- Open access
- Published: 03 September 2022
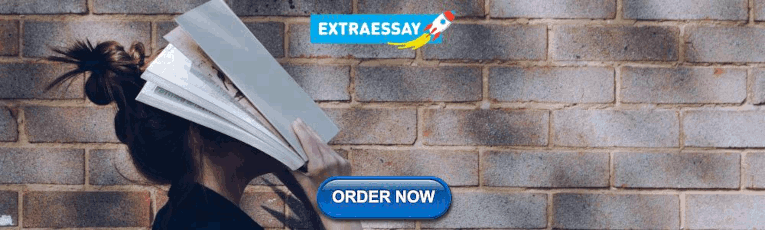
A literature review of risk, regulation, and profitability of banks using a scientometric study
- Shailesh Rastogi 1 ,
- Arpita Sharma 1 ,
- Geetanjali Pinto 2 &
- Venkata Mrudula Bhimavarapu ORCID: orcid.org/0000-0002-9757-1904 1 , 3
Future Business Journal volume 8 , Article number: 28 ( 2022 ) Cite this article
12k Accesses
1 Citations
Metrics details
This study presents a systematic literature review of regulation, profitability, and risk in the banking industry and explores the relationship between them. It proposes a policy initiative using a model that offers guidelines to establish the right mix among these variables. This is a systematic literature review study. Firstly, the necessary data are extracted using the relevant keywords from the Scopus database. The initial search results are then narrowed down, and the refined results are stored in a file. This file is finally used for data analysis. Data analysis is done using scientometrics tools, such as Table2net and Sciences cape software, and Gephi to conduct network, citation analysis, and page rank analysis. Additionally, content analysis of the relevant literature is done to construct a theoretical framework. The study identifies the prominent authors, keywords, and journals that researchers can use to understand the publication pattern in banking and the link between bank regulation, performance, and risk. It also finds that concentration banking, market power, large banks, and less competition significantly affect banks’ financial stability, profitability, and risk. Ownership structure and its impact on the performance of banks need to be investigated but have been inadequately explored in this study. This is an organized literature review exploring the relationship between regulation and bank performance. The limitations of the regulations and the importance of concentration banking are part of the findings.
Introduction
Globally, banks are under extreme pressure to enhance their performance and risk management. The financial industry still recalls the ignoble 2008 World Financial Crisis (WFC) as the worst economic disaster after the Great Depression of 1929. The regulatory mechanism before 2008 (mainly Basel II) was strongly criticized for its failure to address banks’ risks [ 47 , 87 ]. Thus, it is essential to investigate the regulation of banks [ 75 ]. This study systematically reviews the relevant literature on banks’ performance and risk management and proposes a probable solution.
Issues of performance and risk management of banks
Banks have always been hailed as engines of economic growth and have been the axis of the development of financial systems [ 70 , 85 ]. A vital parameter of a bank’s financial health is the volume of its non-performing assets (NPAs) on its balance sheet. NPAs are advances that delay in payment of interest or principal beyond a few quarters [ 108 , 118 ]. According to Ghosh [ 51 ], NPAs negatively affect the liquidity and profitability of banks, thus affecting credit growth and leading to financial instability in the economy. Hence, healthy banks translate into a healthy economy.
Despite regulations, such as high capital buffers and liquidity ratio requirements, during the second decade of the twenty-first century, the Indian banking sector still witnessed a substantial increase in NPAs. A recent report by the Indian central bank indicates that the gross NPA ratio reached an all-time peak of 11% in March 2018 and 12.2% in March 2019 [ 49 ]. Basel II has been criticized for several reasons [ 98 ]. Schwerter [ 116 ] and Pakravan [ 98 ] highlighted the systemic risk and gaps in Basel II, which could not address the systemic risk of WFC 2008. Basel III was designed to close the gaps in Basel II. However, Schwerter [ 116 ] criticized Basel III and suggested that more focus should have been on active risk management practices to avoid any impending financial crisis. Basel III was proposed to solve these issues, but it could not [ 3 , 116 ]. Samitas and Polyzos [ 113 ] found that Basel III had made banking challenging since it had reduced liquidity and failed to shield the contagion effect. Therefore, exploring some solutions to establish the right balance between regulation, performance, and risk management of banks is vital.
Keeley [ 67 ] introduced the idea of a balance among banks’ profitability, regulation, and NPA (risk-taking). This study presents the balancing act of profitability, regulation, and NPA (risk-taking) of banks as a probable solution to the issues of bank performance and risk management and calls it a triad . Figure 1 illustrates the concept of a triad. Several authors have discussed the triad in parts [ 32 , 96 , 110 , 112 ]. Triad was empirically tested in different countries by Agoraki et al. [ 1 ]. Though the idea of a triad is quite old, it is relevant in the current scenario. The spirit of the triad strongly and collectively admonishes the Basel Accord and exhibits new and exhaustive measures to take up and solve the issue of performance and risk management in banks [ 16 , 98 ]. The 2008 WFC may have caused an imbalance among profitability, regulation, and risk-taking of banks [ 57 ]. Less regulation , more competition (less profitability ), and incentive to take the risk were the cornerstones of the 2008 WFC [ 56 ]. Achieving a balance among the three elements of a triad is a real challenge for banks’ performance and risk management, which this study addresses.
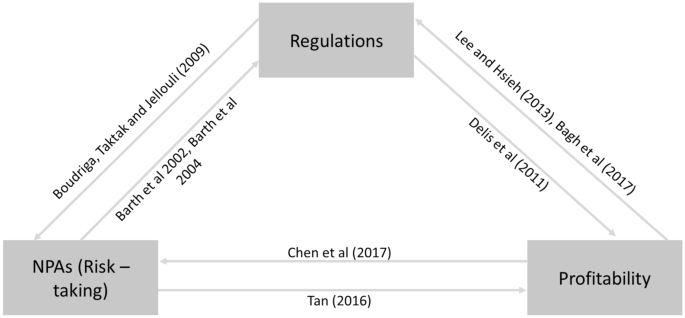
Triad of Profitability, regulation, and NPA (risk-taking). Note The triad [ 131 ] of profitability, regulation, and NPA (risk-taking) is shown in Fig. 1
Triki et al. [ 130 ] revealed that a bank’s performance is a trade-off between the elements of the triad. Reduction in competition increases the profitability of banks. However, in the long run, reduction in competition leads to either the success or failure of banks. Flexible but well-expressed regulation and less competition add value to a bank’s performance. The current review paper is an attempt to explore the literature on this triad of bank performance, regulation, and risk management. This paper has the following objectives:
To systematically explore the existing literature on the triad: performance, regulation, and risk management of banks; and
To propose a model for effective bank performance and risk management of banks.
Literature is replete with discussion across the world on the triad. However, there is a lack of acceptance of the triad as a solution to the woes of bank performance and risk management. Therefore, the findings of the current papers significantly contribute to this regard. This paper collates all the previous studies on the triad systematically and presents a curated view to facilitate the policy makers and stakeholders to make more informed decisions on the issue of bank performance and risk management. This paper also contributes significantly by proposing a DBS (differential banking system) model to solve the problem of banks (Fig. 7 ). This paper examines studies worldwide and therefore ensures the wider applicability of its findings. Applicability of the DBS model is not only limited to one nation but can also be implemented worldwide. To the best of the authors’ knowledge, this is the first study to systematically evaluate the publication pattern in banking using a blend of scientometrics analysis tools, network analysis tools, and content analysis to understand the link between bank regulation, performance, and risk.
This paper is divided into five sections. “ Data and research methods ” section discusses the research methodology used for the study. The data analysis for this study is presented in two parts. “ Bibliometric and network analysis ” section presents the results obtained using bibliometric and network analysis tools, followed by “ Content Analysis ” section, which presents the content analysis of the selected literature. “ Discussion of the findings ” section discusses the results and explains the study’s conclusion, followed by limitations and scope for further research.
Data and research methods
A literature review is a systematic, reproducible, and explicit way of identifying, evaluating, and synthesizing relevant research produced and published by researchers [ 50 , 100 ]. Analyzing existing literature helps researchers generate new themes and ideas to justify the contribution made to literature. The knowledge obtained through evidence-based research also improves decision-making leading to better practical implementation in the real corporate world [ 100 , 129 ].
As Kumar et al. [ 77 , 78 ] and Rowley and Slack [ 111 ] recommended conducting an SLR, this study also employs a three-step approach to understand the publication pattern in the banking area and establish a link between bank performance, regulation, and risk.
Determining the appropriate keywords for exploring the data
Many databases such as Google Scholar, Web of Science, and Scopus are available to extract the relevant data. The quality of a publication is associated with listing a journal in a database. Scopus is a quality database as it has a wider coverage of data [ 100 , 137 ]. Hence, this study uses the Scopus database to extract the relevant data.
For conducting an SLR, there is a need to determine the most appropriate keywords to be used in the database search engine [ 26 ]. Since this study seeks to explore a link between regulation, performance, and risk management of banks, the keywords used were “risk,” “regulation,” “profitability,” “bank,” and “banking.”
Initial search results and limiting criteria
Using the keywords identified in step 1, the search for relevant literature was conducted in December 2020 in the Scopus database. This resulted in the search of 4525 documents from inception till December 2020. Further, we limited our search to include “article” publications only and included subject areas: “Economics, Econometrics and Finance,” “Business, Management and Accounting,” and “Social sciences” only. This resulted in a final search result of 3457 articles. These results were stored in a.csv file which is then used as an input to conduct the SLR.
Data analysis tools and techniques
This study uses bibliometric and network analysis tools to understand the publication pattern in the area of research [ 13 , 48 , 100 , 122 , 129 , 134 ]. Some sub-analyses of network analysis are keyword word, author, citation, and page rank analysis. Author analysis explains the author’s contribution to literature or research collaboration, national and international [ 59 , 99 ]. Citation analysis focuses on many researchers’ most cited research articles [ 100 , 102 , 131 ].
The.csv file consists of all bibliometric data for 3457 articles. Gephi and other scientometrics tools, such as Table2net and ScienceScape software, were used for the network analysis. This.csv file is directly used as an input for this software to obtain network diagrams for better data visualization [ 77 ]. To ensure the study’s quality, the articles with 50 or more citations (216 in number) are selected for content analysis [ 53 , 102 ]. The contents of these 216 articles are analyzed to develop a conceptual model of banks’ triad of risk, regulation, and profitability. Figure 2 explains the data retrieval process for SLR.
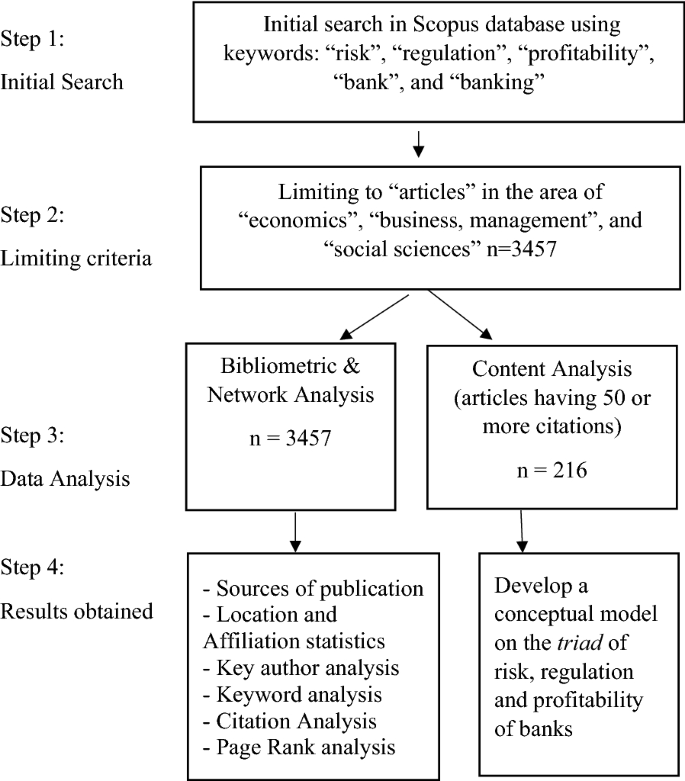
Data retrieval process for SLR. Note Stepwise SLR process and corresponding results obtained
Bibliometric and network analysis
Figure 3 [ 58 ] depicts the total number of studies that have been published on “risk,” “regulation,” “profitability,” “bank,” and “banking.” Figure 3 also depicts the pattern of the quality of the publications from the beginning till 2020. It undoubtedly shows an increasing trend in the number of articles published in the area of the triad: “risk” regulation” and “profitability.” Moreover, out of the 3457 articles published in the said area, 2098 were published recently in the last five years and contribute to 61% of total publications in this area.
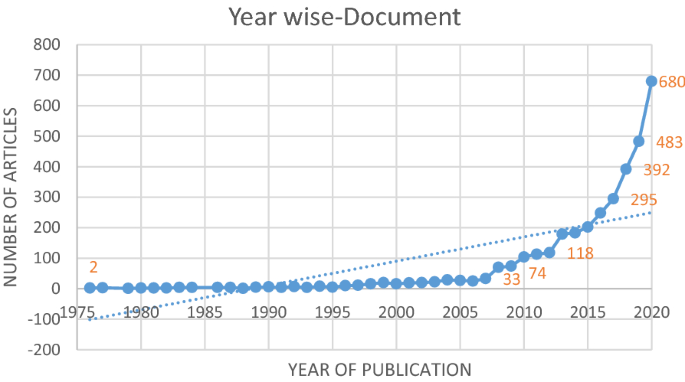
Articles published from 1976 till 2020 . Note The graph shows the number of documents published from 1976 till 2020 obtained from the Scopus database
Source of publications
A total of 160 journals have contributed to the publication of 3457 articles extracted from Scopus on the triad of risk, regulation, and profitability. Table 1 shows the top 10 sources of the publications based on the citation measure. Table 1 considers two sets of data. One data set is the universe of 3457 articles, and another is the set of 216 articles used for content analysis along with their corresponding citations. The global citations are considered for the study from the Scopus dataset, and the local citations are considered for the articles in the nodes [ 53 , 135 ]. The top 10 journals with 50 or more citations resulted in 96 articles. This is almost 45% of the literature used for content analysis ( n = 216). Table 1 also shows that the Journal of Banking and Finance is the most prominent in terms of the number of publications and citations. It has 46 articles published, which is about 21% of the literature used for content analysis. Table 1 also shows these core journals’ SCImago Journal Rank indicator and H index. SCImago Journal Rank indicator reflects the impact and prestige of the Journal. This indicator is calculated as the previous three years’ weighted average of the number of citations in the Journal since the year that the article was published. The h index is the number of articles (h) published in a journal and received at least h. The number explains the scientific impact and the scientific productivity of the Journal. Table 1 also explains the time span of the journals covering articles in the area of the triad of risk, regulation, and profitability [ 7 ].
Figure 4 depicts the network analysis, where the connections between the authors and source title (journals) are made. The network has 674 nodes and 911 edges. The network between the author and Journal is classified into 36 modularities. Sections of the graph with dense connections indicate high modularity. A modularity algorithm is a design that measures how strong the divided networks are grouped into modules; this means how well the nodes are connected through a denser route relative to other networks.
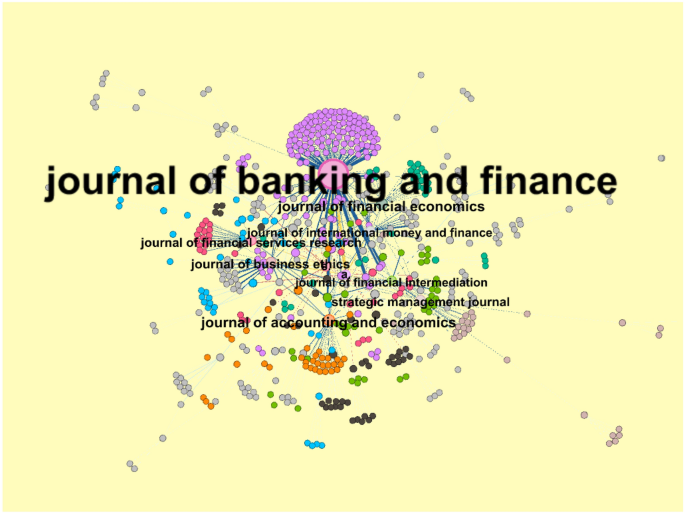
Network analysis between authors and journals. Note A node size explains the more linked authors to a journal
The size of the nodes is based on the rank of the degree. The degree explains the number of connections or edges linked to a node. In the current graph, a node represents the name of the Journal and authors; they are connected through the edges. Therefore, the more the authors are associated with the Journal, the higher the degree. The algorithm used for the layout is Yifan Hu’s.
Many authors are associated with the Journal of Banking and Finance, Journal of Accounting and Economics, Journal of Financial Economics, Journal of Financial Services Research, and Journal of Business Ethics. Therefore, they are the most relevant journals on banks’ risk, regulation, and profitability.
Location and affiliation analysis
Affiliation analysis helps to identify the top contributing countries and universities. Figure 5 shows the countries across the globe where articles have been published in the triad. The size of the circle in the map indicates the number of articles published in that country. Table 2 provides the details of the top contributing organizations.
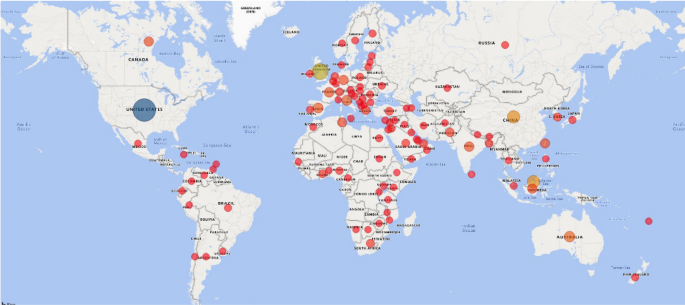
Location of articles published on Triad of profitability, regulation, and risk
Figure 5 shows that the most significant number of articles is published in the USA, followed by the UK. Malaysia and China have also contributed many articles in this area. Table 2 shows that the top contributing universities are also from Malaysia, the UK, and the USA.
Key author analysis
Table 3 shows the number of articles written by the authors out of the 3457 articles. The table also shows the top 10 authors of bank risk, regulation, and profitability.
Fadzlan Sufian, affiliated with the Universiti Islam Malaysia, has the maximum number, with 33 articles. Philip Molyneux and M. Kabir Hassan are from the University of Sharjah and the University of New Orleans, respectively; they contributed significantly, with 20 and 18 articles, respectively.
However, when the quality of the article is selected based on 50 or more citations, Fadzlan Sufian has only 3 articles with more than 50 citations. At the same time, Philip Molyneux and Allen Berger contributed more quality articles, with 8 and 11 articles, respectively.
Keyword analysis
Table 4 shows the keyword analysis (times they appeared in the articles). The top 10 keywords are listed in Table 4 . Banking and banks appeared 324 and 194 times, respectively, which forms the scope of this study, covering articles from the beginning till 2020. The keyword analysis helps to determine the factors affecting banks, such as profitability (244), efficiency (129), performance (107, corporate governance (153), risk (90), and regulation (89).
The keywords also show that efficiency through data envelopment analysis is a determinant of the performance of banks. The other significant determinants that appeared as keywords are credit risk (73), competition (70), financial stability (69), ownership structure (57), capital (56), corporate social responsibility (56), liquidity (46), diversification (45), sustainability (44), credit provision (41), economic growth (41), capital structure (39), microfinance (39), Basel III (37), non-performing assets (37), cost efficiency (30), lending behavior (30), interest rate (29), mergers and acquisition (28), capital adequacy (26), developing countries (23), net interest margin (23), board of directors (21), disclosure (21), leverage (21), productivity (20), innovation (18), firm size (16), and firm value (16).
Keyword analysis also shows the theories of banking and their determinants. Some of the theories are agency theory (23), information asymmetry (21), moral hazard (17), and market efficiency (16), which can be used by researchers when building a theory. The analysis also helps to determine the methodology that was used in the published articles; some of them are data envelopment analysis (89), which measures technical efficiency, panel data analysis (61), DEA (32), Z scores (27), regression analysis (23), stochastic frontier analysis (20), event study (15), and literature review (15). The count for literature review is only 15, which confirms that very few studies have conducted an SLR on bank risk, regulation, and profitability.
Citation analysis
One of the parameters used in judging the quality of the article is its “citation.” Table 5 shows the top 10 published articles with the highest number of citations. Ding and Cronin [ 44 ] indicated that the popularity of an article depends on the number of times it has been cited.
Tahamtan et al. [ 126 ] explained that the journal’s quality also affects its published articles’ citations. A quality journal will have a high impact factor and, therefore, more citations. The citation analysis helps researchers to identify seminal articles. The title of an article with 5900 citations is “A survey of corporate governance.”
Page Rank analysis
Goyal and Kumar [ 53 ] explain that the citation analysis indicates the ‘popularity’ and ‘prestige’ of the published research article. Apart from the citation analysis, one more analysis is essential: Page rank analysis. PageRank is given by Page et al. [ 97 ]. The impact of an article can be measured with one indicator called PageRank [ 135 ]. Page rank analysis indicates how many times an article is cited by other highly cited articles. The method helps analyze the web pages, which get the priority during any search done on google. The analysis helps in understanding the citation networks. Equation 1 explains the page rank (PR) of a published paper, N refers to the number of articles.
T 1,… T n indicates the paper, which refers paper P . C ( Ti ) indicates the number of citations. The damping factor is denoted by a “ d ” which varies in the range of 0 and 1. The page rank of all the papers is equal to 1. Table 6 shows the top papers based on page rank. Tables 5 and 6 together show a contrast in the top ranked articles based on citations and page rank, respectively. Only one article “A survey of corporate governance” falls under the prestigious articles based on the page rank.
Content analysis
Content Analysis is a research technique for conducting qualitative and quantitative analyses [ 124 ]. The content analysis is a helpful technique that provides the required information in classifying the articles depending on their nature (empirical or conceptual) [ 76 ]. By adopting the content analysis method [ 53 , 102 ], the selected articles are examined to determine their content. The classification of available content from the selected set of sample articles that are categorized under different subheads. The themes identified in the relationship between banking regulation, risk, and profitability are as follows.
Regulation and profitability of banks
The performance indicators of the banking industry have always been a topic of interest to researchers and practitioners. This area of research has assumed a special interest after the 2008 WFC [ 25 , 51 , 86 , 114 , 127 , 132 ]. According to research, the causes of poor performance and risk management are lousy banking practices, ineffective monitoring, inadequate supervision, and weak regulatory mechanisms [ 94 ]. Increased competition, deregulation, and complex financial instruments have made banks, including Indian banks, more vulnerable to risks [ 18 , 93 , 119 , 123 ]. Hence, it is essential to investigate the present regulatory machinery for the performance of banks.
There are two schools of thought on regulation and its possible impact on profitability. The first asserts that regulation does not affect profitability. The second asserts that regulation adds significant value to banks’ profitability and other performance indicators. This supports the concept that Delis et al. [ 41 ] advocated that the capital adequacy requirement and supervisory power do not affect productivity or profitability unless there is a financial crisis. Laeven and Majnoni [ 81 ] insisted that provision for loan loss should be part of capital requirements. This will significantly improve active risk management practices and ensure banks’ profitability.
Lee and Hsieh [ 83 ] proposed ambiguous findings that do not support either school of thought. According to Nguyen and Nghiem [ 95 ], while regulation is beneficial, it has a negative impact on bank profitability. As a result, when proposing regulations, it is critical to consider bank performance and risk management. According to Erfani and Vasigh [ 46 ], Islamic banks maintained their efficiency between 2006 and 2013, while most commercial banks lost, furthermore claimed that the financial crisis had no significant impact on Islamic bank profitability.
Regulation and NPA (risk-taking of banks)
The regulatory mechanism of banks in any country must address the following issues: capital adequacy ratio, prudent provisioning, concentration banking, the ownership structure of banks, market discipline, regulatory devices, presence of foreign capital, bank competition, official supervisory power, independence of supervisory bodies, private monitoring, and NPAs [ 25 ].
Kanoujiya et al. [ 64 ] revealed through empirical evidence that Indian bank regulations lack a proper understanding of what banks require and propose reforming and transforming regulation in Indian banks so that responsive governance and regulation can occur to make banks safer, supported by Rastogi et al. [ 105 ]. The positive impact of regulation on NPAs is widely discussed in the literature. [ 94 ] argue that regulation has multiple effects on banks, including reducing NPAs. The influence is more powerful if the country’s banking system is fragile. Regulation, particularly capital regulation, is extremely effective in reducing risk-taking in banks [ 103 ].
Rastogi and Kanoujiya [ 106 ] discovered evidence that disclosure regulations do not affect the profitability of Indian banks, supported by Karyani et al. [ 65 ] for the banks located in Asia. Furthermore, Rastogi and Kanoujiya [ 106 ] explain that disclosure is a difficult task as a regulatory requirement. It is less sustainable due to the nature of the imposed regulations in banks and may thus be perceived as a burden and may be overcome by realizing the benefits associated with disclosure regulation [ 31 , 54 , 101 ]. Zheng et al. [ 138 ] empirically discovered that regulation has no impact on the banks’ profitability in Bangladesh.
Governments enforce banking regulations to achieve a stable and efficient financial system [ 20 , 94 ]. The existing literature is inconclusive on the effects of regulatory compliance on banks’ risks or the reduction of NPAs [ 10 , 11 ]. Boudriga et al. [ 25 ] concluded that the regulatory mechanism plays an insignificant role in reducing NPAs. This is especially true in weak institutions, which are susceptible to corruption. Gonzalez [ 52 ] reported that firm regulations have a positive relationship with banks’ risk-taking, increasing the probability of NPAs. However, Boudriga et al. [ 25 ], Samitas and Polyzos [ 113 ], and Allen et al. [ 3 ] strongly oppose the use of regulation as a tool to reduce banks’ risk-taking.
Kwan and Laderman [ 79 ] proposed three levels in regulating banks, which are lax, liberal, and strict. The liberal regulatory framework leads to more diversification in banks. By contrast, the strict regulatory framework forces the banks to take inappropriate risks to compensate for the loss of business; this is a global problem [ 73 ].
Capital regulation reduces banks’ risk-taking [ 103 , 110 ]. Capital regulation leads to cost escalation, but the benefits outweigh the cost [ 103 ]. The trade-off is worth striking. Altman Z score is used to predict banks’ bankruptcy, and it found that the regulation increased the Altman’s Z-score [ 4 , 46 , 63 , 68 , 72 , 120 ]. Jin et al. [ 62 ] report a negative relationship between regulation and banks’ risk-taking. Capital requirements empowered regulators, and competition significantly reduced banks’ risk-taking [ 1 , 122 ]. Capital regulation has a limited impact on banks’ risk-taking [ 90 , 103 ].
