Advertisement
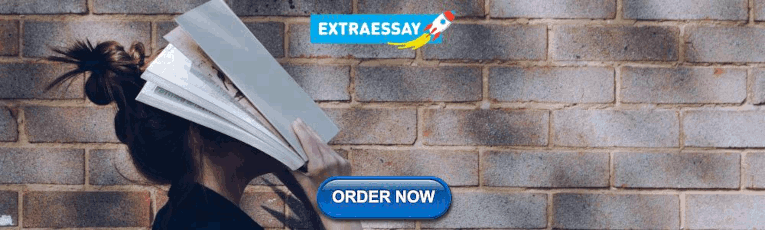
Unemployment Scarring Effects: An Overview and Meta-analysis of Empirical Studies
- Review article
- Published: 17 May 2023
Cite this article
- Mattia Filomena ORCID: orcid.org/0000-0002-4099-9168 1 , 2
769 Accesses
3 Altmetric
Explore all metrics
This article reviews the empirical literature on the scarring effects of unemployment, by first presenting an overview of empirical evidence relating to the impact of unemployment spells on subsequent labor market outcomes and then exploiting meta-regression techniques. Empirical evidence is homogeneous in highlighting significant and often persistent wage losses and strong unemployment state dependence. This is confirmed by a meta-regression analysis under the assumption of a common true effect. Heterogeneous findings emerge in the literature, related to the magnitude of these detrimental effects, which are particularly penalizing in terms of labor earnings in case of unemployment periods experienced by laid-off workers. We shed light on further sources of heterogeneity and find that unemployment is particularly scarring for men and when studies’ identification strategy is based on selection on observables.
This is a preview of subscription content, log in via an institution to check access.
Access this article
Price includes VAT (Russian Federation)
Instant access to the full article PDF.
Rent this article via DeepDyve
Institutional subscriptions
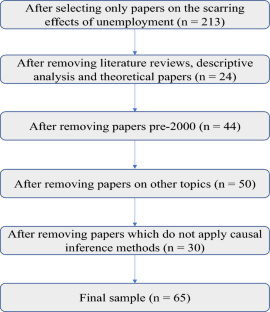
Similar content being viewed by others
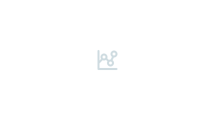
The gender pay gap in the USA: a matching study
Katie Meara, Francesco Pastore & Allan Webster
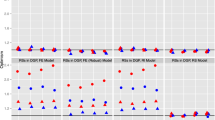
Fixed and random effects models: making an informed choice
Andrew Bell, Malcolm Fairbrother & Kelvyn Jones
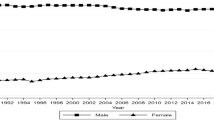
Patterns and Causes of Female Labor Force Participation: An Age–Period–Cohort Analysis for Pakistan
Hina Amber & Bezawit Beyene Chichaibelu
Data Availability
The datasets generated and analyzed during the current study are available from the corresponding author on reasonable request.
Moreover, further outcomes discussed by the literature on scarring are family formation, crime and negative psychological implications in terms of well-being, life satisfaction and mental health (see e.g. Helbling and Sacchi 2014 ; Strandh et al. 2014 ; Mousteri et al. 2018 ; Clark and Lepinteur 2019 ).
A further strand of the recent literature focuses on the effect of adverse labor market conditions at graduation, for example focusing on the effect of local unemployment rate or graduating during a recession (see e.g. Raaum and Roed, 2006 ; Kahn 2010 ; Oreopoulos et al. 2012 ; Kawaguchi and Murao 2014 ; Altonji et al. 2016 ). The consequences of economic downturns on wages, labor supply and social outcomes for young labor market entrants have been recently surveyed by Cockx ( 2016 ), Von Wachter (2020) and Rodriguez et al. ( 2020 ).
The stigma effect means that individuals who have been unemployed face lower chances of being hired because employers may use their past history of unemployment as a negative signal.
Thus, papers using traditional multivariate descriptive analysis, duration models, or OLS regressions with a reduced number of controls which do not properly address endogeneity issues and are unlikely to have a causal interpretation (endogeneity issues are discussed in SubSect. 3.2 ).
For intergenerational scars we mean that studies focused on the effect of parents’ unemployment experiences on the children’ future employment status (see e.g. Karhula et al. 2017 ). For macroeconomic conditions at graduation we mean that we excluded that literature focused on the local unemployment rate at graduation or other local labor market conditions, rather than on individual unemployment experience and state dependence (see e.g. Oreopoulos et al. 2012 ; Raaem and Roed, 2006 ).
When we could not directly retrieve the t -statistics because not reported among the study results, we computed them as the ratio between the estimated unemployment effects ( \({\beta }_{i}\) ) and their standard errors. If studies only displayed the estimated effects and their 95% confidence intervals, the standard error can be calculated by SE = ( ub − lb )/(2 × 1.96), where ub and lb are the upper bound and the lower bound, respectively.
We removed from the meta-regression analysis 8 articles because they did not contain sufficient information to compute the t -statistic of the estimated scarring effect. They are reported in italics in Tables 5 and 6 .
For employment outcomes we mean the likelihood of experiencing future unemployment, the probability to have a job later (employability), the fraction of days spent at work or the hours worked during the following years (labor market participation), the call-backs from employers in case of field experiment. Earning outcomes include hourly wages, labor earnings, income, etc.
Since many studies did not provide precise information on the number of covariates, we approximated \({dk}_{i}\) with the number of observations minus 2. Indeed, given that in microeconometric applications the sample sizes are very often much larger than the number of the parameters, the calculation of the partial correlation coefficient is quite robust to errors in deriving \({dk}_{i}\) (Picchio 2022 ).
The publication bias is the bias arising from the tendency of editors to publish more easily findings consistent with a conventional view or with statistically significant results, whereas studies that find small or no significant effects tend to remain unpublished (Card and Krueger 1995 ).
We employed the Precision Effect Estimate with Standard Error (PEESE) specification because its quadratic form of the standard errors has been proven to be less biased and often more efficient to check for heterogeneity than the FAT-PET specification when there is a nonzero genuine effect (Stanley and Doucouliagos 2014 ). Nevertheless, the results from the FAT-PET specification are very similar to the ones from the PEESE model.
Abebe DS, Hyggen C (2019) Moderators of unemployment and wage scarring during the transition to young adulthood: evidence from Norway. In: Hvinden B, Oreilly J, Schoyen MA, Hyggen C (eds) Negotiating early job insecurity. Elgar Publishing, Cheltenham
Google Scholar
Adascalitei D, Morano CP (2016) Drivers and effects of labour market reforms: evidence from a novel policy compendium. IZA J Labor Policy 5(1):1–32
Article Google Scholar
Ahmad N (2014) State dependence in unemployment. Int J Econ Financ Issues 4(1):93
Altonji JG, Kahn LB, Speer JD (2016) Cashier or consultant? Entry labor market conditions, field of study, and career success. J Law Econ 34(1):S361–S401
Arranz JM, García-Serrano C (2003) Non-employment and subsequent wage losses. Instituto de Estudios Fiscales, No. 19-03
Arranz JM, Davia MA, García-Serrano C (2005) Labour market transitions and wage dynamics in Europe. ISER Working Paper Series, No. 2005-17
Arulampalam W (2001) Is unemployment really scarring? Effects of unemployment experiences on wages. Econ J 111(475):F585–F606
Arulampalam W, Booth AL, Taylor MP (2000) Unemployment persistence. Oxf Econ Pap 52(1):24–50
Arulampalam W, Gregg P, Gregory M (2001) Introduction: unemployment scarring. Econ J 111(475):F577–F584
Ayllón S (2013) Unemployment persistence: not only stigma but discouragement too. Appl Econ Lett 20(1):67–71
Ayllón S, Valbuena J, Plum A (2021) Youth unemployment and stigmatization over the business cycle in Europe. Oxford Bull Econ Stat 84(1):103–129
Baert S, Verhaest D (2019) Unemployment or overeducation: which is a worse signal to employers? De Econ 167(1):1–21
Baumann I (2016) The debate about the consequences of job displacement. Springer, Cham, pp 1–33
Becker, Gary. 1975. Human capital: a theoretical and empirical analysis, with special reference to education. Second Edition. National Bureau of Economic Research, Inc.
Biewen M, Steffes S (2010) Unemployment persistence: is there evidence for stigma effects? Econ Lett 106(3):188–190
Birkelund GE, Heggebø K, Rogstad J (2017) Additive or multiplicative disadvantage? The scarring effects of unemployment for ethnic minorities. Eur Sociol Rev 33(1):17–29
Borland J (2020) Scarring effects: a review of Australian and international literature. Aust J Labour Econ 23(2):173–188
Bratberg E, Nilsen OA (2000) Transitions from school to work and the early labour market experience. Oxford Bull Econ Stat 62:909–929
Brodeur A, Cook N, Heyes A (2020) Methods matter: p-hacking and publication bias in causal analysis in economics. Am Econ Rev 110(11):3634–3660
Brodeur A, Lé M, Sangnier M, Zylberberg Y (2016) Star wars: the empirics strike back. Am Econ J Appl Econ 8(1):1–32
Burda MC, Mertens A (2001) Estimating wage losses of displaced workers in Germany. Labour Econ 8(1):15–41
Burdett K (1978) A theory of employee job search and quit rates. Am Econ Rev 68(1):212–220
Böheim R, Taylor MP (2002) The search for success: do the unemployed find stable employment? Labour Econ 9(6):717–735
Cameron CA, Gelbach JB, Miller DL (2008) Bootstrap-based improvements for inference with clustered errors. Rev Econ Stat 90(3):414–427
Card D, Krueger AB (1995) Time-series minimum-wage studies: a meta-analysis. Am Econ Rev 85(2):238–243
Chamberlain G (1984) Panel data. Handb Econ 2:1247–1318
Clark AE, Lepinteur A (2019) The causes and consequences of early-adult unemployment: evidence from cohort data. J Econ Behav Organ 166:107–124
Cockx B, Picchio M (2013) Scarring effects of remaining unemployed for long-term unemployed school-leavers. J R Stat Soc A Stat Soc 176(4):951–980
Cockx B (2016) Do youths graduating in a recession incur permanent losses? IZA World Labor
Couch KA (2001) Earnings losses and unemployment of displaced workers in Germany. ILR Rev 54(3):559–572
Deelen A, de Graaf-Zijl M, van den Berge W (2018) Labour market effects of job displacement for prime-age and older workers. IZA J Labor Econ 7(1):3
Dieckhoff M (2011) The effect of unemployment on subsequent job quality in Europe: a comparative study of four countries. Acta Sociol 54(3):233–249
Doiron D, Gørgens T (2008) State dependence in youth labor market experiences, and the evaluation of policy interventions. J Econometr 145(1–2):81–97
Dorsett R, Lucchino P (2018) Young people’s labour market transitions: the role of early experiences. Labour Econ 54:29–46
Doucouliagos H (1995) Worker participation and productivity in labor-managed and participatory capitalist firms: a meta-analysis. ILR Rev 49(1):58–77
Eicher TS, Papageorgiou C, Raftery AE (2011) Default priors and predictive performance in Bayesian model averaging, with application to growth determinants. J Appl Economet 26(1):30–55
Eliason M, Storrie D (2006) Lasting or latent scars? Swedish evidence on the long-term effects of job displacement. J Law Econ 24(4):831–856
Eriksson S, Rooth D-O (2014) Do employers use unemployment as a sorting criterion when hiring? Evidence from a field experiment. Am Econ Rev 104(3):1014–1039
Fallick BC (1996) A review of the recent empirical literature on displaced workers. ILR Rev 50(1):5–16
Farber HS, Herbst CM, Silverman D, Von Watcher T (2019) Whom do employers want? The role of recent employment and unemployment status and age. J Law Econ 37(2):323–349
Farber HS, Silverman D, Von Watcher T (2016) Determinants of callbacks to job applications: an audit study. Am Econ Rev 106(5):314–318
Farber HS, Silverman D, Von Watcher T (2017) Factors determining callbacks to job applications by the unemployed: an audit study. RSF Russell Sage Found J Soc Sci 3(3):168–201
Filomena M, Picchio M (2022) Retirement and health outcomes in a meta-analytical framework. J Econ Surv (forthcoming)
Fraja De, Gianni SL, Rockey J (2021) The wounds that do not heal: the lifetime scar of youth unemployment. Economica 88(352):896–941
Gangji A, Plasman R (2008) Microeconomic analysis of unemployment persistence in Belgium. Int J Manpow 29(3):280–298
Gangji A, Plasman R (2007) The Matthew effect of unemployment: how does it affect wages in Belgium. DULBEA Working Papers 07-19.RS, ULB—Universite Libre de Bruxelles
Gangl M (2004) Welfare states and the scar effects of unemployment: a comparative analysis of the United States and West Germany. Am J Sociol 109(6):1319–1364
Gangl M (2006) Scar effects of unemployment: an assessment of institutional complementarities. Am Sociol Rev 71(6):986–1013
Gartell M (2009) Unemployment and subsequent earnings for Swedish college graduates. A study of scarring effects. Arbetsrapport 2009:2, Institute for Futures Studies
Gaure S, Røed K, Westlie L (2008) The impacts of labor market policies on job search behavior and post-unemployment job quality. IZA Discussion Papers 3802, Institute of Labor Economics (IZA)
Ghirelli C (2015) Scars of early non-employment for low educated youth: evidence and policy lessons from Belgium. IZA J Eur Labor Stud 4(1):20
Gibbons R, Katz LF (1991) Layoffs and lemons. J Law Econ 9(4):351–380
Gregg P (2001) The impact of youth unemployment on adult unemployment in the NCDS. Econ J 111(475):F626–F653
Gregg P, Tominey E (2005) The wage scar from male youth unemployment. Labour Econ 12(4):487–509
Gregory M, Jukes R (2001) Unemployment and subsequent earnings: estimating scarring among British men 1984–94. Econ J 111(475):607–625
Guvenen F, Karahan F, Ozkan S, Song J (2017) Heterogeneous scarring effects of full-year nonemployment. Am Econ Rev 107(5):369–373
Hamermesh DS (1989) What do we know about worker displacement in the US? Ind Relat J Econ Soc 28(1):51–59
Havránek T, Horvath R, Irsova Z, Rusnak M (2015) Cross-country heterogeneity in intertemporal substitution. J Int Econ 96(1):100–118
Havránek T, Stanley TD, Doucouliagos H, Bom P, Geyer-Klingeberg J, Ichiro Iwasaki W, Reed R, Rost K, van Aert RCM (2020) Reporting guidelines for meta-analysis in economics. J Econ Surv 34(3):469–475
Heckman JJ (1979) Sample selection bias as a specification error. Econometrica 47(1):153–161
Helbling LA, Sacchi S (2014) Scarring effects of early unemployment among young workers with vocational credentials in Switzerland. Emp Res Voc Educ Train 6(1):12
Heylen V (2011) Scarring, the effects of early career unemployment. In ECPR General conference, 2011/08/24–2011/08/27, University of Iceland, Reykjavik
Hämäläinen K (2003) Education and unemployment: state dependence in unemployment among young people in the 1990s. VATT Institute for Economic Research, No. 312
Jacobson LS, LaLonde RJ, Sullivan DG (1993) Earnings losses of displaced workers. Am Econ Rev 83(4):685–709
Jovanovic B (1979a) Firm-specific capital and turnover. J Polit Econ 87(6):1246–1260
Jovanovic B (1979b) Job matching and the theory of turnover. J Polit Econ 87(5, Part 1):972–990
Kahn LB (2010) The long-term labor market consequences of graduating from college in a bad economy. Labour Econ 17(2):303–316
Karhula A, Lehti H, Erola J (2017) Intergenerational scars? The long-term effects of parental unemployment during a depression. Res Finn Soc 10:87–99
Kawaguchi D, Murao T (2014) Labor-market institutions and long-term effects of youth unemployment. J Money Credit Bank 46(S2):95–116
Kletzer LG (1998) Job displacement. J Econ Perspect 12(1):115–136
Kletzer LG, Fairlie RW (2003) The long-term costs of job displacement for young adult workers. ILR Rev 56(4):682–698
Knights S, Harris MN, Loundes J (2002) Dynamic relationships in the Australian labour market: heterogeneity and state dependence. Econ Record 78(242):284–298
Kroft K, Lange F, Notowidigdo MJ (2013) Duration dependence and labor market conditions: evidence from a field experiment. Q J Econ 128(3):1123–1167
Kuchibhotla M, Orazem PF, Ravi S (2020) The scarring effects of youth joblessness in Sri Lanka. Rev Dev Econ 24(1):269–287
Lazear EP (1986) Raids and offer matching. In: Ehrenberg R (ed) Research in Labor Economics, vol 8. JAI Press, Greenwich
Lockwood B (1991) Information externalities in the labour market and the duration of unemployment. Rev Econ Stud 58(4):733–753
De Luca G, Magnus JR (2011) Bayesian model averaging and weighted-average least squares: equivariance, stability, and numerical issues. Stata Journal 11(4):518–544
Lupi C, Ordine P (2002) Unemployment scarring in high unemployment regions. Econ Bull 10(2):1–8
Magnus JR, De Luca G (2016) Weighted-average least squares (WALS): a survey. J Econ Surv 30(1):117–148
Magnus JR, Powell O, Prüfer P (2010) A comparison of two model averaging techniques with an application to growth empirics. J Econometr 154(2):139–153
Manzoni A, Mooi-Reci I (2011) Early unemployment and subsequent career complexity: a sequence-based perspective. Schmollers Jahrbuch J Appl Soc Sci Stud ZeitschrFür Wirtschaftsund Sozialwissenschaften 131(2):339–348
Mavromaras K, Sloane P, Wei Z (2015) The scarring effects of unemployment, low pay and skills under-utilization in Australia compared. Appl Econ 47(23):2413–2429
Mincer J (1974) Schooling, experience, and earnings. National Bureau of Economic Research Inc, Cambridge
Mooi-Reci I, Ganzeboom HB (2015) Unemployment scarring by gender: human capital depreciation or stigmatization? Longitudinal evidence from the Netherlands, 1980–2000. Soc Sci Res 52:642–658
Mortensen DT (1987) Job search and labor market analysis. In: Ashenfelter O, Layard R (eds) Handbook of labor economics, vol 2, Chapter 15, pp 849–919, Elsevier
Mortensen DT (1988) Wages, separations, and job tenure: on-the-job specific training or matching? J Law Econ 6(4):445–471
Mousteri V, Daly M, Delaney L (2018) The scarring effect of unemployment on psychological well-being across Europe. Soc Sci Res 72:146–169
Mroz TA, Savage TH (2006) The long-term effects of youth unemployment. J Hum Resour 41(2):259–293
Mundlak Y (1978) On the pooling of time series and cross section data. Econometrica 69–85
Möller J, Umkehrer M (2015) Are there long-term earnings scars from youth unemployment in Germany? Jahrbücher Für Nationalökon Und Stat 235(4–5):474–498
Nekoei A, Weber A (2017) Does extending unemployment benefits improve job quality? American Economic Review 107(2):527–561
Nickell S, Jones P, Quintini G (2002) A picture of job insecurity facing British men. Econ J 112(476):1–27
Nilsen ØA, Reiso KH (2014) Scarring effects of early-career unemployment. Nord Econ Policy Rev 1:13–46
Nordström Skans O (2011) Scarring effects of the first labor market experience. IZA Discussion Papers 5565, Institute of Labor Economics (IZA)
Nunley JM, Pugh A, Romero N, Alan Seals R (2017) The effects of unemployment and underemployment on employment opportunities: results from a correspondence audit of the labor market for college graduates. ILR Rev 70(3):642–669
Nüß P (2018) Duration dependence as an unemployment stigma: evidence from a field experiment in Germany. Technical report, Economics Working Paper.
