- Browse All Articles
- Newsletter Sign-Up
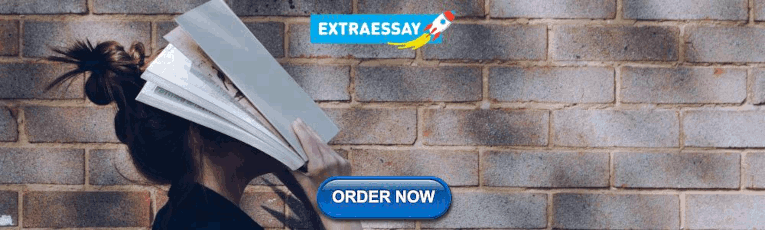
TechnologyAdoption →
No results found in working knowledge.
- Were any results found in one of the other content buckets on the left?
- Try removing some search filters.
- Use different search filters.

An official website of the United States government
The .gov means it’s official. Federal government websites often end in .gov or .mil. Before sharing sensitive information, make sure you’re on a federal government site.
The site is secure. The https:// ensures that you are connecting to the official website and that any information you provide is encrypted and transmitted securely.
- Publications
- Account settings
Preview improvements coming to the PMC website in October 2024. Learn More or Try it out now .
- Advanced Search
- Journal List
- Springer Nature - PMC COVID-19 Collection

Educational Technology Adoption: A systematic review
Andrina granić.
Faculty of Science, Department of Computer Science, University of Split, Rudera Boskovica 33, 21000 Split, Croatia
During the past decades a respectable number and variety of theoretical perspectives and practical approaches have been advanced for studying determinants for prediction and explanation of user’s behavior towards acceptance and adoption of educational technology. Aiming to identify the most prominent factors affecting and reliably predicting successful educational technology adoption, this systematic review offers succinct account of technology adoption and acceptance theories and models related to and widely applied in educational research. Recognised journals of the Web of Science (WoS) database were searched with no time frame limit, and a total of 47 studies published between 2003 and 2021 were critically analysed. The key research findings revealed that in educational context a vast majority of selected studies explore the validity of Technology Acceptance Model (TAM) and its many different extensions (N=37), along with TAM’s integrations with other contributing theories and models (N=5). It was exposed that among numerous predictors, thematically grouped into user aspects, task & technology aspects, and social aspects, self-efficacy, subjective norm, (perceived) enjoyment, facilitating conditions, (computer) anxiety, system accessibility, and (technological) complexity were the most frequent predictive factors (i.e. antecedents) affecting educational technology adoption. Considering types of technologies, e-learning was found to be the most common validated mode of delivery, followed by m-learning, Learning Management Systems (LMSs), and social media services. The results also revealed that the majority of analysed studies were conducted in higher education environments. New directions of research along with potential challenges in educational technology acceptance, adoption, and actual use are discussed as well.
Introduction
Over the last half-century, a vast number of adoption theories and technology acceptance models, along with a plethora of their extensions and modifications has been advanced. Aiming to explore their applicability, as well as to enhance their predictive validity, proposed theories and models have been extensively used in assessment of various Information and Communication Technology (ICT) products and services. Commonly, technology adoption is a term that refers to the acceptance, integration, and embracement of any types of new technology. Technology acceptance, as the first step of technology adoption, is an attitude towards technology, and it is influenced by various factors. According to the Innovation Diffusion Theory (IDT) (Rogers, 1962 , 1995 ), adoption is a decision to make full use of technology innovation as the best course of action available. The key to adoption is that the adopter (individual or organization) must perceive the idea, behavior, or product as new or innovative. As for technology adoption research at the individual level, numerous theories and models have been used to predict and explain human behavior towards technology acceptance, adoption and usage.
Education presents an area of great interest in incorporating new technologies, thus technology acceptance and adoption theories and models are often used to inform research in educational context. Such setting is characterised by a great variety of potential users of various types of technology embraced in the process of learning, teaching, and assessment. Some of the most influential theoretical approaches involve (listed in chronological order with relevant illustrative example research):
- Technology Acceptance Model (TAM) (Davis, 1986 , 1989 ), the widely used reliable model, to explore new facilitating technologies in educational context, ranging from social media platforms (Yu, 2020 ) to the technology aimed at helping the learning process through teaching assistant robots (Park and Kwon, 2016 ), simulators (Lemay, Morin, Bazelais & Doleck, 2018 ), and virtual reality (Jang, Ko, Shin & Han, 2021 );
- Decomposed Theory of Planned Behavior (DTPB) (Taylor & Todd, 1995 ) to understand university students’ adoption of WhatsApp in learning (Nyasulu & Chawinga, 2019 ), to explore factors that influence teachers’ intentions to integrate digital literacy (Sadaf & Gezer, 2020 ), as well as to examine factors that impact the acceptance and usage of e-assessment by academics (Alruwais, Wills & Wald, 2017 );
- Unified Theory of Acceptance and Use of Technology (UTAUT) (Venkatesh, Morris, Davis & Davis, 2003 ) to study core factors affecting the university students’ attitude towards adoption of online classes during COVID-19 (Tiwari, 2020 ), to explore the factors that influence preservice teachers’ acceptance of ICT integration in the classroom (Birch & Irvine, 2009 ), and students’ usage of e-learning systems in developing countries (Abbad, 2021 );
- Extended UTAUT (UTAUT2) (Venkatesh, Thong & Xu, 2012 ) to evaluate acceptance of blended learning in executive education (Dakduk, Santalla-Banderali & van der Woude, 2018 ), and to examine preservice teachers’ acceptance of learning management software (Raman & Don, 2013 ).
Several reviews and meta-analysis that summarize empirical research have been focused on specific topics in the field of education, for example: (i) particular technology adoption model , like the meta-analysis dealing with TAM in prediction of teachers’ adoption of technology (Scherer, Siddiq & Tondeur, 2019 ), and the quantitative meta-analysis to identify the most commonly used external factors of TAM in the context of e-learning adoption (Abdullah & Ward, 2016 ); (ii) specific type of users , like reviews conducted to understand factors influencing academics’ adoption of learning technologies (Liu, Geertshuis & Grainger, 2020 ), to explore factors that affect teachers’ acceptance and use of ICT in the classroom (Gamage & Tanwar, 2018 ), as well as to study factors affecting students’ adoption and continuation of technology use in online learning (Panigrahi, Srivastava & Sharma, 2018 ); (iii) particular technology and mode of delivery , like reviews carried on to explore factors affecting blended learning adoption and implementation in higher education (Anthony, et al. 2020 ), to study technical factors affecting users’ intentions to use mobile phones as learning tools (Alghazi, Wong, Kamsin, Yadegaridehkordi & Shuib, 2020 ), as well as to examine the most prominent external factors affecting learning management systems (LMSs) adoption in higher educational institutions (Al-Nuaimi & Al-Emran, 2021 ). Besides, some theoretical work aimed to identify determinants of learning technology acceptance, but it was more focused on original constructs of reviewed technology adoption theories, like in the study conducted by Kaushik and Verma ( 2020 ).
However, to the best of authors’ knowledge, currently there is hardly a holistic view of factors that affect and reliably predict successful acceptance and adoption of technology engaged in educational process. Understanding these aspects can be beneficial and can help in an improvement of both, research and educational practices. Hence, this concept-centric review aims at addressing this concern with the following two main research questions (RQs):
- RQ1. Which technology acceptance and adoption theories and models are widely applied in educational research?
- RQ2. Which are the most prominent predictive factors (i.e. antecedents) affecting educational technology adoption?
Research Approach
The research scope of this systematic review is narrowed and piloted towards understanding the most recognized and applied theoretical models, as well as the most influential predictive factors affecting various technologies used to support the process of knowledge transfer and acquisition. Due to massive work worldwide, this study is used to offer succinct account of predominant predictors in educational technology adoption, and certainly cannot be all-encompassing. With the aim to filter and narrow the search, but at the same time to cover representative literature from recognised journals, the Web of Science (WoS) Current Contents Connect (CCC) database was searched. The search was not limited to a precise timespan. To denote different technology acceptance models and theories, the search was conducted using relevant terms connected with Boolean operators “OR” and “AND”, specifically (“theor*” OR “model”) AND (“technolog*”) AND (“adoption” OR “acceptance”) . To locate education related studies, (“education*” OR “learn*”) search terms were joined with the aforementioned ones by means of the operator “AND”. Truncation was used to cover all variations of some keywords, for example, the search term “ technolog* ” was used to search for literature that included the word “technology” as well as “technologies”.
It was searched for studies that have specified search terms in publication title (the filter “TITLE” was selected). For the purpose of this review, specified inclusion criteria enabled selection of studies that report on technology acceptance and adoption theories and models in which some type of ICT products and services to support the process of learning and teaching was used (in this context indicating all classes of technologies, interactive systems, environments, tools, applications, services, platforms, and devices). To be included, studies had to report on empirically evaluated research model and related research hypothesis. Besides, studies must be published as peer-reviewed journal articles written in English language. On the subject of exclusion criteria , studies that do not clearly and credibly describe model/theory constructs or variables, and the relationships among them, were not considered as valid to be selected and included in the analysis. In addition, theoretical studies published as peer-reviewed journal articles, specifically reviews and meta-analysis, were excluded as well.
The literature search was conducted in August 2021. No time frame period was specified; 1998-2021 is the full range of the CCC database search engine. In this inquiry, 71 publications that included specified search terms in the publication title were identified. Considering only peer-reviewed journal articles written in English, the number of 67 journal and review articles was reached. Title, abstract and full text of the filtered literature were screened to ensure publication suitability and relevance. Accordingly, the qualified publications were retained and eleven unrelated ones were excluded, thus narrowing the number and leaving for further detailed analysis 56 publications. Out of 56 identified journal articles, 47 publications were found to be compliant with the purpose of this study, while 9 publications offered theoretical work which summarized empirical research focused on specific topics in educational technology acceptance and adoption.
In view of the identified theoretical work, the majority of studies offered meta-analysis and reviews of Technology Acceptance Model (TAM) based studies in education (N=6), specifically (Dimitrijević & Devedžić, 2021 ; Granić & Marangunić, 2019 ; Kemp, Palmer & Strelan, 2019 ; Scherer et al., 2019 ; Al-Emran, Mezhuyev & Kamaludin, 2018 ; Abdullah & Ward,2016), while just few publications addressed other acceptance models and theories, in particular Unified Theory of Acceptance and Use of Technology (UTAUT) (Bervell & Umar, 2017 ), Senior Technology Exploration, Learning and Acceptance (STELA) model (Tsai, Rikard, Cotton & Shillair, 2019 ), along with Straub’s ( 2009 ) study in a context of informal learning which examined adoption processes through the lenses of Innovation Diffusion Theory (IDT), Concerns-Based Adoption Model (CBAM), TAM and UTAUT.
Results and Discussion
The analysis of 47 publications found to be compliant with the purpose of this study is presented and discussed in the following.
Publication History and Distribution by Countries
Considering the history of publishing, Fig. 1 shows the trend of publication frequency which started in 2003, and can be followed until 2021. The majority of studies has been published in the last decade thus reflecting an increased attention given to the researched domain. It can be noticed that there are only three identified studies in 2021, but this is connected with the fact that the search was undertaken in August 2021, and several potentially relevant articles/studies are not published yet.

Publication history
The interest of researchers worldwide in educational technology acceptance and adoption is evident (see Fig. 2 ). Most of the identified studies were conducted in Taiwan (N=7), followed by relevant research carried out in South Korea and USA (N=4), Spain (N=3), Canada, China, Hong Kong, Malaysia, Pakistan, Singapore and Turkey (N=2). In the rest of illustrated countries only single studies were piloted (alphabetical order): Azerbaijan, Cyprus, France, Hungary, Lebanon, Libya, Netherlands, Nigeria, Oman, Philippines, South Africa, UK, United Arab Emirates, as well as Qatar & USA.

Distribution of selected articles by countries
Type of Technologies and Modes of Delivery
This research revealed a diversity of ICT products and services employed in educational context, here referring to all classes of technologies, interactive systems, environments, tools, applications, services, platforms, and devices used in the selected research. Considering types of technologies and modes of delivery used to support the process of learning and teaching, it is noticeable that almost half of the analysed studies (N=20) validated e-learning technologies, in selected research referred to as e-learning systems (Hanif, Jamal & Imran 2018 ), e-learning platforms (Song & Kong, 2017 ), e-learning environments (Esteban-Millat, Martinez-Lopez, Pujol-Jover, Gazquez-Abad & Alegret 2018 ), e-learning tools (Tarhini, Hone, Liu & Tarhini 2016 ), web-based learning systems (Calisir, Gumussoy, Bayraktaroglu & Karaali 2014 ), Internet-based learning systems (Saade & Bahli, 2005 ), or just e-learning (Abdou & Jasimuddin, 2020 ). Many studies dealt with mobile learning (N=6) in which context mobile computing devices (Lai, 2020 ), mobile technology and apps (Briz-Ponce & Garcia-Penalvo, 2015 ), tablet personal computers (Moran, Hawkes & El Gayar, 2010 ), or just m-learning (Iqbal & Bhatti, 2015 ) was validated. Learning Management Systems (LMSs) in general, along with specific LMSs in particular, like Blackboard (Yi & Hwang, 2003 ), Moodle (Nagy, 2018 ), and Moodle gamification training platform (Vanduhe, Nat & Hasan, 2020 ), were also frequently researched (N=6).
Besides, some studies (N=5) counted on social media services/platforms at large (Al-Rahmi, Shamsuddin, Alturki, Aldraiweesh, Yusof, Al-Rahmi & Aljeraiwi, 2021), as well as on WeChat (Yu, 2020 ) and YouTube (Lee & Lehto, 2013 ) in particular. Since educational possibilities of virtual reality (VR) and augmented reality (AR) are getting more attention, few studies (N=3) were focused on VR technology (Lin and Yeh, 2019), VR and AR technology (Jang, Ko, Shin & Han, 2021 ), while one earlier study concerned virtual world Second Life (Chow, Herold, Choo & Chan, 2012 ). Use of computer technology in general was examined in a couple of studies (N=2) (e.g. Teo, 2010 ), while a number of single studies considered also assistive technology (Nam, Bahn & Lee, 2013 ), collaborative technology, specifically Google applications for collaborative learning (Cheung & Vogel, 2013 ), simulation-based learning environment (Lemay, Morin, Bazelais & Doleck, 2018 ), university communication model (UCOM) which works similar to Massive Open Online Course (MOOC) (Tawafak, Romli & Arshah, 2018 ), as well as Open Educational Resources (OER) (Kelly, 2014 ). Figure 3 provides insight into a variety of validated technologies and modes of delivery.

Validated technologies and modes of delivery
Type of Participants & Sample Size
Another aspect refers to different types of involved participants/users. In a great majority of analysed research (N=29) university students were the most commonly chosen sample group, since most data from web-based questionnaires and/or mailed surveys was collected from the universities (e.g. Salloum, Alhamad, Al-Emran, Monem & Shaalan, 2019 ; Park, 2009 ). Several studies involved employees (N=7) from a variety of organizations/companies, specifically faculty & educational stakeholders (Aburagaga, Agoyi & Elgedawy, 2020 ), bank officials (Abdou & Jasimuddin, 2020 ), business workforce (Lee, Hsieh & Hsu, 2011 ), blue-collar workers (Calisir et al., 2014 ), health nurses (Chen, Yang, Tang, Huang & Yu, 2008 ), along with employees from four international agencies of the United Nations (Roca, Chiu & Martinez, 2006 ), as well as from four industries, specifically manufacturing, information technology, marketing and government agencies (Lee, Hsieh & Chen, 2013 ). Quite a few studies engaged teachers (N=5), to be specific pre-service (Teo, 2010 ) and in-service teachers (Jang et al., 2021 ), special education teachers (Nam et al., 2013 ), as well as K-12 educators (Kelly, 2014 ). A small number of research also involved other participants, in particular university instructors (N=2) (Vanduhe et al., 2020 ), older adults (Lai, 2020 ), and senior high school students (Prasetyo, Ong, Concepcion, Navata, Robles, Tomagos, Young, Diaz, Nadlifatin & Redi, 2021). Finally, in one study information about the type of participants who took part in the conducted research was not provided (see Fig. 4 ).

Type of involved participants
It can be seen that sample size varied from the smallest sample of 72 students (Lin & Yeh, 2019) to the largest one of 2574 students involved in the study conducted by Esteban-Millat et al. ( 2018 ). However, the domination of smaller sample sizes up to 400 participants (N=30) compared to the number of larger sample sizes is notable.
Employed Technology Acceptance and Adoption Models
The conducted review clearly indicated that the vast majority of identified research used TAM model (N=42), in particular the core TAM (N=1), the extended TAM (N=36), along with some studies which integrated TAM with other individual models/theories aiming to advance TAM’s explanatory power (N=5), in particular with:
- Innovation Diffusion Theory (IDT) proposed by Rogers ( 1962 , 1995 ) as the most popular model in investigating innovation acceptance and adoption (N=2), specifically (Lee et al., 2011 ; Al-Rahmi, Yahaya, Aldraiweesh, Alamri, Aljarboa, Alturki & Aljeraiwi, 2019),
- Information Systems Success Model (ISSM) introduced by DeLone and McLean ( 1992 ) as a robust theoretical basis for the study of technology post-adoption (N=2), specifically (Prasetyo et al., 2021 ; Al-Rahmi et al., 2021 ),
- combination of ISSM and Expectation-Confirmation Theory (ECT), a post-adoption theory offered by Oliver ( 1980 ), in work conducted by Roca, Chiu, and Martinez ( 2006 ).
Besides TAM-based research, a few studies explored also the core (N=2) and the extended (N=2) UTAUT model, along with a single research which employed extended UTAUT2 model (refer to Fig. 5 ).

Used technology acceptance and adoption models
Factors Affecting Educational Technology Adoption
This study revealed that, aiming to increase the predictive validity of TAM and UTAUT, in most selected studies (N=44) the models have been extended with different predictive (antecedent) factors . In view of UTAUT model on the one hand, those factors are related to the behavioral intention (BI) variable/construct. On the other hand, when considering TAM, the majority of identified factors represent antecedents of the two core variables of TAM, perceived ease of use (PEU) and perceived usefulness (PU), while a minor number predicts behavioral intention (BI). Among selected research, only three studies have used original models without any modifications and enhancements, in particular the core TAM (Chipps, Kerr, Brysiewicz & Walters, 2015 ) and the core UTAUT (Lai, 2020 ; Yakubu & Dasuki, 2019 ).
In addition, besides a variety of introduced predictors for the core TAM constructs, as well as TAM’s and UTAUT’s behavioral intention variable, the results exposed also a number of incorporated supplementary factors which aimed to moderate relationships among TAM’s constructs. Consequently, categorization of identified factors from models’ modifications and enhancements included in this review is conducted, and three pools of factors affecting educational technology adoption are documented:
- antecedents of perceived ease of use (PEU) and perceived usefulness (PU),
- behavioral intention (BI) antecedents, and.
- moderating factors.
To shed-light-on, numerous identified predictive factors are thematically grouped into: (i) user aspects (individual attributes, and pleasure & usefulness), (ii) task & technology aspects , and (iii) social aspects . The categorised antecedents of TAM variables (PEU and PU), as well as TAM’s and UTAUT’s BI antecedents, along with related illustrative example research are presented in Tables 1 and and2, 2 , respectively.
Predictors of the two core TAM variables (PEU and PU) along with relevant example research
Predictors of TAM’s and UTAUT’s behavioral intention (BI) variable along with example research
Antecedents of Perceived Ease of Use and Perceived Usefulness. By analysing the selected publications, self-efficacy was found as the most widely introduced predictive factor of TAM (N=16). In various empirical studies conducted in educational context it was revealed that self-efficacy, i.e. an individual judgement of one’s capability to use computer (e.g. Salloum et al., 2019 ; Teo, 2009 ), Internet (e.g. Nagy, 2018 ), m-learning (e.g. Park, Nam & Cha, 2012 ), e-learning (e.g. Chen et al., 2008 ) or specific application (e.g. Yi & Hwang, 2003 ), had a significant impact on the perceived usefulness and the perceived ease of use. Another widely researched predictive factors were subjective norm (N=9), defined as the degree to which an individual believes that people who are important to him/her think he/she should or should not perform the behavior in question, as well as perceived enjoyment (N=8) considered as the extent to which the activity of using the computer is perceived to be enjoyable in its own right, apart from any performance consequences that may be anticipated. It has been revealed that the subjective norm (Song & Kong, 2017 ), and enjoyment (Salloum et al., 2019 ), positively and significantly influence students’ perceived usefulness of e-learning, as well as perceived ease of use of e-learning systems (Hanif et al., 2018 ; Chang, Hajiyev & Su, 2017 )
The results indicated that system quality (e.g. Salloum et al., 2019 ) and system accessibility (e.g. Park et al., 2012 ; Hanif et al., 2018 ), along with technological complexity (e.g. Teo, 2009 ) have a significant influence on perceived ease of use. Besides, facilitating conditions , which originally provide resource factors (such as time and money needed) and technology factors regarding compatibility issues that may constrain usage, were indicated to be an essential factor that affect e-learning system (e.g. Song & Kong, 2017 ) or computer technology (e.g. Teo, 2009 ) acceptance. Finally, while the perceived playfulness, which operationalizes the question of how intrinsic motives affect the individual’s acceptance of technology, had a direct impact on the variables perceived usefulness and perceived ease of use (e.g. Padilla-Melendez, del Aguila-Obra & Garrido-Moreno, 2013 ), anxiety as a personal trait explained as evoking anxious or emotional reactions when it comes to performing a behavior, negatively affects the two core TAM variables (e.g. Chang et al., 2017 ; Calisir et al., 2014 )
Behavioral Intention Antecedents. Both self-efficacy and subjective norm were among frequently employed factors affecting attitude towards technology and behavioral intention. The results indicated that self-efficacy was found to have a direct effect and a positive influence on behavioral intention to use e-learning (e.g. Tarhini, Hone & Liu, 2014 ; Yi & Hwang, 2003 ), m-learning (e.g. Moran et al., 2010 ; Park et al., 2012 ), as well as collaborative technology (e.g. Cheung & Vogel, 2013 ), and computers (e.g. Nam et al., 2013 ; Teo, 2009 ). Subjective norm , as another important construct in providing an understanding of the determinants of usage in educational context, is shown to have strong influence on the behavioral intention to use e-learning systems/platforms (e.g. Song & Kong, 2017 ). It has been revealed that subjective norm represented by peers significantly moderate the relationship between attitude and intention toward the technology (Cheung & Vogel, 2013 )
Furthermore, perceived playfulness is found to be one of the key drivers for the adoption and use of blended learning system depending of user’s gender (Padilla-Melendez et al., 2013 ). Also, direct and indirect effect of perceived playfulness on the intention to use a computer-assisted training program has been confirmed (Lin & Yeh, 2019). Finally, the research has exposed that system accessibility was one of the dominant exogenous constructs affecting behavioral intention to use mobile learning (e.g. Park et al., 2012 )
Moderating Factors. Although the majority of selected research has been focused on finding PEU, PU and BI antecedents, there is also a growing need for understanding incorporated supplementary factors aiming to moderate the relationships among TAM variables, on the one hand, as well as those which have an impact on the model itself, on the other. In the investigation of the moderating effect of gender and age on e-learning acceptance Tarhini and colleagues ( 2014 ) have found that age moderates the effect of perceived ease of use, perceived usefulness and self-efficacy on behavioral intention, and that gender moderates the effect of perceived ease of use and social norms on behavioral intention. Yet, unexpectedly, no significant moderating effect of age on the relationship between social norms and behavioral intention was found; results also revealed no moderating of gender on perceived usefulness or self-efficacy and behavioral intention. Padilla-Melendez et al. ( 2013 ) argued that there exist gender differences in attitude and intentions to use. The main contribution of their study is provided evidence that there exist gender differences in the effect of playfulness in the student attitude toward technology and the intention to use it. In females, playfulness influences attitude toward using the system. In males, playfulness influences attitude moderated by perceived usefulness
When examining the moderating effect of individual-level cultural values on users’ acceptance of e-learning in developing countries, Tarhini et al. ( 2016 ) demonstrated that the relationship between social norms and behavioral intention was particularly sensitive to differences in individual cultural values, with significant moderating effects observed for all studied cultural dimensions, in particular masculinity/femininity , individualism/collectivism , power distance and uncertainty avoidance . As a final point, in an empirical study of the use of the General Extended Technology Acceptance Model for E-learning (GETAMEL) to determine the factors that affect students’ intention to use an e-learning system, Chang and colleagues ( 2017 ) found that technological innovation significantly moderates the relationship between subjective norm and perceived usefulness, as well as perceived usefulness and behavioral intention to use e-learning.
Integration with Other Models & Theories
Although TAM proved to be a powerful model applicable to various technologies and contexts at the individual level, research also revealed its successful integration with other contributing theories and models within a range of different application fields (Al-Emran & Granić, 2021 ). To evaluate students’ adoption of smartwatches for educational purposes, TAM has been successfully combined with Goodhue and Thompson’s ( 1995 ) Task-Technology Fit (TTF) (Al-Emran, 2021 ), and Rogers ( 1975 ) Protection Motivation Theory (PMT) (Al-Emran, Granić, Al-Sharafi, Nisreen & Sarrab, 2021 ). In addition, the Innovation Diffusion Theory (IDT) has been combined with TAM in an empirical investigation on university students’ intention to use e-learning systems (Al-Rahmi et al., 2019 ), to investigate factors affecting business employees’ behavioral intentions to use the e-learning system (Lee et al., 2011 ), as well as to explore diffusion and adoption of an open source learning platform (Huang, Wang, Yang & Shiau, 2020 ). The Information Systems Success Model (ISSM), as one of the post-adoption theories, has been integrated with TAM to help in determining factors which affected acceptance of e-learning platforms during the COVID-19 pandemic (Prasetyo et al., 2021 ), and in exploring students’ behavioral intention to use social media, specifically the perception of their academic performance and satisfaction (Al-Rahmi et al., 2021 ). Lastly, to understand e-learning continuance intention, TAM has been integrated with ISSM and Oliver’s ( 1980 ) Expectation-Confirmation Theory (ECT) (Roca et al., 2006 ).
Limitations of the Conducted Review
In the conducted review, specific criteria were used to search the WoS CCC database for relevant studies to be included and analysed. The applied research approach allowed to capture and cover only a representative selection of studies published in numerous recognized journals, and undoubtedly cannot be all-inclusive. Specification of other search criteria along with a selection of other databases might bring more and/or slightly different selection of relevant journal articles and proceeding papers. Hence, this review should be regarded as an attempt to explore relevant challenges and emerged topics in educational technology adoption field during the past. Finally, it should be noted that this study does not describe or pass any judgement on research methods and approaches employed in the analysed literature since this is out of the scope of this review.
Conclusion and Future Research
Over the past decades a variety of theoretical perspectives have been advanced to provide an understanding of the determinants of acceptance, adoption and usage of various technologies used to support the process of knowledge transfer and acquisition. However, it has been shown that over the years TAM has emerged as a leading scientific paradigm for studying the determinants affecting human behavior and usage of various technologies through beliefs about two factors: the perceived ease of use and the perceived usefulness (Al-Emran & Granić, 2021 ; Marangunić & Granić, 2015 ). Moreover, the conducted review once again exposed TAM predomination in educational context as well; refer also to (Granić & Marangunić, 2019 ). This study confirmed that TAM is the most widely used powerful and valid model for prediction and explanation of user’s behavior towards acceptance and adoption of various technologies used to support the process of learning and teaching.
Continuous development of new technologies, along with a growing number and diversity of users in educational context, opens new directions of research that could raise understanding of the technology acceptance, adoption, and actual use. Thus, despite the fact that extensive work has already been conducted, there is still a huge potential for further advancements, exploration and practice in this field of research. In light of current research findings, future work could follow new research directions:
- to explore predictive validity of technology acceptance models and theories when applied to various supporting ICT technologies employed in a number of emerging teaching strategies , like flipped learning, gamification-based learning, and visual scaffolding, favourable communication support , like chats, discussion forums, and discussion boards, as well as relevant facilitative tools , like blogs and wikis used in educational context;
- to further empirically validate predictive factors (antecedents) influencing the acceptance and adoption of technology in education which have not been so widely explored, for example perceived playfulness which has been associated with a high level of perceived usefulness (Lin & Yeh, 2019), social media usage which has indicated a positive and constructive influence on satisfaction and academic performance (Al-Rahmi et al., 2021 ), as well as psychological influence factors such as conformity behavior and self-esteem due to their positive and direct effect on perceived ease of use, perceived usefulness, perceived enjoyment and continuance intention (Yu, 2020 );
- to explore some possibly significant predictive factors that still have not been adequately examined, but could be important in understanding educational technology adoption as for example, the factor dealing with task & technology aspects, that can be described as cost-effective/pennyworth , here referring to employment of efficient solutions in educational context with relatively limited budget (e.g. simulation, VR, AR, visual scaffolding/visualization);
- to advance the explanatory power of individual technology acceptance and adoption models by reviewing and integrating them with already established theories and models from other fields, like social psychology – Bagozzi and Warshaw’s ( 1990 ) Theory of Trying (TofT), cognitive psychology – Bhattacherjee’s ( 2001 ) Expectation-Confirmation Model (ECM), along with information technology – Goodhue and Thompson’s (1995) Task-Technology Fit (TTF).
