- Browse All Articles
- Newsletter Sign-Up
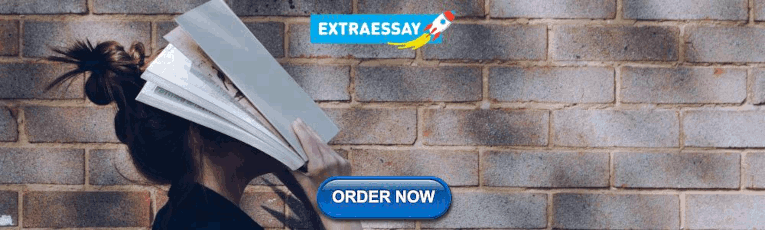
HumanResources →
No results found in working knowledge.
- Were any results found in one of the other content buckets on the left?
- Try removing some search filters.
- Use different search filters.
Academia.edu no longer supports Internet Explorer.
To browse Academia.edu and the wider internet faster and more securely, please take a few seconds to upgrade your browser .
Enter the email address you signed up with and we'll email you a reset link.
- We're Hiring!
- Help Center
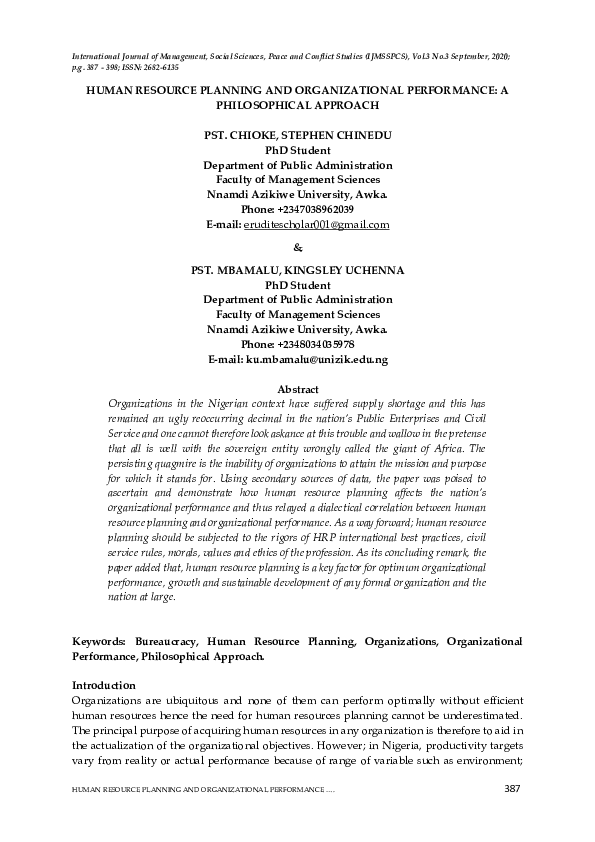
HUMAN RESOURCE PLANNING AND ORGANIZATIONAL PERFORMANCE: A PHILOSOPHICAL APPROACH

Related Papers
International Journal of Research and Innovation in Social Science (IJRISS)
Ndomokidem M Sokari
The study was to investigate the influence of Human resource planning on organizational performance. The objective of this research is to determine the influence of human resource planning on organizational performance in the telecom company in Port Harcourt. The study used a well structured questionnaire to obtain data from 160 top managers and middle level managers. Statistical package for social sciences (SPSS) version 23.0 was utilized for data analysis and regression analysis for the hypotheses. Findings from the study disclosed. HRP has a significant influence on organizational performance (R=0.563, R 2 = 0.318, P=0.000). Furthermore, finding suggests that an effective human resource planning such as anticipating manpower factors of job satisfaction, understanding peoples need, utilization, motivation, compensation and training and development packages have the capacity to assist the organization in modifying the behavior, increase creativity and increase its workers level of innovativeness and commitment towards improving organizational performance. It was recommended that Companies should spend more on Human Resources; which can lead towards High performance achievement.
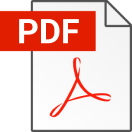
QUEST JOURNALS
This research is on "the impact of human resource planning on organizational goals attainment." The study aims is to determine the extent at which effective human resource planning can impact positively on the organizational goals attainment and also in order to minimized poor performance or productivity in organization to determine if human resource planning have any significant impact on organizational performance, and the relationship between human resource planning and employees performance in the organization. Fifty (50) research questions, two (2) hypotheses and two objectives of the study were formulated in relation with the objectives of the studies. To achieve the aims and objectives of the studies, data were collected through both the primary and secondary sources of data collection. Data collected were analyzed by the used of chi-square method of data analysis. The two hypotheses were tested. Based on the analysis, the study found out that human resource planning is very essential or crucial in any organizations whether small or large scale business enterprise since it is well known that no any business organization can survive or exist entirely or completely without human being, also that one of the major functions of human resource planning is the process of engaging right people with ability, skills and technical knowhow to work in the organization in order to achieve profitability and sales growth. Based on the findings of the study, the researcher recommend that organization should always plan for human resource need of the organization before recruitment, selection or placement in order to know the exact quantity, and quality of human resource need of the organization.
Ohuche Chimezie
ABSTRACT Human Resource Management (HRM) is the term used to describe formal systems devised for the management of people within an organization. Essentially the purpose of HRM is to maximize the productivity of an organization by optimizing the effectiveness of employees. The effectiveness of Human Resources management in Nigeria is hampered by a myriad of challenges ranging from; Labour and Social Legislation, Neglect of Staff welfare programme and Quality of work life (QWL),Lack of skills by HR practitioners or professionals, Casualization of Labour and Outsourcing of services, Lack /Obsolete HR policies, Lack of Business understanding, to HR practitioners/professional as career counselor and health and safety custodian to Quota System in Employment, Values of HR practitioners/professional, Line managers as partners in business, Lack of management support, Pulse sensing and employee priorities, Organizational changes, Labour Dissatisfaction at Work, Changing Values of the Employees, generational combination, Changing Demands of the Employers and lastly Rapid advances in HR technology. Several recommendation are developed that could be a solution to the various challenges facing the effectiveness of Human Resources Management in Nigeria. Organizations, even in the face of globalization should have the right attitude towards HR activities and provide adequate support (financial and otherwise) in ensuring that human resources management becomes effective. HRM would also be more effective if HR professionals receive more attention in form of grants and aids to carry out meaningful research related to all areas of human performance for tomorrow’s organizations.
Nigeria Journal of Management Sciences (NJMS)
Ndubuisi Uchechukwu , elizabeth otusile
Tensure sustainable productivity through human resource management which will ensure optimum service delivery and national development. Questionnaire designed in 5 point Likert scale was used to source the data. The result of data analysis shows that human resource management has significant effect on public sector productivity and that human resource management also has significant effect on employee productivity. It was recommended that the government should search and engage the best people suited for the work, develop and sustain them, not considering the ever present constraining factors. Keywords:Human resource, public sector, organization, productivity
Stephen Dugguh
Bukola Babayeju
This study focused on the impact of human resource management practices in Nigeria organisations using the manufacturing industry as a case study. This study tries to understand the need for HRM practices and specifically questions how HRM practices, such as the importance of HR department, objectives, recruitment, Promotion, Transfer and Staff Separation, performance appraisal and compensation, enhance organisational performance. A multiple-respondent survey of 120 staff of the selected manufacturing companies in Nigeria was undertaken to study the impact of HRM practices on organization performance. The data was collected using structured questionnaire and was analysed using simple percentages and hypotheses tested using chi-square (X 2) analysis. The survey found that a well coordinated HRM
Journal for Studies in Management and Planning
Stephen Pembi
This study examines the Role of Human Resource Planning in Repositioning Nigerian Economic Growth and Development. The paper was carried along two research objectives were to specifically find out the factors underlying the increased interest in the need for human resource planning and as well as to highlights the relevance of human resource planning toward repositioning economic growth and development in Nigeria. Secondary sources of data were used for this study. Finding from the study reveals that; getting the right people in the right place at the right time is the basis for rapid organisational growth and development. It was also found that human resource planning is a vital tool for estimating the demand and evaluating the size, nature and the sources of supply of labour in Nigeria. Uncertainty arising from environmental forces, globalization, new technology, economic conditions and changing workforce were identified, and the factors behind the increased interest in the needs...
International Journal of Social Sciences and Humanities Review
AYOBAMI ELEGUNDE
Journal of Management …
tunde elegbede
Dr. Sajuyigbe Ademola
Effective utilization of human resources has been recognised as a strong factor that paves the path to achieve organizational goals. This study investigates the influence of human resource management practices on organizational performance with particular reference to Nigerian insurance industry. A structured questionnaire was used to collect the data from two hundred (200) respondents through purposive sampling technique. Descriptive Statistics and Ordinary Least Square method were used to analyse the data. Results show that human resource management practices jointly influence organizational performance at 1% level of significant. Results also indicate that human resource management practices contribute 88.3% to organizational performance. The study concludes that human resource management practices are major factors that influence organizational performance in Nigerian insurance industry. Study also confirms that employee participation has the highest influence on organizational performance followed by career planning systems and performance appraisal. Study also reveals that selection processes and compensation systems in Nigerian insurance industry are still below industry standard. Subsequently, the study recommends that the human resource department should be given free hand without undue interference to select suitable candidates and management should review salaries, commissions, and allowances upwardly on a regular basis.
RELATED PAPERS
Nephrology Dialysis Transplantation
A Figueiredo
X. Briffault
Husni Syahrudin
Zbornik Radova Pravnog Fakulteta U Splitu
Sanja Baric
Ioachim Romanovich Chabibullin
Water Research
Mette Myrmel
Deepak Doraiswamy
Verhaltenstherapie
Andreas Hillert
Frontiers in Psychology
Maryam Farooq
Optimization Methods and Software
Emre Alper Yildirim
The American Journal of Occupational Therapy
Debbie Amini
International Journal of Contemporary Pediatrics
Prof.Santosh Kumar Swain
Proceedings of the 3 Simpósio Brasileiro de Geofísica
Danusa Mayara de Souza
Nature Communications
Rick Afonso-Rocha
USA. International Institute of Acoustics and Vibration ( IIAV ). Curran Associates, Inc.