Oberholzer-Gee F (2008) Nonemployment stigma as rational herding: a field experiment. J Econ Behav Organ 65(1):30–40
Omori Y (1997) Stigma effects of nonemployment. Econ Inq 35(2):394–416
Ordine P, Rose G (2015) Educational mismatch and unemployment scarring. Int J Manpower 36(5):733
Oreopoulos P, Von Watcher T, Heisz A (2012) The short- and long-term career effects of graduating in a recession. Am Econ J Appl Econ 4(1):1–29
Pastore F, Quintano C, Rocca A (2021) Some young people have all the luck! The duration dependence of the school-to-work transition in Europe. Labour Econ 70:101982
Petreski M, Mojsoska-Blazevski N, Bergolo M (2017) Labor-market scars when youth unemployment is extremely high: evidence from Macedonia. East Eur Econ 55(2):168–196
Picchio M, van Ours JC (2013) Retaining through training even for older workers. Econ Educ Rev 32(1):29–48
Picchio M (2022) Meta-analysis. In: Zimmermann KF (eds) Handbook of labor, human resources and population economics. Springer, Cham. (forthcoming)
Pissarides CA (1992) Loss of skill during unemployment and the persistence of employment shocks. Q J Econ 107(4):1371–1391
Plum A, Ayllón S (2015) Heterogeneity in unemployment state dependence. Econ Lett 136:85–87
Raaum O, Røed K (2006) Do business cycle conditions at the time of labor market entry affect future employment prospects? Rev Econ Stat 88(2):193–210
Rodriguez JS, Colston J, Wu Z, Chen Z (2020) Graduating during a recession: a literature review of the effects of recessions for college graduates. Centre for College Workforce Transitions (CCWT), University of Wisconsin
Schmillen A, Umkehrer M (2017) The scars of youth: effects of early-career unemployment on future unemployment experience. Int Labour Rev 156(3–4):465–494
Shi LP, Wang S (2021) Demand-side consequences of unemployment and horizontal skill mismatches across national contexts: an employer-based factorial survey experiment. Soc Sci Res 104:102668
Spence M (1973) Job market signaling. Q J Econ 87(3):355–374
Spivey C (2005) Time off at what price? The effects of career interruptions on earnings. ILR Rev 59(1):119–140
Stanley TD (2005) Beyond publication bias. J Econ Surv 19(3):309–345
Stanley TD (2008) Meta-regression methods for detecting and estimating empirical effects in the presence of publication selection. Oxford Bull Econ Stat 70(1):103–127
Stanley TD, Doucouliagos H (2014) Meta-regression approximations to reduce publication selection bias. Res Synth Methods 5(1):60–78
Stewart MB (2007) The interrelated dynamics of unemployment and low-wage employment. J Appl Economet 22(3):511–531
Strandh M, Winefield A, Nilsson K, Hammarström A (2014) Unemployment and mental health scarring during the life course. Eur J Pub Health 24(3):440–445
Tanzi GM (2022) Scars of youth non-employment and labour market conditions. Italian Econ J (forthcoming)
Tatsiramos K (2009) Unemployment insurance in Europe: unemployment duration and subsequent employment stability. J Eur Econ Assoc 7(6):1225–1260
Tumino A (2015) The scarring effect of unemployment from the early’90s to the great recession. ISER Working Paper Series, No. 2015-05
Ugur M (2014) Corruption’s direct effects on per-capita income growth: a meta-analysis. J Econ Surv 28(3):472–490
Verho J (2008) Scars of recession: the long-term costs of the Finnish economic crisis. Working Paper Series 2008:9, IFAU—Institute for Evaluation of Labour Market and Education Policy
Vishwanath T (1989) Job search, stigma effect, and escape rate from unemployment. J Law Econ 7(4):487–502
Von Wachter T (2020) The persistent effects of initial labor market conditions for young adults and their sources. J Econ Perspect 34(4):168–194
Vooren M, Haelermans C, Groot W, van den Brink HM (2019) The effectiveness of active labor market policies: a meta-analysis. J Econ Surv 33(1):125–149
Webb MD (2014) Reworking wild bootstrap based inference for clustered errors. Queen’s Economics Department Working Paper No. 1315, Kingston, Canada
Wooldridge JM (2005) Simple solutions to the initial conditions problem in dynamic, nonlinear panel data models with unobserved heterogeneity. J Appl Economet 20(1):39–54
Xue X, Cheng M, Zhang W (2021) Does education really improve health? A meta-analysis. J Econ Surv 35(1):71–105
Download references
Acknowledgements
The author acknowledges financial support from the Cariverona Foundation Ph.D. research scholarship. He is particularly grateful to Matteo Picchio and Claudia Pigini for their comments and suggestions on a preliminary version of this paper. He also thanks the Associate Editor Massimiliano Bratti and two anonymous reviewers, whose comments were very useful for an important improvement of the paper.
Author information
Authors and affiliations.
Department of Economics and Social Sciences, Marche Polytechnic University, Piazzale Martelli 8, 60121, Ancona, Italy
Mattia Filomena
Department of Public Economics, Masaryk University, Lipová 41a, 60200, Brno, Czech Republic
You can also search for this author in PubMed Google Scholar
Corresponding author
Correspondence to Mattia Filomena .
Ethics declarations
Conflict of interest.
The author has no competing interests to declare that are relevant to the content of this article.
Additional information
Publisher's note.
Springer Nature remains neutral with regard to jurisdictional claims in published maps and institutional affiliations.
See Tables 5 and 6 .
Rights and permissions
Springer Nature or its licensor (e.g. a society or other partner) holds exclusive rights to this article under a publishing agreement with the author(s) or other rightsholder(s); author self-archiving of the accepted manuscript version of this article is solely governed by the terms of such publishing agreement and applicable law.
Reprints and permissions
About this article
Filomena, M. Unemployment Scarring Effects: An Overview and Meta-analysis of Empirical Studies. Ital Econ J (2023). https://doi.org/10.1007/s40797-023-00228-4
Download citation
Received : 09 April 2022
Accepted : 15 April 2023
Published : 17 May 2023
DOI : https://doi.org/10.1007/s40797-023-00228-4
Share this article
Anyone you share the following link with will be able to read this content:
Sorry, a shareable link is not currently available for this article.
Provided by the Springer Nature SharedIt content-sharing initiative
- Unemployment scarring effects
- State dependence
- Earning penalties
- Causal effect
- Meta-analysis
JEL Classification
- Find a journal
- Publish with us
- Track your research

An official website of the United States government
The .gov means it’s official. Federal government websites often end in .gov or .mil. Before sharing sensitive information, make sure you’re on a federal government site.
The site is secure. The https:// ensures that you are connecting to the official website and that any information you provide is encrypted and transmitted securely.
- Publications
- Account settings
Preview improvements coming to the PMC website in October 2024. Learn More or Try it out now .
- Advanced Search
- Journal List
- Elsevier - PMC COVID-19 Collection

Unemployment in the time of COVID-19: A research agenda ☆
David l. blustein.
a Boston College, United States of America
b University of Florida, United States of America
Joaquim A. Ferreira
c University of Coimbra, Portugal
Valerie Cohen-Scali
d Conservatoire National des Arts et Métiers, France
Rachel Gali Cinamon
e University of Tel Aviv, Israel
Blake A. Allan
f Purdue University, United States of America
This essay represents the collective vision of a group of scholars in vocational psychology who have sought to develop a research agenda in response to the massive global unemployment crisis that has been evoked by the COVID-19 pandemic. The research agenda includes exploring how this unemployment crisis may differ from previous unemployment periods; examining the nature of the grief evoked by the parallel loss of work and loss of life; recognizing and addressing the privilege of scholars; examining the inequality that underlies the disproportionate impact of the crisis on poor and working class communities; developing a framework for evidence-based interventions for unemployed individuals; and examining the work-family interface and unemployment among youth.
This essay reflects the collective input from members of a community of vocational psychologists who share an interest in psychology of working theory and related social-justice oriented perspectives ( Blustein, 2019 ; Duffy, Blustein, Diemer, & Autin, 2016 ). Each author of this article has contributed a specific set of ideas, which individually and collectively reflect some promising directions for research about the rampant unemployment that sadly defines this COVID-19 crisis.
Our efforts cohere along several assumptions and values. First, we share a view that unemployment has devastating effects on the psychological, economic, and social well-being of individuals and communities ( Blustein, 2019 ). Second, we seek to build on the exemplary research on unemployment that has documented its impact on mental health ( Paul & Moser, 2009 ; Wanberg, 2012 ) and its equally pernicious impact on communities ( International Labor Organization, 2020b ). Third, we hope that this contribution charts a research agenda that will inform practice at individual and systemic levels to support and sustain people as they grapple with the daunting challenge of seeking work and recovering from the psychological and vocational fallout of this pandemic.
The advent of this period of global unemployment is connected causally and temporally to considerable loss of life and illness, which is creating an intense level of grief and trauma for many people. The first step in developing a research agenda for unemployment during the COVID-19 era is to describe the nature of this process of loss in so many critical sectors of life. A major research question, therefore, is to what extent does this unemployment crisis vary from previous bouts of unemployment which were linked to economic fluctuations? In addition, exploring the role of loss and trauma during this crisis should yield research findings that can inform psychological and vocational interventions as well as policy guidance to support people via civic institutions and communities.
1. Recognizing and channeling our own privilege
In Joe Pinker's (2020) Atlantic essay entitled, “ The Pandemic Will Cleave America in Two”, he highlights two distinct experiences of the pandemic. One is an experience felt by those with high levels of education in stable jobs where telework is possible. Lives are now more stressful, work has been turned upside down, childcare is challenging, and leaving the house feels ominous. The other is an experience felt by the rest of the working public – those who cannot work from home and thus are putting themselves at risk every day, whose jobs have been either lost or downsized, and who are wondering not only if they will catch the virus but whether they have the means and resources to survive. As psychologists and professors, the vast majority of “us” (those writing this essay and those reading it) are extremely fortunate to be in the first group. The pandemic has only served to exacerbate the extent of this privilege.
Given our relative position of power, what are ways we can change our research to be more meaningful and impactful to those outside of our bubble? We propose that the recent work on radical healing in communities of color – where the research is often done in collaboration with the participants and building participant agency is an explicit goal - can inform our path forward ( French et al., 2020 ; Mosley et al., 2020 ). Work has always been a domain where individuals experience distress and marginalization. However, in the current pandemic and into the unforeseeable future, this will only exponentially increase. Sure, we can do surveys about people's experiences and provide incentives for their time. And of course qualitative work will allow us to more directly connect with participants and hear their voices. But what is most needed is research where participants receive tangible benefits to improve their work lives. We, as privileged scholars, need to think about how we can use our expertise in studying work to infuse our studies with real world benefits. We see this as occurring on a spectrum in terms of scholars' time and resources available – from information sharing about resources to providing job-seeking or work-related interventions. In our view, now is the time to truly commit to using work-related research not just as a way to build scholarly knowledge, but as a way to improve lives.
2. Inequality and unemployment
Focusing research efforts on real-world benefits means acknowledging how the COVID-19 pandemic has exposed and exacerbated existing inequities in the labor market. Millions of workers in the U.S. have precarious jobs that are uncertain in the continuity and amount of work, do not pay a living wage, do not give workers power to advocate for their needs, or do not provide access to basic benefits ( Kalleberg, 2009 ). Power and privilege are major determinants of who is at risk for precarious work, with historically marginalized communities being disproportionately vulnerable to these job conditions ( International Labor Organization, 2020a ). In turn, people with precarious work experience chronic stress and uncertainty, putting them at risk for mental health, physical, and relational problems ( Blustein, 2019 ). These risk factors may further worsen the effects of the COVID-19 crisis while simultaneously exposing inequities that existed before the crises.
The COVID-19 pandemic is an opportunity for researchers to define and describe how precarious work creates physical, relational, behavioral, psychological, economic, and emotional vulnerabilities that worsen outcomes from crises like the COVID-19 pandemic (e.g., unemployment, psychological distress). For example, longitudinal studies can examine how precarious work creates vulnerabilities in different domains, which in turn predict outcomes of the COVID-19 pandemic, including unemployment and mental health. This may include larger scale cohort studies that examine how the COVID-19 crisis has created a generation of precarity among people undergoing the school-to-work transition. Researchers can also study how governmental and nonprofit interventions reduce vulnerability and buffer the relations between precarious work and various outcomes. For example, direct cash assistance is becoming increasingly popular as an efficient way to help people in poverty ( Evans & Popova, 2014 ). However, dominant social narratives (e.g., the myth of meritocracy, the American dream) blame people with poor quality work for their situations. Psychologists have a critical role in (a) documenting false social narratives, (b) studying interventions to provide accurate counter narratives (e.g., people who receive direct cash assistance do not spend money on alcohol or drugs; most people who need assistance are working; Evans & Popova, 2014 ), and (c) studying how to effectively change attitudes among the public to create support for effective interventions.
3. Work-family interface
Investigating the work-family interface during unemployment may appear contradictory. It can be argued that because there is no paid work, the work-family interface does not exist. But ‘work’ is an integral part of people's lives, even during unemployment; for example, working to find a job is a daunting task that is usually done from home. Thus, the work-family interface also exists during unemployment, but our knowledge about this is limited. Our current knowledge on the work-family interface primarily focuses on people who work full-time and usually among working parents with young children ( Cinamon, 2018 ). As such, focusing on the work-family interface during periods of unemployment represents a needed research agenda that can inform public policy and scholarship in work-family relationships.
The rise in unemployment due to COVID-19 relates not only to the unemployed, but also to other family members. Important research questions to consider are how are positive and negative feelings and thoughts about the absence of work conveyed and co-constructed by family members? What family behaviors and dynamics promote and serve as social capital for the unemployed and for the other members of the family? Do job search behaviors serve as a form of modeling for other family members? What are the experiences of unemployed spouses and children, and how do these experiences shape their own career development? These issues can be discerned among unemployed people of different ages, communities, and cultures.
Several research methods can promote this agenda. Participatory action research can enable vocational researchers to be proactive and involved in increasing social solidarity. This approach requires mutual collaboration between the researcher and families wherein one of the parents is unemployed. By giving them voice to describe their experiences, thoughts, ideas, and suggested solutions, we affirm inclusion of the individuals living through the new reality, thereby conveying respect and acknowledgment. At the same time, we can bring ideas, knowledge, and social connections to the families that can serve as social capital. In addition, longitudinal quantitative studies among unemployed families that explore some of the issues noted above would be important as a means of exploring how the new unemployment experience is shaping both work and relationships. We also advocate that meaningful incentives be offered to participants in all of these studies, such as online job search workshops and career education interventions for adolescents.
4. Strategies for dealing with unemployment in the pandemic of 2020
Forward-looking governments and organizations (such as universities) should begin thinking about how to deal with the immediate and long-term consequences of the economic crisis created by COVID-19, especially in the area of unemployment. Creating meaningful interventions to assist the newly unemployed will be difficult because of the unprecedented number of individuals and families that are affected and because of the diverse contextual and personal factors that characterize this new population. Because of this diversity of contextual and personal factors, different interventions will be required for different patterns of individual/contextual characteristics ( Ferreira et al., 2015 ).
In broad outline, a research program to address the diversity of issues identified above could be envisioned to consist of several distinct phases: First, it would be necessary to carefully assess the external circumstances of the unemployed individual's job loss, including the probability of re-employment, financial condition, family composition, and living conditions, among others. Second, an assessment should be made of the individual's strengths and growth edges, particularly as they impact the current situation. These assessments could be performed via paper or online questionnaire. Based on these initial assessments, the third phase would involve using statistical analyses such as cluster analysis to form distinct groups of unemployed individuals, perhaps based in part on the probability of re-employment following the pandemic. The fourth phase would focus on determining the types (and/or combinations) of intervention most appropriate for each group (e.g., temporary government assistance; emotional support counseling; retraining for better future job prospects; relocation, etc.). Because access to specific types of assistance is frequently a serious challenge, especially for underprivileged individuals, the fifth phase should emphasize facilitating individuals' access to the specific assistance they need. Finally, the sixth phase of research should evaluate the efficacy of this approach, although designing such a large research program in a crisis situation requires ongoing process evaluation throughout the design and implementation stages of the research program.
5. Unemployment among youth
As reflected in a recent International Labor Organization (2020a) report on the impact of the COVID-19 crisis, youth were already vulnerable within the workforce prior to the crisis; the recent advent of massive job losses and growing precarity of work is having particularly painful impacts on young people across the globe. The COVID-19 economic crisis with vast increases in unemployment (and competition between workers) and the probable growth of digitalization may result in a major dislocation of young workers from the labor market for some time ( International Labor Organization, 2020b ). To provide knowledge to meet this daunting challenge, researchers should develop an agenda focusing on two major components—the first is a participatory mode of understanding the experience of youth and the second is the development of evidence-based interventions that are derived from this research process.
The data gathering aspect of this research agenda optimally should focus on understanding unemployed youths' perception of their situation (opportunities, barriers, fears, and intentions) and of the new labor market. We propose that research is needed to unpack how youth are constructing this new reality, their relationship to society, to others, and to the world. This crisis may have changed their priorities, the meaning of work, and their lifestyle. For example, this crisis may have led to an awareness of the necessity of developing more environmentally responsible behaviors ( Cohen-Scali et al., 2018 ). These new life styles could result in skills development and increased autonomy and adaptability among young people. In addition, the focus on understanding youths' experience, which can encompass qualitative and quantitative methods, should also include explorations of shifts in youths' sense of identity and purpose, which may be dramatically affected by the crisis. The young people who are without work should be involved at each step of the research process in order to improve their capacities, knowledge, and agency and to ensure that the research is designed from their lived experiences.
Building on these research efforts, interventions may be designed that include individual counseling strategies as well as systemic interventions based on analyses of the communities in which young people are involved (for example, families and couples and not only individuals). In addition, we need more research to learn about the process of collective empowerment and critical consciousness development, which can inform youths' advocacy efforts and serve as a buffer in their career development ( Blustein, 2019 ).
6. Conclusion
The research ideas presented in this contribution have been offered as a means of stimulating needed scholarship, program development, and advocacy efforts. Naturally, these ideas are not intended to be exhaustive. We hope that readers will find ideas and perspectives in our essay that may stimulate a broad-based research agenda for our field, optimally informing transformative interventions and needed policy interventions for individuals and communities suffering from the loss of work (and loss of loved ones in this pandemic). A common thread in our essay is the recommendation that research efforts be constructed from the lived experiences of the individuals who are now out of work. As we have noted here, their experiences may not be similar to other periods of extensive unemployment, which argues strongly for experience-near, participatory research. We are also advocating for the use of rigorous quantitative methods to develop new understanding of the nature of unemployment during this period and to develop and assess interventions. In addition, we would like to advocate that the collective scholarly efforts of our community include incentives and outcomes that support unemployed individuals. For example, online workshops and resources can be shared with participants and other communities as a way of not just dignifying their participation, but of also providing tangible support during a crisis.
In closing, we are humbled by the stories that we hear from our communities about the job loss of this pandemic period. Our authorship team shares a deep commitment to research that matters; in this context, we believe that our work now matters more than we can imagine.
☆ The order of authorship for authors two through six was determined randomly; each of these authors contributed equally to this paper.
