Have a language expert improve your writing
Run a free plagiarism check in 10 minutes, generate accurate citations for free.
- Knowledge Base
Methodology
- Mixed Methods Research | Definition, Guide & Examples
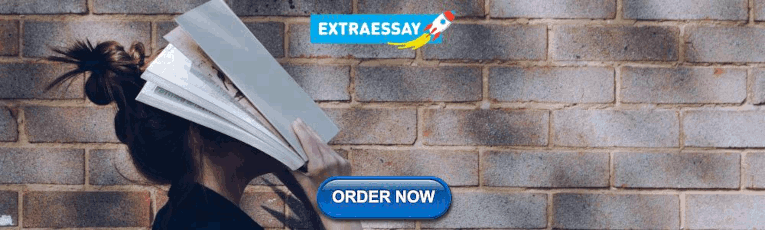
Mixed Methods Research | Definition, Guide & Examples
Published on August 13, 2021 by Tegan George . Revised on June 22, 2023.
Mixed methods research combines elements of quantitative research and qualitative research in order to answer your research question . Mixed methods can help you gain a more complete picture than a standalone quantitative or qualitative study, as it integrates benefits of both methods.
Mixed methods research is often used in the behavioral, health, and social sciences, especially in multidisciplinary settings and complex situational or societal research.
- To what extent does the frequency of traffic accidents ( quantitative ) reflect cyclist perceptions of road safety ( qualitative ) in Amsterdam?
- How do student perceptions of their school environment ( qualitative ) relate to differences in test scores ( quantitative ) ?
- How do interviews about job satisfaction at Company X ( qualitative ) help explain year-over-year sales performance and other KPIs ( quantitative ) ?
- How can voter and non-voter beliefs about democracy ( qualitative ) help explain election turnout patterns ( quantitative ) in Town X?
- How do average hospital salary measurements over time (quantitative) help to explain nurse testimonials about job satisfaction (qualitative) ?
Table of contents
When to use mixed methods research, mixed methods research designs, advantages of mixed methods research, disadvantages of mixed methods research, other interesting articles, frequently asked questions.
Mixed methods research may be the right choice if your research process suggests that quantitative or qualitative data alone will not sufficiently answer your research question. There are several common reasons for using mixed methods research:
- Generalizability : Qualitative research usually has a smaller sample size , and thus is not generalizable. In mixed methods research, this comparative weakness is mitigated by the comparative strength of “large N,” externally valid quantitative research.
- Contextualization: Mixing methods allows you to put findings in context and add richer detail to your conclusions. Using qualitative data to illustrate quantitative findings can help “put meat on the bones” of your analysis.
- Credibility: Using different methods to collect data on the same subject can make your results more credible. If the qualitative and quantitative data converge, this strengthens the validity of your conclusions. This process is called triangulation .
As you formulate your research question , try to directly address how qualitative and quantitative methods will be combined in your study. If your research question can be sufficiently answered via standalone quantitative or qualitative analysis, a mixed methods approach may not be the right fit.
But mixed methods might be a good choice if you want to meaningfully integrate both of these questions in one research study.
Keep in mind that mixed methods research doesn’t just mean collecting both types of data; you need to carefully consider the relationship between the two and how you’ll integrate them into coherent conclusions.
Mixed methods can be very challenging to put into practice, and comes with the same risk of research biases as standalone studies, so it’s a less common choice than standalone qualitative or qualitative research.
Receive feedback on language, structure, and formatting
Professional editors proofread and edit your paper by focusing on:
- Academic style
- Vague sentences
- Style consistency
See an example
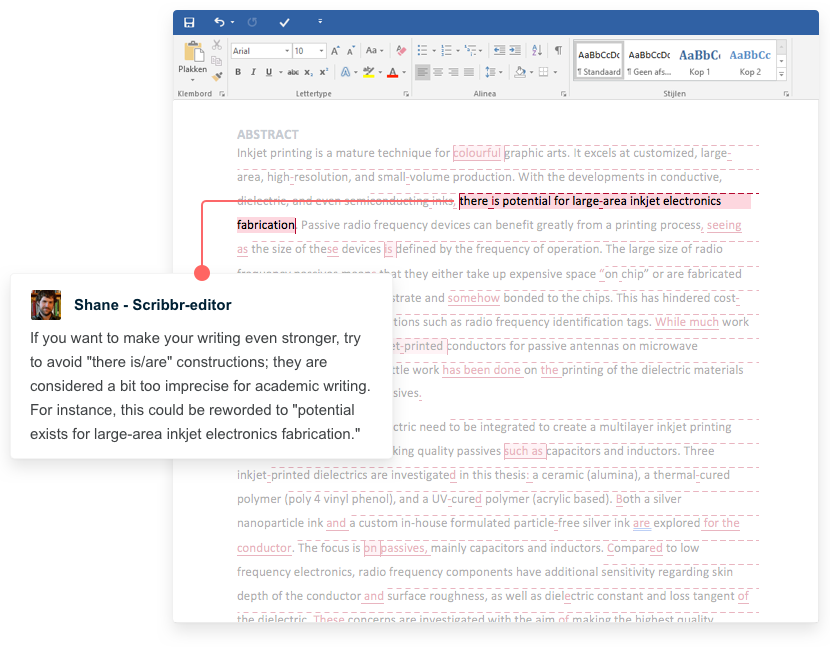
There are different types of mixed methods research designs . The differences between them relate to the aim of the research, the timing of the data collection , and the importance given to each data type.
As you design your mixed methods study, also keep in mind:
- Your research approach ( inductive vs deductive )
- Your research questions
- What kind of data is already available for you to use
- What kind of data you’re able to collect yourself.
Here are a few of the most common mixed methods designs.
Convergent parallel
In a convergent parallel design, you collect quantitative and qualitative data at the same time and analyze them separately. After both analyses are complete, compare your results to draw overall conclusions.
- On the qualitative side, you analyze cyclist complaints via the city’s database and on social media to find out which areas are perceived as dangerous and why.
- On the quantitative side, you analyze accident reports in the city’s database to find out how frequently accidents occur in different areas of the city.
In an embedded design, you collect and analyze both types of data at the same time, but within a larger quantitative or qualitative design. One type of data is secondary to the other.
This is a good approach to take if you have limited time or resources. You can use an embedded design to strengthen or supplement your conclusions from the primary type of research design.
Explanatory sequential
In an explanatory sequential design, your quantitative data collection and analysis occurs first, followed by qualitative data collection and analysis.
You should use this design if you think your qualitative data will explain and contextualize your quantitative findings.
Exploratory sequential
In an exploratory sequential design, qualitative data collection and analysis occurs first, followed by quantitative data collection and analysis.
You can use this design to first explore initial questions and develop hypotheses . Then you can use the quantitative data to test or confirm your qualitative findings.
“Best of both worlds” analysis
Combining the two types of data means you benefit from both the detailed, contextualized insights of qualitative data and the generalizable , externally valid insights of quantitative data. The strengths of one type of data often mitigate the weaknesses of the other.
For example, solely quantitative studies often struggle to incorporate the lived experiences of your participants, so adding qualitative data deepens and enriches your quantitative results.
Solely qualitative studies are often not very generalizable, only reflecting the experiences of your participants, so adding quantitative data can validate your qualitative findings.
Method flexibility
Mixed methods are less tied to disciplines and established research paradigms. They offer more flexibility in designing your research, allowing you to combine aspects of different types of studies to distill the most informative results.
Mixed methods research can also combine theory generation and hypothesis testing within a single study, which is unusual for standalone qualitative or quantitative studies.
Mixed methods research is very labor-intensive. Collecting, analyzing, and synthesizing two types of data into one research product takes a lot of time and effort, and often involves interdisciplinary teams of researchers rather than individuals. For this reason, mixed methods research has the potential to cost much more than standalone studies.
Differing or conflicting results
If your analysis yields conflicting results, it can be very challenging to know how to interpret them in a mixed methods study. If the quantitative and qualitative results do not agree or you are concerned you may have confounding variables , it can be unclear how to proceed.
Due to the fact that quantitative and qualitative data take two vastly different forms, it can also be difficult to find ways to systematically compare the results, putting your data at risk for bias in the interpretation stage.
Here's why students love Scribbr's proofreading services
Discover proofreading & editing
If you want to know more about statistics , methodology , or research bias , make sure to check out some of our other articles with explanations and examples.
- Degrees of freedom
- Null hypothesis
- Discourse analysis
- Control groups
- Non-probability sampling
- Quantitative research
- Inclusion and exclusion criteria
Research bias
- Rosenthal effect
- Implicit bias
- Cognitive bias
- Selection bias
- Negativity bias
- Status quo bias
Quantitative research deals with numbers and statistics, while qualitative research deals with words and meanings.
Quantitative methods allow you to systematically measure variables and test hypotheses . Qualitative methods allow you to explore concepts and experiences in more detail.
In mixed methods research , you use both qualitative and quantitative data collection and analysis methods to answer your research question .
Data collection is the systematic process by which observations or measurements are gathered in research. It is used in many different contexts by academics, governments, businesses, and other organizations.
Triangulation in research means using multiple datasets, methods, theories and/or investigators to address a research question. It’s a research strategy that can help you enhance the validity and credibility of your findings.
Triangulation is mainly used in qualitative research , but it’s also commonly applied in quantitative research . Mixed methods research always uses triangulation.
These are four of the most common mixed methods designs :
- Convergent parallel: Quantitative and qualitative data are collected at the same time and analyzed separately. After both analyses are complete, compare your results to draw overall conclusions.
- Embedded: Quantitative and qualitative data are collected at the same time, but within a larger quantitative or qualitative design. One type of data is secondary to the other.
- Explanatory sequential: Quantitative data is collected and analyzed first, followed by qualitative data. You can use this design if you think your qualitative data will explain and contextualize your quantitative findings.
- Exploratory sequential: Qualitative data is collected and analyzed first, followed by quantitative data. You can use this design if you think the quantitative data will confirm or validate your qualitative findings.
Cite this Scribbr article
If you want to cite this source, you can copy and paste the citation or click the “Cite this Scribbr article” button to automatically add the citation to our free Citation Generator.
George, T. (2023, June 22). Mixed Methods Research | Definition, Guide & Examples. Scribbr. Retrieved April 9, 2024, from https://www.scribbr.com/methodology/mixed-methods-research/
Is this article helpful?
Tegan George
Other students also liked, writing strong research questions | criteria & examples, what is quantitative research | definition, uses & methods, what is qualitative research | methods & examples, what is your plagiarism score.
- What is mixed methods research?
Last updated
20 February 2023
Reviewed by
Miroslav Damyanov
By blending both quantitative and qualitative data, mixed methods research allows for a more thorough exploration of a research question. It can answer complex research queries that cannot be solved with either qualitative or quantitative research .
Analyze your mixed methods research
Dovetail streamlines analysis to help you uncover and share actionable insights
Mixed methods research combines the elements of two types of research: quantitative and qualitative.
Quantitative data is collected through the use of surveys and experiments, for example, containing numerical measures such as ages, scores, and percentages.
Qualitative data involves non-numerical measures like beliefs, motivations, attitudes, and experiences, often derived through interviews and focus group research to gain a deeper understanding of a research question or phenomenon.
Mixed methods research is often used in the behavioral, health, and social sciences, as it allows for the collection of numerical and non-numerical data.
- When to use mixed methods research
Mixed methods research is a great choice when quantitative or qualitative data alone will not sufficiently answer a research question. By collecting and analyzing both quantitative and qualitative data in the same study, you can draw more meaningful conclusions.
There are several reasons why mixed methods research can be beneficial, including generalizability, contextualization, and credibility.
For example, let's say you are conducting a survey about consumer preferences for a certain product. You could collect only quantitative data, such as how many people prefer each product and their demographics. Or you could supplement your quantitative data with qualitative data, such as interviews and focus groups , to get a better sense of why people prefer one product over another.
It is important to note that mixed methods research does not only mean collecting both types of data. Rather, it also requires carefully considering the relationship between the two and method flexibility.
You may find differing or even conflicting results by combining quantitative and qualitative data . It is up to the researcher to then carefully analyze the results and consider them in the context of the research question to draw meaningful conclusions.
When designing a mixed methods study, it is important to consider your research approach, research questions, and available data. Think about how you can use different techniques to integrate the data to provide an answer to your research question.
- Mixed methods research design
A mixed methods research design is an approach to collecting and analyzing both qualitative and quantitative data in a single study.
Mixed methods designs allow for method flexibility and can provide differing and even conflicting results. Examples of mixed methods research designs include convergent parallel, explanatory sequential, and exploratory sequential.
By integrating data from both quantitative and qualitative sources, researchers can gain valuable insights into their research topic . For example, a study looking into the impact of technology on learning could use surveys to measure quantitative data on students' use of technology in the classroom. At the same time, interviews or focus groups can provide qualitative data on students' experiences and opinions.
- Types of mixed method research designs
Researchers often struggle to put mixed methods research into practice, as it is challenging and can lead to research bias. Although mixed methods research can reveal differences or conflicting results between studies, it can also offer method flexibility.
Designing a mixed methods study can be broken down into four types: convergent parallel, embedded, explanatory sequential, and exploratory sequential.
Convergent parallel
The convergent parallel design is when data collection and analysis of both quantitative and qualitative data occur simultaneously and are analyzed separately. This design aims to create mutually exclusive sets of data that inform each other.
For example, you might interview people who live in a certain neighborhood while also conducting a survey of the same people to determine their satisfaction with the area.
Embedded design
The embedded design is when the quantitative and qualitative data are collected simultaneously, but the qualitative data is embedded within the quantitative data. This design is best used when you want to focus on the quantitative data but still need to understand how the qualitative data further explains it.
For instance, you may survey students about their opinions of an online learning platform and conduct individual interviews to gain further insight into their responses.
Explanatory sequential design
In an explanatory sequential design, quantitative data is collected first, followed by qualitative data. This design is used when you want to further explain a set of quantitative data with additional qualitative information.
An example of this would be if you surveyed employees at a company about their satisfaction with their job and then conducted interviews to gain more information about why they responded the way they did.
Exploratory sequential design
The exploratory sequential design collects qualitative data first, followed by quantitative data. This type of mixed methods research is used when the goal is to explore a topic before collecting any quantitative data.
An example of this could be studying how parents interact with their children by conducting interviews and then using a survey to further explore and measure these interactions.
