiple digital modalities for ambulatory care delivery was much discussed [ 67 ]. In the provincial health care system in Ontario, Canada, physician billing for digital care visits was initially permitted on a temporary basis; that permission was extended multiple times [ 68 ]. Importantly, these permissions included the capability of billing for telephone-only digital visits, which has been widely regarded as an equity-promoting policy to ensure those without access to internet-connected digital technologies can retain access to care [ 67 ]. However, the government of Ontario announced in March 2022 that telephone-only physician visits would be reimbursed at a substantially lower rate than video visits by provincial health insurance, instituting a major blow to sustained efforts to enable equity of access to digital health services. In other words, where audio-only provision of direct care serves to enhance access for those without the digital devices and high-speed internet access required for video visits, reimbursing audio-only visits at a lower rate will disincentivize providers to continue with audio-only digital care. This policy issue represents a substantial challenge for policy makers, seeking to maintain a high level of quality care while at the same time promoting broad accessibility. Understanding how to address this challenge while maintaining access to telephone-based care options that promote equitable access to services is a central policy issue for the next 10 years [ 67 ].
Research in Ontario has shown that the telephone was used appropriately for ambulatory care during the pandemic, and the rise in the use of the telephone was not associated with aberrant or unexpected billing practices among physicians (ie, did not lead to substantial fraudulent billing) [ 69 ]. The removal of reimbursement for telephone visits despite this evidence is a symptom of the effort to narrow the scope of government spending, aligning directly with a neoliberal paradigm of public services. However, the question of funding for digital health and digital care is a broader one than reimbursements for telephone visits.
Discussions about funding for telehealth and digital care following the pandemic have emphasized the importance of paying for value, shifting away from volume-based payment toward value-based payment for primary health care [ 70 ]. The policy work associated with shifting toward value-based payment as a paradigm for health care systems is immense; as that work proceeds and telephone visits are defunded in the near term, gaps in access to digital care will widen. This reality is compounded by the lack of affordability of internet access and digital devices and low investments in digital health literacy, as discussed earlier. As a future state, a value-based care approach would ostensibly involve health systems or health care providers being paid block payments to manage a patient roster in the way they see fit, using funds to support various modalities of digital health care when such modalities could contribute to enhanced patient outcomes for all [ 71 ]. We acknowledge this is an important domain of ongoing research and contend that digital health equity must be a core consideration as value-based care develops in research and policy.
Justified Mistrust and Exclusionary Design
Beyond the issues of the availability and affordability of digital health technologies is the issue of the documented harms some technologies have caused. Examples linked to health care and health promotion are common, including racism in an automated health care resource allocation algorithm [ 72 ] and ineffective sensors on heart rate monitors for people with darker skin [ 73 ]. These harms are representations of social systems arising from histories of excluding marginalized communities from commercial activity and quality health care, leading to the development of technologies and services that neglect the needs of such communities or cause them outright harm. Under these circumstances, some communities choose not to engage with digital health technologies as a result of a justified mistrust of their safety and impact [ 16 ].
Strategies to address justified mistrust of digital health technologies are well-established and include community engagement and inclusive co-design with affected communities to ensure the cultural sensitivity, accuracy, and use of digital health offerings [ 16 ]. However, in the current political economic regime, there is a disincentive to adopt inclusive design strategies across types of commercial actors in digital health (ie, from start-ups to large technology companies). These disincentives arise because inclusive design approaches are costly in that they require additional expertise and time and are challenging to accomplish in meaningful ways [ 74 , 75 ]. As such, barriers to inclusive design remain high. Shifting these incentives and lowering barriers to engagement with inclusive design is a primary challenge in the effort to achieve digital health equity.
The challenges to digital health equity arising from a neoliberal political economic regime are multiple and substantial. However, we also find examples across the 6 challenges where efforts are being made within the neoliberal context to prioritize digital health equity by commercial entities, venture capitalists, providers of high-speed internet, digital and health literacy educators, insurers, and inclusive designers. We acknowledge that such examples may be subject to criticism, especially from scholars adopting critical approaches to the digital technology industry. Research in technology ethics, critical data studies, critical digital health studies, and other related fields has emphasized the ways that commercial entities (and especially large technology corporations) use bounded special projects focused on health equity to avoid deeper changes to their business models or the broader health and economic environments [ 13 , 18 , 23 , 76 ]. In these instances of “ethics washing” [ 77 , 78 ], or perhaps more accurately, “equity washing,” business models are critiqued for their drive for profits without clear prioritization of health equity, health outcomes, and the quality of health care systems [ 79 ].
As scholars anchored by the aim of informing innovations that enhance equity and social justice for people’s health, we are closely attuned to these critiques. However, we have also witnessed the effectiveness of multiple points of intervention in the development of digital health technologies that can orient digital health toward more equitable organization and delivery of care. Furthermore, we are encouraged by the growth in awareness since the onset of the COVID-19 pandemic of the urgent need to promote equitable access to and outcomes of digital health. We are aware of mission-driven digital health initiatives that demonstrate a strong understanding of issues of equitable access, informed consent, and ethical business models. Across our 6 areas, we observe promising opportunities for future work regarding immediate investments in changes to the business models that characterize digital technology under neoliberal capitalism as well as broader changes to health system policies that promote universal access to care.
Large technology corporations like Google are becoming more intentional about their influence on digital health equity. The Google Health Equity team is expanding access to health information through initiatives within Google Search and YouTube and is incorporating insights about the social determinants of health within their Fitbit Health Solutions. Google’s Health Equity Tracker, developed by their health equity team in partnership with the Satcher Health Leadership Institute at the Morehouse School of Medicine [ 80 ], 1 of 3 major historically Black medical schools in the United States, provides a real-time dashboard of progress on various health equity indicators. The platform was designed in collaboration with affected communities and is governed by a diverse team of researchers, community members, providers, and technology leaders. The platform presents the opportunity for governments and payers to make strategic investments in enhancing the health of structurally marginalized communities, offering a pathway for digital health technologies to explicitly enhance health equity. Future research could consider how the revenue models that support these collaborations can more equitably yield financial and health benefits for all involved, including the collaborators and individuals sharing data for such initiatives.
Venture capital has explored the potential of social impact investing as a strategy to enhance health equity at local and global levels [ 81 , 82 ]. Social impact investing involves the development of investments in commercial entities that promise to achieve both a financial return on investment and meaningful progress in enhancing social and economic equity [ 81 ]. Such investments have included large initiatives in global health [ 82 ]. Health systems have also invested in the development of digital health technologies that more comprehensively meet the needs of diverse patients and communities served, such as those developed by accountable care organizations to enhance access to care and reduce hospital admissions [ 83 ]. Future research at the nexus of impact investing and digital health should examine the process of venture selection, social, health, and commercial outcomes, and the role of government in directly investing in or developing policies to support equitable digital health venture development [ 84 ].
