Thank you for visiting nature.com. You are using a browser version with limited support for CSS. To obtain the best experience, we recommend you use a more up to date browser (or turn off compatibility mode in Internet Explorer). In the meantime, to ensure continued support, we are displaying the site without styles and JavaScript.
- View all journals
- My Account Login
- Explore content
- About the journal
- Publish with us
- Sign up for alerts
- Open access
- Published: 16 February 2021
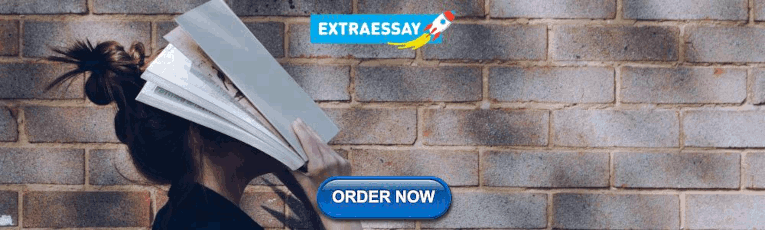
Effects of open-label placebos in clinical trials: a systematic review and meta-analysis
- Melina von Wernsdorff 1 , 2 ,
- Martin Loef 3 ,
- Brunna Tuschen-Caffier 2 &
- Stefan Schmidt 1 , 4
Scientific Reports volume 11 , Article number: 3855 ( 2021 ) Cite this article
26k Accesses
59 Citations
323 Altmetric
Metrics details
- Clinical trials
- Medical research
- Placebo effect
An Author Correction to this article was published on 25 August 2021
This article has been updated
Open-label placebos (OLPs) are placebos without deception in the sense that patients know that they are receiving a placebo. The objective of our study is to systematically review and analyze the effect of OLPs in comparison to no treatment in clinical trials. A systematic literature search was carried out in February 2020. Randomized controlled trials of any medical condition or mental disorder comparing OLPs to no treatment were included. Data extraction and risk of bias rating were independently assessed. 1246 records were screened and thirteen studies were included into the systematic review. Eleven trials were eligible for meta-analysis. These trials assessed effects of OLPs on back pain, cancer-related fatigue, attention deficit hyperactivity disorder, allergic rhinitis, major depression, irritable bowel syndrome and menopausal hot flushes. Risk of bias was moderate among all studies. We found a significant overall effect (standardized mean difference = 0.72, 95% Cl 0.39–1.05, p < 0.0001, I 2 = 76%) of OLP. Thus, OLPs appear to be a promising treatment in different conditions but the respective research is in its infancy. More research is needed, especially with respect to different medical and mental disorders and instructions accompanying the OLP administration as well as the role of expectations and mindsets.
Similar content being viewed by others
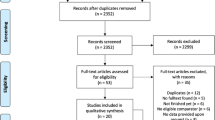
Open-label placebos—a systematic review and meta-analysis of experimental studies with non-clinical samples
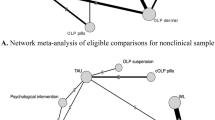
The roles of expectation, comparator, administration route, and population in open-label placebo effects: a network meta-analysis
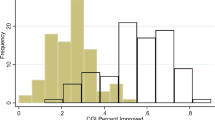
Placebo and nocebo responses in randomised, controlled trials of medications for ADHD: a systematic review and meta-analysis
Introduction.
Placebos have been the subject of many studies in the last two decades 1 and the number of clinical trials to examine a placebo treatment as the primary intervention is rapidly growing 2 . Research has shown that symptoms can be reduced in a significant way by receiving an inert medication 3 , 4 , 5 . Placebos are also increasingly used in medical practice outside of clinical trials 2 , 6 , 7 . A survey in the UK revealed that 77% of general practitioners use placebos regularly 8 . Considering not only the benefits for patients (i.e. no pharmacological side effects) but also economic effects like low priced pills 9 , deceptive placebos appear to be a promising alternative to active substances in medicine.
However, the use of placebos in primary treatment raises ethical concerns because the physicians’ prescriptions may be considered to be deceptive 10 . Patients need to be informed completely, accurately and comprehensively about their treatment 11 , otherwise the essential base for a healthy relationship between physician and patient is jeopardized 12 , 13 . Despite some these ethical concerns, a few researchers contend that deceptive placebos are acceptable in a limited number of circumstances (e.g. 14 , 15 , 16 ) since the therapeutic encounter can still be beneficial to the patient. Others say that physicians are still lying to patients “in order to bring about positive expectations surrounding treatment outcomes” 17 p. 2 which might harm the fiduciary patient-doctor relationship. This dilemma raises the question of whether the deception in placebo treatments is coercively necessary for achieving a placebo effect.
In 1965, Park and Covi 18 were the first researchers who examined if full transparency regarding the placebo treatment would still result in an observable placebo effect. Surprisingly, they found a reduction in symptoms even if patients knew that they received a placebo treatment with inert sugar pills. This line of research was not pursued further until the first randomized controlled trial (RCT) was published in 2008, which examined the placebo-effect without deception (open-label placebo, OLP) as a “dose-extender” in children with attention deficit hyperactivity disorder (ADHD) 19 , 20 . In 2010, a ground-breaking study was published by Kaptchuk et al. 21 , in which they found significant effects of OLPs in patients with irritable bowel syndrome.
Several recent reviews 22 , 23 , 24 , 25 provide an overview of current advances in clinical OLP research and formulate first hypotheses as to why placebos without deception may still have beneficial effects. The general problem in the research of placebo treatments is to differentiate adequately between a placebo effect (effect due to the placebo treatment) and a placebo response . The latter refers to all effects found in the placebo arm of a RCT. This includes, alongside the placebo effect, also the natural tendency of the condition to improve, the statistical artifact of regression to the mean, and the Hawthorne effect due to mere attention and measurement 1 . Blease et al. 22 discuss three methodological challenges to clinical OLP research. These are the choice of control group, potential bias due to unblinded clinican experimenters, and finally the role of the instruction accompanying the OLP administration. Control group OLPs are usually either compared to a ‘treatment as usual’ (TAU) arm or to a ‘no treatment’ (NT) arm, e.g. a wait-list condition. There is often a criticism that patients in the TAU group are not adequately monitored and that, as a result of this, the structural equivalency cannot be guaranteed 22 , 26 . On the other hand, wait-list controls are associated with nocebo effects in psychotherapy research 27 . But even with perfectly paralleled groups, whether TAU or NT, a significant problem remains that participants in the OLP arm and in the control group are treated differently. In one group they receive a placebo often accompanied with a positive instruction (“this placebo pill might help”) and in the control condition they don’t. This difference may result in a Hawthorne effect in the treatment arm and/or cause disappointment in the control group. Another methodological challenge is blinding. If the OLP is administered by a clinician to the patients neither of them are blinded. Thus, an OLP, defined as the administration of an inert pill with an instruction informing the patient about its inertness, cannot be seamlessly integrated into the methodology of a pharmacological RCT. Due to the information given as part of the treatment, an OLP shares aspects of psychological treatments that are beyond a purely biomedical pharmacological approach.
Finally, the accompanying instruction and narrative in the administration of OLPs is an important factor. In almost all OLP trials the provider clearly explained the inactive and inert nature of the pill, (often called a ‘sugar pill’), followed by some positive statements about this kind of treatment based on the circumstances that the placebo effect has been found to be powerful, that many other patients have already benefited from a placebo, that the body can also respond automatically after taking an inert pill, that a positive attitude about the pill might help but is not necessary or that “taking the pills faithfully is critical” 21 p. 2 . In one more recent study, patients were even told that “A few studies have shown that placebos without deception can have beneficial effects” 28 p.10 . Some non-clinical studies examined the OLP-effect, and its dependence on differing instructions, in an experimental setting 29 , 30 . The results of these two studies suggest that a narrative that might raise positive expectations in the participants plays a crucial role in eliciting OLP effects, although it is unclear whether these findings from experimental studies can be generalized to a clinical context.
Despite these methodological challenges, an assessment of the current state of research is crucial. In an earlier systematic review and meta-analysis, Charlesworth et al. 29 summarized five RCTs and found a positive medium-sized effect over all studies for OLPs compared to no treatment (NT) [standardized mean difference (SMD) = 0.88]. Their literature search was conducted in 2015 and there have been several trials published since then. Additionally, the validity of that meta-analysis is comparatively low due to the limited amount of studies and the moderate risk of bias 29 . The research on OLPs is still in its infancy and most of the studies have small sample sizes and short durations 24 . Nevertheless, the amount of studies in this field is growing. There are several recent reviews of OLP research 22 , 23 , 24 , 25 but none of them are systematic. Thus, a systematic overview of the current state of research is missing in the area of OLPs. The aim of the present study is to assess, through a systematic review and meta-analysis, whether the treatment of patients with OLPs is significantly more effective than NT (or treatment as usual (TAU), if both groups are treated) in different intervention forms, patient conditions and outcomes. Additionally, we plan to assess whether the instruction that is given with OLPs is related to their efficacy. Based on this theoretical background the following hypotheses are proposed: (1) treatment with OLPs is more effective than no treatment, and (2) positive instructions increase the effectiveness of OLPs compared to no instruction. Our systematic review was preregistered with the International Prospective Register of Systematic Reviews (PROSPERO) 2020 CRD42020161696.
Study selection
The electronic database search identified 2028 citations (Table 1 ). After removing duplicates, we screened 1246 titles, 313 abstracts and 41 full text articles. Thirteen studies (834 participants) met all of the eligibility criteria and were included into the review. Due to a within-subject design, two studies were excluded from the meta-analysis. A flow chart detailing the process of study identification and selection is shown in Fig. 1 . Tables with the characteristics of included studies (Table 2 ) are shown below. The detailed description of interventions is displayed in Table 3 .
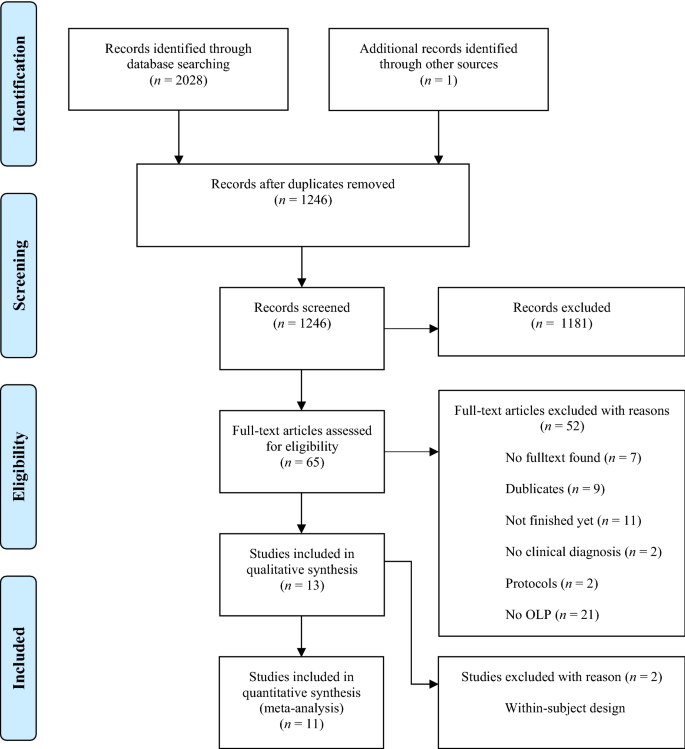
PRISMA flow chart for study selection.
Risk of bias
The risk of bias varies between the studies. The rating of most studies (69%) resulted in “some concerns” 28 , 30 , 31 , 32 , 33 , 34 , 35 , 36 . Four studies (31%) were found to have a “high risk of bias” 19 , 20 , 37 , 38 (see Fig. 2 ). Per definition, none of the participants was blinded to the treatment, so we did not rate this as a risk. When carers and people delivering the intervention were not blinded, we rated the risk with “some concerns”, as the risk of a different handling of patients depending on the group-membership cannot be ruled out. The high risk is mostly due to a considerable amount of missing outcome data and unblinded outcome assessors.
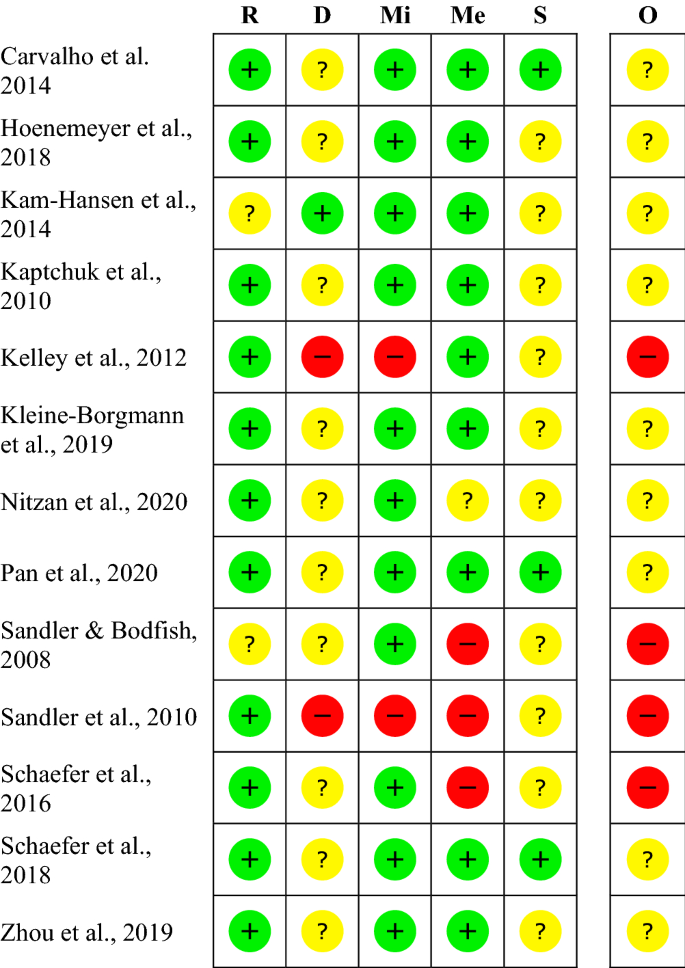
Within studies ‘risk of bias assessment for RCT on five ROB 2 criteria and overall. The risk of bias evaluation in the categories: bias arising from the randomization process (R), bias due to deviations from intended interventions (D), bias due to missing outcome data (Mi), bias in measurement of the outcome (Me), bias in selection of the reported result (S); overall risk of bias (O). Red symbols: high risk of bias; yellow symbols: some concerns; green symbols: low risk of bias.
Publication bias
The funnel plot displaying SMDs and the respective standard error for each RCT can be seen in Fig. 3 . It shows signs of asymmetry. But the Egger’s regression test does not indicate a statistically significant departure from symmetry (intercept 3.44, 95% CI -0.71 – 7.59, p = 0.14). Thus, the risk of publication bias is limited. Nevertheless, the small number of studies (the “small-study effect”, affected by substantial heterogeneity, small samples, short duration, and partially high risk of bias 36 ) may increase the risk of publication bias. The risk for the so-called “time lag bias” is also comparatively high, due to the early state of research in this field. This bias indicated that trials with negative results are published with some delay 39 .
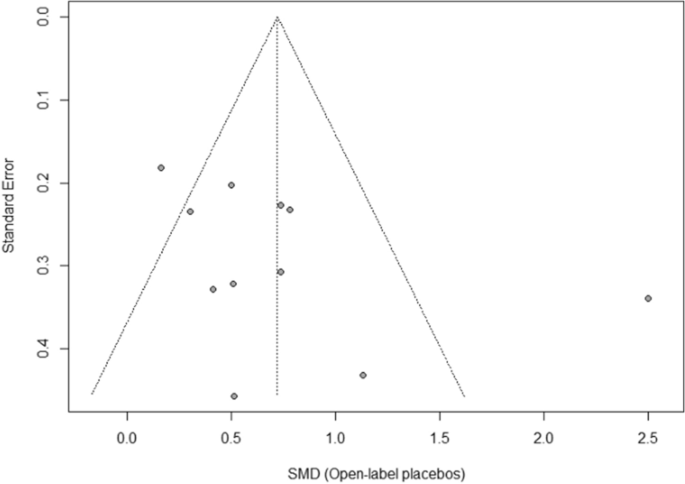
Funnel plot of standardized between-group OLP vs. NT scores. Funnel plot of standardized mean difference (SMD) vs. standard error. The dotted lines indicate the triangular region within which 95% of studies are expected to lie in the absence of publication bias.
Synthesis of results
We included k = 11 studies (N = 654 participants) into the meta-analysis. Two studies were excluded because they have a within-subject design. Due to the exclusion of the full dose group in the study from Sandler et al. 19 , the number of participants was further reduced by N = 31. The test on heterogeneity is significant [χ 2 (df = 10) = 41.14, p = 0.0001, I 2 = 76%], demonstrating some additional variance.
We found a significant positive effect of OLPs compared to no (additional) treatment SMD = 0.72, 95% CI 0.39–1.05, p < 0.0001 (Fig. 4 ).
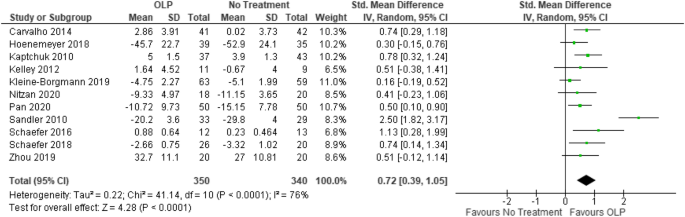
Forest plot for main outcome. Studies with open-label placebo (OLP) group and no treatment group were weighted using sample size (Total), means and standard deviations (SD). The means are shown by the green squares and the whiskers are representing the 95% confidence interval (CI). Overall standardized mean difference was calculated using the random effects model.
Additional analysis
For an explorative sensitivity analysis, we excluded four studies with a high risk of bias 19 , 20 , 37 , 38 in order to obtain a best-evidence synthesis. In this sample the heterogeneity decreased to a non-significant level [χ 2 (df = 7) = 7.32, p = 0.30, I 2 = 4%] and almost all variance could be explained by a sampling error. The corresponding effect size was lower, but still significant (SMD = 0.49, 95% CI 0.32–0.66, p < 0.00001).
We also assessed exploratory whether there is a difference in control conditions. Overall, seven trials had TAU as control group, three trials had ‘no treatment at all’ as control condition and one trial had a wait-list design (see also Table 2 ). TAU controlled trials resulted in SMD = 0.82 (95% CI 0.29–1.34, p < 0.002) with a somewhat larger heterogeneity of I 2 = 85% (χ 2 = 40.15, df = 6, p < 0.00001). The no treatment at all trials resulted in SMD = 0.60 (95% CI 0.33–0.87, p < 0.0001) with no heterogeneity of I 2 = 0% (χ 2 = 0.92, df = 2, p = 0.63).
Furthermore, we conducted a sub-group analysis in which the two studies that were not yet peer-reviewed at the date of inclusion were excluded. This analysis yielded a slightly larger SMD with about the same heterogeneity: SMD = 0.79 (95 CI 0.38–1.20, p < 0.0002), I 2 = 80% (χ 2 = 40.28, df = 8, p < 0.00001).
Results of individual studies
Carvalho et al. 30 tested two randomized groups of patients ( N = 83) with chronic lower back pain. They received either no additional treatment (TAU) or OLPs for 21 days. The investigators measured two primary outcomes, pain intensity and back-related dysfunction assessed by the Roland Morris Disability Questionnaire. At baseline, the TAU group reported lower baseline minimum pain scores, but there were no other significant differences. After 3 weeks, the OLP group had a significantly reduced disability ( p < 0.001) and significantly reduced pain ( p < 0.001).
Hoenemeyer et al. 31 carried out a 21-day RCT with two groups to examine whether OLPs reduce fatigue in cancer survivors ( N = 73). The OLP group ( n = 39) received placebo pills while the control group ( n = 35) received no additional treatment (TAU). The primary outcome fatigue was assessed via the Fatigue Symptom Inventory (FSI-14) and the Multidimensional Fatigue Symptom Inventory Short Form (MFSI-SF30). The difference between the groups concerning fatigue symptoms was significant after 21 days according to FSI-14 ( p = 0.008) and MFSI-SF30 ( p = 0.002).
Kam-Hansen et al. 32 did a randomized study comparing the efficacy of two treatments (placebo/maxalt and no treatment/baseline) along with three different types of information (positive/negative/unclear) in patients with migraine-attacks ( N = 66) using a within-subject design. The outcome was a pain scale from 0–10 two hours after treatment. The pain scores after taking an OLP-pill were significantly lower than those after no treatment ( p = 0.001).
Kaptchuk et al. 21 carried out a RCT with 80 patients suffering from irritable bowel syndrome. One group received OLPs for 21 days, the other group got NT. Differences were measured with the IBS Global Improvement Scale after 3 weeks. The OLP group experienced a significantly higher improvement than the control group ( p < 0.002).
Kelley et al. 37 did a pilot study with two parallel groups examining the OLP effect in patients with Major Depressive Disorder ( N = 20). The OLP group received placebo capsules for 14 days, while the other group was on the wait-list. The primary outcome was the 17-item Hamilton scale for depression. They found no significant difference between the groups after the treatment ( p = 0.26).
Kleine-Borgmann et al. 33 tested the efficacy of OLPs in patients with chronic back pain in a RCT. One group ( n = 63) received OLPs for 21 days and the other group ( n = 59) had no additional treatment (TAU). Changes were measured with a composite pain score from 0–10. Improvement was significant for the OLP group compared to the control group ( p = 0.001).
Nitzan et al. 34 examined whether OLP-treatment is different than TAU in the context of therapy for depression in a parallel-group design. The intervention group ( n = 18) received OLP-pills for 4 weeks, while the control group ( n = 20) got no additional treatment. The primary outcome was the self-report questionnaire for assessment of depressive symptoms (QIDS). Measures taken at the endpoint of the trial showed no significant difference between the groups ( p = 0.203).
Pan et al. 28 carried out a RCT, which tested 100 patients with menopausal hot flushes. One group ( n = 50) received OLPs for 4 weeks, and the other group ( n = 50) got no treatment (NT). After 4 weeks, the OLP group was randomized again into two groups. One received OLPs for another 4 weeks, while the other group got NT. The primary outcome was a hot flush composite score (frequency x intensity). The other primary outcome was change in problem rating measured with the Hot Flush Rating Scale (HFRS). After 4 weeks of OLP treatment, hot flushes were significantly reduced compared to the control group ( p < 0.001). The HFRS did not change ( p = 0.24).
Sandler and Bodfish 20 tested if conditioned OLPs have an effect as dose-extender on children with ADHD in a pilot RCT. Participants ( N = 26) were randomized into two groups and both received a full dose of stimulant medication for one week. Then they received in a cross-over design either (1) a 50% dose in the second week and a 50% dose + open label placebos in the third week, or (2) the reversed order. The most clinical outcome was the Clinical Global Impression scale (CGI) which was completed by the study physician after interviewing the children, the parents, and the blinded teachers. The placebos had a significant effect as a dose extender ( p = 0.004). Other primary outcomes were the IOWA Conners ADHD rating scale (P-IOWA), the Pittsburgh side effects rating scale (PSERS) and the teacher version of the IOWA Conners ADHD rating scale (T-IOWA). The results of the comparison between the 50% dose and the 50% + OLP condition were not reported.
Sandler et al. 19 examined whether conditioned OLPs have an effect on children with ADHD. They were randomized into three groups. One group ( n = 31) received a full dose (FD) of stimulant medication for two months. Another group ( n = 29) received the full dose for one month and a reduced dose (RD) for another month. The third group ( n = 33) also received a full dose for one month with additional placebos, and a reduced dose in the second month with additional placebos (RD/P). The primary outcome was the IOWA Connoers-Rating Scale (parent version). After 8 weeks, the RD group deteriorated significantly compared to the RD/P group ( p = 0.0004), according to the unblinded parents.
Schaefer et al. 38 tested the efficacy of OLPs in patients with allergic rhinitis. Participants ( N = 25) were randomized into two groups. The OLP group was treated for 14 days with inert placebo pills, while the control group received no additional treatment (TAU). The primary outcome was a self-developed symptoms questionnaire. After the treatment, the OLP group had significantly fewer symptoms than the control group ( p = 0.05). The second primary outcome was the SF-36, which examines the quality of life ( p = 0.45).
Schaefer et al. 35 conducted a RCT with four groups of patients with allergic rhinitis in order to test if the briefing combined with the OLP treatment is a significant factor for OLP effects. Two OLP groups, one with briefing ( n = 13) and one without briefing ( n = 13) were compared to two control groups, that received no additional treatment (TAU) either with ( n = 9) or without briefing ( n = 11). The primary outcome was again a self-developed symptoms questionnaire. The OLP treatment was significantly better than the TAU, independent of the briefing ( p = 0.02). The SF-36 showed no significant change (no p-value reported).
Zhou et al. 36 examined whether an OLP treatment had an effect on patients suffering from cancer-related fatigue. The 40 participants were randomized into two groups; the OLP group received OLP-pills for 21 days while the control group got NT. They used the Functional Assessment of Chronic Illness Therapy-Fatigue (FACIT-F) as a primary outcome. Patients who received OLPs significantly benefitted from the treatment ( p = 0.02).
This systematic review and meta-analysis were conducted in order to get an overview of the current body of research, and to find a pooled effect-size estimate of OLPs. We found thirteen studies that met our eligibility criteria. Eleven of them assessed the effect of OLPs in patients compared to no treatment or treatment as usual in two separate groups, making them eligible for the meta-analysis.
The quantitative synthesis of these trials revealed a significant, medium-sized effect of OLPs across those eleven RCTs. All studies included into the meta-analysis examined the efficacy of the OLP treatment, providing an accompanying narrative. Therefore, we were not able to assess the role of the instruction accompanying the placebo administration. Consequently, our hypothesis, that a positive instruction increases the efficacy of OLPs compared to no instruction, could neither be supported nor proven wrong at this stage.
Regarding the interpretation of the overall effect size of SMD = 0.72 one needs to consider some limiting factors. First, we detected hints of a publication bias in the study sample, but the respective test was not significant. The quantitative basis of the meta-analysis is based on a small number of studies, reflecting the early state of research in this field. Moreover, the set of studies showed some heterogeneity. Finally, four studies were rated to have a high risk of bias, and nine to have some concerns.
In order to assess the impact of these high-risk studies we performed an exploratory best-evidence synthesis. We excluded the four studies with a high risk of bias. In this analysis, the heterogeneity could be reduced to a non-critical value and almost all variance in the set of studies could be explained by a sampling error ( I 2 = 4%). With the exclusion of these four studies the mean effect size was reduced to a more conservative SMD = 0.49.
Regardless of this reduction of the overall effect, the same conclusions about the treatment-effect of OLPs can be drawn, although the lack of robustness means that interpretations require some caution. The decrease of heterogeneity shows that methodological impairments might be responsible for the considerable unexplained variance in our results. We abstained from carrying out a further sensitivity analysis for explaining heterogeneity because of the small number of studies.
The positive effect of OLPs is in line with findings of the earlier meta-analysis that analyzed five studies (234 participants) 29 . The updated analysis includes thirteen studies (781 participants) in the systematic review and eleven studies (654 participants) in the meta-analysis and thus, considerably broadens the database. The inclusion of more studies, some of which analyzed larger sample sizes, allows for a higher certainty of the overall OLP effect. We also included one RCT 19 , published in 2010, that was not included by Charleston et al. 29 even though it should have met their eligibility criteria. This indicates a more thorough searching strategy in the present study. Moreover, Charlesworth et al. 29 included a within-subject trial 20 in their meta-analysis without applying a different effect-size formula suitable for this type of study design 37 . By excluding this study from our meta-analysis, our overall effect provides further certainty. We included a second study, published in 2014 32 , in our review which was not included by Charlesworth et al. 31 even though it provides an appropriate comparison of the OLP and NT condition. We excluded it from our meta-analysis because of the within-subject design. In summary, the present review and meta-analysis should result in a more reliable picture of the current body of research on OLPs.
In summarizing OLP trials, we need to also consider different control conditions. In our study sample control conditions for OLP arms were either TAU , ‘ no treatment at all ’ or wait-list . The overall sample was too small to perform sensitivity analyses. Overall TAU controlled trials had slightly larger effect sizes than ‘no treatment at all trials’. For ‘no treatment at all’ trials heterogeneity dropped to zero, indicating a homogenous sub-group. However, one needs to consider that the analysis of TAU trials is confounded with high risk of bias trials. Overall, the choice, type and exact definition of control conditions are open issues in OLP research.
The overall effect of OLPs compared to NT is very promising. The effect of deceptive placebos is estimated as SMD = 0.23 (95% CI 0.17–0.28) in a much larger meta-analysis including 158 trials with more than 10,000 patients 40 . It is doubtful whether this effect size can be compared to the present finding since the meta-analysis by Hróbjartsson and Gøtzsche 40 included a wide range of conditions, including many which are not expected to respond to placebo. Another reason for this comparably larger effect in OLPs could be that in an early state of research, “positive” studies are more likely to be published (time-lag bias). Furthermore, OLPs might produce some additional effects beyond classical conditioning and expectations. Because of the novelty of this kind of treatment, patients seemed to enjoy the treatment and described it as “crazy” according to the intake and exit interviews 23 , 41 . Many patients were frustrated by multiple unsuccessful treatments and chose this counterintuitive intervention from a state of despair 24 , which may have produced new hope after the previous psychological strain. The patients were, by the very definition of the open-label placebo, not blinded, and all outcomes were self-reported, which facilitates the impact of these factors. According to Ongaro and Kaptchuk 42 , the contradictory messages embedded in the provided narrative “this placebo pill may help; it’s an inert pill without physiological effect” can produce a cognitive dissonance, which disturbs central sensitization. This effect is based on the idea of the so called “Bayesian Brain”, which creates a prediction-driven perception of the world 23 , 42 . The research around this field suggests that the perception of body sensations and the environment is cognitively modulated by expectations rather than “a bottom-up readout of sensory signals” 42 p.1 . The cognitive dissonance due to the OLP instruction might alter the familiar interpretation of symptoms and can cause a less intense sensation. A similar effect could be also shown empirically in an active placebo study 43 . The extent to which the decrease of central sensitization is actually affecting measurable health-related symptoms should be examined in OLP studies with objective outcomes. Also, further research on the distinguishing features of deceptive and open placebos is required.
With respect to the small number of studies included, there are some features that should be noted. The research in OLPs to date has been carried out by only a few authors. Some authors are therefore involved in several of the included studies, which reduces the independence of the different trials. It would be preferable if more independent replications were conducted in the future.