Maji and De [ 90 ] suggested that human capital is more effective in managing banks’ credit risks. Besanko and Kanatas [ 21 ] highlighted that regulation on capital requirements might not mitigate risks in all scenarios, especially when recapitalization has been enforced. Klomp and De Haan [ 72 ] proposed that capital requirements and supervision substantially reduce banks’ risks.
A third-party audit may impart more legitimacy to the banking system [ 23 ]. The absence of third-party intervention is conspicuous, and this may raise a doubt about the reliability and effectiveness of the impact of regulation on bank’s risk-taking.
NPA (risk-taking) in banks and profitability
Profitability affects NPAs, and NPAs, in turn, affect profitability. According to the bad management hypothesis [ 17 ], higher profits would negatively affect NPAs. By contrast, higher profits may lead management to resort to a liberal credit policy (high earnings), which may eventually lead to higher NPAs [ 104 ].
Balasubramaniam [ 8 ] demonstrated that NPA has double negative effects on banks. NPAs increase stressed assets, reducing banks’ productive assets [ 92 , 117 , 136 ]. This phenomenon is relatively underexplored and therefore renders itself for future research.
Triad and the performance of banks
Regulation and triad.
Regulations and their impact on banks have been a matter of debate for a long time. Barth et al. [ 12 ] demonstrated that countries with a central bank as the sole regulatory body are prone to high NPAs. Although countries with multiple regulatory bodies have high liquidity risks, they have low capital requirements [ 40 ]. Barth et al. [ 12 ] supported the following steps to rationalize the existing regulatory mechanism on banks: (1) mandatory information [ 22 ], (2) empowered management of banks, and (3) increased incentive for private agents to exert corporate control. They show that profitability has an inverse relationship with banks’ risk-taking [ 114 ]. Therefore, standard regulatory practices, such as capital requirements, are not beneficial. However, small domestic banks benefit from capital restrictions.
DeYoung and Jang [ 43 ] showed that Basel III-based policies of liquidity convergence ratio (LCR) and net stable funding ratio (NSFR) are not fully executed across the globe, including the US. Dahir et al. [ 39 ] found that a decrease in liquidity and funding increases banks’ risk-taking, making banks vulnerable and reducing stability. Therefore, any regulation on liquidity risk is more likely to create problems for banks.
Concentration banking and triad
Kiran and Jones [ 71 ] asserted that large banks are marginally affected by NPAs, whereas small banks are significantly affected by high NPAs. They added a new dimension to NPAs and their impact on profitability: concentration banking or banks’ market power. Market power leads to less cost and more profitability, which can easily counter the adverse impact of NPAs on profitability [ 6 , 15 ].
The connection between the huge volume of research on the performance of banks and competition is the underlying concept of market power. Competition reduces market power, whereas concentration banking increases market power [ 25 ]. Concentration banking reduces competition, increases market power, rationalizes the banks’ risk-taking, and ensures profitability.
Tabak et al. [ 125 ] advocated that market power incentivizes banks to become risk-averse, leading to lower costs and high profits. They explained that an increase in market power reduces the risk-taking requirement of banks. Reducing banks’ risks due to market power significantly increases when capital regulation is executed objectively. Ariss [ 6 ] suggested that increased market power decreases competition, and thus, NPAs reduce, leading to increased banks’ stability.
Competition, the performance of banks, and triad
Boyd and De Nicolo [ 27 ] supported that competition and concentration banking are inversely related, whereas competition increases risk, and concentration banking decreases risk. A mere shift toward concentration banking can lead to risk rationalization. This finding has significant policy implications. Risk reduction can also be achieved through stringent regulations. Bolt and Tieman [ 24 ] explained that stringent regulation coupled with intense competition does more harm than good, especially concerning banks’ risk-taking.
Market deregulation, as well as intensifying competition, would reduce the market power of large banks. Thus, the entire banking system might take inappropriate and irrational risks [ 112 ]. Maji and Hazarika [ 91 ] added more confusion to the existing policy by proposing that, often, there is no relationship between capital regulation and banks’ risk-taking. However, some cases have reported a positive relationship. This implies that banks’ risk-taking is neutral to regulation or leads to increased risk. Furthermore, Maji and Hazarika [ 91 ] revealed that competition reduces banks’ risk-taking, contrary to popular belief.
Claessens and Laeven [ 36 ] posited that concentration banking influences competition. However, this competition exists only within the restricted circle of banks, which are part of concentration banking. Kasman and Kasman [ 66 ] found that low concentration banking increases banks’ stability. However, they were silent on the impact of low concentration banking on banks’ risk-taking. Baselga-Pascual et al. [ 14 ] endorsed the earlier findings that concentration banking reduces banks’ risk-taking.
Concentration banking and competition are inversely related because of the inherent design of concentration banking. Market power increases when only a few large banks are operating; thus, reduced competition is an obvious outcome. Barra and Zotti [ 9 ] supported the idea that market power, coupled with competition between the given players, injects financial stability into banks. Market power and concentration banking affect each other. Therefore, concentration banking with a moderate level of regulation, instead of indiscriminate regulation, would serve the purpose better. Baselga-Pascual et al. [ 14 ] also showed that concentration banking addresses banks’ risk-taking.
Schaeck et al. [ 115 ], in a landmark study, presented that concentration banking and competition reduce banks’ risk-taking. However, they did not address the relationship between concentration banking and competition, which are usually inversely related. This could be a subject for future research. Research on the relationship between concentration banking and competition is scant, identified as a research gap (“ Research Implications of the study ” section).
Transparency, corporate governance, and triad
One of the big problems with NPAs is the lack of transparency in both the regulatory bodies and banks [ 25 ]. Boudriga et al. [ 25 ] preferred to view NPAs as a governance issue and thus, recommended viewing it from a governance perspective. Ahmad and Ariff [ 2 ] concluded that regulatory capital and top-management quality determine banks’ credit risk. Furthermore, they asserted that credit risk in emerging economies is higher than that of developed economies.
Bad management practices and moral vulnerabilities are the key determinants of insolvency risks of Indian banks [ 95 ]. Banks are an integral part of the economy and engines of social growth. Therefore, banks enjoy liberal insolvency protection in India, especially public sector banks, which is a critical issue. Such a benevolent insolvency cover encourages a bank to be indifferent to its capital requirements. This indifference takes its toll on insolvency risk and profit efficiency. Insolvency protection makes the bank operationally inefficient and complacent.
Foreign equity and corporate governance practices help manage the adverse impact of banks’ risk-taking to ensure the profitability and stability of banks [ 33 , 34 ]. Eastburn and Sharland [ 45 ] advocated that sound management and a risk management system that can anticipate any impending risk are essential. A pragmatic risk mechanism should replace the existing conceptual risk management system.
Lo [ 87 ] found and advocated that the existing legislation and regulations are outdated. He insisted on a new perspective and asserted that giving equal importance to behavioral aspects and the rational expectations of customers of banks is vital. Buston [ 29 ] critiqued the balance sheet risk management practices prevailing globally. He proposed active risk management practices that provided risk protection measures to contain banks’ liquidity and solvency risks.
Klomp and De Haan [ 72 ] championed the cause of giving more autonomy to central banks of countries to provide stability in the banking system. Louzis et al. [ 88 ] showed that macroeconomic variables and the quality of bank management determine banks’ level of NPAs. Regulatory authorities are striving hard to make regulatory frameworks more structured and stringent. However, the recent increase in loan defaults (NPAs), scams, frauds, and cyber-attacks raise concerns about the effectiveness [ 19 ] of the existing banking regulations in India as well as globally.
Discussion of the findings
The findings of this study are based on the bibliometric and content analysis of the sample published articles.
The bibliometric study concludes that there is a growing demand for researchers and good quality research
The keyword analysis suggests that risk regulation, competition, profitability, and performance are key elements in understanding the banking system. The main authors, keywords, and journals are grouped in a Sankey diagram in Fig. 6 . Researchers can use the following information to understand the publication pattern on banking and its determinants.
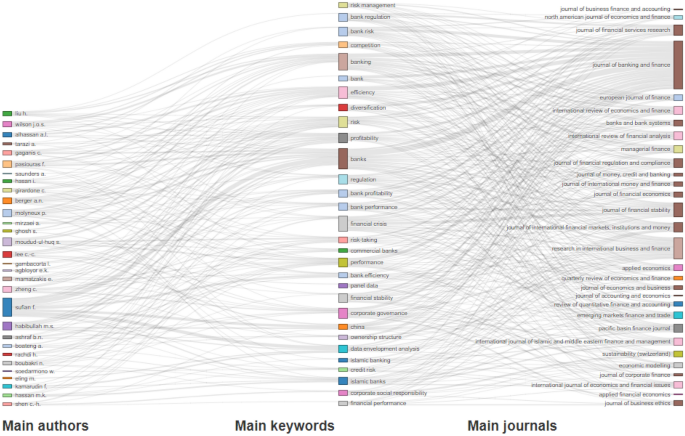
Sankey Diagram of main authors, keywords, and journals. Note Authors contribution using scientometrics tools
Research Implications of the study
The study also concludes that a balance among the three components of triad is the solution to the challenges of banks worldwide, including India. We propose the following recommendations and implications for banks:
This study found that “the lesser the better,” that is, less regulation enhances the performance and risk management of banks. However, less regulation does not imply the absence of regulation. Less regulation means the following:
Flexible but full enforcement of the regulations
Customization, instead of a one-size-fits-all regulatory system rooted in a nation’s indigenous requirements, is needed. Basel or generic regulation can never achieve what a customized compliance system can.
A third-party audit, which is above the country's central bank, should be mandatory, and this would ensure that all three aspects of audit (policy formulation, execution, and audit) are handled by different entities.
Competition
This study asserts that the existing literature is replete with poor performance and risk management due to excessive competition. Banking is an industry of a different genre, and it would be unfair to compare it with the fast-moving consumer goods (FMCG) or telecommunication industry, where competition injects efficiency into the system, leading to customer empowerment and satisfaction. By contrast, competition is a deterrent to the basic tenets of safe banking. Concentration banking is more effective in handling the multi-pronged balance between the elements of the triad. Concentration banking reduces competition to lower and manageable levels, reduces banks’ risk-taking, and enhances profitability.
No incentive to take risks
It is found that unless banks’ risk-taking is discouraged, the problem of high NPA (risk-taking) cannot be addressed. Concentration banking is a disincentive to risk-taking and can be a game-changer in handling banks’ performance and risk management.
Research on the risk and performance of banks reveals that the existing regulatory and policy arrangement is not a sustainable proposition, especially for a country where half of the people are unbanked [ 37 ]. Further, the triad presented by Keeley [ 67 ] is a formidable real challenge to bankers. The balance among profitability, risk-taking, and regulation is very subtle and becomes harder to strike, just as the banks globally have tried hard to achieve it. A pragmatic intervention is needed; hence, this study proposes a change in the banking structure by having two types of banks functioning simultaneously to solve the problems of risk and performance of banks. The proposed two-tier banking system explained in Fig. 7 can be a great solution. This arrangement will help achieve the much-needed balance among the elements of triad as presented by Keeley [ 67 ].
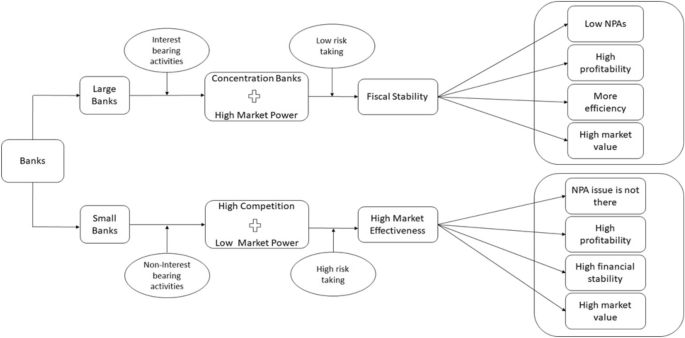
Conceptual Framework. Note Fig. 7 describes the conceptual framework of the study
The first set of banks could be conventional in terms of their structure and should primarily be large-sized. The number of such banks should be moderate. There is a logic in having only a few such banks to restrict competition; thus, reasonable market power could be assigned to them [ 55 ]. However, a reduction in competition cannot be over-assumed, and banks cannot become complacent. As customary, lending would be the main source of revenue and income for these banks (fund based activities) [ 82 ]. The proposed two-tier system can be successful only when regulation especially for risk is objectively executed [ 29 ]. The second set of banks could be smaller in size and more in number. Since they are more in number, they would encounter intense competition for survival and for generating more business. Small is beautiful, and thus, this set of banks would be more agile and adaptable and consequently more efficient and profitable. The main source of revenue for this set of banks would not be loans and advances. However, non-funding and non-interest-bearing activities would be the major revenue source. Unlike their traditional and large-sized counterparts, since these banks are smaller in size, they are less likely to face risk-taking and NPAs [ 74 ].
Sarmiento and Galán [ 114 ] presented the concerns of large and small banks and their relative ability and appetite for risk-taking. High risk could threaten the existence of small-sized banks; thus, they need robust risk shielding. Small size makes them prone to failure, and they cannot convert their risk into profitability. However, large banks benefit from their size and are thus less vulnerable and can convert risk into profitable opportunities.
India has experimented with this Differential Banking System (DBS) (two-tier system) only at the policy planning level. The execution is impending, and it highly depends on the political will, which does not appear to be strong now. The current agenda behind the DBS model is not to ensure the long-term sustainability of banks. However, it is currently being directed to support the agenda of financial inclusion by extending the formal credit system to the unbanked masses [ 107 ]. A shift in goal is needed to employ the DBS as a strategic decision, but not merely a tool for financial inclusion. Thus, the proposed two-tier banking system (DBS) can solve the issue of profitability through proper regulation and less risk-taking.
The findings of Triki et al. [ 130 ] support the proposed DBS model, in this study. Triki et al. [ 130 ] advocated that different component of regulations affect banks based on their size, risk-taking, and concentration banking (or market power). Large size, more concentration banking with high market power, and high risk-taking coupled with stringent regulation make the most efficient banks in African countries. Sharifi et al. [ 119 ] confirmed that size advantage offers better risk management to large banks than small banks. The banks should modify and work according to the economic environment in the country [ 69 ], and therefore, the proposed model could help in solving the current economic problems.
This is a fact that DBS is running across the world, including in India [ 60 ] and other countries [ 133 ]. India experimented with DBS in the form of not only regional rural banks (RRBs) but payments banks [ 109 ] and small finance banks as well [ 61 ]. However, the purpose of all the existing DBS models, whether RRBs [ 60 ], payment banks, or small finance banks, is financial inclusion, not bank performance and risk management. Hence, they are unable to sustain and are failing because their model is only social instead of a much-needed dual business-cum-social model. The two-tier model of DBS proposed in the current paper can help serve the dual purpose. It may not only be able to ensure bank performance and risk management but also serve the purpose of inclusive growth of the economy.
Conclusion of the study
The study’s conclusions have some significant ramifications. This study can assist researchers in determining their study plan on the current topic by using a scientific approach. Citation analysis has aided in the objective identification of essential papers and scholars. More collaboration between authors from various countries/universities may help countries/universities better understand risk regulation, competition, profitability, and performance, which are critical elements in understanding the banking system. The regulatory mechanism in place prior to 2008 failed to address the risk associated with banks [ 47 , 87 ]. There arises a necessity and motivates authors to investigate the current topic. The present study systematically explores the existing literature on banks’ triad: performance, regulation, and risk management and proposes a probable solution.
To conclude the bibliometric results obtained from the current study, from the number of articles published from 1976 to 2020, it is evident that most of the articles were published from the year 2010, and the highest number of articles were published in the last five years, i.e., is from 2015. The authors discovered that researchers evaluate articles based on the scope of critical journals within the subject area based on the detailed review. Most risk, regulation, and profitability articles are published in peer-reviewed journals like; “Journal of Banking and Finance,” “Journal of Accounting and Economics,” and “Journal of Financial Economics.” The rest of the journals are presented in Table 1 . From the affiliation statistics, it is clear that most of the research conducted was affiliated with developed countries such as Malaysia, the USA, and the UK. The researchers perform content analysis and Citation analysis to access the type of content where the research on the current field of knowledge is focused, and citation analysis helps the academicians understand the highest cited articles that have more impact in the current research area.
Practical implications of the study
The current study is unique in that it is the first to systematically evaluate the publication pattern in banking using a combination of scientometrics analysis tools, network analysis tools, and content analysis to understand the relationship between bank regulation, performance, and risk. The study’s practical implications are that analyzing existing literature helps researchers generate new themes and ideas to justify their contribution to literature. Evidence-based research knowledge also improves decision-making, resulting in better practical implementation in the real corporate world [ 100 , 129 ].
Limitations and scope for future research
The current study only considers a single database Scopus to conduct the study, and this is one of the limitations of the study spanning around the multiple databases can provide diverse results. The proposed DBS model is a conceptual framework that requires empirical testing, which is a limitation of this study. As a result, empirical testing of the proposed DBS model could be a future research topic.
Availability of data and materials
SCOPUS database.
Abbreviations
Systematic literature review
World Financial Crisis
Non-performing assets
Differential banking system
SCImago Journal Rank Indicator
Liquidity convergence ratio
Net stable funding ratio
Fast moving consumer goods
Regional rural banks
Agoraki M-EK, Delis MD, Pasiouras F (2011) Regulations, competition and bank risk-taking in transition countries. J Financ Stab 7(1):38–48
Google Scholar
Ahmad NH, Ariff M (2007) Multi-country study of bank credit risk determinants. Int J Bank Financ 5(1):35–62
Allen B, Chan KK, Milne A, Thomas S (2012) Basel III: Is the cure worse than the disease? Int Rev Financ Anal 25:159–166
Altman EI (2018) A fifty-year retrospective on credit risk models, the Altman Z-score family of models, and their applications to financial markets and managerial strategies. J Credit Risk 14(4):1–34
Alvarez F, Jermann UJ (2000) Efficiency, equilibrium, and asset pricing with risk of default. Econometrica 68(4):775–797
Ariss RT (2010) On the implications of market power in banking: evidence from developing countries. J Bank Financ 34(4):765–775
Aznar-Sánchez JA, Piquer-Rodríguez M, Velasco-Muñoz JF, Manzano-Agugliaro F (2019) Worldwide research trends on sustainable land use in agriculture. Land Use Policy 87:104069
Balasubramaniam C (2012) Non-performing assets and profitability of commercial banks in India: assessment and emerging issues. Nat Mon Refereed J Res Commer Manag 1(1):41–52
Barra C, Zotti R (2017) On the relationship between bank market concentration and stability of financial institutions: evidence from the Italian banking sector, MPRA working Paper No 79900. Last Accessed on Jan 2021 https://mpra.ub.uni-muenchen.de/79900/1/MPRA_paper_79900.pdf
Barth JR, Caprio G, Levine R (2004) Bank regulation and supervision: what works best? J Financ Intermed 2(13):205–248
Barth JR, Caprio G, Levine R (2008) Bank regulations are changing: For better or worse? Comp Econ Stud 50(4):537–563
Barth JR, Dopico LG, Nolle DE, Wilcox JA (2002) Bank safety and soundness and the structure of bank supervision: a cross-country analysis. Int Rev Financ 3(3–4):163–188
Bartolini M, Bottani E, Grosse EH (2019) Green warehousing: systematic literature review and bibliometric analysis. J Clean Prod 226:242–258
Baselga-Pascual L, Trujillo-Ponce A, Cardone-Riportella C (2015) Factors influencing bank risk in Europe: evidence from the financial crisis. N Am J Econ Financ 34(1):138–166
Beck T, Demirgüç-Kunt A, Levine R (2006) Bank concentration, competition, and crises: first results. J Bank Financ 30(5):1581–1603
Berger AN, Demsetz RS, Strahan PE (1999) The consolidation of the financial services industry: causes, consequences, and implications for the future. J Bank Financ 23(2–4):135–194
Berger AN, Deyoung R (1997) Problem loans and cost efficiency in commercial banks. J Bank Financ 21(6):849–870
Berger AN, Udell GF (1998) The economics of small business finance: the roles of private equity and debt markets in the financial growth cycle. J Bank Financ 22(6–8):613–673
Berger AN, Udell GF (2002) Small business credit availability and relationship lending: the importance of bank organisational structure. Econ J 112(477):F32–F53
Berger AN, Udell GF (2006) A more complete conceptual framework for SME finance. J Bank Financ 30(11):2945–2966
Besanko D, Kanatas G (1996) The regulation of bank capital: Do capital standards promote bank safety? J Financ Intermed 5(2):160–183
Beyer A, Cohen DA, Lys TZ, Walther BR (2010) The financial reporting environment: review of the recent literature. J Acc Econ 50(2–3):296–343
Bikker JA (2010) Measuring performance of banks: an assessment. J Appl Bus Econ 11(4):141–159
Bolt W, Tieman AF (2004) Banking competition, risk and regulation. Scand J Econ 106(4):783–804
Boudriga A, BoulilaTaktak N, Jellouli S (2009) Banking supervision and non-performing loans: a cross-country analysis. J Financ Econ Policy 1(4):286–318
Bouzon M, Miguel PAC, Rodriguez CMT (2014) Managing end of life products: a review of the literature on reverse logistics in Brazil. Manag Environ Qual Int J 25(5):564–584. https://doi.org/10.1108/MEQ-04-2013-0027
Article Google Scholar
Boyd JH, De Nicolo G (2005) The theory of bank risk taking and competition revisited. J Financ 60(3):1329–1343
Brealey RA, Myers SC, Allen F, Mohanty P (2012) Principles of corporate finance. Tata McGraw-Hill Education
Buston CS (2016) Active risk management and banking stability. J Bank Financ 72:S203–S215
Casu B, Girardone C (2006) Bank competition, concentration and efficiency in the single European market. Manch Sch 74(4):441–468
Charumathi B, Ramesh L (2020) Impact of voluntary disclosure on valuation of firms: evidence from Indian companies. Vision 24(2):194–203
Chen X (2007) Banking deregulation and credit risk: evidence from the EU. J Financ Stab 2(4):356–390
Chen H-J, Lin K-T (2016) How do banks make the trade-offs among risks? The role of corporate governance. J Bank Financ 72(1):S39–S69
Chen M, Wu J, Jeon BN, Wang R (2017) Do foreign banks take more risk? Evidence from emerging economies. J Bank Financ 82(1):20–39
Claessens S, Laeven L (2003) Financial development, property rights, and growth. J Financ 58(6):2401–2436. https://doi.org/10.1046/j.1540-6261.2003.00610.x
Claessens S, Laeven L (2004) What drives bank competition? Some international evidence. J Money Credit Bank 36(3):563–583
Cnaan RA, Moodithaya M, Handy F (2012) Financial inclusion: lessons from rural South India. J Soc Policy 41(1):183–205
Core JE, Holthausen RW, Larcker DF (1999) Corporate governance, chief executive officer compensation, and firm performance. J Financ Econ 51(3):371–406
Dahir AM, Mahat FB, Ali NAB (2018) Funding liquidity risk and bank risk-taking in BRICS countries: an application of system GMM approach. Int J Emerg Mark 13(1):231–248
Dechow P, Ge W, Schrand C (2010) Understanding earnings quality: a review of the proxies, their determinants, and their consequences. J Acc Econ 50(2–3):344–401
Delis MD, Molyneux P, Pasiouras F (2011) Regulations and productivity growth in banking: evidence from transition economies. J Money Credit Bank 43(4):735–764
Demirguc-Kunt A, Laeven L, Levine R (2003) Regulations, market structure, institutions, and the cost of financial intermediation (No. w9890). National Bureau of Economic Research.