Government, large corporations, and digital health ventures are contributing to digital infrastructure initiatives to improve inequitable access to high-speed internet and digital technologies. For example, the US Affordable Connectivity Program of 2022 recently gave over 48 million households (40% of the country) access to high-speed internet for US $30 per month [ 85 ], with a similar government-subsidized program announced in Canada [ 86 ]. Certain health plans in the United States allow for free smartphones for Medicaid patients [ 87 ], with similar programs occurring only through charitable organizations in Canada [ 62 ]. Securing long-term investments and publicizing these offerings to marginalized communities represent crucial investments in digital health equity. Future research should examine factors that promote and inhibit the system-wide scaling of necessary digital infrastructure.
The need for deeper investment in digital health literacy is closely related to the perennial struggle of governments and professional groups to organize systems of health care in ways that support access to care and health promotion for structurally marginalized communities [ 41 ]. In our case, this means fractured investments in digital health literacy, applications, and infrastructure that reinforce the existing fragmentation in health systems, with harmful effects magnified for those who are most marginalized [ 15 ]. Where initiatives aimed at supporting equitable digital health literacy do exist, they are often temporary, local, and not coordinated across the parties needed to ensure their effective implementation in practice. Digital health literacy may be bolstered not only through health agencies but also through broader educational initiatives. For example, the digital literacy efforts by the US Department of Education’s Literacy Information and Communication System and the Canadian Digital Literacy Exchange program could be expanded to explicitly include digital health literacy. Future research is needed on approaches to the governance of digital health that enable innovation in digital health literacy, with an explicit focus on the needs of structurally marginalized communities.
Questions about inequitable reimbursement for digital health care point to the need for broader changes to health system policies that promote universal access to care. These changes are fundamentally longer-term and deeply intertwined with the distribution of power in the health care and digital technology industries. Given the concentrations of power in these domains, strategies to achieve such high-level change are not immediately clear. Building on the strong critiques provided by critical studies of both the health care and digital technology industries and leveraging insights from diverse fields of work such as institutional change, social movements, and sustainability transitions, urgent work is needed on practical strategies to mobilize change at the industry and policy levels. We suggest that research on these changes in the sphere of digital health has much to learn from interdisciplinary social sciences on the green energy transition, exploring multiple points of intervention to modify business models and social habits that support more sustainable ways of life.
Finally, we see a need for support for the implementation and spread of an inclusive design to address mistrust, and with high urgency. Counteracting our aforementioned disincentives for inclusive design, the US Food and Drug Administration is developing plans to enroll more participants from underrepresented racial and ethnic populations into clinical trials [ 88 ]. Design approaches like value-sensitive design are gaining attention from health researchers, such that tensions between stakeholder values can be identified and addressed earlier in design processes [ 89 ]. Remaining aware of current critiques and attuned to the need for broader institutional change, this immediate practical work remains focused on collaboration with digital health developers, governments, health systems, and commercial entities to support equity-focused design and culture changes. Future research is needed that acknowledges critiques of co-design in health care while building strong approaches to inclusive design and values-based collaboration with commercial providers of digital health technologies.
Conclusions
Digital health has become a central feature of health system transformation, presenting an opportunity to steer this transformation in directions that are equity-promoting as a fundamental principle. The role of growth and profit motives and fragmented policy contexts complicate efforts to build coordinated approaches to digital health equity. Nevertheless, the community of researchers, clinicians, community members, and health care leaders committed to health equity holds the power to influence the course of digital health.
As outlined in our paper, there is a substantial body of literature that has drawn on community-engaged and other research approaches to generate strategies to combat digital health inequities at multiple levels. The intellectual work to identify these strategies has already been done and continues to grow. Acknowledging where power wields influence in the broader landscape of digital health, we have proposed future lines of immediate and long-term work aiming to actively promote the advancement of digital health equity. Our recommendations are based on both immediate collaborative work with digital health developers and longer-term efforts to achieve institutional change in the health care and technology industries. Acknowledging the challenges outlined here and investing in the strategies proposed represent important steps toward achieving the goal of digital health equity.
Acknowledgments
Funding for JS was provided by the Canadian Institutes of Health Research Canada Research Chairs program (CRC-2021-00312).
Authors' Contributions
JS and WG contributed to the conceptualization, writing, critical review, and approval of the manuscript.
Conflicts of Interest
None declared.