Sandler et al. 19 , 20 tested children, while all other studies tested adults. Children are more suggestible and have a higher placebo response than adults in the context of trials with patients suffering from mental disorders 44 . Furthermore, they administered OLPs as “dose extenders”. This procedure draws mainly on placebo effects due to classical conditioning and is different from all other studies in the meta-analysis, which are expectancy-based. The differences between these two approaches to OLP effects would be an interesting subject to address in future meta-analyses on larger databases.
We also included the study of Pan et al. 28 , even though the diagnosis for menopausal hot flushes was not validated by gynecologists. The patients filled out a screening questionnaire and were seen by a psychologist who confirmed the diagnosis during the study. According to the respective guidelines 45 most practitioners rely on women´s self-reports in the treatment of menopausal hot flushes. Hormone tests are not primarily recommended.
Even though this study shows the promising potential of OLP treatments, the overall pooled effect estimate gives only a broad hint of the real effectiveness of OLPs. Due to the short history of this field of research and the small amount of studies, we are still far from being able to understand the full implications for clinical decisions. Even though there are positive findings for OLPs in a range of physical and mental conditions (back pain, migraine, cancer-related fatigue, ADHD, allergic rhinitis and irritable bowel syndrome), most of the studies still have small sample sizes and a short duration of treatment and follow-up measures.
Additionally, all primary outcomes that were included into the meta-analysis are based on self-reports. Subjective outcomes can be biased (e.g. wishing to please the examiner). Due to the lack of blinding, patients knew about their treatment and it is difficult to interpret if the impact of this knowledge is relevant. This applies especially for the control group, whose reports could be influenced by disappointment. As mentioned before, these effects are not clearly differentiated from placebo and nocebo effect. However, all studies examined conditions (major depression, cancer-related fatigue, pain, menopausal hot flushes, irritable bowel syndrome, ADHD, allergic rhinitis), which are mostly diagnosed and rated by self-reports. Only one study 33 measured an objective outcome of mobility parameters in chronic lower back pain that demonstrated no significant effects while the self-reports did show significant subjective changes through OLPs. Another study included a questionnaire as an outcome, which was completed by blinded teachers 19 . The results were also not significant. Further research with objective outcomes and objective diagnostic tools in OLP treatment would be recommended to draw further conclusions about the measurable extent of OLP effects.
As the treatment with OLP is not blinded per definition, the advert for the recruitment of participants spoke of a “novel body-mind” treatment, which probably attracted only participants that were willing to try this kind of treatment. Such a self-selection lowers the generalizability of the results for all patients with the same condition, but open-label studies always need to deal with this selection bias.
This systematic review and meta-analysis were conducted in an early state of the research of OLPs. Therefore, we examined the intervention on a meta-level based on studies measuring different conditions, and thus different outcomes were combined. Since there is no appropriate method for these kinds of systematic reviews we followed the methodological approach of the PICO philosophy 46 . These guidelines (e.g. Cochrane) are aligned to examine a specific population with one condition and one outcome. Therefore, conceptualizations like ROB 2 needed to be adjusted to our studies, which lowered the informative value. The studies are maybe too different to be compared with the common methods, which reduces the reliability of the effect. Future clinical research on meta-level interventions would benefit from appropriate methods. Independently of this, a meta-analysis of OLPs would be desirable for studies based on the same condition and same outcome. At the moment, such a meta-analysis would include a maximum of two studies, which does not result in a reliable picture.
Our study has some limitations. We did not explicitly search for grey literature, like unpublished but completed studies, dissertations, and conference abstracts. This limitation may have led to a potential publication bias of the included studies. However, the database research provided not only results from published studies, but also registered trials that were still ongoing or never finished or published. The authors of potentially eligible titles were contacted via e-mail, which allowed us to include two completed trials that were under review. In the meantime, both of these studies have been published. We made a sub-group analysis of only the studies published before the inclusion date, which revealed no substantial differences. Another limitation is that we were not able to find evidence regarding the role of the instruction accompanying the placebo administration, since this was assessed in only one trial. Future studies should take into account whether the instruction influences the treatment effect of OLPs in the clinical context. The role of suggestion prior to the treatment should be examined, especially in contrast to an instruction that only contains the information that the pill is a placebo. It would also be interesting to study whether these suggestions actually influence already established expectations and also more general mindsets 47 . Generally, the role of expectations should be considered prior to the treatment, both in medical care and psychotherapy. A qualitative interview of patients receiving OLP treatments would provide further information about the patient’s attitude and the modes of actions in this treatment. Due to the lack of blinding of OLPs, positive expectations are particularly crucial.
The treatment with OLPs might have a significant effect. Patients that suffer from pain, allergic rhinitis, cancer-related fatigue, menopausal hot flushes, and ADHD benefited from the OLP treatment. OLPs might be as effective, or even more effective, than deceptive placebos. The current research in this field is not yet sufficient to adequately explain the responsible modes of action. More studies with a longer duration and more participants are required in this field, but the results of this study suggest a promising and novel treatment approach in the context of placebos. It also emphasizes the role and power of contextual factors in the treatment of patients such as narratives, instructions, expectations and interactions. OLP treatment takes the patient’s autonomy into account and addresses the self-healing process of the body. Beyond that, patients do not need to be blinded to their treatment, which allows them to be more aware of their conscious and unconscious reactions to the treatment. It also gives healthcare-providers the possibility to administer placebos without deception and thus, with fewer ethical concerns. However, this does not mean that the use of OLPs is free from ethical problems. Two recent publications 48 , 49 have pointed to other ethical issues in the use of OLPs such as self-stigmatization, testimonial injustice and the risk of a medicalization of issues that are more socially or environmentally determined. Nevertheless, even if the current body of research on OLP treatments does not yet allow for clinical recommendations, it supports the conclusion that it is a promising approach that is worth pursuing.
Eligibility criteria
Studies were included if they were randomized controlled trials, which also includes certain within-subject designs as, for example, in cross-over trials. The control group or condition is defined as receiving either no treatment (NT) or treatment as usual (TAU) while TAU must have been the same in both groups. Patients needed to have a medical condition or mental disorder, diagnosed by a clinician or psychologist. Studies needed to provide the necessary information for effect size calculation. We did not apply language, age, or date restrictions.
We excluded studies, which tested participants with a condition that was only diagnosed by self-report as well as studies with healthy volunteers.
Information sources
On the 24th February 2020 we searched for studies using the databases EMBASE via Elsevier Medline via PubMed, PsycINFO via EBSCO, and The Cochrane Central Register of Controlled Trials (CENTRAL). We also screened the Journal of Interdisciplinary placebo Studies DATABASE (JIPS) and the Program in Placebo Studies & Therapeutic Encounter (PiPS). No additional search was done after February 2020. Nevertheless, we included studies that were found in our search but published later. After e-mail contact with two authors (Y. Pan, and U. Nitzan) they provided their submitted manuscripts.
Search strategy
In order to update the review from Charlesworth et al. 29 we used a similar search strategy. We additionally searched the database PsycINFO and expanded our search string for a more sensitive search. For the databases Medline and EMBASE we searched with less proximity operators. The search strategy for all databases is listed in the appendix (Tables S1 to S4 ).
After removing duplicates, two investigators independently screened all remaining titles, abstracts, and full records for eligibility. Differences in results were discussed between the investigators. In addition, a third person was consulted for two studies. The main reasons for exclusion were that the placebo arm was a control condition for a treatment arm in an open-label trial, the absence of a RCT or the fact that there were no clinical patients as participants. For some titles, no abstract or full texts were found. After sending requests via ResearchGate and e-mail to the authors, most studies were either not yet finished or no reply was received.
Only studies with independent groups for each treatment condition (OLP and no (added) treatment) were included into the meta-analysis. Two submitted manuscripts 28 , 34 were provided by the authors, and in the meantime one trial was also published.
Data extraction
We extracted data about the author, year, country of trial execution, duration of treatment, number of participants, exact intervention and control condition, number of primary outcomes and type of outcome used. Additionally, we extracted information about the exact verbal instruction that was given to the patients. Data extraction was done by two independent investigators. The means and standard deviations of the OLP and control condition, as well as the number of participants in each group were extracted. We defined the endpoint as the end of the OLP treatment. In some studies, the control-group also received OLPs after two weeks 37 or after four weeks 34 . Due to the need for a NT control, we decided to choose the endpoint where the control group still received NT. We did not compare follow-up endpoint because of high heterogeneity between the study-designs. In one study 19 we only compared the reduced dose group with the reduced dose/placebo group (detailed information in Table 1 ) because the full-dose group did not meet our eligibility criteria for the control group (NT).
We chose the primary outcome if there was only one reported (see Table 2 ). Other outcomes were not included in our meta-analysis. In several studies, two primary outcomes were reported. In most cases, this was a symptom-oriented assessment and a scale referring to disease-related quality of life. In these cases, we selected the most clinically relevant outcome. This was in all studies the relevant symptom-related scale, with one exception. In the trial on chronic back pain by Carvalho 30 an unusual average of numerical rating scales was applied as the primary outcome in combination with the well-established and well-validated Roland–Morris Disability Questionnaire. Here the latter was selected as the more clinically relevant outcome.
Risk of bias assessment
The risk of bias assessment of individual studies was performed by two independent reviewers. Discrepancies were resolved by an expert’s opinion. We used the revised Cochrane risk of bias tool (ROB 2) for randomized trials 50 . We evaluated biases that arose from the randomization process, deviations from intended interventions, missing outcome data, measurement of the outcome and selection of the reported result. We desisted from increasing the risk of bias due to the lack of blinding of participants for two reasons. First, patients in the OLP treatment cannot be blinded by definition. Second, the additional effect of knowing about the group allocation cannot be separated from the placebo or nocebo effect (excitement or disappointment respectively). Even if this may affect the results of the study, it cannot be rated as a bias because it is the object of investigation in our study.
Risk of bias across studies
We did not conduct the risk of bias across studies using the GRADE (Grading of Recommendations, Assessment, Development and Evaluations) approach from Cochrane because it addresses the quality of evidence for the corporate outcome. The present study is conducted on a meta-level, which means that the studies have various outcomes and thus GRADE is not a suitable assessment. Therefore, we fell back on the PRISMA guidelines 51 for addressing the risk of bias across studies.
We examined the possibility that the included trials are biased by availability (publication bias). Therefore, we investigated publication bias of the meta-analysis by plotting the effect by the inverse of its standard error and visualizing it in a funnel plot. To evaluate asymmetry, we used visual inspection as well as Egger’s regression test 52 .
The detection of selective reporting bias addresses those studies which are excluded as they do not provide sufficient information to compute effect sizes. None of our studies were excluded for this reason, which eliminates the risk of this bias.
Statistical procedures
We conducted our meta-analysis in RevMan version 5.4 53 using the random effects model according to the diversity of patients, study designs and outcomes. The computations of Egger's Regression test and the display of figure 3 were made with the packages meta and dmetar of the statistical software R . All studies reported continuous outcomes.
In the study by Sandler et al. 19 , we used only a subset of data, contrasting the reduced-dose condition (RD) and the reduced-dose/placebo condition (RD/P). Schaefer et al. 35 randomized participants into four groups, using a 2 × 2 design, providing for each intervention (OLP or NT) either a positive briefing or not. For the meta-analysis, we used combined data for the OLP and NT groups.
We calculated the overall standardized mean-difference (SMD) by dividing the difference in mean outcome between the groups by the standard deviation of outcome among participants, and the 95% confidence interval (CI). Additionally, we took the heterogeneity between the studies into account, measuring the χ 2 test Cochran’s Q and Higgins I 2 51 in order to determine the amount of unexplained variance. In order to adjust scales which increase or decrease with disease severity in the same direction, we multiplied the mean values with − 1 in some studies 19 , 28 , 31 , 33 , 34 , 35 according to the Cochrane handbook 54 .
Additional analyses
We planned to compare studies with and without a positive narrative in a sub-group analysis. However, the number of studies (k = 1) was not sufficient to examine this pre-specified analysis. Therefore, our hypothesis that the positive narrative provided increased the efficacy of the OLP treatment could not be tested.
We decided to undertake an explorative best-evidence synthesis by excluding studies with a high risk of bias. We further performed two exploratory sub-group analyses to compare different control conditions as well as peer-reviewed vs. not peer-reviewed trials.
Data availability
All data from this study will be uploaded to a public repository upon acceptance of the manuscript for publication.
Change history
25 august 2021.
A Correction to this paper has been published: https://doi.org/10.1038/s41598-021-96604-0
Evers, A. W. M. et al. Implications of placebo and nocebo effects for clinical practice: expert consensus. PPS 87 , 204–210 (2018).
Google Scholar
Price, D. D., Finniss, D. G. & Benedetti, F. A comprehensive review of the placebo effect: recent advances and current thought. Annu. Rev. Psychol. 59 , 565–590 (2008).
PubMed Google Scholar
Beecher, H. K. The powerful placebo. JAMA 159 , 1602–1606 (1955).
CAS Google Scholar
Zhang, W., Robertson, J., Jones, A. C., Dieppe, P. A. & Doherty, M. The placebo effect and its determinants in osteoarthritis: meta-analysis of randomised controlled trials. Ann. Rheum. Dis. 67 , 1716–1723 (2008).
CAS PubMed Google Scholar
Kaptchuk, T. J. & Miller, F. G. Placebo effects in medicine. N. Engl. J. Med. 373 , 8–9 (2015).
Finniss, D. G., Kaptchuk, T. J., Miller, F. & Benedetti, F. Biological, clinical, and ethical advances of placebo effects. Lancet 375 , 686–695 (2010).
PubMed PubMed Central Google Scholar
Hróbjartsson, A. & Gøtzsche, P. C. Is the placebo powerless? Update of a systematic review with 52 new randomized trials comparing placebo with no treatment. J. Intern. Med. 256 , 91–100 (2004).
Howick, J. et al. Placebo use in the United Kingdom: results from a national survey of primary care practitioners. PLoS ONE 8 , e58247 (2013).
ADS CAS PubMed PubMed Central Google Scholar
Hamberger, J., Meissner, K., Hinterberger, T., Loew, T. & Weimer, K. Placebo economics: a systematic review about the economic potential of utilizing the placebo effect. Front. Psych. 10 , 653 (2019).
Annoni, M. The ethics of placebo effects in clinical practice and research. Int. Rev. Neurobiol. 139 , 463–484 (2018).
Blease, C., Bishop, F. L. & Kaptchuk, T. J. Informed consent and clinical trials: where is the placebo effect?. BMJ 356 , j463 (2017).
CAS PubMed PubMed Central Google Scholar
Miller, F. G. & Colloca, L. The legitimacy of placebo treatments in clinical practice: evidence and ethics. Am. J. Bioethics 9 , 39–47 (2009).
Wendler, D. & Miller, F. G. Deception in the pursuit of science. Arch. Intern. Med. 164 , 597–600 (2004).
Barnhill, A. What it takes to defend deceptive placebo use. Kennedy Inst. Ethics J. 21 , 219–250 (2011).
Kihlbom, U. Autonomy and negatively informed consent. J. Med. Ethics 34 , 146–149 (2008).
Shaw, D. M. Prescribing placebos ethically: the appeal of negatively informed consent. J. Med. Ethics 35 , 97–99 (2009).
Friesen, P. Placebos as a source of agency: evidence and implications. Front. Psych. 10 , 721–721 (2019).
Park, L. C. & Covi, L. Nonblind placebo trial: an exploration of neurotic patients’ responses to placebo when its inert content is disclosed. Arch. Gen. Psych. 12 , 336–345 (1965).
Sandler, A., Glesne, C. E. & Bodfish, J. W. Conditioned placebo dose reduction: a new treatment in ADHD?. J. Dev. Behav. Pediatr. 31 , 369–375 (2010).
Sandler, A. & Bodfish, J. W. Open-label use of placebos in the treatment of ADHD: a pilot study. Child Care Health Dev 34 , 104–110 (2008).
Kaptchuk, T. J. et al. Placebos without deception: a randomized controlled trial in irritable bowel syndrome. PLoS ONE 5 , e15591 (2010).
Blease, C. R., Bernstein, M. H. & Locher, C. Open-label placebo clinical trials: is it the rationale, the interaction or the pill?. BMJ Evid. Based Med. 25 , 159–165 (2020).
Kaptchuk, T. J. Open-label placebo: reflections on a research agenda. Perspect. Biol. Med. 61 , 311–334 (2018).
Kaptchuk, T. J. & Miller, F. G. Open label placebo: can honestly prescribed placebos evoke meaningful therapeutic benefits?. BMJ 363 , k3889 (2018).
Colloca, L. & Howick, J. Placebos without deception: outcomes, mechanisms, and ethics. Int. Rev. Neurobiol. 138 , 219–240 (2018).
Watts, S. E., Turnell, A., Kladnitski, N., Newby, J. M. & Andrews, G. Treatment-as-usual (TAU) is anything but usual: a meta-analysis of CBT versus TAU for anxiety and depression. J. Affect. Disord. 175 , 152–167 (2015).
Furukawa, T. A. et al. Waiting list may be a nocebo condition in psychotherapy trials: a contribution from network meta-analysis. Acta Psychiatr. Scand. 130 , 181–192 (2014).
Pan, Y. et al. Open-label placebos for menopausal hot flushes: a randomized controlled trial. Sci. Rep. 10 , 20090 (2020).
Charlesworth, J. E. G. et al. Effects of placebos without deception compared with no treatment: a systematic review and meta-analysis. J. Evid. Based Med. 10 , 97–107 (2017).
Carvalho, C. et al. Open-label placebo treatment in chronic low back pain: a randomized controlled trial. Pain 157 , 2766–2772 (2016).
Hoenemeyer, T. W., Kaptchuk, T. J., Mehta, T. S. & Fontaine, K. R. Open-label placebo treatment for cancer-related fatigue: a randomized-controlled clinical trial. Sci. Rep. 8 , 1–8 (2018).
ADS CAS Google Scholar
Kam-Hansen, S. et al. Altered placebo and drug labeling changes the outcome of episodic migraine attacks. Sci. Transl. Med. 6 , 218ra5 (2014).
Kleine-Borgmann, J., Schmidt, K., Hellmann, A. & Bingel, U. Effects of open-label placebo on pain, functional disability and spine mobility in chronic back pain patients: a randomized controlled trial. Pain https://doi.org/10.1097/j.pain.0000000000001683 (2019).
Article PubMed Google Scholar
Nitzan, U. et al. Open-Label placebo for the treatment of unipolar depression: results from a randomized controlled trial. J. Affect. Disord. 276 , 707–710 (2020).
Schaefer, M., Sahin, T. & Berstecher, B. Why do open-label placebos work? A randomized controlled trial of an open-label placebo induction with and without extended information about the placebo effect in allergic rhinitis. PLoS ONE 13 , e0192758 (2018).
Zhou, E. S. et al. Open-label placebo reduces fatigue in cancer survivors: a randomized trial. Support Care Cancer 27 , 2179–2187 (2019).
Kelley, J. M., Kaptchuk, T. J., Cusin, C., Lipkin, S. & Fava, M. Open-label placebo for major depressive disorder: a pilot randomized controlled trial. Psychother. Psychosom. 81 , 312–314 (2012).
Schaefer, M., Harke, R. & Denke, C. Open-label placebos improve symptoms in allergic rhinitis: a randomized controlled trial. Psychother. Psychosom. 85 , 373–374 (2016).
Schünemann, H., Brożek, J., Guyatt, G. & Oxman, A. GRADE handbook. https://gdt.gradepro.org/app/handbook/handbook.html#h.m9385o5z3li7 (2013).
Hróbjartsson, A. & Gøtzsche, P. C. Placebo interventions for all clinical conditions. Cochrane Database Syst. Rev. https://doi.org/10.1002/14651858.CD003974.pub3 (2010).
Article PubMed PubMed Central Google Scholar
Ballou, S. et al. Open-label versus double-blind placebo treatment in irritable bowel syndrome: study protocol for a randomized controlled trial. Trials 18 , 234 (2017).
Ongaro, G. & Kaptchuk, T. J. Symptom perception, placebo effects, and the Bayesian brain. Pain 160 , 1–4 (2019).
Flaten, M. A., Simonsen, T. & Olsen, H. Drug-related information generates placebo and nocebo responses that modify the drug response. Psychosom. Med. 61 , 250–255 (1999).
Parellada, M. et al. Placebo effect in child and adolescent psychiatric trials. Eur. Neuropsychopharmacol. 22 , 787–799 (2012).
German Society of Gynecology and Obstetrics. Peri- and postmenopause—diagnosis and interventions. https://www.dggg.de/leitlinien-stellungnahmen/leitlinien/ (2018).
Richardson, W. S., Wilson, M. C., Nishikawa, J. & Hayward, R. S. A. The well-built clinical question: a key to evidence-based decisions. ACP J. Club 123 , A12 (1995).
Mothes, H. et al. Expectations affect psychological and neurophysiological benefits even after a single bout of exercise. J. Behav. Med. 40 , 293–306 (2017).
Sullivan, L. S. More than consent for ethical open-label placebo research. J. Med. Ethics https://doi.org/10.1136/medethics-2019-105893 (2020).
Article Google Scholar
Blease, C. R. The role of placebos in family medicine: Implications of evidence and ethics for general practitioners. Aust. J. Gen. Pract. 48 , 700–705 (2019).
Sterne, J. A. C. et al. RoB 2: a revised tool for assessing risk of bias in randomised trials. BMJ Br. Med. J. 366 , l4898 (2019).
Moher, D., Liberati, A., Tetzlaff, J., Altman, D. G. & Group, T. P. Preferred reporting items for systematic reviews and meta-analyses: the PRISMA statement. PLoS Med. 6 , e1000097 (2009).
Egger, M., Smith, G. D., Schneider, M. & Minder, C. Bias in meta-analysis detected by a simple, graphical test. BMJ 315 , 629–634 (1997).
Review Manager (RevMan). Copenhagen: The Nordic Cochrane Centre, The Cochrane Collaboration, 2020.
Higgins, J. P. T. & Green, S. Cochrane Handbook for Systematic Reviews of Interventions (Wiley, New York, 2011).
Download references
Open Access funding enabled and organized by Projekt DEAL.
Author information
Authors and affiliations.
Department of Psychosomatic Medicine and Psychotherapy, Medical Center Freiburg, Faculty of Medicine, University of Freiburg, Hauptstr. 8, 79104, Freiburg, Germany
Melina von Wernsdorff & Stefan Schmidt
Department of Psychology, Clinical Psychology and Psychotherapy, University of Freiburg, Freiburg, Germany
Melina von Wernsdorff & Brunna Tuschen-Caffier
CHS-Institut, Berlin, Germany
Martin Loef
Institute for Frontier Areas and Mental Health, Freiburg, Germany
Stefan Schmidt
You can also search for this author in PubMed Google Scholar
Contributions
Mv.W. conducted the literature search, study inclusion, data extraction, and systematic review and computed the meta-analysis. S.S. initiated and planned the review and assisted in the study inclusion and bias assessment. S.S. and B.T. supervised the work. M.L. supervised the search strategy and assisted in the study inclusion. Mv.W. wrote first draft of the manuscript and all authors contributed to it.
Corresponding author
Correspondence to Stefan Schmidt .
Ethics declarations
Competing interests.
The authors declare no competing interests.
Additional information
Publisher's note.
Springer Nature remains neutral with regard to jurisdictional claims in published maps and institutional affiliations.
The original online version of this Article was revised: The original version of this Article contained errors, where the number of participants from Sandler et al. 19 was incorrectly utilized. Modifications have been made to the Results, Figure 3 and Discussion. Full information regarding the corrections made can be found in the correction for this Article.
Supplementary Information
Supplementary tables., rights and permissions.
Open Access This article is licensed under a Creative Commons Attribution 4.0 International License, which permits use, sharing, adaptation, distribution and reproduction in any medium or format, as long as you give appropriate credit to the original author(s) and the source, provide a link to the Creative Commons licence, and indicate if changes were made. The images or other third party material in this article are included in the article's Creative Commons licence, unless indicated otherwise in a credit line to the material. If material is not included in the article's Creative Commons licence and your intended use is not permitted by statutory regulation or exceeds the permitted use, you will need to obtain permission directly from the copyright holder. To view a copy of this licence, visit http://creativecommons.org/licenses/by/4.0/ .
Reprints and permissions
About this article
Cite this article.
von Wernsdorff, M., Loef, M., Tuschen-Caffier, B. et al. Effects of open-label placebos in clinical trials: a systematic review and meta-analysis. Sci Rep 11 , 3855 (2021). https://doi.org/10.1038/s41598-021-83148-6
Download citation
Received : 08 October 2020
Accepted : 29 January 2021
Published : 16 February 2021
DOI : https://doi.org/10.1038/s41598-021-83148-6
Share this article
Anyone you share the following link with will be able to read this content:
Sorry, a shareable link is not currently available for this article.
Provided by the Springer Nature SharedIt content-sharing initiative
This article is cited by
Open-label placebos enhance test performance and reduce anxiety in learner drivers: a randomized controlled trial.
- Michael Schaefer
Scientific Reports (2024)
Watermelon and dietary advice compared to dietary advice alone following hospitalization for hyperemesis gravidarum: a randomized controlled trial
- Peng Chiong Tan
- Gayaithiri Ramasandran
- Maherah Kamarudin
BMC Pregnancy and Childbirth (2023)
Study protocol: combined N-of-1 trials to assess open-label placebo treatment for antidepressant discontinuation symptoms [FAB-study]
- Amke Müller
- Stefan Konigorski
- Yvonne Nestoriuc
BMC Psychiatry (2023)
- Sarah Buergler
- Dilan Sezer
- Cosima Locher
Scientific Reports (2023)
Effects of an open-label placebo intervention on reactions to social exclusion in healthy adults: a randomized controlled trial
- Leonie Stumpp
- Melissa Jauch
- Rainer Greifeneder
By submitting a comment you agree to abide by our Terms and Community Guidelines . If you find something abusive or that does not comply with our terms or guidelines please flag it as inappropriate.
Quick links
- Explore articles by subject
- Guide to authors
- Editorial policies
Sign up for the Nature Briefing newsletter — what matters in science, free to your inbox daily.