Peter Brancevich
Journal of Animal Science
Ranee Miller
shamsuddeen sani
Polar Biology
Paul Wassmann
Bulletin Français de la Pêche et de la Pisciculture
Michel Louis Jégu
Hippocampus
Asta Håberg
Wireless Personal Communications
raziyeh okhovat
Journal of Biological Chemistry
Barry Oemar
Journal of investigational allergology & clinical immunology
valentina panetta
- We're Hiring!
- Help Center
- Find new research papers in:
- Health Sciences
- Earth Sciences
- Cognitive Science
- Mathematics
- Computer Science
- Academia ©2024

An official website of the United States government
The .gov means it’s official. Federal government websites often end in .gov or .mil. Before sharing sensitive information, make sure you’re on a federal government site.
The site is secure. The https:// ensures that you are connecting to the official website and that any information you provide is encrypted and transmitted securely.
- Publications
- Account settings
Preview improvements coming to the PMC website in October 2024. Learn More or Try it out now .
- Advanced Search
- Journal List
- Comput Intell Neurosci
- v.2022; 2022

Human Resource Planning and Configuration Based on Machine Learning
1 School of Management Engineering and Business, Hebei University of Engineering, Handan 056000, China
2 School of Artificial Intelligence, Beijing University of Posts and Telecommunications, Beijing 100000, China
Enliang Dai
3 School of Labor and Human Resources, Renmin University of China, Beijing 100000, China
Yongfeng Liang
4 Beijing Miss Fresh E-Commerce Co. Ltd, Beijing 100000, China
Associated Data
The dataset used to support the findings of this study is available from the corresponding author upon request.
Human resources are the core resources of an enterprise, and the demand forecasting plays a vital role in the allocation and optimization of human resources. Starting from the basic concepts of human resource forecasting, this paper employs the backpropagation neural network (BPNN) and radial basis function neural network (RBFNN) to analyze human resource needs and determine the key elements of the company's human resource allocation through predictive models. With historical data as reference, the forecast value of current human resource demand is obtained through the two types of neural networks. Based on the prediction results, the company managers can carry out targeted human resource planning and allocation to improve the efficiency of enterprise operations. In the experiment, the actual human resource data of a certain company are used as the experimental basic samples to train and test the two types of machine learning tools. The experimental results show that the method proposed in this paper can effectively predict the number of personnel required and can support the planning and allocation of human resources.
1. Introduction
Human resources are valuable corporate resources and are of great significance to their predictive analysis. Many experts and scholars all over the world have conducted relevant research studies [ 1 – 4 ]. Human resource demand forecasting generally needs to follow the principle of correlation and the principle of inertia. The principle of correlation is based on the correlation between the research objects and uses other objects to predict the targeted object [ 5 – 12 ]. For example, there is a clear correlation between A, B, and C. In the research process, rich data of A, B, and C can be obtained. At the same time, the trend value of B and C can be predicted by suitable forecasting methods, and finally the correctness can be achieved by making predictions based on A. The principle of inertia specifically refers to the slow progress of A or its regular development, and some valid past data can be obtained. Under this premise, you can choose appropriate means to predict the trend value of A. The human resource demand forecasting model is mainly based on qualitative and quantitative analysis. Qualitative analysis methods mainly include the Delphi method, subjective judgment method, microintegration method, and so on. The above methods rely more on experts or experienced people, and they all have the disadvantage of subjective components. As a result, the prediction results are prone to be non-consensual judgments. The quantitative analysis methods mainly include the production function method, ratio method, trend method, regression analysis method, and so on. The above quantitative analysis is based on the existing data for predictive analysis. The prediction logic is rigorous and overcomes the shortcomings of qualitative methods. However, there are also problems such as low prediction accuracy and difficulty in collecting data. Therefore, the combination of quantitative and qualitative methods has naturally become the research direction of enterprise human resource forecasting [ 13 – 17 ].
Zhandong and Chi [ 18 ] used the Delphi method to study the human resource forecasting of enterprises and explained the importance of human resource forecasting in enterprise management. Belhaj et al. [ 1 ] used the Markov model to predict the human resources of the enterprise and obtained the demand for the human resources of the enterprise in the future [ 19 ]. Wu and Nagahashi [ 20 ] used the grey forecasting model to carry out human resource forecasting analysis for enterprises, which provided a reference for its human resource planning. Qu et al. [ 21 ] predicted the human resources of enterprises based on backpropagation neural network (BPNN) and believed that network can obtain better prediction results. There are many factors affecting human resource demand and non-linear correlation. At the same time, traditional analysis and forecasting methods such as the analytic hierarchy process, multiple linear regression method, and Delphi method have shortcomings and shortcomings such as low prediction accuracy and biased subjective analysis. Therefore, common predictive analysis methods cannot truly reflect the nature of the problem. As a remedy, researchers began to study prediction methods based on machine learning models, which have higher superiority compared with traditional prediction methods [ 21 – 25 ]. Common modern machine learning methods include BPNN, K-nearest neighbors (KNN), support vector machine (SVM), and so on. In addition, the machine learning methods based on tree models were also widely used for data prediction, such as basic decision tree models and related integrated models such as random forests. Therefore, the research on human resource forecasting using machine learning plays an important role in improving forecasting accuracy [ 26 – 30 ].
This paper studies the method of personnel resource planning and allocation based on machine learning. The basic idea of this method is to use machine learning algorithms to predict enterprise human resource needs and dynamically adjust staffing accordingly. Specifically, this paper uses two machine learning models, BPNN and radial basis function neural network (RBFNN). Both types of models are based on neural networks, and robust model parameters are obtained by optimizing training algorithms. For the company's human resource needs, the historical data are used as training samples to train the two models to obtain a robust prediction model. Finally, the corresponding predicted value of human resource demand can be obtained under current conditions, which can be used as a reference for enterprise management personnel in personnel planning and configuration. The experiment uses human resource data of a certain company as the samples. Also, the validity of the proposed method can be verified according to the experimental results.
2. Machine Learning Models
BPNN is a kind of multilayer feedforward neural network with signal forward transmission and error backward propagation [ 11 – 15 ]. It is widely used in the fields of function approximation and pattern recognition. The topological structure of BPNN includes input layer, hidden layer, and output layer, and each layer is composed of neuron connections. In the forward calculation process of the BPNN, information is input by the input layer and processed and calculated by the hidden layers, and the output layer outputs the processing results. In the backward propagation of the error, the error of the processing result of the output layer is calculated. The error signal is propagated back, and the weight of the connection between each neuron is corrected by the method of gradient descent, so as to realize the network optimization.
The training of BPNN includes two processes: forward propagation of signal and backward feedback of error. The forward propagation means that in the calculation, the signal enters the hidden layer after parallel weighting calculation from the input layer and then enters the output layer through weighting processing to obtain the output. The direction of the reverse feedback process is carried out from output to input. The weights and thresholds are adjusted according to the actual error. Through continuous repetition of forward calculation and reverse feedback, the output result can finally meet the requirements. A basic model of BPNN is shown in Figure 1 . In this figure, ( x 1 , x 2 ,…, x j ,…, x m ) is the input quantity, and the subscript is the input quantity number, which corresponds to the input layer node; ( y 1 , y 2 ,…, y k ,…, y t ) is the output quantity, and the subscript is the output quantity number, which corresponds to the output layer node; ( θ 1 , θ 2 ,…, θ i ,…, θ q ) are the thresholds introduced for the hidden layer, and the subscript is the hidden node number; there may be multiple hidden layers in the neural network; ( a 1 , a 2 ,…, a k ,…, a t ) are the threshold values introduced for the output layer, and the subscript is the output node number. There is only one output layer in the neural network; ε denotes the error value.

Basic structure of BPNN.
In the process of forward propagation, the input n i and output o i of the i th node in the hidden layer can be calculated as follows:
In the process of forward propagation, the input net k and output of the k th in the output layer are calculated as follows:
where w ij is the weight of the j th input variable at the i th node of the hidden layer; ϕ is the activation function of the hidden layer; and ψ is the activation function of the output layer. In order to enhance the large-scale non-linear fitting ability, according to actual use experience, the hidden layer activation function selects the bipolar S-shaped tansig function, and the output layer activation function selects the linear purelin function.
The error function E P of the P th sample is shown in equation ( 3 ), where T k is the expected output value of the k th node.
The total error E of P training samples is
In the feedback process, the weight correction amount and threshold correction amount of the hidden layer and the output layer can be written as
where η is the coefficient determining adjustment rate.
The specific steps of BPNN are as follows:
- Step 1: Network initialization: the necessary network parameters are determined. Generally, there are the number of input layer nodes, the number of hidden layer nodes, the number of output layer nodes, connection weights and thresholds, transfer function types, and so on.
- Step 2: According to the parameters determined in Step 1, the hidden layer output calculation is carried out.
- Step 3: Same as above, the input calculation of the output layer is carried out.
- Step 4: The network error is calculated as follows: the expected output-network predicted output.
- Step 5: The connection weight and threshold are updated according to the network error and network learning rate.
- Step 6: The judgment is made on whether the algorithm is terminated. If it is not over, return to Step 2 to continue network training.
It should be noted that there are many kinds of transfer functions, and the threshold transfer function (Hardlim) is generally used. The second is that the network data need normalized data during the training process, which requires that the data are normalized to (0, 1). Also, the data are restored when the data are output. Third, in general, the number of hidden layer nodes needs to be determined manually. Set the number of input layer nodes to be n , the number of hidden layer nodes to be l , and the number of output layer nodes to be m , and they satisfy the following relationship:
Furthermore, because the BPNN generally needs to iteratively determine the connection weights, a relatively large time delay will be generated for a large amount of data processing [ 16 – 20 ]. The RBFNN obtains a value by calculating the norm of the input sample and the hidden layer point (center point) and substituting it into the radial basis function (Gaussian function, quadratic function, inverse quadratic function, and so on). After the weights are multiplied and added, the corresponding output is obtained. The network is simple, and the learning convergence speed is faster, which can make up for the deficiencies of BPNN.