Integrating data in mixed methods studies can be challenging, but it can be done successfully with careful planning.
No matter which type of design you choose, understanding and applying these principles can help you draw meaningful conclusions from your research.
- Strengths of mixed methods research
Mixed methods research designs combine the strengths of qualitative and quantitative data, deepening and enriching qualitative results with quantitative data and validating quantitative findings with qualitative data. This method offers more flexibility in designing research, combining theory generation and hypothesis testing, and being less tied to disciplines and established research paradigms.
Take the example of a study examining the impact of exercise on mental health. Mixed methods research would allow for a comprehensive look at the issue from different angles.
Researchers could begin by collecting quantitative data through surveys to get an overall view of the participants' levels of physical activity and mental health. Qualitative interviews would follow this to explore the underlying dynamics of participants' experiences of exercise, physical activity, and mental health in greater detail.
Through a mixed methods approach, researchers could more easily compare and contrast their results to better understand the phenomenon as a whole.
Additionally, mixed methods research is useful when there are conflicting or differing results in different studies. By combining both quantitative and qualitative data, mixed methods research can offer insights into why those differences exist.
For example, if a quantitative survey yields one result while a qualitative interview yields another, mixed methods research can help identify what factors influence these differences by integrating data from both sources.
Overall, mixed methods research designs offer a range of advantages for studying complex phenomena. They can provide insight into different elements of a phenomenon in ways that are not possible with either qualitative or quantitative data alone. Additionally, they allow researchers to integrate data from multiple sources to gain a deeper understanding of the phenomenon in question.
- Challenges of mixed methods research
Mixed methods research is labor-intensive and often requires interdisciplinary teams of researchers to collaborate. It also has the potential to cost more than conducting a stand alone qualitative or quantitative study .
Interpreting the results of mixed methods research can be tricky, as it can involve conflicting or differing results. Researchers must find ways to systematically compare the results from different sources and methods to avoid bias.
For example, imagine a situation where a team of researchers has employed an explanatory sequential design for their mixed methods study. After collecting data from both the quantitative and qualitative stages, the team finds that the two sets of data provide differing results. This could be challenging for the team, as they must now decide how to effectively integrate the two types of data in order to reach meaningful conclusions. The team would need to identify method flexibility and be strategic when integrating data in order to draw meaningful conclusions from the conflicting results.
- Advanced frameworks in mixed methods research
Mixed methods research offers powerful tools for investigating complex processes and systems, such as in health and healthcare.
Besides the three basic mixed method designs—exploratory sequential, explanatory sequential, and convergent parallel—you can use one of the four advanced frameworks to extend mixed methods research designs. These include multistage, intervention, case study , and participatory.
This framework mixes qualitative and quantitative data collection methods in stages to gather a more nuanced view of the research question. An example of this is a study that first has an online survey to collect initial data and is followed by in-depth interviews to gain further insights.
Intervention
This design involves collecting quantitative data and then taking action, usually in the form of an intervention or intervention program. An example of this could be a research team who collects data from a group of participants, evaluates it, and then implements an intervention program based on their findings .
This utilizes both qualitative and quantitative research methods to analyze a single case. The researcher will examine the specific case in detail to understand the factors influencing it. An example of this could be a study of a specific business organization to understand the organizational dynamics and culture within the organization.
Participatory
This type of research focuses on the involvement of participants in the research process. It involves the active participation of participants in formulating and developing research questions, data collection, and analysis.
An example of this could be a study that involves forming focus groups with participants who actively develop the research questions and then provide feedback during the data collection and analysis stages.
The flexibility of mixed methods research designs means that researchers can choose any combination of the four frameworks outlined above and other methodologies , such as convergent parallel, explanatory sequential, and exploratory sequential, to suit their particular needs.
Through this method's flexibility, researchers can gain multiple perspectives and uncover differing or even conflicting results when integrating data.
When it comes to integration at the methods level, there are four approaches.
Connecting involves collecting both qualitative and quantitative data during different phases of the research.
Building involves the collection of both quantitative and qualitative data within a single phase.
Merging involves the concurrent collection of both qualitative and quantitative data.
Embedding involves including qualitative data within a quantitative study or vice versa.
- Techniques for integrating data in mixed method studies
Integrating data is an important step in mixed methods research designs. It allows researchers to gain further understanding from their research and gives credibility to the integration process. There are three main techniques for integrating data in mixed methods studies: triangulation protocol, following a thread, and the mixed methods matrix.
Triangulation protocol
This integration method combines different methods with differing or conflicting results to generate one unified answer.
For example, if a researcher wanted to know what type of music teenagers enjoy listening to, they might employ a survey of 1,000 teenagers as well as five focus group interviews to investigate this. The results might differ; the survey may find that rap is the most popular genre, whereas the focus groups may suggest rock music is more widely listened to.
The researcher can then use the triangulation protocol to come up with a unified answer—such as that both rap and rock music are popular genres for teenage listeners.
Following a thread
This is another method of integration where the researcher follows the same theme or idea from one method of data collection to the next.
A research design that follows a thread starts by collecting quantitative data on a specific issue, followed by collecting qualitative data to explain the results. This allows whoever is conducting the research to detect any conflicting information and further look into the conflicting information to understand what is really going on.
For example, a researcher who used this research method might collect quantitative data about how satisfied employees are with their jobs at a certain company, followed by qualitative interviews to investigate why job satisfaction levels are low. They could then use the results to explore any conflicting or differing results, allowing them to gain a deeper understanding of job satisfaction at the company.
By following a thread, the researcher can explore various research topics related to the original issue and gain a more comprehensive view of the issue.
Mixed methods matrix
This technique is a visual representation of the different types of mixed methods research designs and the order in which they should be implemented. It enables researchers to quickly assess their research design and adjust it as needed.
The matrix consists of four boxes with four different types of mixed methods research designs: convergent parallel, explanatory sequential, exploratory sequential, and method flexibility.
For example, imagine a researcher who wanted to understand why people don't exercise regularly. To answer this question, they could use a convergent parallel design, collecting both quantitative (e.g., survey responses) and qualitative (e.g., interviews) data simultaneously.
If the researcher found conflicting results, they could switch to an explanatory sequential design and collect quantitative data first, then follow up with qualitative data if needed. This way, the researcher can make adjustments based on their findings and integrate their data more effectively.
Mixed methods research is a powerful tool for understanding complex research topics. Using qualitative and quantitative data in one study allows researchers to understand their subject more deeply.
Mixed methods research designs such as convergent parallel, explanatory sequential, and exploratory sequential provide method flexibility, enabling researchers to collect both types of data while avoiding the limitations of either approach alone.
However, it's important to remember that mixed methods research can produce differing or even conflicting results, so it's important to be aware of the potential pitfalls and take steps to ensure that data is being correctly integrated. If used effectively, mixed methods research can offer valuable insight into topics that would otherwise remain largely unexplored.
What is an example of mixed methods research?
An example of mixed methods research is a study that combines quantitative and qualitative data. This type of research uses surveys, interviews, and observations to collect data from multiple sources.
Which sampling method is best for mixed methods?
It depends on the research objectives, but a few methods are often used in mixed methods research designs. These include snowball sampling, convenience sampling, and purposive sampling. Each method has its own advantages and disadvantages.
What is the difference between mixed methods and multiple methods?
Mixed methods research combines quantitative and qualitative data in a single study. Multiple methods involve collecting data from different sources, such as surveys and interviews, but not necessarily combining them into one analysis. Mixed methods offer greater flexibility but can lead to differing or conflicting results when integrating data.
Get started today
Go from raw data to valuable insights with a flexible research platform
Editor’s picks
Last updated: 21 December 2023
Last updated: 16 December 2023
Last updated: 6 October 2023
Last updated: 5 March 2024
Last updated: 25 November 2023
Last updated: 15 February 2024
Last updated: 11 March 2024
Last updated: 12 December 2023
Last updated: 6 March 2024
Last updated: 10 April 2023
Last updated: 20 December 2023
Latest articles
Related topics, log in or sign up.
Get started for free
Qualitative Research
- What is Qualitative Research
- PEO for Qualitative Questions
- SPIDER for Mixed Methods Qualitative Research Questions
- Finding Qualitative Research Articles
- Critical Appraisal of Qualitative Research Articles
- Mixed Methods Research
- Qualitative Synthesis
SPIDER: Mixed Methods Qualitative Research Questions
SPIDER is a search strategy for finding research to answer a mixed-method qualitative research question.

- Sample: Sample is similar to Patient/Population of PICO. This is the group of people you are interesting in studying qualitatively. For example, in the qualitative research question, "What are the barriers felt by clinicians that lead to the reluctance to use EBP in practice?", S = clinicians
- Phenomenon of Interest: The Phenomenon of Interest can be similar to Intervention of PICO. This can be viewed as the topic of the research. For example, in the qualitative research question, "What are the barriers felt by clinicians that lead to the reluctance to use EBP in practice?", PI = Use of EBP
- Focus Groups
- Observations
- Evaluation: Evaluation is similar to Outcomes of PICO. For example, in the qualitative research question, "What are the barriers felt by clinicians that lead to the reluctance to use EBP in practice?", E = Barriers to use of EBP
- Phenomenology
- Ethnography
- Grounded theory
Using SPIDER for Search Terms
Now that you have your qualitative research questions broken into SPiDER, you can now think about your search strategy and search terms.
For example, in the qualitative research question, " What are the barriers felt by clinicians that lead to the reluctance to use EBP in practice?"
NOTE: You might not use all these terms in your search.
FINAL BOOLEAN SEARCH:
(clinician* OR health care professional OR health care provider) AND (evidence-based practice OR EBP) AND ( interview* OR focus group*) AND (barrier* OR hinder* OR resist*) AND phenomenology
Cooke, A., Smith, D., & Booth, A. (2012). Beyond PICO: The SPIDER tool for qualitative evidence synthesis. Quality Health Research, 22 (10), 1435-1443. doi: 10.1177/1049732312452938
- << Previous: PEO for Qualitative Questions
- Next: Finding Qualitative Research Articles >>
- Last Updated: Aug 28, 2023 2:47 PM
- URL: https://researchguides.gonzaga.edu/qualitative
The Use of Mixed Methods in Research
- Reference work entry
- First Online: 13 January 2019
- Cite this reference work entry
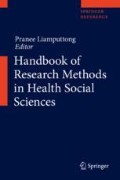
- Kate A. McBride 2 ,
- Freya MacMillan 3 ,
- Emma S. George 4 &
- Genevieve Z. Steiner 5
2448 Accesses
9 Citations
Mixed methods research is becoming increasingly popular and is widely acknowledged as a means of achieving a more complex understanding of research problems. Combining both the in-depth, contextual views of qualitative research with the broader generalizations of larger population quantitative approaches, mixed methods research can be used to produce a rigorous and credible source of data. Using this methodology, the same core issue is investigated through the collection, analysis, and interpretation of both types of data within one study or a series of studies. Multiple designs are possible and can be guided by philosophical assumptions. Both qualitative and quantitative data can be collected simultaneously or sequentially (in any order) through a multiphase project. Integration of the two data sources then occurs with consideration is given to the weighting of both sources; these can either be equal or one can be prioritized over the other. Designed as a guide for novice mixed methods researchers, this chapter gives an overview of the historical and philosophical roots of mixed methods research. We also provide a practical overview of its application in health research as well as pragmatic considerations for those wishing to undertake mixed methods research.
This is a preview of subscription content, log in via an institution to check access.
Access this chapter
- Available as PDF
- Read on any device
- Instant download
- Own it forever
- Available as EPUB and PDF
- Durable hardcover edition
- Dispatched in 3 to 5 business days
- Free shipping worldwide - see info
Tax calculation will be finalised at checkout
Purchases are for personal use only
Institutional subscriptions
Appleton JV, King L. Journeying from the philosophical contemplation of constructivism to the methodological pragmatics of health services research. J Adv Nurs. 2002;40(6):641–8.
Article Google Scholar
Baba CT, Oliveira IM, Silva AEF, Vieira LM, Cerri NC, Florindo AA, de Oliveira Gomes GA. Evaluating the impact of a walking program in a disadvantaged area: using the RE-AIM framework by mixed methods. BMC Public Health. 2017;17(1):709. https://doi.org/10.1186/s12889-017-4698-5 .
Bryman A. The research question in social research: what is its role? Int J Soc Res Methodol. 2007;10(1):5–20. https://doi.org/10.1080/13645570600655282 .
Bryman A. The end of the paradigm wars. In: Alasuutari P, Bickman L, Brannen J, editors. The Sage handbook of social research methods. Thousand Oaks: Sage; 2008. p. 13–25.
Google Scholar
Caracelli VJ, Greene JC. Data-analysis strategies for mixed-method evaluation designs. Educ Eval Policy Anal. 1993;15(2):195–207. https://doi.org/10.3102/01623737015002195 .
Castro FG, Kellison JG, Boyd SJ, Kopak A. A methodology for conducting integrative mixed methods research and data analyses. J Mixed Methods Res. 2010;4(4):342–60. https://doi.org/10.1177/1558689810382916 .
Center for Innovation in Teaching in Research. Choosing a mixed methods design. 2017. Retrieved 15 Nov 2017 from https://cirt.gcu.edu/research/developmentresources/research_ready/mixed_methods/choosing_design .
Creswell JW, Plano Clark VL. Designing and conducting mixed methods research. 3rd ed. Thousand Oaks: Sage; 2018.
Curry LA, Krumholz HM, O’Cathain A, Clark VLP, Cherlin E, Bradley EH. Mixed methods in biomedical and health services research. Circ-Cardiovasc Qual Outcomes. 2013;6(1):119–23. https://doi.org/10.1161/circoutcomes.112.967885 .
Denscombe M. Communities of practice: a research paradigm for the mixed methods approach. J Mixed Methods Res. 2008;2(3):270–83.
Doyle L, Brady A-M, Byrne G. An overview of mixed methods research. J Res Nurs. 2009;14(2):175–85.
Foster NE, Bishop A, Bartlam B, Ogollah R, Barlas P, Holden M, … Young J. Evaluating acupuncture and standard carE for pregnant women with back pain (EASE back): a feasibility study and pilot randomised trial. Health Technol Assess. 2016;20(33):1–236. https://doi.org/10.3310/hta20330 .