Here’s how you know
- U.S. Department of Health and Human Services
- National Institutes of Health
Placebo Effect
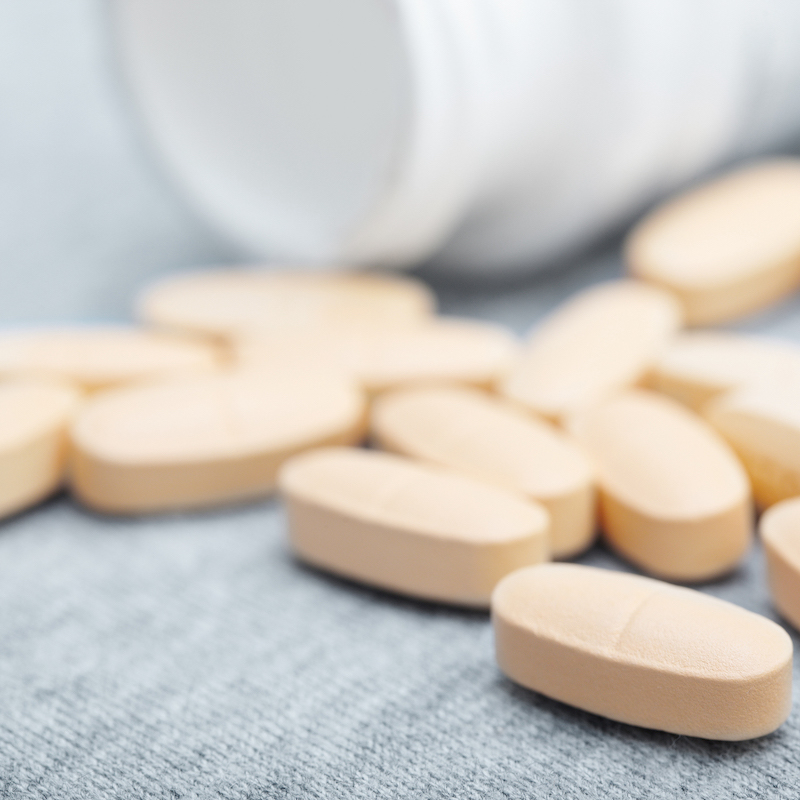
The “gold standard” for testing interventions in people is the “randomized, placebo-controlled” clinical trial, in which volunteers are randomly assigned to a test group receiving the experimental intervention or a control group receiving a placebo (an inactive substance that looks like the drug or treatment being tested). Comparing results from the two groups suggests whether changes in the test group result from the treatment or occur by chance.
The placebo effect is a beneficial health outcome resulting from a person’s anticipation that an intervention will help. How a health care provider interacts with a patient also may bring about a positive response that’s independent of any specific treatment.
Research supported by NCCIH has explored several aspects of the placebo effect. One study identified a genetic marker that may predict whether someone will respond to a placebo, another supported the idea that placebo responses may occur outside of conscious awareness, and a third suggested that placebos may be helpful even if patients know they’re receiving placebos.
- Spotlighted Research Results
- What Is a Placebo? Q and A with Ted Kaptchuk
.header_greentext{color:green!important;font-size:24px!important;font-weight:500!important;}.header_bluetext{color:blue!important;font-size:18px!important;font-weight:500!important;}.header_redtext{color:red!important;font-size:28px!important;font-weight:500!important;}.header_darkred{color:#803d2f!important;font-size:28px!important;font-weight:500!important;}.header_purpletext{color:purple!important;font-size:31px!important;font-weight:500!important;}.header_yellowtext{color:yellow!important;font-size:20px!important;font-weight:500!important;}.header_blacktext{color:black!important;font-size:22px!important;font-weight:500!important;}.header_whitetext{color:white!important;font-size:22px!important;font-weight:500!important;}.header_darkred{color:#803d2f!important;}.Green_Header{color:green!important;font-size:24px!important;font-weight:500!important;}.Blue_Header{color:blue!important;font-size:18px!important;font-weight:500!important;}.Red_Header{color:red!important;font-size:28px!important;font-weight:500!important;}.Purple_Header{color:purple!important;font-size:31px!important;font-weight:500!important;}.Yellow_Header{color:yellow!important;font-size:20px!important;font-weight:500!important;}.Black_Header{color:black!important;font-size:22px!important;font-weight:500!important;}.White_Header{color:white!important;font-size:22px!important;font-weight:500!important;} For More Information
Nccih clearinghouse.
The NCCIH Clearinghouse provides information on NCCIH and complementary and integrative health approaches, including publications and searches of Federal databases of scientific and medical literature. The Clearinghouse does not provide medical advice, treatment recommendations, or referrals to practitioners.
Toll-free in the U.S.: 1-888-644-6226
Telecommunications relay service (TRS): 7-1-1
Website: https://www.nccih.nih.gov
Email: [email protected] (link sends email)
Know the Science
NCCIH and the National Institutes of Health (NIH) provide tools to help you understand the basics and terminology of scientific research so you can make well-informed decisions about your health. Know the Science features a variety of materials, including interactive modules, quizzes, and videos, as well as links to informative content from Federal resources designed to help consumers make sense of health information.
Explaining How Research Works (NIH)
Know the Science: How To Make Sense of a Scientific Journal Article
Understanding Clinical Studies (NIH)
A service of the National Library of Medicine, PubMed® contains publication information and (in most cases) brief summaries of articles from scientific and medical journals. For guidance from NCCIH on using PubMed, see How To Find Information About Complementary Health Approaches on PubMed .
Website: https://pubmed.ncbi.nlm.nih.gov/
This publication is not copyrighted and is in the public domain. Duplication is encouraged.
NCCIH has provided this material for your information. It is not intended to substitute for the medical expertise and advice of your health care provider(s). We encourage you to discuss any decisions about treatment or care with your health care provider. The mention of any product, service, or therapy is not an endorsement by NCCIH.
For Health Care Providers
Placebo Effect - Systematic Reviews/Reviews/Meta-analyses (PubMed®)
Placebo Effect - Randomized Controlled Trials (PubMed®)
Click through the PLOS taxonomy to find articles in your field.
For more information about PLOS Subject Areas, click here .
Loading metrics
Open Access
Peer-reviewed
Research Article
Explaining placebo effects in an online survey study: Does ‘Pavlov’ ring a bell?
Roles Conceptualization, Data curation, Formal analysis, Investigation, Methodology, Project administration, Visualization, Writing – original draft
* E-mail: [email protected]
Affiliations Health, Medical and Neuropsychology unit, Leiden University, Leiden, The Netherlands, Leiden Institute for Brain and Cognition, Leiden, The Netherlands, Pediatric Immunology and Rheumatology, Wilhelmina Children’s Hospital, Utrecht, The Netherlands

