
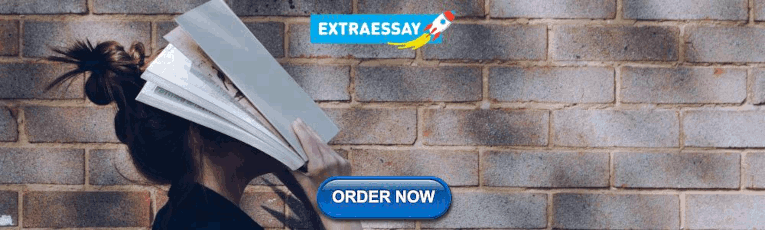
21 Great Examples of Discourse Analysis
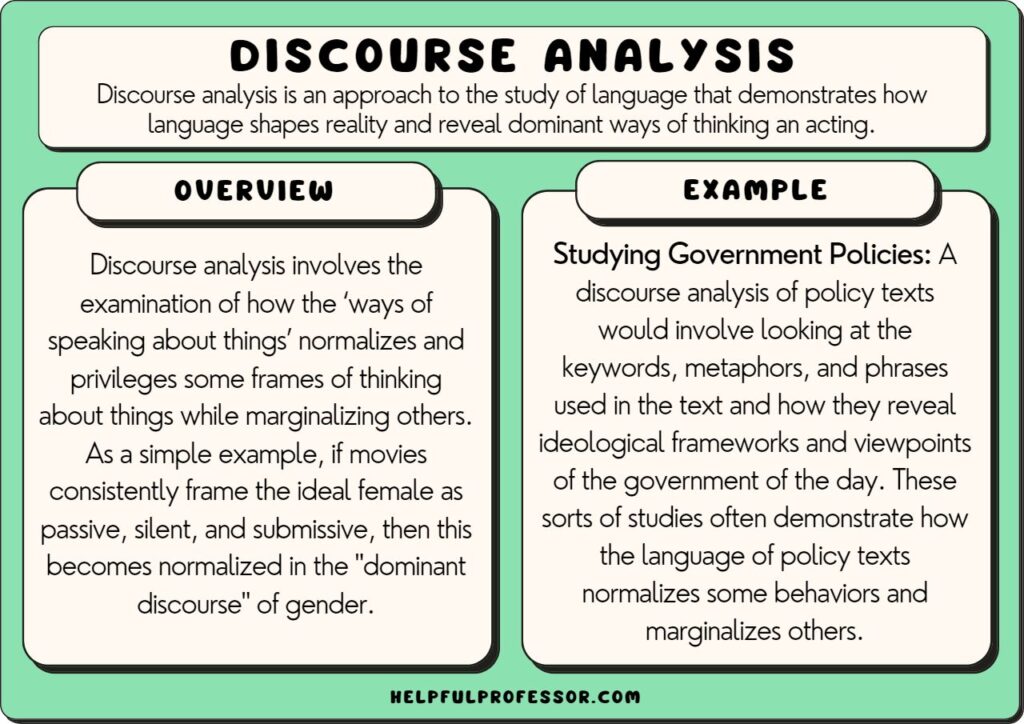
Discourse analysis is an approach to the study of language that demonstrates how language shapes reality. It usually takes the form of a textual or content analysis .
Discourse is understood as a way of perceiving, framing, and viewing the world.
For example:
- A dominant discourse of gender often positions women as gentle and men as active heroes.
- A dominant discourse of race often positions whiteness as the norm and colored bodies as ‘others’ (see: social construction of race )
Through discourse analysis, scholars look at texts and examine how those texts shape discourse.
In other words, it involves the examination of how the ‘ways of speaking about things’ normalizes and privileges some frames of thinking about things while marginalizing others.
As a simple example, if movies consistently frame the ideal female as passive, silent, and submissive, then society comes to think that this is how women should behave and makes us think that this is normal , so women who don’t fit this mold are abnormal .
Instead of seeing this as just the way things are, discourse analysts know that norms are produced in language and are not necessarily as natural as we may have assumed.
Examples of Discourse Analysis
1. language choice in policy texts.
A study of policy texts can reveal ideological frameworks and viewpoints of the writers of the policy. These sorts of studies often demonstrate how policy texts often categorize people in ways that construct social hierarchies and restrict people’s agency .
Examples include:
2. Newspaper Bias
Conducting a critical discourse analysis of newspapers involves gathering together a quorum of newspaper articles based on a pre-defined range and scope (e.g. newspapers from a particular set of publishers within a set date range).
Then, the researcher conducts a close examination of the texts to examine how they frame subjects (i.e. people, groups of people, etc.) from a particular ideological, political, or cultural perspective.
3. Language in Interviews
Discourse analysis can also be utilized to analyze interview transcripts. While coding methods to identify themes are the most common methods for analyzing interviews, discourse analysis is a valuable approach when looking at power relations and the framing of subjects through speech.
4. Television Analysis
Discourse analysis is commonly used to explore ideologies and framing devices in television shows and advertisements.
Due to the fact advertising is not just textual but rather multimodal , scholars often mix a discourse analytic methodology (i.e. exploring how television constructs dominant ways of thinking) with semiotic methods (i.e. exploration of how color, movement, font choice, and so on create meaning).
I did this, for example, in my PhD (listed below).
5. Film Critique
Scholars can explore discourse in film in a very similar way to how they study discourse in television shows. This can include the framing of sexuality gender, race, nationalism, and social class in films.
A common example is the study of Disney films and how they construct idealized feminine and masculine identities that children should aspire toward.
6. Analysis of Political Speech
Political speeches have also been subject to a significant amount of discourse analysis. These studies generally explore how influential politicians indicate a shift in policy and frame those policy shifts in the context of underlying ideological assumptions.
9. Examining Marketing Texts
Advertising is more present than ever in the context of neoliberal capitalism. As a result, it has an outsized role in shaping public discourse. Critical discourse analyses of advertising texts tend to explore how advertisements, and the capitalist context that underpins their proliferation, normalize gendered, racialized, and class-based discourses.
11. Analyzing Lesson Plans
As written texts, lesson plans can be analyzed for how they construct discourses around education as well as student and teacher identities. These texts tend to examine how teachers and governing bodies in education prioritize certain ideologies around what and how to learn. These texts can enter into discussions around the ‘history wars’ (what and whose history should be taught) as well as ideological approaches to religious and language learning.
12. Looking at Graffiti
One of my favorite creative uses of discourse analysis is in the study of graffiti. By looking at graffiti, researchers can identify how youth countercultures and counter discourses are spread through subversive means. These counterdiscourses offer ruptures where dominant discourses can be unsettled and displaced.
Get a Pdf of this article for class
Enjoy subscriber-only access to this article’s pdf
The Origins of Discourse Analysis
1. foucault.
French philosopher Michel Foucault is a central thinker who shaped discourse analysis. His work in studies like Madness and Civilization and The History of Sexuality demonstrate how our ideas about insanity and sexuality have been shaped through language.
The ways the church speaks about sex, for example, shapes people’s thoughts and feelings about it.
The church didn’t simply make sex a silent taboo. Rather, it actively worked to teach people that desire was a thing of evil, forcing them to suppress their desires.
Over time, society at large developed a suppressed normative approach to the concept of sex that is not necessarily normal except for the fact that the church reiterates that this is the only acceptable way of thinking about the topic.
Similarly, in Madness and Civilization , a discourse around insanity was examined. Medical discourse pathologized behaviors that were ‘abnormal’ as signs of insanity. Were the dominant medical discourse to change, it’s possible that abnormal people would no longer be seen as insane.
One clear example of this is homosexuality. Up until the 1990s, being gay was seen in medical discourse as an illness. Today, most of Western society sees that this way of looking at homosexuality was extremely damaging and exclusionary, and yet at the time, because it was the dominant discourse, people didn’t question it.
2. Norman Fairclough
Fairclough (2013), inspired by Foucault, created some key methodological frameworks for conducting discourse analysis.
Fairclough was one of the first scholars to articulate some frameworks around exploring ‘text as discourse’ and provided key tools for scholars to conduct analyses of newspaper and policy texts.
Today, most methodology chapters in dissertations that use discourse analysis will have extensive discussions of Fairclough’s methods.
Discourse analysis is a popular primary research method in media studies, cultural studies, education studies, and communication studies. It helps scholars to show how texts and language have the power to shape people’s perceptions of reality and, over time, shift dominant ways of framing thought. It also helps us to see how power flows thought texts, creating ‘in-groups’ and ‘out-groups’ in society.
Key examples of discourse analysis include the study of television, film, newspaper, advertising, political speeches, and interviews.
Al Kharusi, R. (2017). Ideologies of Arab media and politics: a CDA of Al Jazeera debates on the Yemeni revolution. PhD Dissertation: University of Hertfordshire.
Alaazi, D. A., Ahola, A. N., Okeke-Ihejirika, P., Yohani, S., Vallianatos, H., & Salami, B. (2021). Immigrants and the Western media: a CDA of newspaper framings of African immigrant parenting in Canada. Journal of Ethnic and Migration Studies , 47 (19), 4478-4496. Doi: https://doi.org/10.1080/1369183X.2020.1798746
Al-Khawaldeh, N. N., Khawaldeh, I., Bani-Khair, B., & Al-Khawaldeh, A. (2017). An exploration of graffiti on university’s walls: A corpus-based discourse analysis study. Indonesian Journal of Applied Linguistics , 7 (1), 29-42. Doi: https://doi.org/10.17509/ijal.v7i1.6856
Alsaraireh, M. Y., Singh, M. K. S., & Hajimia, H. (2020). Critical DA of gender representation of male and female characters in the animation movie, Frozen. Linguistica Antverpiensia , 104-121.
Baig, F. Z., Khan, K., & Aslam, M. J. (2021). Child Rearing and Gender Socialisation: A Feminist CDA of Kids’ Popular Fictional Movies. Journal of Educational Research and Social Sciences Review (JERSSR) , 1 (3), 36-46.
Barker, M. E. (2021). Exploring Canadian Integration through CDA of English Language Lesson Plans for Immigrant Learners. Canadian Journal of Applied Linguistics/Revue canadienne de linguistique appliquée , 24 (1), 75-91. Doi: https://doi.org/10.37213/cjal.2021.28959
Coleman, B. (2017). An Ideological Unveiling: Using Critical Narrative and Discourse Analysis to Examine Discursive White Teacher Identity. AERA Online Paper Repository .
Drew, C. (2013). Soak up the goodness: Discourses of Australian childhoods on television advertisements, 2006-2012. PhD Dissertation: Australian Catholic University. Doi: https://doi.org/10.4226/66/5a9780223babd
Fairclough, N. (2013). Critical discourse analysis: The critical study of language . London: Routledge.
Foucault, M. (1990). The history of sexuality: An introduction . London: Vintage.
Foucault, M. (2003). Madness and civilization . New York: Routledge.
Hahn, A. D. (2018). Uncovering the ideologies of internationalization in lesson plans through CDA. The New English Teacher , 12 (1), 121-121.
Isti’anah, A. (2018). Rohingya in media: CDA of Myanmar and Bangladesh newspaper headlines. Language in the Online and Offline World , 6 , 18-23. Doi: http://repository.usd.ac.id/id/eprint/25962
Khan, M. H., Adnan, H. M., Kaur, S., Qazalbash, F., & Ismail, I. N. (2020). A CDA of anti-Muslim rhetoric in Donald Trump’s historic 2016 AIPAC policy speech. Journal of Muslim Minority Affairs , 40 (4), 543-558. Doi: https://doi.org/10.1080/13602004.2020.1828507
Louise Cooper, K., Luck, L., Chang, E., & Dixon, K. (2021). What is the practice of spiritual care? A CDA of registered nurses’ understanding of spirituality. Nursing Inquiry , 28 (2), e12385. Doi: https://doi.org/10.1111/nin.12385
Mohammadi, D., Momeni, S., & Labafi, S. (2021). Representation of Iranians family’s life style in TV advertising (Case study: food ads). Religion & Communication , 27 (58), 333-379.
Munro, M. (2018) House price inflation in the news: a CDA of newspaper coverage in the UK. Housing Studies, 33(7), pp. 1085-1105. doi: 10.1080/02673037.2017.1421911
Ravn, I. M., Frederiksen, K., & Beedholm, K. (2016). The chronic responsibility: a CDA of Danish chronic care policies. Qualitative Health Research , 26 (4), 545-554. Doi: https://doi.org/10.1177%2F1049732315570133
Sengul, K. (2019). Critical discourse analysis in political communication research: a case study of right-wing populist discourse in Australia. Communication Research and Practice , 5 (4), 376-392. Doi: https://doi.org/10.1080/22041451.2019.1695082
Serafis, D., Kitis, E. D., & Archakis, A. (2018). Graffiti slogans and the construction of collective identity: evidence from the anti-austerity protests in Greece. Text & Talk , 38 (6), 775-797. Doi: https://doi.org/10.1515/text-2018-0023
Suphaborwornrat, W., & Punkasirikul, P. (2022). A Multimodal CDA of Online Soft Drink Advertisements. LEARN Journal: Language Education and Acquisition Research Network , 15 (1), 627-653.
Symes, C., & Drew, C. (2017). Education on the rails: a textual ethnography of university advertising in mobile contexts. Critical Studies in Education , 58 (2), 205-223. Doi: https://doi.org/10.1080/17508487.2016.1252783
Thomas, S. (2005). The construction of teacher identities in educational policy documents: A critical discourse analysis. Critical Studies in Education , 46 (2), 25-44. Doi: https://doi.org/10.1080/17508480509556423
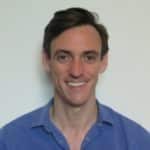
Chris Drew (PhD)
Dr. Chris Drew is the founder of the Helpful Professor. He holds a PhD in education and has published over 20 articles in scholarly journals. He is the former editor of the Journal of Learning Development in Higher Education. [Image Descriptor: Photo of Chris]
- Chris Drew (PhD) https://helpfulprofessor.com/author/chris-drew-phd/ 5 Top Tips for Succeeding at University
- Chris Drew (PhD) https://helpfulprofessor.com/author/chris-drew-phd/ 50 Durable Goods Examples
- Chris Drew (PhD) https://helpfulprofessor.com/author/chris-drew-phd/ 100 Consumer Goods Examples
- Chris Drew (PhD) https://helpfulprofessor.com/author/chris-drew-phd/ 30 Globalization Pros and Cons
Leave a Comment Cancel Reply
Your email address will not be published. Required fields are marked *
Have a language expert improve your writing
Run a free plagiarism check in 10 minutes, generate accurate citations for free.
- Knowledge Base
Methodology
- Content Analysis | Guide, Methods & Examples
Content Analysis | Guide, Methods & Examples
Published on July 18, 2019 by Amy Luo . Revised on June 22, 2023.
Content analysis is a research method used to identify patterns in recorded communication. To conduct content analysis, you systematically collect data from a set of texts, which can be written, oral, or visual:
- Books, newspapers and magazines
- Speeches and interviews
- Web content and social media posts
- Photographs and films
Content analysis can be both quantitative (focused on counting and measuring) and qualitative (focused on interpreting and understanding). In both types, you categorize or “code” words, themes, and concepts within the texts and then analyze the results.
Table of contents
What is content analysis used for, advantages of content analysis, disadvantages of content analysis, how to conduct content analysis, other interesting articles.
Researchers use content analysis to find out about the purposes, messages, and effects of communication content. They can also make inferences about the producers and audience of the texts they analyze.
Content analysis can be used to quantify the occurrence of certain words, phrases, subjects or concepts in a set of historical or contemporary texts.
Quantitative content analysis example
To research the importance of employment issues in political campaigns, you could analyze campaign speeches for the frequency of terms such as unemployment , jobs , and work and use statistical analysis to find differences over time or between candidates.
In addition, content analysis can be used to make qualitative inferences by analyzing the meaning and semantic relationship of words and concepts.
Qualitative content analysis example
To gain a more qualitative understanding of employment issues in political campaigns, you could locate the word unemployment in speeches, identify what other words or phrases appear next to it (such as economy, inequality or laziness ), and analyze the meanings of these relationships to better understand the intentions and targets of different campaigns.
Because content analysis can be applied to a broad range of texts, it is used in a variety of fields, including marketing, media studies, anthropology, cognitive science, psychology, and many social science disciplines. It has various possible goals:
- Finding correlations and patterns in how concepts are communicated
- Understanding the intentions of an individual, group or institution
- Identifying propaganda and bias in communication
- Revealing differences in communication in different contexts
- Analyzing the consequences of communication content, such as the flow of information or audience responses
Here's why students love Scribbr's proofreading services
Discover proofreading & editing
- Unobtrusive data collection
You can analyze communication and social interaction without the direct involvement of participants, so your presence as a researcher doesn’t influence the results.
- Transparent and replicable
When done well, content analysis follows a systematic procedure that can easily be replicated by other researchers, yielding results with high reliability .
- Highly flexible
You can conduct content analysis at any time, in any location, and at low cost – all you need is access to the appropriate sources.
Focusing on words or phrases in isolation can sometimes be overly reductive, disregarding context, nuance, and ambiguous meanings.
Content analysis almost always involves some level of subjective interpretation, which can affect the reliability and validity of the results and conclusions, leading to various types of research bias and cognitive bias .
- Time intensive
Manually coding large volumes of text is extremely time-consuming, and it can be difficult to automate effectively.
If you want to use content analysis in your research, you need to start with a clear, direct research question .
Example research question for content analysis
Is there a difference in how the US media represents younger politicians compared to older ones in terms of trustworthiness?
Next, you follow these five steps.
1. Select the content you will analyze
Based on your research question, choose the texts that you will analyze. You need to decide:
- The medium (e.g. newspapers, speeches or websites) and genre (e.g. opinion pieces, political campaign speeches, or marketing copy)
- The inclusion and exclusion criteria (e.g. newspaper articles that mention a particular event, speeches by a certain politician, or websites selling a specific type of product)
- The parameters in terms of date range, location, etc.
If there are only a small amount of texts that meet your criteria, you might analyze all of them. If there is a large volume of texts, you can select a sample .
2. Define the units and categories of analysis
Next, you need to determine the level at which you will analyze your chosen texts. This means defining:
- The unit(s) of meaning that will be coded. For example, are you going to record the frequency of individual words and phrases, the characteristics of people who produced or appear in the texts, the presence and positioning of images, or the treatment of themes and concepts?
- The set of categories that you will use for coding. Categories can be objective characteristics (e.g. aged 30-40 , lawyer , parent ) or more conceptual (e.g. trustworthy , corrupt , conservative , family oriented ).
Your units of analysis are the politicians who appear in each article and the words and phrases that are used to describe them. Based on your research question, you have to categorize based on age and the concept of trustworthiness. To get more detailed data, you also code for other categories such as their political party and the marital status of each politician mentioned.
3. Develop a set of rules for coding
Coding involves organizing the units of meaning into the previously defined categories. Especially with more conceptual categories, it’s important to clearly define the rules for what will and won’t be included to ensure that all texts are coded consistently.
Coding rules are especially important if multiple researchers are involved, but even if you’re coding all of the text by yourself, recording the rules makes your method more transparent and reliable.
In considering the category “younger politician,” you decide which titles will be coded with this category ( senator, governor, counselor, mayor ). With “trustworthy”, you decide which specific words or phrases related to trustworthiness (e.g. honest and reliable ) will be coded in this category.
4. Code the text according to the rules
You go through each text and record all relevant data in the appropriate categories. This can be done manually or aided with computer programs, such as QSR NVivo , Atlas.ti and Diction , which can help speed up the process of counting and categorizing words and phrases.
Following your coding rules, you examine each newspaper article in your sample. You record the characteristics of each politician mentioned, along with all words and phrases related to trustworthiness that are used to describe them.
5. Analyze the results and draw conclusions
Once coding is complete, the collected data is examined to find patterns and draw conclusions in response to your research question. You might use statistical analysis to find correlations or trends, discuss your interpretations of what the results mean, and make inferences about the creators, context and audience of the texts.
Let’s say the results reveal that words and phrases related to trustworthiness appeared in the same sentence as an older politician more frequently than they did in the same sentence as a younger politician. From these results, you conclude that national newspapers present older politicians as more trustworthy than younger politicians, and infer that this might have an effect on readers’ perceptions of younger people in politics.
If you want to know more about statistics , methodology , or research bias , make sure to check out some of our other articles with explanations and examples.
- Normal distribution
- Measures of central tendency
- Chi square tests
- Confidence interval
- Quartiles & Quantiles
- Cluster sampling
- Stratified sampling
- Thematic analysis
- Cohort study
- Peer review
- Ethnography
Research bias
- Implicit bias
- Cognitive bias
- Conformity bias
- Hawthorne effect
- Availability heuristic
- Attrition bias
- Social desirability bias
Cite this Scribbr article
If you want to cite this source, you can copy and paste the citation or click the “Cite this Scribbr article” button to automatically add the citation to our free Citation Generator.
Luo, A. (2023, June 22). Content Analysis | Guide, Methods & Examples. Scribbr. Retrieved April 2, 2024, from https://www.scribbr.com/methodology/content-analysis/
Is this article helpful?
Other students also liked
Qualitative vs. quantitative research | differences, examples & methods, descriptive research | definition, types, methods & examples, reliability vs. validity in research | difference, types and examples, what is your plagiarism score.
- Privacy Policy
Buy Me a Coffee

Home » Discourse Analysis – Methods, Types and Examples
Discourse Analysis – Methods, Types and Examples
Table of Contents

Discourse Analysis
Definition:
Discourse Analysis is a method of studying how people use language in different situations to understand what they really mean and what messages they are sending. It helps us understand how language is used to create social relationships and cultural norms.
It examines language use in various forms of communication such as spoken, written, visual or multi-modal texts, and focuses on how language is used to construct social meaning and relationships, and how it reflects and reinforces power dynamics, ideologies, and cultural norms.
Types of Discourse Analysis
Some of the most common types of discourse analysis are:
Conversation Analysis
This type of discourse analysis focuses on analyzing the structure of talk and how participants in a conversation make meaning through their interaction. It is often used to study face-to-face interactions, such as interviews or everyday conversations.
Critical discourse Analysis
This approach focuses on the ways in which language use reflects and reinforces power relations, social hierarchies, and ideologies. It is often used to analyze media texts or political speeches, with the aim of uncovering the hidden meanings and assumptions that are embedded in these texts.
Discursive Psychology
This type of discourse analysis focuses on the ways in which language use is related to psychological processes such as identity construction and attribution of motives. It is often used to study narratives or personal accounts, with the aim of understanding how individuals make sense of their experiences.
Multimodal Discourse Analysis
This approach focuses on analyzing not only language use, but also other modes of communication, such as images, gestures, and layout. It is often used to study digital or visual media, with the aim of understanding how different modes of communication work together to create meaning.
Corpus-based Discourse Analysis
This type of discourse analysis uses large collections of texts, or corpora, to analyze patterns of language use across different genres or contexts. It is often used to study language use in specific domains, such as academic writing or legal discourse.
Descriptive Discourse
This type of discourse analysis aims to describe the features and characteristics of language use, without making any value judgments or interpretations. It is often used in linguistic studies to describe grammatical structures or phonetic features of language.
Narrative Discourse
This approach focuses on analyzing the structure and content of stories or narratives, with the aim of understanding how they are constructed and how they shape our understanding of the world. It is often used to study personal narratives or cultural myths.
Expository Discourse
This type of discourse analysis is used to study texts that explain or describe a concept, process, or idea. It aims to understand how information is organized and presented in such texts and how it influences the reader’s understanding of the topic.
Argumentative Discourse
This approach focuses on analyzing texts that present an argument or attempt to persuade the reader or listener. It aims to understand how the argument is constructed, what strategies are used to persuade, and how the audience is likely to respond to the argument.
Discourse Analysis Conducting Guide
Here is a step-by-step guide for conducting discourse analysis:
- What are you trying to understand about the language use in a particular context?
- What are the key concepts or themes that you want to explore?
- Select the data: Decide on the type of data that you will analyze, such as written texts, spoken conversations, or media content. Consider the source of the data, such as news articles, interviews, or social media posts, and how this might affect your analysis.
- Transcribe or collect the data: If you are analyzing spoken language, you will need to transcribe the data into written form. If you are using written texts, make sure that you have access to the full text and that it is in a format that can be easily analyzed.
- Read and re-read the data: Read through the data carefully, paying attention to key themes, patterns, and discursive features. Take notes on what stands out to you and make preliminary observations about the language use.
- Develop a coding scheme : Develop a coding scheme that will allow you to categorize and organize different types of language use. This might include categories such as metaphors, narratives, or persuasive strategies, depending on your research question.
- Code the data: Use your coding scheme to analyze the data, coding different sections of text or spoken language according to the categories that you have developed. This can be a time-consuming process, so consider using software tools to assist with coding and analysis.
- Analyze the data: Once you have coded the data, analyze it to identify patterns and themes that emerge. Look for similarities and differences across different parts of the data, and consider how different categories of language use are related to your research question.
- Interpret the findings: Draw conclusions from your analysis and interpret the findings in relation to your research question. Consider how the language use in your data sheds light on broader cultural or social issues, and what implications it might have for understanding language use in other contexts.
- Write up the results: Write up your findings in a clear and concise way, using examples from the data to support your arguments. Consider how your research contributes to the broader field of discourse analysis and what implications it might have for future research.
Applications of Discourse Analysis
Here are some of the key areas where discourse analysis is commonly used:
- Political discourse: Discourse analysis can be used to analyze political speeches, debates, and media coverage of political events. By examining the language used in these contexts, researchers can gain insight into the political ideologies, values, and agendas that underpin different political positions.
- Media analysis: Discourse analysis is frequently used to analyze media content, including news reports, television shows, and social media posts. By examining the language used in media content, researchers can understand how media narratives are constructed and how they influence public opinion.
- Education : Discourse analysis can be used to examine classroom discourse, student-teacher interactions, and educational policies. By analyzing the language used in these contexts, researchers can gain insight into the social and cultural factors that shape educational outcomes.
- Healthcare : Discourse analysis is used in healthcare to examine the language used by healthcare professionals and patients in medical consultations. This can help to identify communication barriers, cultural differences, and other factors that may impact the quality of healthcare.
- Marketing and advertising: Discourse analysis can be used to analyze marketing and advertising messages, including the language used in product descriptions, slogans, and commercials. By examining these messages, researchers can gain insight into the cultural values and beliefs that underpin consumer behavior.
When to use Discourse Analysis
Discourse analysis is a valuable research methodology that can be used in a variety of contexts. Here are some situations where discourse analysis may be particularly useful:
- When studying language use in a particular context: Discourse analysis can be used to examine how language is used in a specific context, such as political speeches, media coverage, or healthcare interactions. By analyzing language use in these contexts, researchers can gain insight into the social and cultural factors that shape communication.
- When exploring the meaning of language: Discourse analysis can be used to examine how language is used to construct meaning and shape social reality. This can be particularly useful in fields such as sociology, anthropology, and cultural studies.
- When examining power relations: Discourse analysis can be used to examine how language is used to reinforce or challenge power relations in society. By analyzing language use in contexts such as political discourse, media coverage, or workplace interactions, researchers can gain insight into how power is negotiated and maintained.
- When conducting qualitative research: Discourse analysis can be used as a qualitative research method, allowing researchers to explore complex social phenomena in depth. By analyzing language use in a particular context, researchers can gain rich and nuanced insights into the social and cultural factors that shape communication.
Examples of Discourse Analysis
Here are some examples of discourse analysis in action:
- A study of media coverage of climate change: This study analyzed media coverage of climate change to examine how language was used to construct the issue. The researchers found that media coverage tended to frame climate change as a matter of scientific debate rather than a pressing environmental issue, thereby undermining public support for action on climate change.
- A study of political speeches: This study analyzed political speeches to examine how language was used to construct political identity. The researchers found that politicians used language strategically to construct themselves as trustworthy and competent leaders, while painting their opponents as untrustworthy and incompetent.
- A study of medical consultations: This study analyzed medical consultations to examine how language was used to negotiate power and authority between doctors and patients. The researchers found that doctors used language to assert their authority and control over medical decisions, while patients used language to negotiate their own preferences and concerns.
- A study of workplace interactions: This study analyzed workplace interactions to examine how language was used to construct social identity and maintain power relations. The researchers found that language was used to construct a hierarchy of power and status within the workplace, with those in positions of authority using language to assert their dominance over subordinates.
Purpose of Discourse Analysis
The purpose of discourse analysis is to examine the ways in which language is used to construct social meaning, relationships, and power relations. By analyzing language use in a systematic and rigorous way, discourse analysis can provide valuable insights into the social and cultural factors that shape communication and interaction.
The specific purposes of discourse analysis may vary depending on the research context, but some common goals include:
- To understand how language constructs social reality: Discourse analysis can help researchers understand how language is used to construct meaning and shape social reality. By analyzing language use in a particular context, researchers can gain insight into the cultural and social factors that shape communication.
- To identify power relations: Discourse analysis can be used to examine how language use reinforces or challenges power relations in society. By analyzing language use in contexts such as political discourse, media coverage, or workplace interactions, researchers can gain insight into how power is negotiated and maintained.
- To explore social and cultural norms: Discourse analysis can help researchers understand how social and cultural norms are constructed and maintained through language use. By analyzing language use in different contexts, researchers can gain insight into how social and cultural norms are reproduced and challenged.
- To provide insights for social change: Discourse analysis can provide insights that can be used to promote social change. By identifying problematic language use or power imbalances, researchers can provide insights that can be used to challenge social norms and promote more equitable and inclusive communication.
Characteristics of Discourse Analysis
Here are some key characteristics of discourse analysis:
- Focus on language use: Discourse analysis is centered on language use and how it constructs social meaning, relationships, and power relations.
- Multidisciplinary approach: Discourse analysis draws on theories and methodologies from a range of disciplines, including linguistics, anthropology, sociology, and psychology.
- Systematic and rigorous methodology: Discourse analysis employs a systematic and rigorous methodology, often involving transcription and coding of language data, in order to identify patterns and themes in language use.
- Contextual analysis : Discourse analysis emphasizes the importance of context in shaping language use, and takes into account the social and cultural factors that shape communication.
- Focus on power relations: Discourse analysis often examines power relations and how language use reinforces or challenges power imbalances in society.
- Interpretive approach: Discourse analysis is an interpretive approach, meaning that it seeks to understand the meaning and significance of language use from the perspective of the participants in a particular discourse.
- Emphasis on reflexivity: Discourse analysis emphasizes the importance of reflexivity, or self-awareness, in the research process. Researchers are encouraged to reflect on their own positionality and how it may shape their interpretation of language use.
Advantages of Discourse Analysis
Discourse analysis has several advantages as a methodological approach. Here are some of the main advantages:
- Provides a detailed understanding of language use: Discourse analysis allows for a detailed and nuanced understanding of language use in specific social contexts. It enables researchers to identify patterns and themes in language use, and to understand how language constructs social reality.
- Emphasizes the importance of context : Discourse analysis emphasizes the importance of context in shaping language use. By taking into account the social and cultural factors that shape communication, discourse analysis provides a more complete understanding of language use than other approaches.
- Allows for an examination of power relations: Discourse analysis enables researchers to examine power relations and how language use reinforces or challenges power imbalances in society. By identifying problematic language use, discourse analysis can contribute to efforts to promote social justice and equality.
- Provides insights for social change: Discourse analysis can provide insights that can be used to promote social change. By identifying problematic language use or power imbalances, researchers can provide insights that can be used to challenge social norms and promote more equitable and inclusive communication.
- Multidisciplinary approach: Discourse analysis draws on theories and methodologies from a range of disciplines, including linguistics, anthropology, sociology, and psychology. This multidisciplinary approach allows for a more holistic understanding of language use in social contexts.
Limitations of Discourse Analysis
Some Limitations of Discourse Analysis are as follows:
- Time-consuming and resource-intensive: Discourse analysis can be a time-consuming and resource-intensive process. Collecting and transcribing language data can be a time-consuming task, and analyzing the data requires careful attention to detail and a significant investment of time and resources.
- Limited generalizability: Discourse analysis is often focused on a particular social context or community, and therefore the findings may not be easily generalized to other contexts or populations. This means that the insights gained from discourse analysis may have limited applicability beyond the specific context being studied.
- Interpretive nature: Discourse analysis is an interpretive approach, meaning that it relies on the interpretation of the researcher to identify patterns and themes in language use. This subjectivity can be a limitation, as different researchers may interpret language data differently.
- Limited quantitative analysis: Discourse analysis tends to focus on qualitative analysis of language data, which can limit the ability to draw statistical conclusions or make quantitative comparisons across different language uses or contexts.
- Ethical considerations: Discourse analysis may involve the collection and analysis of sensitive language data, such as language related to trauma or marginalization. Researchers must carefully consider the ethical implications of collecting and analyzing this type of data, and ensure that the privacy and confidentiality of participants is protected.
About the author
Muhammad Hassan
Researcher, Academic Writer, Web developer
You may also like

Cluster Analysis – Types, Methods and Examples

Discriminant Analysis – Methods, Types and...

MANOVA (Multivariate Analysis of Variance) –...

Documentary Analysis – Methods, Applications and...

ANOVA (Analysis of variance) – Formulas, Types...

Graphical Methods – Types, Examples and Guide
Have a language expert improve your writing
Run a free plagiarism check in 10 minutes, automatically generate references for free.
- Knowledge Base
- Methodology
- Critical Discourse Analysis | Definition, Guide & Examples
Critical Discourse Analysis | Definition, Guide & Examples
Published on 5 May 2022 by Amy Luo . Revised on 5 December 2022.
Discourse analysis is a research method for studying written or spoken language in relation to its social context. It aims to understand how language is used in real-life situations.
When you do discourse analysis, you might focus on:
- The purposes and effects of different types of language
- Cultural rules and conventions in communication
- How values, beliefs, and assumptions are communicated
- How language use relates to its social, political, and historical context
Discourse analysis is a common qualitative research method in many humanities and social science disciplines, including linguistics, sociology, anthropology, psychology, and cultural studies. It is also called critical discourse analysis.
Table of contents
What is discourse analysis used for, how is discourse analysis different from other methods, how to conduct discourse analysis.
Conducting discourse analysis means examining how language functions and how meaning is created in different social contexts. It can be applied to any instance of written or oral language, as well as non-verbal aspects of communication, such as tone and gestures.
Materials that are suitable for discourse analysis include:
- Books, newspapers, and periodicals
- Marketing material, such as brochures and advertisements
- Business and government documents
- Websites, forums, social media posts, and comments
- Interviews and conversations
By analysing these types of discourse, researchers aim to gain an understanding of social groups and how they communicate.
Prevent plagiarism, run a free check.
Unlike linguistic approaches that focus only on the rules of language use, discourse analysis emphasises the contextual meaning of language.
It focuses on the social aspects of communication and the ways people use language to achieve specific effects (e.g., to build trust, to create doubt, to evoke emotions, or to manage conflict).
Instead of focusing on smaller units of language, such as sounds, words, or phrases, discourse analysis is used to study larger chunks of language, such as entire conversations, texts, or collections of texts. The selected sources can be analysed on multiple levels.
Discourse analysis is a qualitative and interpretive method of analysing texts (in contrast to more systematic methods like content analysis ). You make interpretations based on both the details of the material itself and on contextual knowledge.
There are many different approaches and techniques you can use to conduct discourse analysis, but the steps below outline the basic structure you need to follow.
Step 1: Define the research question and select the content of analysis
To do discourse analysis, you begin with a clearly defined research question . Once you have developed your question, select a range of material that is appropriate to answer it.
Discourse analysis is a method that can be applied both to large volumes of material and to smaller samples, depending on the aims and timescale of your research.
Step 2: Gather information and theory on the context
Next, you must establish the social and historical context in which the material was produced and intended to be received. Gather factual details of when and where the content was created, who the author is, who published it, and whom it was disseminated to.
As well as understanding the real-life context of the discourse, you can also conduct a literature review on the topic and construct a theoretical framework to guide your analysis.
Step 3: Analyse the content for themes and patterns
This step involves closely examining various elements of the material – such as words, sentences, paragraphs, and overall structure – and relating them to attributes, themes, and patterns relevant to your research question.
Step 4: Review your results and draw conclusions
Once you have assigned particular attributes to elements of the material, reflect on your results to examine the function and meaning of the language used. Here, you will consider your analysis in relation to the broader context that you established earlier to draw conclusions that answer your research question.
Cite this Scribbr article
If you want to cite this source, you can copy and paste the citation or click the ‘Cite this Scribbr article’ button to automatically add the citation to our free Reference Generator.
Luo, A. (2022, December 05). Critical Discourse Analysis | Definition, Guide & Examples. Scribbr. Retrieved 2 April 2024, from https://www.scribbr.co.uk/research-methods/discourse-analysis-explained/
Is this article helpful?
Other students also liked
Case study | definition, examples & methods, how to do thematic analysis | guide & examples, content analysis | a step-by-step guide with examples.