Declarations
The data of the systematic review consist of articles published in journals and conferences. Many of these are freely available online, others can be accessed for a fee or through subscription.
The authors declare no conflicts of interest.
No ethics review was required to undertake this literature review.
The original online version of this article was revised: Figures 2, 3 and 4 were incorrectly captured in the html version.
Publisher’s Note
Springer Nature remains neutral with regard to jurisdictional claims in published maps and institutional affiliations.
Change history
A Correction to this paper has been published: 10.1007/s10639-022-11053-0
- Abbad MM. Using the UTAUT model to understand students’ usage of e-learning systems in developing countries. Education and Information Technologies. 2021 doi: 10.1007/s10639-021-10573-5. [ PMC free article ] [ PubMed ] [ CrossRef ] [ Google Scholar ]
- Abdou D, Jasimuddin SM. The use of the UTAUT model in the adoption of e-learning technologies: An empirical study in France based banks. Journal of Global Information Management. 2020; 28 (4):38–51. doi: 10.4018/JGIM.2020100103. [ CrossRef ] [ Google Scholar ]
- Abdullah F, Ward R. Developing a General Extended Technology Acceptance Model for E-Learning (GETAMEL) by analysing commonly used external factors. Computers in Human Behavior. 2016; 56 :238–256. doi: 10.1016/j.chb.2015.11.036. [ CrossRef ] [ Google Scholar ]
- Aburagaga I, Agoyi M, Elgedawy I. Assessing faculty’s use of social network tools in Libyan higher education via a Technology Acceptance Model. IEEE ACCESS. 2020; 8 :116415–116430. doi: 10.1109/ACCESS.2020.3004200. [ CrossRef ] [ Google Scholar ]
- Al-Emran M. Evaluating the use of smartwatches for learning purposes through the integration of the Technology Acceptance Model and Task-Technology Fit. International Journal of Human–Computer Interaction. 2021 doi: 10.1080/10447318.2021.1921481. [ CrossRef ] [ Google Scholar ]
- Al-Emran, M., & Granić, A. (2021). Is it still valid or outdated? A bibliometric analysis of the Technology Acceptance Model and its applications from 2010 to 2020. In Al-Emran, N., & Shaalan, K. (Eds.) Recent Advances in Technology Acceptance Models and Theories. Studies in Systems, Decision and Control , Springer Nature Switzerland AG. 1-12. doi:10.1007/978-3-030-64987-6_1
- Al-Emran M, Granić A, Al-Sharafi M, Nisreen A, Sarrab M. Examining the roles of students’ beliefs and security concerns for using smartwatches in higher education. Journal of Enterprise Information Management. 2021; 34 (4):1229–1251. doi: 10.1108/JEIM-02-2020-0052. [ CrossRef ] [ Google Scholar ]
- Al-Emran M, Mezhuyev V, Kamaludin A. Technology Acceptance Model in m-learning context: A systematic review. Computers & Education. 2018; 125 :389–412. doi: 10.1016/j.compedu.2018.06.008. [ CrossRef ] [ Google Scholar ]
- Alghazi, S. S., Wong, S. Y., Kamsin, A., Yadegaridehkordi, E., & Shuib, L. (2020). Towards sustainable mobile learning: A brief review of the factors influencing acceptance of the use of mobile phones as learning tools. Sustainability , 12(24), 10.3390/su122410527
- Al-Nuaimi MN, Al-Emran M. Learning management systems and technology acceptance models: A systematic review. Education and Information Technologies. 2021; 26 :5499–5533. doi: 10.1007/s10639-021-10513-3. [ CrossRef ] [ Google Scholar ]
- Al-Rahmi AM, Shamsuddin A, Alturki U, Aldraiweesh A, Yusof FM, Al-Rahmi WM, Aljeraiwi AA. The influence of Information System Success and Technology Acceptance Model on social media factors in education. Sustainability. 2021; 13 (14):7770–7770. doi: 10.3390/su13147770. [ CrossRef ] [ Google Scholar ]
- Al-Rahmi WM, Yahaya N, Aldraiweesh AA, Alamri MM, Aljarboa NA, Alturki U, Aljeraiwi AA. Integrating Technology Acceptance Model with Innovation Diffusion Theory: An empirical investigation on students’ intention to use e-learning systems. IEEE ACCESS. 2019; 7 :26797–26809. doi: 10.1109/ACCESS.2019.2899368. [ CrossRef ] [ Google Scholar ]
- Alruwais NM, Wills G, Wald M. Validating Factors that impact the acceptance and usage of e-assessment by academics in Saudi universities. International Journal of Information and Education Technology. 2017; 7 (10):716–721. doi: 10.18178/ijiet.2017.7.10.960. [ CrossRef ] [ Google Scholar ]
- Anthony B, Kamaludin A, Romli A, et al. blended learning adoption and implementation in higher education: a theoretical and systematic review. Technology, Knowledge and Learning. 2020 doi: 10.1007/s10758-020-09477-z. [ CrossRef ] [ Google Scholar ]
- Bagozzi RP, Warshaw PR. Trying to consume. Journal of Consumer Research. 1990; 17 (2):127–140. doi: 10.1086/208543. [ CrossRef ] [ Google Scholar ]
- Bervell B, Umar IN. Validation of the UTAUT model: Re-considering non-linear relationships of exogeneous variables in higher education technology acceptance research. EURASIA Journal of Mathematics Science and Technology Education. 2017; 13 (10):6471–6490. doi: 10.12973/ejmste/78076. [ CrossRef ] [ Google Scholar ]
- Bhattacherjee A. Understanding information systems continuance: An Expectation-Confirmation Model. MIS Quarterly. 2001; 25 (3):351–370. doi: 10.2307/3250921. [ CrossRef ] [ Google Scholar ]
- Birch A, Irvine V. Preservice teachers’ acceptance of ICT integration in the classroom: Applying the UTAUT model. Educational Media International. 2009; 46 (4):295–315. doi: 10.1080/09523980903387506. [ CrossRef ] [ Google Scholar ]
- Briz-Ponce L, Garcia-Penalvo FJ. An empirical assessment of a Technology Acceptance Model for apps in medical education. Journal of Medical Systems. 2015; 39 (11):176–176. doi: 10.1007/s10916-015-0352-x. [ PubMed ] [ CrossRef ] [ Google Scholar ]
- Calisir F, Gumussoy CA, Bayraktaroglu AE, Karaali D. Predicting the intention to use a web-based learning system: perceived content quality, anxiety, perceived system quality, image, and the Technology Acceptance Model. Human Factors and Ergonomics in Manufacturing & Service Industries. 2014; 24 (5):515–531. doi: 10.1002/hfm.20548. [ CrossRef ] [ Google Scholar ]
- Chang CT, Hajiyev J, Su CR. Examining the students’ behavioral intention to use e-learning in Azerbaijan? The General Extended Technology Acceptance Model for E-Learning approach. Computers & Education. 2017; 111 :128–143. doi: 10.1016/j.compedu.2017.04.010. [ CrossRef ] [ Google Scholar ]
- Chen IJ, Yang KF, Tang FI, Huang CH, Yu S. Applying the technology acceptance model to explore public health nurses’ intentions towards web-based learning: A cross-sectional questionnaire survey. International Journal of Nursing Studies. 2008; 45 (6):869–878. doi: 10.1016/j.ijnurstu.2006.11.011. [ PubMed ] [ CrossRef ] [ Google Scholar ]
- Cheung R, Vogel D. Predicting user acceptance of collaborative technologies: An extension of the technology acceptance model for e-learning. Computers & Education. 2013; 63 :160–175. doi: 10.1016/j.compedu.2012.12.003. [ CrossRef ] [ Google Scholar ]
- Chipps J, Kerr J, Brysiewicz P, Walters F. Survey of university students’ perceptions of learning management systems in a low-resource setting using a Technology Acceptance Model. Computers Informatics Nursing. 2015; 33 (2):71–77. doi: 10.1097/CIN.0000000000000123. [ PubMed ] [ CrossRef ] [ Google Scholar ]
- Chow M, Herold DK, Choo TM, Chan K. Extending the technology acceptance model to explore the intention to use Second Life for enhancing healthcare education. Computers & Education. 2012; 59 (4):1136–1144. doi: 10.1016/j.compedu.2012.05.011. [ CrossRef ] [ Google Scholar ]
- Dakduk, S., Santalla-Banderali, Z., & van der Woude, D. (2018). Acceptance of blended learning in executive education. Online Teaching . SAGE Open, 1-16. doi:10.1177/2158244018800647
- Davis, F. D. (1986). A technology acceptance model for empirically testing new end-user information systems: theory and results. Doctoral dissertation. MIT Sloan School of Management, Cambridge, MA. Retrieved from https://dspace.mit.edu/handle/1721.1/15192
- Davis FD. perceived usefulness, perceived ease of use, and user acceptance of information technology. MIS Quarterly. 1989; 13 (3):319–340. doi: 10.2307/249008. [ CrossRef ] [ Google Scholar ]
- DeLone WH, McLean ER. Information systems success: The quest for the dependent variable. Information System Research. 1992; 3 (1):60–95. doi: 10.1287/isre.3.1.60. [ CrossRef ] [ Google Scholar ]
- Dimitrijević S, Devedžić V. Utilitarian and experiential aspects in acceptance models for learning technology. Educational Technology Research and Development. 2021; 69 (2):627–654. doi: 10.1007/s11423-021-09970-x. [ CrossRef ] [ Google Scholar ]
- El-Masri M, Tarhini A. Factors affecting the adoption of e-learning systems in Qatar and USA: Extending the Unified Theory of Acceptance and Use of Technology 2 (UTAUT2) Educational Technology Research and Development. 2017; 65 (3):743–763. doi: 10.1007/s11423-016-9508-8. [ CrossRef ] [ Google Scholar ]
- Esteban-Millat I, Martinez-Lopez FJ, Pujol-Jover M, Gazquez-Abad JC, Alegret A. An extension of the technology acceptance model for online learning environments. Interactive Learning Environments. 2018; 26 (7):895–910. doi: 10.1080/10494820.2017.1421560. [ CrossRef ] [ Google Scholar ]
- Gamage, S. N., & Tanwar, T. (2018). Factors affecting teachers’ use of ICTs in the classroom: A systematic review of the literature. Information Technologies & International Development, 14, 105-115. Retrieved from https://itidjournal.org/index.php/itid/article/download/1487/1487-4980-1-PB.pdf
- Goodhue DL, Thompson RL. Task-technology fit and individual performance. MIS Quarterly. 1995; 19 (2):213–236. doi: 10.2307/249689. [ CrossRef ] [ Google Scholar ]
- Granić A, Marangunić N. Technology acceptance model in educational context: A systematic literature review. British Journal of Educational Technology. 2019; 50 (5):2572–2593. doi: 10.1111/bjet.12864. [ CrossRef ] [ Google Scholar ]
- Hanif A, Jamal FQ, Imran M. Extending the Technology Acceptance Model for use of e-learning systems by digital learners. IEEE ACCESS. 2018; 6 :73395–73404. doi: 10.1109/ACCESS.2018.2881384. [ CrossRef ] [ Google Scholar ]
- Huang, C. Y., Wang, H. Y., Yang, C. L., & Shiau, S. (2020). A derivation of factors influencing the diffusion and adoption of an open source learning platform. Sustainability , 12(18), doi:10.3390/su12187532
- Huang JH, Lin YR, Chuang ST. Elucidating user behavior of mobile learning - A perspective of the extended Technology Acceptance Model. Electronic Library. 2007; 25 (5):585–598. doi: 10.1108/02640470710829569. [ CrossRef ] [ Google Scholar ]
- Iqbal S, Bhatti ZA. An investigation of university student readiness towards m-learning using Technology Acceptance Model. International Review of Research in Open and Distributed Learning. 2015; 16 (4):83–103. doi: 10.19173/irrodl.v16i4.2351. [ CrossRef ] [ Google Scholar ]
- Jang J, Ko Y, Shin WS, Han I. Augmented reality and virtual reality for learning: An examination using an extended Technology Acceptance Model. IEEE ACCESS. 2021; 9 :6798–6809. doi: 10.1109/ACCESS.2020.3048708. [ CrossRef ] [ Google Scholar ]
- Kaushik MK, Verma D. Determinants of digital learning acceptance behavior: A systematic review of applied theories and implications for higher education. Journal of Applied Research in Higher Education. 2020; 12 (4):659–672. doi: 10.1108/JARHE-06-2018-0105. [ CrossRef ] [ Google Scholar ]
- Kelly H. Path analysis of educator perceptions of open educational resources using the Technology Acceptance Model. International Review of Research in Open and Distance Learning. 2014; 15 (2):26–42. doi: 10.19173/irrodl.v15i2.1715. [ CrossRef ] [ Google Scholar ]
- Kemp A, Palmer E, Strelan P. A taxonomy of factors affecting attitudes towards educational technologies for use with technology acceptance models. British Journal of Educational Technology. 2019; 50 (5):2394–2413. doi: 10.1111/bjet.12833. [ CrossRef ] [ Google Scholar ]
- Lai HJ. Investigating older adults’ decisions to use mobile devices for learning, based on the unified theory of acceptance and use of technology. Interactive Learning Environments. 2020; 28 (7):890–901. doi: 10.1080/10494820.2018.1546748. [ CrossRef ] [ Google Scholar ]
- Lee DY, Lehto MR. User acceptance of You Tube for procedural learning: An extension of the Technology Acceptance Model. Computers & Education. 2013; 61 :193–208. doi: 10.1016/j.compedu.2012.10.001. [ CrossRef ] [ Google Scholar ]
- Lee YH, Hsieh YC, Chen YH. An investigation of employees’ use of e-learning systems: applying the technology acceptance model. Behaviour & Information Technology. 2013; 32 (2):173–189. doi: 10.1080/0144929X.2011.577190. [ CrossRef ] [ Google Scholar ]
- Lee, Y. H., Hsieh, Y. C., & Hsu, C. N. (2011). Adding Innovation Diffusion Theory to the Technology Acceptance Model: Supporting employees’ intentions to use e-learning systems. Educational Technology & Society, 14 (4), 124-137. Retrieved from https://www.ds.unipi.gr/et&s/journals/14_4/12.pdf
- Lemay DJ, Morin MM, Bazelais P, Doleck T. Modeling students’ perceptions of simulation-based learning using the Technology Acceptance Model. Clinical Simulation in Nursing. 2018; 20 :28–37. doi: 10.1016/j.ecns.2018.04.004. [ CrossRef ] [ Google Scholar ]
- Lin, P.H. &; Yeh, S.C. (2019). How motion-control influences a VR-supported technology for mental rotation learning: from the perspectives of playfulness, gender difference and Technology Acceptance Model. International Journal of Human-Computer Interaction, 35 (18), 1736-1746. doi:10.1080/10447318.2019.1571784
- Liu Q, Geertshuis S, Grainger R. Understanding academics’ adoption of learning technologies: A systematic review. Computers & Education. 2020; 151 :103857. doi: 10.1016/j.compedu.2020.103857. [ CrossRef ] [ Google Scholar ]
- Marangunić N, Granić A. Technology acceptance model: a literature review from 1986 to 2013. Universal Access in the Information Society. 2015; 14 (1):81–95. doi: 10.1007/s10209-014-0348-1. [ CrossRef ] [ Google Scholar ]
- Moran M, Hawkes M, El Gayar O. Tablet personal computer integration in higher education: Applying the Unified Theory of Acceptance and Use Technology model to understand supporting factors. Journal of Educational Computing Research. 2010; 42 (1):79–101. doi: 10.2190/EC.42.1.d. [ CrossRef ] [ Google Scholar ]
- Nagy JT. Evaluation of Online Video Usage and Learning Satisfaction: An Extension of the Technology Acceptance Model. International Review of Research in Open and Distributed Learning. 2018; 19 (1):160–185. doi: 10.19173/irrodl.v19i1.2886. [ CrossRef ] [ Google Scholar ]
- Nam CS, Bahn S, Lee R. Acceptance of assistive technology by special education teachers: A structural equation model approach. International Journal of Human-Computer Interaction. 2013; 29 (5):365–377. doi: 10.1080/10447318.2012.711990. [ CrossRef ] [ Google Scholar ]
- Nyasulu C, Chawinga WD. Using the decomposed theory of planned behaviour to understand university students’ adoption of WhatsApp in learning. E-Learning and Digital Media. 2019; 16 (5):413–429. doi: 10.1177/2042753019835906. [ CrossRef ] [ Google Scholar ]
- Oliver RL. A cognitive model of the antecedents and consequences of satisfaction decisions. Journal of Marketing Research. 1980; 17 (4):460–469. doi: 10.2307/3150499. [ CrossRef ] [ Google Scholar ]
- Padilla-Melendez A, del Aguila-Obra AR, Garrido-Moreno A. Perceived playfulness, gender differences and technology acceptance model in a blended learning scenario. Computers & Education. 2013; 63 :306–317. doi: 10.1016/j.compedu.2012.12.014. [ CrossRef ] [ Google Scholar ]
- Panigrahi R, Srivastava PR, Sharma D. Online learning: Adoption, continuance, and learning outcome - A review of literature. International Journal of Information Management. 2018; 43 :1–14. doi: 10.1016/j.ijinfomgt.2018.05.005. [ CrossRef ] [ Google Scholar ]
- Park E, Kwon SJ. The adoption of teaching assistant robots: a technology acceptance model approach. Program-Electronic Library and Information Systems. 2016; 50 (4):354–366. doi: 10.1108/PROG-02-2016-0017. [ CrossRef ] [ Google Scholar ]
- Park, S. Y. (2009). An Analysis of the Technology Acceptance Model in Understanding University Students’ Behavioral Intention to Use e-Learning. Educational Technology & Society, 12 (3), 150-162. Retrieved from: https://www.jstor.org/stable/jeductechsoci.12.3.150
- Park SY, Nam MW, Cha SB. University students’ behavioral intention to use mobile learning: Evaluating the technology acceptance model. British Journal of Educational Technology. 2012; 43 (4):592–605. doi: 10.1111/j.1467-8535.2011.01229.x. [ CrossRef ] [ Google Scholar ]
- Prasetyo YT, Ong AKS, Concepcion GKF, Navata FMB, Robles RAV, Tomagos IJT, Redi AANP. Determining factors affecting acceptance of e-learning platforms during the COVID-19 pandemic: Integrating extended Technology Acceptance Model and DeLone & McLean IS Success Model. Sustainability. 2021; 13 (15):8365–8365. doi: 10.3390/su13158365. [ CrossRef ] [ Google Scholar ]
- Raman, A., & Don, Y. (2013). preservice teachers’ acceptance of learning management software: an application of the UTAUT2 Model. International Education Studies , 6(7), doi:10.5539/ies.v6n7p157
- Roca JC, Chiu CM, Martinez FJ. Understanding e-learning continuance intention: An extension of the Technology Acceptance Model. International Journal of Human-Computer Studies. 2006; 64 (8):683–696. doi: 10.1016/j.ijhcs.2006.01.003. [ CrossRef ] [ Google Scholar ]
- Rogers E. Diffusion of Innovations. New York, NY: The Free Press; 1962. [ Google Scholar ]
- Rogers E. Diffusion of Innovations. 4. New York, NY: The Free Press; 1995. [ Google Scholar ]
- Rogers RW. A protection motivation theory of fear appeals and attitude change. Journal of Psychology. 1975; 91 (1):93–114. doi: 10.1080/00223980.1975.9915803. [ PubMed ] [ CrossRef ] [ Google Scholar ]
- Saade R, Bahli B. The impact of cognitive absorption on perceived usefulness and perceived ease of use in on-line learning: an extension of the technology acceptance model. Information & Management. 2005; 42 (2):317–327. doi: 10.1016/j.im.2003.12.013. [ CrossRef ] [ Google Scholar ]
- Sadaf A, Gezer T. Exploring factors that influence teachers’ intentions to integrate digital literacy using the decomposed theory of planned behaviour. Journal of Digital Learning in Teacher Education. 2020; 36 (3):1–22. doi: 10.1080/21532974.2020.1719244. [ CrossRef ] [ Google Scholar ]
- Salloum SA, Alhamad AQM, Al-Emran M, Monem AA, Shaalan K. Exploring students’ acceptance of e-learning through the development of a comprehensive Technology Acceptance Model. IEEE ACCESS. 2019; 7 :128445–128462. doi: 10.1109/ACCESS.2019.2939467. [ CrossRef ] [ Google Scholar ]
- Scherer, R., Siddiq, F., & Tondeur, J. (2019). The technology acceptance model (TAM): A meta-analytic structural equation modeling approach to explaining teachers’ adoption of digital technology in education. Computers & Education, 128 , 13-35. doi:0.1016/j.compedu.2018.09.009
- Schoonenboom J. Using an adapted, task-level technology acceptance model to explain why instructors in higher education intend to use some learning management system tools more than others. Computers & Education. 2014; 71 :247–256. doi: 10.1016/j.compedu.2013.09.016. [ CrossRef ] [ Google Scholar ]
- Shyu SHP, Huang JH. Elucidating usage of e-government learning: A perspective of the extended Technology Acceptance Model. Government Information Quarterly. 2011; 28 (4):491–502. doi: 10.1016/j.giq.2011.04.002. [ CrossRef ] [ Google Scholar ]
- Song YJ, Kong SC. Investigating students’ acceptance of a statistics learning platform using Technology Acceptance Model. Journal of Educational Computing Research. 2017; 55 (6):865–897. doi: 10.1177/0735633116688320. [ CrossRef ] [ Google Scholar ]
- Straub ET. Understanding technology adoption: Theory and future directions for informal learning. Review of Educational Research. 2009; 79 (2):625–649. doi: 10.3102/0034654308325896. [ CrossRef ] [ Google Scholar ]
- Tarhini A, Hone K, Liu XH. Measuring the moderating effect of gender and age on e-learning acceptance in England: A structural equation modeling approach for an extended Technology Acceptance Model. Journal of Educational Computing Research. 2014; 51 (2):163–184. doi: 10.2190/EC.51.2.b. [ CrossRef ] [ Google Scholar ]
- Tarhini A, Hone K, Liu XH, Tarhini T. Examining the moderating effect of individual-level cultural values on users’ acceptance of E-learning in developing countries: A structural equation modeling of an extended technology acceptance model. Interactive Learning Environments. 2016; 25 (3):306–328. doi: 10.1080/10494820.2015.1122635. [ CrossRef ] [ Google Scholar ]
- Tawafak RM, Romli ABT, Arshah RB. Continued intention to use UCOM: Four factors for integrating with a Technology Acceptance Model to moderate the satisfaction of learning. IEEE ACCESS. 2018; 6 :66481–66498. doi: 10.1109/ACCESS.2018.2877760. [ CrossRef ] [ Google Scholar ]
- Taylor, S., & Todd, P. A. (1995). Understanding information technology usage: A test of competing models. Information Systems Research , 6 (2), 144-176. Retrieved from http://www.jstor.org/stable/23011007
- Teo T. Modelling technology acceptance in education: A study of pre-service teachers. Computers & Education. 2009; 52 (2):302–312. doi: 10.1016/j.compedu.2008.08.006. [ CrossRef ] [ Google Scholar ]
- Teo T. A path analysis of pre-service teachers’ attitudes to computer use: applying and extending the technology acceptance model in an educational context. Interactive Learning Environments. 2010; 18 (1):65–79. doi: 10.1080/10494820802231327. [ CrossRef ] [ Google Scholar ]
- Tiwari P. Measuring the impact of students” attitude towards adoption of online classes during COVID 19: Integrating UTAUT Model with perceived cost. Test Engineering and Management. 2020; 83 (1):8374–8382. doi: 10.1007/s10639-021-10573-5. [ CrossRef ] [ Google Scholar ]
- Tsai HYS, Rikard RV, Cotten SR, Shillair R. Senior technology exploration, learning, and acceptance (STELA) model: from exploration to use - a longitudinal randomized controlled trial. Educational Gerontology. 2019; 45 (12):728–743. doi: 10.1080/03601277.2019.1690802. [ CrossRef ] [ Google Scholar ]
- Vanduhe VZ, Nat M, Hasan HF. continuance intentions to use gamification for training in higher education: Integrating the Technology Acceptance Model (TAM), social motivation, and Task Technology Fit (TTF) IEEE ACCESS. 2020; 8 :21473–21484. doi: 10.1109/ACCESS.2020.2966179. [ CrossRef ] [ Google Scholar ]
- Venkatesh V, Thong JY, Xu X. Consumer acceptance and use of information technology: Extending the Unified Theory of Acceptance and Use of Technology. MIS Quarterly. 2012; 36 :157–178. doi: 10.2307/41410412. [ CrossRef ] [ Google Scholar ]
- Venkatesh V, Morris MG, Davis GB, Davis FD. User acceptance of information technology: Toward a unified view. MIS Quarterly. 2003; 27 (3):425–478. doi: 10.2307/30036540. [ CrossRef ] [ Google Scholar ]
- Yakubu MN, Dasuki SI. Factors affecting the adoption of e-learning technologies among higher education students in Nigeria A structural equation modelling approach. Information Development. 2019; 35 (3):492–502. doi: 10.1177/0266666918765907. [ CrossRef ] [ Google Scholar ]
- Yi MY, Hwang YJ. Predicting the use of web-based information systems: self-efficacy, enjoyment, learning goal orientation, and the Technology Acceptance Model. International Journal of Human-Computer Studies. 2003; 59 (4):431–449. doi: 10.1016/S1071-5819(03)00114-9. [ CrossRef ] [ Google Scholar ]
- Yu, Z. G. (2020). Extending the learning Technology Acceptance Model of WeChat by adding new psychological constructs. Journal of Educational Computing Research, 58(6), 1121-1143. doi.10.1177/0735633120923772

- Previous Article
- Next Article
Promises and Pitfalls of Technology
Politics and privacy, private-sector influence and big tech, state competition and conflict, author biography, how is technology changing the world, and how should the world change technology.
- Split-Screen
- Article contents
- Figures & tables
- Supplementary Data
- Peer Review
- Open the PDF for in another window
- Guest Access
- Get Permissions
- Cite Icon Cite
- Search Site
Josephine Wolff; How Is Technology Changing the World, and How Should the World Change Technology?. Global Perspectives 1 February 2021; 2 (1): 27353. doi: https://doi.org/10.1525/gp.2021.27353
Download citation file:
- Ris (Zotero)
- Reference Manager
Technologies are becoming increasingly complicated and increasingly interconnected. Cars, airplanes, medical devices, financial transactions, and electricity systems all rely on more computer software than they ever have before, making them seem both harder to understand and, in some cases, harder to control. Government and corporate surveillance of individuals and information processing relies largely on digital technologies and artificial intelligence, and therefore involves less human-to-human contact than ever before and more opportunities for biases to be embedded and codified in our technological systems in ways we may not even be able to identify or recognize. Bioengineering advances are opening up new terrain for challenging philosophical, political, and economic questions regarding human-natural relations. Additionally, the management of these large and small devices and systems is increasingly done through the cloud, so that control over them is both very remote and removed from direct human or social control. The study of how to make technologies like artificial intelligence or the Internet of Things “explainable” has become its own area of research because it is so difficult to understand how they work or what is at fault when something goes wrong (Gunning and Aha 2019) .
This growing complexity makes it more difficult than ever—and more imperative than ever—for scholars to probe how technological advancements are altering life around the world in both positive and negative ways and what social, political, and legal tools are needed to help shape the development and design of technology in beneficial directions. This can seem like an impossible task in light of the rapid pace of technological change and the sense that its continued advancement is inevitable, but many countries around the world are only just beginning to take significant steps toward regulating computer technologies and are still in the process of radically rethinking the rules governing global data flows and exchange of technology across borders.