Haider AH, Schneider EB, Kodadek LM, Adler RR, Ranjit A, Torain M, … Lau BD. Emergency department query for patient-centered approaches to sexual orientation and gender identity the EQUALITY study. JAMA Intern Med. 2017;177(6):819–828. https://doi.org/10.1001/jamainternmed.2017.0906 .
Harding KE, Taylor NF, Bowers B, Stafford M, Leggat SG. Clinician and patient perspectives of a new model of triage in a community rehabilitation program that reduced waiting time: a qualitative analysis. Aust Health Rev. 2013;37(3):324–30. https://doi.org/10.1071/ah13033 .
Hoddinott P, Britten J, Prescott GJ, Tappin D, Ludbrook A, Godden DJ. Effectiveness of policy to provide breastfeeding groups ( BIG) for pregnant and breastfeeding mothers in primary care: cluster randomised controlled trial. Br Med J. 2009;338:a3026, 10. https://doi.org/10.1136/bmj.a3026 .
Ivankova NV, Creswell JW, Stick SL. Using mixed-methods sequential explanatory design: from theory to practice. Field Methods. 2006;18(1):3–20. https://doi.org/10.1177/1525822x05282260 .
Johnson B, Gray R. A history of philosophical and theoretical issues for mixed methods research. In: Tashakkori A, Teddlie C, editors. Sage handbook of mixed methods in social and behavioral research. 2nd ed. Thousand Oaks: Sage; 2010. p. 69–94.
Chapter Google Scholar
Johnson BR, Onwuegbuzie AJ. Mixed methods research: a research paradigm whose time has come. Educ Res. 2004;33(7):14–26. https://doi.org/10.3102/0013189x033007014 .
Keeney S, McKenna H, Fleming P, McIlfatrick S. Attitudes to cancer and cancer prevention: what do people aged 35–54 years think? Eur J Cancer Care. 2010;19(6):769–77. https://doi.org/10.1111/j.1365-2354.2009.01137.x .
McBride KA, Ballinger ML, Schlub TE, Young MA, Tattersall MHN, Kirk J, et al. Psychosocial morbidity in TP53 mutation carriers: is whole-body cancer screening beneficial? Familial Cancer. 2017;16(3):423–32. https://doi.org/10.1007/s10689-016-9964-7 .
Mertens DM. Transformative mixed methods: addressing inequities. Am Behav Sci. 2012;56(6):802–13. https://doi.org/10.1177/0002764211433797 .
Moffatt S, White M, Mackintosh J, Howel D. Using quantitative and qualitative data in health services research – what happens when mixed method findings conflict? BMC Health Serv Res. 2006;6:28. https://doi.org/10.1186/1472-6963-6-28 .
Morse JM. Approaches to qualitative-quantitative methodological triangulation. Nurs Res. 1991a;40(2):120–3.
Morse JM. Principles of mixed methods and multimethod research design. In: Tashakkori A, Teddilie C, editors. SAGE handbook of mixed methods in social and behavioral research. Thousand Oaks: Sage; 1991b.
Mutrie N, Doolin O, Fitzsimons CF, Grant PM, Granat M, Grealy M, et al. Increasing older adults’ walking through primary care: results of a pilot randomized controlled trial. Fam Pract. 2012;29(6):633–42. https://doi.org/10.1093/fampra/cms038 .
Newman I, Ridenour C, Newman C, De Marco G. A typology of research purposes and its relationship to mixed methods in social and behavioral research. Thousand Oaks: Sage; 2003. p. 167–88.
O’Cathain A, Murphy E, Nicholl J. The quality of mixed methods studies in health services research. J Health Serv Res Policy. 2008;13(2):92–8. https://doi.org/10.1258/jhsrp.2007.007074 .
Onwuegbuzie AJ, Collins KMT. A typology of mixed methods sampling designs in social science research. Qual Rep. 2007;12(2):281–316.
Plano Clark VL, Badiee M. Research questions in mixed methods research. In: Tashakkori A, Teddlie C, editors. SAGE handbook of mixed methods in social and behavioral research. 2nd ed. Thousand Oaks: Sage; 2010. p. 275–304.
Prades, J., Algara, M., Espinas, J. A., Farrus, B., Arenas, M., Reyes, V., . . . Borras, J. M. (2017). Understanding variations in the use of hypofractionated radiotherapy and its specific indications for breast cancer: a mixed-methods study. Radiother Oncol, 123(1), 22–28. doi: https://doi.org/10.1016/j.radonc.2017.01.014 .
Tariq S, Woodman J. Using mixed methods in health research. JRSM Short Rep. 2013; 4 (6):1–8. https://doi.org/10.1177/2042533313479197 .
Tashakkori A, Creswell JW. Editorial: the new era of mixed methods. J Mixed Methods Res. 2007;1(1):3–7. https://doi.org/10.1177/2345678906293042 .
Tashakkori A, Teddlie C. The past and future of mixed methods research: from data triangulation to mixed model designs. In: Tashakkori A, Teddlie C, editors. Handbook of mixed methods in social & behavioral research. Thousand Oaks: Sage; 2003. p. 671–701.
Teddlie C, Tashakkori A. Foundations of mixed methods research: integrating quantitative and qualitative approaches in the social and behavioral sciences. Thousand Oaks: Sage; 2009.
Teddlie C, Yu F. Mixed methods sampling a typology with examples. J Mixed Methods Res. 2007;1(1):77–100. https://doi.org/10.1177/2345678906292430 .
Wellard SJ, Rasmussen B, Savage S, Dunning T. Exploring staff diabetes medication knowledge and practices in regional residential care: triangulation study. J Clin Nurs. 2013;22(13–14):1933–40. https://doi.org/10.1111/jocn.12043 .
Zhang W. Mixed methods application in health intervention research: a multiple case study. Int J Mult Res Approaches. 2014;8(1):24–35. https://doi.org/10.5172/mra.2014.8.1.24 .
Download references
Author information
Authors and affiliations.
School of Medicine and Translational Health Research Institute, Western Sydney University, Sydney, NSW, Australia
Kate A. McBride
School of Science and Health and Translational Health Research Institute (THRI), Western Sydney University, Penrith, NSW, Australia
Freya MacMillan
School of Science and Health, Western Sydney University, Sydney, NSW, Australia
Emma S. George
NICM and Translational Health Research Institute (THRI), Western Sydney University, Penrith, NSW, Australia
Genevieve Z. Steiner
You can also search for this author in PubMed Google Scholar
Corresponding author
Correspondence to Kate A. McBride .
Editor information
Editors and affiliations.
School of Science and Health, Western Sydney University, Penrith, NSW, Australia
Pranee Liamputtong
Rights and permissions
Reprints and permissions
Copyright information
© 2019 Springer Nature Singapore Pte Ltd.
About this entry
Cite this entry.
McBride, K.A., MacMillan, F., George, E.S., Steiner, G.Z. (2019). The Use of Mixed Methods in Research. In: Liamputtong, P. (eds) Handbook of Research Methods in Health Social Sciences. Springer, Singapore. https://doi.org/10.1007/978-981-10-5251-4_97
Download citation
DOI : https://doi.org/10.1007/978-981-10-5251-4_97
Published : 13 January 2019
Publisher Name : Springer, Singapore
Print ISBN : 978-981-10-5250-7
Online ISBN : 978-981-10-5251-4
eBook Packages : Social Sciences Reference Module Humanities and Social Sciences Reference Module Business, Economics and Social Sciences
Share this entry
Anyone you share the following link with will be able to read this content:
Sorry, a shareable link is not currently available for this article.
Provided by the Springer Nature SharedIt content-sharing initiative
- Publish with us
Policies and ethics
- Find a journal
- Track your research
- Privacy Policy
Buy Me a Coffee

Home » Mixed Methods Research – Types & Analysis
Mixed Methods Research – Types & Analysis
Table of Contents

Mixed Methods Research
Mixed methods research is an approach to research that combines both quantitative and qualitative research methods in a single study or research project. It is a methodological approach that involves collecting and analyzing both numerical (quantitative) and narrative (qualitative) data to gain a more comprehensive understanding of a research problem.
Types of Mixed Research

There are different types of mixed methods research designs that researchers can use, depending on the research question, the available data, and the resources available. Here are some common types:
Convergent Parallel Design
This design involves collecting both qualitative and quantitative data simultaneously, analyzing them separately, and then merging the findings to draw conclusions. The qualitative and quantitative data are given equal weight, and the findings are integrated during the interpretation phase.
Sequential Explanatory Design
In this design, the researcher collects and analyzes quantitative data first, and then uses qualitative data to explain or elaborate on the quantitative findings. The researcher may use the qualitative data to clarify unexpected or contradictory results from the quantitative analysis.
Sequential Exploratory Design
This design involves collecting qualitative data first, analyzing it, and then collecting and analyzing quantitative data to confirm or refute the qualitative findings. Qualitative data are used to generate hypotheses that are tested using quantitative data.
Concurrent Triangulation Design
This design involves collecting both qualitative and quantitative data concurrently and then comparing the results to find areas of agreement and disagreement. The findings are integrated during the interpretation phase to provide a more comprehensive understanding of the research question.
Concurrent Nested Design
This design involves collecting one type of data as the primary method and then using the other type of data to elaborate or clarify the primary data. For example, a researcher may use quantitative data as the primary method and qualitative data as a secondary method to provide more context and detail.
Transformative Design
This design involves using mixed methods research to not only understand the research question but also to bring about social change or transformation. The research is conducted in collaboration with stakeholders and aims to generate knowledge that can be used to improve policies, programs, and practices.
Concurrent Embedded Design
Concurrent embedded design is a type of mixed methods research design in which one type of data is embedded within another type of data. This design involves collecting both quantitative and qualitative data simultaneously, with one type of data being the primary method and the other type of data being the secondary method. The secondary method is embedded within the primary method, meaning that it is used to provide additional information or to clarify the primary data.
Data Collection Methods
Here are some common data collection methods used in mixed methods research:
Surveys are a common quantitative data collection method used in mixed methods research. Surveys involve collecting standardized responses to a set of questions from a sample of participants. Surveys can be conducted online, in person, or over the phone.
Interviews are a qualitative data collection method that involves asking open-ended questions to gather in-depth information about a participant’s experiences, perspectives, and opinions. Interviews can be conducted in person, over the phone, or online.
Focus groups
Focus groups are a qualitative data collection method that involves bringing together a small group of participants to discuss a topic or research question. The group is facilitated by a researcher, and the discussion is recorded and analyzed for themes and patterns.
Observations
Observations are a qualitative data collection method that involves systematically watching and recording behavior in a natural setting. Observations can be structured or unstructured and can be used to gather information about behavior, interactions, and context.
Document Analysis
Document analysis is a qualitative data collection method that involves analyzing existing documents, such as reports, policy documents, or media articles. Document analysis can be used to gather information about trends, policy changes, or public attitudes.
Experimentation
Experimentation is a quantitative data collection method that involves manipulating one or more variables and measuring their effects on an outcome. Experiments can be conducted in a laboratory or in a natural setting.
Data Analysis Methods
Mixed methods research involves using both quantitative and qualitative data analysis methods to analyze data collected through different methods. Here are some common data analysis methods used in mixed methods research:
Quantitative Data Analysis
Quantitative data collected through surveys or experiments can be analyzed using statistical methods. Statistical analysis can be used to identify relationships between variables, test hypotheses, and make predictions. Common statistical methods used in quantitative data analysis include regression analysis, t-tests, ANOVA, and correlation analysis.
Qualitative Data Analysis
Qualitative data collected through interviews, focus groups, or observations can be analyzed using a variety of qualitative data analysis methods. These methods include content analysis, thematic analysis, narrative analysis, and grounded theory. Qualitative data analysis involves identifying themes and patterns in the data, interpreting the meaning of the data, and drawing conclusions based on the findings.
Integration of Data
The integration of quantitative and qualitative data involves combining the results from both types of data analysis to gain a more comprehensive understanding of the research question. Integration can involve either a concurrent or sequential approach. Concurrent integration involves analyzing quantitative and qualitative data at the same time, while sequential integration involves analyzing one type of data first and then using the results to inform the analysis of the other type of data.
Triangulation
Triangulation involves using multiple sources or types of data to validate or corroborate findings. This can involve using both quantitative and qualitative data or multiple qualitative methods. Triangulation can enhance the credibility and validity of the research findings.
Mixed Methods Meta-analysis
Mixed methods meta-analysis involves the systematic review and synthesis of findings from multiple studies that use mixed methods designs. This involves combining quantitative and qualitative data from multiple studies to gain a broader understanding of a research question.
How to conduct Mixed Methods Research
Here are some general steps for conducting mixed methods research:
- Identify the research problem: The first step is to clearly define the research problem and determine if mixed methods research is appropriate for addressing it.
- Design the study: The research design should include both qualitative and quantitative data collection and analysis methods. The specific design will depend on the research question and the purpose of the study.
- Collect data : Data collection involves collecting both qualitative and quantitative data through various methods such as surveys, interviews, observations, and document analysis.
- Analyze data: Both qualitative and quantitative data need to be analyzed separately and then integrated. Analysis methods may include coding, statistical analysis, and thematic analysis.
- Interpret results: The results of the analysis should be interpreted, taking into account both the quantitative and qualitative findings. This involves integrating the results and identifying any patterns, themes, or discrepancies.
- Draw conclusions : Based on the interpretation of the results, conclusions should be drawn that address the research question and objectives.
- Report findings: Finally, the findings should be reported in a clear and concise manner, using both quantitative and qualitative data to support the conclusions.
Applications of Mixed Methods Research
Mixed methods research can be applied to a wide range of research fields and topics, including:
- Education : Mixed methods research can be used to evaluate educational programs, assess the effectiveness of teaching methods, and investigate student learning experiences.
- Health and social sciences: Mixed methods research can be used to study health interventions, understand the experiences of patients and their families, and assess the effectiveness of social programs.
- Business and management: Mixed methods research can be used to investigate customer satisfaction, assess the impact of marketing campaigns, and analyze the effectiveness of management strategies.
- Psychology : Mixed methods research can be used to explore the experiences and perspectives of individuals with mental health issues, investigate the impact of psychological interventions, and assess the effectiveness of therapy.
- Sociology : Mixed methods research can be used to study social phenomena, investigate the experiences and perspectives of marginalized groups, and assess the impact of social policies.