- Blustein D.L. Oxford University Press; NY: 2019. The importance of work in an age of uncertainty: The eroding work experience in America. [ Google Scholar ]
- Cinamon R.G. Paper presented at the Society of Vocational Psychology; Arizona: 2018, June. Life span and time perspective of the work-family interface – Critical transitions and suggested interventions. [ Google Scholar ]
- Cohen-Scali V., Pouyaud J., Podgorny M., Drabik-Podgorna V., Aisenson G., Bernaud J.L., Moumoula I.A., Guichard J., editors. Interventions in career design and education. Springer; Cham, Switzerland: 2018. [ Google Scholar ]
- Duffy R.D., Blustein D.L., Diemer M.A., Autin K.L. The psychology of working theory. Journal of Counseling Psychology. 2016; 63 (2):127–148. [ PubMed ] [ Google Scholar ]
- Evans D.K., Popova A. Cash transfers and temptation goods: A review of global evidence. The World Bank. 2014 doi: 10.1596/1813-9450-6886. [ CrossRef ] [ Google Scholar ]
- Ferreira J.A., Reitzle M., Lee B., Freitas R.A., Santos E.R., Alcoforado L., Vondracek F.W. Configurations of unemployment, reemployment, and psychological well-being: A longitudinal study of unemployed individuals in Portugal. Journal of Vocational Behavior. 2015; 91 :54–64. doi: 10.1016/j.jvb.2015.09.004. [ CrossRef ] [ Google Scholar ]
- French B.H., Lewis J.A., Mosley D.V., Adames H.Y., Chavez-Dueñas N.Y., Chen G.A., Neville H.A. Toward a psychological framework of radical healing in communities of color. The Counseling Psychologist. 2020; 48 :14–46. doi: 10.1177/0011000019843506. [ CrossRef ] [ Google Scholar ]
- International Labor Organization World Employment and Social Outlook – Trends. 2020:2020. https://www.ilo.org/global/research/global-reports/weso/2020/lang--en/index.htm [ Google Scholar ]
- International Labor Organization. (2020b). Young workers will be hit hard by COVID-19's economic fallout. https://iloblog.org/2020/04/15/young-workers-will-be-hit-hard-by-covid-19s-economic-fallout/ .
- Kalleberg A.L. Precarious work, insecure workers: Employment relations in transition. American Sociological Review. 2009; 74 (1):1–22. doi: 10.1177/000312240907400101. [ CrossRef ] [ Google Scholar ]
- Mosley D.V., Hargons C.N., Meiller C., Angyal B., Wheeler P., Davis C., Stevens-Watkins D. Critical consciousness of anti-Black racism: A practical model to prevent and resist racial trauma. Journal of Counseling Psychology. 2020 doi: 10.1037/cou0000430. (Advance online publication) [ PMC free article ] [ PubMed ] [ CrossRef ] [ Google Scholar ]
- Paul K.I., Moser K. Unemployment impairs mental health: Meta-analyses. Journal of Vocational Behavior. 2009; 74 :264–282. [ Google Scholar ]
- Pinker J. The Atlantic; 2020. The pandemic will cleave America in two. [ Google Scholar ]
- Wanberg C.R. The individual experience of unemployment. Annual Review of Psychology. 2012; 63 :369–396. [ PubMed ] [ Google Scholar ]
The Pandemic's Impact on Unemployment and Labor Force Participation Trends
Following early 2020 responses to the pandemic, labor force participation declined dramatically and has remained below its 2019 level, whereas the unemployment rate recovered briskly. We estimate the trend of labor force participation and unemployment and find a substantial impact of the pandemic on estimates of trend. It turns out that levels of labor force participation and unemployment in 2021 were approaching their estimated trends. A return to 2019 levels would then represent a tight labor market, especially relative to long-run demographic trends that suggest further declines in the participation rate.
At the end of 2019, the labor market was hotter than it had been in years. Unemployment was at a historic low, and participation in the labor market was finally increasing after a prolonged decline. That tight labor market came to an abrupt halt with the COVID-19 pandemic in the spring of 2020.
Now, two years later, the labor market has mostly recovered from the depths of the pandemic recession. The unemployment rate is close to pre-pandemic lows, and job openings are at record highs. Yet, participation and employment rates have remained persistently below pre-pandemic levels. This suggests the possibility that the pandemic has permanently reduced participation in the economy and that current participation rates reflect a new normal. In this article, we explore how the pandemic has affected labor markets and whether a new normal is emerging.
What Is "Normal"?
One way to define the normal level of a variable is to estimate its trend and compare the observed data with the estimated trend values. Constructing a trend essentially means drawing a smooth line through the variations in the actual data.
But this means that constructing the trend for a point in time typically involves considering what happened both before and after that point in time. Thus, constructing the trend at the end of a sample is especially hard, since we do not yet know how the data will evolve.
We construct trends for three aggregate labor market ratios — the labor force participation (LFP) rate, the unemployment rate and the employment-population ratio (EPOP) — using methods described in our 2019 article " Projecting Unemployment and Demographic Trends ."
First, we estimate statistical models for LFP and unemployment rates of demographic groups defined by age, gender and education. For each gender and education, we decompose its unemployment and LFP into cyclical components common to all age groups and smooth local trends for age and cohort effects.
Second, we aggregate trends from the estimates of the group-specific trends. Specifically, we construct the trend for the aggregate LFP rate as the population-share-weighted sum of the corresponding estimated trends for demographic groups. We construct the aggregate unemployment rate and EPOP trends from the group-specific LFP and unemployment trends and the groups' population shares.
In our previous work, we estimated the trends for the unemployment rate and LFP rate of a gender-education group separately using maximum likelihood methods. The estimates reported in this article are based on the joint estimation of LFP and unemployment rate trends using Bayesian methods.
We separately estimate the trends using data from 1976 to 2019 (pre-pandemic) and from 1976 to 2021 (including the pandemic period). Figures 1, 2 and 3 display annual averages for the three aggregate labor market ratios — the LFP rate, the unemployment rate and EPOP, respectively — from 1976 to 2021.
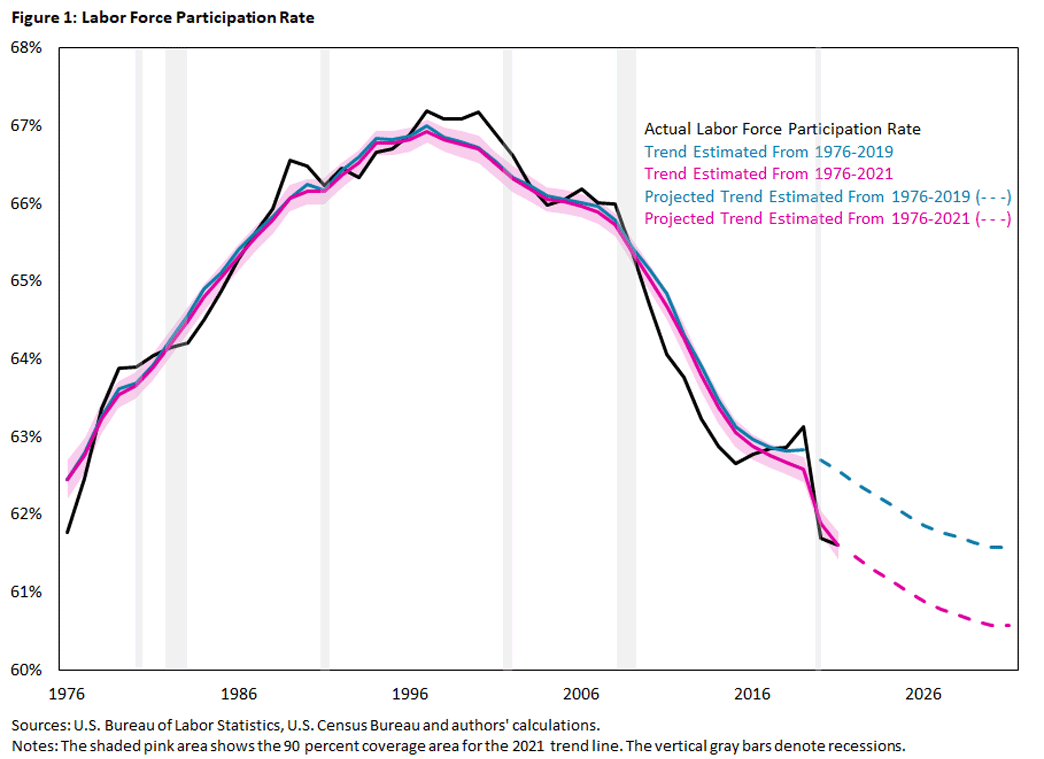
In each figure, the solid black line denotes the observed values, and the blue and pink lines denote the estimated trend using data from 1976 up to and including 2019 and 2021, respectively. The estimated trends are subject to uncertainty, and the plotted trends represent the median estimate of the trend.
For the estimates based on data up to 2021, we also include the 90 percent coverage area shown as the shaded pink area. According to the statistical model, there is a 90 percent probability that the trend is contained in the coverage area. The blue and pink dotted lines represent our projections on how the labor market ratios will evolve until 2031, again based on the estimated trend up to and including 2019 and 2021. The shaded gray vertical areas highlight recessions as defined by the National Bureau of Economic Research (NBER).
Pre-Pandemic Trends: 1976-2019
We start with the pre-pandemic trends for the LFP rate and unemployment rate estimated for data from 1976 through 2019. After a long recovery from the 2007-09 recession, the LFP rate was 63.1 percent in 2019 (slightly above the estimated trend value of 62.8 percent), and the unemployment rate was 3.7 percent (noticeably below its estimated trend value of 4.7 percent).
The LFP rate being above trend and the unemployment rate being below trend reflects the characterization of the 2019 labor market as "hot." But note that even though the LFP rate exceeded its trend value in 2019, it was still lower than during the 2007-09 period. This difference is accounted for by the declining trend in the LFP rate.
As noted in our 2019 article , LFP rates and unemployment rates differ systematically across demographic groups. Participation rates tend to be higher for younger, more-educated workers and for men. Unemployment rates tend to be lower for men and for the older and more-educated population.
Thus, changes in the population composition over time — that is, the relative size of demographic groups — will affect the aggregate LFP and unemployment rates, in addition to changes in the LFP and unemployment rate trends of the demographic groups.
As also noted in our 2019 article, the hump-shaped trend of the aggregate LFP rate reflects a variety of forces:
- Prior to 1990, the aggregate LFP rate was boosted by an upward trend in the LFP rate of women. But after 1990, the LFP rate of women began declining. Combining this with declining trend LFP rates for other demographic groups has reduced the aggregate LFP rate.
- Changes in the age distribution had a limited impact prior to 2005, but the aging population since then has lowered the aggregate LFP rate substantially.
- Increasing educational attainment has contributed positively to aggregate LFP throughout the period.
The steady decline of the unemployment rate trend reflects mostly the contributions from an older and more-educated population and, to some extent, a decline in the trend unemployment rates of demographic groups.
Pre-Pandemic Expectations of Future LFP and Unemployment Trends
Our statistical model of smooth local trends for the LFP and unemployment rates of demographic groups has the property that the best forecast for future trend values of demographic groups is their last estimated trend value. Thus, the model will only predict a change in the trend of aggregate ratios if the population shares of its constituent groups are changing.
We combine the U.S. Census Bureau population forecasts for the gender-age groups with an estimated statistical model of education shares for gender-age groups to forecast population shares of our demographic groups from 2020 to 2031 (the dotted blue lines in Figures 1 and 2).
As we can see, the changing demographics alone imply further reductions of 1 percentage point and 0.2 percentage points in the trend LFP rate and unemployment rate, respectively. This projection is driven by the forecasted aging of the population, which is only partially offset by the forecasted higher educational attainment.
Based on data up to 2019, the same aggregate LFP rates in 2021 as in 2019 would have represented a substantial cyclical deviation upward from the pre-pandemic trends.
It is notable that the unemployment rate is much more volatile relative to its trend than the LFP rate is. In other words, cyclical deviations from trend are much more pronounced for the unemployment rate than for the LFP rate.
In fact, in our estimation, the behavior of the unemployment rate determines the common cyclical component of both the unemployment rate and the LFP rate. Whereas the unemployment rate spikes in recessions, the LFP rate response is more muted and tends to lag recessions. This feature will be important for interpreting how the estimated trend LFP rate changed with the pandemic.
Finally, Figure 3 combines the information from the LFP rate and unemployment rate and plots actual and trend rates for EPOP. On the one hand, given the relatively small trend decline of the unemployment rate, the trend for EPOP mainly reflects the trend for the LFP rate and inherits its hump-shaped path and the projected decline over the next 10 years. On the other hand, EPOP inherits the volatility from the unemployment rate. In 2019, EPOP is notably above trend, by about 1 percentage point.
Unemployment and Labor Force Participation During the Pandemic
The behavior of unemployment resulting from the pandemic-induced recession was different from past recessions:
- The entire increase in unemployment between February and April 2020 was accounted for by the increase in unemployment from temporary layoffs. This differed from previous recessions, when a spike in permanent layoffs led the bulge of unemployment in the trough.
- The recovery started in May 2020, and the speed of recovery was also much faster than in previous recessions. After only seven months, unemployment declined by 8 percentage points.
- The behavior of the unemployment rate is reflected in the 2020 recession being the shortest NBER recession on record: It lasted for two months (March to April 2020).
To summarize, the runup and decline of the unemployment rate during the pandemic were unusually rapid, but the qualitative features were not that different from previous recessions after properly accounting for temporary layoffs, as noted in the 2020 working paper " The Unemployed With Jobs and Without Jobs . "
The decline in the LFP rate was sharp and persistent. The LFP rate dropped from 63.4 percent in February 2020 to 60.2 percent in April 2020, an unprecedented drop during such a short period of time. After a rebound to 61.7 percent in August 2020, the LFP rate essentially moved sideways and remained below 62 percent until the end of 2021.
The large drop in the aggregate LFP rate has been attributed to, among others:
- More people — especially women — leaving the labor force to care for children because of school closings or to care for relatives at increased health risk, as noted in the 2021 work " Assessing Five Statements About the Economic Impact of COVID-19 on Women (PDF) " and the 2021 article " Caregiving for Children and Parental Labor Force Participation During the Pandemic "
- An increase in retirement due to health concerns, as noted in the 2021 working paper " How Has COVID-19 Affected the Labor Force Participation of Older Workers? "
- Generous pandemic income transfers and unemployment insurance programs, as noted in the 2021 article " COVID Transfers Dampening Employment Growth, but Not Necessarily a Bad Thing "
All of these factors might impact the participation trend, but by how much?
The Pandemic's Effect on Trend Estimates for LFP and Unemployment
The aggregate trend assessment for the LFP and unemployment rates has changed considerably as a result of 2020 and 2021. Repeating the estimation of trend and cycle for our demographic groups using data from 1976 up to 2021 yields the pink trend lines in Figures 1 and 2.
The updated trend estimates now put the positive cyclical gap in 2019 for LFP at 0.5 percentage points (rather than 0.3 percentage points) and the negative cyclical gap for the unemployment rate at 1.4 percentage points (rather than 1 percentage point). That is, by this estimate of the trend, the labor market in 2019 was even hotter than by the estimates from the 1976-2019 period.
In 2021, the actual LFP rate is essentially at trend, and the unemployment rate is only slightly above trend. That is, by this estimate of the trend, the labor market is relatively tight.
Notice that even though the new 2021 trend estimates for both the LFP and the unemployment rates differ noticeably from the trend values predicted for 2021 based on data up to 2019, the trend revisions for the LFP rate are limited to more recent years, whereas the trend revisions for the unemployment rate apply to the whole sample.
The difference in revisions is related to how confident we can be about the estimated trends. The 90 percent coverage area is quite narrow for the LFP rate for the entire sample up to the last four years. Thus, there is no need to drastically revise the estimated trend prior to 2017.
On the other hand, the 90 percent coverage area for the trend unemployment rate is quite broad throughout the sample. That is, a wide range of values for trend unemployment is potentially consistent with observed unemployment values. Consequently, the last two observations lead to a wholesale reassessment of the level of the trend unemployment rate.
Another way to frame the 2020-21 trend revisions is as follows. The unemployment rate is very cyclical, deviations from trend are large, and though the sharp increase and decline of the unemployment rate in 2020-21 is unusual, an upward level shift of the trend unemployment rate best reflects the additional pandemic data.
The LFP rate, however, is usually not very cyclical, and it is only weakly related to the unemployment rate. Since the model assumes that the cyclical response does not change over the sample, it then attributes the large 2020-21 drop of the LFP rate to a decline in its trend and ultimately to a decline of the trend LFP rates of most demographic groups.
Finally, the EPOP trend is again mainly determined by the LFP trend, seen in Figure 3. Including the pandemic years noticeably lowers the estimated trend for the years from 2017 onwards. The cyclical gap in 2019 is now estimated to be 1.4 percentage points, and 2021 EPOP is close to its estimated trend.
What Does the Future Hold?
In our framework, current estimates of trend LFP and the unemployment rate for demographic groups are the best forecasts of future rates. Combined with projected demographic changes, this implies a continued noticeable downward trend for the LFP rate and a slight downward trend for the unemployment rate.
The trend unemployment rate is low, independent of how we estimate the trend. But given the highly unusual circumstances of the pandemic, the model may well overstate the decline in the trend LFP rate. Therefore, it is likely that the "true" trend lies somewhere between the trends estimated using data up to 2019 and data up to 2021.
That being a possibility, it remains that labor markets as of now have been unusually tight by most other measures, such as nominal wage growth and posted job openings relative to hires. This suggests that the true trend is closer to the revised 2021 trend than to the 2019 trend. In other words, the LFP rate and unemployment rate at the end of 2021 relative to the 2021 estimate of trend LFP and unemployment rate are consistent with a tight labor market.
Andreas Hornstein is a senior advisor in the Research Department at the Federal Reserve Bank of Richmond. Marianna Kudlyak is a research advisor in the Research Department at the Federal Reserve Bank of San Francisco.
To cite this Economic Brief, please use the following format: Hornstein, Andreas; and Kudlyak, Marianna. (April 2022) "The Pandemic's Impact on Unemployment and Labor Force Participation Trends." Federal Reserve Bank of Richmond Economic Brief , No. 22-12.
This article may be photocopied or reprinted in its entirety. Please credit the authors, source, and the Federal Reserve Bank of Richmond and include the italicized statement below.
V iews expressed in this article are those of the authors and not necessarily those of the Federal Reserve Bank of Richmond or the Federal Reserve System.
Subscribe to Economic Brief
Receive a notification when Economic Brief is posted online.
By submitting this form you agree to the Bank's Terms & Conditions and Privacy Notice.
Thank you for signing up!
As a new subscriber, you will need to confirm your request to receive email notifications from the Richmond Fed. Please click the confirm subscription link in the email to activate your request.
If you do not receive a confirmation email, check your junk or spam folder as the email may have been diverted.
Phone Icon Contact Us
ORIGINAL RESEARCH article
Unemployment, employability and covid19: how the global socioeconomic shock challenged negative perceptions toward the less fortunate in the australian context.
- 1 Research School of Population Health, The Australian National University, Canberra, ACT, Australia
- 2 Institute of Child Protection Studies, The Australian Catholic University, Melbourne, VIC, Australia
- 3 Melbourne Institute of Applied Economic and Social Research, The University of Melbourne, Melbourne, VIC, Australia
Unemployed benefit recipients are stigmatized and generally perceived negatively in terms of their personality characteristics and employability. The COVID19 economic shock led to rapid public policy responses across the globe to lessen the impact of mass unemployment, potentially shifting community perceptions of individuals who are out of work and rely on government income support. We used a repeated cross-sections design to study change in stigma tied to unemployment and benefit receipt in a pre-existing pre-COVID19 sample ( n = 260) and a sample collected during COVID19 pandemic ( n = 670) by using a vignette-based experiment. Participants rated attributes of characters who were described as being employed, working poor, unemployed or receiving unemployment benefits. The results show that compared to employed characters, unemployed characters were rated substantially less favorably at both time points on their employability and personality traits. The difference in perceptions of the employed and unemployed was, however, attenuated during COVID19 with benefit recipients perceived as more employable and more Conscientious than pre-pandemic. These results add to knowledge about the determinants of welfare stigma highlighting the impact of the global economic and health crisis on perception of others.
