- 33 Online Shopping Questionnaire + [Template Examples]

Online shopping is increasingly becoming the most preferred shopping option across the globe. In 2019 alone, an estimated 1.92 billion people purchased goods or services online. This has created a need to offer good customer service to online shoppers, which is why you need an online shopping questionnaire.
An online shopping questionnaire helps you to study users’ behaviors, experiences, and preferences as they shop items from your e-commerce store. In this article, we will discuss 33 questions you should include in your online shopping questionnaire to help you understand your customers’ needs.
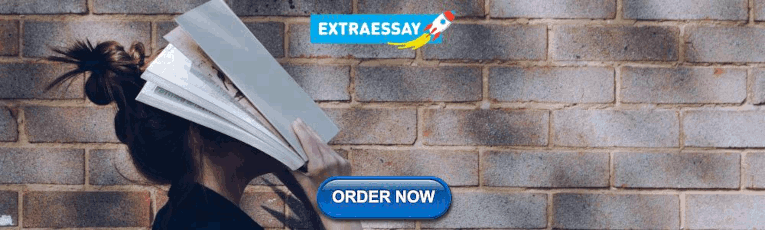
Online Shopping Survey Questions
The types of questions listed in your online shopping survey must reflect the aims and objectives of the data collection process. Also, be sure to ask good survey questions that allow respondents to freely communicate their thoughts and perceptions without boxing them into a corner.
Close-ended questions, open-ended questions , and dichotomous questions are examples of good survey questions that you can add to your online shopping survey.
Close-Ended Questions
A close-ended question is a type of survey question that restricts respondents to a set of answer-options to choose from. In other words, the researcher provides options for you to choose from in response to the question. Close-ended questions help you to gather quantitative data .
11 Close-ended Questions for an Online Shopping Questionnaire
How often do you shop on this site, choose 2 products you buy frequently on this site..
- Accessories
- Perfumes and Oils
- Skincare products
What is the biggest challenge you face with shopping online?
- Slow checkout time
- Lack of products I want
- Slow webpage response time
How likely are you to recommend this site to other online shoppers?
- Very likely
- Somewhat likely
- Very unlikely
What is your biggest concern about online shopping?
- Breach of personal information
- Breach of payment details
- Poor internet connection
How much do you spend on online shopping every month?
- Less than 100 USD
- $100 – $500
- $500 – $1000
- More than 1000 USD
How many times do you shop online in a month?
- More than thrice
Which payment method do you prefer for online shopping?
- Payment Gateways
- Bank transfer
How would you rate your overall online shopping experience?
How likely are you to return to this webpage for your online shopping.
- Highly unlikely
What is your gender?
- Others. Please state
- Prefer not to say
Open-Ended Questions
This is a type of survey question that does not limit respondents to predetermined answers. Open-ended questions allow you to fully communicate your ideas and perceptions in response to a question. You can describe them as free-form survey questions.
Examples of Open-Ended Questions in an Online Shopping Questionnaire
- Describe your online shopping experience with us . This question allows your customer to provide a holistic view of their overall customer experience with your organization.
- Describe a negative experience you had while shopping online . This question allows customers to highlight any areas needing improvement in your online store.
- Describe a positive experience you had while shopping . Let customers identify strong points when it comes to online shopping with your brand.
- Why do you shop online with us? With this question, you would be able to identify the unique selling points of your brand across different customer segments.
- How old are you ? This question helps you to understand who your customers are; that is, the different age groups that your brand appeals to.
- Which products do you buy regularly? The responses to this question will help you to identify fast-moving products and to categorize your stock accordingly.
- Have you experienced any difficulty with adding products to your online cart? This question allows respondents to provide specific feedback on definite aspects of your online shopping operations.
- What do you think about the pricing of our products ? Use this question to collect feedback on product pricing to avoid overcharging or under-charging your customers.
- What do you think about the quality of our products? This question allows you to collect first-hand feedback from customers in terms of the quality of your product(s).
- What other online store do you shop on? The answers to this question make it easy for you to identify your competition. You can leverage this data to create a better customer experience for your clients.
- What major challenges have you encountered while shopping on our site ? This question allows you to identify and address customer dissatisfaction easily. After identifying the challenges faced, you should work on providing sustainable solutions to them.
Dichotomous Question
A dichotomous question is a type of close-ended survey question that provides respondents with 2 opposite answer options for them to choose from. Common answer options in dichotomous questions include true/false, yes/no, fair/unfair, to mention a few.
Dichotomous Question Samples for an Online Shopping Questionnaire
Did you enjoy the online shopping experience on our website.
This question allows customers to provide feedback on the overall shopping experience.
Do you always shop on our website?
This simple question allows you to track consumer retention for your organization.
Would you recommend our website to others?
Positive responses to this question serve as an indicator of a good customer experience.
Our website provided the best online shopping experience.
Just like the first question in this section, this question helps you to gather feedback on your overall online shopping experience.
Do you have any challenges with our checkout method?
Get direct feedback from customers about your e-commerce checkout process on your website.
Our product prices are affordable.
This question allows you to gather feedback from customers about product pricing.
Have you ever had a bad experience while shopping with us?
This question allows you to track and address customer dissatisfaction.
Do you have any concerns about your data privacy while shopping online?
I always use the credit card option for my online shopping transactions..
This question prompts customers to indicate preferred payment options.
I always use the bank transfer method for online shopping transactions.
Just like the question above, customers can provide responses here that allow you to identify preferred payment methods for your e-commerce store.
Do you always shop online?
Responses to this question provide insight into customers’ behaviors and preferences.
Can’t find your preferred Online Shopping survey template? ithCreate yours for free with the easy-to-use Formplus builder
How to Create an Online Shopping Questionnaire with Formplus
With Formplus, you can create a smart online shopping questionnaire and either add the form to your website or share it with your customers using our multiple form sharing options. Formplus makes it easy for you to collect and process data from your customers, and this helps to improve customer experience and consumer satisfaction for your organization.
Follow these steps to create your online shopping questionnaire from scratch using Formplus.
- If you do not have a Formplus account, visit www.formpl.us to sign up for your Formplus account. If you have a Formplus account, visit the aforementioned website and click on the “Access Dashboard” button to gain access to your personalized Formplus dashboard.
- Once you have access to your Formplus dashboard, click on the “create new form” button to start building your online shopping survey. You’d find this button at the top left corner of your dashboard.
- Alternatively, you can modify any of the existing Formplus templates to suit your data collection needs. All you need to do is click on the “template” option on the dashboard navigation bar and then, follow the prompt.
- Now, you should be in the form builder. This is where you create your online shopping form. Start by adding the form title to the builder’s title bar.
- Next, go to the form fields section located on the left side of the form builder. There, you’d find more than 30 form field options including digital signature fields, payment fields, date-time validation, and so on. You can add any of these fields to your form by simply clicking on them or drag and drop the field from the builder’s inputs section.
- After adding the fields, click on the pencil icon just beside each field to access the form fields editing section. Here, you should add your question(s) and/or options.
- When you’ve added and modified all preferred fields accordingly, click on the save icon just at the top right corner of the builder. This automatically saves the form and takes you to the builder’s section.
- The Customize section is where you can change the look and feel of your online shopping survey. You can add preferred background images to your form, embed your organization’s logo, change the form font, or even customize the form layout using CSS.
- To add the online shopping survey to your website simply, go to the form builder’s “Share” page. You’d find it on the builder’s navigation panel right at the top corner.
- Click on the “embed” tab on the sidebar.
- You’d see 4 options here: Use as Pop-up, Use as iFrame embed, Embed in Facebook Page, and Embed in WordPress site.
- Click on “use as iFrame embed” and copy the displayed code.
- Paste the code at the appropriate place to add it to your site.
- If you have a WordPress website, you can embed the form by choosing the “Embed in WordPress site” option, copy the shortcode, and paste it inside your WordPress editor.
- Copy the form link and share it with respondents.
Importance of an Online Shopping Survey
E-commerce businesses, especially, should prioritize online shopping surveys because these data collection tools are key to business optimization, improved customer experience, brand loyalty, and increasing revenue. Here are 6 ways that online shopping surveys can make a difference in your business.
- Understand Consumer Behaviour: With an online shopping survey, you’d have a better understanding of your customers’ online shopping behaviors with specific insights into their preferences, challenges, and experiences. This allows you to place them into distinct customer segments as part of market research.
- Seamless Data Collection: An online shopping survey is a fast, easy, and convenient method of data collection . Unlike paper forms and other traditional survey methods, an online shopping survey can be filled on the go which allows you to gather real-time information from respondents, instantly.
- With a smart online survey, you’d find it easier to highlight current trends and patterns in consumers’ behaviors.
- Improved Customer Experience and Satisfaction: It helps you to immediately identify and address any challenges faced by your customers and to resolve these challenges accordingly. If you embed the survey into your e-commerce website, customers complete the questionnaire once they are done shopping on your webpage.
- Optimized Marketing Plans and Strategies: The data gathered via an online shopping survey can help you create a well-defined marketing plan and strategy for your organization. Having a clear knowledge of who your customers are and what different customer segments prefer typically empowers you to create specifically tailored adverts that appeal to each segment.
- It improves your organization’s response time to customers’ complaints.
Research shows that consumers spend an average of 5 hours shopping online every week and 92% of consumers shop online at least once a year . This, once again, emphasizes how much online shopping has become integral to our everyday lives.
If you want to create unforgettable online shopping experiences for your target audience, you must understand customers’ experiences and expectations. An online shopping questionnaire is a simple but effective data tool that helps you to gather objective data from consumers.
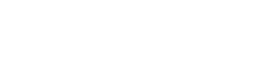
Connect to Formplus, Get Started Now - It's Free!
- compatibility questionnaires
- online questionnaire sample
- online shopping
- online store
- online surveys
- busayo.longe
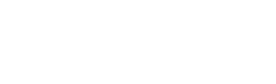
You may also like:
Pilot Testing: Meaning, Importance + [Question Example]
This article will discuss what a pilot test is, best practices for implementing pilot tests and how Formplus can help create stellar...