- Search Menu
- Browse content in Arts and Humanities
- Browse content in Archaeology
- Anglo-Saxon and Medieval Archaeology
- Archaeological Methodology and Techniques
- Archaeology by Region
- Archaeology of Religion
- Archaeology of Trade and Exchange
- Biblical Archaeology
- Contemporary and Public Archaeology
- Environmental Archaeology
- Historical Archaeology
- History and Theory of Archaeology
- Industrial Archaeology
- Landscape Archaeology
- Mortuary Archaeology
- Prehistoric Archaeology
- Underwater Archaeology
- Urban Archaeology
- Zooarchaeology
- Browse content in Architecture
- Architectural Structure and Design
- History of Architecture
- Residential and Domestic Buildings
- Theory of Architecture
- Browse content in Art
- Art Subjects and Themes
- History of Art
- Industrial and Commercial Art
- Theory of Art
- Biographical Studies
- Byzantine Studies
- Browse content in Classical Studies
- Classical History
- Classical Philosophy
- Classical Mythology
- Classical Literature
- Classical Reception
- Classical Art and Architecture
- Classical Oratory and Rhetoric
- Greek and Roman Papyrology
- Greek and Roman Epigraphy
- Greek and Roman Law
- Greek and Roman Archaeology
- Late Antiquity
- Religion in the Ancient World
- Digital Humanities
- Browse content in History
- Colonialism and Imperialism
- Diplomatic History
- Environmental History
- Genealogy, Heraldry, Names, and Honours
- Genocide and Ethnic Cleansing
- Historical Geography
- History by Period
- History of Emotions
- History of Agriculture
- History of Education
- History of Gender and Sexuality
- Industrial History
- Intellectual History
- International History
- Labour History
- Legal and Constitutional History
- Local and Family History
- Maritime History
- Military History
- National Liberation and Post-Colonialism
- Oral History
- Political History
- Public History
- Regional and National History
- Revolutions and Rebellions
- Slavery and Abolition of Slavery
- Social and Cultural History
- Theory, Methods, and Historiography
- Urban History
- World History
- Browse content in Language Teaching and Learning
- Language Learning (Specific Skills)
- Language Teaching Theory and Methods
- Browse content in Linguistics
- Applied Linguistics
- Cognitive Linguistics
- Computational Linguistics
- Forensic Linguistics
- Grammar, Syntax and Morphology
- Historical and Diachronic Linguistics
- History of English
- Language Evolution
- Language Reference
- Language Acquisition
- Language Variation
- Language Families
- Lexicography
- Linguistic Anthropology
- Linguistic Theories
- Linguistic Typology
- Phonetics and Phonology
- Psycholinguistics
- Sociolinguistics
- Translation and Interpretation
- Writing Systems
- Browse content in Literature
- Bibliography
- Children's Literature Studies
- Literary Studies (Romanticism)
- Literary Studies (American)
- Literary Studies (Asian)
- Literary Studies (European)
- Literary Studies (Eco-criticism)
- Literary Studies (Modernism)
- Literary Studies - World
- Literary Studies (1500 to 1800)
- Literary Studies (19th Century)
- Literary Studies (20th Century onwards)
- Literary Studies (African American Literature)
- Literary Studies (British and Irish)
- Literary Studies (Early and Medieval)
- Literary Studies (Fiction, Novelists, and Prose Writers)
- Literary Studies (Gender Studies)
- Literary Studies (Graphic Novels)
- Literary Studies (History of the Book)
- Literary Studies (Plays and Playwrights)
- Literary Studies (Poetry and Poets)
- Literary Studies (Postcolonial Literature)
- Literary Studies (Queer Studies)
- Literary Studies (Science Fiction)
- Literary Studies (Travel Literature)
- Literary Studies (War Literature)
- Literary Studies (Women's Writing)
- Literary Theory and Cultural Studies
- Mythology and Folklore
- Shakespeare Studies and Criticism
- Browse content in Media Studies
- Browse content in Music
- Applied Music
- Dance and Music
- Ethics in Music
- Ethnomusicology
- Gender and Sexuality in Music
- Medicine and Music
- Music Cultures
- Music and Media
- Music and Religion
- Music and Culture
- Music Education and Pedagogy
- Music Theory and Analysis
- Musical Scores, Lyrics, and Libretti
- Musical Structures, Styles, and Techniques
- Musicology and Music History
- Performance Practice and Studies
- Race and Ethnicity in Music
- Sound Studies
- Browse content in Performing Arts
- Browse content in Philosophy
- Aesthetics and Philosophy of Art
- Epistemology
- Feminist Philosophy
- History of Western Philosophy
- Metaphysics
- Moral Philosophy
- Non-Western Philosophy
- Philosophy of Language
- Philosophy of Mind
- Philosophy of Perception
- Philosophy of Science
- Philosophy of Action
- Philosophy of Law
- Philosophy of Religion
- Philosophy of Mathematics and Logic
- Practical Ethics
- Social and Political Philosophy
- Browse content in Religion
- Biblical Studies
- Christianity
- East Asian Religions
- History of Religion
- Judaism and Jewish Studies
- Qumran Studies
- Religion and Education
- Religion and Health
- Religion and Politics
- Religion and Science
- Religion and Law
- Religion and Art, Literature, and Music
- Religious Studies
- Browse content in Society and Culture
- Cookery, Food, and Drink
- Cultural Studies
- Customs and Traditions
- Ethical Issues and Debates
- Hobbies, Games, Arts and Crafts
- Lifestyle, Home, and Garden
- Natural world, Country Life, and Pets
- Popular Beliefs and Controversial Knowledge
- Sports and Outdoor Recreation
- Technology and Society
- Travel and Holiday
- Visual Culture
- Browse content in Law
- Arbitration
- Browse content in Company and Commercial Law
- Commercial Law
- Company Law
- Browse content in Comparative Law
- Systems of Law
- Competition Law
- Browse content in Constitutional and Administrative Law
- Government Powers
- Judicial Review
- Local Government Law
- Military and Defence Law
- Parliamentary and Legislative Practice
- Construction Law
- Contract Law
- Browse content in Criminal Law
- Criminal Procedure
- Criminal Evidence Law
- Sentencing and Punishment
- Employment and Labour Law
- Environment and Energy Law
- Browse content in Financial Law
- Banking Law
- Insolvency Law
- History of Law
- Human Rights and Immigration
- Intellectual Property Law
- Browse content in International Law
- Private International Law and Conflict of Laws
- Public International Law
- IT and Communications Law
- Jurisprudence and Philosophy of Law
- Law and Politics
- Law and Society
- Browse content in Legal System and Practice
- Courts and Procedure
- Legal Skills and Practice
- Primary Sources of Law
- Regulation of Legal Profession
- Medical and Healthcare Law
- Browse content in Policing
- Criminal Investigation and Detection
- Police and Security Services
- Police Procedure and Law
- Police Regional Planning
- Browse content in Property Law
- Personal Property Law
- Study and Revision
- Terrorism and National Security Law
- Browse content in Trusts Law
- Wills and Probate or Succession
- Browse content in Medicine and Health
- Browse content in Allied Health Professions
- Arts Therapies
- Clinical Science
- Dietetics and Nutrition
- Occupational Therapy
- Operating Department Practice
- Physiotherapy
- Radiography
- Speech and Language Therapy
- Browse content in Anaesthetics
- General Anaesthesia
- Neuroanaesthesia
- Clinical Neuroscience
- Browse content in Clinical Medicine
- Acute Medicine
- Cardiovascular Medicine
- Clinical Genetics
- Clinical Pharmacology and Therapeutics
- Dermatology
- Endocrinology and Diabetes
- Gastroenterology
- Genito-urinary Medicine
- Geriatric Medicine
- Infectious Diseases
- Medical Toxicology
- Medical Oncology
- Pain Medicine
- Palliative Medicine
- Rehabilitation Medicine
- Respiratory Medicine and Pulmonology
- Rheumatology
- Sleep Medicine
- Sports and Exercise Medicine
- Community Medical Services
- Critical Care
- Emergency Medicine
- Forensic Medicine
- Haematology
- History of Medicine
- Browse content in Medical Skills
- Clinical Skills
- Communication Skills
- Nursing Skills
- Surgical Skills
- Browse content in Medical Dentistry
- Oral and Maxillofacial Surgery
- Paediatric Dentistry
- Restorative Dentistry and Orthodontics
- Surgical Dentistry
- Medical Ethics
- Medical Statistics and Methodology
- Browse content in Neurology
- Clinical Neurophysiology
- Neuropathology
- Nursing Studies
- Browse content in Obstetrics and Gynaecology
- Gynaecology
- Occupational Medicine
- Ophthalmology
- Otolaryngology (ENT)
- Browse content in Paediatrics
- Neonatology
- Browse content in Pathology
- Chemical Pathology
- Clinical Cytogenetics and Molecular Genetics
- Histopathology
- Medical Microbiology and Virology
- Patient Education and Information
- Browse content in Pharmacology
- Psychopharmacology
- Browse content in Popular Health
- Caring for Others
- Complementary and Alternative Medicine
- Self-help and Personal Development
- Browse content in Preclinical Medicine
- Cell Biology
- Molecular Biology and Genetics
- Reproduction, Growth and Development
- Primary Care
- Professional Development in Medicine
- Browse content in Psychiatry
- Addiction Medicine
- Child and Adolescent Psychiatry
- Forensic Psychiatry
- Learning Disabilities
- Old Age Psychiatry
- Psychotherapy
- Browse content in Public Health and Epidemiology
- Epidemiology
- Public Health
- Browse content in Radiology
- Clinical Radiology
- Interventional Radiology
- Nuclear Medicine
- Radiation Oncology
- Reproductive Medicine
- Browse content in Surgery
- Cardiothoracic Surgery
- Gastro-intestinal and Colorectal Surgery
- General Surgery
- Neurosurgery
- Paediatric Surgery
- Peri-operative Care
- Plastic and Reconstructive Surgery
- Surgical Oncology
- Transplant Surgery
- Trauma and Orthopaedic Surgery
- Vascular Surgery
- Browse content in Science and Mathematics
- Browse content in Biological Sciences
- Aquatic Biology
- Biochemistry
- Bioinformatics and Computational Biology
- Developmental Biology
- Ecology and Conservation
- Evolutionary Biology
- Genetics and Genomics
- Microbiology
- Molecular and Cell Biology
- Natural History
- Plant Sciences and Forestry
- Research Methods in Life Sciences
- Structural Biology
- Systems Biology
- Zoology and Animal Sciences
- Browse content in Chemistry
- Analytical Chemistry
- Computational Chemistry
- Crystallography
- Environmental Chemistry
- Industrial Chemistry
- Inorganic Chemistry
- Materials Chemistry
- Medicinal Chemistry
- Mineralogy and Gems
- Organic Chemistry
- Physical Chemistry
- Polymer Chemistry
- Study and Communication Skills in Chemistry
- Theoretical Chemistry
- Browse content in Computer Science
- Artificial Intelligence
- Computer Architecture and Logic Design
- Game Studies
- Human-Computer Interaction
- Mathematical Theory of Computation
- Programming Languages
- Software Engineering
- Systems Analysis and Design
- Virtual Reality
- Browse content in Computing
- Business Applications
- Computer Security
- Computer Games
- Computer Networking and Communications
- Digital Lifestyle
- Graphical and Digital Media Applications
- Operating Systems
- Browse content in Earth Sciences and Geography
- Atmospheric Sciences
- Environmental Geography
- Geology and the Lithosphere
- Maps and Map-making
- Meteorology and Climatology
- Oceanography and Hydrology
- Palaeontology
- Physical Geography and Topography
- Regional Geography
- Soil Science
- Urban Geography
- Browse content in Engineering and Technology
- Agriculture and Farming
- Biological Engineering
- Civil Engineering, Surveying, and Building
- Electronics and Communications Engineering
- Energy Technology
- Engineering (General)
- Environmental Science, Engineering, and Technology
- History of Engineering and Technology
- Mechanical Engineering and Materials
- Technology of Industrial Chemistry
- Transport Technology and Trades
- Browse content in Environmental Science
- Applied Ecology (Environmental Science)
- Conservation of the Environment (Environmental Science)
- Environmental Sustainability
- Environmentalist Thought and Ideology (Environmental Science)
- Management of Land and Natural Resources (Environmental Science)
- Natural Disasters (Environmental Science)
- Nuclear Issues (Environmental Science)
- Pollution and Threats to the Environment (Environmental Science)
- Social Impact of Environmental Issues (Environmental Science)
- History of Science and Technology
- Browse content in Materials Science
- Ceramics and Glasses
- Composite Materials
- Metals, Alloying, and Corrosion
- Nanotechnology
- Browse content in Mathematics
- Applied Mathematics
- Biomathematics and Statistics
- History of Mathematics
- Mathematical Education
- Mathematical Finance
- Mathematical Analysis
- Numerical and Computational Mathematics
- Probability and Statistics
- Pure Mathematics
- Browse content in Neuroscience
- Cognition and Behavioural Neuroscience
- Development of the Nervous System
- Disorders of the Nervous System
- History of Neuroscience
- Invertebrate Neurobiology
- Molecular and Cellular Systems
- Neuroendocrinology and Autonomic Nervous System
- Neuroscientific Techniques
- Sensory and Motor Systems
- Browse content in Physics
- Astronomy and Astrophysics
- Atomic, Molecular, and Optical Physics
- Biological and Medical Physics
- Classical Mechanics
- Computational Physics
- Condensed Matter Physics
- Electromagnetism, Optics, and Acoustics
- History of Physics
- Mathematical and Statistical Physics
- Measurement Science
- Nuclear Physics
- Particles and Fields
- Plasma Physics
- Quantum Physics
- Relativity and Gravitation
- Semiconductor and Mesoscopic Physics
- Browse content in Psychology
- Affective Sciences
- Clinical Psychology
- Cognitive Psychology
- Cognitive Neuroscience
- Criminal and Forensic Psychology
- Developmental Psychology
- Educational Psychology
- Evolutionary Psychology
- Health Psychology
- History and Systems in Psychology
- Music Psychology
- Neuropsychology
- Organizational Psychology
- Psychological Assessment and Testing
- Psychology of Human-Technology Interaction
- Psychology Professional Development and Training
- Research Methods in Psychology
- Social Psychology
- Browse content in Social Sciences
- Browse content in Anthropology
- Anthropology of Religion
- Human Evolution
- Medical Anthropology
- Physical Anthropology
- Regional Anthropology
- Social and Cultural Anthropology
- Theory and Practice of Anthropology
- Browse content in Business and Management
- Business Ethics
- Business Strategy
- Business History
- Business and Technology
- Business and Government
- Business and the Environment
- Comparative Management
- Corporate Governance
- Corporate Social Responsibility
- Entrepreneurship
- Health Management
- Human Resource Management
- Industrial and Employment Relations
- Industry Studies
- Information and Communication Technologies
- International Business
- Knowledge Management
- Management and Management Techniques
- Operations Management
- Organizational Theory and Behaviour
- Pensions and Pension Management
- Public and Nonprofit Management
- Strategic Management
- Supply Chain Management
- Browse content in Criminology and Criminal Justice
- Criminal Justice
- Criminology
- Forms of Crime
- International and Comparative Criminology
- Youth Violence and Juvenile Justice
- Development Studies
- Browse content in Economics
- Agricultural, Environmental, and Natural Resource Economics
- Asian Economics
- Behavioural Finance
- Behavioural Economics and Neuroeconomics
- Econometrics and Mathematical Economics
- Economic History
- Economic Systems
- Economic Methodology
- Economic Development and Growth
- Financial Markets
- Financial Institutions and Services
- General Economics and Teaching
- Health, Education, and Welfare
- History of Economic Thought
- International Economics
- Labour and Demographic Economics
- Law and Economics
- Macroeconomics and Monetary Economics
- Microeconomics
- Public Economics
- Urban, Rural, and Regional Economics
- Welfare Economics
- Browse content in Education
- Adult Education and Continuous Learning
- Care and Counselling of Students
- Early Childhood and Elementary Education
- Educational Equipment and Technology
- Educational Strategies and Policy
- Higher and Further Education
- Organization and Management of Education
- Philosophy and Theory of Education
- Schools Studies
- Secondary Education
- Teaching of a Specific Subject
- Teaching of Specific Groups and Special Educational Needs
- Teaching Skills and Techniques
- Browse content in Environment
- Applied Ecology (Social Science)
- Climate Change
- Conservation of the Environment (Social Science)
- Environmentalist Thought and Ideology (Social Science)
- Natural Disasters (Environment)
- Social Impact of Environmental Issues (Social Science)
- Browse content in Human Geography
- Cultural Geography
- Economic Geography
- Political Geography
- Browse content in Interdisciplinary Studies
- Communication Studies
- Museums, Libraries, and Information Sciences
- Browse content in Politics
- African Politics
- Asian Politics
- Chinese Politics
- Comparative Politics
- Conflict Politics
- Elections and Electoral Studies
- Environmental Politics
- European Union
- Foreign Policy
- Gender and Politics
- Human Rights and Politics
- Indian Politics
- International Relations
- International Organization (Politics)
- International Political Economy
- Irish Politics
- Latin American Politics
- Middle Eastern Politics
- Political Behaviour
- Political Economy
- Political Institutions
- Political Methodology
- Political Communication
- Political Philosophy
- Political Sociology
- Political Theory
- Politics and Law
- Public Policy
- Public Administration
- Quantitative Political Methodology
- Regional Political Studies
- Russian Politics
- Security Studies
- State and Local Government
- UK Politics
- US Politics
- Browse content in Regional and Area Studies
- African Studies
- Asian Studies
- East Asian Studies
- Japanese Studies
- Latin American Studies
- Middle Eastern Studies
- Native American Studies
- Scottish Studies
- Browse content in Research and Information
- Research Methods
- Browse content in Social Work
- Addictions and Substance Misuse
- Adoption and Fostering
- Care of the Elderly
- Child and Adolescent Social Work
- Couple and Family Social Work
- Developmental and Physical Disabilities Social Work
- Direct Practice and Clinical Social Work
- Emergency Services
- Human Behaviour and the Social Environment
- International and Global Issues in Social Work
- Mental and Behavioural Health
- Social Justice and Human Rights
- Social Policy and Advocacy
- Social Work and Crime and Justice
- Social Work Macro Practice
- Social Work Practice Settings
- Social Work Research and Evidence-based Practice
- Welfare and Benefit Systems
- Browse content in Sociology
- Childhood Studies
- Community Development
- Comparative and Historical Sociology
- Economic Sociology
- Gender and Sexuality
- Gerontology and Ageing
- Health, Illness, and Medicine
- Marriage and the Family
- Migration Studies
- Occupations, Professions, and Work
- Organizations
- Population and Demography
- Race and Ethnicity
- Social Theory
- Social Movements and Social Change
- Social Research and Statistics
- Social Stratification, Inequality, and Mobility
- Sociology of Religion
- Sociology of Education
- Sport and Leisure
- Urban and Rural Studies
- Browse content in Warfare and Defence
- Defence Strategy, Planning, and Research
- Land Forces and Warfare
- Military Administration
- Military Life and Institutions
- Naval Forces and Warfare
- Other Warfare and Defence Issues
- Peace Studies and Conflict Resolution
- Weapons and Equipment

- < Previous chapter
- Next chapter >

19 Content Analysis
Lindsay Prior, School of Sociology, Social Policy, and Social Work, Queen's University
- Published: 02 September 2020
- Cite Icon Cite
- Permissions Icon Permissions
In this chapter, the focus is on ways in which content analysis can be used to investigate and describe interview and textual data. The chapter opens with a contextualization of the method and then proceeds to an examination of the role of content analysis in relation to both quantitative and qualitative modes of social research. Following the introductory sections, four kinds of data are subjected to content analysis. These include data derived from a sample of qualitative interviews ( N = 54), textual data derived from a sample of health policy documents ( N = 6), data derived from a single interview relating to a “case” of traumatic brain injury, and data gathered from fifty-four abstracts of academic papers on the topic of “well-being.” Using a distinctive and somewhat novel style of content analysis that calls on the notion of semantic networks, the chapter shows how the method can be used either independently or in conjunction with other forms of inquiry (including various styles of discourse analysis) to analyze data and also how it can be used to verify and underpin claims that arise from analysis. The chapter ends with an overview of the different ways in which the study of “content”—especially the study of document content—can be positioned in social scientific research projects.
What Is Content Analysis?
In his 1952 text on the subject of content analysis, Bernard Berelson traced the origins of the method to communication research and then listed what he called six distinguishing features of the approach. As one might expect, the six defining features reflect the concerns of social science as taught in the 1950s, an age in which the calls for an “objective,” “systematic,” and “quantitative” approach to the study of communication data were first heard. The reference to the field of “communication” was nothing less than a reflection of a substantive social scientific interest over the previous decades in what was called public opinion and specifically attempts to understand why and how a potential source of critical, rational judgment on political leaders (i.e., the views of the public) could be turned into something to be manipulated by dictators and demagogues. In such a context, it is perhaps not so surprising that in one of the more popular research methods texts of the decade, the terms content analysis and communication analysis are used interchangeably (see Goode & Hatt, 1952 , p. 325).
Academic fashions and interests naturally change with available technology, and these days we are more likely to focus on the individualization of communications through Twitter and the like, rather than of mass newspaper readership or mass radio audiences, yet the prevailing discourse on content analysis has remained much the same as it was in Berleson’s day. Thus, Neuendorf ( 2002 ), for example, continued to define content analysis as “the systematic, objective, quantitative analysis of message characteristics” (p. 1). Clearly, the centrality of communication as a basis for understanding and using content analysis continues to hold, but in this chapter I will try to show that, rather than locate the use of content analysis in disembodied “messages” and distantiated “media,” we would do better to focus on the fact that communication is a building block of social life itself and not merely a system of messages that are transmitted—in whatever form—from sender to receiver. To put that statement in another guise, we must note that communicative action (to use the phraseology of Habermas, 1987 ) rests at the very base of the lifeworld, and one very important way of coming to grips with that world is to study the content of what people say and write in the course of their everyday lives.
My aim is to demonstrate various ways in which content analysis (henceforth CTA) can be used and developed to analyze social scientific data as derived from interviews and documents. It is not my intention to cover the history of CTA or to venture into forms of literary analysis or to demonstrate each and every technique that has ever been deployed by content analysts. (Many of the standard textbooks deal with those kinds of issues much more fully than is possible here. See, for example, Babbie, 2013 ; Berelson, 1952 ; Bryman, 2008 , Krippendorf, 2004 ; Neuendorf, 2002 ; and Weber, 1990 ). Instead, I seek to recontextualize the use of the method in a framework of network thinking and to link the use of CTA to specific problems of data analysis. As will become evident, my exposition of the method is grounded in real-world problems. Those problems are drawn from my own research projects and tend to reflect my academic interests—which are almost entirely related to the analysis of the ways in which people talk and write about aspects of health, illness, and disease. However, lest the reader be deterred from going any further, I should emphasize that the substantive issues that I elect to examine are secondary if not tertiary to my main objective—which is to demonstrate how CTA can be integrated into a range of research designs and add depth and rigor to the analysis of interview and inscription data. To that end, in the next section I aim to clear our path to analysis by dealing with some issues that touch on the general position of CTA in the research armory, especially its location in the schism that has developed between quantitative and qualitative modes of inquiry.
The Methodological Context of Content Analysis
Content analysis is usually associated with the study of inscription contained in published reports, newspapers, adverts, books, web pages, journals, and other forms of documentation. Hence, nearly all of Berelson’s ( 1952 ) illustrations and references to the method relate to the analysis of written records of some kind, and where speech is mentioned, it is almost always in the form of broadcast and published political speeches (such as State of the Union addresses). This association of content analysis with text and documentation is further underlined in modern textbook discussions of the method. Thus, Bryman ( 2008 ), for example, defined CTA as “an approach to the analysis of documents and texts , that seek to quantify content in terms of pre-determined categories” (2008, p. 274, emphasis in original), while Babbie ( 2013 ) stated that CTA is “the study of recorded human communications” (2013, p. 295), and Weber referred to it as a method to make “valid inferences from text” (1990, p. 9). It is clear then that CTA is viewed as a text-based method of analysis, though extensions of the method to other forms of inscriptional material are also referred to in some discussions. Thus, Neuendorf ( 2002 ), for example, rightly referred to analyses of film and television images as legitimate fields for the deployment of CTA and by implication analyses of still—as well as moving—images such as photographs and billboard adverts. Oddly, in the traditional or standard paradigm of CTA, the method is solely used to capture the “message” of a text or speech; it is not used for the analysis of a recipient’s response to or understanding of the message (which is normally accessed via interview data and analyzed in other and often less rigorous ways; see, e.g., Merton, 1968 ). So, in this chapter I suggest that we can take things at least one small step further by using CTA to analyze speech (especially interview data) as well as text.
Standard textbook discussions of CTA usually refer to it as a “nonreactive” or “unobtrusive” method of investigation (see, e.g., Babbie, 2013 , p. 294), and a large part of the reason for that designation is because of its focus on already existing text (i.e., text gathered without intrusion into a research setting). More important, however (and to underline the obvious), CTA is primarily a method of analysis rather than of data collection. Its use, therefore, must be integrated into wider frames of research design that embrace systematic forms of data collection as well as forms of data analysis. Thus, routine strategies for sampling data are often required in designs that call on CTA as a method of analysis. These latter can be built around random sampling methods or even techniques of “theoretical sampling” (Glaser & Strauss, 1967 ) so as to identify a suitable range of materials for CTA. Content analysis can also be linked to styles of ethnographic inquiry and to the use of various purposive or nonrandom sampling techniques. For an example, see Altheide ( 1987 ).
The use of CTA in a research design does not preclude the use of other forms of analysis in the same study, because it is a technique that can be deployed in parallel with other methods or with other methods sequentially. For example, and as I will demonstrate in the following sections, one might use CTA as a preliminary analytical strategy to get a grip on the available data before moving into specific forms of discourse analysis. In this respect, it can be as well to think of using CTA in, say, the frame of a priority/sequence model of research design as described by Morgan ( 1998 ).
As I shall explain, there is a sense in which CTA rests at the base of all forms of qualitative data analysis, yet the paradox is that the analysis of content is usually considered a quantitative (numerically based) method. In terms of the qualitative/quantitative divide, however, it is probably best to think of CTA as a hybrid method, and some writers have in the past argued that it is necessarily so (Kracauer, 1952 ). That was probably easier to do in an age when many recognized the strictly drawn boundaries between qualitative and quantitative styles of research to be inappropriate. Thus, in their widely used text Methods in Social Research , Goode and Hatt ( 1952 ), for example, asserted that “modern research must reject as a false dichotomy the separation between ‘qualitative’ and ‘quantitative’ studies, or between the ‘statistical’ and the ‘non-statistical’ approach” (p. 313). This position was advanced on the grounds that all good research must meet adequate standards of validity and reliability, whatever its style, and the message is well worth preserving. However, there is a more fundamental reason why it is nonsensical to draw a division between the qualitative and the quantitative. It is simply this: All acts of social observation depend on the deployment of qualitative categories—whether gender, class, race, or even age; there is no descriptive category in use in the social sciences that connects to a world of “natural kinds.” In short, all categories are made, and therefore when we seek to count “things” in the world, we are dependent on the existence of socially constructed divisions. How the categories take the shape that they do—how definitions are arrived at, how inclusion and exclusion criteria are decided on, and how taxonomic principles are deployed—constitute interesting research questions in themselves. From our starting point, however, we need only note that “sorting things out” (to use a phrase from Bowker & Star, 1999 ) and acts of “counting”—whether it be of chromosomes or people (Martin & Lynch, 2009 )—are activities that connect to the social world of organized interaction rather than to unsullied observation of the external world.
Some writers deny the strict division between the qualitative and quantitative on grounds of empirical practice rather than of ontological reasoning. For example, Bryman ( 2008 ) argued that qualitative researchers also call on quantitative thinking, but tend to use somewhat vague, imprecise terms rather than numbers and percentages—referring to frequencies via the use of phrases such as “more than” and “less than.” Kracauer ( 1952 ) advanced various arguments against the view that CTA was strictly a quantitative method, suggesting that very often we wished to assess content as being negative or positive with respect to some political, social, or economic thesis and that such evaluations could never be merely statistical. He further argued that we often wished to study “underlying” messages or latent content of documentation and that, in consequence, we needed to interpret content as well as count items of content. Morgan ( 1993 ) argued that, given the emphasis that is placed on “coding” in almost all forms of qualitative data analysis, the deployment of counting techniques is essential and we ought therefore to think in terms of what he calls qualitative as well as quantitative content analysis. Naturally, some of these positions create more problems than they seemingly solve (as is the case with considerations of “latent content”), but given the 21st-century predilection for mixed methods research (Creswell, 2007 ), it is clear that CTA has a role to play in integrating quantitative and qualitative modes of analysis in a systematic rather than merely ad hoc and piecemeal fashion. In the sections that follow, I will provide some examples of the ways in which “qualitative” analysis can be combined with systematic modes of counting. First, however, we must focus on what is analyzed in CTA.
Units of Analysis
So, what is the unit of analysis in CTA? A brief answer is that analysis can be focused on words, sentences, grammatical structures, tenses, clauses, ratios (of, say, nouns to verbs), or even “themes.” Berelson ( 1952 ) gave examples of all of the above and also recommended a form of thematic analysis (cf., Braun & Clarke, 2006 ) as a viable option. Other possibilities include counting column length (of speeches and newspaper articles), amounts of (advertising) space, or frequency of images. For our purposes, however, it might be useful to consider a specific (and somewhat traditional) example. Here it is. It is an extract from what has turned out to be one of the most important political speeches of the current century.
Iraq continues to flaunt its hostility toward America and to support terror. The Iraqi regime has plotted to develop anthrax and nerve gas and nuclear weapons for over a decade. This is a regime that has already used poison gas to murder thousands of its own citizens, leaving the bodies of mothers huddled over their dead children. This is a regime that agreed to international inspections then kicked out the inspectors. This is a regime that has something to hide from the civilized world. States like these, and their terrorist allies, constitute an axis of evil, arming to threaten the peace of the world. By seeking weapons of mass destruction, these regimes pose a grave and growing danger. They could provide these arms to terrorists, giving them the means to match their hatred. They could attack our allies or attempt to blackmail the United States. In any of these cases, the price of indifference would be catastrophic. (George W. Bush, State of the Union address, January 29, 2002)
A number of possibilities arise for analyzing the content of a speech such as the one above. Clearly, words and sentences must play a part in any such analysis, but in addition to words, there are structural features of the speech that could also figure. For example, the extract takes the form of a simple narrative—pointing to a past, a present, and an ominous future (catastrophe)—and could therefore be analyzed as such. There are, in addition, several interesting oppositions in the speech (such as those between “regimes” and the “civilized” world), as well as a set of interconnected present participles such as “plotting,” “hiding,” “arming,” and “threatening” that are associated both with Iraq and with other states that “constitute an axis of evil.” Evidently, simple word counts would fail to capture the intricacies of a speech of this kind. Indeed, our example serves another purpose—to highlight the difficulty that often arises in dissociating CTA from discourse analysis (of which narrative analysis and the analysis of rhetoric and trope are subspecies). So how might we deal with these problems?
One approach that can be adopted is to focus on what is referenced in text and speech, that is, to concentrate on the characters or elements that are recruited into the text and to examine the ways in which they are connected or co-associated. I shall provide some examples of this form of analysis shortly. Let us merely note for the time being that in the previous example we have a speech in which various “characters”—including weapons in general, specific weapons (such as nerve gas), threats, plots, hatred, evil, and mass destruction—play a role. Be aware that we need not be concerned with the veracity of what is being said—whether it is true or false—but simply with what is in the speech and how what is in there is associated. (We may leave the task of assessing truth and falsity to the jurists). Be equally aware that it is a text that is before us and not an insight into the ex-president’s mind, or his thinking, or his beliefs, or any other subjective property that he may have possessed.
In the introductory paragraph, I made brief reference to some ideas of the German philosopher Jürgen Habermas ( 1987 ). It is not my intention here to expand on the detailed twists and turns of his claims with respect to the role of language in the “lifeworld” at this point. However, I do intend to borrow what I regard as some particularly useful ideas from his work. The first is his claim—influenced by a strong line of 20th-century philosophical thinking—that language and culture are constitutive of the lifeworld (Habermas, 1987 , p. 125), and in that sense we might say that things (including individuals and societies) are made in language. That is a simple justification for focusing on what people say rather than what they “think” or “believe” or “feel” or “mean” (all of which have been suggested at one time or another as points of focus for social inquiry and especially qualitative forms of inquiry). Second, Habermas argued that speakers and therefore hearers (and, one might add, writers and therefore readers), in what he calls their speech acts, necessarily adopt a pragmatic relation to one of three worlds: entities in the objective world, things in the social world, and elements of a subjective world. In practice, Habermas ( 1987 , p. 120) suggested all three worlds are implicated in any speech act, but that there will be a predominant orientation to one of them. To rephrase this in a crude form, when speakers engage in communication, they refer to things and facts and observations relating to external nature, to aspects of interpersonal relations, and to aspects of private inner subjective worlds (thoughts, feelings, beliefs, etc.). One of the problems with locating CTA in “communication research” has been that the communications referred to are but a special and limited form of action (often what Habermas called strategic acts). In other words, television, newspaper, video, and Internet communications are just particular forms (with particular features) of action in general. Again, we might note in passing that the adoption of the Habermassian perspective on speech acts implies that much of qualitative analysis in particular has tended to focus only on one dimension of communicative action—the subjective and private. In this respect, I would argue that it is much better to look at speeches such as George W Bush’s 2002 State of the Union address as an “account” and to examine what has been recruited into the account, and how what has been recruited is connected or co-associated, rather than use the data to form insights into his (or his adviser’s) thoughts, feelings, and beliefs.
In the sections that follow, and with an emphasis on the ideas that I have just expounded, I intend to demonstrate how CTA can be deployed to advantage in almost all forms of inquiry that call on either interview (or speech-based) data or textual data. In my first example, I will show how CTA can be used to analyze a group of interviews. In the second example, I will show how it can be used to analyze a group of policy documents. In the third, I shall focus on a single interview (a “case”), and in the fourth and final example, I will show how CTA can be used to track the biography of a concept. In each instance, I shall briefly introduce the context of the “problem” on which the research was based, outline the methods of data collection, discuss how the data were analyzed and presented, and underline the ways in which CTA has sharpened the analytical strategy.
Analyzing a Sample of Interviews: Looking at Concepts and Their Co-associations in a Semantic Network
My first example of using CTA is based on a research study that was initially undertaken in the early 2000s. It was a project aimed at understanding why older people might reject the offer to be immunized against influenza (at no cost to them). The ultimate objective was to improve rates of immunization in the study area. The first phase of the research was based on interviews with 54 older people in South Wales. The sample included people who had never been immunized, some who had refused immunization, and some who had accepted immunization. Within each category, respondents were randomly selected from primary care physician patient lists, and the data were initially analyzed “thematically” and published accordingly (Evans, Prout, Prior, Tapper-Jones, & Butler, 2007 ). A few years later, however, I returned to the same data set to look at a different question—how (older) lay people talked about colds and flu, especially how they distinguished between the two illnesses and how they understood the causes of the two illnesses (see Prior, Evans, & Prout, 2011 ). Fortunately, in the original interview schedule, we had asked people about how they saw the “differences between cold and flu” and what caused flu, so it was possible to reanalyze the data with such questions in mind. In that frame, the example that follows demonstrates not only how CTA might be used on interview data, but also how it might be used to undertake a secondary analysis of a preexisting data set (Bryman, 2008 ).
As with all talk about illness, talk about colds and flu is routinely set within a mesh of concerns—about causes, symptoms, and consequences. Such talk comprises the base elements of what has at times been referred to as the “explanatory model” of an illness (Kleinman, Eisenberg, & Good, 1978 ). In what follows, I shall focus almost entirely on issues of causation as understood from the viewpoint of older people; the analysis is based on the answers that respondents made in response to the question, “How do you think people catch flu?”
Semistructured interviews of the kind undertaken for a study such as this are widely used and are often characterized as akin to “a conversation with a purpose” (Kahn & Cannell, 1957 , p. 97). One of the problems of analyzing the consequent data is that, although the interviewer holds to a planned schedule, the respondents often reflect in a somewhat unstructured way about the topic of investigation, so it is not always easy to unravel the web of talk about, say, “causes” that occurs in the interview data. In this example, causal agents of flu, inhibiting agents, and means of transmission were often conflated by the respondents. Nevertheless, in their talk people did answer the questions that were posed, and in the study referred to here, that talk made reference to things such as “bugs” (and “germs”) as well as viruses, but the most commonly referred to causes were “the air” and the “atmosphere.” The interview data also pointed toward means of transmission as “cause”—so coughs and sneezes and mixing in crowds figured in the causal mix. Most interesting, perhaps, was the fact that lay people made a nascent distinction between facilitating factors (such as bugs and viruses) and inhibiting factors (such as being resistant, immune, or healthy), so that in the presence of the latter, the former are seen to have very little effect. Here are some shorter examples of typical question–response pairs from the original interview data.
(R:32): “How do you catch it [the flu]? Well, I take it its through ingesting and inhaling bugs from the atmosphere. Not from sort of contact or touching things. Sort of airborne bugs. Is that right?” (R:3): “I suppose it’s [the cause of flu] in the air. I think I get more diseases going to the surgery than if I stayed home. Sometimes the waiting room is packed and you’ve got little kids coughing and spluttering and people sneezing, and air conditioning I think is a killer by and large I think air conditioning in lots of these offices.” (R:46): “I think you catch flu from other people. You know in enclosed environments in air conditioning which in my opinion is the biggest cause of transferring diseases is air conditioning. Worse thing that was ever invented that was. I think so, you know. It happens on aircraft exactly the same you know.”
Alternatively, it was clear that for some people being cold, wet, or damp could also serve as a direct cause of flu; thus: Interviewer: “OK, good. How do you think you catch the flu?”
(R:39): “Ah. The 65 dollar question. Well, I would catch it if I was out in the rain and I got soaked through. Then I would get the flu. I mean my neighbour up here was soaked through and he got pneumonia and he died. He was younger than me: well, 70. And he stayed in his wet clothes and that’s fatal. Got pneumonia and died, but like I said, if I get wet, especially if I get my head wet, then I can get a nasty head cold and it could develop into flu later.”
As I suggested earlier, despite the presence of bugs and germs, viruses, the air, and wetness or dampness, “catching” the flu is not a matter of simple exposure to causative agents. Thus, some people hypothesized that within each person there is a measure of immunity or resistance or healthiness that comes into play and that is capable of counteracting the effects of external agents. For example, being “hardened” to germs and harsh weather can prevent a person getting colds and flu. Being “healthy” can itself negate the effects of any causative agents, and healthiness is often linked to aspects of “good” nutrition and diet and not smoking cigarettes. These mitigating and inhibiting factors can either mollify the effects of infection or prevent a person “catching” the flu entirely. Thus, (R:45) argued that it was almost impossible for him to catch flu or cold “cos I got all this resistance.” Interestingly, respondents often used possessive pronouns in their discussion of immunity and resistance (“my immunity” and “my resistance”)—and tended to view them as personal assets (or capital) that might be compromised by mixing with crowds.
By implication, having a weak immune system can heighten the risk of contracting colds and flu and might therefore spur one to take preventive measures, such as accepting a flu shot. Some people believe that the flu shot can cause the flu and other illnesses. An example of what might be called lay “epidemiology” (Davison, Davey-Smith, & Frankel, 1991 ) is evident in the following extract.
(R:4): “Well, now it’s coincidental you know that [my brother] died after the jab, but another friend of mine, about 8 years ago, the same happened to her. She had the jab and about six months later, she died, so I know they’re both coincidental, but to me there’s a pattern.”
Normally, results from studies such as this are presented in exactly the same way as has just been set out. Thus, the researcher highlights given themes that are said to have emerged from the data and then provides appropriate extracts from the interviews to illustrate and substantiate the relevant themes. However, one reasonable question that any critic might ask about the selected data extracts concerns the extent to which they are “representative” of the material in the data set as a whole. Maybe, for example, the author has been unduly selective in his or her use of both themes and quotations. Perhaps, as a consequence, the author has ignored or left out talk that does not fit the arguments or extracts that might be considered dull and uninteresting compared to more exotic material. And these kinds of issues and problems are certainly common to the reporting of almost all forms of qualitative research. However, the adoption of CTA techniques can help to mollify such problems. This is so because, by using CTA, we can indicate the extent to which we have used all or just some of the data, and we can provide a view of the content of the entire sample of interviews rather than just the content and flavor of merely one or two interviews. In this light, we must consider Figure 19.1 , which is based on counting the number of references in the 54 interviews to the various “causes” of the flu, though references to the flu shot (i.e., inoculation) as a cause of flu have been ignored for the purpose of this discussion. The node sizes reflect the relative importance of each cause as determined by the concept count (frequency of occurrence). The links between nodes reflect the degree to which causes are co-associated in interview talk and are calculated according to a co-occurrence index (see, e.g., SPSS, 2007 , p. 183).
What causes flu? A lay perspective. Factors listed as causes of colds and flu in 54 interviews. Node size is proportional to number of references “as causes.” Line thickness is proportional to co-occurrence of any two “causes” in the set of interviews.
Given this representation, we can immediately assess the relative importance of the different causes as referred to in the interview data. Thus, we can see that such things as (poor) “hygiene” and “foreigners” were mentioned as a potential cause of flu—but mention of hygiene and foreigners was nowhere near as important as references to “the air” or to “crowds” or to “coughs and sneezes.” In addition, we can also determine the strength of the connections that interviewees made between one cause and another. Thus, there are relatively strong links between “resistance” and “coughs and sneezes,” for example.
In fact, Figure 19.1 divides causes into the “external” and the “internal,” or the facilitating and the impeding (lighter and darker nodes). Among the former I have placed such things as crowds, coughs, sneezes, and the air, while among the latter I have included “resistance,” “immunity,” and “health.” That division is a product of my conceptualizing and interpreting the data, but whichever way we organize the findings, it is evident that talk about the causes of flu belongs in a web or mesh of concerns that would be difficult to represent using individual interview extracts alone. Indeed, it would be impossible to demonstrate how the semantics of causation belong to a culture (rather than to individuals) in any other way. In addition, I would argue that the counting involved in the construction of the diagram functions as a kind of check on researcher interpretations and provides a source of visual support for claims that an author might make about, say, the relative importance of “damp” and “air” as perceived causes of disease. Finally, the use of CTA techniques allied with aspects of conceptualization and interpretation has enabled us to approach the interview data as a set and to consider the respondents as belonging to a community, rather than regarding them merely as isolated and disconnected individuals, each with their own views. It has also enabled us to squeeze some new findings out of old data, and I would argue that it has done so with advantage. There are other advantages to using CTA to explore data sets, which I will highlight in the next section.
Analyzing a Sample of Documents: Using Content Analysis to Verify Claims
Policy analysis is a difficult business. To begin, it is never entirely clear where (social, health, economic, environmental) policy actually is. Is it in documents (as published by governments, think tanks, and research centers), in action (what people actually do), or in speech (what people say)? Perhaps it rests in a mixture of all three realms. Yet, wherever it may be, it is always possible, at the very least, to identify a range of policy texts and to focus on the conceptual or semantic webs in terms of which government officials and other agents (such as politicians) talk about the relevant policy issues. Furthermore, insofar as policy is recorded—in speeches, pamphlets, and reports—we may begin to speak of specific policies as having a history or a pedigree that unfolds through time (think, e.g., of U.S. or U.K. health policies during the Clinton years or the Obama years). And, insofar as we consider “policy” as having a biography or a history, we can also think of studying policy narratives.
Though firmly based in the world of literary theory, narrative method has been widely used for both the collection and the analysis of data concerning ways in which individuals come to perceive and understand various states of health, ill health, and disability (Frank, 1995 ; Hydén, 1997 ). Narrative techniques have also been adapted for use in clinical contexts and allied to concepts of healing (Charon, 2006 ). In both social scientific and clinical work, however, the focus is invariably on individuals and on how individuals “tell” stories of health and illness. Yet narratives can also belong to collectives—such as political parties and ethnic and religious groups—just as much as to individuals, and in the latter case there is a need to collect and analyze data that are dispersed across a much wider range of materials than can be obtained from the personal interview. In this context, Roe ( 1994 ) demonstrated how narrative method can be applied to an analysis of national budgets, animal rights, and environmental policies.
An extension of the concept of narrative to policy discourse is undoubtedly useful (Newman & Vidler, 2006 ), but how might such narratives be analyzed? What strategies can be used to unravel the form and content of a narrative, especially in circumstances where the narrative might be contained in multiple (policy) documents, authored by numerous individuals, and published across a span of time rather than in a single, unified text such as a novel? Roe ( 1994 ), unfortunately, was not in any way specific about analytical procedures, apart from offering the useful rule to “never stray too far from the data” (p. xii). So, in this example, I will outline a strategy for tackling such complexities. In essence, it is a strategy that combines techniques of linguistically (rule) based CTA with a theoretical and conceptual frame that enables us to unravel and identify the core features of a policy narrative. My substantive focus is on documents concerning health service delivery policies published from 2000 to 2009 in the constituent countries of the United Kingdom (that is, England, Scotland, Wales, and Northern Ireland—all of which have different political administrations).
Narratives can be described and analyzed in various ways, but for our purposes we can say that they have three key features: they point to a chronology, they have a plot, and they contain “characters.”
All narratives have beginnings; they also have middles and endings, and these three stages are often seen as comprising the fundamental structure of narrative text. Indeed, in his masterly analysis of time and narrative, Ricoeur ( 1984 ) argued that it is in the unfolding chronological structure of a narrative that one finds its explanatory (and not merely descriptive) force. By implication, one of the simplest strategies for the examination of policy narratives is to locate and then divide a narrative into its three constituent parts—beginning, middle, and end.
Unfortunately, while it can sometimes be relatively easy to locate or choose a beginning to a narrative, it can be much more difficult to locate an end point. Thus, in any illness narrative, a narrator might be quite capable of locating the start of an illness process (in an infection, accident, or other event) but unable to see how events will be resolved in an ongoing and constantly unfolding life. As a consequence, both narrators and researchers usually find themselves in the midst of an emergent present—a present without a known and determinate end (see, e.g., Frank, 1995 ). Similar considerations arise in the study of policy narratives where chronology is perhaps best approached in terms of (past) beginnings, (present) middles, and projected futures.
According to Ricoeur ( 1984 ), our basic ideas about narrative are best derived from the work and thought of Aristotle, who in his Poetics sought to establish “first principles” of composition. For Ricoeur, as for Aristotle, plot ties things together. It “brings together factors as heterogeneous as agents, goals, means, interactions, circumstances, unexpected results” (p. 65) into the narrative frame. For Aristotle, it is the ultimate untying or unraveling of the plot that releases the dramatic energy of the narrative.
Characters are most commonly thought of as individuals, but they can be considered in much broader terms. Thus, the French semiotician A. J. Greimas ( 1970 ), for example, suggested that, rather than think of characters as people, it would be better to think in terms of what he called actants and of the functions that such actants fulfill within a story. In this sense, geography, climate, and capitalism can be considered characters every bit as much as aggressive wolves and Little Red Riding Hood. Further, he argued that the same character (actant) can be considered to fulfill many functions, and the same function may be performed by many characters. Whatever else, the deployment of the term actant certainly helps us to think in terms of narratives as functioning and creative structures. It also serves to widen our understanding of the ways in which concepts, ideas, and institutions, as well “things” in the material world, can influence the direction of unfolding events every bit as much as conscious human subjects. Thus, for example, the “American people,” “the nation,” “the Constitution,” “the West,” “tradition,” and “Washington” can all serve as characters in a policy story.
As I have already suggested, narratives can unfold across many media and in numerous arenas—speech and action, as well as text. Here, however, my focus is solely on official documents—all of which are U.K. government policy statements, as listed in Table 19.1 . The question is, How might CTA help us unravel the narrative frame?
It might be argued that a simple reading of any document should familiarize the researcher with elements of all three policy narrative components (plot, chronology, and character). However, in most policy research, we are rarely concerned with a single and unified text, as is the case with a novel; rather, we have multiple documents written at distinctly different times by multiple (usually anonymous) authors that notionally can range over a wide variety of issues and themes. In the full study, some 19 separate publications were analyzed across England, Wales, Scotland, and Northern Ireland.
Naturally, listing word frequencies—still less identifying co-occurrences and semantic webs in large data sets (covering hundreds of thousands of words and footnotes)—cannot be done manually, but rather requires the deployment of complex algorithms and text-mining procedures. To this end, I analyzed the 19 documents using “Text Mining for Clementine” (SPSS, 2007 ).
Text-mining procedures begin by providing an initial list of concepts based on the lexicon of the text but that can be weighted according to word frequency and that take account of elementary word associations. For example, learning disability, mental health, and performance management indicate three concepts, not six words. Using such procedures on the aforementioned documents gives the researcher an initial grip on the most important concepts in the document set of each country. Note that this is much more than a straightforward concordance analysis of the text and is more akin to what Ryan and Bernard ( 2000 ) referred to as semantic analysis and Carley ( 1993 ) has referred to as concept and mapping analysis.
So, the first task was to identify and then extract the core concepts, thus identifying what might be called “key” characters or actants in each of the policy narratives. For example, in the Scottish documents, such actants included “Scotland” and the “Scottish people,” as well as “health” and the “National Health Service (NHS),” among others, while in the Welsh documents it was “the people of Wales” and “Wales” that figured largely—thus emphasizing how national identity can play every bit as important a role in a health policy narrative as concepts such as “health,” “hospitals,” and “well-being.”
Having identified key concepts, it was then possible to track concept clusters in which particular actants or characters are embedded. Such cluster analysis is dependent on the use of co-occurrence rules and the analysis of synonyms, whereby it is possible to get a grip on the strength of the relationships between the concepts, as well as the frequency with which the concepts appear in the collected texts. In Figure 19.2 , I provide an example of a concept cluster. The diagram indicates the nature of the conceptual and semantic web in which various actants are discussed. The diagrams further indicate strong (solid line) and weaker (dashed line) connections between the various elements in any specific mix, and the numbers indicate frequency counts for the individual concepts. Using Clementine , the researcher is unable to specify in advance which clusters will emerge from the data. One cannot, for example, choose to have an NHS cluster. In that respect, these diagrams not only provide an array in terms of which concepts are located, but also serve as a check on and to some extent validation of the interpretations of the researcher. None of this tells us what the various narratives contained within the documents might be, however. They merely point to key characters and relationships both within and between the different narratives. So, having indicated the techniques used to identify the essential parts of the four policy narratives, it is now time to sketch out their substantive form.
Concept cluster for “care” in six English policy documents, 2000–2007. Line thickness is proportional to the strength co-occurrence coefficient. Node size reflects relative frequency of concept, and (numbers) refer to the frequency of concept. Solid lines indicate relationships between terms within the same cluster, and dashed lines indicate relationships between terms in different clusters.
It may be useful to note that Aristotle recommended brevity in matters of narrative—deftly summarizing the whole of the Odyssey in just seven lines. In what follows, I attempt—albeit somewhat weakly—to emulate that example by summarizing a key narrative of English health services policy in just four paragraphs. Note how the narrative unfolds in relation to the dates of publication. In the English case (though not so much in the other U.K. countries), it is a narrative that is concerned to introduce market forces into what is and has been a state-managed health service. Market forces are justified in terms of improving opportunities for the consumer (i.e., the patients in the service), and the pivot of the newly envisaged system is something called “patient choice” or “choice.” This is how the story unfolds as told through the policy documents between 2000 and 2008 (see Table 19.1 ). The citations in the following paragraphs are to the Department of Health publications (by year) listed in Table 19.1 .
The advent of the NHS in 1948 was a “seminal event” (2000, p. 8), but under successive Conservative administrations, the NHS was seriously underfunded (2006, p. 3). The (New Labour) government will invest (2000) or already has (2003, p. 4) invested extensively in infrastructure and staff, and the NHS is now on a “journey of major improvement” (2004, p. 2). But “more money is only a starting point” (2000, p. 2), and the journey is far from finished. Continuation requires some fundamental changes of “culture” (2003, p. 6). In particular, the NHS remains unresponsive to patient need, and “all too often, the individual needs and wishes are secondary to the convenience of the services that are available. This ‘one size fits all’ approach is neither responsive, equitable nor person-centred” (2003, p. 17). In short, the NHS is a 1940s system operating in a 21st-century world (2000, p. 26). Change is therefore needed across the “whole system” (2005, p. 3) of care and treatment.
Above all, we must recognize that we “live in a consumer age” (2000, p. 26). People’s expectations have changed dramatically (2006, p. 129), and people want more choice, more independence, and more control (2003, p. 12) over their affairs. Patients are no longer, and should not be considered, “passive recipients” of care (2003, p. 62), but wish to be and should be (2006, p. 81) actively “involved” in their treatments (2003, p. 38; 2005, p. 18)—indeed, engaged in a partnership (2003, p. 22) of respect with their clinicians. Furthermore, most people want a personalized service “tailor made to their individual needs” (2000, p. 17; 2003, p. 15; 2004, p. 1; 2006, p. 83)—“a service which feels personal to each and every individual within a framework of equity and good use of public money” (2003, p. 6).
To advance the necessary changes, “patient choice” must be and “will be strengthened” (2000, p. 89). “Choice” must be made to “happen” (2003), and it must be “real” (2003, p. 3; 2004, p. 5; 2005, p. 20; 2006, p. 4). Indeed, it must be “underpinned” (2003, p. 7) and “widened and deepened” (2003, p. 6) throughout the entire system of care.
If “we” expand and underpin patient choice in appropriate ways and engage patients in their treatment systems, then levels of patient satisfaction will increase (2003, p. 39), and their choices will lead to a more “efficient” (2003, p. 5; 2004, p. 2; 2006, p. 16) and effective (2003, p. 62; 2005, p. 8) use of resources. Above all, the promotion of choice will help to drive up “standards” of care and treatment (2000, p. 4; 2003, p. 12; 2004, p. 3; 2005, p. 7; 2006, p. 3). Furthermore, the expansion of choice will serve to negate the effects of the “inverse care law,” whereby those who need services most tend to get catered to the least (2000, p. 107; 2003, p. 5; 2006, p. 63), and it will thereby help in moderating the extent of health inequalities in the society in which we live. “The overall aim of all our reforms,” therefore, “is to turn the NHS from a top down monolith into a responsive service that gives the patient the best possible experience. We need to develop an NHS that is both fair to all of us, and personal to each of us” (2003, p. 5).
We can see how most—though not all—of the elements of this story are represented in Figure 19.2. In particular, we can see strong (co-occurrence) links between care and choice and how partnership, performance, control, and improvement have a prominent profile. There are some elements of the web that have a strong profile (in terms of node size and links), but to which we have not referred; access, information, primary care, and waiting times are four. As anyone well versed in English healthcare policy would know, these elements have important roles to play in the wider, consumer-driven narrative. However, by rendering the excluded as well as included elements of that wider narrative visible, the concept web provides a degree of verification on the content of the policy story as told herein and on the scope of its “coverage.”