RBFNN is a three-layer forward network, and its network structure is shown in Figure 2 . Among them, W 1 and b 1 are the connection weight matrix and bias vector from the input layer to the hidden layer. W 2 and b 2 are the connection weight matrix and the connection weight matrix from the hidden layer to the output layer. The input layer is composed of signal nodes, and the number of neurons is the dimension of the input sample. The activation function of the hidden layer neurons is a RBF that is radially symmetric and attenuated to the center point and is commonly used as Gauss function, reflected sigmoidal function, inverse multiquadric function, etc. The output layer responds to the input pattern, and the number of neurons is equal to the dimension of the output sample. The transformation from the input space to the hidden layer space is non-linear, and the transformation from the hidden layer space to the output layer space is linear, so the mapping of RBFNN from input to output is also non-linear.

Basic structure of RBFNN.
The basic idea of RBFNN is to use RBF as the activation function of the hidden layer neurons to form the hidden layer space, so that the input vector can be directly mapped to the hidden layer space. Also, the mapping from the hidden layer space to the output layer space is linear, that is, the network output of RBFNN is the linear weighted sum of the output of hidden layer neurons. The connection weight from the hidden layer to the output layer is an adjustable parameter of RBFNN. It can be seen that although the network input to output mapping is non-linear, the network output is linear for the adjustable parameters, so the adjustable parameters of the network can be solved directly by linear equations, which greatly accelerates the learning speed and avoids the local minima problems. RBFNN is a function approximation network that non-linearly maps the input space to the output space. The weight vector of the array beam design is a non-linear function of the position of the array element, so the use of RBFNN can realize the mapping from the position of the array element to the weight vector of the array.
According to the above discussion, the overall expression of RBFNN is
where ω is the connection weight, which can be iteratively obtained by the least squares method, and the calculation formula is
The specific learning algorithm steps are as follows:
- Step 1: The K-means clustering method is used to solve the center of the radial basis function; generally, after the network initialization, the input data are calculated according to the nearest neighbor rule, and grouping and re-adjusting the clustering center are completed in 3 steps.
- Step 2: The variance of RBF of the RBFNN is calculated.
- Step 3: The least squares algorithm is employed to calculate the weight between the hidden layer and the output layer.
3. Experiment and Analysis
3.1. dataset and comparison method.
Taking a company's personnel data as an example, the original data (2009–2020) are divided into two parts. The first 10-year data are the basic data, and the 11th year data are the verification data, and the model is trained and solved. When judging the pros and cons of different models' predictive performance, some quantitative index systems are needed. According to the existing literature, this paper selects three indicators: mean squared error (MSE), mean absolute percentage error (MAPE), and symmetric mean absolute percentage error (SMAPE), as the evaluation indexes for the predictive performance. The specific calculation formulas of the above three error indicators are as follows:
4. Results and Analysis
In addition to the two models used in this paper, two traditional methods from the literature [ 12 , 18 ] are also selected for comparative analysis in the experiment. Taking selected experimental data as samples, based on the three indicators of MSE, MAPE, and SMAPE to test different methods, the statistics of the results of different methods are shown in Table 1 . It can be seen that the overall performance of the BPNN and RBFNN selected in this paper is better than that of the two traditional methods, reflecting the performance advantages of the machine learning models. Comparing BPNN and RBFNN, the latter has more advantages in overall performance because of the consideration of non-linear factors.
Comparison of different methods in the prediction of human resources.
In the actual process, due to the influence of external factors such as market changes and changes in the international situation, the prediction model may have certain deviations. For this reason, this paper applies a certain degree of noise conditions to the experimental data to reflect the influence of external influences on the allocation of human resources. On this basis, the performance trend of various methods is tested using MSE as the basic evaluation index, and the results are shown in Figure 3 . It can be seen from the figure that the performance of various methods is degraded to a certain extent due to the influence of noise. As the signal-to-noise ratio (SNR) decreases, the MSE keeps increasing. In comparison, the two models used in this paper can maintain the best prediction performance under different noise interference conditions, which further enhances its performance advantages. Comparing BPNN and RBFNN, the latter has better overall noise robustness due to the consideration of the possible non-linear effects of noise.

Performance measured by MSE achieved by different methods under noises.
5. Conclusion
The sustainable demand forecast of human resources is the prerequisite and basis for the correct deployment of personnel planning. This paper proposes a human resource prediction method based on machine learning to address the above problems. Two types of neural networks, BPNN and RBFNN, are used to predict the human resource needs of enterprises. We train the two types of models based on the historical data of human resources to obtain predictive models. According to the current enterprise situation, the current enterprise human resource forecast value can be obtained. In the experiment, the two models are tested and verified using human resource data of a certain enterprise. The experimental results show that the proposed method is effective for enterprise personnel resource forecasting and can support enterprise managers to carry out scientific personnel planning and allocation.
Data Availability
Conflicts of interest.
The authors declare that they have no conflicts of interest.
Get full control of all your budgets in one place
Resource Planning
See where your agency stands this quarter, and in the next few
Get access to over 50 prebuilt agency reports or build and customize on your own
Project Management
Set up, streamline, and deliver projects
Time Tracking
With each tracked hour, get more precise data so you can run a more profitable agency
Shape your agency's sustainable growth
Eliminate hours spent on invoicing
Create documents and collaborate with your teammates
Software Development
Marketing Agency
Design Studio
In-house Team
Success Stories
Read how agencies transform using Productive
Agency management, product updates & how tos
End-to-end Agency Management
Future proof your business with an agency management system
Agency Resource Management
Gain more control over your agency’s resources
Learn how to run a more successful agency in Productive
Billable Hours Calculator
Find out your billable hours, team utilization rate, and ideal billable hourly rate
Get advice and support from the team
The Bold Community
Join the community for agency professionals by Productive
Book a Demo
Try Productive
Comparisons
{{minutes}} min read
Human Resource Planning: Definition & Top Strategies
Lucija Bakić
April 4, 2024
Human resource planning is a key strategy for ensuring long-term business sustainability and resilience.
In this guide, we’ll explore the essentials of human resource planning (HRP), why it’s so important, and the best practices to start your human resource planning process. To learn more about the broader process of resource management, read our guide to business resource planning . Key Takeaways
- HRP is a process that ensures that companies have enough capacity to meet customer demands and business goals.
- The main steps of the process include analyzing current availability, forecasting future demand, identifying capacity gaps, and developing and monitoring HRP strategies.
- Some of the main challenges include ensuring the accuracy of your forecasting with reliable data, maintaining the balance between billable work and capacity building initiatives, and promoting collaboration and transparency.
- The right capacity planning solution can help you address the above with automation features, real-time data, and predictive analytics.
What Is Human Resource Planning (HRP)?
Human resource planning (HRP) is a process used to ensure that businesses have employees with the right skills, at the right time, and with the appropriate capacity to meet strategic goals. Some practical examples of HRP workflows for various businesses include:
- An e-commerce business forecasting the need for IT capacity increases according to seasonal trends and scaling their infrastructure and support team.
- A design agency identifying higher demand for digital media through benchmarking and developing strategies to upskill and reskill its employee pool.
- A law firm initiating a succession planning strategy for the impending employee retirements by developing internal leadership candidates and recruiting external talent.
Why Is HRP Important? Top 4 Benefits
According to research by the Work Institute, 78% of the reasons for voluntary turnover could have been prevented by the employer if identified and addressed on time. Human resource planning helps businesses increase employee engagement and drive various improvements by:
1. Maintaining a Qualified Workforce
HRP aligns talent capabilities with organizational goals through talent acquisition, training, and development initiatives. Ensuring you have a skilled workforce to meet future workforce requirements reduces the risk of inefficient workflows and supports daily business operations. Investing in employee talent and skills can also help increase employee engagement and satisfaction.
2. Improving Risk and Change Management
HRP is a proactive approach that focuses on identifying issues before they occur. Analyzing trends and forecasting future needs helps businesses create contingency plans for various scenarios. This can include high-impact external changes, such as technological advancements, or internal disruptions, like leadership transitions.
Optimize Your Human Resource Planning
Productive is the all-in-one software for workload balancing, scenario planning, and financial management, tailored to businesses of all shapes and sizes.
Start Free Trial
Book a demo
3. Ensuring Your Business Is Competitive
HRP keeps businesses competitive by helping them attract the right talent and ensuring that current employees are skilled and engaged in the workplace. It helps companies adapt to changes quickly and efficiently, fostering the agility and proactiveness needed to stay ahead of industry trends and competitors. See more : The Top 13 Benefits of ERP
4. Optimizing Workforce Costs
HRP optimizes business costs by providing balanced employee utilization so that your agency isn’t spending excess money on non-productive labor costs. It also ensures that your business can do more work with adequate supply. Finally, HRP reduces the chance of unexpected resource gaps through effective forecasting, minimizing the need for last-minute hiring or overtime work.
ON DEMAND WEBINAR
Utilization and forecasting in crisis times.
Learn all about leading your agency through uncertain times by tracking your utilization rate with Productive and The Agency Collective.
The Main Steps of Human Resources Planning
The key steps of HRP include:
- Workforce analysis to determine your company’s current human resource capacity.
- Demand forecasting to future resource demand based on industry trends and internal needs.
- Gap analysis includes finding potential roadblocks in your HRP process and developing strategies to address them.
- Implementation and monitoring of your human resource strategies, usually by tracking key performance indicators.
Analyzing Current Availability
Workforce analysis involves a comprehensive evaluation of the current workforce’s size, skills, and capabilities. It assesses aspects such as:
- Employee productivity
- Job satisfaction
- Skill sets, including technical and soft skills
- Turnover rates
These metrics are used to identify your organization’s strengths and weaknesses. This is the foundation on which you’ll develop actionable steps to improve your HRP processes.
The utilization rate is the key metric for visualizing productivity — use Productive for real-time insights
Projecting Future Demand
Projecting future demand involves estimating the human resource requirements needed for an organization to meet its future goals. This forecast considers factors such as business growth, market expansion, technological advancements, and changes in operational processes. Three tools that are used in this process are:
- Ratio analysis , where historical data on the relationship between business metrics and workforce size is used to predict future staffing needs.
- Trend analysis , which examines patterns in the organization’s workforce data over time.
- Comparative analysis, whether by comparing internal resource planning practices across projects or benchmarking your performance against competitors.