Highest-Paying Survey Sites on the Web
In this post, we will highlight how the paid survey companies operate and we will also list out sites with legit paid surveys.
Survey or Questionnaire? 15 Differences & Similarities
Guide on differences between survey and questionnaires. + Survey Questionnaire tips, tools, advantages template and examples
4 Types of Questionnaire + Free Question Examples
For many years, questionnaires have been a popular data collection tool during research. Questionnaires are very diverse and
Formplus - For Seamless Data Collection
Collect data the right way with a versatile data collection tool. try formplus and transform your work productivity today..
Quantitative market research questions to ask for actionable insights
Types of quantitative market research questions, 36 quantitative research questions and examples, how to write your own quantitative market research questions, how to collect insightful data from your quantitative surveys, receive quantitative insights in weeks, not months.
There’s a big difference between asking “Why do you like our product?” and “On a scale of 1-10, how much do you like our product?” But both ways of asking are valuable in their own way.
Knowing your audience is not about guesswork or intuition, it is about concrete data. And while it’s valuable to learn the ‘why’ behind the ‘what’ with qualitative research, quantitative research is just as necessary — to spot trends, patterns and more.
Unlike qualitative research, which explores attitudes, opinions, and motivations through open-ended questions, quantitative research zeroes in on the numbers (see what we did there?). It’s the difference between gathering general opinions and collecting measurable, specific data.
But when is this approach the way to go? For starters, whenever you need to track factors over time, such as customer satisfaction. Or when assessing the popularity of a potential product feature, understanding demographic preferences, or analyzing consumer purchasing behavior in different locations.
Quantitative research reveals the impact and scale of sentiments for better decision-making. It’s also valuable when you’re looking to quantify the extent of a trend, measure the impact of a marketing campaign, or pin down the specifics of consumer behavior.
But how do you ask quantitative market research questions that don’t just scratch the surface? We’re here to give you some great examples of quantitative survey questions.
In the US? Check out these research platforms
Here are the top market research platforms in the US for reliable insights – check them out and start getting your insights today!
When thinking of quantitative market research questions, people often think ‘ ah, numbers ‘. But there’s more than meets the eye. Here’s how you can categorize the different types of quantitative research questions:
Descriptive quantitative research questions
These are your what , when , and how many types of questions. They help you sketch out the basic landscape of your market. For example, “How often do you shop online in a month?” or “What is your preferred method of payment while shopping online?” When you give answers people can select, it is quantifiable data. That’s different from asking: ”describe what a day out shopping looks like for you”, which is a qualitative question.
Comparative quantitative survey questions
These questions measure differences or changes over time or between groups. For instance, “How has your spending on online shopping changed since last year?” Comparative questions help you understand the dynamics and shifts in your market. Remember that you’re not just trying to find overlap: it’s just as important to know what differences there are.
Relationship-based quantitative survey questions
These questions aim to uncover correlations or relationships between two or more variables. They can reveal insights like, “Is there a link between age and the likelihood of using mobile payments?” These questions help you understand the deeper connections within your market, as well as test assumptions, as long as you dare to ask questions that challenge what you’re hoping to find.
Now, a quick note on reducing bias in quantitative survey questions . Here are some key points to remember:
- The key is in how you frame your questions.
- Always aim for neutrality.
- Avoid leading questions that suggest a particular answer.
- Be specific and clear to avoid confusion.
- Consider the order of your questions, as earlier questions can influence responses to later ones.
And finally, test your survey with a small group before a full rollout, to catch and correct any unintentional bias. This way, you ensure the data you collect is as accurate and reliable as possible, giving you the best insights to make those crucial business decisions.
If you want to make a quantitative survey that hits the spot, don’t just ask generic questions. We’re here with some examples that you can adapt to make your research a success.
Descriptive market research questions
With a descriptive quantitative research question, you can quickly get the most important info for your respondents on anything ranging from buying frequency to satisfaction levels.
- Insight : this question reveals the frequency of use, indicating customer dependency on your product or service.
- Benefit : understanding usage patterns can guide inventory management and marketing strategies.
- Insight : reveals the communication channels most favored by your audience.
- Benefit : tailor your customer service and marketing outreach to your customers’ preferred channels.
- Insight : provides an average spending figure for budget allocation in that category.
- Benefit : helps in pricing strategies and identifying the most lucrative customer segments.
- Insight : uncovers patterns in online shopping behavior.
- Benefit : optimizes the timing of online marketing campaigns and promotions.
- Insight : identifies the most effective channels for brand discovery.
- Benefit : informs where to allocate advertising spend for maximum impact.
- Insight : measures the likelihood (not effectiveness!) of word-of-mouth referrals.
- Benefit : assesses customer satisfaction and the potential for organic growth.
- Insight : highlights your unique selling points from the customer’s perspective.
- Benefit : guides messaging to emphasize what customers value most about your brand.
- Insight : offers a quantifiable measure of customer service satisfaction.
- Benefit : identifies areas for improvement in customer support.
- Insight : sheds light on the most popular aspects of your product.
- Benefit : informs product development and feature enhancement.
- Insight : uncovers the key motivators behind purchasing decisions.
- Benefit : helps create targeted marketing campaigns to focus on these driving factors.
Comparative market research questions
If you want to analyze and compare different variables, these questions can help.
- Insight : highlights changes in consumer spending habits over time.
- Benefit : useful for identifying trends and shifts in consumer behavior, aiding in long-term planning. Especially valuable if you add qualitative insights to this quantitative data.
- Insight : compares consumer preferences between different shopping channels.
- Benefit : guides omnichannel marketing strategies and resource allocation.
- Insight : tracks changing consumer values and preferences over time.
- Benefit : useful for aligning product development and marketing with evolving consumer values.
- Insight : compares the weight of price versus brand in purchasing decisions.
- Benefit : informs pricing strategies and brand positioning efforts.
- Insight : evaluates customer perception of marketing efforts in product packaging.
- Benefit : assesses the impact of packaging on brand image and customer approval.
What are the top research platforms in the UK?
Here’s our list of the pros and cons of key market research platforms for UK brands
Relationship-based questions for quantitative research
In quantitative research, especially when exploring relationship-based aspects, the key is not to cram multiple inquiries into one question but to ask them sequentially.
This approach allows for a clearer and more focused response to each individual question. Later, during the analysis phase, you can then correlate the responses to uncover relationships between different variables.
For instance, instead of asking, “How often do you use our product and how satisfied are you with it?”, split this into two separate questions:
- “How often do you use our product (daily, weekly, monthly)?”
- “On a scale of 1-10, how satisfied are you with our product?”
By asking these questions separately, you ensure that respondents clearly focus on each aspect without being overwhelmed or confused by a dual-focused question. This approach yields more accurate and reliable data.
After the survey, you can analyze the results to see if there’s a correlation between usage frequency and satisfaction levels.
Here are some examples of combinations that can work well:
- What is your age group?
- Insight : correlates age with shopping preferences.
- Benefit : you can tailor marketing and sales strategies to different age demographics based on their preferred shopping channels.
- How long have you been using our products/services?
- Insight : links customer tenure with brand loyalty.
- Benefit : assesses the impact of long-term use on loyalty, informing customer retention initiatives.
- What is your approximate annual income?
- Insight : examines the relationship between income levels and purchasing behavior for premium products.
- Benefit : guides product and pricing strategies targeting different income segments.
- How often do you use social media for product discovery?
- Insight : assesses if frequent social media use for product discovery actually influences online shopping behavior.
- Benefit : informs the effectiveness of social media marketing in driving online sales in your target market.
- How would you rate your satisfaction with our post-purchase customer service (scale of 0-10)?
- Insight : links the level of service post-purchase with the likelihood of repeat purchases.
- Benefit : identifies if customer service is negatively or positively affecting repeat custom rates.
Brand tracking questions for quantitative insights
One thing you should definitely gather numerical data on, is your brand’s health. Just like your own health, stats, and numbers matter and can show you where to further investigate to ask qualitative research questions about. Learn if your brand stands strong through market trends and gain insights on whether your brand is growing in terms of awareness — and in which segments.
- Insight : measures brand awareness among the target audience.
- Benefit : helps assess the effectiveness of your marketing and branding efforts.
- Insight : evaluates brand loyalty and the potential for organic growth through word-of-mouth.
- Benefit : indicates customer satisfaction and the potential for brand advocacy.
- Insight: Identifies the most effective channels for brand discovery.
- Benefit: Informs where to focus marketing efforts for increased brand exposure.
- Insight: Measures brand visibility and frequency of encounters with the brand.
- Benefit: Helps evaluate the reach and frequency of marketing campaigns.
- Insight: Determines which brand values resonate most with the audience.
- Benefit: Aids in refining brand messaging and aligning it with customer values.
Quantitative consumer segmentation questions
Quantitative questions about customer segments can go beyond age group and gender. King Charles III is the same age as Ozzy Osbourne – would you say they’re very similar?
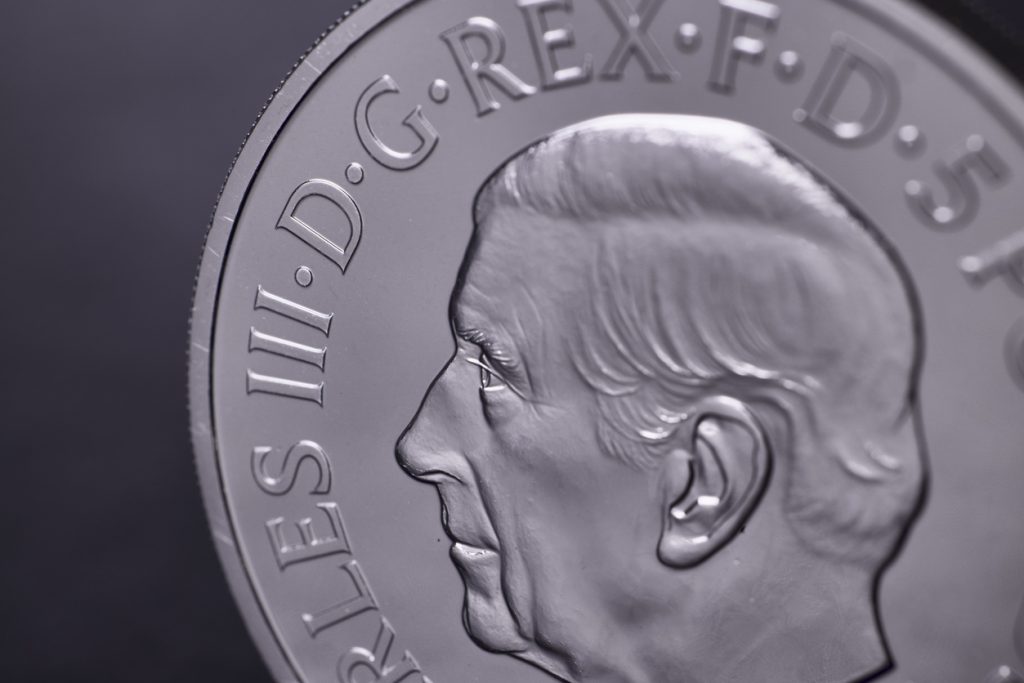
It is vital that you look at more variables so you can really tell the difference between your respondents, and make informed decisions based on the whole truth. Putting these consumer profiling questions and answers in specific ranges helps you create segments to tailor your marketing and customer experience for, rather than just aiming at the entire population.
- Insight : helps understand the economic demographics of your customers.
- Benefit : assists in pricing strategies and identifying which income groups are most engaged with your brand.
- Insight : reveals geographical spread and regional preferences.
- Benefit : guides regional marketing efforts and product distribution strategies.
- Insight : helps categorize customers by education level.
- Benefit : useful for tailoring communication and content complexity to different education backgrounds.
- Insight : provides insights into the professional background of your customers.
- Benefit : helps in creating industry-specific marketing campaigns and products.
- Insight : gives an idea of household size and composition.
- Benefit : useful for targeting products and services aimed at families or individuals.
- Insight : identifies customers who are parents of minors (which is different from parents of young adults, or even grown adults).
- Benefit : informs product and marketing strategies aimed at families with children.
Okay, so now you got the gist of it and have seen what quantitative questions can look like — as they come in all shapes and sizes. But they might feel too generic for your research, or you’re looking for something specific.
Here’s how you can whip up your own quantitative questions that deliver the insights you need for data-driven decisions.
Identify the key variables you need to measure
Start by pinpointing exactly what you want to know. Is it customer satisfaction, buying behavior, or brand awareness? Determining the specific variables you need to measure sets the foundation for your entire survey.
Choose the right survey distribution method
Think about how your questions will reach your audience. Will it be online through email or social media, over the phone, or in person? Your method should align with where your target audience is most active and responsive.
Make sure your questions are crystal-clear and unequivocally unbiased
We’ve mentioned it earlier, and we’ll do it again if we have to. The way you phrase your questions can make or break your survey. Aim for clarity and simplicity – questions should be easy to understand and answer. Avoid leading or loaded questions that might sway a respondent’s answer. Remember: it’s a survey, not a sales pitch.
Know where to ask for more detailed information and qualitative data
Quantitative market research questions only tell part of the story. If you see interesting trends in say purchase behavior or price sensitivity, or a particular product gets a bad rating, dig a little deeper. Follow up important questions with qualitative research questions to analyze what’s going on behind the numbers.
If you don’t want to end up with a pile of quantitative data that doesn’t do much for you or breaks the bank unnecessarily, it’s vital you choose a form of distributing the survey that makes sense. You can work with UK market research companies to outsource it all, or do it yourself. Here’s a brief look at the pros and cons of popular methods:
Telephone surveys:
- Pros : good for less tech-savvy demographics.
- Cons : time-consuming, potentially costly, and declining response rates. They might be better for qualitative research.
In-person surveys:
- Pros : also avoids any confusion with tech.
- Cons : logistically demanding and expensive, not suited for quick data collection.
Online survey software:
- Pros : cost-effective, broad reach, real-time data analysis, and versatile formats.
- Cons : it’s extra important to pay close attention to survey design, so people don’t get the urge to give false answers just to get to the end.
The choice is yours, but generally, quantitative research thrives when done with online surveys and it’s the go-to method for most international market research . And here at Attest, we help you get even more out of it by giving you a chock-full toolkit. From various types of questions to robust analytical tools (and a dedicated research expert for when you need a little extra help) — we set you up for measurable success.
Speed and accuracy in market research matter — but we don’t want you to sacrifice quality. With Attest, you get fast, actionable and high-quality insights.
Which market analysis tool is right for you?
Check our rundown of the top platforms for market analysis – and start making better decisions with reliable insights in no time!
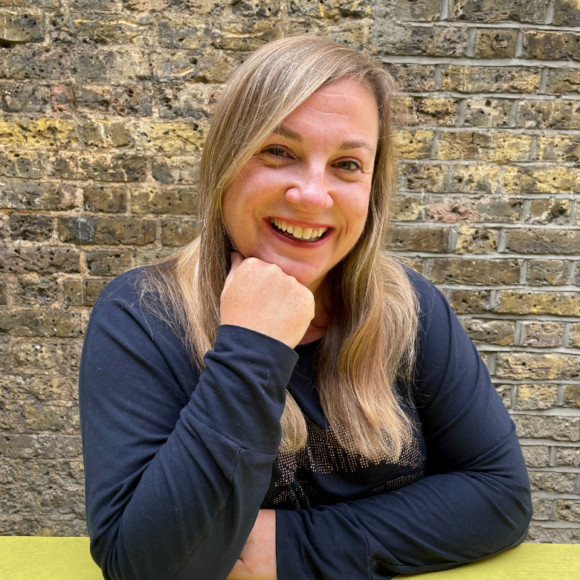
VP Customer Success
Sam joined Attest in 2019 and leads the Customer Research Team. Sam and her team support brands through their market research journey, helping them carry out effective research and uncover insights to unlock new areas for growth.
Related articles
A guide to brand tracking: what it is & how to boost your brand roi, brand tracking, how to gather consumer insights (and use them to improve your business), 12 brand management software, platforms and tools to understand customer perception in 2024, subscribe to our newsletter.
Fill in your email and we’ll drop fresh insights and events info into your inbox each week.
* I agree to receive communications from Attest. Privacy Policy .
You're now subscribed to our mailing list to receive exciting news, reports, and other updates!
Determinants of consumer's online shopping intention during COVID-19
Journal of Electronic Business & Digital Economics
ISSN : 2754-4214
Article publication date: 13 June 2023
Issue publication date: 26 July 2023
This paper aims to determine the factors that influence the consumer’s online shopping intention in the current pandemic context (COVID-19). For this purpose, a conceptual model has been developed by introducing the constructs “attitude,” “perceived utility,” “intention” as well as the variable “perceived risk of contagion.”
Design/methodology/approach
After collecting data from the questionnaire diffused in Moroccan e-commerce websites, this study used various statistical analyses with the multiple regression model on the SPSS statistical software to confirm or refute the research hypotheses.
The results indicate that attitude and perceived utility positively affect online shopping intention. However, the variable “perceived risk of contagion” has a weak effect on such intention, which can be explained by the period in which the survey was started (a few months after the confinement).
Originality/value
The scientific contribution of this study lies in the insertion of a new factor that will be called “perceived risk of contagion” in the research model. This factor has been inspired by the perceived risk theory of Bauer (1960). Furthermore, all studies dealing with this topic have been carried out in developed countries, such as France, Great Britain, Germany and the USA. For this reason, the researcher believe that it is more appropriate to study the intention to buy online during the COVID-19 pandemic in one of the developing countries, such as Morocco. This is based on the fact that to develop theories, it is necessary to examine a given problem in several countries. The context plays a determining role in such situations.
- Online shopping
- Behavioral intention
El Moussaoui, A.E. and Benbba, B. (2023), "Determinants of consumer's online shopping intention during COVID-19", Journal of Electronic Business & Digital Economics , Vol. 2 No. 1, pp. 69-88. https://doi.org/10.1108/JEBDE-01-2023-0002
Emerald Publishing Limited
Copyright © 2023, Alaa Eddine El Moussaoui and Brahim Benbba
Published in Journal of Electronic Business & Digital Economics . Published by Emerald Publishing Limited. This article is published under the Creative Commons Attribution (CC BY 4.0) licence. Anyone may reproduce, distribute, translate and create derivative works of this article (for both commercial and non-commercial purposes), subject to full attribution to the original publication and authors. The full terms of this licence may be seen at http://creativecommons.org/licences/by/4.0/legalcode
1. Introduction
COVID-19 is a contagious disease caused by a virus called coronavirus, which has affected many countries around the world. This pandemic has infected people in 185 countries. As more and more cities are locked down, many businesses are forced to close. Furthermore, the COVID-19 pandemic has led consumers to avoid meeting others and, consequently, there was an increase in the rate of non-contact consumption among both young and old people, who rarely use laptops. They are avoiding crowds and leaving their homes less often due to this crisis. Reducing the purchases needed is becoming a new norm. With the closure of many physical stores, the e-commerce strategy has gained importance during this period ( Sahli, 2020 ).
The COVID-19 pandemic has dramatically changed consumer habits. Although pandemic crises are not common, a single pandemic wave can cause disastrous and unexpected consequences. The majority of studies dealing with diseases and epidemics are medical in nature. Thus, there is little research conducted from a social science perspective. These studies have in turn been carried out in developed countries, such as France, Great Britain, Germany and the USA ( Moon, Choe, & Song, 2021 ). For this reason, we believe that it is more appropriate to study the intention to buy online in the context of the pandemic in one of the developing countries, such as Morocco, so as to complete the theoretical inventory related to this topic, and consequently to give the possibility to other researchers to compare these studies while taking into account the culture, economy and technology. In fact, Ajzen (1991) and Triandis (1979) have conducted some studies on the same phenomenon (adoption of technology). These researchers, who have focused on behavioral intentions, stated that by understanding the determinants of online purchasing, we will be able to develop some decision elements for marketers. The development of our model, which was conducted during the epidemic period (COVID-19), will help businesses willing to enter the online business sector to better understand the behavior of potential customers.
In this article, we intend to answer the following question: what are the factors that have favored the adoption of online shopping by consumers since the announcement of the health alert? To this end, we have proposed a model showing the determinants of the intention to buy online during the period of the health crisis (COVID-19), focusing on the various theoretical models of technology acceptance, which are related to the marketing field and specifically to the consumer behavior area. Thus, our scientific contribution lies in the insertion of a new factor that we will call “perceived risk of contagion” in our research model. This factor has been inspired by the perceived risk theory of Bauer (1960) . Furthermore, all studies dealing with this topic have been carried out in developed countries, such as France, Great Britain, Germany and the USA. For this reason, we believe that it is more appropriate to study the intention to buy online during the COVID-19 pandemic in one of the developing countries, such as Morocco. This is based on the fact that to develop theories, it is necessary to examine a given problem in several countries. The context plays a determining role in such situations.
Our article is structured as follows: first, in Section 2 , we present a related work section that describes previous research. Then, in Section 3 , we present the research methodology used in our study. Next, in Sections 4 and 5 , we discuss the results obtained. In Section 6 , we summarize our study and suggest future research directions.
2. Literature review and hypotheses development
2.1 theories explaining the consumer’s online shopping behavior.
TRA: The precursors of the theory of reasoned action, Ajzen and Fishbein (1975) , have proposed a model that aims to explain and understand the behavior of individuals in various situations. This theory considers that each behavior is directly determined by the individual's intention to emit this behavior. In turn, behavioral intention is affected by two variables: the attitude toward the behavior and the subjective norm. As justified in Ajzen and Fishbein's (1975) work, attitude is determined by behavioral beliefs (beliefs about the probability of consequences) and evaluations of the quality or severity of those consequences. The second determinant of behavioral intention, which is the subjective norm, depends on beliefs about what people think they should do. Indeed, Sutton's (1998) meta-analysis confirms that the TRA has good validity, with the exception of being under-controlled only by intentional behavior, and lacking situational factors. This has led several researchers to test other ways of improving this theory. For these reasons, Ajzen (1991) was motivated to revise the theory of reasoned action while proposing the theory derived from the TRA which is the TPB.
TPB: To improve the TRA, Ajzen (1991) has developed a new theory derived from the TRA, which is the TPB. In this context, an additional determinant has been added: perceived behavioral control ( Ajzen, 1991 ; Emin, 2004 ). According to Ajzen's (1991) theory of planned behavior, any behavior that requires some reflection and/or planning, such as the realization of an online purchase, is affected directly by intention; the latter is determined by three variables: the attitude toward the behavior; the subjective norm; and the perceived behavioral control. The two first variables are previously defined. The third corresponds to the factors that facilitate or inhibit the execution of a given behavior ( Ajzen, 1991 ). In the context of our study, Moon et al. (2021 ) showed that when the consumer adopts a negative attitude toward COVID-19 and strongly perceives the subjective norm, he will choose online shopping channels over traditional ones. In addition, they indicated that the consumer would opt for online shopping channels when perceived behavioral control is significantly high; and vice versa.
TAM: In 1985, Fred Davis suggested a conceptual framework under the name of the TAM. He stated that the actual use of the system constitutes a response likely to be explained or predicted by the user's motivation. Davis, Bagozzi, and Warshaw (1989) showed that the latter can be explained by three factors: perceived ease of use, perceived usefulness and attitude. Davis suggested that the user's attitude constitutes a key factor in determining the user's use or rejection of the system. This attitude, in turn, is influenced by two major beliefs, namely, perceived usefulness and ease of use. Davis et al . (1989) defined the first belief as the level on which the person believes that the use of a particular system would improve their professional performance. Regarding the second belief, as the name suggests, it implies that the person uses a system without any effort ( Sharp, 2006 ). According to Marangunić and Granić (2015) , both beliefs are positively influenced by the design features of the system. Over time, Davis et al . (1989) found that attitude does not directly influence perceived usefulness and perceived ease of use. Based on these complementary results, a parsimonious TAM was suggested. The new model has incorporated behavioral intention as a new variable, which directly influences the perceived usefulness of the system ( Davis et al. , 1989 ). The other change introduced to the original TAM was the inclusion of certain factors called external variables.
IDT: Innovation diffusion theory was introduced in 1962 by Rogers. Its principal aim is to understand why, how and at what rate technologies spread through a social system ( Rogers, 1995 ). According to Al-Rahmi et al. (2019 ), an innovation that brings benefits, low complexity, perceived compatibility with existing beliefs and potential trialability, is likely to be diffused quickly and widely. Innovation diffusion theory adopts an opposite logic to that of behavioral change studies. It sees change primarily as a question of evolving or “reinventing” products and behaviors so that they better meet the individual's and group's requirements ( Wani & Ali, 2015 ). In this approach, it is not the people who change, but the innovations themselves ( Robinson, 2009 ). The innovation diffusion theory can be divided into four main elements, namely: innovation, time, communication system and social system.
CVM: Daily consumption is highly dependent on price-quality ratio, convenience, health concerns, habit and the individual's responses to institutional and social norms ( Biswas, 2017 ). According to Thye Goh, Mohd Suki, and Fam (2014 ), the model of consumption values is based on three major principles: (1) The benefits of each consumption value vary significantly from one situation to another; (2) consumption behavior depends on several consumption values; and (3) all consumption values are fully independent of each other. In fact, CVM takes into account various components of consumption values, such as attractiveness, price, emotions, quality and environmental impact ( Hur, Yoo, & Chung, 2012 ; Phau, Quintal, & Shanka, 2014 ). Some researchers have combined these components into just three factors, such as functional value, psychological value and environmental value ( Biswas & Roy, 2015 ; Biswas, 2017 ).
2.2 Online shopping intention
Today, online shopping has significantly increased the level of competition in the online market ( Akroush & Al-Debei, 2015 ) and changed consumer habits ( Kühn & Petzer, 2018 ; El Moussaoui, Benbba, & El Andaloussi, 2022 ; El Moussaoui, Benbba, & El Andaloussi, 2022 , El Moussaoui, El Moussaoui, Benbba, Jaegler, & El Andaloussi, 2022 ). Indeed, several research studies have concentrated on consumers' online shopping intentions in different contexts. Table 1 summarizes these studies (conducted over the last 5 years), by identifying the main factors influencing online purchase intention, the methodology used including sample size and the theory or model used to explain such intention.
Attitude: a major component of the individual's voluntary behavior was introduced for the first time in the TRA. It is a key variable in understanding decision-making ( Akar & Dalgic, 2018 ). It corresponds mainly to the feelings of pleasure, joy, satisfaction or dissatisfaction that the consumer relates to a given behavior. The literature has shown that there is a positive link between attitude and customers' online purchase intentions ( Singh & Srivastava, 2018 ; Yang, Li, & Zhang, 2018 ; Ha & Nguyen, 2019 ; Rehman, Bhatti, Mohamed, & Ayoup, 2019 ). Indeed, when consumers favor online shopping, they tend to adopt this behavior. According to Karahanna, Straub, & Chervany ( 1999 ), attitude determines the intention to use information technology. Thus, a favorable attitude to Internet usage tends to generate a positive intention to purchase online. Simultaneously, the literature ( Sabik, 2014 ; Ezzahi & Jazi, 2018 ; Bourchich & Nejjar, 2021 ) confirmed that the attitude toward Internet usage positively affects the intention to buy products online. In terms of the context of this study, consumers around the world have all experienced a shift in their shopping behaviors due to health concerns, anxiety and confinement ( Ozturk, 2020 ). Consumers' attitudes toward online shopping had an impact on their intentions to purchase products and services during the pandemic. According to a study conducted in Morocco on the basis of 114 respondents, Hajraoui and Chalabi (2021 ) found that only 4% of consumers surveyed adopted online shopping during the COVID-19 health crisis, against 96% who still prefer to make their purchases in physical stores (supermarkets, souk municipal, retail stores…), this explains that the Moroccan consumers have a low orientation toward the electronic commerce. This is what we seek to confirm through our study while supporting the idea that attitude affects behavioral intention during a health crisis. Hypothesis H1 is developed as follows:
There is a positive and significant relationship between the attitude (favorable, unfavorable) toward online shopping and the intention to buy online during the health crisis.
Perceived risk of contagion: Since the 1960s, perceived risk by consumers has been discussed extensively in the literature. It is demonstrated to affect all purchase decisions, as well as the intention ( Cox, 1967 ). Perceived risk guides consumer behavior significantly since people are interested in avoiding mistakes ( Mitchell, 1999 ). A situation is considered risky if the decision involves discomfort ( Taylor, 1974 ) or uncertainty ( Engel, Blackwell, & Miniard, 1990 ). According to Liao, Palvia, & Lin (2010) , consumers are generally worried when they cannot be sure that purchases will achieve their desired goals. Some research has shown that online shopping presents a risk for consumers ( Bourlakis, Papagiannidis, & Fox, 2008 ; Bianchi & Andrews, 2012 ). In the current epidemiological situation, we can say that the perceived risk variable will allow us to better explain the obstacles that consumers may have before committing to an act of purchase. Bauer (1960) has divided this risk into five types: financial, performance, physical, psychological and finally social. These last two risks are often combined into a single variable, which is the psychosocial risk ( Kaplan, Szybillo, & Jacoby, 1974 ). Similarly, Gabarino and Strahilevitz (2004 ) decomposed perceived risk into two components: uncertainty about the decision's effectiveness, and the probable losses incurred during the purchase or consumption. With the appearance of the Coronavirus, the world has changed completely. COVID-19 has caused instabilities in many sectors of society ( Good, 2020 ). Customers preoccupied with the pandemic had to prevent, cope with or respond to these changes ( Ozturk, 2020 ). In this context, another dimension of risk that has been identified is the risk of contagion. This is the risk of being contaminated through gatherings, for example, in public spaces such as stores, buses, schools... This observation is based on a study conducted by the Criteo6 cabinet in March 2020. The investigation shows that 52% of consumers in the US and UK prefer the Web as a purchasing channel and that more than 70% of consumers in South Korea prefer to buy online. As for the countries that present a moderate rate, we can observe Spain with a percentage of 42% and France with 36.7%. Therefore, we can state that the fear of catching the virus has pushed consumers to adopt online shopping as a channel to make their purchases. Consequently, we would like to study this dimension of risk on the basis of the idea: the perceived risk (risk of contagion) has an impact on the intentions to purchase on the internet. The H2 hypothesis is proposed as follows:
There is a positive and significant relationship between the perceived risk of contagion and behavioral intention to purchase online during the health crisis.
There is a positive and significant relationship between the perceived utility of online shopping in the COVID-19 period and the behavioral purchase intention.
The research model and relationships proposed in this study are illustrated in Figure 1 .
3. Research methodology
3.1 research design and sampling.
In this study, we adopted the quantitative methods approach using the questionnaire as a technique of collecting data. We have chosen this approach for two reasons. First, is to prevent crowds and, therefore, to avoid the risk of being contaminated by the coronavirus. Second, we opted for this method to collect a high number of data and in a minimal time, which is not the case for the qualitative study. The questionnaire is written in French since this language is the second language of the kingdom after Arabic. It is composed of three parts, and each part gives us specific information on the intention to buy online during Covid-19. Firstly, the questionnaire was submitted to a sample of 30 respondents to check its clarity and consistency. Then, we shared it on Linkedin and in the Facebook groups of e-commerce in Morocco (Maroc E-Commerce Communaute and J'ai testé en E-Commerce et je vous le recommande). We specifically selected these two social networks as they are the most popular and most used by consumers in the world ( El Moussaoui et al. , 2023 ). We waited seven weeks to collect the maximum possible number of responses ( Figure 2 ). In total, we received 220 completed questionnaires (Linkedin: 93; Facebook: 127) from 21 October to 8 December 2022.
3.2 Measurement instrument
To develop our measurement instrument, we have focused on the literature review. The existing documentation allowed us to develop our own items, from a set of items used by researchers. Table 2 shows the items chosen with the reference authors. All constructs in the study were operationalized as reflective constructs and adapted from past studies. The construct “Attitude towards online shopping” was measured by adapting six items from Limayem and Rowe (2006) , Sabik (2014) and Bourchich and Nejjar (2021) . The “Perceived utility” was measured by adapting six items from Zaoui (2009) and Ezzahi and Jazi (2018) . The two measurement items for “Perceived risk of contagion” were proposed by us. As for the dependent variable “Online shopping intention during Covid-19,” the four items were adapted from studies conducted by Ajzen (1991) and Moon et al. (2021) . It should be noted that the choice of variables was made on the basis of the acceptance model, incorporating the epidemiological factor “perceived risk of contagion.”
3.3 Data processing method
Following an initial verification of received questionnaires, some weaknesses were found in the dataset, allowing us to eliminate 8 incorrectly completed questionnaires. The total number of correctly filled questionnaires obtained was 220. The data processing was carried out with SPSS statistical software. There were two stages of analysis. The first one concerns the test of the reliability of measurement scales. It was realized through Principal Component Analysis (PCA), which is a factorial analysis technique. The second phase was devoted to testing the research hypotheses on the basis of the data collected ( El Moussaoui, Benbba, & El Andaloussi, 2022 ; El Moussaoui, Benbba, & El Andaloussi, 2022 , El Moussaoui, El Moussaoui, Benbba, Jaegler, & El Andaloussi, 2022 ). There are various statistical tools for testing relational research hypotheses. In this article, we have opted for multiple linear regression, an analytical model designed to explain the variance of a specific factor through a combination of explanatory variables ( El Moussaoui, Benbba, & El Andaloussi, 2022 ; El Moussaoui, Benbba, & El Andaloussi, 2022 , El Moussaoui , El Moussaoui, Benbba, Jaegler, & El Andaloussi, 2022 ).
4. Data analysis and results
4.1 description of consumer profile.
The survey was conducted with a sample of 220 Moroccan consumers of various profiles. As we see in Table 3 , the sample is composed of 101 men and 119 women, mostly aged between 18 and 45 years, with an education level of bac, bachelor, master or PhD.
4.2 Reliability of measurement scales
Before proceeding to the statistical analyses, we checked the internal reliability of the measurement scales by calculating Cronbach's alpha, which is an index that estimates the internal consistency of the items in our constructs. According to Nunnally (1978) , Cronbach's alpha value must be between 0.70 and 0.79 for the scale to be acceptable, between 0.80 and 0.90 to be “perfectly coherent” and above 0.95 to indicate that there are probably “redundant item.” On the other hand, a value between 0.60 and 0.69 is considered “minimally acceptable”; between 0.50 and 0.59, it is qualified as poor; and when the Cronbach's alpha value is lower than 0.50, the scale is “unacceptable.”
As presented in Table 4 , we obtained for the variable “Attitude” a Cronbach's alpha of 0.518, which represents a very low value. Therefore, we removed only one item (Attitude 5) to improve the quality of the model and reached a value of 0.732 ( Table 5 ). Doing the same thing for the variable “Perceived utility,” we obtained a value of 0.698, but with the removal of the item (Utility 4), the Cronbach's alpha value became 0.729. In the end, for the two variables “Perceived risk of contagion” and “Intention,” we kept all the attributed items since we obtained for the perceived risk of contagion a Cronbach's alpha of 0.875 and for the intention 0.870. So, both values are above 0.7, which means that they have a good reliability index. In the following, we will move to test the research hypotheses.
4.3 Hypothesis testing
H1 : There is a positive and significant relationship between the attitude (favorable, unfavorable) toward online shopping and the intention to buy online during the period of the health crisis (C OVID -19). From Table 6 , we can observe that there is a significant relationship between attitude and purchase intention (Sig = 0.000; P = 0.463). Therefore, both variables are positively correlated with a medium intensity. This means that the H1 hypothesis is validated.
H2 : There is a positive and significant relationship between perceived risk of contagion and intention to purchase online during the health crisis period. The examination of the results presented in Table 6 shows that there is a highly significant relationship between the perceived risk of contagion and the intention (Sig = 0.000; P = 0.452). Thus, the two variables are positively correlated with moderate intensity. It can be concluded that the H2 hypothesis is validated.
H3 : There is a positive and significant relationship between the perceived utility of online shopping in the C OVID -19 period and the behavioral purchase intention. The results presented in Table 6 indicate that there is a highly significant relationship between the perceived utility variable and the intention to purchase (Sig = 0.000; P = 0.581). Therefore, the two variables are positively correlated with high intensity. We can conclude that hypothesis H3 is validated.
In addition to the correlation analysis, we will ensure that there is no multi-collinearity effect between the variables. For this purpose, we need to examine the indicator of multi-collinearity which is the VIF (Variance Inflation Factor) (see Table 7 ). Following these results, we observe that all the values of the VIF are lower than 10, meaning that there isn't an inflation of high variance. Therefore, we can conclude that no variable is redundant, as well as no effect of multi-collinearity exists between the variables studied. Therefore, we can continue the analysis.
4.4 Model testing (multiple linear regression)
In the previous section, we tested the relationship of each of the explanatory variables with the variable that we want to explain (Intention). In this part, we will test the entire research model through multiple linear regression, using the “stepwise” method. This method allows us to analyze the influence of a selection of explanatory variables (independent) on the variable that we want to explain (dependent), as well as to keep the most economical model while maintaining only the significant variables in our research model. Table 8 shows a summary of the multiple regression analysis using the “stepwise” method.
In the model quality analysis, we opted for the stepwise method to keep only the significant variables and eliminate the redundant and unimportant variables. Then, we selected the third model with a coefficient of determination R 2 = 0.454, which signifies that the chosen model explains 45.40% of the total variance of the dependent variable “intention.” In terms of Fisher's significance, we have obtained a better level of significance (SIG.F = 0.000). In other words, the regression equation performs very significantly and allows us to conclude that the three explanatory variables (attitude, perceived risk of contagion and perceived utility) contribute significantly to the dependent variable (intention) scores. We did not retain models 1 and 2 for the reason that blocks a and b included only two variables Advantage and Attitude. Whereas, we are looking for a significant model that contains all of the study variables, including the risk of contagion, the latter variable significantly improved model 2 with an R -squared of 0.40 to 0.45 at the level of block c. In Table 8 , we present the importance of each explanatory variable in our research model.
The standardized coefficient “Beta” is interpreted in the same way as the Pearson regression coefficient. If Beta in absolute value is less than 0.29, the effect is low; if Beta is between 0.30 and 0.49, the effect is medium. Finally, if Beta in absolute value is higher than 0.50, the effect is high. It is important to remember that the objective of this step is to extract the variables that contribute significantly to the explanation of the phenomenon studied. Following the analysis of Table 9 , we note that the standardized coefficient of 0.371 of the variable “perceived utility” is the highest coefficient, then comes the variable “attitude” with a standardized coefficient Beta of 0.264 and finally the variable “perceived risk of contagion” comes last with a standardized coefficient Beta 0.252. The results obtained in Table 9 allow us to conclude that the variable “perceived utility” is the most important factor influencing consumers' intentions toward online purchases during the health crisis (COVID-19). This conclusion is perfectly in line with the analysis of Pearson's correlation coefficient since the variable “perceived utility” obtained the highest Pearson's coefficient (P = 0.581).
5. Discussion
5.1 effect of attitude toward online shopping in covid-19 period on behavioral intention.
The hypothesis that assumes a link between attitude and online shopping intention was validated in our sample. This finding is consistent with the TRA ( Ajzen and Fishbein, 1975 ), planned behavior ( Ajzen, 1991 ) and with TAM ( Davis et al ., 1989 ). Our result remains in agreement with previous works. It was found that even with this climate of the pandemic, attitude remains one of the determinants that can predict consumer behavior in the adoption of online shopping. This is exactly the case with the studies conducted by Sabik (2014 ), Ezzahi and Jazi (2018) , and Bourchich and Nejjar (2021 ) which confirmed that the attitude toward internet usage positively affects the intention to buy products online.
5.2 Effect of perceived utility on behavioral intention in the COVID-19 period
The hypothesis that assumes a positive and significant relationship between perceived utility and online purchase intention in the COVID-19 period is supported. The regression analysis shows that perceived utility has the greatest effect on behavioral intentions to purchase products on the Internet during the COVID-19 period. This finding follows the TAM of Davis et al . (1989) , which shows that intention is impacted by the perceived utility through attitude (mediating variable). This means that the consumer develops an intention to shop online during this epidemiological period only when he sees a benefit toward online shopping, such as avoiding crowds, home delivery and time-saving, etc.
5.3 Influence of perceived risk (perceived risk of contagion) on behavioral intention
The hypothesis that assumes a positive and significant relationship between the perceived risk of contagion and the intention to buy online during the COVID-19 period is verified. The regression analysis gives the first variable the last rank in terms of effect. The fact that the variable “perceived risk of contagion” has a weak effect can be explained by the period in which we started our survey (a few months after the confinement), meaning that the panic and fear of the COVID-19 pandemic has visibly diminished among consumers. This result was unexpected, as it was predicted that this variable would have the highest effect, following the worsening epidemiological situation day after day. Indeed, this finding is in line with previous research results, especially the results of the Criteo study in 2020 conducted in five countries, namely, France, Spain, the UK, South Korea and the USA, which confirms that most consumers are oriented toward online shopping for epidemiological reasons. This approves that the perceived risks influence the behavioral intention to buy online ( Vijayasarathy & Jones, 2000 ).
6. Conclusion/implications/limitations
6.1 conclusion.
This paper aims to determine the factors that influence online shopping in the current pandemic context. For this purpose, we developed our conceptual model by referring to Davis et al. ' (1989) TAM. We introduced the constructs “Attitude,” “perceived utility,” “intention” as well as the variable “perceived risk of contagion.” The results indicate that attitude and perceived utility positively affect online shopping intention. However, the variable “perceived risk of contagion” has a weak effect on such intention, which can be explained by the period in which we started our survey (a few months after the confinement).
6.2 Implications
The results of this study provided additional empirical support for the constructs of the technology acceptance model. The inclusion of the variable “perceived risk of contagion” reinforces the original model and provides a contribution to their influence on online shopping intention during the COVID-19 pandemic. This, in turn, will allow us to better understand consumer behavior. This study is one of the first studies that incorporate the variable “perceived risk of contagion” into the TAM. This highlights the necessity of adapting models to different contexts for a better understanding of behavioral intention. Academics have frequently worried about the role of national factors in the adoption of technology by the consumer. Furthermore, they have discouraged researchers from modeling technology acceptance patterns, as they still believe that such a pattern differs completely from one country to another. In the context of the COVID-19 crisis, this study was conducted to fill this gap by providing insights into the factors that determine consumers' online purchase intention. In this way, the study increases the generality potential of the research instrument. This fact once again underlines the central role of context in the design of theories. Indeed, it is crucial to understand the contexts in which theories begin to break down, as these form a basis for future research and knowledge creation.
The results of this paper can be applied and exerted in the context of the new crown pneumonia epidemic. For example, it can guide e-commerce website policymakers to make decisions that are beneficial to consumers. It is recommended that e-commerce actors focus on the experience of the Internet users, by proposing fluidity in the whole process of the orders and making the navigation on the website and the purchase pleasant. We should also note that even though epidemics are no longer epiphenomena, they pose real managerial challenges. In this context, the marketing department of companies must devote its efforts essentially to media and non-media communication, to create a favorable attitude among website visitors. We also suggest that practitioners of the field focus more on the advantages mentioned above (avoiding crowds, home delivery and time-saving) through the different communication tools in order to develop the willingness of consumers to purchase on the Internet, and ultimately to have a good level of conversion in their e-commerce websites. In addition, the managers of online websites are required to clearly communicate the protective actions and measures they take in the preparation and delivery processes to support the consumer's sense of security.
6.3 Limitations/future research
The multiple linear regression model used in the article is mainly designed to explore the correlation between the variables. More rigorous research models and methods are needed to prove the causal relationship between the variables.
The consideration of the variables is not rich enough. Based on the TAM technology acceptance model, this paper investigates the impact of attitude, perceived utility and perceived risk of infection on online shopping intention during COVID-19. Many factors can affect consumers' online purchasing intention, such as perceived benefits, perceived costs, perceived value and other factors. Future research can take these limitations into consideration by including all these variables.
The data collected through the questionnaire is not always accountable for the user's purchasing behavior. During this process, we observed that some questionnaires were not 100% completed, which allowed us to leave them out of the statistical study. Also noting that our research model has been statistically validated, but we cannot generalize it because our sample size is relatively small. Therefore, future research can adopt the same conceptual model we proposed with a large sample size. In addition, all the measurement scales were selected from the literature, except for the scale measuring the variable “perceived risk of contagion” which we proposed ourselves and which has never been used in other works. It should be noted that this variable had the lowest score in the correlation analysis, which calls into question the internal reliability of the scale used, even though we obtained a high Cronbach's alpha. This limitation could be the subject of future research.
The questionnaire is not written in Morocco's first language. Thus, it can be alluded that this study is particularly addressed to people with a high level of education. For better clarity, future research in Morocco should take a significant sample by writing a questionnaire in Arabic, so that all Moroccan citizens will be able to answer it.
Research model
Data collection process
Main research dealing with online shopping intention
Source(s): Table by authors
Addo , P .C. , Jiaming , F. , Kulbo , N. B. , & Liangqiang , L. ( 2020 ). COVID-19: Fear appeal favoring purchase behavior towards personal protective equipment . The Service Industries Journal , 40 ( 7-8 ), 471 – 490 .
Akar , E. , & Dalgic , T. ( 2018 ). Understanding online consumers’ purchase intentions: A contribution from social network theory . Behaviour & Information Technology , 37 ( 5 ), 473 – 487 .
Ait Youssef , A. , Jaafari , M. , & Belhcen , L. ( 2020 ). Factors affecting the online purchase intention during COVID-19 crisis: The case of Morocco . Available at SSRN 3734389 .
Ajzen , I. ( 1991 ). The theory of planned behavior . Organizational Behavior and Human Decision Processes , 50 ( 2 ), 179 – 211 .
Ajzen , I. , & Fishbein , M. ( 1975 ). A Bayesian analysis of attribution processes . Psychological Bulletin , 82 ( 2 ), 261 .
Akroush , M. N. , & Al-Debei , M. M. ( 2015 ). An integrated model of factors affecting consumer attitudes towards online shopping . Business Process Management Journal , 21 ( 6 ), 1353 – 1376 .
Al-Rahmi , W. M. , Yahaya , N. , Aldraiweesh , A. A. , Alamri , M. M. , Aljarboa , N. A. , Alturki , U. , & Aljeraiwi , A. A. ( 2019 ). Integrating technology acceptance model with innovation diffusion theory: An empirical investigation on students’ intention to use E-learning systems . Ieee Access , 7 , 26797 – 26809 .
Audrey , Y. A. , Wiguna , A. S. , Ramadhani , S. A. , & Gui , A. ( 2022 ). Factors influencing online shopping intention in Indonesia . In 2022 International Conference on Information Management and Technology (ICIMTech) (pp. 357 – 362 ). IEEE .
Barkhi , R. , Belanger , F. , & Hicks , J. ( 2008 ). A model of the determinants of purchasing from virtual stores . Journal of Organizational Computing and Electronic Commerce , 18 ( 3 ), 177 – 196 .
Bauer , R. ( 1960 ), “ Consumer behavior as risk taking ”, Dynamic Marketing for a Changing World , American Marketing Association , Chicago , pp. 389 - 298 .
Bianchi , C. , & Andrews , L. ( 2012 ). Risk, trust, and consumer online purchasing behaviour: A Chilean perspective . International Marketing Review , 9 ( 3 ), 253 – 276 .
Biswas , A. ( 2017 ). A consumption value-gap analysis for sustainable consumption . Environmental Science and Pollution Research , 24 ( 8 ), 7714 – 7725 .
Biswas , A. , & Roy , M. ( 2015 ). Leveraging factors for sustained green consumption behavior based on consumption value perceptions: Testing the structural model . Journal of Cleaner Production , 95 , 332 – 340 .
Bourchich , A. , & Nejjar , B. ( 2021 ). Les déterminants de l’achat en ligne en période de la Covid -19 : Approche quantitative « Une étude Post confinement » [The Determinants of Online Shopping during Covid-19: A Quantitative Approach ‘A Post-Containment Study’] . International Journal of Accounting, Finance, Auditing, Management and Economics , 2 ( 5 ), 60 – 77 .
Bourlakis , M. , Papagiannidis , S. , & Fox , H. ( 2008 ). Econsumer behaviour: Past, present and future trajectories of an evolving retail revolution . International Journal of E-Business Research , 4 ( 3 ), 64 – 76 .
Chiu , W. , Kim , T. , & Won , D. ( 2018 ). Predicting consumers’ intention to purchase sporting goods online: An application of the model of goal-directed behavior . Asia Pacific Journal of Marketing and Logistics , 30 ( 2 ), 333 – 351 .
Cox , D. F. ( 1967 ). Risk taking and information handling in consumer behaviour . Boston : Harvard University Press .
Dang , V. T. , & Pham , T. L. ( 2018 ). An empirical investigation of consumer perceptions of online shopping in an emerging economy: Adoption theory perspective . Asia Pacific Journal of Marketing and Logistics , 30 ( 4 ), 952 – 971 .
Davis , F. D. , Bagozzi , R. P. , & Warshaw , P. R. ( 1989 ). User acceptance of computer technology: A comparison of two theoretical models . Management Science , 35 ( 8 ), 982 – 1003 .
El Moussaoui , A. E. , Benbba , B. and El Andaloussi , Z. ( 2022 ). Impact of logistics performance on the store image, consumer satisfaction and loyalty: A quantitative case study . Arab Gulf Journal of Scientific Research, (ahead-of-print ).
El Moussaoui , A. E. , Benbba , B. , & El Andaloussi , Z. ( 2022 ). Perception d’e-consommateur sur les consignes automatiques: Note de recherche [E-consumer perception of automated lockers: Research note] . Revue de Recherches en Economie et en Management Africain , 10 ( 10 ).
El Moussaoui , A. E. , El Moussaoui , T. , Benbba , B. , Jaegler , A. , & El Andaloussi , Z. ( 2022 ). Understanding the choice of collection & delivery point by the E-consumer via a machine learning model: Moroccan case study . Procedia Computer Science , 210 , 204 – 211 .
El Moussaoui , A. E. , Benbba , B. , Jaegler , A. , El Moussaoui , T. , El Andaloussi , Z. , & Chakir , L. ( 2023 ). Consumer perceptions of online shopping and willingness to use pick-up points: A case study of Morocco . Sustainability , 15 ( 9 ), 7405 .
Emin , S. ( 2004 ). Les facteurs déterminant la création d'entreprise par les chercheurs public: Application des modèles d'entention [The factors determining the creation of businesses by public researchers: Application of the training models] . Revue de l'entrepreneuriat , 3 ( 1 ), 1 - 20 .
Engel , J. , Blackwell , R. , & Miniard , P. ( 1990 ). Consumer behavior (pp. 789 ). Chicago : Bryden Press .
Eti , I. A. , Horaira , M. A. , & Bari , M. M. ( 2021 ). Power and stimulus of social media marketing on consumer purchase intention in Bangladesh during the COVID-19 . International Journal of Research in Business and Social Science (2147-4478) , 10 ( 1 ), 28 – 37 .
Ezzahi and Jazi ( 2018 ), “ Influence du bouche à oreille électronique sur l’intention d’achat: Une exploration netnographique ”.
Gabarino , E. , & Strahilevitz , M. ( 2004 ). Gender differences in the perceived risk of buying online and the effects of receiving a site recommendation . Journal of Business Research , 57 .
Good , K. W. ( 2020 ). The COVID-19 Apocalypse – rules for survival . Available from: https://www.jurist.org/commentary/2020/04/the-covid-19-apocalypse-rules-for-survival/ ( accessed 7 May 2023 ).
Ha , N. , & Nguyen , T. ( 2019 ). The effect of trust on consumers’ online purchase intention: An integration of TAM and TPB . Management Science Letters , 9 ( 9 ), 1451 – 1460 .
Hajraoui , K. , & Chalabi , H. ( 2021 ). Impact du confinement dû à la pandémie du SARS-CoV-2 sur le comportement d’achat et la fréquentation des points de vente des produits alimentaires au Maroc [Impact of confinement caused by the SARS-CoV-2 pandemic on purchasing behavior and food store frequency in Morocco] . Revue d’Etudes en Management et Finance d’Organisation , 5 ( 1 ).
Hur , W. M. , Yoo , J. J. , & Chung , T. L. ( 2012 ). The consumption values and consumer innovativeness on convergence products . Industrial Management & Data Systems .
Jain , S. ( 2022 ). Factors influencing online luxury purchase intentions: The moderating role of bandwagon luxury consumption behavior . South Asian Journal of Business Studies , (ahead-of-print) .
Jordan , G. , Leskovar , R. , & Marič , M. ( 2018 ). Impact of fear of identity theft and perceived risk on online purchase intention . Organizacija , 51 ( 2 ), 146 – 155 .
Kaplan , L. B. , Szybillo , G. J. , & Jacoby , J. ( 1974 ). Components of perceived risk in product purchase: A cross-validation . Journal of Applied Psychology , 59 ( 3 ), 287 .
Karahanna , E. , Straub , D. W. , & Chervany , N. L. ( 1999 ). Information technology adoption across time: A cross-sectional comparison of pre-adoption and post-adoption beliefs . MIS Quarterly , 183 – 213 .
Kühn , S. W. , & Petzer , D. J. ( 2018 ). Fostering purchase intentions toward online retailer websites in an emerging market: An SOR perspective . Journal of Internet Commerce , 17 ( 3 ), 255 – 282 .
Li , L. , Wang , Z. , Li , Y. , & Liao , A. ( 2021 ). Impacts of consumer innovativeness on the intention to purchase sustainable products . Sustainable Production and Consumption , 27 , 774 – 786 .
Li , Y. , Xu , Z. , & Xu , F. ( 2018 ). Perceived control and purchase intention in online shopping: The mediating role of self-efficacy . Social Behavior and Personality: An International Journal , 46 ( 1 ), 99 – 105 .
Liao , Palvia and Lin ( 2010 ), “ Stage antecedents of consumer online buying behavior ”, Electronic Markets , Vol. 20 , pp. 53 - 65 .
Limayem , M. , & Rowe , F. ( 2006 ). Comparaison des facteurs influençant les intentions d'achat à partir du Web à Hong Kong et en France: influence sociale , risques et aversion pour la perte du contact [Comparison of factors influencing web-based purchase intentions in Hong Kong and France: social influence, risk and contact loss aversion] . Revue française du marketing .
Ling , H. C. , Chen , H. R. , Ho , K. K. , & Hsiao , K. L. ( 2021 ). Exploring the factors affecting customers’ intention to purchase a smart speaker . Journal of Retailing and Consumer Services , 59 , 102331 .
Liu , H. , Feng , S. , & Hu , X.S. ( 2022 ). Process vs. outcome: Effects of food photo types in online restaurant reviews on consumers’ purchase intention . International Journal of Hospitality Management , 102 , 103179 .
Marangunić , N. , & Granić , A. ( 2015 ). Technology acceptance model: A literature review from 1986 to 2013 . Universal Access in the Information Society , 14 , 81 – 95 .
Mitchell , V. W. ( 1999 ). Consumer perceived risk: Conceptualisations and models . European Journal of Marketing , 33 ( 12 ), 163195 .
Moon , J. , Choe , Y. , & Song , H. ( 2021 ). Determinants of consumers’ online/offline shopping behaviours during the COVID-19 pandemic . International Journal of Environmental Research and Public Health , 18 ( 4 ), 1593 .
Nunnally ( 1978 ), “ An overview of psychological measurement ”, Clinical Diagnosis of Mental Disorders: A Handbook , pp. 97 - 146 .
Ozturk , R. ( 2020 ). Health or death? The online purchase intentions of consumers during the COVID-19 pandemic . Transnational Marketing Journal (TMJ) , 8 ( 2 ), 219 – 241 .
Phau , I. , Quintal , V. , & Shanka , T. ( 2014 ). Examining a consumption values theory approach of young tourists toward destination choice intentions . International Journal of Culture, Tourism and Hospitality Research .
Ramayah , T. , Rahman , S. A. , & Ling , N. C. ( 2018 ). How do consumption values influence online purchase intention among school leavers in Malaysia? . Revista Brasileira de Gestão de Negócios , 20 , 638 – 654 .
Redda , E. H. ( 2019 ). Attitudes towards online shopping: Application of the theory of planned behaviour . Acta Universitatis Danubius. Œconomica , 15 ( 2 ), 148 – 159 .
Rehman , S. U. , Bhatti , A. , Mohamed , R. , & Ayoup , H. ( 2019 ). The moderating role of trust and commitment between consumer purchase intention and online shopping behavior in the context of Pakistan . Journal of Global Entrepreneurship Research , 9 , 1 – 25 .
Robinson , L. ( 2009 ). A summary of diffusion of innovations .
Rogers , E. M. ( 1995 ). Diffusion of innovations: modifications of a model for telecommunications (pp. 25 – 38 ). Die diffusion von innovationen in der telekommunikation .
Sabik , S. ( 2014 ). les facteurs déterminants de l'intention d'achat en ligne: cas des maghrébins immigrés au Canada [Determinants of the intention to buy online: the case of the Maghrebin immigrants in Canada] .
Sahli , F. ( 2020 ). Coronavirus (Covid19): Quel impact sur le comportement d’achat en ligne des consommateurs tunisiens? [Coronavirus (Covid-19): What impact on the online shopping behavior of Tunisian consumers?] . Alternatives Managériales Economiques , 2 ( 3 ), 16 – 36 .
Sharp , J. H. ( 2006 ). Development, extension, and application: A review of the technology acceptance model . Director , 7 ( 9 ), 3 – 11 .
Singh , S. , & Srivastava , S. ( 2018 ). Moderating effect of product type on online shopping behavior and purchase intention: An Indian perspective . Cogent Arts & Humanities , 5 ( 1 ), 1495043 .
Sutton , S. ( 1998 ). Predicting and explaining intentions and behavior: How well are we doing? . Journal of Applied Social Psychology , 28 ( 15 ), 1317 – 1338 .
Taylor , J. W. ( 1974 ). The role of risk in consumer behavior . Journal of Marketing , 38 ( 2 ), 5456 .
Thye Goh , T. , Mohd Suki , N. , & Fam , K. ( 2014 ). Exploring a consumption value model for Islamic mobile banking adoption . Journal of Islamic Marketing , 5 ( 3 ), 344 – 365 .
Triandis , H. ( 1979 ). Values, attitudes, and interpersonal behavior . Nebreska Symposium on motivation , Lincoln . University of Nebreska Press .
Verhagen , T. , & Bloemers , D. ( 2018 ). Exploring the cognitive and affective bases of online purchase intentions: A hierarchical test across product types . Electronic Commerce Research , 18 ( 3 ), 537 – 561 .
Vijayasarathy , L. R. , & Jones , J. M. ( 2000 ). Print and internet catalog shopping: Assessing attitudes and intention . Internet Research: Electronic Networking Applications and Policy , 10 ( 3 ), 191 – 202 .
Wani , T. A. , & Ali , S. W. ( 2015 ). Innovation diffusion theory . Journal of General Management Research , 3 ( 2 ), 101 – 118 .
Yang , S. , Li , L. , & Zhang , J. ( 2018 ). Understanding consumers’ sustainable consumption intention at China’s double-11 online shopping festival: An extended theory of planned behavior model . Sustainability , 10 ( 6 ), 1801 .
Zaoui , I. ( 2009 ). Orientation de shopping et intention d'achat en ligne [Orientation of shopping and intention to buy online] . Actes du 25 ème Congrès international de l'AFM .
Corresponding author
Related articles, we’re listening — tell us what you think, something didn’t work….
Report bugs here
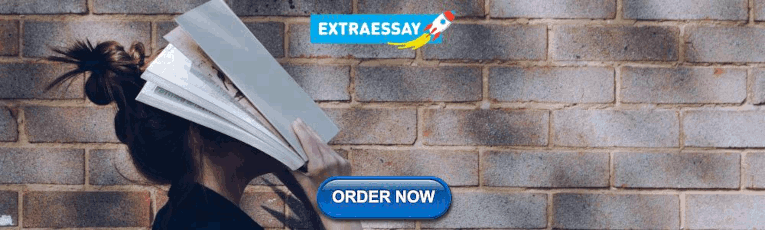
All feedback is valuable
Please share your general feedback
Join us on our journey
Platform update page.
Visit emeraldpublishing.com/platformupdate to discover the latest news and updates
Questions & More Information
Answers to the most commonly asked questions here
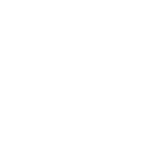
98 Quantitative Research Questions & Examples