These are exciting times not just for technological development but also for technology policy—our technologies may be more advanced and complicated than ever but so, too, are our understandings of how they can best be leveraged, protected, and even constrained. The structures of technological systems as determined largely by government and institutional policies and those structures have tremendous implications for social organization and agency, ranging from open source, open systems that are highly distributed and decentralized, to those that are tightly controlled and closed, structured according to stricter and more hierarchical models. And just as our understanding of the governance of technology is developing in new and interesting ways, so, too, is our understanding of the social, cultural, environmental, and political dimensions of emerging technologies. We are realizing both the challenges and the importance of mapping out the full range of ways that technology is changing our society, what we want those changes to look like, and what tools we have to try to influence and guide those shifts.
Technology can be a source of tremendous optimism. It can help overcome some of the greatest challenges our society faces, including climate change, famine, and disease. For those who believe in the power of innovation and the promise of creative destruction to advance economic development and lead to better quality of life, technology is a vital economic driver (Schumpeter 1942) . But it can also be a tool of tremendous fear and oppression, embedding biases in automated decision-making processes and information-processing algorithms, exacerbating economic and social inequalities within and between countries to a staggering degree, or creating new weapons and avenues for attack unlike any we have had to face in the past. Scholars have even contended that the emergence of the term technology in the nineteenth and twentieth centuries marked a shift from viewing individual pieces of machinery as a means to achieving political and social progress to the more dangerous, or hazardous, view that larger-scale, more complex technological systems were a semiautonomous form of progress in and of themselves (Marx 2010) . More recently, technologists have sharply criticized what they view as a wave of new Luddites, people intent on slowing the development of technology and turning back the clock on innovation as a means of mitigating the societal impacts of technological change (Marlowe 1970) .
At the heart of fights over new technologies and their resulting global changes are often two conflicting visions of technology: a fundamentally optimistic one that believes humans use it as a tool to achieve greater goals, and a fundamentally pessimistic one that holds that technological systems have reached a point beyond our control. Technology philosophers have argued that neither of these views is wholly accurate and that a purely optimistic or pessimistic view of technology is insufficient to capture the nuances and complexity of our relationship to technology (Oberdiek and Tiles 1995) . Understanding technology and how we can make better decisions about designing, deploying, and refining it requires capturing that nuance and complexity through in-depth analysis of the impacts of different technological advancements and the ways they have played out in all their complicated and controversial messiness across the world.
These impacts are often unpredictable as technologies are adopted in new contexts and come to be used in ways that sometimes diverge significantly from the use cases envisioned by their designers. The internet, designed to help transmit information between computer networks, became a crucial vehicle for commerce, introducing unexpected avenues for crime and financial fraud. Social media platforms like Facebook and Twitter, designed to connect friends and families through sharing photographs and life updates, became focal points of election controversies and political influence. Cryptocurrencies, originally intended as a means of decentralized digital cash, have become a significant environmental hazard as more and more computing resources are devoted to mining these forms of virtual money. One of the crucial challenges in this area is therefore recognizing, documenting, and even anticipating some of these unexpected consequences and providing mechanisms to technologists for how to think through the impacts of their work, as well as possible other paths to different outcomes (Verbeek 2006) . And just as technological innovations can cause unexpected harm, they can also bring about extraordinary benefits—new vaccines and medicines to address global pandemics and save thousands of lives, new sources of energy that can drastically reduce emissions and help combat climate change, new modes of education that can reach people who would otherwise have no access to schooling. Regulating technology therefore requires a careful balance of mitigating risks without overly restricting potentially beneficial innovations.
Nations around the world have taken very different approaches to governing emerging technologies and have adopted a range of different technologies themselves in pursuit of more modern governance structures and processes (Braman 2009) . In Europe, the precautionary principle has guided much more anticipatory regulation aimed at addressing the risks presented by technologies even before they are fully realized. For instance, the European Union’s General Data Protection Regulation focuses on the responsibilities of data controllers and processors to provide individuals with access to their data and information about how that data is being used not just as a means of addressing existing security and privacy threats, such as data breaches, but also to protect against future developments and uses of that data for artificial intelligence and automated decision-making purposes. In Germany, Technische Überwachungsvereine, or TÜVs, perform regular tests and inspections of technological systems to assess and minimize risks over time, as the tech landscape evolves. In the United States, by contrast, there is much greater reliance on litigation and liability regimes to address safety and security failings after-the-fact. These different approaches reflect not just the different legal and regulatory mechanisms and philosophies of different nations but also the different ways those nations prioritize rapid development of the technology industry versus safety, security, and individual control. Typically, governance innovations move much more slowly than technological innovations, and regulations can lag years, or even decades, behind the technologies they aim to govern.
In addition to this varied set of national regulatory approaches, a variety of international and nongovernmental organizations also contribute to the process of developing standards, rules, and norms for new technologies, including the International Organization for Standardization and the International Telecommunication Union. These multilateral and NGO actors play an especially important role in trying to define appropriate boundaries for the use of new technologies by governments as instruments of control for the state.
At the same time that policymakers are under scrutiny both for their decisions about how to regulate technology as well as their decisions about how and when to adopt technologies like facial recognition themselves, technology firms and designers have also come under increasing criticism. Growing recognition that the design of technologies can have far-reaching social and political implications means that there is more pressure on technologists to take into consideration the consequences of their decisions early on in the design process (Vincenti 1993; Winner 1980) . The question of how technologists should incorporate these social dimensions into their design and development processes is an old one, and debate on these issues dates back to the 1970s, but it remains an urgent and often overlooked part of the puzzle because so many of the supposedly systematic mechanisms for assessing the impacts of new technologies in both the private and public sectors are primarily bureaucratic, symbolic processes rather than carrying any real weight or influence.
Technologists are often ill-equipped or unwilling to respond to the sorts of social problems that their creations have—often unwittingly—exacerbated, and instead point to governments and lawmakers to address those problems (Zuckerberg 2019) . But governments often have few incentives to engage in this area. This is because setting clear standards and rules for an ever-evolving technological landscape can be extremely challenging, because enforcement of those rules can be a significant undertaking requiring considerable expertise, and because the tech sector is a major source of jobs and revenue for many countries that may fear losing those benefits if they constrain companies too much. This indicates not just a need for clearer incentives and better policies for both private- and public-sector entities but also a need for new mechanisms whereby the technology development and design process can be influenced and assessed by people with a wider range of experiences and expertise. If we want technologies to be designed with an eye to their impacts, who is responsible for predicting, measuring, and mitigating those impacts throughout the design process? Involving policymakers in that process in a more meaningful way will also require training them to have the analytic and technical capacity to more fully engage with technologists and understand more fully the implications of their decisions.
At the same time that tech companies seem unwilling or unable to rein in their creations, many also fear they wield too much power, in some cases all but replacing governments and international organizations in their ability to make decisions that affect millions of people worldwide and control access to information, platforms, and audiences (Kilovaty 2020) . Regulators around the world have begun considering whether some of these companies have become so powerful that they violate the tenets of antitrust laws, but it can be difficult for governments to identify exactly what those violations are, especially in the context of an industry where the largest players often provide their customers with free services. And the platforms and services developed by tech companies are often wielded most powerfully and dangerously not directly by their private-sector creators and operators but instead by states themselves for widespread misinformation campaigns that serve political purposes (Nye 2018) .
Since the largest private entities in the tech sector operate in many countries, they are often better poised to implement global changes to the technological ecosystem than individual states or regulatory bodies, creating new challenges to existing governance structures and hierarchies. Just as it can be challenging to provide oversight for government use of technologies, so, too, oversight of the biggest tech companies, which have more resources, reach, and power than many nations, can prove to be a daunting task. The rise of network forms of organization and the growing gig economy have added to these challenges, making it even harder for regulators to fully address the breadth of these companies’ operations (Powell 1990) . The private-public partnerships that have emerged around energy, transportation, medical, and cyber technologies further complicate this picture, blurring the line between the public and private sectors and raising critical questions about the role of each in providing critical infrastructure, health care, and security. How can and should private tech companies operating in these different sectors be governed, and what types of influence do they exert over regulators? How feasible are different policy proposals aimed at technological innovation, and what potential unintended consequences might they have?
Conflict between countries has also spilled over significantly into the private sector in recent years, most notably in the case of tensions between the United States and China over which technologies developed in each country will be permitted by the other and which will be purchased by other customers, outside those two countries. Countries competing to develop the best technology is not a new phenomenon, but the current conflicts have major international ramifications and will influence the infrastructure that is installed and used around the world for years to come. Untangling the different factors that feed into these tussles as well as whom they benefit and whom they leave at a disadvantage is crucial for understanding how governments can most effectively foster technological innovation and invention domestically as well as the global consequences of those efforts. As much of the world is forced to choose between buying technology from the United States or from China, how should we understand the long-term impacts of those choices and the options available to people in countries without robust domestic tech industries? Does the global spread of technologies help fuel further innovation in countries with smaller tech markets, or does it reinforce the dominance of the states that are already most prominent in this sector? How can research universities maintain global collaborations and research communities in light of these national competitions, and what role does government research and development spending play in fostering innovation within its own borders and worldwide? How should intellectual property protections evolve to meet the demands of the technology industry, and how can those protections be enforced globally?
These conflicts between countries sometimes appear to challenge the feasibility of truly global technologies and networks that operate across all countries through standardized protocols and design features. Organizations like the International Organization for Standardization, the World Intellectual Property Organization, the United Nations Industrial Development Organization, and many others have tried to harmonize these policies and protocols across different countries for years, but have met with limited success when it comes to resolving the issues of greatest tension and disagreement among nations. For technology to operate in a global environment, there is a need for a much greater degree of coordination among countries and the development of common standards and norms, but governments continue to struggle to agree not just on those norms themselves but even the appropriate venue and processes for developing them. Without greater global cooperation, is it possible to maintain a global network like the internet or to promote the spread of new technologies around the world to address challenges of sustainability? What might help incentivize that cooperation moving forward, and what could new structures and process for governance of global technologies look like? Why has the tech industry’s self-regulation culture persisted? Do the same traditional drivers for public policy, such as politics of harmonization and path dependency in policy-making, still sufficiently explain policy outcomes in this space? As new technologies and their applications spread across the globe in uneven ways, how and when do they create forces of change from unexpected places?
These are some of the questions that we hope to address in the Technology and Global Change section through articles that tackle new dimensions of the global landscape of designing, developing, deploying, and assessing new technologies to address major challenges the world faces. Understanding these processes requires synthesizing knowledge from a range of different fields, including sociology, political science, economics, and history, as well as technical fields such as engineering, climate science, and computer science. A crucial part of understanding how technology has created global change and, in turn, how global changes have influenced the development of new technologies is understanding the technologies themselves in all their richness and complexity—how they work, the limits of what they can do, what they were designed to do, how they are actually used. Just as technologies themselves are becoming more complicated, so are their embeddings and relationships to the larger social, political, and legal contexts in which they exist. Scholars across all disciplines are encouraged to join us in untangling those complexities.
Josephine Wolff is an associate professor of cybersecurity policy at the Fletcher School of Law and Diplomacy at Tufts University. Her book You’ll See This Message When It Is Too Late: The Legal and Economic Aftermath of Cybersecurity Breaches was published by MIT Press in 2018.
Recipient(s) will receive an email with a link to 'How Is Technology Changing the World, and How Should the World Change Technology?' and will not need an account to access the content.
Subject: How Is Technology Changing the World, and How Should the World Change Technology?
(Optional message may have a maximum of 1000 characters.)
Citing articles via
Email alerts, affiliations.
- Special Collections
- Review Symposia
- Info for Authors
- Info for Librarians
- Editorial Team
- Emerging Scholars Forum
- Open Access
- Online ISSN 2575-7350
- Copyright © 2024 The Regents of the University of California. All Rights Reserved.
Stay Informed
Disciplines.
- Ancient World
- Anthropology
- Communication
- Criminology & Criminal Justice
- Film & Media Studies
- Food & Wine
- Browse All Disciplines
- Browse All Courses
- Book Authors
- Booksellers
- Instructions
- Journal Authors
- Journal Editors
- Media & Journalists
- Planned Giving
About UC Press
- Press Releases
- Seasonal Catalog
- Acquisitions Editors
- Customer Service
- Exam/Desk Requests
- Media Inquiries
- Print-Disability
- Rights & Permissions
- UC Press Foundation
- © Copyright 2024 by the Regents of the University of California. All rights reserved. Privacy policy Accessibility
This Feature Is Available To Subscribers Only
Sign In or Create an Account
Technology Adoption
- Reference work entry
- First Online: 01 January 2018
- pp 1719–1723
- Cite this reference work entry
- Chris Forman 4 ,
- Avi Goldfarb 5 &
- Shane Greenstein 6
402 Accesses
Using examples from information technology adoption, we emphasize the role of costs, benefits, communications channels and dynamic considerations in the decision to adopt new technology. We discuss differences between adoption by consumers and adoption by firms. We emphasize the adoption of business process innovations, which alter organizational practices and often involve the post-adoption invention of complementary business processes and adaptations. Within the context of business adoption, we discuss the inherent challenges in identifying the decision maker and the role of competition in influencing the benefits to adoption.
This is a preview of subscription content, log in via an institution to check access.
Access this chapter
- Available as PDF
- Read on any device
- Instant download
- Own it forever
- Available as EPUB and PDF
- Durable hardcover edition
- Dispatched in 3 to 5 business days
- Free shipping worldwide - see info
Tax calculation will be finalised at checkout
Purchases are for personal use only
Institutional subscriptions
Astebro, T. 2002. Noncapital investment costs and the adoption of CAD and CNC in U.S. metalworking industries. RAND Journal of Economics 33: 672–688.
Article Google Scholar
Astebro, T. 2004. Sunk costs and the depth and probability of technology adoption. Journal of Industrial Economics 52: 381–399.
Baker, G., and T.N. Hubbard. 2003. Make versus buy in trucking: Asset ownership, job design, and information. American Economic Review 93: 551–572.
Baker, G., and T.N. Hubbard. 2004. Contractibility and asset ownership: On-board computers and governance in US trucking. Quarterly Journal of Economics 119: 1443–1480.
Bloom N, Garicano L, Sadun R, Van Reenen J. 2011. The distinct effects of information technology and communication technology on firm organization. Working paper, Stanford University.
Google Scholar
Bresnahan, T. and Greenstein, S. 1996. Technical progress and co-invention in computing and in the use of computers. Brookings Papers on Economic Activity: Microeconomics 1–78.
Bresnahan, T.F., E. Brynjolfsson, and L.M. Hitt. 2002. Information technology, workplace organization, and the demand for skilled labor: Firm-level evidence. Quarterly Journal of Economics 117: 339–376.
David, P. A. 1969. A contribution to the theory of diffusion. Memorandum No. 71, Stanford Center for Research in Economic Growth, Stanford University.
Forman, C. 2005. The corporate digital divide: Determinants of internet adoption. Management Science 51: 641–654.
Forman, C., and A. Goldfarb. 2006. Diffusion of information and communications technology to business. In Handbooks in information systems , Economics and Information Systems, vol. 1, ed. T. Hendershott. Amsterdam: Elsevier.
Forman, C., A. Goldfarb, and S. Greenstein. 2003a. Which industries use the internet? In Organizing the new industrial economy , ed. M. Baye. Amsterdam: Elsevier.
Forman, C., A. Goldfarb, and S. Greenstein. 2003b. The geographic dispersion of commercial internet use. In Rethinking rights and regulations: Institutional responses to new communications technologies , ed. L. Cranor and S. Wildman. Cambridge, MA: The MIT Press.
Forman, C., A. Goldfarb, and S. Greenstein. 2005. How did location affect adoption of the internet by commercial establishments? Urban density versus global village. Journal of Urban Economics 58: 389–420.
Forman, C., A. Goldfarb, and S. Greenstein. 2008. Understanding inputs into innovation: Do cities substitute for internal firm resources? Journal of Economics and Management Strategy 17: 295–316.
Forman, C., A. Goldfarb, and S. Greenstein. 2012. The internet and local wages: A puzzle. American Economic Review 102: 556–575.
Goldfarb, A. 2006. The (teaching) role of universities in the diffusion of the internet. International Journal of Industrial Organization 24: 203–225.
Goldfarb, A., and J. Prince. 2008. Internet adoption and usage patterns are different: Implications for the digital divide. Information Economics and Policy 20: 2–15.
Goolsbee, A., and P. Klenow. 2002. Evidence on learning and network externalities in the diffusion of home computers. Journal of Law and Economics 45: 317–344.
Hubbard, T. 2000. The demand for monitoring technologies: The case of trucking. Quarterly Journal of Economics 115: 533–560.
Hubbard, T. 2003. Information, decisions, and productivity: On-board computers and capacity utilization in trucking. American Economic Review 93: 1328–1353.
McElheran, K. 2012. Do market leaders lead in business process adoption? Customers and co-invention in e-business adoption. Working paper, Harvard Business School.
Prince, J. 2008. Repeat purchase amid rapid quality improvement: Structural estimation of demand for personal computers. Journal of Economics and Management Strategy 17: 1–33.
Rogers, E. 1995. The diffusion of innovations , 4th ed. New York: Free Press.
Stoneman, P. 2002. The economics of technological diffusion . Oxford: Blackwell.
Download references
Author information
Authors and affiliations.
Georgia Institute of Technology, Atlanta, GA, USA
Chris Forman
University of Toronto, Rotman School of Management, 105St George Street, Toronto, OH, Canada
Avi Goldfarb
Northwestern University, Evanston, IL, USA
Shane Greenstein
You can also search for this author in PubMed Google Scholar
Corresponding author
Correspondence to Chris Forman .
Editor information
Editors and affiliations.
GSBPP, Naval Postgraduate School, Monterey, CA, USA
Berkeley Research Group, LLC, Emeryville, CA, USA
David J. Teece
Haas School of Business, University of California, Berkeley, Berkeley, CA, USA
Copyright information
© 2018 Macmillan Publishers Ltd., part of Springer Nature
About this entry
Cite this entry.
Forman, C., Goldfarb, A., Greenstein, S. (2018). Technology Adoption. In: Augier, M., Teece, D.J. (eds) The Palgrave Encyclopedia of Strategic Management. Palgrave Macmillan, London. https://doi.org/10.1057/978-1-137-00772-8_379
Download citation
DOI : https://doi.org/10.1057/978-1-137-00772-8_379
Published : 01 May 2018
Publisher Name : Palgrave Macmillan, London
Print ISBN : 978-0-230-53721-7
Online ISBN : 978-1-137-00772-8
eBook Packages : Business and Management Reference Module Humanities and Social Sciences Reference Module Business, Economics and Social Sciences
Share this entry
Anyone you share the following link with will be able to read this content:
Sorry, a shareable link is not currently available for this article.
Provided by the Springer Nature SharedIt content-sharing initiative
- Publish with us
Policies and ethics
- Find a journal
- Track your research
- Open access
- Published: 19 April 2024
A scoping review of continuous quality improvement in healthcare system: conceptualization, models and tools, barriers and facilitators, and impact
- Aklilu Endalamaw 1 , 2 ,
- Resham B Khatri 1 , 3 ,
- Tesfaye Setegn Mengistu 1 , 2 ,
- Daniel Erku 1 , 4 , 5 ,
- Eskinder Wolka 6 ,
- Anteneh Zewdie 6 &
- Yibeltal Assefa 1
BMC Health Services Research volume 24 , Article number: 487 ( 2024 ) Cite this article
841 Accesses
Metrics details
The growing adoption of continuous quality improvement (CQI) initiatives in healthcare has generated a surge in research interest to gain a deeper understanding of CQI. However, comprehensive evidence regarding the diverse facets of CQI in healthcare has been limited. Our review sought to comprehensively grasp the conceptualization and principles of CQI, explore existing models and tools, analyze barriers and facilitators, and investigate its overall impacts.
This qualitative scoping review was conducted using Arksey and O’Malley’s methodological framework. We searched articles in PubMed, Web of Science, Scopus, and EMBASE databases. In addition, we accessed articles from Google Scholar. We used mixed-method analysis, including qualitative content analysis and quantitative descriptive for quantitative findings to summarize findings and PRISMA extension for scoping reviews (PRISMA-ScR) framework to report the overall works.
A total of 87 articles, which covered 14 CQI models, were included in the review. While 19 tools were used for CQI models and initiatives, Plan-Do-Study/Check-Act cycle was the commonly employed model to understand the CQI implementation process. The main reported purposes of using CQI, as its positive impact, are to improve the structure of the health system (e.g., leadership, health workforce, health technology use, supplies, and costs), enhance healthcare delivery processes and outputs (e.g., care coordination and linkages, satisfaction, accessibility, continuity of care, safety, and efficiency), and improve treatment outcome (reduce morbidity and mortality). The implementation of CQI is not without challenges. There are cultural (i.e., resistance/reluctance to quality-focused culture and fear of blame or punishment), technical, structural (related to organizational structure, processes, and systems), and strategic (inadequate planning and inappropriate goals) related barriers that were commonly reported during the implementation of CQI.
Conclusions
Implementing CQI initiatives necessitates thoroughly comprehending key principles such as teamwork and timeline. To effectively address challenges, it’s crucial to identify obstacles and implement optimal interventions proactively. Healthcare professionals and leaders need to be mentally equipped and cognizant of the significant role CQI initiatives play in achieving purposes for quality of care.
Peer Review reports
Continuous quality improvement (CQI) initiative is a crucial initiative aimed at enhancing quality in the health system that has gradually been adopted in the healthcare industry. In the early 20th century, Shewhart laid the foundation for quality improvement by describing three essential steps for process improvement: specification, production, and inspection [ 1 , 2 ]. Then, Deming expanded Shewhart’s three-step model into ‘plan, do, study/check, and act’ (PDSA or PDCA) cycle, which was applied to management practices in Japan in the 1950s [ 3 ] and was gradually translated into the health system. In 1991, Kuperman applied a CQI approach to healthcare, comprising selecting a process to be improved, assembling a team of expert clinicians that understands the process and the outcomes, determining key steps in the process and expected outcomes, collecting data that measure the key process steps and outcomes, and providing data feedback to the practitioners [ 4 ]. These philosophies have served as the baseline for the foundation of principles for continuous improvement [ 5 ].
Continuous quality improvement fosters a culture of continuous learning, innovation, and improvement. It encourages proactive identification and resolution of problems, promotes employee engagement and empowerment, encourages trust and respect, and aims for better quality of care [ 6 , 7 ]. These characteristics drive the interaction of CQI with other quality improvement projects, such as quality assurance and total quality management [ 8 ]. Quality assurance primarily focuses on identifying deviations or errors through inspections, audits, and formal reviews, often settling for what is considered ‘good enough’, rather than pursuing the highest possible standards [ 9 , 10 ], while total quality management is implemented as the management philosophy and system to improve all aspects of an organization continuously [ 11 ].
Continuous quality improvement has been implemented to provide quality care. However, providing effective healthcare is a complicated and complex task in achieving the desired health outcomes and the overall well-being of individuals and populations. It necessitates tackling issues, including access, patient safety, medical advances, care coordination, patient-centered care, and quality monitoring [ 12 , 13 ], rooted long ago. It is assumed that the history of quality improvement in healthcare started in 1854 when Florence Nightingale introduced quality improvement documentation [ 14 ]. Over the passing decades, Donabedian introduced structure, processes, and outcomes as quality of care components in 1966 [ 15 ]. More comprehensively, the Institute of Medicine in the United States of America (USA) has identified effectiveness, efficiency, equity, patient-centredness, safety, and timeliness as the components of quality of care [ 16 ]. Moreover, quality of care has recently been considered an integral part of universal health coverage (UHC) [ 17 ], which requires initiatives to mobilise essential inputs [ 18 ].
While the overall objective of CQI in health system is to enhance the quality of care, it is important to note that the purposes and principles of CQI can vary across different contexts [ 19 , 20 ]. This variation has sparked growing research interest. For instance, a review of CQI approaches for capacity building addressed its role in health workforce development [ 21 ]. Another systematic review, based on random-controlled design studies, assessed the effectiveness of CQI using training as an intervention and the PDSA model [ 22 ]. As a research gap, the former review was not directly related to the comprehensive elements of quality of care, while the latter focused solely on the impact of training using the PDSA model, among other potential models. Additionally, a review conducted in 2015 aimed to identify barriers and facilitators of CQI in Canadian contexts [ 23 ]. However, all these reviews presented different perspectives and investigated distinct outcomes. This suggests that there is still much to explore in terms of comprehensively understanding the various aspects of CQI initiatives in healthcare.
As a result, we conducted a scoping review to address several aspects of CQI. Scoping reviews serve as a valuable tool for systematically mapping the existing literature on a specific topic. They are instrumental when dealing with heterogeneous or complex bodies of research. Scoping reviews provide a comprehensive overview by summarizing and disseminating findings across multiple studies, even when evidence varies significantly [ 24 ]. In our specific scoping review, we included various types of literature, including systematic reviews, to enhance our understanding of CQI.
This scoping review examined how CQI is conceptualized and measured and investigated models and tools for its application while identifying implementation challenges and facilitators. It also analyzed the purposes and impact of CQI on the health systems, providing valuable insights for enhancing healthcare quality.
Protocol registration and results reporting
Protocol registration for this scoping review was not conducted. Arksey and O’Malley’s methodological framework was utilized to conduct this scoping review [ 25 ]. The scoping review procedures start by defining the research questions, identifying relevant literature, selecting articles, extracting data, and summarizing the results. The review findings are reported using the PRISMA extension for a scoping review (PRISMA-ScR) [ 26 ]. McGowan and colleagues also advised researchers to report findings from scoping reviews using PRISMA-ScR [ 27 ].
Defining the research problems
This review aims to comprehensively explore the conceptualization, models, tools, barriers, facilitators, and impacts of CQI within the healthcare system worldwide. Specifically, we address the following research questions: (1) How has CQI been defined across various contexts? (2) What are the diverse approaches to implementing CQI in healthcare settings? (3) Which tools are commonly employed for CQI implementation ? (4) What barriers hinder and facilitators support successful CQI initiatives? and (5) What effects CQI initiatives have on the overall care quality?
Information source and search strategy
We conducted the search in PubMed, Web of Science, Scopus, and EMBASE databases, and the Google Scholar search engine. The search terms were selected based on three main distinct concepts. One group was CQI-related terms. The second group included terms related to the purpose for which CQI has been implemented, and the third group included processes and impact. These terms were selected based on the Donabedian framework of structure, process, and outcome [ 28 ]. Additionally, the detailed keywords were recruited from the primary health framework, which has described lists of dimensions under process, output, outcome, and health system goals of any intervention for health [ 29 ]. The detailed search strategy is presented in the Supplementary file 1 (Search strategy). The search for articles was initiated on August 12, 2023, and the last search was conducted on September 01, 2023.
Eligibility criteria and article selection
Based on the scoping review’s population, concept, and context frameworks [ 30 ], the population included any patients or clients. Additionally, the concepts explored in the review encompassed definitions, implementation, models, tools, barriers, facilitators, and impacts of CQI. Furthermore, the review considered contexts at any level of health systems. We included articles if they reported results of qualitative or quantitative empirical study, case studies, analytic or descriptive synthesis, any review, and other written documents, were published in peer-reviewed journals, and were designed to address at least one of the identified research questions or one of the identified implementation outcomes or their synonymous taxonomy as described in the search strategy. Based on additional contexts, we included articles published in English without geographic and time limitations. We excluded articles with abstracts only, conference abstracts, letters to editors, commentators, and corrections.
We exported all citations to EndNote x20 to remove duplicates and screen relevant articles. The article selection process includes automatic duplicate removal by using EndNote x20, unmatched title and abstract removal, citation and abstract-only materials removal, and full-text assessment. The article selection process was mainly conducted by the first author (AE) and reported to the team during the weekly meetings. The first author encountered papers that caused confusion regarding whether to include or exclude them and discussed them with the last author (YA). Then, decisions were ultimately made. Whenever disagreements happened, they were resolved by discussion and reconsideration of the review questions in relation to the written documents of the article. Further statistical analysis, such as calculating Kappa, was not performed to determine article inclusion or exclusion.
Data extraction and data items
We extracted first author, publication year, country, settings, health problem, the purpose of the study, study design, types of intervention if applicable, CQI approaches/steps if applicable, CQI tools and procedures if applicable, and main findings using a customized Microsoft Excel form.
Summarizing and reporting the results
The main findings were summarized and described based on the main themes, including concepts under conceptualizing, principles, teams, timelines, models, tools, barriers, facilitators, and impacts of CQI. Results-based convergent synthesis, achieved through mixed-method analysis, involved content analysis to identify the thematic presentation of findings. Additionally, a narrative description was used for quantitative findings, aligning them with the appropriate theme. The authors meticulously reviewed the primary findings from each included material and contextualized these findings concerning the main themes1. This approach provides a comprehensive understanding of complex interventions and health systems, acknowledging quantitative and qualitative evidence.