In following through on this example, we have moved from CTA to a form of discourse analysis (in this instance, narrative analysis). That shift underlines aspects of both the versatility of CTA and some of its weaknesses—versatility in the sense that CTA can be readily combined with other methods of analysis and in the way in which the results of the CTA help us to check and verify the claims of the researcher. The weakness of the diagram compared to the narrative is that CTA on its own is a somewhat one-dimensional and static form of analysis, and while it is possible to introduce time and chronology into the diagrams, the diagrams themselves remain lifeless in the absence of some form of discursive overview. (For a fuller analysis of these data, see Prior, Hughes, & Peckham, 2012 ).
Analyzing a Single Interview: The Role of Content Analysis in a Case Study
So far, I have focused on using CTA on a sample of interviews and a sample of documents. In the first instance, I recommended CTA for its capacity to tell us something about what is seemingly central to interviewees and for demonstrating how what is said is linked (in terms of a concept network). In the second instance, I reaffirmed the virtues of co-occurrence and network relations, but this time in the context of a form of discourse analysis. I also suggested that CTA can serve an important role in the process of verification of a narrative and its academic interpretation. In this section, however, I am going to link the use of CTA to another style of research—case study—to show how CTA might be used to analyze a single “case.”
Case study is a term used in multiple and often ambiguous ways. However, Gerring ( 2004 ) defined it as “an intensive study of a single unit for the purpose of understanding a larger class of (similar) units” (p. 342). As Gerring pointed out, case study does not necessarily imply a focus on N = 1, although that is indeed the most logical number for case study research (Ragin & Becker, 1992 ). Naturally, an N of 1 can be immensely informative, and whether we like it or not, we often have only one N to study (think, e.g., of the 1986 Challenger shuttle disaster or of the 9/11 attack on the World Trade Center). In the clinical sciences, case studies are widely used to represent the “typical” features of a wider class of phenomena and often used to define a kind or syndrome (as in the field of clinical genetics). Indeed, at the risk of mouthing a tautology, one can say that the distinctive feature of case study is its focus on a case in all of its complexity—rather than on individual variables and their interrelationships, which tends to be a point of focus for large N research.
There was a time when case study was central to the science of psychology. Breuer and Freud’s (2001) famous studies of “hysteria” (originally published in 1895) provide an early and outstanding example of the genre in this respect, but as with many of the other styles of social science research, the influence of case studies waned with the rise of much more powerful investigative techniques—including experimental methods—driven by the deployment of new statistical technologies. Ideographic studies consequently gave way to the current fashion for statistically driven forms of analysis that focus on causes and cross-sectional associations between variables rather than ideographic complexity.
In the example that follows, we will look at the consequences of a traumatic brain injury (TBI) on just one individual. The analysis is based on an interview with a person suffering from such an injury, and it was one of 32 interviews carried out with people who had experienced a TBI. The objective of the original research was to develop an outcome measure for TBI that was sensitive to the sufferer’s (rather than the health professional’s) point of view. In our original study (see Morris et al., 2005 ), interviews were also undertaken with 27 carers of the injured with the intention of comparing their perceptions of TBI to those of the people for whom they cared. A sample survey was also undertaken to elicit views about TBI from a much wider population of patients than was studied via interview.
In the introduction, I referred to Habermas and the concept of the lifeworld. Lifeworld ( Lebenswelt ) is a concept that first arose from 20th-century German philosophy. It constituted a specific focus for the work of Alfred Schutz (see, e.g., Schutz & Luckman, 1974 ). Schutz ( 1974 ) described the lifeworld as “that province of reality which the wide-awake and normal adult simply takes-for-granted in an attitude of common sense” (p. 3). Indeed, it was the routine and taken-for-granted quality of such a world that fascinated Schutz. As applied to the worlds of those with head injuries, the concept has particular resonance because head injuries often result in that taken-for-granted quality being disrupted and fragmented, ending in what Russian neuropsychologist A. R. Luria ( 1975 ) once described as “shattered” worlds. As well as providing another excellent example of a case study, Luria’s work is also pertinent because he sometimes argued for a “romantic science” of brain injury—that is, a science that sought to grasp the worldview of the injured patient by paying attention to an unfolding and detailed personal “story” of the individual with the head injury as well as to the neurological changes and deficits associated with the injury itself. In what follows, I shall attempt to demonstrate how CTA might be used to underpin such an approach.
In the original research, we began analysis by a straightforward reading of the interview transcripts. Unfortunately, a simple reading of a text or an interview can, strangely, mislead the reader into thinking that some issues or themes are more important than is warranted by the contents of the text. How that comes about is not always clear, but it probably has something to do with a desire to develop “findings” and our natural capacity to overlook the familiar in favor of the unusual. For that reason alone, it is always useful to subject any text to some kind of concordance analysis—that is, generating a simple frequency list of words used in an interview or text. Given the current state of technology, one might even speak these days of using text-mining procedures such as the aforementioned Clementine to undertake such a task. By using Clementine , and as we have seen, it is also possible to measure the strength of co-occurrence links between elements (i.e., words and concepts) in the entire data set (in this example, 32 interviews), though for a single interview these aims can just as easily be achieved using much simpler, low-tech strategies.
By putting all 32 interviews into the database, several common themes emerged. For example, it was clear that “time” entered into the semantic web in a prominent manner, and it was clearly linked to such things as “change,” “injury,” “the body,” and what can only be called the “I was.” Indeed, time runs through the 32 stories in many guises, and the centrality of time is a reflection of storytelling and narrative recounting in general—chronology, as we have noted, being a defining feature of all storytelling (Ricoeur, 1984 ). Thus, sufferers both recounted the events surrounding their injury and provided accounts as to how the injuries affected their current life and future hopes. As to time present, much of the patient story circled around activities of daily living—walking, working, talking, looking, feeling, remembering, and so forth.
Understandably, the word and the concept of “injury” featured largely in the interviews, though it was a word most commonly associated with discussions of physical consequences of injury. There were many references in that respect to injured arms, legs, hands, and eyes. There were also references to “mind”—though with far less frequency than with references to the body and to body parts. Perhaps none of this is surprising. However, one of the most frequent concepts in the semantic mix was the “I was” (716 references). The statement “I was,” or “I used to” was, in turn, strongly connected to terms such as “the accident” and “change.” Interestingly, the “I was” overwhelmingly eclipsed the “I am” in the interview data (the latter with just 63 references). This focus on the “I was” appears in many guises. For example, it is often associated with the use of the passive voice: “I was struck by a car,” “I was put on the toilet,” “I was shipped from there then, transferred to [Cityville],” “I got told that I would never be able …,” “I was sat in a room,” and so forth. In short, the “I was” is often associated with things, people, and events acting on the injured person. More important, however, the appearance of the “I was” is often used to preface statements signifying a state of loss or change in the person’s course of life—that is, as an indicator for talk about the patient’s shattered world. For example, Patient 7122 stated,
The main (effect) at the moment is I’m not actually with my children, I can’t really be their mum at the moment. I was a caring Mum, but I can’t sort of do the things that I want to be able to do like take them to school. I can’t really do a lot on my own. Like crossing the roads.
Another patient stated,
Everything is completely changed. The way I was … I can’t really do anything at the moment. I mean my German, my English, everything’s gone. Job possibilities is out the window. Everything is just out of the window … I just think about it all the time actually every day you know. You know it has destroyed me anyway, but if I really think about what has happened I would just destroy myself.
Each of these quotations, in its own way, serves to emphasize how life has changed and how the patient’s world has changed. In that respect, we can say that one of the major outcomes arising from TBI may be substantial “biographical disruption” (Bury, 1982 ), whereupon key features of an individual’s life course are radically altered forever. Indeed, as Becker ( 1997 , p. 37) argued in relation to a wide array of life events, “When their health is suddenly disrupted, people are thrown into chaos. Illness challenges one’s knowledge of one’s body. It defies orderliness. People experience the time before their illness and its aftermath as two separate entities.” Indeed, this notion of a cusp in personal biography is particularly well illustrated by Luria’s patient Zasetsky; the latter often refers to being a “newborn creature” (Luria, 1975 , pp. 24, 88), a shadow of a former self (p. 25), and as having his past “wiped out” (p. 116).
However, none of this tells us about how these factors come together in the life and experience of one individual. When we focus on an entire set of interviews, we necessarily lose the rich detail of personal experience and tend instead to rely on a conceptual rather than a graphic description of effects and consequences (to focus on, say, “memory loss,” rather than loss of memory about family life). The contents of Figure 19.3 attempt to correct that vision. Figure 19.3 records all the things that a particular respondent (Patient 7011) used to do and liked doing. It records all the things that he says he can no longer do (at 1 year after injury), and it records all the consequences that he suffered from his head injury at the time of the interview. Thus, we see references to epilepsy (his “fits”), paranoia (the patient spoke of his suspicions concerning other people, people scheming behind his back, and his inability to trust others), deafness, depression, and so forth. Note that, although I have inserted a future tense into the web (“I will”), such a statement never appeared in the transcript. I have set it there for emphasis and to show how, for this person, the future fails to connect to any of the other features of his world except in a negative way. Thus, he states at one point that he cannot think of the future because it makes him feel depressed (see Figure 19.3 ). The line thickness of the arcs reflects the emphasis that the subject placed on the relevant “outcomes” in relation to the “I was” and the “now” during the interview. Thus, we see that factors affecting his concentration and balance loom large, but that he is also concerned about his being dependent on others, his epileptic fits, and his being unable to work and drive a vehicle. The schism in his life between what he used to do, what he cannot now do, and his current state of being is nicely represented in the CTA diagram.
The shattered world of Patient 7011. Thickness of lines (arcs) is proportional to the frequency of reference to the “outcome” by the patient during the interview.
What have we gained from executing this kind of analysis? For a start, we have moved away from a focus on variables, frequencies, and causal connections (e.g., a focus on the proportion of people with TBI who suffer from memory problems or memory problems and speech problems) and refocused on how the multiple consequences of a TBI link together in one person. In short, instead of developing a narrative of acting variables, we have emphasized a narrative of an acting individual (Abbott, 1992 , p. 62). Second, it has enabled us to see how the consequences of a TBI connect to an actual lifeworld (and not simply an injured body). So the patient is not viewed just as having a series of discrete problems such as balancing, or staying awake, which is the usual way of assessing outcomes, but as someone struggling to come to terms with an objective world of changed things, people, and activities (missing work is not, for example, routinely considered an outcome of head injury). Third, by focusing on what the patient was saying, we gain insight into something that is simply not visible by concentrating on single outcomes or symptoms alone—namely, the void that rests at the center of the interview, what I have called the “I was.” Fourth, we have contributed to understanding a type, because the case that we have read about is not simply a case of “John” or “Jane” but a case of TBI, and in that respect it can add to many other accounts of what it is like to experience head injury—including one of the most well documented of all TBI cases, that of Zatetsky. Finally, we have opened up the possibility of developing and comparing cognitive maps (Carley, 1993 ) for different individuals and thereby gained insight into how alternative cognitive frames of the world arise and operate.
Tracing the Biography of a Concept
In the previous sections, I emphasized the virtues of CTA for its capacity to link into a data set in its entirety—and how the use of CTA can counter any tendency of a researcher to be selective and partial in the presentation and interpretation of information contained in interviews and documents. However, that does not mean that we always must take an entire document or interview as the data source. Indeed, it is possible to select (on rational and explicit grounds) sections of documentation and to conduct the CTA on the chosen portions. In the example that follows, I do just that. The sections that I chose to concentrate on are titles and abstracts of academic papers—rather than the full texts. The research on which the following is based is concerned with a biography of a concept and is being conducted in conjunction with a Ph.D. student of mine, Joanne Wilson. Joanne thinks of this component of the study more in terms of a “scoping study” than of a biographical study, and that, too, is a useful framework for structuring the context in which CTA can be used. Scoping studies (Arksey & O’Malley, 2005 ) are increasingly used in health-related research to “map the field” and to get a sense of the range of work that has been conducted on a given topic. Such studies can also be used to refine research questions and research designs. In our investigation, the scoping study was centered on the concept of well-being. Since 2010, well-being has emerged as an important research target for governments and corporations as well as for academics, yet it is far from clear to what the term refers. Given the ambiguity of meaning, it is clear that a scoping review, rather than either a systematic review or a narrative review of available literature, would be best suited to our goals.
The origins of the concept of well-being can be traced at least as far back as the 4th century bc , when philosophers produced normative explanations of the good life (e.g., eudaimonia, hedonia, and harmony). However, contemporary interest in the concept seemed to have been regenerated by the concerns of economists and, most recently, psychologists. These days, governments are equally concerned with measuring well-being to inform policy and conduct surveys of well-being to assess that state of the nation (see, e.g., Office for National Statistics, 2012 )—but what are they assessing?
We adopted a two-step process to address the research question, “What is the meaning of ‘well-being’ in the context of public policy?” First, we explored the existing thesauri of eight databases to establish those higher order headings (if any) under which articles with relevance to well-being might be cataloged. Thus, we searched the following databases: Cumulative Index of Nursing and Allied Health Literature, EconLit, Health Management Information Consortium, Medline, Philosopher’s Index, PsycINFO, Sociological Abstracts, and Worldwide Political Science Abstracts. Each of these databases adopts keyword-controlled vocabularies. In other words, they use inbuilt statistical procedures to link core terms to a set lexis of phrases that depict the concepts contained in the database. Table 19.2 shows each database and its associated taxonomy. The contents of Table 19.2 point toward a linguistic infrastructure in terms of which academic discourse is conducted, and our task was to extract from this infrastructure the semantic web wherein the concept of well-being is situated. We limited the thesaurus terms to well-being and its variants (i.e., wellbeing or well being). If the term was returned, it was then exploded to identify any associated terms.
To develop the conceptual map, we conducted a free-text search for well-being and its variants within the context of public policy across the same databases. We orchestrated these searches across five time frames: January 1990 to December 1994, January 1995 to December 1999, January 2000 to December 2004, January 2005 to December 2009, and January 2010 to October 2011. Naturally, different disciplines use different words to refer to well-being, each of which may wax and wane in usage over time. The searches thus sought to quantitatively capture any changes in the use and subsequent prevalence of well-being and any referenced terms (i.e., to trace a biography).
It is important to note that we did not intend to provide an exhaustive, systematic search of all the relevant literature. Rather, we wanted to establish the prevalence of well-being and any referenced (i.e., allied) terms within the context of public policy. This has the advantage of ensuring that any identified words are grounded in the literature (i.e., they represent words actually used by researchers to talk and write about well-being in policy settings). The searches were limited to abstracts to increase the specificity, albeit at some expense to sensitivity, with which we could identify relevant articles.
We also employed inclusion/exclusion criteria to facilitate the process by which we selected articles, thereby minimizing any potential bias arising from our subjective interpretations. We included independent, stand-alone investigations relevant to the study’s objectives (i.e., concerned with well-being in the context of public policy), which focused on well-being as a central outcome or process and which made explicit reference to “well-being” and “public policy” in either the title or the abstract. We excluded articles that were irrelevant to the study’s objectives, those that used noun adjuncts to focus on the well-being of specific populations (i.e., children, elderly, women) and contexts (e.g., retirement village), and those that focused on deprivation or poverty unless poverty indices were used to understand well-being as opposed to social exclusion. We also excluded book reviews and abstracts describing a compendium of studies.
Using these criteria, Joanne Wilson conducted the review and recorded the results on a template developed specifically for the project, organized chronologically across each database and timeframe. Results were scrutinized by two other colleagues to ensure the validity of the search strategy and the findings. Any concerns regarding the eligibility of studies for inclusion were discussed among the research team. I then analyzed the co-occurrence of the key terms in the database. The resultant conceptual map is shown in Figure 19.4.
The position of a concept in a network—a study of “well-being.” Node size is proportional to the frequency of terms in 54 selected abstracts. Line thickness is proportional to the co-occurrence of two terms in any phrase of three words (e.g., subjective well-being, economics of well-being, well-being and development).
The diagram can be interpreted as a visualization of a conceptual space. So, when academics write about well-being in the context of public policy, they tend to connect the discussion to the other terms in the matrix. “Happiness,” “health,” “economic,” and “subjective,” for example, are relatively dominant terms in the matrix. The node size of these words suggests that references to such entities is only slightly less than references to well-being itself. However, when we come to analyze how well-being is talked about in detail, we see specific connections come to the fore. Thus, the data imply that talk of “subjective well-being” far outweighs discussion of “social well-being” or “economic well-being.” Happiness tends to act as an independent node (there is only one occurrence of happiness and well-being), probably suggesting that “happiness” is acting as a synonym for well-being. Quality of life is poorly represented in the abstracts, and its connection to most of the other concepts in the space is very weak—confirming, perhaps, that quality of life is unrelated to contemporary discussions of well-being and happiness. The existence of “measures” points to a distinct concern to assess and to quantify expressions of happiness, well-being, economic growth, and gross domestic product. More important and underlying this detail, there are grounds for suggesting that there are in fact a number of tensions in the literature on well-being.
On the one hand, the results point toward an understanding of well-being as a property of individuals—as something that they feel or experience. Such a discourse is reflected through the use of words like happiness, subjective , and individual . This individualistic and subjective frame has grown in influence over the past decade in particular, and one of the problems with it is that it tends toward a somewhat content-free conceptualization of well-being. To feel a sense of well-being, one merely states that one is in a state of well-being; to be happy, one merely proclaims that one is happy (cf., Office for National Statistics, 2012 ). It is reminiscent of the conditions portrayed in Aldous Huxley’s Brave New World , wherein the rulers of a closely managed society gave their priority to maintaining order and ensuring the happiness of the greatest number—in the absence of attention to justice or freedom of thought or any sense of duty and obligation to others, many of whom were systematically bred in “the hatchery” as slaves.
On the other hand, there is some intimation in our web that the notion of well-being cannot be captured entirely by reference to individuals alone and that there are other dimensions to the concept—that well-being is the outcome or product of, say, access to reasonable incomes, to safe environments, to “development,” and to health and welfare. It is a vision hinted at by the inclusion of those very terms in the network. These different concepts necessarily give rise to important differences concerning how well-being is identified and measured and therefore what policies are most likely to advance well-being. In the first kind of conceptualization, we might improve well-being merely by dispensing what Huxley referred to as “soma” (a superdrug that ensured feelings of happiness and elation); in the other case, however, we would need to invest in economic, human, and social capital as the infrastructure for well-being. In any event and even at this nascent level, we can see how CTA can begin to tease out conceptual complexities and theoretical positions in what is otherwise routine textual data.
Putting the Content of Documents in Their Place
I suggested in my introduction that CTA was a method of analysis—not a method of data collection or a form of research design. As such, it does not necessarily inveigle us into any specific forms of either design or data collection, though designs and methods that rely on quantification are dominant. In this closing section, however, I want to raise the issue as to how we should position a study of content in our research strategies as a whole. We must keep in mind that documents and records always exist in a context and that while what is “in” the document may be considered central, a good research plan can often encompass a variety of ways of looking at how content links to context. Hence, in what follows, I intend to outline how an analysis of content might be combined with other ways of looking at a record or text and even how the analysis of content might be positioned as secondary to an examination of a document or record. The discussion calls on a much broader analysis, as presented in Prior ( 2011 ).
I have already stated that basic forms of CTA can serve as an important point of departure for many types of data analysis—for example, as discourse analysis. Naturally, whenever “discourse” is invoked, there is at least some recognition of the notion that words might play a part in structuring the world rather than merely reporting on it or describing it (as is the case with the 2002 State of the Nation address that was quoted in the section “Units of Analysis”). Thus, for example, there is a considerable tradition within social studies of science and technology for examining the place of scientific rhetoric in structuring notions of “nature” and the position of human beings (especially as scientists) within nature (see, e.g., work by Bazerman, 1988 ; Gilbert & Mulkay, 1984 ; and Kay, 2000 ). Nevertheless, little, if any, of that scholarship situates documents as anything other than inert objects, either constructed by or waiting patiently to be activated by scientists.
However, in the tradition of the ethnomethodologists (Heritage, 1991 ) and some adherents of discourse analysis, it is also possible to argue that documents might be more fruitfully approached as a “topic” (Zimmerman & Pollner, 1971 ) rather than a “resource” (to be scanned for content), in which case the focus would be on the ways in which any given document came to assume its present content and structure. In the field of documentation, these latter approaches are akin to what Foucault ( 1970 ) might have called an “archaeology of documentation” and are well represented in studies of such things as how crime, suicide, and other statistics and associated official reports and policy documents are routinely generated. That, too, is a legitimate point of research focus, and it can often be worth examining the genesis of, say, suicide statistics or statistics about the prevalence of mental disorder in a community as well as using such statistics as a basis for statistical modeling.
Unfortunately, the distinction between topic and resource is not always easy to maintain—especially in the hurly-burly of doing empirical research (see, e.g., Prior, 2003 ). Putting an emphasis on “topic,” however, can open a further dimension of research that concerns the ways in which documents function in the everyday world. And, as I have already hinted, when we focus on function, it becomes apparent that documents serve not merely as containers of content but also very often as active agents in episodes of interaction and schemes of social organization. In this vein, one can begin to think of an ethnography of documentation. Therein, the key research questions revolve around the ways in which documents are used and integrated into specific kinds of organizational settings, as well as with how documents are exchanged and how they circulate within such settings. Clearly, documents carry content—words, images, plans, ideas, patterns, and so forth—but the manner in which such material is called on and manipulated, and the way in which it functions, cannot be determined (though it may be constrained) by an analysis of content. Thus, Harper’s ( 1998 ) study of the use of economic reports inside the International Monetary Fund provides various examples of how “reports” can function to both differentiate and cohere work groups. In the same way. Henderson ( 1995 ) illustrated how engineering sketches and drawings can serve as what she calls conscription devices on the workshop floor.
Documents constitute a form of what Latour ( 1986 ) would refer to as “immutable mobiles,” and with an eye on the mobility of documents, it is worth noting an emerging interest in histories of knowledge that seek to examine how the same documents have been received and absorbed quite differently by different cultural networks (see, e.g., Burke, 2000 ). A parallel concern has arisen with regard to the newly emergent “geographies of knowledge” (see, e.g., Livingstone, 2005 ). In the history of science, there has also been an expressed interest in the biography of scientific objects (Latour, 1987 , p. 262) or of “epistemic things” (Rheinberger, 2000 )—tracing the history of objects independent of the “inventors” and “discoverers” to which such objects are conventionally attached. It is an approach that could be easily extended to the study of documents and is partly reflected in the earlier discussion concerning the meaning of the concept of well-being. Note how in all these cases a key consideration is how words and documents as “things” circulate and translate from one culture to another; issues of content are secondary.
Studying how documents are used and how they circulate can constitute an important area of research in its own right. Yet even those who focus on document use can be overly anthropocentric and subsequently overemphasize the potency of human action in relation to written text. In that light, it is interesting to consider ways in which we might reverse that emphasis and instead to study the potency of text and the manner in which documents can influence organizational activities as well as reflect them. Thus, Dorothy Winsor ( 1999 ), for example, examined the ways in which work orders drafted by engineers not only shape and fashion the practices and activities of engineering technicians but also construct “two different worlds” on the workshop floor.
In light of this, I will suggest a typology (Table 19.3 ) of the ways in which documents have come to be and can be considered in social research.
While accepting that no form of categorical classification can capture the inherent fluidity of the world, its actors, and its objects, Table 19.3 aims to offer some understanding of the various ways in which documents have been dealt with by social researchers. Thus, approaches that fit into Cell 1 have been dominant in the history of social science generally. Therein, documents (especially as text) have been analyzed and coded for what they contain in the way of descriptions, reports, images, representations, and accounts. In short, they have been scoured for evidence. Data analysis strategies concentrate almost entirely on what is in the “text” (via various forms of CTA). This emphasis on content is carried over into Cell 2–type approaches, with the key differences being that analysis is concerned with how document content comes into being. The attention here is usually on the conceptual architecture and sociotechnical procedures by means of which written reports, descriptions, statistical data, and so forth are generated. Various kinds of discourse analysis have been used to unravel the conceptual issues, while a focus on sociotechnical and rule-based procedures by means of which clinical, police, social work, and other forms of records and reports are constructed has been well represented in the work of ethnomethodologists (see Prior, 2011 ). In contrast, and in Cell 3, the research focus is on the ways in which documents are called on as a resource by various and different kinds of “user.” Here, concerns with document content or how a document has come into being are marginal, and the analysis concentrates on the relationship between specific documents and their use or recruitment by identifiable human actors for purposeful ends. I have pointed to some studies of the latter kind in earlier paragraphs (e.g., Henderson, 1995 ). Finally, the approaches that fit into Cell 4 also position content as secondary. The emphasis here is on how documents as “things” function in schemes of social activity and with how such things can drive, rather than be driven by, human actors. In short, the spotlight is on the vita activa of documentation, and I have provided numerous example of documents as actors in other publications (see Prior, 2003 , 2008 , 2011 ).
Content analysis was a method originally developed to analyze mass media “messages” in an age of radio and newspaper print, well before the digital age. Unfortunately, CTA struggles to break free of its origins and continues to be associated with the quantitative analysis of “communication.” Yet, as I have argued, there is no rational reason why its use must be restricted to such a narrow field, because it can be used to analyze printed text and interview data (as well as other forms of inscription) in various settings. What it cannot overcome is the fact that it is a method of analysis and not a method of data collection. However, as I have shown, it is an analytical strategy that can be integrated into a variety of research designs and approaches—cross-sectional and longitudinal survey designs, ethnography and other forms of qualitative design, and secondary analysis of preexisting data sets. Even as a method of analysis, it is flexible and can be used either independent of other methods or in conjunction with them. As we have seen, it is easily merged with various forms of discourse analysis and can be used as an exploratory method or as a means of verification. Above all, perhaps, it crosses the divide between “quantitative” and “qualitative” modes of inquiry in social research and offers a new dimension to the meaning of mixed methods research. I recommend it.
Abbott, A. ( 1992 ). What do cases do? In C. C. Ragin & H. S. Becker (Eds.), What is a case? Exploring the foundations of social inquiry (pp. 53–82). Cambridge, England: Cambridge University Press.
Google Scholar
Google Preview
Altheide, D. L. ( 1987 ). Ethnographic content analysis. Qualitative Sociology, 10, 65–77.
Arksey, H. , & O’Malley, L. ( 2005 ). Scoping studies: Towards a methodological framework. International Journal of Sociological Research Methodology, 8, 19–32.
Babbie, E. ( 2013 ). The practice of social research (13th ed.) Belmont, CA: Wadsworth.
Bazerman, C. ( 1988 ). Shaping written knowledge. The genre and activity of the experimental article in science . Madison: University of Wisconsin Press.
Becker, G. ( 1997 ). Disrupted lives. How people create meaning in a chaotic world . London, England: University of California Press.
Berelson, B. ( 1952 ). Content analysis in communication research . Glencoe, IL: Free Press.
Bowker, G. C. , & Star, S. L. ( 1999 ). Sorting things out. Classification and its consequences . Cambridge, MA: MIT Press.
Braun, V. , & Clarke, V. ( 2006 ). Using thematic analysis in psychology. Qualitative Research in Psychology, 3, 77–101.
Breuer, J. , & Freud, S. ( 2001 ). Studies on hysteria. In L. Strachey (Ed.), The standard edition of the complete psychological works of Sigmund Freud (Vol. 2). London, England: Vintage.
Bryman, A. ( 2008 ). Social research methods (3rd ed.). Oxford, England: Oxford University Press.
Burke, P. ( 2000 ). A social history of knowledge. From Guttenberg to Diderot . Cambridge, MA: Polity Press.
Bury, M. ( 1982 ). Chronic illness as biographical disruption. Sociology of Health and Illness, 4, 167–182.
Carley, K. ( 1993 ). Coding choices for textual analysis. A comparison of content analysis and map analysis. Sociological Methodology, 23, 75–126.
Charon, R. ( 2006 ). Narrative medicine. Honoring the stories of illness . New York, NY: Oxford University Press.
Creswell, J. W. ( 2007 ). Designing and conducting mixed methods research . Thousand Oaks, CA: Sage.
Davison, C. , Davey-Smith, G. , & Frankel, S. ( 1991 ). Lay epidemiology and the prevention paradox. Sociology of Health & Illness, 13, 1–19.
Evans, M. , Prout, H. , Prior, L. , Tapper-Jones, L. , & Butler, C. ( 2007 ). A qualitative study of lay beliefs about influenza. British Journal of General Practice, 57, 352–358.
Foucault, M. ( 1970 ). The order of things. An archaeology of the human sciences . London, England: Tavistock.
Frank, A. ( 1995 ). The wounded storyteller: Body, illness, and ethics . Chicago, IL: University of Chicago Press.
Gerring, J. ( 2004 ). What is a case study, and what is it good for? The American Political Science Review, 98, 341–354.
Gilbert, G. N. , & Mulkay, M. ( 1984 ). Opening Pandora’s box. A sociological analysis of scientists’ discourse . Cambridge, England: Cambridge University Press.
Glaser, B. G. , & Strauss, A. L. ( 1967 ). The discovery of grounded theory. Strategies for qualitative research . New York, NY: Aldine de Gruyter.
Goode, W. J. , & Hatt, P. K. ( 1952 ). Methods in social research . New York, NY: McGraw–Hill.
Greimas, A. J. ( 1970 ). Du Sens. Essays sémiotiques . Paris, France: Ėditions du Seuil.
Habermas, J. ( 1987 ). The theory of communicative action: Vol.2, A critique of functionalist reason ( T. McCarthy , Trans.). Cambridge, MA: Polity Press.
Harper, R. ( 1998 ). Inside the IMF. An ethnography of documents, technology, and organizational action . London, England: Academic Press.
Henderson, K. ( 1995 ). The political career of a prototype. Visual representation in design engineering. Social Problems, 42, 274–299.
Heritage, J. ( 1991 ). Garkfinkel and ethnomethodology . Cambridge, MA: Polity Press.
Hydén, L-C. ( 1997 ). Illness and narrative. Sociology of Health & Illness, 19, 48–69.
Kahn, R. , & Cannell, C. ( 1957 ). The dynamics of interviewing. Theory, technique and cases . New York, NY: Wiley.
Kay, L. E. ( 2000 ). Who wrote the book of life? A history of the genetic code . Stanford, CA: Stanford University Press.
Kleinman, A. , Eisenberg, L. , & Good, B. ( 1978 ). Culture, illness & care, clinical lessons from anthropologic and cross-cultural research. Annals of Internal Medicine, 88, 251–258.
Kracauer, S. ( 1952 ). The challenge of qualitative content analysis. Public Opinion Quarterly, Special Issue on International Communications Research (1952–53), 16, 631–642.
Krippendorf, K. ( 2004 ). Content analysis: An introduction to its methodology (2nd ed.). Thousand Oaks, CA: Sage.
Latour, B. ( 1986 ). Visualization and cognition: Thinking with eyes and hands. Knowledge and Society, Studies in Sociology of Culture, Past and Present, 6, 1–40.
Latour, B. ( 1987 ). Science in action. How to follow scientists and engineers through society . Milton Keynes, England: Open University Press.
Livingstone, D. N. ( 2005 ). Text, talk, and testimony: Geographical reflections on scientific habits. An afterword. British Society for the History of Science, 38, 93–100.
Luria, A. R. ( 1975 ). The man with the shattered world. A history of a brain wound ( L. Solotaroff , Trans.). Harmondsworth, England: Penguin.
Martin, A. , & Lynch, M. ( 2009 ). Counting things and counting people: The practices and politics of counting. Social Problems, 56, 243–266.
Merton, R. K. ( 1968 ). Social theory and social structure . New York, NY: Free Press.
Morgan, D. L. ( 1993 ). Qualitative content analysis. A guide to paths not taken. Qualitative Health Research, 2, 112–121.
Morgan, D. L. ( 1998 ). Practical strategies for combining qualitative and quantitative methods. Qualitative Health Research, 8, 362–376.
Morris, P. G. , Prior, L. , Deb, S. , Lewis, G. , Mayle, W. , Burrow, C. E. , & Bryant, E. ( 2005 ). Patients’ views on outcome following head injury: A qualitative study, BMC Family Practice, 6, 30.
Neuendorf, K. A. ( 2002 ). The content analysis guidebook . Thousand Oaks: CA: Sage.
Newman, J. , & Vidler, E. ( 2006 ). Discriminating customers, responsible patients, empowered users: Consumerism and the modernisation of health care, Journal of Social Policy, 35, 193–210.
Office for National Statistics. ( 2012 ). First ONS annual experimental subjective well-being results . London, England: Office for National Statistics. Retrieved from http://www.ons.gov.uk/ons/dcp171766_272294.pdf
Prior, L. ( 2003 ). Using documents in social research . London, England: Sage.
Prior, L. ( 2008 ). Repositioning documents in social research. Sociology. Special Issue on Research Methods, 42, 821–836.
Prior, L. ( 2011 ). Using documents and records in social research (4 vols.). London, England: Sage.
Prior, L. , Evans, M. , & Prout, H. ( 2011 ). Talking about colds and flu: The lay diagnosis of two common illnesses among older British people. Social Science and Medicine, 73, 922–928.
Prior, L. , Hughes, D. , & Peckham, S. ( 2012 ) The discursive turn in policy analysis and the validation of policy stories. Journal of Social Policy, 41, 271–289.
Ragin, C. C. , & Becker, H. S. ( 1992 ). What is a case? Exploring the foundations of social inquiry . Cambridge, England: Cambridge University Press.
Rheinberger, H.-J. ( 2000 ). Cytoplasmic particles. The trajectory of a scientific object. In Daston, L. (Ed.), Biographies of scientific objects (pp. 270–294). Chicago, IL: Chicago University Press.
Ricoeur, P. ( 1984 ). Time and narrative (Vol. 1., K. McLaughlin & D, Pellauer, Trans.). Chicago, IL: University of Chicago Press.
Roe, E. ( 1994 ). Narrative policy analysis, theory and practice . Durham, NC: Duke University Press.
Ryan, G. W. , & Bernard, H. R. ( 2000 ). Data management and analysis methods. In N. K. Denzin & Y. S. Lincoln (Eds.), Handbook of qualitative research (2nd ed., pp. 769–802). Thousand Oaks, CA: Sage.
Schutz, A. , & Luckman, T. ( 1974 ). The structures of the life-world (R. M. Zaner & H. T. Engelhardt, Trans.). London, England: Heinemann.
SPSS. ( 2007 ). Text mining for Clementine . 12.0 User’s Guide. Chicago, IL: SPSS.
Weber, R. P. ( 1990 ). Basic content analysis . Newbury Park, CA: Sage.
Winsor, D. ( 1999 ). Genre and activity systems. The role of documentation in maintaining and changing engineering activity systems. Written Communication, 16, 200–224.
Zimmerman, D. H. , & Pollner, M. ( 1971 ). The everyday world as a phenomenon. In J. D. Douglas (Ed.), Understanding everyday life (pp. 80–103). London, England: Routledge & Kegan Paul.
- About Oxford Academic
- Publish journals with us
- University press partners
- What we publish
- New features
- Open access
- Institutional account management
- Rights and permissions
- Get help with access
- Accessibility
- Advertising
- Media enquiries
- Oxford University Press
- Oxford Languages
- University of Oxford
Oxford University Press is a department of the University of Oxford. It furthers the University's objective of excellence in research, scholarship, and education by publishing worldwide
- Copyright © 2024 Oxford University Press
- Cookie settings
- Cookie policy
- Privacy policy
- Legal notice
This Feature Is Available To Subscribers Only
Sign In or Create an Account
This PDF is available to Subscribers Only
For full access to this pdf, sign in to an existing account, or purchase an annual subscription.
Discourse analysis: Step-by-step guide with examples
What is a discourse analysis, the application of discourse analysis in the academic thesis, discourse analysis with maxqda.
- Step 1: Importing data
- Step 2: Coding data
- Step 3: Creating Codebook
- Step 4: Visualize data
Literature about MAXQDA
Tuesday, September 19, 2023
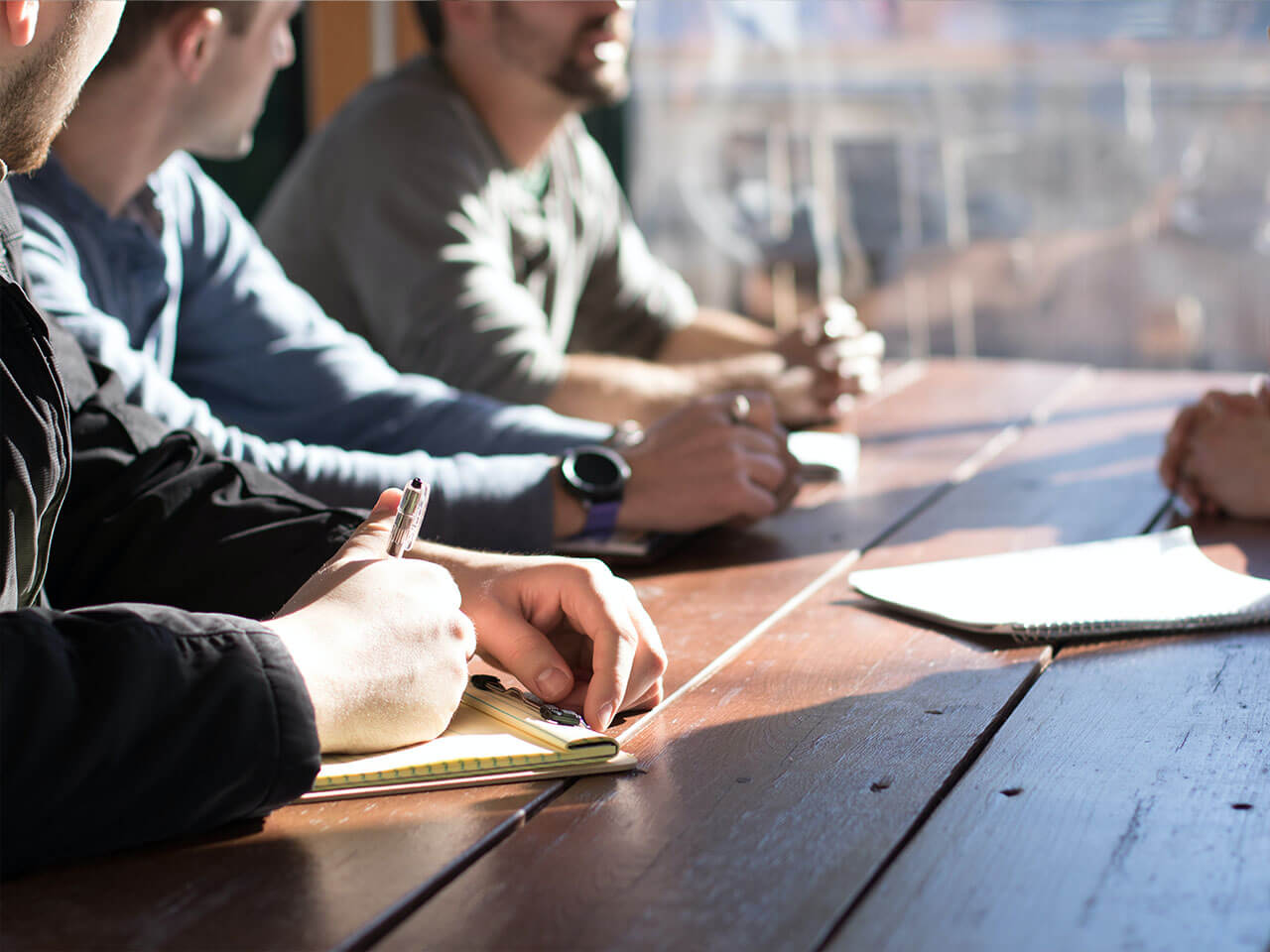
MAXQDA supports various methodological approaches, including discourse analysis. This guide will introduce you to the tools of MAXQDA, which are ideal for performing discourse analysis with MAXQDA quickly and easily. MAXQDA is a qualitative data analysis software that helps you import, code, and identify patterns in your discourse.
Discourse analysis is a multidisciplinary method used in the humanities and social sciences to develop a deeper understanding of the interactions between language, society, and culture. It focuses on the study of linguistic expressions, structures, and practices in order to capture social meanings and power dynamics. Both verbal and nonverbal communication are considered. The overarching goal of discourse analysis is to explore how discourses influence the construction of knowledge, identities, and social relations. It enables the study of the role of language and communication in shaping and influencing social reality. Overall, discourse analysis makes a valuable contribution to the study of social phenomena and processes by providing an in-depth understanding of how language and communication are used to create meanings, shape social relationships, and establish social power dynamics. Discourse analysis contributes to critical reflection and knowledge acquisition in various academic disciplines.
A primary motivation for using discourse analysis is the ability to uncover dominant discourses, ideological assumptions, and power structures in texts, media content, or political speeches. Discourse analysis allows researchers to better understand and critically reflect on the role of language and discourse in society. Another important area of application of discourse analysis in dissertations is the study of the relationship between discourses and identity constructions. For example, gender roles, ethnic identities, or sexual orientations can be studied. Discourse analysis can help to understand how identities are negotiated, constructed, and reproduced in specific social contexts. Another area of application in dissertations is the study of discourses in the media. The analysis of media discourses makes it possible to identify, critically expose and reflect on patterns and trends in reporting. This can contribute to a better understanding of the media’s role in constructing and disseminating discourses. In summary, discourse analysis offers a valuable methodological perspective for the study of complex social phenomena in the context of academic work.
Researchers typically follow these steps in discourse analysis: defining the research question, selecting relevant textual data, coding and categorizing the data, analyzing patterns and meanings within the discourse, interpreting the results, and documenting their findings in written form. The specific steps may vary depending on the research question and methodology.
As mentioned earlier, there are clear advantages to using software like MAXQDA to conduct discourse analysis. With MAXQDA, you can segment data, code it, and develop analytical ideas all at the same time. This makes the process more efficient and allows you to refine your theoretical approaches in real time. If you do not have a MAXQDA License yet, download the free 14-day trial to get started:
Download free trial
Step 1 of the discourse analysis with MAXQDA: Importing data
Importing data into MAXQDA is a crucial step in beginning the analysis of qualitative data. MAXQDA provides several options for importing data into the program, allowing you to effectively organize your research materials. You can import different types of data, such as text documents, transcripts, media content, or existing MAXQDA Projects. MAXQDA gives you the flexibility to import both individual files and entire folders of data, which is especially helpful when working with large data sets. The import process is designed to be simple and user-friendly, making it easier for you to work with your data.Another advantage of MAXQDA is that it supports a wide variety of file formats. You can import files in various formats, including TXT, DOC, PDF, MP3, MP4 and many more. This versatility allows you to work with different types of data and incorporate different media into your analysis.Importing your data into MAXQDA makes it structured and accessible for further analysis. Within MAXQDA, you can organize, code, and link your data with other analytical tools. This makes it easier to navigate and access relevant information during the analysis process.Overall, importing data into MAXQDA is an efficient way to manage your qualitative research materials and prepare them for analysis. It serves as a critical first step in launching your project in MAXQDA and taking full advantage of the program’s extensive analytical capabilities.