As researchers, we know how powerful quantitative research data can be in helping answer strategic questions. Here, I’ve detailed 23 use cases and curated 98 quantitative market research questions with examples – making this a post you should add to your bookmark list 📚, so you can quickly refer back.
I’ve formatted this post to show you 10-15 questions for each use case. At the end of each section, I also share a quicker way to get similar insights using modern market research tools like Similarweb.
What is a quantitative research question?
Quantitative market research questions tell you the what, how, when, and where of a subject. From trendspotting to identifying patterns or establishing averages– using quantitative data is a clear and effective way to start solving business problems.
Types of quantitative research questions
Quantitative market research questions are divided into two main types: descriptive and causal.
- Descriptive research questions seek to quantify a phenomenon by focusing on a certain population or phenomenon to measure certain aspects of it, such as frequency, average, or relationship.
- Causal research questions explore the cause-and-effect relationship between two or more variables.
The ultimate list of questions for quantitative market research
Get clear explanations of the different applications and approaches to quantitative research–with the added bonus of seeing what questions to ask and how they can impact your business.
Examples of quantitative research questions for competitive analysis
A powerful example of quantitative research in play is when it’s used to inform a competitive analysis . A process that’s used to analyze and understand how industry leaders and companies of interest are performing.
Pro Tip: Collect data systematically, and use a competitive analysis framework to record your findings. You can refer back to it when you repeat the process later in the year.
- What is the market share of our major competitors?
- What is the average purchase price of our competitors’ products?
- How often do our competitors release new products?
- What is the total number of customer reviews for our competitors’ products?
- What is the average rating of our competitors’ products?
- What is the average customer satisfaction score for our competitors?
- What is the average return rate of our competitors’ products?
- What is the average shipping time for our competitors’ products?
- What is the average price discount offered by our competitors?
- What is the average lifespan of our competitors’ products?
With this data, you can determine your position in the market and benchmark your performance against rival companies. It can then be used to improve offerings, service standards, pricing, positioning, and operational effectiveness. Notice that all questions can be answered with a numerical response , a key component of all successful examples of quantitative market research questions.
Quantitative research question example: market analysis
🙋♀️ Question: What is the market share of our major competitors?
🤓 Insight sought: Industry market share of leaders and key competitors.
🤯 Challenges with traditional quantitative research methods: Outdated data is a major consideration; data freshness remains critical, yet is often tricky to obtain using traditional research methods. Markets shift fast, so being able to obtain and track market share in real time is a challenge many face.
💡 A new approach: Similarweb enables you to track this key business KPI in real-time using digital data directly from the platform. On any day, you can see what your market share is, along with any players in your market. Plus, you get to see rising stars showing significant growth, who may pose a threat through market disruption or new tactics.
⏰ Time to insight: 30 seconds
✅ How it’s done: Using Similarweb’s Web Industry Analysis, two digital metrics give you the intel needed to decipher the market share in any industry. I’m using the Banking, Credit, and Lending market throughout these examples. I’ve selected the US market, analyzing the performance of the previous 3 months.
- Share of visits