Another potential strategy is utilizing real-time data, such as forecasting charts that depict the impact of resource scheduling on agency analytics.
Productive’s forecasting charts let you predict your company’s revenue and profit margins
Gap Analysis
Gap analysis compares future human resource needs against the current workforce’s capabilities to identify discrepancies or gaps. A way to conduct gap analysis is to monitor where previous projects went wrong to pinpoint inefficiencies in your workflows, such as miscommunication or a deficit of specific skills. You can do this by checking estimated vs real completion times for various tasks — ERP solutions can deliver these insights with time tracking features. Then, by examining your upcoming projects or initiatives, you can identify and forecast potential areas where similar imbalances may occur.
Developing and Implementing Strategies
The final step is developing and implementing HR strategies to cover your company’s specific needs and requirements. These strategies may include:
- Creating a resource plan: A resource plan is an in-depth document that contains information on your employees, their availability, and their scheduled time. It helps businesses follow strategic objectives and monitor their ongoing processes.
Get an in-depth overview of your business resources and their availability
- Employee engagement and retention strategies: For example, drafting career development plans, introducing new benefits packages and competitive compensation, and promoting a healthy organizational mindset.
- Implementing modern software: Resource planning tools can support various steps of the HRP process, with features such as time off management, billable hours tracking, financial forecasting, real-time reporting, workflow automations, and more.
Best Practices for Effective HRP
Once you’ve pinpointed potential gaps and developed strategies to drive improvements, what are some best practices to ensure they stick?
Monitoring Your Progress
Whichever initiatives you decide to implement, monitoring them through key performance indicators (KPIs) is necessary to assess their effectiveness. However, keep in mind that while business metrics are important, some benefits of HRP may be hard to quantify. This includes better work-life balance and improved working environment.
Regular Review
HRP can take a long time to provide results. Agility and flexibility are needed to make sure that your strategies can stay aligned with changing business needs and priorities. Regular review helps identify where your strategies have gone off track to implement timely changes.
Continuous Improvement
HRP is an ongoing process. As such, your strategies will need to evolve alongside your business goals and circumstances. Incremental improvements are always better than sudden, expansive changes — consistently seeking feedback and analyzing outcomes is a way to ensure your HRP strategies remain effective over time.
Types of HR Planning
There are different types or techniques associated with HR planning. Here are some common terms and how you can differentiate them:
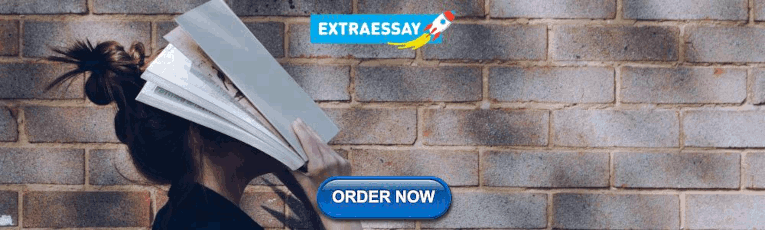
Hard vs Soft HR Planning
- Hard HR Planning focuses on quantitative aspects of human resource management, such as headcount, costs, and labor allocation. This approach often involves in-depth data and forecasting for informed decision-making.
- Soft HR Planning considers qualitative factors of workforce management, such as engagement, development, and well-being. It’s less focused on data and more on fostering a committed and resilient workforce.
Short-Term vs Strategic HRP
- Short-term HRP is more of a reactive approach that addresses immediate staffing needs and focuses on resolving urgent issues. It typically spans a timeframe of up to one year.
- Strategic HRP is a long-term approach that aligns workforce planning with the organization’s future goals and strategies. It involves forecasting workforce requirements, sustainable talent management, and other proactive strategies for business success.
Employee Reskilling vs Upskilling
- Reskilling involves training employees in new skills and capabilities to help them transition to different roles within the company.
- Upskilling focuses on enhancing the current skills and competencies to improve performance, stay competitive, and meet job requirements.
See more : Capacity Building 101
Future Trends in HR Planning
- Remote work is here to stay. According to survey results, 63% of professionals are willing to take a pay cut to work remotely (FlexJobs). If possible, consider including it as one of your benefits to drive a competitive advantage.
- In general, employee well-being initiatives are becoming more and more popular. This can include more flexible hours, hybrid or remote work, health insurance plans, as well as various fitness and wellness programs.
- 72% of professionals agree that all forms of skill-based hiring are more effective than resumes. While the resume is still used to filter the pool of applicants, work-related tasks and technical questions have proven to be the more efficient and cost-effective way of hiring candidates. (Test Gorilla).
- When it comes to daily workflows, 60% of professionals believe that automation helps them fight burnout and work-related stress. It allows for a more flexible work schedule, helps them be more organized at work, frees up their tasks for work they enjoy, and more (Zapier). Consider tools that can provide no-code automations to streamline day-to-day work.
The Challenges of Human Resource Planning
Now that we’ve gone through the main steps of the HRP, it’s time to address some of its main challenges:
- Accurate forecasting: Predicting future needs accurately can be a challenge in itself. Not only does it require having an in-depth understanding of your business circumstances, but it’s also sensitive to changes in market demand and economic conditions.
- Maintaining a flexible workforce: Maintaining a versatile and skilled workforce requires careful management of work hours. This ensures that profitability isn’t compromised, and at the same time, avoids situations where training is neglected entirely for billable work. This balance between billable and non-billable time is crucial for sustainable organizational success.
- Aligning HR Strategy with Business Goals : Keeping track of the overarching business strategy in HRP can be hard, especially in large or rapidly evolving organizations. It requires transparent communication, cross-functional collaboration, and a deep understanding of the organization’s long-term objectives and the role of the workforce in achieving them.
The Solution: Utilizing Software for Enhanced HR Planning
A way to address these potential challenges is using tools with HR and resource management capabilities . Modern software provides a way to visualize and forecast employee hours, activities, and their impact on business financials for more informed decision-making. It also helps businesses view project progress in real time to streamline stakeholder collaboration.
Adapt your project management to your working preferences with Productive
An example of such a tool is Productive , with key HRP features including:
- Time tracking
- Resource scheduling
- Workload balancing
- Time off management
- Financial forecasting
Book a demo today to discover how Productive can help drive efficient human resource management.
What is meant by human resource planning?
Human resource planning (HRP) is the strategic process of ensuring your business has the correct number of skilled employees to meet company goals. It involves talent management, employee performance and data analysis, needs forecasting, and more.
What are the 5 steps in human resource planning?
The five main steps of human resource planning include identifying current organizational availability, demand forecasting, capacity gap analysis, strategy development and implementation, and results monitoring and analysis.
What are the 3 key areas of human resources planning?
The 3 key areas of human resource planning (HRP) include workforce forecasting, talent management, and gap analysis. Workforce forecasting involves analyzing current availability and predicting the future needs of the workforce. Talent management encompasses various strategies, from recruitment, training, and development to succession planning. Gap analysis involves pinpointing areas of improvement by identifying where capacity fell short of meeting demand (skills, quantity, time, etc.).
Why is human resources planning important?
Human resource planning (HRP) is important because it ensures that the workforce is aligned with the organization’s strategic goals. It helps businesses get the most out of their human resources, both by improving acquisition strategies, developing current talent, and increasing retention. HRP also supports organization agility, flexibility, and resilience by building a well-skilled and satisfied workforce.
Connect With Agency Peers
Access agency-related Slack channels, exchange business insights, and join in on members-only live sessions.
Content Specialist
Related articles
Agency workflows 101: the best process for agencies.
Agency Management
Capacity Management: Best Practices for Agency Growth
Project resource management: the ultimate guide.
Questions not answered yet? Our sales is here to help
+1 (415) 287-3073
Integrations
Automations
Permissions and User Access
Business Consultancy
Customer Stories
Product Updates
Agency Valuation Calculator
Building Productive
Brand Guidelines
Trust Center
© 2024 The Productive Company, Inc.
Privacy Policy
Terms & Conditions
We need your consent to continue
Necessary cookies
Cookies for the basic functionality of the Productive website.
Functional cookies
Cookies for additional functionality and increased website security.
Targeting cookies
Advertising and analytics service cookies that create day-to-day statistics and show ads on their site and on the advertiser’s partners websites.
Save changes
Manage cookies and help us deliver our services. By using our services, you agree to our use of cookies.
Try Productive for free
Free 14-day trial. No credit card required. Cancel any time.
Already using Productive? Sign in with an existing account
This paper is in the following e-collection/theme issue:
Published on 11.4.2024 in Vol 26 (2024)
A Perspective on Crowdsourcing and Human-in-the-Loop Workflows in Precision Health
Authors of this article:

- Peter Washington, PhD
Information and Computer Sciences, University of Hawaii at Manoa, Honolulu, HI, United States
Corresponding Author:
Peter Washington, PhD
Information and Computer Sciences
University of Hawaii at Manoa
1680 East-West Road
Honolulu, HI, 96822
United States
Email: [email protected]
Modern machine learning approaches have led to performant diagnostic models for a variety of health conditions. Several machine learning approaches, such as decision trees and deep neural networks, can, in principle, approximate any function. However, this power can be considered to be both a gift and a curse, as the propensity toward overfitting is magnified when the input data are heterogeneous and high dimensional and the output class is highly nonlinear. This issue can especially plague diagnostic systems that predict behavioral and psychiatric conditions that are diagnosed with subjective criteria. An emerging solution to this issue is crowdsourcing, where crowd workers are paid to annotate complex behavioral features in return for monetary compensation or a gamified experience. These labels can then be used to derive a diagnosis, either directly or by using the labels as inputs to a diagnostic machine learning model. This viewpoint describes existing work in this emerging field and discusses ongoing challenges and opportunities with crowd-powered diagnostic systems, a nascent field of study. With the correct considerations, the addition of crowdsourcing to human-in-the-loop machine learning workflows for the prediction of complex and nuanced health conditions can accelerate screening, diagnostics, and ultimately access to care.