Introduction
The onset of COVID19 pandemic saw unemployment climb to the highest rate since the Great Depression in many regions globally 1 . Over just one month, from March to April 2020 unemployment rate in the United States increased from 4.4% to over 14.7% and in Australia the effective rate of unemployment increased from 5.4 to 11.7% ( Australian Bureau of Statistics, 2020 ) 2 . In Australia, a number of economic responses were rapidly introduced including a wage subsidy scheme (Jobkeeper) to enable employees to keep their employees connected to the workforce, one-off payments to many welfare recipients, and a doubling of the usual rate of the unemployment benefits (Jobseeker payment) through a new Coronavirus supplement payment. At the time of writing in July 2020, many countries, including Australia remain in the depths of a health and economic crisis.
A rich research literature from a range of disciplines has documented the pervasive negative community views toward those who are unemployed and receiving unemployment benefits, with the extent of this “welfare stigma” being particularly pronounced in countries with highly targeted benefit systems such as the United States and Australia ( Fiske et al., 2002 ; Baumberg, 2012 ; Contini and Richiardi, 2012 ; Schofield and Butterworth, 2015 ). The stigma and potential discrimination associated with unemployment and benefit receipt are known to have negative impacts on health, employability and equality (for meta-analyses, see Shahidi et al., 2016 ). In addition, the receipt of unemployment benefits co-occurs with other stigmatized characteristics such as poverty and unemployment ( Schofield and Butterworth, 2018a ). The changing context related to the COVID19 crisis provides a novel opportunity to better understand the determinants of stigmatizing perceptions of unemployment and benefit receipt.
Negative community attitudes and perceptions of benefit recipients are commonly explained by the concept of “deservingness” ( van Oorschot and Roosma, 2017 ). The unemployed are typically seen as less deserving of government support than other groups because they are more likely to be seen as responsible for their own plight, ungrateful for support, not in genuine need ( Petersen et al., 2011 ; van Oorschot and Roosma, 2017 ), and lacking reciprocity (i.e., seen as taking more than they have given – or will give – back to society; van Oorschot, 2000 ; Larsen, 2008 ; Petersen et al., 2011 ; Aarøe and Petersen, 2014 ). Given the economic shock associated with COVID19, unemployment and reliance on income support are less likely to seen as an outcome within the individuals control and may therefore amplify perceptions of deservingness. Prior work has shown that experimentally manipulating perceived control over circumstances does indeed change negative stereotypes ( Aarøe and Petersen, 2014 ).
A number of experimental paradigms have been used to investigate perceptions of “welfare recipients” and the “unemployed.” The stereotype content model (SCM; Fiske et al., 2002 ), for example, represents the stereotypes of social groups on two dimensions: warmth, relating to being friendly and well–intentioned (rather than ill–intentioned); and competence, relating to one’s capacity to pursue intentions ( Fiske et al., 2002 ). Using this model, the “unemployed” have been evaluated as low in warmth and competence across a variety of welfare regime types ( Fiske et al., 2002 ; Bye et al., 2014 ). The structure of stereotypes has also been studied using the Big Five personality dimensions ( Schofield and Butterworth, 2018b ; Schofield et al., 2019 ): Openness, Conscientiousness, Extraversion, Agreeableness, and Emotional Stability (for background on the Big Five see: Goldberg, 1993 ; Hogan et al., 1996 ; Saucier and Goldberg, 1996 ; McCrae and Terracciano, 2005 ; Srivastava, 2010 ; Chan et al., 2012 ; Löckenhoff et al., 2014 ). There are parallels between the Big Five and the SCM: warmth relating to the dimension of Agreeableness, and competence relating to Conscientiousness ( Digman, 1997 ; Ward et al., 2006 ; Cuddy et al., 2008 ; Abele et al., 2016 ) and these constructs have been found to predict employability and career success ( Barrick et al., 2001 ; Cuesta and Budría, 2017 ). Warmth and agreeableness have also been linked to the welfare-specific characteristics of deservingness ( Aarøe and Petersen, 2014 ).
The term “employability” has been previously defined as a set of achievements, skills and personal attributes that make a person more likely to gain employment and leading to success in their chosen career pathway ( Pegg et al., 2012 ; O’Leary, 2017 , 2019 ). While there are few studies examining perceptions of others, perceptions of one’s own employability have been recently studied in university students, jobseekers ( Atitsogbe et al., 2019 ) and currently employed workers ( Plomp et al., 2019 ; Yeves et al., 2019 ), consistently showing higher levels of perceived employability being linked to personal and job-related wellbeing as well as career success. Examining other’s perceptions of employability may be more relevant to understand factors impacting on actual employment outcomes. A majority of studies examining other’s perceptions of employability have focused on job specific skills study ( Lowden et al., 2011 ; Dhiman, 2012 ; Saad and Majid, 2014 ).
Building on this previous work, our own research has focused on the effects of unemployment by drawing on frameworks of Big Five, SCM and employability in pre-COVID19 samples ( Schofield and Butterworth, 2018b ; Schofield et al., 2019 ). Our studies consistently show that unemployed individuals receiving government payments are perceived as less employable (poorer “quality” workers and less desirable for employment) and less Conscientious. We found similar but weaker pattern related to Agreeableness, Emotional Stability, and the extent that a person is perceived as “uniquely human” ( Schofield et al., 2019 ). Further, we found that vignette characters described as currently employed but with a history of welfare receipt were indistinguishable from those described as employed and with no reference to benefit receipt ( Schofield et al., 2019 ). Findings such as this provide experimental evidence that welfare stigma is malleable and can be challenged by information inconsistent with negative stereotype ( Schofield and Butterworth, 2018b ; Schofield et al., 2019 ; see also Petersen et al., 2011 ).
The broad aim of the current study was to extend this previous work by examining the impact of COVID19 on person perceptions tied to employment and benefit recipient status. It repeats a pre-COVID19 study of an Australian general population sample in the COVID19 context, drawing on the same sampling frame, materials and study design to maximize comparability. The study design recognizes that the negative perceptions of benefit recipients may reflect a combination of difference sources of stigma: poverty, lack of work, and benefit receipt. Therefore, the original study used four different conditions to seek to differentiate these different sources: (1) Employed ; (2) Working poor ; (3) Unemployed ; and (4) Unemployed benefit recipient . Finally, for the COVID19 sample we added a novel fifth condition: (5) Unemployment benefit recipient also receiving the “Coronavirus” supplement . We except that the reference to a payment specifically applicable to the COVID19 context may lead to more favorable perceptions (more deserving) than the other unemployed and benefit receipt characters.
The study capitalizes on a major exogenous event, the COVID19 crisis, which we hypothesize will alter perceptions of deservingness by fundamentally challenging social identities and perceptions of one’s own vulnerability to unemployment. The study tests three hypotheses, and in doing so makes an important empirical and theoretical contribution to understanding how deservingness influences person perception, and understanding of the potential “real world” barriers experienced by people seeking employment in the COVID19 context.
Hypothesis 1
The pre-COVID19 assessment uses a subset of data from a pre-registered study, but this reuse of the data was not preregistered 3 . We hypothesize that, at Time 1 (pre-COVID19 assessment) we will find that employed characters will be rated more favorably than characters described as unemployed and receiving unemployment benefits, particularly on dimensions of Conscientiousness, Worker and Boss suitability. Moreover, we expect a gradient in perceptions across the four experimental conditions, from employed to working poor, to unemployed to unemployed receiving benefits and to show a similar trend for the other outcome measures included in the study.
Hypothesis 2
We hypothesize that the character in the unemployed condition(s) would be rated less negatively relative to the employed condition(s) at Time 2, compared to Time 1. We predict a two-way interaction between time and condition for the key measures (Conscientiousness, Worker and Boss suitability) and a similar trend on other outcomes.
Hypothesis 3
We expect that explicit reference to the unemployed benefit character receiving the “Coronavirus supplement” payment will increase the salience of the COVID19 context and lead to more positive ratings of this character relative to the standard unemployed benefit condition in the pre-COVID19 and COVID19 occasions.
Materials and Methods
Participants.
Two general population samples (pre-COVID19 and COVID19) were recruited from the same source: The Australian Online Research Unit (ORU) panel. The ORU is an online survey platform that provides access to a cohort of members of the general public who are interested in contributing to research. The ORU randomly selects potential participants who meet study eligibility criteria, and provides the participant with an incentive for their participation. The sample for the Time 1 (pre-COVID19) occasion was part of a larger study (768 participants) collected in November 2018. From this initial dataset, we were able to use data from 260 (50.1% female, M Age = 42.1 [16.7] years, range: 18–82) participants who were presented with the one vignette scenario that we could replicate at the time of the social restrictions applicable in the COVID19 context (i.e., the vignette character was not described as going out and visiting friends, as these behaviors were illegal at Time 2). The sample for Time 2 (COVID19) was collected in May–June 2020, at the height of the lock down measures in Australia and included 670 participants (40.5% female, M Age = 51.0 [15.8] years, range: 18–85). The two samples were broadly similar (see below), though the proportion of male participants at Time 2 was greater than at Time 1.
The pre-COVID assessment at Time 1 was restricted to those participants who completed the social-distancing consistent vignette in the first place to avoid potential order/context effects. This provided, on average, 65 respondents in each of the four experimental conditions. Using the results from our previous published studies as indicators of effect size ( Schofield and Butterworth, 2018b ; Schofield et al., 2019 ). Monte Carlo simulation was used to identify the Time 2 sample size that would provide 90% power to detect an interaction effect that represented a 50% decline in the difference between the two employment and two unemployment conditions on the three-key measures at the COVID occasion relative to the pre-COVID difference. This sample size of 135 per condition also provided between 60 and 90% power to detect a difference of a similar magnitude between the employed and unemployment benefit conditions across the two measurement occasions. Given previous evidence that the differences between employed and unemployed/welfare conditions is robust and large for Conscientiousness and Worker suitability ( Schofield and Butterworth, 2018b ), the current study is also adequately powered to detect the most replicable effects of unemployment and welfare on perceptions of a person’s character (even in the absence of the hypothesized interaction effect).
Materials and Procedure
The procedures were identical on both study occasions. Participants read a brief vignette that described a fictional character, and then rated the character on measures reflecting personality dimensions, their suitability as a worker or boss, morality, warmth, and competence, and the participant’s beliefs the character should feel guilt and shame, or feel angry and disgusted. At Time 1 (pre-COVID19 context) participants then repeated this process with a second vignette, but we do not consider data from the second vignette.
Manipulation
The key experimental conditions were operationalized by a single sentence embedded within the vignette that was randomly allocated to different participants (employed: “S/he is currently working as a sales assistant in a large department store”; working poor: “S/he is currently working as a sales assistant, on a minimum-wage, in a large department store”; unemployed: “S/he is currently unemployed”; and receipt of unemployment benefits: “S/he is currently unemployed, and is receiving government benefits due to his/her unemployment”). The four experimental conditions were identical at both time points. At Time 2, an additional COVID19-specific condition was included (to maximize the salience of the COVID19 context): “S/he is currently unemployed and is receiving government benefits, including the Coronavirus supplement, due to his/her unemployment.”
All three study conditions will imply poverty/low income. In Australia, few minimum-wage jobs are supplemented by tips, and so a minimum-wage job indicates a level of relative poverty. A full-time worker in a minimum wage job is in the bottom quartile of income earners ( Australian Bureau of Statistics, 2017 ). Prior to the COVID19 crisis and the increase in payment level, a single person with no dependents receiving unemployment benefits received approximately 75% of the minimum-wage in cash assistance. During COVID19 and at the time of the data collection, the rate of pay exceeds the minimum-wage.
Several characteristics of the vignette character, including age and relationship status, were balanced across study participants. Age was specified as either 27 or 35 years, relationship status was either “single” or “lives with his/her partner.” The character’s gender was also varied and names were stereotypically White.
For Time 1, manipulated characteristics yielded 32 unique vignettes, comprised of four key experimental conditions (employed, working poor, unemployed, and unemployment benefits) × 2 ages × 2 genders × 2 relationship statuses. For Time 2, manipulated characteristics yielded 40 unique vignettes, comprised of five key experimental conditions (employed, working poor, unemployed, unemployment benefits, and unemployed + coronavirus supplement) × 2 ages × 2 genders × 2 relationship statuses. The vignette template construction is presented in Figure 1 including each component of the vignette that was randomly varied.
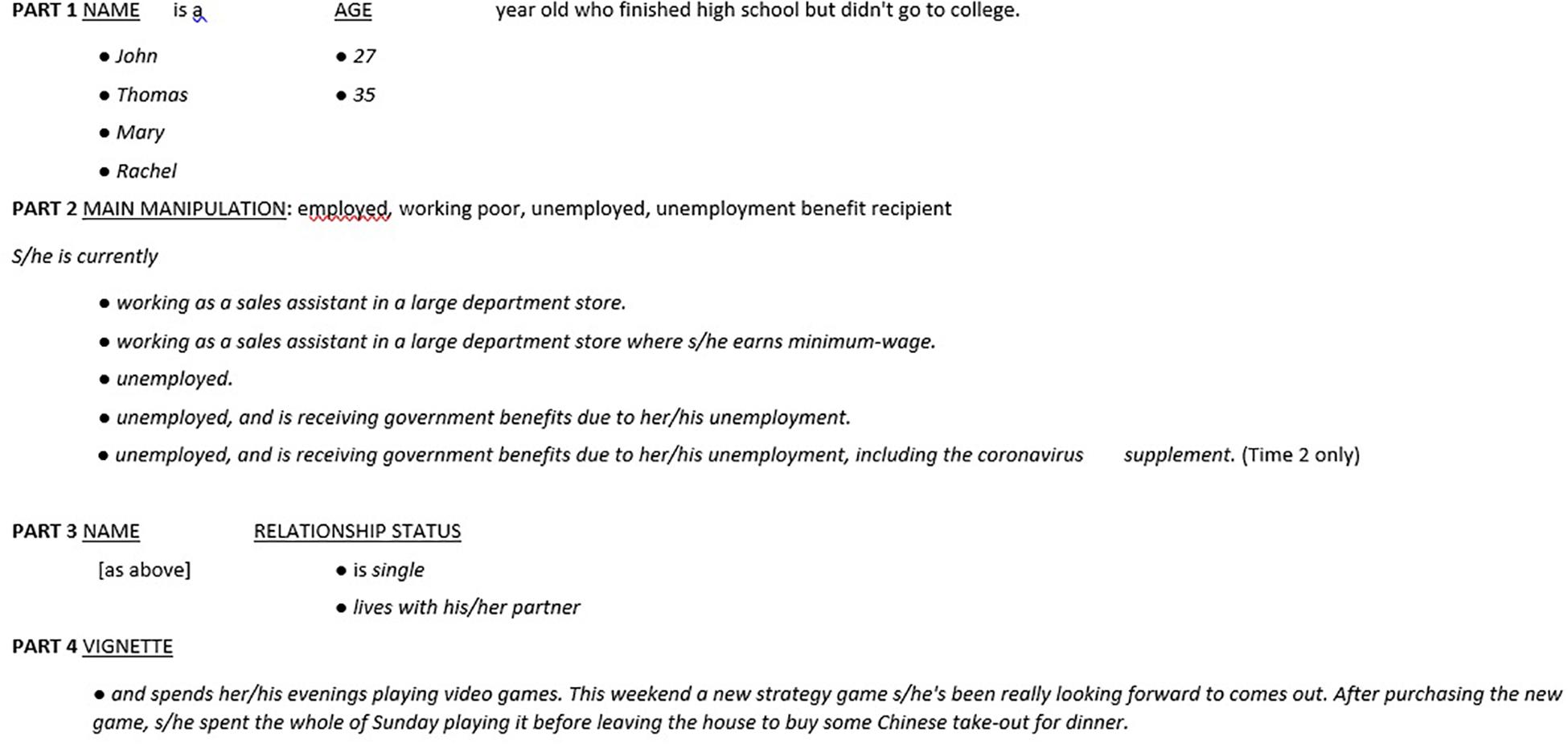
Figure 1. Outline of vignette construction in 4 parts. Bullet pointed options replace the underlined text, with gendered pronouns in each option selected to match character name.
Comprehension Checks
In both studies, participants were required to affirm consent after debriefing or had their data deleted. Participant comprehension of the vignettes was checked via three free-response comprehension questions about the character’s age and weekend activities. Participants who did not answer any questions correctly were not able to continue the study.
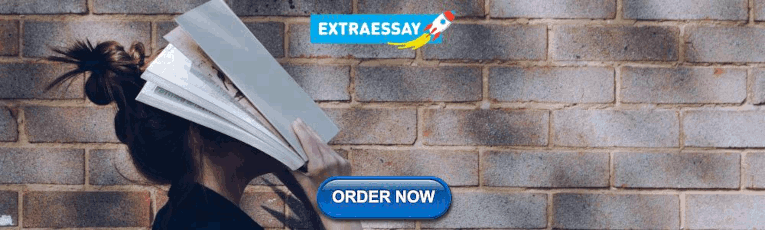
Outcome Measures
Personality, employability (suitability as a worker or boss), communion and agency, cognitive and emotional moral judgments, and dehumanization were included as the study outcomes. While not all personality or character dimension measures can be considered as negative or positive, higher scores were used in the study to indicate more “favorable” perceptions by the participants of the characters.
Personality
The Ten Item Personality Inventory was used to measure the Big Five ( Gosling et al., 2003 ) and adapted to other–oriented wording (i.e., “I felt like the person in the story was…”) ( Schofield et al., 2019 ). Two items measured each trait via two paired attributes. One item contained positive attributes and one contained negative attributes. Participants indicated the extent to which “I think [Name] is [attributes]” from 1 (strongly disagree) to 7 (strongly agree). The order of these 10 items was randomized. Agreeableness (α = 0.54) was assessed from “sympathetic, warm” and “critical, quarrelsome” (reversed); Extraversion (α = 0.50) was assessed from “extraverted, enthusiastic” and “reserved, quiet” (reversed); Conscientiousness (α = 0.76) was assessed from “dependable, self-disciplined” and “disorganized, careless” (reversed); Openness to experience (α = 0.36) was assessed from “open to new experiences, complex” and “conventional, uncreative” (reversed); Emotional stability (α = 0.65) was assessed from “calm, emotionally stable.” and “anxious, easily upset” (reversed). The order of these 10 items was randomized.
Employability
Single item measures: “I think [Name] would be a good worker” ( Worker suitability ) and “I think [Name] would be a good boss” ( Boss suitability ) were rated on the same scale as the personality measure. The order of these two items was randomized. Higher scores indicated better employability.
Communion and Agency
Communion and agency was assessed using Bocian et al. (2018) adaptation of Abele et al. (2016) scale that measures the fundamental dimensions of communion and agency using two-subscales for each dimension. The morality and warmth subscales are seen as measures of communion (referred to as warmth in SCM; Fiske, 2018 ); while the competence and assertiveness subscales measure agency (what Fiske refers to as competence in SCM; Fiske, 2018 ). This subscale structure has been identified in multiple samples. Participants indicated the extent to which “I think [Name] [attributes]” from 1 (not at all) to 5 (very much so). Morality (α = 0.92) was measured with six items, e.g., “is just,” “is fair”; Warmth (α = 0.96) with six items, e.g., “is caring,” “is empathetic”; Competence (α = 0.90) with five items, e.g., “is efficient,” “is capable”; and Assertiveness (α = 0.83) with six items, e.g., “is self-confident,” “stands up well under pressure.” These items were presented in a random order.