Here, I can see the top players in my market based on the number of unique visitors to their sites. On top of the raw data that shows me the volume of visitors as a figure, I can quickly see the two players ( Capital One and Chase ) that have grown and by what percentage. On the side, you can see rising players in the industry. Now, while my initial question was to establish the market share of my major competitors, I can see there are a few disruptive players in my market who I’d want to track too; Synchrony.com being one of particular interest, given their substantial growth and traffic numbers.
- Share of search

Viewing the overall market size based on total search volumes, you can explore industry leaders in more detail. The top websites are the top five players, ranking by traffic share . You can also view the month-over-month change in visits, which shows you who is performing best at any given time . It’s the same five names, with Paypal and Chase leading the pack. However, I see Wells Fargo is better at attracting repeat visitors, while Capital One and Bank of America perform better at drawing in unique visitors.
In answer to my question, what is the market share of my major competitors, I can quickly use Similarweb’s quantitative data to get my answer.

This traffic share visual can be downloaded from the platform. It plots the ten industry leader’s market share and allocates the remaining share to the rest of the market.

I can also download a market quadrant analysis, which takes two key data points, traffic share and unique visitors, and plots the industry leaders. All supporting raw data can be downloaded in .xls format or connected to other business intelligence platforms via the API.
Quantitative research questions for consumer behavior studies
These studies measure and analyze consumer behavior, preferences, and habits . Any type of audience analysis helps companies better understand customer intent, and adjust offerings, messaging, campaigns, SEO, and ultimately offer more relevant products and services within a market.
- What is the average amount consumers spend on a certain product each month?
- What percentage of consumers are likely to purchase a product based on its price?
- How do the demographics of the target audience affect their purchasing behavior?
- What type of incentive is most likely to increase the likelihood of purchase?
- How does the store’s location impact product sales and turnover?
- What are the key drivers of product loyalty among consumers?
- What are the most commonly cited reasons for not buying a product?
- How does the availability of product information impact purchasing decisions?
- What is the average time consumers spend researching a product before buying it?
- How often do consumers use social media when making a purchase decision?
While applying a qualitative approach to such studies is also possible, it’s a great example of quantitative market research in action. For larger corporations, studies that involve a large, relevant sample size of a target market deliver vital consumer insights at scale .
Read More: 83 Qualitative Research Questions & Examples
Quantitative research question and answer: content strategy and analysis
🙋♀️ Question: What type of content performed best in the market this past month?
🤓 Insight sought: Establish high-performing campaigns and promotions in a market.
🤯 Challenges with traditional quantitative research methods: Whether you consider putting together a panel yourself, or paying a company to do it for you, quantitative research at scale is costly and time-consuming. What’s more, you have to ensure that sampling is done right and represents your target audience.
💡 A new approach: Data analysis is the foundation of our entire business. For over 10 years, Similarweb has developed a unique, multi-dimensional approach to understanding the digital world. To see the specific campaigns that resonate most with a target audience, use Similarweb’s Popular Pages feature. Key metrics show which campaigns achieve the best results for any site (including rival firms), campaign take-up, and periodic changes in performance and interest.
✅ How it’s done: I’ve chosen Capital One and Wells Fargo to review. Using the Popular Pages campaign filter, I can view all pages identified by a URL parameter UTM. For clarity, I’ve highlighted specific campaigns showing high-growth and increasing popularity. I can view any site’s trending, new, or best-performing pages using a different filter.

In this example, I have highlighted three campaigns showing healthy growth, covering teen checking accounts, performance savings accounts, and add-cash-in-store. Next, I will perform the same check for another key competitor in my market.

Here, I can see financial health tools campaigns with over 300% month-over-month growth and smarter credit and FICO campaigns showing strong performance. This tells me that campaigns focussing on education and tools are growing in popularity within this market.
Examples of quantitative research questions for brand tracking
These studies are designed to measure customers’ awareness, perceptions, behaviors, and attitudes toward a brand over time. Different applications include measuring brand awareness , brand equity, customer satisfaction, and purchase or usage intent.