- Environmental studies: Mixed methods research can be used to assess the impact of environmental policies, investigate public perceptions of environmental issues, and analyze the effectiveness of conservation strategies.
Examples of Mixed Methods Research
Here are some examples of Mixed-Methods research:
- Evaluating a school-based mental health program: A researcher might use a concurrent embedded design to evaluate a school-based mental health program. The researcher might collect quantitative data through surveys and qualitative data through interviews with students and teachers. The quantitative data might be analyzed using statistical methods, while the qualitative data might be analyzed using thematic analysis. The results of the two types of data analysis could be integrated to provide a comprehensive evaluation of the program’s effectiveness.
- Understanding patient experiences of chronic illness: A researcher might use a sequential explanatory design to investigate patient experiences of chronic illness. The researcher might collect quantitative data through surveys and then use the results of the survey to inform the selection of participants for qualitative interviews. The qualitative data might be analyzed using content analysis to identify common themes in the patients’ experiences.
- Assessing the impact of a new public transportation system : A researcher might use a concurrent triangulation design to assess the impact of a new public transportation system. The researcher might collect quantitative data through surveys and qualitative data through focus groups with community members. The results of the two types of data analysis could be triangulated to provide a more comprehensive understanding of the impact of the new transportation system on the community.
- Exploring teacher perceptions of technology integration in the classroom: A researcher might use a sequential exploratory design to investigate teacher perceptions of technology integration in the classroom. The researcher might collect qualitative data through in-depth interviews with teachers and then use the results of the interviews to develop a survey. The quantitative data might be analyzed using descriptive statistics to identify trends in teacher perceptions.
When to use Mixed Methods Research
Mixed methods research is typically used when a research question cannot be fully answered by using only quantitative or qualitative methods. Here are some common situations where mixed methods research is appropriate:
- When the research question requires a more comprehensive understanding than can be achieved by using only quantitative or qualitative methods.
- When the research question requires both an exploration of individuals’ experiences, perspectives, and attitudes, as well as the measurement of objective outcomes and variables.
- When the research question requires the examination of a phenomenon in its natural setting and context, which can be achieved by collecting rich qualitative data, as well as the generalization of findings to a larger population, which can be achieved through the use of quantitative methods.
- When the research question requires the integration of different types of data or perspectives, such as combining data collected from participants with data collected from stakeholders or experts.
- When the research question requires the validation of findings obtained through one method by using another method.
- When the research question involves studying a complex phenomenon that cannot be understood by using only one method, such as studying the impact of a policy on a community’s well-being.
- When the research question involves studying a topic that has not been well-researched, and using mixed methods can help provide a more comprehensive understanding of the topic.
Purpose of Mixed Methods Research
The purpose of mixed methods research is to provide a more comprehensive understanding of a research problem than can be obtained through either quantitative or qualitative methods alone.
Mixed methods research is particularly useful when the research problem is complex and requires a deep understanding of the context and subjective experiences of participants, as well as the ability to generalize findings to a larger population. By combining both qualitative and quantitative methods, researchers can obtain a more complete picture of the research problem and its underlying mechanisms, as well as test hypotheses and identify patterns that may not be apparent with only one method.
Overall, mixed methods research aims to provide a more holistic and nuanced understanding of the research problem, allowing researchers to draw more valid and reliable conclusions, make more informed decisions, and develop more effective interventions and policies.
Advantages of Mixed Methods Research
Mixed methods research offers several advantages over using only qualitative or quantitative research methods. Here are some of the main advantages of mixed methods research:
- Comprehensive understanding: Mixed methods research provides a more comprehensive understanding of the research problem by combining both qualitative and quantitative data, which allows for a more nuanced interpretation of the data.
- Triangulation : Mixed methods research allows for triangulation, which is the use of multiple sources of data to verify findings. This improves the validity and reliability of the research.
- Addressing limitations: Mixed methods research can address the limitations of qualitative or quantitative research by compensating for the weaknesses of each method.
- Flexibility : Mixed methods research is flexible, allowing researchers to adapt the research design and methods as needed to best address the research question.
- Validity : Mixed methods research can increase the validity of the research by using multiple methods to measure the same concept.
- Generalizability : Mixed methods research can improve the generalizability of the findings by using quantitative data to test the applicability of qualitative findings to a larger population.
- Practical applications: Mixed methods research is useful for developing practical applications, such as interventions or policies, as it provides a more comprehensive understanding of the research problem.
Limitations of Mixed Methods Research
Here are some of the main limitations of mixed methods research:
- Time-consuming: Mixed methods research can be time-consuming and may require more resources than using only one research method.
- Complex data analysis: Integrating qualitative and quantitative data can be challenging and requires specialized skills for data analysis.
- Sampling bias: Mixed methods research can be subject to sampling bias, particularly if the sampling strategies for the qualitative and quantitative components are not aligned.
- Validity and reliability: Mixed methods research requires careful attention to the validity and reliability of both the qualitative and quantitative data, as well as the integration of the two data types.
- Difficulty in balancing the two methods: Mixed methods research can be difficult to balance the qualitative and quantitative methods effectively, particularly if one method dominates the other.
- Theoretical and philosophical issues: Mixed methods research raises theoretical and philosophical questions about the compatibility of qualitative and quantitative research methods and the underlying assumptions about the nature of reality and knowledge.
About the author
Muhammad Hassan
Researcher, Academic Writer, Web developer
You may also like

Questionnaire – Definition, Types, and Examples

Case Study – Methods, Examples and Guide

Observational Research – Methods and Guide

Quantitative Research – Methods, Types and...

Qualitative Research Methods

Explanatory Research – Types, Methods, Guide
Log in using your username and password
- Search More Search for this keyword Advanced search
- Latest content
- Current issue
- BMJ Journals More You are viewing from: Google Indexer
You are here
- Online First
- Grand rounds in methodology: designing for integration in mixed methods research
- Article Text
- Article info
- Citation Tools
- Rapid Responses
- Article metrics

- http://orcid.org/0000-0002-0093-858X Timothy C Guetterman 1 ,
- http://orcid.org/0000-0002-6101-5535 Milisa Manojlovich 2
- 1 University of Michigan , Ann Arbor , Michigan , USA
- 2 School of Nursing , University of Michigan , Ann Arbor , Michigan , USA
- Correspondence to Dr Timothy C Guetterman, University of Michigan, Ann Arbor, Michigan, USA; tguetter{at}med.umich.edu
Mixed methods research is a popular approach used to understand persistent and complex problems related to quality and safety, such as reasons why interventions are not implemented as intended or explaining differential outcomes. However, the quality and rigour of mixed methods research proposals and publications often miss opportunities for integration, which is the core of mixed methods. Achieving integration remains challenging, and failing to integrate reduces the benefits of a mixed methods approach. Therefore, the purpose of this article is to guide quality and safety researchers in planning and designing a mixed methods study that facilitates integration. We highlight how meaningful integration in mixed methods research can be achieved by centring integration at the following levels: research question, design, methods, results and reporting and interpretation levels. A holistic view of integration through all these levels will enable researchers to provide better answers to complex problems and thereby contribute to improvement of safety and quality of care.
- Health services research
- Qualitative research
- Implementation science
- Evaluation methodology
Data availability statement
There are no data in this work.
https://doi.org/10.1136/bmjqs-2023-016112
Statistics from Altmetric.com
Request permissions.
If you wish to reuse any or all of this article please use the link below which will take you to the Copyright Clearance Center’s RightsLink service. You will be able to get a quick price and instant permission to reuse the content in many different ways.
WHAT IS ALREADY KNOWN ON THIS TOPIC
Mixed methods research integrates qualitative and quantitative approaches to more comprehensively address research questions. Despite its growing popularity, integration remains challenging to implement in practice.
WHAT THIS STUDY ADDS
This article bridges the levels of integration with the design stage of a mixed methods research project. It presents major decision points that span research question, design, methods, results and reporting and interpretation levels to achieve integration.
HOW THIS STUDY MIGHT AFFECT RESEARCH, PRACTICE OR POLICY
Researchers using mixed methods research to understand a quality and safety-related situation of practice should consider the fit of mixed methods research and major levels of integration in designing mixed methods research.
The situation in practice
Mixed methods study designs in the health sciences have been popular for over two decades, 1 2 yet researchers continue to miss opportunities to systematically integrate qualitative and quantitative approaches. Integration is the most important characteristic of mixed methods research and refers to the intentional combining of qualitative and quantitative data, methods, results and interpretation such that the two forms of research become interdependent to address research questions. 3 Too many studies purport to use a mixed methods design when, in reality, the design consists of multiple methods that are not integrated or sequential qualitative methods alone. Even when both quantitative and qualitative data are collected, many researchers analyse each type of data separately and fail to integrate them to yield results that are more than just the sum of each type of analysis. No single type of data can fully explain phenomena that exist within a physical environment and affect the people and their interactions within it.
For instance, consider the following example. Barcode medication administration (BCMA) uses a technology that prevents medication errors and promotes patient safety when used appropriately. The medication administration process consists of nurses first using a barcode scanner to scan a patient’s identification (ID) wristband, scanning the medication which is dispensed in a single-unit package and then administering the medication and documenting it in the electronic medical record. As with many interventions, the BCMA implementation process is important for its success but deviations from the process are common and include workarounds that can lead to medication errors and patient harm. Few studies have identified why deviations occur and the influence of the context that surrounds medication administration on these deviations. To address this gap in the literature, Mulac and colleagues conducted a convergent mixed methods study to explore how nurses use the BCMA and identify policy deviations associated with unsafe practice. 4 Researchers used structured observation (eg, a digital observation tool) to collect numerical data and quantify policy deviations. Field notes and nurse comments comprised the qualitative data. Quantitative results revealed that 140 patients were affected by task-related policy deviations during medication dispensing and 152 patients were affected by task-related policy deviations during medication administration. Qualitative results showed, for example, that nurses did not always scan the patient’s ID band prior to medication administration, creating a potential patient safety issue. Had the research team only relied on the quantitative data, results would have been limited to identifying the frequency of medication error, while the qualitative data alone highlighted problems in the process. Integration revealed additional insights by making a linkage between qualitative and quantitative results: nurses did not always scan the patient’s ID band because the medication did not have a barcode or barcode was unreadable for the scanner.
In general, quality and safety research suffers from variations on this problem of examining an issue from a single perspective, which a well-integrated mixed methods approach might solve. Healthcare delivery is complex and can be considered from multiple vantage points using a variety of methods that generate different kinds of knowledge. By using integration in a mixed methods approach, knowledge as a whole is advanced because we learn more about why patient safety and quality problems exist and what can be done to fix them. Mixed methods is a way to leverage complementary strengths of qualitative and quantitative methods, while ideally minimising overlapping weaknesses. For instance, a survey with both quantitative and open-ended qualitative items would have overlapping weaknesses inherent in the survey, such as same source bias. Five common reasons for using a mixed methods approach are in table 1 , although it is worth noting that there are as many reasons as ways to mix methods.
- View inline
Reasons for using a mixed methods approach and possible applications
Despite the growth in the use of mixed methods and the many tutorials on how to perform mixed methods studies, 5–7 there has been relatively little attention paid in quality and safety research to integrating quantitative and qualitative components. Furthermore, the existing literature on integration typically focuses on the procedures employed at a particular phase of the study, rather than taking a holistic approach that encompasses the entire research process. While helpful, the integration procedures do not occur in isolation and are most effective when considered across all phases of research from planning and designing through analysis and reporting. Although new strategies and methods of integration continue to be developed, 3 8 9 many research proposals and empirical articles reporting mixed methods results give little attention to integration, fail to describe integration strategies or omit the results of integration altogether. Researchers continue to experience challenges in achieving meaningful integration, which is essential to the methodology to yield credible results. Integration is particularly important to mixed methods research in quality and safety to develop a comprehensive picture of the key constituents involved in a particular problem. Therefore, the purpose of this article is to guide quality and safety researchers in designing mixed methods research by centring integration through levels of the design process.
Major levels of integration when designing mixed methods research
At its core, integration is synergistic, generating something more than simply the cumulative effects of qualitative or quantitative research alone. The results of quantitative and qualitative analysis yield separate findings that lead to separate conclusions or claims. 10 Integrative analysis in a mixed methods study results in a ‘meta-inference,’ defined as a conclusion that connects claims from both quantitative and qualitative analysis or results. 10 A common misconception is that integration occurs only at the level of data. Rather, integration should occur at many levels of a mixed methods study, including the philosophical level, 11 the research question level, the design level, the methods level, the results level and during interpretation and reporting. 12 Although this article does not address integration at the philosophical level, interested readers should review literature on integrating philosophical perspectives. 13–15 Being aware of these levels is essential for designing for integration in quality and safety studies. In addition, integration can inform follow-up phases, their sampling plans and data collection methods. For example, qualitative results could be used to develop or adapt a quantitative survey, as in the work of researchers who developed a tool to facilitate individualised self-management interventions for adolescents with asthma. Researchers first had teens create photo diaries then participate in semistructured interviews to develop a pool of candidate items for the tool. 16 Numerous authors have discussed concepts of rigour, quality and validity as they apply to mixed methods research, which we will not repeat here. 5 17–20
Integration at the research question level
The need for a close relationship between research design and research question is especially true for mixed methods studies. The aims or research questions drive the decision of what type of mixed methods design is most appropriate based on the intent of integration. Integration of both qualitative and quantitative research questions can serve different functional needs ( box 1 ). Importantly, designing a mixed methods research study involves writing research questions or aims that reflect the need for integration. There are three broad types of mixed methods research questions: those that focus on the method (eg, how do quantitative results contribute to our understanding of qualitative findings?), those focused on content (eg, how can barriers and facilitators to a specific intervention best be explained?) or a combination of the two. 5 Our ‘Situation in Practice’ is an example of the third type of question because the authors wanted to gain an in-depth understanding of how nurses use BCMA technology (qualitative methods) and record the number and types of BCMA policy deviations (quantitative methods). It is important to critically think about how and why various methods can integrate to address aims and research questions. For example, a trial of an intervention likely already has a strong conceptual framework for why it would or would not work. A focus group may not contribute more explanation for why the intervention was effective or not, but it might address other questions about the experiences of participants or implementation issues. When writing aims or research questions, we suggest including at least one that is mixed methods-focused and can only be addressed through integration.