Dehumanization
Dehumanization was measured with a composite scale of two-items drawn from Bastian et al. (2013) . Based on prior research, we measured dehumanization with two items: “I think [Name] is mechanical and cold, like a robot” and “I think [Name] lacked self-restraint, like an animal” order of these two items was randomized. We reverse coded the two items for the analyses for consistency for the other variables, so that higher scores were indicative of more favorable perceptions.
Moral Emotions
Moral emotions were measured by four items that asked about emotional responses to the character that were framed as self-condemning or other-condemning ( Haidt, 2003 ; Giner-Sorolla and Espinosa, 2011 ). Two other-condemning items asked the participant about their own emotional response to the character in the vignette (Anger: “[Name]’s behavior makes me angry”; Disgust: “I think [Name] is someone who makes me feel disgusted,” α = 0.92). The two self-condemning items asked about the character’s emotional response (Guilt: “[Name] should feel guilty about [his/her] behavior”; Shame: “I think [Name] should feel ashamed of [him/her]self”; α = 0.95). We reverse coded the two scales to ensure consistency with other variables, with higher scores indicative of more favorable perceptions.
Analytical Strategy
With the exception of the Moral emotion (and Communion and Agency) scales that are new to this study and the previously tested Openness to Experience, our previous research has demonstrated differences between the ratings of employed and unemployed characters on the included outcome measures ( Schofield and Butterworth, 2018b ; Schofield et al., 2019 ). We undertake the analysis using a four-step process. We use mixed-effects multi-level models, with the 14 outcome measures nested within participants, and predicted by fixed (between-person) terms representing the experimental “Condition,” “Time” (pre-/COVID19) and their interaction, and controlling for measure differences and allowing for random effects at the participant level: i) We initially assessed the effect of condition in the pre-COVID19 occasion to establish the baseline pattern of results; ii) we then evaluated the interaction term and, specifically, the extent to which the baseline difference observed between employment and unemployment conditions is attenuated at Time 2 (COVID19 occasion); iii) we tested the three-way interaction between condition, occasion and measure to assess whether this two-way interaction varies across the outcome measures; and if significant iv) repeated the modeling approach using separate linear regression models for each outcome measure. Our initial model contrasts the two employed (employed and working poor) and unemployed (unemployed and benefit receipt) conditions. The second model examines the four separate vignette conditions separately, differentiating between unemployed and unemployed benefit conditions. Finally, we contrast the three unemployment benefit conditions: (1) unemployment benefit recipients at Time 1; (2) unemployment benefit recipients at Time 2; and (3) unemployment benefit recipients receiving the Coronavirus payment at Time 2. For all models, we consider unadjusted and adjusted results (controlling for participant demographics). To address a potential bias from gender differences between samples, post-stratification weights were calculated for the COVID19 sample to reflecting the gender by age distribution of the pre-COVID19 sample. All models were weighted.
The two samples from Time 1 (pre-COVID19) and Time 2 (COVID19) were comparable on all demographic variables, except for gender (χ 2 [1, 923] = 7.04, p < 0.001) and employment (χ 2 [1, 910] = 27.66, p < 0.001): The gender distribution was more balanced at Time 1 with 49.8% of males, compared to 59.5% of males at Time 2. There was also a significant increase in unemployment with 20.9% of Time 1 participants out of work compared to 39.3% of the Time 2 participants. This was likely reflective of the employment rate nearly doubling in Australia during COVID19 crisis. Bivariate correlations showed significant positive correlations between all 14 outcomes ( p ’s < 0.001), except for Extraversion that was only positively correlated with Emotional Stability, boss suitability, warmth, assertiveness, and competence ( p’ s < 0.05).
Contrasting Employed and Unemployed Characters
The results, both adjusted and unadjusted, from the initial overall multilevel model using a binary indicator of whether vignette characters were employed (those in the employed or working poor conditions) or unemployed (unemployed or welfare) and testing the interaction between vignette Condition and Time (pre-COVID19 vs COVID19) are presented in the Supplementary Table S1 . The adjusted results (holding participant age, gender, employment, and education constant) indicated that the unemployed characters were rated lower than the employed characters at Time 1 ( b = −0.57). This difference in the ratings of employed and unemployed characters was reduced in the COVID19 assessment at Time 2, declining from 0.57 to 0.26, across all the outcome measures. The addition of the three-way interaction between Condition, Time and outcome measure significantly improved overall model fit, χ 2 (52) = 482.94, p < 0.001, indicating the interaction between Condition and Time varied over measures.
A series of separate regression models considering each outcome separately (see Supplementary Table S2 ) showed a significant effect of Condition (employment rated higher than unemployment) at Time 1 (pre-COVID) for all outcomes except Openness and Extraversion. The lower ratings for unemployed relative to employed characters were significantly moderated at Time 2 on the Competence, Worker and Boss suitability, and Guilt/Shame outcomes ( p’ s < 0.05).
COVID19 and Perceptions of Unemployment Benefit Recipients
The next set of analyses consider the four separate vignette conditions, differentiating between the unemployed and unemployed benefit recipient conditions. The overall mixed-effects multilevel model incorporating the four distinct vignette conditions provided evidence of significant effects for Condition and Condition by Time in both adjusted and unadjusted models. The result for the adjusted model ( Table 1 ), averaged across the various outcomes, replicated the previous finding of a difference in ratings of employed and unemployed characters at Time 1 (pre-COVID19): relative to the employed condition, there was no difference in ratings of the working poor, but the unemployed and the unemployed benefit recipient characters were rated less favorably. There was some evidence of a gradient across the unemployed characters: the average rating of the unemployed condition was higher than the unemployed benefit condition, though this difference was not statistically significant. In the presence of the interaction effect, the non-significant effect of Time shows that, averaged across all the outcome measures, there was no difference in the rating of the characters in the employed condition on the pre-COVID19 and COVID19 occasions. We tested for the effect of sociodemographic characteristics as covariates in the adjusted models (employment and benefit receipt status, education, age, and gender) but found no main effects of any of the covariates except for gender: females tended to rate characters higher ( b = 0.13, 95% CI [0.04, 0.21]) compared to males. Testing the heterogeneity of these patterns across outcomes via the inclusion of a three-way interaction between vignette condition, occasion and measure significantly improved overall model fit, χ 2 (104) = 533.40, p < 0.001, prompting analysis of each outcome separately.
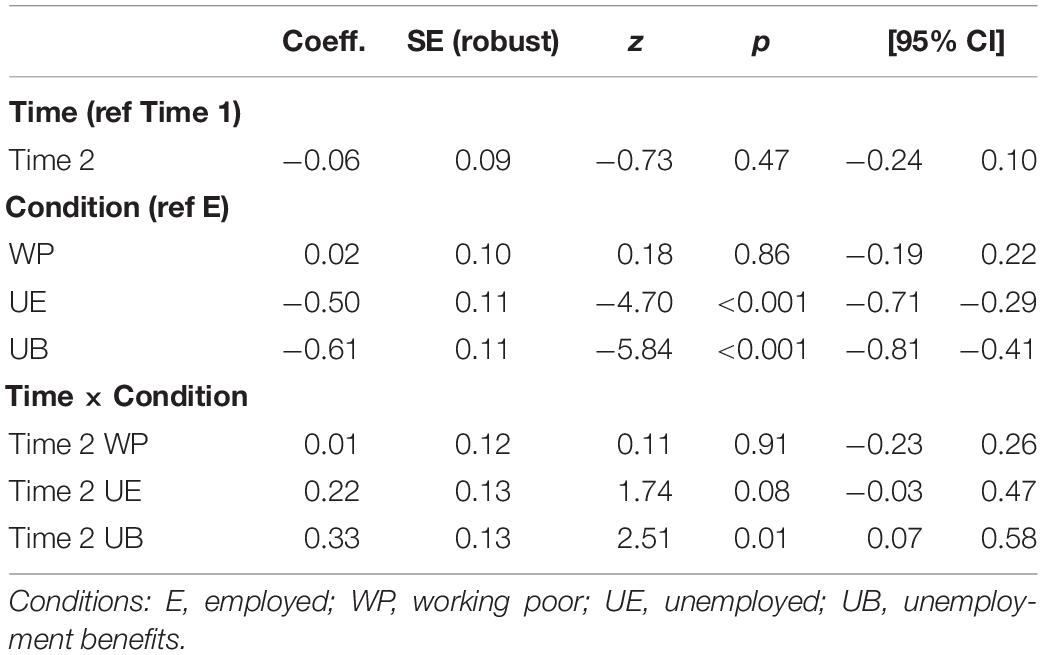
Table 1. Adjusted fixed effects estimates of outcomes as a function of interactions between condition and time.
The separate linear regressions for each outcome measure ( Supplementary Table S3 ) show that ratings of unemployed benefit recipients at the Time 1 (pre-COVID19) were significantly lower than the employed characters for all outcomes except Openness and Extraversion. Statistically significant Condition by Time terms indicated that the unemployed benefit effect was moderated at Time 2 (COVID19) for the three key outcome measures identified in previous research (Conscientiousness, Worker and Boss suitability) and for the measure of Guilt and Shame. Figure 2 depicts this interaction for these four outcomes. These occurred in two profiles. For Conscientiousness, Worker and Boss suitability, COVID19 attenuated the negative perceptions of unemployed relative to employed characters, providing support for Hypothesis 2. By contrast, COVID19 has induced a new difference, such that participants thought employed characters should feel higher levels Guilt and Shame at Time 2, compared to Time 1. While the “working poor” condition was not central to the COVID19 hypotheses, we note that we found no evidence that ratings of these characters on any outcome differed from the standard employed character, or that this difference was changed in assessment at Time 2 (COVID19 occasion).
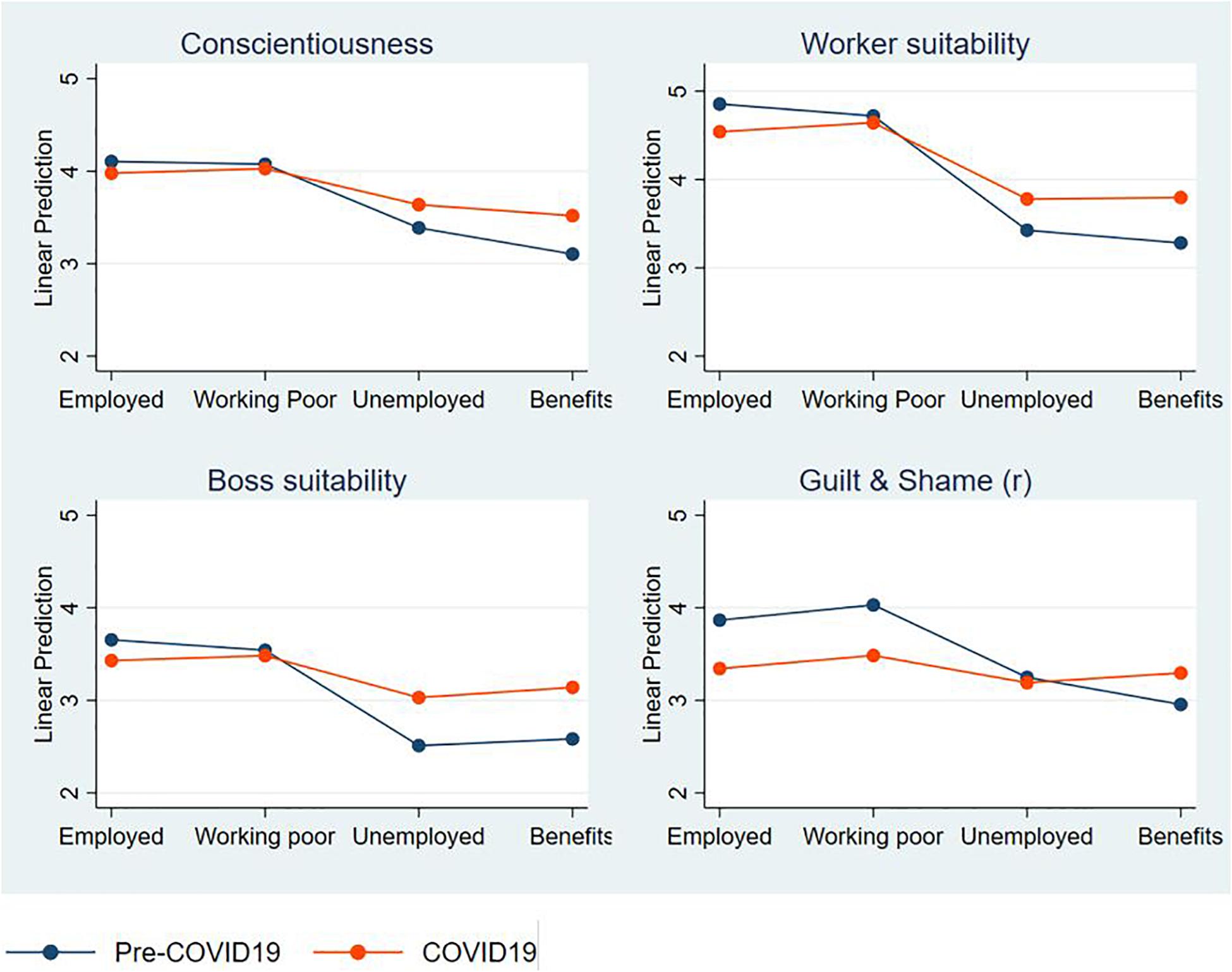
Figure 2. Interaction effect of Time (COVID19) by Condition marginal mean ratings on four outcomes: Conscientiousness, Worker suitability, Boss suitability, and Guilt and Shame (reversed).
The Impact of COVID19 on Perceptions of Unemployment Benefit-Recipients
The inclusion of the fifth COVID19-specific unemployment benefit condition did not generate more positive (or different) ratings than the standard unemployment benefit condition. Overall mixed-effects multilevel models, both adjusted and unadjusted, indicated that participants in the Coronavirus supplement condition (adjusted model: b = 0.26, 95% CI [0.06, 0.45]) and the general unemployed benefit recipient condition at Time 2 (adjusted model: b = 0.28, 95% CI [0.08, 0.48]) were both rated more favorably in comparison to unemployed benefit recipients at Time 1. There was no difference between these two Time 2 benefit recipient groups ( b = 0.03, 95% CI [−0.12, 0.19]). These results did not support hypothesis 3.
Previous research has demonstrated that people who are unemployed, and particularly those receiving unemployment benefits, are perceived more negatively and less employable than those who are employed. However, the economic shock associated with the COVID19 crisis is likely to have challenged people’s sense of their own vulnerability and risk of unemployment, and altered their perceptions of those who are unemployed and receiving government support. The broad aim of the current study was to examine the potential effect of this crisis on person perceptions tied to employment and benefit recipient status. We did this by presenting brief vignettes describing fictional characters, manipulating key experimental conditions related to employment status, and asking study participants to rate the characters’ personality and capability. We contrasted results from two cross-sectional general population samples collected before and during the COVID19 crisis.
The pre-COVID19 assessment replicated our previous findings (e.g., Schofield and Butterworth, 2018b ) showing that employed characters are perceived more favorably than those who were unemployed and receiving government benefits on measures of Conscientiousness and suitability as a worker. These findings supported Hypothesis 1. In comparison, the assessment conducted during the COVID19 crisis showed that unemployed and employed characters were viewed more similarly on these same key measures, with a significant interaction effect providing support for Hypothesis 2. Our third hypothesis, suggesting that n reference to the Coronavirus Supplement (an additional form of income support introduced during the pandemic) would enhance ratings of unemployed benefit recipients at the second assessment occasion, was not supported. We found that benefit recipients at Time 2 were rated more favorably than the benefit group at Time 1, irrespective of whether this COVID19-specific payment was referenced. This suggests the broader context in which the study was conducted was responsible for the change in perceptions.
We sampled participants from the same population, used identical experimental procedures, and found no difference over time in the ratings of employed characters on the key outcome measures of employability (Worker and Boss suitability) and Conscientiousness. The more favorable ratings of unemployed and benefit receiving characters at Time 2 is likely to reflect how the exogenous economic shock brought about by the COVID19 crisis challenged social identities and the stereotypes held of others 4 . The widespread impact and uncontrollable nature of this event are inconsistent with pre-COVID19 views that attribute ill-intent to those receiving to unemployment benefits ( Fiske et al., 2002 ; Baumberg, 2012 ; Contini and Richiardi, 2012 ; Bye et al., 2014 ). We suggest the changing context altered perceptions of the “deservingness” of people who are unemployed as unemployment in the context of COVID19 is less indicative personal failings or a result of one’s “own doing” ( Petersen et al., 2011 ; van Oorschot and Roosma, 2017 ). It is important to recognize, however, that the negative perceptions of unemployed benefit recipients were attenuated in the COVID19 assessment, but they continued to be rated less favorably than those who were employed on the key outcome measures.
In contrast to our findings on the key measures of employability and Conscientiousness, the previous and current research is less conclusive for the other outcome measures. The current study showed a broadly consistent gradient in the perception of employed and unemployed characters for all outcome measures apart from Openness and Extraversion. Findings on these other measures have been weaker and inconsistent across previous studies ( Schofield and Butterworth, 2018b ; Schofield et al., 2019 ), and the current experiment was not designed with sufficient power to demonstrate interaction effects for these measures. There was, however, one measure that showed significant divergence from the expected profile of results. A significant interaction term suggested that study participants at the Time 2 (COVID19) assessment reported that the employed characters should feel greater levels of Guilt and Shame than those who participated in the pre-COVID19 assessment. In contrast, there was consistency in the ratings of unemployed characters on this measure across the two assessment occasions. While not predicted, these results are also interpretable in the context of the pervasive job loss that accompanied the COVID19 crisis. Haller et al. (2020) , for example, argue that the highly distressing, morally difficult, and cumulative nature of COVID19 related stressors presents a perfect storm to result in a guilt and shame responses. The context of mass job losses may leave “surviving” workers feeling increasingly guilty.
The main findings of the current study are consistent with previous experimental studies that show that the stereotypes of unemployed benefit recipients are malleable ( Aarøe, 2011 ; Schofield et al., 2019 ). These previous studies, however, have demonstrated malleability by providing additional information about unemployed individuals that was inconsistent with the unemployed benefit recipient stereotype (e.g., the external causes of their unemployment). In contrast, the current study did not change how the vignette characters were presented or the experimental procedures. Rather, we assessed how the changing context in which study participants were living had altered their perceptions: suggesting the experience of COVID19 altered stereotypical views held by study participants rather than presenting information about the character that would challenge the applicability of the benefit recipient stereotype in this instance.
Perceptions and stereotypes of benefit recipients can be reinforced (and potentially generated) by government actions and policies. Structural stigma can be used as a policy tool to stigmatize benefit receipt as a strategy to reduce dependence on income support and encourage workforce participation ( Moffitt, 1983 ; Stuber and Schlesinger, 2006 ; Baumberg, 2012 ; Contini and Richiardi, 2012 ; Garthwaite, 2013 ). In the current instance, however, the Australian government acted quickly to provide greater support to Australians who lost their jobs (e.g., doubling the rate of payment, removing mandatory reporting to the welfare services) and this may have reduced the stigmatizing structural features of the income support system and contributed to the changed perceptions of benefit recipients identified in this study.
Limitations
The current study took advantage of a natural experimental design and replicated a pre-COVID19 study during the COVID19 crisis. The study is limited by the relatively small sample size at Time 1, which was not designed for current purposes but part of another study. We were not able to include most of the participants from the original Time 1 study as most of the experimental conditions described activities that were illegal/inconsistent with recommend activity at the time of the COVID19 lockdown and social restriction measures. Finally, the data collection for the current study occurred very quickly after the initial and sudden COVID19 lockdowns and economic shock, which is both a strength and a limitation for the generalizability of the results. The pattern of results using the same sampling frame offers compelling support for our hypothesis that the shared economic shock and increase in unemployment attenuates stigmatizing community attitudes toward those who need to receive benefits. Our current conclusions would be further strengthened by a subsequent replication when the public health and economic crises stabilize, to test whether pre-COVID perceptions return.
The current study provides novel information about impact of the COVID19 health and economic crisis, and the impact of the corresponding policy responses on community perceptions. This novel study shows how community perceptions of employment and benefit recipient status have been altered by the COVID19 pandemic. These results add to knowledge about the determinants of welfare stigma, particularly relating to employability, highlighting societal level contextual factors.
Data Availability Statement
The raw data supporting the conclusions of this article will be made available by the authors, without undue reservation.
Ethics Statement
The studies involving human participants were reviewed and approved by Melbourne University Human Research Ethics Committee. The patients/participants provided their written informed consent to participate in this study.
Author Contributions
AS led the review conceptualized by TS and PB. AS and PB conducted the analyses and wrote up the review. TS led the data collection, reviewed and edited the manuscript, and provided data management support. This manuscript is based on previous extensive work by TS and PB on stereotypes toward the unemployed and welfare benefit recipients. All authors contributed to the article and approved the submitted version.
This study was funded by the Australian Research Council (ARC) grant # DP16014178.
Conflict of Interest
The authors declare that the research was conducted in the absence of any commercial or financial relationships that could be construed as a potential conflict of interest.
Supplementary Material
The Supplementary Material for this article can be found online at: https://www.frontiersin.org/articles/10.3389/fpsyg.2020.594837/full#supplementary-material
- ^ https://fred.stlouisfed.org/series/UNRATE
- ^ The Australian figure includes individuals working zero hours who had “no work, not enough work available or were stood down.” The US Bureau of Labor Statistics noted that some people on temporary layoff were not classified as such and the unemployment rate could have been almost 5 percentage points higher.
- ^ https://osf.io/wknb6
- ^ https://pursuit.unimelb.edu.au/articles/our-changing-identities-under-covid-19
Aarøe, L. (2011). Investigating frame strength: the case of episodic and thematic frames. Polit. Commun . 28, 207–226. doi: 10.1080/10584609.2011.568041
CrossRef Full Text | Google Scholar
Aarøe, L., and Petersen, M. B. (2014). Crowding out culture: scandinavians and Americans agree on social welfare in the face of deservingness cues. J. Polit. 76, 684–697. doi: 10.1017/S002238161400019X
Abele, A. E., Hauke, N., Peters, K., Louvet, E., Szymkow, A., and Duan, Y. (2016). Facets of the fundamental content dimensions: agency with competence and assertiveness—Communion with warmth and morality. Front. Psychol. 7:1810. doi: 10.3389/fpsyg.2016.01810
PubMed Abstract | CrossRef Full Text | Google Scholar
Atitsogbe, K. A., Mama, N. P., Sovet, L., Pari, P., and Rossier, J. (2019). Perceived employability and entrepreneurial intentions across university students and job seekers in Togo: the effect of career adaptability and self-efficacy. Front. Psychol. 10:180. doi: 10.3389/fpsyg.2019.00180
Australian Bureau of Statistics (2017). Characteristics of Employment. Australia: Labour Force.
Google Scholar
Australian Bureau of Statistics (2020). Employment and unemployment: International Perspective. Australia: Labour Force.
Barrick, M. R., Mount, M. K., and Judge, T. A. (2001). Personality and performance at the beginning of the new millennium: what do we know and where do we go next? Int. J. Select. Assess. 9, 9–30. doi: 10.1111/1468-2389.00160
Bastian, B., Denson, T. F., and Haslam, N. (2013). The roles of dehumanization and moral outrage in retributive justice. PLoS One 8:e61842. doi: 10.1371/journal.pone.0061842
Baumberg, B. (2012). Three ways to defend welfare in Britain. J. Poverty Soc. Just. 20, 149–161. doi: 10.1332/175982712X652050
Bocian, K., Baryla, W., Kulesza, W. M., Schnall, S., and Wojciszke, B. (2018). The mere liking effect: attitudinal influences on attributions of moral character. J. Exp. Soc. Psychol. 79, 9–20. doi: 10.1016/j.jesp.2018.06.007
Bye, H. H., Herrebrøden, H., Hjetland, G. J., Røyset, G. Ø, and Westby, L. L. (2014). Stereotypes of norwegian social groups. Scand. J. Psychol. 55, 469–476. doi: 10.1111/sjop.12141
Chan, W., McCrae, R. R., De Fruyt, F., Jussim, L., Löckenhoff, C. E., De Bolle, M., et al. (2012). Stereotypes of age differences in personality traits: universal and accurate? J. Personal. Soc. Psychol. 103, 1050–1066. doi: 10.1037/a0029712
Contini, D., and Richiardi, M. G. (2012). Reconsidering the effect of welfare stigma on unemployment. J. Econ. Behav. Organ. 84, 229–244. doi: 10.1016/j.jebo.2012.02.010
Cuddy, A. J., Fiske, S. T., and Glick, P. (2008). Warmth and competence as universal dimensions of social perception: the stereotype content model and the BIAS map. Adv. Exp. Soc. Psychol. 40, 61–149. doi: 10.1016/S0065-2601(07)00002-0
Cuesta, M. B., and Budría, S. (2017). Unemployment persistence: how important are non-cognitive skills? J. Behav. Exp. Econom. 69, 29–37. doi: 10.1016/j.socec.2017.05.006
Dhiman, M. C. (2012). Employers’ perceptions about tourism management employability skills. Anatolia 23, 359–372. doi: 10.1080/13032917.2012.711249
Digman, J. M. (1997). Higher-order factors of the big five. J. Personal. Soc. Psychol. 73:1246.
Fiske, S. T. (2018). Stereotype content: warmth and competence endure. Curr. Direct. Psychol. Sci. 27, 67–73. doi: 10.1177/0963721417738825
Fiske, S. T., Cuddy, A. J., Glick, P., and Xu, J. (2002). A model of (often mixed) stereotype content: competence and warmth respectively follow from perceived status and competition. J. Personal. Soc. Psychol. 82:878. doi: 10.1037/0022-3514.82.6.878
Garthwaite, K. (2013). Fear of the brown envelope: exploring welfare reform with long-term sickness benefits recipients. Soc. Pol. Administration. 48, 782–798. doi: 10.1111/spol.12049
Giner-Sorolla, R., and Espinosa, P. (2011). Social cuing of guilt by anger and of shame by disgust. Psychol. Sci. 22, 49–53. doi: 10.1177/0956797610392925
Goldberg, L. R. (1993). The structure of phenotypic personality traits. Am. Psychol. 48:26. doi: 10.1037/0003-066X.48.1.26
Gosling, S. D., Rentfrow, P. J., and Swann, W. B. Jr. (2003). A very brief measure of the Big-Five personality domains. J. Res. Personal. 37, 504–528. doi: 10.1016/S0092-6566(03)00046-1
Haidt, J. (2003). The moral emotions. Handb. Affect. Sci. 11, 852–870.
Haller, M., Norman, S. B., Davis, B. C., Capone, C., Browne, K., and Allard, C. B. (2020). A model for treating COVID-19–related guilt, shame, and moral injury. Psychol. Trauma 12, S174–S176.
Hogan, R., Hogan, J., and Roberts, B. W. (1996). Personality measurement and employment decisions: questions and answers. Am. Psychol. 51:469. doi: 10.1037/0003-066X.51.5.469
Larsen, K. (2008). Knowledge network hubs and measures of research impact, science structure, and publication output in nanostructured solar cell research. Scientometrics 74, 123–142. doi: 10.1007/s11192-008-0107-2
Löckenhoff, C. E., Chan, W., McCrae, R. R., De Fruyt, F., Jussim, L., De Bolle, M., et al. (2014). Gender stereotypes of personality: universal and accurate? J. Cross-Cult. Psychol. 45, 675–694. doi: 10.1177/0022022113520075
Lowden, K., Hall, S., Elliot, D., and Lewin, J. (2011). Employers’ Perceptions of the Employability Skills of New Graduates. London: Edge Foundation.
McCrae, R. R., and Terracciano, A. (2005). Universal features of personality traits from the observer’s perspective: data from 50 cultures. J. Personal. Soc. Psychol. 88, 547–561. doi: 10.1037/0022-3514.88.3.547
Moffitt, R. (1983). An economic model of welfare stigma. Am. Econom. Rev. 75, 1023–1035.
O’Leary, S. (2017). Graduates’ experiences of, and attitudes towards, the inclusion of employability-related support in undergraduate degree programmes; trends and variations by subject discipline and gender. J. Educ. Work 30, 84–105. doi: 10.1080/13639080.2015.1122181
O’Leary, S. (2019). Gender and management implications from clearer signposting of employability attributes developed across graduate disciplines. Stud. Higher Educ. 1–20. doi: 10.1080/03075079.2019.1640669
Pegg, A., Waldock, J., Hendy-Isaac, S., and Lawton, R. (2012). Pedagogy for Employability. York: Higher Education Academy.
Petersen, M. B., Slothuus, R., Stubager, R., and Togeby, L. (2011). Deservingness versus values in public opinion on welfare: the automaticity of the deservingness heuristic. Eur. J. Political Res. 50, 24–52. doi: 10.1111/j.1475-6765.2010.01923.x
Plomp, J., Tims, M., Khapova, S. N., Jansen, P. G., and Bakker, A. B. (2019). Psychological safety, job crafting, and employability: a comparison between permanent and temporary workers. Front. Psychol. 10:974.
Saad, M. S. M., and Majid, I. A. (2014). Employers’ perceptions of important employability skills required from Malaysian engineering and information and communication technology (ICT) graduates. Global J. Eng. Educ. 16, 110–115.
Saucier, G., and Goldberg, L. R. (1996). “The language of personality: lexical perspectives,” in On the Five-factor Model of Personality: Theoretical Perspectives , ed. J. S. Wiggins (New York, NY: Guilford Press), 21–50.
Schofield, T. P., and Butterworth, P. (2015). Patterns of welfare attitudes in the Australian population. PLoS One 10:0142792. doi: 10.1371/journal.pone.0142792
Schofield, T. P., and Butterworth, P. (2018a). Are negative community attitudes toward welfare recipients associated with unemployment? evidence from an australian cross-sectional sample and longitudinal cohort. Soc. Psychol. Personal. Sci. 9, 503–515. doi: 10.1177/1948550617712031
Schofield, T. P., and Butterworth, P. (2018b). Community attitudes toward people receiving unemployment benefits: does volunteering change perceptions? Basic Appl. Soc. Psychol. 40, 279–292. doi: 10.1080/01973533.2018.1496335
Schofield, T. P., Haslam, N., and Butterworth, P. (2019). The persistence of welfare stigma: does the passing of time and subsequent employment moderate the negative perceptions associated with unemployment benefit receipt? J. Appl. Soc. Psychol. 49, 563–574. doi: 10.1111/jasp.12616
Shahidi, F., Siddiqi, A., and Muntaner, C. (2016). Does social policy moderate the impact of unemployment on health? a multilevel analysis of 23 welfare states. Eur. J. f Public Health 26, 1017–1022. doi: 10.1093/eurpub/ckw050
Srivastava, S. (2010). The five-factor model describes the structure of social perceptions. Psychol. Inquiry 21, 69–75. doi: 10.1080/10478401003648815
Stuber, J., and Schlesinger, M. (2006). Sources of stigma for means-tested government programs. Soc. Sci. Med. 63, 933–945. doi: 10.1016/j.socscimed.2006.01.012
van Oorschot, W. (2000). Who should get what, and why? on deservingness criteria and the conditionality of solidarity among the public. Pol. Pol. 28, 33–48. doi: 10.1332/0305573002500811
van Oorschot, W., and Roosma, F. (2017). The Social Legitimacy of Targeted Welfare. Cheltenham: Edward Elgar Publishing.
Ward, L. C., Thorn, B. E., Clements, K. L., Dixon, K. E., and Sanford, S. D. (2006). Measurement of agency, communion, and emotional vulnerability with the Personal Attributes Questionnaire. J. Pers. Assess . 86, 206–216. doi: 10.1207/s15327752jpa8602_10
Yeves, J., Bargsted, M., Cortes, L., Merino, C., and Cavada, G. (2019). Age and perceived employability as moderators of job insecurity and job satisfaction: a moderated moderation model. Front. Psychol. 10:799. doi: 10.3389/fpsyg.2019.00799
Keywords : COVID19, employability, personality, Big Five, public policy, unemployment
Citation: Suomi A, Schofield TP and Butterworth P (2020) Unemployment, Employability and COVID19: How the Global Socioeconomic Shock Challenged Negative Perceptions Toward the Less Fortunate in the Australian Context. Front. Psychol. 11:594837. doi: 10.3389/fpsyg.2020.594837
Received: 14 August 2020; Accepted: 22 September 2020; Published: 15 October 2020.
Reviewed by:
Copyright © 2020 Suomi, Schofield and Butterworth. This is an open-access article distributed under the terms of the Creative Commons Attribution License (CC BY) . The use, distribution or reproduction in other forums is permitted, provided the original author(s) and the copyright owner(s) are credited and that the original publication in this journal is cited, in accordance with accepted academic practice. No use, distribution or reproduction is permitted which does not comply with these terms.
*Correspondence: Aino Suomi, [email protected]
Disclaimer: All claims expressed in this article are solely those of the authors and do not necessarily represent those of their affiliated organizations, or those of the publisher, the editors and the reviewers. Any product that may be evaluated in this article or claim that may be made by its manufacturer is not guaranteed or endorsed by the publisher.
- Original Article
- Open access
- Published: 08 March 2018
Unemployment among younger and older individuals: does conventional data about unemployment tell us the whole story?
- Hila Axelrad 1 , 2 ,
- Miki Malul 3 &
- Israel Luski 4
Journal for Labour Market Research volume 52 , Article number: 3 ( 2018 ) Cite this article
65k Accesses
30 Citations
14 Altmetric
Metrics details
In this research we show that workers aged 30–44 were significantly more likely than those aged 45–59 to find a job a year after being unemployed. The main contribution is demonstrating empirically that since older workers’ difficulties are related to their age, while for younger individuals the difficulties are more related to the business cycle, policy makers must devise different programs to address unemployment among young and older individuals. The solution to youth unemployment is the creation of more jobs, and combining differential minimum wage levels and earned income tax credits might improve the rate of employment for older individuals.
1 Introduction
Literature about unemployment references both the unemployment of older workers (ages 45 or 50 and over) and youth unemployment (15–24). These two phenomena differ from one another in their characteristics, scope and solutions.
Unemployment among young people begins when they are eligible to work. According to the International Labor Office (ILO), young people are increasingly having trouble when looking for their first job (ILO 2011 ). The sharp increase in youth unemployment and underemployment is rooted in long-standing structural obstacles that prevent many youngsters in both OECD countries and emerging economies from making a successful transition from school to work. Not all young people face the same difficulties in gaining access to productive and rewarding jobs, and the extent of these difficulties varies across countries. Nevertheless, in all countries, there is a core group of young people facing various combinations of high and persistent unemployment, poor quality jobs when they do find work and a high risk of social exclusion (Keese et al. 2013 ). The rate of youth unemployment is much higher than that of adults in most countries of the world (ILO 2011 ; Keese et al. 2013 ; O’Higgins 1997 ; Morsy 2012 ). Official youth unemployment rates in the early decade of the 2010s ranged from under 10% in Germany to around 50% in Spain ( http://www.indexmundi.com/g/r.aspx?v=2229 ; Pasquali 2012 ). The youngest employees, typically the newest, are more likely to be let go compared to older employees who have been in their jobs for a long time and have more job experience and job security (Furlong et al. 2012 ). However, although unemployment rates among young workers are relatively higher than those of older people, the period of time they spend unemployed is generally shorter than that of older adults (O’Higgins 2001 ).
We would like to argue that one of the most important determinants of youth unemployment is the economy’s rate of growth. When the aggregate level of economic activity and the level of adult employment are high, youth employment is also high. Footnote 1 Quantitatively, the employment of young people appears to be one of the most sensitive variables in the labor market, rising substantially during boom periods and falling substantially during less active periods (Freeman and Wise 1982 ; Bell and Blanchflower 2011 ; Dietrich and Möller 2016 ). Several explanations have been offered for this phenomenon. First, youth unemployment might be caused by insufficient skills of young workers. Another reason is a fall in aggregate demand, which leads to a decline in the demand for labor in general. Young workers are affected more strongly than older workers by such changes in aggregate demand (O’Higgins 2001 ). Thus, our first research question is whether young adults are more vulnerable to economic shocks compared to their older counterparts.
Older workers’ unemployment is mainly characterized by difficulties in finding a new job for those who have lost their jobs (Axelrad et al. et al. 2013 ). This fact seems counter-intuitive because older workers have the experience and accumulated knowledge that the younger working population lacks. The losses to society and the individuals are substantial because life expectancy is increasing, the retirement age is rising in many countries, and people are generally in good health (Axelrad et al. 2013 ; Vodopivec and Dolenc 2008 ).
The difficulty that adults have in reintegrating into the labor market after losing their jobs is more severe than that of the younger unemployed. Studies show that as workers get older, the duration of their unemployment lengthens and the chances of finding a job decline (Böheim et al. 2011 ; De Coen et al. 2010 ). Therefore, our second research question is whether older workers’ unemployment stems from their age.
In this paper, we argue that the unemployment rates of young people and older workers are often misinterpreted. Even if the data show that unemployment rates are higher among young people, such statistics do not necessarily imply that it is harder for them to find a job compared to older individuals. We maintain that youth unemployment stems mainly from the characteristics of the labor market, not from specific attributes of young people. In contrast, the unemployment of older individuals is more related to their specific characteristics, such as higher salary expectations, higher labor costs and stereotypes about being less productive (Henkens and Schippers 2008 ; Keese et al. 2006 ). To test these hypotheses, we conduct an empirical analysis using statistics from the Israeli labor market and data published by the OECD. We also discuss some policy implications stemming from our results, specifically, a differential policy of minimum wages and earned income tax credits depending on the worker’s age.
Following the introduction and literary review, the next part of our paper presents the existing data about the unemployment rates of young people and adults in the OECD countries in general and Israel in particular. Than we present the research hypotheses and theoretical model, we describe the data, variables and methods used to test our hypotheses. The regression results are presented in Sect. 4 , the model of Business Cycle is presented in Sect. 5 , and the paper concludes with some policy implications, a summary and conclusions in Sect. 6 .
2 Literature review
Over the past 30 years, unemployment in general and youth unemployment in particular has been a major problem in many industrial societies (Isengard 2003 ). The transition from school to work is a rather complex and turbulent period. The risk of unemployment is greater for young people than for adults, and first jobs are often unstable and rather short-lived (Jacob 2008 ). Many young people have short spells of unemployment during their transition from school to work; however, some often get trapped in unemployment and risk becoming unemployed in the long term (Kelly et al. 2012 ).
Youth unemployment leads to social problems such as a lack of orientation and hostility towards foreigners, which in turn lead to increased social expenditures. At the societal level, high youth unemployment endangers the functioning of social security systems, which depend on a sufficient number of compulsory payments from workers in order to operate (Isengard 2003 ).
Workers 45 and older who have lost their jobs often encounter difficulties in finding a new job (Axelrad et al. 2013 ; Marmora and Ritter 2015 ) although today they are more able to work longer than in years past (Johnson 2004 ). In addition to the monetary rewards, work also offers mental and psychological benefits (Axelrad et al. 2016 ; Jahoda 1982 ; Winkelmann and Winkelmann 1998 ). Working at an older age may contribute to an individual’s mental acuity and provide a sense of usefulness.