Roles Conceptualization, Funding acquisition, Methodology, Supervision, Writing – review & editing
Roles Conceptualization, Writing – review & editing
Affiliation Department of Primary and Community Care, Radboud Institute for Health Sciences, Radboud University Medical Center, Nijmegen, The Netherlands
Affiliations Health, Medical and Neuropsychology unit, Leiden University, Leiden, The Netherlands, Leiden Institute for Brain and Cognition, Leiden, The Netherlands
Roles Conceptualization, Formal analysis, Methodology, Writing – review & editing
Affiliation Research Methods and Statistics, Institute of Education and Child Studies, Leiden University, Leiden, The Netherlands
Roles Writing – review & editing
Affiliation Pediatric Immunology and Rheumatology, Wilhelmina Children’s Hospital, Utrecht, The Netherlands
Affiliations Health, Medical and Neuropsychology unit, Leiden University, Leiden, The Netherlands, Leiden Institute for Brain and Cognition, Leiden, The Netherlands, Department of Psychiatry, Leiden University Medical Center, Leiden, The Netherlands
- Rosanne M. Smits,
- Dieuwke S. Veldhuijzen,
- Tim Olde Hartman,
- Kaya J. Peerdeman,
- Liesbeth M. Van Vliet,
- Henriët Van Middendorp,
- Ralph C. A. Rippe,
- Nico M. Wulffraat,
- Andrea W. M. Evers
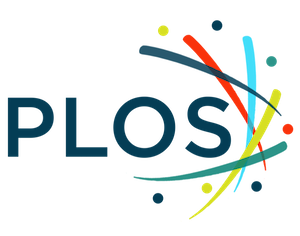
- Published: March 11, 2021
- https://doi.org/10.1371/journal.pone.0247103
- Peer Review
- Reader Comments
Despite the increasing knowledge about placebo effects and their beneficial impact on treatment outcomes, strategies that explicitly employ these mechanisms remain scarce. To benefit from placebo effects, it is important to gain better understanding in how individuals want to be informed about placebo effects (for example about the underlying mechanisms that steer placebo effects). The main aim of this study was to investigate placebo information strategies in a general population sample by assessing current placebo knowledge, preferences for different placebo explanations (built around well-known mechanisms involved in placebo effects), and attitudes and acceptability towards the use of placebo effects in treatment.
Online survey.
Leiden, The Netherlands.
Participants
444 participants (377 completers), aged 16–78 years.
Main outcome measures
Current placebo knowledge, placebo explanation preferences, and placebo attitudes and acceptability.
Participants scored high on current placebo knowledge (correct answers: M = 81.15%, SD = 12.75). Comparisons of 8 different placebo explanations revealed that participants preferred explanations based on brain mechanisms and positive expectations more than all other explanations ( F (7, 368) = 3.618, p = .001). Furthermore, attitudes and acceptability for placebos in treatment varied for the type of the condition (i.e. more acceptant for psychological complaints) and participants indicated that physicians do not always have to be honest while making use of placebo effects for therapeutic benefit.
Our results brought forth new evidence in placebo information strategies, and indicated that explanations based on brain mechanisms and positive expectations were most preferred. These results can be insightful to construct placebo information strategies for both clinical context and research practices.
Citation: Smits RM, Veldhuijzen DS, Olde Hartman T, Peerdeman KJ, Van Vliet LM, Van Middendorp H, et al. (2021) Explaining placebo effects in an online survey study: Does ‘Pavlov’ ring a bell? PLoS ONE 16(3): e0247103. https://doi.org/10.1371/journal.pone.0247103
Editor: Jenny Wilkinson, Endeavour College of Natural Health, AUSTRALIA
Received: June 3, 2020; Accepted: February 1, 2021; Published: March 11, 2021
Copyright: © 2021 Smits et al. This is an open access article distributed under the terms of the Creative Commons Attribution License , which permits unrestricted use, distribution, and reproduction in any medium, provided the original author and source are credited.
Data Availability: The data underlying this study are available on DataVerseNL ( https://doi.org/10.34894/XWKZSN ).
Funding: This work was supported by grants of the Dutch Arthritis Foundation; the European Research Council (ERC Consolidator Grant ERC-2013-CoG-617700), and the Dutch Organization for Scientific Research (NWO-Vici grant 01 6.V I CL770. L52).
Competing interests: The authors declare no conflict of interest.
Introduction
A substantial amount of literature has demonstrated the significant role of placebo effects and their positive influence on treatment outcomes [ 1 – 4 ]. Placebo effects refer to the beneficial effects after administration of an inert treatment or as an additive effect upon active treatments [ 5 ]. The underlying mechanisms involved in placebo effects have become increasingly well-understood and encompass learning mechanisms such as classical conditioning, instructional learning, and social observational learning [ 6 – 8 ]. Other factors that contribute to placebo effects involve the patient-physician relationship, communication styles, and trust [ 9 ]. Neurobiological activation related to placebo effects (i.e. placebo-induced activation of specific brain regions) have demonstrated important insights into bodily responses after placebo administration [ 8 ]. Studies that integrate this wealth of study findings into clinical practice remain scarce, and information strategies that facilitate placebo effects in treatment are limited [ 1 , 3 , 5 ]. To optimize placebo information strategies, it is important to gain a better understanding in how individuals want to be informed about placebo effects (for example, about the underlying mechanisms that steer placebo effects), before this could be used for therapeutic benefit in clinical context.
Translating current knowledge of placebo effects into useful placebo information strategies can be challenging. According to previous studies that focused on attitudes and acceptability about placebo use in patients, several misconceptions exist [ 10 – 16 ]. For example, there seems to be a lack of understanding in what placebo and placebo effects entail. A telephone survey of 853 patients with chronic health problems found that 80.7% were familiar with the term ’placebo’, whereas only about half of the participants (51.5%) had heard of the term ’placebo effects’ [ 15 ]. A misconception regarding terminology also seems to exist. A focus group study of primary care patients indicated that the word ‘placebo’ was frequently associated with ‘ineffective’, which can be challenging when attempting to integrate placebo effects in treatment [ 11 ]. Moreover, attitudes towards acceptability of placebos in treatment are divided. In one study, 50–84% of the participants indicated that if they were informed about the potential benefit of placebo effects in treatment, they deemed placebo treatment acceptable [ 15 ]. However, data from other studies reported more nuances in attitudes, where participants reported this to be strongly dependent on factors such as the type of a condition [ 11 , 17 ]. Another recurrent topic from these patient studies was the need for transparency and shared decision-making [ 11 , 14 , 15 ]. Altogether, studies that investigate placebo attitudes and acceptability stress the need to develop placebo information strategies that are feasible in daily clinical practice and, more importantly, understandable for patients. Only then effective clinical implementation of placebo effects can take place.
Interestingly, the need for transparency is often contrasted by the (mis)conception that placebos solely work in a deceptive manner held by the majority of participants in several studies [ 11 , 13 , 15 ]. However, a growing body of literature demonstrates that even when participants are aware of placebo administration (i.e. open-label placebos), placebo effects are considerable [ 18 – 23 ]. In open-label designs, providing a comprehensive placebo rationale is essential, as this boosts (or induces) treatment effects when combined with placebos [ 24 ]. Several randomized controlled trials that implemented open-label placebos have shown clinically relevant outcomes in chronic low back pain, cancer-related fatigue, irritable bowel syndrome, major depressive disorder, attention deficit hyperactivity disorder, and allergic rhinitis [ 18 – 23 ]. However, it is remarkable that the majority of these studies have employed different explanations about placebo effects, ranging from classical conditioning [ 18 , 19 , 23 , 25 ], the power of expectations [ 16 , 18 , 19 , 23 , 25 , 26 ], neurobiological processes [ 19 , 26 ], mind and body interaction [ 18 , 20 ], or the use and efficacy of non-deceptive placebos [ 19 , 26 ] (see S1 File for the explanations used in previous studies). Because of these variations, it is still unclear which of the explanations can be best used for daily practice, for example with open-label placebos.
This present study aims to investigate placebo information strategies in a general population sample to gain insights in future use for clinical practice. Because previous studies in this line of research have mainly focused on clinical populations [ 10 – 16 ], targeting a general population sample will be insightful as this population has not been influenced too much by specific clinical experiences and can therefore provide new insights in how placebo information strategies can be broadly implemented. First, this study examines the current knowledge of placebos and placebo effects. Secondly, different types of explanations about placebo effects based on their underlying mechanisms are assessed and are rated based on three outcomes; preference for each explanation, perceived efficacy for each explanation, and the willingness to participate in a treatment based on placebo effects for each explanation. Also, the study explores whether participants interpreted all different type of explanations as a single general underlying construct of placebo effects. In addition, for exploratory purposes this study investigated whether factors that have been associated with placebo effects in previous literature (i.e. age [ 27 ], gender [ 28 , 29 ], education [ 16 ], dispositional optimism [ 30 , 31 ], trait anxiety [ 32 ] neuroticism [ 33 ], beliefs about medication [ 34 ], and current placebo knowledge) could also have an impact on the preference for placebo information strategies Third and lastly, this study builds upon previous study findings by further exploring attitudes and acceptability towards the use of placebo effects in treatment [ 13 , 15 – 17 , 24 , 35 ].
Methods/Design
Participants from the age of 16 years and older were recruited via social media (e.g., Facebook and WhatsApp) between April and June 2019. Participants had to be able to speak and understand Dutch. No further in- or exclusion criteria applied. In total, 444 participants started with the online survey, of which 377 participants completed the survey (see Table 1 for the demographic characteristics of the final sample). The first question of the survey contained the information letter and consent form. Participants that did not agree with the consent question, were not able to continue with the survey and were not included in the study. Participants were compensated with a €6.50 monetary reward or two course credits for study participation. This study was approved by the Psychology Research Ethics Committee of Leiden University (CEP19-0204/53). Because this was the first study to compare placebo information strategies, there were no prior effect sizes for a power calculation. Instead, the sample size was based on previous placebo questionnaire studies. We therefore aimed to include 400 participants in line with comparable studies [ 13 , 14 , 17 ].
- PPT PowerPoint slide
- PNG larger image
- TIFF original image
https://doi.org/10.1371/journal.pone.0247103.t001
Participants were invited via a link in an e-mail or social media for an online survey via Qualtrics ( https://leidenuniv.eu.qualtrics.com ) entitled ‘What do you know about placebo?’. The survey could be filled out on a mobile phone or computer and could be paused at any time. The first part of the survey focused on placebo effects and included three subsets presented in a fixed order: a PlaceboQuiz (to assess current placebo knowledge), placebo explanations (each explanation was rated based on their preference, perceived efficacy and willingness to participate in a treatment), and placebo scenarios (to assess acceptability for placebo use in different situations). This order was chosen so that current placebo knowledge was assessed first. After that, placebo explanations were presented in a randomized order to reduce the potential influence of carry-over effects coming from the information provided by the previous placebo explanations. The placebo scenarios were presented last because by then participants had received all placebo explanations and should be able to answer the questions independent of the current placebo knowledge they had. The second part of the survey focused on personal characteristics such as demographic factors, personality traits, and beliefs about medication and medication use. At the end of the survey, participants were thanked for their participation and debriefed that the aim of this study was to gain a better understanding in how placebo effects are perceived and that this may help to optimize future treatment outcomes. The estimated time to fill out the entire online survey was about 45–60 minutes.
Placebo knowledge.
A placebo quiz (‘PlaceboQuiz’) was included and consisted of 14 true or false statements about placebos and placebo effects (e.g., ‘Placebos can trigger a physical response’). Items for this quiz varied in difficulty level from previous placebo surveys [ 10 , 13 – 16 , 26 , 36 ] ranging in questions that were commonly answered correct (e.g., ‘Thoughts can affect health’) and questions that were commonly answered incorrect (e.g., ‘Placebo effects do not work when a person knows he or she is taking a placebo’). Mean total correct scores from the PlaceboQuiz were calculated and percentage scores were computed ranging from 0–100%, where a higher score indicated more general knowledge about placebo effects.
Placebo explanations.
Different explanations about placebo effects were stated, which were all potential options for placebo information strategies. The explanations were comprised of a combination of previously used instructions in open-label studies and additional mechanisms involved in placebo effects [ 10 , 13 – 17 , 21 , 23 , 26 , 36 ] (see S1 File for an overview of previously used explanations, and the explanations used in this current study). The explanations were based on 8 themes: classical conditioning, expectations, brain mechanisms, mind and body healing processes, social learning, trust, transparency, and finally a neutral explanation (which stated that placebo effects work for some people, not all, and that it is not entirely clear why). Eventually, all explanations provided three separate outcome scores: 1) preference scores on a numerical slider from 0–10 (indicating how much participants would like to receive each explanation), 2) perceived efficacy , indicating how effective participants perceived each explanation (5-point Likert scale, strongly disagree to strongly agree), and 3) willingness to participate , indicating the willingness to participate in a treatment based on each placebo explanation (5-point Likert scale, strongly disagree to strongly agree).
Placebo attitudes and acceptability.
Seven different situations of placebo use in a treatment context were provided that varied in the level of openness of a physician about placebo use (3 situations) and the extent of placebos integrated in treatment (4 situations). For example, one question about openness of placebo administration was “The physician only has to disclose placebo use afterwards and only when it works”. For the integration of placebos in treatment, different methods of placebo use from previous literature were presented, for example as ‘dose extenders’ (“I think placebo treatment is acceptable when prescribed after a long period of medication”) or ‘therapeutic boosters’ (“I think placebo treatment is acceptable when combined with another medical treatment”) [ 20 , 22 , 37 ]. In addition to previous research about placebo attitudes indicating that attitudes may be dependent on factors such as the type of a condition [ 11 , 15 , 17 , 38 ], answer categories were added for different types of complaints (i.e., in case of a) psychological complaints, b) a cold, c) chronic diseases, d) terminal diseases, e) never correct, or f) always correct). Participants could then choose for each statement for what type of condition they would deem placebo treatment appropriate. Multiple answers were possible.
Demographic factors.
Demographic information regarding age, sex, education level, and medication use were collected. Education was categorized according to the Dutch educational system in a lower (primary and lower secondary education) and a higher education level (general secondary education, pre-university education, higher vocational education, university and academic degree).
Dispositional optimism.
The Life Orientation Test-Revised (LOT-R) [ 39 ] assessed dispositional optimism and contained six self-report items (and four filler items) rated on a 5-point Likert scale ranging from 0 (strongly disagree) to 4 ( strongly agree) , with total scores ranging from 0–24. Higher scores indicated more dispositional optimism [ 39 ].
Neuroticism.
The Revised NEO Personality Inventory (NEO-PI-R) [ 40 ] was used to assess neuroticism and consisted of eight items that were rated on a 5-point Likert scale ranging from 1 ( strongly disagree ) to 5 ( strongly agree ). The scale resulted in total scores ranging from 8–40, with higher scores indicating a higher sensitivity to stressful situations [ 40 ].
Trait anxiety.
The Spielberger State Trait Anxiety Inventory (STAI) [ 41 ] was assessed to measure trait anxiety of participants. The STAI consisted of 20 items on a 5-point Likert scale ranging from 1 ( strongly disagree) to 5 (strongly agree) . Total scores range from 20 to 80, with higher scores indicating higher trait anxiety levels [ 41 ].
General attitudes towards medication.
The General Attitudes towards Medication Survey (GAMQ) [ 42 ] was used to assess attitudes towards prescription medication in general, and consisted of 12 items on a 5-point Likert scale with scores ranging between 0 ( strongly disagree) and 5 ( strongly agree) . Final scores ranged from 0 to 60, with higher scores indicating a more positive attitude towards medication [ 42 ].
Statistical analysis
Data was analyzed using IBM SPSS Statistics (version 25).
Mean percentage correct scores of the PlaceboQuiz were computed per item. Multiple regression analysis was conducted to analyze potential prediction of placebo knowledge by age, gender, education level, medication use, dispositional optimism, trait anxiety, neuroticism, and general attitudes towards medication.
For the explanation comparisons, 8 placebo explanations were compared based on three outcome measures: preference scores, perceived efficacy scores, and willingness to participate scores. For preference scores, the outcomes were measured on a numerical scale and were entered in a repeated measures ANCOVA with the 8 explanations for each participant as the within-subject factor. The 8 explanations were entered in repeated measures ANCOVA as different timepoints to account for error variance within participants, since every participant had evaluated all 8 explanations. Furthermore, we added 8 placebo correlates: age, gender, education level, dispositional optimism, trait anxiety, neuroticism, placebo knowledge, and attitudes towards medication in the repeated measures ANCOVAs, treated as between-subject variables to explore whether these factors influenced preference scores for each explanation. Perceived efficacy scores and willingness to participate scores were measured on a Likert-scale and were first converted into numerical values using optimal scaling from non-linear principal component analyses (CATPCA) through the multiplication of individual scores with transformed values [ 43 , 44 ]. After transformations, perceived efficacy scores and willingness to participate scores for the 8 explanations were entered into the same repeated measures ANCOVAs as described above.
For visual representation, the placebo correlates were categorized to compute simple slopes in low (-1 SD ), mean, and high (+1 SD ), or were categorized based on previous literature if available [ 39 ]. To measure whether the placebo information strategies were all interpreted by a single general underlying construct of placebo effects, linear principal component analysis (PCA) was conducted for preference scores and CATPCAs were conducted for perceived efficacy and willingness to participate scores with optimal scaling transformations in order to perform a principal component analysis that reproduces as much variance as possible based on the covariance matrix [ 43 , 44 ].
Placebo acceptability.
Percentages were computed to give an overview of which placebo scenarios are deemed acceptable.
We considered a significance level of < .05 to be significant for all analyses and conducted post-hoc Bonferroni corrections for multiple testing. Normality was tested based on skewness and kurtosis. Outliers were specified as exceeding a distance of 1.5 times the interquartile range from the adjacent quartile. For effect sizes, partial eta squared (ηp 2 ) was reported with values of 0.01 considered as a small effect, 0.06 as a moderate effect and 0.14 as a large effect [ 45 ].
Sample characteristics
Of 444 participants, 377 participants completed the survey. Data from 444 participants on the PlaceboQuiz, data from 401 participants on demographic factors and data from 377 participants of the entire survey were used for data analysis. For the analysis of placebo explanations, normality tests indicated an assumption violation. To test for the severity of this violation, we conducted sensitivity analyses to compare the directions of effects of the original dataset with square root transformations versus a dataset where data of outliers based on 1.5 interquartile range of the transformed data were excluded. Exploration of the ‘outlier group’ (>1.5 interquartile range) did not indicate differences from participant data within 1.5 interquartile range in demographic characteristics. Based on this finding, we included the data of the ‘outlier’ group in the analysis, as there were no clear indications why these responses were not plausible, or fitting to this sample, and chose to report the results of the complete dataset (N = 377, see S2 File for comparisons between the group within 1.5 interquartile range and the group that exceeded the 1.5 interquartile range).
Placebo knowledge
Overall, the PlaceboQuiz indicated a mean of correct scores of 81.15% ( SD = 12.75). The lowest score (22.5% correct) was found on the question stating that placebo effects could be induced without deception, and the highest scores (96.8%) were found on the questions stating that thoughts can affect health and that placebo effects do not only occur in alternative medicine. Scores on all questions are depicted in Table 2 .
https://doi.org/10.1371/journal.pone.0247103.t002
Predictor analysis.
To assess potential predictors associated with placebo knowledge, a multiple regression analysis was conducted with age, gender, education level, medication use, dispositional optimism, trait anxiety, general attitudes towards medication, and neuroticism. Results showed that the regression model explained 13.6% of the variance, F (8, 365) = 5.222, p < .001, being mainly attributable to the significant relation between a higher education and more placebo knowledge (see Table 3 ).
https://doi.org/10.1371/journal.pone.0247103.t003
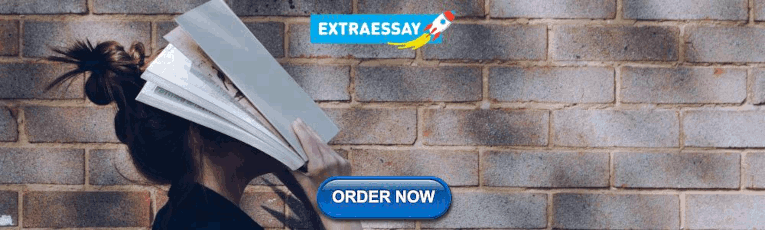
Placebo explanations
Explanation comparisons..
The Huynh-Feldt correction was used to correct for sphericity violations for preference scores, perceived efficacy and willingness to participate. A significant difference in preference scores was found between explanations, F (7, 368) = 3.618, p = .001, ηp 2 = .010. Bonferroni-corrected post-hoc tests for pairwise comparisons indicated that explanations based on brain mechanisms ( M = 6.91, SD = .09, p < .001) and expectations ( M = 6.67, SD = .09, p < .001) had significantly higher preference scores than all other explanations. The neutral explanation received significantly lower preference scores compared to the other explanations ( M = 4.28, SD = .12, p < .001) (see Fig 1 ). No significant differences were found for the explanations based on perceived efficacy scores ( F (7, 367) = 1.645, p = .121, ηp 2 = .004 For willingness to participate, also no significant differences were found based on the explanations ( F (7, 368) = 1.119, p = .348, ηp 2 = .003).
Error bars: 95% CI, *Preference scores for the explanations expectations, brain, and neutral significantly differed from the other explanations (p < .001).
https://doi.org/10.1371/journal.pone.0247103.g001
Analysis of placebo correlates.
Preference scores . Evaluation of the placebo correlates that were entered into the repeated measures ANCOVA as between-subject factors, indicated that preference scores differed based on age (F (1,368) = 10.652, p = .001, ηp 2 = .028), education level (F (1,368) = 12.363, p < .001, ηp 2 = .033), optimism (F (1,368) = 11.461, p = .001, ηp 2 = .030), trait anxiety (F (1,368) = 6.592, p = .011 ηp 2 = .018), and placebo knowledge (F (1,368) = 6.606, p = .011, ηp 2 = .018), but not with gender, neuroticism, and attitudes towards medication. For example, participants who scored 1 SD higher on trait anxiety showed a stronger preference for the explanation based on positive expectations than participants lower on trait anxiety. Participants with higher optimism scores showed a stronger preference towards the explanation based on positive expectations than participants with lower optimism scores. Another example from the placebo correlates analysis shows that participants with lower education levels rated all explanations higher (with the exception of the explanation based on brain mechanisms) than participants with higher education levels. See Fig 2 for a depiction of all significant variables of explanation preference scores.
Explanations were numbered: 1 = conditioning, 2 = expectations, 3 = brain mechanisms, 4 = mind and body, 5 = social learning, 6 = trust, 7 = neutral, 8 = transparency. The y-axis represents mean preference scores.
https://doi.org/10.1371/journal.pone.0247103.g002
Perceived efficacy . The placebo correlates that were entered into the repeated measures ANCOVA as between-subject factors.showed that perceived efficacy was associated with dispositional optimism ( F (1,367) = 9.172, p = .003, ηp 2 = .024), trait anxiety ( F (1,367) = 7.355, p = .007, ηp 2 = .020), placebo knowledge ( F (1,367) = 5.136, p = .024, ηp 2 = .014), and level of education ( F (1,367) = 10.005, p < .002, ηp 2 = .027), indicating that the effectiveness of the explanations varied based on these factors. For example, participants who scored 1 SD higher on trait anxiety perceived almost all explanations as more effective than participants lower in anxiety. Participants with lower scores on dispositional optimism showed considerably more variation in how effective they perceived explanations, favoring the explanation based on expectations, than participants with moderate or high optimism scores who showed a more stable pattern across all explanations. In addition, participants with higher placebo knowledge also showed more variation than in the perceived effectiveness of the explanation, with the explanation based on brain mechanisms receiving the highest score for effectiveness (see Fig 3 ).
Explanations were numbered (1 = conditioning, 2 = expectations, 3 = brain mechanisms, 4 = mind and body, 5 = social learning, 6 = trust, 7 = neutral, 8 = transparency). The y-axis represents transformed values of the perceived efficacy scores.
https://doi.org/10.1371/journal.pone.0247103.g003
Willingness to participate . For willingness to participate placebo correlates trait anxiety ( F (1,368) = 12.835, p < .001, ηp 2 = .034) and general attitudes towards medication ( F (1,368) = 5.340, p = .021, ηp 2 = .014), indicated that the willingness to participate was dependent on the scores of these factors. For example, participants who scored 1 SD higher on trait anxiety showed more willingness to participate in placebo treatments based on explanations that focused on brain mechanisms and transparency, whereas lower scores on trait anxiety did not show this difference. Furthermore, individuals with a more positive attitude towards medication showed more willingness to participate in placebo treatment based on the explanation of brain mechanisms (see Fig 4 ).
Explanations were numbered (1 = conditioning, 2 = expectations, 3 = brain mechanisms, 4 = mind and body, 5 = social learning, 6 = trust, 7 = neutral, 8 = transparency). The y-axis represents transformed values of the willingness to participate scores.
https://doi.org/10.1371/journal.pone.0247103.g004
Component analyses.
The PCA for preference scores revealed that all 8 explanations were interpreted as the same underlying construct, accounting for 36.24% of the total variance, and could not be reduced to a smaller set of interrelated explanations. For perceived efficacy, the CATPCA revealed a two-dimensional structure. Scores of two participants were excluded based on the scatterplot exploration because the scores did not fit either of the two components. Explanations based on expectations, mind and body, trust, social learning, and brain mechanisms described the first component and explained 19.68% of the variance. Explanations about conditioning, transparency and the neutral explanation were classified in a second component and explained 15.41% of the variance. Similar to the analysis for perceived efficacy, the CATPCA for willingness to participate revealed a two-dimensional structure for willingness to participate. Explanations based on expectations, mind and body, trust, conditioning and brain mechanisms described the first component and the explanations based on social learning, transparency and the neutral explanation accounted for the second component. The first component accounted for 22.74% of the variance and the second component for 15.81% of the variance. Because results from the component analyses indicated a dichotomy in interpretations for both outcomes of perceived efficacy and willingness to participate, additional analyses were conducted by repeating the abovementioned analyses to explore differences for the 2 underlying components of the 8 placebo explanations, however similar results were found (see S3 File ).
Placebo attitudes and acceptability
Participants were divided in their opinions about openness for the use of placebos in treatment, with equally high scores in the answer categories ‘always correct’ and ‘never correct” for the different statements. For example, 19.2% of the participants indicated that it is always correct to use deception if the physician assumes that this will benefit the patient, whereas 22.8% of the participants indicated that this is never correct (see Fig 5 ). For the integration of placebos in treatment, highest scores were given when there is no other treatment available. The lowest scores were given when stated that placebos can never be combined with other treatments (see Fig 6 ). Furthermore, there was a clear distinction between conditions in acceptance for placebos in treatment or disclosure of placebos in treatment. Participants were most acceptant of placebo treatment when it comes to complaints of psychological nature and less acceptant towards placebo treatment for chronic or terminal diseases (see Figs 5 and 6 ).
Multiple answers were possible.
https://doi.org/10.1371/journal.pone.0247103.g005
https://doi.org/10.1371/journal.pone.0247103.g006
The primary aim of this study was to investigate placebo information strategies to gain insights in their potential use for clinical practice. First, this study examined the current knowledge about placebos and placebo effects. Results indicated that participants from our sample had an overall good understanding of both concepts, but that participants were less aware of the effectiveness of non-deceptive placebos and nocebo effects. Next, the different explanations about placebo effects based on their underlying mechanisms elucidated that explanations based on brain mechanisms and positive expectancies were most preferred, and that trait anxiety was a significant factor associated with all three explanation outcomes (preference scores, perceived efficacy, and willingness to participate). Moreover, attitudes and acceptability for placebos in treatment varied for the type of the condition (i.e. more acceptant for psychological complaints) and participants indicated that physicians do not always have to be honest while administering placebos.
In light of previous placebo literature, our findings show comparable results regarding overall placebo knowledge and showed that participants were generally well-acquainted with placebo effects, with the exception of non-deceptive placebos and nocebo effects [ 13 , 14 , 16 , 17 ]. The results of the placebo correlates analysis tell us that factors previously associated to contribute to placebo effects, only explain a small portion of variance in placebo knowledge, and further research is warranted to explore this. Altogether, the results provide a clear direction for future placebo education to focus on non-deceptive placebo use and nocebo effects. Moreover, the present study adds to previous literature by demounting different placebo explanations from previous studies and assessing the preference for each explanation separately, to gain more insight in how placebo effects in treatments could be explained [ 16 , 18 , 19 , 23 , 25 , 26 ]. Furthermore, compared with previous studies that focused on attitudes and acceptability about placebo use, this study was the first to our knowledge to examine the extent of placebos integrated in treatment and demonstrated comparable nuances for placebo acceptability for the type of a condition from previous literature [ 11 , 17 ].
For a more in-depth understanding of whether participants interpreted all explanations as one underlying component, namely explaining the placebo effect, a component analysis was conducted. We considered this to be of importance in formulating placebo information strategies because a principal component analysis can serve as an exploration of the homogeneity of the reflection of internal beliefs about the underlying construct using the different formulations. For preference scores, results indicated that participant perceived the explanations as a single underlying construct, but when explanations were rated based on perceived efficacy and the willingness to participate in a treatment, a dichotomy was found. Explanations that were less positively stated (transparency, conditioning, social learning and the neutral explanation) were classified as one subcategory and more positively stated explanations (expectations, mind and body, trust, and brain mechanisms) were classified as a second subcategory. However, no significant differences in perceived efficacy or willingness to participate were found between the categories, indicating that some individuals preferred less positively stated explanations, for example individuals with relatively less positive attitudes towards medication (further explained in S3 File ). Interestingly, the component analysis also indicated that all 8 placebo explanations only accounted for approximately one third of the variance, even though the explanations encompassed well-known mechanisms involved in placebo effects such as classical conditioning, expectations, neurological processes, social learning, trust, and open-label placebos [ 3 , 8 , 46 , 47 ]. This finding raises the question whether participants may have preferred alternative explanations about placebo effects than the explanations used in the current study and previous literature, but further research is warranted to investigate this large amount of unexplained variance.
Besides investigating the internal interpretation of the placebo explanations with a principal component analysis (i.e. as a general underlying construct of placebo effects), this study also assessed whether personal factors contribute to the preference of a certain type of explanation. Our results demonstrated that trait anxiety was consistently associated with all three outcomes of placebo explanations (preference, perceived efficacy and willingness to participate). This indicates that participants that scored relatively highly on trait anxiety had a higher preference for explanations that involved positive expectations (i.e., the explanations about expectations and brain mechanisms), which could be a useful indicator for physicians that want to reassure anxious patients. However, in comparison to relevant norm groups (general population sample from Netherlands) we did find that mean trait anxiety scores from our sample were relatively higher ( M = 47.04, SD = 4.47) than from the norm group ( M = 34.3, SD = 8.3), so therefore results need to be interpreted with caution [ 48 ].
As the first study to our knowledge, the current sample consisted of participants from the general population. This group was chosen because this represents a large part of society that may be representative of a sample that occasionally visits the general practitioner and has not been influenced too much by specific clinical experiences. However, our sample had its limitations in regards to the distribution in age and education. Although the sample was rather large compared to previous placebo questionnaire samples (around 200 participants), most participants were highly educated and of relatively young age [ 13 , 17 ]. Another limitation was the cross-sectional nature of the study, which prevents to assess whether attitudes towards the use of placebo effects can change over time. In order to evaluate whether our findings can be of potential use for clinical practice, a next step would be to target a patient sample or gain further insights from a sample with health care professionals. In addition, since this was one of the first studies that focused on specific placebo information strategies, this provides directions for future research. For instance, in order to utilize the current placebo information strategies it should also be investigated whether optimized placebo information strategies result in larger placebo effects. Lastly, it would furthermore be insightful to develop similar strategies for nocebo effects, since negative expectations can have detrimental effects on treatment outcomes [ 49 ]. Overall, results from our sample showed that participants were amenable towards placebo use in treatment, and provides further guidelines in regards to information strategies and knowledge gaps about placebo effects. These findings are insightful and may contribute to the development of placebo information strategies for future clinical implementation.
This study provides new insights in how placebo effects can be explained, indicating that explanations based on brain mechanisms and positive expectations were most preferred in our sample to explain placebo effects. Moreover, our results brought forth insights when placebo use was deemed acceptable and encourages translation to clinical implementation. In addition, our results showed that even though our sample mainly consisted of higher educated participants, there was a lack of understanding about non-deceptive placebos and nocebo effects. These knowledge gaps are clear directions that need to be addressed to optimize placebo effects in treatments.
Supporting information
S1 file. overview of placebo explanations based on their underlying mechanisms..
https://doi.org/10.1371/journal.pone.0247103.s001
S2 File. Comparisons within 1.5 interquartile range and beyond 1.5 interquartile range.
https://doi.org/10.1371/journal.pone.0247103.s002
S3 File. Additional analyses based on component scores for perceived efficacy and willingness to participate outcomes.
https://doi.org/10.1371/journal.pone.0247103.s003
S4 File. Placebo questionnaire (Dutch version).
https://doi.org/10.1371/journal.pone.0247103.s004
S5 File. Placebo questionnaire (English version).
https://doi.org/10.1371/journal.pone.0247103.s005
- View Article
- PubMed/NCBI
- Google Scholar
- 24. Colloca L, Howick J. Placebos without deception: Outcomes, mechanisms, and ethics. International review of neurobiology. 138: Elsevier; 2018. p. 219–40. https://doi.org/10.1016/bs.irn.2018.01.005 pmid:29681327
- 40. Costa PT, McCrae RR. The NEO personality inventory. 1985.
- 42. Tekampe J, Peerdeman K, van Middendorp H, van Laarhoven AI, Rippe RC, Peters ML, et al. Development and Validation of the General Attitude Towards Medication Questionnaire (GAMQ)-Preprint. 2019.
- 45. Gravetter FJ, Wallnau LB. Statistics for the behavioral sciences: Cengage Learning; 2016.
- 48. Van der Ploeg H, editor Handleiding bij de Zelfbeoordelingsvragenlijst. Een Nederlandstalige bewerking van de State-Trait Anxiety Inventory STAI-DY2005: Second press. Lisse: Swets & Zeitlinger BV 10th International Conference on ….