Search results
A total of 11,251 documents were identified from various databases: SCOPUS ( n = 4,339), PubMed ( n = 2,893), Web of Science ( n = 225), EMBASE ( n = 3,651), and Google Scholar ( n = 143). After removing duplicates ( n = 5,061), 6,190 articles were evaluated by title and abstract. Subsequently, 208 articles were assessed for full-text eligibility. Following the eligibility criteria, 121 articles were excluded, leaving 87 included in the current review (Fig. 1 ).
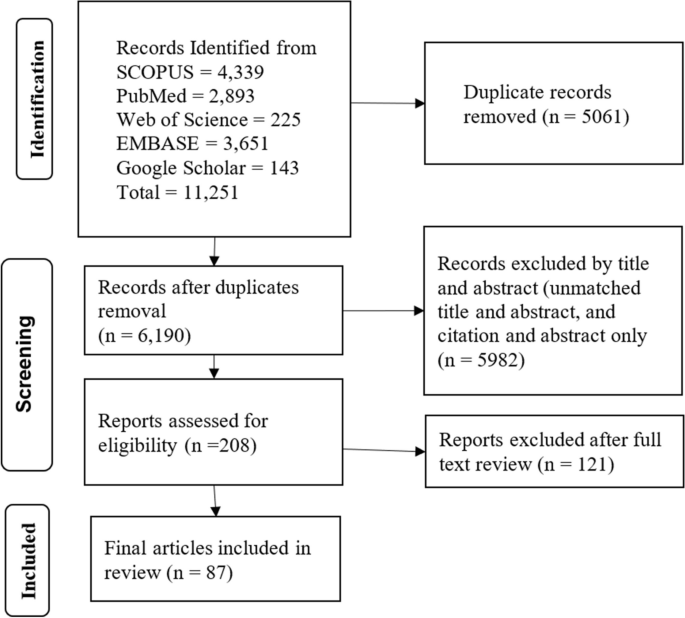
Article selection process
Operationalizing continuous quality improvement
Continuous Quality Improvement (CQI) is operationalized as a cyclic process that requires commitment to implementation, teamwork, time allocation, and celebrating successes and failures.
CQI is a cyclic ongoing process that is followed reflexive, analytical and iterative steps, including identifying gaps, generating data, developing and implementing action plans, evaluating performance, providing feedback to implementers and leaders, and proposing necessary adjustments [ 31 , 32 , 33 , 34 , 35 , 36 , 37 , 38 ].
CQI requires committing to the philosophy, involving continuous improvement [ 19 , 38 ], establishing a mission statement [ 37 ], and understanding quality definition [ 19 ].
CQI involves a wide range of patient-oriented measures and performance indicators, specifically satisfying internal and external customers, developing quality assurance, adopting common quality measures, and selecting process measures [ 8 , 19 , 35 , 36 , 37 , 39 , 40 ].
CQI requires celebrating success and failure without personalization, leading each team member to develop error-free attitudes [ 19 ]. Success and failure are related to underlying organizational processes and systems as causes of failure rather than blaming individuals [ 8 ] because CQI is process-focused based on collaborative, data-driven, responsive, rigorous and problem-solving statistical analysis [ 8 , 19 , 38 ]. Furthermore, a gap or failure opens another opportunity for establishing a data-driven learning organization [ 41 ].
CQI cannot be implemented without a CQI team [ 8 , 19 , 37 , 39 , 42 , 43 , 44 , 45 , 46 ]. A CQI team comprises individuals from various disciplines, often comprising a team leader, a subject matter expert (physician or other healthcare provider), a data analyst, a facilitator, frontline staff, and stakeholders [ 39 , 43 , 47 , 48 , 49 ]. It is also important to note that inviting stakeholders or partners as part of the CQI support intervention is crucial [ 19 , 38 , 48 ].
The timeline is another distinct feature of CQI because the results of CQI vary based on the implementation duration of each cycle [ 35 ]. There is no specific time limit for CQI implementation, although there is a general consensus that a cycle of CQI should be relatively short [ 35 ]. For instance, a CQI implementation took 2 months [ 42 ], 4 months [ 50 ], 9 months [ 51 , 52 ], 12 months [ 53 , 54 , 55 ], and one year and 5 months [ 49 ] duration to achieve the desired positive outcome, while bi-weekly [ 47 ] and monthly data reviews and analyses [ 44 , 48 , 56 ], and activities over 3 months [ 57 ] have also resulted in a positive outcome.
Continuous quality improvement models and tools
There have been several models are utilized. The Plan-Do-Study/Check-Act cycle is a stepwise process involving project initiation, situation analysis, root cause identification, solution generation and selection, implementation, result evaluation, standardization, and future planning [ 7 , 36 , 37 , 45 , 47 , 48 , 49 , 50 , 51 , 53 , 56 , 57 , 58 , 59 , 60 , 61 , 62 , 63 , 64 , 65 , 66 , 67 , 68 , 69 , 70 ]. The FOCUS-PDCA cycle enhances the PDCA process by adding steps to find and improve a process (F), organize a knowledgeable team (O), clarify the process (C), understand variations (U), and select improvements (S) [ 55 , 71 , 72 , 73 ]. The FADE cycle involves identifying a problem (Focus), understanding it through data analysis (Analyze), devising solutions (Develop), and implementing the plan (Execute) [ 74 ]. The Logic Framework involves brainstorming to identify improvement areas, conducting root cause analysis to develop a problem tree, logically reasoning to create an objective tree, formulating the framework, and executing improvement projects [ 75 ]. Breakthrough series approach requires CQI teams to meet in quarterly collaborative learning sessions, share learning experiences, and continue discussion by telephone and cross-site visits to strengthen learning and idea exchange [ 47 ]. Another CQI model is the Lean approach, which has been conducted with Kaizen principles [ 52 ], 5 S principles, and the Six Sigma model. The 5 S (Sort, Set/Straighten, Shine, Standardize, Sustain) systematically organises and improves the workplace, focusing on sorting, setting order, shining, standardizing, and sustaining the improvement [ 54 , 76 ]. Kaizen principles guide CQI by advocating for continuous improvement, valuing all ideas, solving problems, focusing on practical, low-cost improvements, using data to drive change, acknowledging process defects, reducing variability and waste, recognizing every interaction as a customer-supplier relationship, empowering workers, responding to all ideas, and maintaining a disciplined workplace [ 77 ]. Lean Six Sigma, a CQI model, applies the DMAIC methodology, which involves defining (D) and measuring the problem (M), analyzing root causes (A), improving by finding solutions (I), and controlling by assessing process stability (C) [ 78 , 79 ]. The 5 C-cyclic model (consultation, collection, consideration, collaboration, and celebration), the first CQI framework for volunteer dental services in Aboriginal communities, ensures quality care based on community needs [ 80 ]. One study used meetings involving activities such as reviewing objectives, assigning roles, discussing the agenda, completing tasks, retaining key outputs, planning future steps, and evaluating the meeting’s effectiveness [ 81 ].
Various tools are involved in the implementation or evaluation of CQI initiatives: checklists [ 53 , 82 ], flowcharts [ 81 , 82 , 83 ], cause-and-effect diagrams (fishbone or Ishikawa diagrams) [ 60 , 62 , 79 , 81 , 82 ], fuzzy Pareto diagram [ 82 ], process maps [ 60 ], time series charts [ 48 ], why-why analysis [ 79 ], affinity diagrams and multivoting [ 81 ], and run chart [ 47 , 48 , 51 , 60 , 84 ], and others mentioned in the table (Table 1 ).
Barriers and facilitators of continuous quality improvement implementation
Implementing CQI initiatives is determined by various barriers and facilitators, which can be thematized into four dimensions. These dimensions are cultural, technical, structural, and strategic dimensions.
Continuous quality improvement initiatives face various cultural, strategic, technical, and structural barriers. Cultural dimension barriers involve resistance to change (e.g., not accepting online technology), lack of quality-focused culture, staff reporting apprehensiveness, and fear of blame or punishment [ 36 , 41 , 85 , 86 ]. The technical dimension barriers of CQI can include various factors that hinder the effective implementation and execution of CQI processes [ 36 , 86 , 87 , 88 , 89 ]. Structural dimension barriers of CQI arise from the organization structure, process, and systems that can impede the effective implementation and sustainability of CQI [ 36 , 85 , 86 , 87 , 88 ]. Strategic dimension barriers are, for example, the inability to select proper CQI goals and failure to integrate CQI into organizational planning and goals [ 36 , 85 , 86 , 87 , 88 , 90 ].
Facilitators are also grouped to cultural, structural, technical, and strategic dimensions to provide solutions to CQI barriers. Cultural challenges were addressed by developing a group culture to CQI and other rewards [ 39 , 41 , 80 , 85 , 86 , 87 , 90 , 91 , 92 ]. Technical facilitators are pivotal to improving technical barriers [ 39 , 42 , 53 , 69 , 86 , 90 , 91 ]. Structural-related facilitators are related to improving communication, infrastructure, and systems [ 86 , 92 , 93 ]. Strategic dimension facilitators include strengthening leadership and improving decision-making skills [ 43 , 53 , 67 , 86 , 87 , 92 , 94 , 95 ] (Table 2 ).
Impact of continuous quality improvement
Continuous quality improvement initiatives can significantly impact the quality of healthcare in a wide range of health areas, focusing on improving structure, the health service delivery process and improving client wellbeing and reducing mortality.
Structure components
These are health leadership, financing, workforce, technology, and equipment and supplies. CQI has improved planning, monitoring and evaluation [ 48 , 53 ], and leadership and planning [ 48 ], indicating improvement in leadership perspectives. Implementing CQI in primary health care (PHC) settings has shown potential for maintaining or reducing operation costs [ 67 ]. Findings from another study indicate that the costs associated with implementing CQI interventions per facility ranged from approximately $2,000 to $10,500 per year, with an average cost of approximately $10 to $60 per admitted client [ 57 ]. However, based on model predictions, the average cost savings after implementing CQI were estimated to be $5430 [ 31 ]. CQI can also be applied to health workforce development [ 32 ]. CQI in the institutional system improved medical education [ 66 , 96 , 97 ], human resources management [ 53 ], motivated staffs [ 76 ], and increased staff health awareness [ 69 ], while concerns raised about CQI impartiality, independence, and public accountability [ 96 ]. Regarding health technology, CQI also improved registration and documentation [ 48 , 53 , 98 ]. Furthermore, the CQI initiatives increased cleanliness [ 54 ] and improved logistics, supplies, and equipment [ 48 , 53 , 68 ].
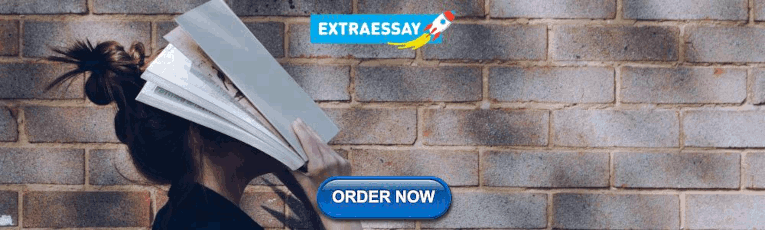
Process and output components
The process component focuses on the activities and actions involved in delivering healthcare services.
Service delivery
CQI interventions improved service delivery [ 53 , 56 , 99 ], particularly a significant 18% increase in the overall quality of service performance [ 48 ], improved patient counselling, adherence to appropriate procedures, and infection prevention [ 48 , 68 ], and optimised workflow [ 52 ].
Coordination and collaboration
CQI initiatives improved coordination and collaboration through collecting and analysing data, onsite technical support, training, supportive supervision [ 53 ] and facilitating linkages between work processes and a quality control group [ 65 ].
Patient satisfaction
The CQI initiatives increased patient satisfaction and improved quality of life by optimizing care quality management, improving the quality of clinical nursing, reducing nursing defects and enhancing the wellbeing of clients [ 54 , 76 , 100 ], although CQI was not associated with changes in adolescent and young adults’ satisfaction [ 51 ].
CQI initiatives reduced medication error reports from 16 to 6 [ 101 ], and it significantly reduced the administration of inappropriate prophylactic antibiotics [ 44 ], decreased errors in inpatient care [ 52 ], decreased the overall episiotomy rate from 44.5 to 33.3% [ 83 ], reduced the overall incidence of unplanned endotracheal extubation [ 102 ], improving appropriate use of computed tomography angiography [ 103 ], and appropriate diagnosis and treatment selection [ 47 ].
Continuity of care
CQI initiatives effectively improve continuity of care by improving client and physician interaction. For instance, provider continuity levels showed a 64% increase [ 55 ]. Modifying electronic medical record templates, scheduling, staff and parental education, standardization of work processes, and birth to 1-year age-specific incentives in post-natal follow-up care increased continuity of care to 74% in 2018 compared to baseline 13% in 2012 [ 84 ].
The CQI initiative yielded enhanced efficiency in the cardiac catheterization laboratory, as evidenced by improved punctuality in procedure starts and increased efficiency in manual sheath-pulls inside [ 78 ].
Accessibility
CQI initiatives were effective in improving accessibility in terms of increasing service coverage and utilization rate. For instance, screening for cigarettes, nutrition counselling, folate prescription, maternal care, immunization coverage [ 53 , 81 , 104 , 105 ], reducing the percentage of non-attending patients to surgery to 0.9% from the baseline 3.9% [ 43 ], increasing Chlamydia screening rates from 29 to 60% [ 45 ], increasing HIV care continuum coverage [ 51 , 59 , 60 ], increasing in the uptake of postpartum long-acting reversible contraceptive use from 6.9% at the baseline to 25.4% [ 42 ], increasing post-caesarean section prophylaxis from 36 to 89% [ 62 ], a 31% increase of kangaroo care practice [ 50 ], and increased follow-up [ 65 ]. Similarly, the QI intervention increased the quality of antenatal care by 29.3%, correct partograph use by 51.7%, and correct active third-stage labour management, a 19.6% improvement from the baseline, but not significantly associated with improvement in contraceptive service uptake [ 61 ].
Timely access
CQI interventions improved the time care provision [ 52 ], and reduced waiting time [ 62 , 74 , 76 , 106 ]. For instance, the discharge process waiting time in the emergency department decreased from 76 min to 22 min [ 79 ]. It also reduced mean postprocedural length of stay from 2.8 days to 2.0 days [ 31 ].
Acceptability
Acceptability of CQI by healthcare providers was satisfactory. For instance, 88% of the faculty, 64% of the residents, and 82% of the staff believed CQI to be useful in the healthcare clinic [ 107 ].
Outcome components
Morbidity and mortality.
CQI efforts have demonstrated better management outcomes among diabetic patients [ 40 ], patients with oral mucositis [ 71 ], and anaemic patients [ 72 ]. It has also reduced infection rate in post-caesarean Sect. [ 62 ], reduced post-peritoneal dialysis peritonitis [ 49 , 108 ], and prevented pressure ulcers [ 70 ]. It is explained by peritonitis incidence from once every 40.1 patient months at baseline to once every 70.8 patient months after CQI [ 49 ] and a 63% reduction in pressure ulcer prevalence within 2 years from 2008 to 2010 [ 70 ]. Furthermore, CQI initiatives significantly reduced in-hospital deaths [ 31 ] and increased patient survival rates [ 108 ]. Figure 2 displays the overall process of the CQI implementations.
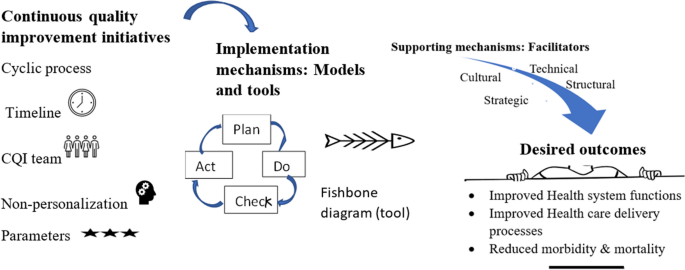
The overall mechanisms of continuous quality improvement implementation
In this review, we examined the fundamental concepts and principles underlying CQI, the factors that either hinder or assist in its successful application and implementation, and the purpose of CQI in enhancing quality of care across various health issues.
Our findings have brought attention to the application and implementation of CQI, emphasizing its underlying concepts and principles, as evident in the existing literature [ 31 , 32 , 33 , 34 , 35 , 36 , 39 , 40 , 43 , 45 , 46 ]. Continuous quality improvement has shared with the principles of continuous improvement, such as a customer-driven focus, effective leadership, active participation of individuals, a process-oriented approach, systematic implementation, emphasis on design improvement and prevention, evidence-based decision-making, and fostering partnership [ 5 ]. Moreover, Deming’s 14 principles laid the foundation for CQI principles [ 109 ]. These principles have been adapted and put into practice in various ways: ten [ 19 ] and five [ 38 ] principles in hospitals, five principles for capacity building [ 38 ], and two principles for medication error prevention [ 41 ]. As a principle, the application of CQI can be process-focused [ 8 , 19 ] or impact-focused [ 38 ]. Impact-focused CQI focuses on achieving specific outcomes or impacts, whereas process-focused CQI prioritizes and improves the underlying processes and systems. These principles complement each other and can be utilized based on the objectives of quality improvement initiatives in healthcare settings. Overall, CQI is an ongoing educational process that requires top management’s involvement, demands coordination across departments, encourages the incorporation of views beyond clinical area, and provides non-judgemental evidence based on objective data [ 110 ].
The current review recognized that it was not easy to implement CQI. It requires reasonable utilization of various models and tools. The application of each tool can be varied based on the studied health problem and the purpose of CQI initiative [ 111 ], varied in context, content, structure, and usability [ 112 ]. Additionally, overcoming the cultural, technical, structural, and strategic-related barriers. These barriers have emerged from clinical staff, managers, and health systems perspectives. Of the cultural obstacles, staff non-involvement, resistance to change, and reluctance to report error were staff-related. In contrast, others, such as the absence of celebration for success and hierarchical and rational culture, may require staff and manager involvement. Staff members may exhibit reluctance in reporting errors due to various cultural factors, including lack of trust, hierarchical structures, fear of retribution, and a blame-oriented culture. These challenges pose obstacles to implementing standardized CQI practices, as observed, for instance, in community pharmacy settings [ 85 ]. The hierarchical culture, characterized by clearly defined levels of power, authority, and decision-making, posed challenges to implementing CQI initiatives in public health [ 41 , 86 ]. Although rational culture, a type of organizational culture, emphasizes logical thinking and rational decision-making, it can also create challenges for CQI implementation [ 41 , 86 ] because hierarchical and rational cultures, which emphasize bureaucratic norms and narrow definitions of achievement, were found to act as barriers to the implementation of CQI [ 86 ]. These could be solved by developing a shared mindset and collective commitment, establishing a shared purpose, developing group norms, and cultivating psychological preparedness among staff, managers, and clients to implement and sustain CQI initiatives. Furthermore, reversing cultural-related barriers necessitates cultural-related solutions: development of a culture and group culture to CQI [ 41 , 86 ], positive comprehensive perception [ 91 ], commitment [ 85 ], involving patients, families, leaders, and staff [ 39 , 92 ], collaborating for a common goal [ 80 , 86 ], effective teamwork [ 86 , 87 ], and rewarding and celebrating successes [ 80 , 90 ].
The technical dimension barriers of CQI can include inadequate capitalization of a project and insufficient support for CQI facilitators and data entry managers [ 36 ], immature electronic medical records or poor information systems [ 36 , 86 ], and the lack of training and skills [ 86 , 87 , 88 ]. These challenges may cause the CQI team to rely on outdated information and technologies. The presence of barriers on the technical dimension may challenge the solid foundation of CQI expertise among staff, the ability to recognize opportunities for improvement, a comprehensive understanding of how services are produced and delivered, and routine use of expertise in daily work. Addressing these technical barriers requires knowledge creation activities (training, seminar, and education) [ 39 , 42 , 53 , 69 , 86 , 90 , 91 ], availability of quality data [ 86 ], reliable information [ 92 ], and a manual-online hybrid reporting system [ 85 ].
Structural dimension barriers of CQI include inadequate communication channels and lack of standardized process, specifically weak physician-to-physician synergies [ 36 ], lack of mechanisms for disseminating knowledge and limited use of communication mechanisms [ 86 ]. Lack of communication mechanism endangers sharing ideas and feedback among CQI teams, leading to misunderstandings, limited participation and misinterpretations, and a lack of learning [ 113 ]. Knowledge translation facilitates the co-production of research, subsequent diffusion of knowledge, and the developing stakeholder’s capacity and skills [ 114 ]. Thus, the absence of a knowledge translation mechanism may cause missed opportunities for learning, inefficient problem-solving, and limited creativity. To overcome these challenges, organizations should establish effective communication and information systems [ 86 , 93 ] and learning systems [ 92 ]. Though CQI and knowledge translation have interacted with each other, it is essential to recognize that they are distinct. CQI focuses on process improvement within health care systems, aiming to optimize existing processes, reduce errors, and enhance efficiency.
In contrast, knowledge translation bridges the gap between research evidence and clinical practice, translating research findings into actionable knowledge for practitioners. While both CQI and knowledge translation aim to enhance health care quality and patient outcomes, they employ different strategies: CQI utilizes tools like Plan-Do-Study-Act cycles and statistical process control, while knowledge translation involves knowledge synthesis and dissemination. Additionally, knowledge translation can also serve as a strategy to enhance CQI. Both concepts share the same principle: continuous improvement is essential for both. Therefore, effective strategies on the structural dimension may build efficient and effective steering councils, information systems, and structures to diffuse learning throughout the organization.
Strategic factors, such as goals, planning, funds, and resources, determine the overall purpose of CQI initiatives. Specific barriers were improper goals and poor planning [ 36 , 86 , 88 ], fragmentation of quality assurance policies [ 87 ], inadequate reinforcement to staff [ 36 , 90 ], time constraints [ 85 , 86 ], resource inadequacy [ 86 ], and work overload [ 86 ]. These barriers can be addressed through strengthening leadership [ 86 , 87 ], CQI-based mentoring [ 94 ], periodic monitoring, supportive supervision and coaching [ 43 , 53 , 87 , 92 , 95 ], participation, empowerment, and accountability [ 67 ], involving all stakeholders in decision-making [ 86 , 87 ], a provider-payer partnership [ 64 ], and compensating staff for after-hours meetings on CQI [ 85 ]. The strategic dimension, characterized by a strategic plan and integrated CQI efforts, is devoted to processes that are central to achieving strategic priorities. Roles and responsibilities are defined in terms of integrated strategic and quality-related goals [ 115 ].
The utmost goal of CQI has been to improve the quality of care, which is usually revealed by structure, process, and outcome. After resolving challenges and effectively using tools and running models, the goal of CQI reflects the ultimate reason and purpose of its implementation. First, effectively implemented CQI initiatives can improve leadership, health financing, health workforce development, health information technology, and availability of supplies as the building blocks of a health system [ 31 , 48 , 53 , 68 , 98 ]. Second, effectively implemented CQI initiatives improved care delivery process (counselling, adherence with standards, coordination, collaboration, and linkages) [ 48 , 53 , 65 , 68 ]. Third, the CQI can improve outputs of healthcare delivery, such as satisfaction, accessibility (timely access, utilization), continuity of care, safety, efficiency, and acceptability [ 52 , 54 , 55 , 76 , 78 ]. Finally, the effectiveness of the CQI initiatives has been tested in enhancing responses related to key aspects of the HIV response, maternal and child health, non-communicable disease control, and others (e.g., surgery and peritonitis). However, it is worth noting that CQI initiative has not always been effective. For instance, CQI using a two- to nine-times audit cycle model through systems assessment tools did not bring significant change to increase syphilis testing performance [ 116 ]. This study was conducted within the context of Aboriginal and Torres Strait Islander people’s primary health care settings. Notably, ‘the clinics may not have consistently prioritized syphilis testing performance in their improvement strategies, as facilitated by the CQI program’ [ 116 ]. Additionally, by applying CQI-based mentoring, uptake of facility-based interventions was not significantly improved, though it was effective in increasing community health worker visits during pregnancy and the postnatal period, knowledge about maternal and child health and exclusive breastfeeding practice, and HIV disclosure status [ 117 ]. The study conducted in South Africa revealed no significant association between the coverage of facility-based interventions and Continuous Quality Improvement (CQI) implementation. This lack of association was attributed to the already high antenatal and postnatal attendance rates in both control and intervention groups at baseline, leaving little room for improvement. Additionally, the coverage of HIV interventions remained consistently high throughout the study period [ 117 ].
Regarding health care and policy implications, CQI has played a vital role in advancing PHC and fostering the realization of UHC goals worldwide. The indicators found in Donabedian’s framework that are positively influenced by CQI efforts are comparable to those included in the PHC performance initiative’s conceptual framework [ 29 , 118 , 119 ]. It is clearly explained that PHC serves as the roadmap to realizing the vision of UHC [ 120 , 121 ]. Given these circumstances, implementing CQI can contribute to the achievement of PHC principles and the objectives of UHC. For instance, by implementing CQI methods, countries have enhanced the accessibility, affordability, and quality of PHC services, leading to better health outcomes for their populations. CQI has facilitated identifying and resolving healthcare gaps and inefficiencies, enabling countries to optimize resource allocation and deliver more effective and patient-centered care. However, it is crucial to recognize that the successful implementation of Continuous Quality Improvement (CQI) necessitates optimizing the duration of each cycle, understanding challenges and barriers that extend beyond the health system and settings, and acknowledging that its effectiveness may be compromised if these challenges are not adequately addressed.
Despite abundant literature, there are still gaps regarding the relationship between CQI and other dimensions within the healthcare system. No studies have examined the impact of CQI initiatives on catastrophic health expenditure, effective service coverage, patient-centredness, comprehensiveness, equity, health security, and responsiveness.
Limitations
In conducting this review, it has some limitations to consider. Firstly, only articles published in English were included, which may introduce the exclusion of relevant non-English articles. Additionally, as this review follows a scoping methodology, the focus is on synthesising available evidence rather than critically evaluating or scoring the quality of the included articles.
Continuous quality improvement is investigated as a continuous and ongoing intervention, where the implementation time can vary across different cycles. The CQI team and implementation timelines were critical elements of CQI in different models. Among the commonly used approaches, the PDSA or PDCA is frequently employed. In most CQI models, a wide range of tools, nineteen tools, are commonly utilized to support the improvement process. Cultural, technical, structural, and strategic barriers and facilitators are significant in implementing CQI initiatives. Implementing the CQI initiative aims to improve health system blocks, enhance health service delivery process and output, and ultimately prevent morbidity and reduce mortality. For future researchers, considering that CQI is context-dependent approach, conducting scale-up implementation research about catastrophic health expenditure, effective service coverage, patient-centredness, comprehensiveness, equity, health security, and responsiveness across various settings and health issues would be valuable.
Availability of data and materials
The data used and/or analyzed during the current study are available in this manuscript and/or the supplementary file.
Shewhart WA, Deming WE. Memoriam: Walter A. Shewhart, 1891–1967. Am Stat. 1967;21(2):39–40.
Article Google Scholar
Shewhart WA. Statistical method from the viewpoint of quality control. New York: Dover; 1986. ISBN 978-0486652320. OCLC 13822053. Reprint. Originally published: Washington, DC: Graduate School of the Department of Agriculture, 1939.
Moen R, editor Foundation and History of the PDSA Cycle. Asian network for quality conference Tokyo. https://www.deming.org/sites/default/files/pdf/2015/PDSA_History_Ron_MoenPdf . 2009.
Kuperman G, James B, Jacobsen J, Gardner RM. Continuous quality improvement applied to medical care: experiences at LDS hospital. Med Decis Making. 1991;11(4suppl):S60–65.
Article CAS PubMed Google Scholar
Singh J, Singh H. Continuous improvement philosophy–literature review and directions. Benchmarking: An International Journal. 2015;22(1):75–119.
Goldstone J. Presidential address: Sony, Porsche, and vascular surgery in the 21st century. J Vasc Surg. 1997;25(2):201–10.
Radawski D. Continuous quality improvement: origins, concepts, problems, and applications. J Physician Assistant Educ. 1999;10(1):12–6.
Shortell SM, O’Brien JL, Carman JM, Foster RW, Hughes E, Boerstler H, et al. Assessing the impact of continuous quality improvement/total quality management: concept versus implementation. Health Serv Res. 1995;30(2):377.
CAS PubMed PubMed Central Google Scholar
Lohr K. Quality of health care: an introduction to critical definitions, concepts, principles, and practicalities. Striving for quality in health care. 1991.