On average, throughout the OECD, the hiring rate of workers aged 50 and over is less than half the rate for workers aged 25–49. The low re-employment rates among older job seekers reflect, among other things, the reluctance of employers to hire older workers. Lahey ( 2005 ) found evidence of age discrimination against older workers in labor markets. Older job applicants (aged 50 or older), are treated differently than younger applicants. A younger worker is more than 40% more likely to be called back for an interview compared to an older worker. Age discrimination is also reflected in the time it takes for older adults to find a job. Many workers aged 45 or 50 and older who have lost their jobs often encounter difficulties in finding a new job, even if they are physically and intellectually fit (Hendels 2008 ; Malul 2009 ). Despite the fact that older workers are considered to be more reliable (McGregor and Gray 2002 ) and to have better business ethics, they are perceived as less flexible or adaptable, less productive and having higher salary expectations (Henkens and Schippers 2008 ). Employers who hesitated in hiring older workers also mentioned factors such as wages and non-wage labor costs that rise more steeply with age and the difficulties firms may face in adjusting working conditions to meet the requirements of employment protection rules (Keese et al. 2006 ).
Thus, we have a paradox. On one hand, people live longer, the retirement age is rising, and older people in good health want or need to keep working. At the same time, employers seek more and more young workers all the time. This phenomenon might marginalize skilled and experience workers, and take away their ability to make a living and accrue pension rights. Thus, employers’ reluctance to hire older workers creates a cycle of poverty and distress, burdening the already overcrowded social institutions and negatively affecting the economy’s productivity and GDP (Axelrad et al. 2013 ).
2.1 OECD countries during the post 2008 crisis
The recent global economic crisis took an outsized toll on young workers across the globe, especially in advanced economies, which were hit harder and recovered more slowly than emerging markets and developing economies. Does this fact imply that the labor market in Spain and Portugal (with relatively high youth unemployment rates) is less “friendly” toward younger individuals than the labor market in Israel and Germany (with a relatively low youth unemployment rate)? Has the market in Spain and Portugal become less “friendly” toward young people during the last 4 years? We argue that the main factor causing the increasing youth unemployment rates in Spain and Portugal is the poor state of the economy in the last 4 years in these countries rather than a change in attitudes toward hiring young people.
OECD data indicate that adult unemployment is significantly lower than youth unemployment. The global economic crisis has hit young people very hard. In 2010, there were nearly 15 million unemployed youngsters in the OECD area, about four million more than at the end of 2007 (Scarpetta et al. 2010 ).
From an international perspective, and unlike other developed countries, Israel has a young age structure, with a high birthrate and a small fraction of elderly population. Israel has a mandatory retirement age, which differs for men (67) and women (62), and the labor force participation of older workers is relatively high (Stier and Endeweld 2015 ), therefore, we believe that Israel is an interesting case for studying.
The Israeli labor market is extremely flexible (e.g. hiring and firing are relatively easy), and mobile (workers can easily move between jobs) (Peretz 2016 ). Focusing on Israel’s labor market, we want to check whether this is true for older Israeli workers as well, and whether there is a difference between young and older workers.
The problem of unemployment among young people in Israel is less severe than in most other developed countries. This low unemployment rate is a result of long-term processes that have enabled the labor market to respond relatively quickly to changes in the economic environment and have reduced structural unemployment. Footnote 2 Furthermore, responsible fiscal and monetary policies, and strong integration into the global market have also promoted employment at all ages. With regard to the differences between younger and older workers in Israel, Stier and Endeweld ( 2015 ) determined that older workers, men and women alike, are indeed less likely to leave their jobs. This finding is similar to other studies showing that older workers are less likely to move from one employer to another. According to the U.S. Bureau of Labor Statistics, the median employee tenure is generally higher among older workers than younger ones (BLS 2014 ). Movement in and out of the labor market is highest among the youngest workers. However, these young people are re-employed quickly, while older workers have the hardest time finding jobs once they become unemployed. The Bank of Israel calculated the chances of unemployed people finding work between two consecutive quarters using a panel of the Labor Force Survey for the years 1996–2011. Their calculations show that since the middle of the last decade the chances of unemployed people finding a job between two consecutive quarters increased. Footnote 3 However, as noted earlier, as workers age, the duration of their unemployment lengthens. Prolonged unemployment erodes the human capital of the unemployed (Addison et al. 2004 ), which has a particularly deleterious effect on older workers. Thus, the longer the period of unemployment of older workers, the less likely they will find a job (Axelrad and Luski 2017 ). Nevertheless, as Fig. 1 shows, the rates of youth unemployment in Israel are higher than those of older workers.
(Source: Calculated by the authors by using data from the Labor Force survey of the Israeli CBS, 2011)
Unemployed persons and discouraged workers as percentages of the civilian labor force, by age group (Bank of Israel 2011 ). We excluded those living outside settled communities or in institutions. The percentages of discouraged workers are calculated from the civilian labor force after including them in it
We argue that the main reason for this situation is the status quo in the labor market, which is general and not specific to Israel. It applies both to older workers and young workers who have a job. The status quo is evident in the situation in which adults (and young people) already in the labor market manage to keep their jobs, making the entrance of new young people into the labor market more difficult. What we are witnessing is not evidence of a preference for the old over the young, but the maintaining of the status quo.
The rate of employed Israelis covered by collective bargaining agreements increases with age: up to age 35, the rate is less than one-quarter, and between 50 and 64 the rate reaches about one-half. In effect, in each age group between 25 and 60, there are about 100,000 covered employees, and the lower coverage rate among the younger ages derives from the natural growth in the cohorts over time (Bank of Israel 2013 ). The wave of unionization in recent years is likely to change only the age profile of the unionization rate and the decline in the share of covered people over the years, to the extent that it strengthens and includes tens of thousands more employees from the younger age groups. Footnote 4
The fact that the percentage of employees covered by collective agreement increases with age implies that there is a status quo effect. Older workers are protected by collective agreements, and it is hard to dismiss them (Culpepper 2002 ; Palier and Thelen 2010 ). However, young workers enter the workforce with individual contracts and are not protected, making it is easier to change their working conditions and dismiss them.
To complete the picture, Fig. 2 shows that the number of layoffs among adults is lower, possibly due to their protection under collective bargaining agreements.
(Source: Israeli Central Bureau of Statistics, 2008, data processed by the authors)
Dismissal of employees in Israel, by age. Percentage of total employed persons ages 20–75 and over including those dismissed
In order to determine the real difference between the difficulties of older versus younger individuals in finding work, we have to eliminate the effect of the status quo in the labor market. For example, if we removed all of the workers from the labor market, what would be the difference between the difficulties of older people versus younger individuals in finding work? In the next section we will analyze the probability of younger and older individuals moving from unemployment to employment when we control for the status quo. We will do so by considering only individuals who have not been employed at least part of the previous year.
3 Estimating the chances of finding a job and research hypotheses
Based on the literature and the classic premise that young workers are more vulnerable to economic shocks (ILO 2011 ), we posit that:
H 1 : The unemployment rate of young people stems mainly from the characteristics of the labor market and less from their personal attributes.
Based on the low hiring rate of older workers (OECD 2006 ) and the literature about age discrimination against older workers in labor markets (Axelrad et al. 2013 ; Lahey 2005 ), we hypothesis that:
H 2 : The difficulty face by unemployed older workers searching for a job stems mainly from their age and less from the characteristics of the labor market.
To assess the chances of younger and older workers finding a job, we used a logit regression model that has been validated in previous studies (Brander et al. 2002 ; Flug and Kassir 2001 ). Being employed was the dependent variable, and the characteristics of the respondents (age, gender, ethnicity and education) were the independent variables. The dependent variable was nominal and dichotomous with two categories: 0 or 1. We defined the unemployed as those who did not work at all during the last year or worked less than 9 months last year. The dependent variable was a dummy variable of the current employment situation, which received the value of 1 if the individual worked last week and 0 otherwise.
3.1 The model
i—individual i, P i —the chances that individual i will have a full or part time job (at the time of the survey). \(\underset{\raise0.3em\hbox{$\smash{\scriptscriptstyle-}$}}{\text{X}}_{\text{i}}\) —vector of explanatory variables of individual i. Each of the variables in vector \(\underset{\raise0.3em\hbox{$\smash{\scriptscriptstyle-}$}}{X}_{i}\) was defined as a dummy variable with the value of 1 or 0. β—vector of marginal addition to the log of the odds ratio. For example, if the explanatory variable was the log of 13 years or more of schooling, then the log odds ratio refers to the marginal addition of 13 years of education to the chances of being employed, compared with 12 years of education or less.
The regression allowed us to predict the probability of an individual finding a job. The dependent variable was the natural base log of the probability ratio P divided by (1 − P) that a particular individual would find a job. The odds ratio from the regression answers the question of how much more likely it is that an individual will find a job if he or she has certain characteristics. The importance of the probability analysis is the consideration of the marginal contribution of each feature to the probability of finding a job.
3.2 The sample
We used data gathered from the 2011 Labor Force Survey Footnote 5 of the Israeli Central Bureau of Statistics (CBS), Footnote 6 which is a major survey conducted annually among households. The survey follows the development of the labor force in Israel, its size and characteristics, as well as the extent of unemployment and other trends. Given our focus on working age individuals, we excluded all of the respondents under the age of 18 or over the age of 59. The data sample includes only the Jewish population, because structural problems in the non-Jewish sector made it difficult to estimate this sector using the existing data only. The sample does not include the ultra-Orthodox population because of their special characteristics, particularly the limited involvement of men in this population in the labor market.
The base population is individuals who did not work at all during the past year or worked less than 9 months last year (meaning that they worked but were unemployed at least part of last year). To determine whether they managed to find work after 1 year of unemployment, we used the question on the ICBS questionnaire, “Did you work last week?” We used the answer to this question to distinguish between those who had succeeded in finding a job and those who did not. The data include individuals who were out of the labor force Footnote 7 at the time of the survey, but exclude those who were not working for medical reasons (illness, disability or other medical restrictions) or due to their mandatory military service. Footnote 8
3.3 Data and variables
The survey contains 104,055 respondents, but after omitting all of the respondents under the age of 18 or above 59, those who were outside the labor force for medical reasons or due to mandatory military service, non-Jews, the ultra-Orthodox, and those who worked more than 9 months last year, the sample includes 13,494 individuals (the base population). Of these, 9409 are individuals who had not managed to find work, and 4085 are individuals who were employed when the survey was conducted.
The participants’ ages range between 18 and 59, with the average age being 33.07 (SD 12.88) and the median age being 29. 40.8% are males; 43.5% have an academic education; 52.5% are single, and 53.5% of the respondents have no children under 17.
3.4 Dependent and independent variables
While previous studies have assessed the probability of being unemployed in the general population, our study examines a more specific case: the probability of unemployed individuals finding a job. Therefore, we use the same explanatory variables that have been used in similar studies conducted in Israel (Brander et al. 2002 ; Flug and Kassir 2001 ), which were also based on an income survey and the Labor Force Survey of the Central Bureau of Statistics.
3.5 The dependent variable—being employed
According to the definition of the CBS, employed persons are those who worked at least 1 h during a given week for pay, profit or other compensation.
3.6 Independent variables
We divided the population into sub-groups of age intervals: 18–24, 25–29, 30–44, 45–54 and 55–59, according to the sub-groups provided by the CBS. We then assigned a special dummy variable to each group—except the 30–44 sub-group, which is considered as the base group. Age is measured as a dummy variable, and is codded as 1 if the individual belongs to the age group, and 0 otherwise. Age appears in the regression results as a variable in and of itself. Its significance is the marginal contribution of each age group to the probability of finding work relative to the base group (ages 30–44), and also as an interaction variable.
3.6.2 Gender
This variable is codded as 1 if the individual is female and 0 otherwise. Gender also appears in the interaction with age.
3.6.3 Marital status
Two dummy variables are used: one for married respondents and one for those who are divorced or widowed. In accordance with the practice of the CBS, we combined the divorced and the widowed into one variable. This variable is a dummy variable that is codded as 1 if the individual belongs to the appropriate group (divorced/widowed or married) and 0 otherwise. The base group is those who are single.
3.6.4 Education
This variable is codded as 1 if the individual has 13 or more years of schooling, and 0 otherwise. The variable also appears in interactions between it and the age variable.
3.6.5 Vocational education
This variable is codded as 1 if the individual has a secondary school diploma that is not an academic degree or another diploma, and 0 otherwise.
3.6.6 Academic education
This variable is codded as 1 if the individual has any university degree (bachelors, masters or Ph.D.) and 0 otherwise.
3.6.7 Children
In accordance with similar studies that examined the probability of employment in Israel (Brander et al. 2002 ), we define children as those up to age 17. This variable is a dummy variable that is codded as 1 if the respondents have children under the age of 17, and 0 otherwise.
3.6.8 Ethnicity
This variable is codded as 1 if the individual was born in an Arabic-speaking country, in an African country other than South Africa, or in an Asian country, or was born in Israel but had a father who was born in one of these countries. Israel generally refers to such individuals as Mizrahim. Respondents who were not Mizrahim received a value of 0. The base group in our study are men aged 30–44 who are not Mizrahim.
We also assessed the interactions between the variables. For example, the interaction between age and the number of years of schooling is the contribution of education (i.e., 13 years of schooling) to the probability of finding a job for every age group separately relative to the situation of having less education (i.e., 12 years of education). The interaction between age and gender is the contribution of gender (i.e., being a female respondent) to the probability of finding a job for each age group separately relative to being a man.
To demonstrate the differences between old and young individuals in their chances of finding a job, we computed the rates of those who managed to find a job relative to all of the respondents in the sample. Table 1 shows that the rate of those who found a job declines with age. For example, 36% of the men age 30–44 found a job, but those rates drop to 29% at the age of 45–54 and decline again to 17% at the age of 55–59. As for women, 31% of them aged 30–44 found a job, but those rates drop to 20% at the age of 45–54 and decline again to 9% at the age of 55–59.
In an attempt to determine the role of education in finding employment, we created Model 1 and Model 2, which differ only in terms of how we defined education. In Model 1 the sample is divided into two groups: those with up to 12 years of schooling (the base group) and those with 13 or more years of schooling. In Model 2 there are three sub-groups: those with a university degree, those who have a vocational education, and the base group that has only a high school degree.
Table 2 shows that the probability of a young person (age 18–24) getting a job is larger than that of an individual aged 30–44 who belongs to the base group (the coefficient of the dummy variable “age 18–24” is significant and positive). Similarly, individuals who are older than 45 are less likely than those in the base group to find work.
Women aged 30–44 are less likely to be employed than men in the same age group. Additionally, when we compare women aged 18–24 to women aged 30–44, we see that the chances of the latter being employed are lower. Older women (45+) are much less likely than men of the same age group to find work. Additionally, having children under the age of 17 at home reduces the probability of finding a job.
A university education increases the probability of being employed for both men and women aged 30–44. Furthermore, for older people (55+) an academic education reduces the negative effect of age on the probability of being employed. While a vocational education increases the likelihood of finding a job for those aged 30–44, such a qualification has no significant impact on the prospects of older people.
Interestingly, being a Mizrahi Jew increases the probability of being employed.
In addition, we estimated the models separately twice—for the male and for the female population. For male and female, the probability of an unemployed individual finding a job declines with age.
Analyzing the male population (Table 3 ) reveals that those aged 18–24 are more likely than the base group (ages 30–44) to find a job. However, the significance level is relatively low, and in Model 2, this variable is not significant at all. Those 45 and older are less likely than the base group (ages 30–44) to find a job. Married men are more likely than single men to be employed. However, divorced and widowed men are less likely than single men to find a job. For men, the presence in their household of children under the age of 17 further reduces the probability of their being employed. Mizrahi men aged 18–24 are more likely to be employed than men of the same age who are from other regions.
Table 3 illustrates that educated men are more likely to find work than those who are not. However, in Model 1, at the ages 18–29 and 45–54, the probability of finding a job for educated men is less than that of uneducated males. Among younger workers, this might be due to excess supply—the share of academic degree owners has risen, in contrast to almost no change in the overall share of individuals receiving some other post-secondary certificate (Fuchs 2015 ). Among older job seeking men, this might be due to the fact that the increase in employment among men during 2002–2010 occurred mainly in part-time jobs (Bank of Israel 2011 ). In Model 2, men with an academic or vocational education have a better chance of finding a job, but at the group age of 18–24, those with a vocational education are less likely to find a job compared to those without a vocational education. The reason might be the lack of experience of young workers (18–24), experience that is particularly needed in jobs that require vocational education (Salvisberg and Sacchi 2014 ).
Analyzing the female population (Table 3 ) reveals that women between 18 and 24 are more likely to be employed than those who are 30–44, and those who are 45–59 are less likely to be employed than those who are 30–44. The probability of finding a job for women at the age of 25 to 29 is not significantly different from the probability of the base group (women ages 30–44).
Married women are less likely than single women to be employed. Women who have children under the age of 17 are less likely to be employed than women who do not have dependents that age. According to Model 2, Mizrahi women are more likely to be employed compared to women from other regions. According to both models, women originally from Asia or Africa ages 25–29 have a better chance of being employed than women the same age from other regions. Future research should examine this finding in depth to understand it.
With regard to education, in Model 1 (Table 3 ), where we divided the respondents simply on the question of whether they had a post-high school education, women who were educated were more likely to find work than those who were not. However, in the 18–29 age categories, educated women were less likely to find a job compared to uneducated women, probably due to the same reason cited above for men in the same age group—the inflation of academic degrees (Fuchs 2015 ). These findings become more nuanced when we consider the results of Model 2. There, women with an academic or vocational education have a better chance of finding a job, but at the ages of 18–24 those with an academic education are less likely to find a job than those without an academic education. Finally, at the ages of 25–29, those with a vocational education have a better chance of finding a job than those without a vocational education, due to the stagnation in the overall share of individuals receiving post-secondary certificate (Fuchs 2015 ).
Thus, based on the results in Table 3 , we can draw several conclusions. First, the effect of aging on women is more severe than the impact on men. In addition, the “marriage premium” is positive for men and negative for women. Divorced or widowed men lose their “marriage premium”. Finally, having children at home has a negative effect on both men and women—almost at the same magnitude.
5 Unemployment as a function of the business cycle
To determine whether unemployment of young workers is caused by the business cycle, we examined the unemployment figures in 34 OECD countries in 2007–2009, years of economic crisis, and in 2009–2011, years of recovery and economic growth. For each country, we considered the data on unemployment among young workers (15–24) and older adults (55–64) and calculated the difference between 2009 and 2007 and between 2011 and 2009 for both groups. The data were taken from OECD publications and included information about the growth rates from 2007 to 2011. Our assessment of unemployment rates in 34 OECD countries reveals that the average rate of youth unemployment in 2007 was 13.4%, compared to 18.9% in 2011, so the delta of youth unemployment before and after the economic crisis was 5.55. The average rate of adult unemployment in 2007 was 4% compared to 5.8% in 2011, so the delta for adults was 1.88. Both of the differences are significantly different from zero, and the delta for young people is significantly larger than the delta for adults. These results indicate that among young people (15–24), the increase in unemployment due to the crisis was very large.
An OLS model of the reduced form was estimated to determine whether unemployment is a function of the business cycle, which is represented by the growth rate. The variables GR2007, GR2009 and GR2011 are the rate of GDP growth in 2007, 2009 and 2011 respectively ( Appendix ). The explanatory variable is either GR2009 minus GR2007 or GR2011 minus GR2009. In both periods, 2007–2009 and 2009–2011, the coefficient of the change in growth rates is negative and significant for young people, but insignificant for adults. Thus, it seems that the unemployment rates of young people are affected by the business cycle, but those of older workers are not. In a time of recession (2007–2009), unemployment among young individuals increases whereas for older individuals the increase in unemployment is not significant. In recovery periods (2009–2011), unemployment among young individuals declines, whereas the drop in unemployment among older individuals is not significant (Table 4 ).