A monthly newsletter from the National Institutes of Health, part of the U.S. Department of Health and Human Services
Search form
January 2023
Print this issue
The Powerful Placebo
Helping the Brain Heal the Body
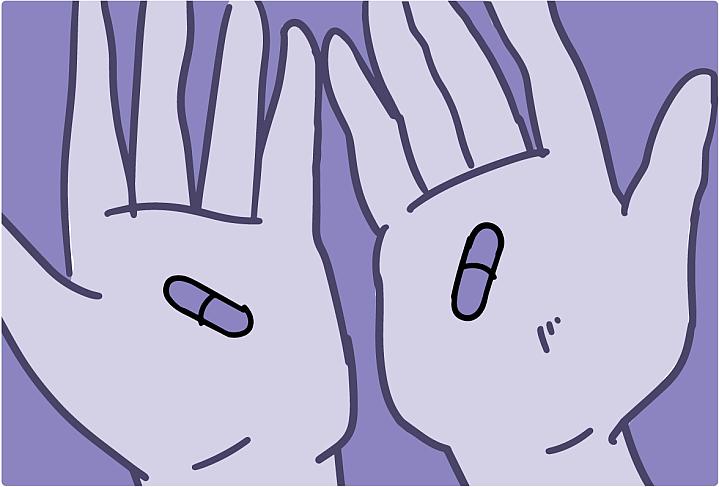
If you’re feeling unwell, you may turn to medicine to find relief. But how do you know it was the drug that made you feel better? Sometimes, when you expect a treatment to work, it will. This phenomenon is called the placebo effect. Scientists are looking for ways to harness this effect for medical treatments.
A placebo is an inactive substance or action that resembles a drug or medical treatment. But it isn’t meant to actually fix anything in your body. A pill that doesn’t contain any medicine is one example.
Historically, placebos have been a key part of testing if a new treatment works. In some types of clinical trials, participants are given either an active treatment or a placebo. But they aren’t told which one they’re getting. The treatment must do more to improve the participants’ condition than the placebo. If both groups show similar improvement, it may be from the placebo effect, not the drug.
The placebo effect works by turning on the body’s natural mechanisms for helping us feel better. Our brains make many substances that can lessen pain, stress, anxiety, and other unpleasant feelings.
Dr. Luana Colloca, a physician-scientist at the University of Maryland, Baltimore, calls this our “inner pharmacy.” Just expecting to feel better can cause the release of these substances.
“Our mindset is so critical,” Colloca says, “because our thoughts are not independent from our bodies’ responses.”
The placebo effect can be powerful. It can help with pain, fatigue, depression, anxiety, or nausea. But our inner pharmacy can’t treat everything. It can’t, for instance, make tumors go away, lower your cholesterol, or get rid of infections.
NIH-funded researchers are trying to understand the brain pathways underlying the placebo effect. They’re also looking for ways to use it to improve treatments.
Recent studies have been exploring if placebos can be used to cut down on how much medication people take. People with a chronic disease A disease or condition that lasts for three months or longer. may need to take a drug for a long time. Researchers are testing if placebos can be used to replace some drug doses. These are called dose-extending placebos. The drug effects might continue working for some time as if the patient had taken a real dose.
Dose-extending placebos may be particularly useful with opioids. Opioids are sometimes used to treat chronic pain. But they can be highly addictive and may pose risk for overdose or even death. Scientists are studying whether dose-extending placebos can reduce the chances of opioid addiction.
But for a placebo to work, do you need to believe you’re taking the real thing? Recent research suggests that may not be the case. That’s because your expectations can also affect how well a treatment works.
For instance, if you’re given a drug for pain, it may work better if you’re told that it’s a potent pain treatment. This approach can work for placebos, too—if you’re truthfully told that it has been shown to help.
A drug may also be more effective if you’ve had a good experience with it before. Colloca’s research has shown that even seeing someone else get relief from a treatment can make it more effective.
For these reasons, good communication between patients and health care providers is an essential part of treatment. Having a provider you trust, who is supportive and has empathy, can produce better treatment results.
Related Stories