Berwick DM. The clinical process and the quality process. Qual Manage Healthc. 1992;1(1):1–8.
Article CAS Google Scholar
Gift B. On the road to TQM. Food Manage. 1992;27(4):88–9.
CAS PubMed Google Scholar
Greiner A, Knebel E. The core competencies needed for health care professionals. health professions education: A bridge to quality. 2003:45–73.
McCalman J, Bailie R, Bainbridge R, McPhail-Bell K, Percival N, Askew D et al. Continuous quality improvement and comprehensive primary health care: a systems framework to improve service quality and health outcomes. Front Public Health. 2018:6 (76):1–6.
Sheingold BH, Hahn JA. The history of healthcare quality: the first 100 years 1860–1960. Int J Afr Nurs Sci. 2014;1:18–22.
Google Scholar
Donabedian A. Evaluating the quality of medical care. Milbank Q. 1966;44(3):166–206.
Institute of Medicine (US) Committee on Quality of Health Care in America. Crossing the Quality Chasm: A New Health System for the 21st Century. Washington (DC): National Academies Press (US). 2001. 2, Improving the 21st-century Health Care System. Available from: https://www.ncbi.nlm.nih.gov/books/NBK222265/ .
Rubinstein A, Barani M, Lopez AS. Quality first for effective universal health coverage in low-income and middle-income countries. Lancet Global Health. 2018;6(11):e1142–1143.
Article PubMed Google Scholar
Agency for Healthcare Reserach and Quality. Quality Improvement and monitoring at your fingertips USA,: Agency for Healthcare Reserach and Quality. 2022. Available from: https://qualityindicators.ahrq.gov/ .
Anderson CA, Cassidy B, Rivenburgh P. Implementing continuous quality improvement (CQI) in hospitals: lessons learned from the International Quality Study. Qual Assur Health Care. 1991;3(3):141–6.
Gardner K, Mazza D. Quality in general practice - definitions and frameworks. Aust Fam Physician. 2012;41(3):151–4.
PubMed Google Scholar
Loper AC, Jensen TM, Farley AB, Morgan JD, Metz AJ. A systematic review of approaches for continuous quality improvement capacity-building. J Public Health Manage Pract. 2022;28(2):E354.
Hill JE, Stephani A-M, Sapple P, Clegg AJ. The effectiveness of continuous quality improvement for developing professional practice and improving health care outcomes: a systematic review. Implement Sci. 2020;15(1):1–14.
Candas B, Jobin G, Dubé C, Tousignant M, Abdeljelil AB, Grenier S, et al. Barriers and facilitators to implementing continuous quality improvement programs in colonoscopy services: a mixed methods systematic review. Endoscopy Int Open. 2016;4(02):E118–133.
Peters MD, Marnie C, Colquhoun H, Garritty CM, Hempel S, Horsley T, et al. Scoping reviews: reinforcing and advancing the methodology and application. Syst Reviews. 2021;10(1):1–6.
Arksey H, O’Malley L. Scoping studies: towards a methodological framework. Int J Soc Res Methodol. 2005;8(1):19–32.
Tricco AC, Lillie E, Zarin W, O’Brien KK, Colquhoun H, Levac D, et al. PRISMA extension for scoping reviews (PRISMA-ScR): checklist and explanation. Ann Intern Med. 2018;169(7):467–73.
McGowan J, Straus S, Moher D, Langlois EV, O’Brien KK, Horsley T, et al. Reporting scoping reviews—PRISMA ScR extension. J Clin Epidemiol. 2020;123:177–9.
Donabedian A. Explorations in quality assessment and monitoring: the definition of quality and approaches to its assessment. Health Administration Press, Ann Arbor. 1980;1.
World Health Organization. Operational framework for primary health care: transforming vision into action. Geneva: World Health Organization and the United Nations Children’s Fund (UNICEF); 2020 [updated 14 December 2020; cited 2023 Nov Oct 17]. Available from: https://www.who.int/publications/i/item/9789240017832 .
The Joanna Briggs Institute. The Joanna Briggs Institute Reviewers’ Manual :2014 edition. Australia: The Joanna Briggs Institute. 2014:88–91.
Rihal CS, Kamath CC, Holmes DR Jr, Reller MK, Anderson SS, McMurtry EK, et al. Economic and clinical outcomes of a physician-led continuous quality improvement intervention in the delivery of percutaneous coronary intervention. Am J Manag Care. 2006;12(8):445–52.
Ade-Oshifogun JB, Dufelmeier T. Prevention and Management of Do not return notices: a quality improvement process for Supplemental staffing nursing agencies. Nurs Forum. 2012;47(2):106–12.
Rubenstein L, Khodyakov D, Hempel S, Danz M, Salem-Schatz S, Foy R, et al. How can we recognize continuous quality improvement? Int J Qual Health Care. 2014;26(1):6–15.
O’Neill SM, Hempel S, Lim YW, Danz MS, Foy R, Suttorp MJ, et al. Identifying continuous quality improvement publications: what makes an improvement intervention ‘CQI’? BMJ Qual Saf. 2011;20(12):1011–9.
Article PubMed PubMed Central Google Scholar
Sibthorpe B, Gardner K, McAullay D. Furthering the quality agenda in Aboriginal community controlled health services: understanding the relationship between accreditation, continuous quality improvement and national key performance indicator reporting. Aust J Prim Health. 2016;22(4):270–5.
Bennett CL, Crane JM. Quality improvement efforts in oncology: are we ready to begin? Cancer Invest. 2001;19(1):86–95.
VanValkenburgh DA. Implementing continuous quality improvement at the facility level. Adv Ren Replace Ther. 2001;8(2):104–13.
Loper AC, Jensen TM, Farley AB, Morgan JD, Metz AJ. A systematic review of approaches for continuous quality improvement capacity-building. J Public Health Manage Practice. 2022;28(2):E354–361.
Ryan M. Achieving and sustaining quality in healthcare. Front Health Serv Manag. 2004;20(3):3–11.
Nicolucci A, Allotta G, Allegra G, Cordaro G, D’Agati F, Di Benedetto A, et al. Five-year impact of a continuous quality improvement effort implemented by a network of diabetes outpatient clinics. Diabetes Care. 2008;31(1):57–62.
Wakefield BJ, Blegen MA, Uden-Holman T, Vaughn T, Chrischilles E, Wakefield DS. Organizational culture, continuous quality improvement, and medication administration error reporting. Am J Med Qual. 2001;16(4):128–34.
Sori DA, Debelew GT, Degefa LS, Asefa Z. Continuous quality improvement strategy for increasing immediate postpartum long-acting reversible contraceptive use at Jimma University Medical Center, Jimma, Ethiopia. BMJ Open Qual. 2023;12(1):e002051.
Roche B, Robin C, Deleaval PJ, Marti MC. Continuous quality improvement in ambulatory surgery: the non-attending patient. Ambul Surg. 1998;6(2):97–100.
O’Connor JB, Sondhi SS, Mullen KD, McCullough AJ. A continuous quality improvement initiative reduces inappropriate prescribing of prophylactic antibiotics for endoscopic procedures. Am J Gastroenterol. 1999;94(8):2115–21.
Ursu A, Greenberg G, McKee M. Continuous quality improvement methodology: a case study on multidisciplinary collaboration to improve chlamydia screening. Fam Med Community Health. 2019;7(2):e000085.
Quick B, Nordstrom S, Johnson K. Using continuous quality improvement to implement evidence-based medicine. Lippincotts Case Manag. 2006;11(6):305–15 ( quiz 16 – 7 ).
Oyeledun B, Phillips A, Oronsaye F, Alo OD, Shaffer N, Osibo B, et al. The effect of a continuous quality improvement intervention on retention-in-care at 6 months postpartum in a PMTCT Program in Northern Nigeria: results of a cluster randomized controlled study. J Acquir Immune Defic Syndr. 2017;75(Suppl 2):S156–164.
Nyengerai T, Phohole M, Iqaba N, Kinge CW, Gori E, Moyo K, et al. Quality of service and continuous quality improvement in voluntary medical male circumcision programme across four provinces in South Africa: longitudinal and cross-sectional programme data. PLoS ONE. 2021;16(8):e0254850.
Article CAS PubMed PubMed Central Google Scholar
Wang J, Zhang H, Liu J, Zhang K, Yi B, Liu Y, et al. Implementation of a continuous quality improvement program reduces the occurrence of peritonitis in PD. Ren Fail. 2014;36(7):1029–32.
Stikes R, Barbier D. Applying the plan-do-study-act model to increase the use of kangaroo care. J Nurs Manag. 2013;21(1):70–8.
Wagner AD, Mugo C, Bluemer-Miroite S, Mutiti PM, Wamalwa DC, Bukusi D, et al. Continuous quality improvement intervention for adolescent and young adult HIV testing services in Kenya improves HIV knowledge. AIDS. 2017;31(Suppl 3):S243–252.
Le RD, Melanson SE, Santos KS, Paredes JD, Baum JM, Goonan EM, et al. Using lean principles to optimise inpatient phlebotomy services. J Clin Pathol. 2014;67(8):724–30.
Manyazewal T, Mekonnen A, Demelew T, Mengestu S, Abdu Y, Mammo D, et al. Improving immunization capacity in Ethiopia through continuous quality improvement interventions: a prospective quasi-experimental study. Infect Dis Poverty. 2018;7:7.
Kamiya Y, Ishijma H, Hagiwara A, Takahashi S, Ngonyani HAM, Samky E. Evaluating the impact of continuous quality improvement methods at hospitals in Tanzania: a cluster-randomized trial. Int J Qual Health Care. 2017;29(1):32–9.
Kibbe DC, Bentz E, McLaughlin CP. Continuous quality improvement for continuity of care. J Fam Pract. 1993;36(3):304–8.
Adrawa N, Ongiro S, Lotee K, Seret J, Adeke M, Izudi J. Use of a context-specific package to increase sputum smear monitoring among people with pulmonary tuberculosis in Uganda: a quality improvement study. BMJ Open Qual. 2023;12(3):1–6.
Hunt P, Hunter SB, Levan D. Continuous quality improvement in substance abuse treatment facilities: how much does it cost? J Subst Abuse Treat. 2017;77:133–40.
Azadeh A, Ameli M, Alisoltani N, Motevali Haghighi S. A unique fuzzy multi-control approach for continuous quality improvement in a radio therapy department. Qual Quantity. 2016;50(6):2469–93.
Memiah P, Tlale J, Shimabale M, Nzyoka S, Komba P, Sebeza J, et al. Continuous quality improvement (CQI) institutionalization to reach 95:95:95 HIV targets: a multicountry experience from the Global South. BMC Health Serv Res. 2021;21(1):711.
Yapa HM, De Neve JW, Chetty T, Herbst C, Post FA, Jiamsakul A, et al. The impact of continuous quality improvement on coverage of antenatal HIV care tests in rural South Africa: results of a stepped-wedge cluster-randomised controlled implementation trial. PLoS Med. 2020;17(10):e1003150.
Dadi TL, Abebo TA, Yeshitla A, Abera Y, Tadesse D, Tsegaye S, et al. Impact of quality improvement interventions on facility readiness, quality and uptake of maternal and child health services in developing regions of Ethiopia: a secondary analysis of programme data. BMJ Open Qual. 2023;12(4):e002140.
Weinberg M, Fuentes JM, Ruiz AI, Lozano FW, Angel E, Gaitan H, et al. Reducing infections among women undergoing cesarean section in Colombia by means of continuous quality improvement methods. Arch Intern Med. 2001;161(19):2357–65.
Andreoni V, Bilak Y, Bukumira M, Halfer D, Lynch-Stapleton P, Perez C. Project management: putting continuous quality improvement theory into practice. J Nurs Care Qual. 1995;9(3):29–37.
Balfour ME, Zinn TE, Cason K, Fox J, Morales M, Berdeja C, et al. Provider-payer partnerships as an engine for continuous quality improvement. Psychiatric Serv. 2018;69(6):623–5.
Agurto I, Sandoval J, De La Rosa M, Guardado ME. Improving cervical cancer prevention in a developing country. Int J Qual Health Care. 2006;18(2):81–6.
Anderson CI, Basson MD, Ali M, Davis AT, Osmer RL, McLeod MK, et al. Comprehensive multicenter graduate surgical education initiative incorporating entrustable professional activities, continuous quality improvement cycles, and a web-based platform to enhance teaching and learning. J Am Coll Surg. 2018;227(1):64–76.
Benjamin S, Seaman M. Applying continuous quality improvement and human performance technology to primary health care in Bahrain. Health Care Superv. 1998;17(1):62–71.
Byabagambi J, Marks P, Megere H, Karamagi E, Byakika S, Opio A, et al. Improving the quality of voluntary medical male circumcision through use of the continuous quality improvement approach: a pilot in 30 PEPFAR-Supported sites in Uganda. PLoS ONE. 2015;10(7):e0133369.
Hogg S, Roe Y, Mills R. Implementing evidence-based continuous quality improvement strategies in an urban Aboriginal Community Controlled Health Service in South East Queensland: a best practice implementation pilot. JBI Database Syst Rev Implement Rep. 2017;15(1):178–87.
Hopper MB, Morgan S. Continuous quality improvement initiative for pressure ulcer prevention. J Wound Ostomy Cont Nurs. 2014;41(2):178–80.
Ji J, Jiang DD, Xu Z, Yang YQ, Qian KY, Zhang MX. Continuous quality improvement of nutrition management during radiotherapy in patients with nasopharyngeal carcinoma. Nurs Open. 2021;8(6):3261–70.
Chen M, Deng JH, Zhou FD, Wang M, Wang HY. Improving the management of anemia in hemodialysis patients by implementing the continuous quality improvement program. Blood Purif. 2006;24(3):282–6.
Reeves S, Matney K, Crane V. Continuous quality improvement as an ideal in hospital practice. Health Care Superv. 1995;13(4):1–12.
Barton AJ, Danek G, Johns P, Coons M. Improving patient outcomes through CQI: vascular access planning. J Nurs Care Qual. 1998;13(2):77–85.
Buttigieg SC, Gauci D, Dey P. Continuous quality improvement in a Maltese hospital using logical framework analysis. J Health Organ Manag. 2016;30(7):1026–46.
Take N, Byakika S, Tasei H, Yoshikawa T. The effect of 5S-continuous quality improvement-total quality management approach on staff motivation, patients’ waiting time and patient satisfaction with services at hospitals in Uganda. J Public Health Afr. 2015;6(1):486.
PubMed PubMed Central Google Scholar
Jacobson GH, McCoin NS, Lescallette R, Russ S, Slovis CM. Kaizen: a method of process improvement in the emergency department. Acad Emerg Med. 2009;16(12):1341–9.
Agarwal S, Gallo J, Parashar A, Agarwal K, Ellis S, Khot U, et al. Impact of lean six sigma process improvement methodology on cardiac catheterization laboratory efficiency. Catheter Cardiovasc Interv. 2015;85:S119.
Rahul G, Samanta AK, Varaprasad G A Lean Six Sigma approach to reduce overcrowding of patients and improving the discharge process in a super-specialty hospital. In 2020 International Conference on System, Computation, Automation and Networking (ICSCAN) 2020 July 3 (pp. 1-6). IEEE
Patel J, Nattabi B, Long R, Durey A, Naoum S, Kruger E, et al. The 5 C model: A proposed continuous quality improvement framework for volunteer dental services in remote Australian Aboriginal communities. Community Dent Oral Epidemiol. 2023;51(6):1150–8.
Van Acker B, McIntosh G, Gudes M. Continuous quality improvement techniques enhance HMO members’ immunization rates. J Healthc Qual. 1998;20(2):36–41.
Horine PD, Pohjala ED, Luecke RW. Healthcare financial managers and CQI. Healthc Financ Manage. 1993;47(9):34.
Reynolds JL. Reducing the frequency of episiotomies through a continuous quality improvement program. CMAJ. 1995;153(3):275–82.
Bunik M, Galloway K, Maughlin M, Hyman D. First five quality improvement program increases adherence and continuity with well-child care. Pediatr Qual Saf. 2021;6(6):e484.
Boyle TA, MacKinnon NJ, Mahaffey T, Duggan K, Dow N. Challenges of standardized continuous quality improvement programs in community pharmacies: the case of SafetyNET-Rx. Res Social Adm Pharm. 2012;8(6):499–508.
Price A, Schwartz R, Cohen J, Manson H, Scott F. Assessing continuous quality improvement in public health: adapting lessons from healthcare. Healthc Policy. 2017;12(3):34–49.
Gage AD, Gotsadze T, Seid E, Mutasa R, Friedman J. The influence of continuous quality improvement on healthcare quality: a mixed-methods study from Zimbabwe. Soc Sci Med. 2022;298:114831.
Chan YC, Ho SJ. Continuous quality improvement: a survey of American and Canadian healthcare executives. Hosp Health Serv Adm. 1997;42(4):525–44.
Balas EA, Puryear J, Mitchell JA, Barter B. How to structure clinical practice guidelines for continuous quality improvement? J Med Syst. 1994;18(5):289–97.
ElChamaa R, Seely AJE, Jeong D, Kitto S. Barriers and facilitators to the implementation and adoption of a continuous quality improvement program in surgery: a case study. J Contin Educ Health Prof. 2022;42(4):227–35.
Candas B, Jobin G, Dubé C, Tousignant M, Abdeljelil A, Grenier S, et al. Barriers and facilitators to implementing continuous quality improvement programs in colonoscopy services: a mixed methods systematic review. Endoscopy Int Open. 2016;4(2):E118–133.
Brandrud AS, Schreiner A, Hjortdahl P, Helljesen GS, Nyen B, Nelson EC. Three success factors for continual improvement in healthcare: an analysis of the reports of improvement team members. BMJ Qual Saf. 2011;20(3):251–9.
Lee S, Choi KS, Kang HY, Cho W, Chae YM. Assessing the factors influencing continuous quality improvement implementation: experience in Korean hospitals. Int J Qual Health Care. 2002;14(5):383–91.
Horwood C, Butler L, Barker P, Phakathi S, Haskins L, Grant M, et al. A continuous quality improvement intervention to improve the effectiveness of community health workers providing care to mothers and children: a cluster randomised controlled trial in South Africa. Hum Resour Health. 2017;15(1):39.
Hyrkäs K, Lehti K. Continuous quality improvement through team supervision supported by continuous self-monitoring of work and systematic patient feedback. J Nurs Manag. 2003;11(3):177–88.
Akdemir N, Peterson LN, Campbell CM, Scheele F. Evaluation of continuous quality improvement in accreditation for medical education. BMC Med Educ. 2020;20(Suppl 1):308.
Barzansky B, Hunt D, Moineau G, Ahn D, Lai CW, Humphrey H, et al. Continuous quality improvement in an accreditation system for undergraduate medical education: benefits and challenges. Med Teach. 2015;37(11):1032–8.
Gaylis F, Nasseri R, Salmasi A, Anderson C, Mohedin S, Prime R, et al. Implementing continuous quality improvement in an integrated community urology practice: lessons learned. Urology. 2021;153:139–46.
Gaga S, Mqoqi N, Chimatira R, Moko S, Igumbor JO. Continuous quality improvement in HIV and TB services at selected healthcare facilities in South Africa. South Afr J HIV Med. 2021;22(1):1202.
Wang F, Yao D. Application effect of continuous quality improvement measures on patient satisfaction and quality of life in gynecological nursing. Am J Transl Res. 2021;13(6):6391–8.
Lee SB, Lee LL, Yeung RS, Chan J. A continuous quality improvement project to reduce medication error in the emergency department. World J Emerg Med. 2013;4(3):179–82.
Chiang AA, Lee KC, Lee JC, Wei CH. Effectiveness of a continuous quality improvement program aiming to reduce unplanned extubation: a prospective study. Intensive Care Med. 1996;22(11):1269–71.
Chinnaiyan K, Al-Mallah M, Goraya T, Patel S, Kazerooni E, Poopat C, et al. Impact of a continuous quality improvement initiative on appropriate use of coronary CT angiography: results from a multicenter, statewide registry, the advanced cardiovascular imaging consortium (ACIC). J Cardiovasc Comput Tomogr. 2011;5(4):S29–30.
Gibson-Helm M, Rumbold A, Teede H, Ranasinha S, Bailie R, Boyle J. A continuous quality improvement initiative: improving the provision of pregnancy care for Aboriginal and Torres Strait Islander women. BJOG: Int J Obstet Gynecol. 2015;122:400–1.
Bennett IM, Coco A, Anderson J, Horst M, Gambler AS, Barr WB, et al. Improving maternal care with a continuous quality improvement strategy: a report from the interventions to minimize preterm and low birth weight infants through continuous improvement techniques (IMPLICIT) network. J Am Board Fam Med. 2009;22(4):380–6.
Krall SP, Iv CLR, Donahue L. Effect of continuous quality improvement methods on reducing triage to thrombolytic interval for Acute myocardial infarction. Acad Emerg Med. 1995;2(7):603–9.
Swanson TK, Eilers GM. Physician and staff acceptance of continuous quality improvement. Fam Med. 1994;26(9):583–6.
Yu Y, Zhou Y, Wang H, Zhou T, Li Q, Li T, et al. Impact of continuous quality improvement initiatives on clinical outcomes in peritoneal dialysis. Perit Dial Int. 2014;34(Suppl 2):S43–48.
Schiff GD, Goldfield NI. Deming meets Braverman: toward a progressive analysis of the continuous quality improvement paradigm. Int J Health Serv. 1994;24(4):655–73.
American Hospital Association Division of Quality Resources Chicago, IL: The role of hospital leadership in the continuous improvement of patient care quality. American Hospital Association. J Healthc Qual. 1992;14(5):8–14,22.
Scriven M. The Logic and Methodology of checklists [dissertation]. Western Michigan University; 2000.
Hales B, Terblanche M, Fowler R, Sibbald W. Development of medical checklists for improved quality of patient care. Int J Qual Health Care. 2008;20(1):22–30.
Vermeir P, Vandijck D, Degroote S, Peleman R, Verhaeghe R, Mortier E, et al. Communication in healthcare: a narrative review of the literature and practical recommendations. Int J Clin Pract. 2015;69(11):1257–67.
Eljiz K, Greenfield D, Hogden A, Taylor R, Siddiqui N, Agaliotis M, et al. Improving knowledge translation for increased engagement and impact in healthcare. BMJ open Qual. 2020;9(3):e000983.
O’Brien JL, Shortell SM, Hughes EF, Foster RW, Carman JM, Boerstler H, et al. An integrative model for organization-wide quality improvement: lessons from the field. Qual Manage Healthc. 1995;3(4):19–30.
Adily A, Girgis S, D’Este C, Matthews V, Ward JE. Syphilis testing performance in Aboriginal primary health care: exploring impact of continuous quality improvement over time. Aust J Prim Health. 2020;26(2):178–83.
Horwood C, Butler L, Barker P, Phakathi S, Haskins L, Grant M, et al. A continuous quality improvement intervention to improve the effectiveness of community health workers providing care to mothers and children: a cluster randomised controlled trial in South Africa. Hum Resour Health. 2017;15:1–11.
Veillard J, Cowling K, Bitton A, Ratcliffe H, Kimball M, Barkley S, et al. Better measurement for performance improvement in low- and middle-income countries: the primary Health Care Performance Initiative (PHCPI) experience of conceptual framework development and indicator selection. Milbank Q. 2017;95(4):836–83.
Barbazza E, Kringos D, Kruse I, Klazinga NS, Tello JE. Creating performance intelligence for primary health care strengthening in Europe. BMC Health Serv Res. 2019;19(1):1006.
Assefa Y, Hill PS, Gilks CF, Admassu M, Tesfaye D, Van Damme W. Primary health care contributions to universal health coverage. Ethiopia Bull World Health Organ. 2020;98(12):894.
Van Weel C, Kidd MR. Why strengthening primary health care is essential to achieving universal health coverage. CMAJ. 2018;190(15):E463–466.
Download references
Acknowledgements
Not applicable.
The authors received no fund.
Author information
Authors and affiliations.
School of Public Health, The University of Queensland, Brisbane, Australia
Aklilu Endalamaw, Resham B Khatri, Tesfaye Setegn Mengistu, Daniel Erku & Yibeltal Assefa
College of Medicine and Health Sciences, Bahir Dar University, Bahir Dar, Ethiopia
Aklilu Endalamaw & Tesfaye Setegn Mengistu
Health Social Science and Development Research Institute, Kathmandu, Nepal
Resham B Khatri
Centre for Applied Health Economics, School of Medicine, Grifth University, Brisbane, Australia
Daniel Erku
Menzies Health Institute Queensland, Grifth University, Brisbane, Australia
International Institute for Primary Health Care in Ethiopia, Addis Ababa, Ethiopia
Eskinder Wolka & Anteneh Zewdie
You can also search for this author in PubMed Google Scholar
Contributions
AE conceptualized the study, developed the first draft of the manuscript, and managing feedbacks from co-authors. YA conceptualized the study, provided feedback, and supervised the whole processes. RBK provided feedback throughout. TSM provided feedback throughout. DE provided feedback throughout. EW provided feedback throughout. AZ provided feedback throughout. All authors read and approved the final manuscript.
Corresponding author
Correspondence to Aklilu Endalamaw .
Ethics declarations
Ethics approval and consent to participate.
Not applicable because this research is based on publicly available articles.
Consent for publication
Competing interests.
The authors declare that they have no competing interests.
Additional information
Publisher’s note.
Springer Nature remains neutral with regard to jurisdictional claims in published maps and institutional affiliations.
Supplementary Information
Supplementary material 1., supplementary material 2., rights and permissions.
Open Access This article is licensed under a Creative Commons Attribution 4.0 International License, which permits use, sharing, adaptation, distribution and reproduction in any medium or format, as long as you give appropriate credit to the original author(s) and the source, provide a link to the Creative Commons licence, and indicate if changes were made. The images or other third party material in this article are included in the article's Creative Commons licence, unless indicated otherwise in a credit line to the material. If material is not included in the article's Creative Commons licence and your intended use is not permitted by statutory regulation or exceeds the permitted use, you will need to obtain permission directly from the copyright holder. To view a copy of this licence, visit http://creativecommons.org/licenses/by/4.0/ . The Creative Commons Public Domain Dedication waiver ( http://creativecommons.org/publicdomain/zero/1.0/ ) applies to the data made available in this article, unless otherwise stated in a credit line to the data.
Reprints and permissions
About this article
Cite this article.
Endalamaw, A., Khatri, R.B., Mengistu, T.S. et al. A scoping review of continuous quality improvement in healthcare system: conceptualization, models and tools, barriers and facilitators, and impact. BMC Health Serv Res 24 , 487 (2024). https://doi.org/10.1186/s12913-024-10828-0
Download citation
Received : 27 December 2023
Accepted : 05 March 2024
Published : 19 April 2024
DOI : https://doi.org/10.1186/s12913-024-10828-0
Share this article
Anyone you share the following link with will be able to read this content:
Sorry, a shareable link is not currently available for this article.
Provided by the Springer Nature SharedIt content-sharing initiative
- Continuous quality improvement
- Quality of Care
BMC Health Services Research
ISSN: 1472-6963
- General enquiries: [email protected]
Generative AI Transforming South African Business Landscape
A brand new research paper highlights the fact that big corporations in South Africa are adopting Generate Artificial Intelligence (GenAI) at a continually increasing rate. This is a massive and quite important transformation in the country’s business strategy. According to the periodic publication of South African Generative AI Roadmap 2024 by World Wide Worx in alliance with Dell Technologies and Intel, 90% of decision-makers that respondents have used or plan to implicate in their business operations are now using or are going to utilize GenAI.
Rapid adoption and strategic deployment
The report, which explores the initial reactions of 100 entrepreneurs from sizable organizations to GenAI, reveals a lively connection. While just over half of the respondents (45%) have already materialized the technologies, the other respondents (45%) are equally evaluating their possible future applications. However, only 10% of the respondents said they have yet to make plans for the immediate utilization of GenAI, which is actually unsurprising. The report provides evidence of such a trend, and businesses that mostly use public GenAI services are experimenting with it, while some are going forward into the more advanced cloud and premises-based services.
GenAI is definitely for productivity, boosting competitiveness, and eventually reorganizing all industries’ jobs. A staggering 95.6% of the interviewees expressed the view that GenAI is a powerful instrument for optimizing production lines, sales, customer service, and business performance. Besides, the technology can automate monotonous tasks, enhance work processes, and offer decision data, which are essential in the realization of innovation and long-term development.
As demonstrated in the report, the most popular use cases involve reaching customers and improving customer relationships through product research, market analysis, and content creation. These applications demonstrate GenAI’s ability to not only facilitate existing processes but also design processes that create new opportunities for business development.
Success factors and Dell’s role in GenAI integration
According to the report, a successful GenAI deployment is largely dependent on the following crucial issues i.e. strategic approach quality security, a supportive organization culture resource allocation, and information sharing. It is now clear why Dell’s contribution served as a key enabler of AI adoption. Applying its AI consulting to conduct evaluation and integration to optimize AI contexts, Dell assists firms from the start to the end.
Customers have access to Dell’s Exploration and Validation Facilities (EVF), within our customer centers and are provided with not only the expertise but also the infrastructure, platforms, and tools necessary to conduct proofs of concept and validate their designs without risking their data. Thus, personalization of this approach eliminates the chances of rivalry and safeguards intellectual property and copyrights while ensuring that the issues of the organization are solved collectively and they can innovate collectively.
Embracing the GenAI wave
The South African Generative AI Roadmap 2024 isn’t only an influential guide for establishments that are ready to work with and derive benefits from GenAI. Still, it is also proof that South Africa is ever in search of innovative technologies. Companies should be aware of these impending waves, as the report has unambiguously made it clear that businesses that do not embrace these revolutionary approaches must bow to the stern tides of an AI-driven international economy.
The availability of GenAI lends itself to unprecedented productivity breakthroughs. At the same time, it allows any South African business to vie for a number one position in the global market. Those who have a keen interest in becoming a part of this transforming tale are welcome to attend the webcast conducted by World Wide Worx, Dell Technologies, and Intel to gain an in-depth understanding of the growing trends observed in the current tech market. The realization plan, thus, provides for an optimistic future that will lead South African businesses into a new horizon of business innovation and strategic advantage with the enhancement of GenAI.
This article originally appeared in Bizcommunity
Numbers, Facts and Trends Shaping Your World
Read our research on:
Full Topic List
Regions & Countries
- Publications
- Our Methods
- Short Reads
- Tools & Resources
Read Our Research On:
Millennials stand out for their technology use, but older generations also embrace digital life
Our approach to generational analysis has evolved to incorporate new considerations. Learn more about how we currently report on generations , and read tips for consuming generations research .
Millennials have often led older Americans in their adoption and use of technology, and this largely holds true today. But there has been significant growth in tech adoption since 2012 among older generations – particularly Gen Xers and Baby Boomers.
More than nine-in-ten Millennials (93% of those who turn ages 23 to 38 this year ) own smartphones, compared with 90% of Gen Xers (those ages 39 to 54 this year), 68% of Baby Boomers (ages 55 to 73) and 40% of the Silent Generation (74 to 91), according to a new analysis of a Pew Research Center survey of U.S. adults conducted in early 2019.
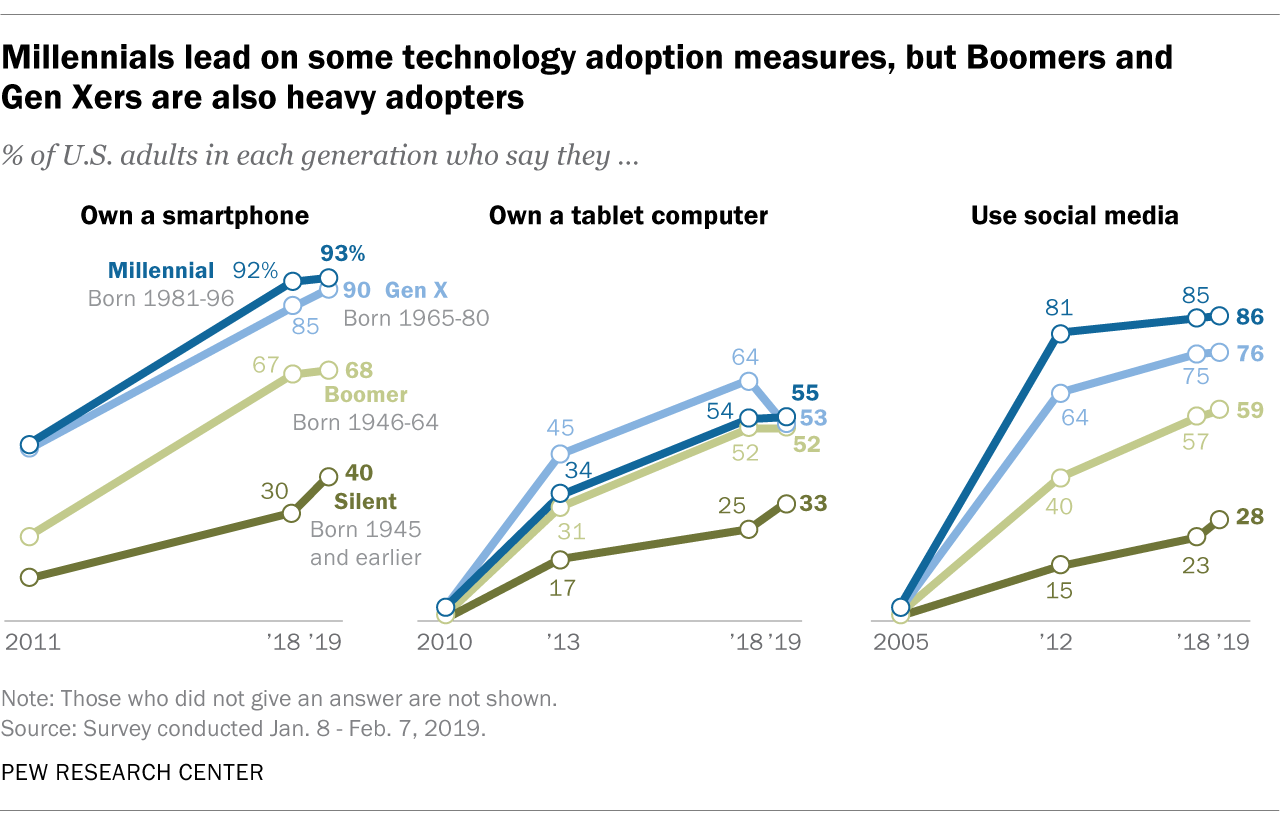
Similarly, the vast majority of Millennials (86%) say they use social media, compared with smaller shares among older generations. While the share of Millennials who say they use social media has remained largely unchanged since 2012, the shares of Gen Xers, Boomers and Silents who use social media all have increased by at least 10 percentage points during this period.
Unlike with smartphones and social media, tablet ownership is now comparable across most generations. Today, 55% of Gen Xers, 53% of Millennials and 52% of Boomers say they own tablets. A smaller share of Silents (33%) report owning tablets.
Those in the Silent Generation also lag when it comes to having broadband service at home. Whereas most Millennials (78%), Gen Xers (78%) and Boomers (74%) say they subscribe to home broadband, fewer than half of Silents (45%) say this.
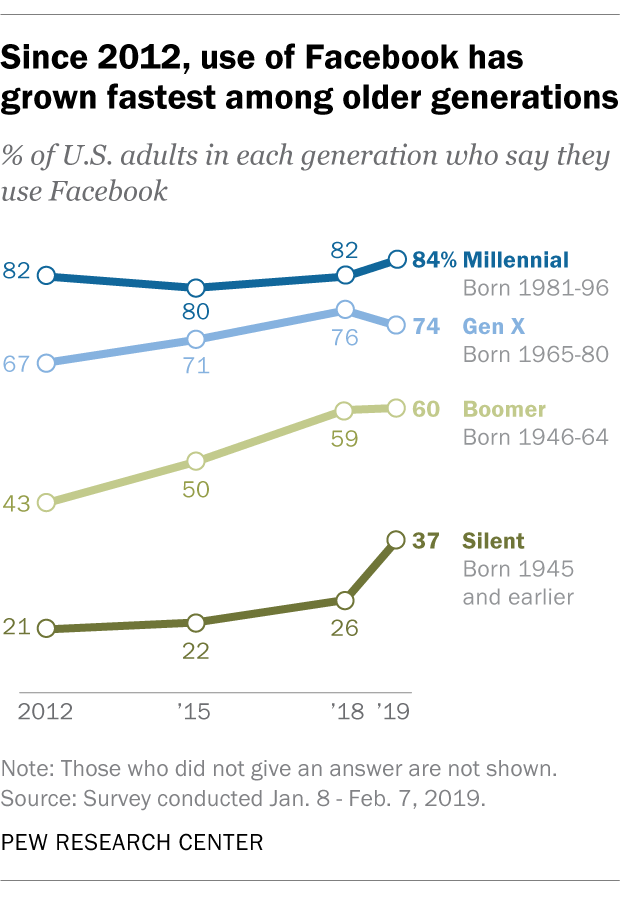
In terms of specific platforms, around three-fourths or more of both Millennials and Gen Xers now report using Facebook (84% vs. 74%, respectively). Boomers and Silents have both increased their Facebook use by double digits since 2015. In fact, the share of Silents using Facebook has nearly doubled in the past four years, from 22% to 37%.
Almost all Millennials (nearly 100%) now say they use the internet, and 19% of them are smartphone-only internet users – that is, they own a smartphone but do not have broadband internet service at home. Large shares of Gen Xers (91%) and Boomers (85%) use the internet, compared with just 62% of Silents. When it comes to smartphone-only internet users, 17% of Gen Xers go online primarily via a smartphone, as do 11% of Boomers and 15% of Silents.
Baby Boomers continue to trail both Gen Xers and Millennials on most measures of technology adoption, but adoption rates for this group have been growing rapidly in recent years. For example, Boomers are now far more likely to own a smartphone than they were in 2011 (68% now vs. 25% then).
Although Boomers have been adopting a range of technologies in recent years, members of the Silent Generation are less likely to have done so. Four-in-ten Silents (40%) report owning a smartphone, and fewer (33%) indicate that they have a tablet computer or use social media (28%). Previous Pew Research Center surveys have found that the oldest adults face some unique barriers to adopting new technologies – from a lack of confidence in using new technologies to physical challenges manipulating various devices.
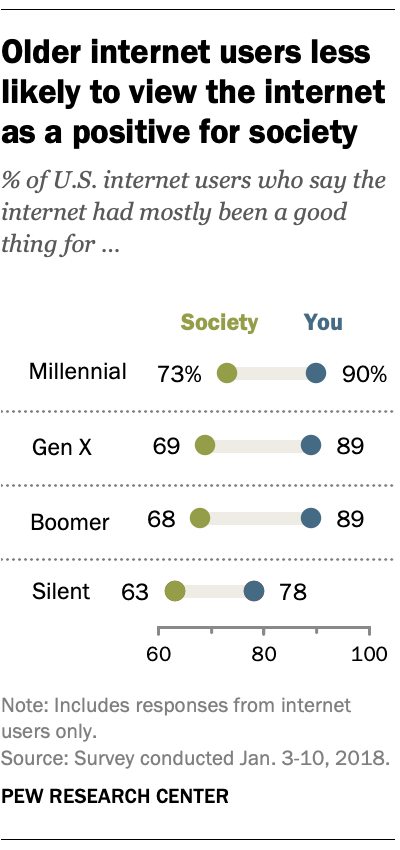
While generations differ in their use of various technologies, a 2018 Center survey found that younger internet users also were more likely than older Americans who use the internet to say the internet has had a positive impact on society: 73% of online Millennials said the internet has been mostly a good thing for society, compared with 63% of users in the Silent Generation.
Americans were also less positive about the societal impact of the internet last year than four years earlier. Gen Xers’ views of the internet’s impact on society declined the most in that time. In 2014, 80% of Gen X internet users believed the internet had been mostly a positive thing for society, a number that dropped to 69% in 2018. Millennial and Silent internet users were also somewhat less optimistic last year than in 2014.
Note: This is an update of a post originally published May 2, 2018, and written by Jingjing Jiang, a former research analyst focusing on internet and technology. See full topline results and methodology here .
- Age & Generations
- Baby Boomers
- Comparison of Generations
- Generation X
- Millennials
- Older Adults & Tech
- Silent Generation
- Social Media
Emily A. Vogels is a former research associate focusing on internet and technology at Pew Research Center
How Teens and Parents Approach Screen Time
Who are you the art and science of measuring identity, u.s. centenarian population is projected to quadruple over the next 30 years, older workers are growing in number and earning higher wages, teens, social media and technology 2023, most popular.
1615 L St. NW, Suite 800 Washington, DC 20036 USA (+1) 202-419-4300 | Main (+1) 202-857-8562 | Fax (+1) 202-419-4372 | Media Inquiries
Research Topics
- Coronavirus (COVID-19)
- Economy & Work
- Family & Relationships
- Gender & LGBTQ
- Immigration & Migration
- International Affairs
- Internet & Technology
- Methodological Research
- News Habits & Media
- Non-U.S. Governments
- Other Topics
- Politics & Policy
- Race & Ethnicity
- Email Newsletters
ABOUT PEW RESEARCH CENTER Pew Research Center is a nonpartisan fact tank that informs the public about the issues, attitudes and trends shaping the world. It conducts public opinion polling, demographic research, media content analysis and other empirical social science research. Pew Research Center does not take policy positions. It is a subsidiary of The Pew Charitable Trusts .
Copyright 2024 Pew Research Center
Terms & Conditions
Privacy Policy
Cookie Settings
Reprints, Permissions & Use Policy
Asking the better questions that unlock new answers to the working world's most complex issues.
Trending topics
AI insights
EY podcasts
EY webcasts
Operations leaders
Technology leaders
Marketing and growth leaders
Cybersecurity and privacy leaders
Risk leaders
EY Center for Board Matters
EY helps clients create long-term value for all stakeholders. Enabled by data and technology, our services and solutions provide trust through assurance and help clients transform, grow and operate.
Artificial Intelligence (AI)
Strategy, transaction and transformation consulting
Technology transformation
Tax function operations
Climate change and sustainability services
EY Ecosystems
Supply chain and operations
EY Partner Ecosystem
Explore Services
We bring together extraordinary people, like you, to build a better working world.
Experienced professionals
MBA and advanced-degree students
Student and entry level programs
Contract workers
EY-Parthenon careers
Discover how EY insights and services are helping to reframe the future of your industry.
Case studies
Energy and resources
How data analytics can strengthen supply chain performance
13-Jul-2023 Ben Williams
How Takeda harnessed the power of the metaverse for positive human impact
26-Jun-2023 Edwina Fitzmaurice
Banking and Capital Markets
How cutting back infused higher quality in transaction monitoring
11-Jul-2023 Ron V. Giammarco
At EY, our purpose is building a better working world. The insights and services we provide help to create long-term value for clients, people and society, and to build trust in the capital markets.
EY is now carbon negative
19-Sep-2022 Carmine Di Sibio
Our commitment to audit quality
13-Nov-2023 Julie A. Boland
No results have been found
Recent Searches
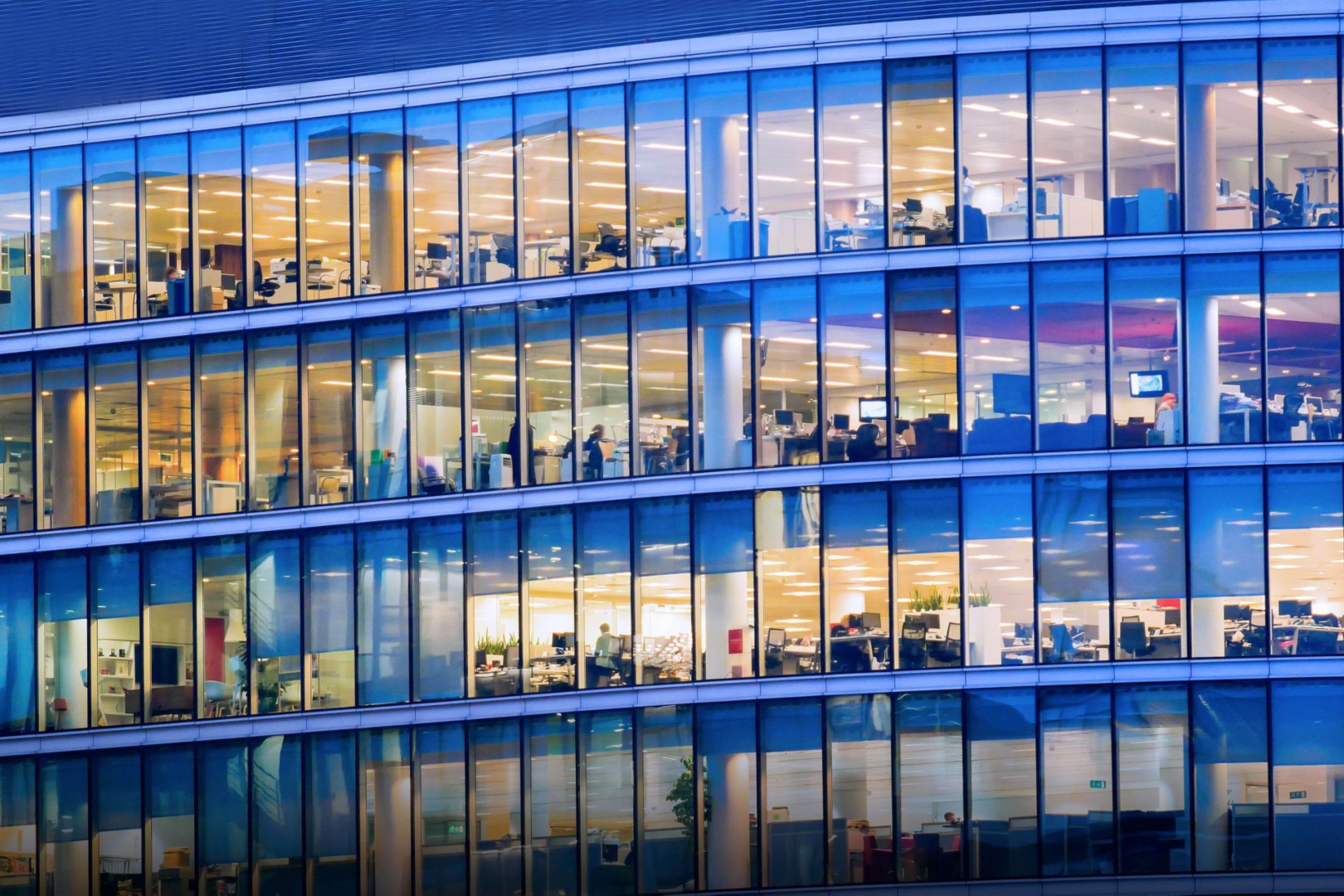
BEPS 2.0: as policies evolve, engagement is key
It remains to be seen whether the US will align its tax law with the OECD/G20’s global BEPS 2.0 rules. MNEs will feel the impact in 2024. Learn more.
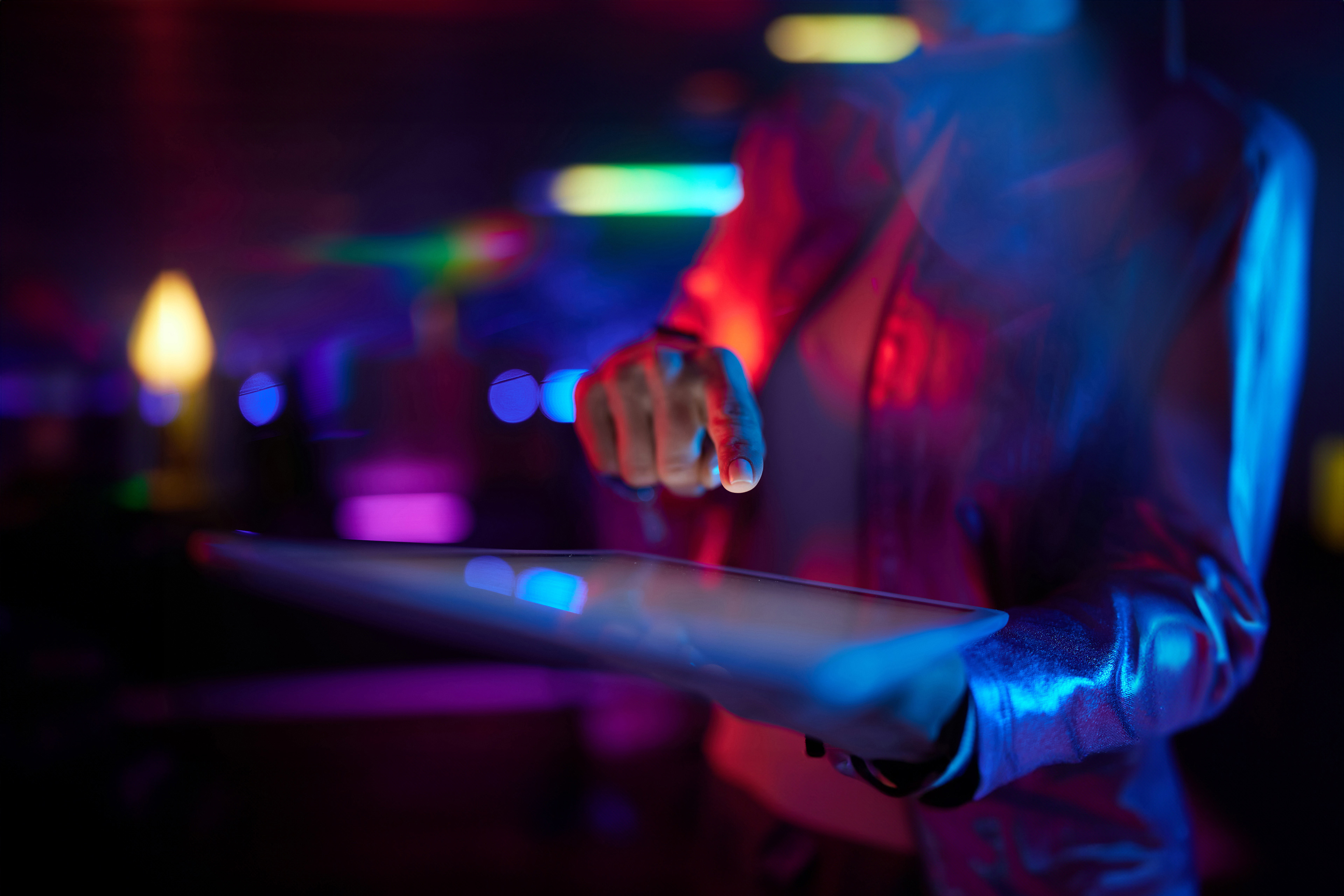
How GenAI strategy can transform innovation
Companies considering or investing in a transformative GenAI strategy should tie generative artificial intelligence use cases to revenue, cost and expense. Learn more
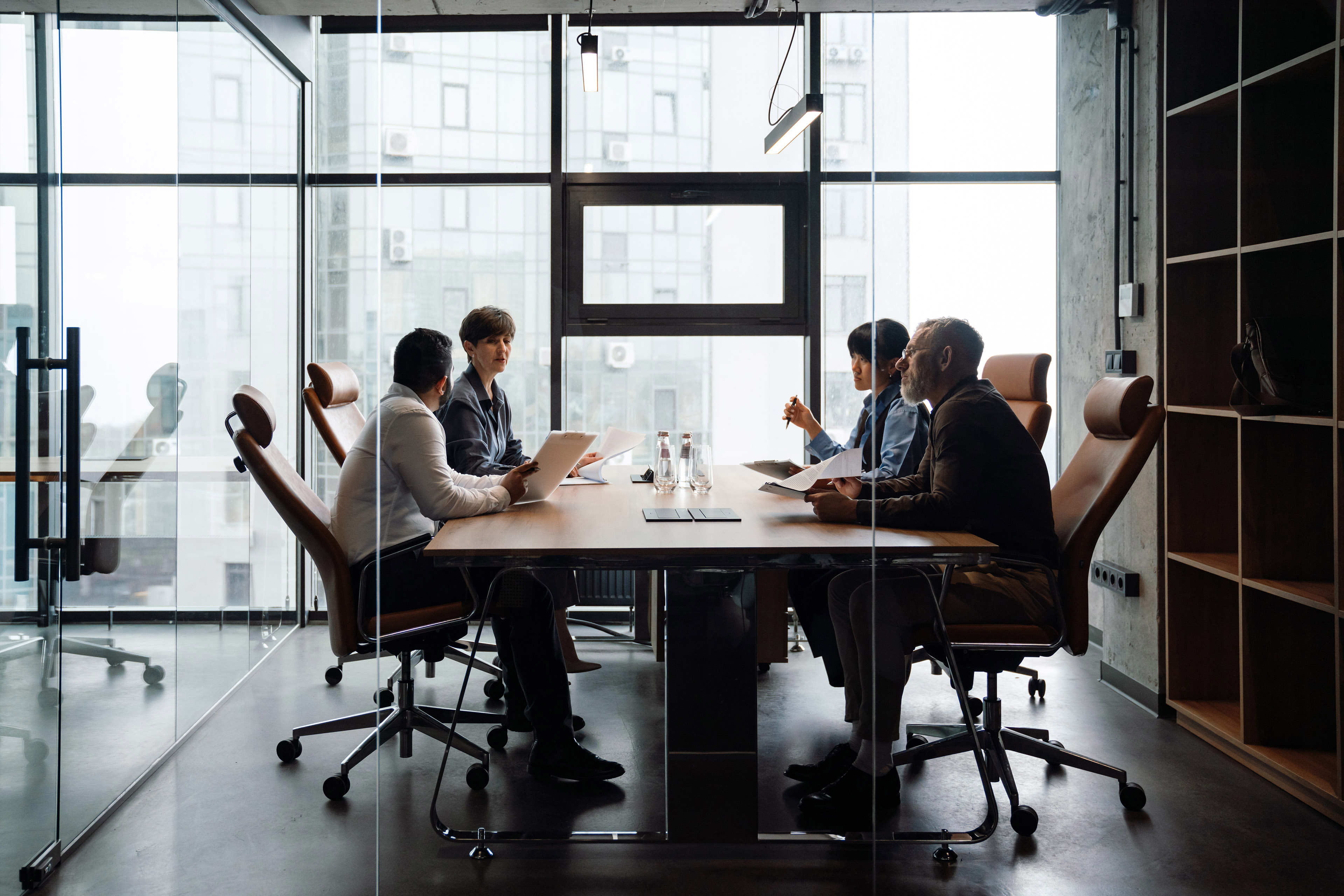
Top five private equity trends for 2024
Read about the five key trends private equity firms will emphasize in 2024 as they create value
Select your location
close expand_more
Banking & Capital Markets
The bank of the future will integrate disruptive technologies with an ecosystem of partners to transform their business and achieve growth.
Disruption is creating opportunities and challenges for global banks. While the risk and regulatory protection agenda remains a major focus, banks must also address financial performance and heightened customer and investor expectations, as they reshape and optimize operational and business models to deliver sustainable returns. Innovation and business-led transformation will be critical for future growth. To remain competitive and relevant, every bank must embrace disruption and strategically build a better ecosystem — not a bigger bank.
Our worldwide team of industry-focused assurance, tax, transaction and consulting professionals integrates sector knowledge and technical experience. We work with clients to navigate digital innovation, new business models and ecosystem partnerships, helping banks become the nimble, responsive organizations that customers demand.
Five priorities for harnessing the power of GenAI in banking</p> "> Five priorities for harnessing the power of GenAI in banking
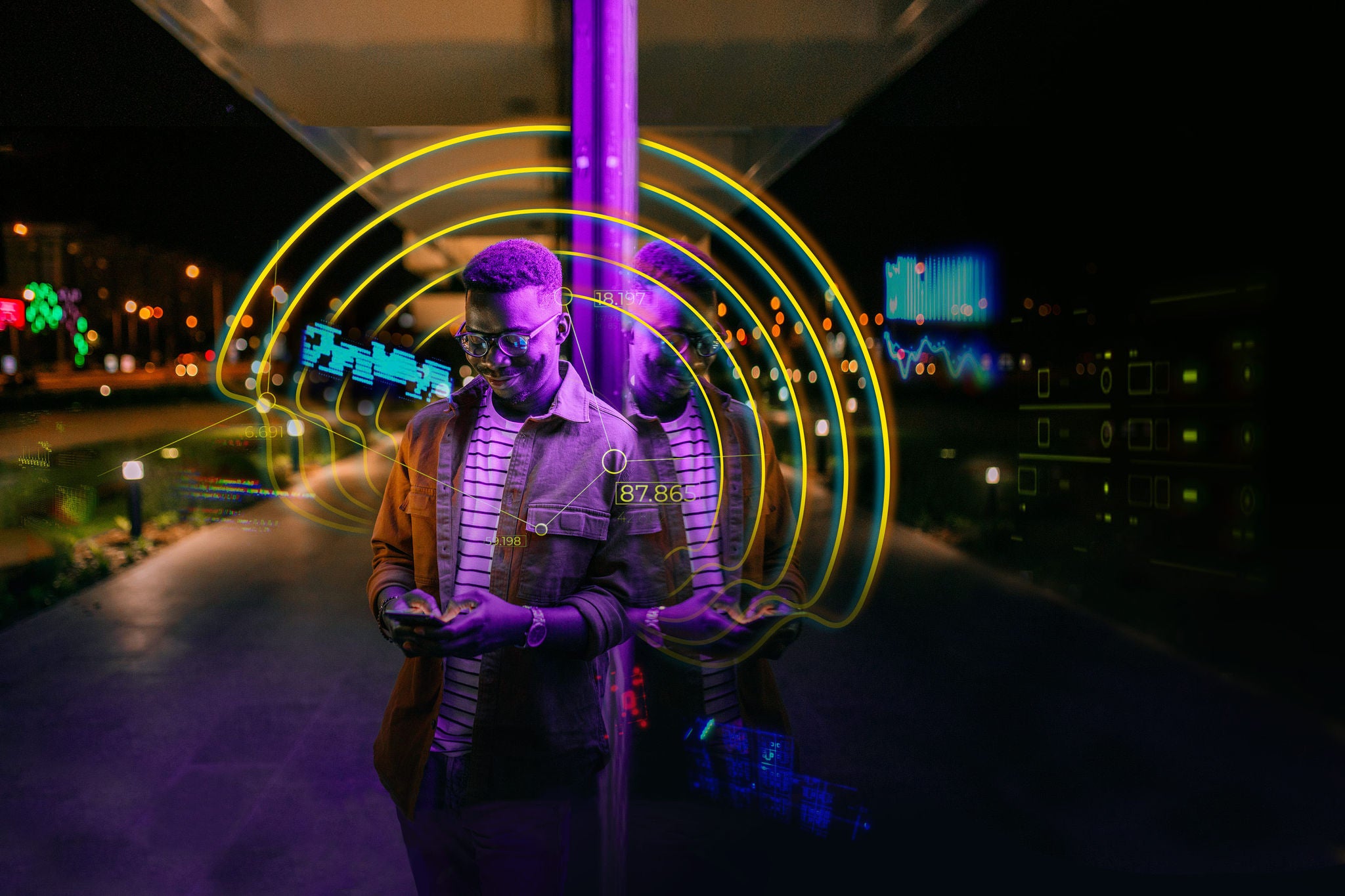
What to expect from global financial services in 2024 — Americas and EMEIA
In this webcast for Americas and EMEIA audiences, the EY Global Regulatory Network will discuss the direction of travel for regulators across key areas and how to prepare for what's coming.
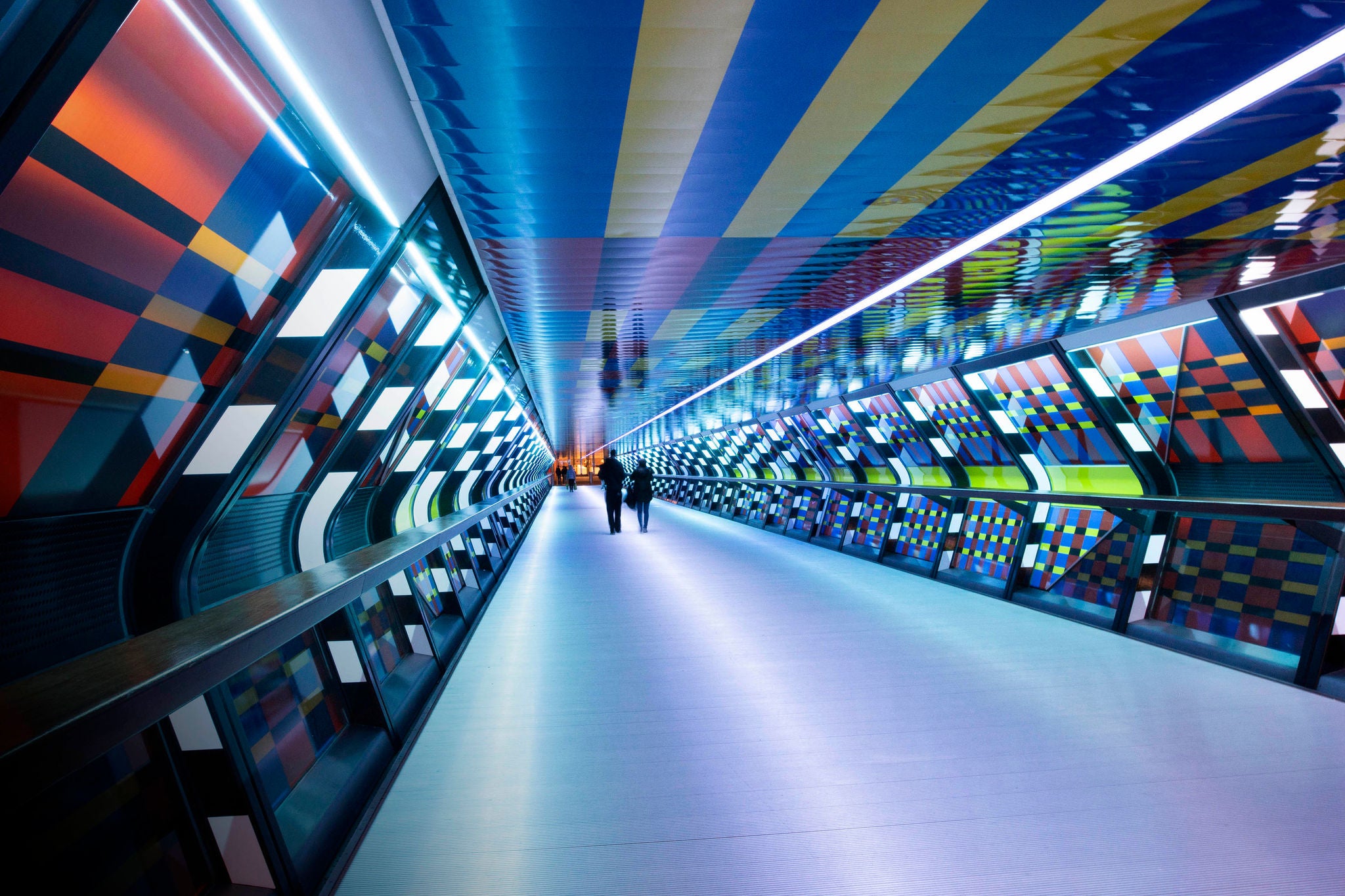
Our latest thinking on Banking & Capital Markets
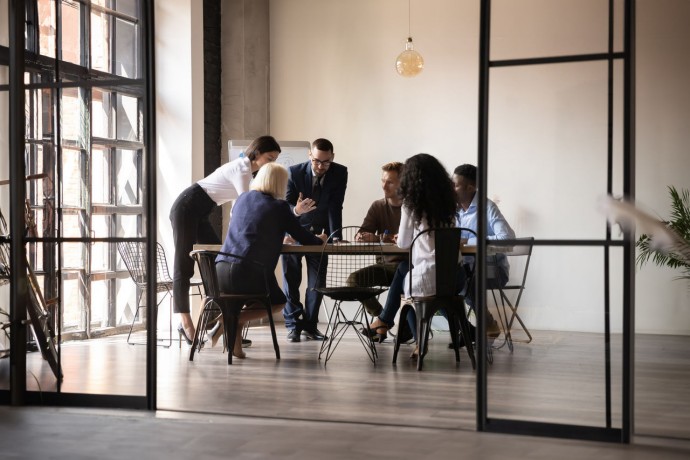
Impacts of Central Clearing of US Treasuries and Repo
In this webcast, panelists will discuss key themes and high-level requirements of the US Treasury and repo central clearing rules.
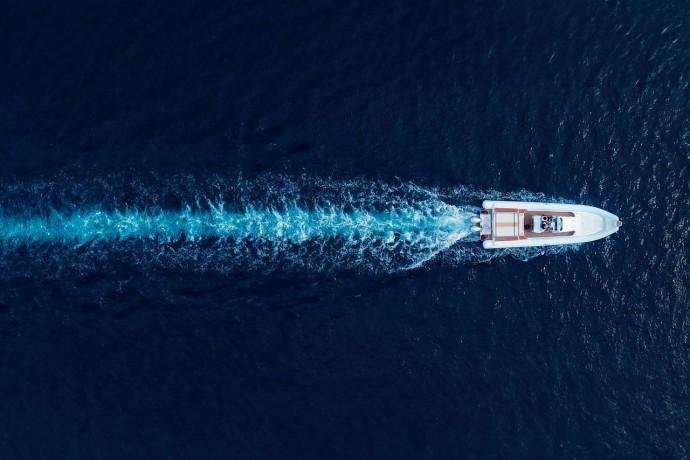
Can core platform modernization position a bank for future success?
Case study: how one regional bank used core platform modernization to build a strong foundation for future profitability.
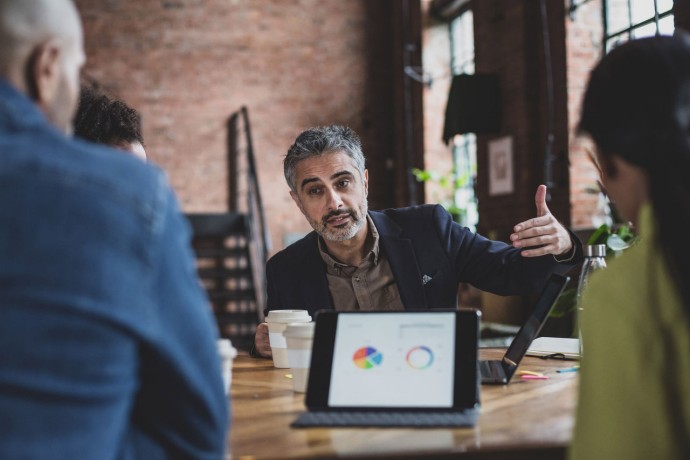
The case for a modern transaction banking platform
The evolution of corporate treasury management needs presents an opportunity for corporate banks. Learn from an industry approach.
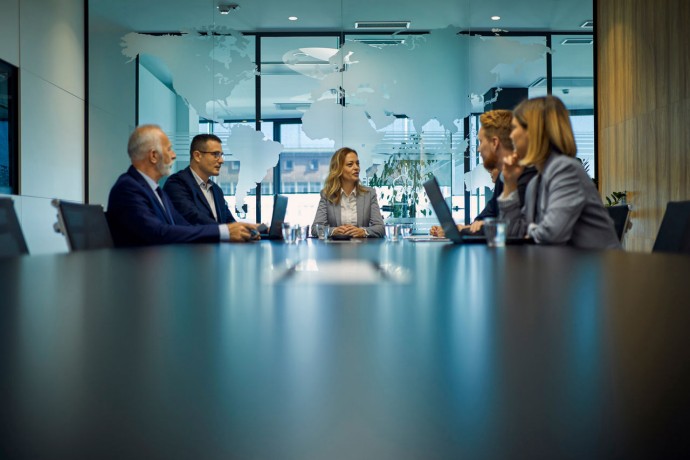
How to transition from a tactical to strategic adoption of ISO 20022
With ISO 20022 adoption lagging amid competing global deadlines, a successful migration may hinge on changing from a tactical to a strategic mindset.
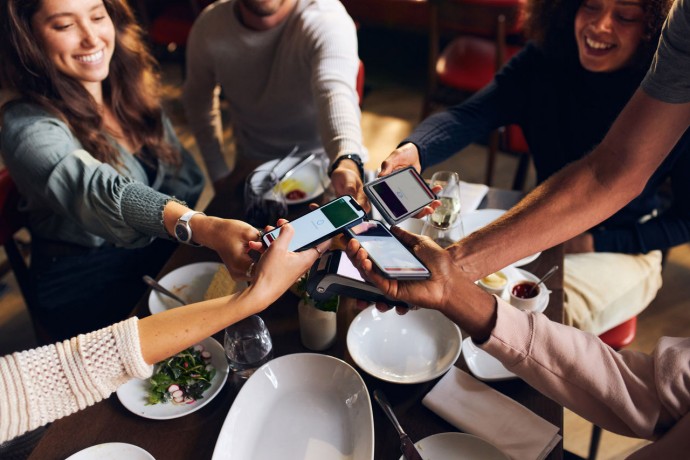
How Gen Z’s preference for digital is changing the payments landscape
EY survey shows Gen Z embraces simple, seamless payment methods. Learn more.
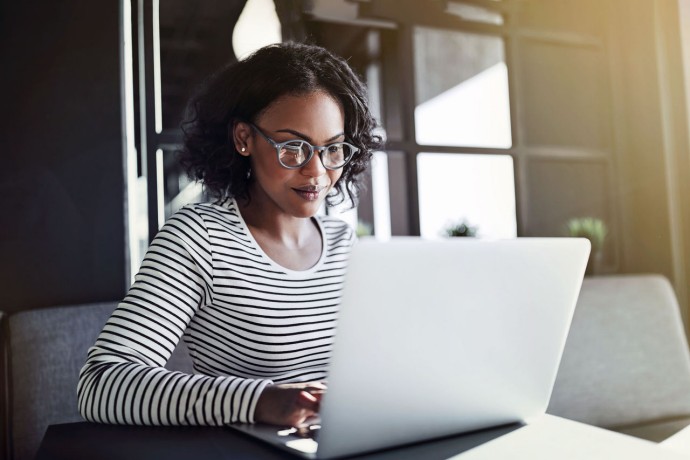
How can financial institutions modernize their fair-lending practices?
FIs that disregard fair banking are lagging behind FIs that enhance compliance procedures, lending models and data analytics to become more compliant. Read more.
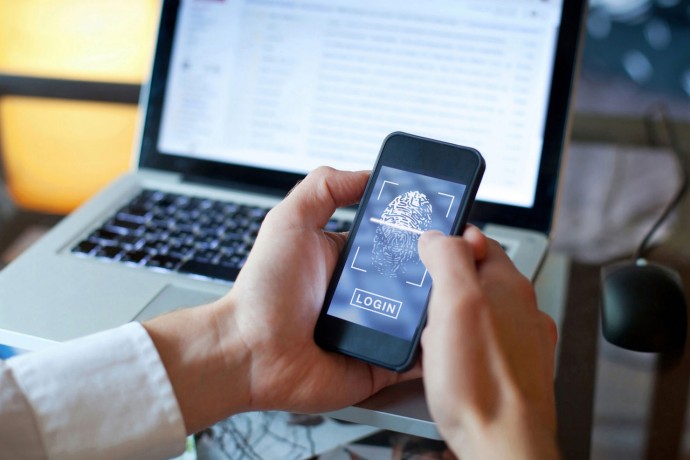
Digital identity opportunities in financial services
Exploring the policy and regulatory trends shaping digital identity and opportunities for financial services companies in a changing payment landscape.
Explore our Banking & Capital Markets case studies
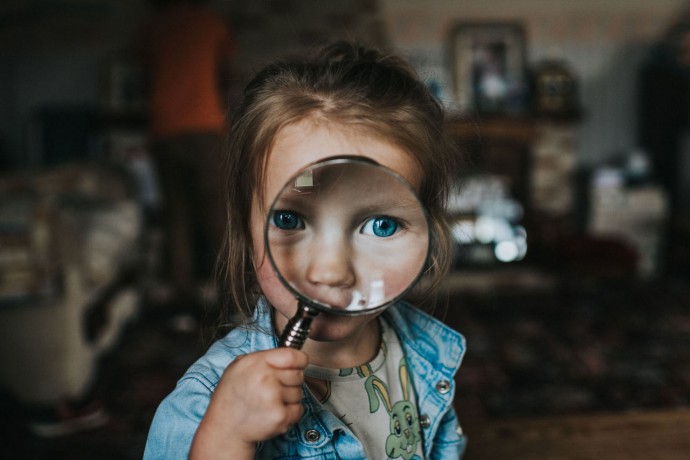
Using AI to augment pricing intelligence for banks
How an AI-powered digital tool, Smart Advisor (SA), helped one bank deliver better client service while maximizing value creation.
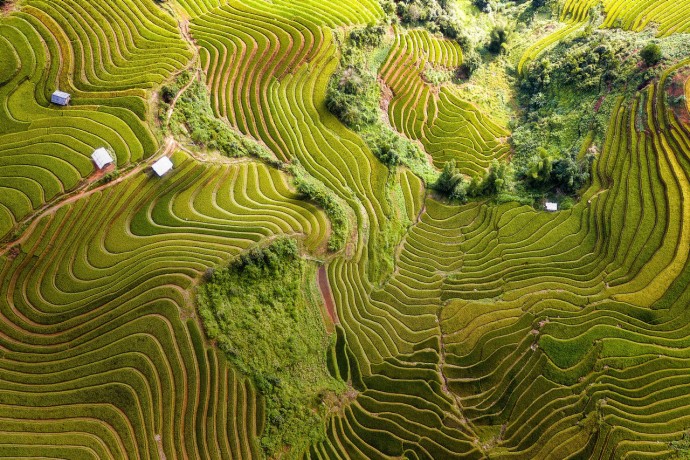
How a global FinTech captured growth in the SME segment
A global Fintech captured growth in an opportunistic SME segment with a differentiated, holistic strategy. Learn more in this case study.
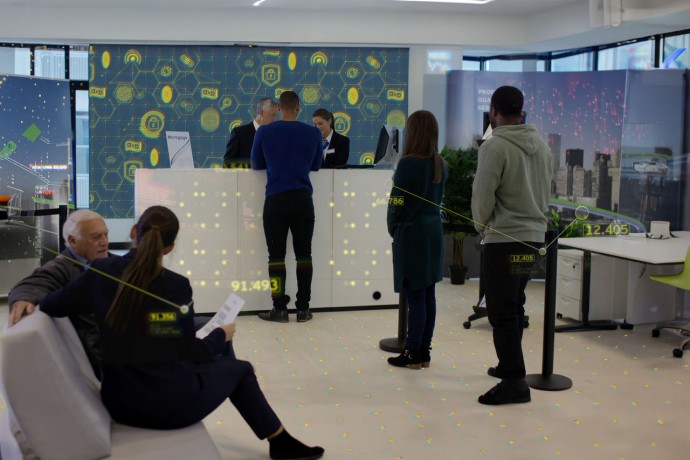
Using AI to improve a bank’s agent effectiveness
Leveraging the power of AI and machine learning, one bank mined sales agents’ calls for performance-boosting insights. Learn more in this case study.
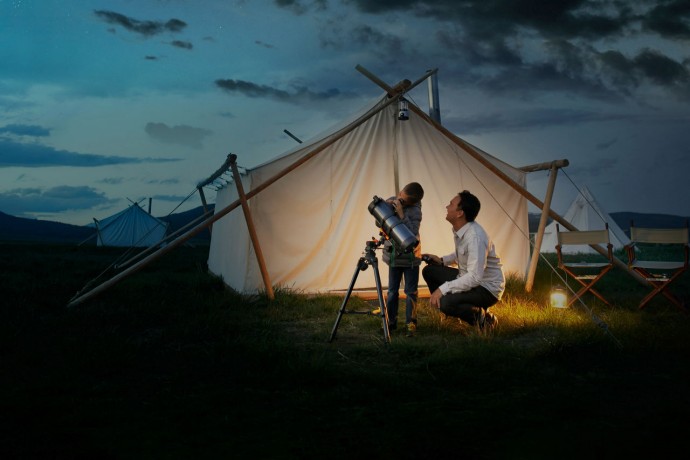
After cloud migration, investment bank sees potential for big dividends
A leading investment bank sought to move vital assets to the clouds by building an experienced, cross-functional team. Find out how.
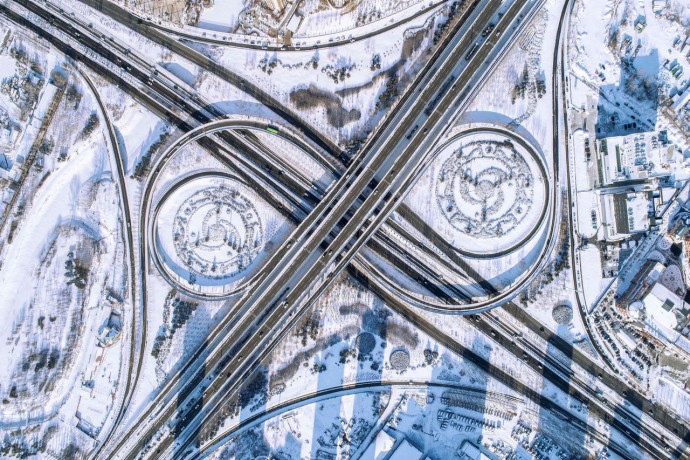
How digital transformation is redesigning trade finance
Banks that adopt an agile, design-based approach to digital transformation can boost the success of their trade finance functions.
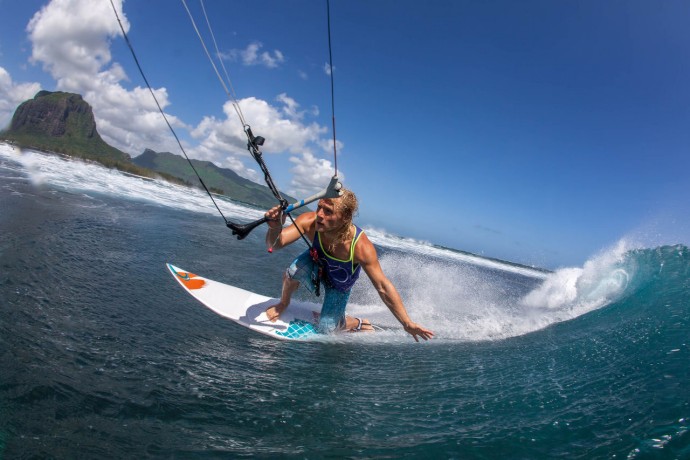
How to transform product development to outperform the competition
EY Nexus is a cloud-based platform offering access to the most advanced technologies to launch new products, businesses and services.
How EY can help
Capital Markets Services
Know how our Capital Markets consulting team can help your business grow, manage costs and meet regulatory requirements.
Consumer banking and wealth services
EY consumer banking and wealth technology solutions are designed to drive operational excellence and profitable growth. Learn more.
Corporate, Commercial and SME Banking services
Our Corporate, Commercial and SME (CCSB) Banking services team can help your business navigate through rising market expectation. Learn more.
Cost transformation
EY cost transformation teams help banks to optimize profits and fund transformation. Find out more.
Consumer lending services
Our consumer lending team can help navigate the complexities of unique lending propositions. Find out how.
EY Nexus for Banking
A transformative solution that accelerates innovation, unlocks value in your ecosystem, and powers frictionless business. Learn more.
Finance transformation
We help clients transform finance functions to be a strategic business partner for the business via value creation and controllership activities.
EY Financial Crime solutions
Our skilled teams, operational efficiencies enabled by innovative technology and flexible global delivery service centers can help you manage financial crime risk in a cost-effective, sustainable way.
Financial services risk management
Discover how EY can help the banking & capital markets, insurance, wealth & asset management and private equity sectors tackle the challenges of risk management.
IBOR transition services
EY helps global institutions prepare for the imminent transition away from Interbank Offered Rates (IBORs) to Alternate Reference Rates (ARRs). We also play a leading role in supporting regulators, trade associations and others to increase awareness and education.
Open banking services
Our open banking professionals can help your business maintain a trusted and secure open banking ecosystem while managing its risks. Learn more.
Payment services
Our payments professionals can help your business enhance innovation, drive growth and improve performance. Find out more.
Third-party risk management services
Discover how EY's Third Party Risk Management team can enable your business to make better decisions about the third parties they choose to work with.
Direct to your inbox
Stay up to date with our Editor‘s picks newsletter.
The Banking & Capital Markets team
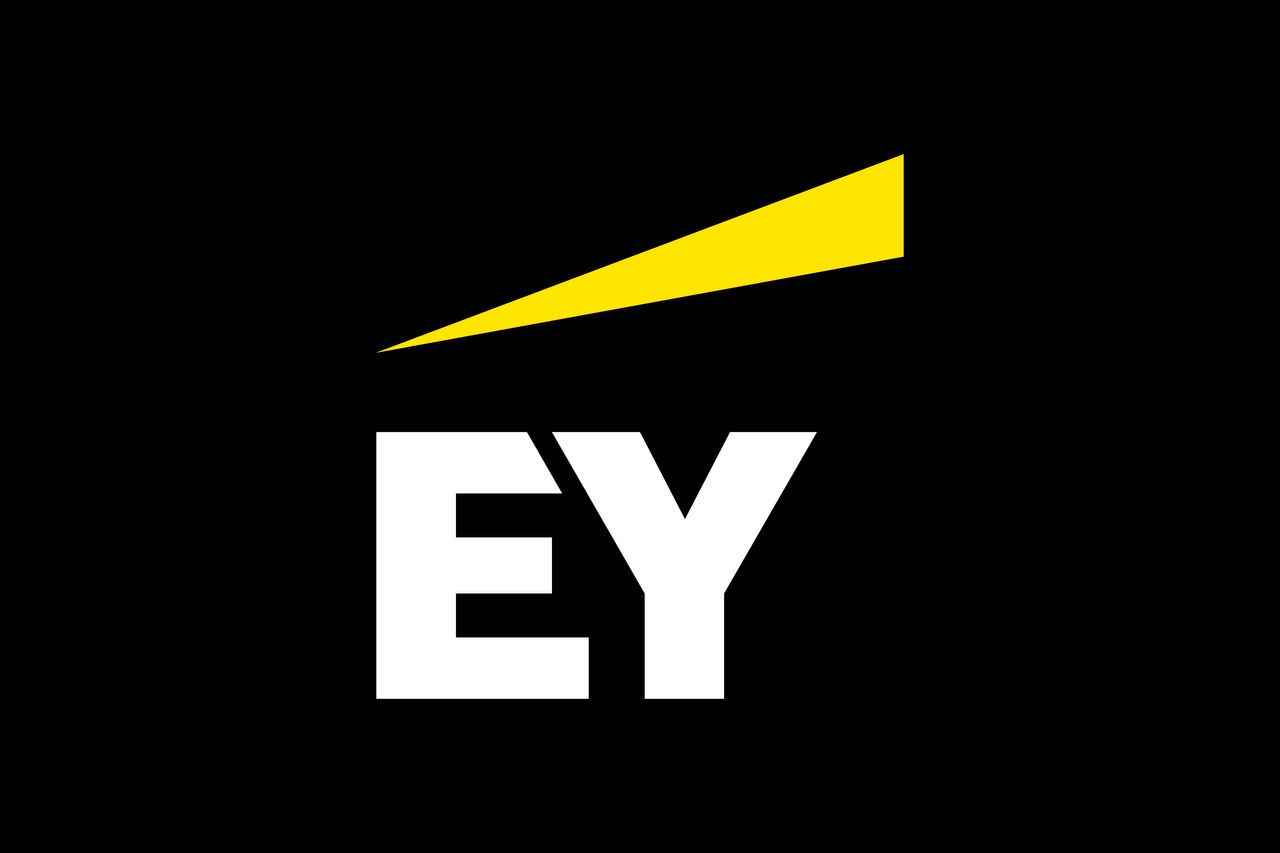
Enjoys traveling with family, and coaching his daughters’ basketball and soccer teams. Enjoys running and playing basketball and golf.