These types of research surveys ask questions about brand knowledge, brand attributes, brand perceptions, and brand loyalty . The data collected can then be used to understand the current state of a brand’s performance, identify improvements, and track the success of marketing initiatives.
- To what extent is Brand Z associated with innovation?
- How do consumers rate the quality of Brand Z’s products and services?
- How has the awareness of Brand Z changed over the past 6 months?
- How does Brand Z compare to its competitors in terms of customer satisfaction?
- To what extent do consumers trust Brand Z?
- How likely are consumers to recommend Brand Z?
- What factors influence consumers’ purchase decisions when considering Brand Z?
- What is the average customer satisfaction score for equity?
- How does equity’s customer service compare to its competitors?
- How do customer perceptions of equity’s brand values compare to its competitors?
Quantitative research question example and answer: brand tracking
🙋♀️ Question: How has the awareness of Brand Z changed over the past 6 months?
🤓 Insight sought: How has brand awareness changed for my business and competitors over time.
⏰ Time to insight: 2 minutes
✅ How it’s done: Using Similarweb’s search overview , I can quickly identify which brands in my chosen market have the highest brand awareness over any time period or location. I can view these stats as a custom market or examine brands individually.

Here, I’ve chosen a custom view that shows me five companies side-by-side. In the top right-hand corner, under branded traffic, you get a quick snapshot of the share of website visits that were generated by branded keywords. A branded keyword is when a consumer types the brand name + a search term.
Below that, you will see the search traffic and engagement section. Here, I’ve filtered the results to show me branded traffic as a percentage of total traffic. Similarweb shows me how branded search volumes grow or decline monthly. Helping me answer the question of how brand awareness has changed over time.
Quantitative research questions for consumer ad testing
Another example of using quantitative research to impact change and improve results is ad testing. It measures the effectiveness of different advertising campaigns. It’s often known as A/B testing , where different visuals, content, calls-to-action, and design elements are experimented with to see which works best. It can show the impact of different ads on engagement and conversions.
A range of quantitative market research questions can be asked and analyzed to determine the optimal approach.
- How does changing the ad’s headline affect the number of people who click on the ad?
- How does varying the ad’s design affect its click-through rate?
- How does altering the ad’s call-to-action affect the number of conversions?
- How does adjusting the ad’s color scheme influence the number of people who view the ad?
- How does manipulating the ad’s text length affect the average amount of time a user spends on the landing page?
- How does changing the ad’s placement on the page affect the amount of money spent on the ad?
- How does varying the ad’s targeting parameters affect the number of impressions?
- How does altering the ad’s call-to-action language impact the click-through rate?
Quantitative question examples for social media monitoring
Quantitative market research can be applied to measure and analyze the impact of social media on a brand’s awareness, engagement, and reputation . By tracking key metrics such as the number of followers, impressions, and shares, brands can:
- Assess the success of their social media campaigns
- Understand what content resonates with customers
- Spot potential areas for improvement
- How often are people talking about our brand on social media channels?
- How many times has our brand been mentioned in the past month?
- What are the most popular topics related to our brand on social media?
- What is the sentiment associated with our brand across social media channels?
- How do our competitors compare in terms of social media presence?
- What is the average response time for customer inquiries on social media?
- What percentage of followers are actively engaging with our brand?
- What are the most popular hashtags associated with our brand?
- What types of content generate the most engagement on social media?
- How does our brand compare to our competitors in terms of reach and engagement on social media?
Example of quantitative research question and answer: social media monitoring
🙋♀️ Question: How does our brand compare to our competitors in terms of reach and engagement on social media?
🤓 Insight sought: The social channels that most effectively drive traffic and engagement in my market
✅ How it’s done: Similarweb Digital Research Intelligence shows you a marketing channels overview at both an industry and market level. With it, you can view the most effective social media channels in any industry and drill down to compare social performance across a custom group of competitors or an individual company.
Here, I’ve taken the five closest rivals in my market and clicked to expand social media channel data. Wells Fargo and Bank of America have generated the highest traffic volume from social media, with over 6.6 million referrals this year. Next, I can see the exact percentage of traffic generated by each channel and its relative share of traffic for each competitor. This shows me the most effective channels are Youtube, Facebook, LinkedIn, and Reddit – in that order.

In 30-seconds, I’ve discovered the following:
- YouTube is the most popular social network in my market.
- Facebook and LinkedIn are the second and third most popular channels.
- Wells Fargo is my primary target for a more in-depth review, with the highest performance on the top two channels.
- Bank of America is outperforming all key players significantly on LinkedIn.
- American Express has found a high referral opportunity on Reddit that others have been unable to match.
Power-up Your Market Research with Similarweb Today
Examples of quantitative research questions for online polls.
This is one of the oldest known uses of quantitative market research. It dates back to the 19th century when they were first used in America to try and predict the outcome of the presidential elections.

Polls are just short versions of surveys but provide a point-in-time perspective across a large group of people. You can add a poll to your website as a widget, to an email, or if you’ve got a budget to spend, you might use a company like YouGov to add questions to one of their online polls and distribute it to an audience en-masse.
- What is your annual income?
- In what age group do you fall?
- On average, how much do you spend on our products per month?
- How likely are you to recommend our products to others?
- How satisfied are you with our customer service?
- How likely are you to purchase our products in the future?
- On a scale of 1 to 10, how important is price when it comes to buying our products?
- How likely are you to use our products in the next six months?
- What other brands of products do you purchase?
- How would you rate our products compared to our competitors?
Quantitative research questions for eye tracking studies
These research studies measure how people look and respond to different websites or ad elements. It’s traditionally an example of quantitative research used by enterprise firms but is becoming more common in the SMB space due to easier access to such technologies.
- How much time do participants spend looking at each visual element of the product or ad?
- How does the order of presentation affect the impact of time spent looking at each visual element?
- How does the size of the visual elements affect the amount of time spent looking at them?
- What is the average time participants spend looking at the product or ad as a whole?
- What is the average number of fixations participants make when looking at the product or ad?
- Are there any visual elements that participants consistently ignore?
- How does the product’s design or advertising affect the average number of fixations?
- How do different types of participants (age, gender, etc.) interact with the product or ad differently?
- Is there a correlation between the amount of time spent looking at the product or ad and the participants’ purchase decision?
- How does the user’s experience with similar products or ads affect the amount of time spent looking at the current product or ad?
Quantitative question examples for customer segmentation
Segmentation is becoming more important as organizations large and small seek to offer more personalized experiences. Effective segmentation helps businesses understand their customer’s needs–which can result in more targeted marketing, increased conversions, higher levels of loyalty, and better brand awareness.

If you’re just starting to segment your market, and want to know the best quantitative research questions to ask to help you do this, here are 20 to choose from.
Examples of quantitative research questions to segment customers
- What is your age range?
- What is your annual household income?
- What is your preferred online shopping method?
- What is your occupation?
- What types of products do you typically purchase?
- Are you a frequent shopper?
- How often do you purchase products online?
- What is your typical budget for online purchases?
- What is your primary motivation for purchasing products online?
- What factors influence your decision to purchase a product online?
- What device do you use most often when shopping online?
- What type of product categories are you most interested in?
- Do you prefer to shop online for convenience or for a better price?
- What type of discounts or promotions do you look for when making online purchases?
- How do you prefer to receive notifications about product promotions or discounts?
- What type of payment methods do you prefer when shopping online?
- What methods do you use to compare different products and prices when shopping online?
- What type of customer service do you expect when shopping online?
- What type of product reviews do you consider when making online purchases?
- How do you prefer to interact with a brand when shopping online?
Examples of quantitative research questions for analyzing customer segments
- What is the average age of customers in each segment?
- How do spending habits vary across customer segments?
- What is the average length of time customers spend in each segment?
- How does loyalty vary across customer segments?
- What is the average purchase size in each segment?
- What is the average frequency of purchases in each segment?
- What is the average customer lifetime value in each segment?
- How does customer satisfaction vary across customer segments?
- What is the average response rate to campaigns in each segment?
- How does customer engagement vary across customer segments?
These questions are ideal to ask once you’ve already defined your segments. We’ve written a useful post that covers the ins and outs of what market segmentation is and how to do it.
Additional applications of quantitative research questions
I’ve covered ten use cases for quantitative questions in detail. Still, there are other instances where you can put quantitative research to good use.
Product usage studies: Measure how customers use a product or service.
Preference testing: Testing of customer preferences for different products or services.
Sales analysis: Analysis of sales data to identify trends and patterns.
Distribution analysis: Analyzing distribution channels to determine the most efficient and effective way to reach customers.
Focus groups: Groups of consumers brought together to discuss and provide feedback on a particular product, service, or marketing campaign.
Consumer interviews: Conducted with customers to understand their behavior and preferences better.
Mystery shopping: Mystery shoppers are sent to stores to measure customer service levels and product availability.
Conjoint analysis: Analysis of how consumers value different attributes of a product or service.
Regression analysis: Statistical analysis used to identify relationships between different variables.
A/B testing: Testing two or more different versions of a product or service to determine which one performs better.
Brand equity studies: Measure, compare and analyze brand recognition, loyalty, and consumer perception.
Exit surveys: Collect numerical data to analyze employee experience and reasons for leaving, providing insight into how to improve the work environment and retain employees.
Price sensitivity testing: Measuring responses to different pricing models to find the optimal pricing model, and identify areas if and where discounts or incentives might be beneficial.
Quantitative market research survey examples
A recent GreenBook study shows that 89% of people in the market research industry use online surveys frequently–and for good reason. They’re quick and easy to set up, the cost is minimal, and they’re highly scalable too.

Questions are always formatted to provide close-ended answers that can be quantified. If you wish to collect free-text responses, this ventures into the realm of qualitative research . Here are a few examples.
Brand Loyalty Surveys: Companies use online surveys to measure customers’ loyalty to their brand. They include questions about how long an individual has been a customer, their overall satisfaction with the service or product, and the likelihood of them recommending the brand to others.
Customer Satisfaction Surveys: These surveys may include questions about the customer’s experience, their overall satisfaction, and the likelihood they will recommend a product or service to others.
Pricing Studies: This type of research reveals how customers value their products or services. These surveys may include questions about the customer’s willingness to pay for the product, the customer’s perception of the price and value, and their comparison of the price to other similar items.
Product/Service Usage Studies: These surveys measure how customers use their products or services. They can include questions about how often customers use a product, their preferred features, and overall satisfaction.
Here’s an example of a typical survey we’ve used when testing out potential features with groups of clients. After they’ve had the chance to use the feature for a period, we send a short survey, then use the feedback to determine the viability of the feature for future release.
Employee Experience Surveys: Another great example of quantitative data in action, and one we use at Similarweb to measure employee satisfaction. Many online platforms are available to help you conduct them; here, we use Culture AMP . The ability to manipulate the data, spot patterns or trends, then identify the core successes and development areas are astounding.

Read a connected post that shows 18 ways to use market research surveys .
How to answer quantitative research questions with Similarweb
For the vast majority of applications I’ve covered in this post, there’s a more modern, quicker, and more efficient way to obtain similar insights online. Gone are the days when companies need to use expensive outdated data or pay hefty sums of money to market research firms to conduct broad studies to get the answers they need.
By this point, I hope you’ve seen how quick and easy it is to use Similarweb to do market research the modern way. But I’ve only scratched the surface of its capabilities.
Take two to watch this introductory video and see what else you can uncover.
Added bonus: Similarweb API
If you need to crunch large volumes of data and already use tools like Tableau or PowerBI, you can seamlessly connect Similarweb via the API and pipe in the data. So for faster analysis of big data, you can leverage Similarweb data to use alongside the visualization tools you already know and love.
Similarweb’s suite of market intelligence solutions offers unbiased, accurate, honest insights you can trust. With a world of data at your fingertips, use Similarweb Digital Research Intelligence to uncover telling facts that help inform your research and strengthen your position.
Use it for:
Market Research
Benchmarking
Audience Insights
Company Research
Consumer Journey Tracking
Wrapping up
Today’s markets change at lightning speed. To keep up and succeed, companies need access to insights and intel they can depend on to be timely and on-point. While quantitative market research questions can and should always be asked, it’s important to leverage technology to increase your speed to insight, and thus improve reaction times and response to market shifts.
What is quantitative market research?
Quantitative market research is a form of research that uses numerical data to gain insights into the behavior and preferences of customers. It is used to measure and track the performance of products, services, and campaigns.
How does quantitative market research help businesses?
Quantitative market research can help businesses identify customer trends, measure customer satisfaction, and develop effective marketing strategies. It can also provide valuable insights into customer behavior, preferences, and attitudes.
What types of questions should be included in a quantitative market research survey?
Questions in a quantitative market research survey should be focused, clear, and specific. Questions should be structured to collect quantitative data, such as numbers, percentages, or frequency of responses.
What methods can be used to collect quantitative market research data?
Common methods used to collect quantitative market research data include surveys, interviews, focus groups, polls, and online questionnaires.
What are the advantages and disadvantages of using quantitative market research?
The advantages of using quantitative market research include the ability to collect data quickly, the ability to analyze data in a structured way, and the ability to identify trends. Disadvantages include the potential for bias, the cost of collecting data, and the difficulty in interpreting results.
Related Posts