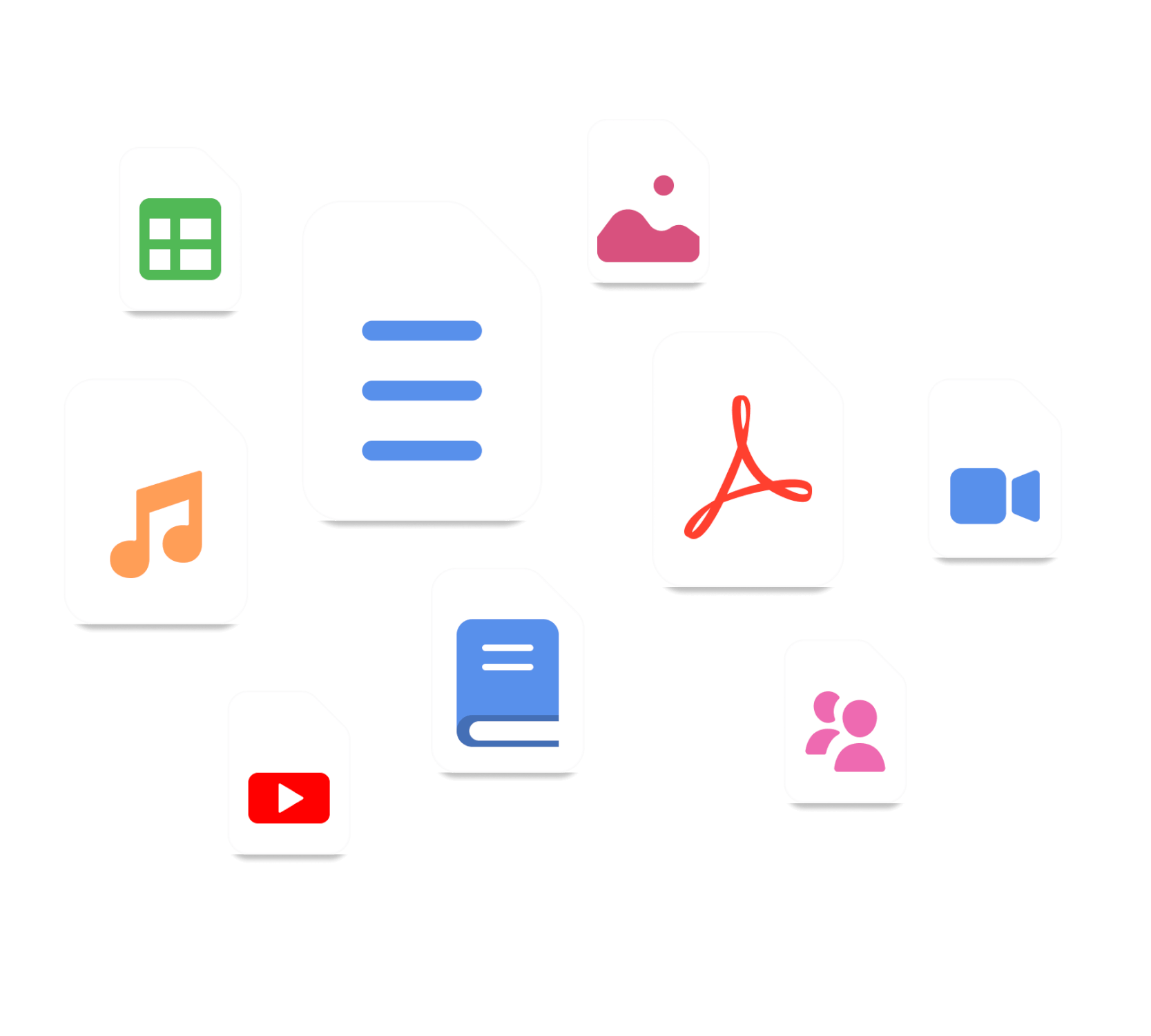
Importing data into MAXQA plays a crucial role in conducting discourse analysis. With MAXQDA, you can segment your data into documents and annotate them with relevant metadata such as title, author, and date. This allows you to organize your texts during the analysis phase. You can sort, filter, and group your data based on various criteria to access specific texts. In addition, MAXQDA provides the ability to annotate the imported text with notes, comments, or memos. This feature is invaluable for capturing important information, thoughts, or interpretations that arise during analysis. You can document your observations and insights directly in MAXQDA, thus fostering a comprehensive understanding of the discourse being analyzed.In MAXQDA, you can assign meaningful titles to your data and include relevant metadata such as author and date in the document names. This ensures a clear organization of your texts during the analysis phase. You can sort, filter, and group your data according to various criteria to access specific texts. In addition, MAXQDA allows you to annotate the imported texts with comments and notes using memos. This feature is very useful for capturing key information, thoughts, or interpretations that emerge during the analysis. You can document your observations and insights directly in MAXQDA and develop a thorough understanding of the discourse being analyzed. Importing data into MAXQDA is fundamental to conducting a systematic and comprehensive discourse analysis.The structured organization of data in MAXQDA facilitates the effective application of various analysis methods and techniques. You can create codes to identify and analyze important themes, terms, or patterns within the discourse. Importing data into MAXQDA provides a central platform where you can manage, analyze, and interpret your data. This greatly streamlines the entire process of discourse analysis, allowing you to make informed statements about social meanings, power dynamics, and identity constructions within the discourse you are analyzing.
Step 2 of the discourse analysis with MAXQDA: Coding data
Coding data in MAXQDA plays a critical role in the analysis process. Coding involves identifying and marking specific themes, categories, or concepts within the data. This allows researchers to systematically organize and extract relevant information from the data. In MAXQDA, different types of data can be coded, such as text passages, images, videos, or audio files. Codes can be used to associate these data segments with specific content or meanings. Researchers can use codes to identify and mark certain phenomena or themes in the data, allowing for targeted access later. Coding in MAXQDA allows researchers to identify complex relationships and patterns within the data.By linking and combining codes and organizing them hierarchically, researchers can establish relationships between different elements. These connections provide new insights and help understand the relationships within the data. The coded data can be further used in MAXQDA for additional analysis. For example, complex queries or filters can be applied to examine specific aspects of the discourse in detail. By analyzing the coded data, researchers can identify patterns, trends, and significant relationships that lead to valuable insights.MAXQDA provides an intuitive and easy-to-use platform to efficiently perform the coding and analysis process. The program offers several tools and features that allow researchers to customize the coding process and tailor the analysis to their specific needs. Overall, coding data in MAXQDA is a critical step in analyzing and understanding qualitative data.
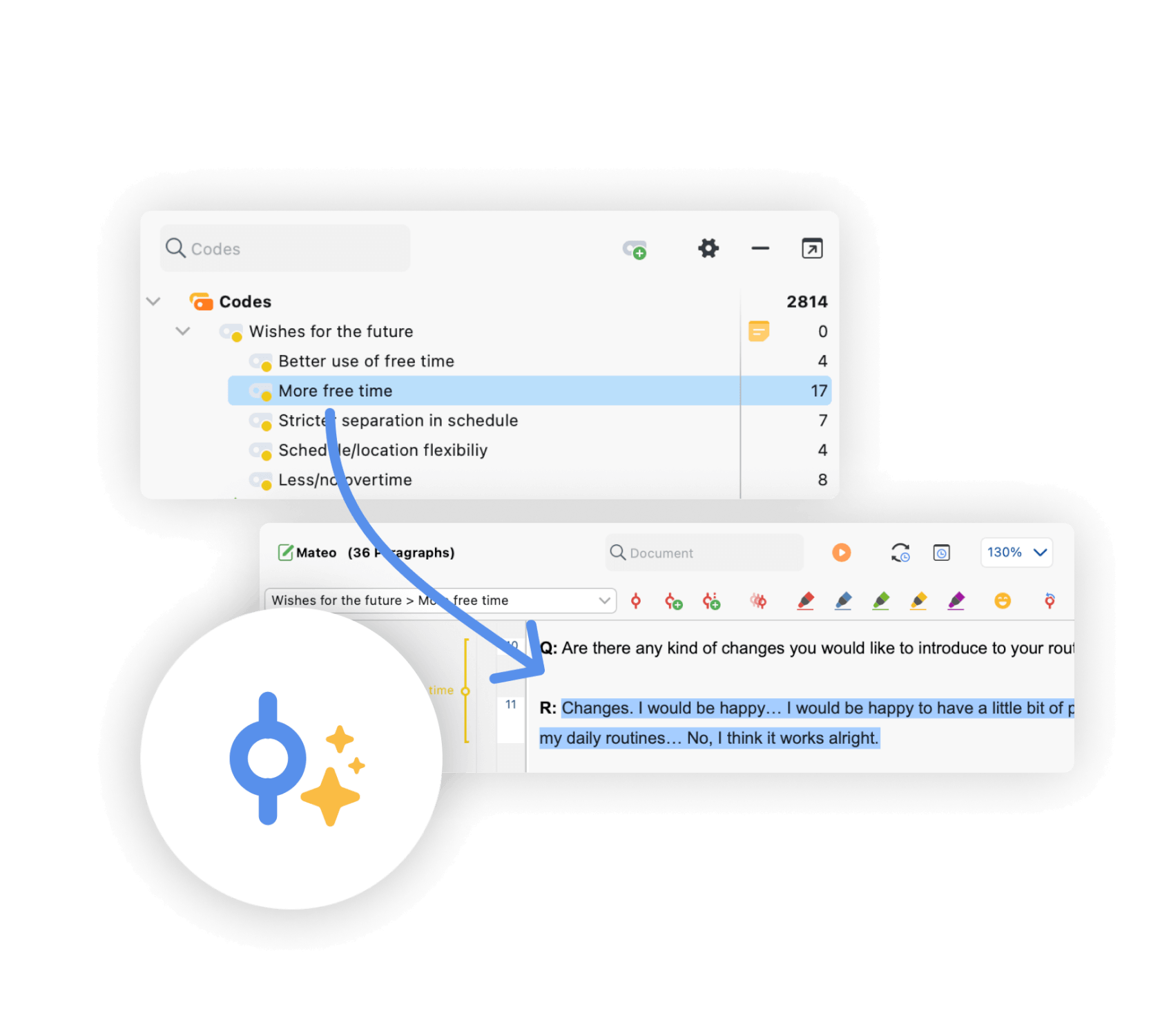
Coding data in MAXQDA allows researchers to identify and analyze specific discursive elements such as themes, arguments, or language strategies in the texts under study. To code data in MAXQDA, researchers can select relevant text passages and assign them codes that represent specific meanings or categories. These codes can be organized hierarchically to illustrate relationships between different discursive elements. In addition to coding, MAXQDA offers features such as text annotation, the ability to create memos, and options for visual data presentation at later stages. These features facilitate the organization and interpretation of coded data, enabling researchers to gain deep insights into the discourse under study and to visualize their findings. MAXQDA provides a comprehensive and efficient platform for coding and analyzing data in discourse analysis.
Step 3 of the discourse analysis with MAXQDA: Creating Codebook
A Codebook in MAXQDA defines codes for units of meaning within data. It enables structured and consistent coding, improves traceability and reproducibility, increases the efficiency of data analysis, facilitates comparisons and cross-references between codes and data, and provides flexibility and adaptability. In summary, a codebook promotes structured, consistent, and efficient data analysis, improving traceability and identification of relationships and patterns.
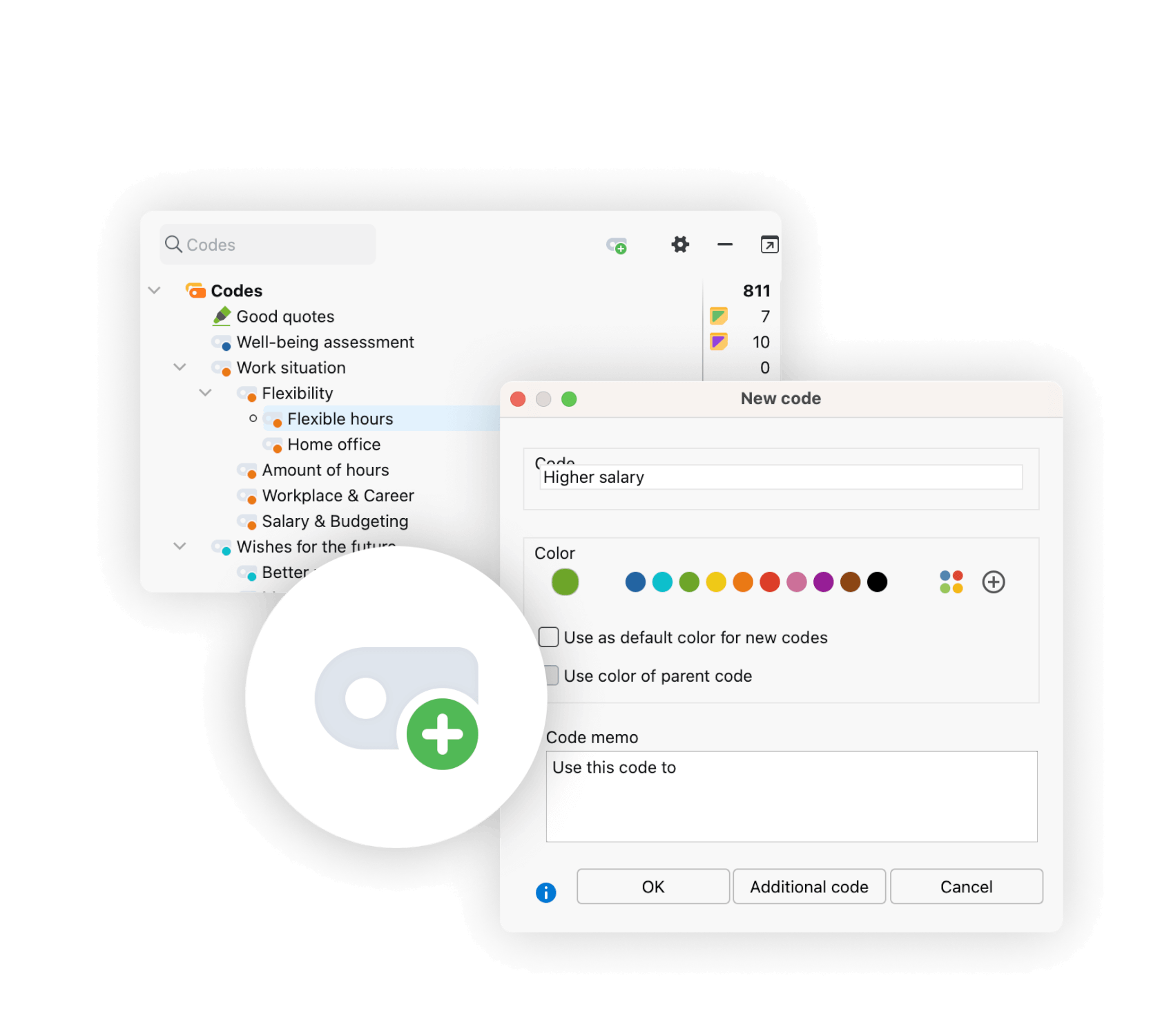
A Codebook is also very useful for discourse analysis in MAXQDA. Here are some reasons why:
- Structured coding of discourse features: A Codebook establishes uniform rules and definitions for coding data. This ensures that coding is structured and consistent across researchers and stages of analysis. This increases the reliability of results and facilitates the comparison and integration of data.
- Improved traceability and reproducibility: By clearly defining the codes and their use in the Codebook, the traceability of the coding process is improved. Other researchers can understand and trace the coding, increasing the reproducibility of the analysis. In addition, a Codebook facilitates effective collaboration and sharing of data and analysis among researchers.
- Identification and comparison of discourse patterns: A Codebook allows for the systematic identification and comparison of discourse patterns. This makes it possible to identify connections, patterns, and differences in the data, thus facilitating the interpretation of the results.
- Efficient data analysis: A Codebook provides a structured view of the codes used and their meanings. This allows researchers to work more efficiently by applying the codes quickly and specifically to relevant data. Using a codebook saves time and makes it easier to organize and navigate the coded data.
- Flexibility and adaptability: A Codebook in MAXQDA is flexible and customizable. Researchers can add, modify, or remove codes to meet the needs of their specific research questions. This allows for dynamic and iterative data analysis, where the Codebook can be continually updated and expanded.
In summary, a well-designed codebook in MAXQDA promotes structured, consistent, and efficient data analysis.
Step 4 of the discourse analysis with MAXQDA: Visualize data
MAXQDA offers a wide range of visualization tools to help you present your research data in an engaging and meaningful way. These include not only different types of charts, such as bar or pie charts for visualizing numerical data, but also other innovative visualization tools that help you identify and analyze complex relationships.
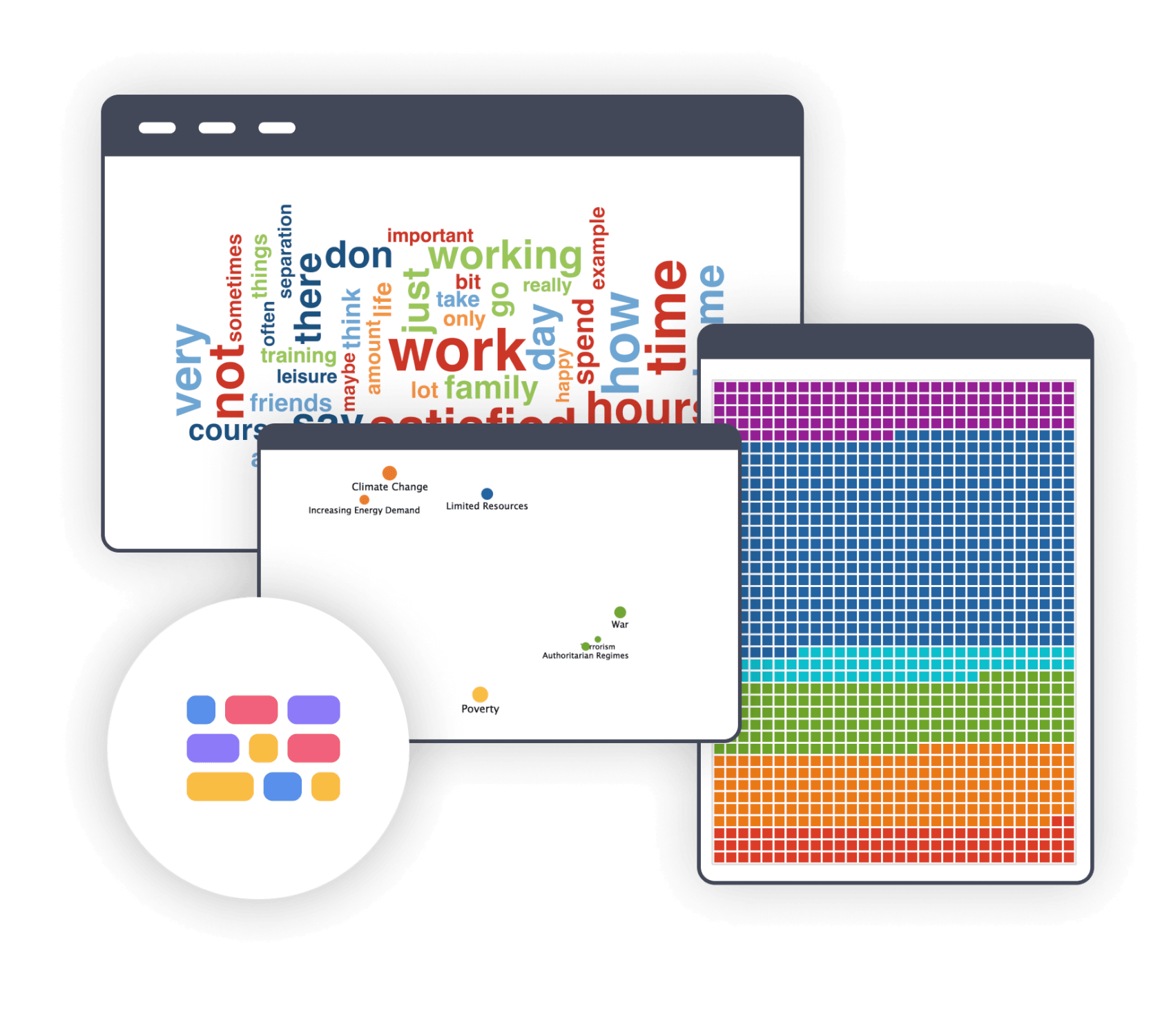
Code Matrix Browser
With the Code Matrix Browser , in MAXQDA, you can visually display and analyze the occurrence of codes in your data. This feature is invaluable for identifying similarities, differences, and patterns in discourse. Here are some of the ways the Code Matrix Browser can help you:
- Visualization of codings: The Code Matrix Browser displays a matrix where codes are arranged along the rows and documents along the columns. This visual representation allows you to quickly see which codes were used in which documents. This allows you to identify similarities and differences in the coding, which makes it easier to make connections.
- Pattern recognition: By analyzing codings in the Code Relations Browser, you can identify patterns in discourse. For example, you can observe which codes are particularly prevalent in certain documents. These patterns may indicate important themes, arguments, or language strategies, helping you to develop a more comprehensive understanding of the discourse.
- Comparison: With the Code Matrix Browser, you can compare how often certain codes were assigned in each document and display the corresponding information in the matrix. This allows you to analyze relationships between different elements in the discourse and to make connections between different topics or arguments.
Code Relations Browser
The Code Relations Browser , in MAXQDA allows you to visually display and analyze the connections and dependencies between the codes in your discourse. This feature is extremely valuable for understanding the interactions and hierarchy between codes. Here are some of the ways the Code Relations Browser can help you:
- Visualize code relationships: The Code Relations Browser visually displays the relationships between codes. You can see which codes are linked and how they are related to each other. These relationships can be hierarchical, associative, or several other types. This visual representation helps you better understand the structure and organization of codes within the discourse.
- Analyze interactions: The Code Relations Browser lets you analyze the interactions between codes. You can observe which codes occur frequently or how they influence each other. This can help you identify specific themes, arguments, or concepts in the discourse and examine their interrelationships. Analyzing these interactions can provide a deeper understanding of the discourse and the connections between codes.
The Code Map in MAXQDA visualizes selected codes as a map, showing the similarity of codes based on overlaps in the data material. Each code is represented by a circle, and the distance between the circles indicates their similarity. Larger circles represent more instances of coding with the code. Colors can highlight group membership, and connecting lines indicate overlap between codes, with thicker lines indicating more significant overlap.Visualizing the similarities between codes in the data provides an overview of different discursive elements. Grouping codes into clusters allows for the identification of specific discourse themes or dimensions. The connecting lines also show how codes interact and which codes frequently appear together. This allows for a detailed examination of the relationships between discursive elements, facilitating the interpretation and analysis of the discourse.
Document Map
The Document Map visualizes selected documents like a map. The positioning of the circles on the map is based on the similarity of the code assignments between the documents. Documents with similar code mappings are placed closer together, while those with different code mappings are placed further apart. Variable values from the documents can be used to determine similarity. Optionally, similar documents can be color-coded. Larger circles represent documents with more of the analyzed codes. The Document Map is a useful tool for visually grouping cases and can be used for typing or further investigation of the identified groups. The Document Map can be used in several ways in discourse analysis:
- Discourse group identification: By positioning documents on the map based on their code assignments, similar discourse groups can be identified. Documents with similar code assignments are placed closer together, indicating common discursive features.
- Recognition of discourse patterns: The visual representation of documents and their similarities on the map allows for the detection of patterns in discourse. Clusters of documents with similar codings may indicate common themes, arguments, or language patterns.
- Exploration of discourse dynamics: The use of connecting lines between codes on the map can reveal which codes overlap within documents. Thick connecting lines indicate frequent overlap and may suggest discursive relationships or connections.”
- Typification: The Document Map can serve as a basis for typology in discourse analysis. By grouping documents with similar code assignments, different discourse types can be identified and described”.
Profile Comparison Chart
The Profile Comparison Chart MAXQDA allows you to select multiple documents and compare the use of codes within those documents. This comparison allows you to identify differences or similarities in discourse between the selected documents. Below are some steps for using the Profile Comparison Chart:
- Document selection: Select the documents you want to compare. You can choose single documents or a group of documents. These documents should represent the discourse you want to analyze.
- Code selection: Select the codes you wish to compare in the selected documents. These can be specific themes, concepts or discursive elements that are of interest in the discourse.
- Create the comparison chart: Create the comparison graph in MAXQDA. The graph shows the occurrence of codes in individual paragraphs of the documents.
- Analysis of the chart: Analyze the comparison chart to identify differences or similarities in the discourse of the selected documents. Examine the assignment of codes in the paragraphs of the documents. Different patterns or variations in frequency may indicate differences in discourse, while similar patterns may indicate similarities in discourse.
Document Portrait
The Document Portrait feature in MAXQDA allows you to visually represent important features, themes, or characteristics of a document by visualizing the sequence of coding within that document. This feature allows you to identify relevant aspects of the discourse and analyze their weight in this particular document. Below are some steps for using the Document Portrait:
- Document Selection: Select the document for which you want to create a document portrait. The document selected should be representative of the discourse you are analyzing.
- Identify relevant features: Identify the codes that you want to visualize. These may be specific relevant features, themes or characteristics of the document, or other elements relevant to the discourse.
- Weighting of Features: The length of the segment is used as a weighting factor for the Document Portrait.
- Creation of the Document Portrait: Generate the Document Portrait in MAXQDA. The portrait visualizes the identified features and their weighting in the selected document. As a result, you obtain a visual representation of the sequence of coding performed within the document.
- Analysis of the Portrait: Analyze the Document Portrait to identify important features, themes, or characteristics of the document. This allows you to locate and understand relevant aspects of the discourse within a particular document.
The Codeline is a powerful tool in MAXQDA that allows you to visually represent the use of different codes within a document. By displaying the sequence of codes, you can see the flow and development of the discourse. With the Codeline, you can not only see which codes were used in specific sections of the document, but you can also track the progression of codings within a document. This allows you to identify crucial stages, turning points, or focal points in the discourse.The Codeline also allows you to analyze coded segments over time. You can examine specific codes and their occurrences or changes over time. This allows you to examine and interpret trends, patterns, or changes in the discourse more closely. The Codeline is therefore a valuable tool for considering the temporal progression and development of discourse in your analysis.By analyzing coded segments over time, you can gain a deeper understanding of the dynamics and context of the discourse, leading to more informed interpretations.
The Word Cloud is a powerful visualization tool in MAXQDA that helps you visually represent frequently occurring words or terms in the discourse. By looking at the size or weight of the words in the Word Cloud, you can quickly see which terms are particularly prevalent or significant in the discourse. By analyzing the Word Cloud, you can identify key terms in the discourse and examine their weight or frequency in relation to other terms. This allows you to identify and understand important themes, trends, or focuses in the discourse. In addition, you can use the Word Cloud to identify connections between different terms. If certain words occur frequently together or are used in similar contexts, you can identify associations or links in the discourse. The Word Cloud is thus a valuable tool for getting a quick and clear representation of the most common words or terms in the discourse. By analyzing the key terms and their weighting, you can gain important insights into the content and structure of the discourse and make a well-informed interpretation.
We offer a variety of free learning materials to help you get started with MAXQDA. Check out our Getting Started Guide to get a quick overview of MAXQDA and step-by-step instructions on setting up your software and creating your first project with your brand new QDA software. In addition, the free Literature Reviews Guide explains how to conduct a literature review with MAXQDA.
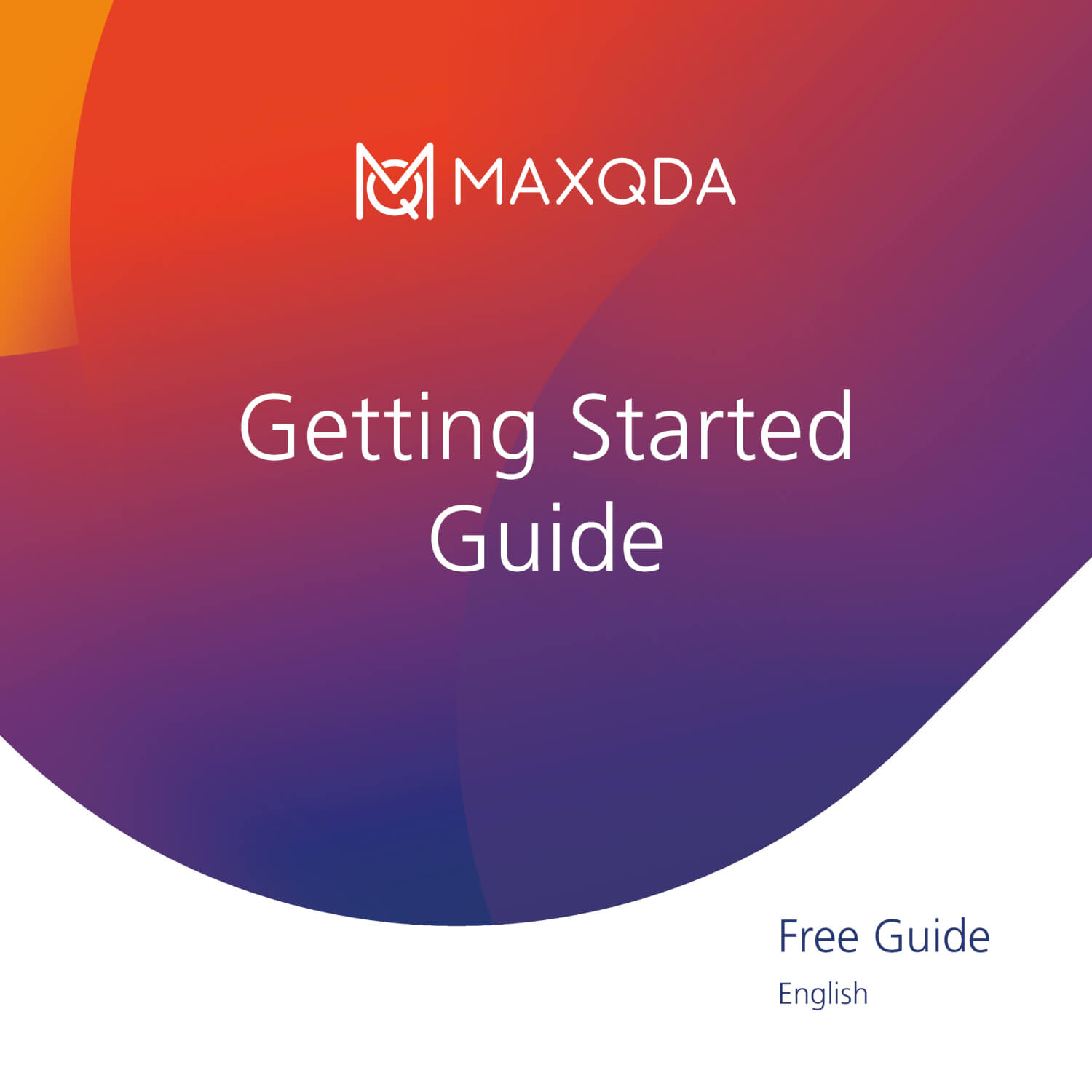
Getting Started with MAXQDA
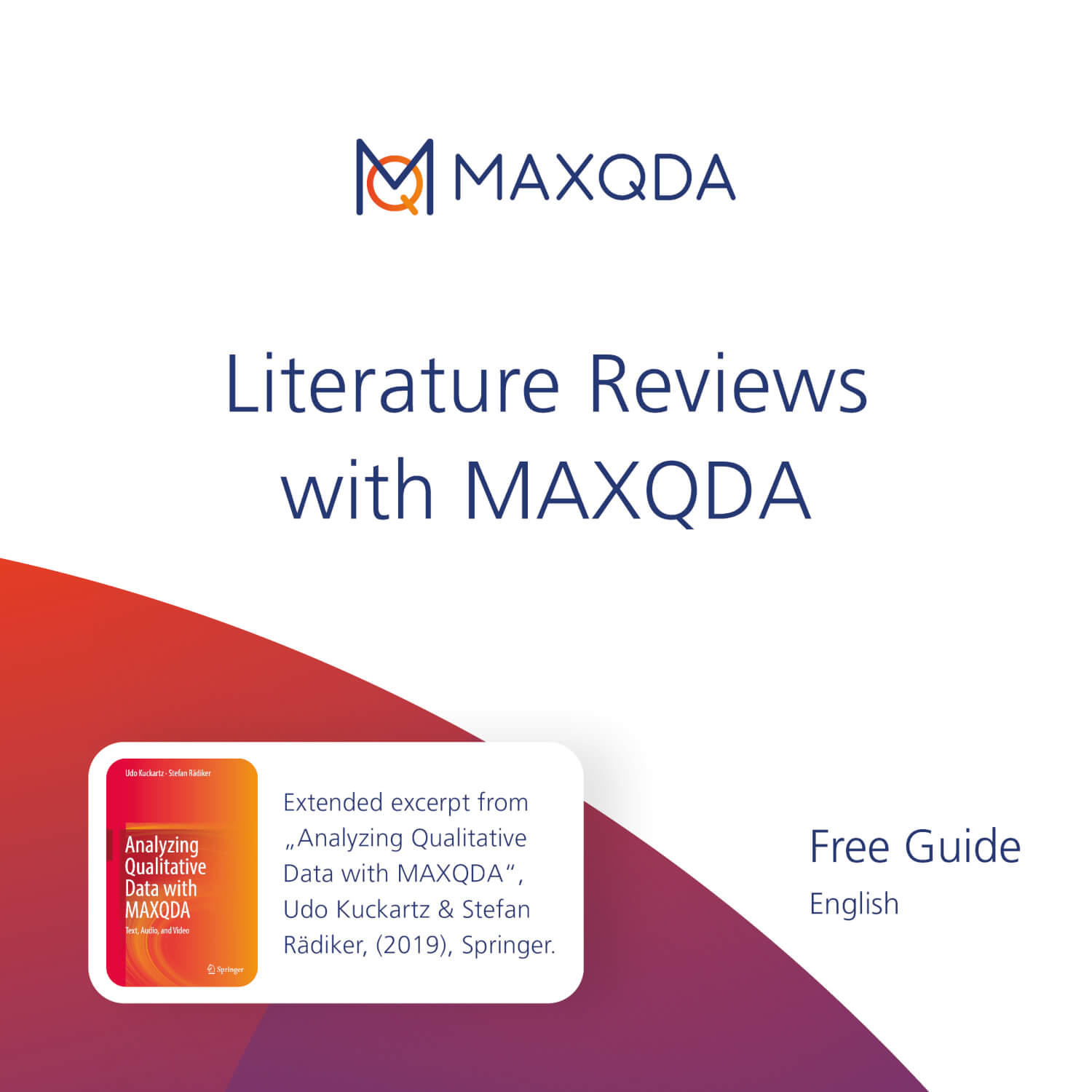
Literature Reviews with MAXQDA
MAXQDA Newsletter
Our research and analysis tips, straight to your inbox.
- By submitting the form I accept the Privacy Policy.
For an exact search, surround your words with double quotes.
Critical Discourse Analysis: Qualitative Content Approach
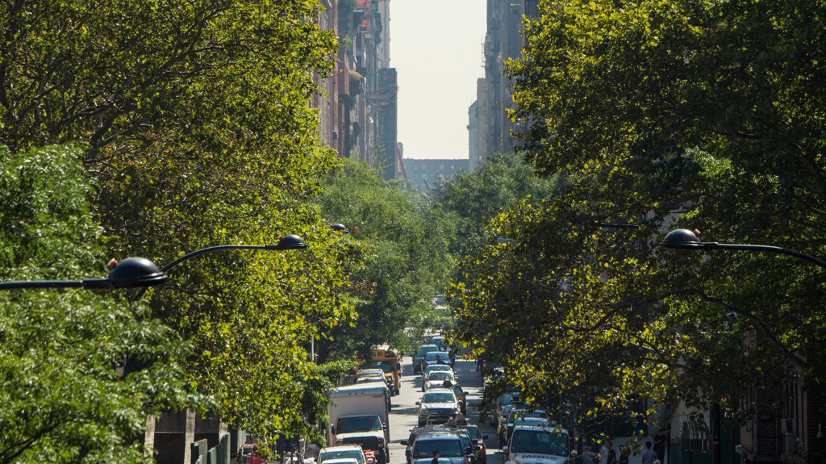
A downloadable version of this explainer is available here:
Document Explainer – CDA Qualitative Content Analysis.pdf
Previous explainers have introduced the topics of narrative and visual discourse analysis, which socio-environmental (S-E) researchers may use to identify the rhetorical impacts of images and text within a particular social context. Qualitative content analysis 1 (QCA) is an extension of narrative analysis that integrates specialized software. QCA blends qualitative and quantitative methods to organize a large number of texts into a standardized system of thematic coding, which can reveal discursive bias and cultural or organizational trends. QCA may reveal historical, geographical, and demographic patterns, as well as the ways in which archival documents may represent and preserve existing power structures and the priorities of managers invested in the status quo. By coding for categories that focus on trade-offs (for example, categorical choices among economic, social justice, and hydrological priorities), QCA can show commonalities among a wide range of texts, including links between disparate sectors, strategies used by various actors, and the regulations governing activities in socio-environmental systems.
QCA researchers often begin with quantitative methods, such as software that allows them to analyze the number of times a keyword is used in a text. Software-enabled QCA allows researchers to amplify narrative analysis by generating codes that represent a large number of specific keywords, which aid researchers in grouping the textual content into themes, categories, and subcategories. By coding keywords and themes, researchers may reveal both coding frequency (number of keyword hits), and relative thematic distribution across publications with particular topical and regional foci. The goal is to find a range of terms with similar meanings that are expressed in different ways in various media forms. Researchers decide how to code their target papers based on qualitative choices of which themes interest them and what types of sentiments they believe could be reflected in the texts.
Burke et al. (2015) showed how a team of three authors can conduct a simple qualitative analysis of theme, content, and tone within a single periodical to reveal how competing environmental discourses affect public knowledge and create or diffuse a collective desire to act toward conservation. They conducted a narrative and keyword analysis of the environmental columns of an influential regional newspaper in southern Appalachia to explore how the journalists discursively construct the environment, its interrelation with human activities, and currently favored forms of environmental governance. The authors read a large store of recent issues and agreed-upon keyword codes that indicated the content that covered target themes, including environment, science, and policy or governance. They identified 53 relevant coded segments that reveal the articles’ thematic content, which they cross-analyzed using a series of variables: the articles’ overall goal; emotional tone; people depicted; spatial and temporal scales; inclusion of environmental politics and value systems; the representation of risk; and change in the environment (such as suburbanization) or governance (forms of regulation).
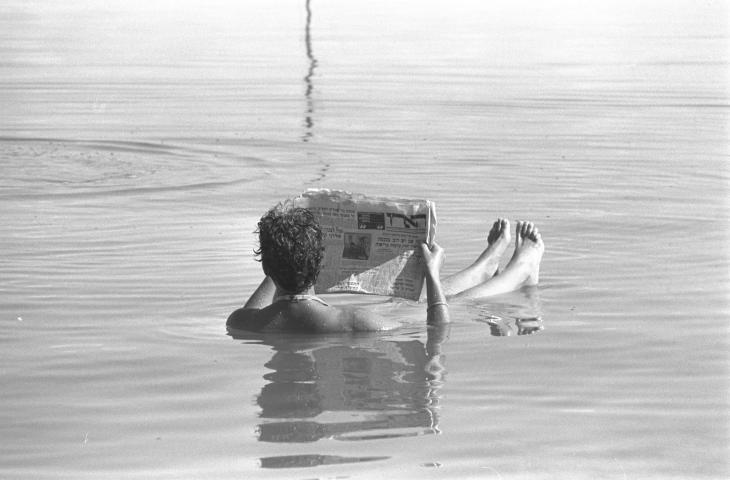
The values revealed by this QCA showed the newspaper’s dominant discourse of showcasing passive pleasure within the acculturated environment (including golf courses and gardens) rather than observing problems or suggesting active interventions to preserve natural resources. This choice of an “outdoor life” discourse with limited human agency notably departs from more activist or conservationist discourse that would attune the regional conversation to ongoing stressors like suburbanization, climate change, and inequality.
Burke et al. noted how this discursive tone aligns with a local culture of uncontroversial politeness, but the content does not represent the diversity of view nor the fundamental changes in land use and demographics that endanger many aspects of the Appalachian ecosystem. Their approach exemplifies how a small team may analyze the social implications of environmental content in a comprehensive way by limiting themselves to one target publication and using themes to cut through content and reveal the cultural values that the discourse supports. This simple reader-review approach is most effective when focusing on the characteristics of a regional discourse and a limited stock of texts (one newspaper) rather than a cross-comparison that traverses regions, document types, and the views of different disciplines or stakeholders. This SESYNC lesson guides learners through a similar discourse analysis exercise.
1 Not to be confused with Qualitative Comparative Analysis (also QCA), which combines quantitative and qualitative information from case studies to model causal conditions that can account for a full range of observed outcomes . See SESYNC’s learning resource that explains this method in detail.
QCA and Big Data
When handling larger or more diverse stores of discourse, a blend of computer-based and reader-review strategies is most effective. Software like Atlas.TI and the R packages allow researchers to upload the full text from high volumes of documents and analyze their content based on themes, coded segments, and variables like geography or document type. This strategy allows researchers to highlight cross-sector themes that may be overlooked by single discipline or single stakeholder analysis, and it may be revised iteratively as new concepts and terms emerge.
For example, Hoover et al. (2021) employed a dataset of 119 planning documents in 19 U.S. cities to examine the siting criteria for Green Infrastructure (GI) projects. The researchers were interested in how environmental justice considerations may or may not influence GI siting across cities. They used an initial keyword search of “green infrastructure” to identify relevant sections in the documents. They standardized further analysis of these hits using spreadsheets that described in narrative the types of siting criteria (environmental justice (EJ), water quality, feasibility, etc.) cross-referenced with document codes that specify the city, document, and thematic code group (hydrologic, social, economic, etc.). They then used Atlas.TI software to apply the descriptive coding regime to the list of general siting criteria codes based on GI planning documents. From this iterative coding process, they visualized 1,805 text segments across 12 siting categories and 35 subcategories (using R program’s “ggpubr” package) to examine the coding frequency and distribution across cities. This step allowed the team to create a series of visuals that illustrated the factors that influence GI siting criteria.
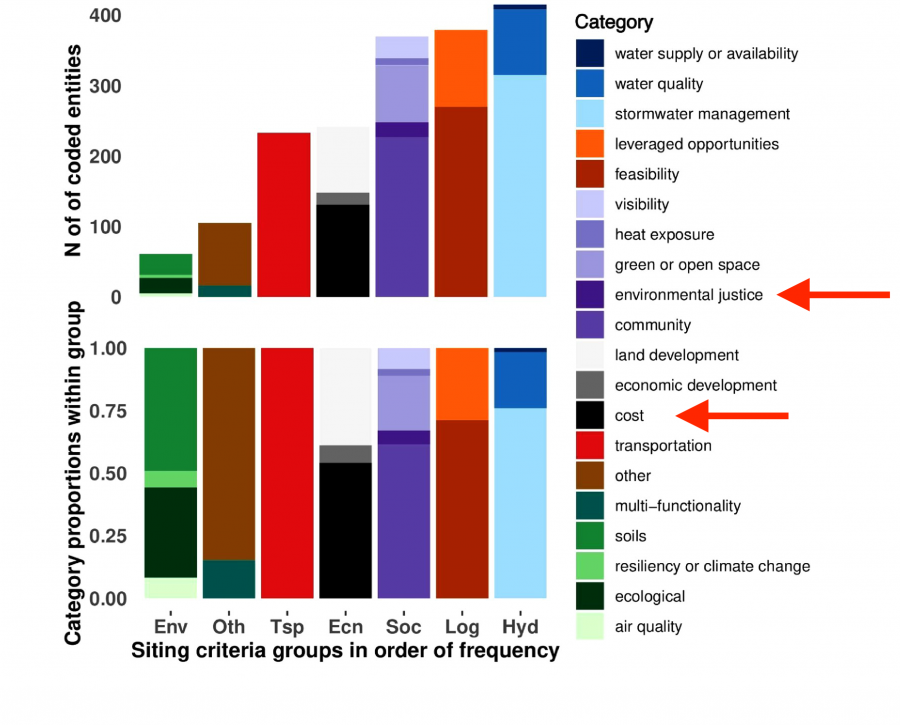
In Figure 3 from Hoover et al. (above), all 19 target cities used cost or economics as siting criteria for GI; 16 used hydrology or stormwater management; and only 7 included environmental justice or equity as criteria for the placement of GI projects. The text “environmental justice” accounted for only 1.2% of coded criteria in the seven cities in which EJ was mentioned at all in planning documents. The authors concluded that the low prevalence of EJ discourse in GI planning documents may cause unjust outcomes that prioritize investing amenities in privileged communities instead of addressing the underlying structural inequalities that give EJ communities less access to environmental benefits. By using this QCA approach, enhanced by software-driven document processing and visuals, the team generated clear and actionable results out of a large, complex discourse sample that cuts across cities, criteria, and categories. They acknowledged a key assumption of the study: the frequency and quality of specific kinds of discourse in planning documents (here, EJ inclusion) reflects actual municipal priorities and future designs for GI siting.
Furthermore, some researchers wish to contextualize official policy or planning documents with candid interviews of people with first-hand experience of S-E systems governance and operation. For example, Lund et al. (2022) used a combined QCA, narrative, and interview-based analysis approach to assess archival documents in the Senegal River Basin’s (SRB) food-energy-water (FEW) nexus with the additional consideration of health (FEW+H). For the QCA, they used deduction to develop a FEW+H nexus-specific coding scheme based on keywords and concepts they expected to encounter in planning documents. They divided excerpts among the five members of the team for coding and edited the scheme iteratively based on revised expectations of keywords and concepts after an initial analysis. Coding categories focused on trade-offs, links between FEW+H sectors, strategies used by different actors, and the regulations governing basin activities. Interviews with environmental health and hydrologic engineering staff supplemented, validated, and contextualized their QCA findings.
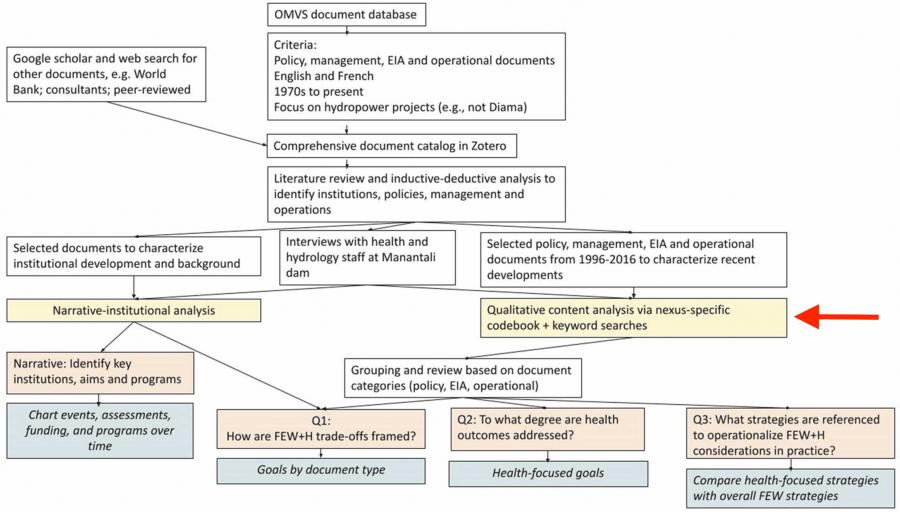
See Figure 2 above from Lund et al. The QCA (indicated by the red arrow added) shows that they identified in the policy and governance documents more goals related to FEW resources than those related to improving socio-economic, environmental, or health measures. Their complementary narrative analysis of policy and governance documents revealed that programs were designed to facilitate the mitigation of health and environmental impacts but they ultimately failed to integrate health into river basin operations. In the documents, heath is discussed as an externality to FEW priorities, and health-related excerpts represented only a small portion of coded data. In the authors’ analysis of archival documents since 1970, they found where in the planning cycle health is overlooked—between dam and reservoir operations and in key areas of decision making, such as impact assessments and basin-wide programs. Note that in their combined approach, the authors’ narrative analysis provided a history of the institution’s aims and programs while the interviews established a gap between official documents and actual operations. Lund et al.’s blended discourse analysis created an understanding of how SRB actors view the health impacts of dams: they are aware of them but fall short of managing them. The authors identify key barriers to achieving integrated sustainable development: 1) the need to generate and synthesize knowledge across sectors and 2) the use of that knowledge as a basis for decision making and implementation. These insights provided critical context for the keyword-and-code-based QCA. Without all forms of analysis, the team would not have been able to situate their findings within particular contexts of the SRB’s regulatory history.
Lund et al. noted that their findings are limited to the quality and accuracy of the documents they analyzed, a point worth considering across these discourse analysis cases. Institutional documents are assumed to reflect the policy-to-practice continuum and discuss priorities accurately, but choices in the moment are not always recorded in official documents. By adding an interview component, the team integrated more candid views of SRB dam operations than may exist in official publications.
QCA is an approachable technique for S-E researchers who possess or wish to develop basic software skills to analyze documents on a large scale. As the QCA approach reduces text to codes and excerpts to take on big archives, other forms of discourse analysis like narrative analysis and interviews may complement the QCA and provide a more textured and contextual view of the range of perspectives on an S-E issue.
Burke, B.J., Welch-Divine, M., & Gustafson, S. (2015). Nature Talk in an Appalachian Newspaper: What Environmental Discourse Analysis Reveals about Efforts to Address Exurbanization and Climate Change. Human Organization, 74 ( 2), 185- 196. http://doi.org/10.17730/0018-7259-74.2.185
Hoover, F.A., Meerow, S., Grabowski, Z.J. et al. (2021). Environmental justice implications of siting criteria in urban green infrastructure planning. Journal of Environmental Policy & Planning, 23 (5), 665-682. https://doi.org/10.1080/1523908X.2021.1945916
Lund, A.J., Harrington, E., Albrecht, T.R. (2022). Tracing the inclusion of health as a component of the food-energy-water nexus in dam management in the Senegal River Basin. Environmental Science and Policy,133 , 74–86. https://doi.org/10.1016/j.envsci.2022.03.005
Heidi Scott, SESYNC
Related Content
Critical discourse analysis resources, adaptation to resilience planning: alternative pathways to prepare for climate change, news media and fisheries-independent data reveal hidden impacts of hurricanes.

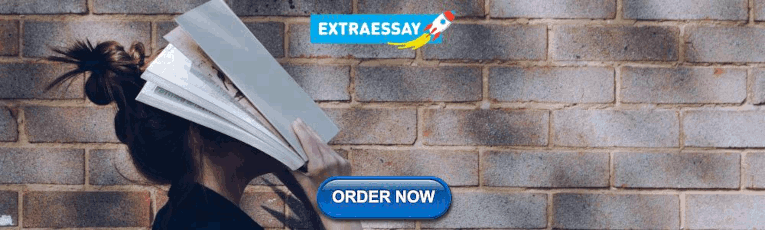
What (Exactly) Is Discourse Analysis? A Plain-Language Explanation & Definition (With Examples)
By: Jenna Crosley (PhD). Expert Reviewed By: Dr Eunice Rautenbach | June 2021
Discourse analysis is one of the most popular qualitative analysis techniques we encounter at Grad Coach. If you’ve landed on this post, you’re probably interested in discourse analysis, but you’re not sure whether it’s the right fit for your project, or you don’t know where to start. If so, you’ve come to the right place.
Overview: Discourse Analysis Basics
In this post, we’ll explain in plain, straightforward language :
- What discourse analysis is
- When to use discourse analysis
- The main approaches to discourse analysis
- How to conduct discourse analysis
What is discourse analysis?
Let’s start with the word “discourse”.
In its simplest form, discourse is verbal or written communication between people that goes beyond a single sentence . Importantly, discourse is more than just language. The term “language” can include all forms of linguistic and symbolic units (even things such as road signs), and language studies can focus on the individual meanings of words. Discourse goes beyond this and looks at the overall meanings conveyed by language in context . “Context” here refers to the social, cultural, political, and historical background of the discourse, and it is important to take this into account to understand underlying meanings expressed through language.
A popular way of viewing discourse is as language used in specific social contexts, and as such language serves as a means of prompting some form of social change or meeting some form of goal.
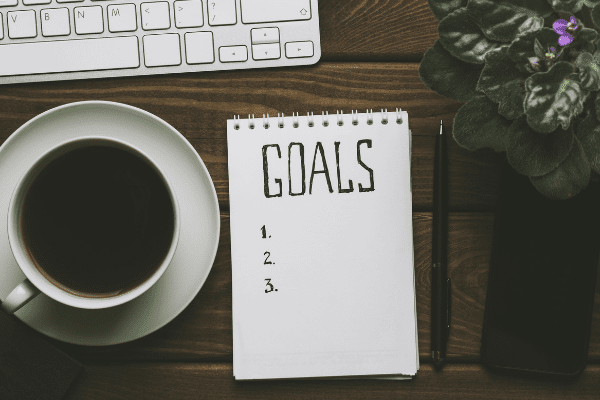
Now that we’ve defined discourse, let’s look at discourse analysis .
Discourse analysis uses the language presented in a corpus or body of data to draw meaning . This body of data could include a set of interviews or focus group discussion transcripts. While some forms of discourse analysis center in on the specifics of language (such as sounds or grammar), other forms focus on how this language is used to achieve its aims. We’ll dig deeper into these two above-mentioned approaches later.
As Wodak and Krzyżanowski (2008) put it: “discourse analysis provides a general framework to problem-oriented social research”. Basically, discourse analysis is used to conduct research on the use of language in context in a wide variety of social problems (i.e., issues in society that affect individuals negatively).
For example, discourse analysis could be used to assess how language is used to express differing viewpoints on financial inequality and would look at how the topic should or shouldn’t be addressed or resolved, and whether this so-called inequality is perceived as such by participants.
What makes discourse analysis unique is that it posits that social reality is socially constructed , or that our experience of the world is understood from a subjective standpoint. Discourse analysis goes beyond the literal meaning of words and languages
For example, people in countries that make use of a lot of censorship will likely have their knowledge, and thus views, limited by this, and will thus have a different subjective reality to those within countries with more lax laws on censorship.
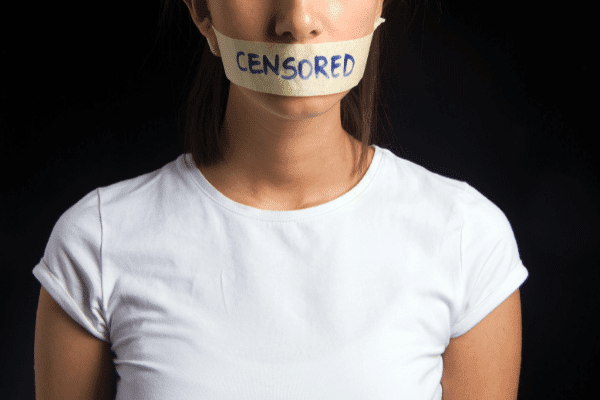
When should you use discourse analysis?
There are many ways to analyze qualitative data (such as content analysis , narrative analysis , and thematic analysis ), so why should you choose discourse analysis? Well, as with all analysis methods, the nature of your research aims, objectives and research questions (i.e. the purpose of your research) will heavily influence the right choice of analysis method.
The purpose of discourse analysis is to investigate the functions of language (i.e., what language is used for) and how meaning is constructed in different contexts, which, to recap, include the social, cultural, political, and historical backgrounds of the discourse.
For example, if you were to study a politician’s speeches, you would need to situate these speeches in their context, which would involve looking at the politician’s background and views, the reasons for presenting the speech, the history or context of the audience, and the country’s social and political history (just to name a few – there are always multiple contextual factors).
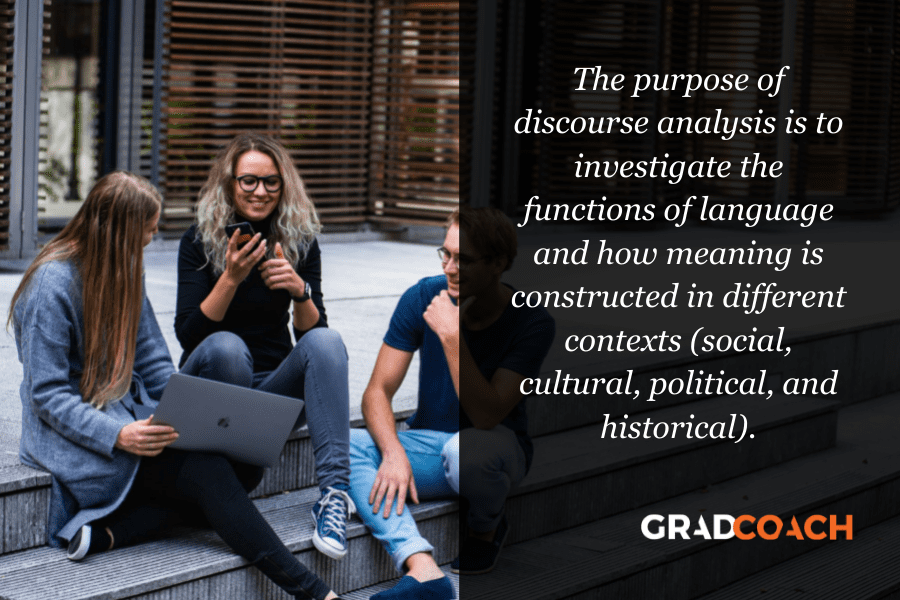
Discourse analysis can also tell you a lot about power and power imbalances , including how this is developed and maintained, how this plays out in real life (for example, inequalities because of this power), and how language can be used to maintain it. For example, you could look at the way that someone with more power (for example, a CEO) speaks to someone with less power (for example, a lower-level employee).
Therefore, you may consider discourse analysis if you are researching:
- Some form of power or inequality (for example, how affluent individuals interact with those who are less wealthy
- How people communicate in a specific context (such as in a social situation with colleagues versus a board meeting)
- Ideology and how ideas (such as values and beliefs) are shared using language (like in political speeches)
- How communication is used to achieve social goals (such as maintaining a friendship or navigating conflict)
As you can see, discourse analysis can be a powerful tool for assessing social issues , as well as power and power imbalances . So, if your research aims and objectives are oriented around these types of issues, discourse analysis could be a good fit for you.
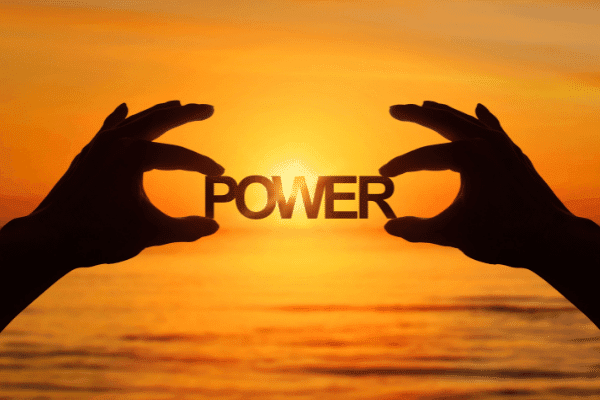
Discourse Analysis: The main approaches
There are two main approaches to discourse analysis. These are the language-in-use (also referred to as socially situated text and talk ) approaches and the socio-political approaches (most commonly Critical Discourse Analysis ). Let’s take a look at each of these.
Approach #1: Language-in-use
Language-in-use approaches focus on the finer details of language used within discourse, such as sentence structures (grammar) and phonology (sounds). This approach is very descriptive and is seldom seen outside of studies focusing on literature and/or linguistics.
Because of its formalist roots, language-in-use pays attention to different rules of communication, such as grammaticality (i.e., when something “sounds okay” to a native speaker of a language). Analyzing discourse through a language-in-use framework involves identifying key technicalities of language used in discourse and investigating how the features are used within a particular social context.
For example, English makes use of affixes (for example, “un” in “unbelievable”) and suffixes (“able” in “unbelievable”) but doesn’t typically make use of infixes (units that can be placed within other words to alter their meaning). However, an English speaker may say something along the lines of, “that’s un-flipping-believable”. From a language-in-use perspective, the infix “flipping” could be investigated by assessing how rare the phenomenon is in English, and then answering questions such as, “What role does the infix play?” or “What is the goal of using such an infix?”
Need a helping hand?
Approach #2: Socio-political
Socio-political approaches to discourse analysis look beyond the technicalities of language and instead focus on the influence that language has in social context , and vice versa. One of the main socio-political approaches is Critical Discourse Analysis , which focuses on power structures (for example, the power dynamic between a teacher and a student) and how discourse is influenced by society and culture. Critical Discourse Analysis is born out of Michel Foucault’s early work on power, which focuses on power structures through the analysis of normalized power .
Normalized power is ingrained and relatively allusive. It’s what makes us exist within society (and within the underlying norms of society, as accepted in a specific social context) and do the things that we need to do. Contrasted to this, a more obvious form of power is repressive power , which is power that is actively asserted.
Sounds a bit fluffy? Let’s look at an example.
Consider a situation where a teacher threatens a student with detention if they don’t stop speaking in class. This would be an example of repressive power (i.e. it was actively asserted).
Normalized power, on the other hand, is what makes us not want to talk in class . It’s the subtle clues we’re given from our environment that tell us how to behave, and this form of power is so normal to us that we don’t even realize that our beliefs, desires, and decisions are being shaped by it.
In the view of Critical Discourse Analysis, language is power and, if we want to understand power dynamics and structures in society, we must look to language for answers. In other words, analyzing the use of language can help us understand the social context, especially the power dynamics.
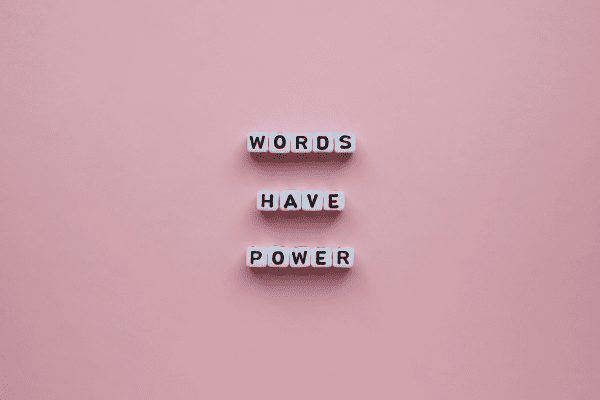
While the above-mentioned approaches are the two most popular approaches to discourse analysis, other forms of analysis exist. For example, ethnography-based discourse analysis and multimodal analysis. Ethnography-based discourse analysis aims to gain an insider understanding of culture , customs, and habits through participant observation (i.e. directly observing participants, rather than focusing on pre-existing texts).
On the other hand, multimodal analysis focuses on a variety of texts that are both verbal and nonverbal (such as a combination of political speeches and written press releases). So, if you’re considering using discourse analysis, familiarize yourself with the various approaches available so that you can make a well-informed decision.
How to “do” discourse analysis
As every study is different, it’s challenging to outline exactly what steps need to be taken to complete your research. However, the following steps can be used as a guideline if you choose to adopt discourse analysis for your research.
Step 1: Decide on your discourse analysis approach
The first step of the process is to decide on which approach you will take in terms. For example, the language in use approach or a socio-political approach such as critical discourse analysis. To do this, you need to consider your research aims, objectives and research questions . Of course, this means that you need to have these components clearly defined. If you’re still a bit uncertain about these, check out our video post covering topic development here.
While discourse analysis can be exploratory (as in, used to find out about a topic that hasn’t really been touched on yet), it is still vital to have a set of clearly defined research questions to guide your analysis. Without these, you may find that you lack direction when you get to your analysis. Since discourse analysis places such a focus on context, it is also vital that your research questions are linked to studying language within context.
Based on your research aims, objectives and research questions, you need to assess which discourse analysis would best suit your needs. Importantly, you need to adopt an approach that aligns with your study’s purpose . So, think carefully about what you are investigating and what you want to achieve, and then consider the various options available within discourse analysis.
It’s vital to determine your discourse analysis approach from the get-go , so that you don’t waste time randomly analyzing your data without any specific plan.
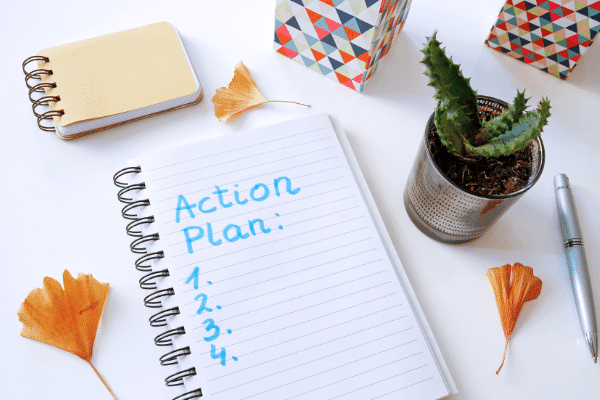
Step 2: Design your collection method and gather your data
Once you’ve got determined your overarching approach, you can start looking at how to collect your data. Data in discourse analysis is drawn from different forms of “talk” and “text” , which means that it can consist of interviews , ethnographies, discussions, case studies, blog posts.
The type of data you collect will largely depend on your research questions (and broader research aims and objectives). So, when you’re gathering your data, make sure that you keep in mind the “what”, “who” and “why” of your study, so that you don’t end up with a corpus full of irrelevant data. Discourse analysis can be very time-consuming, so you want to ensure that you’re not wasting time on information that doesn’t directly pertain to your research questions.
When considering potential collection methods, you should also consider the practicalities . What type of data can you access in reality? How many participants do you have access to and how much time do you have available to collect data and make sense of it? These are important factors, as you’ll run into problems if your chosen methods are impractical in light of your constraints.
Once you’ve determined your data collection method, you can get to work with the collection.
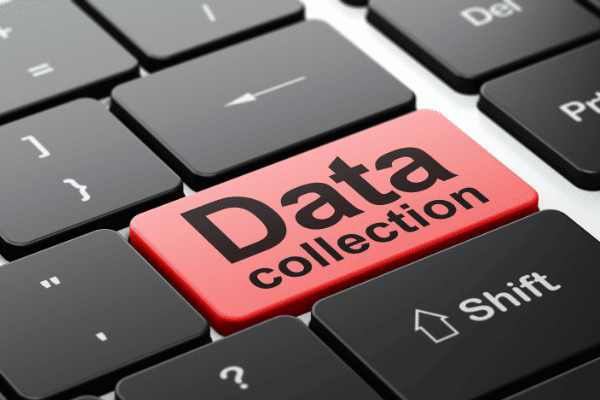
Step 3: Investigate the context
A key part of discourse analysis is context and understanding meaning in context. For this reason, it is vital that you thoroughly and systematically investigate the context of your discourse. Make sure that you can answer (at least the majority) of the following questions:
- What is the discourse?
- Why does the discourse exist? What is the purpose and what are the aims of the discourse?
- When did the discourse take place?
- Where did it happen?
- Who participated in the discourse? Who created it and who consumed it?
- What does the discourse say about society in general?
- How is meaning being conveyed in the context of the discourse?
Make sure that you include all aspects of the discourse context in your analysis to eliminate any confounding factors. For example, are there any social, political, or historical reasons as to why the discourse would exist as it does? What other factors could contribute to the existence of the discourse? Discourse can be influenced by many factors, so it is vital that you take as many of them into account as possible.
Once you’ve investigated the context of your data, you’ll have a much better idea of what you’re working with, and you’ll be far more familiar with your content. It’s then time to begin your analysis.
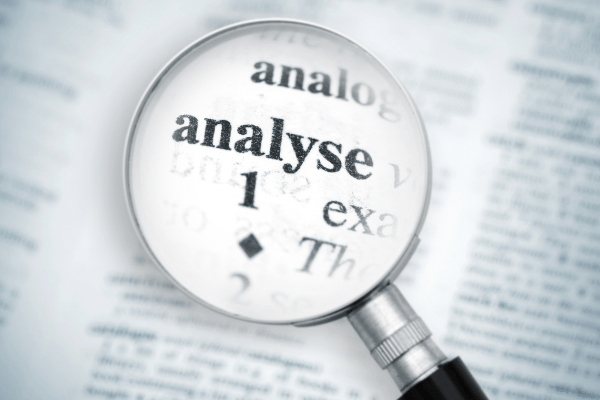
Step 4: Analyze your data
When performing a discourse analysis, you’ll need to look for themes and patterns . To do this, you’ll start by looking at codes , which are specific topics within your data. You can find more information about the qualitative data coding process here.
Next, you’ll take these codes and identify themes. Themes are patterns of language (such as specific words or sentences) that pop up repeatedly in your data, and that can tell you something about the discourse. For example, if you’re wanting to know about women’s perspectives of living in a certain area, potential themes may be “safety” or “convenience”.
In discourse analysis, it is important to reach what is called data saturation . This refers to when you’ve investigated your topic and analyzed your data to the point where no new information can be found. To achieve this, you need to work your way through your data set multiple times, developing greater depth and insight each time. This can be quite time consuming and even a bit boring at times, but it’s essential.
Once you’ve reached the point of saturation, you should have an almost-complete analysis and you’re ready to move onto the next step – final review.
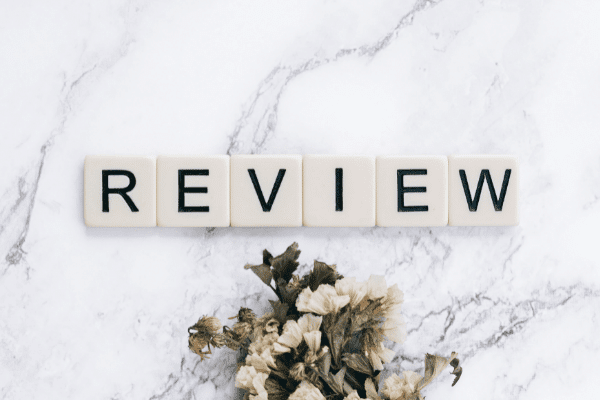
Step 5: Review your work
Hey, you’re nearly there. Good job! Now it’s time to review your work.
This final step requires you to return to your research questions and compile your answers to them, based on the analysis. Make sure that you can answer your research questions thoroughly, and also substantiate your responses with evidence from your data.
Usually, discourse analysis studies make use of appendices, which are referenced within your thesis or dissertation. This makes it easier for reviewers or markers to jump between your analysis (and findings) and your corpus (your evidence) so that it’s easier for them to assess your work.
When answering your research questions, make you should also revisit your research aims and objectives , and assess your answers against these. This process will help you zoom out a little and give you a bigger picture view. With your newfound insights from the analysis, you may find, for example, that it makes sense to expand the research question set a little to achieve a more comprehensive view of the topic.
Let’s recap…
In this article, we’ve covered quite a bit of ground. The key takeaways are:
- Discourse analysis is a qualitative analysis method used to draw meaning from language in context.
- You should consider using discourse analysis when you wish to analyze the functions and underlying meanings of language in context.
- The two overarching approaches to discourse analysis are language-in-use and socio-political approaches .
- The main steps involved in undertaking discourse analysis are deciding on your analysis approach (based on your research questions), choosing a data collection method, collecting your data, investigating the context of your data, analyzing your data, and reviewing your work.
If you have any questions about discourse analysis, feel free to leave a comment below. If you’d like 1-on-1 help with your analysis, book an initial consultation with a friendly Grad Coach to see how we can help.
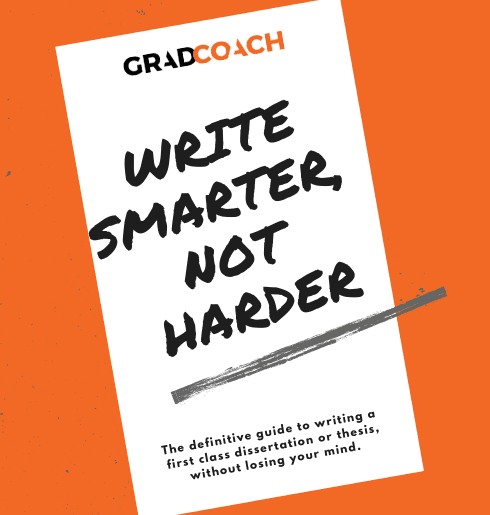
Psst… there’s more (for free)
This post is part of our dissertation mini-course, which covers everything you need to get started with your dissertation, thesis or research project.
You Might Also Like:
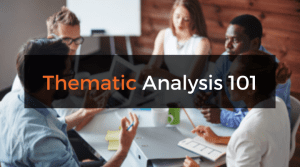
30 Comments
This was really helpful to me
I would like to know the importance of discourse analysis analysis to academic writing
In academic writing coherence and cohesion are very important. DA will assist us to decide cohesiveness of the continuum of discourse that are used in it. We can judge it well.
Thank you so much for this piece, can you please direct how I can use Discourse Analysis to investigate politics of ethnicity in a particular society
Fantastically helpful! Could you write on how discourse analysis can be done using computer aided technique? Many thanks
I would like to know if I can use discourse analysis to research on electoral integrity deviation and when election are considered free & fair
I also to know the importance of discourse analysis and it’s purpose and characteristics
Thanks, we are doing discourse analysis as a subject this year and this helped a lot!
Please can you help explain and answer this question? With illustrations,Hymes’ Acronym SPEAKING, as a feature of Discourse Analysis.
What are the three objectives of discourse analysis especially on the topic how people communicate between doctor and patient
Very useful Thank you for your work and information
thank you so much , I wanna know more about discourse analysis tools , such as , latent analysis , active powers analysis, proof paths analysis, image analysis, rhetorical analysis, propositions analysis, and so on, I wish I can get references about it , thanks in advance
Its beyond my expectations. It made me clear everything which I was struggling since last 4 months. 👏 👏 👏 👏
Thank you so much … It is clear and helpful
Thanks for sharing this material. My question is related to the online newspaper articles on COVID -19 pandemic the way this new normal is constructed as a social reality. How discourse analysis is an appropriate approach to examine theese articles?
This very helpful and interesting information
This was incredible! And massively helpful.
I’m seeking further assistance if you don’t mind.
Found it worth consuming!
What are the four types of discourse analysis?
very helpful. And I’d like to know more about Ethnography-based discourse analysis as I’m studying arts and humanities, I’d like to know how can I use it in my study.
Amazing info. Very happy to read this helpful piece of documentation. Thank you.
is discourse analysis can take data from medias like TV, Radio…?
I need to know what is general discourse analysis
Direct to the point, simple and deep explanation. this is helpful indeed.
Thank you so much was really helpful
really impressive
Thank you very much, for the clear explanations and examples.
It is really awesome. Anybody within just in 5 minutes understand this critical topic so easily. Thank you so much.
Thank you for enriching my knowledge on Discourse Analysis . Very helpful thanks again
This was extremely helpful. I feel less anxious now. Thank you so much.
Submit a Comment Cancel reply
Your email address will not be published. Required fields are marked *
Save my name, email, and website in this browser for the next time I comment.
- Print Friendly
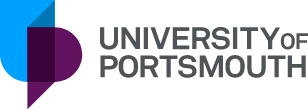
Discourse analysis
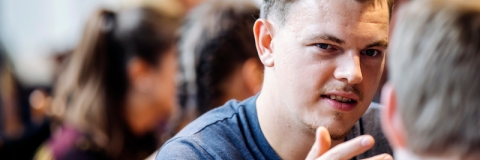
Discourse analysis research
We're exploring how language represents ideas, concepts and people
Language is not neutral, but helps us make sense of our world. How we represent ideas, concepts and people through language is of interest to discourse analysts – and our research in discourse analysis explores how language is used in real-life contexts.
In response to increased concerns about student mental health and wellbeing in higher education, we're researching narratives of loneliness – based on student interviews – that can help us unpack the potential causes of these issues, and help educators to address them.
In online spaces, where misogynistic trolling of women is a growing issue, we're analysing the discourse of hate speech, and creating a database of online hate crime examples. With this data, we're developing diagnostic tools for the police and other bodies that could further their investigations and help to eradicate the problem.
Our discourse analysis research is regularly published in leading academic journals within the field, including Discourse & Society, Gender & Language, Discourse, Context & Media, Language & Literature, and Language & Discrimination.
Our research covers the following topics
- Critical discourse analysis
- Gender and sexuality
- Mental health discourse
- Translation studies
- Systemic functional linguistics
- Media discourse
- Online discourse
Methods and memberships
Our researchers in discourse analysis use a range of qualitative and quantitative methods (often combining both for the purposes of triangulation), such as focus groups, interviews, close linguistic analysis using methods from systemic functional linguistics and critical discourse analysis, corpus linguistics and “big data” approaches.
Many of our researchers in discourse analysis are members of the Poetics and Linguistics Association (PALA), the British Association of Applied Linguistics (BAAL) and the International Gender and Language Association (IGALA).
Their membership of these bodies enables our work to reach wider audiences, and opens up opportunities for research collaborations with other members at other institutions.
Publication highlights
Exploring student loneliness in higher education: a discursive psychology approach.
Oakley, L.J. (2020) "Exploring Student Loneliness in Higher Education: A Discursive Psychology Approach", Palgrave Macmillan Ltd
Contemporary Media Stylistics
Ringrow, H. (Editor), Pihlaja, S. (Editor) (2020) "Contemporary Media Stylistics", Contemporary Studies in Linguistics
'Environment' submissions in the UK’s REF 2014
Thorpe, A., Craig, R., Tourish, D., Hadikin, G., Batistic, S. (2018) "'Environment' submissions in the UK's Research Excellence Framework 2014", British Journal of Management
Our members
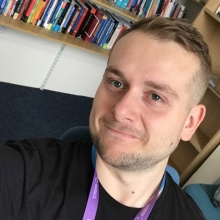
Dr Lee Oakley
Senior Lecturer
School of Education, Languages and Linguistics
Faculty of Humanities and Social Sciences
PhD Supervisor
Discover our areas of expertise
Discourse analysis is one of our six areas of expertise within our Linguistics research area. Explore the others below.
Corpus linguistics
We're looking at huge datasets of natural language – often many billions of words – to explore how language is used in different regions, genres and situations.
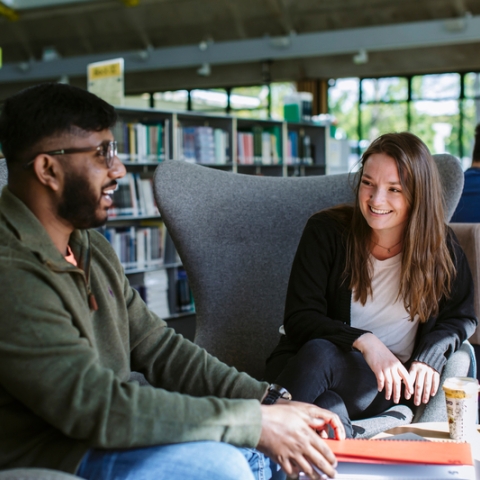
Translation
We're exploring how texts are translated and the practices around the translation of texts, including professional training, the use of technologies, and non-professional translation communities.
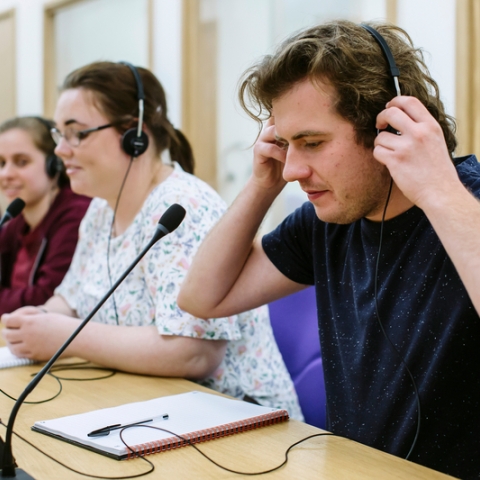
Professional communication
Our research in professional communication explores how spoken and written language is used in workplaces to develop relationships and achieve institutional objectives.
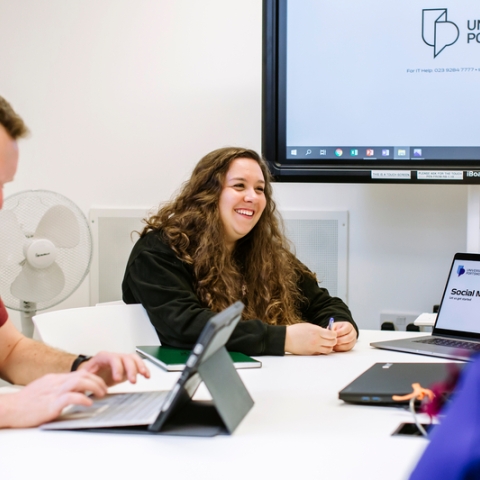
Sociolinguistics
Through our work in sociolinguistics, we're studying the ways in which language can affect, and is affected, by social phenomena.
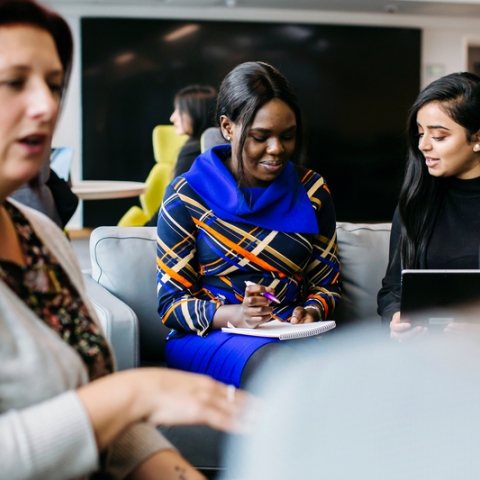
Teaching English to speakers of other languages (TESOL)
We're focusing on the learning and teaching of English as a second or foreign language, in primary, secondary and adult learning contexts.
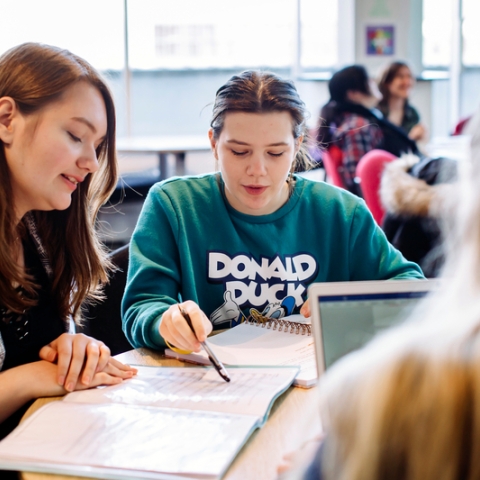
Interested in a PhD in Languages and Linguistics?
Browse our postgraduate research degrees – including PhDs and MPhils – at our Languages and Linguistics postgraduate research degrees page.
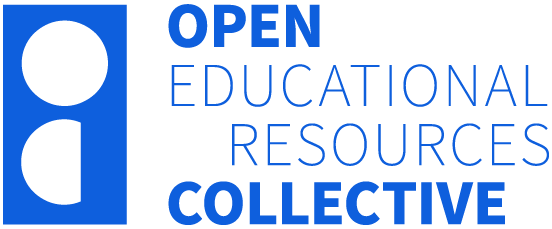
Want to create or adapt books like this? Learn more about how Pressbooks supports open publishing practices.
Chapter 23: Discourse analysis
Tess Tsindos
Learning outcomes
Upon completion of this chapter, you should be able to:
- Describe discourse analysis.
- Understand how to conduct discourse analysis.
- Identify the strengths and limitations of discourse analysis.
What is discourse analysis?
Discourse analysis is a field of qualitative analysis that has its origins in disciplines such as linguistics, philosophy, psychology, anthropology. 1 It is an interdisciplinary field that deals with ‘language’ and meaning. 2
According to Jaworski and Coupland, the purpose of discourse analysis is that it ‘offers a means of exposing or deconstructing the social practices that constitute ‘social structure’ and what we might call the conventional meaning structures of social life. It is a sort of forensic activity’. 3 ( p5 ) There are three domains of discourse analysis: the study of social interaction; the study of minds, selves and sense-making; and the study of culture and social relations. 4 ( p5 )
Discourse analysis is the study of texts such as transcribed interviews, websites, forums, books, newspapers, government documents (and many more), and the analysis of those texts to understand different accounts and the meanings behind those accounts. Qualitative researchers strive to understand the relationships between text (discourse) and social constructs. As text is analysed, the meaning behind the text is also explored, often as the ‘voices’ in the text. For example, when a participant is asked about their eating habits and they discuss their joy in eating as well as feelings of guilt from eating high-calorific foods, they may be voicing their parents’ disapproval of this eating behaviour. The relationship between text and social constructs can also be seen in alcohol advertising: an advertisement may be promoting alcohol consumption as a fun behaviour, but also cautions listeners to drink ‘responsibly’, because the advertiser is required to do so by advertising standards authorities. This inherent contradiction in the advertising is part of the meaning-making regarding alcohol consumption. This meaning-making is contextual and differs between countries, such as Australia (a high alcohol consumption culture) and Canada (a lower alcohol consumption culture). Another example of context is in the use of the word ‘just’ by an interview participant; the term can mean many things, but if the researcher is asking about job title, ‘just’ may the participant’s implication or inference that the title does not reflect an important position (e.g. ‘I’m just an editor’). In discourse analysis, texts, meanings and inferences are important.
Following is an example of media articles and two distinct discourses about violence towards women. The first media article, published by The Guardian on 15 June 2018 , 5 presents a discourse about how it is the responsibility of women to prevent men from being violent towards them. The second article about the same incident, published by The Age on 25 May 2019, 6 presents a discourse that it is the responsibility of men not to be violent towards women.
Meanings of texts are particularly important when participants use metaphors. The researcher needs to examine the implications of the metaphor, deliberate or inadvertent. For example, when the researcher asks the participant how they felt about their life and the participant replies, ‘life is a highway’, the researcher needs to look beyond what was said to understand the participant’s meaning.
As an interdisciplinary method, discourse analysis can be complex and intricate. Gee 7 provides 72 tools to assist with various types of discourse analysis, ranging from identifying what is being said and what is not being said, to examining ‘how the person is using language, as well as ways of acting, interacting, believing, valuing, dressing, and using various objects, tools, and technologies in certain sorts of environments to enact a specific socially recognizable identity and engage in one or more socially recognizable activities’. 7 ( p201 ) Gee also includes a helpful table (see Table 23.1) populated with his 7 building tasks for researchers to examine their discourses, and provides the answers. 8
Table 23.1. Seven Building Tasks and associated discourse analysis questions
How to conduct discourse analysis.
Discourse analysis, as in all other qualitative methods, is used depending on the research topic and question(s) or aim(s). The following steps are recommended:
Step 1: Have a clearly defined topic and research question, because this informs the types of research materials that will be used.
Step 2: Conduct wide-ranging searches for materials that will inform the research topic.
Step 3: Determine which theory and framework will be used as the underpinning foundation for the analyses (see Section 1 chapters 1–4).
Step 4: Analyse the content of the materials. This analysis is different (but similar) to content analysis, which is a research technique to systematically classify codes and identify themes or patterns within the data. Discourse analysis is concerned with identifying themes and patterns within the texts that relate to the social contexts reflected in the research topic and within the theoretical lens chosen for analyses.
Step 5: Interpret and draw conclusions. Reflect on your work and examine how the various texts use language within the context of the research topic to answer the research question(s).
As an example, Table 23.3 includes a study on girls’ experience of competitive dancing . 9 The authors progressed through the steps as follows:
Step 1: The topic is eating disorders and young dancers. The research question is ‘ How does experience in the world of competitive dance shape the relationship that young girls have with their bodies ?’
Step 2: The author conducted wide – ranging literature searches on eating disorders, ballet dancers, body image, thinness, Western culture, dieting, media influences and many more topics.
Step 3: Feminism was the theoretical underpinning of the text ual analys i s. As described by the authors, ‘ a feminist post structural approach was chosen to provide a critical lens to explore the beliefs, values, and practices of young dancers… aimed to provide an understanding of the dominant and competing discourses present in the world of dance and discover how these discourses are constituted, perpetuated, and form ways of knowing in relation to body and body image.’ 9(p 7 )
Ste p 4: T he transcripts were analysed in 5 steps , following Aston 10 a nd presented in table 23.2 :
Table 23.2. A guide to using feminist poststructuralism informed by discourse analysis
*Note: This table is from an Open Access article distributed under the terms of the Creative Commons Attribution License (http://creativecommons.org/licenses/by/4.0), which permits copy and redistribution of material in any medium or format, remix, transform and build upon the material for any purpose, even commercially provided the original work is properly cited.
Step 5: Results were first interpreted within an ‘environmental’ context (competitive culture, ideal dancer’s body, mirrors, and dance attire and costumes) , which was predominately negative due to the competitive culture. The second context was ‘parents’ , which encompassed body monitoring, joking, and parents and support. Although most of the dancers stated that their parents did not influence their relationship with their body, discourse analysis demonstrated that parents did influence them. The third context was ‘ coaches’ . Coaches had a very strong influence on participants’ body image. While the dancers believed their coaches were supportive, the discourse demonstrated that most coach es’ comments were negative. ‘Peers’ represented in the final context for analysis. Again, the dancers believed their peers were supportive ; however , discourse analysis demonstrated that many peer comments were negative. The conclusions drawn from the research were that ‘ all participants experienced negative physical, mental, and/or emotional repercussions throughout their competitive dance experience. It was also determined that environment, parents, coaches, and peers largely shaped the dancer’s relationship with body and body image in the world of dance. These influences generated and perpetuated the dominant negative body image discourse that dancers were often unable to resist, and consequently their relationship with body and body image suffered.’ 9(p p22-23 )
This is a good example of situating a topic (body image) within a context (young women dancing) underpinned by a theoretical framework that explores the dancers’ beliefs, values and practices.
Table 23.3. Discourse analysis examples
Advantages and challenges of discourse analysis.
Discourse analysis can be used to analyse small and large data sets with homogenous and heterogenous samples. It can be applied to any type of data source, from interviews and focus groups to diary entries, news reports and online discussion forums. However, interpretation in discourse analysis can lead to limitations and challenges that tend to occur when discourse analysis is misapplied or done poorly. Discourse analysis can be highly flexible and is best used when anchored in a theoretical approach. Because discourse analysis involves subjective interpretation, training and support from a qualitative researcher with expertise in the method is required to ensure that the interpretation of the data is meaningful. Finally, discourse analysis can be time-consuming when analysing large volumes of texts.
Discourse analysis is a process whereby texts are examined and interpreted. It looks for the meanings ‘behind’ text in cultural and social contexts. Discourse analysis is flexible, and the researcher has scope to interpret the text(s) based on the research topic and aim(s). Having a theoretical approach assists the researcher to position the discourse in cultural and social grounding.
- Schiffrin D, Tannen D et al . , ed s . The Handbook of Discourse Analysis . Blackwell ; 2001.
- Jaworski A, Coupland N. eds. The Discourse Reader . 2nd ed. Routledge; 2006.
- Jaworski A, Coupland N. Introduction: perspectives on discourse analysis. In: Jaworski A, Coupland N, eds. The Discourse Reader . 2nd ed. Routledge; 2006.
- Wetherell M, Taylor S, Yates S. (2001) Discourse Theory and Practice: A Reader . 2nd ed. Sage. 2001.
- Davey M. ‘Men need to change’: anger grows over police response to Eurydice Dixon’s murder. Guardian . June 15, 2018. Accessed April 28, 2023. https://www.theguardian.com/australia-news/2018/jun/15/men-need-to-change-anger-grows-over-police-response-to-comedians#:~:text=Melbourne
- Fowler M. ‘This is about men’s behaviour’, says top policy offer after another woman’s murder . Age . May 25, 2019. Accessed April 28, 2023. https://www.theage.com.au/national/victoria/this-is-about-men-s-behaviour-says-top-police-officer-after-another-woman-s-murder-20190525-p51r46.html
- Gee J. How t o d o Discourse Analysis: A Toolkit . 2nd ed. Routledge; 2014.
- Gee J. An Introduction to Discourse Analysis: Theory and Method . 3rd ed. Routledge; 2011.
- Doria N, Numer M. Dancing in a culture of disordered eating: a feminist poststructural analysis of body and body image among young girls in the world of dance. PLoS ONE . 2022;17(1): e0247651. doi:10.1371/journal.pone.0247651
- Aston M. Teaching feminist poststructuralism: founding scholars still relevant today. Creative Education . 2016;7(15):2251-2267. doi: 10.4236/ce.2016.715220
- Öhman A, Burman M, Carbin M et al . ‘The public health turn on violence against women’: analysing Swedish healthcare law, public health and gender-equality policies. BMC Public Health . 2020;20:753. doi:10.1186/s12889-020-08766-7
- Carrasco JM, Gómez-Baceiredo B, Navas A et al. Social representation of palliative care in the Spanish printed media: a qualitative analysis. PLoS ONE . 2019;14(1):e0211106. doi:10.1371/journal.pone.0211106
Qualitative Research – a practical guide for health and social care researchers and practitioners Copyright © 2023 by Tess Tsindos is licensed under a Creative Commons Attribution-NonCommercial 4.0 International License , except where otherwise noted.
Share This Book
Corpus-Based Discourse Analysis: Titles in Civil Engineering Research Articles
- First Online: 11 January 2022
Cite this chapter
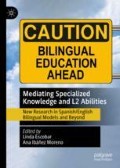
- Ana Roldan-Riejos 3
194 Accesses
The study of titles in technical discourse and, specifically, in civil engineering research articles (CERA) is a pending issue within Languages for Specific Purposes (LSP). Research into this area can benefit researchers and students of engineering. This chapter aims to fill this gap by focusing on the most common phraseological and rhetorical features of these titles. A corpus of 60 titles of CERA was compiled from six recently published journals indexed in the Web of Science (ISI). The corpus was examined both structurally (i.e. word count, word function and syntactic encoding of components) and phraseologically (i.e. word combination, word position and meaning analysis). The main research questions that this work aims to address are twofold: (i) Are the structure and content of CERA titles characterized by certain conventions (such as the degree of technical explicitness)? (ii) Do these titles contain specific and recurrent phraseological patterns, and if so, what are they? The findings of this study suggest the existence of specific traits in CERA titles, such as the correlation between title length, title type and degree of technical explicitness. The results outline the features that shape this particular engineering subgenre and could serve to inform future LSP teaching and learning material.
- Research article titles
- CERA titles
- Title length
- Title typology
- Title phraseology
This is a preview of subscription content, log in via an institution to check access.
Access this chapter
- Available as PDF
- Read on any device
- Instant download
- Own it forever
- Available as EPUB and PDF
- Compact, lightweight edition
- Dispatched in 3 to 5 business days
- Free shipping worldwide - see info
- Durable hardcover edition
Tax calculation will be finalised at checkout
Purchases are for personal use only
Institutional subscriptions
Afful, J., & Mwinlaaru, I. (2010). Commonality and individuality in academic writing: An analysis of conference paper titles of four scholars. ESP World, 9 (27), 1–30.
Google Scholar
Alcaraz, M. Á., & Méndez, D. I. (2016). When astrophysics meets lay and specialized audiences: Titles in popular and scientific papers. Journal of Language and Communication, 3 (2), 109–120.
Anthony, L. (2001). Characteristic features of research article titles in Computer Science. IEEE Transactions on Professional Communication, 44 (3), 187–194.
Article Google Scholar
Baicchi, A. (2004). The cataphoric indexicality of titles. In K. Aijmer & A. Stenström (Eds.), Discourse patterns in spoken and written corpora (pp. 17–38). John Benjamins.
Chapter Google Scholar
Bhatia, V. K. (1993). Analysing genre: Language use in professional settings . Longman.
Biber, D. (2015). Corpus-based and corpus-driven analyses of language variation and use. In: Heine, B., & Narrog, H. (Eds.). The Oxford handbook of linguistic analysis (2nd ed., pp. 1–32). Oxford University Press. Retrieved February 16, 2021, from https://www.oxfordhandbooks.com/view/10.1093/oxfordhb/9780199677078.001.0001/oxfordhb-9780199677078-e-8?print=pdf
Cheng, S. W., Kuo, C. W., & Kuo, C. H. (2012). Research article titles in applied linguistics. Journal of Academic Language and Learning, 6 (1), A1–A14.
Doykova, I. (2016, October). Title structures in research articles [Conference presentation]. 10th Jubilee International Scientific Conference “The Power of Knowledge”. Agia Triada, Greece. Retrieved June 7, 2020, from https://www.researchgate.net/publication/315760130_TITLE_STRUCTURES_IN_RESEARCH_ARTICLES
Gesuato, S. (2008). Encoding of information in titles: Practices across four genres in linguistics. In C. Taylor (Ed.), The Role E-corpora in Translation and Language Learning (pp. 127–157). Edizioni Università di Trieste.
Givón, T. (1992). Isomorphism in the grammatical code: Cognitive and biological considerations. Studies in Language, 15 (1), 85–114. Retrieved May 20, 2020, from https://www.jbe-platform.com/content/journals/10.1075/sl.15.1.04giv
Gledhill, C., & Kübler, N. (2016). What can linguistic approaches bring to English for specific purposes? Asp Journal, 69 , 65–95.
Gries, S. (2008). Phraseology and linguistic theory: A brief survey. In S. Granger & F. Meunier (Eds.), Phraseology: An interdisciplinary perspective (pp. 3–25). Amsterdam, The Netherlands: John Benjamins.
Hartley, J. (2007). Planning that title: Practices and preferences for titles with colons in academic articles. Library and Information Science Research, 29 (4), 553–568.
Hudson, J. (2016). An analysis of the titles of papers submitted to the UK REF in 2014: Authors, disciplines, and stylistic details. Scientometrics, 109 (2), 871–889.
Jamali, H. R., & Nikzad, M. (2011). Article title type and its relation with the number of downloads and citations. Scientometrics, 88 (2), 653–661.
Lewinson, G., & Hartley, J. (2005). What’s in a title? Number of words and the presence of colons. Scientometrics, 63 (2), 341–356.
Moattarian, A., & Alibabaee, A. (2015). Syntactic structures in research article titles from three different disciplines: Applied linguistics, civil engineering, and dentistry. The Journal of Teaching Language Skills (JTLS), 7 (1), Ser. 78(4), 27–50.
Picht, H. (1987). Terms and their LSP environment—LSP phraseology. Meta , 32 (2), 149–155. Retrieved January 30, 2021, from https://doi.org/10.7202/003836ar
Pípalová, R. (2017). Encoding the global theme in research articles: Syntactic and FSP parameters of academic titles and keyword sets. AUC Philologica, 1 , 91–113. https://doi.org/10.14712/24646830.2017.6
Quirk, R., & Greenbaum, S. (1979). A University Grammar of English . Longman.
Roy, I., & Soare, E. (2011). Nominalisations: New insights and theoretical implications. Recherches linguistiques de Vincennes, 40 , 7–23.
Salager-Meyer, F., & Alcaraz Ariza, M. A. (2013). Titles are “serious stuff”: A historical study of academic titles. JAHR, 4 (7), 257–271.
Salager-Meyer, F., Lewin, B., & Luzardo Briceño, M. (2017). Neutral, risky or provocative? Trends in titling practices in complementary and alternative medicine articles (1995–2016). Revista de Lenguas para Fines Específicos, 23 (2), 263–289.
Slougui, D. (2018). Dissertation titles in EFL and UK-based contexts: How much do they differ? Asp 74, 135–161. Retrieved March 15, 2020, from http://journals.openedition.org/asp/5466
Soler, V. (2007). Writing titles in science: An exploratory study. English for Specific Purposes, 26 (1), 90–102.
Swales, J. M. (1990). Genre analysis: English in academic and research setting . Cambridge University Press.
Trimble, L. (1985). English for science and technology: A discourse approach . Cambridge University Press.
Yakhontova, T. (2002). Titles of conference presentation abstracts: A crosscultural perspective. In E. Ventola, C. Shalom, & S. Thompson (Eds.), The language of conferencing (pp. 277–300). Peter Lang.
Yang, W. (2019). A diachronic keyword analysis in research article titles and cited article titles in applied linguistics from 1990 to 2016. English Text Construction, 12 (1), 84–102.
Zeng, H. (2019). A cognitive-pragmatic approach to metaphor and metonymy in brand names: A case study of film titles. Taiwan Journal of Linguistics, 17 (1), 1–47.
Download references
Author information
Authors and affiliations.
Universidad Politécnica de Madrid, Madrid, Spain
Ana Roldan-Riejos
You can also search for this author in PubMed Google Scholar
Corresponding author
Correspondence to Ana Roldan-Riejos .
Editor information
Editors and affiliations.
Faculty of Humanities, National University of Distance Education, Madrid, Spain
Linda Escobar
Ana Ibáñez Moreno
Appendix: List of Analyzed Titles
Civil Engineering Journal
Drought Scenario Analysis Using RiverWare: A Case Study in Urumqi River Basin, China
Investigation of Performance of Soil-Cement Pile in Support of Foundation Systems for High-Rise Buildings
Numerical Study of the Wake Flow of a Wind Turbine with Consideration of the Inflow Turbulence
Performance of Post-Fire Composite Prestressed Concrete Beam Topped with Reinforced Concrete Flange
A Comparative Study between Pseudo-static and Dynamic Analyses on Rock Wedge Stability of an Arch Dam
Experimental Study of Silty Clay Plane Strain Tri-axial Test under RTC Path and Modified Cam-clay Model
Laser Drilling of Small Holes in Different Kinds of Concrete
Predicting the Earthquake Magnitude Using the Multilayer Perceptron Neural Network with Two Hidden Layers
Investigation on the Mechanical Properties of Fiber Reinforced Recycled Concrete
An Experimental and Numerical Comparison of Flow Hydraulic Parameters in Circular Crested Weir Using Flow3D
International Journal of Civil Engineering
Nonlinear Dynamic Response of a RC Frame for Different Structural Conditions Including the Effect of FE Model Updating
RC Beam–Column Connections Retrofitted by Steel Prop: Experimental and Analytical Studies
Effect of Porous Media on Hydraulic Jump Characteristics by Using Smooth Particle Hydrodynamics Method
Effect of Recycled PET (Polyethylene Terephthalate) on the Electrochemical Properties of Rebar in Concrete
Flexural Capacity of Reinforced Recycled Aggregate Concrete Columns Under Seismic Loading: Database and a Simplified Model
Internal Erosion in Dams: Studies and Rehabilitation
Assessment of Foundation Mass and Earthquake Input Mechanism Effect on Dam–Reservoir–Foundation System Response
Constitutive Modelling of Wetting Deformation of Rockfill Materials
Nonlinear Seismic Response Analysis of High Arch Dams to Spatially-Varying Ground motions
Research on Rock-Filled Concrete Dam
Open Journal of Civil Engineering
Environmental and Cost Advantages of Using Polyethylene Terephthalate Fibre Reinforced Concrete with Fly Ash as a Partial Cement Replacement
Patron Survey of Acceptable Wait Times at Transit Bus Stops in the District of Columbia
Research on Construction Monitoring of Large-Span Steel Pipe Truss Structure
Comparative Analysis of Energy Performance for Residential Wall Systems with Conventional and Innovative Insulation Materials: A Case Study
An Assessment of the Seismic Performance of the Historic Tigris Bridge
Assessment of Seismic Indirect Losses Based on Utility Curves
Kinematic Storage Model (KSM) for Groundwater Development in Highly Permeable Hill Slope-Laboratory Study
Developing Suitable Proportions for the Production of Pineapple Leaf Fibers Reinforced Normal Strength Concrete
Effect of Waste Bamboo Fiber Addition on Mechanical Properties of Soil
Experimental Study of Runoff Coefficients for Different Hill Slope Soil Profiles
Advances in Civil Engineering
A Comprehensive Review on Reasons for Tailings Dam Failures Based on Case History
BIM Use by Architecture, Engineering, and Construction (AEC) Industry in Educational Facility Projects
Spatial temporal Characteristics of Tunnel Traffic Accidents in China from 2001 to Present
Stability Analysis of Slope with Multiple Sliding Surfaces Based on Dynamic Strength-Reduction DDA Method
Freeze-Thaw Cycle Effect on Sputtering Rate of Water-Saturated Yellow Sandstone under Impact Loading
Laboratory Experiments on Breaching Characteristics of Natural Dams on Sloping Beds
Updating Soil Spatial Variability and Reducing Uncertainty in Soil Excavations by Kriging and Ensemble Kalman Filter
Rethinking the Water Leak Incident of Tunnel LUO09 to Prepare for a Challenging Future
Correlation Analysis of Macroscopic and Microscopic Parameters of Coal Measure Soil Based on Discrete Element
Accuracy Assessment of Nonlinear Seismic Displacement Demand Predicted by Simplified Methods for the Plateau Range of Design Response Spectra
The Open Civil Engineering Journal
Intelligent Computing Based Formulas to Predict the Settlement of Shallow Foundations on Cohesionless Soils
Development of Ductile Truss System Using Double Small Buckling-Restrained Braces: Analytical Study
Experimental Investigation of Skirt Footing Subjected to Lateral Loading
Seismic Behaviour of 17th Century Khusro Tomb due to Site-Specific Ground Motion
Adequacy of the ASTM C1240 Specifications for Nanosilica Pozzolans
Challenges Facing Small-sized Construction Firms in the Gaza Strip
Simulation of Pressure Head and Chlorine Decay in a Water Distribution Network: A Case Study
Application of ANN Predictive Model for the Design of Batch Adsorbers—Equilibrium Simulation of Cr(VI) Adsorption onto Activated Carbon
Properties of Modified High Permeable Concrete with a Crumb Rubber
Structural Behavior of Geopolymer Concrete Thin Wall Panels Based on Metakaolin and Recycled Concrete Aggregate
International Journal of Concrete Structures and Materials
Experimental and Numerical Assessment of Reinforced Concrete Beams with Disturbed Depth
Seismic Performance of Precast Concrete Columns with Improved U-type Reinforcement Ferrule Connections
Experimental Studies on Bond Performance of BFRP Bars Reinforced Coral Aggregate Concrete
Axial–Flexural Interaction in FRP-Wrapped RC Columns
A Hysteretic Constitutive Model for Reinforced Concrete Panel Elements
Shear Friction Characteristics and Modification Factor of Concrete Prepared Using Expanded Bottom Ash and Dredged Soil Granules
Experimental Quantification on the Residual Seismic Capacity of Damaged RC Column Members
A New 3D Empirical Plastic and Damage Model for Simulating the Failure of Concrete Structure
Estimation of Compressive Strength and Member Size of Steel Fiber Reinforced Concrete Using Stress Wave-Driven Nondestructive Test Methods
Shear Resistance Prediction of Post-fire Reinforced Concrete Beams Using Artificial Neural Network
Rights and permissions
Reprints and permissions
Copyright information
© 2021 The Author(s), under exclusive license to Springer Nature Switzerland AG
About this chapter
Roldan-Riejos, A. (2021). Corpus-Based Discourse Analysis: Titles in Civil Engineering Research Articles. In: Escobar, L., Ibáñez Moreno, A. (eds) Mediating Specialized Knowledge and L2 Abilities. Palgrave Macmillan, Cham. https://doi.org/10.1007/978-3-030-87476-6_15
Download citation
DOI : https://doi.org/10.1007/978-3-030-87476-6_15
Published : 11 January 2022
Publisher Name : Palgrave Macmillan, Cham
Print ISBN : 978-3-030-87475-9
Online ISBN : 978-3-030-87476-6
eBook Packages : Social Sciences Social Sciences (R0)
Share this chapter
Anyone you share the following link with will be able to read this content:
Sorry, a shareable link is not currently available for this article.
Provided by the Springer Nature SharedIt content-sharing initiative
- Publish with us
Policies and ethics
- Find a journal
- Track your research
Introducing Discourse Analysis for Qualitative Research
Qualitative researchers often try to understand the world by listening to how people talk, but it can be really revealing to look at not just what people say, but how. This is how discourse analysis (DA) can be used to examine qualitative data.
Daniel Turner
Qualitative research often focuses on what people say: be that in interviews , focus-groups , diaries , social media or documents . Qualitative researchers often try to understand the world by listening to how people talk, but it can be really revealing to look at not just what people say, but how. Essentially this is the how discourse analysis (DA) can be used to examine qualitative data. Discourse is the complete system by which people communicate, it’s the widest interpretation of what we call ‘language’. It includes both written, verbal and non-verbal communication, as well as the wider social concepts that underpin what language means, and how it changes. For example, it can be revealing to look at how some people use a particular word, or terms from a particular local dialect. This can show their upbringing and life history, or influences from other people and workplace culture. It can also be interesting to look at non-verbal communication: people’s facial expressions and hand movements are an important part of the context of what people say. But language is also a dynamic part of culture, and the meanings behind terms change over time. How we understand terms like ‘fake news’ or ‘immigration’ or ‘freedom’ tells us a lot, not just about the times we live in or the people using those terms, but groups that have power to change the discourse on such issues. We will look at all these as separate types of discourse analysis. But first it’s important to understand why language is so important; it is much more than just a method of communication.
“Language allows us to do things. It allows us to engage in actions and activities. We promise people things, we open committee meetings, we propose to our lovers, we argue over politics, and we “talk to God”…
Language allows us to be things. It allows us to take on different socially significant identities. We can speak as experts—as doctors, lawyers, anime aficionados, or carpenters—or as ‘everyday people’. To take on any identity at a given time and place we have to ‘talk the talk’…” - Gee 2011
Language is more than a neutral way of communicating, it’s deeply connected with actions and personal identity, and can even shape the way we think about and understand the world. Who we are, what we do, and our beliefs are all shaped by the language we use. This makes it a very rich avenue for analysis.
Types of discourse analysis Just like so many blanket qualitative terms , there are a lot of different practices and types of analysis called ‘discourse’ analysis, and many different ways of applying them. Hodges et al. (2008) identify 3 meta-types, broadly going from more face-value to conceptual analysis: • Formal linguistic (basically looking at words/phrases, grammar or semantics) • Empirical (social practice constructed through text) • Critical (language constructing and limiting thought)
Tannen et al., 2015 categorise three similar broad types of analysis, again becoming increasingly socially conceptual:
• language use
• anything beyond the sentence
• a broader range of social practice that includes non-linguistic and non-specific instances of language
However Gee (2011) only recognises two main categories, essentially those that look at the use of words, and ‘critical discourse analysis’: like the latter of both groupings above, this is analysis of how language is situated in cultural and contextual power dynamics. But before we get there, let’s start with an example of some more obvious linguistic level discourse analysis.
Example Imagine the following scenario from your favourite fictional medical drama. A patient is wheeled into the ER/casualty unit, conscious but suffering from burns. The doctor attending says three things:
To Patient: “We’re just going to give you a little injection to help with the pain.”
To Nurse: “10cc’s of sodium pentothal, stat!”
To Surgeon: “We’ve got severe second-degree chemical burns, GA administered”
In this situation, the doctor has said essentially the same thing 3 times, but each time using a different response for each recipient. Firstly, when talking to the patient, the doctor doesn’t use any medical terminology, and uses calming and minimising language to comfort the patient. This is a classic type of discourse we are familiar with from medical TV dramas, the ‘good bed-side manner’.
To the nurse, the doctor has a different tone, more commanding and even condescending. It’s a barked command, finished with the term ‘stat!’ - a commonly used medial slang word (actually from the Latin word ‘statum’ meaning immediately, that’s your linguistic analysis!). This is interesting, because it’s not a term you’d hear used in other professional places like a busy kitchen. It shows there is a specific discourse for the setting (a hospital) and for different people in the setting. The ‘10cc of sodium pentothal’ is a commonly used anaesthetic: the same ‘something to help with the pain’ but now with a (trademarked) pharmacological name and dose.
Finally, to the surgeon the same prescription is described by the doctor as an abbreviation (GA for General Anaesthetic). Between senior health professionals, abbreviations might be used more often, in this case actually hiding the specific drug given, perhaps on the basis that the surgeon doesn’t need to know. It could also imply that since only that basic first step has been made, there has been little assessment or intervention so far, telling to an experienced ear what stage of the proceedings they are walking in on. The use of the term ‘we’ might imply the doctor and surgeon are on the same level, as part of the team, a term not used when addressing the nurse.
Even in this small example, there are a lot of different aspects of discourse to unpack. It is very contextually dependent, none of the phrases or manners are likely to be adopted by the doctor in the supermarket or at home. This shows how the identity and performativity of the doctor is connected to their job (and shaped by it, and contextual norms). It also shows differences in discourse between different actors, and power dynamics which are expressed and created through discursive norms.
At a very basic level, we could probably do an interesting study on TV shows and the use of the term ‘stat!’. We could look at how often the term was used, how often it was used by doctors to nurses (often) and by nurses to doctors (rarely). This would probably be more like a basic linguistic analysis, possibly even quantitative. It’s one of the few occasions that a keyword search in a qualitative corpus can be useful – because you are looking at the use of a single, non-replaceable word. If someone says ‘now please’ or ‘as soon as you can’ it has a very different meaning and power dynamic, so we are not interested in synonyms here. However, we probably still want to trawl through the whole text to look at different phrases that are used, and why ‘stat!’ was not the command in all situations. This would be close to the ‘formal linguistic’ approach listed above.
But a more detailed, critical and contextual examination of the discourse might show that nurses struggle with out-moded power dynamics in hospitals (eg Fealy and McNamara 2007 , Turner et al 2007 ). Both of these papers are described as ‘critical’ discourse analysis. However, this term is used in many different ways.
Critical discourse analysis is probably the most often cited, but often used in the most literal sense – that it looks at discourse critically, and takes a comparative and critical analytic stance. It’s another term like ‘grounded theory’ that is used as a catch-all for many different nuanced approaches. But there is another ‘level’ of critical discourse analysis, influenced by Foucault (1972, 1980) and others, that goes beyond reasons for use and local context, to examine how thought processes in society influenced by the control of language and meanings.
Critical discourse analysis (hardcore mode)
“What we commonly accept as objective or obviously true is only so because of negotiated agreement among people” – Gee (2011)
Language and discourse are not absolute. Gee (2011) notes at least three different ways that the positionality of discourse can be shown to be constructed and non-universal: meanings and reality can change over time, between cultures, and finally with ‘discursive construction’ – due to power dynamics in setting language that controls how we understand concepts. Gee uses the term ‘deconstruction’ in the Derridian sense of the word, advocating for the critical examining and dismantling of unquestioned assumptions about what words mean and where they come from.
But ‘deep’ critical discourse analysis also draws heavily from Foucault and an examination of how language is a result of power dynamics, and that the discourse of society heavily regulates what words are understood to mean, as well as who can use them. It also implies that because of these systems of control, discourse is used to actually change and reshape thought and expression. But the key jump is to understand and explain that “what we take to be the truth about the world importantly depends on the social relationships of which we are a part” (Gergen 2015). This is social construction, and a key part of the philosophy behind much critical discourse analysis.
Think of the use of the term ‘freedom’ in mainstream and political discourse in the United States. It is one of the most powerful words used by politicians, and has been for centuries (eg Chanley and Chanley 2015 ) However, it’s use and meaning have changed over time, and what different people from different parts of the political spectrum understand to be enshrined under this concept can be radically different, and even exclusionary. Those in powerful political and media positions are able to change the rhetoric around words like freedom, and sub-terms like ‘freedom of speech’ and ‘freedom of religion’ are both being shifted in public discourse, even on a daily basis, and taking our own internal concepts and ideas with them. It may be that there has never been an age when so much power to manipulate discourse is concentrated in so few places, and able to shift it so rapidly.
Doing Discourse
So do we ‘do’ discourse analysis? How can we start examining complex qualitative data from many voices from a point of view of discourse? Like so many qualitative analytical techniques , researchers will usually adopt a blend of approaches: doing some elements of linguistic analysis, as well as critical discourse analysis for some parts or research questions. They may also draw on narrative and thematic analysis . But discourse analysis is often comparative, it lends itself to differences in the use of language between individuals, professionals and contexts.
From a practical point of view, it can be started by a close reading of key words and terms, especially if it is not clear from the outset what the important and illustrative ones are going to be. For building a complete picture of discourse, a line-by-line approach can be adopted, but it’s also useful to use ‘codes’ or ‘themes’ to tag every use of some terms, or just significant ones. A qualitative software tool like Quirkos can help you do this.

For critical discourse analysis, examination of primary data is rarely enough – it needs to be deeply contextualised within the wider societal or environmental norms that govern a particular subset of discourse. So policy and document analysis are often entwined and can be analysed in the same project. From here, it’s difficult to describe a single technique further, as it will greatly vary by type of source. It is possible in discourse analysis for a single sentence or word to be the major focus of the study, or it may look widely across many different people and data sources.
The textbooks below are all classic works on discourse analysis, each a rabbit hole in itself to digest (especially the new edition of Gergen (2015) which goes much wider into social construction). However, Hodges et al. (2008) is a nice short, practical overview to start your journey.
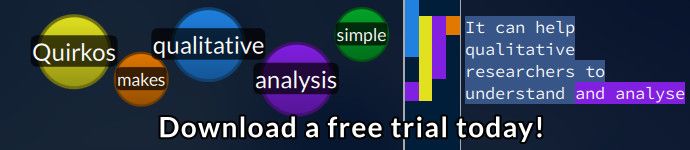
If you are looking for a tool to help your qualitative discourse analysis, why not give Quirkos a try? It was designed by qualitative researchers to be the software they wanted to use, and is flexible enough for a whole number of analytical approaches, including discourse analysis. Download a free trial , or read more about it here .
Gee, J., P., 2011. An Introduction to Discourse Analysis . Routledge, London.
Gergen, K. J., 2015, An invitation to Social Construction . Sage, London.
Hodges, B. D., Kuper, A., Reeves, S. 2008. Discourse Analysis. BMJ , a879.
Johnstone, B., 2017. Discourse Analysis . Wiley, London.
Paltridge, B., 2012. Discourse Analysis: An Introduction . Bloomsbury.
Tannen, D., Hamilton, H., Schiffrin, D. 2015. The Handbook of Discourse Analysis . Wiley, Chichester.
Sign up for more like this.
Thank you for visiting nature.com. You are using a browser version with limited support for CSS. To obtain the best experience, we recommend you use a more up to date browser (or turn off compatibility mode in Internet Explorer). In the meantime, to ensure continued support, we are displaying the site without styles and JavaScript.
- View all journals
- My Account Login
- Explore content
- About the journal
- Publish with us
- Sign up for alerts
- Open access
- Published: 20 March 2024
Persistent interaction patterns across social media platforms and over time
- Michele Avalle ORCID: orcid.org/0009-0007-4934-2326 1 na1 ,
- Niccolò Di Marco 1 na1 ,
- Gabriele Etta 1 na1 ,
- Emanuele Sangiorgio ORCID: orcid.org/0009-0003-1024-3735 2 ,
- Shayan Alipour 1 ,
- Anita Bonetti 3 ,
- Lorenzo Alvisi 1 ,
- Antonio Scala 4 ,
- Andrea Baronchelli 5 , 6 ,
- Matteo Cinelli ORCID: orcid.org/0000-0003-3899-4592 1 &
- Walter Quattrociocchi ORCID: orcid.org/0000-0002-4374-9324 1
Nature ( 2024 ) Cite this article
13k Accesses
229 Altmetric
Metrics details
- Mathematics and computing
- Social sciences
Growing concern surrounds the impact of social media platforms on public discourse 1 , 2 , 3 , 4 and their influence on social dynamics 5 , 6 , 7 , 8 , 9 , especially in the context of toxicity 10 , 11 , 12 . Here, to better understand these phenomena, we use a comparative approach to isolate human behavioural patterns across multiple social media platforms. In particular, we analyse conversations in different online communities, focusing on identifying consistent patterns of toxic content. Drawing from an extensive dataset that spans eight platforms over 34 years—from Usenet to contemporary social media—our findings show consistent conversation patterns and user behaviour, irrespective of the platform, topic or time. Notably, although long conversations consistently exhibit higher toxicity, toxic language does not invariably discourage people from participating in a conversation, and toxicity does not necessarily escalate as discussions evolve. Our analysis suggests that debates and contrasting sentiments among users significantly contribute to more intense and hostile discussions. Moreover, the persistence of these patterns across three decades, despite changes in platforms and societal norms, underscores the pivotal role of human behaviour in shaping online discourse.
Similar content being viewed by others
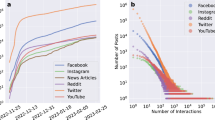
Cross-platform social dynamics: an analysis of ChatGPT and COVID-19 vaccine conversations
Shayan Alipour, Alessandro Galeazzi, … Walter Quattrociocchi
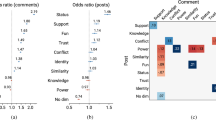
The language of opinion change on social media under the lens of communicative action
Corrado Monti, Luca Maria Aiello, … Francesco Bonchi
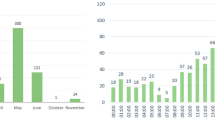
A social media network analysis of trypophobia communication
Xanat Vargas Meza & Shinichi Koyama
The advent and proliferation of social media platforms have not only transformed the landscape of online participation 2 but have also become integral to our daily lives, serving as primary sources for information, entertainment and personal communication 13 , 14 . Although these platforms offer unprecedented connectivity and information exchange opportunities, they also present challenges by entangling their business models with complex social dynamics, raising substantial concerns about their broader impact on society. Previous research has extensively addressed issues such as polarization, misinformation and antisocial behaviours in online spaces 5 , 7 , 12 , 15 , 16 , 17 , revealing the multifaceted nature of social media’s influence on public discourse. However, a considerable challenge in understanding how these platforms might influence inherent human behaviours lies in the general lack of accessible data 18 . Even when researchers obtain data through special agreements with companies like Meta, it may not be enough to clearly distinguish between inherent human behaviours and the effects of the platform’s design 3 , 4 , 8 , 9 . This difficulty arises because the data, deeply embedded in platform interactions, complicate separating intrinsic human behaviour from the influences exerted by the platform’s design and algorithms.
Here we address this challenge by focusing on toxicity, one of the most prominent aspects of concern in online conversations. We use a comparative analysis to uncover consistent patterns across diverse social media platforms and timeframes, aiming to shed light on toxicity dynamics across various digital environments. In particular, our goal is to gain insights into inherently invariant human patterns of online conversations.
The lack of non-verbal cues and physical presence on the web can contribute to increased incivility in online discussions compared with face-to-face interactions 19 . This trend is especially pronounced in online arenas such as newspaper comment sections and political discussions, where exchanges may degenerate into offensive comments or mockery, undermining the potential for productive and democratic debate 20 , 21 . When exposed to such uncivil language, users are more likely to interpret these messages as hostile, influencing their judgement and leading them to form opinions based on their beliefs rather than the information presented and may foster polarized perspectives, especially among groups with differing values 22 . Indeed, there is a natural tendency for online users to seek out and align with information that echoes their pre-existing beliefs, often ignoring contrasting views 6 , 23 . This behaviour may result in the creation of echo chambers, in which like-minded individuals congregate and mutually reinforce shared narratives 5 , 24 , 25 . These echo chambers, along with increased polarization, vary in their prevalence and intensity across different social media platforms 1 , suggesting that the design and algorithms of these platforms, intended to maximize user engagement, can substantially shape online social dynamics. This focus on engagement can inadvertently highlight certain behaviours, making it challenging to differentiate between organic user interaction and the influence of the platform’s design. A substantial portion of current research is devoted to examining harmful language on social media and its wider effects, online and offline 10 , 26 . This examination is crucial, as it reveals how social media may reflect and amplify societal issues, including the deterioration of public discourse. The growing interest in analysing online toxicity through massive data analysis coincides with advancements in machine learning capable of detecting toxic language 27 . Although numerous studies have focused on online toxicity, most concentrate on specific platforms and topics 28 , 29 . Broader, multiplatform studies are still limited in scale and reach 12 , 30 . Research fragmentation complicates understanding whether perceptions about online toxicity are accurate or misconceptions 31 . Key questions include whether online discussions are inherently toxic and how toxic and non-toxic conversations differ. Clarifying these dynamics and how they have evolved over time is crucial for developing effective strategies and policies to mitigate online toxicity.
Our study involves a comparative analysis of online conversations, focusing on three dimensions: time, platform and topic. We examine conversations from eight different platforms, totalling about 500 million comments. For our analysis, we adopt the toxicity definition provided by the Perspective API, a state-of-the-art classifier for the automatic detection of toxic speech. This API considers toxicity as “a rude, disrespectful or unreasonable comment likely to make someone leave a discussion”. We further validate this definition by confirming its consistency with outcomes from other detection tools, ensuring the reliability and comparability of our results. The concept of toxicity in online discourse varies widely in the literature, reflecting its complexity, as seen in various studies 32 , 33 , 34 . The efficacy and constraints of current machine-learning-based automated toxicity detection systems have recently been debated 11 , 35 . Despite these discussions, automated systems are still the most practical means for large-scale analyses.
Here we analyse online conversations, challenging common assumptions about their dynamics. Our findings reveal consistent patterns across various platforms and different times, such as the heavy-tailed nature of engagement dynamics, a decrease in user participation and an increase in toxic speech in lengthier conversations. Our analysis indicates that, although toxicity and user participation in debates are independent variables, the diversity of opinions and sentiments among users may have a substantial role in escalating conversation toxicity.
To obtain a comprehensive picture of online social media conversations, we analysed a dataset of about 500 million comments from Facebook, Gab, Reddit, Telegram, Twitter, Usenet, Voat and YouTube, covering diverse topics and spanning over three decades (a dataset breakdown is shown in Table 1 and Supplementary Table 1 ; for details regarding the data collection, see the ‘Data collection’ section of the Methods ).
Our analysis aims to comprehensively compare the dynamics of diverse social media accounting for human behaviours and how they evolved. In particular, we first characterize conversations at a macroscopic level by means of their engagement and participation, and we then analyse the toxicity of conversations both after and during their unfolding. We conclude the paper by examining potential drivers for the emergence of toxic speech.
Conversations on different platforms
This section provides an overview of online conversations by considering user activity and thread size metrics. We define a conversation (or a thread) as a sequence of comments that follow chronologically from an initial post. In Fig. 1a and Extended Data Fig. 1 , we observe that, across all platforms, both user activity (defined as the number of comments posted by the user) and thread length (defined as the number of comments in a thread) exhibit heavy-tailed distributions. The summary statistics about these distributions are reported in Supplementary Tables 1 and 2 .
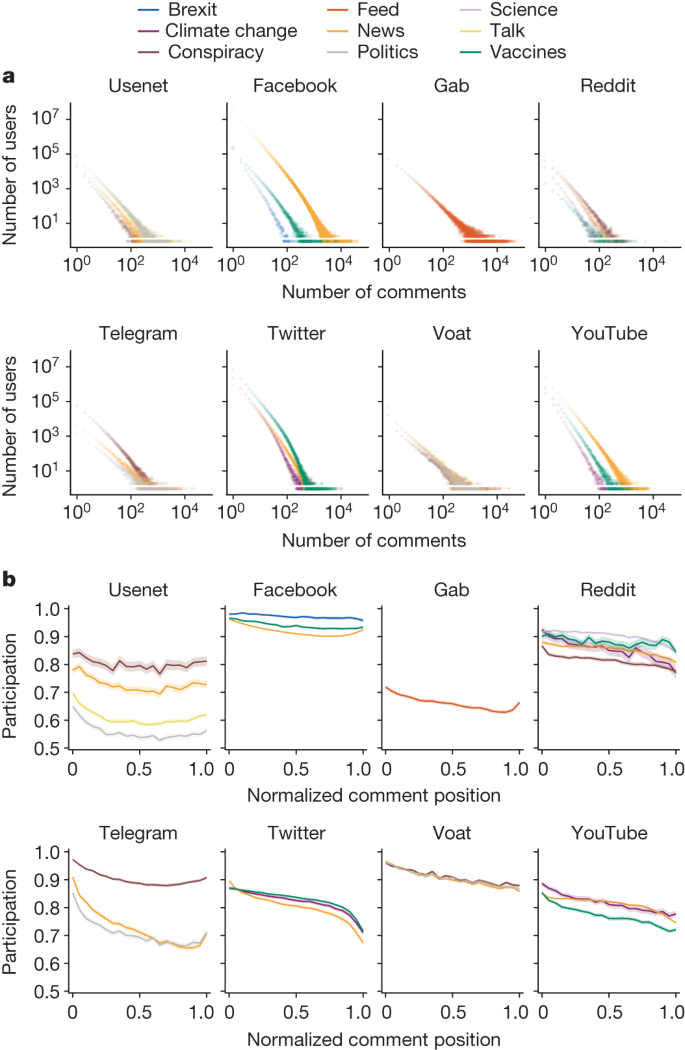
a , The distributions of user activity in terms of comments posted for each platform and each topic. b , The mean user participation as conversations evolve. For each dataset, participation is computed for the threads belonging to the size interval [0.7–1] (Supplementary Table 2 ). Trends are reported with their 95% confidence intervals. The x axis represents the normalized position of comment intervals in the threads.
Consistent with previous studies 36 , 37 our analysis shows that the macroscopic patterns of online conversations, such as the distribution of users/threads activity and lifetime, are consistent across all datasets and topics (Supplementary Tables 1 – 4 ). This observation holds regardless of the specific features of the diverse platforms, such as recommendation algorithms and moderation policies (described in the ‘Content moderation policies’ of the Methods ), as well as other factors, including the user base and the conversation topics. We extend our analysis by examining another aspect of user activity within conversations across all platforms. To do this, we introduce a metric for the participation of users as a thread evolves. In this analysis, threads are filtered to ensure sufficient length as explained in the ‘Logarithmic binning and conversation size’ section of the Methods .
The participation metric, defined over different conversation intervals (that is, 0–5% of the thread arranged in chronological order, 5–10%, and so on), is the ratio of the number of unique users to the number of comments in the interval. Considering a fixed number of comments c , smaller values of participation indicate that fewer unique users are producing c comments in a segment of the conversation. In turn, a value of participation equal to 1 means that each user is producing one of the c comments, therefore obtaining the maximal homogeneity of user participation. Our findings show that, across all datasets, the participation of users in the evolution of conversations, averaged over almost all considered threads, is decreasing, as indicated by the results of Mann–Kendall test—a nonparametric test assessing the presence of a monotonic upward or downward tendency—shown in Extended Data Table 1 . This indicates that fewer users tend to take part in a conversation as it evolves, but those who do are more active (Fig. 1b ). Regarding patterns and values, the trends in user participation for various topics are consistent across each platform. According to the Mann–Kendall test, the only exceptions were Usenet Conspiracy and Talk, for which an ambiguous trend was detected. However, we note that their regression slopes are negative, suggesting a decreasing trend, even if with a weaker effect. Overall, our first set of findings highlights the shared nature of certain online interactions, revealing a decrease in user participation over time but an increase in activity among participants. This insight, consistent across most platforms, underscores the dynamic interplay between conversation length, user engagement and topic-driven participation.
Conversation size and toxicity
To detect the presence of toxic language, we used Google’s Perspective API 34 , a state-of-the-art toxicity classifier that has been used extensively in recent literature 29 , 38 . Perspective API defines a toxic comment as “A rude, disrespectful, or unreasonable comment that is likely to make people leave a discussion”. On the basis of this definition, the classifier assigns a toxicity score in the [0,1] range to a piece of text that can be interpreted as an estimate of the likelihood that a reader would perceive the comment as toxic ( https://developers.perspectiveapi.com/s/about-the-api-score ). To define an appropriate classification threshold, we draw from the existing literature 39 , which uses 0.6 as the threshold for considering a comment as toxic. A robustness check of our results using different threshold and classification tools is reported in the ‘Toxicity detection and validation of employed models’ section of the Methods , together with a discussion regarding potential shortcomings deriving from automatic classifiers. To further investigate the interplay between toxicity and conversation features across various platforms, our study first examines the prevalence of toxic speech in each dataset. We then analyse the occurrence of highly toxic users and conversations. Lastly, we investigate how the length of conversations correlates with the probability of encountering toxic comments. First of all, we define the toxicity of a user as the fraction of toxic comments that she/he left. Similarly, the toxicity of a thread is the fraction of toxic comments it contains. We begin by observing that, although some toxic datasets exist on unmoderated platforms such as Gab, Usenet and Voat, the prevalence of toxic speech is generally low. Indeed, the percentage of toxic comments in each dataset is mostly below 10% (Table 1 ). Moreover, the complementary cumulative distribution functions illustrated in Extended Data Fig. 2 show that the fraction of extremely toxic users is very low for each dataset (in the range between 10 −3 and 10 −4 ), and the majority of active users wrote at least one toxic comment, as reported in Supplementary Table 5 , therefore suggesting that the overall volume of toxicity is not a phenomenon limited to the activity of very few users and localized in few conversations. Indeed, the number of users versus their toxicity decreases sharply following an exponential trend. The toxicity of threads follows a similar pattern. To understand the association between the size and toxicity of a conversation, we start by grouping conversations according to their length to analyse their structural differences 40 . The grouping is implemented by means of logarithmic binning (see the ‘Logarithmic binning and conversation size’ section of the Methods ) and the evolution of the average fraction of toxic comments in threads versus the thread size intervals is reported in Fig. 2 . Notably, the resulting trends are almost all increasing, showing that, independently of the platform and topic, the longer the conversation, the more toxic it tends to be.
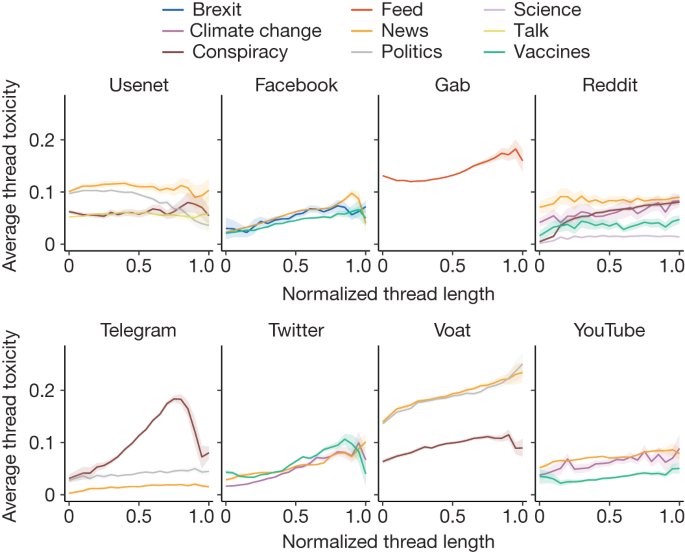
The mean fraction of toxic comments in conversations versus conversation size for each dataset. Trends represent the mean toxicity over each size interval and their 95% confidence interval. Size ranges are normalized to enable visual comparison of the different trends.
We assessed the increase in the trends by both performing linear regression and applying the Mann–Kendall test to ensure the statistical significance of our results (Extended Data Table 2 ). To further validate these outcomes, we shuffled the toxicity labels of comments, finding that trends are almost always non-increasing when data are randomized. Furthermore, the z -scores of the regression slopes indicate that the observed trends deviate from the mean of the distributions resulting from randomizations, being at least 2 s.d. greater in almost all cases. This provides additional evidence of a remarkable difference from randomness. The only decreasing trend is Usenet Politics. Moreover, we verified that our results are not influenced by the specific number of bins as, after estimating the same trends again with different intervals, we found that the qualitative nature of the results remains unchanged. These findings are summarized in Extended Data Table 2 . These analyses have been validated on the same data using a different threshold for identifying toxic comments and on a new dataset labelled with three different classifiers, obtaining similar results (Extended Data Fig. 5 , Extended Data Table 5 , Supplementary Fig. 1 and Supplementary Table 8 ). Finally, using a similar approach, we studied the toxicity content of conversations versus their lifetime—that is, the time elapsed between the first and last comment. In this case, most trends are flat, and there is no indication that toxicity is generally associated either with the duration of a conversation or the lifetime of user interactions (Extended Data Fig. 4 ).
Conversation evolution and toxicity
In the previous sections, we analysed the toxicity level of online conversations after their conclusion. We next focus on how toxicity evolves during a conversation and its effect on the dynamics of the discussion. The common beliefs that (1) online interactions inevitably devolve into toxic exchanges over time and (2) once a conversation reaches a certain toxicity threshold, it would naturally conclude, are not modern notions but they were also prevalent in the early days of the World Wide Web 41 . Assumption 2 aligns with the Perspective API’s definition of toxic language, suggesting that increased toxicity reduces the likelihood of continued participation in a conversation. However, this observation should be reconsidered, as it is not only the peak levels of toxicity that might influence a conversation but, for example, also a consistent rate of toxic content. To test these common assumptions, we used a method similar to that used for measuring participation; we select sufficiently long threads, divide each of them into a fixed number of equal intervals, compute the fraction of toxic comments for each of these intervals, average it over all threads and plot the toxicity trend through the unfolding of the conversations. We find that the average toxicity level remains mostly stable throughout, without showing a distinctive increase around the final part of threads (Fig. 3a (bottom) and Extended Data Fig. 3 ). Note that a similar observation was made previously 41 , but referring only to Reddit. Our findings challenge the assumption that toxicity discourages people from participating in a conversation, even though this notion is part of the definition of toxicity used by the detection tool. This can be seen by checking the relationship between trends in user participation, a quantity related to the number of users in a discussion at some point, and toxicity. The fact that the former typically decreases while the latter remains stable during conversations indicates that toxicity is not associated with participation in conversations (an example is shown in Fig. 3a ; box plots of the slopes of participation and toxicity for the whole dataset are shown in Fig. 3b ). This suggests that, on average, people may leave discussions regardless of the toxicity of the exchanges. We calculated the Pearson’s correlation between user participation and toxicity trends for each dataset to support this hypothesis. As shown in Fig. 3d , the resulting correlation coefficients are very heterogeneous, indicating no consistent pattern across different datasets. To further validate this analysis, we tested the differences in the participation of users commenting on either toxic or non-toxic conversations. To split such conversations into two disjoint sets, we first compute the toxicity distribution T i of long threads in each dataset i , and we then label a conversation j in dataset i as toxic if it has toxicity t ij ≥ µ ( T i ) + σ ( T i ), with µ ( T i ) being mean and σ ( T i ) the standard deviation of T i ; all of the other conversations are considered to be non-toxic. After splitting the threads, for each dataset, we compute the Pearson’s correlation of user participation between sets to find strongly positive values of the coefficient in all cases (Fig. 3c,e ). This result is also confirmed by a different analysis of which the results are reported in Supplementary Table 8 , in which no significant difference between slopes in toxic and non-toxic threads can be found. Thus, user behaviour in toxic and non-toxic conversations shows almost identical patterns in terms of participation. This reinforces our finding that toxicity, on average, does not appear to affect the likelihood of people participating in a conversation. These analyses were repeated with a lower toxicity classification threshold (Extended Data Fig. 5 ) and on additional datasets (Supplementary Fig. 2 and Supplementary Table 11 ), finding consistent results.
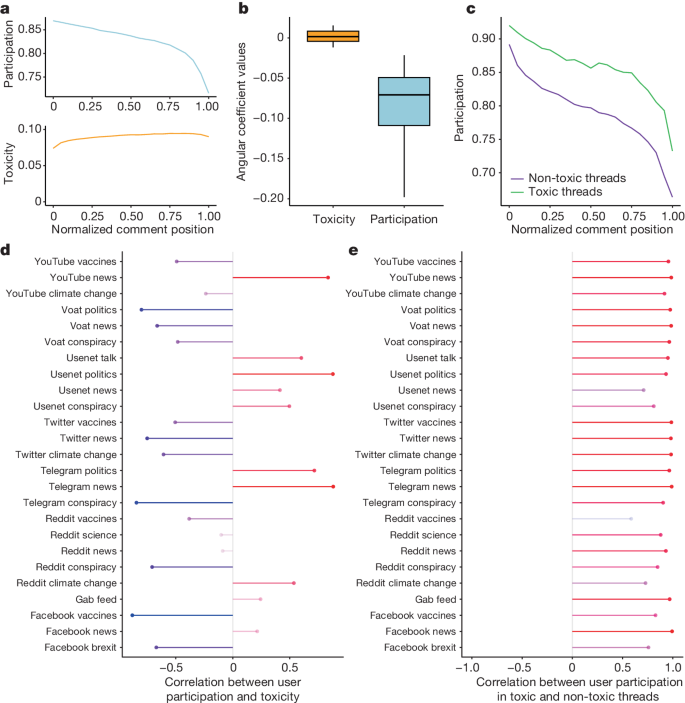
a , Examples of a typical trend in averaged user participation (top) and toxicity (bottom) versus the normalized position of comment intervals in the threads (Twitter news dataset). b , Box plot distributions of toxicity ( n = 25, minimum = −0.012, maximum = 0.015, lower whisker = −0.012, quartile 1 (Q1) = − 0.004, Q2 = 0.002, Q3 = 0.008, upper whisker = 0.015) and participation ( n = 25, minimum = −0.198, maximum = −0.022, lower whisker = −0.198, Q1 = − 0.109, Q2 = − 0.071, Q3 = − 0.049, upper whisker = −0.022) trend slopes for all datasets, as resulting from linear regression. c , An example of user participation in toxic and non-toxic thread sets (Twitter news dataset). d , Pearson’s correlation coefficients between user participation and toxicity trends for each dataset. e , Pearson’s correlation coefficients between user participation in toxic and non-toxic threads for each dataset.
Controversy and toxicity
In this section, we aim to explore why people participate in toxic online conversations and why longer discussions tend to be more toxic. Several factors could be the subject matter. First, controversial topics might lead to longer, more heated debates with increased toxicity. Second, the endorsement of toxic content by other users may act as an incentive to increase the discussion’s toxicity. Third, engagement peaks, due to factors such as reduced discussion focus or the intervention of trolls, may bring a higher share of toxic exchanges. Pursuing this line of inquiry, we identified proxies to measure the level of controversy in conversations and examined how these relate to toxicity and conversation size. Concurrently, we investigated the relationship between toxicity, endorsement and engagement.
As shown previously 24 , 42 , controversy is likely to emerge when people with opposing views engage in the same debate. Thus, the presence of users with diverse political leanings within a conversation could be a valid proxy for measuring controversy. We operationalize this definition as follows. Exploiting the peculiarities of our data, we can infer the political leaning of a subset of users in the Facebook News, Twitter News, Twitter Vaccines and Gab Feed datasets. This is achieved by examining the endorsement, for example, in the form of likes, expressed towards news outlets of which the political inclinations have been independently assessed by news rating agencies (see the ‘Polarization and user leaning attribution’ section of the Methods ). Extended Data Table 3 shows a breakdown of the datasets. As a result, we label users with a leaning score l ∈ [−1, 1], −1 being left leaning and +1 being right leaning. We then select threads with at least ten different labelled users, in which at least 10% of comments (with a minimum of 20) are produced by such users and assign to each of these comments the same leaning score of those who posted them. In this setting, the level of controversy within a conversation is assumed to be captured by the spread of the political leaning of the participants in the conversation. A natural way for measuring such a spread is the s.d. σ ( l ) of the distribution of comments possessing a leaning score: the higher the σ ( l ), the greater the level of ideological disagreement and therefore controversy in a thread. We analysed the relationship between controversy and toxicity in online conversations of different sizes. Figure 4a shows that controversy increases with the size of conversations in all datasets, and its trends are positively correlated with the corresponding trends in toxicity (Extended Data Table 3 ). This supports our hypothesis that controversy and toxicity are closely related in online discussions.
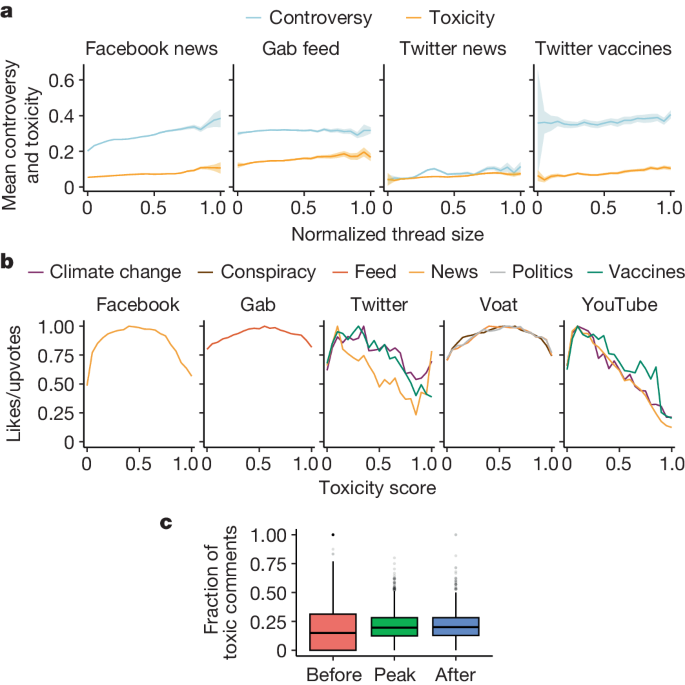
a , The mean controversy ( σ ( l )) and mean toxicity versus thread size (log-binned and normalized) for the Facebook news, Twitter news, Twitter vaccines and Gab feed datasets. Here toxicity is calculated in the same conversations in which controversy could be computed (Extended Data Table 3 ); the relative Pearson’s, Spearman’s and Kendall’s correlation coefficients are also provided in Extended Data Table 3 . Trends are reported with their 95% confidence interval. b , Likes/upvotes versus toxicity (linearly binned). c , An example (Voat politics dataset) of the distributions of the frequency of toxic comments in threads before ( n = 2,201, minimum = 0, maximum = 1, lower whisker = 0, Q1 = 0, Q2 = 0.15, Q3 = 0.313, upper whisker = 0.769) at the peak ( n = 2,798, minimum = 0, maximum = 0.8, lower whisker = 0, Q1 = 0.125, Q2 = 0.196, Q3 = 0.282, upper whisker = 0.513) and after the peak ( n = 2,791, minimum = 0, maximum = 1, lower whisker = 0, Q1 = 0.129, Q2 = 0.200, Q3 = 0.282, upper whisker = 0.500) of activity, as detected by Kleinberg’s burst detection algorithm.
As a complementary analysis, we draw on previous results 43 . In that study, using a definition of controversy operationally different but conceptually related to ours, a link was found between a greater degree of controversy of a discussion topic and a wider distribution of sentiment scores attributed to the set of its posts and comments. We quantified the sentiment of comments using a pretrained BERT model available from Hugging Face 44 , used also in previous studies 45 . The model predicts the sentiment of a sentence through a scoring system ranging from 1 (negative) to 5 (positive). We define the sentiment attributed to a comment c as its weighted mean \(s(c)=\sum _{i=1.5}{x}_{i}{p}_{i}\) , where x i ∈ [1, 5] is the output score from the model and p i is the probability associated to that value. Moreover, we normalize the sentiment score s for each dataset between 0 and 1. We observe the trends of the mean s.d. of sentiment in conversations, \(\bar{\sigma }(s)\) , and toxicity are positively correlated for moderated platforms such as Facebook and Twitter but are negatively correlated on Gab (Extended Data Table 3 ). The positive correlation observed in Facebook and Twitter indicates that greater discrepancies in sentiment of the conversations can, in general, be linked to toxic conversations and vice versa. Instead, on unregulated platforms such as Gab, highly conflicting sentiments seem to be more likely to emerge in less toxic conversations.
As anticipated, another factor that may be associated with the emergence of toxic comments is the endorsement they receive. Indeed, such positive reactions may motivate posting even more comments of the same kind. Using the mean number of likes/upvotes as a proxy of endorsement, we have an indication that this may not be the case. Figure 4b shows that the trend in likes/upvotes versus comments toxicity is never increasing past the toxicity score threshold (0.6).
Finally, to complement our analysis, we inspect the relationship between toxicity and user engagement within conversations, measured as the intensity of the number of comments over time. To do so, we used a method for burst detection 46 that, after reconstructing the density profile of a temporal stream of elements, separates the stream into different levels of intensity and assigns each element to the level to which it belongs (see the ‘Burst analysis’ section of the Methods ). We computed the fraction of toxic comments at the highest intensity level of each conversation and for the levels right before and after it. By comparing the distributions of the fraction of toxic comments for the three intervals, we find that these distributions are statistically different in almost all cases (Fig. 4c and Extended Data Table 4 ). In all datasets but one, distributions are consistently shifted towards higher toxicity at the peak of engagement, compared with the previous phase. Likewise, in most cases, the peak shows higher toxicity even if compared to the following phase, which in turn is mainly more toxic than the phase before the peak. These results suggest that toxicity is likely to increase together with user engagement.
Here we examine one of the most prominent and persistent characteristics online discussions—toxic behaviour, defined here as rude, disrespectful or unreasonable conduct. Our analysis suggests that toxicity is neither a deterrent to user involvement nor an engagement amplifier; rather, it tends to emerge when exchanges become more frequent and may be a product of opinion polarization. Our findings suggest that the polarization of user opinions—intended as the degree of opposed partisanship of users in a conversation—may have a more crucial role than toxicity in shaping the evolution of online discussions. Thus, monitoring polarization could indicate early interventions in online discussions. However, it is important to acknowledge that the dynamics at play in shaping online discourse are probably multifaceted and require a nuanced approach for effective moderation. Other factors may influence toxicity and engagement, such as the specific subject of the conversation, the presence of influential users or ‘trolls’, the time and day of posting, as well as cultural or demographic aspects, such as user average age or geographical location. Furthermore, even though extremely toxic users are rare (Extended Data Fig. 2 ), the relationship between participation and toxicity of a discussion may in principle be affected also by small groups of highly toxic and engaged users driving the conversation dynamics. Although the analysis of such subtler aspects is beyond the scope of this Article, they are certainly worth investigating in future research.
However, when people encounter views that contradict their own, they may react with hostility and contempt, consistent with previous research 47 . In turn, it may create a cycle of negative emotions and behaviours that fuels toxicity. We also show that some online conversation features have remained consistent over the past three decades despite the evolution of platforms and social norms.
Our study has some limitations that we acknowledge and discuss. First, we use political leaning as a proxy for general leaning, which may capture only some of the nuances of online opinions. However, political leaning represents a broad spectrum of opinions across different topics, and it correlates well with other dimensions of leaning, such as news preferences, vaccine attitudes and stance on climate change 48 , 49 . We could not assign a political leaning to users to analyse controversies on all platforms. Still, those considered—Facebook, Gab and Twitter—represent different populations and moderation policies, and the combined data account for nearly 90% of the content in our entire dataset. Our analysis approach is based on breadth and heterogeneity. As such, it may raise concerns about potential reductionism due to the comparison of different datasets from different sources and time periods. We acknowledge that each discussion thread, platform and context has unique characteristics and complexities that might be diminished when homogenizing data. However, we aim not to capture the full depth of every discussion but to identify and highlight general patterns and trends in online toxicity across platforms and time. The quantitative approach used in our study is similar to numerous other studies 15 and enables us to uncover these overarching principles and patterns that may otherwise remain hidden. Of course, it is not possible to account for the behaviours of passive users. This entails, for example, that even if toxicity does not seem to make people leave conversations, it could still be a factor that discourages them from joining them. Our study leverages an extensive dataset to examine the intricate relationship between persistent online human behaviours and the characteristics of different social media platforms. Our findings challenge the prevailing assumption by demonstrating that toxic content, as traditionally defined, does not necessarily reduce user engagement, thereby questioning the assumed direct correlation between toxic content and negative discourse dynamics. This highlights the necessity for a detailed examination of the effect of toxic interactions on user behaviour and the quality of discussions across various platforms. Our results, showing user resilience to toxic content, indicate the potential for creating advanced, context-aware moderation tools that can accurately navigate the complex influence of antagonistic interactions on community engagement and discussion quality. Moreover, our study sets the stage for further exploration into the complexities of toxicity and its effect on engagement within online communities. Advancing our grasp of online discourse necessitates refining content moderation techniques grounded in a thorough understanding of human behaviour. Thus, our research adds to the dialogue on creating more constructive online spaces, promoting moderation approaches that are effective yet nuanced, facilitating engaging exchanges and reducing the tangible negative effects of toxic behaviour.
Through the extensive dataset presented here, critical aspects of the online platform ecosystem and fundamental dynamics of user interactions can be explored. Moreover, we provide insights that a comparative approach such as the one followed here can prove invaluable in discerning human behaviour from platform-specific features. This may be used to investigate further sensitive issues, such as the formation of polarization and misinformation. The resulting outcomes have multiple potential impacts. Our findings reveal consistent toxicity patterns across platforms, topics and time, suggesting that future research in this field should prioritize the concept of invariance. Recognizing that toxic behaviour is a widespread phenomenon that is not limited by platform-specific features underscores the need for a broader, unified approach to understanding online discourse. Furthermore, the participation of users in toxic conversations suggests that a simple approach to removing toxic comments may not be sufficient to prevent user exposure to such phenomena. This indicates a need for more sophisticated moderation techniques to manage conversation dynamics, including early interventions in discussions that show warnings of becoming toxic. Furthermore, our findings support the idea that examining content pieces in connection with others could enhance the effectiveness of automatic toxicity detection models. The observed homogeneity suggests that models trained using data from one platform may also have applicability to other platforms. Future research could explore further into the role of controversy and its interaction with other elements contributing to toxicity. Moreover, comparing platforms could enhance our understanding of invariant human factors related to polarization, disinformation and content consumption. Such studies would be instrumental in capturing the drivers of the effect of social media platforms on human behaviour, offering valuable insights into the underlying dynamics of online interactions.
Data collection
In our study, data collection from various social media platforms was strategically designed to encompass various topics, ensuring maximal heterogeneity in the discussion themes. For each platform, where feasible, we focus on gathering posts related to diverse areas such as politics, news, environment and vaccinations. This approach aims to capture a broad spectrum of discourse, providing a comprehensive view of conversation dynamics across different content categories.
We use datasets from previous studies that covered discussions about vaccines 50 , news 51 and brexit 52 . For the vaccines topic, the resulting dataset contains around 2 million comments retrieved from public groups and pages in a period that ranges from 2 January 2010 to 17 July 2017. For the news topic, we selected a list of pages from the Europe Media Monitor that reported the news in English. As a result, the obtained dataset contains around 362 million comments between 9 September 2009 and 18 August 2016. Furthermore, we collect a total of about 4.5 billion likes that the users put on posts and comments concerning these pages. Finally, for the brexit topic, the dataset contains around 460,000 comments from 31 December 2015 to 29 July 2016.
We collect data from the Pushshift.io archive ( https://files.pushshift.io/gab/ ) concerning discussions taking place from 10 August 2016, when the platform was launched, to 29 October 2018, when Gab went temporarily offline due to the Pittsburgh shooting 53 . As a result, we collect a total of around 14 million comments.
Data were collected from the Pushshift.io archive ( https://pushshift.io/ ) for the period ranging from 1 January 2018 to 31 December 2022. For each topic, whenever possible, we manually identified and selected subreddits that best represented the targeted topics. As a result of this operation, we obtained about 800,000 comments from the r/conspiracy subreddit for the conspiracy topic. For the vaccines topic, we collected about 70,000 comments from the r/VaccineDebate subreddit, focusing on the COVID-19 vaccine debate. We collected around 400,000 comments from the r/News subreddit for the news topic. We collected about 70,000 comments from the r/environment subreddit for the climate change topic. Finally, we collected around 550,000 comments from the r/science subreddit for the science topic.
We created a list of 14 channels, associating each with one of the topics considered in the study. For each channel, we manually collected messages and their related comments. As a result, from the four channels associated with the news topic (news notiziae, news ultimora, news edizionestraordinaria, news covidultimora), we obtained around 724,000 comments from posts between 9 April 2018 and 20 December 2022. For the politics topic, instead, the corresponding two channels (politics besttimeline, politics polmemes) produced a total of around 490,000 comments between 4 August 2017 and 19 December 2022. Finally, the eight channels assigned to the conspiracy topic (conspiracy bennyjhonson, conspiracy tommyrobinsonnews, conspiracy britainsfirst, conspiracy loomeredofficial, conspiracy thetrumpistgroup, conspiracy trumpjr, conspiracy pauljwatson, conspiracy iononmivaccino) produced a total of about 1.4 million comments between 30 August 2019 and 20 December 2022.
We used a list of datasets from previous studies that includes discussions about vaccines 54 , climate change 49 and news 55 topics. For the vaccines topic, we collected around 50 million comments from 23 January 2010 to 25 January 2023. For the news topic, we extend the dataset used previously 55 by collecting all threads composed of less than 20 comments, obtaining a total of about 9.5 million comments for a period ranging from 1 January 2020 to 29 November 2022. Finally, for the climate change topic, we collected around 9.7 million comments between 1 January 2020 and 10 January 2023.
We collected data for the Usenet discussion system by querying the Usenet Archive ( https://archive.org/details/usenet?tab=about ). We selected a list of topics considered adequate to contain a large, broad and heterogeneous number of discussions involving active and populated newsgroups. As a result of this selection, we selected conspiracy, politics, news and talk as topic candidates for our analysis. For the conspiracy topic, we collected around 280,000 comments between 1 September 1994 and 30 December 2005 from the alt.conspiracy newsgroup. For the politics topics, we collected around 2.6 million comments between 29 June 1992 and 31 December 2005 from the alt.politics newsgroup. For the news topic, we collected about 620,000 comments between 5 December 1992 and 31 December 2005 from the alt.news newsgroup. Finally, for the talk topic, we collected all of the conversations from the homonym newsgroup on a period that ranges from 13 February 1989 to 31 December 2005 for around 2.1 million contents.
We used a dataset presented previously 56 that covers the entire lifetime of the platform, from 9 January 2018 to 25 December 2020, including a total of around 16.2 million posts and comments shared by around 113,000 users in about 7,100 subverses (the equivalent of a subreddit for Voat). Similarly to previous platforms, we associated the topics to specific subverses. As a result of this operation, for the conspiracy topic, we collected about 1 million comments from the greatawakening subverse between 9 January 2018 and 25 December 2020. For the politics topic, we collected around 1 million comments from the politics subverse between 16 June 2014 and 25 December 2020. Finally, for the news topic, we collected about 1.4 million comments from the news subverse between 21 November 2013 and 25 December 2020.
We used a dataset proposed in previous studies that collected conversations about the climate change topic 49 , which is extended, coherently with previous platforms, by including conversations about vaccines and news topics. The data collection process for YouTube is performed using the YouTube Data API ( https://developers.google.com/youtube/v3 ). For the climate change topic, we collected around 840,000 comments between 16 March 2014 and 28 February 2022. For the vaccines topic, we collected conversations between 31 January 2020 and 24 October 2021 containing keywords about COVID-19 vaccines, namely Sinopharm, CanSino, Janssen, Johnson&Johnson, Novavax, CureVac, Pfizer, BioNTech, AstraZeneca and Moderna. As a result of this operation, we gathered a total of around 2.6 million comments to videos. Finally, for the news topic, we collected about 20 million comments between 13 February 2006 and 8 February 2022, including videos and comments from a list of news outlets, limited to the UK and provided by Newsguard (see the ‘Polarization and user leaning attribution’ section).
Content moderation policies
Content moderation policies are guidelines that online platforms use to monitor the content that users post on their sites. Platforms have different goals and audiences, and their moderation policies may vary greatly, with some placing more emphasis on free expression and others prioritizing safety and community guidelines.
Facebook and YouTube have strict moderation policies prohibiting hate speech, violence and harassment 57 . To address harmful content, Facebook follows a ‘remove, reduce, inform’ strategy and uses a combination of human reviewers and artificial intelligence to enforce its policies 58 . Similarly, YouTube has a similar set of community guidelines regarding hate speech policy, covering a wide range of behaviours such as vulgar language 59 , harassment 60 and, in general, does not allow the presence of hate speech and violence against individuals or groups based on various attributes 61 . To ensure that these guidelines are respected, the platform uses a mix of artificial intelligence algorithms and human reviewers 62 .
Twitter also has a comprehensive content moderation policy and specific rules against hateful conduct 63 , 64 . They use automation 65 and human review in the moderation process 66 . At the date of submission, Twitter’s content policies have remained unchanged since Elon Musk’s takeover, except that they ceased enforcing their COVID-19 misleading information policy on 23 November 2022. Their policy enforcement has faced criticism for inconsistency 67 .
Reddit falls somewhere in between regarding how strict its moderation policy is. Reddit’s content policy has eight rules, including prohibiting violence, harassment and promoting hate based on identity or vulnerability 68 , 69 . Reddit relies heavily on user reports and volunteer moderators. Thus, it could be considered more lenient than Facebook, YouTube and Twitter regarding enforcing rules. In October 2022, Reddit announced that they intend to update their enforcement practices to apply automation in content moderation 70 .
By contrast, Telegram, Gab and Voat take a more hands-off approach with fewer restrictions on content. Telegram has ambiguity in its guidelines, which arises from broad or subjective terms and can lead to different interpretations 71 . Although they mentioned they may use automated algorithms to analyse messages, Telegram relies mainly on users to report a range of content, such as violence, child abuse, spam, illegal drugs, personal details and pornography 72 . According to Telegram’s privacy policy, reported content may be checked by moderators and, if it is confirmed to violate their terms, temporary or permanent restrictions may be imposed on the account 73 . Gab’s Terms of Service allow all speech protected under the First Amendment to the US Constitution, and unlawful content is removed. They state that they do not review material before it is posted on their website and cannot guarantee prompt removal of illegal content after it has been posted 74 . Voat was once known as a ‘free-speech’ alternative to Reddit and allowed content even if it may be considered offensive or controversial 56 .
Usenet is a decentralized online discussion system created in 1979. Owing to its decentralized nature, Usenet has been difficult to moderate effectively, and it has a reputation for being a place where controversial and even illegal content can be posted without consequence. Each individual group on Usenet can have its own moderators, who are responsible for monitoring and enforcing their group’s rules, and there is no single set of rules that applies to the entire platform 75 .
Logarithmic binning and conversation size
Owing to the heavy-tailed distributions of conversation length (Extended Data Fig. 1 ), to plot the figures and perform the analyses, we used logarithmic binning. Thus, according to its length, each thread of each dataset is assigned to 1 out of 21 bins. To ensure a minimal number of points in each bin, we iteratively change the left bound of the last bin so that it contains at least N = 50 elements (we set N = 100 in the case of Facebook news, due to its larger size). Specifically, considering threads ordered in increasing length, the size of the largest thread is changed to that of the second last largest one, and the binning is recalculated accordingly until the last bin contains at least N points.
For visualization purposes, we provide a normalization of the logarithmic binning outcome that consists of mapping discrete points into coordinates of the x axis such that the bins correspond to {0, 0.05, 0.1, ..., 0.95, 1}.
To perform the part of the analysis, we select conversations belonging to the [0.7, 1] interval of the normalized logarithmic binning of thread length. This interval ensures that the conversations are sufficiently long and that we have a substantial number of threads. Participation and toxicity trends are obtained by applying to such conversations a linear binning of 21 elements to a chronologically ordered sequence of comments, that is, threads. A breakdown of the resulting datasets is provided in Supplementary Table 2 .
Finally, to assess the equality of the growth rates of participation values in toxic and non-toxic threads (see the ‘Conversation evolution and toxicity’ section), we implemented the following linear regression model:
where the term β 2 accounts for the effect that being a toxic conversation has on the growth of participation. Our results show that β 2 is not significantly different from 0 in most original and validation datasets (Supplementary Tables 8 and 11 )
Toxicity detection and validation of the models used
The problem of detecting toxicity is highly debated, to the point that there is currently no agreement on the very definition of toxic speech 64 , 76 . A toxic comment can be regarded as one that includes obscene or derogatory language 32 , that uses harsh, abusive language and personal attacks 33 , or contains extremism, violence and harassment 11 , just to give a few examples. Even though toxic speech should, in principle, be distinguished from hate speech, which is commonly more related to targeted attacks that denigrate a person or a group on the basis of attributes such as race, religion, gender, sex, sexual orientation and so on 77 , it sometimes may also be used as an umbrella term 78 , 79 . This lack of agreement directly reflects the challenging and inherent subjective nature of the concept of toxicity. The complexity of the topic makes it particularly difficult to assess the reliability of natural language processing models for automatic toxicity detection despite the impressive improvements in the field. Modern natural language processing models, such as Perspective API, are deep learning models that leverage word-embedding techniques to build representations of words as vectors in a high-dimensional space, in which a metric distance should reflect the conceptual distance among words, therefore providing linguistic context. A primary concern regarding toxicity detection models is their limited ability to contextualize conversations 11 , 80 . These models often struggle to incorporate factors beyond the text itself, such as the participant’s personal characteristics, motivations, relationships, group memberships and the overall tone of the discussion 11 . Consequently, what is considered to be toxic content can vary significantly among different groups, such as ethnicities or age groups 81 , leading to potential biases. These biases may stem from the annotators’ backgrounds and the datasets used for training, which might not adequately represent cultural heterogeneity. Moreover, subtle forms of toxic content, like indirect allusions, memes and inside jokes targeted at specific groups, can be particularly challenging to detect. Word embeddings equip current classifiers with a rich linguistic context, enhancing their ability to recognize a wide range of patterns characteristic of toxic expression. However, the requirements for understanding the broader context of a conversation, such as personal characteristics, motivations and group dynamics, remain beyond the scope of automatic detection models. We acknowledge these inherent limitations in our approach. Nonetheless, reliance on automatic detection models is essential for large-scale analyses of online toxicity like the one conducted in this study. We specifically resort to the Perspective API for this task, as it represents state-of-the-art automatic toxicity detection, offering a balance between linguistic nuance and scalable analysis capabilities. To define an appropriate classification threshold, we draw from the existing literature 64 , which uses 0.6 as the threshold for considering a comment to be toxic. This threshold can also be considered a reasonable one as, according to the developer guidelines offered by Perspective, it would indicate that the majority of the sample of readers, namely 6 out of 10, would perceive that comment as toxic. Due to the limitations mentioned above (for a criticism of Perspective API, see ref. 82 ), we validate our results by performing a comparative analysis using two other toxicity detectors: Detoxify ( https://github.com/unitaryai/detoxify ), which is similar to Perspective, and IMSYPP, a classifier developed for a European Project on hate speech 16 ( https://huggingface.co/IMSyPP ). In Supplementary Table 14 , the percentages of agreement among the three models in classifying 100,000 comments taken randomly from each of our datasets are reported. For Detoxify we used the same binary toxicity threshold (0.6) as used with Perspective. Although IMSYPP operates on a distinct definition of toxicity as outlined previously 16 , our comparative analysis shows a general agreement in the results. This alignment, despite the differences in underlying definitions and methodologies, underscores the robustness of our findings across various toxicity detection frameworks. Moreover, we perform the core analyses of this study using all classifiers on a further, vast and heterogeneous dataset. As shown in Supplementary Figs. 1 and 2 , the results regarding toxicity increase with conversation size and user participation and toxicity are quantitatively very similar. Furthermore, we verify the stability of our findings under different toxicity thresholds. Although the main analyses in this paper use the threshold value recommended by the Perspective API, set at 0.6, to minimize false positives, our results remain consistent even when applying a less conservative threshold of 0.5. This is demonstrated in Extended Data Fig. 5 , confirming the robustness of our observations across varying toxicity levels. For this study, we used the API support for languages prevalent in the European and American continents, including English, Spanish, French, Portuguese, German, Italian, Dutch, Polish, Swedish and Russian. Detoxify also offers multilingual support. However, IMSYPP is limited to English and Italian text, a factor considered in our comparative analysis.
Polarization and user leaning attribution
Our approach to measuring controversy in a conversation is based on estimating the degree of political partisanship among the participants. This measure is closely related to the political science concept of political polarization. Political polarization is the process by which political attitudes diverge from moderate positions and gravitate towards ideological extremes, as described previously 83 . By quantifying the level of partisanship within discussions, we aim to provide insights into the extent and nature of polarization in online debates. In this context, it is important to distinguish between ‘ideological polarization’ and ‘affective polarization’. Ideological polarization refers to divisions based on political viewpoints. By contrast, affective polarization is characterized by positive emotions towards members of one’s group and hostility towards those of opposing groups 84 , 85 . Here we focus specifically on ideological polarization. The subsequent description of our procedure for attributing user political leanings will further clarify this focus. On online social media, the individual leaning of a user toward a topic can be inferred through the content produced or the endorsement shown toward specific content. In this study, we consider the endorsement of users to news outlets of which the political leaning has been evaluated by trustworthy external sources. Although not without limitations—which we address below—this is a standard approach that has been used in several studies, and has become a common and established practice in the field of social media analysis due to its practicality and effectiveness in providing a broad understanding of political dynamics on these online platforms 1 , 43 , 86 , 87 , 88 . We label news outlets with a political score based on the information reported by Media Bias/Fact Check (MBFC) ( https://mediabiasfactcheck.com ), integrating with the equivalent information from Newsguard ( https://www.newsguardtech.com/ ). MBFC is an independent fact-checking organization that rates news outlets on the basis of the reliability and the political bias of the content that they produce and share. Similarly, Newsguard is a tool created by an international team of journalists that provides news outlet trust and political bias scores. Following standard methods used in the literature 1 , 43 , we calculated the individual leaning of a user l ∈ [−1, 1] as the average of the leaning scores l c ∈ [−1, 1] attributed to each of the content it produced/shared, where l c results from a mapping of the news organizations political scores provided by MBFC and Newsguard, respectively: [left, centre-left, centre, centre-right, right] to [−1, − 0.5, 0, 0.5, 1], and [far left, left, right, far right] to [−1, −0.5, 0.5, 1]). Our datasets have different structures, so we have to evaluate user leanings in different ways. For Facebook News, we assign a leaning score to users who posted a like at least three times and commented at least three times under news outlet pages that have a political score. For Twitter News, a leaning is assigned to users who posted at least 15 comments under scored news outlet pages. For Twitter Vaccines and Gab, we consider users who shared content produced by scored news outlet pages at least three times. A limitation of our approach is that engaging with politically aligned content does not always imply agreement; users may interact with opposing viewpoints for critical discussion. However, research indicates that users predominantly share content aligning with their own views, especially in politically charged contexts 87 , 89 , 90 . Moreover, our method captures users who actively express their political leanings, omitting the ‘passive’ ones. This is due to the lack of available data on users who do not explicitly state their opinions. Nevertheless, analysing active users offers valuable insights into the discourse of those most engaged and influential on social media platforms.
Burst analysis
We used the Kleinberg burst detection algorithm 46 (see the ‘Controversy and toxicity’ section) to all conversations with at least 50 comments in a dataset. In our analysis, we randomly sample up to 5,000 conversations, each containing a specific number of comments. To ensure the reliability of our data, we exclude conversations with an excessive number of double timestamps—defined as more than 10 consecutive or over 100 within the first 24 h. This criterion helps to mitigate the influence of bots, which could distort the patterns of human activity. Furthermore, we focus on the first 24 h of each thread to analyse streams of comments during their peak activity period. Consequently, Usenet was excluded from our study. The unique usage characteristics of Usenet render such a time-constrained analysis inappropriate, as its activity patterns do not align with those of the other platforms under consideration. By reconstructing the density profile of the comment stream, the algorithm divides the entire stream’s interval into subintervals on the basis of their level of intensity. Labelled as discrete positive values, higher levels of burstiness represent higher activity segments. To avoid considering flat-density phases, threads with a maximum burst level equal to 2 are excluded from this analysis. To assess whether a higher intensity of comments results in a higher comment toxicity, we perform a Mann–Whitney U -test 91 with Bonferroni correction for multiple testing between the distributions of the fraction of toxic comments t i in three intensity phases: during the peak of engagement and at the highest levels before and after. Extended Data Table 4 shows the corrected P values of each test, at a 0.99 confidence level, with H1 indicated in the column header. An example of the distribution of the frequency of toxic comments in threads at the three phases of a conversation considered (pre-peak, peak and post-peak) is reported in Fig. 4c .
Toxicity detection on Usenet
As discussed in the section on toxicity detection and the Perspective API above, automatic detectors derive their understanding of toxicity from the annotated datasets that they are trained on. The Perspective API is predominantly trained on recent texts, and its human labellers conform to contemporary cultural norms. Thus, although our dataset dates back to no more than the early 1990s, we provide a discussion on the viability of the application of Perspective API to Usenet and validation analysis. Contemporary society, especially in Western contexts, is more sensitive to issues of toxicity, including gender, race and sexual orientation, compared with a few decades ago. This means that some comments identified as toxic today, including those from older platforms like Usenet, might not have been considered as such in the past. However, this discrepancy does not significantly affect our analysis, which is centred on current standards of toxicity. On the other hand, changes in linguistic features may have some repercussions: there may be words and locutions that were frequently used in the 1990s that instead appear sparsely in today’s language, making Perspective potentially less effective in classifying short texts that contain them. We therefore proceeded to evaluate the impact that such a possible scenario could have on our results. In light of the above considerations, we consider texts labelled as toxic as correctly classified; instead, we assume that there is a fixed probability p that a comment may be incorrectly labelled as non-toxic. Consequently, we randomly designate a proportion p of non-toxic comments, relabel them as toxic and compute the toxicity versus conversation size trend (Fig. 2 ) on the altered dataset across various p . Specifically, for each value, we simulate 500 different trends, collecting their regression slopes to obtain a null distribution for them. To assess if the probability of error could lead to significant differences in the observed trend, we compute the fraction f of slopes lying outside the interval (−| s |,| s |), where s is the slope of the observed trend. We report the result in Supplementary Table 9 for different values of p . In agreement with our previous analysis, we assume that the slope differs significantly from the ones obtained from randomized data if f is less than 0.05.
We observed that only the Usenet Talk dataset shows sensitivity to small error probabilities, and the others do not show a significant difference. Consequently, our results indicate that Perspective API is suitable for application to Usenet data in our analyses, notwithstanding the potential linguistic and cultural shifts that might affect the classifier’s reliability with older texts.
Toxicity of short conversations
Our study focuses on the relationship between user participation and the toxicity of conversations, particularly in engaged or prolonged discussions. A potential concern is that concentrating on longer threads overlooks conversations that terminate quickly due to early toxicity, therefore potentially biasing our analysis. To address this, we analysed shorter conversations, comprising 6 to 20 comments, in each dataset. In particular, we computed the distributions of toxicity scores of the first and last three comments in each thread. This approach helps to ensure that our analysis accounts for a range of conversation lengths and patterns of toxicity development, providing a more comprehensive understanding of the dynamics at play. As shown in Supplementary Fig. 3 , for each dataset, the distributions of the toxicity scores display high similarity, meaning that, in short conversations, the last comments are not significantly more toxic than the initial ones, indicating that the potential effects mentioned above do not undermine our conclusions. Regarding our analysis of longer threads, we notice here that the participation quantity can give rise to similar trends in various cases. For example, high participation can be achieved because many users take part in the conversation, but also with small groups of users in which everyone is equally contributing over time. Or, in very large discussions, the contributions of individual outliers may remain hidden. By measuring participation, these and other borderline cases may not be distinct from the statistically highly likely discussion dynamics but, ultimately, this lack of discriminatory power does not have any implications on our findings nor on the validity of the conclusions that we draw.
Reporting summary
Further information on research design is available in the Nature Portfolio Reporting Summary linked to this article.
Data availability
Facebook, Twitter and YouTube data are made available in accordance with their respective terms of use. IDs of comments used in this work are provided at Open Science Framework ( https://doi.org/10.17605/osf.io/fq5dy ). For the remaining platforms (Gab, Reddit, Telegram, Usenet and Voat), all of the necessary information to recreate the datasets used in this study can be found in the ‘Data collection’ section.
Code availability
The code used for the analyses presented in the Article is available at Open Science Framework ( https://doi.org/10.17605/osf.io/fq5dy ). The repository includes dummy datasets to illustrate the required data format and make the code run.
Cinelli, M., Morales, G. D. F., Galeazzi, A., Quattrociocchi, W. & Starnini, M. The echo chamber effect on social media. Proc. Natl Acad. Sci. USA 118 , e2023301118 (2021).
Article CAS PubMed PubMed Central Google Scholar
Tucker, J. A. et al. Social media, political polarization, and political disinformation: a review of the scientific literature. Preprint at SSRN https://doi.org/10.2139/ssrn.3144139 (2018).
González-Bailón, S. et al. Asymmetric ideological segregation in exposure to political news on Facebook. Science 381 , 392–398 (2023).
Article PubMed ADS Google Scholar
Guess, A. et al. How do social media feed algorithms affect attitudes and behavior in an election campaign? Science 381 , 398–404 (2023).
Article CAS PubMed ADS Google Scholar
Del Vicario, M. et al. The spreading of misinformation online. Proc. Natl Acad. Sci. USA 113 , 554–559 (2016).
Article PubMed PubMed Central ADS Google Scholar
Bakshy, E., Messing, S. & Adamic, L. A. Exposure to ideologically diverse news and opinion on Facebook. Science 348 , 1130–1132 (2015).
Article MathSciNet CAS PubMed ADS Google Scholar
Bail, C. A. et al. Exposure to opposing views on social media can increase political polarization. Proc. Natl Acad. Sci. USA 115 , 9216–9221 (2018).
Article CAS PubMed PubMed Central ADS Google Scholar
Nyhan, B. et al. Like-minded sources on Facebook are prevalent but not polarizing. Nature 620 , 137–144 (2023).
Guess, A. et al. Reshares on social media amplify political news but do not detectably affect beliefs or opinions. Science 381 , 404–408 (2023).
Castaño-Pulgaŕın, S. A., Suárez-Betancur, N., Vega, L. M. T. & López, H. M. H. Internet, social media and online hate speech. Systematic review. Aggress. Viol. Behav. 58 , 101608 (2021).
Article Google Scholar
Sheth, A., Shalin, V. L. & Kursuncu, U. Defining and detecting toxicity on social media: context and knowledge are key. Neurocomputing 490 , 312–318 (2022).
Lupu, Y. et al. Offline events and online hate. PLoS ONE 18 , e0278511 (2023).
Gentzkow, M. & Shapiro, J. M. Ideological segregation online and offline. Q. J. Econ. 126 , 1799–1839 (2011).
Aichner, T., Grünfelder, M., Maurer, O. & Jegeni, D. Twenty-five years of social media: a review of social media applications and definitions from 1994 to 2019. Cyberpsychol. Behav. Social Netw. 24 , 215–222 (2021).
Lazer, D. M. et al. The science of fake news. Science 359 , 1094–1096 (2018).
Cinelli, M. et al. Dynamics of online hate and misinformation. Sci. Rep. 11 , 22083 (2021).
González-Bailón, S. & Lelkes, Y. Do social media undermine social cohesion? A critical review. Soc. Issues Pol. Rev. 17 , 155–180 (2023).
Roozenbeek, J. & Zollo, F. Democratize social-media research—with access and funding. Nature 612 , 404–404 (2022).
Article CAS PubMed Google Scholar
Dutton, W. H. Network rules of order: regulating speech in public electronic fora. Media Cult. Soc. 18 , 269–290 (1996).
Papacharissi, Z. Democracy online: civility, politeness, and the democratic potential of online political discussion groups. N. Media Soc. 6 , 259–283 (2004).
Coe, K., Kenski, K. & Rains, S. A. Online and uncivil? Patterns and determinants of incivility in newspaper website comments. J. Commun. 64 , 658–679 (2014).
Anderson, A. A., Brossard, D., Scheufele, D. A., Xenos, M. A. & Ladwig, P. The “nasty effect:” online incivility and risk perceptions of emerging technologies. J. Comput. Med. Commun. 19 , 373–387 (2014).
Garrett, R. K. Echo chambers online?: Politically motivated selective exposure among internet news users. J. Comput. Med. Commun. 14 , 265–285 (2009).
Del Vicario, M. et al. Echo chambers: emotional contagion and group polarization on Facebook. Sci. Rep. 6 , 37825 (2016).
Garimella, K., De Francisci Morales, G., Gionis, A. & Mathioudakis, M. Echo chambers, gatekeepers, and the price of bipartisanship. In Proc. 2018 World Wide Web Conference , 913–922 (International World Wide Web Conferences Steering Committee, 2018).
Johnson, N. et al. Hidden resilience and adaptive dynamics of the global online hate ecology. Nature 573 , 261–265 (2019).
Fortuna, P. & Nunes, S. A survey on automatic detection of hate speech in text. ACM Comput. Surv. 51 , 85 (2018).
Phadke, S. & Mitra, T. Many faced hate: a cross platform study of content framing and information sharing by online hate groups. In Proceedings of the 2020 CHI Conference on Human Factors in Computing Systems 1–13 (Association for Computing Machinery, 2020).
Xia, Y., Zhu, H., Lu, T., Zhang, P. & Gu, N. Exploring antecedents and consequences of toxicity in online discussions: a case study on Reddit. Proc. ACM Hum. Comput. Interact. 4 , 108 (2020).
Sipka, A., Hannak, A. & Urman, A. Comparing the language of qanon-related content on Parler, GAB, and Twitter. In Proc. 14th ACM Web Science Conference 2022 411–421 (Association for Computing Machinery, 2022).
Fortuna, P., Soler, J. & Wanner, L. Toxic, hateful, offensive or abusive? What are we really classifying? An empirical analysis of hate speech datasets. In Proc. 12th Language Resources and Evaluation Conference (eds Calzolari, E. et al.) 6786–6794 (European Language Resources Association, 2020).
Davidson, T., Warmsley, D., Macy, M. & Weber, I. Automated hate speech detection and the problem of offensive language. In Proc. International AAAI Conference on Web and Social Media 11 (Association for the Advancement of Artificial Intelligence, 2017).
Kolhatkar, V. et al. The SFU opinion and comments corpus: a corpus for the analysis of online news comments. Corpus Pragmat. 4 , 155–190 (2020).
Article PubMed Google Scholar
Lees, A. et al. A new generation of perspective API: efficient multilingual character-level transformers. In KDD'22: The 28th ACM SIGKDD Conference on Knowledge Discovery and Data Mining 3197–3207 (Association for Computing Machinery, 2022).
Vidgen, B. & Derczynski, L. Directions in abusive language training data, a systematic review: garbage in, garbage out. PLoS ONE 15 , e0243300 (2020).
Ross, G. J. & Jones, T. Understanding the heavy-tailed dynamics in human behavior. Phys. Rev. E 91 , 062809 (2015).
Article MathSciNet ADS Google Scholar
Choi, D., Chun, S., Oh, H., Han, J. & Kwon, T. T. Rumor propagation is amplified by echo chambers in social media. Sci. Rep. 10 , 310 (2020).
Beel, J., Xiang, T., Soni, S. & Yang, D. Linguistic characterization of divisive topics online: case studies on contentiousness in abortion, climate change, and gun control. In Proc. International AAAI Conference on Web and Social Media Vol. 16, 32–42 (Association for the Advancement of Artificial Intelligence, 2022).
Saveski, M., Roy, B. & Roy, D. The structure of toxic conversations on Twitter. In Proc. Web Conference 2021 (eds Leskovec, J. et al.) 1086–1097 (Association for Computing Machinery, 2021).
Juul, J. L. & Ugander, J. Comparing information diffusion mechanisms by matching on cascade size. Proc. Natl Acad. Sci. USA 118 , e2100786118 (2021).
Fariello, G., Jemielniak, D. & Sulkowski, A. Does Godwin’s law (rule of Nazi analogies) apply in observable reality? An empirical study of selected words in 199 million Reddit posts. N. Media Soc. 26 , 14614448211062070 (2021).
Qiu, J., Lin, Z. & Shuai, Q. Investigating the opinions distribution in the controversy on social media. Inf. Sci. 489 , 274–288 (2019).
Garimella, K., Morales, G. D. F., Gionis, A. & Mathioudakis, M. Quantifying controversy on social media. ACM Trans. Soc. Comput. 1 , 3 (2018).
NLPTown. bert-base-multilingual-uncased-sentiment, huggingface.co/nlptown/bert-base-multilingual-uncased-sentiment (2023).
Ta, H. T., Rahman, A. B. S., Najjar, L. & Gelbukh, A. Transfer Learning from Multilingual DeBERTa for Sexism Identification CEUR Workshop Proceedings Vol. 3202 (CEUR-WS, 2022).
Kleinberg, J. Bursty and hierarchical structure in streams. Data Min. Knowl. Discov. 7 , 373–397 (2003).
Article MathSciNet Google Scholar
Zollo, F. et al. Debunking in a world of tribes. PLoS ONE 12 , e0181821 (2017).
Article PubMed PubMed Central Google Scholar
Albrecht, D. Vaccination, politics and COVID-19 impacts. BMC Publ. Health 22 , 96 (2022).
Article CAS Google Scholar
Falkenberg, M. et al. Growing polarization around climate change on social media. Nat. Clim. Change 12 , 1114–1121 (2022).
Schmidt, A. L., Zollo, F., Scala, A., Betsch, C. & Quattrociocchi, W. Polarization of the vaccination debate on Facebook. Vaccine 36 , 3606–3612 (2018).
Schmidt, A. L. et al. Anatomy of news consumption on Facebook. Proc. Natl Acad. Sci. USA 114 , 3035–3039 (2017).
Del Vicario, M., Zollo, F., Caldarelli, G., Scala, A. & Quattrociocchi, W. Mapping social dynamics on Facebook: the brexit debate. Soc. Netw. 50 , 6–16 (2017).
Hunnicutt, T. & Dave, P. Gab.com goes offline after Pittsburgh synagogue shooting. Reuters , www.reuters.com/article/uk-pennsylvania-shooting-gab-idUKKCN1N20QN (29 October 2018).