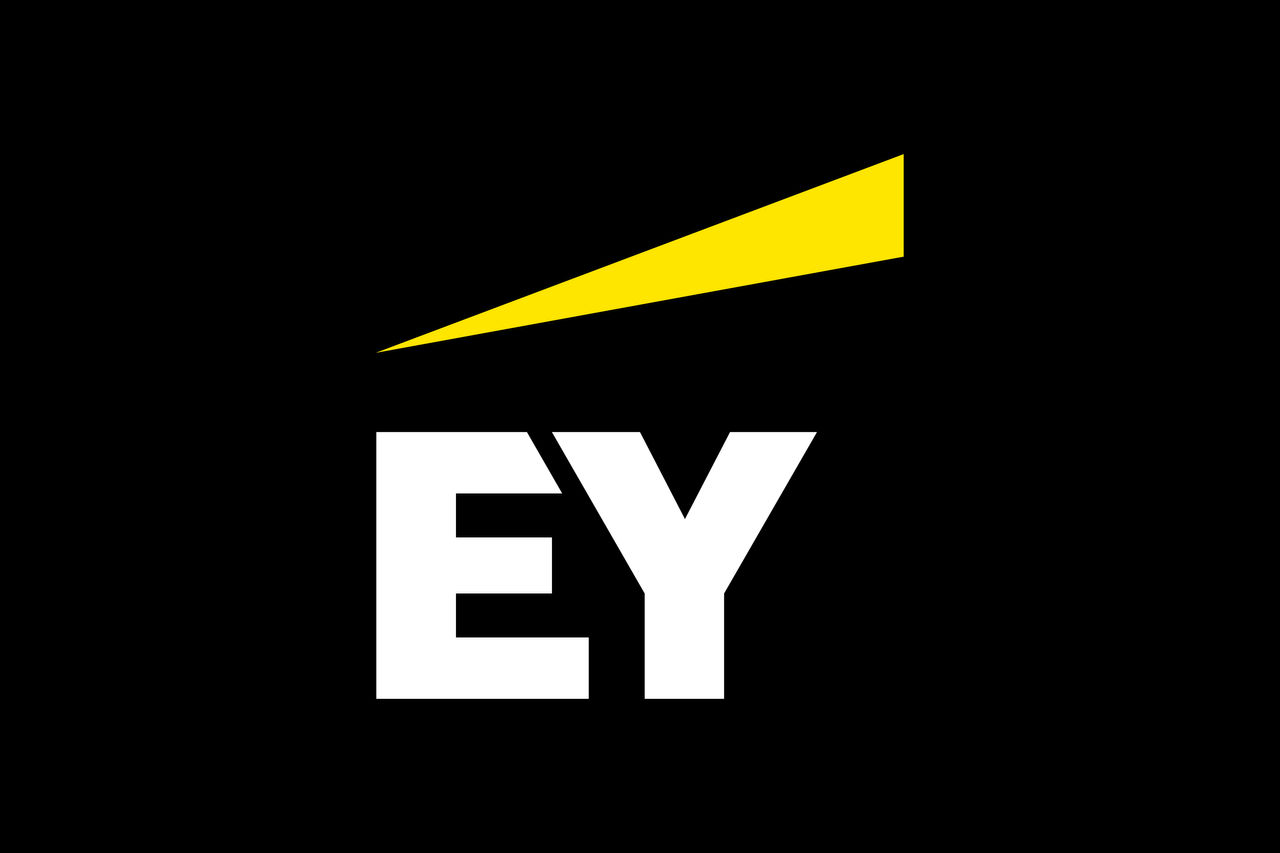
Lee Ann Lednik
People-focused leader committed to building trust and transparency amid increasing complexity. Passionate working mom of three. Aspiring photographer. Avid sports fan.
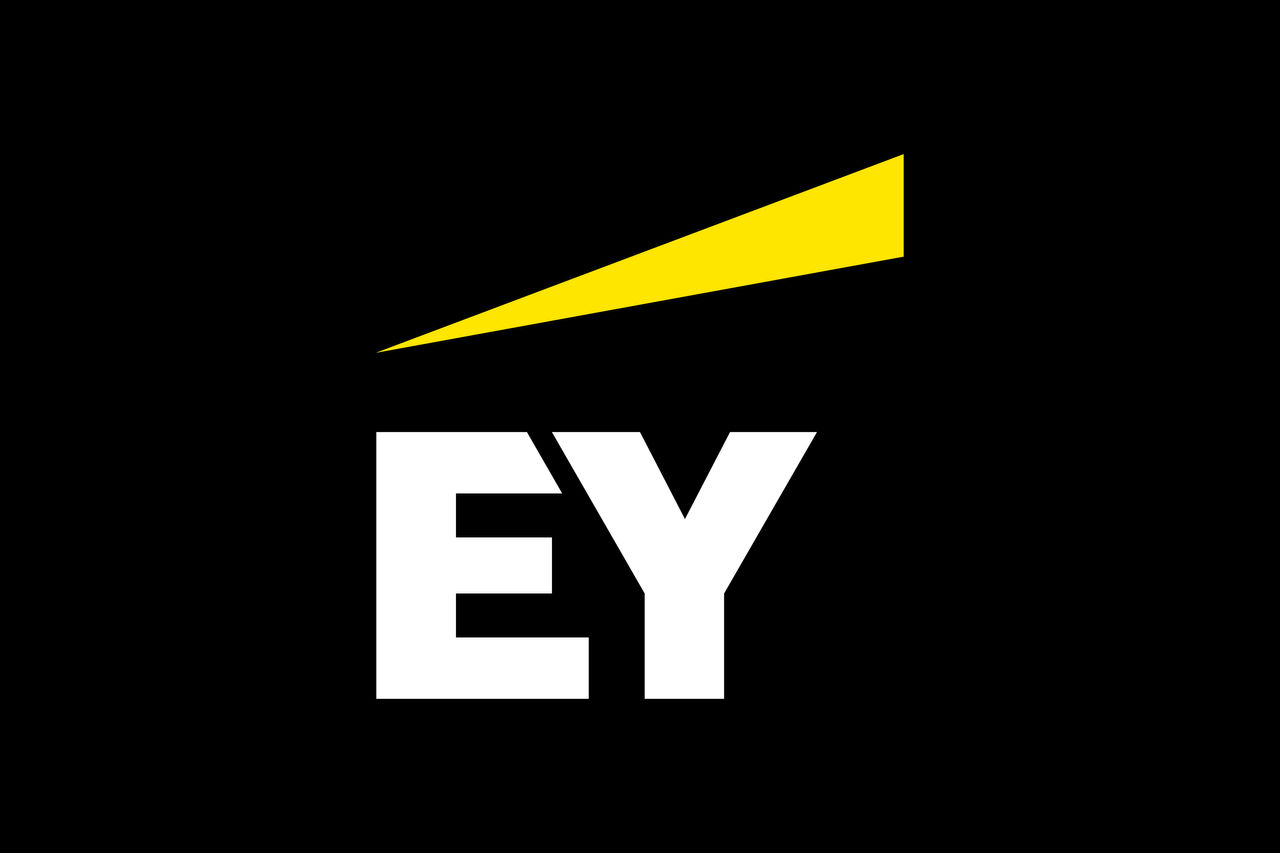
David Kadio-Morokro
Passionate about technology, innovation, and leading EY people to solve clients’ most challenging problems.
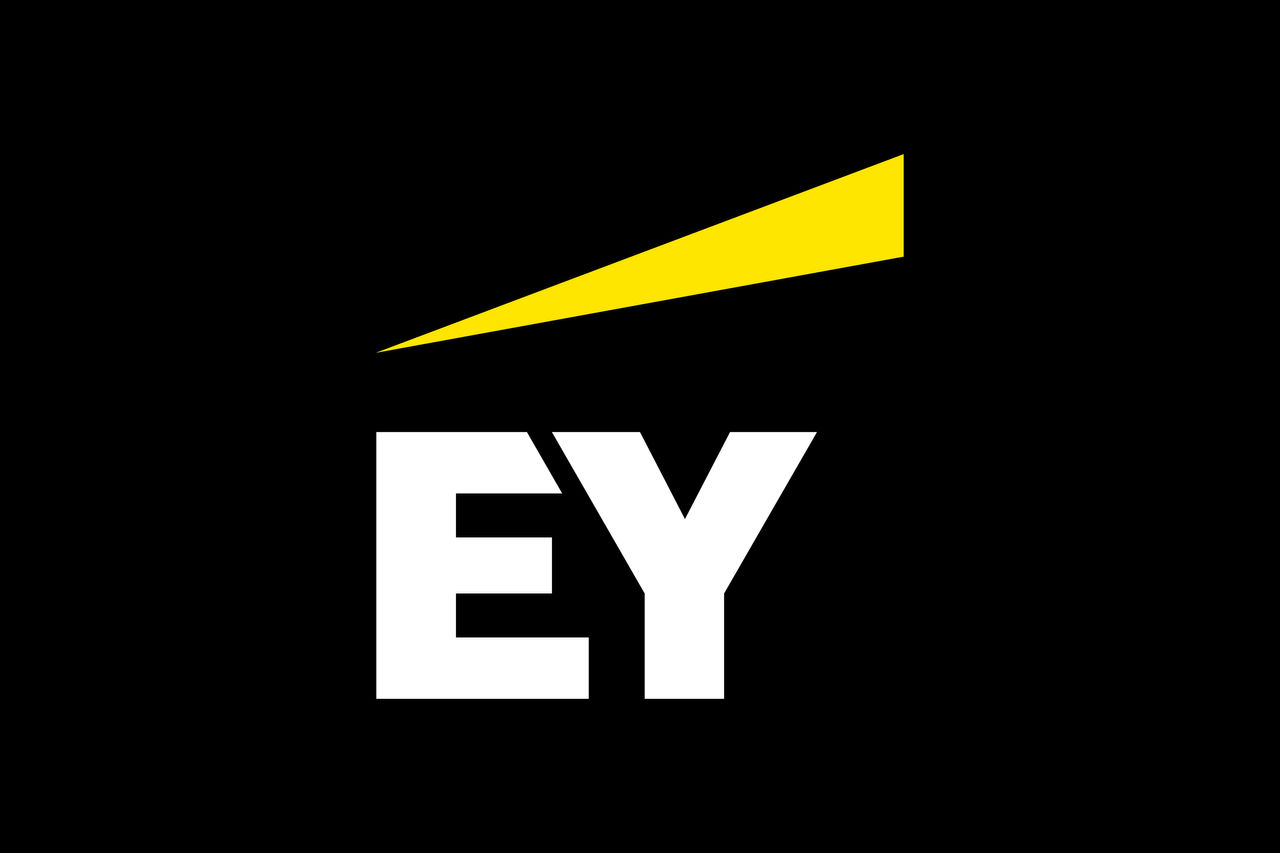
Heidi Boyle
Passionate about helping people thrive in the workplace and creating a sense of belonging for all. Writer. Musician. Cooking enthusiast.
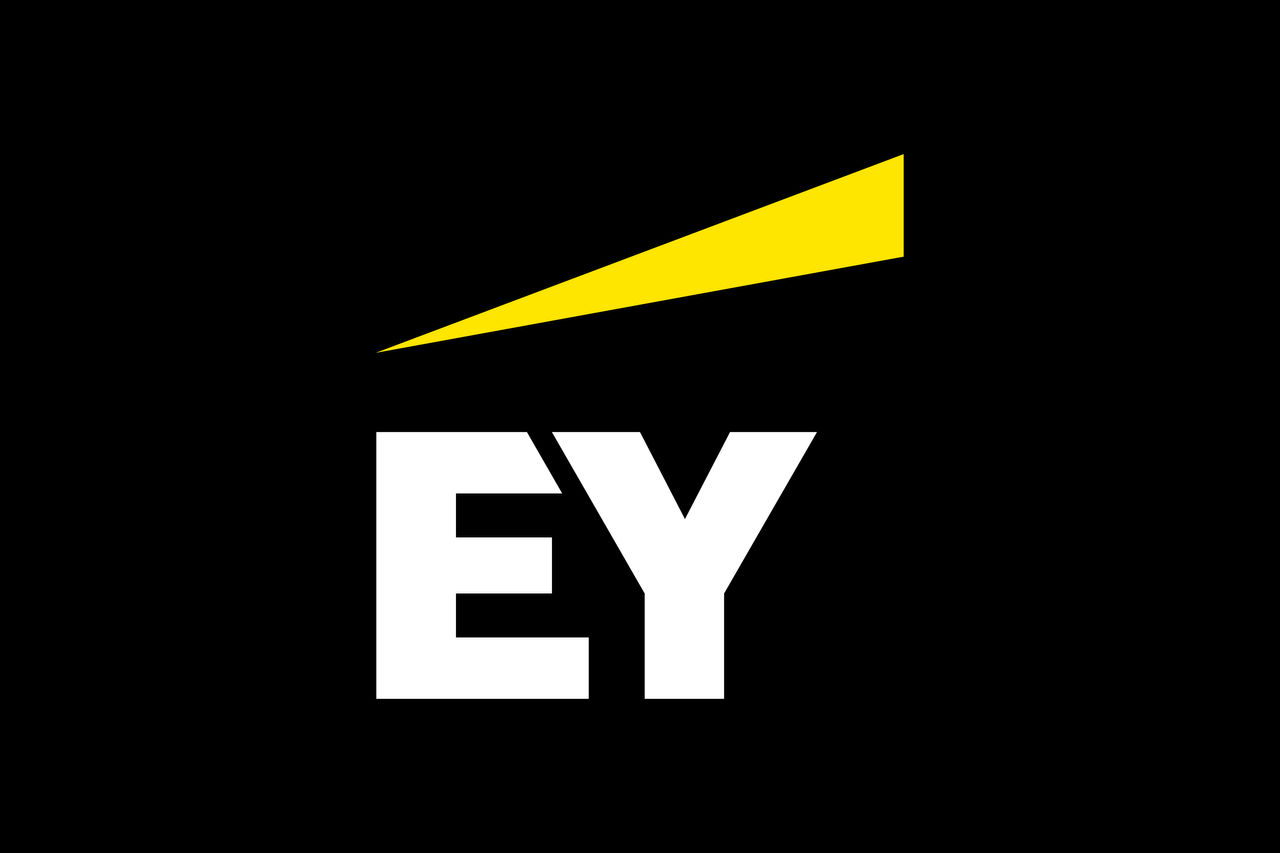
Seasoned financial services professional. Resides in Massachusetts with her husband and three children.
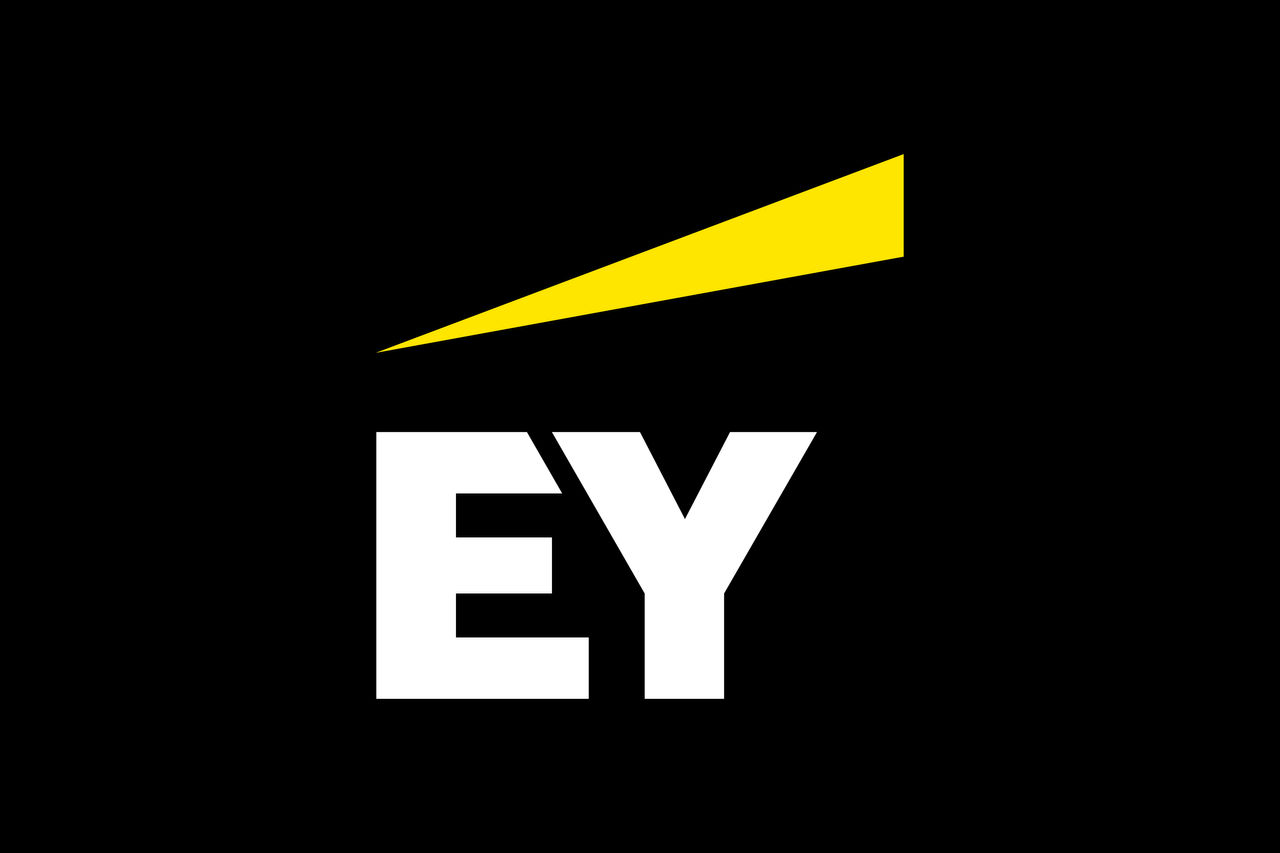
Kellen Maia de Sá
Collaborator and problem-solver with the desire to do the right thing. Leads efforts to help financial services clients with the disruption and impact of COVID-19.
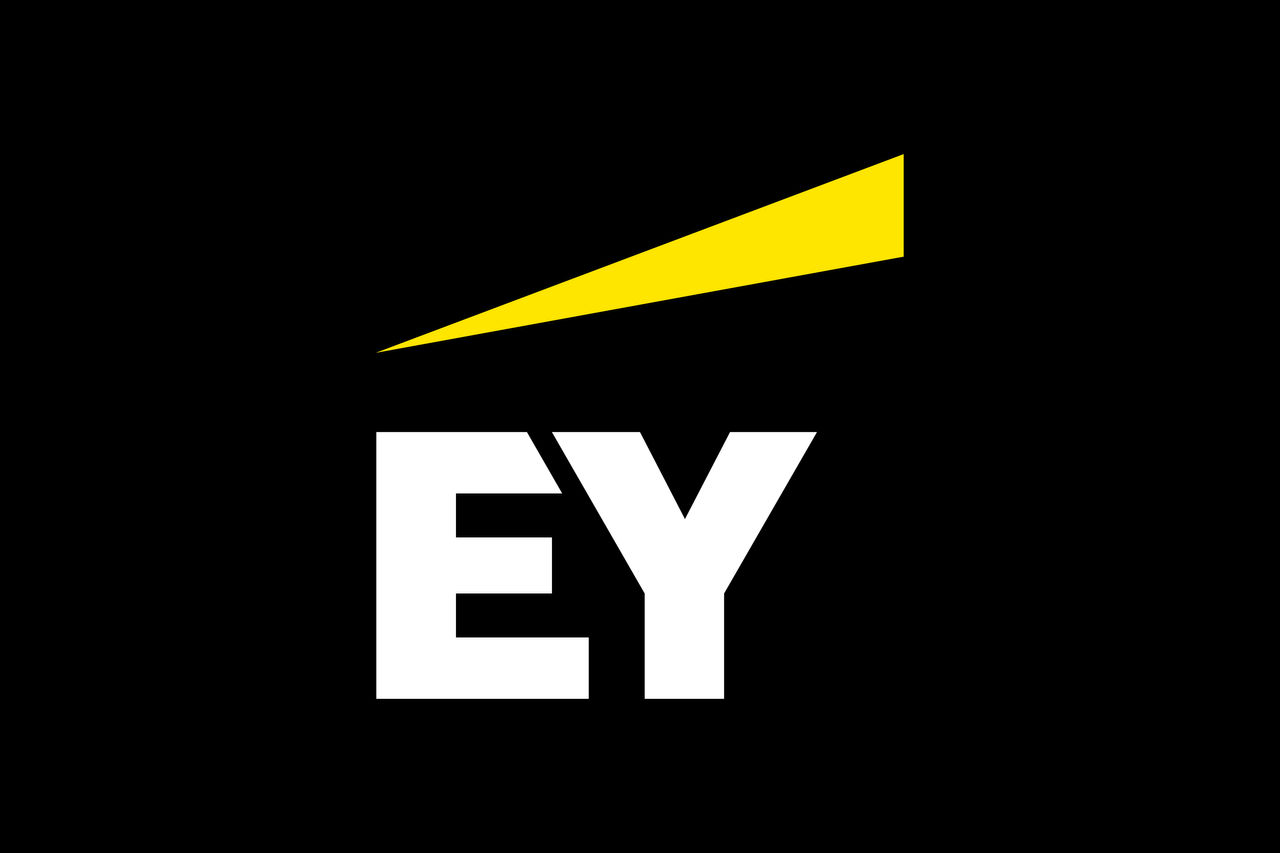
Terry Cardew
Builds trust by helping banks solve business issues and stay competitive. Devoted husband. Father of six. Avid skier. NY Giants and Yankees fan. Supporter of The Fresh Air Fund and Lynne’s Kids.
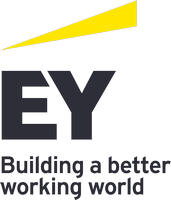
- Connect with us
- Our locations
- Do Not Sell or Share My Personal Information
- Legal and privacy
- Accessibility
- Open Facebook profile
- Open X profile
- Open LinkedIn profile
- Open Youtube profile
EY refers to the global organization, and may refer to one or more, of the member firms of Ernst & Young Global Limited, each of which is a separate legal entity. Ernst & Young Global Limited, a UK company limited by guarantee, does not provide services to clients.
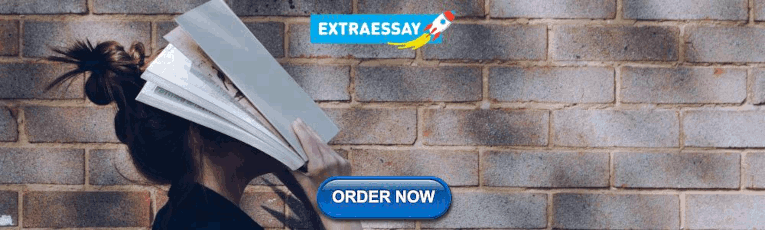
IMAGES
VIDEO
COMMENTS
This research note condenses prior comprehensive literature reviews on technology adoption conducted by Lee and Coughlin (2015), and Lee et al. (2018). Drawing from these papers, we will focus on three models of technology adoption that were published in 1989, 2003, and 2015. Technology Acceptance Model (TAM)
How and why individuals adopt innovations has motivated a great deal of research. This article examines individuals' computing adoption processes through the lenses of three adoption theories: Rogers's innovation diffusion theory, the Concerns-Based Adoption Model, the Technology Acceptance Model, and the United Theory of Acceptance and Use of Technology.
Technology adoption researches often conceptualized emotions as negative effects such as computer anxiety [46, 47, 49], fears [50] and worries [51, 52]. In contrary, positive emotions like happiness, interest, joy, contentment and enthusiasm have been largely neglected [46].
The research scope was narrowed to six perspectives, namely year of publication, theories underlining the technology adoption, level of research, dependent variables, context of the technology adoption, and independent variables. In this research, information on trends in IT adoption is provided by examining related research works to provide ...
The interest of researchers worldwide in educational technology acceptance and adoption is evident (see Fig. 2).Most of the identified studies were conducted in Taiwan (N=7), followed by relevant research carried out in South Korea and USA (N=4), Spain (N=3), Canada, China, Hong Kong, Malaysia, Pakistan, Singapore and Turkey (N=2).
2.1 Defining Technology Acceptance and Adoption. Technology acceptance is defined as the intention to use a technology or the actual use of a technology [].[] described technology acceptance as the critical factor in determining the success or failure of any technology and acceptance has been conceptualized as an outcome variable in a psychological process that users go through in making ...
Read Articles about Technology Adoption- HBS Working Knowledge: The latest business management research and ideas from HBS faculty. ... New research on technology adoption from Harvard Business School faculty on issues including the use of educational technology, effects of the increasing ubiquity of smartphones, and methods for increasing ...
Future research on adoption may examine the consequences of technology to. create a holistic understanding of how technology change influences the organiza- tion and the individual. Whereas technology adoption may be viewed in terms of. ramp-up time, or how much time is lost in the learning of technology, researchers.
As for technology adoption research at the individual level, numerous theories and models have been used to predict and explain human behavior towards technology acceptance, adoption and usage. Education presents an area of great interest in incorporating new technologies, thus technology acceptance and adoption theories and models are often ...
Previous ICT adoption and use research streams emphasized the cognitive basis for an individuals' decision about technology adoption and use. Early post-adoption research used the same theories used in adoption research. Also, the post-adoption theories and models employed similar theoretical frameworks as adoption focused theories.
Shifting our tech adoption studies to NPORS ensures we're keeping up with the latest advances in the Center's methods toolkit, with quality at the forefront of this important work. The internet hasn't just transformed Americans' everyday lives - it's also transformed the way researchers study its impact. The changes we've made ...
The research methodology employed in this study aimed to provide a comprehensive and in-depth exploration of the interplay between in ation, service quality, and technology adoption in the context ...
Mahdi Yadegari is a PhD Candidate in Information Technology (area of e-Commerce) at K.N. Toosi University of Technology, Iran. He is conducting research on innovation management, technology adoption models, blockchain and cryptocurrency.
In other words, adoption is a level of. technology publication in which a person or an organization decides to select and apply. the technology over the previous method s. Thus, technology ...
This paper pres ents a framework for technology adoption based on an interaction perspective, resulted from a literature study on technology adop tion. In studying technology ado ption, it is ...
As AI Spreads, Experts Predict the Best and Worst Changes in Digital Life by 2035. As they watch the splashy emergence of generative artificial intelligence and an array of other AI applications, experts participating in a new Pew Research Center canvassing say they have deep concerns about people's and society's overall well-being.
Technologies are becoming increasingly complicated and increasingly interconnected. Cars, airplanes, medical devices, financial transactions, and electricity systems all rely on more computer software than they ever have before, making them seem both harder to understand and, in some cases, harder to control. Government and corporate surveillance of individuals and information processing ...
Abstract. Numerous theories and models exist in iInformation sSystems (IS) research to examine the variables that influence the adoption of new technologies. This study compares different technology adoption models, and then builds a model on the modified uUnified tTheory of aAcceptance and uUse of tTechnology (UTAUT2).
The main aim of this research is to understand the impact of collaborative knowledge creation and learning practices for the adoption of technology-based innovations for teaching and learning. We implement the current research in a SUP context where teachers co-create and learn together with university didactics and educational technology ...
The technology adoption lifecycle is a sociological model that describes the adoption or acceptance of a new product or innovation, according to the demographic and psychological characteristics of defined adopter groups. ... This research built on prior work by Neal C. Gross and Bryce Ryan. Rogers ...
Follow these links for more in-depth analysis of the impact of mobile technology on American life. Americans' Social Media Use Jan. 31, 2024; Americans' Use of Mobile Technology and Home Broadband Jan. 31 2024; Q&A: How and why we're changing the way we study tech adoption Jan. 31, 2024
Technology has become the lifeblood of organizations, a vital tool used regularly in essentially every function. But while 60% of business and risk leaders see one new technology tool, generative AI (GenAI), as an opportunity, 57% say that preparing for investments in new technology is the single biggest trigger to review the risk landscape, according to the PwC 2023 Global Risk Survey.
This process is commonly known as the Technology Adoption Decision Process and consists of five stages. In knowledge stage, individuals become aware of the innovation and understand its features ...
Technology adoption occurs when an individual, firm or other agent first makes use of a new technology. In this setting, technology can refer to a new product, service or management innovation. There is a vast economics literature studying the use of newly available technologies.
The growing adoption of continuous quality improvement (CQI) initiatives in healthcare has generated a surge in research interest to gain a deeper understanding of CQI. However, comprehensive evidence regarding the diverse facets of CQI in healthcare has been limited. Our review sought to comprehensively grasp the conceptualization and principles of CQI, explore existing models and tools ...
A brand new research paper highlights the fact that big corporations in South Africa are adopting Generate Artificial Intelligence (GenAI) at a continually increasing rate. This is a massive and ...
Millennials have often led older Americans in their adoption and use of technology. But there has been significant growth in tech adoption among older generations. ... This is an update of a post originally published May 2, 2018, and written by Jingjing Jiang, a former research analyst focusing on internet and technology. See full topline ...
Technology adoption among indigenous tourism stakeholders is nascent and has not gained momentum due to inherent and exogenous constraints. As the role of information technology (IT) for development is well evidenced in the tourism sector, encouraging its adoption among all stakeholders is mandated. ... He has 16 years of teaching and research ...
With ISO 20022 adoption lagging amid competing global deadlines, a successful migration may hinge on changing from a tactical to a strategic mindset. ... Our skilled teams, operational efficiencies enabled by innovative technology and flexible global delivery service centers can help you manage financial crime risk in a cost-effective ...
Well-defined and stable property rights play a pivotal role in shaping human economic behavior by averting the tragedy of the commons. This study employs micro-survey data from Heilongjiang Province, China, to empirically investigate the impact and mechanisms of land approval on the adoption of straw returning tstraw-returning technology by farmers. Utilizing the Probit model and mediation and ...