The Future of UK Finance: Top Trends to Watch in 2024

From AI to Buy: The Role of Artificial Intelligence in Retail

How to Conduct a Social Media Competitor Analysis: 5 Quick Steps

Industry Research: The Data-Backed Approach

How to Do a Competitive Analysis: A Complete Guide

How to Check Website Traffic: Analyzing the Digital Data
Wondering what similarweb can do for you.
Here are two ways you can get started with Similarweb today!
From clicks to consequences: a multi-method review of online grocery shopping
- Published: 23 October 2023
Cite this article
- Arvind Shroff ORCID: orcid.org/0000-0002-8544-5361 1 ,
- Satish Kumar ORCID: orcid.org/0000-0001-5200-1476 2 ,
- Luisa M. Martinez 3 , 4 &
- Nitesh Pandey 5
2747 Accesses
14 Altmetric
Explore all metrics
The academic interest in Online Grocery Shopping (OGS) has proliferated in retailing and business management over the past two decades. Previous research on OGS was primarily focused on consumer-level consequences such as purchase intention, purchase decision, and post-purchase behavior. However, there is a lack of literature integrating intrinsic and extrinsic factors that influence the growth of OGS and its impact on purchase outcomes. To address this, we conduct a multi-method review combining traits of a systematic literature review and bibliometric analysis. Analyzing 145 articles through word cloud and keyword co-occurrence analysis, we identify publication trends (top journals, articles) and nine thematic clusters. We develop an integrated conceptual framework encompassing the antecedents, mediators, moderators, and consequences of OGS. Finally, we outline future research directions using Theory-Context-Characteristics-Methods framework to serve as a reference point for future researchers working in OGS.
This is a preview of subscription content, log in via an institution to check access.
Access this article
Price includes VAT (Russian Federation)
Instant access to the full article PDF.
Rent this article via DeepDyve
Institutional subscriptions
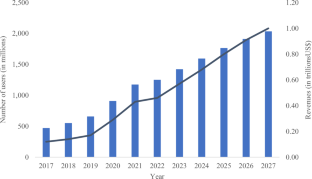
Statista Digital Market Insights, November 2022
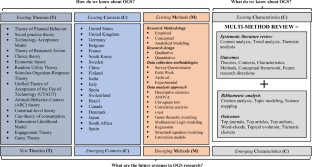
Similar content being viewed by others
Beyond Kirana Stores: A Study on Consumer Purchase Intention for Buying Grocery Online
Factors Influencing Consumers’ Online Grocery Shopping Under the New Normal
Online Shopping Motives - An Empirical Investigation of Consumer Buying Behavior in Germany’s Main Online Retail Segments
Bernstein, F., DeCroix, G. A., & Keskin, N. B. (2021). Competition between two-sided platforms under demand and supply congestion effects. Manufacturing and Service Operations Management, 23 (5), 1043–1061. https://doi.org/10.1287/msom.2020.0866
Article Google Scholar
Mody, M. A., Hanks, L., & Cheng, M. (2021). Sharing economy research in hospitality and tourism: A critical review using bibliometric analysis, content analysis and a quantitative systematic literature review. International Journal of Contemporary Hospitality Management, 33 (5), 1711–1745. https://doi.org/10.1108/IJCHM-12-2020-1457
Shroff, A., Shah, B. J., & Gajjar, H. (2022). Online food delivery research: A systematic literature review. International Journal of Contemporary Hospitality Management, 34 (8), 2852–2883. https://doi.org/10.1108/IJCHM-10-2021-1273
Jen-Hui Wang, R., Malthouse, E. C., & Krishnamurthi, L. (2015). On the go: How mobile shopping affects customer purchase behavior. Journal of Retailing, 91 (2), 217–234. https://doi.org/10.1016/j.jretai.2015.01.002
Hui, T. K., & Wan, D. (2009). Who are the online grocers? Service Industries Journal, 29 (11), 1479–1489. https://doi.org/10.1080/02642060902793334
Ramus, K., & Nielsen, N. A. (2005). Online grocery retailing: What do consumers think? Internet Research . https://doi.org/10.1108/10662240510602726
Anckar, B., Walden, P., & Jelassi, T. (2002). Creating customer value in online grocery shopping. International Journal of Retail and Distribution Management, 30 (4), 211–220. https://doi.org/10.1108/09590550210423681
Kaufman-Scarborough, C., & Lindquist, J. D. (2002). E-shopping in a multiple channel environment. Journal of Consumer Marketing, 19 (4), 333–350. https://doi.org/10.1108/07363760210433645
Shrivastava, A. (2021). Food-delivery commissions in India among the highest globally. The CapTable . Retrieved August 29, 2022, from https://the-captable.com/2021/05/food-delivery-commissions-india-among-highest-swiggy-zomato/ .
Hansen, T. (2005). Consumer adoption of online grocery buying: A discriminant analysis. International Journal of Retail and Distribution Management, 33 (2), 101–121. https://doi.org/10.1108/09590550510581449
Breugelmans, E., & Campo, K. (2016). Cross-channel effects of price promotions: An empirical analysis of the multi-channel grocery retail sector. Journal of Retailing, 92 , 333–351. https://doi.org/10.1016/j.jretai.2016.02.003
Gruntkowski, L. M., & Martinez, L. F. (2022). Online grocery shopping in Germany: Assessing the impact of COVID-19. Journal of Theoretical and Applied Electronic Commerce Research, 17 (3), 984–1002. https://doi.org/10.3390/jtaer17030050
Brüggemann, P., & Olbrich, R. (2022). The impact of COVID-19 pandemic restrictions on offline and online grocery shopping: New normal or old habits? Electronic Commerce Research . https://doi.org/10.1007/s10660-022-09658-1
Edible grocery global sales by channel 2021–2026|Statista. (2022). Retrieved May 24, 2023, from https://www.statista.com/statistics/1268769/global-edible-grocery-store-based-and-e-commerce-sales/ .
Global online food delivery market size 2027|Statista. (2023). Retrieved May 24, 2023, from https://www.statista.com/statistics/1170631/online-food-delivery-market-size-worldwide/ .
Online food delivery users worldwide 2017–2027|Statista. (2023). Retrieved May 24, 2023, from https://www.statista.com/forecasts/891088/online-food-delivery-users-by-segment-worldwide .
Martinez, L. F., Pauwels, K., & Brüggemann, P. (2023). Call for papers on online grocery shopping—current and future challenges and opportunities. Electronic Commerce Research . Retrieved May 24, 2023, from https://www.springer.com/journal/10660/updates/23919876 .
Paul, J., Lim, W. M., O’Cass, A., Hao, A. W., & Bresciani, S. (2021). Scientific procedures and rationales for systematic literature reviews (SPAR-4-SLR). International Journal of Consumer Studies, 45 (4), O1–O16. https://doi.org/10.1111/IJCS.12695
Singh, K., & Basu, R. (2023). Online consumer shopping behaviour: A review and research agenda. International Journal of Consumer Studies, 47 (3), 815–851. https://doi.org/10.1111/IJCS.12899
Donthu, N., Kumar, S., Mukherjee, D., Pandey, N., & Lim, W. M. (2021). How to conduct a bibliometric analysis: An overview and guidelines. Journal of Business Research, 133 , 285–296. https://doi.org/10.1016/j.jbusres.2021.04.070
Aria, M., & Cuccurullo, C. (2017). Bibliometrix: An R-tool for comprehensive science mapping analysis. Journal of Informetrics, 11 (4), 959–975. https://doi.org/10.1016/j.joi.2017.08.007
Kumar, S., Lim, W. M., Pandey, N., & Christopher Westland, J. (2021). 20 years of electronic commerce research. Electronic Commerce Research, 21 (1), 1–40. https://doi.org/10.1007/s10660-021-09464-1
Lim, W. M., Kumar, S., & Ali, F. (2022). Advancing knowledge through literature reviews: ‘what’, ‘why’, and ‘how to contribute.’ Service Industries Journal, 42 (7–8), 481–513. https://doi.org/10.1080/02642069.2022.2047941
Snyder, H. (2019). Literature review as a research methodology: An overview and guidelines. Journal of Business Research, 104 , 333–339. https://doi.org/10.1016/j.jbusres.2019.07.039
Kraus, S., Breier, M., Lim, W. M., Dabić, M., Kumar, S., Kanbach, D., & Ferreira, J. J. (2022). Literature reviews as independent studies: Guidelines for academic practice. Review of Managerial Science, 16 (8), 2577–2595. https://doi.org/10.1007/s11846-022-00588-8
Palmatier, R. W., Houston, M. B., & Hulland, J. (2018). Review articles: Purpose, process, and structure. Journal of the Academy of Marketing Science, 46 (1), 1–5. https://doi.org/10.1007/s11747-017-0563-4
Lim, W. M., Yap, S. F., & Makkar, M. (2021). Home sharing in marketing and tourism at a tipping point: What do we know, how do we know, and where should we be heading? Journal of Business Research, 122 , 534–566. https://doi.org/10.1016/J.JBUSRES.2020.08.051
Jebarajakirthy, C., Maseeh, H. I., Morshed, Z., Shankar, A., Arli, D., & Pentecost, R. (2021). Mobile advertising: A systematic literature review and future research agenda. International Journal of Consumer Studies, 45 (6), 1258–1291. https://doi.org/10.1111/ijcs.12728
Donthu, N., Kumar, S., Pandey, N., Pandey, N., & Mishra, A. (2021). Mapping the electronic word-of-mouth (eWOM) research: A systematic review and bibliometric analysis. Journal of Business Research, 135 (July), 758–773. https://doi.org/10.1016/j.jbusres.2021.07.015
Davis, R., Buchanan-Oliver, M., & Brodie, R. J. (2000). Retail service branding in electronic-commerce environments. Journal of Service Research, 3 (2), 178–186. https://doi.org/10.1177/109467050032006
Morganosky, M. A., & Cude, B. J. (2000). Consumer response to online grocery shopping. International Journal of Retail and Distribution Management, 28 (1), 17–26. https://doi.org/10.1108/09590550010306737
Brand, C., Schwanen, T., & Anable, J. (2020). Online omnivores or Willing but struggling? Identifying online grocery shopping behavior segments using attitude theory. Journal of Retailing and Consumer Services . https://doi.org/10.1016/J.JRETCONSER.2020.102195
Thomas-Francois, K., Jo, W. M., Somogyi, S., Li, Q., & Nixon, A. (2023). Virtual grocery shopping intention: an application of the model of goal-directed behaviour. British Food Journal . https://doi.org/10.1108/BFJ-06-2022-0510
Piroth, P., Ritter, M. S., & Rueger-Muck, E. (2020). Online grocery shopping adoption: Do personality traits matter? British Food Journal, 122 (3), 957–975. https://doi.org/10.1108/BFJ-08-2019-0631
Stenius, M., & Eriksson, N. (2022). What beliefs underlie decisions to buy groceries online? International Journal of Consumer Studies, 47 (3), 922–935. https://doi.org/10.1111/ijcs.12874
Hansson, L., Holmberg, U., Post, A., Hansson, L., & Holmberg, U. (2022). Reorganising grocery shopping practices—the case of elderly consumers. The International Review of Retail, Distribution and Consumer Research, 32 (4), 351–369. https://doi.org/10.1080/09593969.2022.2085137
Berg, J., & Henriksson, M. (2020). In search of the ‘good life’: Understanding online grocery shopping and everyday mobility as social practices. Journal of Transport Geography, 83 , 102633. https://doi.org/10.1016/J.JTRANGEO.2020.102633
Driediger, F., & Bhatiasevi, V. (2019). Online grocery shopping in Thailand: Consumer acceptance and usage behavior. Journal of Retailing and Consumer Services, 48 , 224–237. https://doi.org/10.1016/J.JRETCONSER.2019.02.005
Badenhop, A., & Frasquet, M. (2021). Online grocery shopping at multichannel supermarkets: the impact of retailer brand equity. Journal of Food Products Marketing, 27 (2), 89–104. https://doi.org/10.1080/10454446.2021.1894296
Hood, N., Urquhart, R., Newing, A., & Heppenstall, A. (2020). Sociodemographic and spatial disaggregation of e-commerce channel use in the grocery market in Great Britain. Journal of Retailing and Consumer Services, 55 , 102076. https://doi.org/10.1016/J.JRETCONSER.2020.102076
Shi, S. W., Zhang, J., & Smith, R. H. (2014). Usage experience with decision aids and evolution of online purchase behavior. Marketing Science, 33 (6), 871–882. https://doi.org/10.1287/mksc.2014.0872
Yokoyama, N., Azuma, N., & Kim, W. (2023). The impact of e-retail usage on relative retail patronage formation. International Journal of Retail and Distribution Management, 51 (13), 16–32. https://doi.org/10.1108/IJRDM-04-2022-0142
Ponte, D., & Sergi, D. (2023). E-grocery delivery channels: Acceptance of the click and collect solutions. Technology Analysis and Strategic Management . https://doi.org/10.1080/09537325.2022.2163890
Weinstein, A. T., Anti, K., & Ochoa, E. (2022). World’s biggest retailer launches Walmart Plus and customers have their say. Journal of Business Strategy, 43 (6), 381–390. https://doi.org/10.1108/JBS-07-2021-0133
Bezirgani, A., & Lachapelle, U. (2021). Online grocery shopping for the elderly in Quebec, Canada: The role of mobility impediments and past online shopping experience. Travel Behaviour and Society, 25 , 133–143. https://doi.org/10.1016/J.TBS.2021.07.001
Campbell, J. M., & Fairhurst, A. (2014). Billion dollar baby: Local foods and U.S. grocery. Journal of Food Products Marketing, 20 (3), 215–228. https://doi.org/10.1080/10454446.2012.728985
De Kervenoael, R., Soopramanien, D., Hallsworth, A., & Elms, J. (2007). Personal privacy as a positive experience of shopping an illustration through the case of online grocery shopping. International Journal of Retail and Distribution Management, 35 (7), 583–599. https://doi.org/10.1108/09590550710755958
Burningham, K., Venn, S., Christie, I., Jackson, T., & Gatersleben, B. (2014). New motherhood: A moment of change in everyday shopping practices? Young Consumers, 15 (3), 211–226. https://doi.org/10.1108/YC-11-2013-00411
Loketkrawee, P., & Bhatiasevi, V. (2018). Elucidating the behavior of consumers toward online grocery shopping: The role of shopping orientation. Journal of Internet Commerce, 17 (4), 418–445. https://doi.org/10.1080/15332861.2018.1496390
Khan, A., & Khan, S. (2022). Purchasing grocery online in a nonmetro city: Investigating the role of convenience, security, and variety. Journal of Public Affairs, 22 (2), e2497. https://doi.org/10.1002/PA.2497
Goethals, F., Leclercq-Vandelannoitte, A., & Tütüncü, Y. (2012). French consumers’ perceptions of the unattended delivery model for e-grocery retailing. Journal of Retailing and Consumer Services, 19 (1), 133–139. https://doi.org/10.1016/j.jretconser.2011.11.002
Cervellon, M. C., Sylvie, J., & Ngobo, P. V. (2015). Shopping orientations as antecedents to channel choice in the French grocery multichannel landscape. Journal of Retailing and Consumer Services, 27 , 31–51. https://doi.org/10.1016/J.JRETCONSER.2015.06.008
Hansen, T. (2006). Determinants of consumers’ repeat online buying of groceries. International Review of Retail, Distribution and Consumer Research, 16 (1), 93–114. https://doi.org/10.1080/09593960500453617
Huyghe, E., Verstraeten, J., Geuens, M., & Van Kerckhove, A. (2017). Clicks as a healthy alternative to bricks: How online grocery shopping reduces vice purchases. Journal of Marketing Research, 54 (1), 61–74. https://doi.org/10.1509/jmr.14.0490
Cebollada, J., Chu, Y., & Jiang, Z. (2019). Online category pricing at a multichannel grocery retailer. Journal of Interactive Marketing, 46 , 52–69. https://doi.org/10.1016/j.intmar.2018.12.004
Chu, J., Chintagunta, P., & Cebollada, J. (2008). Research note-A comparison of within-household price sensitivity across online and offline channels. Marketing Science, 27 (2), 283–299. https://doi.org/10.1287/mksc.1070.0288
Ayadi, K., & Muratore, I. (2020). Digimums’ online grocery shopping: The end of children’s influence? International Journal of Retail and Distribution Management, 48 (4), 348–362. https://doi.org/10.1108/IJRDM-09-2019-0291
Elms, J., & Tinson, J. (2012). Consumer vulnerability and the transformative potential of Internet shopping: An exploratory case study. Journal of Marketing Management, 28 (11–12), 1354–1376. https://doi.org/10.1080/0267257X.2012.691526
Rossolov, A. (2021). A last-mile delivery channel choice by E-shoppers: Assessing the potential demand for automated parcel lockers. International Journal of Logistics Research and Applications . https://doi.org/10.1080/13675567.2021.2005004
Maltese, I., Le Pira, M., Marcucci, E., Gatta, V., & Evangelinos, C. (2021). Grocery or @grocery: A stated preference investigation in Rome and Milan. Research in Transportation Economics, 87 , 101096. https://doi.org/10.1016/J.RETREC.2021.101096
Kolesova, S., & Singh, R. (2019). One Vs. Many: who wins? An empirical investigation of online product display. International Review of Retail, Distribution and Consumer Research, 29 (3), 285–305. https://doi.org/10.1080/09593969.2019.1598465
Li, J., Hallsworth, A. G., & Coca-Stefaniak, J. A. (2020). Changing grocery shopping behaviours among chinese consumers at the outset of the COVID-19 outbreak. Tijdschrift voor economische en sociale geografie, 111 (3), 574–583. https://doi.org/10.1111/TESG.12420
Singh, R., & Söderlund, M. (2022). There is no place like home: Home satisfaction and customer satisfaction in online grocery retailing. International Review of Retail, Distribution and Consumer Research, 32 (4), 370–387. https://doi.org/10.1080/09593969.2022.2073555
Motte-Baumvol, B., Belton Chevallier, L., & Bonin, O. (2022). Does e-grocery shopping reduce CO 2 emissions for working couples’ travel in England? International Journal of Sustainable Transportation, 17 (5), 515–526. https://doi.org/10.1080/15568318.2022.2074326
Van Hove, L. (2022). Consumer characteristics and e-grocery services: The primacy of the primary shopper. Electronic Commerce Research, 22 (2), 241–266. https://doi.org/10.1007/s10660-022-09551-x
Dayarian, I., & Pazour, J. (2022). Crowdsourced order-fulfillment policies using in-store customers. Production and Operations Management, 31 (11), 4075–4094. https://doi.org/10.1111/poms.13805
Samudio Lezcano, M., Harper, C. D., Nock, D., Lowry, G. V., & Michalek, J. J. (2023). Online grocery delivery: Sustainable practice, or congestion generator and environmental burden? Transportation Research Part D: Transport and Environment, 119 (March), 103722. https://doi.org/10.1016/j.trd.2023.103722
Neumayr, L., & Moosauer, C. (2021). How to induce sales of sustainable and organic food: The case of a traffic light eco-label in online grocery shopping. Journal of Cleaner Production, 328 , 129584. https://doi.org/10.1016/J.JCLEPRO.2021.129584
Lim, B., Xie, Y., & Haruvy, E. (2022). The impact of mobile app adoption on physical and online channels. Journal of Retailing, 98 (3), 453–470. https://doi.org/10.1016/J.JRETAI.2021.10.001
Pan, F., Pan, S., Zhou, W., & Fan, T. (2022). Perishable product bundling with logistics uncertainty: Solution based on physical internet. International Journal of Production Economics, 244 , 108386. https://doi.org/10.1016/J.IJPE.2021.108386
Hand, C., Riley, F. D. O., Harris, P., Singh, J., & Rettie, R. (2009). Online grocery shopping: The influence of situational factors. European Journal of Marketing, 43 (9), 1205–1219. https://doi.org/10.1108/03090560910976447
McNeill, L. S. (2006). The influence of culture on retail sales promotion use in Chinese supermarkets. Australasian Marketing Journal, 14 (2), 34–46. https://doi.org/10.1016/S1441-3582(06)70059-3
McClatchey, J., Cattell, K., & Michell, K. (2007). The impact of online retail grocery shopping on retail space: A Cape Town case study. Facilities, 25 (3–4), 115–126. https://doi.org/10.1108/02632770710729700
Itani, O. S., & Hollebeek, L. D. (2020). Consumers’ health-locus-of-control and social distancing in pandemic-based e-tailing services. Journal of Services Marketing, 35 (8), 1073–1091. https://doi.org/10.1108/JSM-10-2020-0410
Handayani, P. W., Nurahmawati, R. A., Pinem, A. A., & Azzahro, F. (2020). Switching intention from traditional to online groceries using the moderating effect of gender in Indonesia. Journal of Food Products Marketing, 26 (6), 425–439. https://doi.org/10.1080/10454446.2020.1792023
Van Droogenbroeck, E., & Van Hove, L. (2020). Intra-household task allocation in online grocery shopping: Together alone. Journal of Retailing and Consumer Services . https://doi.org/10.1016/J.JRETCONSER.2020.102153
Anshu, K., Gaur, L., & Singh, G. (2022). Impact of customer experience on attitude and repurchase intention in online grocery retailing: A moderation mechanism of value Co-creation. Journal of Retailing and Consumer Services, 64 , 102798. https://doi.org/10.1016/J.JRETCONSER.2021.102798
Suher, J., Huang, S. C., & Lee, L. (2019). Planning for multiple shopping goals in the marketplace. Journal of Consumer Psychology, 29 (4), 642–651. https://doi.org/10.1002/JCPY.1130
Mirhoseini, M., Pagé, S. A., Léger, P. M., & Sénécal, S. (2021). What deters online grocery shopping? Investigating the effect of arithmetic complexity and product type on user satisfaction. Journal of Theoretical and Applied Electronic Commerce Research, 16 (4), 1–18. https://doi.org/10.3390/jtaer16040047
Wang, K., Gao, Y., Liu, Y., & Nurul Habib, K. (2023). Exploring the choice between in-store versus online grocery shopping through an application of semi-compensatory independent availability logit (SCIAL) model with latent variables. Journal of Retailing and Consumer Services, 71 , 103191. https://doi.org/10.1016/J.JRETCONSER.2022.103191
Hillen, J., & Fedoseeva, S. (2021). E-commerce and the end of price rigidity? Journal of Business Research, 125 , 63–73. https://doi.org/10.1016/J.JBUSRES.2020.11.052
Chintagunta, P. K., Chu, J., & Cebollada, J. (2012). Quantifying transaction costs in online/off-line grocery channel choice. Marketing Science, 31 (1), 96–114. https://doi.org/10.1287/mksc.1110.0678
Breugelmans, E., & Campo, K. (2016). Cross-channel effects of price promotions: An empirical analysis of the multi-channel grocery retail sector. Journal of Retailing, 92 (3), 333–351. https://doi.org/10.1016/j.jretai.2016.02.003
Kumar, A., Chakraborty, S., & Bala, P. K. (2023). Text mining approach to explore determinants of grocery mobile app satisfaction using online customer reviews. Journal of Retailing and Consumer Services, 73 , 103363. https://doi.org/10.1016/j.jretconser.2023.103363
Belavina, E., Girotra, K., & Kabra, A. (2017). Online grocery retail: Revenue models and environmental impact. Management Science, 63 (6), 1781–1799. https://doi.org/10.1287/mnsc.2016.2430
Bjørgen, A., Bjerkan, K. Y., & Hjelkrem, O. A. (2021). E-groceries: Sustainable last mile distribution in city planning. Research in Transportation Economics, 87 , 100805. https://doi.org/10.1016/J.RETREC.2019.100805
Melis, K., Campo, K., Breugelmans, E., & Lamey, L. (2015). The impact of the multi-channel retail mix on online store choice: Does online experience matter? Journal of Retailing, 91 (2), 272–288. https://doi.org/10.1016/J.JRETAI.2014.12.004
Zissis, D., Aktas, E., & Bourlakis, M. (2018). Collaboration in urban distribution of online grocery orders. International Journal of Logistics Management, 29 (4), 1196–1214. https://doi.org/10.1108/IJLM-11-2017-0303
Harris-lagoudakis, K. (2022). Online shopping and the healthfulness of grocery purchases. American Journal of Agricultural Economics, 104 (3), 1050–1076. https://doi.org/10.1111/ajae.12262
Kim, H. (2021). Use of mobile grocery shopping application: Motivation and decision-making process among South Korean consumers. Journal of Theoretical and Applied Electronic Commerce Research, 16 (7), 2672–2693. https://doi.org/10.3390/JTAER16070147
Suel, E., & Polak, J. W. (2017). Development of joint models for channel, store, and travel mode choice: Grocery shopping in London. Transportation Research Part A: Policy and Practice, 99 , 147–162. https://doi.org/10.1016/J.TRA.2017.03.009
Singh, R., & Söderlund, M. (2020). Extending the experience construct: An examination of online grocery shopping. European Journal of Marketing, 54 (10), 2419–2446. https://doi.org/10.1108/EJM-06-2019-0536
Gielens, K., Gijsbrechts, E., & Geyskens, I. (2021). Navigating the last mile: The demand effects of click-and-collect order fulfillment. Journal of Marketing, 85 (4), 158–178. https://doi.org/10.1177/0022242920960430
Mortimer, G., Fazal e Hasan, S., Andrews, L., & Martin, J. (2016). Online grocery shopping: The impact of shopping frequency on perceived risk. International Review of Retail, Distribution and Consumer Research, 26 (2), 202–223. https://doi.org/10.1080/09593969.2015.1130737
Wang, X. C., Kim, W., Holguín-Veras, J., & Schmid, J. (2021). Adoption of delivery services in light of the COVID pandemic: Who and how long? Transportation Research Part A: Policy and Practice, 154 , 270–286. https://doi.org/10.1016/J.TRA.2021.10.012
Hansen, T. (2008). Consumer values, the theory of planned behaviour and online grocery shopping. International Journal of Consumer Studies, 32 (2), 128–137. https://doi.org/10.1111/J.1470-6431.2007.00655.X
Delasay, M., Jain, A., & Kumar, S. (2022). Impacts of the COVID-19 pandemic on grocery retail operations: An analytical model. Production and Operations Management, 31 (5), 2237–2255. https://doi.org/10.1111/POMS.13717
Van Droogenbroeck, E., & Van Hove, L. (2020). Triggered or evaluated? A qualitative inquiry into the decision to start using e-grocery services. International Review of Retail, Distribution and Consumer Research, 30 (2), 103–122. https://doi.org/10.1080/09593969.2019.1655085
Sousa, R., Horta, C., Ribeiro, R., & Rabinovich, E. (2020). How to serve online consumers in rural markets: Evidence-based recommendations. Business Horizons, 63 (3), 351–362. https://doi.org/10.1016/J.BUSHOR.2020.01.007
Van Droogenbroeck, E., & Van Hove, L. (2020). Intra-household task allocation in online grocery shopping: Together alone. Journal of Retailing and Consumer Services, 56 , 102153. https://doi.org/10.1016/j.jretconser.2020.102153
Dawes, J., & Nenycz-Thiel, M. (2014). Comparing retailer purchase patterns and brand metrics for in-store and online grocery purchasing. Journal of Marketing Management, 30 (3–4), 364–382. https://doi.org/10.1080/0267257X.2013.813576
Dias, F. F., Lavieri, P. S., Sharda, S., Khoeini, S., Bhat, C. R., Pendyala, R. M., & Srinivasan, K. K. (2020). A comparison of online and in-person activity engagement: The case of shopping and eating meals. Transportation Research Part C: Emerging Technologies, 114 , 643–656. https://doi.org/10.1016/J.TRC.2020.02.023
van Ewijk, B. J., Steenkamp, J. B. E. M., & Gijsbrechts, E. (2020). The rise of online grocery shopping in China: Which brands will benefit? Journal of International Marketing, 28 (2), 20–39. https://doi.org/10.1177/1069031X20914265
Shroff, A., Shah, B. J., & Gajjar, H. (2021). Shelf space allocation game with private brands: A profit-sharing perspective. Journal of Revenue and Pricing Management, 20 (2), 116–133. https://doi.org/10.1057/s41272-021-00295-1
Harris, P., Dall’Olmo Riley, F., Riley, D., & Hand, C. (2017). Online and store patronage: A typology of grocery shoppers. International Journal of Retail and Distribution Management, 45 (4), 419–445. https://doi.org/10.1108/IJRDM-06-2016-0103
Aagja, J. P., Mammen, T., & Saraswat, A. (2011). Validating service convenience scale and profiling customers: A study in the indian retail context. Vikalpa, 36 (4), 25–49. https://doi.org/10.1177/0256090920110403
Lim, H., Widdows, R., & Hooker, N. H. (2009). Web content analysis of e-grocery retailers: A longitudinal study. International Journal of Retail and Distribution Management, 37 (10), 839–851. https://doi.org/10.1108/09590550910988020
Hallikainen, H., Luongo, M., Dhir, A., & Laukkanen, T. (2022). Consequences of personalized product recommendations and price promotions in online grocery shopping. Journal of Retailing and Consumer Services, 69 (July), 103088. https://doi.org/10.1016/j.jretconser.2022.103088
de Bellis, E., & Venkataramani Johar, G. (2020). Autonomous shopping systems: Identifying and overcoming barriers to consumer adoption. Journal of Retailing, 96 (1), 74–87. https://doi.org/10.1016/J.JRETAI.2019.12.004
Xu, L., & Saphores, J. D. (2022). Grocery shopping in California and COVID-19: Transportation, environmental justice, and policy implications. Transportation Research Part D: Transport and Environment, 113 , 103537. https://doi.org/10.1016/J.TRD.2022.103537
Blitstein, J. L., Frentz, F., & Jilcott Pitts, S. B. (2020). A mixed-method examination of reported benefits of online grocery shopping in the United States and Germany: Is health a factor? Journal of Food Products Marketing, 26 (3), 212–224. https://doi.org/10.1080/10454446.2020.1754313
Singh, R. (2019). Why do online grocery shoppers switch or stay? An exploratory analysis of consumers’ response to online grocery shopping experience. International Journal of Retail and Distribution Management, 47 (12), 1300–1317. https://doi.org/10.1108/IJRDM-10-2018-0224
Seitz, C., Pokrivčák, J., Tóth, M., & Plevný, M. (2017). Online grocery retailing in Germany: An explorative analysis. Journal of Business Economics and Management, 18 (6), 1243–1263. https://doi.org/10.3846/16111699.2017.1410218
Fedoseeva, S., Herrmann, R., & Nickolaus, K. (2017). Was the economics of information approach wrong all the way? Evidence from German grocery r(E)tailing. Journal of Business Research, 80 , 63–72. https://doi.org/10.1016/J.JBUSRES.2017.07.006
Van Droogenbroeck, E., & Van Hove, L. (2017). Adoption of online grocery shopping: Personal or household characteristics? Journal of Internet Commerce, 16 (3), 255–286. https://doi.org/10.1080/15332861.2017.1317149
Ruggeri, G., Orsi, L., & Corsi, S. (2019). A bibliometric analysis of the scientific literature on Fairtrade labelling. International Journal of Consumer Studies, 43 (2), 134–152. https://doi.org/10.1111/ijcs.12492
Aguinis, H., Gottfredson, R. K., & Wright, T. A. (2011). Best-practice recommendations for estimating interaction effects using meta-analysis. Journal of Organizational Behavior, 32 (8), 1033–1043. https://doi.org/10.1002/JOB.719
Download references
Acknowledgements
This study was partially funded by UNIDCOM under a Grant by the Fundação para a Ciência e a Tecnologia (UIDB/DES/00711/2020) attributed to UNIDCOM/IADE – Unidade de Investigação em Design e Comunicação, Lisbon, Portugal.
Author information
Authors and affiliations.
Indian Institute of Management Lucknow, Lucknow, India
Arvind Shroff
Indian Institute of Management Nagpur, Nagpur, India
Satish Kumar
Instituto Português de Administração de Marketing – IPAM Lisboa, Lisbon, Portugal
Luisa M. Martinez
UNIDCOM/IADE, Unidade de Investigação em Design e Comunicação, Lisbon, Portugal
Amrita School of Business, Coimbatore, India
Nitesh Pandey
You can also search for this author in PubMed Google Scholar
Corresponding author
Correspondence to Satish Kumar .
Ethics declarations
Conflict of interest.
On behalf of all authors, the corresponding author states that there is no conflict of interest.
Supplementary Information
Below is the link to the electronic supplementary material.
Supplementary file1 (DOCX 45 KB)
Rights and permissions.
Springer Nature or its licensor (e.g. a society or other partner) holds exclusive rights to this article under a publishing agreement with the author(s) or other rightsholder(s); author self-archiving of the accepted manuscript version of this article is solely governed by the terms of such publishing agreement and applicable law.
Reprints and permissions
About this article
Shroff, A., Kumar, S., Martinez, L.M. et al. From clicks to consequences: a multi-method review of online grocery shopping. Electron Commer Res (2023). https://doi.org/10.1007/s10660-023-09761-x
Download citation
Accepted : 10 September 2023
Published : 23 October 2023
DOI : https://doi.org/10.1007/s10660-023-09761-x
Share this article
Anyone you share the following link with will be able to read this content:
Sorry, a shareable link is not currently available for this article.
Provided by the Springer Nature SharedIt content-sharing initiative
- Online grocery shopping
- Systematic literature review
- Bibliometric analysis
- Co-occurrence analysis
- Conceptual framework
- Find a journal
- Publish with us
- Track your research