Sore, Scratchy Throat?

Testing an mRNA Vaccine to Treat Pancreatic Cancer

Living With Gout

Digging Into Vitamin D
NIH Office of Communications and Public Liaison Building 31, Room 5B52 Bethesda, MD 20892-2094 [email protected] Tel: 301-451-8224
Editor: Harrison Wein, Ph.D. Managing Editor: Tianna Hicklin, Ph.D. Illustrator: Alan Defibaugh
Attention Editors: Reprint our articles and illustrations in your own publication. Our material is not copyrighted. Please acknowledge NIH News in Health as the source and send us a copy.
For more consumer health news and information, visit health.nih.gov .
For wellness toolkits, visit www.nih.gov/wellnesstoolkits .
- U.S. Department of Health & Human Services

- Virtual Tour
- Staff Directory
- En Español
You are here
Nih research matters.
October 19, 2015
Placebo Effect in Depression Treatment
At a glance.
- People with depression who benefited from a placebo showed signature changes in the brain and also responded better to subsequent medication.
- Gaining a better understanding of how placebos work could lead to the development of more effective therapies for a variety of mental disorders.

A placebo is a substance, such as a pill or shot, that doesnt contain any active medicine. Scientists typically use placebos as controls in research studies. This helps them understand how much of a medicines effects are due to the drug itself, versus how much are due to participants expectations or other factors.
People who are given a placebo may report improvements in symptoms, sometimes even when they know they’re taking something that doesn’t contain real medicine. To better understand the neurochemical mechanisms underlying the placebo effect, a team led by Dr. Jon-Kar Zubieta, formerly at the University of Michigan School of Medicine and now at the University of Utah, examined such effects in depression treatment. The study was funded in part by NIH’s National Institute of Mental Health (NIMH). Results appeared online on September 30, 2015, in JAMA Psychiatry.
The scientists enrolled 35 people with major depression who weren’t taking any medications. In the first phase of the study, the participants were randomly assigned to receive placebo pills that were described as a potentially fast-acting antidepressant (“active” placebo group) or identical pills described as a placebo with no antidepressant effects (“inactive” placebo group). Each group took the pills for a week, and then after a few days, the groups switched.
At the end of each week of treatment, the participants completed a questionnaire about their depression symptoms. They also underwent a PET brain scan to measure the activity of µ-opioid receptors, which are known to be involved in emotion, stress, social rewards, and depression. During the scan, the active placebo group received intravenous doses of saline with the understanding that it might activate brain systems involved in mood improvement. This was done to monitor the acute effects of an active placebo on brain function. The inactive placebo group received no infusions during the scan.
In the second phase of the study, all participants were treated for 10 weeks with antidepressants (usually selective serotonin reuptake inhibitors), and their depression symptoms were monitored. At the end of the study, each person was fully briefed on the study design and use of placebos.
The researchers found that the participants reported significant decreases in depression symptoms when they took the active placebo, compared to when they took the inactive placebo. These reductions were linked to increased µ-opioid receptor brain activity in regions of the brain associated with emotion and stress regulation. Notably, the increased µ-opioid activity induced by the active placebo was also associated with significantly better responses to the subsequent antidepressant treatment.
“These results suggest that some people are more responsive to the intention to treat their depression, and may do better if psychotherapies or cognitive therapies that enhance the clinician-patient relationship are incorporated into their care as well as antidepressant medications,” Zubieta says. “We need to find out how to enhance the natural resiliency that some people appear to have.”
—by Carol Torgan, Ph.D.
Related Links
- Brain Scan May Predict Best Depression Treatment
- Predicting Treatment Response to a Rapid Antidepressant
- Brain Basics
- Placebo Effect
References: Association Between Placebo-Activated Neural Systems and Antidepressant Responses: Neurochemistry of Placebo Effects in Major Depression. Peciña M, Bohnert AS, Sikora M, Avery ET, Langenecker SA, Mickey BJ, Zubieta JK. JAMA Psychiatry . 2015 Sep 30:1-8. doi: 10.1001/jamapsychiatry.2015.1335. [Epub ahead of print]. PMID: 26421634.
Funding: NIH's National Institute of Mental Health (NIMH), Phil F. Jenkins Foundation, and Michigan Institute for Clinical & Health Research.
Connect with Us
- More Social Media from NIH
- Bipolar Disorder
- Therapy Center
- When To See a Therapist
- Types of Therapy
- Best Online Therapy
- Best Couples Therapy
- Best Family Therapy
- Managing Stress
- Sleep and Dreaming
- Understanding Emotions
- Self-Improvement
- Healthy Relationships
- Student Resources
- Personality Types
- Guided Meditations
- Verywell Mind Insights
- 2023 Verywell Mind 25
- Mental Health in the Classroom
- Editorial Process
- Meet Our Review Board
- Crisis Support
The Placebo Effect: Fake Treatment, Real Response
Kendra Cherry, MS, is a psychosocial rehabilitation specialist, psychology educator, and author of the "Everything Psychology Book."
:max_bytes(150000):strip_icc():format(webp)/IMG_9791-89504ab694d54b66bbd72cb84ffb860e.jpg)
solidcolours / Getty Images
The mind can trick you into believing that a fake treatment has real therapeutic results, a phenomenon known as the placebo effect. In some cases, placebos can exert an influence powerful enough to mimic the effects of real medical treatments.
In this phenomenon, some people experience a benefit after the administration of an inactive lookalike substance or treatment. This substance, or placebo, has no known medical effect and can be in the form of a pill (sugar pill), injection (saline solution), or consumable liquid.
In most cases, the person does not know that the treatment they're receiving is actually a placebo. Instead, they believe they've received the real treatment. The placebo is designed to seem exactly like the real treatment, yet the substance has no actual effect on the condition it purports to treat.
The placebo effect is much more than just positive thinking , however. When this occurs, many people have no idea they are responding to what is essentially a sugar pill. Placebos are often used in medical research to help doctors and scientists discover and understand the physiological and psychological effects of new medications.
Here's why the placebo effect is important, how it happens, and why it works.
Placebo vs. Placebo Effect
It is important to note that a "placebo" and the "placebo effect" are different things. The term placebo refers to the inactive substance itself, while the term placebo effect refers to any effects of taking a medicine that cannot be attributed to the treatment itself.
Causes of the Placebo Effect
Although researchers know that the placebo effect is real, they do not yet fully understand how and why it occurs. Various factors might contribute to this phenomenon.
Hormonal Response
One possible explanation is that taking the placebo triggers a release of endorphins. Endorphins have a structure similar to that of morphine and other opiate painkillers and act as the brain's own natural painkillers.
Researchers have demonstrated the placebo effect in action using brain scans, showing that areas with many opiate receptors were activated in both the placebo and treatment groups. Naloxone is an opioid antagonist that blocks both natural endorphins and opioid drugs. After people received naloxone, placebo pain relief was reduced.
Conditioning
Other possible explanations include classical conditioning , or when you form an association between two stimuli resulting in a learned response. In some cases, a placebo can be paired with an actual treatment until it evokes the desired effect.
For example, if you're regularly given the same arthritis pill to relieve stiff, sore joints, you may begin to associate that pill with pain relief. If you're given a placebo that looks similar to your arthritis pill, you may still believe it provides pain relief because you've been conditioned to do so.
Expectation
Expectations, or what we believe we will experience, have been found to play a significant role in the placebo effect. People who are highly motivated and expect the treatment to work may be more likely to experience a placebo effect.
A prescribing physician's enthusiasm for treatment can even impact how a patient responds. If a doctor seems very positive that a treatment will have a desirable effect, a patient may be more likely to see benefits from taking the drug. This demonstrates that the placebo effect can even take place when a patient is taking real medications to treat an illness.
Verbal, behavioral, and social cues can contribute to a person's expectations of whether the medication will have an effect.
- Behavioral : The act of taking a pill or receiving an injection to improve your condition
- Social : Reassuring body language, eye contact, and speech from a doctor or nurse
- Verbal : Listing to a health care provider talk positively about treatment
Genes may also influence how people respond to placebo treatments. Some people are genetically predisposed to respond more to placebos. One study found that people with a gene variant that codes for higher levels of the brain chemical dopamine are more prone to the placebo effect than those with the low-dopamine version. People with the high-dopamine version of this gene also tend to have higher levels of pain perception and reward-seeking.
The Nocebo Effect
Conversely, individuals can experience more symptoms or side effects as a response to a placebo, a response that is sometimes referred to as the " nocebo effect ." For example, a patient might report having headaches, nausea, or dizziness in response to a placebo.
The placebo effect can be used in a variety of ways, including in medical research and psychology research to learn more about the physiological and psychological effects of new medications.
In Medical Research
In medical research, some people in a study may be given a placebo, while others get the new treatment being tested. The purpose of doing this is to determine the effectiveness of the new treatment. If participants taking the actual drug demonstrate a significant improvement over those taking the placebo, the study can help support the claim for the drug's effectiveness.
When testing new medications or therapies, scientists want to know if the new treatment works and if it's better than what's already available. Through their research, they learn the sort of side effects the new treatment might produce, which patients may benefit the most, and if the potential benefits outweigh the risks.
By comparing the effects of a treatment to a placebo, researchers hope to be able to determine if the effects of the medicine are due to the treatment itself or caused by some other variable.
In Psychology Experiments
In a psychology experiment, a placebo is an inert treatment or substance that has no known effects. Researchers might utilize a placebo control group , which is a group of participants who are exposed to the placebo or fake independent variable . The impact of this placebo treatment is then compared to the results of the experimental group .
Even though placebos contain no real treatment, researchers have found they can have a variety of both physical and psychological effects. Participants in placebo groups have displayed changes in heart rate, blood pressure, anxiety levels, pain perception, fatigue, and even brain activity. These effects point to the brain's role in health and well-being.
Benefits of Using a Placebo
The major advantage of using a placebo when evaluating a new drug is that it weakens or eliminates the effect that expectations can have on the outcome. If researchers expect a certain result, they may unknowingly give clues to participants about how they should behave. This can affect the results of the study.
To minimize this, researchers sometimes conduct what is known as a double-blind study . In this type of study, neither the study participants nor the researchers know who is getting the placebo and who is getting the real treatment. By minimizing the risk of these subtle biases influencing the study, researchers are better able to look at the effects of the drug and the placebo.
One of the most studied and strongest placebo effects is in the reduction of pain. According to some estimates, approximately 30% to 60% of people will feel that their pain has diminished after taking a placebo pill.
For example, imagine that a participant has volunteered for a study to determine the effectiveness of a new headache drug. After taking the drug, she finds that her headache quickly dissipates, and she feels much better. However, she later learns that she was in the placebo group and that the drug she was given was just a sugar pill.
Placebo Effect Outcomes
While placebos can affect how a person feels, studies suggest that they do not have a significant impact on underlying illnesses. A major review of more than 150 clinical trials involving placebos found that placebos had no major clinical effects on illnesses. Instead, the placebo effect had a small influence on patient-reported outcomes, particularly of perceptions of nausea and pain.
However, another review conducted nearly 10 years later found that in similar populations, both placebos and treatments had similar effects. The authors concluded that placebos, when used appropriately, could potentially benefit patients as part of a therapeutic plan.
- Depression : The placebo effect has been found to impact people with major depression disorder. In one study, participants who weren’t currently taking any other medication were given placebo pills labeled as either fast-acting antidepressants or placebo for one week. After the week, the researchers took PET scans and told the participants they were receiving an injection to improve mood. Participants who took the placebo labeled as an antidepressant as well as the injection reported decreased depression symptoms and increased brain activity in areas of the brain linked to emotion and stress regulation.
- Pain management : A small 2014 study tested the placebo effect on 66 people with episodic migraine, who were asked to take an assigned pill—either a placebo or Maxalt (rizatriptan), which is a known migraine medication—and rate their pain intensity. Some people were told the pill was a placebo, some were told it was Maxalt, and others were told it could be either. Researchers found that the expectations set by the pill labeling influenced the participants responses. Even when Maxalt was labeled as a placebo, participants gave it the same rating as a placebo that was labeled Maxalt.
- Symptom relief : The placebo effect has also been studied on cancer survivors who experience cancer-related fatigue. Participants received three weeks of treatment, either their regular treatment or a pill labeled as a placebo. The study found that the placebo (despite being labeled as such) was reported to improve symptoms while taking the medication and three weeks after discontinuation.
A Word From Verywell
The placebo effect can have a powerful influence on how people feel, but it is important to remember that they are not a cure for an underlying condition.
Healthcare providers aren't allowed to use placebos in actual practice without informing patients (this would be considered unethical care), which reduces or eliminates the desired placebo effect.
However, by using placebos in research, during which they don't have to inform the participant, scientists are able to get a better idea of how treatments impact patients and whether new medications and treatment approaches are safe and effective.
Eippert F, Bingel U, Schoell ED, et al. Activation of the opioidergic descending pain control system underlies placebo analgesia . Neuron . 2009;63(4):533-543. doi:10.1016/j.neuron.2009.07.014
Bąbel P. Classical conditioning as a distinct mechanism of placebo effects . Front Psychiatry . 2019;10:449. doi:10.3389/fpsyt.2019.00449
Brown WA. Expectation, the placebo effect and the response to treatment . R I Med J (2013) . 2015;98(5):19-21.
Hall KT, Lembo AJ, Kirsch I, et al. Catechol-O-methyltransferase val158met polymorphism predicts placebo effect in irritable bowel syndrome . PLoS One . 2012;7(10):e48135. doi:10.1371/journal.pone.0048135
Colloca L. The placebo effect in pain therapies . Annu Rev Pharmacol Toxicol . 2019;59:191-211. doi:10.1146/annurev-pharmtox-010818-021542
Hróbjartsson A, Gøtzsche PC. Placebo interventions for all clinical conditions . Cochrane Database Syst Rev . 2004;(3):CD003974. doi:10.1002/14651858.CD003974.pub2
Howick J, Friedemann C, Tsakok M, et al. Are treatments more effective than placebos? A systematic review and meta-analysis . PLoS One . 2013;8(5):e62599. doi:10.1371/journal.pone.0062599
Peciña M, Bohnert ASB, Sikora M, et al. Association between placebo-activated neural systems and antidepressant responses: Neurochemistry of placebo effects in major depression . JAMA Psychiatry . 2015;72(11):1087. doi:10.1001/jamapsychiatry.2015.1335
Kam-Hansen S, Jakubowski M, Kelley JM, et al. Altered placebo and drug labeling changes the outcome of episodic migraine attacks . Science Translational Medicine . 2014;6(218):218ra5-218ra5. doi:10.1126/scitranslmed.3006175
Hoenemeyer TW, Kaptchuk TJ, Mehta TS, Fontaine KR. Open-label placebo treatment for cancer-related fatigue: A randomized-controlled clinical trial . Sci Rep . 2018;8(1):2784. doi:10.1038/s41598-018-20993-y
Weiner IB, Craighead WE. The Corsini Encyclopedia of Psychology, Volume 3 . Hoboken, NJ: John Wiley & Sons. 2010.
By Kendra Cherry, MSEd Kendra Cherry, MS, is a psychosocial rehabilitation specialist, psychology educator, and author of the "Everything Psychology Book."

An official website of the United States government
The .gov means it’s official. Federal government websites often end in .gov or .mil. Before sharing sensitive information, make sure you’re on a federal government site.
The site is secure. The https:// ensures that you are connecting to the official website and that any information you provide is encrypted and transmitted securely.
- Publications
- Account settings
Preview improvements coming to the PMC website in October 2024. Learn More or Try it out now .
- Advanced Search
- Journal List

Placebo response and effect in randomized clinical trials: meta-research with focus on contextual effects
Sigurlaug h. hafliðadóttir.
1 Section for Biostatistics and Evidence-Based Research, the Parker Institute, Bispebjerg and Frederiksberg Hospital, Nordre Fasanvej 57, DK-2000 Copenhagen F, Denmark
2 SEARCH Research Group, Research Unit of Musculoskeletal Function and Physiotherapy, Institute of Sports Science and Clinical Biomechanics, Faculty of Health Sciences, University of Southern Denmark, Odense, Denmark
Carsten B. Juhl
3 Department of Physiotherapy and Occupational Therapy, University Hospital of Copenhagen, Herlev, Gentofte Denmark
Sabrina M. Nielsen
4 Research Unit of Rheumatology, Department of Clinical Research, University of Southern Denmark, Odense University Hospital, Odense, Denmark
Marius Henriksen
5 The Parker Institute, Copenhagen University Hospital Bispebjerg and Frederiksberg, Copenhagen, Denmark
Ian A. Harris
6 Whitlam Orthopaedic Research Centre, Ingham Institute for Applied Medical Research, Sydney, New South Wales Australia
7 Faculty of Medicine, South Western Sydney Clinical School, The University of New South Wales, Sydney, New South Wales Australia
8 Institute of Musculoskeletal Health, School of Public Health, The University of Sydney, Sydney, New South Wales Australia
Henning Bliddal
Robin christensen, associated data.
All data generated or analyzed during this study are included in this published article and its supplementary information files.
Contextual effects (i.e., placebo response ) refer to all health changes resulting from administering an apparently inactive treatment. In a randomized clinical trial (RCT), the overall treatment effect (i.e., the post-treatment effect in the intervention group) can be regarded as the true effect of the intervention plus the impact of contextual effects. This meta-research was conducted to examine the average proportion of the overall treatment effect attributable to contextual effects in RCTs across clinical conditions and treatments and explore whether it varies with trial contextual factors.
Data was extracted from trials included in the main meta-analysis from the latest update of the Cochrane review on “ Placebo interventions for all clinical conditions” (searched from 1966 to March 2008). Only RCTs reported in English having an experimental intervention group, a placebo comparator group, and a no-treatment control group were eligible.
In total, 186 trials (16,655 patients) were included. On average, 54% (0.54, 95%CI 0.46 to 0.64) of the overall treatment effect was attributable to contextual effects. The contextual effects were higher for trials with blinded outcome assessor and concealed allocation. The contextual effects appeared to increase proportional to the placebo effect, lower mean age, and proportion of females.
Approximately half of the overall treatment effect in RCTs seems attributable to contextual effects rather than to the specific effect of treatments. As the study did not include all important contextual factors (e.g., patient-provider interaction), the true proportion of contextual effects could differ from the study’s results. However, contextual effects should be considered when assessing treatment effects in clinical practice.
Trial registration
PROSPERO CRD42019130257 . Registered on April 19, 2019.
Supplementary Information
The online version contains supplementary material available at 10.1186/s13063-021-05454-8.
The importance of patients’ expectations, emotions, and clinical context in medical practice should not be ignored, but their impact on health care outcomes has only recently been evaluated [ 1 – 6 ]. Patients’ expectations and memories, the place in which the treatment is delivered, and the interaction between the patient and provider are just some of many factors in a “ therapeutic environment ” that can affect the treatment outcome [ 7 , 8 ] and are linked to the placebo response [ 1 , 7 ].
The Society for Interdisciplinary Placebo Studies defines placebo effects as changes specifically attributable to placebo mechanisms (e.g., the neurobiological and psychological mechanisms of expectations), whereas placebo response refers to all health changes resulting from administering an inactive treatment, including regression towards the mean and natural course of the disease [ 9 ]. Hence, the placebo response includes the placebo effect and is also referred to as contextual effects ; see Fig. Fig.1 1 .

Explanatory diagram illustrating the contribution of the placebo effect and placebo response relative to the estimated effect of active treatment
Because any positive health change is of importance to patients, clinicians should acknowledge both the placebo effect (i.e. in research) and the placebo response (i.e. in practice). In clinical trials, the difference between the group receiving the experimental intervention and the placebo (comparator) group indicates the strength of the active treatment (i.e., net benefit). By looking only at the difference between these two groups, the clinical impact of the placebo response (i.e., the contextual effects) gets overlooked [ 10 ]. This omission can result in an ‘efficacy paradox’—a discrepancy between treatment effects reported in randomized clinical trials (RCTs), and the overall treatment effect experienced by patients and observed in clinical practice [ 11 ].
Contextual effects have been quantified by using the proportional contextual effect (PCE)—the proportion of the overall treatment effect attributable to contextual effects [ 12 ]. While the net benefit remains an important goal for any randomized trial, a shift in focus to the overall benefit and the PCE would mitigate the efficacy paradox and highlight the contribution of contextual effects, both in research and clinical practice.
Our primary objective of this meta-epidemiological study was to examine the average proportion of the overall treatment effect that may be explained as contextual effects, in an attempt to address the aforementioned “efficacy paradox” [ 10 , 11 ]. Our secondary objective was to examine whether the contextual effects differ for different contextual factors; i.e., factors related to study design, type of intervention, and patients included. Our third objective was to explore the association between the contextual effects and placebo effect.
Study selection, assessment of eligibility criteria, data extraction, and statistical analysis were performed based on a predefined protocol (PROSPERO registration no. CRD42019130257, Additional file 1 ), in accordance with the methodology guidelines from Cochrane. The findings are reported according to the Preferred Reporting Items for Systematic reviews and Meta-Analysis (PRISMA) statement [ 13 ] (Additional file 2 ).
Data sources and searches
Only trials included in the latest update of the Cochrane review “ Placebo interventions for all clinical conditions ” by Hróbjartsson and Gøtzsche [ 14 ] were considered eligible, and therefore, no new literature search was performed [ 15 , 16 ].
Study selection
Only randomized trials having an experimental intervention group, a placebo comparator group, and a no-treatment (control) group were considered eligible. As in the original meta-analysis [ 14 , 17 ], participants were patients with any somatic or psychiatric disease or symptoms. Besides the exclusion criteria described in detail in the Cochrane review [ 14 ], trials without an intervention group and trials written in languages other than English were excluded.
Data collection process and data items
A data-extraction form was developed for data collection. One reviewer (S.H.H.) extracted data and selected the outcome of interest based on the description in the Cochrane review [ 14 ]. A second reviewer (R.C) was consulted when necessary, and doubts were discussed to consensus. The extracted data included the year of publication, study design, number of participants randomized and analyzed, baseline characteristics (average age, proportion of women, diagnosis, chronic or non-chronic condition), time of outcome measurement, type of placebo (pharmacological, physical, or psychological), experimental and no-treatment intervention and primary outcome.
Further, the type of outcome was categorized in ( i ) patient-reported outcomes (e.g., pain); ( ii ) observable patient-reported outcomes (e.g., vomiting); ( iii ) observer-reported outcomes dependent on cooperation of the patient (e.g., forced expiratory volume); ( iv ) observer-reported outcomes not dependent on patient cooperation (e.g., blood pressure); and ( v ) laboratory data (e.g., hemoglobin concentration). Other extracted information included dropout rate; blinding of participants, providers, and observers; allocation concealment; settings (i.e., single-center or multicenter); and information given to participants (i.e., whether participants were informed that the trial involved a placebo intervention). End-of-treatment data were preferred over follow-up data to reduce bias due to patients leaving the primary trial and effects consequentially diminishing. Change from baseline was preferred, but if only final values were available, these were used. For crossover trials, data were extracted from the first treatment period only, to avoid any carry-over effect. If that was not possible, summary data were used (naively) as if they had been derived from a parallel-group trial.
Risk of bias in individual studies
Risk of bias of the included studies was assessed and compared to those from the original Cochrane review [ 14 ]. This approach enabled us to rate the risk of bias in domains from the Cochrane Risk of Bias tool [ 18 ] as High , Low , or Unclear risk . In addition, a simple risk-of-bias assessment proposed in the Cochrane review [ 14 ] was used, where trials with low risk of bias were defined as fulfilling the three following criteria: ( i ) adequate concealment of allocation, ( ii ) dropout rate no more than 15%, and ( iii ) inclusion of at least 50 patients.
Summary measures
Proportional contextual effect (pce).
For each trial, the PCE was calculated by dividing the improvement in the placebo control group (∆ m C ) by the improvement in the experimental intervention (∆ m I ) group (i.e., PCE=∆ m C /∆ m I ) [ 19 ]. For trials with continuous outcomes, the improvement was defined as the mean change from baseline in the group, in the unit of standard deviation (SD). For trials with binary outcomes, improvement was defined as the number of participants improved in each group (placebo: n C , and intervention: n I ), divided by the number of participants randomized to that particular group ( N C and N I ). For trials with more than one relevant experimental intervention group (or placebo group), where all arms were relevant, treatment groups were combined into one group by calculating a weighted mean prior to perform the meta-analysis techniques. The PCE ratio was log e -transformed to normalize the distribution for the analysis and back-transformed for reporting. Theoretically, the PCE should range from 0 to 1, where 0 indicates no contribution from contextual effects while 1 indicates 100% contribution from contextual effects [ 10 ].
In trials with continuous outcomes, where either the intervention or placebo group showed no improvement (change score = 0) or worsening (negative score) from baseline, a miniscule effect (i.e., 1% benefit [multiplying by 1.01]) was imputed to enable estimation of the ratio (rather than excluding the trial from the primary analysis). In trials with binary outcomes, where no participants improved in the placebo group, it was not possible to log-transform the PCE. In these cases, we applied a “modified Woolf approach” where 0.5 was added to all cells as if 0.5 participant had improved and corresponding 0.5 participant had worsened, making it possible to include the trial in the primary quantitative synthesis.
Placebo effect in Cochrane review
For our third objective, exploring the association between the PCE and placebo effect, we used the estimates directly from the Cochrane review [ 14 ], odds ratio (OR) for binary outcomes, and standardized mean difference (SMD) for continuous outcomes—estimated by comparing the placebo comparator group and the no treatment control group. As a second step, in order to enable a meta-analytic combination of results, these placebo effect results were converted from OR to SMD. This conversion was done by converting the ln(OR) to the corresponding SMD, dividing by 1.81 (i.e., π / 3 ), as suggested by Chinn [ 20 ].
Data synthesis and analysis
A Restricted Maximum Likelihood (REML) mixed-effects model was applied to combine the log e (PCEs) across trials. To evaluate the degree of heterogeneity, the I 2 index was estimated describing the percentage of total variation attributable to heterogeneity [ 21 ].
As outlined in the protocol, a number of pre-specified stratified analyses were performed: ( i ) time of outcome measurement, ( ii ) type of intervention, ( iii ) type of outcome, ( iv ) blinding of participants and treatment providers, ( v ) blinding of outcome assessor, ( vi ) allocation concealment, ( vii ) risk of bias, ( viii ) information to participants, and ( ix ) trial settings. Furthermore, the patient’s condition (i.e., chronic or non-chronic) was assessed at trial-level (based on the trial’s eligibility criteria).
Meta-regression analysis (REML models) was conducted involving covariates at trial-level to investigate whether individual covariates could explain heterogeneity (i.e., reduce variability) of the PCE among studies. These variables were ( i ) patient age, ( ii ) proportion of females, ( iii ) sample size, and ( iv ) year of publication. A meta-regression analysis was also performed to investigate the association between PCE and the corresponding placebo effect (i.e., SMD), as reported in the Cochrane review [ 14 ]. Small-study bias was examined using a funnel plot and Egger’s test [ 22 ]. Furthermore, based on the various risk-of-bias assessments for each trial, pre-specified exploratory sensitivity analyses were conducted in order to assess whether possible biases could affect the estimates. Trial characteristics, such as overall risk of bias, sample size [ 23 ], and trial settings (single-center or multicenter trial) [ 24 ], were further used in the pre-specified analyses to evaluate possible bias across studies. P values were obtained as part of the meta-regression analysis; i.e., based on F tests if more than two groups were compared and t tests if only two groups were compared. All statistical tests were performed using STATA/IC 15.1 (Stata Corp LLC, TX, USA).
Search results
All 202 trials included in the main analysis of the Cochrane review by Hróbjartsson and Gøtzsche [ 14 ] were screened for inclusion (Fig. (Fig.2). 2 ). Five trials [ 25 – 29 ] were not in English. The remaining 197 trials were read in full text, of which 7 trials [ 30 – 36 ] did not meet the inclusion criteria. Relevant outcome data were not accessible in 4 trials [ 37 – 40 ], leaving 186 trials for inclusion in the meta-analysis.

Flow diagram of study selection criteria.
a Trials where either intervention or placebo group showed no improvement or got worse, requiring adjustment in the form of imputation of a small treatment effect
Included studies
The included trials were conducted in 23 countries and published between 1961 and 2008, with the majority published after 1994. There were 11 crossover trials [ 41 – 51 ], of which 10 (total of 250 patients) had no data available from the first period only, and thus were handled as parallel trials by using summary data (ignoring the design). There were 54 trials (total of 5,160 patients) having more than three arms. In 18 of those [ 52 – 69 ], one or more additional arms were disregarded (a total of 796 patients) in order to calculate the PCE for groups that had comparable interventions. In the remaining 36 trials with more than three arms, all arms were relevant and therefore included which left a total number of 16,655 patients for the meta-analysis.
Trial characteristics are summarized in Table Table1. 1 . The mean age of participants ranged from 0 (infants) to 83.8 years, with an average mean age of 39.9 years (age was not reported in 11 trials). The percentage of females ranged from 0 to 100, with mean percentage of 58.6 (female/male proportions was not reported in 23 trials). Patients included in the trials had a broad spectrum of conditions, such as depression, hypertension, obesity, or headaches. Pain was the most common outcome measure (reported in 53 trials). Other frequent measures were for anxiety, medication use, smoking cessation, and nausea. Interventions were categorized into three types: pharmacological (e.g., medication or supplements given orally or via injection/inhalation), physical (e.g., acupuncture or transcutaneous electrical nerve stimulation), and psychological (e.g., cognitive therapy or hypnosis). In 22 trials, patients were not informed that the study involved placebo. There were 97 single-center trials and 33 multicenter trials (unclear in 56 studies). Explicit trial characteristics are presented in Additional file 3 .
Summary of trial characteristics
a Conditions investigated in less than three trials, b Outcomes reported in less than three trials
Risk of bias within studies
Methodological characteristics were assessed by Hróbjartsson and Gøtzsche [ 14 ] and are listed in detail in the original Cochrane review. In summary, adequate sequence generation was reported in 39 trials (21%); 28 trials (15%) reported adequate concealment of allocation; 59 trials (32%) were judged to have adequate blinding of patients and providers; and 81 trials (44%) to have adequate blinding of outcome assessor. Overall, 81 trials (44%) had a sample size of at least 50 patients, and 80 trials (43%) had a dropout rate of 15% or lower. Following the simple risk-of-bias assessment proposed in the Cochrane review, 16 trials [ 70 – 85 ] (9%) fulfilled all three criteria and were therefore judged as having low risk of bias among the included trials.
Proportional contextual effect
As illustrated in Additional file 4 , there was a considerable degree of heterogeneity across PCE’s from various trials ( I 2 =93.6). The pooled PCE across all 186 trials corresponded to 54% of the observed effect (0.54, 95%CI 0.46 to 0.64). There was statistically significantly higher PCE in trials with pharmacological and physical interventions, compared to psychological, and PCE was non-significantly higher for patient-involved outcomes (patient-reported outcomes and observer-reported outcomes dependent on patient cooperation). Trials where patients were not informed that the study involved placebo had non-significantly higher PCE. Trials with binary outcomes had statistically non-significant, but potentially higher PCE’s compared to trials with continuous outcomes. None of these factors, however, explained much of the observed heterogeneity. Publication year and sample size did not have any effect on heterogeneity either, and no association was found with PCE (see Additional file 5 ). There was a significant association between PCE and mean age and percentage of females, where PCE decreased with higher mean age of participants (slope = 0.986; 95%CI 0.976 to 0.995) and increased with higher percentage of females (slope = 1.006; 95%CI 1.000 to 1.012). However, only the proportion of females in the trial populations seemed to explain some of the between-study variance (reduction in τ 2 = 14.1%, I 2 = 92.5); see Table Table2 2 .
Results from random-effect meta-analysis and meta-regression analysis
k , number of trials; n , number of patients analyzed; τ 2 , estimate of between-study variance; I 2 , variation in PCE attributable to heterogeneity, estimated by random-effect subgroup analysis
a Sample size analyzed by dividing the trials in two groups, 70.5 (the median) being the cut-point
Neither patient’s condition/diagnosis (e.g., fibromyalgia, cancer, smoking; increase in τ 2 = 4.5%, I 2 = 93.8) nor outcome domain (e.g., pain, nausea, smoking cessation; increase in τ 2 = 0.7%, I 2 = 93.5) was an important factor in reducing the between-study variance. However, whether the condition was chronic or not reduced between-study variance slightly (slope = 0.69; 95%CI 0.49 to 0.96; reduction in τ 2 = 3.0%); chronic conditions had a significantly lower PCE.
Proportional contextual effects and placebo effect
As illustrated in Fig. Fig.3, 3 , there was an association between PCE and the corresponding placebo effect, estimated as SMD and reported in the Cochrane review [ 14 ], where PCE increased with an increasing placebo effect (slope = 1.55; 95%CI 1.07 to 2.24).