Deyoung R, Jang KY (2016) Do banks actively manage their liquidity? J Bank Financ 66:143–161
Ding Y, Cronin B (2011) Popularand/orprestigious? Measures of scholarly esteem. Inf Process Manag 47(1):80–96
Eastburn RW, Sharland A (2017) Risk management and managerial mindset. J Risk Financ 18(1):21–47
Erfani GR, Vasigh B (2018) The impact of the global financial crisis on profitability of the banking industry: a comparative analysis. Economies 6(4):66
Erkens DH, Hung M, Matos P (2012) Corporate governance in the 2007–2008 financial crisis: evidence from financial institutions worldwide. J Corp Finan 18(2):389–411
Fahimnia B, Sarkis J, Davarzani H (2015) Green supply chain management: a review and bibliometric analysis. Int J Prod Econ 162:101–114
Financial Stability Report (2019) Financial stability report (20), December 2019. https://www.rbi.org.in/Scripts/PublicationReportDetails.aspx?UrlPage=&ID=946 Accesses on March 2020
Fink A (2005) Conducting Research Literature Reviews:From the Internet to Paper, 2nd edn. SAGE Publications
Ghosh A (2015) Banking-industry specific and regional economic determinants of non-performing loans: evidence from US states. J Financ Stab 20:93–104. https://doi.org/10.1016/j.jfs.2015.08.004
Gonzalez F (2005) Bank regulation and risk-taking incentives: an international comparison of bank risk. J Bank Financ 29(5):1153–1184
Goyal K, Kumar S (2021) Financial literacy: a systematic review and bibliometric analysis. Int J Consum Stud 45(1):80–105
Grassa R, Moumen N, Hussainey K (2020) Do ownership structures affect risk disclosure in Islamic banks? International evidence. J Financ Rep Acc 19(3):369–391
Haque F, Shahid R (2016) Ownership, risk-taking and performance of banks in emerging economies: evidence from India. J Financ Econ Policy 8(3):282–297
Hellmann TF, Murdock KC, Stiglitz JE (2000) Liberalization, moral hazard in banking, and prudential regulation: Are capital requirements enough? Am Econ Rev 90(1):147–165
Hirshleifer D (2001) Investor psychology and asset pricing. J Financ 56(4):1533–1597
Huang J, You JX, Liu HC, Song MS (2020) Failure mode and effect analysis improvement: a systematic literature review and future research agenda. Reliab Eng Syst Saf 199:106885
Ibáñez Zapata A (2017) Bibliometric analysis of the regulatory compliance function within the banking sector (Doctoral dissertation). Last Accessed on Jan 2021 https://riunet.upv.es/bitstream/handle/10251/85952/Bibliometric%20analysis_AIZ_v4.pdf?sequence=1
Ibrahim MS (2010) Performance evaluation of regional rural banks in India. Int Bus Res 3(4):203–211
Jayadev M, Singh H, Kumar P (2017) Small finance banks: challenges. IIMB Manag Rev 29(4):311–325
Jin JY, Kanagaretnam K, Lobo GJ, Mathieu R (2013) Impact of FDICIA internal controls on bank risk taking. J Bank Financ 37(2):614–624
Joshi MK (2020) Financial performance analysis of select Indian Public Sector Banks using Altman’s Z-Score model. SMART J Bus Manag Stud 16(2):74–87
Kanoujiya J, Bhimavarapu VM, Rastogi S (2021) Banks in India: a balancing act between profitability, regulation and NPA. Vision, 09722629211034417
Karyani E, Dewo SA, Santoso W, Frensidy B (2020) Risk governance and bank profitability in ASEAN-5: a comparative and empirical study. Int J Emerg Mark 15(5):949–969
Kasman S, Kasman A (2015) Bank competition, concentration and financial stability in the Turkish banking industry. Econ Syst 39(3):502–517
Keeley MC (1990) Deposit insurance, risk, and market power in banking. Am Econ Rev 1:1183–1200
Khaddafi M, Heikal M, Nandari A (2017) Analysis Z-score to predict bankruptcy in banks listed in indonesia stock exchange. Int J Econ Financ Issues 7(3):326–330
Khanna T, Yafeh Y (2007) Business groups in emerging markets: Paragons or parasites? J Econ Lit 45(2):331–372
King RG, Levine R (1993) Finance and growth: schumpeter might be right. Q J Econ 108(3):717–737
Kiran KP, Jones TM (2016) Effect of non performing assets on the profitability of banks–a selective study. Int J Bus Gen Manag 5(2):53–60
Klomp J, De Haan J (2015) Banking risk and regulation: Does one size fit all? J Bank Financ 36(12):3197–3212
Koehn M, Santomero AM (1980) Regulation of bank capital and portfolio risk. J Financ 35(5):1235–1244
Köhler M (2015) Which banks are more risky? The impact of business models on bank stability. J Financ Stab 16(1):195–212
Kothari SP (2001) Capital markets research in accounting. J Account Econ 31(1–3):105–231
Kumar S, Goyal N (2015) Behavioural biases in investment decision making – a systematic literature review. Qual Res Financ Mark 7(1):88–108
Kumar S, Kamble S, Roy MH (2020) Twenty-five years of Benchmarking: an International Journal (BIJ): a bibliometric overview. Benchmarking Int J 27(2):760–780. https://doi.org/10.1108/BIJ-07-2019-0314
Kumar S, Sureka R, Colombage S (2020) Capital structure of SMEs: a systematic literature review and bibliometric analysis. Manag Rev Q 70(4):535–565. https://doi.org/10.1007/s11301-019-00175-4
Kwan SH, Laderman ES (1999) On the portfolio effects of financial convergence-a review of the literature. Econ Rev 2:18–31
Lado AA, Boyd NG, Hanlon SC (1997) Competition, cooperation, and the search for economic rents: a syncretic model. Acad Manag Rev 22(1):110–141
Laeven L, Majnoni G (2003) Loan loss provisioning and economic slowdowns: Too much, too late? J Financ Intermed 12(2):178–197
Laeven L, Ratnovski L, Tong H (2016) Bank size, capital, and systemic risk: Some international evidence. J Bank Finance 69(1):S25–S34
Lee C-C, Hsieh M-F (2013) The impact of bank capital on profitability and risk in Asian banking. J Int Money Financ 32(1):251–281
Leech D, Leahy J (1991) Ownership structure, control type classifications and the performance of large British companies. Econ J 101(409):1418–1437
Levine R (1997) Financial development and economic growth: views and agenda. J Econ Lit 35(2):688–726
Lim CY, Woods M, Humphrey C, Seow JL (2017) The paradoxes of risk management in the banking sector. Br Acc Rev 49(1):75–90
Lo AW (2009) Regulatory reform in the wake of the financial crisis of 2007–2008. J Financ Econ Policy 1(1):4–43
Louzis DP, Vouldis AT, Metaxas VL (2012) Macroeconomic and bank-specific determinants of non-performing loans in Greece: a comparative study of mortgage, business and consumer loan portfolios. J Bank Financ 36(4):1012–1027
Maddaloni A, Peydró J-L (2011) Bank risk-taking, securitization, supervision, and low interest rates: evidence from the Euro-area and the U.S. lending standards. Rev Financ Stud 24(6):2121–2165. https://doi.org/10.1093/rfs/hhr015
Maji SG, De UK (2015) Regulatory capital and risk of Indian banks: a simultaneous equation approach. J Financ Econ Policy 7(2):140–156
Maji SG, Hazarika P (2018) Capital regulation, competition and risk-taking behavior of Indian banks in a simultaneous approach. Manag Financ 44(4):459–477
Messai AS, Jouini F (2013) Micro and macro determinants of non-performing loans. Int J Econ Financ Issues 3(4):852–860
Mitra S, Karathanasopoulos A, Sermpinis G, Dunis C, Hood J (2015) Operational risk: emerging markets, sectors and measurement. Eur J Oper Res 241(1):122–132
Mohsni S, Otchere I (2018) Does regulatory regime matter for bank risk-taking? A comparative analysis of US and Canada, d/Seas Working Papers-ISSN 2611-0172 1(1):28–28
Nguyen TPT, Nghiem SH (2015) The interrelationships among default risk, capital ratio and efficiency: evidence from Indian banks. Manag Financ 41(5):507–525
Niinimäki J-P (2004) The effects of competition on banks’ risk taking. J Econ 81(3):199–222
Page L, Brin S, Motwani R, Winograd T (1999) The PageRank citation ranking: bringing order to the web. Stanford InfoLab
Pakravan K (2014) Bank capital: the case against Basel. J Financ Regul Compl 22(3):208–218
Palacios-Callender M, Roberts SA, Roth-Berghofer T (2016) Evaluating patterns of national and international collaboration in Cuban science using bibliometric tools. J Doc 72(2):362–390. https://doi.org/10.1108/JD-11-2014-0164
Pinto G, Rastogi S, Kadam S, Sharma A (2019) Bibliometric study on dividend policy. Qual Res Financ Mark 12(1):72–95
Polizzi S, Scannella E (2020) An empirical investigation into market risk disclosure: Is there room to improve for Italian banks? J Financ Regul Compl 28(3):465–483
Prasad P, Narayanasamy S, Paul S, Chattopadhyay S, Saravanan P (2019) Review of literature on working capital management and future research agenda. J Econ Surv 33(3):827–861
Rahman MM, Zheng C, Ashraf BN, Rahman MM (2018) Capital requirements, the cost of financial intermediation and bank risk-taking: empirical evidence from Bangladesh. Res Int Bus Financ 44(1):488–503
Rajan RG (1994) Why bank credit policies fluctuate: a theory and some evidence. Q J Econ 109(2):399–441
Rastogi S, Gupte R, Meenakshi R (2021) A holistic perspective on bank performance using regulation, profitability, and risk-taking with a view on ownership concentration. J Risk Financ Manag 14(3):111
Rastogi S, Kanoujiya J (2022) Does transparency and disclosure (T&D) improve the performance of banks in India? Int J Product Perform Manag. https://doi.org/10.1108/IJPPM-10-2021-0613
Rastogi S, Ragabiruntha E (2018) Financial inclusion and socioeconomic development: gaps and solution. Int J Soc Econ 45(7):1122–1140
RBI (2001) Prudential Norms on income recognition, asset classification, and provisioning -pertaining to advances. Accessed on Apr 2020. https://rbidocs.rbi.org.in/rdocs/notification/PDFs/23068.pdf
Reddy S (2018) Announcement of payment banks and stock performance of commercial banks in India. J Internet Bank Commer 23(1):1–12
Repullo R (2004) Capital requirements, market power, and risk-taking in banking. J Financ Intermed 13(2):156–182
Rowley J, Slack F (2004) Conducting a literature review. Manag Res News 27(6):31–39. https://doi.org/10.1108/01409170410784185
Salas V, Saurina J (2003) Deregulation, market power and risk behaviour in Spanish banks. Eur Econ Rev 47(6):1061–1075
Samitas A, Polyzos S (2015) To Basel or not to Basel? Banking crises and contagion. Journal of Financial Regulation and Compliance 23(3):298–318
Sarmiento M, Galán JE (2017) The influence of risk-taking on bank efficiency: evidence from Colombia. Emerg Mark Rev 32:52–73. https://doi.org/10.1016/j.ememar.2017.05.007
Schaeck K, Cihak M, Wolfe S (2009) Are competitive banking systems more stable? J Money Credit Bank 41(4):711–734
Schwerter S (2011) Basel III’s ability to mitigate systemic risk. J Financ Regul Compl 19(4):337–354
Sen S, Sen RL (2014) Impact of NPAs on bank profitability: an empirical study. In: Ray N, Chakraborty K (eds) Handbook of research on strategic business infrastructure development and contemporary issues in finance. IGI Global, pp 124–134. https://doi.org/10.4018/978-1-4666-5154-8.ch010
Chapter Google Scholar
Shajahan K (1998) Non-performing assets of banks: Have they really declined? And on whose account? Econ Pol Wkly 33(12):671–674
Sharifi S, Haldar A, Rao SN (2016) Relationship between operational risk management, size, and ownership of Indian banks. Manag Financ 42(10):930–942
Sharma A, Theresa L, Mhatre J, Sajid M (2019) Application of altman Z-Score to RBI defaulters: Indian case. Asian J Res Bus Econ Manag 9(4):1–11
Shehzad CT, De Haan J (2015) Supervisory powers and bank risk taking. J Int Finan Markets Inst Money 39(1):15–24
Shen L, Xiong B, Hu J (2017) Research status, hotspotsandtrends forinformation behavior in China using bibliometric and co-word analysis. J Doc 73(4):618–633
Shleifer A, Vishny RW (1997) A survey of corporate governance. J Financ 52(2):737–783
Singh HP, Kumar S (2014) Working capital management: a literature review and research agenda. Qual Res Financ Mark 6(2):173–197
Tabak BM, Fazio DM, Cajueiro DO (2013) Systemically important banks and financial stability: the case of Latin America. J Bank Financ 37(10):3855–3866
Tahamtan I, SafipourAfshar A, Ahamdzadeh K (2016) Factors affecting number of citations: a comprehensive review of the literature. Scientometrics 107(3):1195–1225
Thakor AV (2018) Post-crisis regulatory reform in banking: Address insolvency risk, not illiquidity! J Financ Stab 37(1):107–111
Thomsen S, Pedersen T (2000) Ownership structure and economic performance in the largest European companies. Strategic Manag J 21(6):689–705
Tranfield D, Denyer D, Smart P (2003) Towards a methodology for developing evidence-informed management knowledge by means of systematic review. Br J Manag 14(3):207–222
Triki T, Kouki I, Dhaou MB, Calice P (2017) Bank regulation and efficiency: What works for Africa? Res Int Bus Financ 39(1):183–205
Tsay M, Shu Z (2011) Journal bibliometric analysis: a case study on the journal of documentation. J Doc 67(5):806–822
Vento GA, La Ganga P (2009) Bank liquidity risk management and supervision: which lessons from recent market turmoil. J Money Invest Bank 10(10):78–125
Wahid ANM (1994) The grameen bank and poverty alleviation in Bangladesh: theory, evidence and limitations. Am J Econ Sociol 53(1):1–15
Xiao Y, Watson M (2019) Guidance on conducting a systematic literature review. J Plan Educ Res 39(1):93–112
Xu X, Chen X, Jia F, Brown S, Gong Y, Xu Y (2018) Supply chain finance: a systematic literature review and bibliometric analysis. Int J Prod Econ 204:160–173
Yadav M (2011) Impact of non performing assets on profitability and productivity of public sector banks in India. AFBE J 4(1):232–239
Yong-Hak J (2013), Web of Science, Thomson Reuters
Zheng C, Rahman MM, Begum M, Ashraf BN (2017) Capital regulation, the cost of financial intermediation and bank profitability: evidence from Bangladesh. J Risk Financ Manag 10(2):9
Download references
Acknowledgements
Not Applicable.
Author information
Authors and affiliations.
Symbiosis Institute of Business Management, Symbiosis International (Deemed University), Pune, India
Shailesh Rastogi, Arpita Sharma & Venkata Mrudula Bhimavarapu
SIES School of Business Studies, Navi Mumbai, India
Geetanjali Pinto
School of Commerce and Management, D Y Patil International University, Akurdi, Pune, India
Venkata Mrudula Bhimavarapu
You can also search for this author in PubMed Google Scholar
Contributions
‘SR’ performed Abstract, Introduction, and Data methodology sections and was the major contributor; ‘AS’ performed Bibliometric and Network analysis and conceptual framework; ‘GP’ performed citation analysis and discussion section; ‘VMB’ collated data from the database and concluded the article. All authors read and approved the final manuscript.
Corresponding author
Correspondence to Venkata Mrudula Bhimavarapu .
Ethics declarations
Ethics approval and consent to participate, consent for publication, competing interests.
The authors declare that they have no competing interests.
Additional information
Publisher's note.
Springer Nature remains neutral with regard to jurisdictional claims in published maps and institutional affiliations.
Rights and permissions
Open Access This article is licensed under a Creative Commons Attribution 4.0 International License, which permits use, sharing, adaptation, distribution and reproduction in any medium or format, as long as you give appropriate credit to the original author(s) and the source, provide a link to the Creative Commons licence, and indicate if changes were made. The images or other third party material in this article are included in the article's Creative Commons licence, unless indicated otherwise in a credit line to the material. If material is not included in the article's Creative Commons licence and your intended use is not permitted by statutory regulation or exceeds the permitted use, you will need to obtain permission directly from the copyright holder. To view a copy of this licence, visit http://creativecommons.org/licenses/by/4.0/ .
Reprints and permissions
About this article
Cite this article.
Rastogi, S., Sharma, A., Pinto, G. et al. A literature review of risk, regulation, and profitability of banks using a scientometric study. Futur Bus J 8 , 28 (2022). https://doi.org/10.1186/s43093-022-00146-4
Download citation
Received : 11 March 2022
Accepted : 16 August 2022
Published : 03 September 2022
DOI : https://doi.org/10.1186/s43093-022-00146-4
Share this article
Anyone you share the following link with will be able to read this content:
Sorry, a shareable link is not currently available for this article.
Provided by the Springer Nature SharedIt content-sharing initiative
- Bank performance
- Profitability
- Bibliometric analysis
- Scientometric analysis
Click through the PLOS taxonomy to find articles in your field.
For more information about PLOS Subject Areas, click here .
Loading metrics
Open Access
Peer-reviewed
Research Article
Financial sector and economic growth amid external uncertainty shocks: Insights into emerging economies
Roles Conceptualization, Data curation, Formal analysis, Methodology, Writing – original draft
* E-mail: [email protected]
Affiliation Department of Finance, School of Business, University of Cape Coast, Cape Coast, Ghana

Roles Conceptualization, Data curation, Investigation, Visualization, Writing – original draft
Roles Investigation, Supervision, Visualization
Roles Data curation, Methodology, Software, Supervision, Validation, Visualization, Writing – review & editing
Roles Supervision, Validation, Visualization, Writing – review & editing
- Emmanuel Asafo-Adjei,
- Ebenezer Boateng,
- Zangina Isshaq,
- Anthony Adu-Asare Idun,
- Peterson Owusu Junior,
- Anokye M. Adam
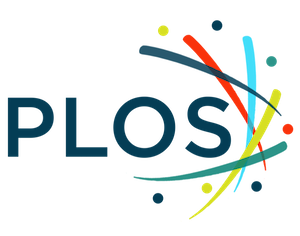
- Published: November 11, 2021
- https://doi.org/10.1371/journal.pone.0259303
- Reader Comments
The study aims to shed new lights on the lead-lag relationships between the financial sector (RFSI) and economic growth (GDP) in the midst of global economic policy uncertainty (GEPU) shocks for BRICS economies. Hence, the bivariate, partial, and wavelet multiple correlations techniques are employed. From the bivariate analysis, we document positive bi-directional causality between the RFSI and economic growth over the sample period. The partial wavelet reveals that GEPU shocks distort the significance and directional comovements between the RFSI and GDP. Moreover, the outcome from the wavelet multiple cross correlations (WMCC) indicates that the RFSI is a first mover at most time scales for the BRICS economies. This is followed by GEPU which either leads or lags for most scales, especially for South Africa. The impact of GEPU on RFSI and GDP is worst for South Africa in about four cases in the medium-, and long-terms. This signifies that South Africa’s financial markets and economic growth are vulnerable to GEPU. However, the impetus for GEPU to drive the comovements between the financial sector and economic activity was less pronounced in the pre-COVID analysis conducted with the WMCC. The study supports both the supply-leading and demand-following hypotheses. Our findings also underscore the need for policymakers, investors and academics alike to incessantly observe the dynamics between finance and growth across time and periodicity while considering adverse shocks from global economic policy uncertainty in tandem.
Citation: Asafo-Adjei E, Boateng E, Isshaq Z, Idun AA-A, Owusu Junior P, Adam AM (2021) Financial sector and economic growth amid external uncertainty shocks: Insights into emerging economies. PLoS ONE 16(11): e0259303. https://doi.org/10.1371/journal.pone.0259303
Editor: J E. Trinidad Segovia, University of Almeria, SPAIN
Received: May 31, 2021; Accepted: October 15, 2021; Published: November 11, 2021
Copyright: © 2021 Asafo-Adjei et al. This is an open access article distributed under the terms of the Creative Commons Attribution License , which permits unrestricted use, distribution, and reproduction in any medium, provided the original author and source are credited.
Data Availability: All relevant data are within the manuscript and its Supporting Information files.
Funding: The authors received no specific funding for this work.
Competing interests: The authors have declared that no competing interests exist.
1. Introduction
The global financial crisis (GFC) in 2007–2009 has brought to light the importance of an efficient financial sector to the global economy. Following a series of recessions witnessed by emerging markets and the GFC, it has become blatant to practitioners, regulators, and researchers that the financial sector and macroeconomic variables are interrelated. Meanwhile, sustained economic growth is the primary focus of every economy. Haldane [ 1 ] re-echoed this when he averred that sustaining economic growth is the single most important determinant of the living standards of the society. Due to this, a copious amount of academic literature from finance and economic scholars has arguably been devoted to exploring the determinants of economic growth [ 2 – 5 ]. In this regard, the finance-growth nexus has become one of the oldest debates in finance and economic literature.
Early economists sharply disagreed on how the financial sector affects growth and development. Bauer, Meier, and Seers [ 3 ] did not consider finance worthy of discussion in their series of essays on “pioneers in economic development”. Similarly, Lucas [ 6 ] on the “mechanics of economic development” dismissed finance as an exaggerated factor in the determinants of growth. In the extreme form, Miller [ 7 ] remarks that the contribution of financial markets to economic growth should not be contemplated in any serious discussions at sophisticated fora. However, other economists in the late 1800s and the 1900s argued that excluding the role of the financial system in any discourse on economic growth will give a limited and myopic view of the phenomenon [ 8 – 11 ].
The financial sector ensures efficient allocation of financial resources by facilitating the transfer of financial resources to undertake productive investments in an economy. Hence, a well-functioning financial sector is needed to facilitate investment in the real sector, which ultimately leads to growth in economies [ 9 ]. In the financial intermediation process, financial intermediaries also reduce information asymmetry and carry the burden that individual investors will encounter in assessing firms, managers, and conditions in the macroeconomic environment [ 12 ]. Due to this function, they can identify and direct funds to entrepreneurs with higher chances of introducing a new production process and goods [ 13 , 14 ]. Furthermore, financial markets also play a key role in corporate governance [ 15 ]. The monitoring role of banks through debt covenants with firms strengthens corporate governance structures. Also, the monitoring role of financial intermediaries reduces the cost of the monitoring to the individual investors as it becomes the delegated monitor, saving the costs that the individual investors would have to pay, thereby reducing aggregate monitoring costs and eliminating the free-rider problem because the intermediary is doing the monitoring on the investors’ behalf [ 12 ]. Aside from economising the monitoring process, Bencivenga and Smith [ 16 ] show that institutional monitoring by financial intermediaries ensures that capital rationing is reduced, resulting in increased productivity and growth. Furthermore, financial intermediaries play a key role in ameliorating risk, facilitating exchanges, and pooling savings which, in turn, results in growth in the real sector.
In sharp contrast, Robinson [ 17 ] pioneered in his model of expanding economy that the growth in the financial sector will depend on the performance of the economy. In support of this, Gurley and Shaw [ 18 ] argued that in periods of economic growth, countries also experience rapid growth in financial assets. Further, they averred that the development of the financial sector is contingent on conditions of supply and demand of financial assets which are very sensitive to economic development. Likewise some authors also proffer that the financial sector may be an important indicator of economic growth and not necessarily the cause of growth. For instance, Rajan and Zingales [ 19 ] divulged that financial markets can anticipate future economic growth and respond in like manner. Thus, the theoretical channels on finance-growth literature have become complex and puzzling. In analysing these contrasting perspectives, Patrick [ 20 ] coined the term “demand-following” to explain the phenomenon in which the financial sector develops in response to growth from the real economy and “supply-leading” to represent the condition where a sustained real economic growth is driven by finance. Further, he proposed the “stage of development hypothesis” to explain the interrelationship in the supply-leading and demand-following phenomena. According to Patrick [ 20 ], by supporting innovative investments, the financial sector becomes a key driver to real economic growth as countries kick-start modern growth. However, as real growth in the economy persists, the impetus of the financial sector gradually diminishes and the demand-following dominates the relationship.
In the quest to empirically examine and ascertain the validity of these channels, several conflicting results have been documented in the contemporary literature. However, since empirical results on the phenomenon can be sensitive to proxies for the financial sector [ 21 ], an underlying issue that may explain disparities in the results is the absence of a consensus on whether market-based or bank-based measures provide an accurate proxy for the financial sector. On the one hand, financial markets permit greater diversification because they provide a richer set of tools to manage risks. As economies are maturing, the need for richer risk management tools and several investment vehicles become blatant, hence a legal and regulatory environment that encourages the development of a market-based system is prioritised [ 12 ], and therefore, a market-based measure correctly captures the financial sector of emerging economies. On the other hand, the liquidity that stock markets provide can adversely affect the allocation of resources. Shleifer and Summers [ 22 ] show that liquid markets create incentives for unhealthy takeover attempts that are socially inexpedient. Moreover, excess liquidity in capital markets may encourage an investment climate where investors, in an attempt to avoid the costly and careful process of monitoring, sell their shares inexpensively. This hinders corporate governance and induces inefficiencies in resource allocation. Consequent to this, a bank-based system offers better insights into the contributions of the financial sector to economic growth [ 23 , 24 ]. As a result, a potpourri of measures such as broad money, domestic credit to the private sector, venture capital availability, ease of access to loans, stock market turnover, market capitalization, and other stock market indices and bank-based indices have been used as proxies for the financial sector development to discriminate the finance-growth nexus [ 21 , 23 – 28 ].
Despite the arguments purporting to show the superiority of one measure over the other, stock markets and banks may play a complementary role in enhancing economic growth [ 29 – 32 ]. Corollary to this, a nascent and fledgling body of literature has directed their attention towards using the financial sector indices of countries [ 33 – 35 ] to measure the overall performance of the financial sectors of economies. Although the indices are a market-based measure, it captures the performance of banks, insurance services, real estate investment trusts, capital markets, and other providers of financial services whose services are germane to the financial sector and matter for risk diversification, resource allocation and dealing with information asymmetry. This is important because the different contributions of the various actors could have synergistic benefits on economic growth [ 36 ]. Hence, the financial sector indices enable us to capture the multidimensionality of the financial sectors of the economies of BRICS. Thus, we proxy the performance and growth of the indices which capture a broad array of firms offering financial services as a measure of financial development.
This paper offers different insights into the finance-growth nexus in two ways. First, we employ the financial sector index as a proxy for the financial sectors of the BRICS economies. This enables us to disentangle the contribution of the financial sector from the real sector in the finance-growth nexus and still capture the performance of banks and other providers of financial services. Second, we explore the time- and frequency-varying relationship between the financial sector index and economic growth in emerging markets with a bi-wavelet, partial wavelet, and wavelet multiple correlations (WMC). The extant literature that examines the relationship between the financial sector and economic growth assesses this phenomenon with less concentration on time-frequency analysis which has the potential to influence the results [ 32 , 37 – 41 ]. However, the intrinsic complexity in time series analysis has increased the time-frequency domain, thus, wavelet analysis is becoming a usual instrument for examining confined variations of power within a time series to define both prevailing modes of variability and how the modes change in time through decomposition. Thus, the application of bivariate, partial and wavelet multiple correlations (WMC) is important in this study. While bivariate wavelet coherence is a technique that shows the correlation between two variables, partial wavelet shows the comovements between two variables relative to a common interdependence, and the resulting wavelet transformation coherence of several variables is ideal for WMC [ 42 ]. Specifically, we seek to examine the lead-lag relationships at the diverse time and frequency domains using the bivariate and partial wavelet analysis. This will enable us to assess the time and frequency at which two variables lead or lag and further control for a common interdependence. We additionally assess the degree of integration between all the variables simultaneously for each of the selected emerging economies by applying the WMC. Thus, the WMC will be used to determine the leading/lagging variable relative to the scales through linear combinations for more than two variables to provide the full picture of the nexus [ 43 ].
In particular, we examine the time-varying relationship between the financial sector and economic growth and control for external uncertainty shocks for several reasons. The stage of development hypothesis implies that the finance-growth nexus could be time-varying. Specifically, Patrick [ 20 ] divulged that, for a start, the financial sector would drive economic growth, but such impetus will diminish over time and then, economic growth will lead the relationship. Similarly, Grochowska, Diaconescu, Margerit, and Tomova [ 44 ] proffered that the relationship between finance and growth is complex, unstable over time, and varies over the cycle. Furthermore, external uncertainty may undermine activities of the financial sector and can be detrimental to growth in BRICS economies. Bhattarai, Chatterjee, and Park [ 45 ] note that external uncertainty shocks exhibit significant uncertainty spillovers to emerging economies. Previously, Horvath and Zhong [ 46 ] had documented that external shocks have a sizeable impact on macroeconomic fluctuations in emerging countries and that a considerable fraction of this impact is through the domestic stock market.
Global policy uncertainty can negatively affect banks’ liquidity creation [ 47 ] and negatively influence stock market performance [ 48 ] which can hurt the stability of the financial sector [ 49 ] in BRICS. In another vein, economic policy uncertain can have severe implications on investments, consumption and employment [ 47 ] and consequently economic growth [ 50 ]. Thus, in analysing the time-varying dynamics in the finance-growth nexus, we also note that global uncertainty shocks can affect the comovements between the financial sector and economic activity, and has implications on the extent to which the financial sector indices and economic growth leads/lags in a linear combination at multiscales (short-, medium-, and long-term). Accordingly, the partial and wavelet multiple techniques accurately delineate the interdependencies demonstrated by the finance-growth dynamics in the midst of global economic policy uncertainty shocks.