Four common functions of integrating qualitative and quantitative research 47
Address the same research questions.
Address related questions for the purpose of elaboration, or questions raised by the other approach (ie, qualitative or quantitative).
Use qualitative methods to develop a quantitative measure or intervention.
Use one form of research to inform sampling of the other.
Integration at the design level
Mixed methods designs provide a map for when qualitative and quantitative data collection and analysis occur and where points of integration occur. What is most important is designing the study to address aims or research questions through integration, which can be done in a variety of ways. Onwuegbuzie and Leech have stressed that timing, sequence and priority are common factors across design types. 21 Authors have described different types of mixed methods designs that follow these principles. 5 22 Creswell and Plano Clark have advanced a parsimonious set of three core mixed methods designs: convergent, explanatory sequential and exploratory sequential. 5 Convergent designs involve collecting and analysing quantitative and qualitative data and typically merging the results together to more completely understand an issue. Explanatory sequential designs use a phased approach and begin with quantitative data collection and analysis, which is followed by a second phase where qualitative data are collected and analysed to explain quantitative results. Exploratory sequential designs are also phased but begin with a qualitative phase and use results to develop an instrument or intervention that is then tested with quantitative data collection and analysis. 5 These designs are distinguished by their intent, which also guides the sequence of qualitative and quantitative procedures and the points at which integration occur.
The core mixed method designs provide a foundation for identifying points of integration to address research questions and can be combined to create variations that would more thoroughly address unique research questions. Mixed methods researchers often intersect core designs with other approaches such as randomised controlled trials (RCT) or case studies, which are examples of complex designs. In implementation science, a mixed methods intervention design might involve implementing an RCT of an intervention to deliver self-management support to patients with cancer in three regional cancer centres, for example, and also integrating qualitative methods to understand barriers and facilitators to the implementation. 23 Understanding participant experiences within a trial can also yield insight into mechanisms that might explain how or why an intervention was or was not effective.
Implementation science questions that focus on understanding implementation factors often require integrating methods. 6 Our ‘Situation in Practice’ example, by including nurses’ comments related to medication administration, uncovered how poor implementation of the BCMA technology contributed to workflow disruptions, increased workload for nurses and possible medication errors. RCTs, case studies and other approaches can further intersect with implementation science with varying degrees of focus on assessing implementation strategies or the intervention effectiveness as determined by the research questions. 24 Implementation research may involve quantitative measures of effectiveness and implementation variables, informed by a framework such as Reach Effectiveness, Adoption, Implementation, and Maintenance (RE-AIM) 25 or the Consolidated Framework for Implementation Research (CFIR). 26 Mixing qualitative with quantitative methods can also help to unpack reasons for successful implementation or illuminate how implementation characteristics relate to outcomes and experiences of participants. For example, examining a paediatric patient safety intervention in Guatemala, researchers integrated qualitative and quantitative results, guided by the CFIR, to understand barriers and facilitators to implementation. 27 They gathered surveys about attitudes towards patient safety programme adoption and interviews with staff focused on their views of implementation early in the programme followed by focus group interviews a year later with the goal of identifying opportunities for improvement.
A challenge of designing mixed methods research is that the procedures are not necessarily fixed but may intentionally change. For example, depending on the results of an initial quantitative phase in an explanatory sequential design, researchers decide what specific sampling plans or interview guides in the qualitative phase will best understand results. This decision is an example of the integration threaded throughout a mixed methods design. Naming a design is helpful, but more important is describing the design and providing relevant citations. We recommend articulating a rationale at the outset for why a mixed methods design is needed to justify the added complexity, resources and cost. Drawing a procedural diagram is recommended because it can summarise detailed information in a small space and clarify the points of integration in the design. 28 Figure 1 displays a procedural diagram for a convergent design in a proposed mixed methods study whose purpose is to develop interventions to improve cancer patients’ medication adherence. By convention, boxes are used to represent data collection and analysis, whereas circles represent mixed methods integration and interpretation. Arrows indicate the direction and flow of activities. The amount and type of information within the boxes can vary. Although Creswell argues for simplicity, 28 we provide more information in both data collection boxes and the interpretation circle for illustrative purposes.
- Download figure
- Open in new tab
- Download powerpoint
Procedural diagram for a convergent mixed methods design.
Integration at the methods level
One of the factors that contributes to the versatility of mixed methods is the countless ways in which both types of data can be collected. Alternatively, data can be collected from a single data set but analysed both qualitatively and quantitatively. The qualitative and quantitative components need to be rigorous to ensure that the results of integration generate valid insights. For example, a mixed methods study in which the only qualitative data collected is through open-ended survey responses may be insufficiently rigorous if the response rate is low. Similarly, an inadequately powered quantitative sample could make it difficult to discriminate between high and low-performing hospitals on some aspect of patient safety (eg, hospital-acquired conditions).
Major procedures for integration at the methods level include connecting, building and merging. 12 Connecting is a procedure for using the results of one form of research to inform the sampling of the other. For example, in a study exploring causes of variation for in-hospital cardiac arrest survival, researchers stratified hospitals based on survival rates and used connecting to sample hospitals that represented high, middle and lower performers. 29 30 Another procedure, building integration, involves using the results of one form of research to inform data collection of the other. In the classic example, qualitative data gathered from interviews or focus groups on a specific topic (pain management, eg) are used to develop themes that become converted to items for a quantitative instrument that is tested in the population of interest (patients with postoperative pain). 31
Merging is another common integration procedure, discussed in the next section, that typically involves integrating results to develop meta-inferences. However, designing for merging integration necessitates attention at the methods level. Quantitative and qualitative data fall on a continuum with quantitative data tending to be more closed ended and qualitative more open ended. To design for integration, it may help to brainstorm all possible data sources that could be identified to address research questions because phenomena being studied are not inherently quantitative or qualitative. Quantitative data might consist of lab results, registry data, test results, psychological assessments and educational measures. Because of how data are generated qualitative data are open ended and come from semistructured individual interviews, focus groups, open-ended survey questions, written participant journals, field observations and photographs. Thinking in an integrative manner while planning data collection helps to prepare for integrative analysis. Matching is a preintegration procedure for planning data collection about related concepts or domains both quantitatively and qualitatively. 32 For example, in a study of quality of life during cancer diagnosis, at the design stage, researchers matched items on the European Organisation for Research and Treatment of Cancer quality of life questionnaire to questions in individual interviews to prepare for merging as an integration strategy. 33 The matching does not have to be 1:1, yet it promotes thinking systematically about how to collect quantitative and qualitative data for the purpose of integration on common domains.
Integration at the results level
Mixed methods studies involve three types of analysis—qualitative, quantitative and integrative mixed methods—to produce results. Planning the qualitative and quantitative analysis has implications for integration. If the intent is to compare qualitative and quantitative results, the analysis should yield results that are comparable on the same level of analysis. For example, statistical analysis may produce an aggregated result such as descriptive statistics for variables or domains or the results of hypothesis testing. These types of results should be compared with an aggregated or grouped qualitative result, such as a theme, rather than raw qualitative data like quotes that are unanalysed.
Merging integration is a procedure most often used for integrating at the results level. When merging, researchers might compare quantitative and qualitative results on common domains to develop meta-inferences. Alternatively, they may examine patterns such as whether themes differ by statistical differences or categorical levels of a variable (eg, good vs poor outcomes) to develop meta-inferences. For instance, Hall and colleagues merged qualitative and quantitative implementation results to identify patient centredness, staff receptivity and desire for protocols as facilitators in addition to numerous barriers to implementing a paediatrics safety programme. 27 In our ‘Situation in Practice’ the five domains from the Systems Engineering Initiative for Patient Safety model (ie, tasks, organisational factors, technology, physical environment and individuals) were used to develop meta-inferences. 34 In the environmental domain, for example, quantitative results from the observation tool revealed that the medication room was far away from the nurse station. Qualitative results from nurses’ comments revealed that nurses stored medication at their station to avoid going back and forth to the medication room. The meta-inference here was that the location of the medication room affected task efficiency and time spent administering medications.
Integration during interpretation and reporting
Integration in mixed methods research leads to more than the sum of its parts. 35 In our ‘Situation in Practice’ example, one might initially be appalled to learn of the numerous medication dispensing and administration policy deviations. Through integration, researchers develop a new interpretation of why and how such deviations occurred: patient medication drawers were too small to hold all patient medications (leading to dispensing omissions), a slow BCMA process led to nurses skipping use of the BCMA, and scanners were attached to the computer-on-wheels (COW) instead of being wireless. This meant that the COW had to be brought into a patient’s room to use the scanner but the COW’s large size made it unwieldy to move, so that nurses left the COW and attached scanner outside of a patient’s room, and administered medication without scanning the patient’s ID band.
Several tools, such as joint displays, can help quality and safety researchers develop meta-inferences (ie, conclusions that connect quantitative and qualitative claims) from the integration of results. Joint displays are a visual means of integrating quantitative and qualitative data, methods or results through matrices and figures 9 36 and are a way to help researchers develop meta-inferences. Joint displays are used to represent the integration procedures in addition to the results of integration and meta-inferences where applicable. Software programs, such as Dedoose, HyperResearch, MAXQDA, NVivo and QDAMiner, can be helpful in developing joint displays.
The construction of a joint display encourages researchers to interrogate the entirety of results systematically in order to identify meta-inferences. Two popular types of joint displays of results are side-by-side joint displays that juxtapose quantitative and qualitative results on common domains along with resultant meta-inferences and themes-by-statistics joint displays that array qualitative themes by quantitative statistics similar to a crosstab.
For example, Hall et al 27 examined implementation of safety programmes at a hospital in Guatemala by comparing CFIR constructs from qualitative and quantitative data sets. Using a side-by-side joint display, researchers first conducted qualitative (ie, semistructured interviews and focus groups) and quantitative (ie, Evidence-Based Practice Attitude Scale) analysis and summarised the results in the cells, as in table 2 , an adapted joint display derived from Hall. 27 The next step was to compare quantitative and qualitative results on a similar domain, which was based on CFIR. This process facilitated systematically comparing quantitative and qualitative results on each domain and led to new insights into their integration.
An example side-by-side joint display to develop meta-inferences
A themes-by-statistics joint display ( table 3 ) was used to array medical students’ communication performance in a simulation measured by an objective-structured clinical examination (OSCE) by themes of those participating in the simulation. 37 In this example, researchers also begin with qualitative and quantitative analysis, but continue by examining relationships, such as how themes differ based on levels of performance on the OSCE. The cells of the joint display could contain themes, subthemes, codes and illustrative quotes in addition to statistics. In table 3 , the rows are themes of participant experiences in the communication simulation and columns represent quantitative levels of performance based on an OSCE score. The cells contain exemplar quotes for each theme to facilitate comparison by performance level. This cross-tabulation of results lends well to examining how a theme or category may differ across quantitative levels. In addition, it may be helpful to focus on a quantitative level and look across themes to generate meta-inferences.
A joint display of qualitative themes by quantitative performance level 37
In another example, to understand variation in nursing roles for in-hospital cardiac arrest response among hospitals with higher versus lower survival rates, researchers developed a themes-by-statistics joint display. 30 Using the MAXQDA segment matrix (formerly Interactive Quote Matrix), 38 researchers reviewed snippets of coded text for each major theme to examine whether there was variation between higher compared with lower performing hospitals. Although we have discussed side-by-side and statistics-by-themes joint displays, numerous other types appear in published studies. 9 36 39–41
Reporting integration is one of the most important aspects of writing mixed methods research proposals or publications. Keeping this end product in mind is essential when designing for integration. Reporting is achieved through written results sections in a narrative form of weaving 12 together quantitative and qualitative results along with meta-inferences and through joint displays. Meta-inferences often appear within the text of the results section of a manuscript or as the final column or row of a joint display. Writing mixed methods manuscripts can be very complicated but providing greater detail about the reporting process is beyond the scope of this paper and is discussed in other publications. 32 42 43
Mixed methods research studies have the potential to provide more insight and a more comprehensive understanding of many complex phenomena that quality and safety science addresses. Considering integration as an afterthought at the end of a mixed methods study fails to capitalise on its strengths and limits its impact. Advances in patient safety may depend on answers to research questions that incorporate integration at multiple levels into mixed methods approaches. A holistic view of integration across levels of the research design process helps to take full advantage of the strengths of mixed methods. We encourage quality and safety researchers to continue to innovate methods, create new integration procedures and consider integration holistically.
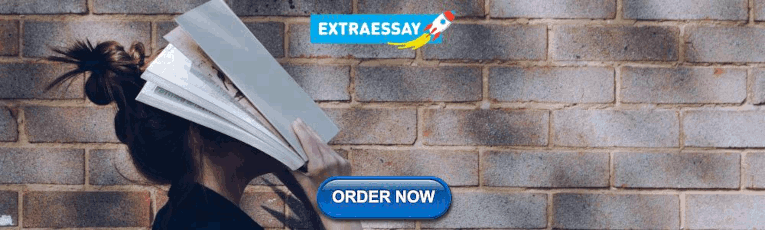
Ethics statements
Patient consent for publication.
Not applicable.
Ethics approval
Acknowledgments.
We are very grateful for comments on earlier versions of the manuscript by reviewers and editors in addition to two of our colleagues, Dr. Melissa DeJonckheere and Dr. Sergi Fàbregues. Their thoughtful and critical feedback was very helpful in shaping this manuscript.