Meta-regression plot illustrating the association between the PCE and the placebo effect (SMD). Larger SMD indicates a larger placebo effect. ( k = 186). PCE, proportional contextual effect; SMD, standardized mean difference
Risk of bias across studies
The risk of small-study bias across trials was assessed using funnel plot, followed by Egger’s test [ 22 ]. The funnel plot was asymmetrical (Fig. (Fig.4), 4 ), which Egger’s test confirmed ( p < 0.001). Small studies tended to show smaller PCE. Furthermore, the funnel plot showed a vertical line of points (ln[PCE] = − 4.6) that indicated the trials where either the intervention group or the placebo group showed no improvement or got worse, requiring adjustment in the form of the described imputation of a small treatment effect. For sensitivity, when comparing the fixed-effect estimate for the PCE to the random-effects estimate, the PCE increased from 0.54 (95%CI 0.46 to 0.64) to 0.82 (95%CI 0.80 to 0.83). This indicates a discrepancy between random and fixed-effect models. However, a subsequent visual inspection of the funnel plot did not indicate an important small study bias.

Funnel plot of all trials included in the main meta-analysis ( k = 186). The vertical line shows the average effect size. The median SE(ln[PCE]) is 0.27; i.e., SE(ln[PCE]) larger than this are “smaller studies.” PCE, proportional contextual effect
When conducting the pre-specified sensitivity analyses according to the risk-of-bias assessment, the blinding of the outcome assessor was the most important factor, being associated with a significantly higher PCE. Furthermore, the PCE was significantly higher in trials with concealed allocation, and there was a non-significant increased PCE in trials with an overall low risk of bias. Neither trial setting (single-center or multicenter) nor sample size had an important effect on PCE or explained much of the reported heterogeneity, with blinding of outcome assessor apparently being the most important factor (reduction in τ 2 = 5.8%).
What influenced the heterogeneity the most were the 18 trials [ 41 , 46 , 48 , 86 – 100 ] (including 890 patients) where either the intervention or placebo group showed no improvement or got worse; these trials required imputing a very small treatment effect in order to calculate PCE so they could be included in the meta-analysis (reduction in τ 2 = 80.6%, I 2 = 84.4). Due to this imputation technique, a sensitivity analysis was performed where the aforementioned 18 trials were excluded.
Sensitivity analysis
A meta-analysis of the remaining 168 studies (total of 15,765 patients) resulted in PCE of 0.72 (95%CI 0.67 to 0.79), τ 2 = 0.2080, and a slightly lower heterogeneity ( I 2 = 85.7). Many of the trends apparent in the main analysis (e.g., higher PCE in trials with concealed allocation) diminished or disappeared (see Additional file 6 ). Ten of the 18 excluded trials had psychological interventions [ 87 – 90 , 92 , 93 , 95 , 97 , 99 , 100 ], which led to a radical shift in PCE for the remaining trials with psychological interventions. This shift in PCE, in turn, led to a non-significantly higher PCE in trials with physical interventions compared to pharmacological and psychological interventions. The PCE was still higher in trials with concealed allocation, blinded outcome assessor, and overall low risk of bias, but only the blinding of outcome assessor resulted in significantly higher PCE (slope = 1.22; 95%CI 1.03 to 1.44). The significantly higher PCE in trials of non-chronic conditions diminished and was no longer significant. None of these factors were important in explaining the between-study variance. Neither sample size, publication year, whether trials had binary or continuous outcomes, mean age of participants, nor percentage of females seemed to have any effect on heterogeneity, and no association was found with PCE in the sensitivity analyses.
For the sensitivity analysis of the association between PCE and placebo effect, an additional five trials with binary outcomes were excluded because in these trials, no improvement was seen in the no-treatment group. In order to be eligible for inclusion in the synthesis, these trials needed adjustment (0.5 added to each cell of the 2 × 2 table) prior to calculating the OR, and afterwards SMD. The association found earlier between PCE and placebo effect diminished and was non-significant after the exclusion of all 23 trials that needed adjustment. The association between PCE and placebo effect (SMD) for the sensitivity analysis is illustrated in Fig. Fig.5 5 .

Meta-regression plot. Meta-regression plot, illustrating association between the PCE and the placebo effect (SMD) after exclusion of the 23 trials that needed adjustment prior to analysis. Larger SMD indicates a larger placebo effect ( k = 163). PCE, proportional contextual effect
A new funnel plot and Egger’s test was conducted for the remaining 163 trials in the sensitivity analysis, which also resulted in an asymmetrical funnel plot (Additional file 7 ; Egger´s test, p < 0.001), suggesting that small studies report smaller PCE.
Summary of the evidence
This study shows, based on 186 trials, that the majority (average PCE: 54%) of the overall treatment effect of diverse interventions across conditions was attributable to contextual effects. Several contextual factors and trial characteristics were found to impact the observed variation in PCE. The factors that increased the PCE, and thus are potentially valuable, were adequate allocation concealment, blinded outcome assessor, lower mean age of participants, higher proportion of females, larger placebo effect, and trials of non-chronic conditions. As anticipated the PCE was higher in trials with low risk of bias and using patient-reported outcomes. The proportion of females was the only pre-specified covariate that significantly reduced the between-study variance; other factors had only minimal impact on the between-study variance.
The sensitivity analysis showed insignificant variation of PCE for the most part; only blinding of outcome assessor remained significant. No factors were found to significantly reduce the between-study variance, and no association was found between PCE and mean age, percentage of females, or placebo effects, respectively.
Our findings support those reported in earlier PCE study by Zou et al. [ 12 ], who also found larger PCE in studies with concealed allocation. Neither study could explain, however, whether this is due to greater contextual effects or smaller experimental (intervention group) effects. Also, our findings support those found by Whiteside et al. [ 19 ], who reported an increase in PCE with higher proportions of females. There are, however, two crucial differences between this study and previous PCE studies. First, the two previous studies examined a single condition, (osteoarthritis [ 12 ] and fibromyalgia [ 19 ]), where the primary outcome was pain. Second, both studies excluded trials where one or more groups did not improve or even worsened. Due to these exclusions, it might be more appropriate to compare the findings from the sensitivity analysis in this study to the previous PCE studies. The overall PCEs reported in previous studies are similar to the findings from the present sensitivity analysis (75% in osteoarthritis and 60% in fibromyalgia compared to 72% in the present study). Zou et al. [ 12 ] reported the lowest PCE for treatments delivered with oral medications and higher PCE for treatments delivered via physical means and needles/injections. In the present sensitivity analysis, there was also insignificantly higher PCE in physical interventions compared to pharmacological. The categories in this study, however, cover a broader range of interventions than the categories used by Zou et al. [ 12 ]; nevertheless, our study generally corroborates earlier findings. We found that there was a direct association between PCE and the placebo effect reported in the Cochrane review [ 14 ]. This association, however, was not apparent in the sensitivity analysis, meaning that the PCE was constant even though the placebo effect diminished.
There are several similarities between the findings in the Cochrane review [ 14 ] and the findings in this study. The Cochrane review reported a larger placebo effect in trials with patient-reported outcomes, in trials where patients were falsely informed that no placebo was involved in the study, and in trials with concealed allocation. Similar results were seen in the sensitivity analysis in the present study, where trials with patient-reported outcomes suggested a higher PCE compared to outcomes not dependent on patient involvement. Trials with concealed allocation had significantly higher PCE in the main analysis but not in the sensitivity analysis. These similarities between PCE and placebo effect reported in the Cochrane review indicate that the difference between the improvements seen in the intervention and placebo groups is less prominent in studies with a large placebo effect.
Limitations
A major limitation of this study was the inclusion of studies with high risk of bias, mainly due to lack of sequence generation and allocation concealment in 79% and 85% of trials, respectively. Only 16 trials (9%) out of 186 fulfilled all three predefined criteria for low risk of bias. An updated literature search may have added more studies at low risk of bias, as the majority of the included trials did not describe factors such as sequence generation and allocation concealment adequately. Since there were trends for higher PCE in trials with a low risk of bias this could have affected the outcomes. Furthermore, the meta-analytic methods used did not allow for patient-and clinical-related variables changes over time. Many changes in the patient populations could be experienced in 47 years.
This study correlates with previous studies of bias, reporting that bias due to unblinded assessor or inadequate allocation concealment tends to overestimate treatment effect [ 101 – 103 ]. Furthermore, a small-study bias was detected, whereby small-sample studies report smaller PCE. Based on the presence of small-study bias, and that studies with a lower risk of bias tended to have higher PCE, the PCE reported in this study could have been underestimated.
As described above, there was a considerably high degree of heterogeneity, which may have affected our study’s outcomes. Although subgroup analyses were carried out, the heterogeneity remained high, and no factors were able to explain the heterogeneity, except for the 18 trials that needed adjustment prior to analysis. Furthermore, not all the important contextual factors were included in the model. This could lead to uncontrolled confounding in the meta-analytic study, leading to biased effect estimates. Thus, the conclusions claimed in this article could be incorrect. A more in-depth sensitivity analysis would have been helpful to assess the magnitude of these biases. However, many contextual factors that are known to be important, such as the patient-provider interaction and patient’s expectations, were not considered because these factors are rarely reported in RCTs.
In contrast to earlier PCE studies, we decided to also include trials where either the intervention or placebo group showed no improvement or even worsened (i.e., where the PCE became negative). That decision might have been both a strength and a limitation. Earlier PCE studies excluded such trials because the measure of PCE does not allow negative values when the ratio is log-transformed, and worsening in a group could indicate side/nocebo effect, which is not the focus of interest for the PCE [ 12 ]. However, we viewed the exclusion of studies that did not fit to the pre-specified hypothesis as a source of bias; we therefore decided to add a miniscule effect to the groups that had not improved, in order to be able to perform the necessary calculations. This was the case for 17 trials with continuous outcomes and one trial with binary outcomes. Afterwards, it was clear these 18 trials had great impact on the results, and it was questionable whether this kind of adjustment was optimal for retaining the trials in our study.
This study suggests that at least half of the overall treatment effect observed in clinical trials across conditions is attributable to contextual effects rather than to the specific experimental intervention on trial. Factors such as blinding of outcome assessor, concealed allocation, lower mean age, and higher proportion of females had the most impact on the PCE. This analysis in our study did not include all known important contextual factors (e.g., patient’s expectations), so the true proportion of contextual effects could have been underestimated. The association between PCE and methodological quality (lower risk of bias) indicates that the true PCE may be higher than estimated. These findings highlight the importance of contextual effects in clinical practice and their large impact on patient care. In reporting of RCTs, it is important to not only focus on the net benefit of a treatment but also to consider the PCE when translating findings from clinical trials to clinical practice. The findings could encourage health care professionals to consider potentially modifiable contextual factors, such as their patient-provider interaction, in any attempt to enhance the overall treatment response to interventions. Contextual effects are important contributors to the overall treatment effect and should be embraced in both clinical trials and practice for their potential benefits to patients.
Acknowledgements
Not applicable.
Data sharing
The dataset has been published as additional information (Additional file 8 ).
Abbreviations
Authors’ contributions.
SHH and RC conceptualized the study. All authors participated in the development and design of the study. SHH, CBJ, and RC performed the statistical analysis and interpretation of data. SHH and RC drafted the manuscript. All authors were substantially involved in the critical revision of the manuscript. All authors read and approved the final version of the manuscript.
We would like to acknowledge the support that the Parker Institute, Bispebjerg and Frederiksberg Hospital receives via a core grant from the Oak Foundation (OCAY-18-774-OFIL): the Oak Foundation commits its resources to address issues of global, social and environmental concern, particularly those that have a major impact on the lives of the disadvantaged. The Oak Foundation had no role in the study design, data collection and analysis, or preparation of the manuscript.
Availability of data and materials
Declarations.
The authors declare that they have no competing interests.
Publisher’s Note
Springer Nature remains neutral with regard to jurisdictional claims in published maps and institutional affiliations.
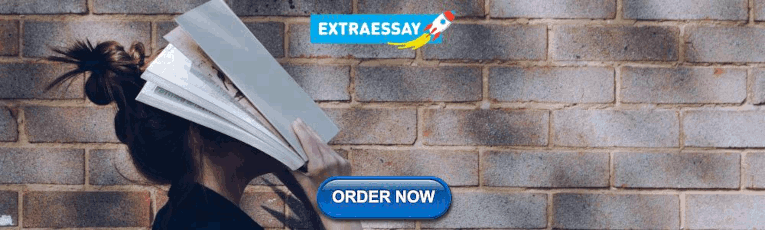
IMAGES
VIDEO
COMMENTS
Understanding placebo and nocebo effects is important for both clinicians and neuroscientists. Placebo responses are substantial across diverse clinical disorders 2-4 and, in some cases, are related to objective pathology 5 and survival 6.A large part of the overall therapeutic response to drugs 7-10, surgery 11,12, psychotherapy 13 and other treatments may be due to the treatment context ...
It is becoming clear that any treatment is significantly modulated by placebo effects in clinical settings. Placebo effects are positive outcomes that are attributable to the psychosocial context and individual treatment expectations rather than the action of the medication or intervention (Colloca & Benedetti, 2005).Placebo effects also occur when placebos are given following the ...
The use of placebo in clinical trials is fundamental, although the ethics of its use is under discussion. The placebo may behave like a drug from the pharmacokinetic and pharmacodynamic point of view and can also be associated with adverse events (nocebo effect). Placebo can modify treatment by increasing or decreasing the effects of drugs.
Placebo and nocebo effects are essential components of clinical practice and efficacy research [].They occur in both experimental and clinical contexts when a pure inert treatment is administered ...
The placebo effect is the beneficial outcome caused by a treatment that is not attributable to the pharmacological properties of the treament itself. This effect occurs if, for example ...
Neurobiologic Mechanisms of Placebo and Nocebo Effects. Placebo effects have been shown to be associated with the release of substances such as endogenous opioids, 13,14 endocannabinoids, 15 ...
Placebos have been the subject of many studies in the last two decades 1 and the number of clinical trials to examine a placebo treatment as the primary intervention is rapidly growing 2.Research ...
The placebo effect is a beneficial health outcome resulting from a person's anticipation that an intervention will help. How a health care provider interacts with a patient also may bring about a positive response that's independent of any specific treatment. Research supported by NCCIH has explored several aspects of the placebo effect.
Introduction. A substantial amount of literature has demonstrated the significant role of placebo effects and their positive influence on treatment outcomes [1-4].Placebo effects refer to the beneficial effects after administration of an inert treatment or as an additive effect upon active treatments [].The underlying mechanisms involved in placebo effects have become increasingly well ...
Placebo effect examples. The placebo effect illustrates how the mind can trigger changes in the body. Example: The power of suggestion. In a study, participants are given a placebo but are told it's a stimulant. While talking about the "medication," researchers are convincing and positive about the expected results.
Several research studies have demonstrated the placebo effect's role as a powerful determinant of health in certain disease conditions. Migraines, joint pain, arthritis, asthma, high blood pressure, and depression are some disease conditions that are more sensitive to the placebo effect. The placebo effect is a complex phenomenon with several ...
The placebo effect works by turning on the body's natural mechanisms for helping us feel better. Our brains make many substances that can lessen pain, stress, anxiety, and other unpleasant feelings. ... Help Advance Placebo Research. You may be able to help scientists learn more about the placebo effect: To participate in a clinical trial, ...
Real-life placebo effects. Today, scientists define these so-called placebo effects as the positive outcomes that cannot be scientifically explained by the physical effects of the treatment. Research suggests that the placebo effect is caused by positive expectations, the provider-patient relationship and the rituals around receiving medical care.
To better understand the neurochemical mechanisms underlying the placebo effect, a team led by Dr. Jon-Kar Zubieta, formerly at the University of Michigan School of Medicine and now at the University of Utah, examined such effects in depression treatment. The study was funded in part by NIH's National Institute of Mental Health (NIMH).
The placebo is designed to seem exactly like the real treatment, yet the substance has no actual effect on the condition it purports to treat. The placebo effect is much more than just positive thinking, however. When this occurs, many people have no idea they are responding to what is essentially a sugar pill.
The placebo effect is a complex phenomenon that can be described from neurobiological, psychosocial, and epistemological perspectives. Different leaders in the field have proposed multiple theories and models that attempt to describe both the nature and the mechanisms of action underlying placebo effects. ... However, most of the research has ...
Countless studies include the use of placebos. Some studies that have shown placebo effects include: Advertisement. Chronic pain. Nausea. Sleep conditions. Depression and other mood disorders. One ...
As Benedetti (2009, p.75) notes, mechanistic research on the placebo effect, beginning with experiments in the late 1970s indicating that placebo analgesia is mediated by release of endogenous opioids, gave "scientific credibility to the placebo phenomenon by unraveling the underlying biological mechanisms." This scientific credibility is ...
The Placebo Effect in Medicine The placebo effect has been studied in various medical fields, from pain management to depression treatment. In many cases, it has shown that believing in a ...
Background. Contextual effects (i.e., placebo response) refer to all health changes resulting from administering an apparently inactive treatment.In a randomized clinical trial (RCT), the overall treatment effect (i.e., the post-treatment effect in the intervention group) can be regarded as the true effect of the intervention plus the impact of contextual effects.