6 Summary and conclusions
The purpose of this paper was to show that while the unemployment rates of young workers are higher than those of older workers, the data alone do not necessarily tell the whole story. Our findings confirm our first hypothesis, that the high unemployment rate of young people stems mainly from the characteristics of the labor market and less from their personal attributes. Using data from Israel and 34 OECD countries, we demonstrated that a country’s growth rate is the main factor that determines youth unemployment. However, the GDP rate of growth cannot explain adult unemployment. Our results also support our second hypothesis, that the difficulties faced by unemployed older workers when searching for a job are more a function of their age than the overall business environment.
Indeed, one limitation of the study is the fact that we could not follow individuals over time and capture individual changes. We analyze a sample of those who have been unemployed in the previous year and then analyze the probability of being employed in the subsequent year but cannot take into account people could have found a job in between which they already lost again. Yet, in this sample we could isolate and analyze those who did not work last year and look at their employment status in the present. By doing so, we found out that the rate of those who found a job declines with age, and that the difficulties faced by unemployed older workers stems mainly from their age.
To solve both of these problems, youth unemployment and older workers unemployment, countries need to adopt different methods. Creating more jobs will help young people enter the labor market. Creating differential levels for the minimum wage and supplementing the income of older workers with earned income tax credits will help older people re-enter the job market.
Further research may explore the effect of structural and institutional differences which can also determine individual unemployment vs. employment among different age groups.
In addition to presenting a theory about the factors that affect the differences in employment opportunities for young people and those over 45, the main contribution of this paper is demonstrating the validity of our contention that it is age specifically that works to keep older people out of the job market, whereas it is the business cycle that has a deleterious effect on the job prospects of younger people. Given these differences, these two sectors of unemployment require different approaches for solving their employment problems. The common wisdom maintains that the high level of youth unemployment requires policy makers to focus on programs targeting younger unemployed individuals. However, we argue that given the results of our study, policy makers must adopt two different strategies to dealing with unemployment in these two groups.
6.1 Policy implications
In order to cope with the problem of youth unemployment, we must create more jobs. When the recession ends in Portugal and Spain, the problem of youth unemployment should be alleviated. Since there is no discrimination against young people—evidenced by the fact that when the aggregate level of economic activity and the level of adult employment are high, youth employment is also high—creating more jobs in general by enhancing economic growth should improve the employment rates of young workers.
In contrast, the issue of adult unemployment requires a different solution due to the fact that their chances of finding a job are related specifically to their age. One solution might be a differential minimum wage for older and younger individuals and earned income tax credits (EITC) Footnote 9 for older individuals, as Malul and Luski ( 2009 ) suggested.
According to this solution, the government should reduce the minimum wage for older individuals. As a complementary policy and in order to avoid differences in wages between older and younger individuals, the former would receive an earned income tax credit so that their minimum wage together with their EITC would be equal to the minimum wage of younger individuals. Earned income tax credits could increase employment among older workers while increasing their income. For older workers, EITCs are more effective than a minimum wage both in terms of employment and income. Such policies of a differential minimum wage plus an EITC can help older adults and constitute a kind of social safety net for them. Imposing a higher minimum wage exclusively for younger individuals may be beneficial in encouraging them to seek more education.
Young workers who face layoffs as a result of their high minimum wage (Kalenkoski and Lacombe 2008 ) may choose to increase their investment in their human capital (Nawakitphaitoon 2014 ). The ability of young workers to improve their professional level protects them against the unemployment that might result from a higher minimum wage (Malul and Luski 2009 ). For older workers, if the minimum wage is higher than their productivity, they will be unemployed. This will be true even if their productivity is higher than the value of their leisure. Such a situation might result in an inefficient allocation between work and leisure for this group. One way to fix this inefficient allocation without reducing the wages of older individuals is to use the EITC, which is actually a subsidy for this group. This social policy might prompt employers to substitute older workers with a lower minimum wage for more expensive younger workers, making it possible for traditional factories to continue their domestic production. However, a necessary condition for this suggestion to work is the availability of efficient systems of training and learning. Axelrad et al. ( 2013 ) provided another justification for subsidizing the work of older individuals. They found that stereotypes about older workers might lead to a distorted allocation of the labor force. Subsidizing the work of older workers might correct this distortion. Ultimately, however, policy makers must understand that they must implement two different approaches to dealing with the problems of unemployment among young people and in the older population.
For example, in the US, the UK and Portugal, we witnessed higher rates of growth during late 1990 s and lower rates of youth unemployment compared to 2011.
Bank of Israel Annual Report—2013, http://www.boi.org.il/en/NewsAndPublications/RegularPublications/Research%20Department%20Publications/BankIsraelAnnualReport/Annual%20Report-2013/p5-2013e.pdf .
http://www.boi.org.il/en/NewsAndPublications/RegularPublications/Research%20Department%20Publications/RecentEconomicDevelopments/develop136e.pdf .
The Labor Force Survey is a major survey conducted by the Israeli Central Bureau of Statistics among households nationwide. The survey follows the development of the labor force in Israel, its size and characteristics, as well as the extent of unemployment and other trends. The publication contains detailed data on labor force characteristics such as their age, years of schooling, type of school last attended, and immigration status. It is also a source of information on living conditions, mobility in employment, and many other topics.
The survey population is the permanent (de jure) population of Israel aged 15 and over. For more details see: http://www.cbs.gov.il/publications13/1504/pdf/intro04_e.pdf .
When we looked at those who had not managed to find a job at the time of the survey, we included all individuals who were not working, regardless of whether they were discouraged workers, volunteers or had other reasons. As long as they are not out of the labor force due to medical reasons or their mandatory military service, we classified them as "did not manage to find a job."
Until 2012, active soldiers were considered outside the labor force in the samples of the CBS.
EITC is a refundable tax credit for low to moderate income working individuals and couples.
Addison, J.T., Centeno, M., Portugal, P.: Reservation wages, search duration, and accepted wages in Europe (No. 1252). IZA Discussion paper series (2004)
Axelrad, H., Luski, I., Malul, M.: Difficulties of integrating older workers into the labor market: exploring the Israeli labor market. Int. J. Soc. Econ. 40 (12), 1058–1076 (2013). https://doi.org/10.1108/ijse-12-2011-0098
Article Google Scholar
Axelrad, H., Luski, I., Malul, M.: Behavioral biases in the labor market differences between older and younger individuals. J. Behav. Exp. Econ. 60 , 23–28 (2016). https://doi.org/10.1016/j.socec.2015.11.003
Axelrad, H., Luski, I., Malul, M.: Reservation wages and unemployment among older workers. J. Labor Res. 38 (2), 206–227 (2017). https://doi.org/10.1007/s12122-017-9247-6
Bank of Israel.: Bank of Israel report, Bank of Israel Research Department Jerusalem, March 2011. https://www.boi.org.il/he/NewsAndPublications/RegularPublications/Doch2010/p5.pdf (2011). Retrieved 17 Jan 2018
Bank of Israel.: Recent economic developments 136, Bank of Israel Research Department Jerusalem, December 2013. http://www.boi.org.il/en/NewsAndPublications/RegularPublications/Research%20Department%20Publications/BankIsraelAnnualReport/Annual%20Report-2013/p5-2013e.pdf (2013). Retrieved 16 July 2014
Bell, D.N., Blanchflower, D.G.: Young people and the great recession. Oxford Rev. Econ. Pol. 27 (2), 241–267 (2011). https://doi.org/10.1093/oxrep/grr011
Böheim, R., Horvath, G.T., Winter-Ebmer, R.: Great expectations: past wages and unemployment durations. Labour Econ. 18 (6), 778–785 (2011). https://doi.org/10.1016/j.labeco.2011.06.009
Brander, A., Peled Levy, O., Kassir, N.: Government policy and labor force participation rates of the working age population Israel and the OECD countries in the 90s. Bank of Israel Survey, August 2002 (2002), 7–61 ISSN 0552-2761 (Hebrew)
Bureau of Labor Statistics.: Employee tenure summary employee tenure in 2014, Bureau of Labor Statistics, U.S. Department of Labor. http://www.bls.gov/news.release/tenure.nr0.htm (2014). Retrieved 22 June 2015
Culpepper, P.D.: Powering, puzzling, and ‘pacting’: the informational logic of negotiated reforms. J. Eur. Public Policy 9 (5), 774–790 (2002)
De Coen, A., Forrier, A., Sels, L.: The impact of age on the reservation wage: the role of employability. FBE Research Report MO_1001, pp. 1–36. (2010). https://ssrn.com/abstract=1620368 or http://dx.doi.org/10.2139/ssrn.1620368
Dietrich, H., Möller, J.: Youth unemployment in Europe—business cycle and institutional effects. Int. Econ. Econ. Policy 13 (1), 5–25 (2016). https://doi.org/10.1007/s10368-015-0331-1
Flug, K., Kassir, N.: On poverty, work and everything in between. Research Department Bank of Israel (2001) (Hebrow)
Freeman, R.B., Wise, D.A.: The youth labor market problem: its nature causes and consequences. In: The youth labor market problem: Its nature, causes, and consequences, pp. 1–16. University of Chicago Press, Chicago (1982)
Fuchs, H.: The socioeconomic situation of young adults in Israel. State of the nation report: society, economy and policy in Israel, pp. 139–181 (2015)
Furlong, A.: Youth studies: an introduction, pp. 72–97. Routledge, New York (2012)
Hendeles, S.: The Center for Adult Employment. National Insurance Institute, Research and Planning, Development Services Division (2008) (Hebrew)
Henkens, K., Schippers, J.: Labor market policies regarding older workers in the Netherlands. In: Taylor, P. (ed.) The ageing labor force: promises and prospects, pp. 141–157. Edward Elgar, Cheltenham (2008)
Google Scholar
International Labor Office: Global employment trends for youth: 2011 update. International Labor Office, Geneva (2011)
Isengard, B.: Youth unemployment: Individual risk factors and institutional determinants. A case study of Germany and the United Kingdom. J. Youth Stud. 6 (4), 357–376 (2003). https://doi.org/10.1080/1367626032000162096
Jacob, M.: Unemployment benefits and parental resources: what helps the young unemployed with labor market integration? J. Youth Stud. 11 (2), 147–163 (2008). https://doi.org/10.1080/13676260701863413
Jahoda, M. (ed.): Employment and unemployment”. Cambridge University Press, Cambridge (1982)
Johnson, R.W.: Trends in job demands among older workers, 1992–2002. Mon. Labor Rev. 127 , 48 (2004)
Kalenkoski, C.M., Lacombe, D.J.: Effects of minimum wages on youth employment: the importance of accounting for spatial correlation. J. Labor Res. 29 (4), 303–317 (2008). https://doi.org/10.1007/s12122-007-9038-6
Keese, M., Queisser, M., Whitehouse, E.: Older workers living longer, working longer, DELSA Newsletter 2, OECD (2006)
Keese, M., Roseveare, D., Giguere, S.: The OECD action plan for youth, giving youth a better start in the labor market. OECD, Paris (2013)
Kelly, E., McGuinness, S., O'Connell, P.: J: Transitions to long-term unemployment risk among young people: evidence from Ireland. J Youth Stud 15 (6), 780–801 (2012). https://doi.org/10.1080/13676261.2012.678047
Lahey, J.N.: Do older workers face discrimination? Center for Retirement Research at Boston College (2005)
Malul, M.: Older workers’ employment in dynamic technology changes. J. Socio Econ. 38 (5), 809–813 (2009). https://doi.org/10.1016/j.socec.2009.05.005
Malul, M., Luski, I.: The optimal policy combination of the minimum wage and the earned income tax credit. BE J. Econ. Anal. Policy 9 , 1 (2009). https://doi.org/10.2202/1935-1682.1953
Marmora, P., Ritter, M.: Unemployment and the retirement decisions of older workers. J. Labor Res. 36 (3), 274–290 (2015). https://doi.org/10.1007/s12122-015-9207-y
McGregor, J., Gray, L.: Stereotypes and older workers: The New Zealand experience. Soc. Policy J. NZ. 163–177 (2002).
Morsy, H.: Scarred generation. Financ. Dev. 49 , 1 (2012)
Nawakitphaitoon, K.: Occupational human capital and wages: the role of skills transferability across occupations. J. Labor Res. 35 (1), 63–87 (2014). https://doi.org/10.1007/s12122-013-9172-2
OECD.: Live longer, work longer—ISBN-92-64-035877 (2006)
O’Higgins, N.: The challenge of youth unemployment. Int. Soc. Secur. Rev. 50 (4), 63–93 (1997). https://doi.org/10.1111/j.1468-246X.1997.tb01084.x
O’Higgins, N.: Youth unemployment and employment policy: a global perspective. International Labor Office, Geneva (2001)
Palier, B., Thelen, K.: Institutionalizing dualism: complementarities and change in France and Germany. Politics Soc. 38 (1), 119–148 (2010)
Pasquali, V.: Unemployment rates in countries around the world. Global Finance. https://www.gfmag.com/global-data/economic-data/worlds-unemployment-ratescom (2012). Retrieved 4 Nov 2013
Peretz, S.: The secret of the Israeli labor market’s flexibility. Haaretz. https://www.haaretz.com/israel-news/business/opinion/.premium-1.698583 (2016). Accessed 7 Jan 2018
Salvisberg, A., Sacchi, S.: Labour market prospects of Swiss career entrants after completion of vocational education and training. Eur. Soc. 16 (2), 255–274 (2014). https://doi.org/10.1080/14616696.2013.821623
Scarpetta, S., Sonnet, A., Manfredi, T.: Rising youth unemployment during the crisis: how to prevent negative long-term consequences on a generation?, OECD Social, Employment and Migration Working Papers, No. 106, OECD Publishing (2010). https://doi.org/10.1787/5kmh79zb2mmv-en
Stier, H., Endeweld, M.: Employment transitions and labor market exits: age and gender in the Israeli labor market. Res. Soc. Stratif. Mobil. 30 (41), 93–103 (2015). https://doi.org/10.1016/j.rssm.2015.01.002
Vodopivec, M., Dolenc, P.: Live longer, work longer: making it happen in the labor market. Financ. Theory Pract. 32 (1), 65–81 (2008)
Winkelmann, L., Winkelmann, R.: Why are the unemployed so unhappy? Evidence from panel data. Economica 65 (257), 1–15 (1998)
Download references
Authors’ contributions
HA, MM and IL conceptualized and designed the study. HA collected and managed study data, HA and IL carried out statistical analyses. HA drafted the initial manuscript. MM and IL reviewed and revised the manuscript. All authors read and approved the final manuscript.
Competing interests
The authors declare that they have any no competing interests.
Ethics approval and consent to participate
Not applicable.
Publisher’s Note
Springer Nature remains neutral with regard to jurisdictional claims in published maps and institutional affiliations.
Author information
Authors and affiliations.
Center on Aging & Work, Boston College, Chestnut Hill, MA, 02467, USA
Hila Axelrad
The School of Social and Policy Studies, The Faculty of Social Sciences, Tel Aviv University, P.O. Box 39040, 6997801, Tel Aviv, Israel
Department of Public Policy & Administration, Guilford Glazer Faculty of Business & Management, Ben-Gurion University of the Negev, Beer Sheva, Israel
Department of Economics, The Western Galilee College, Akko, Israel
Israel Luski
You can also search for this author in PubMed Google Scholar
Corresponding author
Correspondence to Hila Axelrad .
See Table 5 .
Rights and permissions
Open Access This article is distributed under the terms of the Creative Commons Attribution 4.0 International License ( http://creativecommons.org/licenses/by/4.0/ ), which permits unrestricted use, distribution, and reproduction in any medium, provided you give appropriate credit to the original author(s) and the source, provide a link to the Creative Commons license, and indicate if changes were made.
Reprints and permissions
About this article
Cite this article.
Axelrad, H., Malul, M. & Luski, I. Unemployment among younger and older individuals: does conventional data about unemployment tell us the whole story?. J Labour Market Res 52 , 3 (2018). https://doi.org/10.1186/s12651-018-0237-9
Download citation
Received : 22 September 2017
Accepted : 25 February 2018
Published : 08 March 2018
DOI : https://doi.org/10.1186/s12651-018-0237-9
Share this article
Anyone you share the following link with will be able to read this content:
Sorry, a shareable link is not currently available for this article.
Provided by the Springer Nature SharedIt content-sharing initiative
- Unemployment
- Older workers
JEL Classification
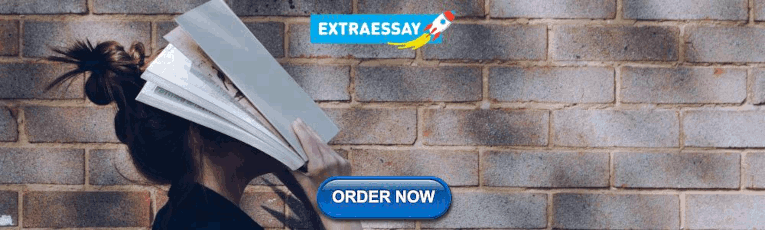
COMMENTS
Overall, the literature indicates gaps in research on unemployment and specific types of underemployment, and on indicators of health and well-being in specific population groups, such as young workers, women, and low-income people of color living in neighborhoods who are disproportionately burdened by job loss.
unemployment agree that seeking jobs and the ability to work. are the main characteristics of unemployed people. Since. unemployment leads to negative e conomic, social, and. security outcomes [5 ...
Abstract. Job loss is an involuntary disruptive life event with a far-reaching impact on workers’ life trajectories. Its incidence among growing segments of the workforce, alongside the recent era of severe economic upheaval, has increased attention to the effects of job loss and unemployment. As a relatively exogenous labor market shock, the ...
3.1 Selection Criteria and Study Features. Following a set of standards in summarizing the literature suggested by the Meta-Analysis of Economics Research Network (MAER-Net) guidelines (Havránek et al. 2020), we carried out our literature search through a comprehensive search in Web of Science and Google Scholar databases and focused only on articles in English, for the sake of accessibility ...
The framework of a career planning concept and career paths over time (Wanberg, 2012) could be considered as yet another approach through which it is possible to examine job loss, by pointing out the dynamic career planning activities over the course of one's unemployment. Furthermore, research focusing on career exploration during the ...
Abstract. This essay represents the collective vision of a group of scholars in vocational psychology who have sought to develop a research agenda in response to the massive global unemployment crisis that has been evoked by the COVID-19 pandemic. The research agenda includes exploring how this unemployment crisis may differ from previous ...
This paper presents a flow-based methodology for real-time unemployment rate projections and shows that this approach performed considerably better at the onset of the COVID-19 recession in the spring 2020 in predicting the peak unemployment rate as well as its rapid decline over the
April 2022, No. 22-12. Following early 2020 responses to the pandemic, labor force participation declined dramatically and has remained below its 2019 level, whereas the unemployment rate recovered briskly. We estimate the trend of labor force participation and unemployment and find a substantial impact of the pandemic on estimates of trend.
Introduction. The onset of COVID19 pandemic saw unemployment climb to the highest rate since the Great Depression in many regions globally 1.Over just one month, from March to April 2020 unemployment rate in the United States increased from 4.4% to over 14.7% and in Australia the effective rate of unemployment increased from 5.4 to 11.7% (Australian Bureau of Statistics, 2020) 2.
In this research we show that workers aged 30–44 were significantly more likely than those aged 45–59 to find a job a year after being unemployed. The main contribution is demonstrating empirically that since older workers’ difficulties are related to their age, while for younger individuals the difficulties are more related to the business cycle, policy makers must devise different ...