- Schulman-Green D ,
- Feder S , et al
- Plano Clark VL
- Mathiesen L ,
- Taxis K , et al
- Creswell JW ,
- Palinkas LA ,
- Aarons GA ,
- Horwitz S , et al
- Johnson RE ,
- Guetterman TC ,
- Fàbregues S ,
- Sakakibara R
- Hitchcock JH ,
- Onwuegbuzie AJ
- Fetters MD ,
- Molina-Azorin JF
- Creswell JW
- Shannon-Baker P
- Byczkowski TL ,
- Vockell A-LB ,
- Krier DJ , et al
- Onwuegbuzie AJ ,
- Johnson RB ,
- Collins KMT
- O’Cathain A
- Howell Smith MC ,
- Babchuk WA , et al
- Molina-Azorín JF
- Teddlie C ,
- Tashakkori A
- Kirkby R , et al
- Curran GM ,
- Mittman B , et al
- Glasgow RE ,
- Harden SM ,
- Gaglio B , et al
- Damschroder LJ ,
- Reardon CM ,
- Widerquist MAO , et al
- Aguilar A , et al
- Nallamothu BK ,
- Harrod M , et al
- Kellenberg JE ,
- Krein SL , et al
- Towsley GL ,
- Berry PH , et al
- Moseholm E ,
- Rydahl-Hansen S ,
- Lindhardt BØ , et al
- Holden RJ ,
- Carayon P ,
- Gurses AP , et al
- Freshwater D
- Sakakibara R ,
- Baireddy S , et al
- Plano Clark VL ,
- Guetterman TC
- Pedersen M ,
- Dahlberg B ,
- Wittink MN ,
- Lockhart S ,
- Reed K , et al
- Manojlovich M ,
- Forman J , et al
- Bettencourt AP ,
- Mangus CW , et al
- Horwitz SM ,
- Chamberlain P , et al
Twitter @tc_g, @mmanojlo
Contributors Both authors conceived this study. TG wrote the first draft and revision and is guarantor. Both authors critically reviewed the manuscript and approved the final version.
Funding The authors have not declared a specific grant for this research from any funding agency in the public, commercial or not-for-profit sectors.
Competing interests None declared.
Provenance and peer review Commissioned; externally peer-reviewed.
Read the full text or download the PDF:

Developing Metadiscursive Thinking About Research Methods Through a Mixed-Methods Research Project
- Ellen M. Rigsby Saint Mary's College of California Author
- Makiko Imamura Saint Mary's College of California Author
This study provides an overview of the shifting epistemological terrain regarding methodological approaches to the research process in the field of communication and discusses how a mixed-methods design could improve methods comprehension in undergraduate education. The authors describe how they incorporated a mixed-methods project into the curriculum and discuss the student experience of learning qualitative and quantitative methods through the mixed-methods project as evidenced by surveys and reflective writing assignments. Specifically, the project reorients students' thinking from qualitative and quantitative methods as separate paradigms. Instead, it fosters a metadiscursive perspective on methods in which research designs and questions are in conversation with each other (Creswell, 2011). The project is structured using the Dimensions of Research in Undergraduate Learning framework (DRUL; Healey & Jenkins, 2009; Ozay, 2012) to foster this conversation by creating an explicit path for the transfer of knowledge between specific methods.
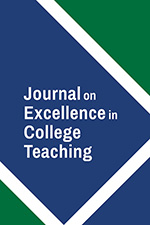
- Requires Subscription PDF
Access Agreement Journal on Excellence in College Teaching
Before proceeding you must agree to the terms and conditions of usage as outlined below by clicking on the Accept button and/or by both parties’ signatures below. You will have to do this only once. After agreement, you will be redirected back to the main Journal page. A pdf copy of the terms is available for download.
This Access Agreement (the "Agreement") is effective upon processing of payment ("Effective Date") and is entered into by and between the Journal on Excellence in College Teaching (“JECT”) and the Customer (“Customer").
This Agreement constitutes the entire agreement and supersedes and voids all prior communications, understandings, and agreements relating to the Product(s), including any terms of use displayed to Authorized Users via the online site of the Product(s). Alterations to the Agreement and to any Addendum to the Agreement are only valid and binding if they are recorded in writing and signed by both parties
. I. Definitions
"Authorized Users" shall mean individuals who are authorized by the Customer (which shall include those individuals authorized by the Institutions hereunder) to access the Customer's information services whether on-site or off-site via secure authentication and who are affiliated with the Customer as a student (undergraduates and postgraduates), employee (whether on a permanent or temporary basis), or a contractor of the Customer. Individuals who are not a current student, employee, or a contractor of the Customer, but who are permitted to access the Customer's information services from computer terminals within the physical premises of the Customer ("Walk-In Users"), are also deemed to be Authorized Users, but only for the time they are within the physical premises of the Customer. Walk-In Users may not be given means to access the Product(s) when they are not within the physical premises of the Customer.
"Commercial Use" shall mean use for the purpose of monetary reward (whether by or for the Customer or an Authorized User) by means of the sale, resale, loan, transfer, hire, or other form of exploitation of the Product(s). For the avoidance of doubt, neither recovery of direct cost by the Customer from Authorized Users, nor use by the Customer or Authorized Users of the Product(s) in the course of research funded by a commercial organization, shall be deemed to constitute Commercial Use.
"Educational Purposes" shall mean for the purpose of education, teaching, distance learning, private study and/or research as described in Section II below.
"Institutions" shall mean the Customer's participating institutions, if applicable.
"License" shall mean the non-exclusive, non-transferable right to access and use the Product(s) pursuant to the specific terms and conditions set forth in this Agreement.
"Product(s)" shall mean the products, materials and/or information contained therein that are subject to this Agreement. Product(s) include the Journal on Excellence in College Teaching and the archive of the Learning Communities Journal.
"Reasonable Amount" shall be determined based on guidelines set forth by 17 U.S. Code § 107 (Limitations on exclusive rights, Fair use).
"Secure Authentication" shall mean access to the Product(s) by Internet Protocol ("IP") ranges or by another means of authentication agreed between the Publisher and Customer or Institutions (if applicable) from time to time.
II. Authorized Use of Product(s)
Customer, the Institutions (if applicable), and Authorized Users may use the Product(s) for Educational Purposes as follows:
Analysis . Authorized Users shall be permitted to extract or use information contained in the Product(s) for Educational Purposes, including, but not limited to, text and data mining, extraction and manipulation of information for the purposes of illustration, explanation, example, comment, criticism, teaching, research, or analysis.
Course Packs . Customer, the Institutions, and Authorized Users may use a Reasonable Amount of the Product(s) in the preparation of course packs or other educational materials.
Digital Copy . Customer, the Institutions, and Authorized Users may download and digitally copy a Reasonable Amount of the Product(s).
Display . Customer, the Institutions, and Authorized Users shall have the right to electronically display the Product(s) to the extent necessary to further the intent and purpose of this Agreement.
Electronic Reserve . Customer, the Institutions, and Authorized Users may use a Reasonable Amount of each of the Product(s) in connection with specific courses of instruction offered by Customer.
Interlibrary Loan . The Customer and the Institutions shall be permitted to use Reasonable Amounts of the Content to fulfill occasional requests from other, non-participating institutions, a practice commonly called Interlibrary Loan ("ILL"). Customer and the Institutions shall fulfill such requests in compliance with Section 108 of the United States Copyright Law (17 USC S108, "Limitations on exclusive rights: Reproduction by libraries and archives") and the Guidelines for the Proviso of Subsection 108(2g)(2) prepared by the National Commission on New Technological Uses of Copyrighted Works (CONTU).
The electronic form of the Product(s) may be used as a source for ILL. Customer and the Institutions shall include copyright notices on all ILL transmissions. Notwithstanding anything herein to the contrary, in no event shall any non-secure electronic transmission of files be permitted.
Print Copy . Customer, the Institutions, and Authorized Users may print a Reasonable Amount of the Product(s).
Recover Copying Costs . Customer and the Institutions may charge a reasonable fee to cover costs of copying or printing portions of Product(s) for Authorized Users.
Scholarly Sharing . Authorized Users may transmit to a third party colleague in hard copy or electronically, Reasonable Amounts of the Product(s) for personal use, professional use, or Educational Purposes but in no event for Commercial Use. In addition, Authorized Users have the right to use, with appropriate credit, figures, tables, and brief excerpts from the Product(s) in the Authorized User's own scientific, scholarly, and educational works.
Text Mining . Authorized Users may use the licensed material to perform and engage in text mining/data mining activities for legitimate academic research and other Educational Purposes. Those uses beyond educational use shall require permission from the Publisher.
III. Restrictions
Except as provided herein, the institution shall make reasonable efforts to inform its authorized users not to use, alter, decompile, modify, display, or distribute the Product(s) as follows:
Alter Identification . Remove, obscure, or modify copyright notices, text acknowledging, attributions, or other means of identification or disclaimers as they appear. Alter Product(s).
Alter, decompile, adapt, or modify the Product(s), except to the extent necessary to make it perceptible on a computer screen, or as otherwise permitted in this Agreement. Alteration of words or their order is strictly prohibited.
Commercial Use . No Commercial Use of the Product(s) shall be permitted unless the Customer or an Authorized User has been granted prior written consent by an authorized representative of the Product(s). Use of all or any part of the Product(s) for any Commercial Use or for any purpose other than Educational Purposes.
Distribution . Display or distribute any part of the Product(s) on any electronic network, including without limitation, the Internet, and any other distribution medium now in existence or hereinafter created, other than by a Secure Authentication; print and distribute any portion(s) of the Product(s)s to persons or entities other than the Customer or Authorized Users, except as provided in Section II.
JECT acknowledges that the Customer cannot police or control the actions of its students, faculty, and other Authorized Users with respect to their use of the Product(s). In the event of abuse, the institution shall make prompt and reasonable efforts to heal the breach and notify the publisher.
IV. Term and Termination
This agreement shall commence on the Effective Date and shall remain in effect unless and until terminated as permitted herein (the "Term"). There is no perpetual electronic access to content made available during the term of the agreement.
JECT may terminate this Agreement if Customer violates any of the terms and conditions set forth herein. In the event of any termination of access, JECT will promptly notify the Customer of the basis for termination.
The Customer may terminate this Agreement if sufficient funds are not provided or allotted in future government-approved budgets of the Customer (or reasonably available or expected to become available from other sources at the time the Customer’s payment obligation attaches) to permit the Subscriber, in the exercise of its reasonable administrative discretion, to continue this Agreement.
In the event of any unauthorized use of the Product(s) by an Authorized User, Customer shall cooperate with JECT in the investigation of any unauthorized use of the Product(s) of which it is made aware and shall use reasonable efforts to remedy such unauthorized use and prevent its recurrence. JECT may terminate such Authorized User's access to the Product(s) after first providing reasonable notice to the Customer (in no event less than two (2) weeks) and cooperating with the Customer to avoid recurrence of any unauthorized use. In the event of any termination of access, JECT will promptly notify the Customer
. V. Refunds
In the event that a subscription is canceled by the Customer prior to the subscription end date, the following will be used as guidelines for refunds.
Electronic subscriptions . The Customer shall be entitled to a full refund within 14 days of the start of the most recent subscription term. Refunds requested after 14 days but no later than 60 days from the start of the most recent subscription term will be allowed, minus a 30% processing fee. Refunds will not be granted if requested more than 60 days after the start of the most recent subscription term.
Journal on Excellence in College Teaching Center for Teaching Excellence Miami University 317 Laws Hall Oxford, Ohio 45056
All rights reserved | Miami University | The Journal on Excellence in College Teaching
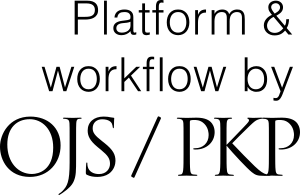
This paper is in the following e-collection/theme issue:
Published on 11.4.2024 in Vol 26 (2024)
Evaluating the Digital Health Experience for Patients in Primary Care: Mixed Methods Study
Authors of this article:

Original Paper
- Melinda Ada Choy 1, 2 , BMed, MMed, DCH, MD ;
- Kathleen O'Brien 1 , BSc, GDipStats, MBBS, DCH ;
- Katelyn Barnes 1, 2 , BAPSC, MND, PhD ;
- Elizabeth Ann Sturgiss 3 , BMed, MPH, MForensMed, PhD ;
- Elizabeth Rieger 1 , BA, MClinPsych, PhD ;
- Kirsty Douglas 1, 2 , MBBS, DipRACOG, Grad Cert HE, MD
1 School of Medicine and Psychology, College of Health and Medicine, The Australian National University, Canberra, Australia
2 Academic Unit of General Practice, Office of Professional Leadership and Education, ACT Health Directorate, Canberra, Australia
3 School of Primary and Allied Health Care, Monash University, Melbourne, Australia
Corresponding Author:
Melinda Ada Choy, BMed, MMed, DCH, MD
School of Medicine and Psychology
College of Health and Medicine
The Australian National University
Phone: 61 51244947
Email: [email protected]
Background: The digital health divide for socioeconomic disadvantage describes a pattern in which patients considered socioeconomically disadvantaged, who are already marginalized through reduced access to face-to-face health care, are additionally hindered through less access to patient-initiated digital health. A comprehensive understanding of how patients with socioeconomic disadvantage access and experience digital health is essential for improving the digital health divide. Primary care patients, especially those with chronic disease, have experience of the stages of initial help seeking and self-management of their health, which renders them a key demographic for research on patient-initiated digital health access.
Objective: This study aims to provide comprehensive primary mixed methods data on the patient experience of barriers to digital health access, with a focus on the digital health divide.
Methods: We applied an exploratory mixed methods design to ensure that our survey was primarily shaped by the experiences of our interviewees. First, we qualitatively explored the experience of digital health for 19 patients with socioeconomic disadvantage and chronic disease and second, we quantitatively measured some of these findings by designing and administering a survey to 487 Australian general practice patients from 24 general practices.
Results: In our qualitative first phase, the key barriers found to accessing digital health included (1) strong patient preference for human-based health services; (2) low trust in digital health services; (3) high financial costs of necessary tools, maintenance, and repairs; (4) poor publicly available internet access options; (5) reduced capacity to engage due to increased life pressures; and (6) low self-efficacy and confidence in using digital health. In our quantitative second phase, 31% (151/487) of the survey participants were found to have never used a form of digital health, while 10.7% (52/487) were low- to medium-frequency users and 48.5% (236/487) were high-frequency users. High-frequency users were more likely to be interested in digital health and had higher self-efficacy. Low-frequency users were more likely to report difficulty affording the financial costs needed for digital access.
Conclusions: While general digital interest, financial cost, and digital health literacy and empowerment are clear factors in digital health access in a broad primary care population, the digital health divide is also facilitated in part by a stepped series of complex and cumulative barriers. Genuinely improving digital health access for 1 cohort or even 1 person requires a series of multiple different interventions tailored to specific sequential barriers. Within primary care, patient-centered care that continues to recognize the complex individual needs of, and barriers facing, each patient should be part of addressing the digital health divide.
Introduction
The promise of ehealth.