Valensise, C. M. et al. Lack of evidence for correlation between COVID-19 infodemic and vaccine acceptance. Preprint at arxiv.org/abs/2107.07946 (2021).
Quattrociocchi, A., Etta, G., Avalle, M., Cinelli, M. & Quattrociocchi, W. in Social Informatics (eds Hopfgartner, F. et al.) 245–256 (Springer, 2022).
Mekacher, A. & Papasavva, A. “I can’t keep it up” a dataset from the defunct voat.co news aggregator. In Proc. International AAAI Conference on Web and Social Media Vol. 16, 1302–1311 (AAAI, 2022).
Facebook Community Standards , transparency.fb.com/policies/community-standards/hate-speech/ (Facebook, 2023).
Rosen, G. & Lyons, T. Remove, reduce, inform: new steps to manage problematic content. Meta , about.fb.com/news/2019/04/remove-reduce-inform-new-steps/ (10 April 2019).
Vulgar Language Policy , support.google.com/youtube/answer/10072685? (YouTube, 2023).
Harassment & Cyberbullying Policies , support.google.com/youtube/answer/2802268 (YouTube, 2023).
Hate Speech Policy , support.google.com/youtube/answer/2801939 (YouTube, 2023).
How Does YouTube Enforce Its Community Guidelines? , www.youtube.com/intl/enus/howyoutubeworks/policies/community-guidelines/enforcing-community-guidelines (YouTube, 2023).
The Twitter Rules , help.twitter.com/en/rules-and-policies/twitter-rules (Twitter, 2023).
Hateful Conduct , help.twitter.com/en/rules-and-policies/hateful-conduct-policy (Twitter, 2023).
Gorwa, R., Binns, R. & Katzenbach, C. Algorithmic content moderation: technical and political challenges in the automation of platform governance. Big Data Soc. 7 , 2053951719897945 (2020).
Our Range of Enforcement Options , help.twitter.com/en/rules-and-policies/enforcement-options (Twitter, 2023).
Elliott, V. & Stokel-Walker, C. Twitter’s moderation system is in tatters. WIRED (17 November 2022).
Reddit Content Policy , www.redditinc.com/policies/content-policy (Reddit, 2023).
Promoting Hate Based on Identity or Vulnerability , www.reddithelp.com/hc/en-us/articles/360045715951 (Reddit, 2023).
Malik, A. Reddit acqui-hires team from ML content moderation startup Oterlu. TechCrunch , tcrn.ch/3yeS2Kd (4 October 2022).
Terms of Service , telegram.org/tos (Telegram, 2023).
Durov, P. The rules of @telegram prohibit calls for violence and hate speech. We rely on our users to report public content that violates this rule. Twitter , twitter.com/durov/status/917076707055751168?lang=en (8 October 2017).
Telegram Privacy Policy , telegram.org/privacy (Telegram, 2023).
Terms of Service , gab.com/about/tos (Gab, 2023).
Salzenberg, C. & Spafford, G. What is Usenet? , www0.mi.infn.it/ ∼ calcolo/Wis usenet.html (1995).
Castelle, M. The linguistic ideologies of deep abusive language classification. In Proc. 2nd Workshop on Abusive Language Online (ALW2) (eds Fišer, D. et al.) 160–170, aclanthology.org/W18-5120 (Association for Computational Linguistics, 2018).
Tontodimamma, A., Nissi, E. & Sarra, A. E. A. Thirty years of research into hate speech: topics of interest and their evolution. Scientometrics 126 , 157–179 (2021).
Sap, M. et al. Annotators with attitudes: how annotator beliefs and identities bias toxic language detection. In Proc. 2022 Conference of the North American Chapter of the Association for Computational Linguistics: Human Language Technologies (eds. Carpuat, M. et al.) 5884–5906 (Association for Computational Linguistics, 2022).
Pavlopoulos, J., Sorensen, J., Dixon, L., Thain, N. & Androutsopoulos, I. Toxicity detection: does context really matter? In Proc. 58th Annual Meeting of the Association for Computational Linguistics (eds Jurafsky, D. et al.) 4296–4305 (Association for Computational Linguistics, 2020).
Yin, W. & Zubiaga, A. Hidden behind the obvious: misleading keywords and implicitly abusive language on social media. Online Soc. Netw. Media 30 , 100210 (2022).
Sap, M., Card, D., Gabriel, S., Choi, Y. & Smith, N. A. The risk of racial bias in hate speech detection. In Proc. 57th Annual Meeting of the Association for Computational Linguistics (eds Kohonen, A. et al.) 1668–1678 (Association for Computational Linguistics, 2019).
Rosenblatt, L., Piedras, L. & Wilkins, J. Critical perspectives: a benchmark revealing pitfalls in PerspectiveAPI. In Proc. Second Workshop on NLP for Positive Impact (NLP4PI) (eds Biester, L. et al.) 15–24 (Association for Computational Linguistics, 2022).
DiMaggio, P., Evans, J. & Bryson, B. Have American’s social attitudes become more polarized? Am. J. Sociol. 102 , 690–755 (1996).
Fiorina, M. P. & Abrams, S. J. Political polarization in the American public. Annu. Rev. Polit. Sci. 11 , 563–588 (2008).
Iyengar, S., Gaurav, S. & Lelkes, Y. Affect, not ideology: a social identity perspective on polarization. Publ. Opin. Q. 76 , 405–431 (2012).
Cota, W., Ferreira, S. & Pastor-Satorras, R. E. A. Quantifying echo chamber effects in information spreading over political communication networks. EPJ Data Sci. 8 , 38 (2019).
Bessi, A. et al. Users polarization on Facebook and Youtube. PLoS ONE 11 , e0159641 (2016).
Bessi, A. et al. Science vs conspiracy: collective narratives in the age of misinformation. PLoS ONE 10 , e0118093 (2015).
Himelboim, I., McCreery, S. & Smith, M. Birds of a feather tweet together: integrating network and content analyses to examine cross-ideology exposure on Twitter. J. Comput. Med. Commun. 18 , 40–60 (2013).
An, J., Quercia, D. & Crowcroft, J. Partisan sharing: Facebook evidence and societal consequences. In Proc. Second ACM Conference on Online Social Networks, COSN ′ 14 13–24 (Association for Computing Machinery, 2014).
Mann, H. B. & Whitney, D. R. On a test of whether one of two random variables is stochastically larger than the other. Ann. Math. Stat. 18 , 50–60 (1947).
Download references
Acknowledgements
We thank M. Samory for discussions; T. Quandt and Z. Zhang for suggestions during the review process; and Geronimo Stilton and the Hypnotoad for inspiring the data analysis and result interpretation. The work is supported by IRIS Infodemic Coalition (UK government, grant no. SCH-00001-3391), SERICS (PE00000014) under the NRRP MUR program funded by the EU NextGenerationEU project CRESP from the Italian Ministry of Health under the program CCM 2022, PON project ‘Ricerca e Innovazione’ 2014-2020, and PRIN Project MUSMA for Italian Ministry of University and Research (MUR) through the PRIN 2022CUP G53D23002930006 and EU Next-Generation EU, M4 C2 I1.1.
Author information
These authors contributed equally: Michele Avalle, Niccolò Di Marco, Gabriele Etta
Authors and Affiliations
Department of Computer Science, Sapienza University of Rome, Rome, Italy
Michele Avalle, Niccolò Di Marco, Gabriele Etta, Shayan Alipour, Lorenzo Alvisi, Matteo Cinelli & Walter Quattrociocchi
Department of Social Sciences and Economics, Sapienza University of Rome, Rome, Italy
Emanuele Sangiorgio
Department of Communication and Social Research, Sapienza University of Rome, Rome, Italy
Anita Bonetti
Institute of Complex Systems, CNR, Rome, Italy
Antonio Scala
Department of Mathematics, City University of London, London, UK
Andrea Baronchelli
The Alan Turing Institute, London, UK
You can also search for this author in PubMed Google Scholar
Contributions
Conception and design: W.Q., M.A., M.C., G.E. and N.D.M. Data collection: G.E. and N.D.M. with collaboration from M.C., M.A. and S.A. Data analysis: G.E., N.D.M., M.A., M.C., W.Q., E.S., A. Bonetti, A. Baronchelli and A.S. Code writing: G.E. and N.D.M. with collaboration from M.A., E.S., S.A. and M.C. All of the authors provided critical feedback and helped to shape the research, analysis and manuscript, and contributed to the preparation of the manuscript.
Corresponding authors
Correspondence to Matteo Cinelli or Walter Quattrociocchi .
Ethics declarations
Competing interests.
The authors declare no competing interests.
Peer review
Peer review information.
Nature thanks Thorsten Quandt, Ziqi Zhang and the other, anonymous, reviewer(s) for their contribution to the peer review of this work.
Additional information
Publisher’s note Springer Nature remains neutral with regard to jurisdictional claims in published maps and institutional affiliations.
Extended data figures and tables
Extended data fig. 1 general characteristics of online conversations..
a . Distributions of conversation length (number of comments in a thread). b . Distributions of the time duration (days) of user activity on a platform for each platform and each topic. c . Time duration (days) distributions of threads. Colour-coded legend on the side.
Extended Data Fig. 2 Extremely toxic authors and conversations are rare.
a . Complementary cumulative distribution functions (CCDFs) of the toxicity of authors who posted more than 10 comments. Toxicity is defined as usual as the fraction of toxic comments over the total of comments posted by a user. b . CCDFs of the toxicity of conversations containing more than 10 comments. Colour-coded legend on the side.
Extended Data Fig. 3 User toxicity as conversations evolve.
Mean fraction of toxic comments as conversations progress. The x-axis represents the normalized position of comment intervals in the threads. For each dataset, toxicity is computed in the thread size interval [0.7−1] (see main text and Tab. S 2 in SI). Trends are reported with their 95% confidence interval. Colour-coded legend on the side.
Extended Data Fig. 4 Toxicity is not associated with conversation lifetime.
Mean toxicity of a . users versus their time of permanence in the dataset and b . threads versus their time duration. Trends are reported with their 95% confidence interval and they are reported using a normalized log-binning. Colour-coded legend on the side.
Extended Data Fig. 5 Results hold for a different toxicity threshold.
Core analyses presented in the paper repeated employing a lower (0.5) toxicity binary classification threshold. a . Mean fraction of toxic comments in conversations versus conversation size, for each dataset (see Fig. 2 ). Trends are reported with their 95% confidence interval. b . Pearson’s correlation coefficients between user participation and toxicity trends for each dataset. c . Pearson’s correlation coefficients between users’ participation in toxic and non-toxic thread sets, for each dataset. d . Boxplot of the distribution of toxicity ( n = 25, min = −0.016, max = 0.020, lower whisker = −0.005, Q 1 = − 0.005, Q 2 = 0.004, Q 3 = 0.012, upper whisker = 0.020) and participation ( n = 25, min = −0.198, max = −0.022, lower whisker = −0.198, Q 1 = − 0.109, Q 2 = − 0.070, Q 3 = − 0.049, upper whisker = −0.022) trend slopes for all datasets, as resulting from linear regression. The results of the relative Mann-Kendall tests for trend assessment are shown in Extended Data Table 5 .
Supplementary information
Supplementary information.
Supplementary Information 1–4, including details regarding data collection for validation dataset, Supplementary Figs. 1–3, Supplementary Tables 1–17 and software and coding specifications.
Reporting Summary
Rights and permissions.
Open Access This article is licensed under a Creative Commons Attribution 4.0 International License, which permits use, sharing, adaptation, distribution and reproduction in any medium or format, as long as you give appropriate credit to the original author(s) and the source, provide a link to the Creative Commons licence, and indicate if changes were made. The images or other third party material in this article are included in the article’s Creative Commons licence, unless indicated otherwise in a credit line to the material. If material is not included in the article’s Creative Commons licence and your intended use is not permitted by statutory regulation or exceeds the permitted use, you will need to obtain permission directly from the copyright holder. To view a copy of this licence, visit http://creativecommons.org/licenses/by/4.0/ .
Reprints and permissions
About this article
Cite this article.
Avalle, M., Di Marco, N., Etta, G. et al. Persistent interaction patterns across social media platforms and over time. Nature (2024). https://doi.org/10.1038/s41586-024-07229-y
Download citation
Received : 30 April 2023
Accepted : 22 February 2024
Published : 20 March 2024
DOI : https://doi.org/10.1038/s41586-024-07229-y
Share this article
Anyone you share the following link with will be able to read this content:
Sorry, a shareable link is not currently available for this article.
Provided by the Springer Nature SharedIt content-sharing initiative
By submitting a comment you agree to abide by our Terms and Community Guidelines . If you find something abusive or that does not comply with our terms or guidelines please flag it as inappropriate.
Quick links
- Explore articles by subject
- Guide to authors
- Editorial policies
Sign up for the Nature Briefing newsletter — what matters in science, free to your inbox daily.

University of South Florida
College of Education
Tampa | St. Petersburg | Sarasota-Manatee
Main Navigation
87 usf research faculty and students presenting at aera 2024 conference.
- April 2, 2024
- College of Education News
It is the premiere event for educational research. The USF College of Education has 87 faculty and students presenting or speaking in 99 different sessions during the American Educational Research Association (AERA) Annual Meeting in Philadelphia from April 11-14.
View our USF researchers and their AERA presentation schedule organized by date and time below.
Thursday, APRIL 11
Dr. Michael Berson Engaging with the Past: Archival Photographs as Tools for Data and Analysis in Rural Middle School Social Studies (Poster 2) Thu, April 11, 9:00 to 10:30am, Pennsylvania Convention Center, Floor: Level 100, Room 115B
Dr. Yiting Chu A Pioneer Scholar’s Five Decades of Curriculum Theorizing and Praxis Thu, April 11, 9:00 to 10:30am, Pennsylvania Convention Center, Floor: Level 200, Room 201A
Dr. Lindsay E. Persohn, Leah Burger (Student), Kritin Valle Geren (Student) Pod Clubs for Professional Learning and Collegial Conversation: Knowledge, Advocacy, and Agency Thu, April 11, 9:00 to 10:30am, Pennsylvania Convention Center, Floor: Level 200, Exhibit Hall B
Dr. Brenda Townsend Walker "We Are Our History”: Historicizing Current Black-White Disparities in Exclusionary Discipline Thu, April 11, 9:00 to 10:30am, Pennsylvania Convention Center, Floor: Level 100, Room 103B
Dr. Meghan Bratkovich Great Expectations: A Discourse Analysis of Entrustable Behaviors in the EPA (Entrustable Professional Activities) Curriculum Developer's Guide Thu, April 11, 10:50am to 12:20pm, Philadelphia Marriott Downtown, Floor: Level 4, Room 415
Dr. Wendy Guo Fostering Racial Literacy Through Children’s Literature: Chinese American Students Interrogating Anti-Asian Racism in a Community-Based Book Club Thu, April 11, 10:50am to 12:20pm, Pennsylvania Convention Center, Floor: Level 200, Exhibit Hall B
Dr. Yiting Chu Asian American Teachers Negotiating Double Marginalization in Teaching and Solidarity Building Thu, April 11, 12:40 to 2:10pm, Pennsylvania Convention Center, Floor: Level 100, Room 105A
Dr. Wendy Guo International Relations Committee Invited Session: Amplifying Diasporan Literacies in Educational Research and Teaching Thu, April 11, 12:40 to 2:10pm, Pennsylvania Convention Center, Floor: Level 100, Room 120A
Dr. Patriann Smith Race, Language, and Nationality as Geopolitical, Occupational, and Pedagogical Borders in Teaching and Teacher Education Thu, April 11, 12:40 to 2:10pm, Pennsylvania Convention Center, Floor: Level 100, Room 113C
Dr. Kimberly Defusco, Dr. Michael B. Sherry, Dr. Glenn Smith How Alternative Online Discussion Forums Could Benefit Collaborative Written Argumentation Thu, April 11, 12:40 to 2:10pm, Philadelphia Marriott Downtown, Floor: Level 3, Room 310
Dr. Vonzell Agosto & Maria Migueliz Valcarlos (Student) Non-Joking Matters: The Ethics of Performance-Based Research Thu, April 11, 2:30 to 4:00pm, Philadelphia Marriott Downtown, Floor: Level 5, Salon J
Dr. Sara Barnard Flory A Hope and a Prayer: First-Year Experiences of a Black Male Physical Educator Thu, April 11, 2:30 to 4:00pm, Pennsylvania Convention Center, Floor: Level 200, Exhibit Hall
Dr. Sara Barnard Flory & Dr. Craigory Nieman “Put Yourself in Their Shoes”: Developing a Knowledge Base in Urban Physical Education Thu, April 11, 2:30 to 4:00pm, Pennsylvania Convention Center, Floor: Level 200, Exhibit Hall
Dr. Yiting Chu An AsianCrit Analysis of Asian American Teacher Motivations Thu, April 11, 2:30 to 4:00pm, Pennsylvania Convention Center, Floor: Level 200, Exhibit Hall B
Dr. Lorien Jordan A Critical Whiteness Discourse Analysis of Family Interactions on Racism and Whiteness Thu, April 11, 2:30 to 4:00pm, Pennsylvania Convention Center, Floor: Level 100, Room 107A
Dr. Sanghoon Park Augmented Reality-Based Classroom Management Simulation (Poster 38) Thu, April 11, 2:30 to 4:00pm, Pennsylvania Convention Center, Floor: Level 200, Exhibit Hall A
Dr. Charles Vanover Performing Ethics for Scholar/Artists Thu, April 11, 2:30 to 4:00pm, Philadelphia Marriott Downtown, Floor: Level 5, Salon J
Daria Smirnova (Student) Wondering, Seducing . . . Thematic Nonsense (Poster 5) Thu, April 11, 2:30 to 4:00pm, Pennsylvania Convention Center, Floor: Level 100, Room 115B
Dr. Susan Bennett Multicultural Children's Literature as a Tool for Hearing All Voices in the Classroom Thu, April 11, 4:20 to 5:50pm, Pennsylvania Convention Center, Floor: Level 200, Exhibit Hall B
Dr. Cynthia Castro-Minnehan Teaching for Social Justice in Mathematics Education Doctoral Preparation in the United States Thu, April 11, 4:20 to 5:50pm, Pennsylvania Convention Center, Floor: Level 100, Room 118A
Dr. Mandie Dunn Disaffected Teachers: Disrupting Normalized Feelings of Race and Gender in Teacher Education Research Thu, April 11, 4:20 to 5:50pm, Philadelphia Marriott Downtown, Floor: Level 4, Room 411
Dr. AnnMarie Gunn Multicultural Children's Literature as a Tool for Hearing All Voices in the Classroom Thu, April 11, 4:20 to 5:50pm, Pennsylvania Convention Center, Floor: Level 200, Exhibit Hall B
Dr. Sophia Han Examining Translanguaging Play-Based Family Literacy at Home Through the Suda Approach (Poster 16) Thu, April 11, 4:20 to 5:50pm, Pennsylvania Convention Center, Floor: Level 200, Exhibit Hall A
Xilong Jing (Student) Within the Context of “Double Reduction” Policy, Exploring Parent’s Behaviors by ABC Model (Poster 33) Thu, April 11, 4:20 to 5:50pm, Pennsylvania Convention Center, Floor: Level 200, Exhibit Hall A
Friday, April 12
Dr. Cheryl Ellerbrock & Dr. Karyn Z. Mendez A Secret Society of Readers: Constructing Possibilities Through Mentored Reading Experiences Fri, April 12, 7:45 to 9:15am, Pennsylvania Convention Center, Floor: Level 200, Exhibit Hall B
Dr. Dulsey Hunter & Dr. Lindsay E. Persohn A Secret Society of Readers: Constructing Possibilities Through Mentored Reading Experiences Fri, April 12, 7:45 to 9:15am, Pennsylvania Convention Center, Floor: Level 200, Exhibit Hall B
Dr. Jennifer Jacobs Reimagining Leadership Through Teacher Leadership Fri, April 12, 7:45 to 9:15am, Pennsylvania Convention Center, Floor: Level 100, Room 115A
Dr. Karen Ramlackhan Leadership Strategies and Instructional Practices That Enhance Equity and Cultural Responsiveness Fri, April 12, 7:45 to 9:15am, Pennsylvania Convention Center, Floor: Level 100, Room 118A
Dr. Patriann Smith Black Englishes and the Global Multilingual Imperative Fri, April 12, 7:45 to 9:15am, Pennsylvania Convention Center, Floor: Level 200, Exhibit Hall B
Dr. Brenda Townsend Walker Loud, Proud, and Love a Crowd—Remix Fri, April 12, 7:45 to 9:15am, Philadelphia Marriott Downtown, Floor: Level 4, Franklin 8
Dr. Sophia Han, Dr. Jennifer Jacobs, Dr. Zorka Karanxha, Dr. Eugenia Vomvoridi-Ivanovic Continued Exploration and Envisioning of Culturally Responsive Pedagogy in Higher Education: A Collaborative Self-Study Fri, April 12, 9:35 to 11:05am, Pennsylvania Convention Center, Floor: Level 200, Exhibit Hall B
Dr. Veselina Lambrev Learning to Write as Scholars: A Collaborative Inquiry Into Ed.D. Students’ Academic Writing Development Fri, April 12, 9:35 to 11:05am, Pennsylvania Convention Center, Floor: Level 200, Exhibit Hall B
Dr. Susan Bennett Cross-Cultural Book Clubs and Culturally Sustaining Pedagogy Fri, April 12, 11:25am to 12:55pm, Philadelphia Marriott Downtown, Floor: Level 3, Room 307
Dr. Ilene Berson Ethical Imperatives and Pedagogical Potentials: AI Integration and Primary Source Analysis in Early Childhood and Elementary Education Fri, April 12, 11:25am to 12:55pm, Philadelphia Marriott Downtown, Floor: Level 4, Franklin 11
Dr. Wendy Guo Educational Justice Within and Beyond School Buildings (Table 28) Fri, April 12, 11:25am to 12:55pm, Pennsylvania Convention Center, Floor: Level 200, Exhibit Hall B
Dr. Sophia Han Exploring Prospective Early Education Professionals' Cultural Competence Working With Asian/Asian American Communities Fri, April 12, 11:25am to 12:55pm, Philadelphia Marriott Downtown, Floor: Level 3, Room 307
Dr. Glenn Smith Harnessing ChatGPT to Craft Custom Learning Games Fri, April 12, 11:25am to 12:55pm, Philadelphia Marriott Downtown, Floor: Level 4, Franklin 11
Dr. Karen Ramlackhan, Marisa Gourley (Student), Sanora L. White (Student) Transforming Doctoral Student Mentorship Through Creative and Arts-Based Approaches Fri, April 12, 11:25am to 12:55pm, Pennsylvania Convention Center, Floor: Level 200, Exhibit Hall B
Dr. John Ferron Methodological Innovations and Complexities in the Analysis and Meta-Analysis of Single-Subject Experimental Design Data Fri, April 12, 3:05 to 4:35pm, Pennsylvania Convention Center, Floor: Level 100, Room 115B
Dr. Alexandra Panos, Katharine Hull (Student), Kritin Valle Geren (Student) Supporting Preservice Teachers’ Navigation of Policy: Research-Based Design to Develop Policy Literacy Fri, April 12, 3:05 to 4:35pm, Pennsylvania Convention Center, Floor: Level 100, Room 104A
Lodi Lipien (Student) Confidence Intervals for Fine-Grained Effect Sizes (Poster 4) Fri, April 12, 3:05 to 4:35pm, Pennsylvania Convention Center, Floor: Level 100, Room 115B
Dr. Marie Byrd Urban Black/African American Teenagers: Culturally Relevant Social and Emotional Development in Out-of-School-Time Settings Fri, April 12, 4:55 to 6:25pm, Pennsylvania Convention Center, Floor: Level 200, Exhibit Hall B
Dr. Robert Dedrick, Dr. Constance Hines, Dr. Eunsook Kim Content Validation of the Elementary School Climate Assessment Instrument for Use in the Caribbean Fri, April 12, 4:55 to 6:25pm, Philadelphia Marriott Downtown, Floor: Level 4, Room 402
Dr. Amber Dumford Dismantling the Color Lines of the 21st Century: Race and Racism in AIEnabled Social and Virtual Spaces Fri, April 12, 4:55 to 6:25pm, Pennsylvania Convention Center, Floor: Level 200, Room 201C
Dr. Courtney Howard-Kirby Trifactor Mixture Modeling for Multi-Informant Assessment: A Simulation Study Fri, April 12, 4:55 to 6:25pm, Philadelphia Marriott Downtown, Floor: Level 4, Room 403
Dr. Lorien Jordan Illusory Presence: A Critical Assessment of Culturally Responsive Qualitative Research Fri, April 12, 4:55 to 6:25pm, Pennsylvania Convention Center, Floor: Level 200, Exhibit Hall B
Dr. Sanghoon Park Virtual Training Simulation: Influences of Physical Fidelity of Virtual Reality Controllers on Task Load and Performance Fri, April 12, 4:55 to 6:25pm, Philadelphia Marriott Downtown, Floor: Level 3, Room 310
Dr. Lindsay E. Persohn & Leah Burger (Student) A Model for Podcasting as Knowledge Dissemination: From Concept, Through Production, to Promotion Fri, April 12, 4:55 to 6:25pm, Pennsylvania Convention Center, Floor: Level 200, Exhibit Hall B
Dr. Karen Ramlackhan Challenges and Possibilities of U.S. State Education Policy for Culture, Race, and Intersectionality Fri, April 12, 4:55 to 6:25pm, Philadelphia Marriott Downtown, Floor: Level 5, Salon B
Dr. Dana Thompson Dorsey & Darlshawn Patterson (Student) Graduate Student Council Division G Fireside Chat: Unraveling Tensions in Educational Policies: Book Banning, Curriculum, DEI, and Affirmative Action – A Call to Action Fri, April 12, 4:55 to 6:25pm, Philadelphia Marriott Downtown, Floor: Level 5, Salon H
Tara Indar (Student) Urban Black/African American Teenagers: Culturally Relevant Social and Emotional Development in Out-of-School-Time Settings Fri, April 12, 4:55 to 6:25pm, Pennsylvania Convention Center, Floor: Level 200, Exhibit Hall B
Sarah Putnam (Student) Within- and Cross-Language Relationships Between Oral Language Skills and Literacy Achievement for Spanish-English-Speaking Dual Language Learners Fri, April 12, 4:55 to 6:25pm, Pennsylvania Convention Center, Floor: Level 200, Exhibit Hall B
Michelle Rocha-Angelo (Student) Crossing Language Borders: The Testimonios of Latinx and Caribbean Immigrant Mothers in K–12 Public Schools Fri, April 12, 4:55 to 6:25pm, Pennsylvania Convention Center, Floor: Level 200, Exhibit Hall B
Dr. Yiting Chu Multicultural/Multiethnic Education: Theory, Research, and Practice 2024 SIG Business Meeting and Reception Fri, April 12, 6:45 to 8:15pm, Philadelphia Marriott Downtown, Floor: Level 4, Room 411
Saturday, APRIL 13
Dr. Allan Feldman The Use of the FEW (Food-Energy-Water) Nexus as a Basis for Authentic Secondary School STEM Activities (Poster 6) Sat, April 13, 7:45 to 9:15am, Pennsylvania Convention Center, Floor: Level 100, Room 118B
Dr. Alexandra Panos, Dr. Michael B. Sherry, Katharine Hull (Student), Kritin Valle Geren (Student) Reaching for Mangoes: Mobilizing Stories of Ecojustice and Teaching in Politically and Ecologically Vulnerable Places Sat, April 13, 7:45 to 9:15am, Pennsylvania Convention Center, Floor: Level 200, Exhibit Hall B
Rita G Ortiz (Student) The Use of the FEW (Food-Energy-Water) Nexus as a Basis for Authentic Secondary School STEM Activities (Poster 6) Sat, April 13, 7:45 to 9:15am, Pennsylvania Convention Center, Floor: Level 100, Room 118B
Dr. Oscar Aliaga, Dr. David Allsopp, Dr. Lyman Dukes Transitioning to Postsecondary Education: Influence of Career and Technical Education on Students With Learning Disabilities Sat, April 13, 9:35 to 11:05am, Philadelphia Marriott Downtown, Floor: Level 3, Room 303
Dr. Ilene Berson Children, Parents, and Teachers in the Digital Age: Post-Pandemic Challenges and Preventative Solutions Sat, April 13, 9:35 to 11:05am, Philadelphia Marriott Downtown, Floor: Level 4, Franklin 12
Dr. Amber Dumford & Siyu Liu (Student) Estimating the Causal Effect of Learning Approaches on College Students' Four-Year Graduation Sat, April 13, 9:35 to 11:05am, Pennsylvania Convention Center, Floor: Level 100, Room 108A
Dr. Mandie Dunn Deepening Dialogue: How White Preservice Teachers Revised Assumptions in an Online Class About Linguistic Racism Sat, April 13, 9:35 to 11:05am, Pennsylvania Convention Center, Floor: Level 100, Room 115A
Dr. Allan Feldman Educational Action Research Sat, April 13, 9:35 to 11:05am, Philadelphia Marriott Downtown, Floor: Level 5, Salon G
Dr. Jarrett Gupton The School of DeSantis: A Critical Policy Analysis of Florida Educational Policy From 2020 to 2023 Sat, April 13, 9:35 to 11:05am, Pennsylvania Convention Center, Floor: Level 100, Room 110B
Dr. Sarah Kiefer & Kerrijo Ellis (Student) Culturally Sustaining and Motivating Practices: Fostering Equitable Learning Environments and Student Academic Success (Poster 44) Sat, April 13, 9:35 to 11:05am, Pennsylvania Convention Center, Floor: Level 200, Exhibit Hall A
Dr. Csaba Osvath Art, Words, and Existence: Unveiling the Transformative Power of Incarnational Literacy Sat, April 13, 9:35 to 11:05am, Pennsylvania Convention Center, Floor: Level 200, Exhibit Hall B
Dr. Michael B. Sherry Deepening Dialogue: How White Preservice Teachers Revised Assumptions in an Online Class About Linguistic Racism Sat, April 13, 9:35 to 11:05am, Pennsylvania Convention Center, Floor: Level 100, Room 115A
Dr. Brenda Townsend Walker Understanding and Addressing Racialized History and Violent Extremism Across P–12 Systems and Settings Sat, April 13, 9:35 to 11:05am, Pennsylvania Convention Center, Floor: Level 200, Room 201B
Kerrijo Ellis (Student) Directions for New Research: Middle Level Teacher Development Sat, April 13, 9:35 to 11:05am, Philadelphia Marriott Downtown, Floor: Level 4, Room 407
Dove Maria Elena Wimbish (Student) Online Discussion and Interaction Sat, April 13, 11:25am to 12:55pm, Philadelphia Marriott Downtown, Floor: Level 4, Franklin 11
Dr. Teresa Bergstrom The Wide Divide: Educator Reflections on Bridging the Gap Between Social Studies Theory and Practice Sat, April 13, 1:15 to 2:45pm, Pennsylvania Convention Center, Floor: Level 200, Exhibit Hall B
Dr. Richard Chapman The Power and Flexibility of Photovoice: Engaging Youth With Intellectual and Developmental Disabilities in Multiple Contexts Sat, April 13, 1:15 to 2:45pm, Pennsylvania Convention Center, Floor: Level 200, Exhibit Hall B
Dr. Lorien Jordan Of Pods and Possibilities: Reimagining Podcasting as a Qualitative DisCrit Methodology Sat, April 13, 1:15 to 2:45pm, Philadelphia Marriott Downtown, Floor: Level 3, Room 304
Dr. Sarah Kiefer Educational Psychology and Teacher Education: Reflections and Self-Study of a Professor Sat, April 13, 1:15 to 2:45pm, Philadelphia Marriott Downtown, Floor: Level 5, Salon I
Kerrijo Ellis (Student) Emerging Educational Psychologist: A Narrative Inquiry of a Doctoral Candidate’s Journey Sat, April 13, 1:15 to 2:45pm, Philadelphia Marriott Downtown, Floor: Level 5, Salon I
Dr. Fatima Almuthibi, Dr. Jolyn Blank, Dr. Amanda Mirabello, Terri Hubbard (Student), Leslie Reed (Student) Examining Play as Action Texts: Multimodal Interactions in Preschool Sat, April 13, 3:05 to 4:35pm, Pennsylvania Convention Center, Floor: Level 200, Exhibit Hall B
Dr. Allan Feldman Dialogic Collaborative Action Research: A Sustainable Form of Teacher Action Research Sat, April 13, 3:05 to 4:35pm, Philadelphia Marriott Downtown, Floor: Level 4, Room 404
Dr. Jenifer Jasinski Schneider, Dr. James R. King, Leah Burger (Student) Negotiating AI Outputs to Engineer Future Cities: Black Youth as Composing Agents Sat, April 13, 3:05 to 4:35pm, Pennsylvania Convention Center, Floor: Level 200, Exhibit Hall B
Dr. Karen Ramlackhan Critical Perceptions of Undocumented Students for Inclusion, Justice, and Opportunity Sat, April 13, 3:05 to 4:35pm, Pennsylvania Convention Center, Floor: Level 100, Room 111B
Dr. Jennifer R. Wolgemuth Qualitative Research SIG Business Meeting and Reception Sat, April 13, 6:45 to 8:15pm, Pennsylvania Convention Center, Floor: Level 100, Room 112B
Sunday, APRIL 14
Dr. Michael Berson & Dr. Ilene Berson Fragments of the Past: Exploring Multimodal Creative Inquiry in Early Childhood with AI-Powered Painting (Poster 31) Sun, April 14, 7:45 to 9:15am, Pennsylvania Convention Center, Floor: Level 200, Exhibit Hall A
Dr. Ann Cranston-Gingras & Dr. Karen Ramlackhan “So, I Am Back”: Adjudicated Youths’ School Reentry Experience (Poster 53) Sun, April 14, 7:45 to 9:15am, Pennsylvania Convention Center, Floor: Level 200, Exhibit Hall A
Christopher Darby (Student) Decision-Making Processes in Instrument Development and Implementation Sun, April 14, 7:45 to 9:15am, Pennsylvania Convention Center, Floor: Level 200, Room 201A
Dr. Dana Thompson Dorsey & Christopher Darby (Student) We Hear You: Amplifying Participants’ Voices in Race-Focused Survey Development Sun, April 14, 7:45 to 9:15am, Pennsylvania Convention Center, Floor: Level 200, Room 201A
Dr. Dana Thompson Dorsey Building a Racial Equity Survey: From Conceptualization to Implementation Sun, April 14, 7:45 to 9:15am, Pennsylvania Convention Center, Floor: Level 200, Room 201A
Dr. Arianna Banack Developing Racial Literacy Through a Racialized Reader Response Framework Sun, April 14, 9:35 to 11:05am, Pennsylvania Convention Center, Floor: Level 200, Exhibit Hall B
Dr. Lorien Jordan Aesthetic Experiencing: The Sandtray as Qualitative Method Sun, April 14, 9:35 to 11:05am, Pennsylvania Convention Center, Floor: Level 200, Exhibit Hall B
Dr. Michael B. Sherry Opening and Sustaining Dialogic Space of Possibilities: Examining Talk in and Across International Classrooms Sun, April 14, 9:35 to 11:05am, Philadelphia Marriott Downtown, Floor: Level 3, Room 305
Xilong Jing (Student) & Gen Li (Student) Vocational Student Burnout and Its Relationships With Parental Support, Psychological Capital, and Career Adaptability Sun, April 14, 9:35 to 11:05am, Pennsylvania Convention Center, Floor: Level 200, Exhibit Hall B
Dr. Marie Byrd & Tara Indar (Student) Cultural Competence as the Foundation for Culturally Relevant and Culturally Responsive Leadership Sun, April 14, 11:25am to 12:55pm, Pennsylvania Convention Center, Floor: Level 100, Room 118A
Dr. Wendy Guo Disrupting the Single Story: Transformative Children’s Literature for Social Justice Sun, April 14, 1:15 to 2:45pm, Philadelphia Marriott Downtown, Floor: Level 4, Franklin 7
Dr. Wendy Guo Chinese American Students Interrogating Racial Discourse With Children’s Literature in a Community-Based Book Club Sun, April 14, 1:15 to 2:45pm, Philadelphia Marriott Downtown, Floor: Level 4, Franklin 7
Dr. Karen Ramlackhan Decolonizing Teaching and Learning in Higher Education: Educators’ Realities and Transformative Possibilities Sun, April 14, 1:15 to 2:45pm, Pennsylvania Convention Center, Floor: Level 100, Room 108A
Dr. David Allsopp & Dr. Sarah A. van Ingen Lauer We Must Do Better: An Interdisciplinary Revision of Specific Learning Disabilities Evaluation Standards Sun, April 14, 1:15 to 2:45pm, Pennsylvania Convention Center, Floor: Level 200, Exhibit Hall B
Dr. Jennifer R. Wolgemuth #Scholar #Famous #Monster Sun, April 14, 1:15 to 2:45pm, Pennsylvania Convention Center, Floor: Level 200, Exhibit Hall B
Dr. Reham Abuemira & Dr. Meghan Bratkovich Supporting University Faculty in Teaching International Students: Findings From a Faculty Learning Community Sun, April 14, 3:05 to 4:35pm, Pennsylvania Convention Center, Floor: Level 200, Exhibit Hall B
Dr. Amber Dumford Why Is Everyone Packing Their Bags? COVID-19, Mental Health, Basic Needs, and Intent to Return Sun, April 14, 3:05 to 4:35pm, Pennsylvania Convention Center, Floor: Level 100, Room 112B
Dr. Bo Pei Beyond Surviving to Thriving: An Intelligent Assistance Performance Group Gap Analytics (PGGA) in a Gateway Course Sun, April 14, 3:05 to 4:35pm, Philadelphia Marriott Downtown, Floor: Level 3, Room 307
Daria Smirnova (Student) Teaching for Citizenship in the United States: Teachers’ Beliefs About the Aims of Civic Education Sun, April 14, 3:05 to 4:35pm, Pennsylvania Convention Center, Floor: Level 200, Exhibit Hall B
Dr. Jennifer R. Wolgemuth Teaching and Learning Research Methods SIG Business Meeting and Reception. Invited Panel About Teaching Research Methods Since the Pandemic Sun, April 14, 4:55 to 6:25pm, Pennsylvania Convention Center, Floor: Level 100, Room 109B
Lisa M. Lopez (Non Presenting) Radhika Sundar (Non Presenting) (Student) Tony Xing Tan (Non Presenting)
Return to article listing
Education Research
- Alumni and Friends
- Centers and Institutes
- Community Engagement
- Faculty and Staff
- Resources for Educators
- Student Life
About the USF College of Education:
As the home for more than 2,200 students and 130 faculty members across three campuses, the University of South Florida College of Education offers state-of-the-art teacher training and collegial graduate studies designed to empower educational leaders. Our college is nationally accredited by the Council for the Accreditation of Educator Preparation (CAEP), and our educator preparation programs are fully approved by the Florida Department of Education.
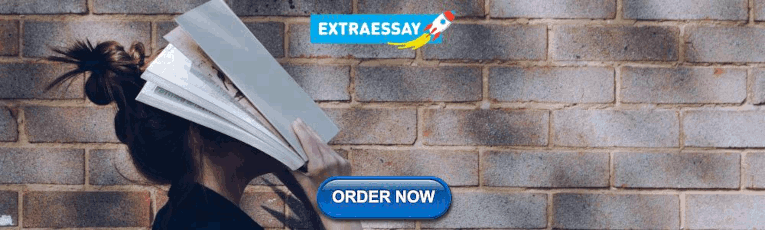
IMAGES
VIDEO
COMMENTS
It usually takes the form of a textual or content analysis. Discourse is understood as a way of perceiving, framing, and viewing the world. ... Sengul, K. (2019). Critical discourse analysis in political communication research: a case study of right-wing populist discourse in Australia. Communication Research and Practice, 5(4), 376-392.
Revised on June 22, 2023. Critical discourse analysis (or discourse analysis) is a research method for studying written or spoken language in relation to its social context. It aims to understand how language is used in real life situations. When you conduct discourse analysis, you might focus on: The purposes and effects of different types of ...
This chapter is structured from general to specific. There is some broad background first, making the analysis stage distinct from the data-gathering stages; then a close study of one example of a content analysis; and finally the second half of this chapter engages in a more detailed way with how a critical realist would approach discourse analysis within the mixed-methods setting.
There is a research about the top 100 cited discourse studies and in this study, the research finds out that educational discourses and news media coverage discourses are the most popular themes ...
Discourse analysis is a well-established qualitative research methodology that is used in a range of disciplines. Although there are a diversity of approaches within discourse analysis (including linguistic, ethnomethodological, semiotic, Althusserian, Gramscian, social constructionist, psychoanalytic, and poststructuralist variations), the commonalities underpinning these various methods ...
Content analysis is a research method used to identify patterns in recorded communication. To conduct content analysis, you systematically collect data from a set of texts, which can be written, oral, or visual: Books, newspapers and magazines. Speeches and interviews. Web content and social media posts. Photographs and films.
Interpretive approach: Discourse analysis is an interpretive approach, meaning that it seeks to understand the meaning and significance of language use from the perspective of the participants in a particular discourse. Emphasis on reflexivity: Discourse analysis emphasizes the importance of reflexivity, or self-awareness, in the research process.
Discourse analysis is a method that can be applied both to large volumes of material and to smaller samples, depending on the aims and timescale of your research. Example: Defining research question and selecting content You want to study how a particular regime change from dictatorship to democracy has affected the public relations rhetoric of ...
Content analysis was a method originally developed to analyze mass media "messages" in an age of radio and newspaper print, well before the digital age. Unfortunately, CTA struggles to break free of its origins and continues to be associated with the quantitative analysis of "communication.".
This chapter illustrates the analysis of texts and discourses in a mixed-methods context, starting with a content-analysis example. For systematic mixed-methods research, it can be advantageous to ...
Discourse analysis is an effective method to approach a wide range of research questions in health care and the. health professions. What underpins all variants of. discourse analysis is the idea of examining segments, or frames of communication, and using this to understand.
The analysis of written or transcribedspoken words, a subset of content analysis, is called text analysis. Its computer-aided form (now supported by more than 20 soft-wares) is called CATA (computer-aided text analysis), a fast-growing segment of the CA literature. CA is limited to a focus on messages.
Chapter 5, Discourse and the Strategic usage of Europe elaborates a research strategy allowing for the study of the strategic use of discourse for political purposes and serves as an illustration of the role of discursive agency politics. Chapter 6, Discourse, Myths and Emotions in EU Politics develops an analytical strategy for the study of ...
Qualitative researchers have developed a wide range of methods of analysis to make sense of textual data, one of the most common forms of data used in qualitative research (Attride-Stirling, 2001; Cho & Trent, 2006; Stenvoll & Svensson, 2011).As a result, qualitative text and discourse analysis (QTDA) has become a thriving methodological space characterized by the diversity of its approaches ...
A primary motivation for using discourse analysis is the ability to uncover dominant discourses, ideological assumptions, and power structures in texts, media content, or political speeches. Discourse analysis allows researchers to better understand and critically reflect on the role of language and discourse in society.
Overview. Previous explainers have introduced the topics of narrative and visual discourse analysis, which socio-environmental (S-E) researchers may use to identify the rhetorical impacts of images and text within a particular social context. Qualitative content analysis 1 (QCA) is an extension of narrative analysis that integrates specialized software. . QCA blends qualitative and ...
As Wodak and Krzyżanowski (2008) put it: "discourse analysis provides a general framework to problem-oriented social research". Basically, discourse analysis is used to conduct research on the use of language in context in a wide variety of social problems (i.e., issues in society that affect individuals negatively).
Methods and memberships. Our researchers in discourse analysis use a range of qualitative and quantitative methods (often combining both for the purposes of triangulation), such as focus groups, interviews, close linguistic analysis using methods from systemic functional linguistics and critical discourse analysis, corpus linguistics and "big ...
Jan. 18 Content+Discourse Analysis •Babbie‐Unobtrusive Research •Van Dijk‐Discourse Analysis •Krippendorf‐Content Analysis" Jan. 25 Content Analysis Theory and Design •Berelson‐Content Analysis in Comm Research •Holsti‐Content Analysis: An Introduction
This analysis is different (but similar) to content analysis, which is a research technique to systematically classify codes and identify themes or patterns within the data. Discourse analysis is concerned with identifying themes and patterns within the texts that relate to the social contexts reflected in the research topic and within the ...
Abstract. The study of titles in technical discourse and, specifically, in civil engineering research articles (CERA) is a pending issue within Languages for Specific Purposes (LSP). Research into this area can benefit researchers and students of engineering. This chapter aims to fill this gap by focusing on the most common phraseological and ...
Qualitative research often focuses on what people say: be that in interviews, focus-groups, diaries, social media or documents. Qualitative researchers often try to understand the world by listening to how people talk, but it can be really revealing to look at not just what people say, but how. Essentially this is the how discourse analysis (DA ...
Designed for instructor-led courses taught online or on campus, Cambridge courseware is a complete digital course solution built to increase student engagement. Choose from ready-made adaptive courses or partner with us to develop custom courseware for your institution. Browse Discourse Analysis higher education textbooks, teaching and learning ...
Growing concern surrounds the impact of social media platforms on public discourse 1,2,3,4 and their influence on social dynamics 5,6,7,8,9, especially in the context of toxicity 10,11,12.Here, to ...
In social science research (and especially in a socially engaged discourse analysis), critique is a methodological stance and an imminent characteristic of discourse analysis (Nonhoff, Citation 2019). Discourse analysis intervenes into the thematic field it deals with when it reconstructs how knowledge, identities and social positions are ...
It is the premiere event for educational research. ... A Critical Whiteness Discourse Analysis of Family Interactions on Racism and Whiteness Thu, April 11, 2:30 to 4:00pm, Pennsylvania Convention Center, Floor: Level 100, Room 107A ... Content Validation of the Elementary School Climate Assessment Instrument for Use in the Caribbean Fri, April ...