Introduction
Crowdsourcing, a term first coined in 2006 [ 1 ], is the use of distributed human workers to accomplish a central task. Crowdsourcing exploits the “power of the crowd” to achieve goals that are only feasible with a distributed group of humans collaborating, either explicitly or implicitly, toward a common goal. Crowdsourcing has often been applied to public health surveillance [ 2 ], such as for tracking epidemics [ 3 , 4 ], quantifying tobacco use [ 5 ], monitoring water quality [ 6 ], tracking misinformation [ 7 ], and understanding the black-market price of prescription opioids [ 8 ]. In the context of health care, crowdsourcing is most often used for public health, a domain that can clearly benefit from scalable and distributed assessments of health status. Although sampling bias can be an issue in epidemiological uses of crowdsourcing [ 9 ], approaches that account for these issues have performed quite robustly.
A smaller but potentially transformative effort to apply crowdsourcing to precision health rather than population health has recently emerged. In precision health contexts, the goal is to provide a diagnosis using information labeled by crowd workers. There are several variations to this basic setup. Crowdsourcing workflows for diagnostics can diverge with respect to the underlying task, worker motivation strategies, worker training, worker filtering, and privacy requirements.
Here, I describe the existing research in the relatively small and early but growing field of crowdsourcing for precision health. I then discuss ongoing challenges and corresponding opportunities that must be addressed as this field matures.
Existing Examples of Crowdsourcing in and Adjacent to Health Care
There are relatively few examples of crowdsourcing in precision health. The vast successes of machine learning for health [ 10 - 15 ] and the human labor costs required for crowdsourcing make purely automated approaches more appealing when they are possible and feasible. However, the crowdsourcing approaches that have been tested tend to perform well for prediction tasks that are beyond the scope of current automated approaches, especially in psychiatry and the behavioral sciences.
I want to begin by highlighting successes in science, as they can often be applied to health and have started to lead to improvements in diagnostics. Framing crowdsourcing tasks as “citizen science” opportunities can be an effective incentive mechanism [ 16 ]. Oftentimes, these projects are “gamified.” Gamification refers to the incorporation of engaging elements into traditionally burdensome workflows, and in particular game-like affordances, to foster increased participation. A combination of large crowd sizes, worker training procedures, and easy identification tasks have led to previous success in the existing gamified citizen science experiments applied to precision health. For example, in a study involving nearly 100,000 crowd workers who scored images on a citizen science platform, cancer was correctly identified with an area under the receive operating characteristic of around 95% [ 17 ]. In the BioGames app, users who performed with greater than 99% accuracy in a training tutorial were invited to diagnose malaria [ 18 , 19 ]. It was discovered that with a large crowd size, the aggregated diagnostic accuracy of nonexpert crowd workers approached that of experts [ 20 ]. Another citizen science malaria diagnosis application, MalariaSpot, resulted in 99% accuracy in the diagnosis of malaria from blood films [ 21 ]. If the annotation task is relatively simple and nonexperts can be trained with minimal onboarding efforts, then citizen science can be an effective and affordable approach.
“Gamified” crowdsourcing for citizen science has also been successful without explicitly requiring workers to undergo a formal training process. Foldit [ 22 - 25 ] and EteRNA [ 26 - 31 ] are 2 games where players with no biology or chemistry background can explore the design space of protein and RNA folding, respectively. These are both NP-hard (ie, computationally complex) problems, and human players in aggregate have designed solutions that outcompete state-of-the-art computational approaches. These solutions have been used to solve health challenges, such as finding a gene signature for active tuberculosis, which can potentially be used in tuberculosis diagnostics [ 32 ]. Other gamified experiences have been used to build training libraries for complex classification tasks in precision psychiatry. Notably, GuessWhat is a mobile charades game played between children with autism and their parents [ 33 , 34 ]. While the game provides therapeutic benefits to the child with autism [ 35 ], the game simultaneously curates automatic labels of behaviors related to autism through the metadata associated with gameplay [ 36 , 37 ]. These automatically annotated video data have been used to develop state-of-the-art computer vision models for behaviors related to the diagnosis of autism, such as facial expression evocation [ 38 - 41 ], eye gaze [ 42 ], atypical prosody [ 43 ], and atypical body movements [ 44 , 45 ].
An alternative incentive mechanism is paid crowdsourcing. The most popular paid crowdsourcing platform, by far, is Amazon Mechanical Turk (MTurk) [ 46 ]. While paid crowdsourcing specifically for precision health is a relatively nascent field, the general study of paid crowdsourcing (particularly on MTurk) is quite mature. Studies have explored worker quality management [ 47 ], understanding crowd worker demographics [ 48 ], the generation of annotations for use in the training of machine learning models [ 49 - 53 ], the rights of crowd workers [ 54 - 56 ], and understanding crowd worker communities and economics [ 57 - 59 ]. Preliminary studies of paid crowdsourcing have yielded mixed success. Around 81% of images were correctly classified on MTurk in a study involving the grading of diabetic retinopathy from images, with workers failing to correctly indicate the level of severity [ 60 ]. In a separate binary labeling task for glaucomatous optic neuropathy, workers achieved sensitivity in the 80s but reached a specificity below 50% [ 61 ].
In a broader classification task of various medical conditions, workers consistently labeled the “easy” cases while struggling to correctly label and even refusing to label more complicated and nuanced tasks [ 62 ]. Clearly, there is a need for extensive innovations to the traditional paid crowdsourcing workflow to translate this methodology to precision health.
I have extensively investigated the utility of paid crowdsourcing for the diagnosis of autism from unstructured home videos, achieving relatively high diagnostic performance [ 63 - 66 ]. In these experiments, untrained annotators watched short videos depicting children with and without autism and answered questions about the behaviors depicted within the videos. These annotations were provided as input into previously developed machine learning models, achieving binary test performance in the 90s across performance metrics due to the reduction of the complex feature space (unstructured videos) into a low-dimensional representation (vectors of a few categorical ordinal values). This pipeline combining crowdsourcing and machine learning can possibly be extended to other diagnostic domains in psychiatry where the input feature space is complex, heterogeneous, and subjective.
Ongoing Challenges and Corresponding Opportunities
Since crowdsourcing for precision health care is an emerging field of study, numerous challenges must be solved for clinical translation to develop. In the proceeding sections, I highlight several areas that are pressing for the field and for which preliminary work has been published.
Worker Identification and Training
While traditional crowdsourcing can work with minimal to no worker training, complex annotation tasks require the identification of qualified workers. I have found that worker identification can occur through the quantification of their performance on test tasks [ 67 , 68 ] and training promising workers [ 66 ]. Such crowd filtration paradigms will require domain-specific procedures. There is ample room to develop new crowdsourcing systems that inherently support natural worker identification and training procedures for crowdsourcing workflows that require well-designed training processes.
Worker Retention
Once proficient workers are identified, continually engaging and retaining these workers is critical. I have found that workers who are repeatedly encouraged by a human (or human-like chatbot) and treated as members of a broader research team tend to enjoy paid work and even ask for more tasks after the completion of the study [ 69 ]. Thus, it is possible that the guarantee of job security can lead to long-term worker retention. However, worker retention in unpaid settings that rely on intrinsic motivation will require additional innovations. For example, there exists an opportunity to explore the creation of crowd worker communities to provide a means of intrinsic motivation leading to worker retention.
Task Assignment
Certain workers perform exceptionally well on a subset of tasks while underperforming on other assigned tasks [ 70 , 71 ]. There is an opportunity to develop algorithmic innovations involving the effective and optimal assignment of workers to subtasks in a dynamic manner. Reinforcement learning could be a promising approach but has yet to be explored in such scenarios.
Privacy of Human Participants
Data in psychiatry and behavioral sciences are particularly sensitive. Ensuring that sensitive health information is handled appropriately and that workers’ privacy is maintained is essential from an ethical perspective. There are 2 general families of approaches to achieving privacy in crowd-powered health care. First, the data can be modified to obscure sensitive information without removing information required for a diagnosis. I have explored privacy-preserving alterations to video data that obfuscate the identity of participants while maximizing the capacity for workers to annotate behaviors of interest [ 70 , 71 ]. For example, in the case of video analytics on bodily movements, the face can be tracked and blurred, or the body can be converted to a stick figure using a pose-based computer vision library. Sometimes, however, it is impossible to modify the data without severely degrading the diagnostic performance. Therefore, the second family of approaches involves carefully vetting crowd workers, training them, and onboarding them into a secure computing environment. In my previous experiences with this process [ 40 ], I discovered that crowd workers were enthusiastic about the prospect of the “job security” that is implied from the thorough vetting procedure and were, therefore, willing to complete extra privacy and security training (in our case, Research, Ethics, Compliance, and Safety training). There is ample room to expand upon these methods and to develop new paradigms and systems for crowdsourcing involving identifiable and protected health information.
Ensuring Reliability and Reproducibility
An intrinsic challenge when incorporating human workers into precision health workflows is the variability in human responses, both within workers and between workers. I have found that while most crowd workers are inconsistent in their annotation patterns, there are workers who provide consistently sensitive and specific annotations across a wide spectrum of data points [ 67 ]. It is therefore critical to measure both internal consistency and consistency against a gold standard when recruiting crowd workers for precision health care workflows.
Handling Financial Constraints
The crowdsourcing method with the lowest setup barriers is paid crowdsourcing. In such scenarios, financial constraints can limit the scalability of crowdsourcing workflows. One approach is to migrate from a paid system to a gamified system or another means of providing intrinsic motivation to crowd workers. However, achieving critical mass for large-scale pipelines is likely unattainable for such unpaid solutions. Paid crowd workers who consistently perform well could be recruited as full-time or long-term part-time employees for companies and organizations providing crowd-powered services. Integrating such workflows into a Food and Drug Administration (FDA)–approved process can be challenging, but it is worth exploring if it turns out that crowd-powered solutions for digital psychiatry continue to remain superior to pure-artificial intelligence (AI) approaches in the coming years.
Translation Outside of Research Contexts
While pure machine learning approaches for precision health are beginning to translate to clinical settings through formal FDA approval procedures, the prospect of translating human-in-the-loop methods that integrate crowd workers rather than expert clinicians is daunting, especially in light of the challenges mentioned above. However, if such approaches lead to clinical-grade performance for certain conditions that are challenging to diagnose using machine learning alone, then the extra implementation and regulatory effort required to migrate these methods into production-level workflows are likely to be warranted.