The rapid growth of digital health, sped up by the COVID-19 pandemic and associated lockdowns, brings the promise of improved health care efficiency, empowerment of consumers, and health care equity [ 1 ]. Digital health is the use of information and communication technology to improve health [ 2 ]. eHealth, which is a type of digital health, refers to the use of internet-based technology for health care and can be used by systems, providers, and patients [ 2 ]. At the time of this study (before COVID-19), examples of eHealth used by patients in Australia included searching for web-based health information, booking appointments on the web, participating in online peer-support health forums, using mobile phone health apps (mobile health), emailing health care providers, and patient portals for electronic health records.
Digital health is expected to improve chronic disease management and has already shown great potential in improving chronic disease health outcomes [ 3 , 4 ]. Just under half of the Australian population (47.3%) has at least 1 chronic disease [ 5 ]. Rates of chronic disease and complications from chronic disease are overrepresented among those with socioeconomic disadvantage [ 6 ]. Therefore, patients with chronic disease and socioeconomic disadvantage have a greater need for the potential benefits of digital health, such as an improvement in their health outcomes. However, there is a risk that those who could benefit most from digital health services are the least likely to receive them, exemplifying the inverse care law in the digital age by Hart [ 7 ].
Our Current Understanding of the Digital Health Divide
While the rapid growth of digital health brings the promise of health care equity, it may also intensify existing inequities [ 8 ]. The digital health divide for socioeconomic disadvantage describes a pattern in which patients considered socioeconomically disadvantaged who are already marginalized through poor access to traditional health care are additionally hindered through poor access to digital health [ 9 ]. In Australia, only 67.4% of households in the lowest household income quintile have home internet access, compared to 86% of the general population and 96.9% of households in the highest household income quintile [ 10 ]. Survey-based studies have also shown that even with internet access, effective eHealth use is lower in populations considered disadvantaged, which speaks to broader barriers to digital health access [ 11 ].
The ongoing COVID-19 global pandemic has sped up digital health transitions with the rapid uptake of telephone and video consultations, e-prescription, and the ongoing rollout of e-mental health in Australia. These have supported the continuation of health care delivery while limiting physical contact and the pandemic spread; however, the early evidence shows that the digital health divide remains problematic. A rapid review identified challenges with reduced digital access and digital literacy among the older adults and racial and ethnic minority groups, which are both groups at greater health risk from COVID-19 infections [ 12 ]. An Australian population study showed that the rapid uptake of telehealth during peak pandemic was not uniform, with the older adults, very young, and those with limited English language proficiency having a lower uptake of general practitioner (GP) telehealth services [ 13 ].
To ensure that digital health improves health care outcome gaps, it is essential to better understand the nature and nuance of the digital health divide for socioeconomic disadvantage. The nature of the digital health divide for socioeconomic disadvantage has been explored primarily through quantitative survey data, some qualitative papers, a few mixed methods papers, and systematic reviews [ 11 , 14 - 16 ]. Identified barriers include a lack of physical hardware and adequate internet bandwidth, a reduced inclination to seek out digital health, and a low ability and confidence to use digital health effectively [ 16 ]. The few mixed methods studies that exist on the digital health divide generally triangulate quantitative and qualitative data on a specific disease type or population subgroup to draw a combined conclusion [ 17 , 18 ]. These studies have found digital health access to be associated with education, ethnicity, and gender as well as trust, complementary face-to-face services, and the desire for alternative sources of information [ 17 , 19 ].
What This Work Adds
This project sought to extend previous research by using an exploratory mixed methods design to ensure that the first step and driver of our survey of a larger population was primarily shaped by the experiences of our interviewees within primary care. This differs from the triangulation method, which places the qualitative and quantitative data as equal first contributors to the findings and does not allow one type of data to determine the direction of the other [ 18 ]. We qualitatively explored the experience of digital health for patients with socioeconomic disadvantage and chronic disease and then quantitatively measured some of the qualitative findings via a survey of the Australian general practice patient population. Our key objective was to provide comprehensive primary mixed methods data, describing the experience and extent of barriers to accessing digital health and its benefits, with a focus on the digital health divide. We completed this research in a primary care context to investigate a diverse community-based population with conceivable reasons to seek digital help in managing their health. Findings from this mixed methods study were intended to provide health care providers and policy makers with a more detailed understanding of how specific barriers affect different aspects or steps of accessing digital health. Ultimately, understanding digital health access can influence the future design and implementation of digital health services by more effectively avoiding certain barriers or building in enablers to achieve improved digital health access not only for everyone but also especially for those in need.
Study Design
We conducted a sequential exploratory mixed methods study to explore a complex phenomenon in depth and then measure its prevalence. We qualitatively explored the experience of digital health for patients with chronic disease and socioeconomic disadvantage in the first phase. Data from the first phase informed a quantitative survey of the phenomenon across a wider population in the second phase [ 18 ]. Both stages of research were conducted before the COVID-19 pandemic in Australia.
Recruitment
Qualitative phase participants.
The eligibility criteria for the qualitative phase were as follows: English-speaking adults aged ≥18 years with at least 1 self-reported chronic disease and 1 marker of socioeconomic disadvantage (indicated by ownership of a Health Care Card or receiving a disability pension, unemployment, or a user of public housing). A chronic disease was defined to potential participants as a diagnosed long-term health condition that had lasted at least 6 months (or is expected to last for at least 6 months; examples are listed in Multimedia Appendix 1 ). The markers of socioeconomic disadvantage we used to identify potential participants were based on criteria typically used by local general practices to determine which patients can have lower or no out-of-pocket expenses. Apart from unemployment, the 3 other criteria to identify socioeconomic disadvantage are means-tested government-allocated public social services [ 20 ]. Qualitative phase participants were recruited from May to July 2019 through 3 general practices and 1 service organization that serve populations considered socioeconomically disadvantaged across urban, regional, and rural regions in the Australian Capital Territory and South Eastern New South Wales. A total of 2 recruitment methods were used in consultation with and as per the choice of the participating organizations. Potential participants were either provided with an opportunity to engage with researchers (KB and MAC) in the general practice waiting room or identified by the practice or organization as suitable for an interview. Interested participants were given a detailed verbal and written description of the project in a private space before providing written consent to be interviewed. All interview participants received an Aus $50 (US $32.68) grocery shopping voucher in acknowledgment of their time.
Quantitative Phase Participants
Eligibility for the quantitative phase was English-speaking adults aged ≥18 years. The eligibility criteria for the quantitative phase were deliberately broader than those for the qualitative phase to achieve a larger sample size within the limitations of recruitment and with the intention that the factors of socioeconomic disadvantage and having a chronic disease could be compared to the digital health access of a more general population. The quantitative phase participants were recruited from November 2019 to February 2020. Study information and paper-based surveys were distributed and collected through 24 general practices across the Australian Capital Territory and South Eastern New South Wales regions, with an option for web-based completion.
Ethical Considerations
Qualitative and quantitative phase research protocols, including the participant information sheet, were approved by the Australian Capital Territory Health Human Research Ethics Committee (2019/ETH/00013) and the Australian National University Human Research Ethics Committee (2019/ETH00003). Qualitative phase participants were given a verbal and written explanation of the study, including how and when they could opt out, before they provided written consent. All interview participants received an Aus $50 (US $32.68) grocery shopping voucher in acknowledgment of their time. Quantitative participants were given a written explanation and their informed consent was implied by return of a completed survey. Participants in both phases of the study were told that all their data was deidentified. Consent was implied through the return of a completed survey.
Qualitative Data Collection and Analysis
Participants were purposively sampled to represent a range in age, gender, degree of socioeconomic disadvantage, and experience of digital health. The sampling and sample size were reviewed regularly by the research team as the interviews were being completed to identify potential thematic saturation.
The interview guide was developed by the research team based on a review of the literature and the patient dimensions of the framework of access by Levesque et al [ 21 ]. The framework by Levesque et al [ 21 ] is a conceptualization of health care access comprising 5 service and patient dimensions of accessibility and ability. The patient dimensions are as follows: (1) ability to perceive, (2) ability to seek, (3) ability to reach, (4) ability to pay, and (5) ability to engage [ 21 ]. The key interview topics included (1) digital health use and access, including facilitators and barriers; (2) attitudes toward digital health; and (3) self-perception of digital health skills and potential training. The interview guide was reviewed for face and content validity by the whole research team, a patient advocate, a digital inclusion charity representative, and the general practices where recruitment occurred. The questions and guide were iteratively refined by the research team to ensure relevance and support reaching data saturation. The interview guide has been provided as Multimedia Appendix 1 . The interviews, which took 45 minutes on average, were taped and transcribed. An interview summary sheet and reflective journal were completed by the interviewer after each interview to also capture nonverbal cues and tone.
Interview transcriptions were coded and processed by inductive thematic analysis. Data collection and analysis were completed in parallel to support the identification of data saturation. Data saturation was defined as no significant new information arising from new interviews and was identified by discussion with the research team [ 22 ]. The 2 interviewers (MAC and KB) independently coded the first 5 transcripts and reflected on them with another researcher (EAS) to ensure intercoder validity and reliability. The rest of the interviews were coded independently by the 2 interviewers, who regularly met to reflect on emerging themes and thematic saturation. Data saturation was initially indicated after 15 interviews and subsequently confirmed with a total of 19 interviews. Coding disagreements and theme development were discussed with at least 1 other researcher (EAS, ER, and KD). Thematic saturation and the final themes were agreed upon by the entire research team.
Quantitative Survey Development
The final themes derived in the qualitative phase of the project guided the specific quantitative phase research questions. The final themes were a list of ordered cumulative barriers experienced by participants in accessing digital health and its benefits ( Figure 1 ). The quantitative survey was designed to test the association between barriers to access and the frequency of use of digital health as a proxy measure for digital health access.

In the survey, the participants were asked about their demographic details, health and chronic diseases, knowledge, use and experience of digital health tools, internet access, perception of digital resource affordability, trust in digital health and traditional health services, perceived capability, health care empowerment, eHealth literacy, and relationship with their GP.
Existing scales and questions from the literature and standardized Australian-based surveys were used whenever possible. We used selected questions and scales from the Australian Bureau of Statistics standards, the eHealth Literacy Scale (eHEALS), the eHealth Literacy Questionnaire, and the Southgate Institute for Health Society and Equity [ 17 , 23 - 26 ]. We adapted other scales from the ICEpop Capability Measure for Adults, the Health Care Empowerment Inventory (HCEI), the Patient-Doctor Relationship Questionnaire, and the Chao continuity questionnaire [ 23 , 27 - 29 ]. Where an existing scale to measure a barrier or theme did not exist, the research team designed the questions based on the literature. Our questions around the frequency of digital health use were informed by multiple existing Australian-based surveys on general technology use [ 30 , 31 ]. Most of the questions used a Likert scale. Every choice regarding the design, adaptation, or copy of questions for the survey was influenced by the qualitative findings and decided on by full agreement among the 2 researchers who completed and coded the interviews. A complete copy of the survey is provided in Multimedia Appendix 2 .
Pilot-testing of the survey was completed with 5 patients, 2 experts on digital inclusion, and 3 local GPs for both the paper surveys and web-based surveys via Qualtrics Core XM (Qualtrics LLC). The resulting feedback on face and content validity, functionality of the survey logic, and feasibility of questionnaire completion was incorporated into the final version of the survey.
The survey was offered on paper with a participant information sheet, which gave the patients the option to complete the web-based survey. The survey was handed out to every patient on paper to avoid sampling bias through the exclusion of participants who could not complete the web-based survey [ 32 ].
Quantitative Data Treatment and Analysis
Data were exported from Qualtrics Core XM to an SPSS (version 26; IBM Corp) data set. Data cleaning and screening were undertaken (KB and KO).
Descriptive statistics (number and percentage) were used to summarize participant characteristics, preference measures, and frequency of eHealth use. Significance testing was conducted using chi-square tests, with a threshold of P <.05; effect sizes were measured by the φ coefficient for 2×2 comparisons and Cramer V statistic for all others. Where the cells sizes were too small, the categories were collapsed for the purposes of significance testing. The interpretation of effect sizes was as per the study by Cohen [ 33 ]. The analysis was conducted in SPSS and SAS (version 9.4; SAS Institute).
Participant Characteristics
Participants’ self-reported characteristics included gender, indigenous status, income category, highest level of education, marital status, and language spoken at home.
Age was derived from participant-reported year of birth and year of survey completion as of 2019 and stratified into age groups. The state or territory of residence was derived from the participant-reported postcode. The remoteness area was derived using the postcode reported by the participants and mapped to a modified concordance from the Australian Bureau of Statistics. Occupation-free text responses were coded using the Australian Bureau of Statistics Census statistics level 1 and 2 descriptors. The country of birth was mapped to Australia, other Organisation for Economic Cooperation and Development countries, and non–Organisation for Economic Cooperation and Development countries.
Frequency of eHealth Use
A summary measure of the frequency of eHealth use was derived from the questions on the use of different types of eHealth.
Specifically, respondents were asked if they had ever used any form of web-based health (“eHealth“) and, if so, to rate how often (never, at least once, every now and then, and most days) against 6 types of “eHealth” (searching for health information online, booking appointments online, emailing health care providers, using health-related mobile phone apps, accessing My Health Record, and accessing online health forums). The frequency of eHealth use was then classified as follows:
- High user: answered “most days” to at least 1 question on eHealth use OR answered “every now and then” to at least 2 questions on eHealth use
- Never user: answered “no” to having ever used any form of eHealth OR “never” to all 6 questions on eHealth use
- Low or medium user: all other respondents.
The frequency of eHealth use was reported as unweighted descriptive statistics (counts and percentages) against demographic characteristics and for the elements of each of the themes identified in phase 1.
Overview of Key Themes
Data were reported against the 6 themes from the phase 1 results of preference, trust, cost, structural access, capacity to engage, and self-efficacy. Where the components of trust, cost, capacity to engage, and self-efficacy had missing data (for less than half of the components only), mean imputation was used to minimize data loss. For each theme, the analysis excluded those for whom the frequency of eHealth use was unknown.
Preference measures (survey section D1 parts 1 to 3) asked participants to report against measures with a 4-point Likert scale (strongly disagree, disagree, agree, and strongly agree). Chi-square tests were conducted after the categories were condensed into 2 by combining strongly disagree and as well as combining strongly agree and agree.