- Bhatia RS, Chu C, Pang A, Tadrous M, Stamenova V, Cram P. Virtual care use before and during the COVID-19 pandemic: a repeated cross-sectional study. CMAJ Open. 2021;9(1):E107-E114. [ FREE Full text ] [ CrossRef ] [ Medline ]
- Zachrison KS, Yan Z, Schwamm LH. Changes in virtual and in-person health care utilization in a large health system during the COVID-19 pandemic. JAMA Netw Open. 2021;4(10):e2129973. [ FREE Full text ] [ CrossRef ] [ Medline ]
- De Vera K, Challa P, Liu RH, Fuller K, Feroz AS, Gamble A, et al. Virtual primary care implementation during COVID-19 in high-income countries: a scoping review. Telemed J E Health. 2022;28(7):920-931. [ CrossRef ] [ Medline ]
- Mathews SC, McShea MJ, Hanley CL, Ravitz A, Labrique AB, Cohen AB. Digital health: a path to validation. NPJ Digit Med. 2019;2(1):38. [ FREE Full text ] [ CrossRef ] [ Medline ]
- Murray E, Hekler EB, Andersson G, Collins LM, Doherty A, Hollis C, et al. Evaluating digital health interventions: key questions and approaches. Am J Prev Med. 2016;51(5):843-851. [ FREE Full text ] [ CrossRef ] [ Medline ]
- Sharon T. When digital health meets digital capitalism, how many common goods are at stake? Big Data Soc. 2018;5(2):2053951718819032. [ FREE Full text ] [ CrossRef ]
- Veinot TC, Mitchell H, Ancker JS. Good intentions are not enough: how informatics interventions can worsen inequality. J Am Med Inform Assoc. 2018;25(8):1080-1088. [ FREE Full text ] [ CrossRef ] [ Medline ]
- Weiss D, Rydland HT, Øversveen E, Jensen MR, Solhaug S, Krokstad S. Innovative technologies and social inequalities in health: a scoping review of the literature. PLoS One. 2018;13(4):e0195447. [ FREE Full text ] [ CrossRef ] [ Medline ]
- Shaw J, Brewer LC, Veinot T. Recommendations for health equity and virtual care arising from the COVID-19 pandemic: narrative review. JMIR Form Res. 2021;5(4):e23233. [ FREE Full text ] [ CrossRef ] [ Medline ]
- Nouri S, Khoong EC, Lyles CR, Karliner L. Addressing equity in telemedicine for chronic disease management during the COVID-19 pandemic. NEJM Catal Innov Care Deliv. 2020;1(3). [ FREE Full text ]
- Veinot TC, Ancker JS, Cole-Lewis H, Mynatt ED, Parker AG, Siek KA, et al. Leveling up: on the potential of upstream health informatics interventions to enhance health equity. Med Care. 2019;57(Suppl 6 Suppl 2):S108-S114. [ FREE Full text ] [ CrossRef ] [ Medline ]
- Cruz TM. Perils of data-driven equity: safety-net care and big data’s elusive grasp on health inequality. Big Data Soc. 2020;7(1):2053951720928097. [ FREE Full text ] [ CrossRef ]
- Lupton D. Critical perspectives on digital health technologies. Sociol Compass. 2014;8(12):1344-1359. [ FREE Full text ] [ CrossRef ]
- Wamsley D, Chin-Yee B. COVID-19, digital health technology and the politics of the unprecedented. Big Data Soc. 2021;8(1):20539517211019441. [ FREE Full text ]
- Richardson S, Lawrence K, Schoenthaler AM, Mann D. A framework for digital health equity. NPJ Digit Med. 2022;5(1):119. [ FREE Full text ] [ CrossRef ] [ Medline ]
- Brewer LC, Fortuna KL, Jones C, Walker R, Hayes SN, Patten CA, et al. Back to the future: achieving health equity through health informatics and digital health. JMIR Mhealth Uhealth. 2020;8(1):e14512. [ FREE Full text ] [ CrossRef ] [ Medline ]
- Budhwani S, Fujioka J, Thomas-Jacques T, De Vera K, Challa P, De Silva R, et al. Challenges and strategies for promoting health equity in virtual care: findings and policy directions from a scoping review of reviews. J Am Med Inform Assoc. 2022;29(5):990-999. [ FREE Full text ] [ CrossRef ] [ Medline ]
- Shaw JA, Donia J. The sociotechnical ethics of digital health: a critique and extension of approaches from bioethics. Front Digit Health. 2021;3:725088. [ FREE Full text ] [ CrossRef ] [ Medline ]
- Kruse CS, Karem P, Shifflett K, Vegi L, Ravi K, Brooks M. Evaluating barriers to adopting telemedicine worldwide: a systematic review. J Telemed Telecare. 2018;24(1):4-12. [ FREE Full text ] [ CrossRef ] [ Medline ]
- Marent B, Henwood F. Digital health. In: Routledge International Handbook of Critical Issues in Health and Illness. London, UK. Routledge; 2021.
- Elenko E, Underwood L, Zohar D. Defining digital medicine. Nat Biotechnol. 2015;33(5):456-461. [ FREE Full text ] [ CrossRef ] [ Medline ]
- Klein S, Hostetter M, McCarthy D. A vision for using digital health technologies to empower consumers and transform the US health care system. Candid. 2014. URL: https://www.issuelab.org/resources/25081/25081.pdf [accessed 2024-03-08]
- Lupton D. The digitally engaged patient: self-monitoring and self-care in the digital health era. Soc Theory Health. 2013;11(3):256-270. [ FREE Full text ] [ CrossRef ]
- Gray CS, Barnsley J, Gagnon D, Belzile L, Kenealy T, Shaw J, et al. Using information communication technology in models of integrated community-based primary health care: learning from the iCOACH case studies. Implement Sci. 2018;13(1):87. [ FREE Full text ] [ CrossRef ] [ Medline ]
- Latulippe K, Hamel C, Giroux D. Social health inequalities and eHealth: a literature review with qualitative synthesis of theoretical and empirical studies. J Med Internet Res. 2017;19(4):e136. [ FREE Full text ] [ CrossRef ] [ Medline ]
- Fujioka JK, Budhwani S, Thomas-Jacques T, De Vera K, Challa P, Fuller K, et al. Challenges and strategies for promoting health equity in virtual care: protocol for a scoping review of reviews. JMIR Res Protoc. 2020;9(12):e22847. [ FREE Full text ] [ CrossRef ] [ Medline ]
- Storeng KT, Fukuda‐Parr S, Mahajan M, Venkatapuram S. Digital technology and the political determinants of health inequities: special issue introduction. Glob Policy. 2021;12(S6):5-11. [ FREE Full text ] [ CrossRef ]
- Parker S, Prince A, Thomas L, Song H, Milosevic D, Harris M, et al. IMPACT Study Group. Electronic, mobile and telehealth tools for vulnerable patients with chronic disease: a systematic review and realist synthesis. BMJ Open. 2018;8(8):e019192. [ FREE Full text ] [ CrossRef ] [ Medline ]
- Jones L, Jacklin K, O'Connell ME. Development and use of health-related technologies in Indigenous communities: critical review. J Med Internet Res. 2017;19(7):e256. [ FREE Full text ] [ CrossRef ] [ Medline ]
- Arsenijevic J, Tummers L, Bosma N. Adherence to electronic health tools among vulnerable groups: systematic literature review and meta-analysis. J Med Internet Res. 2020;22(2):e11613. [ FREE Full text ] [ CrossRef ] [ Medline ]
- Fraser S, Mackean T, Grant J, Hunter K, Towers K, Ivers R. Use of telehealth for health care of Indigenous peoples with chronic conditions: a systematic review. Rural Remote Health. 2017;17(3):4205. [ FREE Full text ] [ CrossRef ] [ Medline ]
- Truong M, Yeganeh L, Cook O, Crawford K, Wong P, Allen J. Using telehealth consultations for healthcare provision to patients from non-Indigenous racial/ethnic minorities: a systematic review. J Am Med Inform Assoc. 2022;29(5):970-982. [ FREE Full text ] [ CrossRef ] [ Medline ]
- Chang JE, Lai AY, Gupta A, Nguyen AM, Berry CA, Shelley DR. Rapid transition to telehealth and the digital divide: implications for primary care access and equity in a post-COVID era. Milbank Q. 2021;99(2):340-368. [ FREE Full text ] [ CrossRef ] [ Medline ]
- Ortega G, Rodriguez JA, Maurer LR, Witt EE, Perez N, Reich A, et al. Telemedicine, COVID-19, and disparities: policy implications. Health Policy Technol. 2020;9(3):368-371. [ FREE Full text ] [ CrossRef ] [ Medline ]
- Rodriguez JA, Clark CR, Bates DW. Digital health equity as a necessity in the 21st century cures act era. JAMA. 2020;323(23):2381-2382. [ FREE Full text ] [ CrossRef ] [ Medline ]
- Yao R, Zhang W, Evans R, Cao G, Rui T, Shen L. Inequities in health care services caused by the adoption of digital health technologies: scoping review. J Med Internet Res. 2022;24(3):e34144. [ FREE Full text ] [ CrossRef ] [ Medline ]
- Wood BR, Young JD, Abdel-Massih RC, McCurdy L, Vento TJ, Dhanireddy S, et al. Advancing digital health equity: a policy paper of the Infectious Diseases Society of America and the HIV Medicine Association. Clin Infect Dis. 2021;72(6):913-919. [ FREE Full text ] [ CrossRef ] [ Medline ]
- Ghobarah HA, Huth P, Russett B. Comparative public health: the political economy of human misery and well-being. Int Studies Q. 2004;48(1):73-94. [ FREE Full text ] [ CrossRef ]
- McCartney G, Hearty W, Arnot J, Popham F, Cumbers A, McMaster R. Impact of political economy on population health: a systematic review of reviews. Am J Public Health. 2019;109(6):e1-e12. [ CrossRef ] [ Medline ]
- Sell SK, Williams OD. Health under capitalism: a global political economy of structural pathogenesis. Rev Int Polit Econ. 2019;27(1):1-25. [ FREE Full text ] [ CrossRef ]
- Friel S, Townsend B, Fisher M, Harris P, Freeman T, Baum F. Power and the people's health. Soc Sci Med. 2021;282:114173. [ FREE Full text ] [ CrossRef ] [ Medline ]
- Kickbusch I, Allen L, Franz C. The commercial determinants of health. Lancet Glob Health. 2016;4(12):e895-e896. [ FREE Full text ] [ CrossRef ]
- McKee M, Stuckler D. Revisiting the corporate and commercial determinants of health. Am J Public Health. 2018;108(9):1167-1170. [ CrossRef ] [ Medline ]
- Jacobs D, Myers L. Union strength, neoliberalism, and inequality. Am Sociol Rev. 2014;79(4):752-774. [ FREE Full text ] [ CrossRef ]
- Scott-Samuel A, Smith KE. Fantasy paradigms of health inequalities: utopian thinking? Soc Theory Health. 2015;13(3-4):418-436. [ FREE Full text ] [ CrossRef ]
- Thomason J. Big tech, big data and the new world of digital health. Glob Health J. 2021;5(4):165-168. [ FREE Full text ] [ CrossRef ]
- Sharon T. Blind-sided by privacy? Digital contact tracing, the Apple/Google API and big tech's newfound role as global health policy makers. Ethics Inf Technol. 2021;23(Suppl 1):45-57. [ FREE Full text ] [ CrossRef ] [ Medline ]
- The big tech in healthcare report: how Amazon, Google, Microsoft, Apple, and Oracle are fighting for the $11T market. CB Insights. 2022. URL: https://www.cbinsights.com/research/report/famga-big-tech-healthcare/ [accessed 2022-01-21]
- Powles J, Hodson H. Google DeepMind and healthcare in an age of algorithms. Health Technol (Berl). 2017;7(4):351-367. [ FREE Full text ] [ CrossRef ] [ Medline ]
- Costa A, Milne R. Understanding 'passivity' in digital health through imaginaries and experiences of coronavirus disease 2019 contact tracing apps. Big Data Soc. 2022;9(1):20539517221091138. [ FREE Full text ] [ CrossRef ] [ Medline ]
- The digital health 150: the most promising digital health companies of 2022. CB Insights Res. 2022. URL: https://www.cbinsights.com/research/report/digital-health-startups-redefining-healthcare/ [accessed 2022-01-22]
- Lehoux P, Daudelin G, Williams-Jones B, Denis JL, Longo C. How do business model and health technology design influence each other? Insights from a longitudinal case study of three academic spin-offs. Research Policy. 2014;43(6):1025-1038. [ FREE Full text ] [ CrossRef ]
- Safavi KC, Cohen AB, Ting DY, Chaguturu S, Rowe JS. Health systems as venture capital investors in digital health: 2011-2019. NPJ Digit Med. 2020;3(1):103. [ FREE Full text ] [ CrossRef ] [ Medline ]
- 5 digital health categories led VC investment in 2021. Digital Health Business Technology. 2022. URL: https://digitalhealth.modernhealthcare.com/finance/5-digital-health-categories-led-vc-investment-2021 [accessed 2022-01-22]
- Lehoux P, Miller FA, Daudelin G. How does venture capital operate in medical innovation? BMJ Innov. 2016;2(3):111-117. [ FREE Full text ] [ CrossRef ] [ Medline ]
- Lehoux P, Miller FA, Daudelin G, Urbach DR. How venture capitalists decide which new medical technologies come to exist. Sci Public Policy. 2015;43(3):375-385. [ FREE Full text ] [ CrossRef ]
- Parthasarathy S. Health innovation policy for the people. Democracy Collaborative. URL: https://thenextsystem.org/sites/default/files/2021-11/Health-innovation-policy-FINAL.pdf [accessed 2024-03-08]
- Benda NC, Veinot TC, Sieck CJ, Ancker JS. Broadband internet access is a social determinant of health!. Am J Public Health. 2020;110(8):1123-1125. [ FREE Full text ] [ CrossRef ] [ Medline ]
- Greene D. The Promise of Access: Technology, Inequality, and the Political Economy of Hope. Cambridge, MA. MIT Press; 2021.
- Chen W, Li X. Digital inequalities in American disadvantaged urban communities: access, skills, and expectations for digital inclusion programs. Inf Commun Soc. 2021;25(13):1916-1933. [ FREE Full text ] [ CrossRef ]
- Vogels EA. Digital divide persists even as Americans with lower incomes make gains in tech adoption. Pew Research Center. URL: https://www.pewresearch.org/short-reads/2021/06/22/digital-divide-persists-even-as-americans-with-lower-incomes-make-gains-in-tech-adoption/ [accessed 2022-05-25]
- Durocher K, Boparai N, Jankowicz D, Strudwick G. Identifying technology industry-led initiatives to address digital health equity. Digit Health. 2021;7:20552076211056156. [ FREE Full text ] [ CrossRef ] [ Medline ]
- Azzopardi-Muscat N, Sørensen K. Towards an equitable digital public health era: promoting equity through a health literacy perspective. Eur J Public Health. 2019;29(Supplement_3):13-17. [ FREE Full text ] [ CrossRef ] [ Medline ]
- Hughes OE. Public Management and Administration: An Introduction. London, UK. Macmillan International Higher Education; 2012.
- Digital health literacy. National Library of Medicine All of Us Program. 2022. URL: https://allofus.nnlm.gov/digital-health-literacy [accessed 2022-03-14]
- Health and digital literacy partnership provides local learning for better health. NHS England. 2023. URL: https://library.nhs.uk/our-news/ [accessed 2023-01-20]
- Thomas-Jacques T, Jamieson T, Shaw J. Telephone, video, equity and access in virtual care. NPJ Digit Med. 2021;4(1):159. [ FREE Full text ] [ CrossRef ] [ Medline ]
- OHIP–bulletins–health care professionals–MOHLTC. Government of Ontario, Ministry of Health and Long-Term Care. URL: https://www.health.gov.on.ca/en/pro/programs/ohip/bulletins/redux/bul210704.aspx [accessed 2022-03-14]
- Stamenova V, Chu C, Pang A, Tadrous M, Bhatia RS, Cram P. Using administrative data to explore potentially aberrant provision of virtual care during COVID-19: retrospective cohort study of ontario provincial data. J Med Internet Res. 2021;23(9):e29396. [ FREE Full text ] [ CrossRef ] [ Medline ]
- Mehrotra A, Bhatia RS, Snoswell CL. Paying for telemedicine after the pandemic. JAMA. 2021;325(5):431-432. [ FREE Full text ] [ CrossRef ] [ Medline ]
- Modi PK, Kaufman SR, Portney DS, Ryan AM, Hollenbeck BK, Ellimoottil C. Telemedicine utilization by providers in accountable care organizations. Mhealth. 2019;5:10. [ FREE Full text ] [ CrossRef ] [ Medline ]
- Obermeyer Z, Powers B, Vogeli C, Mullainathan S. Dissecting racial bias in an algorithm used to manage the health of populations. Science. 2019;366(6464):447-453. [ CrossRef ] [ Medline ]
- Shcherbina A, Mattsson CM, Waggott D, Salisbury H, Christle JW, Hastie T, et al. Accuracy in wrist-worn, sensor-based measurements of heart rate and energy expenditure in a diverse cohort. J Pers Med. 2017;7(2):3. [ FREE Full text ] [ CrossRef ] [ Medline ]
- Costanza-Chock S. Design Justice: towards an intersectional feminist framework for design theory and practice. 2018. Presented at: Design as a Catalyst for Change-DRS International Conference 2018; June 25-28, 2018; Limerick, Ireland. [ CrossRef ]
- Donia J, Shaw JA. Ethics and values in design: a structured review and theoretical critique. Sci Eng Ethics. 2021;27(5):57. [ FREE Full text ] [ CrossRef ] [ Medline ]
- Holzmeyer C. Beyond ‘AI for Social Good’ (AI4SG): social transformations—not tech-fixes—for health equity. Interdiscip Sci Rev. 2021;46(1-2):94-125. [ FREE Full text ] [ CrossRef ]
- Bietti E. From ethics washing to ethics bashing: a view on tech ethics from within moral philosophy. 2020. Presented at: FAT* '20: Conference on Fairness, Accountability, and Transparency; January 27-30, 2020;210-219; Barcelona, Spain. URL: https://dl.acm.org/doi/abs/10.1145/3351095.3372860 [ CrossRef ]
- van Maanen G. AI ethics, ethics washing, and the need to politicize data ethics. Digit Soc. 2022;1(2):9. [ FREE Full text ] [ CrossRef ] [ Medline ]
- Safavi K, Mathews SC, Bates DW, Dorsey ER, Cohen AB. Top-funded digital health companies and their impact on high-burden, high-cost conditions. Health Aff (Millwood). 2019;38(1):115-123. [ FREE Full text ] [ CrossRef ] [ Medline ]
- Advancing health justice. Health Equity Tracker. 2022. URL: https://healthequitytracker.org [accessed 2022-05-25]
- Höchstädter A, Scheck B. What’s in a name: an analysis of impact investing understandings by academics and practitioners. J Bus Ethics. 2014;132(2):449-475. [ FREE Full text ] [ CrossRef ]
- Brest P, Born K. When can impact investing create real impact. Stanf Soc Innov Rev. 2013;11(4):31. [ FREE Full text ]
- Cassatly S, Cassatly M. The affordable care act and digital health applications. J Med Pract Manage. 2016;32(3):198-201. [ Medline ]
- Agrawal A, Hockerts K. Impact investing: review and research agenda. J Small Bus Entrep. 2019;33(2):153-181. [ FREE Full text ] [ CrossRef ]
- Low-wage earners to get high-speed Internet for $30 in Biden program. The Washington Post. 2022. URL: https://www.washingtonpost.com/business/2022/05/09/biden-internet-discount/ [accessed 2022-05-25]
- Government of Canada announces affordable high-speed Internet to help connect low-income families and seniors. Innovation, Science and Economic Development Canada. 2022. URL: https://tinyurl.com/mrpyc3c8 [accessed 2022-05-25]
- Medicaid | Lifeline smartphone. Sentara Health Plans. 2022. URL: https://www.optimahealth.com/plans/medicaid/lifeline-smartphone [accessed 2022-05-25]
- Diversity plans to improve enrollment of participants from underrepresented racial and ethnic populations in clinical trials; draft guidance for industry; availability. Food and Drug Administration. URL: https://tinyurl.com/rfvpd7xj [accessed 2023-06-30]
- Glover WJ, Hendricks-Sturrup R. Ethics and equity-centred perspectives in engineering systems design. In: Maier A, Oehmen J, Vermaas PE, editors. Handbook of Engineering Systems Design. Cham, Switzerland. Springer; 2023;1-24.
Edited by T Leung; submitted 03.03.23; peer-reviewed by L Husain, C Lyles PhD; comments to author 16.06.23; revised version received 30.06.23; accepted 27.02.24; published 26.03.24.
©James Shaw, Wiljeana Glover. Originally published in the Journal of Medical Internet Research (https://www.jmir.org), 26.03.2024.
This is an open-access article distributed under the terms of the Creative Commons Attribution License (https://creativecommons.org/licenses/by/4.0/), which permits unrestricted use, distribution, and reproduction in any medium, provided the original work, first published in the Journal of Medical Internet Research, is properly cited. The complete bibliographic information, a link to the original publication on https://www.jmir.org/, as well as this copyright and license information must be included.
Thank you for visiting nature.com. You are using a browser version with limited support for CSS. To obtain the best experience, we recommend you use a more up to date browser (or turn off compatibility mode in Internet Explorer). In the meantime, to ensure continued support, we are displaying the site without styles and JavaScript.
- View all journals
Bioinformatics articles from across Nature Portfolio
Bioinformatics is a field of study that uses computation to extract knowledge from biological data. It includes the collection, storage, retrieval, manipulation and modelling of data for analysis, visualization or prediction through the development of algorithms and software.
Latest Research and Reviews
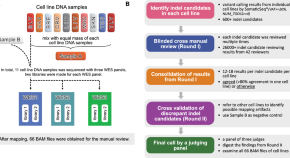
Extend the benchmarking indel set by manual review using the individual cell line sequencing data from the Sequencing Quality Control 2 (SEQC2) project
- Binsheng Gong
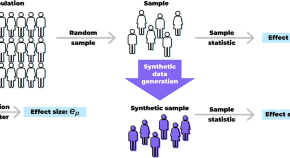
An evaluation of the replicability of analyses using synthetic health data
- Khaled El Emam
- Lucy Mosquera
- Alaa El-Hussuna
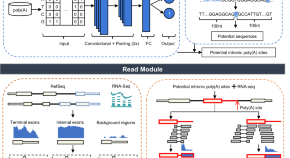
InPACT: a computational method for accurate characterization of intronic polyadenylation from RNA sequencing data
Intronic polyadenylation (IPA) can produce transcripts with truncated coding regions and has been implicated in diverse biological processes and diseases. Here, the authors present a computational method for the accurate delineation of IPA events using RNA-sequencing data.
- Xiaochuan Liu
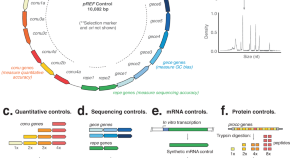
A universal molecular control for DNA, mRNA and protein expression
Multi-omics analyses powerfully combine gene expression and translation, however no available controls can be used across these techniques. Here the authors develop pREF, a universal control construct designed for use in DNA, RNA and protein analyses.
- Helen M. Gunter
- Scott E. Youlten
- Tim R. Mercer
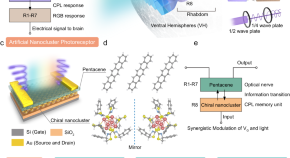
Biomimetic nanocluster photoreceptors for adaptative circular polarization vision
All-in-one multi-task photoperception is desirable for artificial vision systems. Wen et al. present wafer-scale high density integration of artificial photoreceptors that combine photoadaptation and circular polarized light vision, enabled by chiral-nanocluster-conjugated molecule heterostructures.
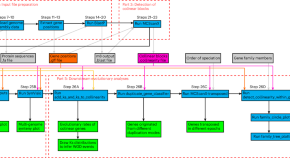
Detection of colinear blocks and synteny and evolutionary analyses based on utilization of MCScanX
Synteny and colinearity are important parameters that delineate the evolution of genomes and gene families. This protocol describes MCScanX, a user-friendly toolkit that facilitates rapid evolutionary analysis of chromosomal structural changes.
- Yupeng Wang
- Haibao Tang
- Andrew H. Paterson
News and Comment
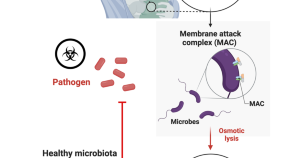
Complement(ing) the microbiome in infants through breastmilk
- Samuel P. Nobs
- Eran Elinav
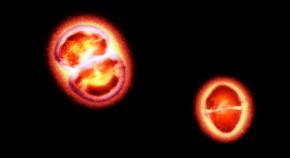
‘Wildly weird’ RNA bits discovered infesting the microbes in our guts
Rod-shaped structures named ‘obelisks’ are even smaller than viruses but can still transmit instructions to cells.
- Saima Sidik
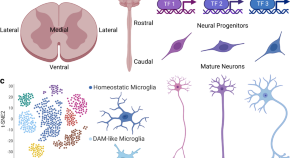
It’s me, hi, I solved the problem, it’s TF-seqFISH
- Olivia Gautier
- Aaron D. Gitler
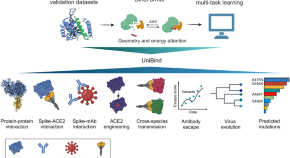
UniBind: a novel artificial intelligence-based prediction model for SARS-CoV-2 infectivity and variant evolution
- Jincun Zhao
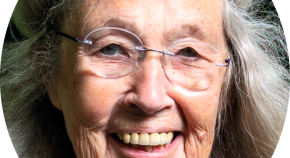
From inception to current challenges in bioinformatics
Dr Paulien Hogeweg — professor of bioinformatics at Utrecht University, who in the 1970s, together with Ben Hesper, coined the term ‘bioinformatics’ — talks to Nature Computational Science about her work on the Cellular Potts model, the integration of spatial information in modeling approaches, and her ongoing research on multilevel evolution.
- Ananya Rastogi
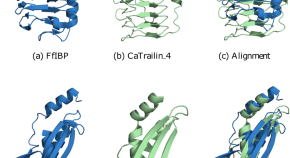
Is Protein BLAST a thing of the past?
Will protein structure search tools like AlphaFold replace protein sequence search with BLAST? We discuss the promises, using structure search for remote homology detection, and why protein BLAST, as the leading sequence search tool, should strive to incorporate structural information
- Ali Al-Fatlawi
- Martin Menzel
- Michael Schroeder
Quick links
- Explore articles by subject
- Guide to authors
- Editorial policies

Intermittent fasting linked to higher risk of cardiovascular death, research suggests
Intermittent fasting, a diet pattern that involves alternating between periods of fasting and eating, can lower blood pressure and help some people lose weight , past research has indicated.
But an analysis presented Monday at the American Heart Association’s scientific sessions in Chicago challenges the notion that intermittent fasting is good for heart health. Instead, researchers from Shanghai Jiao Tong University School of Medicine in China found that people who restricted food consumption to less than eight hours per day had a 91% higher risk of dying from cardiovascular disease over a median period of eight years, relative to people who ate across 12 to 16 hours.
It’s some of the first research investigating the association between time-restricted eating (a type of intermittent fasting) and the risk of death from cardiovascular disease.
The analysis — which has not yet been peer-reviewed or published in an academic journal — is based on data from the Centers for Disease Control and Prevention’s National Health and Nutrition Examination Survey collected between 2003 and 2018. The researchers analyzed responses from around 20,000 adults who recorded what they ate for at least two days, then looked at who had died from cardiovascular disease after a median follow-up period of eight years.
However, Victor Wenze Zhong, a co-author of the analysis, said it’s too early to make specific recommendations about intermittent fasting based on his research alone.
“Practicing intermittent fasting for a short period such as 3 months may likely lead to benefits on reducing weight and improving cardiometabolic health,” Zhong said via email. But he added that people “should be extremely cautious” about intermittent fasting for longer periods of time, such as years.
Intermittent fasting regimens vary widely. A common schedule is to restrict eating to a period of six to eight hours per day, which can lead people to consume fewer calories, though some eat the same amount in a shorter time. Another popular schedule is the "5:2 diet," which involves eating 500 to 600 calories on two nonconsecutive days of the week but eating normally for the other five.
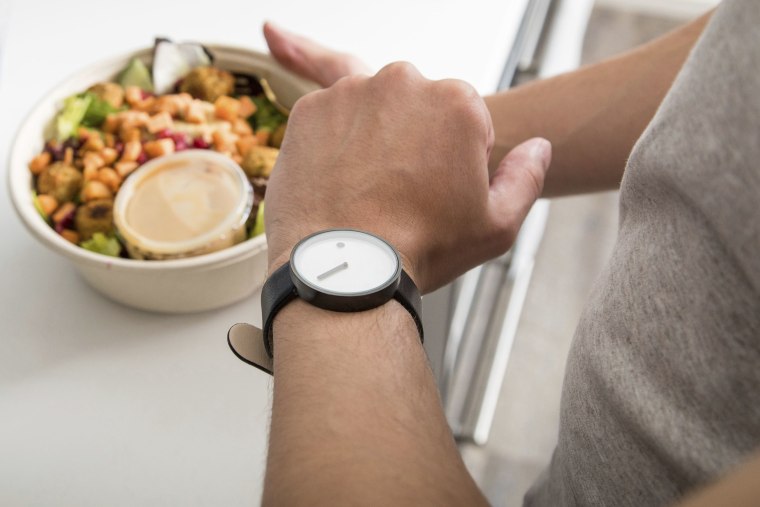
Zhong said it’s not clear why his research found an association between time-restricted eating and a risk of death from cardiovascular disease. He offered an observation, though: People who limited their eating to fewer than eight hours per day had less lean muscle mass than those who ate for 12 to 16 hours. Low lean muscle mass has been linked to a higher risk of cardiovascular death .
Cardiovascular and nutrition experts who were not involved in the analysis offered several theories about what might explain the results.
Dr. Benjamin Horne, a research professor at Intermountain Health in Salt Lake City, said fasting can increase stress hormones such as cortisol and adrenaline, since the body doesn’t know when to expect food next and goes into survival mode. That added stress may raise the short-term risk of heart problems among vulnerable groups, he said, particularly elderly people or those with chronic health conditions.
Horne’s research has shown that fasting twice a week for four weeks, then once a week for 22 weeks may increase a person’s risk of dying after one year but decrease their 10-year risk of chronic disease.
“In the long term, what it does is reduces those risk factors for heart disease and reduces the risk factors for diabetes and so forth — but in the short term, while you’re actually doing it, your body is in a state where it’s at a higher risk of having problems,” he said.
Even so, Horne added, the analysis “doesn’t change my perspective that there are definite benefits from fasting, but it’s a cautionary tale that we need to be aware that there are definite, potentially major, adverse effects.”
Intermittent fasting gained popularity about a decade ago, when the 5:2 diet was touted as a weight loss strategy in the U.K. In the years to follow, several celebrities espoused the benefits of an eight-hour eating window for weight loss, while some Silicon Valley tech workers believed that extreme periods of fasting boosted productivity . Some studies have also suggested that intermittent fasting might help extend people’s lifespans by warding off disease .
However, a lot of early research on intermittent fasting involved animals. In the last seven years or so, various clinical trials have investigated potential benefits for humans, including for heart health.
“The purpose of intermittent fasting is to cut calories, lose weight,” said Penny Kris-Etherton, emeritus professor of nutritional sciences at Penn State University and a member of the American Heart Association nutrition committee. “It’s really how intermittent fasting is implemented that’s going to explain a lot of the benefits or adverse associations.”
Dr. Francisco Lopez-Jimenez, a cardiologist at Mayo Clinic, said the timing of when people eat may influence the effects they see.
“I haven’t met a single person or patient that has been practicing intermittent fasting by skipping dinner,” he said, noting that people more often skip breakfast, a schedule associated with an increased risk of heart disease and death .
The new research comes with limitations: It relies on people’s memories of what they consumed over a 24-hour period and doesn’t consider the nutritional quality of the food they ate or how many calories they consumed during an eating window.
So some experts found the analysis too narrow.
“It’s a retrospective study looking at two days’ worth of data, and drawing some very big conclusions from a very limited snapshot into a person’s lifestyle habits,” said Dr. Pam Taub, a cardiologist at UC San Diego Health.
Taub said her patients have seen “incredible benefits” from fasting regimens.
“I would continue doing it,” she said. “For people that do intermittent fasting, their individual results speak for themselves. Most people that do intermittent fasting, the reason they continue it is they see a decrease in their weight. They see a decrease in blood pressure. They see an improvement in their LDL cholesterol.”
Kris-Etherton, however, urged caution: “Maybe consider a pause in intermittent fasting until we have more information or until the results of the study can be better explained,” she said.

Aria Bendix is the breaking health reporter for NBC News Digital.
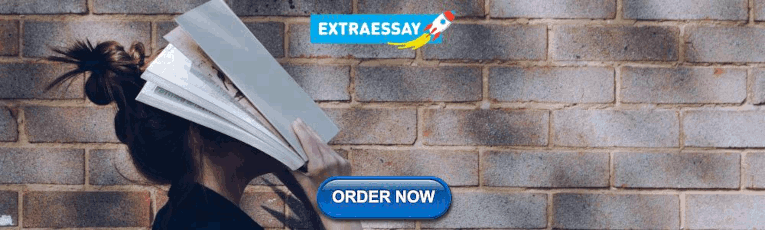
IMAGES
VIDEO
COMMENTS
Get a visual overview of a new academic field. Enter a typical paper and we'll build you a graph of similar papers in the field. Explore and build more graphs for interesting papers that you find - soon you'll have a real, visual understanding of the trends, popular works and dynamics of the field you're interested in.
The Journal of Information Technology (JIT) is a top-ranked journal, focused on new research addressing information, management, and communications technologies as applied to the digital worlds of business, government and non-governmental enterprises. View full journal description. This journal is a member of the Committee on Publication Ethics ...
Papers With Code highlights trending Machine Learning research and the code to implement it. Browse State-of-the-Art Datasets ; Methods; More ... Subscribe to the PwC Newsletter ×. Stay informed on the latest trending ML papers with code, research developments, libraries, methods, and datasets. Read previous issues. Subscribe.
New research on information technology from Harvard Business School faculty on issues including the HealthCare.gov fiasco, online privacy concerns, and the civic benefits of technologies that utilize citizen-created data. Page 1 of 59 Results →. 12 Mar 2024. HBS Case.
Information technology is the design and implementation of computer networks for data processing and communication. This includes designing the hardware for processing information and connecting ...
Call for Papers. Journal of Cybersecurity is soliciting papers for a special collection on the philosophy of information security. This collection will explore research at the intersection of philosophy, information security, and philosophy of science. Find out more.
Explores research from 2010 to February 2022 related to AI applications for cybersecurity from a descriptive point of view, and a detailed state-of-the-art analysis: We performed a systematic literature review (SLR) ... The article is a full research paper (i.e., not a presentation or supplement to a poster). ...
This research paper reviews the existing literature and open data sources related to cybersecurity and cyber risk, focusing on the datasets used to improve academic understanding and advance the current state-of-the-art in cybersecurity. ... Due to the spread of related articles across multiple databases, the literature search was limited to ...
In the penultimate section, we highlight several research issues and potential future directions, and the final section concludes this paper. Types of Real-World Data and Machine Learning Techniques Machine learning algorithms typically consume and process data to learn the related patterns about individuals, business processes, transactions ...
1.711 - SNIP (Source Normalized Impact per Paper) 0.976 - SJR (SCImago Journal Rank) 2023 Speed 10 days submission to first editorial decision for all manuscripts (Median) 116 days submission to accept (Median) 2023 Usage 733,672 downloads 49 Altmetric mentions
The Internet of Things (IoT)-centric concepts like augmented reality, high-resolution video streaming, self-driven cars, smart environment, e-health care, etc. have a ubiquitous presence now. These applications require higher data-rates, large bandwidth, increased capacity, low latency and high throughput. In light of these emerging concepts, IoT has revolutionized the world by providing ...
OpenAI's debut of its impressive Sora text-to-video tool has raised important questions. Jonathan O'Callaghan. News 12 Mar 2024 Nature. Volume: 627, P: 475-476. All News & Comment. Nature.com.
Research by Edward McFowland III, Karim Lakhani, Fabrizio Dell'Acqua, and colleagues. 24 Oct 2023; Cold Call Podcast How the United States Air Force Accelerated AI Adoption. Re: Maria P. Roche. In August 2022, the Pentagon tasked U.S. Air Force Captain Victor Lopez with launching a new Air Force innovation unit that leveraged commercial ...
The authors in have conducted a systematic exploration of serverless computing-related research papers. As they mentioned, their work is not a survey, but it is a supporting source for future research papers. They proposed an open dataset for serverless computing papers. The dataset includes 60 papers for the period (2016-July 2018).
Finally the conclusion of the paper is presented. 2. ... and tangible, it will be described as an attack, and it is at this stage that the rules of international law in related areas and fields (the right to appeal to ... Manz D.O. (Eds.), Research Methods for Cyber Security, Syngress (2017), pp. 33-62 (Chapter 2) View PDF View article Google ...
Access 160+ million publications and connect with 25+ million researchers. Join for free and gain visibility by uploading your research.
Papers most frequently reported results on interventions around pedagogical techniques, curriculum, community, or tools. There was a split in papers that had some type of comparison between an intervention and some other dataset or baseline. Most papers reported related work, following the expectations for doing so in the SIGCSE and CER community.
Find the research you need | With 160+ million publications, 1+ million questions, and 25+ million researchers, this is where everyone can access science
1. Google Scholar. Google Scholar is the clear number one when it comes to academic search engines. It's the power of Google searches applied to research papers and patents. It not only lets you find research papers for all academic disciplines for free but also often provides links to full-text PDF files. Coverage: approx. 200 million articles.
Explore the latest full-text research PDFs, articles, conference papers, preprints and more on BUSINESS ANALYTICS. Find methods information, sources, references or conduct a literature review on ...
Impact of metabolic syndrome on bone mineral density in men over 50 and postmenopausal women according to U.S. survey results. Mo-Yao Tan. Si-Xuan Zhu. Zhong-Xing Liu. Article Open Access 25 Mar 2024.
Founded in 1920, the NBER is a private, non-profit, non-partisan organization dedicated to conducting economic research and to disseminating research findings among academics, public policy makers, and business professionals.
Digital technologies have produced many innovations in care delivery and enabled continuity of care for many people when in-person care was impossible. However, a growing body of research suggests that digital health can also exacerbate health inequities for those excluded from its benefits for reasons of cost, digital literacy, and structural discrimination related to characteristics such as ...
Download This Paper. Open PDF in Browser. Share: Permalink. ... Do you have negative results from your research you'd like to share? Submit Negative Results. Paper statistics. Downloads. 15. Abstract Views. 38. PlumX Metrics. Related eJournals. European Economics: Microeconomics & Industrial Organization eJournal. Follow. European Economics ...
The present research paper reviews the strategic documents related to international, national, maritime and cyber security, foreign policy, intelligence, risk analysis, and foresight, which were ...
Bioinformatics is a field of study that uses computation to extract knowledge from biological data. It includes the collection, storage, retrieval, manipulation and modelling of data for analysis ...
Colorectal cancer is the third most diagnosed cancer and second leading cause of cancer-related death in adults in the United States. 1,2 The lifetime risk of colorectal cancer in the United ...
Intermittent fasting is one of the many trendy ways people try to lose or maintain their weight. Also known as time-restricted eating, the practice is a method of weight loss that confines a ...
Horne's research has shown that fasting twice a week for four weeks, then once a week for 22 weeks may increase a person's risk of dying after one year but decrease their 10-year risk of ...