While machine learning for health has enabled and will continue to enable more efficient, precise, and scalable diagnostics for a variety of conditions, such models are unlikely to generalize to more difficult scenarios such as psychiatry and the behavioral sciences, which require the ability to identify complex and nuanced social human behavior. Crowd-powered human-in-the-loop workflows have the potential to mitigate some of these current limitations while still offering a high degree of automation. I invite researchers in the fields of digital phenotyping [ 72 - 76 ], mobile sensing [ 77 - 83 ], affective computing [ 84 - 90 ], and related subjects to consider integrating crowdsourcing and human-in-the-loop approaches into their methods when pure-AI leads to suboptimal performance.
Acknowledgments
This project is funded by the NIH Director’s New Innovator Award (DP2) from the National Institutes of Health (award DP2-EB035858).
Conflicts of Interest
None declared.
- Howe J. The rise of crowdsourcing. Wired. Jun 01, 2006. URL: https://sistemas-humano-computacionais.wdfiles.com/local--files/capitulo%3Aredes-sociais/Howe_The_Rise_of_Crowdsourcing.pdf [accessed 2024-03-29]
- Brabham DC, Ribisl KM, Kirchner TR, Bernhardt JM. Crowdsourcing applications for public health. Am J Prev Med. 2014;46(2):179-187. [ CrossRef ] [ Medline ]
- Leung GM, Leung K. Crowdsourcing data to mitigate epidemics. Lancet Digit Health. 2020;2(4):e156-e157. [ FREE Full text ] [ CrossRef ] [ Medline ]
- Stockham N, Washington P, Chrisman B, Paskov K, Jung JY, Wall DP. Causal modeling to mitigate selection bias and unmeasured confounding in internet-based epidemiology of COVID-19: model development and validation. JMIR Public Health Surveill. 2022;8(7):e31306. [ FREE Full text ] [ CrossRef ] [ Medline ]
- Kraemer JD, Strasser AA, Lindblom EN, Niaura RS, Mays D. Crowdsourced data collection for public health: a comparison with nationally representative, population tobacco use data. Prev Med. 2017;102:93-99. [ FREE Full text ] [ CrossRef ] [ Medline ]
- Jakositz S, Pillsbury L, Greenwood S, Fahnestock M, McGreavy B, Bryce J, et al. Protection through participation: crowdsourced tap water quality monitoring for enhanced public health. Water Res. 2020;169:115209. [ CrossRef ] [ Medline ]
- Ghenai A, Mejova Y. Catching Zika fever: application of crowdsourcing and machine learning for tracking health misinformation on Twitter. arXiv. Preprint posted online Jul 12, 2017. [ CrossRef ]
- Dasgupta N, Freifeld C, Brownstein JS, Menone CM, Surratt HL, Poppish L, et al. Crowdsourcing black market prices for prescription opioids. J Med Internet Res. 2013;15(8):e178. [ FREE Full text ] [ CrossRef ] [ Medline ]
- Wazny K. "Crowdsourcing" ten years in: a review. J Glob Health. 2017;7(2):020602. [ FREE Full text ] [ CrossRef ] [ Medline ]
- Chen PHC, Liu Y, Peng L. How to develop machine learning models for healthcare. Nat Mater. 2019;18(5):410-414. [ CrossRef ] [ Medline ]
- Dua S, Acharya UR, Dua P, editors. Machine Learning in Healthcare Informatics, Volume 56. Berlin, Heidelberg. Springer; 2014.
- Esteva A, Robicquet A, Ramsundar B, Kuleshov V, DePristo M, Chou K, et al. A guide to deep learning in healthcare. Nat Med. 2019;25(1):24-29. [ CrossRef ] [ Medline ]
- Ghassemi M, Naumann T, Schulam P, Beam AL, Chen IY, Ranganath R. A review of challenges and opportunities in machine learning for health. AMIA Jt Summits Transl Sci Proc. 2020;2020:191-200. [ FREE Full text ] [ Medline ]
- Shailaja K, Seetharamulu B, Jabbar MA. Machine learning in healthcare: a review. 2018. Presented at: 2018 Second International Conference on Electronics, Communication and Aerospace Technology (ICECA); March 29-31, 2018;910-914; Coimbatore, India. [ CrossRef ]
- Yu KH, Beam AL, Kohane IS. Artificial intelligence in healthcare. Nat Biomed Eng. 2018;2(10):719-731. [ CrossRef ] [ Medline ]
- Das R, Keep B, Washington P, Riedel-Kruse IH. Scientific discovery games for biomedical research. Annu Rev Biomed Data Sci. 2019;2(1):253-279. [ FREE Full text ] [ CrossRef ] [ Medline ]
- Dos Reis FJC, Lynn S, Ali HR, Eccles D, Hanby A, Provenzano E, et al. Crowdsourcing the general public for large scale molecular pathology studies in cancer. EBioMedicine. 2015;2(7):681-689. [ FREE Full text ] [ CrossRef ] [ Medline ]
- Mavandadi S, Dimitrov S, Feng S, Yu F, Sikora U, Yaglidere O, et al. Distributed medical image analysis and diagnosis through crowd-sourced games: a malaria case study. PLoS One. 2012;7(5):e37245. [ FREE Full text ] [ CrossRef ] [ Medline ]
- Ozcan A. Educational games for malaria diagnosis. Sci Transl Med. 2014;6(233):233ed9. [ FREE Full text ] [ CrossRef ] [ Medline ]
- Feng S, Woo M, Chandramouli K, Ozcan A. A game-based platform for crowd-sourcing biomedical image diagnosis and standardized remote training and education of diagnosticians. In: Optics and Biophotonics in Low-Resource Settings, Volume 9314. 2015. Presented at: SPIE BIOS; February 7-12, 2015; San Francisco, CA. [ CrossRef ]
- Luengo-Oroz MA, Arranz A, Frean J. Crowdsourcing malaria parasite quantification: an online game for analyzing images of infected thick blood smears. J Med Internet Res. 2012;14(6):e167. [ FREE Full text ] [ CrossRef ] [ Medline ]
- Cooper S, Khatib F, Makedon I, Lu H, Barbero J, Baker D, et al. Analysis of social gameplay macros in the Foldit cookbook. 2011. Presented at: FDG '11: Proceedings of the 6th International Conference on Foundations of Digital Games; June 29, 2011 - July 1, 2011;9-14; Bordeaux, France. [ CrossRef ]
- Curtis V. Motivation to participate in an online citizen science game: a study of Foldit. Sci Commun. 2015;37(6):723-746. [ CrossRef ]
- Eiben CB, Siegel JB, Bale JB, Cooper S, Khatib F, Shen BW, et al. Increased Diels-Alderase activity through backbone remodeling guided by Foldit players. Nat Biotechnol. 2012;30(2):190-192. [ FREE Full text ] [ CrossRef ] [ Medline ]
- Kleffner R, Flatten J, Leaver-Fay A, Baker D, Siegel JB, Khatib F, et al. Foldit standalone: a video game-derived protein structure manipulation interface using Rosetta. Bioinformatics. 2017;33(17):2765-2767. [ FREE Full text ] [ CrossRef ] [ Medline ]
- Andreasson JOL, Gotrik MR, Wu MJ, Wayment-Steele HK, Kladwang W, Portela F, et al. Crowdsourced RNA design discovers diverse, reversible, efficient, self-contained molecular switches. Proc Natl Acad Sci U S A. 2022;119(18):e2112979119. [ FREE Full text ] [ CrossRef ] [ Medline ]
- Krüger A, Watkins AM, Wellington-Oguri R, Romano J, Kofman C, DeFoe A, et al. Community science designed ribosomes with beneficial phenotypes. Nat Commun. 2023;14(1):961. [ FREE Full text ] [ CrossRef ] [ Medline ]
- Lee J, Kladwang W, Lee M, Cantu D, Azizyan M, Kim H, et al. RNA design rules from a massive open laboratory. Proc Natl Acad Sci U S A. 2014;111(6):2122-2127. [ FREE Full text ] [ CrossRef ] [ Medline ]
- Shi J, Das R, Pande VS. SentRNA: improving computational RNA design by incorporating a prior of human design strategies. arXiv. Preprint posted online Mar 06, 2019. [ CrossRef ]
- Treuille A, Das R. Scientific rigor through videogames. Trends Biochem Sci. 2014;39(11):507-509. [ FREE Full text ] [ CrossRef ] [ Medline ]
- Wayment-Steele HK, Kladwang W, Strom AI, Lee J, Treuille A, Becka A, et al. RNA secondary structure packages evaluated and improved by high-throughput experiments. Nat Methods. 2022;19(10):1234-1242. [ FREE Full text ] [ CrossRef ] [ Medline ]
- Sweeney TE, Braviak L, Tato CM, Khatri P. Genome-wide expression for diagnosis of pulmonary tuberculosis: a multicohort analysis. Lancet Respir Med. 2016;4(3):213-224. [ FREE Full text ] [ CrossRef ] [ Medline ]
- Kalantarian H, Jedoui K, Washington P, Wall DP. A mobile game for automatic emotion-labeling of images. IEEE Trans Games. 2020;12(2):213-218. [ FREE Full text ] [ CrossRef ] [ Medline ]
- Kalantarian H, Washington P, Schwartz J, Daniels J, Haber N, Wall D. A gamified mobile system for crowdsourcing video for autism research. 2018. Presented at: 2018 IEEE International Conference on Healthcare Informatics (ICHI); June 4-7, 2018;350-352; New York, NY. [ CrossRef ]
- Penev Y, Dunlap K, Husic A, Hou C, Washington P, Leblanc E, et al. A mobile game platform for improving social communication in children with autism: a feasibility study. Appl Clin Inform. 2021;12(5):1030-1040. [ FREE Full text ] [ CrossRef ] [ Medline ]
- Kalantarian H, Washington P, Schwartz J, Daniels J, Haber N, Wall DP. Guess what? Towards understanding autism from structured video using facial affect. J Healthc Inform Res. 2019;3(1):43-66. [ FREE Full text ] [ CrossRef ] [ Medline ]
- Kalantarian H, Jedoui K, Washington P, Tariq Q, Dunlap K, Schwartz J, et al. Labeling images with facial emotion and the potential for pediatric healthcare. Artif Intell Med. 2019;98:77-86. [ FREE Full text ] [ CrossRef ] [ Medline ]