An official website of the United States government
The .gov means it’s official. Federal government websites often end in .gov or .mil. Before sharing sensitive information, make sure you’re on a federal government site.
The site is secure. The https:// ensures that you are connecting to the official website and that any information you provide is encrypted and transmitted securely.
- Publications
- Account settings
Preview improvements coming to the PMC website in October 2024. Learn More or Try it out now .
- Advanced Search
- Journal List
- v.29; 2020 Apr
Survey data on students’ online shopping behaviour: A focus on selected university students in Indonesia
Associated data.
The data presented in this paper is used to examine the factors influencing students' online shopping behaviour and to identify the students' segmentation on the important factors. The survey was conducted in the Institut Teknologi Sepuluh Nopember (ITS) Surabaya, the biggest science and technology university in East Indonesia, with multicultural and diverse socio-economic students' backgrounds. The total number of population is 20448 students. Using Yamane's formula, a sample size of 393 students was surveyed online, and 83 of them experienced doing online shopping. A quantitative method with a descriptive research design was adopted to explore insights in the data related to the objective of the research. The survey data were analyzed by linear regression and hierarchical clustering. The conceptual framework of the variables are given, and reliability and validity have been confirmed. Data were analyzed with MINITAB and SPSS software.
Specifications Table
As shown in Table 1 , the survey was administered to 393 students representing the sample size used in the selected university (ITS). Among these numbers, 83 (21%) of them indicated that they have experience in doing online shopping. Meanwhile 310 (79%) respondents had no experience with online shopping. The analyzed data in this paper involved only information collected from respondents with experience of doing online shopping.
Analysis of general response dealing with experience in online shopping.
Table 2 shows the distribution of the respondents based on their semester level. We see that the majority (86.8%) of the respondents were in the 5th and 7th semester. The rest (13.2%) were students in the 1st, 3rd and 4th semester.
Descriptive statistics on respondents’ semester level.
Table 3 describes the respondents’ responses from those 83 students, where 41% of them are male students and 59% are female students, as shown in Fig. 1 . It basically shows that female students did shopping more than male students.
Percentage distibution of gender of the surveyed students.

Dendogram of hierarchical clustering.
Table 4 shows that most (58%) of the respondents did online shopping an average of one time in a month, 30% did online shopping two times in a month and the rest (12%) did more than two transactions per month.
Average frequency of online shopping within a month.
Table 5 presents the statistics of the respondents in more detail based on gender. We see that female students did shopping more than male students did. Based on the favourite online marketplace, the male students choose Tokopedia, Lazada and Bukalapak while female students mostly shopped at Sophee.
Descriptive statistics based on gender.
Table 5 also revealed that the students spent mostly about 100000 IDR to 200000 IDR (the current exchange rate is 1 USD equivalent to about 14500 IDR). The male students mostly bought electronics (29%) while female students mostly purchased fashion (63%).
Based on the dataset, we can perform cluster analysis to identify the segmentation of the students. Fig. 1 depicts a dendogram created by using complete linkage with Euclidean distance measure. It provides cluster members depending on the number of clusters. Fig. 2 shows of boxplots of the segments assuming that we perform three clusters. In most cases, cluster 1 and cluster 2 relatively have similar characteristics (see also Table 6 ). Therefore, there might be only two clusters of students with significantly different characterstics. This fact is supported by the summary statistics in Table 3 .

Boxplot of cluster characteristics for each attribute.
Descriptive statistics of each cluster in each attribute.
Table 7 presents the output of multiple linear regression analysis to investigate the factors influencing the online shopping behaviour. The hypothesis to be tested is as follow:
Anaysis of variance.
Ho: There are no variables influencing online shopping behaviour.
We see that the ANOVA produces P-value of the regression = 0.000, which is less than 0.05 significant level. This leads to the rejection of the null hypothesis, meaning that at least one of the predictors significantly influences the purchasing behaviour. The R-square is 47.26%, meaning that the predictors have an effect of 47.26% on onine shopping behaviour.
The coefficients in Table 8 show the individual effect of each variable. We see that the P-values of POR, EJY, SIF and OAD are less than 0.05 significant level. This means that the purchasing behaviour is significantly infuenced by the perception of risk (POR), enjoyment (EJY), social influence (SIF) and online advertisment (OAD). Meamwhile, two other variables, i.e. trust and security (TAS) and quality of website (QOW), did not significantly influence the online shopping behaviour (see Table 9 ).
R-square of the regression.
Coefficients of the regression.
2. Experimental design, materials, and methods
Institut Teknologi Sepuluh Nopember (ITS) was selected in East Java, Indonesia. The total number of students is 20448 students. Using Yamane's formula of Yamane [ 2 ] with 95% confidence level, three hundred and ninety-three students were selected as the respondents. The students were selected randomly by sampling the student registration number, assuming that the students are homogeneous on their perception and understanding about online shopping behaviour. Furthermore, the students were asked to fill in the online questionnaire through the provided link. The data presented in this paper is focused only on the students who experienced online shopping. Among those 393 students, there were 83 students who did online shopping. The research was conducted according to and complies with all regulations established in the ethical guidelines by the ITS Research Ethics Committee in the “code of ethics”. All participants provided written informed consent.
The questionnaire was made following the conceptual framework of Moshref et al. [ 3 ], as can be seen in Fig. 3 . The questionnaire comprises students characteristics and their perceptions on online shopping behaviour with a Likert scale (strongly disagree (1) – strongly agree (5)). The perception variables were measured for online shopping behaviour (OSB) as the response and six predictiors, i.e. perception of risk (POR), trust and security (TAS), enjoyment (EJY), quality of website (QOW), online advertisment (OAD), and social influence (SIF). The list of questions (indicators) for each variable can be seen in the labels of the SPSS file for the corresponding variable. Mean of each perception variables are given in the data for the sake of building regression model. Validity and reliability of the data are confirmed by the test, as can be seen in Table 10 and Table 11 , respectively. All reliability indicators are greater than 0.5 indicating that the data is reliable.

Conceptual framework of the variables.
Validity test.
Reliability test.
3. Policy implications
The data revealed that the students’ online shopping behaviour is significantly influenced by the perception of risk (POR), enjoyment (EJY), social influence (SIF) and online advertisment (OAD). Considering the fact that students are a potential market, the online marketplace should put more focus on those variables. Market segmentation is also important to formulate an efficient marketing strategy. To this end, the data presented in this article is useful for further comprehensive analysis.
Acknowledgments
This research is partially funded by the Indonesian Ministry of Research and Technology, and Indonesian Ministry of Education and Culture under World Class University (WCU) Program managed by Institut Teknologi bandung.
Supplementary data to this article can be found online at https://doi.org/10.1016/j.dib.2019.105073 .
Conflict of interest
The authors declare that they have no known competing financial interests or personal relationships that could have appeared to influence the work reported in this paper.
Appendix A. Supplementary data
The following are the Supplementary data to this article:
Academia.edu no longer supports Internet Explorer.
To browse Academia.edu and the wider internet faster and more securely, please take a few seconds to upgrade your browser .
Enter the email address you signed up with and we'll email you a reset link.
- We're Hiring!
- Help Center
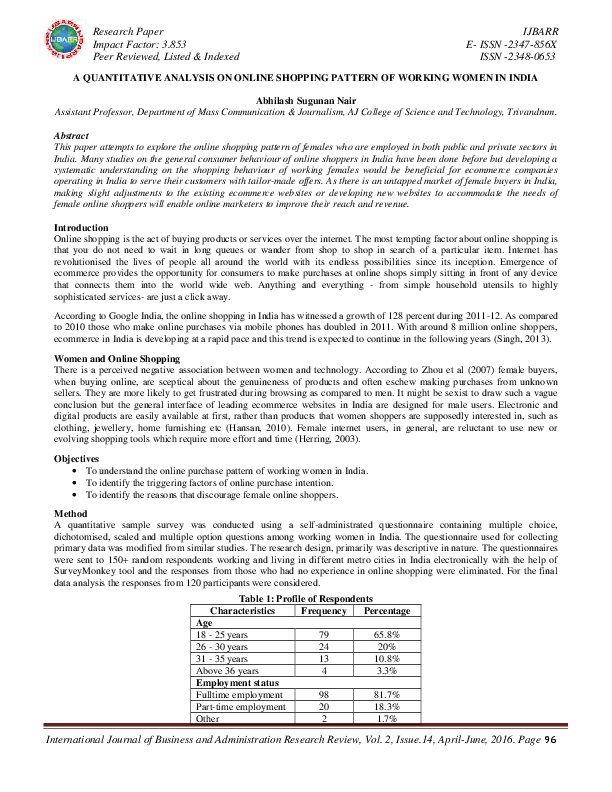
A QUANTITATIVE ANALYSIS ON ONLINE SHOPPING PATTERN OF WORKING WOMEN IN INDIA