We focus on examining the time-varying relationships between the financial sector index and economic growth in BRICS (Brazil, Russia, India, China, and South Africa) for two key reasons. First, these countries are considered among the leading emerging economies in the world and are also viewed as building blocks for innovation. Although these economies have experienced recent development in their financial sectors, their financial sectors still lag behind that of other developed countries. Second, the economies of the BRICS countries have received little attention on the finance-growth nexus. The long-aged debate on the finance-growth nexus in the BRICS countries has largely been some other stock market-based or bank-based discussion, paying limited attention to the financial sector index, and the time-varying dynamics in the relationships. In our attempt to fill this gap, we note that these five countries command about 42% and a third of the world’s population and GDP respectively. Furthermore, they represent the leading emerging countries across several continents (Africa, Asia, Europe, and South America), and hence, the economies of BRICS provide valuable insights into the global emerging market economy. Accordingly, this paper investigates the time and frequency lead-lag relationships between the financial sector index and economic growth. We also control for a common interdependence (global economic uncertainty shocks) and examine the degree of integration among all the variables for each of the BRICS economies.
Our empirical results show that the financial sector indices and economic growth exhibit a bi-directional causality. Furthermore, we document overwhelming evidence that suggests that economic policy uncertainty significantly influence the relationship between the financial sector indices and economic growth as indicated by the partial wavelet. Moreover, the outcome from the wavelet multiple cross correlations indicates that the RFSI is the first mover at most time scales for the BRICS economies. We confirmed from the findings that the COVID-19 pandemic had an adverse impact on the contribution of RFSI to GDP when the pre-COVID-19 pandemic analysis was conducted for the multiple wavelet. Consequently, it was clear that the GEPU had less tendency to drive the RFSI and GDP for all economies in the pre-COVID-19 pandemic sample. In this sense, our study demonstrates that the relationship between the financial sector index and economic growth is time-varying and must be observed with external uncertainty shocks in perspective.
The next of this paper reviews brief literature on existing empirical studies. This is followed by a discussion of the methodology employed in the study. Further, the study also presents and discusses the findings and finally, provides some implications for policy and practice.
2. Literature review
The quest to ascertain a relationship between the financial sector and economic growth began as early as the 18th century. However, the modern empirical literature on the finance-growth nexus developed from the 1900s. The theoretical linkages have already been discussed and therefore for want of space, we present a summary of the extant empirical studies that have examined the phenomenon. These studies, which have mainly tested the direction of the causality between the variables have resulted in competing results and hypotheses.
The first strand of studies has given empirical support to the supply-leading hypothesis, where the financial sector is the main driver of economic growth. Notable amongst such are the studies of King and Levine [ 13 ], Levine, Loayza, and Beck [ 51 ], Chortareas et al. [ 52 ]. The demand-following hypothesis, which also argues that real economic growth is the driver of the financial sector has also received several empirical backing [ 53 – 55 ]. Furthermore, Chortareas et al. [ 52 ] documented evidence of a bi-directional relationship between the financial sector and economic growth in emerging markets. This is broadly classified as the feedback hypothesis [ 52 , 55 – 58 ]. In addition, the neutrality hypothesis, which states that there is no relationship between the financial sector and the banking sector has also been given attention in the extant literature [ 59 ]. In the wake of the disparities in the extant literature on the phenomenon, Vogiazas, and Nellis [ 60 ] argue that the exact role played by the financial sector in the finance-growth nexus is uncertain and complex. Although most of the aforementioned studies investigated the finance-growth nexus with panel estimation techniques, the relationship between the financial sector and economic growth may depend on country-specific specific characteristics [ 61 ].
Empirical evidence on the finance-growth nexus in BRICS countries reveals contrasting findings. In Brazil, Stefani [ 38 ] employed a vector autoregressive (VAR) model and documented evidence of finance-led growth. Da Silva [ 62 ] also employed a spatial-autoregressive disturbance model and documented that the financial sector in Brazil is positively linked to growth in the area. Using a non-linear ARDL model, Nyasha and Odhiambo [ 63 ] found evidence to conclude that that the stock markets in Brazil drive growth rather than the banking sector. This was also confirmed by Moyo et al. [ 37 ] who found stock market variables to affect economic growth in Brazil. For Russia, Ono [ 39 ] used a VAR model and documented evidence of causality from economic growth to money supply and bank lending (demand-following) hypothesis. Conversely, Ono [ 64 ] had found evidence of a supply-leading hypothesis. Similarly, Fidrmuc, Fungáčová, and Weill [ 65 ] revealed that bank’s liquidity creation positively affected growth in Russia. In India, several studies have recorded evidence to support a finance-led growth [ 66 , 67 ] and the supply-leading hypothesis [ 68 , 69 ]. However, Nain and Kamaiah [ 70 ] do not find any evidence of causation. Concerning China, the empirical evidence from the extant literature is also not so different from those garnered from Brazil, Russia and India. Pan and Mishr [ 23 ] found evidence of a growth-led stock market development in China. However, a meta-analysis performed by Guo and He [ 71 ] divulge that the banking sector is a key driver of economic growth than the stock market in China. Meanwhile, Tang [ 72 ] documents no evidence of a finance-led growth in China but contingent on industrialization. Similarly, Sunde [ 73 ] revealed evidence of bi-directional causality between financial development and economic growth in South Africa. Meanwhile, Odhiambo [ 74 ] had earlier rejected any possibility of a supply-leading relationship and concluded overwhelming evidence for a demand-following hypothesis. Further, Nyasha and Odhiambo [ 75 ] showed that bank-based measures exhibited a positive relationship with economic growth while market-based financial development had no relationship with growth in South Africa.
Although it is widely accepted in the literature that the financial sector is important to the economy. The summary of some empirical studies conducted in BRICS confirms that it is still unclear the exact contribution of the financial sector to economic growth. The disparities in existing empirical evidence could be because the relationship between the financial sector and economic growth is not stable over time [ 44 , 56 ]. Moreover, Guru and Yadav [ 21 ] show that proxies for the financial sector also explains differences in existing results. The extant literature has discriminated against the finance-growth nexus with various bank-based and other stock market-based proxies with little attention paid to the financial sector indices. In this regard, we attempt to explain how the relationship between the financial sector and economic growth varies over the business cycles and financial cycles in BRICS countries. We examine the lead-lag relationships at a different time and frequency domains using the bivariate wavelet analysis. We also note that the extent of global uncertainty shocks could affect economic activity and the financial sector [ 76 ] and has implications on the extent to which the financial sector indices and economic growth comove. In consequence of that, we also employ the partial wavelet analysis to assess the time and frequency at which two variables lead or lag and further control for a common interdependence. Finally, we assess the degree of integration between all the variables simultaneously for each of the selected emerging economies by applying the wavelet multiple correlations.
3. Methodology
The study examines the time-frequency co-movement between financial sector index and economic growth in BRICS using both Bivariate, Partial and Wavelet Multiple Analysis. In the following pages, we address the continuous wavelet transform (CWTs), Partial wavelet and “maximal overlap discrete wavelet transform” (MODWT) which is the initial point of the wavelet multiple correlations (WMC) and cross-correlations (WMCC) as indicated by Gençay et al. [ 77 ].
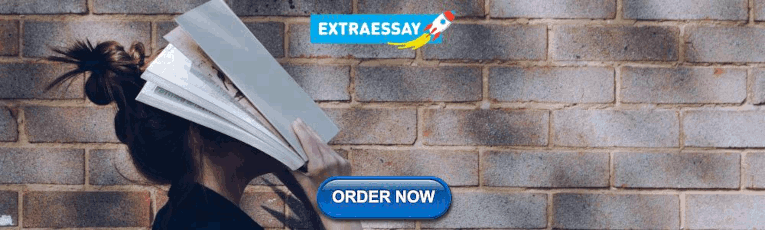
3.1. Bivariate wavelet analysis
3.1.1. continuous wavelet transform (cwt)..
3.1.2. Wavelet transform coherence.
3.1.3. WTC phase difference.
3.2. Partial wavelet coherence ( PWC )
Partial Wavelet Coherence can help solve the problem of “pure” correlation between international stock markets and eliminate the influence of time series z(t) on the wavelet coherence between x ( t ) and y ( t ) [ 81 ].
3.3. Wavelet multiple correlation (WMC)
4. Empirical analysis
4.1. data description.
The study employed monthly data on financial sector index (FSI) of BRICS economies which are made up of Brazil (Brazil IFinanceiro Index), Russia (Moscow Exchange Financial Index), India (NIFTY Financial Services Index), China (Shanghai Financials Sector Index) and South Africa (FTSE/JSE Financial 15 Index). We further consider the gross domestic product (GDP) of each country and the global economic policy uncertainty.
Owing to the quarterly release of GDP, the Organization for Economic Corporation and Development (OECD) constructed the monthly GDP to gauge its short-term dynamics. The monthly GDP is developed from chained volume estimates of quarterly GDP series in US dollars. Through a linear interpolation, the monthly GDP is obtained to align with the required month of a quarter. The data on financial sector indices were obtained from EquityRT database, economic growth data was gleaned from OECD database, and data on global economic policy uncertainty developed by Baker, Bloom, and Davis [ 83 ] was extracted from the website https://www.policyuncertainty.com/index.html . Both the GEPU and GDP are normalised to ensure stability and effective comparison over time. In addition, the GDP as provided by the OECD for each country is seasonally adjusted to deal with periodic swings in the GDP. Consequently, fluctuations that normally occur across time which includes inflation are expunged to better reflect the correct configurations in economic activity. In order to ensure a smooth comparison, we remove inflation (CPI) from the FSI observations for each country to obtain the real financial sector index (RFSI). As shown below, the study was based on monthly returns– r t = ln P t − lnP t −1 , where r t is the continuously compounded return, P t and P t −1 are current and previous indexes respectively. The suggested period as shown in Table 1 was chosen due to consistent data availability, hitherto it considers the COVID-19 pandemic period. The differences in sample window as shown in Table 1 do not distort the analysis of this study because each country is separately assessed to reveal its distinct finance-growth nexus dynamics. Notwithstanding, the bi-wavelet and partial wavelet techniques reveal chunks of time-frequencies which facilitate comparisons where dates overlap.
- PPT PowerPoint slide
- PNG larger image
- TIFF original image
https://doi.org/10.1371/journal.pone.0259303.t001
4.2. Descriptive statistics
Fig 1 illustrates both the price and log-return plots of the BRICS economies for inflation (CPI), financial sector index (FSI), economic activities (GDP) and global economic policy uncertainty (GEPU). We find that the CPI of Brazil, Russia and South Africa trends downwards, and upwards for China and India. The fluctuations in inflation for each country is quite substantial, and its absence from the analysis may confound the results. The significant variations in the monthly prices are apparent in Fig 1 especially during the global financial crisis of 2007–2009, and the COVID-19 pandemic period across markets. In addition, the log-return plots exhibit volatility clustering as anticipated due to the stylised facts of financial time series.
https://doi.org/10.1371/journal.pone.0259303.g001
Table 2 exhibits the summary of statistics for the CPI, financial sector index, economic growth (GDP) and global economic policy uncertainty (GEPU) during the period under study. The skewness values observed shows non-normality across board with the financial sector index of China exhibiting a skewness close to normality of -0.0268. On the other hand, kurtosis values further show leptokurtic behaviour in the values across variables especially global economic policy uncertainty. In terms of the stationarity test, the Augmented Dicky-Fuller (ADF) and the Kwiatkowski-Phillips-Schmidt-Shin (KPSS) are used. The observations from both the ADF and the KPSS reveal that all the data series explicitly fulfil the stationarity requirements. The White Neural Network test of linearity indicates that the GDP for BRICS is non-linear. This puts the time-frequency and frequency dependent analysis of this study into perspective.
https://doi.org/10.1371/journal.pone.0259303.t002
4.3. Main results
4.3.1. bi-wavelet and partial wavelet..
The statistical interpretations and the availability of codes for analysis were found in the biwavelet package provided by Gouhier et al. [ 84 ]. Right-pointing arrows and left-pointing arrows indicate when real financial sector index (RFSI) and gross domestic product (GDP) are respectively in-phase (movement in identical direction) and antiphase (movements in the reverse direction) to ensure the smooth interpretation of the results. Right-pointing arrows upwards and left-pointing arrows downwards mean that the first variable leads, while the left-pointing arrows upwards and the right-pointing arrows downwards mean that the second variable leads. The intensity of the interdependence between the paired series is indicated by the surface colour and the colour pallet is represented. The red (warm) colour signifies parts that have major interactions, while the blue (cold) colour shows a lower series correlation. The cone of influence (COI) within the bivariate plots indicates the region where interpretation of the wavelet becomes important. The results outside the COI may be distorted or unreliable because of the end-points influence of finite length signals.
Fig 2 presents the comovements between RFSI and GDP via the bivariate technique as well as the influence of GEPU on the nexus between RFSI and GDP through the partial wavelet coherence each for BRICS economies. In general, the combined influence of RFSI and GDP appears more dominating for BRICS economies except for China and possibly India from the Asian continent. The remaining BRICS economies exhibit a strong sensitivity for the period 2005–2021, mostly from the medium to the long-term domain (beyond 8 months scale). This is to say India and China demonstrate less intensity of significant influence for most time and frequency horizons. This is probably because even though China and India are in the top five biggest economies in the world, their financial sectors are still maturing [ 85 ]. Consequent to this, the size of the financial sector may not contribute immensely to commensurate the size of their GDP. More importantly, these economies rely heavily on sectors largely driven by foreign direct investment inflows [ 86 ].
https://doi.org/10.1371/journal.pone.0259303.g002
The right-pointing arrows upwards as shown for Brazil, Russia and South Africa from the medium-to-long terms horizon signify that the RFSI drives economic growth during these periods. As a result, the aforementioned countries’ economic development hinges on the effective development of their financial sector. Accordingly, the development of the financial system contributes to economic growth via fund inflow by offering capital accumulation and technological development infrastructure to economies [ 87 ]. The few right-pointing arrows downwards for Russia from 2007–2010 at scales 8–16 months demonstrates that economic growth significantly drives the financial sector. The 2007–2009 Global Financial Crisis and Euro Crisis of 2010 could be a plausible attribution. Notwithstanding, the right-pointing arrows for most BRICS economies depict that the RFSI and economic growth exhibit a positive relationship between them. This suggests that as either the financial sector or economic growth is improved, it contributes to the development of the other. This is to say, there exists a positive interdependency between the financial sector and economic growth, which is mainly realised from the medium to long-term perspective for most economies. In this regard, the development of the financial system would urge global investors to maximise their returns via more productive investments [ 9 , 10 , 88 ]. However, China exhibits the least dependency on finance growth nexus followed by India. Nonetheless, countries with the less developed financial system have a higher likelihood of experiencing market failure issues as a result of incomplete competition and asymmetric information [ 89 ].
Following the impact of the COVID-19 pandemic on most financial markets [ 90 ], we recognise some patches of right-pointing arrows downwards from 2019 to 2021 for most economies, especially in the long-term perspective. At this point that financial markets are adversely affected as indicated by extant literature [ 91 , 92 ] coupled with contagion effects from external shocks, there is a turn of event where economic growth now drives the financial sector which continues to establish the positive interdependency across time. This establishes that where economic growth increases, the demand for financial services (financial growth) is elicited by economic growth [ 93 ]. In the COVID-19 period, financial activities have been depressed with investors unwilling to pursue new investments due to uncertainty in economic conditions [ 94 ]. Consequent to this, investors and subsequent demand for financial assets and investment vehicles will be driven by positive news of economic growth.
Moreover, taking cognisance of shocks from external policy uncertainties on the relationship between RFSI and GDP, the significance of the already established relationship diminishes. That is, with the aid of the partial wavelet coherence, global economic policy uncertainty distorts the significance and directional comovements between RFSI and GDP. This signifies that the relationship between RFSI and GDP is more vulnerable to global economic policy uncertainty which the latter has been revealed by extant literature to have a significant influence on financial markets and economic activity [ 46 , 74 , 95 , 96 ]. The details of the statistical interpretations are saved for readers, however, a more extensive review could be made with the aid of the guidance provided in the initial paragraph of this section.
In summary, we document a bi-directional causality between the RFSI and economic growth. This supports both the supply-side perspective engineered by Schumpeter [ 9 ], which posits causality from finance to economic growth and the demand-side approach led by Robinson [ 17 ] which proposes that economic growth causes financial development. The outcome of the study also supports prior empirical studies within the field of financial development and economic growth [ 97 , 98 ], while other studies also indicate a unidirectional relationship [ 87 , 99 ]. We, therefore, advocate that finance-growth nexus as well as causality dynamics are time-varying, and are likely to be influenced by external policy uncertainty shocks.
4.3.2. Frequency-dependent analysis.
We present the wavelet multiple analysis separately for each BRICS economy based on the three main indicators, which are; real financial sector index (RFSI), gross domestic product (GDP) and global economic policy uncertainty (GEPU). We use a monthly data and set, lj , j = 1…4, of the wavelet factors, which are connected to times of, respectively, “2–4 months (short term), 4–8 months (medium-term), 8–16 months (medium-term), above 16 months (long-term)” from Figs 4 and 5 , following Li, Li, Yuan, and Yu [ 100 ].
4 . 3 . 2 . 1 . Wavelet bivariate correlations matrix . At 5 wavelet scales, the bivariate contemporary correlations are considered. Wavelet bivariate correlations matrix. The codes for the variables are GEPU (C1), GDP (C2) and RFSI (C3). For calculating wavelet correlation coefficients, the horizontal axis displays the possible combinations. If we switch from left to right, the similarities between the pairs of FSI, GDP and GEPU nexus become weaker. On the vertical axis, the wavelet scales reflect periods. The purpose of the bivariate contemporary correlation matrix in this paper addresses the correlation between the realizations of two possible combinations of time series in the same period (wavelet scale). Thus, the bivariate contemporary correlation matrix is frequency (scale) dependent other than having the features of both time-frequency domains as illustrated in Fig 2 .
From Fig 3 , we present the wavelet correlation matrix for RFSI, GEPU and GDP returns across the five wavelet scales, which does not seem to differ significantly from the bivariate analysis from Fig 2 . We find a mix of positive and negative relationships among the pairs. The RFSI and economic growth of Brazil demonstrate the maximum degrees of co-movement with coefficients fluctuating over 0.19 to 0.45 at diverse time scales averaging 0.31 with minimal extreme correlational values. This is followed by Russia, India, South Africa and China for the RFSI and GDP in the short-, medium-, and long-terms. However, the RFSI and GEPU of India seem to be highly correlated at 0.71 in the long-term horizon, with less correlation between the financial sector and economic growth. This pattern of dynamics of less connectedness among RFSI, GEPU and GDP from the short-medium terms (2–16 months) does not deviate from China. This means that, on average, India and China from the Asian continent seem to have low interdependencies between their RFSI and GDP, and a little high dependency with external uncertainty shocks relative to other BRICS economies. This is not startling as China and India have demonstrated similarities in many respect [ 101 ]. This developed relationship should be of much concern to investors and policymakers within this region to continuously monitor the patterns of change between these variables over time. The overall correlation analysis for each of the BRICS economies is attached as part of supporting information to the paper. Notwithstanding, evidence from the Wavelet bivariate correlations matrix contributes to the reasons why financial time series are not static.
Wavelet bivariate correlations matrix. The codes for the variables are GEPU (C1), GDP (C2) and RFSI (C3).
https://doi.org/10.1371/journal.pone.0259303.g003
4 . 3 . 2 . 2 . Wavelet multiple correlations (WMC) . Fig 4 ( Table 3 ) and Fig 5 ( Table 4 ) denote the wavelet multiple correlations and wavelet multiple cross-correlations respectively for the FSI, GEPU and GDP nexus returns series into frequency localization by the MODWT [ 82 ].
U-upper limits, L- lower (at 95% confidence interval).
https://doi.org/10.1371/journal.pone.0259303.g004
https://doi.org/10.1371/journal.pone.0259303.g005
https://doi.org/10.1371/journal.pone.0259303.t003
https://doi.org/10.1371/journal.pone.0259303.t004
Fig 4 and Table 3 establish the degree of integration between the study variables (FSI, GDP & GEPU) from the short-term to the long-term dynamics in a continuous fashion. It does not necessarily indicate which variable is leading or lagging, but the overall connection of the study variables. The degree of integration is comparatively high for the monthly return series reaching as high as approximately 0.9047 (South Africa) for the wavelet multiple correlations, 0.0000 (most BRICS economies) for the lower panel and 0.9860 (South Africa) for the upper panel. There is a continuous increment in multiple correlations over the horizon for only Russia and South Africa. Generally, there is very low integration between FSI, GDP and GEPU from the short-long term, except South Africa which recorded multiple correlations as high as above 90%. Thus, from South Africa, monthly returns of one variable can be explained by the remaining two (2) variables to a degree of about 90% from monthly, leading up to scale 16 months interdependence.
4 . 3 . 2 . 3 . Wavelet multiple cross correlations (WMCC) . The wavelet multiple cross-correlation coefficients are presented in Table 4 depicting four wavelet scales. From Fig 5 , the scales at the y-axis have similar meanings as indicated at the preliminary stage of the wavelet multiple analysis discussion. Following Asafo-Adjei, Adam and Darkwa [ 102 ], the x-axis, however, represents the lag length of the series. In this case, 12 months for positive and negative lags each. We need both positive and negative to confirm the potential leading and lagging variables. Localisations at positive lag denote lagging variable and negative lag denote leading variable at the respective scales. At the zero-lag of localisation (dashed) lines, there is no lead or lag. Localisation implies the maximum values in the linear combination of all variables at the wavelet scales, which are indicated by dashed lines within the dotted lines (at all lags). A variable listed on a scale indicates the variable with the potential to lead or lag all the other variables. It implies that, at that scale, it has the maximum value in the linear combination of all the variables at the respective scales. When a dashed line accompanies a listed variable in the heat map, then it becomes an actual lead (negative lag) or lag (positive lag) unless the dashed line is on the zero-lag which implies neither lead nor lag. Accordingly, the economic implication of the wavelet multiple cross-correlations (WMCC) is that it indicates the degree of interdependence between the variables, and determines the most influential variable at a specified wavelet scale to act as either a leading (first mover to respond to shocks) or lagging (the last variable to respond to shocks after the remaining variables) variable.
We find that the RFSI maximizes the multiple cross-correlations from a linear combination of the remaining variables (GDP and GEPU) at diverse scales for Brazil, Russia, China and India. Specifically for Brazil, the RFSI lags (at time 2 months) in the medium-term at scale 2, but leads (at times -4months) from the long-term at scale 4. On the other hand, the RFSI in Russia has the potential to lead/lag (at time 0 month) in the short-term, lags (at time 7 months) in the medium-term, and leads (at time -7 months) in the long-term. Further, there is no leading nor lagging RFSI in South Africa for the short-, medium-, and long-terms. The RFSI leads in the short-term for India, but in the medium-term for China. This indicates that the RFSI is a first mover at most time scales for most BRICS economies. Again,
This is followed by GDP which can be found at only one scale for Brazil and South Africa. Specifically, economic growth has the potential to lead the RFSI and GEPU for Brazil (at time 0 months), but lags for South Africa (at time 2 months). This clearly indicates that GDP does not maximise the multiple cross-correlations from a linear combination relative to the remaining variables for most economies.
It can be seen from the wavelet multiple cross-correlation plots in Fig 5 and Table 4 that global economic policy uncertainty leads or lags the RFSI and economic growth for the BRICS economies except Brazil. The impact of GEPU on FSI and GDP is worst for South Africa in about four cases in the medium-, and long-terms. This signifies that South Africa’s financial markets and economic growth are vulnerable to external uncertainty shocks. However, GEPU lags for India, but has a potential to lead or lag for Russia and China in the medium-term. Cui et al. [ 103 ] observed that external uncertainty shocks has the potential to undermine corporate innovativeness in China as investments in innovation become stifled.
4.3.3. Robustness check for COVID-19 pandemic shocks.
We further present a confirmatory analysis to establish whether the identified degree of integration between the RFSI and GDP in the midst of GEPU are mostly driven by the COVID-19 pandemic shock. We take insights from the World Health Organization’s (WHO) communiqué, which designated the COVID-19 outbreak a Public Health Emergency of International Concern (PHEIC) on January 30, 2020, and a pandemic on March 11, 2020. We, therefore, segregate the data based on the announcement by the WHO on March 11, 2020 as a global pandemic where the Pandemic had reached its peak, and largely impacted most economies. Consequently, analysis below are conducted prior to March, 2020.
It can be observed from Fig 6 that the relationship between the RFSI and GDP has improved as compared to the outcome of the full sample. We also find that the negative impact of GEPU on RFSI and GDP has minimised. This is to say, the COVID-19 pandemic has a significant impact on RFSI and GDP which heightens the adverse impact of GEPU as found in the full sample case.
The codes for the variables are GEPU (C1), GDP (C2) and RFSI (C3).
https://doi.org/10.1371/journal.pone.0259303.g006
Fig 7 ( Table 5 ) provides that the degree of integration between the RFSI and GDP in the midst of GEPU has improved for the BRICS economies, except India which is developing its financial sector. This indicates that prior to the COVID-19 pandemic, the contribution of RFSI to GDP was effective despite the adverse impact of GEPU. Specifically, the degree of integration for South Africa is quite phenomenal with high convergence in the long-term.
https://doi.org/10.1371/journal.pone.0259303.g007
https://doi.org/10.1371/journal.pone.0259303.t005
It is clearly shown in Fig 8 ( Table 6 ) that the tendency for GEPU to drive the RFSI and GDP has minimised for all economies relative to the full sample scenario. Consequently, we see the RFSI acting as a first mover in the long-term for the BRICS economies, except South Africa. Thus, in line with the arguments of Aghion, Howitt, and Levine [ 104 ], we confirm that the financial sector exhibits first-order impact on economic growth by shaping the severity of credit constraints and the rate of technological innovation [ 105 ]. Also, the RFSI maximizes the multiple cross-correlations from a linear combination of the remaining variables (GDP and GEPU) at diverse scales. This indicates that the COVID-19 pandemic has distorted most economic activities and heightened the level of uncertainties rendering effective assets allocation and portfolio diversification as part of the optimum choice for investors.
https://doi.org/10.1371/journal.pone.0259303.g008
https://doi.org/10.1371/journal.pone.0259303.t006
5. Conclusion and policy implication
The analysis of the study was performed with the aid of three econometric techniques via wavelet. These are the bivariate, partial and wavelet multiple correlations analysis. We, therefore, contribute to existing knowledge on time-frequency domain co-movement between RFSI and GDP, while incorporating shocks from global economic policy uncertainty (GEPU). The techniques enabled us to determine the lead-lag relationships between the study variables and to assess their interdependencies toward investment decisions, especially, portfolio diversifications, as well as for policy decision making.
Findings from the bivariate analysis suggest a bi-directional causality between RFSI and GDP. This supports both the supply-side perspective, which hypothesises causality from finance to economic growth and the demand-side approach which proposes that economic growth causes financial development. Again, there exists a positive interdependency between the financial sector and economic growth, and this is mainly realised from the medium to long-term perspective for most economies. In this aspect, financial system expansion would necessitate global investors maximizing their profits through more productive investments. However, China exhibits the least dependency concerning finance growth nexus, followed by India. Policymakers and other regulators of China and India should take a critical look at this outcome to ensure that their financial sector activities proceed with caution. This is because, countries with less developed financial systems have a higher likelihood of experiencing market failure issues as a result of incomplete competition and information asymmetry [ 89 ].
Evidence from the wavelet multiple correlations analysis indicates that the RFSI is the first mover at most time scales for the BRICS economies. This is followed by GEPU which either leads or lags for most scales, especially for South Africa. Consequently, we found that GDP lags for South Africa which is vulnerable to direct shocks from the GEPU. The lagging property for GDP in South Africa signifies that it is the last variable to respond to shocks, but the impact will most likely be significant. However, GDP has the potential to lead/lag the variables for Russia. The impact of GEPU on RFSI and GDP is worst for South Africa in about four cases in the medium-, and long-terms. This signifies that South Africa’s financial markets and economic growth are vulnerable to external uncertainty shocks. However, GEPU lags for India, but has a potential to lead or lag for Russia and China in the medium-term. This outcome contributes to the findings of Osei et al. [ 106 ] that EPU from China responds quickly to a rise in value of other ASIAN countries’ EPU indices, which may require possible policy uncertainty synergies and spillovers among the Asian economies. Despite the result of this study, Carré and L’œillet [ 107 ] in their review indicated that the nexus between finance-growth nexus is inconclusive.
We confirmed from the findings that the COVID-19 pandemic had an adverse impact on the contribution of RFSI to GDP when the pre-COVID-19 pandemic analysis was conducted for the multiple wavelet. The pre-COVID-19 pandemic sample analysis was needed for only multiple wavelet technique because its econometric properties allow us to assess only frequency domain. The bi-wavelet and partial-wavelet techniques which present the analysis for time-frequency domain also indicated that the COVID-19 pandemic had a significant impact on the BRICS economies’ RFSI, GDP and GEPU. Consequently, it was clear that the GEPU had the less tendency to drive the RFSI and GDP for all economies in the pre-COVID-19 pandemic sample relative to the full sample scenario. This indicates that the COVID-19 pandemic has distorted most economic activities and heightened the level of uncertainties rendering effective assets allocation and portfolio diversification as part of the optimum choice for investors.
The study offers an important empirical contribution to prior literature on finance-growth nexus, and also has practical consequences. First, we demonstrate that the RFSI of these emerging markets can be used to measure the performance of the financial sector. This enables a decoupling of the growth in the financial sector from the other sectors of their economies for a better assessment of the finance-growth nexus. Second, the outcomes from the study reveal the importance of the financial market to global investors by maximising their investment potentials to increase financial market integration among emerging economies. These developed relationship will enable economic growth to cushion the development of the financial sector against adverse external uncertainty shocks on financial markets. That is, from the demand-following perspective, economic growth would tend to increase the demand for financial services leading to financial sector development. It is recommended that policymakers, investors and researchers across the globe should incessantly observe the relationship between financial sector development and economic growth across time while considering adverse shocks from global economic policy uncertainty. To reveal hidden relationships, further studies may apply quantile regression [ 108 ] or transfer entropy [ 109 ] to examine the finance-growth nexus.
Supporting information
https://doi.org/10.1371/journal.pone.0259303.s001
- View Article
- Google Scholar
- 3. Bauer PT, Meier GM, Seers D. Pioneers in development: Second series. Oxford University Press. 1984.
- 5. Demirgüç-Kunt A, Levine R. Finance and growth. Edward Elgar Publishing Limited. 2018.
- 8. Bagehot W. Lombard Street: a Description of the Money Market: By Walter Bagehot. Henry S. King & Company 65 Cornhill & 12 Paternoster Row, London. 1873.
- 9. Schumpeter JA. Theory of economic development. Cambridge: Harvard University Press. 1911.
- 11. Goldsmith RW. Financial Structure and Development, New Haven, Yale University Press. Recuperado de http://www.econ.yale.edu/growth_pdf/cdp236.pdf . 1969.
- 22. Shleifer A, Summers LH. Breach of Trust in Hostile Takeovers. University of Chicago Press. 2013; 2: 33–68.
- 24. Allen F, Gale D. Comparing financial systems. MIT press. 2000.
- 47. Berger AN, Guedhami O, Kim HH, Li X. Economic policy uncertainty and bank liquidity creation. SSRN Scholarly Paper. Social Science Research Network, Rochester, NY. 2018.
- 77. Gençay R, Selçuk F, Whitcher BJ. An introduction to wavelets and other filtering methods in finance and economics. Elsevier. 2001.
- PubMed/NCBI
Cybersecurity hazards and financial system vulnerability: a synthesis of literature
- Original Article
- Published: 18 August 2020
- Volume 22 , pages 239–309, ( 2020 )
Cite this article
- Md. Hamid Uddin ORCID: orcid.org/0000-0003-3025-0206 1 , 2 ,
- Md. Hakim Ali 2 &
- Mohammad Kabir Hassan 3
2581 Accesses
24 Citations
1 Altmetric
Explore all metrics
In this paper, we provide a systematic review of the growing body of literature exploring the issues related to pervasive effects of cybersecurity risk on the financial system. As the cybersecurity risk has appeared as a significant threat to the financial sector, researchers and analysts are trying to understand this problem from different perspectives. There are plenty of documents providing conceptual discussions, technical analysis, and survey results, but empirical studies based on real data are yet limited. Besides, the international and national regulatory bodies suggest guidelines to help banks and financial institutions managing cyber risk exposure. In this paper, we synthesize relevant articles and policy documents on cybersecurity risk, focusing on the dimensions detrimental to the banking system’s vulnerability. Finally, we propose five new research avenues for consideration that may enhance our knowledge of cybersecurity risk and help practitioners develop a better cyber risk management framework.
This is a preview of subscription content, log in via an institution to check access.
Access this article
Price includes VAT (Russian Federation)
Instant access to the full article PDF.
Rent this article via DeepDyve
Institutional subscriptions
Similar content being viewed by others
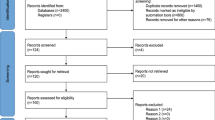
Machine learning-driven credit risk: a systemic review
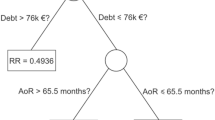
Applications of Explainable Artificial Intelligence in Finance—a systematic review of Finance, Information Systems, and Computer Science literature
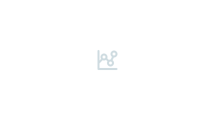
The 2007–2009 Financial Crisis: An Erosion of Ethics: A Case Study
The systems should be designed to ensure a high degree of security and operational reliability. Also, the system should be strong enough to predict the likelihood of future cyber events and take auto protective measures in anticipation of a cyber breach.
It is because cybersecurity risk cannot be mitigated by mere application of upgraded hardware or software as no technology is perfect, and perpetrators can always break the system. Therefore, cybersecurity is now a boardroom issue. It means the corporate managers of financial institutions need to consider the amount of investment in cyber technology more rationally. Otherwise, it might adversely affect the financial performance and stability of banks.
The operational efficiency declines because of unavoidable cybersecurity hazards that often require the users to apply more complex passwords or two-stage authentication. Since complex passwords and multi-stage authorization processes are difficult to memorize, the chances of access denial to genuine users increase that has an implication of time and cost for the users.
The asymmetry of cyber knowledge (between criminals and innocent users) allows criminals to exploit the innocent users to breach the system, suggesting a positive association between faster and widespread use of cyber technology and security risk exposure (Willison and Warkentin 2013 ; Longstaff et al. 2020 ).
Bouveret ( 2018 ) proposed a broader framework of cyber risk assessment that captures the different dimension of cybersecurity hazards such as (i) security threat level, (ii) system vulnerability status, and (iii) financial consequences of a security breach.
The expert report suggests that the DDoS attack can be prevented by increasing bandwidth, creating redundancy in cyber infrastructure, configuring hardware, and deploying anti-DDoS hardware and software, using the DDoS protection appliance, move to a cloud-based DNS provider (Rubens 2018 ).
Without technological support, hybrid securities cannot be developed.
For example, General Data Protection Regulation 2016 (for EU), the UK Data Protection Act 2018, the Personal Data Protection Act 2010 of Malaysia.
Abraham, S., and P.J. Shrives. 2014. Improving the relevance of risk factor disclosure in corporate annual reports. The British accounting review 46 (1): 91–107.
Google Scholar
ACSS. 2016. Australia’s cyber security strategy. Commonwealth of Australia, Department of the Prime Minister and Cabinet. https://cybersecuritystrategy.homeaffairs.gov.au/ .
Ahmad, N., and P. Schreyer. 2016. Measuring GDP in a digitalised economy . Paris: OECD Publishing. https://doi.org/10.1787/18152031 .
Book Google Scholar
AIG. 2016. December. Is cyber risk systemic? New York: American International Group. https://www.aig.com/content/dam/aig/america-canada/us/documents/business/cyber/aig-cyber-risk-systemic-final.pdf .
Akhawe, D., A. Barth, P.E. Lam, J. Mitchell, and D. Song. 2010. Towards a formal foundation of web security. In 2010 23rd IEEE computer security foundations symposium , 290–304. IEEE.
Akhisar, İ., K.B. Tunay, and N. Tunay. 2015. The effects of innovations on bank performance: The case of electronic banking services. Procedia—Social and Behavioral Sciences 195: 369–375.
Aldasoro, I., L. Gambacorta, P. Giudici, and T. Leach. 2020a. Operational and cyber risks in the financial sector. BIS Working Paper No. 840 . Basel, Switzerland: Bank for International Settlements.
Aldasoro, I., L. Gambacorta, P. Giudici, and T. Leach. 2020b. The drivers of cyber risk. BIS Working Paper No. 865 . Basel, Switzerland: Bank for International Settlements.
Alex Johnson. 2018, May 9. Equifax breaks down just how bad last year’s data breach was. NBC News. https://www.nbcnews.com/news/us-news/equifax-breaks-down-just-how-bad-last-year-s-data-n872496 .
Allen, F., and D. Gale. 2004. Competition and financial stability. Journal of Money, Credit and Banking 36 (3): 453–480.
Almansi, A.A. 2018. Financial sector’s cybersecurity: Regulations and supervision . Washington, United States of America: World Bank Group.
Almansi, A.A., Y.C. Lee, and J. Lincoln. 2017. Financial sector’s cybersecurity: A regulatory digest. World Bank . Washington: Financial Sector Advisory Center.
Ames, M., T. Schuermann, and H.S. Scott. 2015. Bank capital for operational risk: A tale of fragility and instability. Journal of Risk Management in Financial Institutions 8 (3): 227–243.
Antonescua, M., and R. Birău. 2015. Financial and non-financial implications of cybercrimes in emerging countries. Procedia Economics and Finance 32: 618–621.
Arner, D.W., J. Barberis, and R.P. Buckley. 2016. FinTech, RegTech, and the reconceptualization of financial regulation. Northwestern Journal of International Law & Business 37 (3).
Aseef, N., P. Davis, M. Mittal, K. Sedky, and A. Tolba. 2005. Cyber-criminal activity and analysis . Washington Education: White paper.
Ashford, W. 2019, July 31. Financial services top cyber attack target. Computer Weekly . https://www.computerweekly.com .
Aziz, A.S., M.A. Salama, A.E. Hassanien, and S.E.O. Hanaf. 2012. Artificial immune system inspired intrusion detection system using genetic algorithm. Informatica 36: 347–357.
Banker, R.D., R.J. Kauffman, and R.C. Morey. 1990. Measuring gains in operational efficiency from information technology: A study of the Positran deployment at Hardee’s Inc. Journal of Management Information Systems 7 (2): 29–54.
Barrett, M., E. Davidson, J. Prabhu, and S.L. Vargo. 2015. Service innovation in the digital age: Key contributions and future directions. MIS quarterly 39 (1): 135–154.
Barthelemy, J. 2001. The hidden costs of IT outsourcing: Lessons from 50 IT-outsourcing efforts show that unforeseen costs can undercut anticipated benefits. Understanding the issues can lead to better outsourcing decisions. MIT Sloan Management Review 42 (3): 60–72.
BDO. 2017. Cyber security in banking industry . India: BDO.
Beccalli, E. 2007. Does IT investment improve bank performance? Evidence from Europe. Journal of Banking & Finance 31 (7): 2205–2230.
Beitollahi, H., and G. Deconinck. 2012. Analyzing well-known countermeasures against distributed denial of service attacks. Computer Communications 35 (11): 1312–1332.
Benaroch, M., A. Chernobai, and J. Goldstein. 2012. An internal control perspective on the market value consequences of IT operational risk events. International Journal of Accounting Information Systems 13: 357–381.
Berger, A.N., and R.D. Young. 1997. Problem loans and cost efficiency in commercial banks. Journal of Banking & Finance 21 (6): 849–870.
Berkman, H., J. Jona, G. Lee, and N. Soderstrom. 2018. Cybersecurity awareness and market valuations. Journal of Accounting and Public Policy 37 (6): 508–526.
Bernik, I. 2014. Cybercrime: The cost of investments into protection. Journal of Criminal Justice and Security 16 (2): 105–116.
Biener, C., M. Eling, and J.H. Wirfs. 2015. Insurability of cyber risk: An empirical analysis. The Geneva Papers on Risk and Insurance-Issues and Practice 40 (1): 131–158.
BIS. 2004. Consolidated KYC risk management. Basel Committee on Banking Supervision. https://www.bis.org/publ/bcbs110.pdf .
BIS. 2013. The road to a more resilient banking sector. BIS. https://www.bis.org/publ/arpdf/ar2013e.htm .
BIS. 2016, June. Bank for international settlements. www.bis.org . https://www.bis.org/cpmi/publ/d146.pdf .
Boer, M., and J. Vazquez. 2017. Cyber security & financial stability: How cyber-attacks could materially impact the global financial system . Washington: The Institute of International Finance.
Böhme, R. 2010. Security Metrics and Security Investment Models. In Advances in information and computer security , ed. I. Echizen, N. Kunihiro, and R. Sasaki, 10–24. Berlin: Springer.
Böhme, R. 2012, February. Security audits revisited. in International conference on financial cryptography and data security , 129–147. Berlin: Springer.
Boin, A., and A. McConnell. 2007. Preparing for critical infrastructure breakdowns: The limits of crisis management and the need for resilience. Journal of Contingencies and Crisis Management 15 (1): 50–59.
Bouveret, A. 2018. Cyber risk for the financial sector: A framework for quantitative assessment. IMF Working Paper No. WP/18/143 . International Monetary Fund.
Bouveret, A. 2019a. Cyber risk for the financial services sector. Journal of Financial Transformation 49.
Bouveret, A. 2019b. Estimation of losses due to cyber risk for financial institutions. Journal of Operational Risk , Forthcoming.
Brechbuhl, H., R. Bruce, S. Dynes, and M.E. Johnson. 2010. Protecting critical information infrastructure: Developing cybersecurity policy. Information Technology for Development 16 (1): 83–91.
Brown, C.S. 2015. Investigating and prosecuting cyber crime: Forensic dependencies and barriers to justice. International Journal of Cyber Criminology 9 (01): 55–119. https://doi.org/10.5281/zenodo.22387 .
Article Google Scholar
Burden, K., and C. Palmer. 2003. Internet crime: Cyber crime—A new breed of criminal? Computer Law & Security Review 19 (3): 222–227.
Cabinet Decision. 2015. Cybersecurity strategy. The Government of Japan. https://www.nisc.go.jp/eng/pdf/cs-strategy-en.pdf .
Carey, M., and R.M. Stulz. 2008. The risks of financial institutions. Journal of Contingencies and Crisis Management 16 (1): 65–66. https://doi.org/10.1111/j.1468-5973.2008.00532_2.x .
CarlColwill, 2009. Human factors in information security: The insider threat—Who can you trust these days? Information Security Technical Report 14 (4): 186–196.
Caron, F. 2015. Cyber risk management in financial market infrastructures: Elements for a holistic and risk - based approach to cyber security. Belgium: National Bank of Belgium. https://lirias.kuleuven.be/1834699?limo=0 .
Carter, W.A., and D.E. Zheng. 2015. The evolution of cybersecurity requirements for the U.S. financial industry . USA: Center for Strategic and International Studies.
Caruana, J. 2009, February. Lessons of the financial crisis for future regulation of financial institutions and markets and for liquidity management . Washington, DC: IMF.
Casu, B., A. Ferrari, C. Girardone, and J.O. Wilson. 2016. Integration, productivity and technological spillovers: Evidence for eurozone banking industries. European Journal of Operational Research 255 (3): 971–983.
Cavusoglu, H., S. Raghunathan, and W. Yue. 2008. Decision-theoretic and game-theoretic approaches to IT security investment. Journal of Management Information Systems 25 (2): 281–304.
Cebula, J.J., and L.R. Young. 2010. A taxonomy of operational cyber. Carnegie-Mellon Univ Pittsburgh PA Software Engineering Inst.
Cetorelli, N., B. Hirtle, D. Morgan, S. Peristiani, and A.J. Santos. 2007. Trends in financial market concentration and their implications for market stability. Federal Reserve Bank of New York Policy Review 33–51.
Chauhan, Y., and S.B. Kumar. 2018. Do investors value the nonfinancial disclosure in emerging markets? Emerging Markets Review 37: 32–46.
Cherdantseva, Y., P. Burnap, A. Blyth, P. Eden, K. Jones, H. Soulsby, and K. Stoddart. 2016. A review of cyber security risk assessment methods for SCADA systems. Computers & Security 56: 1–27.
Choo, K.-K.R. 2011. The cyber threat landscape: Challenges and future research directions. Computers & Security 33 (8): 719–731.
Choo, K.-K.R., R.G. Smith, and R. McCusker. 2007. Future directions in technology-enabled crime: 2007–09 . Canberra: Australian Institute of Criminology.
Chowdhury, A. 2003. Information technology and productivity payoff in the banking industry: Evidence from the emerging markets. Journal of International Development 15 (6): 693–708.
Clare Sullivan, E.B. 2017. “In the public interest”: The privacy implications of international business-to-business sharing of cyber-threat intelligence. Computer Law & Security Review 33: 14–29.
Committee on Payments and Market Infrastructures. 2016. Guidance on cyber resilience for financial market infrastructures. Bank for International Settlements. https://www.bis.org/cpmi/publ/d146.pdf .
Crisanto, J.C., and J. Prenio. 2017, August. Regulatory approaches to enhance banks’ cyber - security frameworks. Bank for International Settlements. https://www.bis.org/fsi/publ/insights2.pdf .
Das, S., A. Mukhopadhyay, and M. Anand. 2012. Stock market response to information security breach: A study using firm and attack characteristics. Journal of Information Privacy and Security 8 (4): 27–55.
Deloitte. 2014. Transforming cybersecurity in the Financial Services Industry. Deloitte. https://www2.deloitte.com/content/dam/Deloitte/za/Documents/risk/ZA_Transforming_Cybersecurity_05122014.pdf .
Demirgüç-Kunt, A., L. Klapper, D. Singer, S. Ansar, and J. Hess. 2018. The global findex database 2017: Measuring financial inclusion and the Fintech revolution. The World Bank.
Derek Young, J.L. 2016. A framework for incorporating insurance in critical infrastructure cyber risk strategies. International Journal of Critical Infrastructure Protection 14: 43–57.
Diamond, D.W., and P.H. Dybvig. 1983. Bank runs, deposit insurance, and liquidity. Journal of Political Economy 91 (3): 401–419.
Diamond, D.W., and P.H. Dybvig. 1986. Banking theory, deposit insurance, and bank regulation. The Journal of Business 59 (1): 55–68.
Donge, Z., F. Luo, and G. Liang. 2018. Blockchain: A secure, decentralized, trusted cyber infrastructure solution for future energy systems. Journal of Modern Power Systems and Clean Energy 1–10.
Duffie, D., and J. Younger. 2019. Cyber runs. Hutchins Center Working Paper #51 . Washington, DC: The Hutchins Center on Fiscal & Monetary Policy, Brookings Institution.
Dufwenberg, M., and M.A. Dufwenberg. 2018. Lies in disguise—A theoretical analysis of cheating. Journal of Economic Theory 175: 248–264.
Duncan, N.B. 1995. Capturing flexibility of information technology infrastructure: A study of resource characteristics and their measure. Journal of Management Information Systems 12 (2): 37–57.
Duran, R.E., & P. Griffin. 2019. Smart contracts: Will Fintech be the catalyst for the next global financial crisis? Journal of Financial Regulation and Compliance (in press)
Dutta, A., and K. McCrohan. 2002. Management’s role in information security in a cyber economy. California Management Review . https://doi.org/10.2307/41166154 .
Eling, M., and M. Lehmann. 2018. The impact of digitalization on the insurance value chain and the insurability of risks. The Geneva Papers on Risk and Insurance-Issues and Practice 43 (3): 359–396.
Eling, M., and J. Wirfs. 2019. What are the actual costs of cyber risk events? European Journal of Operational Research 272 (3): 1109–1119.
Embrechts, P., H. Furrer, and R. Kauffman. 2003. Quantifying regulatory capital for operational risk. Derivatives Use, Trading and Regulation 9 (3): 217–233.
EU. 2018, May. The Directive on security of network and information systems (NIS Directive). https://ec.europa.eu/digital-single-market/en/network-and-information-security-nis-directive .
Euromoney. 2017, August 1. Technology investments drive up banks’ costs. Euromoney Magazine . London.
Fed. 2017, September. Federal reserve policy on payment system risk. Washington: Federal Reserve System.
Federal Office for Information Security. 2017. The state of IT security in Germany 2017. FOIS.
Fitch. 2017, April. Cybersecurity an increasing focus for financial institutions. https://www.fitchratings.com/site/pr/1022468 .
Francis, L., and V.R. Prevosto. 2010. Data and disaster: The role of data in the financial crisis. In casualty actuarial society e-forum, 62. New York: Springer.
Garg, A., J. Curtis, and H. Halper. 2003. The financial impact of IT security breaches: What do investors think? Information Systems Security 12 (1): 22–33.
Gatzlaff, K.M., and K.A. McCullough. 2010. The effect of data breaches on shareholder wealth. Risk Management and Insurance Review 13 (1): 61–83.
Gelenbe, E., and G. Loukas. 2007. A self-aware approach to denial of service defence. Computer Networks 51: 1299–1314.
Germano, J.H. 2014. Cybersecurity partnerships: A new era of public-private collaboration . New York: New York University School of Law.
Geyres, S., and M. Orozco. 2016. Think banking cybersecurity is just a technology issue? Think again. Accenture strategy. https://www.accenture.com/t20160419t004021__w__/us-en/_acnmedia/pdf-13/accenture-strategy-cybersecurity-in-banking.pdf .
Gladstone, R. 2016, March 15. Bangladesh Bank chief resigns after cyber theft of $81 million. The New York Times .
Glaessner, T., T. Kellermann, and V. McNevin. 2002. Electronic security: Risk mitigation in financial transactions — Public policy issues. The World Bank.
Goel, S., and H.A. Shawky. 2009. Estimating the market impact of security breach announcements on firm values. Information & Management 46 (7): 404–410.
Goldman, D. 2012, September 28. Major banks hit with biggest cyberattacks in history. CNN Business . Altanta.
Gommans, L., J. Vollbrecht, B.G.-D. Bruijn, and C.D. Laat. 2015. The service provider group framework a framework for arranging trust and power to facilitate authorization of network services. Future Generation Computer Systems 45: 176–192.
Goodman, S.E., and R. Ramer. 2007. Identify and mitigate the risks of global IT outsourcing. Journal of Global Information Technology Management 10 (4): 1–6.
Gopalakrishnan, R., and M. Mogato. 2016, May 19. Bangladesh Bank official’s computer was hacked to carry out $81 million heist: Diplomat. Reuters: Business News . Thomson Reuters.
Gordon, L.A., and M.P. Loeb. 2002a. The economics of information security investment. ACM Transactions on Information and Systems Security 5 (4): 438–457.
Gordon, L.A., and M.P. Loeb. 2002b. Return on information security investments, myths vs realities. Strategic Finance 84 (5): 26–31.
Gordon, L.A., M.P. Loeb, W. Lucyshyn, and T. Sohail. 2006. The impact of the Sarbanes-Oxley Act on the corporate disclosures of information security activities. Journal of Accounting and Public Policy 25 (5): 503–530.
Gracie, A. 2015. Cyber resilience: A financial stability perspective. Cyber defence and network security conference. London. https://www.bankofengland.co.uk/speech/2015/cyber-resilience-a-financial-stability-perspective .
Granåsen, M., and D. Andersson. 2016. Measuring team effectiveness in cyber-defense exercises: A cross-disciplinary case study. Cognition, Technology & Work 18 (1): 121–143.
Gupta, U.G., and A. Gupta. 2007. Outsourcing the is function: Is it necessary for your organization? Information Systems Management 9 (3): 44–47.
Gutu, L.M. 2014. The impact of Internet technology on the Romanian banks performance. In Proceedings of international academic conferences (No. 0702397). International Institute of Social and Economic Sciences.
Hall, C., R.J. Anderson, R. Clayton, E. Ouzounis, and P. Trimintzios. 2013. Resilience of the internet interconnection ecosystem. Economics of Information Security and Privacy III: 119–148.
Heeks, R. 2002. Information systems and developing countries: Failure, success, and local improvisations. The Information Society 18: 101–112.
Hemphill, T.A., and P. Longstreet. 2016. Financial data breaches in the U.S. retail economy: Restoring. Technology in Society 44: 30–38.
Herath, T., and H.R. Rao. 2009. Encouraging information security behaviors in organizations: Role of penalties, pressures and perceived effectiveness. Decision Support System 47 (02): 154–165.
HKMA. 2016. Enhanced competency framework on cybersecurity. Hong Kong: Hong Kong Monetary Authority. https://www.hkma.gov.hk/media/eng/doc/key-information/guidelines-and-circular/2016/20161219e1.pdf .
Ho, S.J., and S.K. Mallick. 2010. The impact of information technology on the banking industry. Journal of the Operational Research Society 61 (2): 211–221.
Holt, T.J., and E. Lampke. 2010. Exploring stolen data markets online: Products and market forces. Criminal Justice Studies 23 (1): 33–50.
Hon, W.K., and C. Millard. 2018. Banking in the cloud: Part 1—Banks’ use of cloud services. Computer Law & Security Review 34: 4–24.
Horne, R. 2014. The cyber threat to banking. PWC. https://www.bba.org.uk/wp-content/uploads/2014/06/BBAJ2110_Cyber_report_May_2014_WEB.pdf .
Hovav, A., and J. D’Arcy. 2004. The impact of virus attack announcements on the market value of firms. Information Systems Security 13 (3): 32–40.
Hsu, A.W.-H., H. Pourjalali, and Y.-J. Song. 2018. Fair value disclosures and crash risk. Journal of Contemporary Accounting & Economics 14 (3): 358–372.
Humayun, M., N. J. Mahmood Niazi, M. Alshayeb, and S. Mahmood. 2020. Cyber security threats and vulnerabilities: A systematic mapping study. Arabian Journal for Science and Engineering 1–19.
Hyytinen, A., and T. Takalo. 2002. Enhancing bank transparency: A re-assessment. Review of Finance 6 (3): 429–445.
IDSA. 2012. India’s cyber security challenge. New Delhi: Institute for Defence Studies and Analyses. https://idsa.in/system/files/book/book_indiacybersecurity.pdf .
IOSC. 2016. Cyber security in securities markets — An international perspective. International Organization of Securities Commissions. https://www.iosco.org/library/pubdocs/pdf/IOSCOPD528.pdf .
Ismail, N. 2018. The financial impact of data breaches is just the beginning . www.information-age.com . https://www.information-age.com/data-breaches-financial-impact-123470254/ .
ITU. 2012a. Cyberwellness profile hong kong. Hong Kong: ITU. https://www.itu.int/dms_pub/itu-d/opb/str/D-STR-SECU-2015-PDF-E.pdf .
ITU. 2012b. Cyberwellness profile poland. Poland: ITU. https://www.itu.int/en/ITU-D/Cybersecurity/Documents/Country_Profiles/Poland.pdf .
ITU. 2013. Cyberwellness profile Hungary. Hungary: ITU. https://www.itu.int/en/ITU-D/Cybersecurity/Documents/Country_Profiles/Hungary.pdf .
ITU Slovakia. 2012. Cyberwellness profile slovakia. ITU. https://www.itu.int/en/ITU-D/Cybersecurity/Documents/Country_Profiles/Slovakia.pdf .
Javaid, M.A. 2013. Cyber security: Challenges ahead. Available SSRN 2339594 . http://nexusacademicpublishers.com/uploads/portals/Cyber_Security_Challenged_Ahead.pdf .
Jayawardhena, C., and P. Foley. 2000. Changes in the banking sector—The case of Internet banking in the UK. Internet Research 10 (1): 19–31.
Johnson, K.N. 2015. Managing cyber risk. Georgia Law Review 50 (2): 548–592.
Jordan, J.S., J. Peek, and E.S. Rosengren. 2000. The market reaction to the disclosure of supervisory actions: Implications for bank transparency. Journal of Financial Intermediation 9 (3): 298–319.
Juma’h, A.H., and Y. Alnsour. 2020. The effect of data breaches on company performance. International Journal of Accounting & Information Management 28 (2): 275–301.
Kamiya, S., KangJun-Koo, K. Jungmin, A. Milidonis, and R. M. Stulz. 2020. Risk management, firm reputation, and the impact of successful cyberattacks on target firms. Journal of Financial Economics
Kark, K., A. Shaikh, and C. Brown. 2017, November 28. Technology budgets: From value preservation to value creation. Deloitte Insight. London.
Kauffman, R.J., J. Liu, and D. Ma. 2015. Technology investment decision-making under uncertainty. Information Technology and Management 16 (2): 153–172.
Kayworth, T., and D. Whitten. 2012. Effective information security requires a balance of social and technology factors. MIS Quarterly Executive 9(3).
Kesswani, N., and S. Kumar. 2015. Maintaining cyber security: Implications, cost and returns. Proceedings of the 2015 ACM SIGMIS Conference on Computers and People Research. New York: Association for Computer Machinery, 161–164.
Khoury, S., and E. Rolland. 2006. Conceptual model for explaining the IT investment paradox in the banking sector. International Journal of Technology, Policy and Management 6 (3): 309–326.
King, R.G., and R. Levine. 1993. Finance, entrepreneurship, and growth. Journal of Monetary Economics 3 (32): 513–542.
Ko, M., and C. Dorantes. 2006. The impact of information security breaches on financial performance of the breached firms: An empirical investigation. Journal of Information Technology Management 17 (2): 13–22.
Koette, M., and T. Poghosyan. 2009. The identification of technology regimes in banking: Implications for the market power-fragility nexus. Journal of Banking & Finance 33 (8): 1413–1422.
Kopp, E., L. Kaffenberger, and C. Wilson. 2017. Cyber risk, market failures, and financial stability, working paper. International Monetary Fund (WP/17/185).
Kox, H. L. 2013. Cybersecurity in the perspective of Internet traffic growth. Working paper. CPB Netherlands Bureau for Economic Policy Analysis. https://mpra.ub.uni-muenchen.de/47994/ .
Kröger, W. 2008. Critical infrastructures at risk: A need for a new conceptual approach and extended analytical tools. Reliability Engineering & System Safety 93 (12): 1781–1787.
Kunreuther, H., and G. Heal. 2003. Interdependent security. Journal of Risk and Uncertainty 26 (2–3): 231–249.
Kwast, M.L., and J.T. Rose. 1982. Pricing, operating efficiency, and profitability among large commercial banks. Journal of Banking & Finance 6 (2): 233–254.
Lagazio, M., N. Sherif, and A.M. Cushman. 2014. A multi-level approach to understanding the impact of cyber crime on the financial sector. Computers & Security 45: 58–74.
Lages, L.F. 2016. VCW-value creation wheel: Innovation, technology, business, and society. Journal of Business Research 69: 4849–4855.
Langton, J. 2018, June 4. Data breaches credit negative for BMO and CIBC: Moody’s . www.investmentexecutive.com : https://www.investmentexecutive.com/news/industry-news/data-breaches-credit-negative-for-bmo-and-cibc-moodys/ .
Lee, D., and S. Mithas. 2014. IT investments, alignment and firm performance: Evidence from an emerging economy. ICIS Conference Proceedings. Association for Information Systems. https://aisel.aisnet.org/icis2014/proceedings/ISStrategy/29/ .
Lever, K.E., and K. Kifayat. 2020. Identifying and mitigating security risks for secure and robust NGI networks. Sustainable Cities and Society 59: 102098.
Levine, R.G. 1993. Finance and growth: Schumpeter might be right. The Quarterly Journal of Economics 108 (3): 717–737.
Lewis, J.A. 2002. Assessing the risks of cyber terrorism, cyber war and other cyber threats . Washington, DC: Center for Strategic & International Studies.
Lewis, J., and S. Baker. 2013. The economic impact of cybercrime and cyber espionage. McAfee.
Li, H., W.G. No, and T. Wang. 2018. SEC’s cybersecurity disclosure guidance and disclosed cybersecurity risk factors. International Journal of Accounting Information Systems 30: 40–55.
Linsley, P.M., and P.J. Shrives. 2005. Transparency and the disclosure of risk information in the banking sector. Journal of Financial Regulation and Compliance 13 (3): 205–214.
Longstaff, T., C. Chittister, R. Pethia, and Y. Haimes. 2020. Are we forgetting the risks of information technology. Computer 33 (12): 43–51.
Low, P. 2017. Insuring against cyber-attacks. Computer Fraud & Security 2017: 18–20.
Macaulay, T. 2018. Critical infrastructure: Understanding its component parts, vulnerabilities, operating risks, and interdependencies , 1st ed. Boca Raton: Taylor and Francis Group.
Mandeville, T. 1998. An information economics perspective on innovation. International Journal of Social Economics 25 (2/3/4): 357–364.
Mayahi, A., and I. Humaid. 2016. Development of a comprehensive information security system for UAE e-Government. PhD thesis, Prifysgol Bangor University
McConnell, Patrick, and Keith Blacker. 2013. Systemic operational risk: Does it exist and if so, how do we regulate it? The Journal of Operational Risk 8 (1): 59–99.
McGraw, G. 2013. Cyber war is inevitable (unless we build security in). Journal of Strategic Studies 36 (1): 109–119. https://doi.org/10.1080/01402390.2012.742013 .
MCI. 2017. Public consultation paper on the draft cybersecurity bill. The Ministry of Communications and Information (MCI) and the Cyber Security Agency of Singapore. https://www.csa.gov.sg/~/media/csa/cybersecurity_bill/consult_document.pdf .
Ministry of Digital Affairs. 2017. National framework of cybersecurity policy of Republic of Poland for 2017-22 . Warsaw: Government of Poland.
Mohammed, A.-M., B. Idris, G. Saridakis, and V. Benson. 2020. Chapter 8—Information and communication technologies: A curse or blessing for SMEs? . New York: Academic Press.
Moore, T. 2010. The economics of cybersecurity: Principles and policy options. International Journal of Critical Infrastructure Protection 3 (3–4): 103–117.
Moore, T., S. Dynes, and F. Chang. 2015. Identifying how firms manage cybersecurity investment . Dallas: Southern Methodist University.
Morton, M., J. Werner, P. Kintis, K. Snow, M. Antonakakis, M. Polychronakis, and F. Monrose. 2018. Security risks in asynchronous web servers: When performance optimizations amplify the impact of data-oriented attacks. IEEE European Symposium on Security and Privacy , pp. 167–182.
Moumen, N., H.B. Othman, and K. Hussainey. 2015. The value relevance of risk disclosure in annual reports: Evidence from MENA emerging markets. Research in International Business and Finance 34: 177–204.
Mugarura, N., and E. Ssali. 2020. Intricacies of anti-money laundering and cyber-crimes regulation in a fluid global system. Journal of Money Laundering Control .
Mukhopadhyay, A., D.S. Samir Chatterjee, A. Mahanti, and A.S. Sadhukhan. 2013. Cyber-risk decision models: To insure IT or not? Decision Support Systems 56: 11–26.
NCG. 2016. 4 important cybersecurity focus areas for banks. Portland: Northcross Group. http://www.northcrossgroup.com .
NCSB. 2014. National cybersecurity strategy. Dhaka: ICT Ministry. https://sherloc.unodc.org/cld/lessons-learned/bgd/the_national_cybersecurity_strategy_of_bangladesh.html ?.
NCSC. 2018. The cyber threat to UK business. UK: The National Cyber Security Centre. https://www.ncsc.gov.uk/home .
Ngonzi, T.T. 2016. Theorizing ICT-based social innovation on development in the context of developing countries of Africa . Captown: University of Cape Town.
Ni, J., X. Lin, and X. Shen. 2019. Towards edge-assisted internet of things: From security and efficiency perspectives. IEEE Network 33 (2): 50–57.
OECD. 2015. Digital security risk management for economic and social prosperity: OECD recommendation and companion document . Paris: OECD Publishing.
OFR. 2017. Cybersecurity and financial stability: Risks and resilience. Office of Financial Research. https://www.financialresearch.gov/viewpoint-papers/files/OFRvp_17-01_Cybersecurity.pdf .
Page, J., M. Kaur, and E. Waters. 2017. Directors’ liability survey: Cyber attacks and data loss—A growing concern. Journal of Data Protection & Privacy 1 (2): 173–182.
Park, I., J. Lee, H.R. Rao, and S.J. Upadhyaya. 2006. Part 2: Emerging issues for secure knowledge management-results of a Delphi study. IEEE Transactions on Systems, Man, and Cybernetics-Part A: Systems and Humans 36 (3): 421–428.
Patterson, D., A. Brown, P. Broadwell, G. Candea, and J.C. Mike Chen. 2002. Recovery oriented computing (ROC): Motivation, definition, techniques, and case studies. UC Berkeley Computer Science.
Paul, J.A., and X. Wang. 2019. Socially optimal IT investment for cybersecurity. Decision Support Systems 122: 113069.
Pavlou, P.A., H. Liang, and Y. Xue. 2007. Understanding and mitigating uncertainty in online exchange relationships: A PrincipalAgent perspective. MIS Quarterly, 105–136.
Peeters, G. 2017. Strengthening the digital Achilles heel of the European Union: Make use of ethical hackers to find vulnerabilities in information systems? Master thesis .
Peng, C., M. Xu, S. Xu, and T. Hu. 2017. Modeling and predicting extreme cyber attack rates via marked point processes. Jornal of Applied Statistics 44 (14): 2534–2563.
Pirounias, S., D. Mermigas, and C. Patsakis. 2014. The relation between information security events and firm market value, empirical evidence on recent disclosures: An extension of the GLZ study. Journal of Information Security and Applications 19 (4–5): 257–271.
Power, M. 2005. The invention of operational risk. Review of International Political Economy 12 (4): 577–599.
PWC. 2014. Threats to the Financial Services sector. PWC. https://www.pwc.com/gx/en/financial-services/publications/assets/pwc-gecs-2014-threats-to-the-financial-services-sector.pdf .
PWC. 2015. Information security breaches survey. London: The UK Government. https://www.pwc.co.uk/assets/pdf/2015-isbs-technical-report-blue-digital.pdf .
Quinn, J.B., and M.N. Baily. 1994. Information technology: Increasing productivity in services. Academy of Management Perspectives 8 (3): 28–48.
Rackof, N., C. Wiseman, and W.A. Ullrich. 1985. Information systems for competitive advantage: implementation of a planning process. MIS Quarterly, 285-294.
Ralston, P., J. Graham, and J. Hieb. 2007. Cyber security risk assessment for SCADA and DCS networks. ISA Transactions 46: 583–594.
ReedSmith. 2017. China cybersecurity law. ReedSmith. https://www.reedsmith.com/en/perspectives/2017/01/chinas-cybersecurity-law .
Reuters. 2018, June 1. Bank of Chile trading down after hackers rob millions in cyberattack. Santiago. https://www.reuters.com/article/us-chile-banks-cyberattack/bank-of-chile-trading-down-after-hackers-rob-millions-in-cyberattack-idUSKBN1J72FC .
Rezek, T., T. Szatkowski, J. Świątkowska, J. Vyskoč, and M. Ziare. 2012. V4 cooperation in ensuring cyber security—Analysis and recommendations . Poland: The Kosciuszko Institute.
Ring, T. 2014. Threat intelligence: Why people don’t share. Computer Fraud & Security. 3: 5–9.
Risk.net. 2016, Jan 20. Top 10 operational risks for 2016 . www.risk.net . https://www.risk.net/risk-management/2441306/top-10-operational-risks-for-2016#risk1 .
Roth, A.V., and W.E. Jackson-III. 1995. Strategic determinants of service quality and performance: Evidence from the banking industry. Management Science 41 (11): 1720–1733.
Rubens, P. 2018, June 26. How to prevent DDoS attacks: 6 tips to keep your website safe. Nashville: eSecurity Planet, TechnologyAdvice. https://www.esecurityplanet.com/network-security/how-to-prevent-ddos-attacks.html .
Schwartz, M.J. 2013, March 21. South Korea Bank hacks: 7 key facts. Dark Reading. https://www.darkreading.com .
SCM. 2016. Guidelines on management of cyber risk. Securities Commission Malaysia. https://www.sc.com.my/api/documentms/download.ashx?id=9aaddb2e-aa13-409a-a47f-8d0124afd229 .
Scott, S.V., J.V. Reenen, and M. Zachariadis. 2017. The long-term effect of digital innovation on bank performance: An empirical study of SWIFT adoption in financial services. Research Policy 46 (5): 984–1004.
SecurityScoreboard. 2016. Financial industry cybersecurity report . New York: SecurityScoreboard.
Shackelford, S.J. 2012. Should your firm invest in cyber risk insurance? Business Horizons 55: 349–356.
Sharma, A., and P. Tandekar. 2018. Cyber security and business growth. IGI Global, 1208–1221.
Siegel, C.A., T.R. Sagalow, and P. Serritella. 2002. Cyber-risk management: Technical and insurance controls for enterprise-level security. Information Systems Security 11 (4): 33–49.
Skinner, D.J., and R.G. Sloan. 2002. Earnings surprises, growth expectations, and stock returns or don’t let an earnings torpedo sink your portfolio. Review of Accounting Studies 7: 289–312.
Smedinghoff, T.J. 2012. Solving the legal challenges of trustworthy online identity. Computer Law & Security Review 28: 532–541.
Solms, B.V. 2006. Information security—The fourth wave. Computers & Security 25 (3): 165–168.
Sommer, P., and I. Brown. 2011. “Future global shocks” reducing systemic cybersecurity risk . OECD/IFP: OECD.
Soomro, Z.A., M.H. Shah, and J. Ahmed. 2016. Information security management needs more holistic approach: A literature review. International Journal of Information Management 36 (2): 215–225.
Srinidhi, B., J. Yan, and G.K. Tayi. 2015. Allocation of resources to cyber-security: The effect of misalignment of interest between managers and investors. Decision Support Systems 75: 49–62.
State Bank of Pakistan. 2012. Guidelines on information technology security. The State Bank of Pakistan. http://www.sbp.org.pk/bsd/2004/Guidelines_on_IT_Security.pdf .
Stoneburner, G., A. Goguen, and A. Feringa. 2002. Risk management guide for information technology systems. Recommendations of the National Institute of Standards and Technology . Singapore: National Institute of Standards and Technology.
Teece, D.J. 2018. Profiting from innovation in the digital economy: Enabling technologies, standards, and licensing models in the wireless world. Research Policy 47 (8): 1367–1387.
Tendulkar, R. 2013. Cyber-crime, securities markets and systemic risk. CFA Digest 43 (4): 35–43.
Toivanen, H. 2015. Case study of why information security investment fail?. Master’s Thesis , 76. Jyväskylä: University of Jyväskylä.
Trautman, L.J., and K. Altenbaumer-Price. 2010. The board’s responsibility for information technology governance. John Marshall Journal of Computer and Information Law 28: 313.
Vagle, J. 2020. Cybersecurity and moral hazard. Stanford Technology Law Review 23: 71.
Veijalainen, J., V. Terziyan, and H. Tirri. 2006. Transaction management for m-commerce at a mobile terminal. Electronic Commerce Research and Applications 5: 229–245.
Watkins, B. 2014. The impact of cyber attacks on the private sector. Briefing Paper, Association for International Affair , 12.
Watters, P.A., S. McCombie, R. Layton, and J. Pieprzyk. 2012. Characterising and predicting cyber attacks using the cyber attacker model profile. Journal of Money Laundering Control 15 (4): 430–441.
Willison, R., and M. Warkentin. 2013. Beyond deterrence: An expanded view of employee computer abuse. MIS Quarterly 37 (1): 1–20.
Wright, D., S. Gutwirth, M. Friedewald, P.D. Hert, M. Langheinrich, and A. Moscibroda. 2009. Privacy, trust and policy-making: Challenges and responses. Computer Law & Security Review 25: 69–83.
Yang, S.J., S. Byers, J. Holsopple, B. Argauer, and D. Fava. 2008. Intrusion activity projection for cyber situational awareness. 2008 IEEE international conference on intelligence and security informatics , 167–172. Taiwan: IEEE.
Zephirin, M.G. 1994. Switching costs in the deposit market. The Economic Journal 104 (423): 455–461.
Download references
Acknowledgements
This paper is the first output of Taylor’s University’s flagship research project # TUFR/2017/004/05. Md Hamid Uddin led this project over the last three years. He recently moved to the University of Southampton - Malaysia Campus yet engaged with the project until completion of research outputs and obligations under the funding contract. Md Hakim Ali worked as the research scholar while Mohammad Kabir Hassan is an external collaborator who has intellectual contribution in developing this manuscript. The first version of this paper was presented at the Annual Financial Liquidity Conference 2018, Budapest, Hungary. We acknowledge the valuable suggestions received from Igor Lončarski (University of Ljubljana) and Seema Narayan (RMIT University Australia). We thank Mohammed Sawkat Hossain, who helped us at the beginning of literature searching. We appreciate the contribution of David Asirvatham Nor Shaipah Abdul Wahab and Vinitha Guptan at Taylor’s University and Sabur Mollah at Sheffield University in launching this project three years ago. We are grateful to David Asirvatham (leader of Data Analytics, Modeling and Visualization research program) and Centre for Research Management at Taylor’s University for extending full support in implementing the project.
Author information
Authors and affiliations.
University of Southampton, Malaysia Campus, No. 3, Persiaran Canselor 1, Kota Ilmu Educity, Iskandar, 79200, Nusajaya, Johor, Malaysia
Md. Hamid Uddin
Faculty of Business and Law, Taylor’s University, No 1, Jalan Taylor’s, 47500, Subang Jaya, Selangor, Malaysia
Md. Hamid Uddin & Md. Hakim Ali
Department of Economics and Finance, University of New Orleans, 438 Kirschman Hall, 2000 Lakeshore Drive, New Orleans, LA, 70148, USA
Mohammad Kabir Hassan
You can also search for this author in PubMed Google Scholar
Corresponding author
Correspondence to Md. Hamid Uddin .
Ethics declarations
Conflict of interest.
On behalf of all authors, the corresponding author states that there is no conflict of interest.
Additional information
Publisher's note.
Springer Nature remains neutral with regard to jurisdictional claims in published maps and institutional affiliations.
This paper is the output of Taylor's University's flagship research project # TUFR/2017/004/05. Md Hamid Uddin led this project over the last three years. He recently moved to the University of Southampton – Malaysia Campus while maintaining engagement for this project until all outputs are published. Md Hakim Ali worked as the research scholar while M. Kabir Hassan is an external collaborator who has intellectual contribution in developing this manuscript. The first version of this paper was presented at the Annual Financial Liquidity Conference 2018, Budapest, Hungary. We acknowledge the valuable suggestions received from Igor Lončarski (University of Ljubljana) and Seema Narayan (RMIT University Australia). We thank Mohammed Sawkat Hossain, who helped us at the beginning of literature searching. We appreciate the contribution of David Asirvatham Nor Shaipah Abdul Wahab and Vinitha Guptan at Taylor's University and Sabur Mollah at Sheffield University in launching this project three years ago. We are grateful to David Asirvatham (leader of Data Analytics, Modeling & Visualization research program) and Centre for Research Management at Taylor's University for extending full support in implementing the project. All comments should be sent to Md Hamid Uddin at [email protected].
Appendix 1: literatures related to cyber security and operational costs
Appendix 2: literatures related to cybersecurity and institutional performance, appendix 3: literatures related to cybersecurity and operational risk, appendix 4: literatures related to cybersecurity disclosure and governance, appendix 5: cybersecurity guidelines in the global perspective, rights and permissions.
Reprints and permissions
About this article
Uddin, M.H., Ali, M.H. & Hassan, M.K. Cybersecurity hazards and financial system vulnerability: a synthesis of literature. Risk Manag 22 , 239–309 (2020). https://doi.org/10.1057/s41283-020-00063-2
Download citation
Published : 18 August 2020
Issue Date : December 2020
DOI : https://doi.org/10.1057/s41283-020-00063-2
Share this article
Anyone you share the following link with will be able to read this content:
Sorry, a shareable link is not currently available for this article.
Provided by the Springer Nature SharedIt content-sharing initiative
- Cybersecurity
- Banking stability
- Institutional performance
- Bank operational risks
- Find a journal
- Publish with us
- Track your research

An official website of the United States government
The .gov means it’s official. Federal government websites often end in .gov or .mil. Before sharing sensitive information, make sure you’re on a federal government site.
The site is secure. The https:// ensures that you are connecting to the official website and that any information you provide is encrypted and transmitted securely.
- Publications
- Account settings
Preview improvements coming to the PMC website in October 2024. Learn More or Try it out now .
- Advanced Search
- Journal List
- Front Psychol
Coronavirus pandemic impact on bank performance
1 School of Economics, Shandong University of Finance and Economics (SDUFE), Jinan, Shandong, China
2 Youth League Committee of Shandong University of Finance and Economics, (SDUFE), Jinan, Shandong, China
Mohsin Shabir
3 School of International Trade and Economics, Shandong University of Finance and Economics (SDUFE), Jinan, Shandong, China
Associated Data
Publicly available datasets were analyzed in this study. This data can be found at: Bankscope Database, World Governance Indicators, and World Development Indicators.
This study examines the effects of the coronavirus (COVID-19) epidemic on the performance of the banking sector. Our sample consists of 1,575 banks in 85 countries from 2020Q1 to 2021Q4. The findings demonstrate that the COVID-19 outbreak has significantly decreased bank performance. Moreover, the adverse impact of COVID-19 on the bank’s performance depends on the bank’s and country-specific aspects. The adverse effect of the COVID-19 outbreak on bank performance is higher in smaller, undercapitalized, and less diversified banks. At the same time, a better institutional environment and financial development have significantly increased the strength and resilience of banks. The results are quite robust to using the alternative bank performance measures and estimation techniques. These findings provide practical implications for regulators and policymakers in the face of unprecedented uncertainty caused by COVID-19 epidemics.
JEL Classification: G01, G21, L50.
Introduction
In early December 2019, Wuhan City, China, first observed the beginning of the novel coronavirus (COVID-19; Demir and Danisman, 2021 ; Padhan and Prabheesh, 2021 ). Since its origin in Wuhan, COVID-19 has wreaked havoc worldwide because it is a highly transmittable and pathogenic viral infection ( Zaremba et al., 2020 ). Countries including China, Italy, Spain, France, the United Kingdom, and the United States have been hit hard by the severe COVID-19 outbreak ( Padhan and Prabheesh, 2021 ; Song et al., 2021 ). Therefore, on March 11, 2020, the World Health Organization (WHO) stated that COVID-19 is a global pandemic outbreak and is considered a “once-in-a-century pathogen” for the following reasons. Firstly, COVID-19 is perhaps a unique outcome in terms of its global scope as a pandemic. This disease’s rapid transmission rate shows that COVID-19 is more dangerous than any other pandemic ( Padhan and Prabheesh, 2021 ). Secondly, the mortality risk of COVID-19 is 1%, which is worse than normal influenza because it can kill healthy and older people. This fatality risk is comparable to the 1,857 influenza pandemic (0.6%) and the 1,918 Spanish flu (2%). However, due to the absence of pharmaceutical innovations, the actual death rate of COVID-19 is unpredictable ( Padhan and Prabheesh, 2021 ).
The outbreak of COVID-19 brought seriously affected health care, economy, transportation, and other fields in different industries and regions ( Shen et al., 2020 ). Moreover, the COVID-19 pandemic has reverberated across economies and financial markets and greatly impacted real economic activity. The economic impact of COVID-19 can be generally divided into two aspects: supply and demand impacts. The supply impact is the outcome of reductions in working hours and aggregate demand resulting from reduced incomes due to unemployment related to the lockdown. However, Maliszewska et al. (2020) highlight the four key channels through which a pandemic affects the economy. First, it directly impacts through the decrease in employment, which drives a reduction in the demand for capital, leading to a loss in output. Second, the rise in transaction costs increases the costs of imports and exports for goods and services, resulting in a drop in trade and productivity. Third, with a sharp reduction in travel, governments have imposed several restrictions to reduce infections that disrupt international tourism and lead to lower incomes and loss of productivity. Finally, the declined in demand for services. The demand seems to have taken a big hit, as these emergency shutdowns have also locked households into their homes, dramatically reducing consumer spending.
However, this ongoing COVID-19 has taken significant losses for countless businesses, leading to serious disruptions in various industries ( Khlystova et al., 2022 ). A growing body of literature observes the potential impact of the COVID-19 pandemic on different aspects. In this regard, one group of researchers focuses on the short-term effect of COVID-19 on stock market returns or volatility. They show that COVID-19 significantly reduces stock market returns and increases stock market volatility ( Al-Awadhi et al., 2020 ; Baek et al., 2020 ; Zaremba et al., 2020 ; Harjoto et al., 2021 ). At the same time, the other group of researchers has investigated the COVID-19 pandemic’s impact on the firm’s financial performance in various sectors ( Shen et al., 2020 ; Hu and Zhang, 2021 ; Atayah et al., 2022 ; Wellalage et al., 2022 ). Shen et al. (2020) show that firm performance worsens during the COVID-19 pandemic, which is more significant when a firm’s sales revenue or investment scale is low. At the same time, Hu and Zhang (2021) reported that the adverse effect of COVID-19 on firm performance is less pronounced in countries with better institutional environments, well-developed financial systems, and better health care systems.
While this unexpected shock is likely to impact banks, little is yet known about how it might affect the resilience and performance of the banking system as a whole ( Goodell, 2020 ; Duan et al., 2021 ). This is because the bank generally faces a broader range of risks compared to other financial institutions and is more closely connected with economic agents’ day-to-day activities ( Barua and Barua, 2020 ). Banks traditionally deal with a wide range of risks. The pandemic is set to exacerbate them through liquidity shortages, credit reduction, falling returns from investments, and rises in non-performing loans and default rates ( Barua and Barua, 2020 ; Goodell, 2020 ). This may be worse in nations where banks support millions of individuals and firms with comparatively low financial and economic capacity under a weak policy environment and high market competition ( Barua and Barua, 2020 ).
Coronavirus can affect banks in different ways. For example, banks worldwide hold large US dollar-denominated borrowings to fund international trade and financial investments ( Aldasoro and Ehlers, 2018 ). Financial crises tighten the money markets that lend dollars, implying risks for the global banking system. However, as a first response to the pandemic, central banks stretched current swap lines and formed new lines to reduce the cost of dollar funding ( Bahaj and Reis, 2020 ; Demir and Danisman, 2021 ). Bank prudential regulatory actions, such as relaxing the treatment of non-performing loans and reducing capital buffers, mitigate the adverse effect of COVID-19 on the financial system’s stability ( Demir and Danisman, 2021 ; Bitar and Tarazi, 2022 ). Danisman et al. (2021) presented that equity markets in countries with more strict regulatory requirements on capital and liquidity are more resilient to COVID-19. However, due to Basel III capital and liquidity reforms since 2008, banks are well situated to engage the extreme effect of COVID-19. At the same time, facilitation of the behavior of non-performing loans and capital buffers during a pandemic can put banks’ solvency at risk. The possibility of an increase in non-performing loans and substantial withdrawal of deposits by firms and households will adversely affect the performance of banks ( Danisman et al., 2021 ; Goodell, 2020 ). Besides, COVID-19 could adversely affect the efficiency of firms across all businesses, and there could be spillover effects on banks, which would increase their exposure to credit risk. This would threaten their stability and create some obstacles to future intermediation with some potential spillovers to the real economy ( Demir and Danisman, 2021 ).
This study contributes to the literature in the following aspects. First, prior literature examined the impact of COVID-19 on macroeconomic prospectives, such as economic growth ( Apergis and Apergis, 2021 ), International Trade ( Gruszczynski, 2020 ; Vidya and Prabheesh, 2020 ), oil price ( Mensi et al., 2020 ; Gharib et al., 2021 ), and gold price ( Mensi et al., 2020 ). While at the firm level, most existing studies focus on the impact of COVID-19 on a firm’s performance ( Fu and Shen, 2020 ; Gu et al., 2020 ; Shen et al., 2020 ; Xiong et al., 2020 ; Škare et al., 2021 ) and stock market returns and volatility ( Al-Awadhi et al., 2020 ; Baek et al., 2020 ; Zaremba et al., 2020 ; Harjoto et al., 2021 ). At the same time, the impact of COVID-19 on bank performance is scarce. In this study, we shifted the perspective to bank performance at the international level. Secondly, we explored the mechanisms through which COVID-19 affects bank performance. Lastly, as COVID-19 spreads globally, we determine whether the impact of COVID-19 on bank performance varies with institutional quality and level of financial development.
This study examines how the COVID-19 outbreak impacts the banking sector’s performance worldwide. Our sample comprises 1,575 listed and unlisted banks in 85 countries from 2020Q1 to 2021Q4. We use numerous alternative bank performance measures for a comprehensive examination and robustness. The findings illustrate that COVID-19 has significantly decreased bank performance. Moreover, the COVID-19 epidemic’s effects on the bank’s performance vary in the bank’s and country-specific aspects. The finding shows that adverse effects of COVID-19 on bank performance are more pronounced in smaller, undercapitalized, and less diversified banks. Also, a better institutional environment and financial development diminish the negative effects of COVID-19 on banks’ performance. Our primary results continue across alternative model specifications (i.e., GMM).
The rest of the paper is organized as follows. The section “Literature review” provides an overview of the relevant literature. The section “Data and methodology” defines our sample, study variables, econometric model, and summary statistics. The sections “Results and discussion” and “Robustness checks” report the empirical outcomes and robustness tests, respectively. The section “Conclusion” presents the conclusion of the paper.
Literature review
Coronavirus is a major health emergency worldwide. The outbreak of COVID-19 has severely affected healthcare, economy, transportation, and other sectors in various industries and regions ( Padhan and Prabheesh, 2021 ). This increasing pandemic in emerging and developed countries has led to strict lockdowns and unprecedented economic activity disruptions ( Baldwin and di Mauro, 2020 ; Padhan and Prabheesh, 2021 ). For example, in the second quarter of 2020, global GDP fell by more than 4.9 percent due to economic disruption ( Padhan and Prabheesh, 2021 ). The deterioration in the international trade in goods and services was possibly greater than during the global financial crisis of 2007–08 ( IMFC, 2020 ). Therefore, due to weak supply and demand, international trade was constricted by 3.5 percent in the second quarter of 2020 ( Vidya and Prabheesh, 2020 ). A rapid drop in consumption of goods and services was observed due to a sharp drop in income and weak consumer confidence. Moreover, emerging countries experienced substantial capital outflows and reduced investment and productivity due to the pandemic ( BIS, 2019 ; Padhan and Prabheesh, 2021 ).
Moreover, prior literature analyzed the COVID-19 impact in several ways. For example, Choi (2020) and Njindan Iyke (2020) stated that due to COVID-19, production and credit were reduced. Bauer and Weber (2020) , Liu et al. (2020) , and Yu et al. (2020) proved a significant reduction in consumption, investment, and labor force participation rate. Moreover, COVID-19 has unfavorably affected corporate performance ( Gu et al., 2020 ; Shen et al., 2020 ) and herding behavior ( Espinosa-Méndez and Arias, 2020 ). Additionally, some researchers have explored the impact of COVID-19 on the price of oil ( Fu and Shen, 2020 ; Narayan, 2020 ). They highlighted that the deterioration in oil prices due to the pandemic unfavorably influenced the energy sector’s performance. Fu and Shen (2020) and Narayan (2020) argued that COVID-19 increased oil price volatility and negatively affected energy industries,
The COVID-19 pandemic also amplified worldwide financial risks and destructively affected international financial markets ( Al-Awadhi et al., 2020 ; Cao et al., 2020 ; Harjoto et al., 2020 ). COVID-19 has adversely impacted the stock market in the form of ambiguity and a decline in global stock returns, decreasing capital inflows, and creating constraints on investment, new project financing, and accessibility to liquidity in the international financial system ( Padhan and Prabheesh, 2021 ). Guedhami et al. (2021) found that international firms experienced considerably lower stock prices than domestic companies during the pandemic crisis. They also demonstrate that the better financial system of the country moderates these negative performance impacts while real characteristics increase negative crisis returns. Al-Awadhi et al. (2020) and Wang and Enilov (2020) show that COVID-19 significantly reduces stock market returns. Zaremba et al. (2020) illustrate that COVID-19 led to a significant rise in stock market volatility.
Additionally, COVID-19 has a devastating effect on the efficiency of firms across all businesses and may have spillover effects on banks, increasing their exposure to credit risk. Acharya and Steffen (2020) revealed that the increased pace of reducing credit growth, particularly by riskier companies, could damage bank balance sheets and lesser their capital adequacy ratios. This would threaten their stability and create obstacles to upcoming intermediation by possible spillovers to the real economy. Li et al. (2020) reported that U.S. banks significantly amplified their lending if they had more idle loan commitments at the start of the pandemic. However, they argued that though banks improved their credit growth, their total credit supply remained unchanged. In the same way, Greenwald et al. (2020) show that U.S. banks that experienced large credit line drawdowns were more restrictive in lending to small firms during the COVID-19 crisis. Beck and Keil (2021) demonstrate that U.S. banks faced increasing loan loss provisions and non-performing loans. Hasan et al. (2021) illustrate that the spread of syndicated loans increased as the lender or borrower became more vulnerable to epidemics. Ҫolak and Öztekin (2021) and Duan et al. (2021) investigate the effect of the pandemic on global lending and banks’ systemic risk from an international perspective. Demir and Danisman (2021) show that stock returns of banks with a large size, lesser non-performing loans, well capitalization, and higher deposits are more resilient to the pandemic. Dursun-de Neef et al. (2022) show that worse-capitalized banks increased their loan supply significantly more during the pandemic. Elnahass et al. (2021) show that COVID-19 significantly affects financial performance over various financial performance and stability measures. Demirgüç-Kunt et al. (2021) argued that liquidity assistance, borrower support programs, and monetary easing moderated the negative effects of the crisis, but their effects varied significantly across banks and countries. Therefore, based on this evidence in this study, we will find the impact of COVID-19 on bank performance.
Data and methodology
Data and sample selection.
To analyze the impact of COVID-19 on the banking sector, we obtained quarterly balance sheet data of 1,575 listed and unlisted banks in 85 different countries from the Bankscope database for 2020Q1 to 2021Q4. 1 Quarterly frequency data are preferred for the following reasons: (a) The most important reason is that daily and monthly data are unavailable for financial and accounting data; (b) the COVID-19 period covers only two quarters. Hence, our frequency is driven by current financial and accounting data availability in 2020–21. Country-related variables such as GDP per capita , inflation, and bank concentration are taken from IMF and World Bank. Table 1 reports a detailed explanation of all variables and sources. Table 2 displays the summary statistics of the variables of interest.
Variables definition.
This table presents detailed descriptions of study variables.
Descriptive statistics.
This table shows summary statistics for the variables used in this study.
Measurements of variables
Bank performance measurement.
Although banking institutions have become gradually complex, profitability is the underlying driver of bank performance. In this study, we used the two accounting-based measures that are widely used in the earlier literature ( Adesina, 2021 ; Dang and Dang, 2021 ; Elnahass et al., 2021 ) as a dependent variable to evaluate the bank’s performance. These accounting-based measures return on average total assets (ROAA) and return on average equity (ROAE). These are the banking sector’s most accepted financial performance measures ( Simpson and Kohers, 2002 ). Moreover, we also used several alternative proxies of bank performance as robustness.
COVID-19 indicators
In this study, we follow Ҫolak and Öztekin (2021) and use the total number of COVID-19 confirmed cases per million in the country as a proxy for COVID-19.
Bank and country-specific variables
In addition to COVID-19, we have incorporated numerous bank-related and country-related control variables in our model to address the potential omitted variables problem. The bank-related control variables are bank size (SIZ), capitalization (CAP), liquidity (LIQ), asset structure (LTA), and bank diversification (DIV). Bank size (SIZE) is measured through the natural logarithm of a bank’s total assets. Capitalization (CAP) is estimated as equity to total assets. Bank liquidity (LIQ) is calculated as the ratio of liquidity assets to total assets. Net loan to total assets (LTA) is used as the bank’s asset structure proxy. Bank diversification (DIV) is measured by the non-interest income ratio to net operating income. At the same time, the country-related control variables are GDP per capita (GDPpc), inflation (INF) and bank concentration (CON). We use GDP per capita and inflation rates to control business cycles’ overall effects, unobserved factors that vary across countries ( Wu et al., 2020 ). Finally, bank Concentration (CON) controls the country’s market structure.
Empirical framework
In this study, to examine the impact of the COVID-19 pandemic on bank performance, our baseline model is as follows
where I , j t indicate the bank, country and quarter (time). BP denotes our dependent variables bank performance, which is measured as ROAA and ROAE. COVID-19 is our primary explanatory variable measured as the total number of COVID-19 confirmed cases per million in the country. X i t is a vector of our bank-related control variables. Z j t is a vector of country and market structure control variables. β, γ , a n d δ are the parameters of the model. Moreover, μ i , and ʎt are the bank and time effects and ε i t is the error term. We estimate equations (1) with the fixed-effects model. 2
Results and discussion
Baseline regression results.
Our core objective of the study is to determine the possible impacts of the COVID-19 pandemic on bank performance across countries. For this purpose, we regresses the bank performance on COVID19 and show our baseline regression model results in Table 3 . In columns (1) and (5) in Table 3 , we analyze the impact of COVID-19 on bank performance along cross-sectional and time fixed-effects, but we do not incorporate bank and country-related control variables. In columns (2) and (6), we contain the bank-related control variable, while in columns (3) and (7); we comprise country-related control variables. In columns (4) and (8), we incorporate all bank and country-related control variables with cross-sectional and time fixed-effects to examine the impacts of COVID-19 on bank performance. Overall, our findings highlight the significant negative effects of COVID-19 on bank performance in the sampling countries. Columns (4) and (8) in Table 3 show that COVID-19 coefficients are statistically significant with a negative sign with both ROAA and ROAE of bank performance measures. This finding is consistent with Elnahass et al. (2021) and shows that the outbreak of COVID-19 has significantly decreased the banking sector’s profitability. To simplify the economic interpretation of the regression coefficients of our key variables of interest, we implement a log transformation to COVID-19. The coefficient of COVID-19 reflects the βCOVID-19% change in bank performance for a 1% change in the number of disease cases per million.
COVID-19 impact on bank performance.
This table shows the results of the baseline regression on analyzing the effect of the COVID-19 epidemic on bank performance. The sample comprises 1,575 banks in 85 countries from 2020 Q1 to 2021 Q4. Our dependent variable is bank performance measured as ROA and ROE. COVID-19 is our primary explanatory variable of interest, measured as the total number of COVID-19 confirmed cases per million in the country. Robust standard errors are reported in parentheses.
This finding can be interpreted as the spread of the virus forcing governments to initiate several preventive measures, such as social distancing, lockdowns, and business shutdowns ( Duan et al., 2021 ). These activities, in turn, lead to adverse economic impacts on firms and households. As a result, firms have experienced significant declines in revenue and increased cost, and households have experienced job losses and income declines ( Duan et al., 2021 ). Therefore, firms and households may not be able to service their debt, increasing the probability of default ( Bartik et al., 2020 ). These effects are likely to spread to banks, resulting in lost revenue and a surge in non-performing loans, negatively affecting banks’ profits, capital, and solvency ( Beck and Keil, 2021 ). Furthermore, lower demand for bank services may result in lower non-interest income, lowering bank profitability and performance ( Beck and Keil, 2021 ).
Regarding the first set (bank-specific) of control variables, we find that the bank size (SIZE) coefficients are statistically significant and positively linked with ROAA and ROAE. This result aligns with earlier studies by Adesina (2021) and Dang and Dang (2021) and shows that large banks have high ROAA and ROAE. Similarly, capitalization (CAP) also significantly positive impacts ROAA and ROAE. These outcomes supported the empirical finding of Chortareas et al. (2012) and Adesina (2021) , suggesting that better-capitalized banks are highly efficient than those with a lower capital base. Also, the coefficients of asset structure (LTA) significantly positively impact ROAA and ROAE, demonstrating that a better bank asset structure enhances bank profitability. Lastly, bank diversification is also positively associated with ROAA and ROAE. These results support the bank’s diversification advantage and show that reliance on sources of non-interest income enhances the bank’s profits.
In contrast, regarding the country-related control variables. This outcome indicates greater concentration enhances the banking sector’s performance and efficiency. The GDP per capita coefficients show a significant positive relationship with bank performance. At the same time, the estimated inflation coefficients show an adverse and highly significant relationship in all bank performance measures. The bank concentration coefficient is positively linked with ROAA and ROAE.
Bank heterogeneity
Furthermore, we extend our basic analysis to examine how bank characteristics shape the effects of COVID-19 shocks on bank performance. Current studies have shown that bank features such as size, capitalization, liquidity, and diversification have significantly influenced bank performance ( Altunbas et al., 2012 ; Shabir et al., 2021 ). Therefore, to estimate the heterogeneity across the bank, we include the interaction terms of bank size, capitalization, liquidity, and diversification with COIVD-19 in our main regression model. The results are reported in Table 4 . The outcomes show that the coefficients on the interaction term of COVID-19 with size, liquidity, and diversification are positive and statistically significant on ROAA and ROAE. At the same time, the coefficient of interactions of COVID-19 with capitalization is significantly negative with all bank performance measures in ROAA and ROAE. These findings indicate that large size, more liquid and well-diversified banks reduce the adverse impact of COVID-19 on bank performance. In contrast, the poorly capitalized bank increases the adverse impact of COVID-19 on bank performance.
Role of bank heterogeneity.
This table demonstrates how a bank with diverse characteristics responds to the COVID-19 epidemic. The sample comprises 1,575 banks in 85 countries from 2020 Q1 to 2021 Q4. Our dependent variable is bank performance measured as ROA and ROE. COVID-19 is our primary explanatory variable of interest, measured as the total number of COVID-19 confirmed cases per million in the country. Robust standard errors are reported in parentheses.
COVID-19 and bank performance: Role of the institutional quality
The quality of institutions plays an important role during the financial crisis ( Klomp and De Haan, 2014 ; Fazio et al., 2018 ). Numerous recent studies have shown that various aspects of the formal and informal institutional environment significantly affect a bank’s profitability and risk levels. Beck et al. (2006) show that the regulatory policies and institution quality are significantly related to the banking system’s stability. Klomp and De Haan (2014) highlight that tight regulatory policy and higher supervision power reduce bank risk. To capture the institutional quality, we followed the previous literature and used Worldwide Governance Indicators (WGI), which contains six different aspects of institutional quality (i.e., government effectiveness, political stability, regulatory quality, control of corruption, the rule of law, and accountability). Therefore, for a more comprehensive analysis of COVID-19 and bank performance nexus, we include the interaction terms of COVID-19 with institutional quality in the regression model (1). The results are presented in Table 5 , which shows that the coefficients of the institutional quality variable and their interaction terms with COVID-19 are significantly positive at different levels. This indicates that better institutional quality increases the bank’s performance in response to COVID-19 epidemics.
Bank performance during the COVID-19 pandemic. The role of the institutional quality.
The role of the institutional quality. This table presents the country’s institutional quality role during the COVID-19 pandemic. The sample comprises 1,575 banks in 85 countries from 2020 Q1 to 2021 Q4. Our dependent variable is bank performance measured as ROA and ROE. COVID-19 is our primary explanatory variable of interest, measured as the total number of COVID-19 confirmed cases per million in the country. We used the Worldwide Governance Indicators (WGI) to capture the institutional quality, which contains six different aspects of institutional quality such as government effectiveness (GEF), political stability (PST), regulatory quality (RQL), control of corruption (COC), the rule of law (RUL), and voice and accountability (VOA). Robust standard errors are reported in parentheses.
COVID-19 and bank performance: Role of financial development
Additionally, prior empirical and theoretical literature highlight that the development of the financial sector has a positive influence on economic activity by increasing the performance of financial services, capital allocation, technological innovation, the efficiency of resource distribution, risk management, and reducing the risk of crises ( Levine, 1997 ; Vithessonthi and Tongurai, 2016 ). Therefore, we further examine whether the financial development of a country’s banking system mitigates the pandemic’s adverse effect on bank performance. For this reason, we use the financial development index (FDI) from IMF, which summarizes how developed the financial institution is in terms of its depth (FID), access (FIA), and efficiency (FIE). The finding is reported in Table 6 , which shows that the coefficient of COVID-19 remains significantly negative in ROAA and ROAE. At the same time, the coefficient for all financial development measures and their interaction terms are significantly positive with ROAA and ROAE. These findings consistently show that banks in countries with more financial development are less vulnerable to COVID-19 shocks on bank performance than in other countries.
Bank performance during the COVID-19 pandemic The role of financial development.
The role of financial development. This table reports the role of financial development during the COVID-19 pandemic. The sample comprises 1,575 banks in 85 countries from 2020 Q1 to 2021 Q4. Our dependent variable is bank performance measured as ROA and ROE. COVID-19 is our primary explanatory variable of interest, measured as the total number of COVID-19 confirmed cases per million in the country. We have taken the four different indexes from IMF to capture the financial development of a country, such as financial development index (FDI), financial institution depth (FID), financial institution access (FIA), and financial institution efficiency (FIE). Robust standard errors are reported in parentheses.
Robustness checks
Alternative dependent variable.
It is challenging to evaluate and capture a bank’s overall performance using a single measure ( Lee et al., 2014 ; Baselga-Pascual and Vähämaa, 2021 ). Therefore, as robustness, we further examine whether our main findings hold when we use alternative measures of bank performance. For this purpose, we followed existing literature ( Liang et al., 2013 ; Adesina, 2021 ; Dang and Huynh, 2022 ) and used the net interest margin ratio (NIM), cost-to-income ratio (CIN), and non-performing loans (NPL) as an alternative measure of for bank performance. The results are reported in Table 7 , which shows that COVID-19 coefficients remain statistically significant with a negative (positive) sign in NIM (CIN and NPL) of bank performance measures. 3 This finding shows an adverse impact of COVID-19 on bank performance remains consistent with the previous findings in Table 3 .
Alternative dependent variable.
This table presents the result of our baseline regression models by using the net interest margin ratio (NIM), cost-to-income ratio (CIN), and non-performing loans (NPL) as alternative measures of bank performance. The sample comprises 1,575 banks in 85 countries from 2020 Q1 to 2021 Q4. COVID-19 is our primary explanatory variable of interest, measured as the total number of COVID-19 confirmed cases per million in the country. Robust standard errors are reported in parentheses.
Alternative methodology
Our model may have possible endogeneity issues due to reverse causality, omitted variable, and control variable. Therefore, we employ the generalized method of moments (GMMs) and use the two-step system estimator with adjusted standard error for potential heteroskedasticity proposed by Blundell and Bond (1998) as robustness to test our main outcomes are sensitive to estimation approaches. The technique accounts for the unobserved heterogeneity and the dynamic nature of panel data. Moreover, it is more appropriate to deal with possible endogeneity issues and is highly reliable even in reverse causality, omitted variables, and measurement errors ( Bond and Hoeffler, 2001 ). Table 8 describes the results of the System GMM. We find that our baseline finding in Table 3 is still consistent even though we are considering unobserved heterogeneity, simultaneity, and dynamic endogeneity.
Alternative methodology.
This table expresses the impact of the COVID-19 epidemic on bank performance by using the System GMM. The sample comprises 1,575 banks in 85 countries from 2020 Q1 to 2021 Q4. Our dependent variable is bank performance measured as ROA and ROE. COVID-19 is our primary explanatory variable of interest, measured as the total number of COVID-19 confirmed cases per million in the country. Robust standard errors are reported in parentheses.
Coronavirus is not just a global epidemic and public health crisis. There is a widespread consensus among economists that this has devastatingly impacted the global economy. The economic damage led by the COVID-19 epidemics is mainly due to the reductions in income, productivity, unemployment increase, and trade disruptions. This study investigates how the COVID-19 outbreak affects the banking sector’s performance worldwide. Our sample comprises 1,575 listed and unlisted banks in 85 countries from 2020Q1 to 2021Q4. We use numerous alternative bank performance measures for a comprehensive examination and robustness. The findings illustrate that the outbreak of COVID-19 has significantly reduced bank performance.
We also determine whether the COVID-19 epidemic’s influence on the bank’s performance depends on the bank’s and country-specific aspects. For this reason, we find that bank performance is most negatively affected by the COVID-19 outbreak in smaller, undercapitalized, and less diversified banks. Moreover, we find a better institutional environment and financial development diminish the negative effects of COVID-19 on banks’ performance. Our primary results continue across alternative model specifications (i.e., GMM). The current study’s findings have important policy implications for researchers, policymakers, regulators, and financial institutions to manage risks within and across countries. As policy implications, the study suggests that the government should provide larger economic support, loosened capital requirements, and adjust insolvency rules to mitigate the negative impact of COVID-19. The current study’s major limitation is related to the small number of banks in our sample. We use data from only 1,575 banks whose quarterly data are available. Therefore, if our sample size were larger, more favorable results may have emerged. In future research directions, this study can be further expanded by comparing the impact of COVID-19 on Islamic versus conventional banks.
Data availability statement
Author contributions.
All authors listed have made a substantial, direct, and intellectual contribution to the work and approved it for publication.
This work was sponsored by the Natural Science Foundation of Shandong Province, China (grant number: ZR2021MG004) and the Youth Entrepreneurship Talent Introduction and Education Team of Colleges and Universities in Shandong Province, China.
Conflict of interest
The authors declare that the research was conducted in the absence of any commercial or financial relationships that could be construed as a potential conflict of interest.
Publisher’s note
All claims expressed in this article are solely those of the authors and do not necessarily represent those of their affiliated organizations, or those of the publisher, the editors and the reviewers. Any product that may be evaluated in this article, or claim that may be made by its manufacturer, is not guaranteed or endorsed by the publisher.
1 We choose this sample of banks because of the quarterly availability of data on the Bankscope database.
2 Hausman test suggests that the fixed-effects estimator is more appropriate compared to the random-effects estimator in our study.
3 Note that in Table 7 , the bank performance measure variables such as cost-to-income ratio (CIN), and non-performing loans (NPL) are calculated in such a way that increases the variables indicates lower bank performance and increase the risk. In the interest margin ratio (NIM) case, a higher value shows more bank profitability.
- Acharya V. V., Steffen S. (2020). The risk of being a fallen angel and the corporate dash for cash in the midst of COVID . Rev. Corp. Financ. Stud. 9 , 430–471. doi: 10.1093/rcfs/cfaa013 [ CrossRef ] [ Google Scholar ]
- Adesina K. S. (2021). How diversification affects bank performance: the role of human capital . Econ. Model. 94 , 303–319. doi: 10.1016/j.econmod.2020.10.016 [ CrossRef ] [ Google Scholar ]
- Al-Awadhi A. M., Alsaifi K., Al-Awadhi A., Alhammadi S. (2020). Death and contagious infectious diseases: impact of the COVID-19 virus on stock market returns . J. Behav. Exp. Financ. 27 :100326. doi: 10.1016/j.jbef.2020.100326, PMID: [ PMC free article ] [ PubMed ] [ CrossRef ] [ Google Scholar ]
- Aldasoro I., Ehlers T. (2018). The geography of Dollar funding of non-US banks. Available at: https://papers.ssrn.com/abstract=3316358
- Altunbas Y., Gambacorta L., Marques-Ibanez D. (2012). Do bank characteristics influence the effect of monetary policy on bank risk? Econ. Lett. 117 , 220–222. doi: 10.1016/J.ECONLET.2012.04.106 [ CrossRef ] [ Google Scholar ]
- Apergis E., Apergis N. (2021). The impact of COVID-19 on economic growth: evidence from a Bayesian panel vector autoregressive (BPVAR) model . Appl. Econ. 53 , 6739–6751. doi: 10.1080/00036846.2021.1946479 [ CrossRef ] [ Google Scholar ]
- Atayah O. F., Dhiaf M. M., Najaf K., Frederico G. F. (2022). Impact of COVID-19 on financial performance of logistics firms: evidence from G-20 countries . J. Glob. Operat. Strat. Sourc. 15 , 172–196. doi: 10.1108/JGOSS-03-2021-0028/FULL/PDF [ CrossRef ] [ Google Scholar ]
- Baek S., Mohanty S. K., Glambosky M. (2020). COVID-19 and stock market volatility: an industry level analysis . Financ. Res. Lett. 37 :101748. doi: 10.1016/J.FRL.2020.101748, PMID: [ PMC free article ] [ PubMed ] [ CrossRef ] [ Google Scholar ]
- Bahaj S., Reis R. (2020). Central bank swap lines during the Covid-19 pandemic. Covid Economics, April, 1–12. Available at: https://euagenda.eu/upload/publications/covideconomics2.pdf.pdf#page=5
- Baldwin R., di Mauro B.W. (2020). Mitigating the COVID economic crisis: act fast and do whatever it takes in centre for economic policy research. Available at: www.cepr.org
- Bartik A., Bertrand M., Cullen Z., Glaeser E. L., Luca M., Stanton C. (2020). How are small businesses adjusting to COVID-19? Early Evidence from a Survey . SSRN Electron. J. doi: 10.2139/ssrn.3574741 [ CrossRef ] [ Google Scholar ]
- Barua B., Barua S. (2020). COVID-19 implications for banks: evidence from an emerging economy . SN Bus. Econom. 1 , 19–28. doi: 10.1007/S43546-020-00013-W, PMID: [ PMC free article ] [ PubMed ] [ CrossRef ] [ Google Scholar ]
- Baselga-Pascual L., Vähämaa E. (2021). “ Female leadership and bank performance in Latin America ,” in Emerging Markets Review , Vol. 48 (North-Holland: Emerging Markets Review: ), p. 100807. [ Google Scholar ]
- Bauer A., Weber E. (2020). COVID-19: how much unemployment was caused by the shutdown in Germany? 16 doi: 10.1080/13504851.2020.1789544 [ CrossRef ] [ Google Scholar ]
- Beck T., Demirgüç-Kunt A., Levine R. (2006). Bank concentration, competition, and crises: first results . J. Bank. Financ. 30 , 1581–1603. doi: 10.1016/j.jbankfin.2005.05.010 [ CrossRef ] [ Google Scholar ]
- Beck T., Keil J. (2021). Are banks catching Corona? Effects of COVID on lending in the U.S . SSRN Electron. J. doi: 10.2139/ssrn.3766831 [ CrossRef ] [ Google Scholar ]
- BIS (2019). Monetary policy frameworks in EMEs: inflation targeting, the exchange rate and financial stability. In BIS annual economic report. Available at: https://www.bis.org/publ/arpdf/ar2019e2.htm
- Bitar M., Tarazi A. (2022). A note on regulatory responses to COVID-19 pandemic: balancing banks’ solvency and contribution to recovery . J. Financ. Stab. 60 :101009. doi: 10.1016/J.JFS.2022.101009 [ CrossRef ] [ Google Scholar ]
- Blundell R., Bond S. (1998). Initial conditions and moment restrictions in dynamic panel data models . J. Econ. 87 , 115–143. doi: 10.1016/S0304-4076(98)00009-8 [ CrossRef ] [ Google Scholar ]
- Bond S., Hoeffler A. (2001). GMM estimation of empirical growth models. CEPR Discussion Papers/Centre for Economic Policy Research Discussion Papers. Available at: https://papers.ssrn.com/abstract=290522
- Cao K. H., Li Q., Liu Y., Woo C. K. (2020). Covid-19’s adverse effects on a stock market index . Appl. Econ. Lett. 28 , 1157–1161. doi: 10.1080/13504851.2020.1803481 [ CrossRef ] [ Google Scholar ]
- Choi S. Y. (2020). Industry volatility and economic uncertainty due to the COVID-19 pandemic: evidence from wavelet coherence analysis . Financ. Res. Lett. 37 :101783. doi: 10.1016/j.frl.2020.101783, PMID: [ PMC free article ] [ PubMed ] [ CrossRef ] [ Google Scholar ]
- Chortareas G. E., Girardone C., Ventouri A. (2012). Bank supervision, regulation, and efficiency: evidence from the European Union . J. Financ. Stab. 8 , 292–302. doi: 10.1016/j.jfs.2011.12.001 [ CrossRef ] [ Google Scholar ]
- Ҫolak G., Öztekin Ö. (2021). The impact of COVID-19 pandemic on bank lending around the world . J. Bank. Financ. 133 :106207. doi: 10.1016/j.jbankfin.2021.106207 [ CrossRef ] [ Google Scholar ]
- Dang V. D., Dang V. C. (2021). How do bank characteristics affect the bank liquidity creation channel of monetary policy? Financ. Res. Lett. 43 :101984. doi: 10.1016/J.FRL.2021.101984 [ CrossRef ] [ Google Scholar ]
- Dang V. D., Huynh J. (2022). Monetary policy and bank performance: the role of business models . N. Am. J. Econ. Financ. 59 :101602. doi: 10.1016/j.najef.2021.101602 [ CrossRef ] [ Google Scholar ]
- Danisman G. O., Demir E., Zaremba A. (2021). Financial resilience to the covid-19 pandemic: the role of banking market structure . 53 , 4481–4504. doi: 10.1080/00036846.2021.1904118 [ CrossRef ] [ Google Scholar ]
- Demir E., Danisman G. O. (2021). Banking sector reactions to COVID-19: the role of bank-specific factors and government policy responses . Res. Int. Bus. Financ. 58 :101508. doi: 10.1016/j.ribaf.2021.101508 [ PMC free article ] [ PubMed ] [ CrossRef ] [ Google Scholar ]
- Demirgüç-Kunt A., Pedraza A., Ruiz-Ortega C. (2021). Banking sector performance during the COVID-19 crisis . J. Bank. Financ. 133 :106305. doi: 10.1016/j.jbankfin.2021.106305, PMID: [ PMC free article ] [ PubMed ] [ CrossRef ] [ Google Scholar ]
- Duan Y., El Ghoul S., Guedhami O., Li H., Li X. (2021). Bank systemic risk around COVID-19: a cross-country analysis . J. Bank. Financ. 133 :106299. doi: 10.1016/j.jbankfin.2021.106299, PMID: [ PMC free article ] [ PubMed ] [ CrossRef ] [ Google Scholar ]
- Dursun-de Neef H. Ö., Schandlbauer A., Wittig C. (2022). Countercyclical capital buffers and credit supply: evidence from the COVID-19 crisis . SSRN Electron. J. doi: 10.2139/SSRN.4052573 [ PMC free article ] [ PubMed ] [ CrossRef ] [ Google Scholar ]
- Elnahass M., Trinh V. Q., Li T. (2021). Global banking stability in the shadow of Covid-19 outbreak . J. Int. Financ. Mark. Inst. Money 72 :101322. doi: 10.1016/j.intfin.2021.101322 [ CrossRef ] [ Google Scholar ]
- Espinosa-Méndez C., Arias J. (2020). Herding behaviour in Asutralian stock market: evidence on COVID-19 effect . Appl. Econ. Lett. 28 , 1898–1901. doi: 10.1080/13504851.2020.1854659 [ CrossRef ] [ Google Scholar ]
- Fazio D. M., Silva T. C., Tabak B. M., Cajueiro D. O. (2018). Inflation targeting and financial stability: does the quality of institutions matter? Econ. Model. 71 , 1–15. doi: 10.1016/j.econmod.2017.09.011 [ CrossRef ] [ Google Scholar ]
- Fu M., Shen H. (2020). COVID-19 and corporate performance in the energy industry . Energy Res. Lett. 1 :12967. doi: 10.46557/001c.12967 [ CrossRef ] [ Google Scholar ]
- Gharib C., Mefteh-Wali S., Serret V., Ben Jabeur S. (2021). Impact of COVID-19 pandemic on crude oil prices: evidence from Econophysics approach . Res. Policy 74 :102392. doi: 10.1016/j.resourpol.2021.102392, PMID: [ PMC free article ] [ PubMed ] [ CrossRef ] [ Google Scholar ]
- Goodell J. W. (2020). COVID-19 and finance: agendas for future research . Financ. Res. Lett. 35 :101512. doi: 10.1016/J.FRL.2020.101512, PMID: [ PMC free article ] [ PubMed ] [ CrossRef ] [ Google Scholar ]
- Greenwald D. L., Krainer J., Paul P. (2020). The credit Line Channel. Federal Reserve Bank of san Francisco, working paper series, 1.000–96.000.
- Gruszczynski L. (2020). The CoviD-19 pandemic and international trade: temporary turbulence or paradigm shift? Eur. J. Risk Regulat. 11 , 337–342. doi: 10.1017/err.2020.29 [ CrossRef ] [ Google Scholar ]
- Gu X., Ying S., Zhang W., Tao Y. (2020). How do firms respond to COVID-19? First evidence from Suzhou, China . Emerg. Mark. Financ. Trade 56 , 2181–2197. doi: 10.1080/1540496X.2020.1789455 [ CrossRef ] [ Google Scholar ]
- Guedhami O., Knill A. M., Megginson W. L., Senbet L. W. (2021). The dark side of globalization: evidence from the impact of COVID-19 on multinational companies . SSRN Electron. J. doi: 10.2139/ssrn.3868449 [ PMC free article ] [ PubMed ] [ CrossRef ] [ Google Scholar ]
- Harjoto M. A., Rossi F., Lee R., Sergi B. S. (2021). How do equity markets react to COVID-19? Evidence from emerging and developed countries . J. Econ. Bus. 115 :105966. doi: 10.1016/J.JECONBUS.2020.105966, PMID: [ PMC free article ] [ PubMed ] [ CrossRef ] [ Google Scholar ]
- Harjoto M. A., Rossi F., Paglia J. K. (2020). COVID-19: stock market reactions to the shock and the stimulus . Appl. Econ. Lett. 28 , 795–801. doi: 10.1080/13504851.2020.1781767 [ CrossRef ] [ Google Scholar ]
- Hasan I., Politsidis P. N., Sharma Z. (2021). Global syndicated lending during the COVID-19 pandemic . J. Bank. Financ. 133 :106121. doi: 10.1016/J.JBANKFIN.2021.106121, PMID: [ PMC free article ] [ PubMed ] [ CrossRef ] [ Google Scholar ]
- Hu S., Zhang Y. (2021). COVID-19 pandemic and firm performance: cross-country evidence . Int. Rev. Econ. Financ. 74 , 365–372. doi: 10.1016/J.IREF.2021.03.016 [ CrossRef ] [ Google Scholar ]
- IMFC (2020). A crisis like no other, an uncertain recovery. World Economic Outlook Update, June 2020, 1–20. Available at: https://info.nicic.gov/ces/global/coronavirus-economy/crisis-no-other-uncertain-recovery
- Khlystova O., Kalyuzhnova Y., Belitski M. (2022). The impact of the COVID-19 pandemic on the creative industries: a literature review and future research agenda . J. Bus. Res. 139 , 1192–1210. doi: 10.1016/j.jbusres.2021.09.062, PMID: [ PMC free article ] [ PubMed ] [ CrossRef ] [ Google Scholar ]
- Klomp J., De Haan J. (2014). Bank regulation, the quality of institutions, and banking risk in emerging and developing countries: an empirical analysis . Emerg. Mark. Financ. Trade 50 , 19–40. doi: 10.1080/1540496X.2014.1013874 [ CrossRef ] [ Google Scholar ]
- Lee C. C., Hsieh M. F., Yang S. J. (2014). The relationship between revenue diversification and bank performance: do financial structures and financial reforms matterα . Jpn. World Econ. 29 , 18–35. doi: 10.1016/j.japwor.2013.11.002 [ CrossRef ] [ Google Scholar ]
- Levine R. (1997). Financial development and economic growth: views and agenda . J. Econ. Lit. 35 , 688–726. doi: 10.1596/1813-9450-1678 [ CrossRef ] [ Google Scholar ]
- Li L., Strahan P. E., Zhang S. (2020). Banks as lenders of first resort: evidence from the COVID-19 crisis . The Review of Corporate Finance Studies , 9 :472–500. doi: 10.1093/RCFS/CFAA009 [ CrossRef ] [ Google Scholar ]
- Liang Q., Xu P., Jiraporn P. (2013). Board characteristics and Chinese bank performance . J. Bank. Financ. 37 , 2953–2968. doi: 10.1016/j.jbankfin.2013.04.018 [ CrossRef ] [ Google Scholar ]
- Liu T., Pan B., Yin Z. (2020). Pandemic, Mobile payment, and household consumption: micro-evidence from China . Emerg. Mark. Financ. Trade 56 , 2378–2389. doi: 10.1080/1540496X.2020.1788539 [ CrossRef ] [ Google Scholar ]
- Mahi M., Phoong S. W., Ismail I., Isa C. R. (2020). Energy-finance-growth nexus in ASEAN-5 countries: an ARDL bounds test approach . Sustainability 12 , 1–16. doi: 10.3390/SU12010005 [ CrossRef ] [ Google Scholar ]
- Maliszewska M., Mattoo A., van der Mensbrugghe D. (2020). The potential impact of COVID-19 on GDP and trade: a preliminary assessment. In the potential impact of COVID-19 on GDP and trade: a preliminary assessment.
- Mensi W., Sensoy A., Vo X. V., Kang S. H. (2020). Impact of COVID-19 outbreak on asymmetric multifractality of gold and oil prices . Res. Policy 69 :101829. doi: 10.1016/j.resourpol.2020.101829, PMID: [ PMC free article ] [ PubMed ] [ CrossRef ] [ Google Scholar ]
- Narayan P. K. (2020). Oil Price news and COVID-19—is there any connection? Energy Res. Lett. 1 :13176. doi: 10.46557/001c.13176 [ CrossRef ] [ Google Scholar ]
- Njindan Iyke B. (2020). The disease Outbreak Channel of exchange rate return predictability: evidence from COVID-19 56 , 2277–2297. doi: 10.1080/1540496X.2020.1784718, [ CrossRef ] [ Google Scholar ]
- Padhan R., Prabheesh K. P. (2021). The economics of COVID-19 pandemic: a survey . Econom. Analy. Policy 70 , 220–237. doi: 10.1016/J.EAP.2021.02.012, PMID: [ PMC free article ] [ PubMed ] [ CrossRef ] [ Google Scholar ]
- Shabir M., Jiang P., Bakhsh S., Zhao Z. (2021). Economic policy uncertainty and bank stability: threshold effect of institutional quality and competition . Pac. Basin Financ. J. 68 :101610. doi: 10.1016/J.PACFIN.2021.101610 [ CrossRef ] [ Google Scholar ]
- Shen H., Fu M., Pan H., Yu Z., Chen Y. (2020). The impact of the COVID-19 pandemic on firm performance . Emerg. Mark. Financ. Trade 56 , 2213–2230. doi: 10.1080/1540496X.2020.1785863 [ CrossRef ] [ Google Scholar ]
- Simpson W. G., Kohers T. (2002). The link between corporate social and financial performance: evidence from the banking industry . J. Bus. Ethics 35 , 97–109. doi: 10.1023/A:1013082525900 [ CrossRef ] [ Google Scholar ]
- Škare M., Soriano D. R., Porada-Rochoń M. (2021). Impact of COVID-19 on the travel and tourism industry . Technol. Forecast. Soc. Chang. 163 :120469. doi: 10.1016/J.TECHFORE.2020.120469, PMID: [ PMC free article ] [ PubMed ] [ CrossRef ] [ Google Scholar ]
- Song H. J., Yeon J., Lee S. (2021). Impact of the COVID-19 pandemic: evidence from the U.S. restaurant industry . Int. J. Hosp. Manag. 92 :102702. doi: 10.1016/J.IJHM.2020.102702, PMID: [ PMC free article ] [ PubMed ] [ CrossRef ] [ Google Scholar ]
- Vidya C. T., Prabheesh K. P. (2020). Implications of COVID-19 pandemic on the global trade networks . Emerg. Mark. Financ. Trade 56 , 2408–2421. doi: 10.1080/1540496X.2020.1785426 [ CrossRef ] [ Google Scholar ]
- Vithessonthi C., Tongurai J. (2016). Financial markets development, business cycles, and bank risk in South America . Res. Int. Bus. Financ. 36 , 472–484. doi: 10.1016/j.ribaf.2015.10.012 [ CrossRef ] [ Google Scholar ]
- Wang W., Enilov M. (2020). The global impact of COVID-19 on financial markets . SSRN Electron. J. doi: 10.2139/ssrn.3588021 [ CrossRef ] [ Google Scholar ]
- Wellalage N. H., Kumar V., Hunjra A. I., Al-Faryan M. A. S. (2022). Environmental performance and firm financing during COVID-19 outbreaks: evidence from SMEs . Financ. Res. Lett. 47 :102568. doi: 10.1016/J.FRL.2021.102568, PMID: [ PMC free article ] [ PubMed ] [ CrossRef ] [ Google Scholar ]
- Wu J., Yao Y., Chen M., Jeon B. N. (2020). Economic uncertainty and bank risk: evidence from emerging economies . J. Int. Financ. Mark. Inst. Money 68 :101242. doi: 10.1016/j.intfin.2020.101242 [ CrossRef ] [ Google Scholar ]
- Xiong H., Wu Z., Hou F., Zhang J. (2020). Which firm-specific characteristics affect the market reaction of Chinese listed companies to the COVID-19 pandemic? 56 , 2231–2242. doi: 10.1080/1540496X.2020.1787151, [ CrossRef ] [ Google Scholar ]
- Yu Z., Xiao Y., Li Y. (2020). The response of the labor force participation rate to an epidemic: evidence from a cross-country analysis 56 , 2390–2407. doi: 10.1080/1540496X.2020.1787149, [ CrossRef ] [ Google Scholar ]
- Zaremba A., Kizys R., Aharon D. Y., Demir E. (2020). Infected markets: novel coronavirus, government interventions, and stock return volatility around the globe . Financ. Res. Lett. 35 :101597. doi: 10.1016/j.frl.2020.101597, PMID: [ PMC free article ] [ PubMed ] [ CrossRef ] [ Google Scholar ]
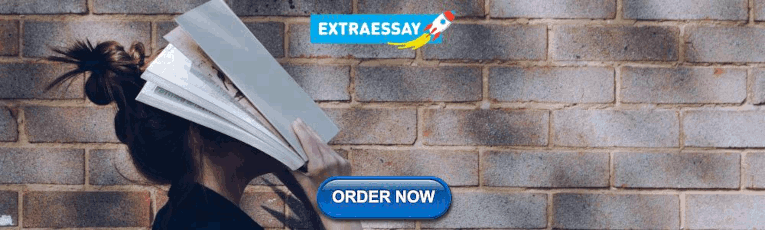
COMMENTS
Literature review. Corporate financial reporting refers to any deliberate release of financial information whether via informal or formal channels, voluntary or required, or in a qualitative or numerical form. ... However, few scholars have highlighted reporting timeliness in the financial sector (A. A. A. Ahmed, Citation 2010; Alali & Elder ...
This study presents a systematic literature review of regulation, profitability, and risk in the banking industry and explores the relationship between them. It proposes a policy initiative using a model that offers guidelines to establish the right mix among these variables. This is a systematic literature review study. Firstly, the necessary data are extracted using the relevant keywords ...
The study is based on the theory background and relevant researches in the areas of performance measures disclosed in financial statements. The sample of the case studies and sorts of literature are specifically collected from the well-known and respected accounting journals investigating in performance measures areas from 2010 to 2016, which are available on open access.
1. Introduction. The financial industry is, without a doubt, of great importance to society and in the daily lives of people worldwide. Although this sector has undergone major transformations over the centuries due to changes in political and geographic regimes and legislation, several authors (Berger, 2003, Mareev, 2016, Shim and Shin, 2016) state that a new era is being born for banks with ...
Literature review. The quest to ascertain a relationship between the financial sector and economic growth began as early as the 18th century. However, the modern empirical literature on the finance-growth nexus developed from the 1900s. ... Although it is widely accepted in the literature that the financial sector is important to the economy ...
Several themes are extracted from the studies that are reviewed, analyzed, and presented in this report. This review covered the themes that include investment, profit, competition, credit risk analysis, banking crime, and fintech. This report also signifies the importance, use of big data, and its function in the banking and financial sector.
The current study is primarily concerned with the developments in financial technology, or fintech, that have significantly altered traditional financial systems, focusing on several risk categories that have emerged in the financial technology sector's digital ecosystem. This paper is a review of existing literature related to the risk landscape in fintech, particularly its publication ...
Impact of Fintech on the Financial Stability of Banks: A Systematic Literature Review. Vol. VII, No. IV (Fall 2022) 35. (Kabir and W orthing, 2007; Albaity et al., 2021) also looked at how market ...
The demand for financial technologies has increased in the last few years. The most impacted sectors are the financial institutions, which are leading the trend. The study's focus was on reviewing the literature about financial technology especially the literature related to the banking sector. Most of the previous studies agreed that ...
We conduct a systematic literature review on environmental and climate-related risk management in the financial sector. We classify the current literature into three categories: (i) the impact of environmental concerns on financial risk; (ii) the current state of environmental risk practices in the financial sector; and (iii) measures to assess the financial exposure to environmental risks.
This paper reviews the theoretical and empirical literature on the role of financial sector development, with a view to deepening understanding of the rationale of development assistance to the financial sector of developing countries. The review leads to the following broad conclusions: (i) there are convincing arguments that financial sector ...
This study provides a holistic and systematic review of the literature on the utilization of articial intelligence (AI) in the banking sector since 2005. In this study, the authors examined 44 articles through a systematic literature review approach and conducted a thematic and content analysis on them. This review identies research themes ...
review the literature to recognize the applicable advantages, the challenges which the Fintech firms could possess for the conventional system of banking. Financial services have altered significantly over the last few generations, owing pri-marily to advances in technology in telecommunications, information and technology, and banking policies.
4.1 The bank fintech. We explore that there are three factors of the bank fintech as below: Pressure of the fintech company. We discuss that the primary factor of the bank fintech is the pressure ...
This article is an attempt to conduct a structured systematic literature review to identify factors impacting women's financial inclusion, the associated gender gap and importance of promoting greater financial inclusion for women. Literature reviews are important to map the existing landscape of a study problem and develop further knowledge.
This paper reviews the theoretical and empirical literature on the role of financial sector development, with a view to deepening understanding of the rationale of development assistance to the financial sector of developing countries. The review leads to the following broad conclusions: (i) there are convincing arguments that financial sector development plays a vital role in facilitating ...
The chatbot technology in the financial sector serves to examine customers' frequently asked questions and the representation of the process using machine learning. In light of this, this study presents a comprehensive systematic literature review of articles focused on text-based chatbots in the financial sector.
Literature review. The quest to ascertain a relationship between the financial sector and economic growth began as early as the 18th century. However, the modern empirical literature on the finance-growth nexus developed from the 1900s. ... Although it is widely accepted in the literature that the financial sector is important to the economy ...
In this paper, we provide a systematic review of the growing body of literature exploring the issues related to pervasive effects of cybersecurity risk on the financial system. As the cybersecurity risk has appeared as a significant threat to the financial sector, researchers and analysts are trying to understand this problem from different perspectives. There are plenty of documents providing ...
Financial literacy is a critical life skill that is essential for achieving financial security and individual well-being, economic growth and overall sustainable development. Based on the analysis of research on financial literacy, we aim to provide a balance sheet of current research and a starting point for future research with the focus on identifying significant predictors of financial ...
1 For a review of the research literature in this area, see Tobias Adrian, Daniel Covitz, and Nellie Liang (2015), "Finan- ... Excessive leverage within the financial sector increases the risk that financial institutions will not have the ability to absorb losses without disruptions to their normal business operations
The section "Literature review" provides an overview of the relevant literature. The section "Data and methodology" defines our sample, study variables, econometric model, and summary statistics. ... (ROAE). These are the banking sector's most accepted financial performance measures (Simpson and Kohers, 2002). Moreover, we also used ...
customer requirements. The chatbot technology in the financial sector serves to examine customers' frequently asked questions and the representation of the process using machine learning. In light of this, this study presents a comprehensive systematic literature review of articles focused on text-based chatbots in the financial sector.