- Hou C, Kalantarian H, Washington P, Dunlap K, Wall DP. Leveraging video data from a digital smartphone autism therapy to train an emotion detection classifier. medRxiv. Preprint posted online Aug 01, 2021. [ CrossRef ]
- Kalantarian H, Jedoui K, Dunlap K, Schwartz J, Washington P, Husic A, et al. The performance of emotion classifiers for children with parent-reported autism: quantitative feasibility study. JMIR Ment Health. 2020;7(4):e13174. [ FREE Full text ] [ CrossRef ] [ Medline ]
- Washington P, Kalantarian H, Kent J, Husic A, Kline A, Leblanc E, et al. Improved digital therapy for developmental pediatrics using domain-specific artificial intelligence: machine learning study. JMIR Pediatr Parent. 2022;5(2):e26760. [ FREE Full text ] [ CrossRef ] [ Medline ]
- Washington P, Kalantarian H, Kent J, Husic A, Kline A, Leblanc E, et al. Training an emotion detection classifier using frames from a mobile therapeutic game for children with developmental disorders. arXiv. Preprint posted online Dec 16, 2020 . [ CrossRef ]
- Varma M, Washington P, Chrisman B, Kline A, Leblanc E, Paskov K, et al. Identification of social engagement indicators associated with autism spectrum disorder using a game-based mobile app: comparative study of gaze fixation and visual scanning methods. J Med Internet Res. 2022;24(2):e31830. [ FREE Full text ] [ CrossRef ] [ Medline ]
- Chi NA, Washington P, Kline A, Husic A, Hou C, He C, et al. Classifying autism from crowdsourced semistructured speech recordings: machine learning model comparison study. JMIR Pediatr Parent. 2022;5(2):e35406. [ FREE Full text ] [ CrossRef ] [ Medline ]
- Lakkapragada A, Kline A, Mutlu OC, Paskov K, Chrisman B, Stockham N, et al. The classification of abnormal hand movement to aid in autism detection: machine learning study. JMIR Biomed Eng. 2022;7(1):e33771. [ FREE Full text ] [ CrossRef ]
- Washington P, Kline A, Mutlu OC, Leblanc E, Hou C, Stockham N, et al. Activity recognition with moving cameras and few training examples: applications for detection of autism-related headbanging. 2021. Presented at: CHI '21: CHI Conference on Human Factors in Computing Systems; May 8-13, 2021;1-7; Yokohama, Japan. [ CrossRef ]
- Paolacci G, Chandler J, Ipeirotis PG. Running experiments on Amazon Mechanical Turk. Judgm Decis Mak. 2010;5(5):411-419. [ FREE Full text ] [ CrossRef ]
- Ipeirotis PG, Provost F, Wang J. Quality management on Amazon Mechanical Turk. 2010. Presented at: HCOMP '10: Proceedings of the ACM SIGKDD Workshop on Human Computation; July 25, 2010;64-67; Washington DC. [ CrossRef ]
- Lee YJ, Arida JA, Donovan HS. The application of crowdsourcing approaches to cancer research: a systematic review. Cancer Med. 2017;6(11):2595-2605. [ FREE Full text ] [ CrossRef ] [ Medline ]
- Ross J, Zaldivar A, Irani L, Tomlinson B. Who are the turkers? Worker demographics in Amazon Mechanical Turk. ResearchGate. 2009. URL: https://www.researchgate.net/publication/268427703_Who_are_the_Turkers_Worker_Demographics_in_Amazon_Mechanical_Turk [accessed 2024-03-22]
- Russakovsky O, Deng J, Su H, Krause J, Satheesh S, Ma S, et al. ImageNet large scale visual recognition challenge. Int J Comput Vis. 2015;115:211-252. [ CrossRef ]
- Sheng VS, Zhang J. Machine learning with crowdsourcing: a brief summary of the past research and future directions. Proc AAAI Conf Artif Intell. 2019;33(01):9837-9843. [ CrossRef ]
- Sorokin A, Forsyth D. Utility data annotation with Amazon Mechanical Turk. 2008. Presented at: 2008 IEEE Computer Society Conference on Computer Vision and Pattern Recognition Workshops; June 23-28, 2008;1-8; Anchorage, AK. [ CrossRef ]
- Xintong G, Hongzhi W, Song Y, Hong G. Brief survey of crowdsourcing for data mining. Expert Syst Appl. 2014;41(17):7987-7994. [ CrossRef ]
- Irani LC, Silberman MS. Turkopticon: interrupting worker invisibility in Amazon Mechanical Turk. 2013. Presented at: CHI '13: Proceedings of the SIGCHI Conference on Human Factors in Computing Systems; April 27, 2013 - May 2, 2013;611-620; Paris, France. [ CrossRef ]
- Irani L, Silberman MS. From critical design to critical infrastructure: lessons from turkopticon. Interactions. 2014;21(4):32-35. [ CrossRef ]
- Kummerfeld JK. Quantifying and avoiding unfair qualification labour in crowdsourcing. arXiv. Preprint posted online May 26, 2021. [ CrossRef ]
- Hansson K, Ludwig T. Crowd dynamics: conflicts, contradictions, and community in crowdsourcing. Comput Support Coop Work. 2019;28:791-794. [ FREE Full text ] [ CrossRef ]
- Shen XL, Lee MKO, Cheung CMK. Exploring online social behavior in crowdsourcing communities: a relationship management perspective. Comput Human Behav. 2014;40:144-151. [ CrossRef ]
- Wu W, Gong X. Motivation and sustained participation in the online crowdsourcing community: the moderating role of community commitment. Internet Res. 2021;31(1):287-314. [ CrossRef ]
- Brady CJ, Villanti AC, Pearson JL, Kirchner TR, Gupta OP, Shah C. Rapid grading of fundus photographs for diabetic retinopathy using crowdsourcing. J Med Internet Res. Oct 30, 2014;16(10):e233. [ FREE Full text ] [ CrossRef ] [ Medline ]
- Mitry D, Peto T, Hayat S, Blows P, Morgan J, Khaw KT, et al. Crowdsourcing as a screening tool to detect clinical features of glaucomatous optic neuropathy from digital photography. PLoS One. 2015;10(2):e0117401. [ FREE Full text ] [ CrossRef ] [ Medline ]
- Cheng J, Manoharan M, Zhang Y, Lease M. Is there a doctor in the crowd? Diagnosis needed! (For less than $5). iConference 2015 Proceedings. 2015. URL: https://www.ideals.illinois.edu/items/73844 [accessed 2024-03-22]
- Leblanc E, Washington P, Varma M, Dunlap K, Penev Y, Kline A, et al. Feature replacement methods enable reliable home video analysis for machine learning detection of autism. Sci Rep. 2020;10(1):21245. [ FREE Full text ] [ CrossRef ] [ Medline ]
- Tariq Q, Daniels J, Schwartz JN, Washington P, Kalantarian H, Wall DP. Mobile detection of autism through machine learning on home video: a development and prospective validation study. PLoS Med. 2018;15(11):e1002705. [ FREE Full text ] [ CrossRef ] [ Medline ]
- Tariq Q, Fleming SL, Schwartz JN, Dunlap K, Corbin C, Washington P, et al. Detecting developmental delay and autism through machine learning models using home videos of Bangladeshi children: development and validation study. J Med Internet Res. 2019;21(4):e13822. [ FREE Full text ] [ CrossRef ] [ Medline ]
- Washington P, Tariq Q, Leblanc E, Chrisman B, Dunlap K, Kline A, et al. Crowdsourced privacy-preserved feature tagging of short home videos for machine learning ASD detection. Sci Rep. 2021;11(1):7620. [ FREE Full text ] [ CrossRef ] [ Medline ]
- Washington P, Leblanc E, Dunlap K, Penev Y, Kline A, Paskov K, et al. Precision telemedicine through crowdsourced machine learning: testing variability of crowd workers for video-based autism feature recognition. J Pers Med. 2020;10(3):86. [ FREE Full text ] [ CrossRef ] [ Medline ]
- Washington P, Leblanc E, Dunlap K, Penev Y, Varma M, Jung JY, et al. Selection of trustworthy crowd workers for telemedical diagnosis of pediatric autism spectrum disorder. Pac Symp Biocomput. 2021;26:14-25. [ FREE Full text ] [ Medline ]
- Washington P, Kalantarian H, Tariq Q, Schwartz J, Dunlap K, Chrisman B, et al. Validity of online screening for autism: crowdsourcing study comparing paid and unpaid diagnostic tasks. J Med Internet Res. 2019;21(5):e13668. [ FREE Full text ] [ CrossRef ] [ Medline ]
- Washington P, Chrisman B, Leblanc E, Dunlap K, Kline A, Mutlu C, et al. Crowd annotations can approximate clinical autism impressions from short home videos with privacy protections. Intell Based Med. 2022;6:100056. [ FREE Full text ] [ CrossRef ] [ Medline ]
- Insel TR. Digital phenotyping: technology for a new science of behavior. JAMA. 2017;318(13):1215-1216. [ CrossRef ] [ Medline ]
- Huckvale K, Venkatesh S, Christensen H. Toward clinical digital phenotyping: a timely opportunity to consider purpose, quality, and safety. NPJ Digit Med. 2019;2:88. [ FREE Full text ] [ CrossRef ] [ Medline ]
- Onnela JP. Opportunities and challenges in the collection and analysis of digital phenotyping data. Neuropsychopharmacology. 2021;46(1):45-54. [ FREE Full text ] [ CrossRef ] [ Medline ]
- Onnela JP, Rauch SL. Harnessing smartphone-based digital phenotyping to enhance behavioral and mental health. Neuropsychopharmacology. 2016;41(7):1691-1696. [ FREE Full text ] [ CrossRef ] [ Medline ]
- Washington P, Mutlu CO, Kline A, Paskov K, Stockham NT, Chrisman B, et al. Challenges and opportunities for machine learning classification of behavior and mental state from images. arXiv. Preprint posted online Jan 26, 2022. [ CrossRef ]
- Baumeister H, Montag C, editors. Digital Phenotyping and Mobile Sensing: New Developments in Psychoinformatics. Cham, Switzerland. Springer International Publishing; 2019.
- Laport-López F, Serrano E, Bajo J, Campbell AT. A review of mobile sensing systems, applications, and opportunities. Knowl Inf Syst. 2020;62(1):145-174. [ CrossRef ]
- Macias E, Suarez A, Lloret J. Mobile sensing systems. Sensors (Basel). 2013;13(12):17292-17321. [ FREE Full text ] [ CrossRef ] [ Medline ]
- Nazir S, Ali Y, Ullah N, García-Magariño I. Internet of things for healthcare using effects of mobile computing: a systematic literature review. Wirel Commun Mob Comput. 2019;2019:1-20. [ FREE Full text ] [ CrossRef ]
- Silva BMC, Rodrigues JJPC, de la Torre Díez I, López-Coronado M, Saleem K. Mobile-health: a review of current state in 2015. J Biomed Inform. 2015;56:265-272. [ FREE Full text ] [ CrossRef ] [ Medline ]
- Sim I. Mobile devices and health. N Engl J Med. 2019;381(10):956-968. [ CrossRef ]
- Yürür O, Liu CH, Sheng Z, Leung VCM, Moreno W, Leung KK. Context-awareness for mobile sensing: a survey and future directions. IEEE Commun Surv Tutor. 2014;18(1):68-93. [ CrossRef ]
- Picard RW. Affective Computing. Cambridge, MA. MIT Press; 2000.
- Picard RW. Affective computing: challenges. Int J Hum-Comput Stud. 2003;59(1-2):55-64. [ CrossRef ]
- Poria S, Cambria E, Bajpai R, Hussain A. A review of affective computing: from unimodal analysis to multimodal fusion. Inf Fusion. 2017;37:98-125. [ CrossRef ]
- Scherer KR, Bänziger T, Roesch E, editors. A Blueprint for Affective Computing: a Sourcebook and Manual. Oxford, United Kingdom. Oxford University Press; 2010.
- Tao J, Tan T. Affective computing: a review. In: Affective Computing and Intelligent Interaction. Berlin, Heidelberg. Springer; 2005. Presented at: First International Conference, ACII 2005; October 22-24, 2005;981-995; Beijing, China. [ CrossRef ]
- Wang Y, Song W, Tao W, Liotta A, Yang D, Li X, et al. A systematic review on affective computing: emotion models, databases, and recent advances. Inf Fusion. 2022;83-84:19-52. [ CrossRef ]
- Zhao S, Wang S, Soleymani M, Joshi D, Ji Q. Affective computing for large-scale heterogeneous multimedia data: a survey. ACM Trans Multimed Comput Commun Appl. 2019;15(3s):1-32. [ CrossRef ]
Abbreviations
Edited by A Mavragani; submitted 22.07.23; peer-reviewed by E Vashishtha, MO Khursheed, L Guo; comments to author 02.09.23; revised version received 15.11.23; accepted 30.01.24; published 11.04.24.
©Peter Washington. Originally published in the Journal of Medical Internet Research (https://www.jmir.org), 11.04.2024.
This is an open-access article distributed under the terms of the Creative Commons Attribution License (https://creativecommons.org/licenses/by/4.0/), which permits unrestricted use, distribution, and reproduction in any medium, provided the original work, first published in the Journal of Medical Internet Research, is properly cited. The complete bibliographic information, a link to the original publication on https://www.jmir.org/, as well as this copyright and license information must be included.
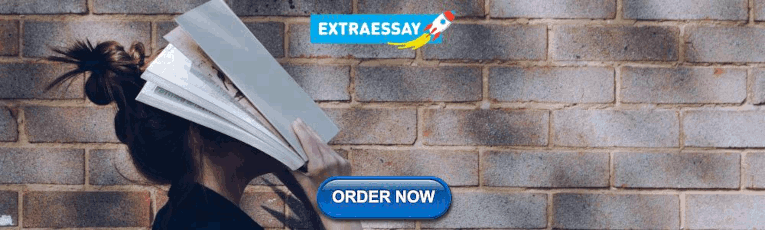
IMAGES
VIDEO
COMMENTS
Strategic human resource management (SHRM) research increasingly focuses on the performance effects of human resource (HR) systems rather than individual HR practices (Combs, Liu, Hall, & Ketchen, 2006).Researchers tend to agree that the focus should be on systems because employees are simultaneously exposed to an interrelated set of HR practices rather than single practices one at a time, and ...
Presented by: Binod Ghimire. Concept. • Human resource planning is the process of. forecasting, development, and controlling human. resource in an organization. • According to Stone, "Human ...
The three fundamental functions of human. resource planning include a) labor forecast, b) managing demand for employees and available. supply in market and c) keep a balance between labor supply ...
Objectives of Human Resource Planning: Recruit, retain & minimize the turnover of human resources. Foresee the impact of technology & improve standards. Anticipate the impact of technology on jobs ...
1. Employee Perceptions of HRM as an Antecedent, Mediator, or Outcome. Nishii and Wright (Citation 2008) developed the SHRM process framework to unravel the link between HRM and performance to shed light on the processes through which HR practices impact organizational performance (Jiang et al., Citation 2013).The starting point of the SHRM process model is the concept of variation.
In this paper, after outlining the evolution of HRM as a discipline, we will systematically review top-tier HRM journals as well as other management journals over a period of two decades. ... i.e. "inter alia, human resource planning, recruitment and selection, team deployment, performance management, and human resource administration" (7 ...
The Human Resource Management Journal has published several research papers exploring various aspects of HR in contexts of change and turmoil from a number of perspectives. This virtual special issue on HRM in times of turmoil brings together a collection of papers which, when viewed together can help shed light on some of the challenges and ...
Human Resource Development Review (HRDR) began in March 2002, starting with Holton's (2002) editorial, The Mandate for Theory in Human Resource Development.As of this writing, HRDR is one of leading journals in organizational research fields.HRDR became a Social Science Citation Index (SSCI) journal in July 2014, thanks to the tremendous efforts of the former editor, Jamie Callahan, and the ...
A Research Paper on Human Resources Planning, Process and Developing. Atyeh Mohammed Alzhrani. Published in International journal of… 30 March 2020. Business, Education. The present work addresses in a clear and simple way, the management of human resources in service organizations where staff is relevant to the achievement of policies, goals ...
Brewster 24 stressed that significant differences can be seen in the HR practices of various nations, and multinational enterprises must adapt to local specifics. The CEEIRT international research's results also attest to this fact. Based on the research examinations conducted in the various countries, it is apparent how the well-performing 'best practices' can't be used alone, as they ...
by Anna Lamb, Harvard Gazette. When COVID pushed service-based businesses to the brink, tipping became a way for customers to show their appreciation. Now that the pandemic is over, new technologies have enabled companies to maintain and expand the use of digital payment nudges, says Jill Avery. 02 Jan 2024.
The strategic human resource planning process is comprised of the following four steps, each of which will be discussed in detail: Assessing the current human resource capacity. Forecasting human resource requirements. Gap analysis. Developing human resource strategies to support college strategies.
Human Resource (HR) practices are an integral part of an organization's management strategy that focuses on. effectively managing the organization's workforce. HR practices enco mpass a wide range ...
A Research Paper on Human Resources Planning, Process and Developing Atyeh Mohammed Alzhrani Abstract The present work addresses in a clear and simple way, the management of human resources in service organizations where staff is relevant to the achievement of policies, goals and objectives. they provide the creative and
International Journal of Research in Business Studies and Management Volume 5, Issue 3, 2018, PP 24-30 ... organization by reviewing different literature on human resource planning. This paper reviews the literature relating to human resource planning and it focuses on the objectives of human resource planning, the process of human resource ...
The paper was carried along two research objectives were to specifically find out the factors underlying the increased interest in the need for human resource planning and as well as to highlights the relevance of human resource planning toward repositioning economic growth and development in Nigeria.
Human Resource is an important corporate asset and overall performance of companies depends upon the way it is put to use. In order to realize company's objectives, it is essential to have Human Resource plan (H.R.P). Planning of Human Resource is essentially matching the appropriate number of qualified and talented people
Therefore, the research on human resource forecasting using machine learning plays an important role in improving forecasting accuracy [ 26 - 30 ]. This paper studies the method of personnel resource planning and allocation based on machine learning. The basic idea of this method is to use machine learning algorithms to predict enterprise ...
This paper explores the strategic importance of human resource (HR) planning and the various techniques employed by organizations to attract talent and thus to gain a competitive edge. In this ...
This paper's goal is to assess the value of human resource planning to the company through a ... International Journal of Research Publication and Reviews, Vol 3, no 11, pp 222-227, ... Human resource planning, according to Cascio (1992), is an endeavor to foresee future economic and environmental demands on an organization and to ...
HR the board alludes to the course of human ability that makes up the association. "It is the managerial capacity through which chiefs enroll, select, train and foster the individuals from the association." According to Akey and Antwi (2017), the HR the board interaction contains six essential exercises. 2.2 Human Resources Planning Process
Human resource planning is a key strategy for ensuring long-term business sustainability and resilience. In this guide, we'll explore the essentials of human resource planning (HRP), why it's so important, and the best practices to start your human resource planning process. ... According to research by the Work Institute, 78% of the ...
This paper is in the following e-collection/theme issue: Demographics of Users, Social & Digital Divide (651) E-Health Policy and Health Systems Innovation (192) Equity and Digital Divide (155) Use and User Demographics of mHealth (299) Health Care Quality and Health Services Research (209) Digital Health, Telehealth and e-Innovation in Clinical Settings (313)
The Human Resource Management (HRM) process comprises the following steps: human resource planning, recruitment, selection, prof essional development, performance appraisal, and compensation. The ...
Modern machine learning approaches have led to performant diagnostic models for a variety of health conditions. Several machine learning approaches, such as decision trees and deep neural networks, can, in principle, approximate any function. However, this power can be considered to be both a gift and a curse, as the propensity toward overfitting is magnified when the input data are ...
The paper is related to career development and the stages of a career program. It reflects on the evolution of the individual's work over time, emphasizing the stages: exploration (attempt of new ...