This paper attempts to explore the online shopping pattern of females who are employed in both public and private sectors in India. Many studies on the general consumer behaviour of online shoppers in India have been done before but developing a systematic understanding on the shopping behaviour of working females would be beneficial for ecommerce companies operating in India to serve their customers with tailor-made offers. As there is an untapped market of female buyers in India, making slight adjustments to the existing ecommerce websites or developing new websites to accommodate the needs of female online shoppers will enable online marketers to improve their reach and revenue.
Related Papers
Global Online shopping-Highly Acknowledged`Current Era Habitòf internet users. This empirical study investigates the reasons for online consumer`s non buyer behaviour. `The Show stopper`can be, the retailer`s own Virtual cart itself. High frequency of non-buyer behaviour has putoff lights on Online marker`s face, as it delays the ROI radically. 88% of online shoppers that is, out of 10 online shoppers 7 individuals abandone the virtual cart. This is critical for this matured state of the technology which has been introduced since 2 decades. The purpose of this study is to find out the dominant factors influencing Virtual Cart Abandonment, confined to Virtual Shopping Cart(VSC) interface and its navigation system. A frame work was created with Structural Equation Model (SEM) with new buyer perspectives, To measure the affinity towards Conventional Shopping Behavior, The study findings show that Information Architecture was negatively related in cart abandonment behavior. Correspondingly other psycological attitudes are also studied to comeup with practical implications and inferences. Introduction Marketers are investing on VSC Development and unable to control Online shopper`s behavioral intention on Virtual shopping Cart Abandonment. Industry reports (Forrester) says that 88% of online shoppers had abandoned their cart after selecting their favorite products. There can be number of possible distractions from the Virtual shopping cart interface module itself, due to complex information, as well as from the Indian shopper`s belief system and attitudes. Though retailer can fetch product relevant demands and the profile of its target consumers with this abandoned cart data, It is not reliable to make assumptions for any future guaranteed sale. Despite comfort, and discounts 88% of online shoppers says that they they have abandoned VSC this is same as five years ago, which shows that retailers are still inside the puzzle to find a way. Indian shoppers are likely to spend almost 85% more on global online marketplaces in 2017 across categories, say a report jointly published by PayPal and Ipsos. According to Morgan Stanley Research report (2015) India is the second largest in terms of Internet users, counting 277 Million a 50% increase from the previous year, which probably contributed to a steep growth in the gross merchandise value for Online retailers. Google has predicted that women will increase their share of online shopping, as their contribution will grow by 5 times in the next 4 years (Track.in). Per capita incomes are likely to double by 2025 in India and this should drive higher aspirations of online shopping among the Indian consumers. Exposure to social media and other group chats applications share information about P&S before release, Social networking sites integration with shopping cart also bring in Consumer engagement to share shopping experiences (Forsythe et al., 2010), Also discussions around price advantages, Value for money, new features are analyzed and published as reviews. A recent study on the 'State of Ecommerce' in India conducted by ASSOCHAM and Comcast has unearthed several interesting aspects of Internet usage in the country, and the penetration of retail shopping using digital medium. Adoption of eCommerce is much faster in Tier 2 cities as compared to Tier 1. Virtual Shopping Cart can be an Effective-Technology Enabled Decision Aid (tool). However, marketers are losing money on increasing bounce rate (Cart Abandonment) by online Shoppers. This study will focus to reduce the earlier studied constructs with new perspective and report the dominant factors which will help the marketer to sustain the competition.
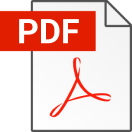
NEETU SINGH
This study aims to find out how and to what extent outlets incorporate visual merchandising that appeals to the customers and lead to a potential purchase. Retail business in India is booming and there is a fierce competition between the retailers. Fashion retailers cannot always depend on the Price, Place, Promotion, and Product cannot always win. An empirical study is aimed to find out the impact of visual merchandising on customer preference analysis in malls in Delhi. The study was based on a primary data and which was done through a questionnaire. The survey data was collected from 100 respondents in retail brands and 60 respondents in Luxury brands between the ages of 18 – 60 years in DLF Emporio, DLF Promenade mall, Ambience Mall Delhi. The dimension of visual merchandising i.e. window display, instore mannequin, floor merchandising promotional signage, proper lighting and good music in stores are an important visual merchandising element. These elements are studied and results reveal the importance of visual merchandising in fashion stores and its comparison in visual merchandising between retail and luxury brands.
UNANZA gulzar
The e-commerce industry in India has seen unprecedented growth in last few years. Eyeing India's substantial e-retail opportunity across multiple segments, investors have been aggressively funding the e-commerce sector. This growth has been fuelled by rapid adoption of technology, improving standards of living, an increasing young population , and economically advancing middle class, besides increasing access to the Internet through broadband and use of smartphones and tablets. The entry of global e-commerce giants has intensified the competition for home-grown players. E-retailers use web atmospherics to differentiate themselves from their competitors and evoke positive cognitive and emotional states of online consumers. However, though this Indian online market is growing at an exponential rate, it is still unexplored in terms of its shopping behaviour. Using structural equation modelling, this study applies the concept of the stimulus– organism–response to explain Indian buyers' online shopping behaviour, besides examining the importance of design elements in enabling website satisfaction (WS). Using a survey method to test the research model, primary data were collected from five Indian metropolitan cities of Delhi, Mumbai, Kolkata, Bengaluru, and Hyderabad during the months of May and June 2015. Confirmatory factory analysis (CFA) was used to estimate the measurement model with respect to convergent and discriminant validities. This was followed by testing the structural model framework and research hypotheses. Findings suggest that both internal and external elements have direct influence on WS. As the mediating variable, WS affects purchase intention. This research highlights on why and how 'satisfaction with website' matters in the contribution of shopping values and website atmospherics to behavioural outcomes by presenting its mediating role.
IJAR Indexing
Consumer behaviors are influenced by different factors such as culture, social class, relation, family, salary level and salary independency, age, gender etc. And so they show different customer behaviors. On-line shopping is a recent phenomenon in the field of E-Business. Most of the companies are selling their products/services on-line through online portals. Though online shopping is very common outside India, its growth in Indian Market, is still not in line with the global market. Companies are using the internet to put across and communicate the information. The main objective is to understand the behavior of consumers on online shopping in India. The results of study reveal that on-line shopping in India is affected by various factors like age, gender, marital status, family size and income. The results of the study could be further used by the researchers and practitioners for conducting future studies in the similar area.
TJPRC Publication
This study titled “A Study on Customer Satisfaction towards Online Shopping in Chennai City” has been undertaken to understand the factors influencing customers’ online shopping decisions and how these factors affect customer satisfaction. Descriptive Research Design has been used for this study. A well-structured questionnaire was designed and administered to collect samples across Chennai. Due to the need for a variety of respondents who have had previous experience with online shopping, judgment sampling technique was chosen. The study has been undertaken with reference to a sample size of 105 respondents. After collecting the data, the data was classified, tabulated and codified. Necessary statistical tools such as Percentage Analysis and Chi Square tests have been used to interpret the data. The results of this study may be of great use to businesses which are looking to expand into or venture into the online shopping environment.
Vivek Pandey
The rapid development of the internet has strongly impact upon the worldwide marketing environment. Currently it has become one of the popular approaches for business and customer to perform trade over the internet. Businesses have been coming up with creative ways to promote their product via online. Thus it describes how modern market is replacing the traditional markets. This study is taking place to identify the factors that may influence customer's online shopping satisfaction. Generally, the success of online shopping essentially depends on the customer satisfaction during their purchase.
Due to the sharp growth in the number of people using internet, online shopping in India also has taken a sharp shoot with increasing trend. Educated people specially who are working in the private sector and are time scarce; prefer to shop online for various reasons. A study conducted by BCG suggests that during the year 2013; out of 1220 million Indians, 169 million Indians were active internet users. The study indicates that by the year 2018 this figure of internet users will shoot up and reach up to 583 million. The popularity of the online shopping trend gave an idea of undertaking this research work to know the preference of people to shop from the three popular shopping websites i.e. Amazon.com, Flipkart.com, Snapdeal.com; one Global Company and two Indian Companies. Wherein, the ‘convenience’ sample of 100 internet users in the age group of 18 to 40 years from Ahmedabad city was chosen. A structured questionnaire was given to each one of them to know the preference of website in the city of Ahmedabad along-with the personal interviews. Descriptive research design was used to know the preferences. The findings revealed that majority of the male as well as female internet users preferred Amazon.com (55%) following Flipkart.com (32%) on the various attributes, factors or services offered by these websites. Amazon topped among the three, on variables like: best payment options for all the products, wide range of products, quality products, variety of products. Flipkart was considered as having the best customer care services among the three and Snapdeal was considered as offering the good packaging. The suggestions from the respondents were that all the companies should display original products, offer better product return policies and provide full and actual product description.
Dr. sumit chaturvedi
The last decade witnessed an explosion of social media networks such as Facebook, twitter, you tube, google + etc. This added a new social dimension to the web. This research paper is an attempt to analysis the Youth buyer behavior for apparels in Jaipur city. Youth is conceivably the most complicated demographic group to communicate with. Not only they have a short attention span, they are also subtle in media consumption, indecisive in brand preference, and simply challenging to engage and entertain. Marketers spend millions in marketing research every year trying to predict, or anticipate, changing youth behaviors. It tries to understand the behavior of youth that whether their buying decision are really affected by the social media promotions done by the companies. This will help the companies to know about social media promotions trend in Jaipur.
Sruthi Antony
RELATED PAPERS
WFL Publisher Ltd
JFAE Journal Administration
Results in Physics
BLDE University Journal of Health Sciences
Subhash Mudanur
Mark de Bruijne
Gede Wirya Wardhana
Marcos Lins
Engenharias e outras práticas técnicas engajadas. Volume 1: Redes e Movimientos
Pedro Reynolds-Cuéllar , Thabiso Mashaba
Neurotoxicology and Teratology
Mary Gilbert
Journal of Clinical Oncology
Nabil Hamed
Journal of Clinical Medicine
SPOUDAI Journal of Economics and Business
Serge Kabongo
Vishal Singh
Laura Pautassi
Hen-Hong Chang
Thin Solid Films
Jean-luc Adam
Journal of Pediatric Surgery
Emile Beuls
International Journal of Health Sciences and Research
Rd. Rohmat Saedudin
Strata: Bulletin of the Anglo-Israeli Archaeological Society 37: 51–59.
Yosef Garfinkel
Business and Human Rights Journal
International Journal of Environmental Research and Public Health
Ashleigh Smith
Process Safety and Environmental Protection
Ashok Dastidar
Bandung Conference Series: Mining Engineering
Noor Fauzi Isniarno
Sourav Ghosh
RELATED TOPICS
- We're Hiring!
- Help Center
- Find new research papers in:
- Health Sciences
- Earth Sciences
- Cognitive Science
- Mathematics
- Computer Science
- Academia ©2024
- 1-800-609-6480

- Your Audience
- Your Industry
- Customer Stories
- Case Studies
- Alchemer Survey
- Alchemer Survey is the industry leader in flexibility, ease of use, and fastest implementation. Learn More
- Alchemer Workflow
- Alchemer Workflow is the fastest, easiest, and most effective way to close the loop with customers. Learn More
- Alchemer Digital
- Alchemer Digital drives omni-channel customer engagement across Mobile and Web digital properties. Learn More
- Additional Products
- Alchemer Mobile
- Alchemer Web
- Email and SMS Distribution
- Integrations
- Panel Services
- Website Intercept
- Onboarding Services
- Business Labs
- Basic Training
- Alchemer University
- Our full-service team will help you find the audience you need. Learn More
- Professional Services
- Specialists will custom-fit Alchemer Survey and Workflow to your business. Learn More
- Mobile Executive Reports
- Get help gaining insights into mobile customer feedback tailored to your requirements. Learn More
- Self-Service Survey Pricing
- News & Press
- Help Center
- Mobile Developer Guides
- Resource Library
- Close the Loop
- Security & Privacy
Adding Quantitative Research Questions in Online Surveys
- Share this post:
One of the things that makes Alchemer a powerful online survey and research platform is the sheer number of question types you have access to as a user. This flexibility also allows you to add different question types to any survey, so you don’t have to choose between quantitative and qualitative questions in your survey. You can have both.
If you’re unsure of the difference between quantitative and qualitative, read the article, Does your Consumer Survey Data Paint The Whole Picture . This blog explores the differences between the two question types but here is the short version:
- Quantitative questions will tell you Who and What.
- Qualitative questions will tell you Why.
Quantitative questions are easier to measure and easier for survey takers to answer. Qualitative questions, on the other hand, are subjective and harder to measure. They are also harder for survey-takers to answer and too many can lead to survey fatigue.
Qualitative questions (like open textboxes or essay questions) are great for the exploratory phase of your research project or to delve deeper into a matter, but you want to use them sparingly. Don’t tire your survey-takers or yourself. Trying to analyze essay question answers to find a common theme can be arduous and time-consuming.
One way to make qualitative questions easier on both of you is to use Video Feedback questions, which allow people to respond with a video, rather than writing out their answers.
If you need hard statistics or quantifiable numbers, use quantitative questions. You can assign numeric values for easy, objective measurement and comparison.
Quantitative questions are close-ended which makes them easy to answer. You can ask a lot of these questions without tiring survey respondents. But you’ll want to mix up the question types to keep your survey interesting and your respondents engaged.
In this article we will explore the different ways to ask quantitative questions in your online survey.
How to Phrase Quantitative Questions
Quantitative questions typically start with how or what. Some common leading phrases include:
- How frequently?
- What percentage?
- What proportion?
- To what extent?
Here are some quantitative question examples:
- How many text messages do you send a day?
- How frequently do you text while driving?
- How often do you send text messages while at work?
Be sure to identify all of the variables that might affect the outcome. Also be sure to include all of the groups you are interested in. Neglecting to recognize variables and groups involved will create gaps in your data that will make it hard for you to base sound decisions on.
In the example above, work and driving are variables that likely alter texting behavior. In this example, you could also collect demographic information such as age, gender, and job function so you can compare texting habits between these groups.
Quantitative Question Types
Most online survey tools offer an array of answer formats. This is good news, as these various options will engage your customers and reduce survey fatigue.
Mix up these close-ended question types to increase your response rate:
Radio Button Example:
Checkbox Example:
Drop Down Menu Example:
Drag and Drop Example:
Likert Scale Example:
Sliding Scale Example:
Star Ranking Example:
Net Promoter Score Example:
Image Select Example:
Matrix Example:
Considerations When Choosing Quantitative Question Types
While it is nice to vary your question types to keep respondents interested, it is important to consider the reporting options. Some question types report in bar and pie charts where others may not. Always test your survey and check the reports to ensure you are collecting the data in the format that best suits your needs.
Also consider the type of device your respondents will be using. Interactive question types are engaging but may not be reliable on all mobile devices. Long matrix tables can be frustrating on a mobile device since the radio buttons or checkboxes are small. Image select questions may not render properly or take too long to load.
Use “Other” as Answer Option When Necessary
Hopefully you have considered all of the relevant answer options when crafting your quantitative question. Of course, it is now always possible to include every answer option.
If you are fearful of not including an answer option, use an “Other” answer choice and provide a textbox so respondents can specify the alternative. These are easy to setup when using a radio button or checkbox question type.
If your question is well designed, the “Other” answer option should be the exception rather than the rule. Analyzing the textbox information should not be too arduous since there are likely only a few of them. If more than 50% selected “Other “ as the answer option than perhaps you needed to do some exploratory research.
Quantifiable Results
So there you have it; 10 different quantitative question types that will keep your survey interesting and your respondents engaged. But the best part is that you will have quantifiable data that you can act on! Related Articles: Does You Consumer Survey Data Paint The Whole Picture: When to Use Qualitative Vs. Quantitative Research Questions Quantitative Vs. Qualitative Research – When to Use Which Using Qualitative Exploration To Create Quantitative Surveys Using Highly Interactive Questions In Online Surveys
- Get Your Free Demo Today Get Demo
- See How Easy Alchemer Is to Use See Help Docs
Start making smarter decisions
Related posts, playing with logic to collect better feedback.
- January 12, 2024
- 4 minute read
Combining Reports – An Answer to One of Your NPS Questions
- October 27, 2022
- 2 minute read
Survey Templates – An Answer to One of Your NPS Questions
- October 11, 2022
- 3 minute read
See it in Action
Request a demo.

- Privacy Overview
- Strictly Necessary Cookies
- 3rd Party Cookies
This website uses cookies so that we can provide you with the best user experience possible. Cookie information is stored in your browser and performs functions such as recognising you when you return to our website and helping our team to understand which sections of the website you find most interesting and useful.
Strictly Necessary Cookie should be enabled at all times so that we can save your preferences for cookie settings.
If you disable this cookie, we will not be able to save your preferences. This means that every time you visit this website you will need to enable or disable cookies again.
This website uses Google Analytics to collect anonymous information such as the number of visitors to the site, and the most popular pages.
Keeping this cookie enabled helps us to improve our website.
Please enable Strictly Necessary Cookies first so that we can save your preferences!
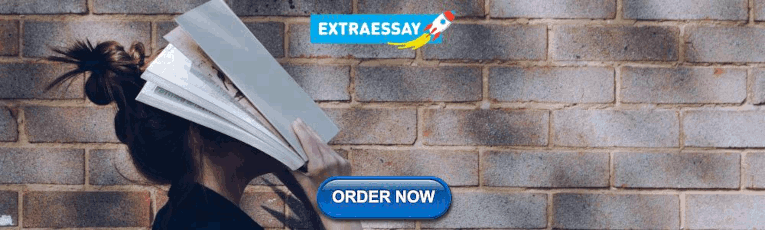
COMMENTS
Close-ended questions help you to gather quantitative data. 11 Close-ended Questions for an Online Shopping Questionnaire ... Research shows that consumers spend an average of 5 hours shopping online every week and 92% of consumers shop online at least once a year. This, once again, emphasizes how much online shopping has become integral to our ...
The impact of online shopping attributes on customer satisfaction and loyalty: Moderating effects of e-commerce experience ... (Brusch et al., Citation 2019), which raises the guiding research questions of this study: ... A quantitative research study that was descriptive in nature was conducted to examine the effects of online shopping ...
3.1.3 About this report. This report represents key findings from the NSW Fair Trading commissioned research into online retail shopping in NSW. Samples in this study are drawn from NSW consumers and businesses (SMBs). Responses from consumers and businesses are examined from both total and subgroup perspectives.
The purpose of the research was to find out the problems that consumers face during their shopping through online stores.,A quantitative research method was adopted for this research in which a survey was conducted among the users of online shopping sites.,As per the results total six factors came out from the study that restrains consumers to ...
differences in there online-shopping cultures as well. To create the best online shopping experiences, cultural values should be considered within (concentrated) marketing approaches. Objective: This research is conducted to examine the online shopping behaviour of generation Y in relation to the cultural values of Germans and Swedes.
We could confirm that consumer online purchasing behavior can be fairly explained by seven factors developed by our study. 4. Managerial implications. As there is an increasing growth of e-commerce retail market (Statista, Citation 2018 ), it is predicted that the number of e-commerce stores will raise, too.
A research on the E-shopping behaviours of British and American consumers has also shown that E-shopping is a determinant of online shopping. Likewise, consumer research on E-shopping behaviour accepts that attitude represents a description of the positive or negative self-appraisal of a client's behaviour, values, feelings, and patterns during ...
Quantitative market research questions to ask for actionable insights. February 16, 2024. 14 min read. In this article. There's a big difference between asking "Why do you like our product?" and "On a scale of 1-10, how much do you like our product?". But both ways of asking are valuable in their own way. Knowing your audience is not ...
Research offers some indication that the online customers' shopping experience (OCSE) can be a strong predictor of online impulsive buying behavior, but there is not much empirical support available to form a holistic understanding; whether, and indeed how, the effects of the OCSE on online impulsive buying behavior are affected by customers' attitudinal loyalty and self-control are not well ...
This article attempts to take stock of this environment to critically assess the research gaps in the domain and provide future research directions. Applying a well-grounded systematic methodology following the TCCM (theory, context, characteristics and methodology) framework, 197 online consumer shopping behaviour articles were reviewed.
This paper aims to determine the factors that influence the consumer's online shopping intention in the current pandemic context (COVID-19). For this purpose, a conceptual model has been developed by introducing the constructs "attitude," "perceived utility," "intention" as well as the variable "perceived risk of contagion.".
This paper aims to propose an approach to evaluate the quality of online shopping services in times of pandemic COVID-19, from the ordering of quality attributes taking into account customers' perception. The proposed approach was developed from a structured questionnaire containing 25 quality attributes adapted from the E-S-QUAL model and applied to consumers of online shopping services ...
The goal of the research is to identify and explore most commonly purchasing products online also influencing factors on the online buying behavior of the MUST university students. Functionality ...
The descriptive quantitative study is a combination of the context of business and psychology. It examined the perceived benefits of online shopping affecting the behavior of online millennial and ...
Here, I've detailed 23 use cases and curated 98 quantitative market research questions with examples - making this a post you should add to your bookmark list , so you can quickly refer back. I've formatted this post to show you 10-15 questions for each use case. At the end of each section, I also share a quicker way to get similar ...
Through research methods such as theoretical deduction, model, and statistical tests, which were used to determine the main characteristics of compulsive purchase behavior in regard to online shopping variables, the main variables affecting social presence, impulsive purchase, and consumer personality tendencies, with core variables focusing on ...
The academic interest in Online Grocery Shopping (OGS) has proliferated in retailing and business management over the past two decades. Previous research on OGS was primarily focused on consumer-level consequences such as purchase intention, purchase decision, and post-purchase behavior. However, there is a lack of literature integrating intrinsic and extrinsic factors that influence the ...
Graduation date: 2011 The purpose of the present study is to examine consumers' privacy concerns in the online shopping context. Drawing from Social Contract Theory, the present study proposed a structure equation model to examine how consumers' evaluations of online shopping experiences (perceived benefit, risk and fairness) and attitudes (trust, moods, and repurchase loyalty) toward ...
The data presented in this paper is focused only on the students who experienced online shopping. Among those 393 students, there were 83 students who did online shopping. The research was conducted according to and complies with all regulations established in the ethical guidelines by the ITS Research Ethics Committee in the "code of ethics".
Page 96 Research Paper Impact Factor: 3.853 Peer Reviewed, Listed & Indexed Frequency of online shopping Very often 13 Often 24 Occasionally 71 Rarely 12 IJBARR E- ISSN -2347-856X ISSN -2348-0653 10.8% 20% 59% 10% Results and Discussion As visualised in figure 1, tickets and clothing are the most preferred products which constitute 41% of the ...
SurveyMonkey is rated 4.5 out of 5 from 18,000+ reviews on G2.com. Whether you're an online retailer or an internet advertiser, it helps to know how people use online shopping websites. With the expert-certified questions in this online shopping attitudes template, you'll get important feedback from online consumers.
This paper intends to examine online shopping. experiences from three aspects: the physical, ideological and pragmatic dimensions. As an exploratory research study, a qualitative research method ...
This question type can be configured to be a single or multi-select answer option. Respondents select an image answer based on a set of set of images. This is great for your market research surveys where you would like respondents to choose which image they find most appealing. Image Select Example: