radiologic technology Recently Published Documents
Total documents.
- Latest Documents
- Most Cited Documents
- Contributed Authors
- Related Sources
- Related Keywords
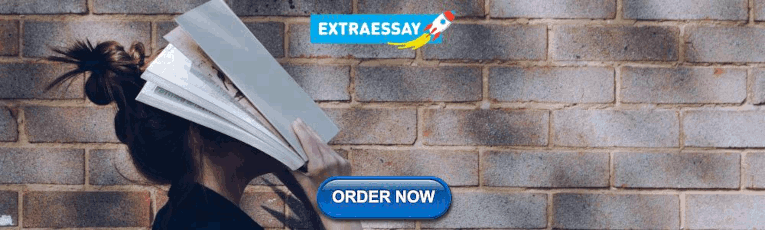
Study Habits and Their Effects with the Academic Performance of Bachelor of Science in Radiologic Technology Students
This study aimed at investigating the effects of students' study habits on their academic performance in professional and general education subjects. To attain this aim, the researcher used a sample of thirty-two (32) students from the Bachelor of Science in Radiologic Technology program under the College of Allied Medical Sciences for the academic year 2019–2020 of a university in Bulacan. The researcher used the descriptive-correlational method of research, which utilized a standardized questionnaire as the primary data gathering technique. Results of the regression analysis indicate that all eight (8) variables of study habits are correlated with academic performance (professional subjects and general education subjects) of the students to a varying extent, as shown by the non-zero B coefficients. The results of the analysis of variance of the regression of study habits on the academic performance of the students revealed an F ratio of 0.939 and 0.900 with an associate probability equal to 0.505 and 0.533, respectively. Since the p-values are greater than alpha, the null hypothesis (Ho) cannot be rejected. It may be safely concluded that the study habits of the students did not produce significant combined effects on the academic performance of the students. Conclusions were drawn, and recommendations were offered.
New solutions for automated image recognition and identification: challenges to radiologic technology and forensic pathology
Predictors of success on the credentialing examination in radiography for first- and non-first-generation students.
Background: Identifying predictors of student success is fundamental across higher education in the United States, particularly for historically underserved first-generation students. In radiologic technology programs, the literature suggests that variables prior to and during matriculation in these programs affects scores on the American Registry of Radiologic Technologists (ARRT) credentialing examination in Radiography. However, the evidence in this area has not considered the educational patterns for first-generation students. Purpose: This study sought to improve our understanding about how select student background characteristics and experiences prior to and during the years enrolled in radiologic technology programs accredited by the Joint Review Committee on Education in Radiologic Technology (JRCERT) affect scores on the ARRT credentialing examination in radiography, especially for first-generation students. Method: The researchers surveyed graduates from radiologic technology programs in 2018 and 2019 who attempted the radiography credentialing examination in these two years. Results: A total of 286 cases were included in the analysis, which revealed different patterns and effects of predictor variables on credentialing examination scores for first- and non-first-generation students. Whereas 10 variables prior to and during matriculation affected examination scores for first-generation students, only 8 did for their non-first-generation peers. Conclusion: Identifying predictors of success in radiologic technology programs helps professionals in these programs design environments that provide opportunities for students to enhance their chances to be successful on the Radiography exam, especially first-generation students.
Radiologic Technology Clinical Manual
A framework for predicting radiologic physics achievement among radiologic technology students.
Radiologic Physics is one of the most challenging professional subjects in the Radiologic Technology (RT) field. It encompasses a wide range of physics concept, calculations, and real-life imaging practices. As observed, most of the students failed in this subject, leading to students dropping out early in the course. To circumvent this daunting issue, a framework for predicting the subject’s achievement should be developed using various tenets of learning strategies and management. This study aims to explore a framework that can predict Radiologic Physics achievement among RT students. Subjects were 954 Radiologic Physics students (480 males and 474 females) randomly selected from 12 Radiologic Technology schools in the Philippines. Their ages ranged from 18 to 22 years (mean age 19.5, SD 2.4). Seven instruments were used to collect data for the study: Physics Learning Strategies Scale, Inventory of Students Attitude Towards Radiologic Physics; Class Involvement Scale; Teacher-Directed Activities Scale; Parental Influence towards Academic Success Scale, and Radiologic Physics Achievement Test. Path analysis was utilized to identify the best fitting framework. The best fitting framework explained 92% of Radiologic Physics achievement variance. Cognitive and metacognitive learning strategies, attitudes towards Radiologic Physics, class involvement, teacher-directed activities, and parental influence exerted a positive effect on Radiologic Physics achievement. Teacher-directed activities, parental influence, and attitudes towards the subject had a positive impact towards cognitive learning strategies. Moreover, teacher-directed activities and parental influence exerted a positive effect on class involvement while parental influence had a positive impact on metacognitive learning strategies. Teacher-direct activities, attitudes towards the subject, and parental influence contributed indirectly to achievement via cognitive learning strategies. Teacher-directed activities contributed indirectly to achievement via class involvement. Finally, parental influence contributed indirectly to achievement via metacognitive learning strategies and class involvement. A framework for predicting Radiologic Physics achievement among RT students could be used to understand the performance of students and innovate learning strategies in the RT education.
Structural Models of Self-Efficacy of Filipino Radiologic Technology Educators, Current Learners, and Prospective Students in the Senior High School
This study aims to explore three different structural models of self-efficacy for Filipino Radiologic Technology educators, current learners, and prospective students in the senior high school. Subjects were 256 Radiologic Technology educators (102 males and 154 females) and 2,451 Radiologic Technology students (1,525 males and 926 females), randomly selected from 22 Radiologic Technology schools in the Philippines. A total of 4,263 prospective Radiologic Technology students from the 30 senior high schools in the Philippines were also sampled as respondents. Six instruments were used to measure help-seeking, self-esteem, social support, motivation, self-regulation, and self-efficacy. Path analysis was utilized to identify the best fitting model. The best fit models of self-efficacy for Filipino Radiologic Technology educators and learners showed that help-seeking, self-esteem, social support, motivation, and self-regulation positively influenced self-efficacy, with social support exerting the greatest causal effect. The best fit model of self-efficacy for prospective Radiologic Technology students from senior high school reported that social support completely mediates the effects of help-seeking, self-esteem, motivation, and self-regulation on self-efficacy. The study highlights different results that could be arrived depending on whether future researchers decide to use the self-efficacy models for Filipino Radiologic Technology educators, current learners, and prospective students in the senior high school. The provision of highly supportive environment for Radiologic Technology educators, current learners, and prospective students in the senior high school is essential to increase self-efficacy, thereby improving their capabilities to hurdle the challenges in the academic milieu.
National Radiologic Technology Licensure Examination Performance: Predicting Success using Discriminant Analysis
This study was designed to identify variables that might be used as predictors for success on the national Radiologic Technology licensure examination. The census sample consisted of 2,036 graduates of a baccalaureate Radiologic Technology program in 2016, 2017, and 2018 from 24 higher education institutions (HEIs) in the Philippines. The investigators examined 12 variables to determine their predictive value for the national Radiologic Technology licensure examination success. Grades in all year levels of Radiologic Technology course were the four best predictors. Results of the discriminant analysis identified seven significant predictor variables leading to successful classification of 99.9 percent of all the passing graduates and 99.8 percent of the failing graduates in the national Radiologic Technology licensure examination. The use of this discriminant function to identify high-risk students has the advantage of early identification of failing. The large amount of 92 percent variance in the national RT licensure examination accounted for in this study may substantiate the claim of high accuracy of the discriminant function used. This is the first study to discriminate passing from failing graduates in the national RT licensure examination based on the selected predictor variables and the astounding precision of classifying graduates is a remarkable result for HEIs included in the analysis.
Adjustment to college and academic performance: Insights from Filipino college freshmen in an allied health science course
This paper aims to know the relationship between the level of adjustment to college and academic performance of first year Radiologic Technology students of a higher education institution in the Philippines. A descriptive-correlational study using survey questionnaire was employed to 132 respondents who were chosen through stratified random sampling and Slovin’s formula. Standard questionnaires were used to gather data on the demographic profile and level of adjustment of the respondents while the academic performance was measured through the Weighted Point Average (WPA) requested from the school’s Registrar. Results showed that the majority of the respondents are female (53.8%), belong to middle income class (34.8%), were from STEM (59.1%) and travel between one kilometer and 10 kilometers to school (34.1%). The study reported a moderate level of adjustment and a 2.63 overall WPA of students. Test of difference showed that there is significant difference in the academic adjustment and academic strand taken during SHS (p<0.05); and in the institutional attachment and proximity of house to school (p<0.05). Bivariate correlation among variables revealed that there is no significant relationship between the level of adjustment to college and academic performance of first year Radiologic Technology students College (p>0.05).
Interprofessional Education Perceptions of Dental Assisting and Radiologic Technology Students Following a Live Patient Experience
Export citation format, share document.
- Open supplemental data
- Reference Manager
- Simple TEXT file
People also looked at
Review article, artificial intelligence for the future radiology diagnostic service.
- Arlington Innovation Center:Health Research, Virginia Tech-Washington DC Area, Arlington, VA, United States
Radiology historically has been a leader of digital transformation in healthcare. The introduction of digital imaging systems, picture archiving and communication systems (PACS), and teleradiology transformed radiology services over the past 30 years. Radiology is again at the crossroad for the next generation of transformation, possibly evolving as a one-stop integrated diagnostic service. Artificial intelligence and machine learning promise to offer radiology new powerful new digital tools to facilitate the next transformation. The radiology community has been developing computer-aided diagnosis (CAD) tools based on machine learning (ML) over the past 20 years. Among various AI techniques, deep-learning convolutional neural networks (CNN) and its variants have been widely used in medical image pattern recognition. Since the 1990s, many CAD tools and products have been developed. However, clinical adoption has been slow due to a lack of substantial clinical advantages, difficulties integrating into existing workflow, and uncertain business models. This paper proposes three pathways for AI's role in radiology beyond current CNN based capabilities 1) improve the performance of CAD, 2) improve the productivity of radiology service by AI-assisted workflow, and 3) develop radiomics that integrate the data from radiology, pathology, and genomics to facilitate the emergence of a new integrated diagnostic service.
Introduction
Radiology was one of the first specialty in healthcare to adopt digital technology. Since the 1970s, radiology has adopted many new digital imaging modalities such as Computed Tomography (CT), Magnetic Resonance Imaging (MRI), Positron Emission Tomography (PET), Computed Radiography (CR), Single Photon Emission Computed Tomography (SPECT), Digital Ultrasound, Digital Mammography and many others. These digital images were initially printed on films for interpretation, sharing, and archiving. As digital technologies for data capture, data storage, image display, and transmission improved, radiology operations began to convert to a filmless digital environment in the late '90s ( Mun et al., 1993 ; Mun et al., 2007 ). Today, x-ray films are gone, and the PACS manages all radiological images ( Alhajeri et al., 2017 ). This massive investment in digital technology transformed the radiology service and made radiology images ubiquitous throughout all aspects of healthcare ( Hricak, 2018 ). Digital radiological images enabled the development of many new image-guided surgeries and radiation oncology. Radiology became global as teleradiology was the first successful telemedicine application globally ( Mun et al., 1998 ; Mun and Turner, 1999 ). Teleradiology, often globally, is a significant portion of radiology operations in the US. Radiology services accumulate massive digital images in their archives, some in the cloud, that laid a technological and human infrastructure for the next digital transformation based on machine learning (ML) and artificial intelligence (AI).
During the mid-'80s, the radiology community began to explore computer aided diagnosis (CAD) as a possible to aid radiologists ( Doi, 2007 ). Since the mid-2010s, there has an overwhelming interest in machine learning techniques in almost all fields involving data classification or analysis. The number of publications using ML has been exponentially increasing from a few thousand per year in the early 2000s to about 35,000 per year in 2018; and nearly 85% were in neural networks, based on Scopus data ( Perrault et al., 2019 ). Several versions of the neural network technique also were used for drug discovery, computational biology, quantum chemistry, autonomous cars, geology, astronomy, and many others.
Many CAD tools were developed in radiology community, with good performance in terms of sensitivity and specificity. However, most of them remained in research labs, and they did not become an integral part of the radiology service. An earlier success in the use of CAD in digital mammography for breast cancer screening generated much excitement in the community for wider clinical adoption of CAD tools. Some speculated that these intelligent systems would soon replace radiologists.
The National Science and Technology Council of the US published a research and development roadmap for medical imaging ( Interagency Working Group on Medical Imaging, Committee on Science, and National Science and Technology Council, 2017 ). The report envisions changes in medical imaging in 4 general areas; 1) patient referral to imaging service, 2) development and use of high-value imaging capability, 3) use of advanced computation and machine learning, and 4) promoting best practice in medical imaging including reorganizing workflows to improve productivity.
In the article, we discuss AI research in medical imaging from a clinical adoption perspective for patient care and suggest several pathways through which AI will be demanded by radiology as it undergoes the next generation digital transformation toward integrated diagnostic service.
Operational Description of Radiology Service
Radiology service is a very complex operation that includes many inefficiencies. There is a great need to improve overall workflow and productivity. The radiology department provides clinical services to referring physicians and patients by managing a complex workflow involving many layers of people, various technology, many types of time-sensitive information. On any given day, a typical radiology department will conduct more than 50 different types of imaging studies covering all body parts using dozens of imaging modalities, including CT, MRI, ultrasound, nuclear medicine, positron emission tomography, and various conventional radiography systems. The acquired images are managed by a picture archiving and communication system (PACS) and radiology information system (RIS) ( Boochever, 2004 ). Orders for imaging studies from the referring physicians are placed by referring physicians based on the patient's medical history and symptoms. A radiologist determines imaging protocol suitable to address the clinical question. When an imaging study is completed at the imaging system, the PACS will collect all images and generate a worklist for each radiologist based on departmental policies/procedures and the radiologist's specialization ( Hricak, 2018 ).
The radiologist's work has three parts: interpreting and analyzing images, generating reports, and providing further consultation for referring physicians and patients ( Halsted and Froehle, 2008 ). In academic departments, training residents and fellows is also a significant responsibility. The interpretation (reading) time varies greatly depending on the types of study. The radiologists are highly skilled and very fast at detecting abnormalities in the image ( Forsberg et al., 2017 ). The reading times in radiology has been steadily increasing. The imaging devices have improved, and they generate increasingly more images per study. For example, the average number of images for a CT exam increased from 82 images in 1999 to 679 images in 2010. For MRI, the numbers increased from 164 images to 570 images, respectively ( McDonald et al., 2015 ). The image interpretation often requires examining previous studies and comparing them to the current study to determine if the patient has gotten better or worse. These comparison analyses are carried out manually by a radiologist, which can be very time-consuming ( Schemmel et al., 2016 ; Doshi et al., 2018 ).
Once the radiologist has completed their interpretation of the study, the results are generally recorded on a voice recognition system (a component of the PACS/RIS) to generate written reports that become part of the patient record ( Boochever, 2004 ). In some cases, the report is sufficient by itself. In other cases, the report becomes part of a more complex analysis ( Kahn et al., 2009 ). For example, a patient might have multiple radiology exams, blood chemistry analysis, and tissue sampling, all of which need to be combined to create a comprehensive diagnosis.
Most of the previous efforts to apply AI to radiology in terms of CAD so far have focused exclusively on the interpretation of single images or a single series of images. In comparison, this is an important task, only a fraction of how a typical radiologist spends their time. When the image's reading/analysis can become a part of subsequent decision-making, radiologists would participate in the decision making beyond the reports ( Brady, 2017 ). In essence, AI in radiology has historically tackled challenging yet narrow problems ( Schemmel et al., 2016 ; Hosny et al., 2018 ). We argue that a refocusing of AI onto different aspects of the radiology workflow and medical error reduction will generated more demand and adoption by radiology community. ( Dikici et al., 2020 ; Montagnon et al., 2020 ).
Clinical Adoption Case Study: Breast Cancer Screening and Diabetic Retinopathy
One has to distinguish between CAD, CADx, and CADe, a family of AI tools based on convolution neural network (CNN). The concept of computed diagnosis (CAD) research in medical imaging has evolved into two distinct clinical applications; computer aided diagnosis (CADx) and computer aided detection (CADe). CADx means the computer provides a diagnosis for physician review. On the other hand, CADe means the computer highlights the area of concern (i.e., cancer) for further diagnostic evaluation without providing a diagnosis. The CADe is used to screen cancers such as lung cancer or breast cancer for the asymptomatic but higher risk population. If cancer is suspected from the screening study, the patients will undergo a higher precision diagnostic study. The CADe is a much more challenging problem compared with CADx.
We will review the clinical adoption experience of two different use of AI, breast cancer screening and diabetic retinopathy screening to highlight that clinical adoption is a multifaceted issue beyond technical success in laboratories.
CADe in Screening Mammography for Cancer Detection
Screening mammography is an ideal application for CADe because it has to review many cases by a limited number of radiologists trained in mammography. It is a single type of exam, and the most basic output is a simple yes (disease present) or no (disease absent). FDA approved the first CADe for mammography in 1998, but its adoption rate was initially less than 5% ( Lehman et al., 2015 ). However, as breast cancer screening became more popular as part of a government policy to promote women's health, there was a shortage of skilled radiologists for mammography. To address the shortage, which resulted from low reimbursement rates for reading mammograms, the Center for Medicare and Medicaid Services (CMS) allowed higher reimbursement rates for using CADe in screening mammography ( Gold et al., 2012 ). This financial incentive has dramatically increased the adoption of CADe for mammography. In fact, in the US today, most breast cancer screening mammograms are interpreted by radiologists with CADe assistance. It is highly unlikely that additional reimbursements would be allowed to use AI tools in radiology in the future.
The widespread use of mammography CADe and the large number of exams performed each year allowed assessment of CADe's impact on mammographic interpretations' accuracy. One of the largest, a study involving 271 radiologists and 323,973 women between Jan 2003 and Dec 2009, compared reading mammograms with and without CADe. The study concluded that CADe does not improve mammography's diagnostic accuracy ( Lehman et al., 2015 ).
A more recent study by Schaffter and colleagues conducted a crowd-sourced trial on the use of deep learning in digital mammography involving 300,000 mammograms from the US and Sweden. The project had 126 teams from 44 countries to see if they could meet or beat the radiologist’s performances. They concluded that AI tools again did not perform better than radiologists ( Schaffter et al., 2020 ).
CADe Use to Screen Diabetic Retinopathy to Prevent Blindness
Diabetic retinopathy is an eye disease when high blood sugar levels of a diabetic patient can cause damage to blood vessels in the retina. Undetected and untreated the patient can become blind. Screening for diabetic retinopathy is an effective way to prevent the blindness. A special camera takes images of the blood vessels in retina. Interpretation of these images requires special expertise, thus it has been a interests of AI community to develop CAD systems.
Recently Google Corporation deployed its AI tool to detect diabetic retinopathy in Thailand. Initial development and testing involved 3,049 patients. In 2018, they deployed the system at 11 clinics in Thailand, involving 7,600 patients ( Beede et al., 2020 ). This large-scale prospective study was halted mainly because of persistent image quality problems. The system performed poorly, mainly due to the variability of retinal scan images obtained at different nurses' different settings ( Beede et al., 2020 ). The success in the lab did not translate well in real-life situations. Initial deployment of an AI system for lung cancer screening experienced a similar situation in dealing with a considerable variability of image quality of real-life clinical cases ( Worrell, 2020 ). Pre-processing of image normalizing before AI application may be an essential step for scalable clinical deployment.
Currently, 46 AI algorithms have approvals from Food and Drug Administrations and Conformité Européenne (CE) ( Tadavarthi et al., 2020 ). The approval process consists of clinical trial demonstration clinical safety and efficacy, often involving receiver operating curve analysis of sensitivity and specificity. The approval, however, does not guarantee successful clinical adoption. Global adoption of these tools is still a few years away. Since there are no insurance reimbursements, except digital mammography in the US, for the use of AI software, return on investment must be assessed based on significant quality improvement or efficiency improvements. AI tools have not shown significant improvements in the quality of diagnosis and operational efficiency for cost savings. Such improvement may require significant changes in the radiology department functions and possible re-configurations of PACS often owned by vendors other than current AI vendors.
The Technology of CNN and Computer-Aided Diagnosis
Artificial intelligence (AI) is the capability of the machine to imitate intelligent human behavior. In contrast, machine learning (ML) is a subfield of AI that allows the machine to learn from data without being explicitly programmed ( Soffer et al., 2019 ). The concept of neural networks emerged from the biologic neuron system. A neural network in the visual cortex can detect the edges of an object seen by the retina. When the receptors' inner parts are activated simultaneously, the cell neutron integrates the signals and transmits an edge detection signal. An artificial neural network (ANN) is composed of interconnected artificial neurons. Each artificial neuron implements a simple classifier model, which outputs a decision signal based on a weighted sum of evidence, and an activation function integrates signals from the neurons. An ANN system can be built with thousands of these basic computing units. The system can be trained by computing these weights using a learning algorithm where pairs of input signals and desired output decisions are presented, mimicking brain functions. An individual artificial neuron is a simple neural network; however, multilayer perceptron can model complex nonlinear functions. The deep learning (DL) concept is based on the use of multilayer architecture of multilayer perceptron. In medical imaging, the number of layers tends to be in the range of dozen.
The convolution neural network (CNN) consists of a series of convolution layers equivalent to compositional convolution layers with a set of large kernels. In effect, a CNN acts as a feature learning based on spatial features with multiple channels ( Lo et al., 1995 ; Lo et al., 2018b ; Lo et al., 2018c ). However, common difficulties in traditional CNN approaches for medical imaging can be grouped into three categories; 1) inability to separate normal from ill-defined abnormal structures, 2) inability to differentiate disease patterns, particularly in subtle cases from a broad spectrum of normal structures, and 3) inability to establish an integrated system between compositional and divide-and-conquer models.
Limitation of Current Generic CNN
Many ML open-source packages such as Tensorflow, Keras, Caffe, and others featuring CNN have been widely used. The core algorithms of CNN in all these packages were designed for general image pattern recognition. They were initially developed for the recognition of alphanumerical handwriting. General image pattern recognition relied on essential graphic pattern features (e.g., edges) and orientation-dependent but size-independent in many situations. On the other hand, medical image pattern recognition should rely more on gray intensity distribution and is orientation independent but size-dependent. Also, some users have experienced inconsistent results from the current CNNs and have tried to use many versions converted from the same input as a part of the augmentation strategy to increase the training samples and stability ( Lo et al., 1998 ).
Improving CNN for Medical Imaging
An ordinary CNN using unconstrained kernel weights entirely based on the backpropagation training ( Lo et al., 2018d ). The use of rotational and translational versions of each input vector as data augmentation was developed by the authors ( Lo et al., 1993 ). However, many investigators reported that the current method requires a long training time and produces unstable results ( Lo et al., 2018b ; Lo et al., 2018c ).
The current CNN software should be redesigned for medical imaging pattern recognition by (i) the use of an activation function without suppression of the composed signal and (ii) the use of symmetric kernels. This is because current activation functions (Relu, Leaky Relu, sigmoid, and Tanh) used in general CNN tools are signal suppression functions (i.e., df/dx < 1). When using them multiple times through multiple convolutional layers, only edge patterns with very few gray value features remained in the feature maps at the end of convolution/activation processing for final classification. These are not acceptable intermediate outcomes for many medical images where subtle gray value differences are used for discerning possible disease characteristics ( Lo et al., 1995 ; Lo et al., 1998 ).
The symmetric kernels within CNN should be used to stabilize the CNN output consistency. The use of kernels with dihedral symmetry of order 8 (Dih4) is an example with a minimum number of free parameters as element coefficients are symmetric with respect to each corner wedges. In other words, elements on other wedges corresponding to the Dih4 symmetric element positions in the wedge would share the same value. The use of symmetric kernels can be expanded to wavelet decomposition. Though it is different from an ordinary convolution process, each compartment's biorthogonal kernels may be different. However, t the absolute value in each element of the kernel is the same. The multi-dimensional wavelet decomposition is made by a one-dimensional convolution process and down sampling one half at a time. The total number of free parameters is much less (the number of elements in 1D kernel plus 1, divided by 2). In effect, kernels to produce low-low (LL) and high-high (HH) compartments are Dih4 transformation-identical (TI) kernels. Kernels to produce low-high (LH) and high-low (HL) compartments are Dih4 TI with an odd number of elements but are anti-symmetric (i.e., 180 o rotation TI) with an even number of elements. Since each compartment is processed through an independent pipeline in the neural network process, for the latter situation, there is still room to make signals from LH and HL be Dih4 TI, if desired. This can be done by inserting a reflective symmetry kernel in each of these two compartment pipelines ( Lo et al., 2018d ).
With symmetric kernels, such as the dihedral symmetry of order 8, the intermediate results throughout all convolutional layers would be equivariant for original input and 90 o rotation increment as the flipping version. With this equivariant property on all convolutional layers, the CNN would produce identical output for all eight input image versions. In summary, the CNN can be treated as a whole function of an input vector Vi (i.e., a 2D image or 3D volume), and the output vector can be expressed as Vo = CNN(T[Vi]) = CNN(Vi) as long as T[K] = K within each of the CNN convolutional processes, where Vi and Vo are input and output vectors, respectively. K denotes the convolution kernel and T[.] is a transformation function. This equivariant property at all convolutional layers can be extended for the CNN to produce identical output results for any arbitrarily rotated images by merging the convolution processing before the classification section in the CNN. The use of symmetric kernels in the convolutional layers in the CNN would be a more appropriate tool to systematically produce highly stable results ( Lo et al., 2018d ).
Open Source CNN
There have been increasing concerns about the ethics, ability to explain and transparency of AI technology ( Tang et al., 2018 ; ESR, 2019 ), especially in healthcare. These concerns are partly due to difficulties in understanding underlying theories, methods, and assumptions used to generate systematic bias results. In this scenario, the use of open-source software (OSS) strategy could help address some of these concerns because, by definition, OSS offers greater transparency of the technology and opportunities for community-based collaboration.
The OSS concept started in the 1980s as a social movement and a philosophy for software development and distribution ( Levine and Prietula, 2014 ). OSS is defined as a software code made available under a legal license in which the copyright holder provides (depending upon the specific terms) various rights to the licensees to study, change, improve and re-distribute the code without any fees. Today there are many different types of OSS licenses depending on the copyright holders' interests and intentions ( Fosfuri et al., 2008 ; Opensource.org, 2020 ). These licenses range from permissive licenses such as Apache-2.0 to strongly protective licenses such as general public license (GPL). OSS is typically available as-is; however, it can be made into commercial products with additional services such as warranty, training, documentation, and maintenance under various commercial contracts.
Some of the more popular packages include TensorFlow, Keras, PyTorch, Caffe2, and many others. They all have varying strengths and weaknesses, depending on users' needs. Keras and TensorFlow have a common or similar core, but Keras is much easier to use with limited options than TensorFlow. PyTorch is fast and flexible for experimentation, and it is tightly integrated with the Python language. An extensive table of available software with detail comparison can be found at Wikipedia ( Multiple-Editor, 2020 ).
These OSS packages are developed and sponsored by various organizations and individuals for their use cases and applications other than medical imaging. However, the packages are initial starting platforms for imaging research. These open source packages should be optimized to be suitable for meaningful medical imaging research as discussed in the earlier section. Additionally, these open source codes' users should form or join collaborative communities based on shared medical imaging interests.
Application of CNN in Medical Imaging:
For research in supervised learning such as CNN, the success depends on three technical factors: 1) underlying science and technology of the code, 2) learning supervised by subject matter experts, and 3) the quantity/quality of data.
Another crucially important factor in CNN research is imaging expertise, both clinical and physic of imaging ( Giger et al., 2008 ). Unlike common everyday objects in AI research, the research team in medical imaging AI has to understand the clinical significance of images and imaging physic.
Availability of Data and Realistic Mix of Data
There are two data issues: access to a sufficient volume of data and enough data diversity representing a realistic case mix of the clinical operational environment ( Yamashita et al., 2018 ).
The imaging data requirement in radiology is relatively modest, less than 10,000 cases per disease category. In the case of the recent AI tool development for lung cancer screening with CT images, approximately 2,000 cases consisting of 300,000 CT images were sufficient for training, and approximately 300 cases of 45,000 images with about 20% subtle cases tested by more than 10 radiologists were sufficient for an FDA specified clinical trial ( Lo et al., 2018a ). For different disease types and imaging modalities, these numbers would be different. If the clinical problem to be addressed has many subtle features, the data volume required would be much higher.
For an AI algorithm to be clinically useful, it must be trained on data that appropriately represent the patient population's variance and diseases' presentation. In a routine data collection effort, the majority of available cases show disease patterns, which are considered relatively easy cases. The cases of subtle disease patterns are relatively rare and thus challenging to collect. It is essential to have a mix of subtle cases in the image archive. If one has a disproportionately large number of similar or easy cases, the system will show bias ( Lo et al., 1995 ; Hosny et al., 2018 ). In supervised learning, algorithms such as CNN learn from labeled data. When the number of categories and/or patterns to be differentiated increases, the required data volumes would increase. The problem of having more dimensions, yet small data volume, can result in overfitting contributing to low generalizability and scalability ( Yamashita et al., 2018 ; Mutasa et al., 2020 ).
Quality of Data: Image Quality
The performance of current CNN is fragile, dealing with varying image quality. In fact, in any data science project, one can spend a significant amount of effort to "clean" the data. The same is true in imaging. The data must be of sufficient quality and acquired with uniform parameters to make certain that conclusions can be validated. The image quality can vary depending on the time and day of imaging, image protocol, imaging system set up, patient conditions, and clinical practice standards in different departments ( Worrell, 2020 ). While human vision is good at reading through the images of varying qualities, AI tools are generally not ( Tang et al., 2018 ). One important task to produce a systematic image AI performance is image pre-processing, including optimization of image quality, noise reduction, clutter removal, and enhancement of essential features for differentiation. Various AI tools are used to standardize image quality ( Zhu et al., 2017 ; Mazurowski et al., 2019 ).
A radiology AI tool for screening or diagnosis of a disease is usually comprised of several components: 1) pre-processing such as image normalization, 2) image segmentation or region of interest (ROI) extraction, and 3) potential disease pattern identification and classification. Various algorithms have been applied to each of these AI sub-components. However, there is a trend to use a fully CNN-based algorithm such as U-net for image segmentation and use a classification CNN- based algorithm for identification and classification of the disease aiming at the ROI. Alternatively, radiomics based classification can be employed on the ROI.
Data Labeling
In radiological imaging, the supervised learning approach is the most popular tool, and it requires labeled data for training and validation. The labeling of images must be done manually by expert radiologists. This process is very labor-intensive and very costly. The truth panel for images is established by having 2 out of 3 radiologists agreeing on the diagnoses and clinical determinations ( Lo et al., 2018a ).
Research Environment - Access to Research Resources
The role of AI will be different in different parts of the world. The AI tools developed for one region of the world using data from that region may not be useful in other regions with different disease prevalence, limited infrastructure, and different healthcare systems.
Collection and curation of images and related data could face several obstacles such as management of privacy, confidentiality, and the question of ownership. In recent years, the realization of clinical images' possible commercial values makes access more costly and difficult. When images need to be collected from multiple organizations, the data sharing process can become more complicated. International collaboration can be difficult when certain countries do not allow clinical data movement beyond their national borders ( Prior et al., 2020 ). One technical solution for such a situation can be a federated learning system where data remains in place while processing code and processed results can move around ( Konečný et al., 2016 )
Government agencies and various consortia have established a growing number of open access data repositories to facilitate better access to clinical image data for research. One of the best-known such repositories is the Cancer Imaging Archive (TCIA) ( Prior et al., 2013 ; The Cancer Imaging Archive (TCIA), 2020 ). It is a publicly available information repository for data about cancer, mostly radiology and pathology data acquired by the lung cancer screening project involving 26,722 participants from 2002 through 2004. It contains 22.3 TB of data. The types and volume of data in the archive are increasing rapidly.
Research and Development Environment in Resource-Limited Regions
The research and development and eventual adoption of AI for medical decision making in global health and low-resource settings are hampered by insufficient infrastructure ( Mollura et al., 2020 ).However, it is essential that local radiology and clinical community, resource-poor or not, have to develop and validate AI tools suitable for their environment. In the resource-poor regions with limited infrastructure, technical and human, such participation could be difficult. However, the research communities in the world's resource-limited regions can access many global imaging AI research resources. The Radiological Society of North America website has a vast amount of information. Many of the AI software is freely available as open-source at no cost to the users. The cancer imaging archive (TCIA) of the National Cancer Institute of the US has many curated radiological images to support imaging research. Most of the CADe products for lung cancer screen started using the openly available images in TCIA, which holds the CT images from the national lung cancer screening trial.
Future of Radiology Service and Radiomics
The digital transformation of radiology services will continue and accelerate. Analog film is gone, and modern imaging systems have evolved far beyond the slow and primitive early MRI and CT systems. PET was only a research tool at a few centers but is now becoming available at small community hospitals. Hybrid scanners that combine multiple modalities and can operate in different healthcare settings will become readily available. The whole-body scanner that could do MRI or CT or PET will be available where the patients receive care ( Pichler et al., 2008 ; Nensa et al., 2018 ). PACS, teleradiology, and Radiology Information Systems all changed the radiology practice.
Nevertheless, the radiology department structure has not fundamentally changed in 30 years ( Kim and Mansfield, 2014 ). The radiology department continues to operate as a centralized resource to which patients come to complete the study, and radiologists dictate and distribute the reports ( Ondategui-Parra et al., 2004 ). This operational model may soon see some changes.
For the next 30 years of radiology in the future, the pace of change will accelerate. The cost of computing will continue to decrease, and connectivity will be fast and ubiquitous. Radiology and the field of diagnosis will evolve together with pathology. Diagnostic imaging, clinical pathology, and genomics could merge as an integrated diagnostic service that can integrate the various reports from these subspecialty sections and synthesize a coherent diagnosis that is communicated to the appropriate physicians with more actionable specifics. Such an integrated system will allow a rapid on-site point of care diagnosis, rather than the serial process of today involving multiple appointments over many days and weeks.
Some expect that a new profession of clinical diagnosticians, who integrate the work of radiologists and pathologists with other specialists with increasing reliance on AI assistance, will begin to grow ( Lundström et al., 2017 ). Pathologists are already doing biopsies under image guidance. There are movements toward the integration of professional service. Simultaneously, there are parallel significant scientific and technical developments underway in radiomics and pathomics that can facilitate this historical evolution.
Radiomics and pathomics are part of quantitative imaging that attempts to extract additional information from radiology and pathology images that may not be visible via visual inspection ( Saltz et al., 2017 ). Radiomics attempts to extract features from radiological images that quantify its phenotype characteristics in an automated high-throughput manner ( Fan et al., 2020 ). Pathomics attempts to extract similar information from pathology images. These two approaches will meet at a shared space to support personalized medicine. It has been hypothesized that such analysis may help prognosticate, predict treatment outcomes, and assess cancer tissue malignancy.
The value of AI in radiomics is two-fold. First, AI can be used for automated image analysis at scale, enabling rapid evaluation of hypothetical radiomic features. Whereas comparison studies involving human radiologists should take into account for a wide range of ergonomic and perception factors (such as the required number of readers or the need to provide time between different readings of the same image), a comparison of radiomics algorithms is only limited by computational speed and power. Similarly, new features can easily be tested against existing data sets. Secondly, unsupervised learning methods can be used to search for new radiomic features that might be very different from what would be noticed by a human observer. The AI can take on the discovery and find new and useful patterns within the existing imaging data ( Miles, 2020 ; Prior et al., 2020 ).
One fundamental issue in radiomics has to address is the standardization of the factors and processing involved in the quantitative analysis. The Image Biomarker Standardization Initiative (IBSI) is a new organization to address many challenges in 4 different specific areas, 1) standard nomenclature and common radiomic features, 2) radiomics image processing schemes, 3) provide data sets for validation and calibration, and 4) set of reporting guidelines ( Zwanenburg et al., 2020 ). This group defined 174 radiomics features commonly used to quantify the morphologic characteristics and numerous others needed to define the quantitative information. The group tries to standardize the image processing steps of data conversion, post-acquisition processing, segmentation, interpolation, masking, and others ( Zwanenburg et al., 2020 )( Zwanenburg et al., 2020 ). Such standardization is expected to make radiomics and pathomics clinically useful and scalable for the integrated diagnosis service ( Kuhl and Truhn, 2020 ).
The clinical adoption of AI can be driven by either technology push or market pull ( Chidamber and Kon, 1994 ; Di Stefano et al., 2012 ). Technology push arises when a new idea or new tool creates a capability that did not previously exist. The market pull is defined by the need to address pain points, inefficiencies, and problems with the current way of doing business. Ideally, these two forces synergistically combine to accelerate technology development and deployment. Over the last 30 years, radiology has benefited from this combination to develop teleradiology, PACS/RIS, and advanced imaging modalities. However, much of CAD's early development has been a technology push; it was usually not well aligned with clinical needs. We have proposed that a better alignment could arise from focusing on the radiology workflow – which includes many tasks beyond image interpretation – and the need to create an integrated diagnostics service combining radiological images, pathological data, and genomics ( Santos et al., 2019 ).
We envision three AI trajectories in radiology, as shown in Figure 1 . First, AI will undergo advances in CADe and CADx to make image interpretation better and faster. Despite the significant progress in developing CNN algorithms, there are still many areas for improvement as proposed in this paper. Second, a variety of AI tools, supervised learning and unsupervised learning, will be needed to improve workflow and increase productivity, and, at the same time, reduce the cost of operation. This operationally focused research will require a holistic understanding of radiology operations. Third, quantitative imaging, including radiomics, pathomics, and genomics, will emerge and become a standardized approach for integrated diagnostics. In summary, we predict that AIs will facilitate the merging of disparate medical and scientific domains into an integrated diagnostic service for personalized precision medicine.
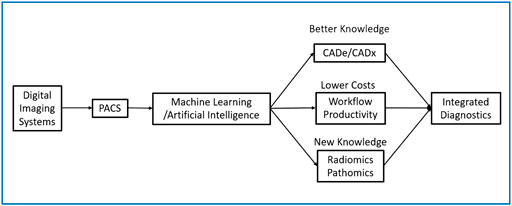
FIGURE 1 . Digital Transformation of Radiology with 3 Categories of Application of ML/AI.
Author Contributions
SM designed the document and integrated different ideas into a single document. KW drafted the section dealing with the clinical adoption of AI and radiomics and edited the final document. SL drafted the section on CNN and data needs. YL did a literature review and developed the list of relevant references. SB was responsible for developing radiology workflow issues.
Conflict of Interest
The authors declare that the research was conducted in the absence of any commercial or financial relationships that could be construed as a potential conflict of interest.
Alhajeri, M., Aldosari, H., and Aldosari, B. (2017). Evaluating latest developments in PACS and their impact on radiology practices: A systematic literature review. Informatics in Medicine Unlocked 9, 181–190. doi:10.1016/j.imu.2017.08.005
CrossRef Full Text | Google Scholar
Beede, E., Baylor, E., Hersch, F., Iurchenko, A., Wilcox, L., Ruamviboonsuk, P., et al. (2020). “A human-centered evaluation of a deep learning system deployed in clinics for the detection of diabetic retinopathy,” in Proceedings of the 2020 CHI Conference on Human Factors in Computing Systems. Honolulu, HI: Hawaii Convention Center, April, 2020, (Accessed August 11, 2020) . doi:10.1145/3313831.3376718
Boochever, S. S. (2004). HIS/RIS/PACS integration: getting to the gold standard. Radiol. Manage. 26 (3), 16–7.
Google Scholar
Brady, A. P. (2017). Error and discrepancy in radiology: inevitable or avoidable? Insights Imaging 8 (1), 171–182. doi:10.1007/s13244-016-0534-1
PubMed Abstract | CrossRef Full Text | Google Scholar
Chidamber, S. R., and Kon, H. B. (1994). A research retrospective of innovation inception and success: the technology–push, demand–pull question. Internat. J. Tech. Manag. 9 (1), 94–112. doi:10.1504/IJTM.1994.025565
Di Stefano, G., Gambardella, A., and Verona, G. (2012). Technology push and demand pull perspectives in innovation studies: Current findings and future research directions. Research policy 41 (8), 1283–1295. doi:10.1016/j.respol.2012.03.021
Dikici, E., Bigelow, M., Prevedello, L. M., White, R. D., and Erdal, B. S. (2020). Integrating AI into radiology workflow: levels of research, production, and feedback maturity. J. Med. Imaging (Bellingham) 7 (1), 016502. doi:10.1117/1.JMI.7.1.016502
Doi, K. (2007). Computer-aided diagnosis in medical imaging: historical review, current status and future potential. Comput. Med. Imaging Graph 31 (4–5), 198–211. doi:10.1016/j.compmedimag.2007.02.002
Doshi, A. M., Moore, W. H., Kim, D. C., Rosenkrantz, A. B., Fefferman, N. R., Ostrow, D. L., et al. (2018). Informatics solutions for driving an effective and efficient radiology practice. RadioGraphics 38 (6), 1810–1822. doi:10.1148/rg.2018180037
ESR (2019). What the radiologist should know about artificial intelligence - an ESR white paper. Insights Imaging 10 (1), 44. doi:10.1186/s13244-019-0738-2
Fan, M, Xia, P., Clarke, R., Wang, Y., and Li, L. (2020). Radiogenomic signatures reveal multiscale intratumour heterogeneity associated with biological functions and survival in breast cancer. Nat. Commun. 11 (1), 4861.doi:10.1038/s41467-020-18703-2
Forsberg, D., Rosipko, B., and Sunshine, J. L. (2017). Radiologists’ variation of time to read across different procedure types. J. Digit. Imaging 30 (1), 86–94. doi:10.1007/s10278-016-9911-z
Fosfuri, A., Giarratana, M. S., and Luzzi, A. (2008). The penguin has entered the building: the commercialization of open source software products. Organization science 19 (2), 292–305. doi:10.1287/orsc.1070.0321
Giger, M. L., Chan, H. P., and Boone, J. (2008). Anniversary paper: history and status of CAD and quantitative image analysis: the role of Medical Physics and AAPM. Med. Phys. 35 (12), 5799–5820. doi:10.1118/1.3013555
Gold, L. S., Klein, G., Carr, L., Kessler, L., and Sullivan, S. D. (2012). The emergence of diagnostic imaging technologies in breast cancer: discovery, regulatory approval, reimbursement, and adoption in clinical guidelines. Cancer Imaging 12 (1), 13–24. doi:10.1102/1470-7330.2012.0003
Halsted, M. J., and Froehle, C. M. (2008). Design, implementation, and assessment of a radiology workflow management system. AJR Am. J Roentgenol. 191 (2), 321–327. doi:10.2214/AJR.08.1566
Hosny, A., Parmar, C., Quackenbush, J., Schwartz, L. H., and Aerts, H. J. W. L. (2018). Artificial intelligence in radiology. Nat. Rev. Cancer 18 (8), 500–510. doi:10.1038/s41568-018-0016-5
Hricak, H. (2018). 2016 new horizons lecture: beyond imaging-radiology of tomorrow. Radiology 286 (3), 764–775. doi:10.1148/radiol.2017171503
Interagency Working Group on Medical Imaging, Committee on Science, and National Science and Technology Council (2017). The interagency working group on medical imaging released a final report in December 2017. Available at: https://www.whitehouse.gov/wp-content/uploads/2017/12/Roadmapfor-Medical-Imaging-Research-and-Development-2017.pdf
Kahn, C. E., Langlotz, C. P., Burnside, E. S., Carrino, J. A., Channin, D. S., Hovsepian, D. M., et al. (2009). Toward best practices in radiology reporting. Radiology 252 (3), 852–856. doi:10.1148/radiol.2523081992
Kim, Y. W., and Mansfield, L. T. (2014). Fool me twice: delayed diagnoses in radiology with emphasis on perpetuated errors. AJR Am. J. Roentgenol. 202 (3), 465–70. doi:10.2214/AJR.13.11493
Konečný, J., McMahan, H. B., Yu, F. X., Richtárik, P., Suresh, A. T., and Bacon, D. (2016). Federated learning: Strategies for improving communication efficiency. arXiv .
Kuhl, C. K., and Truhn, D. (2020). The long route to standardized radiomics: unraveling the knot from the end. Radiology 295 (2), 339–341. doi:10.1148/radiol.2020200059
Lehman, C. D., Wellman, R. D., Buist, D. S., Kerlikowske, K., Tosteson, A. N., Miglioretti, D. L., et al. (2015). Diagnostic accuracy of digital screening mammography with and without computer-aided detection. JAMA Intern. Med. 175 (11), 1828–1837.doi:10.1001/jamainternmed.2015.5231
Levine, S. S., and Prietula, M. J. (2014). Open collaboration for innovation: principles and performance. Organization Science 25 (5), 1414–1433. doi:10.1287/orsc.2013.0872
Lo, S.-C. B., Chan, H.-P., Lin, J.-S., Li, H., Freedman, M. T., and Mun, S. K. (1995). Artificial convolution neural network for medical image pattern recognition. Neural Networks 8 (7–8), 1201–1214. doi:10.1016/0893-6080(95)00061-5
Lo, S.-C. B., Lin, J.-S., Freedman, M. T., and Mun, S. K. (1993). Computer-assisted diagnosis of lung nodule detection using artificial convoultion neural network. Medical Imaging: Image Processing 1898, 859–869. doi:10.1117/12.154572
Lo, S.-C. B., Lin, J.-S.J., Freedman, M. T., and Mun, S. K. (1998). Application of artificial neural networks to medical image pattern recognition: detection of clustered microcalcifications on mammograms and lung cancer on chest radiographs. J. VLSI signal processing systems for signal, image video technology 18 (3), 263–274.
Lo, S. B., Freedman, M. T., Gillis, L. B., White, C. S., and Mun, S. K. (2018a). JOURNAL CLUB: computer-aided detection of lung nodules on CT with a computerized pulmonary vessel suppressed function. AJR Am. J. Roentgenol. 210 (3), 480–488. doi:10.2214/AJR.17.18718
Lo, S. B., Freedman, M. T., and Mun, S. K. (2018b). Transformationally Identical and Invariant Convolutional Neural Networks by Combining Symmetric Operations or Input Vectors. arXiv preprint arXiv:1807.11156.
Lo, S. B., Freedman, M. T., Mun, S.K., and Chan, H.-P. (2018c). Geared Rotationally Identical and Invariant Convolutional Neural Network Systems. arXiv preprint arXiv:1808.01280.
Lo, S. C. B., Freedman, M. T., Mun, S. K., and Gu, S. (2018d). Transformationally identical and invariant convolutional neural networks through symmetric element operators. arXiv preprint arXiv:1806.03636.
Lundström, C. F., Gilmore, H. L., and Ros, P. R. (2017). Integrated diagnostics: the computational revolution catalyzing cross-disciplinary practices in radiology, pathology, and genomics. Radiological 285 (1), 12–15. doi:10.1148/radiol.2017170062
Mazurowski, M. A., Buda, M., Saha, A., and Bashir, M. R. (2019). Deep learning in radiology: An overview of the concepts and a survey of the state of the art with focus on MRI. J. Magn. Reson. Imaging 49 (4), 939–954. doi:10.1002/jmri.26534
McDonald, R. J., Schwartz, K. M., Eckel, L. J., Diehn, F. E., Hunt, C. H., Bartholmai, B. J., et al. (2015). The effects of changes in utilization and technological advancements of cross-sectional imaging on radiologist workload. Acad. Radiol. 22 (9), 1191–1198. doi:10.1016/j.acra.2015.05.007
Miles, K (2020). Radiomics for personalised medicine: the long road ahead. Br J. Cancer 122 (7), 929–930. doi:10.1038/s41416-019-0699-8
Mollura, D. J., Culp, M. P., Pollack, E., Battino, G., Scheel, J. R., Mango, V. L., et al. (2020). Artificial intelligence in low-and middle-income countries: innovating global health radiology. Radiology 297 (3), 513–520. doi:10.1148/radiol.2020201434
Montagnon, E., Cerny, M., Cadrin-Chênevert, A., Hamilton, V., Derennes, T., Ilinca, A., et al. (2020). Deep learning workflow in radiology: a primer. Insights Imaging 11 (1), 22. doi:10.1186/s13244-019-0832-5
Multiple-Editor (2020). Comparison of deep-learning software [Online]. Available from: https://en.wikipedia.org/wiki/Comparison_of_deep-learning_software .
Mun, S. K., Freedman, M., and Kapur, R. (1993). Image management and communications for radiology. IEEE Engineering in Medicine and Biology Magazine 12 (1), 70–80. doi:10.1109/51.195943
Mun, S. K., Levine, B., Cleary, K., and Dai, H. (1998). Deployable teleradiology and telemedicine for the US military. Comput. Methods Programs Biomed . 57 (1–2), 21–27. doi:10.1016/s0169-2607(98)00040-6
Mun, S. K., Prior, F., Caramella, D., and Ratib, O. (2007). Introduction to the special section on image management in the healthcare enterprise. IEEE Trans. Inf. Technol. Biomed. 11 (1), 1–6. doi:10.1109/titb.2006.888236
Mun, S. K., and Turner, J. W. (1999). Telemedicine: Emerging e-medicine. Annu. Rev. Biomed. Eng. 1 (1), 589–610. doi:10.1146/annurev.bioeng.1.1.589
Mutasa, S., Sun, S., and Ha, R. (2020). Understanding artificial intelligence based radiology studies: What is overfitting? Clin. Imaging 65, 96–99. doi:10.1016/j.clinimag.2020.04.025
Nensa, F., Bamberg, F., Rischpler, C., Menezes, L., Poeppel, T.D., La Fougère, C., et al. (2018). Hybrid cardiac imaging using PET/MRI: a joint position statement by the European Society of Cardiovascular Radiology (ESCR) and the European Association of Nuclear Medicine (EANM). European Journal of Hybrid Imaging 28 (1), 4086–4101. doi:10.1007/s00330-017-5008-4
Ondategui-Parra, S., Bhagwat, J. G., Zou, K. H., Gogate, A., Intriere, L. A., Kelly, P., et al. (2004). Practice management performance indicators in academic radiology departments. Radiology 233 (3), 716–722. doi:10.1148/radiol.2333031147
Opensource.org (2020). Open Source Licenses by Category [Online]. Open Source Initiative. Available at: https://opensource.org/licenses/category .
Perrault, R., Shoham, Y., Brynjolfsson, E., Clark, J., Etchemendy, J., Grosz, B., et al. (2019). The AI Index 2019 Annual Report. AI Index Steering Committee . Stanford, CA: Human-Centered AI Institute Stanford University .
Pichler, B. J., Judenhofer, M. S., and Wehrl, H. F. (2008). PET/MRI hybrid imaging: devices and initial results. Eur. Radiol. 18 (6), 1077–1086. doi:10.1007/s00330-008-0857-5
Prior, F., Almeida, J., Kathiravelu, P., Kurc, T., Smith, K., Fitzgerald, T.J., et al. (2020). Open access image repositories: high-quality data to enable machine learning research. Clin. Radiol . 75 (1), 7–12. doi:10.1016/j.crad.2019.04.002
Prior, F. W., Clark, K., Commean, P., Freymann, J., Jaffe, C., Kirby, J., et al. (2013). TCIA: an information resource to enable open science, Annu. Int. Conf. Proc. IEEE Eng. Med. Biol. Soc. 2013, 1282–1285. doi:10.1109/EMBC.2013.6609742
Saltz, J., Almeida, J., Gao, Y., Sharma, A., Bremer, E., DiPrima, T., et al. (2017). Towards generation, management, and exploration of combined radiomics and pathomics datasets for cancer research. AMIA Jt. Summits Transl. Sci. Proc. 2017, 85–94.
PubMed Abstract | Google Scholar
Santos, M. K., Ferreira Júnior, J. R., Wada, D. T., Tenório, A. P. M., Barbosa, M. H. N., and Marques, P. M. A. (2019). Artificial intelligence, machine learning, computer-aided diagnosis, and radiomics: advances in imaging towards to precision medicine. Radiol. Bras. 52 (6), 387–396. doi:10.1590/0100-3984.2019.0049
Schaffter, T., Buist, D. S. M., Lee, C.I., Nikulin, Y., Ribli, D., Guan, Y., et al. (2020). Evaluation of combined artificial intelligence and radiologist assessment to interpret screening mammograms. JAMA Netw. Open 3 (3), e200265. doi:10.1001/jamanetworkopen.2020.0265
Schemmel, A., Lee, M., Hanley, T., Pooler, B.D., Kennedy, T., Field, A., et al. (2016). Radiology workflow disruptors: a detailed analysis. J. Am. Coll. Radiol. 13 (10), 1210–1214.doi:10.1016/j.jacr.2016.04.009
Soffer, S., Ben-Cohen, A., Shimon, O., Amitai, M. M., Greenspan, H., and Klang, E. (2019). Convolutional neural networks for radiologic images: a radiologist's guide. Radiology 290 (3), 590–606. doi:10.1148/radiol.2018180547
Tadavarthi, Y., Vey, B., Krupinski, E., Prater, A., Gichoya, J., Safdar, N., et al. (2020). The state of radiology AI: considerations for purchase decisions and current market offerings. Radiology: Artificial Intelligence 2 (6), e200004. doi:10.1148/ryai.2020200004
Tang, A., Tam, R., Cadrin-Chênevert, A., Guest, W., Chong, J., Barfett, J., et al. (2018). Canadian association of radiologists white paper on artificial intelligence in radiology. Can. Assoc. Radiol. J. 69 (2), 120–135. doi:10.1016/j.carj.2018.02.002
The Cancer Imaging Archive (TCIA) (2020). The Cancer Imaging Archive [Online]. Available at: https://www.cancerimagingarchive.net/ .
Worrell, S. (2020). Making AI Tools Clinically Relevant- Sustainability and Scalability. ( Central VA Health Care System Artificial Intelligence for Medical Imaging Virtual Workshop: Virginia Tech-AIC) . Available at: https://aic.ncr.vt.edu/aic-workshops-and-meetings/aimiworkshophome/aimirecording.html
Yamashita, R., Nishio, M., Do, R. K. G., and Togashi, K. (2018). Convolutional neural networks: an overview and application in radiology. Insights Imaging 9 (4), 611–629. doi:10.1007/s13244-018-0639-9
Zhu, Q., Du, B., Turkbey, B., Choyke, P. L., and Yan, P. (2017). Deeply-supervised CNN for prostate segmentation”, in 2017 International Joint Conference on Neural Networks (Ijcnn) , Anchorage, AK , May 14–19, 2017 ( IEEE ) doi:10.1109/IJCNN.2017.7965852
Zwanenburg, A., Vallières, M., Abdalah, M. A., Aerts, H. J. W. L., Andrearczyk, V., Apte, A., et al. (2020). The image biomarker standardization initiative: standardized quantitative radiomics for high-throughput image-based phenotyping. Radiology 295 (2), 328–338. doi:10.1148/radiol.2020191145
Keywords: artificial intelligence, radiology, CNN, productivity, integrated diagnostics, workflow
Citation: Mun SK, Wong KH, Lo S-CB, Li Y and Bayarsaikhan S (2021) Artificial Intelligence for the Future Radiology Diagnostic Service. Front. Mol. Biosci. 7:614258. doi: 10.3389/fmolb.2020.614258
Received: 05 October 2020; Accepted: 29 December 2020; Published: 28 January 2021.
Reviewed by:
Copyright © 2021 Mun, Wong, Lo, Li and Bayarsaikhan. This is an open-access article distributed under the terms of the Creative Commons Attribution License (CC BY). The use, distribution or reproduction in other forums is permitted, provided the original author(s) and the copyright owner(s) are credited and that the original publication in this journal is cited, in accordance with accepted academic practice. No use, distribution or reproduction is permitted which does not comply with these terms.
*Correspondence: Seong K. Mun, [email protected]
This article is part of the Research Topic
AI in Biological and Biomedical Imaging
Advertisement
The impact of large language models on radiology: a guide for radiologists on the latest innovations in AI
- Invited Review
- Open access
- Published: 29 March 2024
Cite this article
You have full access to this open access article
- Takeshi Nakaura ORCID: orcid.org/0000-0002-9010-0341 1 ,
- Rintaro Ito 2 ,
- Daiju Ueda 3 ,
- Taiki Nozaki 4 ,
- Yasutaka Fushimi 5 ,
- Yusuke Matsui 6 ,
- Masahiro Yanagawa 7 ,
- Akira Yamada 8 ,
- Takahiro Tsuboyama 7 ,
- Noriyuki Fujima 9 ,
- Fuminari Tatsugami 10 ,
- Kenji Hirata 11 ,
- Shohei Fujita 12 ,
- Koji Kamagata 13 ,
- Tomoyuki Fujioka 14 ,
- Mariko Kawamura 2 &
- Shinji Naganawa 2
385 Accesses
Explore all metrics
The advent of Deep Learning (DL) has significantly propelled the field of diagnostic radiology forward by enhancing image analysis and interpretation. The introduction of the Transformer architecture, followed by the development of Large Language Models (LLMs), has further revolutionized this domain. LLMs now possess the potential to automate and refine the radiology workflow, extending from report generation to assistance in diagnostics and patient care. The integration of multimodal technology with LLMs could potentially leapfrog these applications to unprecedented levels.
However, LLMs come with unresolved challenges such as information hallucinations and biases, which can affect clinical reliability. Despite these issues, the legislative and guideline frameworks have yet to catch up with technological advancements. Radiologists must acquire a thorough understanding of these technologies to leverage LLMs’ potential to the fullest while maintaining medical safety and ethics. This review aims to aid in that endeavor.
Similar content being viewed by others
Automated Deep Learning for Medical Imaging
Radiology artificial intelligence: a systematic review and evaluation of methods (RAISE)
Brendan S. Kelly, Conor Judge, … Ronan P. Killeen
Avoid common mistakes on your manuscript.
Introduction
The inception of Deep Learning (DL) has catalyzed a significant progression in artificial intelligence (AI) [ 1 ], unlocking numerous possibilities, especially in diagnostic radiology—an arena pivotal for accurate imaging data interpretation. This progression is attributed mainly to the emergence of Convolutional Neural Networks (CNNs) [ 2 , 3 ], which have markedly enhanced image recognition, segmentation, analysis, and improvement of image quality [ 1 , 4 , 5 , 6 , 7 , 8 , 9 , 10 , 11 , 12 , 13 , 14 , 15 ]. This represents a foundational shift in automated feature extraction from imaging data, consequently reducing the time and expertise required for interpreting medical images. Additionally, DL-powered tools have demonstrated their efficacy in improving diagnostic accuracy by aiding radiologists in precisely detecting anomalies such as tumors, external injuries, and other pathological conditions [ 16 , 17 , 18 , 19 , 20 ]. These advancements not only accelerate the diagnostic process but also contribute substantially to prognostic evaluations, thus playing a crucial role in elevating patient care and outcomes [ 21 ].
The introduction of the Transformer architecture has been a significant milestone in machine learning, paving the way for the development of Large Language Models (LLMs) such as the Generative Pre-trained Transformer (GPT) series. The architecture’s proficiency in handling sequential data efficiently through attention mechanisms has expedited the evolution of LLMs, which now possess the ability to understand and generate human-like text with remarkable accuracy. The subsequent advent of ChatGPT further accentuated the popularity and utility of LLMs by showcasing their capability to engage in more natural, dynamic dialogues, thus expanding the scope of applications across various fields. In diagnostic radiology, LLMs might offer a promising pathway for enhancing multiple aspects of the radiology workflow. Their capability to automate report generation and expedite information retrieval can potentially save significant time for radiologists, thereby ameliorating the efficiency and accuracy of diagnostic processes.
Despite the undeniable utility of LLMs, there has been a scarcity of reviews describing the rapid development of LLMs for clinical radiologists. This article delineates a brief history of contemporary LLMs and provides a synopsis of their application in radiology for the clinical radiologist.
Overview of DL and LLM before transformer architecture
Natural Language Processing (NLP), CNN-based image processing, is a branch of AI. Recently, DL has been employed extensively in NLP tasks. This wide applicability of DL can be attributed to the universal approximation theorem [ 22 ]. This theorem suggests that a neural network, provided with enough layers and neurons, can approximate any reasonable function with a high degree of accuracy. DL thus operates by approximating an ideal function capable of transforming various data types, such as images, music, and text, into other forms of data (Fig. 1 ). In broad terms, the current LLM process involves generating a response sentence from a given request sentence, essentially transforming multi-dimensional vectors representing the request sentence into multi-dimensional vectors representing the response sentence. Despite the development of various DL models for language processing applications, this fundamental concept remains constant.
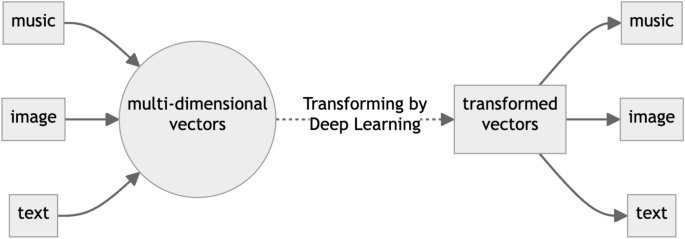
Overview of the Deep Learning Process. If there is some relationship between the matrices representing input and output data, Deep Learning can learn it given a myriad of training data by the “universal approximation theorem”
Before the inception of the Transformer architecture, the domain of NLP chiefly relied on architectural frameworks such as Recurrent Neural Networks (RNNs) [ 2 ], Long Short-Term Memory networks (LSTMs) [ 23 ], and CNNs. RNNs, with their intrinsic capability to encapsulate sequential information, were predominantly employed for an array of NLP tasks including but not limited to translation, sentiment analysis, and named entity recognition. However, they frequently encountered challenges with long-term dependencies owing to the vanishing or exploding gradient dilemma. To alleviate these issues, LSTMs were introduced as a special kind of RNN capable of learning long-term dependencies, providing a more robust framework for handling sequences and time-series data. Nonetheless, while LSTMs mitigated the gradient problem to an extent, they still entailed a significant computational and temporal demand, especially as data complexity increased. Conversely, CNNs were more adept at local pattern recognition within data and found their application in certain NLP tasks, yet they too were encumbered by limitations in capturing long-range dependencies within text sequences. Moreover, the computational and temporal demands of training these networks escalated significantly, especially in the face of the burgeoning size and intricacy of data.
Recently, it has been elucidated that the efficacy of modified RNN can be commensurate with that of newer models [ 24 ], contingent upon the scale. However, the formidable computational costs associated with their operation pose a significant deterrent, leading to their diminished utilization in recent times. It is also recognized that the architectural distinctions among these models exert a lesser impact on performance compared to the magnitude of parameters encompassed [ 25 ]. Hence, the details of the structure of these models are omitted from this review. The cornerstone of operation for these NLP frameworks, inclusive of the subsequent Transformer architecture delineated, hinges on the initial transmutation of textual sentences into a numerical sequence termed as tokens, facilitated by a tokenizer. Figure 2 shows an example of sentence conversion by an online Tokenizer ( https://platform.openai.com/tokenizer ). This token sequence subsequently undergoes a further transmutation into an alternate numerical sequence. This tokenization process has been used in NLP even before the rapid development of Deep Learning, and the basic principle is the same in recent LLM.
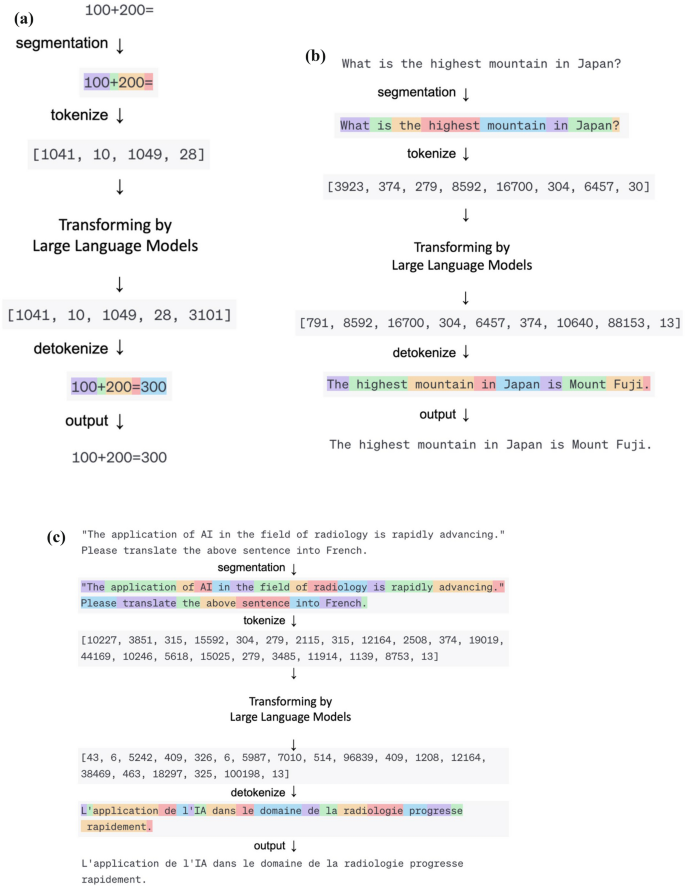
Various Language Processing with Large Language Model. Examples of a computation, b conversation, and c translation, respectively. All of these different language processing tasks can be accomplished using the same process: converting input data into a matrix using a tokenizer, transforming it into another matrix using a Large Language Model, and then converting it back into output data
Advent and evolution of the transformer architecture for LLM
The advent of the Transformer architecture marked a significant milestone in the realms of NLP and machine learning [ 26 , 27 , 28 ]. Unlike previous architectures, the Transformer was designed to efficiently handle parallel processing, making it especially suitable for training on graphics processing units. This novel design facilitated a substantial reduction in training times and effective management of large datasets, enabling the training of large-scale models that were previously unfeasible. Furthermore, this escalation in learning scale elucidated relationships known as scaling laws (Fig. 3 ), which delineate the relationships between model size, dataset size, and the amount of computing used for training [ 25 ]. This study reported the performance of language models on the cross-entropy loss scales according to a power-law to these factors.
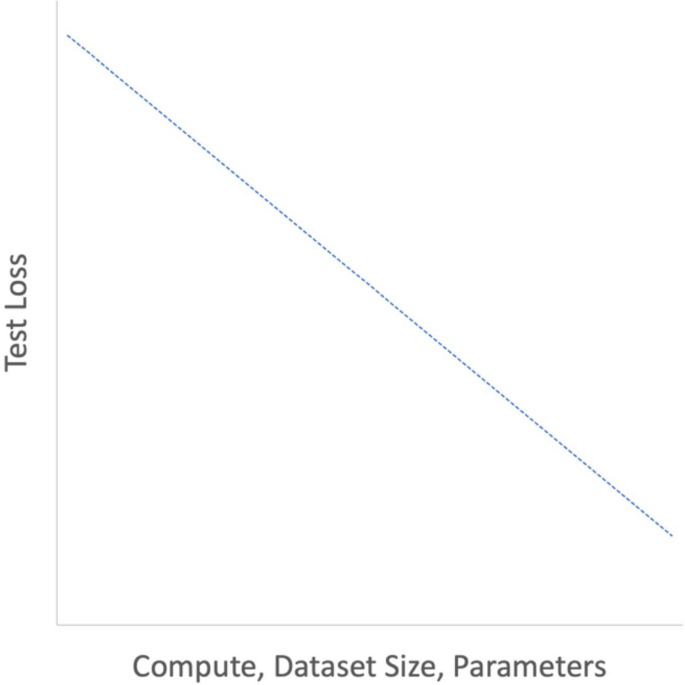
Schema of Scaling Law. The performance of the Transformer follows a simple power law, where the parameters, dataset size and computational resources are considered as variables. For instance, if the other two variables are not the bottleneck, doubling the number of parameters results in a performance improvement by a power of 2. (Graphs are plotted on logarithmic scales)
The scalability and parallel processing capabilities of the Transformer architecture accelerated the development of large-scale neural network models. Notably, the Generative Pre-trained Transformer (GPT) [ 25 , 29 , 30 ] and Bidirectional Encoder Representations from Transformers (BERT) [ 31 ] stand as exemplary embodiments of the large-scale expansion and advancement of the Transformer. GPT, developed by OpenAI, is an LLM based on the Transformer architecture, focusing on predicting the next word in a given text sequence. It is generally pre-trained on a vast text corpus and then fine-tuned for specific tasks. The GPT series (GPT-1, 2, 3, 3.5, and 4) aims to enhance performance by augmenting model size, with GPT-3 boasting 175 billion parameters [ 25 ]. On the other hand, BERT, developed by Google, also leverages the Transformer architecture but adopts a different approach. By considering bidirectional context, BERT achieves superior performance on specific NLP tasks, which is particularly advantageous in tasks like question-answering and named entity recognition.
In any case, as the scale increases, the performance of language models on tasks has significantly improved. Particularly, large-scale models like GPT-3 have been reported to exhibit excellent performance on entirely new tasks without retraining or with just a few demonstrations [ 25 ]. This burgeoning performance with scale underscores the remarkable potential and evolution propelled by the Transformer architecture, contributing to the broad spectrum of advancements in NLP and machine learning fields.
LLM limitations
Despite the remarkable achievements, LLMs have inherent limitations. One of the notable issues is hallucination, where the model generates incorrect or fictional information that wasn’t present in the training data [ 32 , 33 , 34 , 35 , 36 ]. This problem arises due to several underlying factors and poses challenges to the implementation and trustworthiness of LLMs, especially in critical fields like healthcare. A notable cause of hallucination, the source-reference divergence, arises from heuristic data collection methods or inherent challenges in certain natural language generation tasks, leading to deviations from the provided source during text generation. Similarly, exploitation through 'jailbreak' prompts that were not intended by the developers, which manipulate the model’s behavior or output, and reliance on datasets with incomplete or contradictory information significantly influence the LLM’s generated responses. These issues are exacerbated by misleading training data, where incorrect, outdated, or biased information is propagated into the generated outputs, further undermining the reliability of LLMs in a clinical setting. Mitigation strategies aimed at reducing hallucination in LLMs include the employment of regularization techniques, augmenting training data, and leveraging few-shot learning strategies. However, completely preventing hallucination remains a formidable challenge due to the inherent limitations of the current LLM architectures and the vast and varied nature of the training data.
Inductive biases [ 37 ] refer to the set of assumptions that a model makes to predict outputs for unseen data. In LLMs, these biases might arise from the training data, leading the model to generate outputs that may not align with real-world scenarios. The performance and the model’s capacity to generalize across varying contexts can be adversely affected by these biases. Additionally, the “black box” nature of LLMs denotes the lack of transparency in understanding how the model arrives at a particular decision, which is a critical requirement for real-world applications, particularly in fields demanding explainability like medical fields.
The output generated by LLM can be inaccurate and misleading due to these limitations and can lead to misguided clinical problems [ 38 ], and LLM output should be carefully evaluated by professionals.
Release of ChatGPT and its application to medical fields
The public release of ChatGPT on November 30, 2022, developed by Open AI, heralded a new era of accessibility, drawing a plethora of users from diverse fields. The transition to GPT-3.5 used in ChatGPT was a pivotal moment, as the incorporation of Reinforcement Learning from Human Feedback (RLHF) [ 39 ] played a crucial role in refining the model's responses, making them more coherent and contextually appropriate. This widespread adoption triggered a boom, as the model's potential in various applications was explored extensively. Additionally, GPT-4 is slated for public availability on March 14, 2023. While the specific architectural details remain undisclosed, it is anticipated that GPT-4 will herald enhanced performance across diverse domains, marking a substantial advancement from its predecessor, GPT-3.5.
In the medical field, ChatGPT showcased an impressive aptitude by excelling in medical examinations [ 40 ], a testament to its proficiency in handling medical knowledge. Furthermore, studies have highlighted its competence in real-world medication consultations [ 41 ], where it displayed a higher appropriateness rate in responding to public consultation questions compared to those posed by healthcare providers in a hospital setting. Although ChatGPT’s official warnings mention its use in diagnostics, saying, “Making automated decisions in domains that affect an individual’s rights or well-being (e.g., law enforcement, migration, management of critical infrastructure, safety components of products, essential services, credit, employment, housing, education, social scoring, or insurance)” ( https://openai.com/policies/usage-policies ) and the use of ChatGPT on WWW may have a serious concern of data leaking unless user manifest opt-out ( https://privacy.openai.com/policies ), these achievements highlight ChatGPT’s potential in giving important medical insights.
Capable applications of LLM in the radiology field
Radiologists routinely engage with a substantial volume of textual information encompassing diagnostic request forms, medical charts, reports from other examinations, references to various guidelines and past literature, diagnostic imaging reports, and the generation of scholarly articles. However, recent years have witnessed an uptrend in the utilization of imaging diagnostic modalities across numerous countries. The ensuing amplification in image interpretation and reporting duties has precipitated concerns surrounding burnout among radiologists [ 42 ]. Despite the aforementioned limitations, LLMs hold promise as potential adjunctive tools to ameliorate the burden associated with such radiological endeavors.
The accelerated development and refinement of LLMs such as ChatGPT have catalyzed a notable performance in medical examinations. For instance, an evaluation of ChatGPT on the United States Medical Licensing Exam (USMLE) revealed that it performed at or near the passing threshold across all three exams without any specialized training or reinforcement [ 40 ]. Moreover, in a radiology board-style examination, ChatGPT nearly met the passing criteria without specific radiology pre-training, while a GPT-4 demonstrated superior performance compared to GPT-3.5, indicating a significant advancement in model capability [ 43 ]. ChatGPT based on GPT-4 scored 65% when answering Japanese questions from the Japan Radiology Board Examination (JRBE) [ 44 ]. Another study evaluated ChatGPT's performance on the Polish specialty exam in radiology and diagnostic imaging. Although ChatGPT did not reach the passing threshold of 52%, it came close in certain question categories [ 45 ]. Although the precise performance of LLMs may exhibit variance based on language [ 46 ], these facts suggest that LLMs may be able to play a supplementary role even in quite specialized radiology work, even if only for text data at this point.
Given the demonstrated capabilities of LLMs, there exists a potential to significantly enhance radiological workflow. This enhancement may manifest through proficient summarization of medical records, streamlined diagnostic imaging studies, clinical decision-making, rewriting, and generation of radiology reports [ 47 , 48 ]. For instance, while not a general-purpose LLM akin to GPT-4, an LLM trained specifically on medical data, known as PubMedBERT [ 49 ], has been reported to accurately predict mortality within 24 h of admission for patients in intensive care units using solely medical record data [ 50 ]. This demonstrates the capacity of LLMs to adeptly handle and derive meaningful insights from textual data such as medical records, extending promise for their application in critical care settings. Additionally, There is a preliminary study that helps to automatically determine imaging studies and protocols based on the radiology department's request form [ 51 ]. Another paper demonstrates that the DL-based NLP model can accurately classify the status of bone metastasis in Japanese radiology reports, providing a potential tool for the early and efficient detection of patients with bone metastasis. [ 52 ] Given such capabilities, it is conceivable that soon, LLMs could semi-automatically configure protocols for imaging examinations such as CT or MRI, based on the information extracted from examination request forms, medical chart data, and other diagnostic data.
Furthermore, there are several reports of rewriting radiology reports written by radiologists using LLM. The utility of structured reporting in radiology has been acknowledged, yet it has also been reported that LLMs can rewrite free-style reports into structured reports [ 53 ]. This not only holds promise for daily clinical practice but also for the education of radiology residents. Additionally, while the interpretation of radiology reports necessitates specialized knowledge, it has been reported that LLMs are capable of translating these specialized reports into more comprehensible language for a general patient [ 54 ]. This potential application anticipates aiding in patient communication and comprehension, further extending the scope of LLMs in enhancing patient-centered care in radiology.
Report generation assistance through LLMs
One of the most direct applications of LLMs in reducing workload within the clinical setting could arguably be in assisting with the generation of imaging diagnostic reports themselves. While there have been numerous reports on this subject from earlier times [ 55 , 56 , 57 ], the advent of the GPT series has marked a notable advancement. It has been reported that LLMs can autonomously generate human-like radiology reports from merely brief keywords, and the differential diagnoses provided are relatively reliable [ 58 ]. This suggests a significant potential for augmenting the efficiency and accuracy of report generation, a critical component of the radiological workflow.
The approach delineated in this paper [ 58 ] for aiding the generation of radiology reports hinges solely on the utilization of ChatGPT, obviating the need for any specialized training and hence, rendering it a reproducible methodology accessible to all. The requisite inputs for this process are limited to basic demographic data such as age and gender, keywords embodying the imaging findings, and a prompt tailored for report generation. However, it is noteworthy that the prompt necessitates customization for imaging report generation; the prompt utilized in this paper follows a structured format aimed at aiding the generation of radiology reports using ChatGPT. This structure encompasses three pivotal components: (1) establishing the role of the LLM as a radiology specialist, (2) specifying the output sections (Findings, Impression, Differential Diagnosis), and (3) elaborating on the content for each of these sections. This structured approach is predicated on previously reported guidelines for radiology reporting [ 59 ], thereby adhering to the established norms within the radiological community.
Figure 4 shows the simplified prompt for generating radiology reports and usage examples. The prompt used in this review is as follows: “As a radiologist, create a radiology report following the given format. Include up to 5 differential diagnoses based on the information provided. Findings: Describe the factual observations from the imaging study using precise technical language. This sets the groundwork for the diagnosis. Impression: Summarize the meaning of the findings to arrive at a diagnosis or list of possible diagnoses. Give recommendations for the next steps, using clear language. Differential Diagnosis: List up to 5 possible diagnoses without describing the diseases, ranked by level of suspicion.” By typing simple keywords followed by a prompt like this on OpenAI's ChatGPT site ( https://chat.openai.com/ ), anyone can generate something like a radiology report without any additional training.
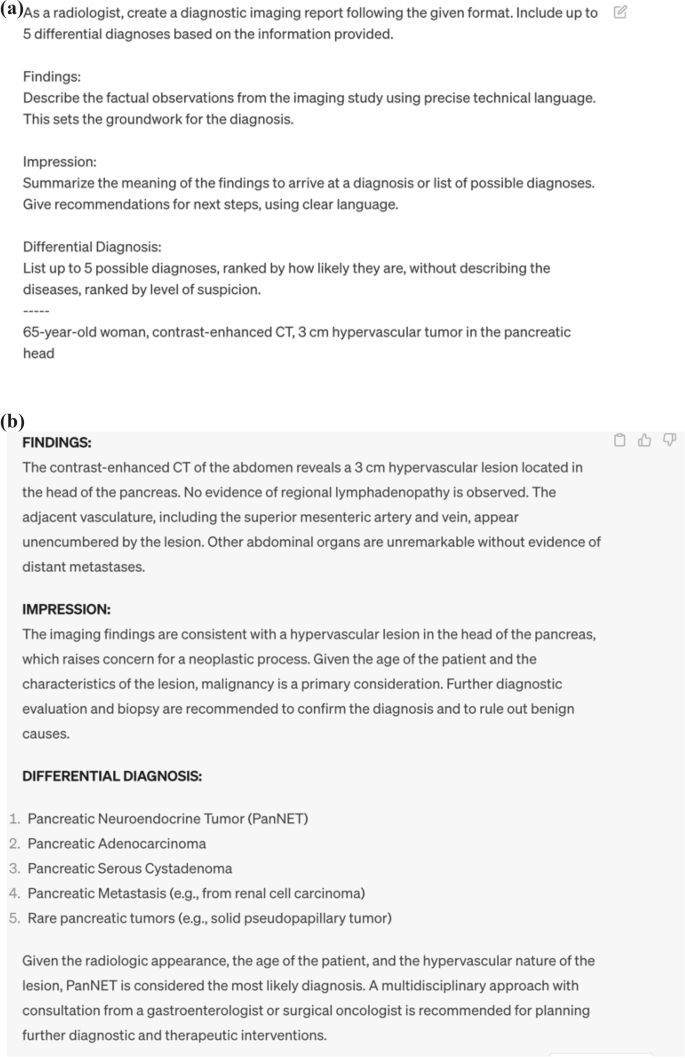
Example of Radiology Report Generated by GPT-4. a Prompts and b Corresponding generated reports. Providing specific instructions on the desired role, format, and content of output items within the prompts can enhance the quality of GPT-4 output
However, there are inherent challenges that must be addressed to ensure the safe and effective deployment of LLMs in this context. One such challenge is the phenomenon of hallucination, where the model generates incorrect or misleading information. This aspect necessitates a cautious approach to employing LLMs for diagnostic reporting. The potential for hallucinations to misguide clinical interpretations underscores the importance of having appropriate regulatory frameworks in place to mitigate risks associated with the use of LLMs in clinical settings.
Moreover, the legal and ethical frameworks surrounding the application of LLMs for diagnostic reporting need to be robustly established. As described above, today, even those without a background in diagnostic radiology can easily generate a large number of reports that are difficult to distinguish from diagnostic reports using LLMs. This has significant implications for the medical field. Given the potential for misinterpretation or misuse of these reports, it is crucial that regulations are put in place to ensure that only qualified professionals are authorized to interpret and apply these findings. Ensuring the responsible use of LLMs while maximizing their potential to reduce the workload and improve the accuracy and efficiency of diagnostic reporting requires a balanced approach. The evolution of legal and professional guidelines, in tandem with technological advancements, is imperative to foster a conducive environment for the integration of LLMs in radiological practice, ensuring both patient safety and enhanced clinical workflow.
Potential of LLMs in research work
The advent of LLMs like ChatGPT might also bring forth a promising avenue for alleviating the burgeoning workload in radiological research. It has been reported that LLM’s text generation capability has reached a level close to that of humans in the research field [ 60 ]. In this study, researchers asked a chatbot to generate 50 medical research abstracts based on excerpts published in JAMA, The New England Journal of Medicine, BMJ, The Lancet, and Nature Medicine. They then compared these generated abstracts with the original ones and asked a group of medical researchers to identify any fabricated abstracts. Scientists fared a correct identification rate of 68% for generated abstracts and 86% for genuine ones; however, they also made mistakes, incorrectly classifying 32% of the generated abstracts as genuine and 14% of the genuine abstracts as generated. An emblematic instance is that a pre-peer review version of the paper evaluating ChatGPT's performance at USMLE added ChatGPT to the authors [ 40 ]. Furthermore, LLMs can serve as invaluable adjuncts in review processes, assisting researchers and reviewers in tasks such as text summarization, extraction, and past literature retrieval. This assistance could be particularly beneficial for non-native authors, facilitating a smoother and more coherent review process [ 61 ].
On the contrary, a very recent pre-peer review paper examines the possibility of replacing the entire peer review process with LLM [ 62 ]. In this study, authors compared the feedback generated by GPT-4 and human peer reviewers, it was found that the overlap rate of points identified by GPT-4 and human reviewers was 30.85% on average in the Nature journal and 39.23% in the International Conference on Learning Representations (ICLR) conference. These rates were comparable to the overlap rate between two human reviewers, which averaged 28.58% in the Nature journal and 35.25% in the ICLR conference. Overall, 57.4% of users evaluated the feedback from GPT-4 as useful or very useful, and 82.4% felt that the feedback from GPT-4 was more beneficial than at least some of the feedback from human reviewers.
However, the integration of LLMs into the scholarly landscape is not devoid of ethical and procedural considerations. One primary concern pertains to authorship, as LLMs, lacking the capacity for responsibility, cannot be listed as authors despite their substantial contribution to manuscript creation [ 63 , 64 ]. Most academic papers’ submission guidelines have acknowledged this concern by mandating a detailed disclosure if LLMs are employed in the manuscript preparation, ensuring a transparent acknowledgment of LLM assistance. Moreover, a cautious approach towards the utilization of LLMs in review processes is advocated to mitigate risks associated with confidentiality and other potential malfeasances. While there has been a paucity of guidelines on the prudent use of LLMs in reviews, the recently published guidelines by Radiology [ 65 ] underline the importance of cautious employment, with a particular emphasis on maintaining confidentiality. This prudent approach towards LLM utilization not only fortifies the integrity of the review process but also sets a precedent for fostering responsible AI integration in radiological research.
Future outlook of LLMs in radiology
LLM is currently evolving rapidly, and multimodal technology seems to be one of the most notable and relevant in the field of radiology. Like LLM, this technology is based on transformer architecture, but it can also handle image data in a unified manner. Microsoft's Bing AI is currently compatible with this multimodal technology and is also available to paid users of Open AI’s GPT-4. Currently, the main reports revolve around annotations of images and videos. However, there is also mention of the potential of AI trained on medical data [ 66 ]. The integration of multimodal technology into LLM, or AI in medical imaging, might bring a new dimension to radiology. According to prior research, the integration of multimodal technology has the potential to revolutionize the precision of image diagnosis in the field of diagnostic radiology [ 67 ]. Moreover, its implementation could substantially reduce the time required for image analysis. However, given that, as with research work, LLMs are not responsible and may produce reports that seem authoritative with a completely different meaning through hallucinations, etc., and given that multimodal technology is prepared to be used by people with no knowledge of the radiology field, it Given that multimodal technology may prepare people with no knowledge of radiology to use it, legal development and guidelines might be needed for the application of this technology in radiology.
Another very promising outcome is the mitigation of LLM hallucinations. One way to overcome hallucinations in LLMs is by improving the training data. The quality and diversity of the training data play a significant role in the performance of these models. There have been reports on LLMs specific to the medical side, and if these models are developed, hallucination could be significantly reduced. Another method is to combine with search. The integration of search into LLMs can help in reducing the frequency of hallucinations by recognizing and rectifying incorrect or nonsensical generated text. The third approach is to refine the model architecture and learning methods of the neural network. Recent literature has reported the potential for significantly improved performance over existing LLMs by combining conventional neural network methods with meta-learning for compositionality [ 68 ]. For instance, it has the potential to operate efficiently even when faced with unfamiliar words or concepts, thereby substantially minimizing the requisite volume of learning data. It is anticipated that such advancements will persistently emerge in the future.
As the development of LLMs is expected to advance further, it is also anticipated that the potential risks associated with this will increase. As mentioned earlier, examples such as radiology report generation, scientific review, and medical consultation on social media, there is a possibility that LLMs will be used not as copilots, but as almost independent agents for some purposes, and in extreme cases, it cannot be denied that even those who have no knowledge of radiology or medicine may provide services. However, there is no method to completely solve the problems of LLMs such as hallucinations and biases at present. In addition, LLMs implicitly memorize the information contained in the training data, and there is a possibility that personal information or medical information may be included in the generated text. Even if LLMs develop, their output may be inaccurate or inappropriate and may affect the health and safety of patients. Developers and users of LLMs in radiology work should use them with a correct understanding of their capabilities and limitations, and checking the output of LLMs by radiologists will continue to be essential in the future.
LLMs have the potential to bring innovation to the medical field, but on the other hand, they also have the potential to bring crisis to the medical field. The development and application of LLMs to the medical field should be done carefully and responsibly, but at present, the rapid development of technology has not caught up with the establishment of guidelines and laws for the use of LLMs in radiology work. As guidelines and submission rules have been changed for the paper submission and the scientific review, similar preparations are urgently needed for daily radiology work considering the future development of LLMs.
As LLM continues to mature and evolve, its incorporation into diagnostic radiology harbors immense potential for advancing this field. However, the speed at which technology has developed has outpaced the establishment of consensus, guidelines, and legislation for LLM use. Currently, LLM models serve a “copilot” role, but shortly, they will gain the ability to function as an autonomous “agent”. as demonstrated by tasks such as report generation and paper review mentioned earlier. Nevertheless, this advancement encompasses an array of potential pitfalls concerning medical safety and ethics. A thorough understanding of LLM by radiologists and collaboration with experts is crucial for successfully integrating LLM into radiology.
Abbreviations
- Artificial intelligence
Bidirectional encoder representations from transformers
Convolutional neural networks
Computed tomography
- Deep learning
Generative pre-trained transformer
International conference on learning representations
Japan radiology board examination
Large language models
Long short-term memory
Magnetic resonance imaging
Natural language processing
Recurrent neural networks
Reinforcement learning from human feedback
Radiological society of North America
United States medical licensing examination
Nakaura T, Higaki T, Awai K, Ikeda O, Yamashita Y. A primer for understanding radiology articles about machine learning and deep learning. Diagn Interv Imaging. 2020;101:765–70.
Article PubMed Google Scholar
Williams RJ, Zipser D. A learning algorithm for continually running fully recurrent neural networks. Neural Comput. 1989;1:270–80.
Article Google Scholar
Lu L, Wang X, Carneiro G, Yang L. Deep learning and convolutional neural networks for medical imaging and clinical informatics. Cham: Springer Nature; 2019.
Book Google Scholar
Higaki T, Nakamura Y, Tatsugami F, Nakaura T, Awai K. Improvement of image quality at CT and MRI using deep learning. Jpn J Radiol. 2019;37:73–80.
Ozaki J, Fujioka T, Yamaga E, Hayashi A, Kujiraoka Y, Imokawa T, et al. Deep learning method with a convolutional neural network for image classification of normal and metastatic axillary lymph nodes on breast ultrasonography. Jpn J Radiol. 2022;40:814–22.
Ishihara M, Shiiba M, Maruno H, Kato M, Ohmoto-Sekine Y, Antoine C, et al. Detection of intracranial aneurysms using deep learning-based CAD system: usefulness of the scores of CNN’s final layer for distinguishing between aneurysm and infundibular dilatation. Jpn J Radiol. 2023;41:131–41.
Koretsune Y, Sone M, Sugawara S, Wakatsuki Y, Ishihara T, Hattori C, et al. Validation of a convolutional neural network for the automated creation of curved planar reconstruction images along the main pancreatic duct. Jpn J Radiol. 2023;41:228–34.
Kitahara H, Nagatani Y, Otani H, Nakayama R, Kida Y, Sonoda A, et al. A novel strategy to develop deep learning for image super-resolution using original ultra-high-resolution computed tomography images of lung as training dataset. Jpn J Radiol. 2022;40:38–47.
Nai Y-H, Loi HY, O’Doherty S, Tan TH, Reilhac A. Comparison of the performances of machine learning and deep learning in improving the quality of low dose lung cancer PET images. Jpn J Radiol. 2022;40:1290–9.
Yasaka K, Akai H, Sugawara H, Tajima T, Akahane M, Yoshioka N, et al. Impact of deep learning reconstruction on intracranial 1.5 T magnetic resonance angiography. Jpn J Radiol. 2022;40:476–83.
Kaga T, Noda Y, Mori T, Kawai N, Miyoshi T, Hyodo F, et al. Unenhanced abdominal low-dose CT reconstructed with deep learning-based image reconstruction: image quality and anatomical structure depiction. Jpn J Radiol. 2022;40:703–11.
Article CAS PubMed PubMed Central Google Scholar
Hosoi R, Yasaka K, Mizuki M, Yamaguchi H, Miyo R, Hamada A, et al. Deep learning reconstruction with single-energy metal artifact reduction in pelvic computed tomography for patients with metal hip prostheses. Jpn J Radiol. 2023;41:863–71.
Article PubMed PubMed Central Google Scholar
Hamabuchi N, Ohno Y, Kimata H, Ito Y, Fujii K, Akino N, et al. Effectiveness of deep learning reconstruction on standard to ultra-low-dose high-definition chest CT images [Internet]. Jpn J Radiol. 2023. https://doi.org/10.1007/s11604-023-01470-7 .
Uematsu T, Nakashima K, Harada TL, Nasu H, Igarashi T. Comparisons between artificial intelligence computer-aided detection synthesized mammograms and digital mammograms when used alone and in combination with tomosynthesis images in a virtual screening setting. Jpn J Radiol. 2022;41:63–70.
Oshima S, Fushimi Y, Miyake KK, Nakajima S, Sakata A, Okuchi S, et al. Denoising approach with deep learning-based reconstruction for neuromelanin-sensitive MRI: image quality and diagnostic performance. Jpn J Radiol. 2023;41:1216–25.
Nakao T, Hanaoka S, Nomura Y, Hayashi N, Abe O. Anomaly detection in chest 18F-FDG PET/CT by Bayesian deep learning. Jpn J Radiol. 2022;40:730–9.
Toda N, Hashimoto M, Iwabuchi Y, Nagasaka M, Takeshita R, Yamada M, et al. Validation of deep learning-based computer-aided detection software use for interpretation of pulmonary abnormalities on chest radiographs and examination of factors that influence readers’ performance and final diagnosis. Jpn J Radiol. 2023;41:38–44.
Azuma M, Nakada H, Takei M, Nakamura K, Katsuragawa S, Shinkawa N, et al. Detection of acute rib fractures on CT images with convolutional neural networks: effect of location and type of fracture and reader’s experience. Emerg Radiol [Internet]. 2022. Accessed 3 Nov 2023;29. Available from: https://pubmed.ncbi.nlm.nih.gov/34855002/
Goto M, Sakai K, Toyama Y, Nakai Y, Yamada K. Use of a deep learning algorithm for non-mass enhancement on breast MRI: comparison with radiologists’ interpretations at various levels. Jpn J Radiol. 2023;41:1094–103.
Chen J, Li K, Peng X, Li L, Yang H, Huang L, et al. A transfer learning approach for staging diagnosis of anterior cruciate ligament injury on a new modified MR dual precision positioning of thin-slice oblique sagittal FS-PDWI sequence. Jpn J Radiol. 2023;41:637–47.
Liu Z, Liu Y, Zhang W, Hong Y, Meng J, Wang J, et al. Deep learning for prediction of hepatocellular carcinoma recurrence after resection or liver transplantation: a discovery and validation study. Hepatol Int. 2022;16:577.
Article CAS PubMed Google Scholar
Zeng GL. A deep-network piecewise linear approximation formula. IEEE Access. 2021;9:120665–74.
Hochreiter S, Schmidhuber J. Long short-term memory. Neural Comput. 1997;9:1735–80.
Peng B, Alcaide E, Anthony Q, Albalak A, Arcadinho S, Cao H, et al. RWKV: reinventing RNNs for the transformer era [Internet]. 2023. Accessed 31 Oct 2023] Available from: http://arxiv.org/abs/2305.13048 .
Brown TB, Mann B, Ryder N, Subbiah M, Kaplan J, Dhariwal P, et al. Language Models are Few-Shot Learners [Internet]. 2020 [Accessed 31 Oct 2023]. Available from: http://arxiv.org/abs/2005.14165 .
Vaswani A, Shazeer N, Parmar N, Uszkoreit J, Jones L, Gomez AN, et al. Attention is all you need [Internet]. 2017 [Accessed 31 Oct 2023]. Available from: http://arxiv.org/abs/1706.03762 .
Jain SM. Introduction to transformers for NLP: with the hugging face library and models to solve problems. Apress; 2022
Ross Gruetzemacher Wichita State University, W. Frank Barton School of Business, David Paradice Auburn University, Harbert College of Business. Deep transfer learning & beyond: transformer language models in information systems research. ACM Comput Surv (CSUR). 2022. https://doi.org/10.1145/3505245 .
Improving language understanding with unsupervised learning [Internet]. Accessed 31 Oct 2023. Available from: https://openai.com/research/language-unsupervised
Radford A, Wu J, Child R, Luan D, Amodei D, Sutskever I. Language Models are unsupervised multitask learners. 2019. Accessed 31 Oct 2023. Available from: https://www.semanticscholar.org/paper/Language-Models-are-Unsupervised-Multitask-Learners-Radford-Wu/9405cc0d6169988371b2755e573cc28650d14dfe
Devlin J, Chang M-W, Lee K, Toutanova K. BERT: Pre-training of Deep Bidirectional Transformers for Language Understanding [Internet]. 2018 [Accessed 31 Oct 2023]. Available from: http://arxiv.org/abs/1810.04805
Agrawal A, Suzgun M, Mackey L, Kalai AT. Do language models know when they’re hallucinating references? [Internet]. 2023. Accessed 31 Oct 2023. Available from: http://arxiv.org/abs/2305.18248
Athaluri SA, Manthena SV, Kesapragada VSRKM, Yarlagadda V, Dave T, Duddumpudi RTS. Exploring the boundaries of reality: investigating the phenomenon of artificial intelligence hallucination in scientific writing through ChatGPT references. Cureus. 2023;15:e37432.
PubMed PubMed Central Google Scholar
McKenna N, Li T, Cheng L, Hosseini MJ, Johnson M, Steedman M. Sources of Hallucination by Large Language Models on Inference Tasks [Internet]. 2023 [Accessed 31 Oct 2023]. Available from: http://arxiv.org/abs/2305.14552
Azamfirei R, Kudchadkar SR, Fackler J. Large language models and the perils of their hallucinations. Crit Care. 2023;27:120.
Thirunavukarasu AJ, Ting DSJ, Elangovan K, Gutierrez L, Tan TF, Ting DSW. Large language models in medicine. Nat Med. 2023;29:1930–40.
Battaglia PW, Hamrick JB, Bapst V, Sanchez-Gonzalez A, Zambaldi V, Malinowski M, et al. Relational inductive biases, deep learning, and graph networks [Internet]. 2018. Accessed Oct 31 2023. Available from: http://arxiv.org/abs/1806.01261
Ueda D, Kakinuma T, Fujita S, Kamagata K, Fushimi Y, Ito R, et al. Fairness of artificial intelligence in healthcare: review and recommendations. Jpn J Radiol. 2023;42:1–13.
Google Scholar
Stiennon N, Ouyang L, Wu J, Ziegler DM, Lowe R, Voss C, et al. Learning to summarize from human feedback [Internet]. 2020. Accessed 31 Oct 2023. Available from: http://arxiv.org/abs/2009.01325
Kung TH, Cheatham M, Medenilla A, Sillos C, De Leon L, Elepaño C, et al. Performance of ChatGPT on USMLE: potential for AI-assisted medical education using large language models. PLOS Digit Health. 2023;2: e0000198.
Ayers JW, Poliak A, Dredze M, Leas EC, Zhu Z, Kelley JB, et al. Comparing physician and artificial intelligence chatbot responses to patient questions posted to a public social media forum. JAMA Intern Med. 2023;183:589–96.
Parikh JR, Wolfman D, Bender CE, Arleo E. Radiologist burnout according to surveyed radiology practice leaders. J Am Coll Radiol. 2020;17:78–81.
Bhayana R, Krishna S, Bleakney RR. Performance of ChatGPT on a radiology board-style examination: insights into current strengths and limitations. Radiology. 2023;307: e230582.
Toyama Y, Harigai A, Abe M, Nagano M, Kawabata M, Seki Y, et al. Performance evaluation of ChatGPT, GPT-4, and Bard on the official board examination of the Japan Radiology Society. Jpn J Radiol. 2023. https://doi.org/10.1007/s11604-023-01491-2 .
Kufel J, Paszkiewicz I, Bielówka M, Bartnikowska W, Janik M, Stencel M, et al. Will ChatGPT pass the Polish specialty exam in radiology and diagnostic imaging? Insights into strengths and limitations. Pol J Radiol. 2023;88:e430–4.
Seghier ML. ChatGPT: not all languages are equal. Nature. 2023;615:216.
Akinci D’Antonoli T, Stanzione A, Bluethgen C, Vernuccio F, Ugga L, Klontzas ME, et al. Large language models in radiology: fundamentals, applications, ethical considerations, risks, and future directions. Diagn Interv Radiol. 2023. https://doi.org/10.4274/dir.2023.232417 .
López-Úbeda P, Martín-Noguerol T, Juluru K, Luna A. Natural language processing in radiology: update on clinical applications. J Am Coll Radiol. 2022;19:1271–85.
Tinn R, Cheng H, Gu Y, Usuyama N, Liu X, Naumann T, et al. Fine-tuning large neural language models for biomedical natural language processing. Patterns (N Y). 2023;4: 100729.
Mahbub M, Srinivasan S, Danciu I, Peluso A, Begoli E, Tamang S, et al. Unstructured clinical notes within the 24 hours since admission predict short, mid & long-term mortality in adult ICU patients. PLoS ONE. 2022;17: e0262182.
Gertz RJ, Bunck AC, Lennartz S, Dratsch T, Iuga A-I, Maintz D, et al. GPT-4 for automated determination of radiological study and protocol based on radiology request forms: a feasibility study. Radiology. 2023;307: e230877.
Doi K, Takegawa H, Yui M, Anetai Y, Koike Y, Nakamura S, et al. Deep learning-based detection of patients with bone metastasis from Japanese radiology reports. Jpn J Radiol. 2023;41:900–8.
Adams LC, Truhn D, Busch F, Kader A, Niehues SM, Makowski MR, et al. Leveraging GPT-4 for post hoc transformation of free-text radiology reports into structured reporting: a multilingual feasibility study. Radiology. 2023;307: e230725.
Lyu Q, Tan J, Zapadka ME, Ponnatapura J, Niu C, Myers KJ, et al. Translating radiology reports into plain language using ChatGPT and GPT-4 with prompt learning: results, limitations, and potential. Vis Comput Ind Biomed Art. 2023;6:9.
Wang X, Peng Y, Lu L, Lu Z, Summers RM. TieNet: Text-image embedding network for common thorax disease classification and reporting in chest X-rays. 2018 IEEE/CVF conference on computer vision and pattern recognition [Internet]. IEEE; 2018 [Accessed 26 Oct 2023]. Available from: https://ieeexplore.ieee.org/document/8579041/ .
Alfarghaly O, Khaled R, Elkorany A, Helal M, Fahmy A. Automated radiology report generation using conditioned transformers. Inform Med Unlocked. 2021;24: 100557.
Sirshar M, Paracha MFK, Akram MU, Alghamdi NS, Zaidi SZY, Fatima T. Attention based automated radiology report generation using CNN and LSTM. PLoS ONE. 2022;17: e0262209.
Nakaura T, Yoshida N, Kobayashi N, Shiraishi K, Nagayama Y, Uetani H, et al. Preliminary assessment of automated radiology report generation with generative pre-trained transformers: comparing results to radiologist-generated reports. Jpn J Radiol. 2023. https://doi.org/10.1007/s11604-023-01487-y .
Hartung MP, Bickle IC, Gaillard F, Kanne JP. How to create a great radiology report. Radiographics. 2020;40:1658–70.
Else H. Abstracts written by ChatGPT fool scientists. Nature. 2023;613:423–423.
Hwang SI, Lim JS, Lee RW, Matsui Y, Iguchi T, Hiraki T, et al. Is ChatGPT a “Fire of prometheus” for non-native english-speaking researchers in academic writing? Korean J Radiol. 2023;24:952–9.
Liang W, Zhang Y, Cao H, Wang B, Ding D, Yang X, et al. Can large language models provide useful feedback on research papers? A large-scale empirical analysis [Internet]. arXiv.org. 2023. Accessed 27 Oct 2023. Available from: https://arxiv.org/pdf/2310.01783.pdf
Stokel-Walker C. ChatGPT listed as author on research papers: many scientists disapprove. Nature. 2023. https://doi.org/10.1038/d41586-023-00107-z .
Thorp HH. ChatGPT is fun, but not an author. Science. 2023;379:313.
Moy L. Guidelines for use of large language models by authors, reviewers, and editors: considerations for imaging journals. Radiology. 2023;309: e239024.
Tu T, Azizi S, Driess D, Schaekermann M, Amin M, Chang P-C, et al. Towards generalist Biomedical AI [Internet]. 2023. Accessed 30 Oct 2023. Available from: http://arxiv.org/abs/2307.14334
Khader F, Müller-Franzes G, Wang T, Han T, Tayebi Arasteh S, Haarburger C, et al. Multimodal deep learning for integrating chest radiographs and clinical parameters: a case for transformers. Radiology. 2023;309: e230806.
Lake BM, Baroni M. Human-like systematic generalization through a meta-learning neural network. Nature. 2023. https://doi.org/10.1038/s41586-023-06668-3 .
Download references
Acknowledgements
We used the DeepL and GPT-4 for Japanese-English translation and English proofreading. The generated text was read, revised, and proofed by the authors.
No funding.
Author information
Authors and affiliations.
Department of Central Radiology, Kumamoto University Hospital, Honjo 1-1-1, Kumamoto, 860-8556, Japan
Takeshi Nakaura
Department of Radiology, Nagoya University Graduate School of Medicine, Nagoya, Aichi, Japan
Rintaro Ito, Mariko Kawamura & Shinji Naganawa
Department of Diagnostic and Interventional Radiology, Graduate School of Medicine, Osaka Metropolitan University, 1‑4‑3 Asahi‑Machi, Abeno‑ku, Osaka, 545‑8585, Japan
Department of Radiology, Keio University School of Medicine, Shinjuku‑ku, Tokyo, Japan
Taiki Nozaki
Department of Diagnostic Imaging and Nuclear Medicine, Kyoto University Graduate School of Medicine, Sakyoku, Kyoto, Japan
Yasutaka Fushimi
Department of Radiology, Faculty of Medicine, Dentistry and Pharmaceutical Sciences, Okayama University, Kita‑ku, Okayama, Japan
Yusuke Matsui
Department of Radiology, Osaka University Graduate School of Medicine, Suita City, Osaka, Japan
Masahiro Yanagawa & Takahiro Tsuboyama
Department of Radiology, Shinshu University School of Medicine, Matsumoto, Nagano, Japan
Akira Yamada
Department of Diagnostic and Interventional Radiology, Hokkaido University Hospital, Sapporo, Japan
Noriyuki Fujima
Department of Diagnostic Radiology, Hiroshima University, Minami‑ku, Hiroshima, Japan
Fuminari Tatsugami
Department of Diagnostic Imaging, Graduate School of Medicine, Hokkaido University, Kita‑ku, Sapporo, Hokkaido, Japan
Kenji Hirata
Department of Radiology, University of Tokyo, Bunkyo‑ku, Tokyo, Japan
Shohei Fujita
Department of Radiology, Juntendo University Graduate School of Medicine, Bunkyo‑ku, Tokyo, Japan
Koji Kamagata
Department of Diagnostic Radiology, Tokyo Medical and Dental University, Bunkyo‑ku, Tokyo, Japan
Tomoyuki Fujioka
You can also search for this author in PubMed Google Scholar
Corresponding author
Correspondence to Takeshi Nakaura .
Ethics declarations
Conflict of interest.
No conflicts of interest statement.
Additional information
Publisher's note.
Springer Nature remains neutral with regard to jurisdictional claims in published maps and institutional affiliations.
Rights and permissions
This article is published under an open access license. Please check the 'Copyright Information' section either on this page or in the PDF for details of this license and what re-use is permitted. If your intended use exceeds what is permitted by the license or if you are unable to locate the licence and re-use information, please contact the Rights and Permissions team .
About this article
Nakaura, T., Ito, R., Ueda, D. et al. The impact of large language models on radiology: a guide for radiologists on the latest innovations in AI. Jpn J Radiol (2024). https://doi.org/10.1007/s11604-024-01552-0
Download citation
Received : 15 November 2023
Accepted : 21 February 2024
Published : 29 March 2024
DOI : https://doi.org/10.1007/s11604-024-01552-0
Share this article
Anyone you share the following link with will be able to read this content:
Sorry, a shareable link is not currently available for this article.
Provided by the Springer Nature SharedIt content-sharing initiative
- Diagnostic radiology
- Large language model
- Radiological workflow
- Find a journal
- Publish with us
- Track your research

- New To ASRT?
- Member ID
- Earn CE Credits
- Track CE Credits
- Request for CE Approval
- Publications
- Professional Practice
- Legislation, Regulations & Advocacy
- Careers in Radiologic Technology
- Plan Your Career
- JobBank®
- Salary Estimator
- Governance, Chapters & Affiliates
- Museum & Archives
- Careers at ASRT
- Advertising & Sponsorship
- ASRT Foundation
- Join or Renew
- Member Benefits
- Membership Categories
- ASRT Home /
News, Research and Publications
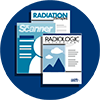
News, Research and Publications Publications
ASRT members receive our publications as part of their membership. If you are a radiologic science professional or student, or you are employed in the technical, educational, managerial or corporate aspects of the industry, we encourage you to join today .
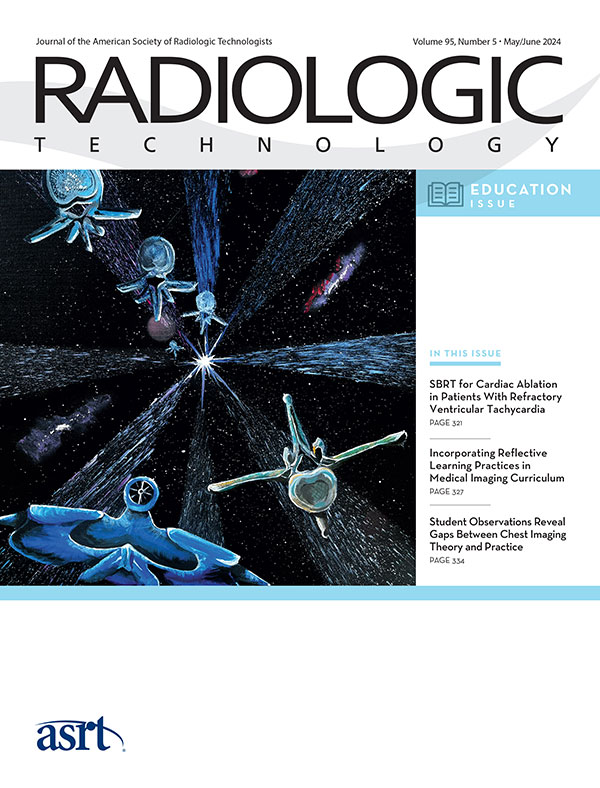
Radiologic Technology

This bimonthly scholarly journal features peer-reviewed research and practical columns spanning the radiologic sciences.
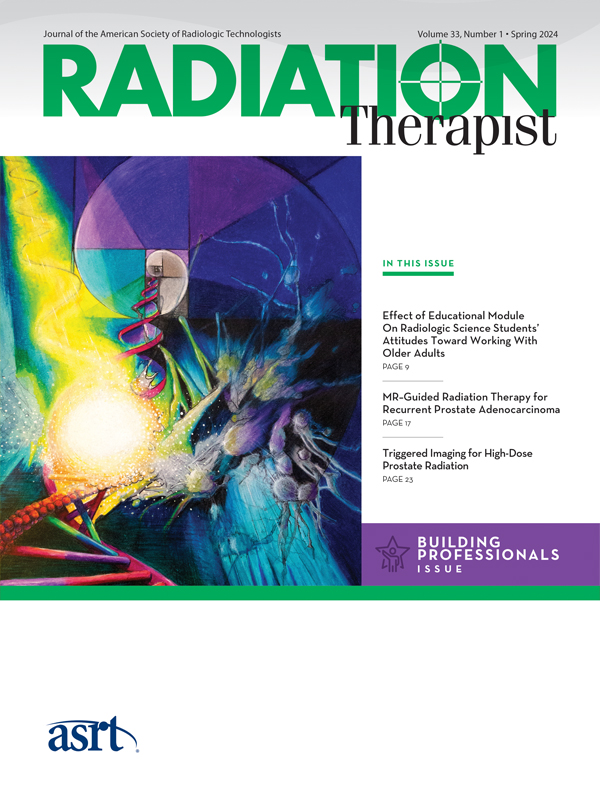
Radiation Therapist
This semiannual scholarly journal includes peer-reviewed research and helpful columns on radiation therapy practice.
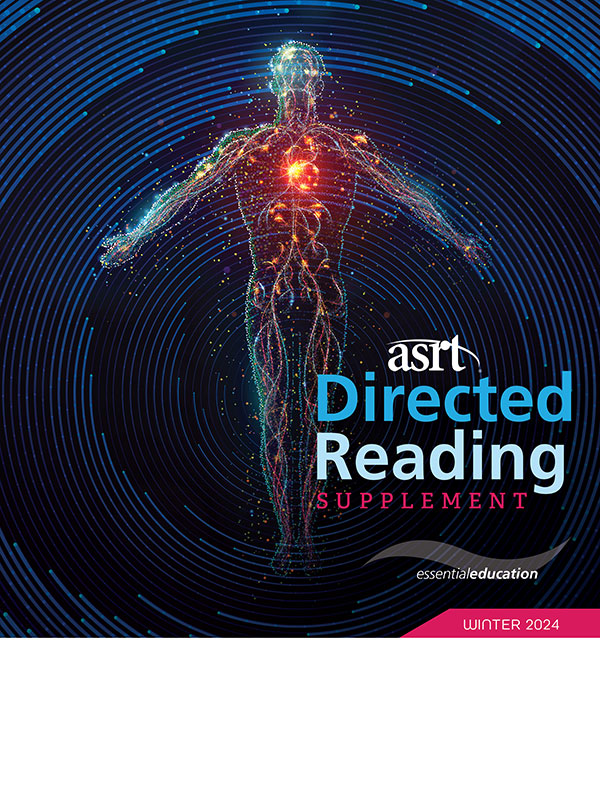
Directed Reading Supplement
The Directed Reading Supplement contains six CE articles per issue and is mailed twice a year.
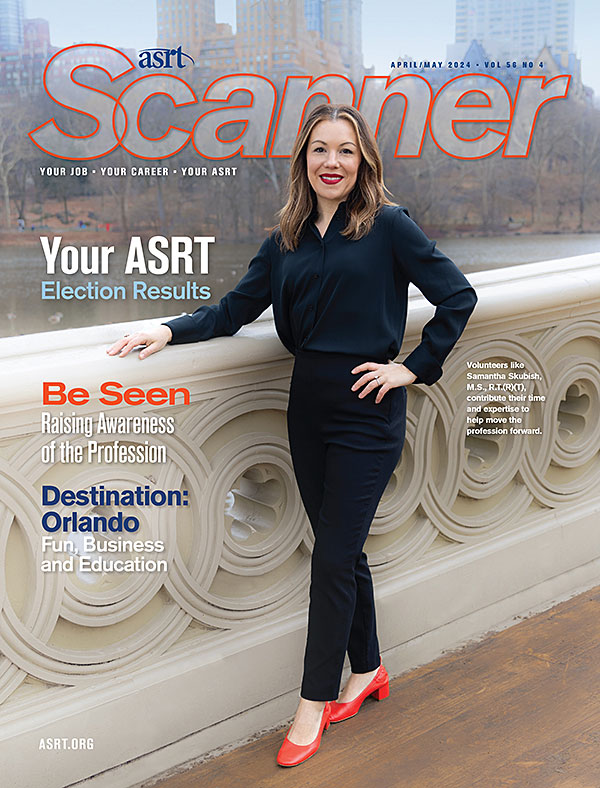
ASRT Scanner
This bimonthly newsmagazine covers the Society and includes information for and about medical imaging and radiation therapy professionals.
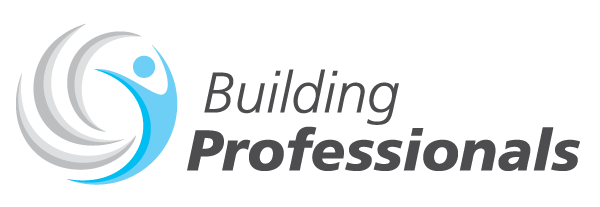
Building Professionals
Learn more about publishing in the student section of the scholarly journals. Articles published in this section focus on fostering professionalism in students and can be written by students or educators.
Author Guide
Whether you'd like to write for ASRT's scholarly journals or for the member newsmagazine, the pages in this section will guide you through ethical considerations, permissions, formatting requirements and other helpful information to make sure your submission is complete and correct.
By clicking the button, you acknowledge that you have read and agreed to ASRT's ethical considerations .
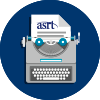
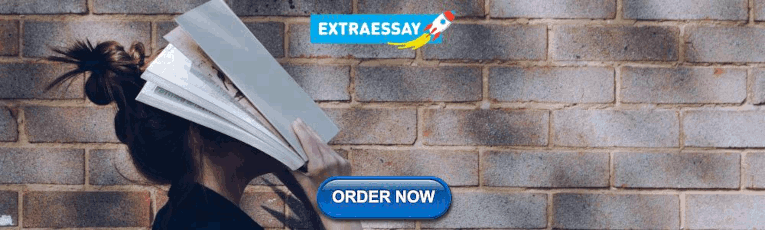
Newsletters
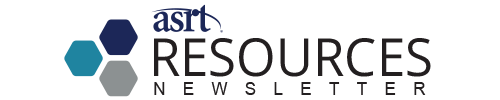
Monthly electronic newsletter for members. Each issue introduces readers to member benefits, advocacy news, upcoming events, continuing education opportunities and other information related to your career and professional development.
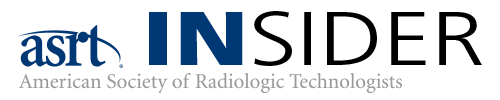
Quarterly newsletter just for members with the Premium membership option. Be the first to know about new research study results, exclusive ASRT news, updates and benefits.
Advertising
Additional Information
- Subscriptions, Reprints and Back Issues
- Radiologic Technology and Radiation Therapist Editorial Review Boards
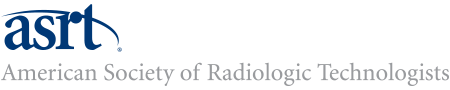
The mission of the American Society of Radiologic Technologists is to advance and elevate the medical imaging and radiation therapy profession and to enhance the quality and safety of patient care.
ASRT strives to be the premier professional association for the medical imaging and radiation therapy community through education, advocacy, research and innovation.
15000 Central Ave. SE Albuquerque, NM 87123-3909
- 800-444-2778
505-298-4500
- 505-298-5063 fax
- [email protected]
Member Services Hours: 7:30 a.m. to 4:30 p.m. Mountain time, Monday-Friday
Earn and Track CE
- ASRT Directed Reading Quizzes
- Events and Conferences
Featured CE Courses
- My Learning
Standards and Regulations
- Regulatory and Legislative News
- ASRT Advocacy
Career Center
- ASRT JobBank®
- Who ASRT Represents
- Why Join ASRT
- ASRT Affiliates
- Doing Business With ASRT
- Computed Tomography
- Mammography Toolkit
- Clinical Instructor Academy
- Fluoroscopy
- Radiography
- California Fluoroscopy Permit
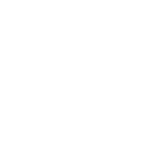
Have a Question?
Member Services Hours: 7:30 a.m. to 4:30 p.m. Mountain Time, Monday-Friday
© 2024 American Society of Radiologic Technologists (ASRT) | Privacy | Refund Policy | Terms | Help | Sitemap | Feedback | Contact
Radiology Research Paper Topics
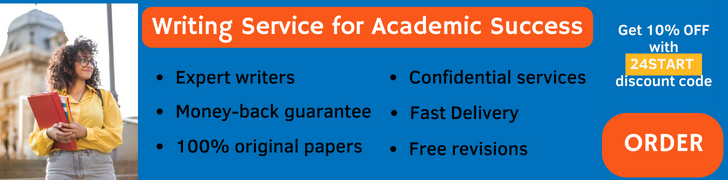
Radiology research paper topics encompass a wide range of fascinating areas within the field of medical imaging. This page aims to provide students studying health sciences with a comprehensive collection of radiology research paper topics to inspire and guide their research endeavors. By delving into various categories and exploring ten thought-provoking topics within each, students can gain insights into the diverse research possibilities in radiology. From advancements in imaging technology to the evaluation of diagnostic accuracy and the impact of radiological interventions, these topics offer a glimpse into the exciting world of radiology research. Additionally, expert advice is provided to help students choose the most suitable research topics and navigate the process of writing a research paper in radiology. By leveraging iResearchNet’s writing services, students can further enhance their research papers with professional assistance, ensuring the highest quality and adherence to academic standards. Explore the realm of radiology research paper topics and unleash your potential to contribute to the advancement of medical imaging and patient care.
100 Radiology Research Paper Topics
Radiology encompasses a broad spectrum of imaging techniques used to diagnose diseases, monitor treatment progress, and guide interventions. This comprehensive list of radiology research paper topics serves as a valuable resource for students in the field of health sciences who are seeking inspiration and guidance for their research endeavors. The following ten categories highlight different areas within radiology, each containing ten thought-provoking topics. Exploring these topics will provide students with a deeper understanding of the diverse research possibilities and current trends within the field of radiology.
Academic Writing, Editing, Proofreading, And Problem Solving Services
Get 10% off with 24start discount code.
Diagnostic Imaging Techniques
- Comparative analysis of imaging modalities: CT, MRI, and PET-CT.
- The role of artificial intelligence in radiological image interpretation.
- Advancements in digital mammography for breast cancer screening.
- Emerging techniques in nuclear medicine imaging.
- Image-guided biopsy: Enhancing accuracy and safety.
- Application of radiomics in predicting treatment response.
- Dual-energy CT: Expanding diagnostic capabilities.
- Radiological evaluation of traumatic brain injuries.
- Imaging techniques for evaluating cardiovascular diseases.
- Radiographic evaluation of pulmonary nodules: Challenges and advancements.
Interventional Radiology
- Minimally invasive treatments for liver tumors: Embolization techniques.
- Radiofrequency ablation in the management of renal cell carcinoma.
- Role of interventional radiology in the treatment of peripheral artery disease.
- Transarterial chemoembolization in hepatocellular carcinoma.
- Evaluation of uterine artery embolization for the treatment of fibroids.
- Percutaneous vertebroplasty and kyphoplasty: Efficacy and complications.
- Endovascular repair of abdominal aortic aneurysms: Long-term outcomes.
- Interventional radiology in the management of deep vein thrombosis.
- Transcatheter aortic valve replacement: Imaging considerations.
- Emerging techniques in interventional oncology.
Radiation Safety and Dose Optimization
- Strategies for reducing radiation dose in pediatric imaging.
- Imaging modalities with low radiation exposure: Current advancements.
- Effective use of dose monitoring systems in radiology departments.
- The impact of artificial intelligence on radiation dose optimization.
- Optimization of radiation therapy treatment plans: Balancing efficacy and safety.
- Radioprotective measures for patients and healthcare professionals.
- The role of radiology in addressing radiation-induced risks.
- Evaluating the long-term effects of radiation exposure in diagnostic imaging.
- Radiation dose tracking and reporting: Implementing best practices.
- Patient education and communication regarding radiation risks.
Radiology in Oncology
- Imaging techniques for early detection and staging of lung cancer.
- Quantitative imaging biomarkers for predicting treatment response in solid tumors.
- Radiogenomics: Linking imaging features to genetic profiles in cancer.
- The role of imaging in assessing tumor angiogenesis.
- Radiological evaluation of lymphoma: Challenges and advancements.
- Imaging-guided interventions in the treatment of hepatocellular carcinoma.
- Assessment of tumor heterogeneity using functional imaging techniques.
- Radiomics and machine learning in predicting treatment outcomes in cancer.
- Multimodal imaging in the evaluation of brain tumors.
- Imaging surveillance after cancer treatment: Optimizing follow-up protocols.
Radiology in Musculoskeletal Disorders
- Imaging modalities in the evaluation of sports-related injuries.
- The role of imaging in diagnosing and monitoring rheumatoid arthritis.
- Assessment of bone health using dual-energy X-ray absorptiometry (DXA).
- Imaging techniques for evaluating osteoarthritis progression.
- Imaging-guided interventions in the management of musculoskeletal tumors.
- Role of imaging in diagnosing and managing spinal disorders.
- Evaluation of traumatic injuries using radiography, CT, and MRI.
- Imaging of joint prostheses: Complications and assessment techniques.
- Imaging features and classifications of bone fractures.
- Musculoskeletal ultrasound in the diagnosis of soft tissue injuries.
Neuroradiology
- Advanced neuroimaging techniques for early detection of neurodegenerative diseases.
- Imaging evaluation of acute stroke: Current guidelines and advancements.
- Role of functional MRI in mapping brain functions.
- Imaging of brain tumors: Classification and treatment planning.
- Diffusion tensor imaging in assessing white matter integrity.
- Neuroimaging in the evaluation of multiple sclerosis.
- Imaging techniques for the assessment of epilepsy.
- Radiological evaluation of neurovascular diseases.
- Imaging of cranial nerve disorders: Diagnosis and management.
- Radiological assessment of developmental brain abnormalities.
Pediatric Radiology
- Radiation dose reduction strategies in pediatric imaging.
- Imaging evaluation of congenital heart diseases in children.
- Role of imaging in the diagnosis and management of pediatric oncology.
- Imaging of pediatric gastrointestinal disorders.
- Evaluation of developmental hip dysplasia using ultrasound and radiography.
- Imaging features and management of pediatric musculoskeletal infections.
- Neuroimaging in the assessment of pediatric neurodevelopmental disorders.
- Radiological evaluation of pediatric respiratory conditions.
- Imaging techniques for the evaluation of pediatric abdominal emergencies.
- Imaging-guided interventions in pediatric patients.
Breast Imaging
- Advances in digital mammography for early breast cancer detection.
- The role of tomosynthesis in breast imaging.
- Imaging evaluation of breast implants: Complications and assessment.
- Radiogenomic analysis of breast cancer subtypes.
- Contrast-enhanced mammography: Diagnostic benefits and challenges.
- Emerging techniques in breast MRI for high-risk populations.
- Evaluation of breast density and its implications for cancer risk.
- Role of molecular breast imaging in dense breast tissue evaluation.
- Radiological evaluation of male breast disorders.
- The impact of artificial intelligence on breast cancer screening.
Cardiac Imaging
- Imaging evaluation of coronary artery disease: Current techniques and challenges.
- Role of cardiac CT angiography in the assessment of structural heart diseases.
- Imaging of cardiac tumors: Diagnosis and treatment considerations.
- Advanced imaging techniques for assessing myocardial viability.
- Evaluation of valvular heart diseases using echocardiography and MRI.
- Cardiac magnetic resonance imaging in the evaluation of cardiomyopathies.
- Role of nuclear cardiology in the assessment of cardiac function.
- Imaging evaluation of congenital heart diseases in adults.
- Radiological assessment of cardiac arrhythmias.
- Imaging-guided interventions in structural heart diseases.
Abdominal and Pelvic Imaging
- Evaluation of hepatobiliary diseases using imaging techniques.
- Imaging features and classification of renal masses.
- Radiological assessment of gastrointestinal bleeding.
- Imaging evaluation of pancreatic diseases: Challenges and advancements.
- Evaluation of pelvic floor disorders using MRI and ultrasound.
- Role of imaging in diagnosing and staging gynecological cancers.
- Imaging of abdominal and pelvic trauma: Current guidelines and techniques.
- Radiological evaluation of genitourinary disorders.
- Imaging features of abdominal and pelvic infections.
- Assessment of abdominal and pelvic vascular diseases using imaging techniques.
This comprehensive list of radiology research paper topics highlights the vast range of research possibilities within the field of medical imaging. Each category offers unique insights and avenues for exploration, enabling students to delve into various aspects of radiology. By choosing a topic of interest and relevance, students can contribute to the advancement of medical imaging and patient care. The provided topics serve as a starting point for students to engage in in-depth research and produce high-quality research papers.
Radiology: Exploring the Range of Research Paper Topics
Introduction: Radiology plays a crucial role in modern healthcare, providing valuable insights into the diagnosis, treatment, and monitoring of various medical conditions. As a dynamic and rapidly evolving field, radiology offers a wide range of research opportunities for students in the health sciences. This article aims to explore the diverse spectrum of research paper topics within radiology, shedding light on the current trends, innovations, and challenges in the field.
Radiology in Diagnostic Imaging : Diagnostic imaging is one of the core areas of radiology, encompassing various modalities such as X-ray, computed tomography (CT), magnetic resonance imaging (MRI), ultrasound, and nuclear medicine. Research topics in this domain may include advancements in imaging techniques, comparative analysis of modalities, radiomics, and the integration of artificial intelligence in image interpretation. Students can explore how these technological advancements enhance diagnostic accuracy, improve patient outcomes, and optimize radiation exposure.
Interventional Radiology : Interventional radiology focuses on minimally invasive procedures performed under image guidance. Research topics in this area can cover a wide range of interventions, such as angioplasty, embolization, radiofrequency ablation, and image-guided biopsies. Students can delve into the latest techniques, outcomes, and complications associated with interventional procedures, as well as explore the emerging role of interventional radiology in managing various conditions, including vascular diseases, cancer, and pain management.
Radiation Safety and Dose Optimization : Radiation safety is a critical aspect of radiology practice. Research in this field aims to minimize radiation exposure to patients and healthcare professionals while maintaining optimal diagnostic image quality. Topics may include strategies for reducing radiation dose in pediatric imaging, dose monitoring systems, the impact of artificial intelligence on radiation dose optimization, and radioprotective measures. Students can investigate how to strike a balance between effective imaging and patient safety, exploring advancements in dose reduction techniques and the implementation of best practices.
Radiology in Oncology : Radiology plays a vital role in the diagnosis, staging, and treatment response assessment in cancer patients. Research topics in this area can encompass the use of imaging techniques for early detection, tumor characterization, response prediction, and treatment planning. Students can explore the integration of radiomics, machine learning, and molecular imaging in oncology research, as well as advancements in functional imaging and image-guided interventions.
Radiology in Neuroimaging : Neuroimaging is a specialized field within radiology that focuses on imaging the brain and central nervous system. Research topics in neuroimaging can cover areas such as stroke imaging, neurodegenerative diseases, brain tumors, neurovascular disorders, and functional imaging for mapping brain functions. Students can explore the latest imaging techniques, image analysis tools, and their clinical applications in understanding and diagnosing various neurological conditions.
Radiology in Musculoskeletal Imaging : Musculoskeletal imaging involves the evaluation of bone, joint, and soft tissue disorders. Research topics in this area can encompass imaging techniques for sports-related injuries, arthritis, musculoskeletal tumors, spinal disorders, and trauma. Students can explore the role of advanced imaging modalities such as MRI and ultrasound in diagnosing and managing musculoskeletal conditions, as well as the use of imaging-guided interventions for treatment.
Pediatric Radiology : Pediatric radiology focuses on imaging children, who have unique anatomical and physiological considerations. Research topics in this field may include radiation dose reduction strategies in pediatric imaging, imaging evaluation of congenital anomalies, pediatric oncology imaging, and imaging assessment of developmental disorders. Students can explore how to tailor imaging protocols for children, minimize radiation exposure, and improve diagnostic accuracy in pediatric patients.
Breast Imaging : Breast imaging is essential for the early detection and diagnosis of breast cancer. Research topics in this area can cover advancements in mammography, tomosynthesis, breast MRI, and molecular imaging. Students can explore topics related to breast density, imaging-guided biopsies, breast cancer screening, and the impact of artificial intelligence in breast imaging. Additionally, they can investigate the use of imaging techniques for evaluating breast implants and assessing high-risk populations.
Cardiac Imaging : Cardiac imaging focuses on the evaluation of heart structure and function. Research topics in this field may include imaging techniques for coronary artery disease, valvular heart diseases, cardiomyopathies, and cardiac tumors. Students can explore the role of cardiac CT, MRI, nuclear cardiology, and echocardiography in diagnosing and managing various cardiac conditions. Additionally, they can investigate the use of imaging in guiding interventional procedures and assessing treatment outcomes.
Abdominal and Pelvic Imaging : Abdominal and pelvic imaging involves the evaluation of organs and structures within the abdominal and pelvic cavities. Research topics in this area can encompass imaging of the liver, kidneys, gastrointestinal tract, pancreas, genitourinary system, and pelvic floor. Students can explore topics related to imaging techniques, evaluation of specific diseases or conditions, and the role of imaging in guiding interventions. Additionally, they can investigate emerging modalities such as elastography and diffusion-weighted imaging in abdominal and pelvic imaging.
Radiology offers a vast array of research opportunities for students in the field of health sciences. The topics discussed in this article provide a glimpse into the breadth and depth of research possibilities within radiology. By exploring these research areas, students can contribute to advancements in diagnostic accuracy, treatment planning, and patient care. With the rapid evolution of imaging technologies and the integration of artificial intelligence, the future of radiology research holds immense potential for improving healthcare outcomes.
Choosing Radiology Research Paper Topics
Introduction: Selecting a research topic is a crucial step in the journey of writing a radiology research paper. It determines the focus of your study and influences the impact your research can have in the field. To help you make an informed choice, we have compiled expert advice on selecting radiology research paper topics. By following these tips, you can identify a relevant and engaging research topic that aligns with your interests and contributes to the advancement of radiology knowledge.
- Identify Your Interests : Start by reflecting on your own interests within the field of radiology. Consider which subspecialties or areas of radiology intrigue you the most. Are you interested in diagnostic imaging, interventional radiology, radiation safety, oncology imaging, or any other specific area? Identifying your interests will guide you in selecting a topic that excites you and keeps you motivated throughout the research process.
- Stay Updated on Current Trends : Keep yourself updated on the latest advancements, breakthroughs, and emerging trends in radiology. Read scientific journals, attend conferences, and engage in discussions with experts in the field. By staying informed, you can identify gaps in knowledge or areas that require further investigation, providing you with potential research topics that are timely and relevant.
- Consult with Faculty or Mentors : Seek guidance from your faculty members or mentors who are experienced in the field of radiology. They can provide valuable insights into potential research areas, ongoing projects, and research gaps. Discuss your research interests with them and ask for their suggestions and recommendations. Their expertise and guidance can help you narrow down your research topic and refine your research question.
- Conduct a Literature Review : Conducting a thorough literature review is an essential step in choosing a research topic. It allows you to familiarize yourself with the existing body of knowledge, identify research gaps, and build a strong foundation for your study. Analyze recent research papers, systematic reviews, and meta-analyses related to radiology to identify areas that need further investigation or where controversies exist.
- Brainstorm Research Questions : Once you have gained an understanding of the current state of research in radiology, brainstorm potential research questions. Consider the gaps or controversies you identified during your literature review. Develop research questions that address these gaps and contribute to the existing knowledge. Ensure that your research questions are clear, focused, and answerable within the scope of your study.
- Consider the Practicality and Feasibility : When selecting a research topic, consider the practicality and feasibility of conducting the study. Evaluate the availability of resources, access to data, research facilities, and ethical considerations. Assess the time frame and potential constraints that may impact your research. Choosing a topic that is feasible within your given resources and time frame will ensure a successful and manageable research experience.
- Collaborate with Peers : Consider collaborating with your peers or forming a research group to enhance your research experience. Collaborative research allows for a sharing of ideas, resources, and expertise, fostering a supportive environment. By working together, you can explore more complex research topics, conduct multicenter studies, and generate more impactful findings.
- Seek Multidisciplinary Perspectives : Radiology intersects with various other medical disciplines. Consider exploring interdisciplinary research topics that integrate radiology with fields such as oncology, cardiology, neurology, or orthopedics. By incorporating multidisciplinary perspectives, you can address complex healthcare challenges and contribute to a broader understanding of patient care.
- Choose a Topic with Clinical Relevance : Select a research topic that has direct clinical relevance. Focus on topics that can potentially influence patient outcomes, improve diagnostic accuracy, optimize treatment strategies, or enhance patient safety. By choosing a clinically relevant topic, you can contribute to the advancement of radiology practice and have a positive impact on patient care.
- Seek Ethical Considerations : Ensure that your research topic adheres to ethical considerations in radiology research. Patient privacy, confidentiality, and informed consent should be prioritized when conducting studies involving human subjects. Familiarize yourself with the ethical guidelines and regulations specific to radiology research and ensure that your study design and data collection methods are in line with these principles.
Choosing a radiology research paper topic requires careful consideration and alignment with your interests, expertise, and the current trends in the field. By following the expert advice provided in this section, you can select a research topic that is engaging, relevant, and contributes to the advancement of radiology knowledge. Remember to consult with mentors, conduct a thorough literature review, and consider practicality and feasibility. With a well-chosen research topic, you can embark on an exciting journey of exploration, innovation, and contribution to the field of radiology.
How to Write a Radiology Research Paper
Introduction: Writing a radiology research paper requires a systematic approach and attention to detail. It is essential to effectively communicate your research findings, methodology, and conclusions to contribute to the body of knowledge in the field. In this section, we will provide you with valuable tips on how to write a successful radiology research paper. By following these guidelines, you can ensure that your paper is well-structured, informative, and impactful.
- Define the Research Question : Start by clearly defining your research question or objective. It serves as the foundation of your research paper and guides your entire study. Ensure that your research question is specific, focused, and relevant to the field of radiology. Clearly articulate the purpose of your study and its potential implications.
- Conduct a Thorough Literature Review : Before diving into writing, conduct a comprehensive literature review to familiarize yourself with the existing body of knowledge in your research area. Identify key studies, seminal papers, and relevant research articles that will support your research. Analyze and synthesize the literature to identify gaps, controversies, or areas for further investigation.
- Develop a Well-Structured Outline : Create a clear and well-structured outline for your research paper. An outline serves as a roadmap and helps you organize your thoughts, arguments, and evidence. Divide your paper into logical sections such as introduction, literature review, methodology, results, discussion, and conclusion. Ensure a logical flow of ideas and information throughout the paper.
- Write an Engaging Introduction : The introduction is the opening section of your research paper and should capture the reader’s attention. Start with a compelling hook that introduces the importance of the research topic. Provide background information, context, and the rationale for your study. Clearly state the research question or objective and outline the structure of your paper.
- Conduct Rigorous Methodology : Describe your research methodology in detail, ensuring transparency and reproducibility. Explain your study design, data collection methods, sample size, inclusion/exclusion criteria, and statistical analyses. Clearly outline the steps you took to ensure scientific rigor and address potential biases. Include any ethical considerations and institutional review board approvals, if applicable.
- Present Clear and Concise Results : Present your research findings in a clear, concise, and organized manner. Use tables, figures, and charts to visually represent your data. Provide accurate and relevant statistical analyses to support your results. Explain the significance and implications of your findings and their alignment with your research question.
- Analyze and Interpret Results : In the discussion section, analyze and interpret your research results in the context of existing literature. Compare and contrast your findings with previous studies, highlighting similarities, differences, and potential explanations. Discuss any limitations or challenges encountered during the study and propose areas for future research.
- Ensure Clear and Coherent Writing : Maintain clarity, coherence, and precision in your writing. Use concise and straightforward language to convey your ideas effectively. Avoid jargon or excessive technical terms that may hinder understanding. Clearly define any acronyms or abbreviations used in your paper. Ensure that each paragraph has a clear topic sentence and flows smoothly into the next.
- Citations and References : Properly cite all the sources used in your research paper. Follow the citation style recommended by your institution or the journal you intend to submit to (e.g., APA, MLA, or Chicago). Include in-text citations for direct quotes, paraphrased information, or any borrowed ideas. Create a comprehensive reference list at the end of your paper, following the formatting guidelines.
- Revise and Edit : Take the time to revise and edit your research paper before final submission. Review the content, structure, and organization of your paper. Check for grammatical errors, spelling mistakes, and typos. Ensure that your paper adheres to the specified word count and formatting guidelines. Seek feedback from colleagues or mentors to gain valuable insights and suggestions for improvement.
Conclusion: Writing a radiology research paper requires careful planning, attention to detail, and effective communication. By following the tips provided in this section, you can write a well-structured and impactful research paper in the field of radiology. Define a clear research question, conduct a thorough literature review, develop a strong outline, and present your findings with clarity. Remember to adhere to proper citation guidelines and revise your paper before submission. With these guidelines in mind, you can contribute to the advancement of radiology knowledge and make a meaningful impact in the field.
iResearchNet’s Writing Services
Introduction: At iResearchNet, we understand the challenges faced by students in the field of health sciences when it comes to writing research papers, including those in radiology. Our writing services are designed to provide you with expert assistance and support throughout your research paper journey. With our team of experienced writers, in-depth research capabilities, and commitment to excellence, we offer a range of services that will help you achieve your academic goals and ensure the success of your radiology research papers.
- Expert Degree-Holding Writers : Our team consists of expert writers who hold advanced degrees in various fields, including radiology and health sciences. They possess extensive knowledge and expertise in their respective areas, allowing them to deliver high-quality and well-researched papers.
- Custom Written Works : We understand that each research paper is unique, and we tailor our services to meet your specific requirements. Our writers craft custom-written research papers that align with your research objectives, ensuring originality and authenticity in every piece.
- In-Depth Research : Research is at the core of any high-quality paper. Our writers conduct comprehensive and in-depth research to gather relevant literature, scientific articles, and other credible sources to support your research paper. They have access to reputable databases and libraries to ensure that your paper is backed by the latest and most reliable information.
- Custom Formatting : Formatting your research paper according to the specified guidelines can be a challenging task. Our writers are well-versed in various formatting styles, including APA, MLA, Chicago/Turabian, and Harvard. They ensure that your paper adheres to the required formatting standards, including citations, references, and overall document structure.
- Top Quality : We prioritize delivering top-quality research papers that meet the highest academic standards. Our writers pay attention to detail, ensuring accurate information, logical flow, and coherence in your paper. We conduct thorough editing and proofreading to eliminate any errors and improve the overall quality of your work.
- Customized Solutions : We understand that every student has unique research requirements. Our services are tailored to provide customized solutions that address your specific needs. Whether you need assistance with topic selection, literature review, methodology, data analysis, or any other aspect of your research paper, we are here to support you at every step.
- Flexible Pricing : We strive to make our services affordable and accessible to students. Our pricing structure is flexible, allowing you to choose the package that suits your budget and requirements. We offer competitive rates without compromising on the quality of our work.
- Short Deadlines : We recognize the importance of meeting deadlines. Our team is equipped to handle urgent orders with short turnaround times. Whether you have a tight deadline or need assistance in a time-sensitive situation, we can deliver high-quality research papers within as little as three hours.
- Timely Delivery : Punctuality is a priority for us. We understand the significance of submitting your research papers on time. Our writers work diligently to ensure that your paper is delivered within the agreed-upon timeframe, allowing you ample time for review and submission.
- 24/7 Support : We provide round-the-clock support to address any queries or concerns you may have. Our customer support team is available 24/7 to assist you with any questions related to our services, order status, or any other inquiries you may have.
- Absolute Privacy : We prioritize your privacy and confidentiality. Rest assured that all your personal information and research paper details are handled with the utmost discretion. We adhere to strict privacy policies to protect your identity and ensure confidentiality throughout the process.
- Easy Order Tracking : We provide a user-friendly platform that allows you to easily track the progress of your order. You can stay updated on the status of your research paper, communicate with your assigned writer, and receive notifications regarding the completion and delivery of your paper.
- Money Back Guarantee : We are committed to your satisfaction. In the rare event that you are not satisfied with the delivered research paper, we offer a money back guarantee. Our aim is to ensure that you are fully content with the final product and receive the value you expect.
At iResearchNet, we understand the challenges students face when it comes to writing research papers in radiology and other health sciences. Our comprehensive range of writing services is designed to provide you with expert assistance, customized solutions, and top-quality research papers. With our team of experienced writers, in-depth research capabilities, and commitment to excellence, we are dedicated to helping you succeed in your academic endeavors. Place your order with iResearchNet and experience the benefits of our professional writing services for your radiology research papers.
Unlock Your Research Potential with iResearchNet
Are you ready to take your radiology research papers to the next level? Look no further than iResearchNet. Our team of expert writers, in-depth research capabilities, and commitment to excellence make us the perfect partner for your academic success. With our range of comprehensive writing services, you can unlock your research potential and achieve outstanding results in your radiology studies.
Why settle for average when you can have exceptional? Our team of expert degree-holding writers is ready to work with you, providing custom-written research papers that meet your specific requirements. We delve deep into the world of radiology, conducting in-depth research and crafting well-structured papers that showcase your knowledge and expertise.
Don’t let the complexities of choosing a research topic hold you back. Our expert advice on selecting radiology research paper topics will guide you through the process, ensuring that you choose a topic that aligns with your interests and has the potential to make a meaningful contribution to the field of radiology.
It’s time to unleash your potential and achieve academic excellence in your radiology studies. Place your trust in iResearchNet and experience the exceptional quality and support that our writing services offer. Let us be your partner in success as you embark on your journey of writing remarkable radiology research papers.
Take the first step towards elevating your radiology research papers by contacting us today. Our dedicated support team is available 24/7 to assist you with any inquiries and guide you through the ordering process. Don’t settle for mediocrity when you can achieve greatness with iResearchNet. Unlock your research potential and exceed your academic expectations.
ORDER HIGH QUALITY CUSTOM PAPER


An official website of the United States government
The .gov means it’s official. Federal government websites often end in .gov or .mil. Before sharing sensitive information, make sure you’re on a federal government site.
The site is secure. The https:// ensures that you are connecting to the official website and that any information you provide is encrypted and transmitted securely.
- Publications
- Account settings
Preview improvements coming to the PMC website in October 2024. Learn More or Try it out now .
- Advanced Search
- Journal List
- v.14(7); 2020 Jul

Technology‐driven research for radiotherapy innovation
Claudio fiorino.
1 Medical Physics, San Raffaele Scientific Institute, Milano Italy
Matthias Guckemberger
2 Department of Radiation Oncology, University Hospital Zurich, University of Zurich, Switzerland
Marco Schwarz
3 Protontherapy Department, Trento Hospital and TIFPA‐INFN, Trento Italy
Uulke A van der Heide
4 Department of Radiation Oncology, The Netherlands Cancer Institute, Amsterdam The Netherlands
5 Department of Radiation Oncology, Leiden University Medical Center, Leiden The Netherlands
Ben Heijmen
6 Department of Radiation Oncology, Erasmus MC Cancer Institute, Rotterdam The Netherlands
Technology has a pivotal role in the continuous development of radiotherapy. The long road toward modern ‘high‐tech’ radiation oncology has been studded with discoveries and technological innovations that resulted from the interaction of various disciplines . In the last decades, a dramatic technology‐driven revolution has hugely improved the capability of accurately and safely delivering complex‐shaped dose distributions. This has contributed to many clinical improvements, such as the successful management of lung cancer and oligometastatic disease through stereotactic body radiotherapy. Technology‐driven research is an active and lively field with promising potential in several domains, including image guidance, adaptive radiotherapy, integration of artificial intelligence, heavy‐particle therapy, and ‘flash’ ultra‐high dose‐rate radiotherapy. The evolution toward personalized Oncology will deeply influence technology‐driven research, aiming to integrate predictive models and omics analyses into fast and efficient solutions to deliver the best treatment for each single patient. Personalized radiation oncology will need affordable technological solutions for middle‐/low‐income countries, as these are expected to experience the highest increase of cancer incidence and mortality. Moreover, technology solutions for automation of commissioning, quality assurance, safety tests, image segmentation, and plan optimization will be required. Although a large fraction of cancer patients receive radiotherapy, this is certainly not reflected in the worldwide budget for radiotherapy research. Differently from the pharmaceutical companies‐driven research, resources for research in radiotherapy are highly limited to equipment vendors, who can, in turn, initiate a limited number of collaborations with academic research centers. Thus, enhancement of investments in technology‐driven radiotherapy research via public funds, national governments, and the European Union would have a crucial societal impact. It would allow for radiotherapy to further strengthen its role as a highly effective and cost‐efficient cancer treatment modality, and it could facilitate a rapid and equalitarian large‐scale transfer of technology to clinic, with direct impact on patient care.
Technology‐driven research has a pivotal role in the advancement of radiotherapy, still more in the current ‘high‐tech’ era. Larger efforts in developing efficient and affordable technology solutions to deliver personalized radiotherapy, even in middle‐/low‐income countries, are necessary. In order to facilitate a rapid and equalitarian large‐scale transfer of technology to clinic, increased investments in technology‐driven radiotherapy research are highly desirable.

Abbreviations
1. technology‐driven research in radiotherapy in a historical context.
Technology has always had an intrinsic and pivotal role in the development of radiotherapy: Since the early days, technological advancements made the history of radiotherapy. After the discovery of X‐rays and radioactivity, the long road toward modern high‐tech radiation oncology was studded with continuous discoveries, integrating innovative ideas and technology solutions from several disciplines, physics first. The integration of amazing advancements from other disciplines—including mechanical and electronic engineering, computer science, mathematics, imaging physics and technology, statistics, and data sciences—has been crucial for the never‐ending improvement of radiotherapy and the success of radiation oncology (Baumann et al. , 2016 ; Bortfeld and Jeraj, 2011 ; Thariat et al. , 2013 ). Often, technology developed in other domains was later applied to radiotherapy, such as the Linear accelerator (Linac) technology that replaced 60Co machines during 1960–1980, or the proton and heavy‐ion accelerators, whose development and improvement was much slower and mainly came from the high‐energy and nuclear physics environment (Durante et al. , 2017 ). Again, the rapid advancements in computer sciences permitted the fast development and integration of IT hardware solutions for radiotherapy, as well as of dramatically improved software for treatment plan optimization and delivery.
The efficient and safe translation of new technology from the research domain to the clinical practice has generally been a relatively rapid process in radiotherapy (Baumann et al. , 2016 ): Among the various players, the presence of physicists inside the hospital environment has been a key point of this process (Bortfeld et al. , 2015 ; Fiorino et al. , 2015 ). Medical physics, originally devoted to hospital radiation safety and dosimetry (including the radiotherapy field), has become a pivotal component of the transfer of technology to the clinics and of its testing and refinement. In addition, the feeding link between Hospital/University medical physics and technology developers has always been crucial, although it changes with time and is still changing nowadays.
2. The technology revolution of the last two decades: an overview
Over the past 20–25 years, a dramatic technology‐driven revolution has hugely improved the radiotherapy potential for accurate and safe delivery of complex‐shaped dose distributions to the tumor. Similarly to external radiotherapy, research driven by recent technological advances has renewed the potential of the old practice of brachytherapy (through which radioactive sources deliver a radiotherapy dose inside the tumor), as recently reviewed elsewhere (Tanderup et al. , 2017 ).
2.1. Conformal radiotherapy
The first step of this revolution was the big jump from a ‘planar imaging‐based’ discipline (i.e., fields were delivered with few open or minimally blocked beams intended to deliver the prescribed dose to very large portions of the body, with ‘high’ probability of including an ‘invisible’ tumor), to the so‐called ‘conformal radiotherapy’ (i.e., the 3D dose distribution is tailored to the target shape). The development and the consequent introduction of the multileaf collimator (MLC) technology during the 1980s and 1990s was a major step toward the potentiation of field shaping according to the shape of tumors. Although the concept of spatially confining the dose strictly around the tumor to spare the surrounding normal tissues (nowadays named ‘Organs at risk’, OARs) was clear since the early days of radiotherapy, it could only materialize after the advent of several technological innovations in imaging, physics, and computer science.
The availability of CTs and, later, of other imaging modalities, including MRI and PET, enabled more accurate identification of targets, namely of both tumors (gross target volume, GTV) and surrounding tissues at risk of micrometastatic spread (clinical target volume, CTV) (ICRU, 2010 ), as well as their incorporation into the optimization process. Concomitant advances in computer science and dose calculation algorithms opened up the road for individual plan optimization. Again, technology‐driven research and innovations translated into a rapid spread of special computer platforms (the ‘treatment planning systems’, TPS) that can simulate what happens inside the patient when delivering radiation beams, using images as a 3D model of the patient. Unlike their ancestors from the 1970s and 1980s, which were solely used for dose calculation in few representative patient slices (often with a rough approximation), modern TPS are used since the 1990s to optimize shape, position, and weight of multiple beams with the goal of tailoring the dose distribution around the target, while minimizing the dose to OARs. Interestingly, personalized radiotherapy, in the sense of the individual adaptation of the spatial (the geometry) and intensity (the dose) radiation delivery features, started a couple of decades before this concept became familiar to the whole oncology community (Chin et al. , 1985 ). Nowadays, nearly all radiotherapy patients get a treatment that is (highly) personalized on their individual anatomy.
2.2. Intensity‐modulated radiotherapy
Another major step for the technological revolution in radiotherapy has been the development of systems for delivering dose distributions strictly tailored to GTV/CTV, thereby extending the ‘conformal radiotherapy’ concept. Modulation of the spatial intensity of the beams in the context of ‘intensity‐modulated radiotherapy’ (IMRT) has hugely increased the degrees of freedom for radiotherapy optimization. Several methods have been implemented to achieve modulation; the most successful and widely used one involves modulation of the 2D intensity of fixed fields through the computer‐controlled motion of MLC systems (Convery and Webb, 1998 ; Kallman et al. , 1988 ). The extension of this approach led to the so‐called IMAT (intensity‐modulated arc therapy), currently named also as VMAT (‘volumetric’ replacing ‘intensity’), where, instead of delivering the treatment with a number of fixed beams, the radiation is continuously delivered by an X‐ray beam that rotates around the patient (Yu, 1995 ). This rotational approach further extended the degrees of freedom for optimizing the treatment and, at the same time, made the delivery of complex‐shaped dose distributions more efficient and faster. IMRT/VMAT delivery was accompanied by the rapid development of TPS that included modulation and rotation into the optimization, replacing the traditional trial and error ‘forward’ plan optimization with the so‐called ‘inverse optimization’ (Brahme, 1988 ), where the theoretically ‘best’ dose distribution was translated into quantitative dose‐volume goals for targets and OARs. Plan optimization of IMRT/VMAT treatments currently resembles a ‘mathematical game’ that determines the optimal ‘physically feasible’ solution for approaching predefined goals.
2.3. Image‐guided radiotherapy
Concomitantly to the development and implementation of IMRT technology, technology‐driven research has been largely devoted to the design of in‐room systems for improving the daily patient setup, gradually leading to the clinical use of image‐guided radiotherapy (IGRT) (Jaffray, 2005 ). Several IGRT approaches have been developed (or are still under development, as shortly described later): planar imaging coupled with the use of internal markers; CT imaging integrated into the Linac (the cone‐beam CT); optical markers; ultrasound‐based systems; and others. These technologies have dramatically improved the accuracy of radiotherapy delivery, and reduced safety margins around GTV/CTV, further enlarging the potential of radiotherapy to spare the healthy tumor‐adjacent tissues. Moreover, many techniques have been developed and implemented to monitor and correct intrafraction changes (including breathing induced motion), so that tumors are effectively tracked during delivery, with a parallel reduction of margins around the target and significantly better sparing of OARs (Korreman, 2012 ).
In Fig. 1 , a schematic cartoon of a concave‐shaped target surrounding a sensitive OAR is used to show how the evolution of technology in the last three decades has improved the ‘conformation’ of the dose distribution around the target, while sparing OARs and adjacent tissues more efficiently.

Schematic plot of the impact of technology in the last decades in delivering dose distributions more tailored to GTV/CTV in a typical case of tumor next to an organ at risk: At each step, the high‐dose region corresponding to the previous technologies is overlaid to better appreciate the net benefit. Nowadays, image‐guided intensity‐modulated radiotherapy (using multifields or arc, IMRT, and VMAT, respectively) may strictly tailor the prescribed dose distribution to the tumor, using reduced margins thanks to the high precision of the delivery permitted by IGRT.
2.4. Extreme hypo‐fractionation and stereotactic body radiotherapy
The widespread implementation of IGRT, mostly in the last decade, and its combination with IMRT/VMAT, largely supported the exploration of extreme hypo‐fractionation. In several relevant situations, as opposed to a conventionally fractionated treatment (with 20–40 fractions), IMRT/VMAT treatment combined with IGRT can be delivered in few fractions (typically 1 to 5), and this has an important impact on both patients and the society, as it is associated with cost reduction and facilitated access to radiotherapy(Atun et al. , 2015 ). This approach represents one of the most valuable successes directly linked to technological innovation and the advancements of IGRT (Jaffray, 2012 ).
Stereotactic body radiotherapy (SBRT) (Lax et al. , 1994 ) has extended the intracranial radiosurgery concept, contributing to the currently changing clinical paradigm for the treatment of several tumors (Aznar et al. , 2018 ; De Bari et al. , 2016 ; Lewis et al. , 2017 ). The recently developed applications of this stereotactic approach to oligometastatic cancer are described also below.
2.5. Recent advancements of delivery technology
In order to make the delivery still more efficient, especially for SBRT applications, flattening‐filter‐free (FFF) Linacs were developed, leading to substantial delivery time reductions (Vassiliev et al. , 2006 ); the replacement of past‐generation machines by FFF Linacs is currently in progress.
The integration of IGRT and IMRT delivery capabilities pushed technology‐driven research to develop improved integrated machines, including ‘special’ dedicated machines that apply various concepts of delivery. Helical tomotherapy (Mackie and Swerdloff, 1993 ) integrates a 6 MV Linac with a CT, through the helical delivery of an intensity‐modulated continuously rotating fan beam, delivered while the couch translates, similarly to a helical CT scanner. Moreover, the Robotic Linac (Schweikard et al. , 2000 ) treats tumors through the delivery of a large number of ‘pencil’ beams. This machine was also the first example of clinical implementation of tracking: The combination of Robotic Linac with a pair of perpendicular flat panels allows for monitoring patient position during beam delivery and, thanks to a fast feedback technology, offers the possibility to correct beam position in real time if the tumor moves.
3. Present and future directions in technology‐driven research
As in part described in the previous section, in modern radiotherapy a range of technologies are in clinical use to treat patients; most of them are highlighted in specific papers of this thematic issue, and Fig. 2 shows in a snapshot most of the available image‐guided equipped technologies actually used in most radiotherapy centers in Europe and North America.

Examples of available ‘High‐Tech image‐guided Linacs’: (A) Conventional Linacs equipped with cone‐beam CT [Zurich UH (left) and NKI‐AvL, Amsterdam (right)]: The kV imaging system is perpendicular to the beam axis and CT images are obtained by rotating the gantry; (B) Helical delivery system (OSR, Milano): The Linac is integrated into a CT ring: Megavoltage images are obtained by using the same treatment fan beam paired to a detector array, delivered in a helicoidal way by moving the couch; (C) Robotic system (Erasmus, Rotterdam): Dose delivery is generated by a large number of small noncoplanar beams delivered by the robotic arms, while image guidance (including tracking during delivery) is driven by a perpendicular pair of flat panel on the floor (in the right corner of the picture) paired to two kV X‐rays tubes positioned on the room ceiling; (D) Hybrid MRI‐Linac machines [Zurich UH (left) and NKI‐AvL, Amsterdam (right)]: The Linac is integrated into an MRI. MRI images can be obtained before and during the treatment delivery. (E) Proton system (Protontherapy, Trento): The gantry (left of the picture) is paired to a robotic couch and a diagnostic CT in the room to setup the patient before treatment delivery
External beam radiotherapy with IMRT/VMAT, particle therapy, and brachytherapy all use different strategies for spatially modulating the deposition of radiation dose in the patient. Nonetheless, they essentially all aim to achieve the same goal: eradicate cancer cells in bulky tumors as well as microscopic regional disease, while at the same time sparing healthy tissue so as to avoid radiation‐induced side effects. This capacity to deposit highly complex‐shaped dose distributions still gives radiotherapy a unique position between the other treatment modalities of cancer: surgery and systemic and targeted drugs. Several themes can be identified in the present and near‐future of technology‐driven research and are here shortly described.
3.1. Outlook for technology‐driven research on image guidance
As radiotherapy is noninvasive, the tumor and surrounding anatomy is not visible during the treatment. This is resolved by integrating medical imaging with radiotherapy delivery systems. Cone‐beam CT is widely used in external beam radiotherapy, allowing the therapist to see the patient anatomy at the time of treatment. As previously discussed, this has dramatically increased the precision of radiotherapy and made it possible to increase radiation dose and shortening treatment time while at the same time reducing treatment‐related side effects (Bujold et al. , 2012 ). While CT‐based image guidance has reached a high level of maturity in external beam radiotherapy, the development of suitable image guidance technology is an active research field in heavy particles therapy and in brachytherapy (Landry and Hua, 2018 ; Tanderup et al. , 2017 ).
A related field of technology‐driven research is still the monitoring and online tracking of tumors, aimed to counteract intrafraction motion, highly relevant in the case of breathing for thoracic and abdominal regions. Much technology‐driven research is oriented to extend the capabilities of fast target recognition as well as of more and more available delivery techniques.
As MRI has superior soft tissue contrast compared to CT, an obvious next step is the integration of MRI systems in image guidance technology. Integrated MRI linear accelerators for external beam radiotherapy are used in tens of institutes worldwide, and this number is increasing rapidly. A key feature of MRI‐guided systems is the capacity to track the cancer not only just prior to irradiation, but while irradiation is ongoing. This allows interrupting the treatment when the tumor moves out of the treatment field, further enhancing precision. Technology for tracking the tumor with the irradiation beams using MRI guidance is currently being developed. Another key feature is the capacity to monitor the response of the tumor to the treatment and to detect changes in tumor characteristics with functional MRI. These techniques are now in development and hold the prospect of further personalizing the treatment to each individual patient (Liney et al. , 2018 ).
3.2. Adaptive radiotherapy
The traditional workflow involves an elaborate series of actions to get from diagnostic images to an executable treatment plan. CT, MRI, and PET images need to be registered and tumor volumes and OARs need to be delineated. Treatment plans are designed to optimize radiation beams and intensities to achieve optimal dose distributions. Patients are usually treated with these fixed plans throughout the course of radiotherapy, disregarding treatment response. To accommodate tumor regression and anatomical changes or adjust to the changing biological characteristics of the cancer, a faster feedback loop is required that translates imaging information into a new treatment plan on a much shorter time scale (Sonke et al. , 2019 ).
The concept of adaptive radiotherapy was introduced in 1997 (Yan et al. , 1997 ) and involves modification of the treatment plans at several instances during the course of fractionated radiotherapy. In this way, changes in anatomy such as shrinkage of the tumor can be accommodated. The state of the art involves repeated imaging with cone‐beam CT or diagnostic CT scanner and adaptation of the treatment at a limited number of instances. Another approach (often reported as the ‘plan‐of‐the‐day’) consists of the preparation of plan libraries before starting the treatment, accounting for different anatomical situations. At each fraction, based on the image‐guided tumor visualization, the best plan is chosen, permitting a higher sparing of normal tissues, especially in those situations where CTV coincides with a highly deforming organ, such as bladder or uterus (Thörnqvist et al. , 2016 ).
The technology is however evolving rapidly. Treatment units with CT on rails or integrated MRI‐Linac systems allow for daily online imaging with a high quality. Software for auto‐contouring of images and automatic generation of treatment plans is becoming available commercially, and TPS is becoming sufficiently fast to allow for online adaptation of the treatment during every treatment fraction. A critical step is a validation and clinical approval of the auto‐segmentation and automatically generated treatment plans by radiation oncologists and medical physicists. To reach the goal of online biological image‐guided adaptive radiotherapy, this validation and approval need to be streamlined so that it can be done in a few minutes rather than in hours.
3.3. Artificial intelligence and big data
Artificial intelligence (AI) techniques will rapidly find relevant and extensive applications in radiotherapy (Thompson et al. , 2018 ). An important example is the ability of AI in supporting planners to generate automated solutions for treatment planning optimization that are integrating (in part replacing) and improving the traditional, manually optimized, planning (Hussein et al. , 2018 ). AI is also particularly promising to support online treatment planning and adaptive radiotherapy (Boon et al. , 2018 ). The potential for fast reconstruction of CT or MR images has been demonstrated as well as the feasibility to generate CT‐like images that are needed for dose calculations from MRI. Many recent studies show its potential for auto‐contouring of organs at risk and, in some cases, tumors. Using AI for quality assurance and outlier detection may help to speed up the process of validation and clinical approval in an online adaptive workflow (McNutt et al. , 2019 ). Increasingly, traditional AI techniques are replaced by deep learning methods (Meyer et al. , 2018 ; Sahiner et al. , 2019 ). Convolutional neural networks have been shown to be superior in auto‐contouring of OARs, and their application for CT and MRI reconstruction and treatment planning are an active field of research. AI also plays an important role in the development of prediction models for outcome based on the images collected during the course of radiation therapy (Bibault et al. , 2016 ). Radiomics uses the extraction of image features from CT, MRI, and PET to find imaging biomarkers and generate prediction models. It is particularly promising to bridge personalized medicine and radiation oncology (Lambin et al. , 2017 ). As with other AI applications, deep learning is increasingly replacing traditional methods.
To harness the potential of AI, large amounts of data are necessary. Particularly, deep learning algorithms tend to be data hungry. Data sharing is therefore necessary and technology to combine data from different sources in a consistent manner is being developed. IT infrastructures that facilitate data sharing are being constructed. To guarantee patient privacy, distributed learning technology is a promising approach. Much research also dealt with the integration of large data base information into decision‐making support systems toward a fully personalized approach to the treatment (Lambin et al. , 2013 ).
3.4. Protons and heavy ions
In the early 2010s, the focus of research and developments was to realize and make available at a large scale the gold standard of beam delivery, that is, pencil beam scanning, which allows to fully take advantage of the possibilities of proton and carbon ion therapy. Currently, big efforts are aimed at improving the imaging tools available for patient positioning and treatment adaptation. When it comes to longer term, research activities in radiotherapy with protons and heavier ions are developing on all fronts (radiobiology, clinic, and technology). Concerning technology, the following main directions can be identified:
- a The size and complexity of a proton therapy facility is typically much larger than a conventional radiotherapy installation, creating economical and logistical barriers to the introduction of this new technology. Therefore, there is continuous interest in developing better/cheaper methods for beam production and delivery, via either incremental or radical changes (Schippers et al., 2018 ).
- b Particles heavier than protons are interesting not only for the superior dose distributions, but also because of a differential radiobiological effect between tumor and healthy tissues that can be used to maximize their beneficial effects. Therefore, a few centers are now working to enable treatments with new ion species (e.g., Helium and Oxygen) (Sokol et al., 2017 ).
- c Delivering ‘Flash’ therapy (see next section) with heavy charged particles, protons, in particular is interesting and, in some cases, should be feasible with relatively minor changes to the existing equipment (Patriarca et al., 2018 ).
- d Since proton and heavier charge particles, unlike X‐rays, stop in the patient, it is of course important to develop better (real time) in vivo range measurement of therapeutic beams (Xie et al., 2017 ).
3.5. ‘Flash’ therapy and spatial fractionation
A quite new and highly promising field of investigation concerns the so‐called ‘Flash’ radiotherapy, consisting in the delivery of ultra‐high (≥40 Gy·s −1 ) dose‐rate beams (Favaudon et al. , 2015 ). Preclinical studies involving cell lines and animals showed a different response of tumor and normal tissues compared to conventional dose rates normally delivered in radiotherapy (typically ranging between 0.01 and 0.1 Gy·s −1 ), with promisingly high benefit in delivering Flash beams. Much preclinical research is nowadays oriented to investigate the basic phenomenon and the related biology effects in preclinical models. In parallel, technology‐driven research is under development to make more easily available ultra‐high dose rates in clinical Linac and few pioneering examples are available, mostly using electron and proton beams (Bourhis et al. , 2019 ). In the case of positive answers from preclinical research, the clinical implementation of Flash radiotherapy could happen quite quickly with the potential to represent a really new and revolutionary approach. Efficient and safe technology needs to be developed with challenging questions to be faced, like the way the beams can be measured, monitored, and possibly delivered in a multifield arrangement. Of note, the first patient, affected by a highly resistant skin lymphoma, was treated with Flash, with an impressive early result (Bourhis et al. , 2020 ).
Another highly promising field of investigation concerns spatial fractionation, including several methods to create dosimetry micropeaks and valleys within a tumor; despite this approach having a long history, most of its aspects remain to be understood (Billena and Khan, 2019 ). Technologies to better adapt this approach to clinical applications are currently available (using grids or MLCs) and others are under development, including the generation and delivery of microbeams with photons or protons (Schültke et al. , 2017 ).
4. Technology to advance cancer care: the example of SBRT for oligometastatic cancer
Radiotherapy technology has been a key driver in oncology, improving outcome of cancer patients in many fields. Oligometastatic disease (OMD) will be here used as an example of how technology recently (and rapidly) changed the picture in a clinically relevant scenario.
OMD is defined as an intermediate cancer state between early stage, where cure is the goal of radical local treatment, and systemic metastasized stage, where local and systemic therapy follows a palliative goal (Hellman and Weichselbaum, 1995 ). In OMD, cancer has spread beyond locoregional areas, but the metastatic capacity is small such that only few metastases have developed. Radical local treatment of all cancer sites combined with systemic therapy for occult micro‐metastases therefore offers a curative potential. Although the term ‘oligometastases’ was coined and defined in 1995, surgical resection of solitary or limited metastases has been performed for decades and has achieved long‐term disease‐free survival and overall survival for selected patients. However, based on a systematic review of oligometastatic non‐small‐cell lung cancer (NSCLC), surgical resection was almost the exclusive local treatment modality until 2003 and the predominant modality until 2007 (Schanne et al. , 2019 ) with radiotherapy used in only very few patients. This is explained by the inability of ‘traditional’ radiotherapy to locally eradicate oligometastases with sufficient safety and efficacy. Only since the development and broad implementation of SBRT into routine clinical practice, radiotherapy became the local treatment modality with the highest level of evidence and simultaneously the most frequently used local treatment modality in OMD. A patient case example is given in Fig. 3 .

(A) Seventy years, female patient with a diagnosis of synchronous oligometastatic NSCLC: cT2 cN1 cM1b (adrenal), adenocarcinoma, EGFR WT, ALK negative. (B) Status after induction/first‐line chemotherapy. (C) Radical radiotherapy in conventional fractionation for the locoregional primary tumor (C1) and SBRT for the adrenal metastasis (C2). (D) Complete metabolic response 3 months after completion of radiotherapy
Stereotactic body radiotherapy has been developed and first clinically introduced at the Karolinska Hospital in Sweden in 1994 (Lax et al. , 1994 ). SBRT was described and characterized by rigid patient positioning and immobilization in a stereotactic frame, control of breathing‐induced target motion, conformal treatment planning by using noncoplanar treatment techniques, inhomogeneous dose distributions in the target, and dose delivery in few fractions of high single fraction doses. Limitations of SBRT as developed in the 90s and early 2000s included the complex methodology that restricted its use to highly specialized academic centers, as well as the toxicity rates in challenging anatomical situations such as the central lung or upper abdominal region. However, within few years all steps of the SBRT treatment chain were optimized integrating all technologies explained above: Respiratory motion became fully integrated into radiotherapy planning and delivery; dynamic intensity‐modulated radiotherapy combined high conformality with rapid dose delivery minimizing intrafractional uncertainties; and online image guidance ensured accurate treatment delivery.
These technological advances of SBRT and its improved accuracy translated into an improved therapeutic ratio with low risk of toxicity and simultaneously high rates of local tumor control. Safety and efficacy of high‐dose SBRT was demonstrated in metastases of histology previously assumed as radioresistant (Guckenberger et al. , 2016 ; Klement et al. , 2018 ). For pulmonary oligometastases of NSCLC, a matched pair analysis reported the identical outcome of SBRT and surgical metastasectomy and similar promising results of SBRT have been described for other frequent oligometastases locations, such as the liver, adrenal gland, bone metastases, and lymph node metastases. Simultaneously, the technological advances of SBRT not only translated into improved clinical outcome but were also the basis for safe and rapid implementation of SBRT outside of clinical trials and outside of specialized academic centers. An international survey among 1000 radiation oncologists in 2017 reported that 61% of all respondents had implemented SBRT for OMD in clinical routine practice (Lewis et al. , 2017 ). Nevertheless, sufficient institutional experience in SBRT is a prerequisite to ensure optimal outcome (Rieber et al. , 2017 ).
The favorable therapeutic ratio combined with rapid adoption of SBRT was key factor to validate the concept of local ablative treatment for OMD in general. Until today, four randomized controlled trials evaluated the value of local ablative treatment of all macroscopic cancer sites in addition to standard of care systemic therapy. Three randomized controlled trials reported improved overall survival in lung cancer (Gomez et al. , 2019 ), colorectal cancer (Ruers et al. , 2017 ), and a disease agnostic setting (Palma et al. , 2019 ); the fourth study was underpowered for overall survival but reported a significantly improved progression‐free survival (Iyengar et al. , 2018 ): The results regarding NSCLC are summarized in Table Table1. 1 . Whereas radiofrequency ablation was the exclusive locally ablative treatment modality in the earliest CLOCC trial (Ruers et al , 2017 ), SBRT was the exclusive locally ablative treatment modality in two studies (Iyengar et al , 2018 ; Palma et al , 2019 ) and the most frequent in one study (Gomez et al , 2019 ). Therefore, the current ESMO guideline for oligometastatic NSCLC states that the ‘relative contribution of surgery versus radiotherapy as local treatment modality has not been established yet’ in OMD. Technological progress in the form of SBRT has consequently changed the standard of care from surgery to radiotherapy for the treatment of OMD.
Summary of the randomized controlled trials comparing standard‐of‐care systemic therapy alone vs systemic therapy plus radical treatment of all cancer lesions for oligometastatic NSCLC (*significant results)
However, considering the current rapid developments in the field of IT, engineering, and imaging, we expect that radiation technology will remain a key factor in improving the outcome of oligometastatic cancer patients.
Whereas current image guidance achieves accurate targeting of most target lesions, metastases located in the upper abdomen and liver are still challenging for cone‐beam CT‐based or planar X‐ray‐based treatment delivery systems. This may at least partially explain the worse local control rates observed in liver SBRT compared to lung SBRT (Klement et al. , 2019 ). In particular, MRI‐based image guidance solutions are expected to address this challenge, especially when combined with online adaptive radiotherapy (Corradini et al. , 2019 ).
5. Challenges and pitfalls of technology‐driven research and of clinical translation
The very strong orientation toward technology‐driven research in radiation oncology has a number of reasons. Although the picture is complex, two general aspects are worthy to be underlined.
First, the process of developing new technology is typically more predictable and with a shorter life cycle than the process of producing and demonstrating improved outcomes. This means that projects focused at generating new devices, or new methods based on software development, are more likely to be successful and their outcome is more easily measurable on a scale of a few years.
Second, the limited dimension and ‘power’ of the radiotherapy community makes it difficult to develop completely new technologies ‘from scratch’. This is the main reason why innovation in the field of radiotherapy‐specific hardware (e.g., new beam delivery systems or radiation detectors) is comparatively slow. However, radiotherapy can greatly benefit from tools and methods initially developed in fields with a much larger ‘critical mass’. Two topical examples are the integration of radiomics (Avanzo et al. , 2017 ) that is benefiting from tools and methods available because of massive investments in the radiology and imaging science domain and, in a more general extent, aimed at developing machine learning in several aspects of life, and the implementation of fast dose calculations and deformable image registration on graphical processing units (GPU) (Pratz and Xing, 2011 ), which is possible because GPUs were developed in the hugely profitable market of video gaming.
The success of technology in radiation oncology has been so significant that it can actually flip the dynamics between technology and medicine, to the point where it is fair to ask whether technology is driving the future of radiotherapy as opposed to the other way around. Of course, this is not a problem of technology per se , but rather an issue that may arise if radiation oncologists and medical physicists are not setting the correct priorities.
In this context, technology‐driven research is prone to issues that roughly belong to three broad categories, as discussed below.
5.1. Issues caused by the (implicit or explicit) assumption that technological improvements will inevitably translate into better outcomes, to the point that they do not need clinical validation
A typical example of this approach is to consider planned dose distributions as a very reliable surrogate for clinical outcome. For instance, the transition from 3DCRT to IMRT has been in fact largely justified based on the superiority of IMRT planned dose distributions. Some level of clinical evidence came only after the transition was over (Veldeman et al. , 2008 ). The same argument has been often proposed in the discussions on photon vs proton therapy (Goitein and Cox, 2008 ).
It is interesting to note, however, that in past few years sophisticated and more quantitative approaches emerged in comparing dose distributions, which may be a good example of how to move forward. Particularly, promising is the so‐called ‘model‐based approach’ (Langendijk et al. , 2013 ) to select patients suitable for a new technology (suggested for instance for proton therapy), which finds a better balance between the possibility of using treatment planning as an outcome predictor and the risk of being overconfident about it. The strength of the model‐based approach is to compare plans based not on pure dosimetric indices but on normal tissue complication probability (NTCP) models taking into account the nonlinear relation between a reduction in dose to the normal tissues and a reduction in radiation side effects. This approach is a good example of how to combine innovative technological approaches (like automatic planning and protons) with the latest knowledge on dose‐effect relations, thus aiming by design at ‘closing the loop’ between technology and clinical outcomes. In addition, even though developed in the context of proton therapy, its domain of applicability is likely to be wider. There are several examples both in the past of radiotherapy (e.g., the early days of IMRT) and in the present (e.g., the MR‐Linac) of situations, where a new technological solution is available, it is theoretically advantageous for a large number of patients, but its availability is scarce, and patient selection is therefore necessary.
5.2. Issues caused by prioritizing research primarily according to the appeal of the technological problem
This issue manifests itself in different ways, in particular in medical physics research. There are instances, where significant resources are needed to tackle a problem in its extreme manifestations, while many/most situations can be handled with simpler methods. For example, the problem of treating moving targets in the minority of patients with large respiratory amplitudes is extremely complex, and technologically very interesting. However, for most patients, planning and treatment in free‐breathing may be fully appropriate, as long as systematic positioning errors are minimized (Sonke et al. , 2008 ).
At the other end of the spectrum, there may be research endeavors that are clinically significant but do not receive the attention they deserve because they are not primarily a technological problem. Is the comparatively limited interest in studies of dose‐effect relation caused also by an excessive focus on technology?
5.3. Issues related to potentially unmotivated costs increase related to technology
Finally yet importantly, an undesired and totally unintended consequence of emphasizing technological hard problems is to favor the industrial development of radiation oncology devices that are increasingly complex and expensive, potentially taking resources away from developments addressing the need of large and disadvantaged populations, where access to basic radiotherapy facilities is a pressing issue (Lievens et al. , 2017 ).
How can we limit the risk of focusing excessively on technology per se ? Tighter interaction between research‐oriented and clinic‐oriented professionals is a crucial first step, and there are examples of this process at work in radiation oncology. For decades, proton therapy has been a field where people worked under the assumption that if just the basic scientists made their technology available to clinicians, most, if not all, problems would be solved. This turned out not to be the case. Only much later, when knowledge of nuclear and accelerator physics was brought to the hospitals and integrated with the essential tools of modern radiation oncology, the field took actually off. Similar processes should happen (hopefully faster than in proton therapy) whenever one wants to introduce a high degree of innovation and apply it to a large number of patients. In such cases, neither basic scientists, nor people exclusively involved in clinical practice, can solve the problem by themselves.
6. Bridging technology innovation with personalized (and efficient) Radiotherapy
As largely debated in the current thematic issue of Molecular Oncology , radiation oncology is a crucial player for the rapid transformation of oncology toward personalized and precision care (Baumann et al. , 2016 ; Krause et al. , 2020 ; Mondini et al. , 2020 ). Among the curative options, it is the only that can merge its increasing ‘surgical’ ability with the potential of sterilizing nodes suspected of infiltration of tumor cells; in addition, the combination with immunotherapy is also expected to extend radiotherapy as a primary player for the ambitious aim of caring (or preventing) metastatic disease (Kroeze et al. , 2017 ). A major element expected to increasingly driving the future is the possibility of more and more using individual (clinical, genetic, other ‘omics’, etc.) information to carefully select patients according to the features of their tumor and to individually predict the risk of toxicity (van der Schaaf et al. , 2015 ). The evolution toward personalization started to influence technology‐driven research and is likely to deeply influence it in the near future, aiming to integrate predictive models into fast and efficient technology solutions for the delivery of the best treatment to single patients. Developments of technology integrating quantitative (morphological and functional) imaging before and during therapy into the optimization and delivery process are expected to be one of the major areas of research of next years. Decision support tools driven by AI‐based platforms integrating large data base information within machine learning systems and data‐sharing communities will also represent a big research item (Lambin et al. , 2013 ), including the selection of the best care and radiotherapy technique. Optimization and delivery are expected to largely benefit of technology solutions permitting a continuously adaptation of the treatment to counteract modifications and incorporating early response into the treatment. In order to efficiently exploit the delivery capabilities, automation will play a key role, pushing technology‐driven research to develop systems that are increasingly able to automatically perform accurate and reproducible patient setup, automatic online planning, and online monitoring of the delivery. The extension of personalized radiation oncology will require affordable technological solutions for high‐, middle‐, and low‐income countries (Lievens et al. , 2020 ), and these last will experience highly increasing cancer incidence and mortality (Atun et al. , 2015 ). Technology solutions for automation of commissioning, quality assurance, safety tests, and planning are likely to play a huge role in technology‐driven research in the field, including new developments in informative science and communication to support remote assistance for therapy personalization.
7. Prioritizing technology‐driven radiotherapy research and investments
It is generally accepted that technology‐driven research has been a driver for most major advancements in radiotherapy in the past decades. Most disruptive technologies (3D conformal radiotherapy, intensity‐modulated radiotherapy, cone‐beam CT, MR‐Linac) have been initiated and developed in academic radiotherapy centers and then commercialized by established or startup companies in collaboration with academic researchers. This routing gives some guarantee that commercialized products are indeed innovative and beneficial to cancer patients.
Around 50% of all cancer patients in high‐income countries receive radiotherapy in some stage of their disease. Although exact numbers are unknown, this is certainly not reflected in the worldwide budget for radiotherapy research, as compared, for example, to investments in medical oncology. Pharmaceutical companies spend vast amounts of money on collaborations with academic centers, as drugs can be sold at price levels that allow for substantial investments in innovation. The situation is completely different in radiotherapy research. Resources for research are highly limited in radiotherapy equipment vendors. They generally do not succeed in selling their products at price levels that allow them to substantially invest in innovation. As a result, the investments they can do in collaborations with academic research centers are also rather restricted. This undoubtedly slows down the development of radiotherapy as an effective treatment modality for cancer patients, which is generally more cost‐efficient than medical oncology interventions. The situation may deteriorate further with the current massive introduction of VMAT and hypofractionated treatments, which allow for efficiently treating higher patient numbers with the current treatment machines, thereby putting pressure on the revenues of the radiotherapy industry.
Probably in part caused by the current difficult market situation, more than 90 % X‐rays Linacs are provided by only two companies. Switching equipment vendors is often considered highly undesirable by radiotherapy centers because of the large investments to be made, for example, in training of personnel. Moreover, working with equipment from both vendors is highly costly. This situation does certainly not contribute to substantial investments in research and innovation by the companies.
In a situation as the one sketched above, enhanced investments in technology‐driven radiotherapy research via public funds from national governments, the European Union, and other international organizations (as well as from not‐for‐profit private funds and charities) could have important societal impact. New research investments would probably also result in new startups, thereby improving the current radiotherapy market. More importantly, increased investment in radiotherapy research could facilitate a faster, and equalitarian, large‐scale technology transfer to clinic, with direct impact on patient care, and would consequently enable radiotherapy to further strengthen its role as a highly effective and cheap treatment modality for cancer patients. As technology transfer is slowed down by the high regulatory pressure, as, for example, the one related to the new European Medical Device Regulation, additional investments are needed to effectively counteract this pressure.
- Atun R, Jaffray DA, Barton MB, Bray F, Baumann M, Vikram B, Hanna TP, Knaul FM, Lievens Y, Lui TYM et al (2015) Expanding global access to radiotherapy . Lancet Oncol 16 , 1153–1186. [ PubMed ] [ Google Scholar ]
- Avanzo M, Stancanello J and El Naqa I (2017) Beyond imaging: The promise of radiomics . Phys Med 38 , 122–139. [ PubMed ] [ Google Scholar ]
- Aznar MC, Warren S, Hoogeman M and Josipovic M (2018) The impact of technology on the changing practice of lung SBRT . Phys Med 47 , 129–138. [ PMC free article ] [ PubMed ] [ Google Scholar ]
- Baumann M, Mechtild R, Overgaard J, Debus J, Bentzen SM, Daartz J, Richter C, Zips D and Bortfeld T (2016) Radiation Oncology in the era of precision medicine . Nature Cancer Rev 16 , 234–249. [ PubMed ] [ Google Scholar ]
- Bibault J‐E, Giraud P and Burgun A (2016) Big data and machine learning in radiation oncology: state of the art and future prospects . Cancer Letters 382 , 110–117. [ PubMed ] [ Google Scholar ]
- Billena C and Khan AJ (2019) A current review of spatial fractionation: Back to the future? Int J Radiat Oncol Biol Phys 104 , 177–187. [ PMC free article ] [ PubMed ] [ Google Scholar ]
- Boon IS, Au Yong TPT and Boon CS (2018) Assessing the role of Artificial Intelligence (AI) in clinical oncology: utility of machine learning in radiotherapy target volume delineation . Medicines (Basel) 5 , E131. [ PMC free article ] [ PubMed ] [ Google Scholar ]
- Bortfeld T and Jeraj R (2011) The physical basis and future of radiation therapy . Br J Radiol 84 , 485–498. [ PMC free article ] [ PubMed ] [ Google Scholar ]
- Bortfeld T, Torresin A, Fiorino C, Andreo P, Gagliardi G, Jeraj R, Muren LP, Paiusco M, Thwaites D and Knoos T (2015) The research versus clinical service role of medical physics . Radiother Oncol 114 , 285–288. [ PubMed ] [ Google Scholar ]
- Bourhis J, Montay‐Gruel P, Gonçalves Jorge P, Bailat C, Petit B, Ollivier J, Jeanneret‐Sozzi W, Ozsahin M, Bochud F, Moeckli R et al (2019) Clinical translation of FLASH radiotherapy: Why and how? Radiother Oncol 139 , 11–17. [ PubMed ] [ Google Scholar ]
- Bourhis J, Sozzi WJ, Jorge PG, Gaide O, Bailat C, Duclos F, Patin D, Ozsahin M, Bochud F, Germond J‐F et al (2020) Treatment of a first patient with FLASH‐radiotherapy . Radiother Oncol 139 , 18–22. [ PubMed ] [ Google Scholar ]
- Brahme A (1988) Optimization of stationary and moving beam radiation therapy techniques . Radiother Oncol 12 , 129–140. [ PubMed ] [ Google Scholar ]
- Bujold A, Craig T, Jaffray D and Dawson LA (2012) Image‐guided radiotherapy: has it influenced patient outcomes? Semin Radiat Oncol 22 , 50–61. [ PubMed ] [ Google Scholar ]
- Chin LM, Siddon RL, Svensson GK and Rose C (1985) Progress in 3‐D treatment planning for photon beam therapy . Int J Radiat Oncol Biol Phys 11 , 2011–2020. [ PubMed ] [ Google Scholar ]
- Convery DJ and Webb S (1998) Generation of discrete beam‐intensity modulation by dynamic multileaf collimation under minimum leaf separation constraints . Phys Med Biol 43 , 2521–2538. [ PubMed ] [ Google Scholar ]
- Corradini S, Alongi F, Andratschke N, Belka C, Boldrini L, Cellini F, Debus J, Guckenberger M, Hörner‐Rieber J, Lagerwaard F et al (2019) MR‐guidance in clinical reality: current treatment challenges and future perspectives . Radiat Oncol 14 , 92. [ PMC free article ] [ PubMed ] [ Google Scholar ]
- De Bari B, Arcangeli S, Ciardo D, Mazzola R, Alongi F, Russi EG, Santoni R, Magrini SM and Jereczek‐Fossa BA (2016) Extreme hypofractionation for early prostate cancer: biology meets technology . Cancer Treat Rev 50 , 48–60. [ PubMed ] [ Google Scholar ]
- Durante M, Orecchia R and Loeffler JS (2017) Charged‐particle therapy in cancer: clinical uses and future perspectives . Nature Rev Clin Oncol 14 , 483–495. [ PubMed ] [ Google Scholar ]
- Favaudon V, Fouillade C and Vozenin MC (2015) Ultrahigh dose‐rate “flash” irradiation minimizes the side effects of radiotherapy . Cancer Radiother 19 , 526–531. [ PubMed ] [ Google Scholar ]
- Fiorino C, Muren LP, Clark CH, van Elmpt W and Jornet N (2015) Expanding the scientific role for medical physics in radiotherapy: time to act . Radiother Oncol 117 , 401–402. [ PubMed ] [ Google Scholar ]
- Goitein M and Cox JD (2008) Should randomized clinical trials be required for proton radiotherapy? J Clin Oncol 26 , 175–176. [ PubMed ] [ Google Scholar ]
- Gomez DR, Tang C, Zhang J, Blumenschein GR, Hernandez M, Lee JJ, Ye R, Palma DA, Louie AV, Camidge DR et al. (2019) Local consolidative therapy Vs. maintenance therapy or observation for patients with oligometastatic non–small‐cell lung cancer: long‐term results of a multi‐institutional, phase II, randomized study . J Clin Oncol 37 , 1558–1565. [ PMC free article ] [ PubMed ] [ Google Scholar ]
- Guckenberger M, Klement RJ, Allgäuer M, Andratschke N, Blanck O, Boda‐Heggemann J, Dieckmann K, Duma M, Ernst I, Ganswindt U et al. (2016) Local tumor control probability modeling of primary and secondary lung tumors in stereotactic body radiotherapy . Radiother Oncol 118 , 485–491. [ PubMed ] [ Google Scholar ]
- Hellman S and Weichselbaum RR (1995) Oligometastases . J Clin Oncol 13 , 8–10. [ PubMed ] [ Google Scholar ]
- Hussein M, Heijmen BJ, Verellen D and Nisbet A (2018) Automation in intensity‐modulated radiotherapy treatment planning – a review of recent innovations . Br J Radiol 91 , 20180270. [ PMC free article ] [ PubMed ] [ Google Scholar ]
- ICRU Report 83 (2010) Journal of the ICRU , Oxford University Press 10 No 1.
- Iyengar P, Wardak Z, Gerber DE, Tumati V, Ahn C, Hughes RS, Dowell JE, Cheedella N, Nedzi L, Westover KD et al (2018) Consolidative radiotherapy for limited metastatic non–small‐cell lung cancer: a phase 2 randomized clinical trial . JAMA Oncol 4 , e173501. [ PMC free article ] [ PubMed ] [ Google Scholar ]
- Jaffray DA (2005) Emergent technologies for 3‐dimensional image‐guided radiation delivery.Sem . Radiat Oncol 15 , 208–216. [ PubMed ] [ Google Scholar ]
- Jaffray DA (2012) Image‐guided radiotherapy: from current concept to future perspectives . Nature Rev Clin Oncol 9 , 688–699. [ PubMed ] [ Google Scholar ]
- Kallman P, Lind B, Eklof A and Brahme A (1988) Shaping of arbitrary dose distributions by dynamic multileaf collimation . Phys Med Biol 33 , 1291–1300. [ PubMed ] [ Google Scholar ]
- Klement RJ, Hoerner‐Rieber J, Adebahr S, Andratschke N, Blanck O, Boda‐Heggemann J, Duma M, Eble M, Eich H, Flentje M et al (2018) Stereotactic body radiotherapy (SBRT) for multiple pulmonary oligometastases: analysis of number and timing of repeat SBRT as impact factors on treatment safety and efficacy . Radiother Oncol 127 , 246–252. [ PubMed ] [ Google Scholar ]
- Klement RJ, Abbasi‐Senger N, Adebahr S, Alheid H, Allgaeuer M, Becker G, Blanck O, Boda‐Heggemann J, Brunner T, Duma M et al (2019) The impact of local control on overall survival after stereotactic body radiotherapy for liver and lung metastases from colorectal cancer: a combined analysis of 388 patients with 500 metastases . BMC Cancer 19 , 173. [ PMC free article ] [ PubMed ] [ Google Scholar ]
- Korreman SS (2012) Motion in radiotherapy: photon therapy . Phys Med Biol 57 , R161–R191. [ PubMed ] [ Google Scholar ]
- Krause M, Alsner J, Linge A, Butof R, Lock S and Bristow R (2020) Specific requirements for translation of biology into clinical radiation oncology . Mol Oncol , (in press). [ PMC free article ] [ PubMed ] [ Google Scholar ]
- Kroeze SGC, Fritz C, Hoyer M, Lo SS, Ricardi U, Sahgal A, Stahel R, Stupp R and Guckenberger M (2017) Toxicity of concurrent stereotactic radiotherapy and targeted therapy or immunotherapy: a systematic review . Cancer Treat Rev 53 , 25–37. [ PubMed ] [ Google Scholar ]
- Lambin P, Leijenaar RTH, Deist TM, Peerlings J, de Jong EEC, van Timmeren J, Sanduleanu S, Larue RTHM, Even AJG, Jochems A et al (2017) Radiomics: the bridge between medical imaging and personalized medicine . Nat Rev Clin Oncol 14 , 749–762. [ PubMed ] [ Google Scholar ]
- Lambin P, Van Stiphout RGPM, Starmans MHW, Rios‐Velazquez E, Nalbantov G, Aerts HJWL, Roelofs E, Van Elmpt W, Boutros PC, Granone P et al (2013) Predicting outcomes in radiation oncology‐multifactorial decision support systems . Nature Rev Clin Oncol 10 , 27–40. [ PMC free article ] [ PubMed ] [ Google Scholar ]
- Landry H and Hua CH (2018) Current state and future applications of radiological image guidance for particle therapy . Med Phys 45 , e1086–e1095. [ PubMed ] [ Google Scholar ]
- Langendijk JA, Lambin P, De Ruysscher D, Widder J, Bos M and Verheij M (2013) Selection of patients for radiotherapy with protons aiming at reduction of side effects: the model‐based approach . Radiother Oncol 107 , 267–273. [ PubMed ] [ Google Scholar ]
- Lax I, Blomgren H, Näslund I and Svanström R (1994) Stereotactic radiotherapy of malignancies in the abdomen: methodological aspects . Acta Oncol 33 , 677–683. [ PubMed ] [ Google Scholar ]
- Lewis S, Porceddu S, Nakamura N, Palma DA, Lo SS, Hoskin P, Moghanaki D, Chmura SJ and Salama JK (2017) Definitive Stereotactic Body Radiotherapy (SBRT) for extracranial oligometastases: an International survey of >1000 radiation oncologists . Am J Clin Oncol 40 , 418–422. [ PubMed ] [ Google Scholar ]
- Lievens Y, Borras JM and Grau C (2020) Provision and use of radiotherapy in Europe . Mol Oncol , (in press). [ PMC free article ] [ PubMed ] [ Google Scholar ]
- Lievens Y, Gospodarowicz M, Grover S, Jaffray D, Rodin D, Torode J, Yap ML and Zubizarreta E (2017) Global impact of radiotherapy in oncology: saving one million lives by 2035 . Radiother Oncol 125 , 175–177. [ PubMed ] [ Google Scholar ]
- Liney GP, Whelan B, Oborn B, Barton M and Keall P (2018) MRI‐Linear accelerator radiotherapy systems . Clin Oncol (R Coll Radiol) 30 , 686–691. [ PubMed ] [ Google Scholar ]
- Mackie TR and Swerdloff S (1993) Tomotherapy: A new concept for the delivery of dynamic conformal radiotherapy . Med Phys 20 , 1709–1719. [ PubMed ] [ Google Scholar ]
- McNutt TR, Moore KL, Wu B and Wright JL (2019) Use of big data for quality assurance in radiation therapy . Semin Radiat Oncol 29 , 326–332. [ PubMed ] [ Google Scholar ]
- Meyer P, Noblet V, Mazzara C and Lallement A (2018) Survey on deep learning for radiotherapy . Comp Biol Med 98 , 126–146. [ PubMed ] [ Google Scholar ]
- Mondini M, Levy A, Meziani L, Milliat F and Deutsch E (2020) Radiotherapy‐immunotherapy combinations: perspectives and challenges . Mol Oncol . 10.1002/1878-0261.12658 [ PMC free article ] [ PubMed ] [ CrossRef ] [ Google Scholar ]
- Palma DA, Olson R, Harrow S, Gaede S, Louie AV, Haasbeek C, Mulroy L, Lock M, Rodrigues GB, Yaremko BP et al (2019) Stereotactic ablative radiotherapy versus standard of care palliative treatment in patients with oligometastatic cancers (SABR‐COMET): a randomised, phase 2, open‐label trial . The Lancet 393 , 2051–2058. [ PubMed ] [ Google Scholar ]
- Patriarca A, Fouillade C, Auger M, Martin F, Pouzoulet F, Nauraye C, Heinrich S, Favaudon V, Meyroneinc S, Dendale R et al (2018) Experimental set‐up for FLASH proton irradiation of small animals using a clinical system . Int J Radiat Oncol Biol Phys 102 , 619–626. [ PubMed ] [ Google Scholar ]
- Pratz G and Xing L (2011) GPU computing in medical physics: a review . Med Phys 38 , 2685–2697. [ PubMed ] [ Google Scholar ]
- Rieber J, Abbassi‐Senger N, Adebahr S, Andratschke N, Blanck O, Duma M, Eble MJ, Ernst I, Flentje M, Gerum S, et al (2017) Influence of Institutional experience and technological advances on outcome of stereotactic body radiation therapy for oligometastatic lung disease . Int J Radiat Oncol 98 , 511–520. [ PubMed ] [ Google Scholar ]
- Ruers T, Van Coevorden F, Punt CJA, Pierie J‐PEN, Borel‐Rinkes I, Ledermann JA, Poston G, Bechstein W, Lentz M‐A, Mauer M et al (2017) Local treatment of unresectable colorectal liver metastases: results of a randomized phase II trial . J Natl Cancer Inst 109 10.1093/jnci/djx015 [ PMC free article ] [ PubMed ] [ CrossRef ] [ Google Scholar ]
- Sahiner B, Pezeshk A, Hadjiiski LM, Wang X, Drukker K, Cha KH, Summers RM and Giger ML (2019) Deep learning in medical imaging and radiation therapy . Med Phys 46 , e1–e36. [ PMC free article ] [ PubMed ] [ Google Scholar ]
- Schanne DH, Heitmann J, Guckenberger M and Andratschke NHJ (2019) Evolution of treatment strategies for oligometastatic NSCLC patients – a systematic review of the literature . Cancer Treat Rev 80 , 101892. [ PubMed ] [ Google Scholar ]
- Schippers JM, Lomax A, Garonna A and Parodi K (2018) Can technological improvements reduce the cost of proton radiation therapy? Semin Radiat Oncol 28 , 150–159. [ PubMed ] [ Google Scholar ]
- Schültke E, Balosso J, Breslin T, Cavaletti G, Djonov V, Esteve F, Grotzer M, Hildebrandt G, Valdman A and Laissue J (2017) Microbeam radiation therapy – grid therapy and beyond: a clinical perspective . Brit J Radiol 90 , art no 20170073. [ PMC free article ] [ PubMed ] [ Google Scholar ]
- Schweikard A, Glosser G, Bodduluri M, Murphy MJ and Adler JR (2000) Robotic motion compensation for respiratory movement during radiosurgery . Comp Aided Surg 5 , 263–277. [ PubMed ] [ Google Scholar ]
- Sokol O, Scifoni E, Tinganelli W, Kraft‐Weyrather W, Wiedemann J, Maier A, Boscolo D, Friedrich T, Brons S, Durante M et al (2017) Oxygen beams for therapy: advanced biological treatment planning and experimental verification . Phys Med Biol 62 , 7798–7813. [ PubMed ] [ Google Scholar ]
- Sonke JJ, Aznar M and Rasch C (2019) Adaptive radiotherapy for anatomical changes . Semin Radiat Oncol 29 , 245–257. [ PubMed ] [ Google Scholar ]
- Sonke JJ, Lebesque JV and Van Herk M (2008) Variability of four‐dimensional computed tomography patient models . Int J Radiat Oncol Biol Phys 70 , 590–598. [ PubMed ] [ Google Scholar ]
- Tanderup K, Ménard C, Polgar C, Lindegaard JC, Kirisits C and Pötter R (2017) Advancements in brachytherapy . Adv Drug Deliv Rev 109 , 15–25. [ PubMed ] [ Google Scholar ]
- Thariat J, Hannoun‐Levi J‐M, Sun Myint A, Vuong T and Gérard J‐P (2013) Past, present, and future of radiotherapy for the benefit of patients . Nature Rev Clin Oncol 10 , 52–60. [ PubMed ] [ Google Scholar ]
- Thompson RF, Valdes G, Fuller CD, Carpenter CM, Morin O, Aneja S, Lindsay WD, Aerts HJWL, Agrimson B, Deville C et al (2018) Artificial intelligence in radiation oncology: a specialty‐wide disruptive transformation? Radiother Oncol 129 , 421–426. [ PMC free article ] [ PubMed ] [ Google Scholar ]
- Thörnqvist S, Hysing LB, Tuomikoski L, Vestergaard A, Tanderup K, Muren LP and Heijmen BJM (2016) Adaptive radiotherapy strategies for pelvic tumors – a systematic review of clinical implementations . Acta Oncol 55 , 943–958. [ PubMed ] [ Google Scholar ]
- Van Der Schaaf A, Langendijk JA, Fiorino C and Rancati T (2015) Embracing phenomenological approaches to normal tissue complication probability modelling: a question of method . Int J Radiat Oncol Biol Phys 91 , 468–471. [ PubMed ] [ Google Scholar ]
- Vassiliev ON, Titt U, Pönisch F, Kry SF, Mohan R and Gillin MT (2006) Dosimetric properties of photon beams from a flattening filter free clinical accelerator . Phys Med Biol 51 , 1907–1917. [ PubMed ] [ Google Scholar ]
- Veldeman L, Madani I, Hulstaert F, De Meerleer G, Mareel M and De Neve W (2008) Evidence behind use of intensity‐modulated radiotherapy: a systematic review of comparative clinical studies . Lancet Oncol 9 , 367–375. [ PubMed ] [ Google Scholar ]
- Xie Y, Bentefour EH, Janssens G, Smeets J, Vander Stappen F, Hotoiu L, Yin L, Dolney D, Avery S, O'Grady F et al (2017) Prompt gamma imaging for in vivo range verification of pencil beam scanning proton therapy . Int J Radiat Oncol Biol Phys 99 , 210–218. [ PubMed ] [ Google Scholar ]
- Yan D, Vicini F, Wong J and Martinez A (1997) Adaptive radiation therapy . Phys Med Biol 42 , 123–132. [ PubMed ] [ Google Scholar ]
- Yu CX (1995) Intensity‐modulated arc therapy with dynamic multileaf collimation: an alternative to tomotherapy . Phys Med Biol 40 , 1435–1449. [ PubMed ] [ Google Scholar ]

Head Start Your Radiology Residency [Online] ↗️
- Radiology Thesis – More than 400 Research Topics (2022)!
Please login to bookmark
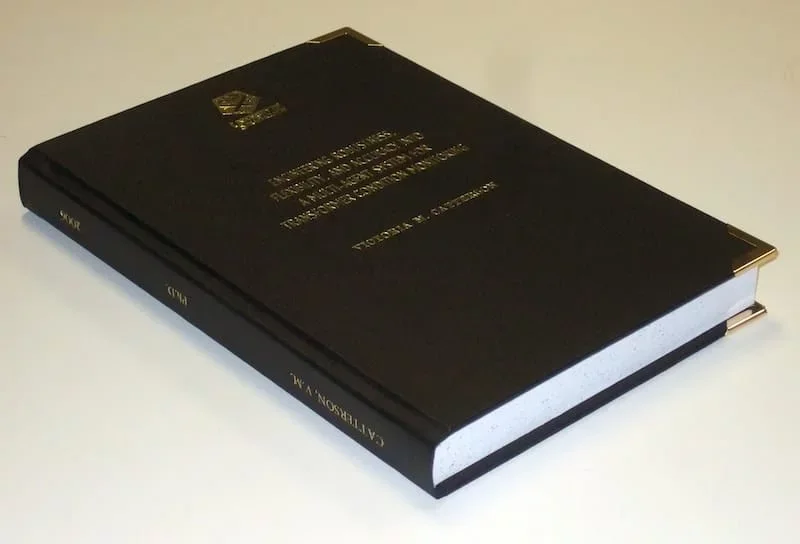
Introduction
A thesis or dissertation, as some people would like to call it, is an integral part of the Radiology curriculum, be it MD, DNB, or DMRD. We have tried to aggregate radiology thesis topics from various sources for reference.
Not everyone is interested in research, and writing a Radiology thesis can be daunting. But there is no escape from preparing, so it is better that you accept this bitter truth and start working on it instead of cribbing about it (like other things in life. #PhilosophyGyan!)
Start working on your thesis as early as possible and finish your thesis well before your exams, so you do not have that stress at the back of your mind. Also, your thesis may need multiple revisions, so be prepared and allocate time accordingly.
Tips for Choosing Radiology Thesis and Research Topics
Keep it simple silly (kiss).
Retrospective > Prospective
Retrospective studies are better than prospective ones, as you already have the data you need when choosing to do a retrospective study. Prospective studies are better quality, but as a resident, you may not have time (, energy and enthusiasm) to complete these.
Choose a simple topic that answers a single/few questions
Original research is challenging, especially if you do not have prior experience. I would suggest you choose a topic that answers a single or few questions. Most topics that I have listed are along those lines. Alternatively, you can choose a broad topic such as “Role of MRI in evaluation of perianal fistulas.”
You can choose a novel topic if you are genuinely interested in research AND have a good mentor who will guide you. Once you have done that, make sure that you publish your study once you are done with it.
Get it done ASAP.
In most cases, it makes sense to stick to a thesis topic that will not take much time. That does not mean you should ignore your thesis and ‘Ctrl C + Ctrl V’ from a friend from another university. Thesis writing is your first step toward research methodology so do it as sincerely as possible. Do not procrastinate in preparing the thesis. As soon as you have been allotted a guide, start researching topics and writing a review of the literature.
At the same time, do not invest a lot of time in writing/collecting data for your thesis. You should not be busy finishing your thesis a few months before the exam. Some people could not appear for the exam because they could not submit their thesis in time. So DO NOT TAKE thesis lightly.
Do NOT Copy-Paste
Reiterating once again, do not simply choose someone else’s thesis topic. Find out what are kind of cases that your Hospital caters to. It is better to do a good thesis on a common topic than a crappy one on a rare one.
Books to help you write a Radiology Thesis
Event country/university has a different format for thesis; hence these book recommendations may not work for everyone.

- Amazon Kindle Edition
- Gupta, Piyush (Author)
- English (Publication Language)
- 206 Pages - 10/12/2020 (Publication Date) - Jaypee Brothers Medical Publishers (P) Ltd. (Publisher)
In A Hurry? Download a PDF list of Radiology Research Topics!
Sign up below to get this PDF directly to your email address.
100% Privacy Guaranteed. Your information will not be shared. Unsubscribe anytime with a single click.
List of Radiology Research /Thesis / Dissertation Topics
- State of the art of MRI in the diagnosis of hepatic focal lesions
- Multimodality imaging evaluation of sacroiliitis in newly diagnosed patients of spondyloarthropathy
- Multidetector computed tomography in oesophageal varices
- Role of positron emission tomography with computed tomography in the diagnosis of cancer Thyroid
- Evaluation of focal breast lesions using ultrasound elastography
- Role of MRI diffusion tensor imaging in the assessment of traumatic spinal cord injuries
- Sonographic imaging in male infertility
- Comparison of color Doppler and digital subtraction angiography in occlusive arterial disease in patients with lower limb ischemia
- The role of CT urography in Haematuria
- Role of functional magnetic resonance imaging in making brain tumor surgery safer
- Prediction of pre-eclampsia and fetal growth restriction by uterine artery Doppler
- Role of grayscale and color Doppler ultrasonography in the evaluation of neonatal cholestasis
- Validity of MRI in the diagnosis of congenital anorectal anomalies
- Role of sonography in assessment of clubfoot
- Role of diffusion MRI in preoperative evaluation of brain neoplasms
- Imaging of upper airways for pre-anaesthetic evaluation purposes and for laryngeal afflictions.
- A study of multivessel (arterial and venous) Doppler velocimetry in intrauterine growth restriction
- Multiparametric 3tesla MRI of suspected prostatic malignancy.
- Role of Sonography in Characterization of Thyroid Nodules for differentiating benign from
- Role of advances magnetic resonance imaging sequences in multiple sclerosis
- Role of multidetector computed tomography in evaluation of jaw lesions
- Role of Ultrasound and MR Imaging in the Evaluation of Musculotendinous Pathologies of Shoulder Joint
- Role of perfusion computed tomography in the evaluation of cerebral blood flow, blood volume and vascular permeability of cerebral neoplasms
- MRI flow quantification in the assessment of the commonest csf flow abnormalities
- Role of diffusion-weighted MRI in evaluation of prostate lesions and its histopathological correlation
- CT enterography in evaluation of small bowel disorders
- Comparison of perfusion magnetic resonance imaging (PMRI), magnetic resonance spectroscopy (MRS) in and positron emission tomography-computed tomography (PET/CT) in post radiotherapy treated gliomas to detect recurrence
- Role of multidetector computed tomography in evaluation of paediatric retroperitoneal masses
- Role of Multidetector computed tomography in neck lesions
- Estimation of standard liver volume in Indian population
- Role of MRI in evaluation of spinal trauma
- Role of modified sonohysterography in female factor infertility: a pilot study.
- The role of pet-CT in the evaluation of hepatic tumors
- Role of 3D magnetic resonance imaging tractography in assessment of white matter tracts compromise in supratentorial tumors
- Role of dual phase multidetector computed tomography in gallbladder lesions
- Role of multidetector computed tomography in assessing anatomical variants of nasal cavity and paranasal sinuses in patients of chronic rhinosinusitis.
- magnetic resonance spectroscopy in multiple sclerosis
- Evaluation of thyroid nodules by ultrasound elastography using acoustic radiation force impulse (ARFI) imaging
- Role of Magnetic Resonance Imaging in Intractable Epilepsy
- Evaluation of suspected and known coronary artery disease by 128 slice multidetector CT.
- Role of regional diffusion tensor imaging in the evaluation of intracranial gliomas and its histopathological correlation
- Role of chest sonography in diagnosing pneumothorax
- Role of CT virtual cystoscopy in diagnosis of urinary bladder neoplasia
- Role of MRI in assessment of valvular heart diseases
- High resolution computed tomography of temporal bone in unsafe chronic suppurative otitis media
- Multidetector CT urography in the evaluation of hematuria
- Contrast-induced nephropathy in diagnostic imaging investigations with intravenous iodinated contrast media
- Comparison of dynamic susceptibility contrast-enhanced perfusion magnetic resonance imaging and single photon emission computed tomography in patients with little’s disease
- Role of Multidetector Computed Tomography in Bowel Lesions.
- Role of diagnostic imaging modalities in evaluation of post liver transplantation recipient complications.
- Role of multislice CT scan and barium swallow in the estimation of oesophageal tumour length
- Malignant Lesions-A Prospective Study.
- Value of ultrasonography in assessment of acute abdominal diseases in pediatric age group
- Role of three dimensional multidetector CT hysterosalpingography in female factor infertility
- Comparative evaluation of multi-detector computed tomography (MDCT) virtual tracheo-bronchoscopy and fiberoptic tracheo-bronchoscopy in airway diseases
- Role of Multidetector CT in the evaluation of small bowel obstruction
- Sonographic evaluation in adhesive capsulitis of shoulder
- Utility of MR Urography Versus Conventional Techniques in Obstructive Uropathy
- MRI of the postoperative knee
- Role of 64 slice-multi detector computed tomography in diagnosis of bowel and mesenteric injury in blunt abdominal trauma.
- Sonoelastography and triphasic computed tomography in the evaluation of focal liver lesions
- Evaluation of Role of Transperineal Ultrasound and Magnetic Resonance Imaging in Urinary Stress incontinence in Women
- Multidetector computed tomographic features of abdominal hernias
- Evaluation of lesions of major salivary glands using ultrasound elastography
- Transvaginal ultrasound and magnetic resonance imaging in female urinary incontinence
- MDCT colonography and double-contrast barium enema in evaluation of colonic lesions
- Role of MRI in diagnosis and staging of urinary bladder carcinoma
- Spectrum of imaging findings in children with febrile neutropenia.
- Spectrum of radiographic appearances in children with chest tuberculosis.
- Role of computerized tomography in evaluation of mediastinal masses in pediatric
- Diagnosing renal artery stenosis: Comparison of multimodality imaging in diabetic patients
- Role of multidetector CT virtual hysteroscopy in the detection of the uterine & tubal causes of female infertility
- Role of multislice computed tomography in evaluation of crohn’s disease
- CT quantification of parenchymal and airway parameters on 64 slice MDCT in patients of chronic obstructive pulmonary disease
- Comparative evaluation of MDCT and 3t MRI in radiographically detected jaw lesions.
- Evaluation of diagnostic accuracy of ultrasonography, colour Doppler sonography and low dose computed tomography in acute appendicitis
- Ultrasonography , magnetic resonance cholangio-pancreatography (MRCP) in assessment of pediatric biliary lesions
- Multidetector computed tomography in hepatobiliary lesions.
- Evaluation of peripheral nerve lesions with high resolution ultrasonography and colour Doppler
- Multidetector computed tomography in pancreatic lesions
- Multidetector Computed Tomography in Paediatric abdominal masses.
- Evaluation of focal liver lesions by colour Doppler and MDCT perfusion imaging
- Sonographic evaluation of clubfoot correction during Ponseti treatment
- Role of multidetector CT in characterization of renal masses
- Study to assess the role of Doppler ultrasound in evaluation of arteriovenous (av) hemodialysis fistula and the complications of hemodialysis vasular access
- Comparative study of multiphasic contrast-enhanced CT and contrast-enhanced MRI in the evaluation of hepatic mass lesions
- Sonographic spectrum of rheumatoid arthritis
- Diagnosis & staging of liver fibrosis by ultrasound elastography in patients with chronic liver diseases
- Role of multidetector computed tomography in assessment of jaw lesions.
- Role of high-resolution ultrasonography in the differentiation of benign and malignant thyroid lesions
- Radiological evaluation of aortic aneurysms in patients selected for endovascular repair
- Role of conventional MRI, and diffusion tensor imaging tractography in evaluation of congenital brain malformations
- To evaluate the status of coronary arteries in patients with non-valvular atrial fibrillation using 256 multirow detector CT scan
- A comparative study of ultrasonography and CT – arthrography in diagnosis of chronic ligamentous and meniscal injuries of knee
- Multi detector computed tomography evaluation in chronic obstructive pulmonary disease and correlation with severity of disease
- Diffusion weighted and dynamic contrast enhanced magnetic resonance imaging in chemoradiotherapeutic response evaluation in cervical cancer.
- High resolution sonography in the evaluation of non-traumatic painful wrist
- The role of trans-vaginal ultrasound versus magnetic resonance imaging in diagnosis & evaluation of cancer cervix
- Role of multidetector row computed tomography in assessment of maxillofacial trauma
- Imaging of vascular complication after liver transplantation.
- Role of magnetic resonance perfusion weighted imaging & spectroscopy for grading of glioma by correlating perfusion parameter of the lesion with the final histopathological grade
- Magnetic resonance evaluation of abdominal tuberculosis.
- Diagnostic usefulness of low dose spiral HRCT in diffuse lung diseases
- Role of dynamic contrast enhanced and diffusion weighted magnetic resonance imaging in evaluation of endometrial lesions
- Contrast enhanced digital mammography anddigital breast tomosynthesis in early diagnosis of breast lesion
- Evaluation of Portal Hypertension with Colour Doppler flow imaging and magnetic resonance imaging
- Evaluation of musculoskeletal lesions by magnetic resonance imaging
- Role of diffusion magnetic resonance imaging in assessment of neoplastic and inflammatory brain lesions
- Radiological spectrum of chest diseases in HIV infected children High resolution ultrasonography in neck masses in children
- with surgical findings
- Sonographic evaluation of peripheral nerves in type 2 diabetes mellitus.
- Role of perfusion computed tomography in the evaluation of neck masses and correlation
- Role of ultrasonography in the diagnosis of knee joint lesions
- Role of ultrasonography in evaluation of various causes of pelvic pain in first trimester of pregnancy.
- Role of Magnetic Resonance Angiography in the Evaluation of Diseases of Aorta and its Branches
- MDCT fistulography in evaluation of fistula in Ano
- Role of multislice CT in diagnosis of small intestine tumors
- Role of high resolution CT in differentiation between benign and malignant pulmonary nodules in children
- A study of multidetector computed tomography urography in urinary tract abnormalities
- Role of high resolution sonography in assessment of ulnar nerve in patients with leprosy.
- Pre-operative radiological evaluation of locally aggressive and malignant musculoskeletal tumours by computed tomography and magnetic resonance imaging.
- The role of ultrasound & MRI in acute pelvic inflammatory disease
- Ultrasonography compared to computed tomographic arthrography in the evaluation of shoulder pain
- Role of Multidetector Computed Tomography in patients with blunt abdominal trauma.
- The Role of Extended field-of-view Sonography and compound imaging in Evaluation of Breast Lesions
- Evaluation of focal pancreatic lesions by Multidetector CT and perfusion CT
- Evaluation of breast masses on sono-mammography and colour Doppler imaging
- Role of CT virtual laryngoscopy in evaluation of laryngeal masses
- Triple phase multi detector computed tomography in hepatic masses
- Role of transvaginal ultrasound in diagnosis and treatment of female infertility
- Role of ultrasound and color Doppler imaging in assessment of acute abdomen due to female genetal causes
- High resolution ultrasonography and color Doppler ultrasonography in scrotal lesion
- Evaluation of diagnostic accuracy of ultrasonography with colour Doppler vs low dose computed tomography in salivary gland disease
- Role of multidetector CT in diagnosis of salivary gland lesions
- Comparison of diagnostic efficacy of ultrasonography and magnetic resonance cholangiopancreatography in obstructive jaundice: A prospective study
- Evaluation of varicose veins-comparative assessment of low dose CT venogram with sonography: pilot study
- Role of mammotome in breast lesions
- The role of interventional imaging procedures in the treatment of selected gynecological disorders
- Role of transcranial ultrasound in diagnosis of neonatal brain insults
- Role of multidetector CT virtual laryngoscopy in evaluation of laryngeal mass lesions
- Evaluation of adnexal masses on sonomorphology and color Doppler imaginig
- Role of radiological imaging in diagnosis of endometrial carcinoma
- Comprehensive imaging of renal masses by magnetic resonance imaging
- The role of 3D & 4D ultrasonography in abnormalities of fetal abdomen
- Diffusion weighted magnetic resonance imaging in diagnosis and characterization of brain tumors in correlation with conventional MRI
- Role of diffusion weighted MRI imaging in evaluation of cancer prostate
- Role of multidetector CT in diagnosis of urinary bladder cancer
- Role of multidetector computed tomography in the evaluation of paediatric retroperitoneal masses.
- Comparative evaluation of gastric lesions by double contrast barium upper G.I. and multi detector computed tomography
- Evaluation of hepatic fibrosis in chronic liver disease using ultrasound elastography
- Role of MRI in assessment of hydrocephalus in pediatric patients
- The role of sonoelastography in characterization of breast lesions
- The influence of volumetric tumor doubling time on survival of patients with intracranial tumours
- Role of perfusion computed tomography in characterization of colonic lesions
- Role of proton MRI spectroscopy in the evaluation of temporal lobe epilepsy
- Role of Doppler ultrasound and multidetector CT angiography in evaluation of peripheral arterial diseases.
- Role of multidetector computed tomography in paranasal sinus pathologies
- Role of virtual endoscopy using MDCT in detection & evaluation of gastric pathologies
- High resolution 3 Tesla MRI in the evaluation of ankle and hindfoot pain.
- Transperineal ultrasonography in infants with anorectal malformation
- CT portography using MDCT versus color Doppler in detection of varices in cirrhotic patients
- Role of CT urography in the evaluation of a dilated ureter
- Characterization of pulmonary nodules by dynamic contrast-enhanced multidetector CT
- Comprehensive imaging of acute ischemic stroke on multidetector CT
- The role of fetal MRI in the diagnosis of intrauterine neurological congenital anomalies
- Role of Multidetector computed tomography in pediatric chest masses
- Multimodality imaging in the evaluation of palpable & non-palpable breast lesion.
- Sonographic Assessment Of Fetal Nasal Bone Length At 11-28 Gestational Weeks And Its Correlation With Fetal Outcome.
- Role Of Sonoelastography And Contrast-Enhanced Computed Tomography In Evaluation Of Lymph Node Metastasis In Head And Neck Cancers
- Role Of Renal Doppler And Shear Wave Elastography In Diabetic Nephropathy
- Evaluation Of Relationship Between Various Grades Of Fatty Liver And Shear Wave Elastography Values
- Evaluation and characterization of pelvic masses of gynecological origin by USG, color Doppler and MRI in females of reproductive age group
- Radiological evaluation of small bowel diseases using computed tomographic enterography
- Role of coronary CT angiography in patients of coronary artery disease
- Role of multimodality imaging in the evaluation of pediatric neck masses
- Role of CT in the evaluation of craniocerebral trauma
- Role of magnetic resonance imaging (MRI) in the evaluation of spinal dysraphism
- Comparative evaluation of triple phase CT and dynamic contrast-enhanced MRI in patients with liver cirrhosis
- Evaluation of the relationship between carotid intima-media thickness and coronary artery disease in patients evaluated by coronary angiography for suspected CAD
- Assessment of hepatic fat content in fatty liver disease by unenhanced computed tomography
- Correlation of vertebral marrow fat on spectroscopy and diffusion-weighted MRI imaging with bone mineral density in postmenopausal women.
- Comparative evaluation of CT coronary angiography with conventional catheter coronary angiography
- Ultrasound evaluation of kidney length & descending colon diameter in normal and intrauterine growth-restricted fetuses
- A prospective study of hepatic vein waveform and splenoportal index in liver cirrhosis: correlation with child Pugh’s classification and presence of esophageal varices.
- CT angiography to evaluate coronary artery by-pass graft patency in symptomatic patient’s functional assessment of myocardium by cardiac MRI in patients with myocardial infarction
- MRI evaluation of HIV positive patients with central nervous system manifestations
- MDCT evaluation of mediastinal and hilar masses
- Evaluation of rotator cuff & labro-ligamentous complex lesions by MRI & MRI arthrography of shoulder joint
- Role of imaging in the evaluation of soft tissue vascular malformation
- Role of MRI and ultrasonography in the evaluation of multifidus muscle pathology in chronic low back pain patients
- Role of ultrasound elastography in the differential diagnosis of breast lesions
- Role of magnetic resonance cholangiopancreatography in evaluating dilated common bile duct in patients with symptomatic gallstone disease.
- Comparative study of CT urography & hybrid CT urography in patients with haematuria.
- Role of MRI in the evaluation of anorectal malformations
- Comparison of ultrasound-Doppler and magnetic resonance imaging findings in rheumatoid arthritis of hand and wrist
- Role of Doppler sonography in the evaluation of renal artery stenosis in hypertensive patients undergoing coronary angiography for coronary artery disease.
- Comparison of radiography, computed tomography and magnetic resonance imaging in the detection of sacroiliitis in ankylosing spondylitis.
- Mr evaluation of painful hip
- Role of MRI imaging in pretherapeutic assessment of oral and oropharyngeal malignancy
- Evaluation of diffuse lung diseases by high resolution computed tomography of the chest
- Mr evaluation of brain parenchyma in patients with craniosynostosis.
- Diagnostic and prognostic value of cardiovascular magnetic resonance imaging in dilated cardiomyopathy
- Role of multiparametric magnetic resonance imaging in the detection of early carcinoma prostate
- Role of magnetic resonance imaging in white matter diseases
- Role of sonoelastography in assessing the response to neoadjuvant chemotherapy in patients with locally advanced breast cancer.
- Role of ultrasonography in the evaluation of carotid and femoral intima-media thickness in predialysis patients with chronic kidney disease
- Role of H1 MRI spectroscopy in focal bone lesions of peripheral skeleton choline detection by MRI spectroscopy in breast cancer and its correlation with biomarkers and histological grade.
- Ultrasound and MRI evaluation of axillary lymph node status in breast cancer.
- Role of sonography and magnetic resonance imaging in evaluating chronic lateral epicondylitis.
- Comparative of sonography including Doppler and sonoelastography in cervical lymphadenopathy.
- Evaluation of Umbilical Coiling Index as Predictor of Pregnancy Outcome.
- Computerized Tomographic Evaluation of Azygoesophageal Recess in Adults.
- Lumbar Facet Arthropathy in Low Backache.
- “Urethral Injuries After Pelvic Trauma: Evaluation with Uretrography
- Role Of Ct In Diagnosis Of Inflammatory Renal Diseases
- Role Of Ct Virtual Laryngoscopy In Evaluation Of Laryngeal Masses
- “Ct Portography Using Mdct Versus Color Doppler In Detection Of Varices In
- Cirrhotic Patients”
- Role Of Multidetector Ct In Characterization Of Renal Masses
- Role Of Ct Virtual Cystoscopy In Diagnosis Of Urinary Bladder Neoplasia
- Role Of Multislice Ct In Diagnosis Of Small Intestine Tumors
- “Mri Flow Quantification In The Assessment Of The Commonest CSF Flow Abnormalities”
- “The Role Of Fetal Mri In Diagnosis Of Intrauterine Neurological CongenitalAnomalies”
- Role Of Transcranial Ultrasound In Diagnosis Of Neonatal Brain Insults
- “The Role Of Interventional Imaging Procedures In The Treatment Of Selected Gynecological Disorders”
- Role Of Radiological Imaging In Diagnosis Of Endometrial Carcinoma
- “Role Of High-Resolution Ct In Differentiation Between Benign And Malignant Pulmonary Nodules In Children”
- Role Of Ultrasonography In The Diagnosis Of Knee Joint Lesions
- “Role Of Diagnostic Imaging Modalities In Evaluation Of Post Liver Transplantation Recipient Complications”
- “Diffusion-Weighted Magnetic Resonance Imaging In Diagnosis And
- Characterization Of Brain Tumors In Correlation With Conventional Mri”
- The Role Of PET-CT In The Evaluation Of Hepatic Tumors
- “Role Of Computerized Tomography In Evaluation Of Mediastinal Masses In Pediatric patients”
- “Trans Vaginal Ultrasound And Magnetic Resonance Imaging In Female Urinary Incontinence”
- Role Of Multidetector Ct In Diagnosis Of Urinary Bladder Cancer
- “Role Of Transvaginal Ultrasound In Diagnosis And Treatment Of Female Infertility”
- Role Of Diffusion-Weighted Mri Imaging In Evaluation Of Cancer Prostate
- “Role Of Positron Emission Tomography With Computed Tomography In Diagnosis Of Cancer Thyroid”
- The Role Of CT Urography In Case Of Haematuria
- “Value Of Ultrasonography In Assessment Of Acute Abdominal Diseases In Pediatric Age Group”
- “Role Of Functional Magnetic Resonance Imaging In Making Brain Tumor Surgery Safer”
- The Role Of Sonoelastography In Characterization Of Breast Lesions
- “Ultrasonography, Magnetic Resonance Cholangiopancreatography (MRCP) In Assessment Of Pediatric Biliary Lesions”
- “Role Of Ultrasound And Color Doppler Imaging In Assessment Of Acute Abdomen Due To Female Genital Causes”
- “Role Of Multidetector Ct Virtual Laryngoscopy In Evaluation Of Laryngeal Mass Lesions”
- MRI Of The Postoperative Knee
- Role Of Mri In Assessment Of Valvular Heart Diseases
- The Role Of 3D & 4D Ultrasonography In Abnormalities Of Fetal Abdomen
- State Of The Art Of Mri In Diagnosis Of Hepatic Focal Lesions
- Role Of Multidetector Ct In Diagnosis Of Salivary Gland Lesions
- “Role Of Virtual Endoscopy Using Mdct In Detection & Evaluation Of Gastric Pathologies”
- The Role Of Ultrasound & Mri In Acute Pelvic Inflammatory Disease
- “Diagnosis & Staging Of Liver Fibrosis By Ultraso Und Elastography In
- Patients With Chronic Liver Diseases”
- Role Of Mri In Evaluation Of Spinal Trauma
- Validity Of Mri In Diagnosis Of Congenital Anorectal Anomalies
- Imaging Of Vascular Complication After Liver Transplantation
- “Contrast-Enhanced Digital Mammography And Digital Breast Tomosynthesis In Early Diagnosis Of Breast Lesion”
- Role Of Mammotome In Breast Lesions
- “Role Of MRI Diffusion Tensor Imaging (DTI) In Assessment Of Traumatic Spinal Cord Injuries”
- “Prediction Of Pre-eclampsia And Fetal Growth Restriction By Uterine Artery Doppler”
- “Role Of Multidetector Row Computed Tomography In Assessment Of Maxillofacial Trauma”
- “Role Of Diffusion Magnetic Resonance Imaging In Assessment Of Neoplastic And Inflammatory Brain Lesions”
- Role Of Diffusion Mri In Preoperative Evaluation Of Brain Neoplasms
- “Role Of Multidetector Ct Virtual Hysteroscopy In The Detection Of The
- Uterine & Tubal Causes Of Female Infertility”
- Role Of Advances Magnetic Resonance Imaging Sequences In Multiple Sclerosis Magnetic Resonance Spectroscopy In Multiple Sclerosis
- “Role Of Conventional Mri, And Diffusion Tensor Imaging Tractography In Evaluation Of Congenital Brain Malformations”
- Role Of MRI In Evaluation Of Spinal Trauma
- Diagnostic Role Of Diffusion-weighted MR Imaging In Neck Masses
- “The Role Of Transvaginal Ultrasound Versus Magnetic Resonance Imaging In Diagnosis & Evaluation Of Cancer Cervix”
- “Role Of 3d Magnetic Resonance Imaging Tractography In Assessment Of White Matter Tracts Compromise In Supra Tentorial Tumors”
- Role Of Proton MR Spectroscopy In The Evaluation Of Temporal Lobe Epilepsy
- Role Of Multislice Computed Tomography In Evaluation Of Crohn’s Disease
- Role Of MRI In Assessment Of Hydrocephalus In Pediatric Patients
- The Role Of MRI In Diagnosis And Staging Of Urinary Bladder Carcinoma
- USG and MRI correlation of congenital CNS anomalies
- HRCT in interstitial lung disease
- X-Ray, CT and MRI correlation of bone tumors
- “Study on the diagnostic and prognostic utility of X-Rays for cases of pulmonary tuberculosis under RNTCP”
- “Role of magnetic resonance imaging in the characterization of female adnexal pathology”
- “CT angiography of carotid atherosclerosis and NECT brain in cerebral ischemia, a correlative analysis”
- Role of CT scan in the evaluation of paranasal sinus pathology
- USG and MRI correlation on shoulder joint pathology
- “Radiological evaluation of a patient presenting with extrapulmonary tuberculosis”
- CT and MRI correlation in focal liver lesions”
- Comparison of MDCT virtual cystoscopy with conventional cystoscopy in bladder tumors”
- “Bleeding vessels in life-threatening hemoptysis: Comparison of 64 detector row CT angiography with conventional angiography prior to endovascular management”
- “Role of transarterial chemoembolization in unresectable hepatocellular carcinoma”
- “Comparison of color flow duplex study with digital subtraction angiography in the evaluation of peripheral vascular disease”
- “A Study to assess the efficacy of magnetization transfer ratio in differentiating tuberculoma from neurocysticercosis”
- “MR evaluation of uterine mass lesions in correlation with transabdominal, transvaginal ultrasound using HPE as a gold standard”
- “The Role of power Doppler imaging with trans rectal ultrasonogram guided prostate biopsy in the detection of prostate cancer”
- “Lower limb arteries assessed with doppler angiography – A prospective comparative study with multidetector CT angiography”
- “Comparison of sildenafil with papaverine in penile doppler by assessing hemodynamic changes”
- “Evaluation of efficacy of sonosalphingogram for assessing tubal patency in infertile patients with hysterosalpingogram as the gold standard”
- Role of CT enteroclysis in the evaluation of small bowel diseases
- “MRI colonography versus conventional colonoscopy in the detection of colonic polyposis”
- “Magnetic Resonance Imaging of anteroposterior diameter of the midbrain – differentiation of progressive supranuclear palsy from Parkinson disease”
- “MRI Evaluation of anterior cruciate ligament tears with arthroscopic correlation”
- “The Clinicoradiological profile of cerebral venous sinus thrombosis with prognostic evaluation using MR sequences”
- “Role of MRI in the evaluation of pelvic floor integrity in stress incontinent patients” “Doppler ultrasound evaluation of hepatic venous waveform in portal hypertension before and after propranolol”
- “Role of transrectal sonography with colour doppler and MRI in evaluation of prostatic lesions with TRUS guided biopsy correlation”
- “Ultrasonographic evaluation of painful shoulders and correlation of rotator cuff pathologies and clinical examination”
- “Colour Doppler Evaluation of Common Adult Hepatic tumors More Than 2 Cm with HPE and CECT Correlation”
- “Clinical Relevance of MR Urethrography in Obliterative Posterior Urethral Stricture”
- “Prediction of Adverse Perinatal Outcome in Growth Restricted Fetuses with Antenatal Doppler Study”
- Radiological evaluation of spinal dysraphism using CT and MRI
- “Evaluation of temporal bone in cholesteatoma patients by high resolution computed tomography”
- “Radiological evaluation of primary brain tumours using computed tomography and magnetic resonance imaging”
- “Three dimensional colour doppler sonographic assessment of changes in volume and vascularity of fibroids – before and after uterine artery embolization”
- “In phase opposed phase imaging of bone marrow differentiating neoplastic lesions”
- “Role of dynamic MRI in replacing the isotope renogram in the functional evaluation of PUJ obstruction”
- Characterization of adrenal masses with contrast-enhanced CT – washout study
- A study on accuracy of magnetic resonance cholangiopancreatography
- “Evaluation of median nerve in carpal tunnel syndrome by high-frequency ultrasound & color doppler in comparison with nerve conduction studies”
- “Correlation of Agatston score in patients with obstructive and nonobstructive coronary artery disease following STEMI”
- “Doppler ultrasound assessment of tumor vascularity in locally advanced breast cancer at diagnosis and following primary systemic chemotherapy.”
- “Validation of two-dimensional perineal ultrasound and dynamic magnetic resonance imaging in pelvic floor dysfunction.”
- “Role of MR urethrography compared to conventional urethrography in the surgical management of obliterative urethral stricture.”
Search Diagnostic Imaging Research Topics
You can also search research-related resources on our custom search engine .
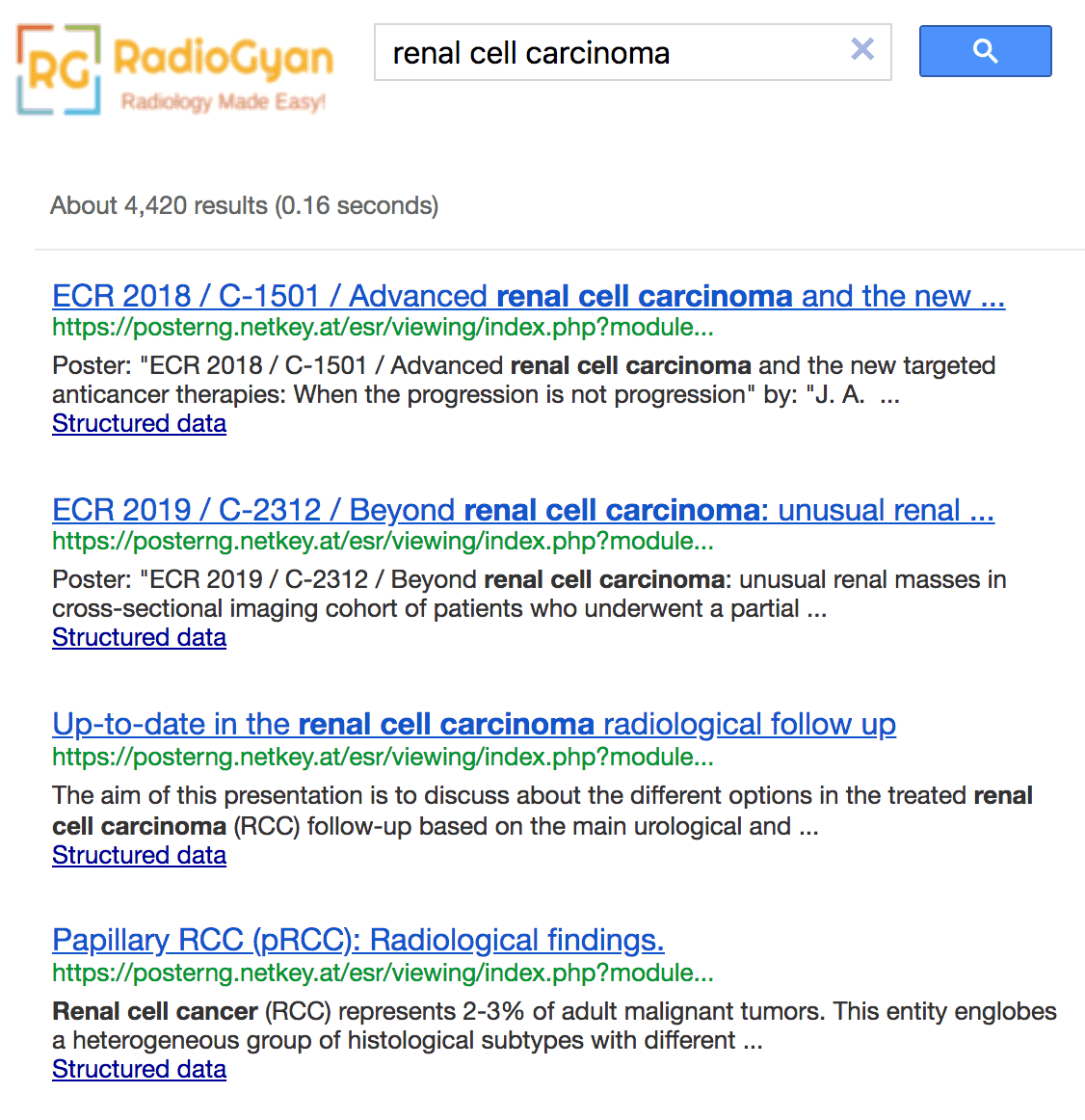
Free Resources for Preparing Radiology Thesis
- Radiology thesis topics- Benha University – Free to download thesis
- Radiology thesis topics – Faculty of Medical Science Delhi
- Radiology thesis topics – IPGMER
- Fetal Radiology thesis Protocols
- Radiology thesis and dissertation topics
- Radiographics
Proofreading Your Thesis:
Make sure you use Grammarly to correct your spelling , grammar , and plagiarism for your thesis. Grammarly has affordable paid subscriptions, windows/macOS apps, and FREE browser extensions. It is an excellent tool to avoid inadvertent spelling mistakes in your research projects. It has an extensive built-in vocabulary, but you should make an account and add your own medical glossary to it.

Guidelines for Writing a Radiology Thesis:
These are general guidelines and not about radiology specifically. You can share these with colleagues from other departments as well. Special thanks to Dr. Sanjay Yadav sir for these. This section is best seen on a desktop. Here are a couple of handy presentations to start writing a thesis:
Read the general guidelines for writing a thesis (the page will take some time to load- more than 70 pages!
A format for thesis protocol with a sample patient information sheet, sample patient consent form, sample application letter for thesis, and sample certificate.
Resources and References:
- Guidelines for thesis writing.
- Format for thesis protocol
- Thesis protocol writing guidelines DNB
- Informed consent form for Research studies from AIIMS
- Radiology Informed consent forms in local Indian languages.
- Sample Informed Consent form for Research in Hindi
- Guide to write a thesis by Dr. P R Sharma
- Guidelines for thesis writing by Dr. Pulin Gupta.
- Preparing MD/DNB thesis by A Indrayan
- Another good thesis reference protocol
Hopefully, this post will make the tedious task of writing a Radiology thesis a little bit easier for you. Best of luck with writing your thesis and your residency too!
More guides for residents :
- Guide for the MD/DMRD/DNB radiology exam!
- Guide for First-Year Radiology Residents
- FRCR Exam: THE Most Comprehensive Guide (2022)!
- Radiology Practical Exams Questions compilation for MD/DNB/DMRD !
Radiology Exam Resources (Oral Recalls, Instruments, etc )!
- Tips and Tricks for DNB/MD Radiology Practical Exam
- FRCR 2B exam- Tips and Tricks !
FRCR exam preparation – An alternative take!
- Why did I take up Radiology?
- Radiology Conferences – A comprehensive guide!
- ECR (European Congress Of Radiology)
- European Diploma in Radiology (EDiR) – The Complete Guide!
- Radiology NEET PG guide – How to select THE best college for post-graduation in Radiology (includes personal insights)!
- Interventional Radiology – All Your Questions Answered!
- What It Means To Be A Radiologist: A Guide For Medical Students!
- Radiology Mentors for Medical Students (Post NEET-PG)
- MD vs DNB Radiology: Which Path is Right for Your Career?
- DNB Radiology OSCE – Tips and Tricks
More radiology resources here: Radiology resources This page will be updated regularly. Kindly leave your feedback in the comments or send us a message here . Also, you can comment below regarding your department’s thesis topics.
Note: All topics have been compiled from available online resources. If anyone has an issue with any radiology thesis topics displayed here, you can message us here , and we can delete them. These are only sample guidelines. Thesis guidelines differ from institution to institution.
Image source: Thesis complete! (2018). Flickr. Retrieved 12 August 2018, from https://www.flickr.com/photos/cowlet/354911838 by Victoria Catterson
About The Author
Dr. amar udare, md, related posts ↓.
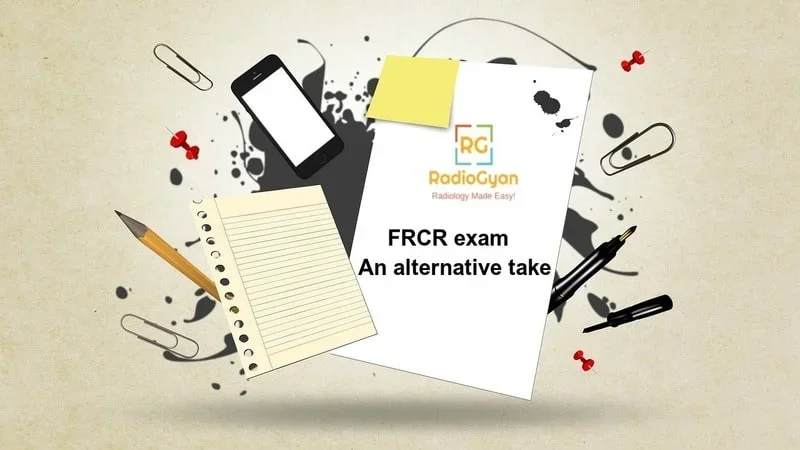
7 thoughts on “Radiology Thesis – More than 400 Research Topics (2022)!”
Amazing & The most helpful site for Radiology residents…
Thank you for your kind comments 🙂
Dr. I saw your Tips is very amazing and referable. But Dr. Can you help me with the thesis of Evaluation of Diagnostic accuracy of X-ray radiograph in knee joint lesion.
Wow! These are excellent stuff. You are indeed a teacher. God bless
Glad you liked these!
happy to see this
Glad I could help :).
Leave a Comment Cancel Reply
Your email address will not be published. Required fields are marked *
Get Radiology Updates to Your Inbox!
This site is for use by medical professionals. To continue, you must accept our use of cookies and the site's Terms of Use. Learn more Accept!
Wish to be a BETTER Radiologist? Join 14000 Radiology Colleagues !
Enter your email address below to access HIGH YIELD radiology content, updates, and resources.
No spam, only VALUE! Unsubscribe anytime with a single click.
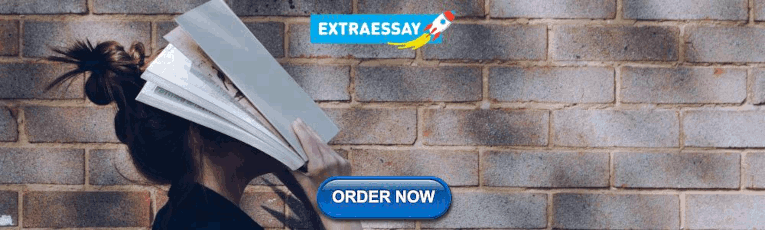
IMAGES
VIDEO
COMMENTS
Abstract. X-ray imaging is a low-cost, powerful technology that has been extensively used in medical diagnosis and industrial nondestructive inspection. The ability of X-rays to penetrate through the body presents great advances for noninvasive imaging of its internal structure. In particular, the technological importance of X-ray imaging has ...
INTRODUCTION. Artificial intelligence (AI) has been revolutionizing the field of healthcare, including radiology, with its potential to transform the way medical professionals diagnose and treat diseases 1, 2.Radiology has traditionally been perceived as a dynamic and cutting-edge field that employs advanced imaging technologies to visualize the internal structures of the human body.
Explore the latest full-text research PDFs, articles, conference papers, preprints and more on RADIOLOGIC TECHNOLOGY. Find methods information, sources, references or conduct a literature review ...
This study aims to explore a framework that can predict Radiologic Physics achievement among RT students. Subjects were 954 Radiologic Physics students (480 males and 474 females) randomly selected from 12 Radiologic Technology schools in the Philippines. Their ages ranged from 18 to 22 years (mean age 19.5, SD 2.4).
OBJECTIVE. Consistent excellence in radiologic technologist performance, including ensuring high technical image quality, patient safety and comfort, and efficient workflow, largely depends on individual technologist skill. However, sustained growth in the size and complexity of health care organizations has increased the difficulty in developing and maintaining technologist expertise. In this ...
Radiology historically has been a leader of digital transformation in healthcare. The introduction of digital imaging systems, picture archiving and communication systems (PACS), and teleradiology transformed radiology services over the past 30 years. Radiology is again at the crossroad for the next generation of transformation, possibly evolving as a one-stop integrated diagnostic service ...
In this era of value-directed care, emphasizing patient primacy and optimizing patient care are paramount to the future of our specialty. The American College of Radiology (ACR) has previously issued white papers on teleradiology [2, 9].In this article, we describe recent changes, current challenges, and future opportunities centered around the ability of teleradiology to improve patient ...
The advent of Deep Learning (DL) has significantly propelled the field of diagnostic radiology forward by enhancing image analysis and interpretation. The introduction of the Transformer architecture, followed by the development of Large Language Models (LLMs), has further revolutionized this domain. LLMs now possess the potential to automate and refine the radiology workflow, extending from ...
We further discuss future research directions and challenges in developing advanced next-generation materials that are crucial to the fabrication of flexible, low-dose, high-resolution X-ray imaging detectors. 1. Introduction. X-rays are a type of ionizing radiation with a wavelength ranging from 0.01 to 10 nm [ 1, 2 ].
The Radiologic Technology Students' Perceptions of the Clinical Learning Environment I verify that my dissertation represents original research, is not falsified or plagiarized, and that I accurately reported, cited, and referenced all sources within this manuscript in strict compliance with APA and Grand Canyon University (GCU) guidelines.
This domain of AI has been the focus of many research papers as it directly relates to the roles and responsibilities of a radiologist. However, discussions on the impact of such technology on the radiography profession are often overlooked. To address this gap in the literature, this paper aims to address the application of AI in radiography ...
WHITE PAPER. 2 ITE PAPER The Arti˜cial Intelligence Era: The Role of Radiologic Technologists and Radiation Therapists ... Dartmouth Summer Research Project on Artificial Intelligence. Also in the 1950s, a team of researchers at Massachusetts Institute of Technology (MIT) began work that would become the MIT Computer Science and Artificial ...
2-year radiologic technology programs in the United States, as well as the state in which the study was conducted, and the limited amount of research previously conducted on the topic of student persistence from enrollment to graduation in 2-year radiologic technology programs. Chapter 1 also includes the problem, purpose, research questions,
Radiologic Technology. This bimonthly scholarly journal features peer-reviewed research and practical columns spanning the radiologic sciences. ... research and innovation. 15000 Central Ave. SE Albuquerque, NM 87123-3909. 800-444-2778; 505-298-4500; 505-298-5063 fax; [email protected]; Member Services Hours: 7:30 a.m. to 4:30 p.m.
From advancements in imaging technology to the evaluation of diagnostic accuracy and the impact of radiological interventions, these topics offer a glimpse into the exciting world of radiology research. ... Choosing a radiology research paper topic requires careful consideration and alignment with your interests, expertise, and the current ...
Technology‐driven research has a pivotal role in the advancement of radiotherapy, still more in the current 'high‐tech' era. Larger efforts in developing efficient and affordable technology solutions to deliver personalized radiotherapy, even in middle‐/low‐income countries, are necessary. In order to facilitate a rapid and ...
This research shows the employment profile of the graduates of Bachelor of Science in Radiologic Technology in Lyceum of the Philippines St. Cabrini school years 2016-2017 and 2017-2018. ... The practice of Radiologic Technology profession however has no preference as to male or female practitioners. It just happened that there are more females ...
Introduction. A thesis or dissertation, as some people would like to call it, is an integral part of the Radiology curriculum, be it MD, DNB, or DMRD. We have tried to aggregate radiology thesis topics from various sources for reference. Not everyone is interested in research, and writing a Radiology thesis can be daunting.