Summary measures for trust were created in 4 domains: trust from the eHealth Literacy Questionnaire (survey section D1 parts 4 to 8), trust from Southgate—GPs, specialists, or allied health (survey section D2 parts 1 to 5), trust from Southgate—digital health (survey section D2 parts 6, 7, 9, and 10), and trust from Southgate—books or pamphlets (survey section D2 part 8). The data were grouped as low, moderate, and high trust based on the assigned scores from the component data. Chi-square tests were conducted comparing low-to-moderate trust against high trust for GP, specialists, or allied health and comparing low trust against moderate-to-high trust for book or pamphlet.
Summary measures for cost were created from survey item C10. To measure cost, participants were asked about whether they considered certain items or services to be affordable. These included cost items mentioned in the qualitative phase interviews relating to mobile phones (1 that connects to the internet, 1 with enough memory space to download apps, downloads or apps requiring payment, repairs, and maintenance costs), having an iPad or tablet with internet connectivity, a home computer or laptop (owning, repairs, and maintenance), home fixed internet access, and an adequate monthly data allowance. These 9 items were scored as “yes definitely”=1 or 0 otherwise. Chi-square tests were conducted with never and low or medium eHealth users combined.
Structural Access
Structural access included asking where the internet is used by participants (survey section C8) and factors relating to internet access (survey section C8 parts 1-3) reporting against a 4-point Likert scale (strongly disagree, disagree, agree, and strongly agree). Chi-square tests were conducted with strongly disagree, disagree, agree, or strongly agree, and never, low, or medium eHealth use combined.
Capacity to Engage
Summary measures for capacity to engage were created from survey section E1. To measure the capacity to engage, participants were asked about feeling “settled and secure,” “being independent,” and “achievement and progress” as an adaptation of the ICEpop Capability Measure for Adults [ 27 ], reporting against a 4-point Likert-like scale. Responses were scored from 1 (“I am unable to feel settled and secure in any areas of my life”) to 4 (“I am able to feel settled and secure in all areas of my life”).
The summary capacity measure was derived by the summation of responses across the 3 questions, which were classified into 4 groups, A to D, based on these scores. Where fewer than half of the responses were missing, mean imputation was used; otherwise, the record was excluded. Groups A and B were combined for significance testing.
Self-Efficacy
Summary measures for self-efficacy were adapted from the eHEALS (E3) and the HCEI (E2) [ 23 , 24 ].
Survey section E3—eHEALS—comprised 8 questions, with participants reporting against a 5-point Likert scale for each (strongly disagree, disagree, neither, agree, and strongly agree). These responses were assigned 1 to 5 points, respectively. The summary eHEALS measure was derived by the summation of responses across the 8 questions, which were classified into 5 groups, A to E, based on these scores. Where fewer than half of the responses were missing, mean imputation was used; otherwise, the record was excluded. Groups A to C and D to E were combined for significance testing.
Survey section E2—HCEI—comprised 5 questions, with participants reporting against a 5-point Likert scale for each (strongly disagree, disagree, neither, agree, and strongly agree). Strongly disagree and disagree and neither were combined, and similarly agree and strongly agree were combined for significance testing.
Qualitative Results
The demographic characteristics of the patients that we interviewed are presented in Table 1 .
The key barriers found to accessing digital health included (1) strong patient preference for human-based health services; (2) low trust in digital health services; (3) high financial costs of necessary tools, maintenance, and repairs; (4) poor publicly available internet access options; (5) reduced capacity to engage due to increased life pressures; and (6) low self-efficacy and confidence in using digital health.
Rather than being an equal list of factors, our interviewees described these barriers as a stepped series of cumulative hurdles, which is illustrated in Figure 1 . Initial issues of preference and trust were foundational to a person even when considering the option of digital health, while digital health confidence and literacy were barriers to full engagement with and optimal use of digital health. Alternatively, interviewees who did use digital health had been enabled by the same factors that were barriers to others.
a GP: general practitioner.
b Multiple answers per respondent.
Strong Patient Preference for Human-Based Health Services
Some patients expressed a strong preference for human-based health services rather than digital health services. In answer to a question about how digital health services could be improved, a patient said the following:
Well, having an option where you can actually bypass actually having to go through the app and actually talk directly to someone. [Participant #10]
For some patients, this preference for human-based health services appeared to be related to a lack of exposure to eHealth. These patients were not at all interested in or had never thought about digital health options. A participant responded the following to the interviewer’s questions:
Interviewer: So when...something feels not right, how do you find out what’s going on?
Respondent: I talk to Doctor XX.
Interviewer: Do you ever Google your symptoms or look online for information?
Respondent: No, I have never even thought of doing that actually. [Participant #11]
For other patients, their preference for human-based health care stemmed from negative experiences with technology. These patients reported actively disliking computers and technology in general and were generally frustrated with what they saw as the pitfalls of technology. A patient stated the following:
If computers and internet weren’t so frigging slow because everything is on like the slowest speed network ever and there’s ads blocking everything. Ads, (expletive) ads. [Participant #9]
A patient felt that he was pushed out of the workforce due his inability to keep up with technology-based changes and thus made a decision to never own a computer:
But, you know, in those days when I was a lot younger those sorts of things weren’t about and they’re just going ahead in leaps and bounds and that’s one of the reasons why I retired early. I retired at 63 because it was just moving too fast and it’s all computers and all those sorts of things and I just couldn’t keep up. [Participant #17]
Low Trust in Digital Health Services
Several patients described low trust levels for digital and internet-based technology in general. Their low trust was generally based on stories they had heard of other people’s negative experiences. A patient said the following:
I don’t trust the internet to be quite honest. You hear all these stories about people getting ripped off and I’ve worked too hard to get what I’ve got rather than let some clown get it on the internet for me. [Participant #11]
Some of this distrust was specific to eHealth. For example, some patients were highly suspicious of the government’s motives with regard to digital health and were concerned about the privacy of their health information, which made them hesitant about the concept of a universal electronic health record. In response to the interviewer’s question, a participant said the following:
Interviewer: Are there any other ways you think that eHealth might help you?
Respondent: I’m sorry but it just keeps coming back to me, Big Brother. [Participant #7]
Another participant said the following:
I just would run a mile from it because I just wouldn’t trust it. It wouldn’t be used to, as I said, for insurance or job information. [Participant #16]
High Financial Costs of the Necessary Tools, Maintenance, and Repairs
A wide variety of patients described affordability issues across several different aspects of the costs involved in digital health. They expressed difficulty in paying for the following items: a mobile phone that could connect to the internet, a mobile phone with enough memory space to download apps, mobile phone apps requiring extra payment without advertisements, mobile phone repair costs such as a broken screen, a computer or laptop, home internet access, and adequate monthly data allowance and speeds to functionally use the internet. Current popular payment systems, such as plans, were not feasible for some patients. A participant stated the following:
I don’t have a computer...I’m not in the income bracket to own a computer really. Like I could, if I got one on a plan kind of thing or if I saved up for x-amount of time. But then like if I was going on the plan I’d be paying interest for having it on like lay-buy kind of thing, paying it off, and if it ever got lost or stolen I would still have to repay that off, which is always a hassle. And yeah. Yeah, I’m like financially not in the state where I’m able to...own a computer right now as I’m kind of paying off a number of debts. [Participant #9]
Poor Publicly Available Internet Access Options
Some patients described struggling without home internet access. While they noted some cost-free public internet access points, such as libraries, hotel bars, and restaurants, they often found these to be inconvenient, lacking in privacy, and constituting low-quality options for digital health. A patient stated the following:
...it’s incredibly slow at the library. And I know why...a friend I went to school with used to belong to the council and the way they set it up, they just got the raw end of the stick and it is really, really slow. It’s bizarre but you can go to the X Hotel and it’s heaps quicker. [Participant #15]
In response to the interviewer's question, a participant said the following:
Interviewer: And do you feel comfortable doing private stuff on computers at the library...?
Respondent: Not really, no, but I don’t have any other choice, so, yeah. [Participant #9]
Reduced Capacity to Engage Due to Increased Life Pressures
When discussing why they were not using digital health or why they had stopped using digital health, patients often described significant competing priorities and life pressures that affected their capacity to engage. An unemployed patient mentioned that his time and energy on the internet were focused primarily on finding work and that he barely had time to focus on his health in general, let alone engage in digital health.
Other patients reported that they often felt that their ability to learn about and spend time on digital health was taken up by caring for sick family members, paying basic bills, or learning English. Some patients said that the time they would have spent learning digital skills when they were growing up had been lost to adverse life circumstances such as being in jail:
So we didn’t have computers in the house when I was growing up. And I didn’t know I’ve never...I’ve been in and out of jail for 28 odd years so it sort of takes away from learning from this cause it’s a whole different… it’s a whole different way of using a telephone from a prison. [Participant #11]
Low Self-Efficacy and Confidence in Starting the Digital Health Process
Some patients had a pervasive self-perception of being slow learners and being unable to use technology. Their stories of being unconfident learners seemed to stem from the fact that they had been told throughout their lives that they were intellectually behind. A patient said the following:
The computer people...wouldn’t take my calls because I’ve always been dumb with that sort of stuff. Like I only found out this later on in life, but I’m actually severely numerically dyslexic. Like I have to triple-check everything with numbers. [Participant #7]
Another patient stated the following:
I like went to two English classes like a normal English class with all the kids and then another English class with about seven kids in there because I just couldn’t I don’t know maybe because I spoke another language at home and they sort of like know I was a bit backward. [Participant #6]
These patients and others had multiple missing pieces of information that they felt made it harder to engage in digital health compared to “easier” human-based services. A patient said the following:
Yeah I’ve heard of booking online but I just I don’t know I find it easier just to ring up. And I’ll answer an email from a health care provider but I wouldn’t know where to start to look for their email address. [Participant #11]
In contrast, the patients who did connect with digital health described themselves as independent question askers and proactive people. Even when they did not know how to use a specific digital health tool, they were confident in attempting to and asking for help when they needed it. A patient said the following:
I’m a “I will find my way through this, no matter how long it takes me” kind of person. So maybe it’s more my personality...If I have to ask for help from somewhere, wherever it is, I will definitely do that. [Participant #3]
Quantitative Results
A total of 487 valid survey responses were received from participants across 24 general practices. The participant characteristics are presented in detail in Table S1 in Multimedia Appendix 3 .
The mean age of the participants was approximately 50 years (females 48.9, SD 19.4 years; males 52.8, SD 20.0 years), and 68.2% (332/487) of the participants identified as female. Overall, 34.3% (151/439) of respondents reported never using eHealth, and 53.8% (236/439) reported high eHealth use.
There were statistically significant ( P <.05) differences in the frequency of eHealth use in terms of age group, gender, state, remoteness, highest level of education, employment status, occupation group, marital status, and language spoken at home, with effect sizes being small to medium. Specifically, high eHealth characteristics were associated with younger age, being female, living in an urban area, and being employed.
Table 2 presents the frequency of eHealth use against 3 internet preference questions.
Preference for using the internet and technology in general and for health needs in particular were significantly related to the frequency of eHealth use ( P <.05 for each), with the effect sizes being small to medium.
a Excludes those for whom frequency of eHealth use is unknown.
b Chi-square tests conducted with strongly disagree and disagree combined, and agree and strongly agree combined.
Table 3 presents the frequency of eHealth use against 4 measures of trust.
The degree of trust was not statistically significantly different for the frequency of eHealth use for any of the domains.
b eHLQ: eHealth Literacy Questionnaire.
c Derived from survey question D1, parts 4 to 8. Mean imputation used where ≤2 responses were missing. If >2 responses were missing, the records were excluded.
d Derived from survey question D2, parts 1 to 5. Mean imputation used where ≤2 responses were missing. If >2 responses were missing, the records were excluded.
e Chi-square test conducted comparing low-to-moderate trust against high trust.
f Derived from survey question D2, parts 6, 7, 9, and 10. Mean imputation used where ≤2 responses were missing. If >2 responses were missing, the records were excluded.
g Derived from survey question D2 part 8.
h Chi-square test conducted comparing low trust against moderate-to-high trust.
Affordability of items and services was reported as No cost difficulty or Cost difficulty. eHealth frequency of use responses were available for 273 participants; among those with no cost difficulty , 1% (2/204) were never users, 14.2% (29/204) were low or medium users, and 84.8% (173/204) were high users of eHealth; among those with cost difficulty , 1% (1/69) were never users, 26% (18/69) were low or medium users, and 73% (50/69) were high users. There was a statistically significant difference in the presence of cost as a barrier between never and low or medium eHealth users compared to high users ( χ 2 1 =5.25; P =.02), although the effect size was small.
Table 4 presents the frequency of eHealth use for elements of structural access.
Quality of internet access and feeling limited in access to the internet were significantly associated with frequency of eHealth use ( P <.05), although the effect sizes were small.
b N/A: not applicable (cell sizes insufficient for chi-square test).
c Chi-square tests conducted with strongly disagree and disagree combined, agree and strongly agree combined, and never and low or medium categories combined.
Table 5 presents the frequency of eHealth use against respondents’ capacity to engage.
Capacity to engage was not significantly different for the frequency of eHealth use ( P =.54).
b Derived from survey item E1. Where 1 response was missing, the mean imputation was used. If >1 response was missing, the record was excluded.
c Chi-square tests conducted with groups A and B combined.
Table 6 presents the frequency of eHealth use for elements of self-efficacy.
Statistically significant results were observed for the relationship between self-efficacy by eHEALS (moderate effect size) and frequency of eHealth use as well as for some of the questions from the HCEI (reliance on health professionals or others to access and explain information; small effect size; P <.05).
b eHEALS: eHealth Literacy Scale.
c eHEALS derived from item E3 (8 parts). Where ≤ 4 responses were missing, mean imputation was used. If >4 responses were missing, the records were excluded. Groups A to C as well as groups D to E were combined for the chi-square test.
d Strongly disagree, disagree, neither, and agree or strongly agree combined for significance testing.
Principal Findings
This paper reports on the findings of a sequential exploratory mixed methods study on the barriers to digital health access for a group of patients in Australian family medicine, with a particular focus on chronic disease and socioeconomic disadvantage.
In the qualitative first phase, the patients with socioeconomic disadvantage and chronic disease described 6 cumulative barriers, as demonstrated in Figure 1 . Many nonusers of digital health preferred human-based services and were not interested in technology, while others were highly suspicious of the technology in general. Some digitally interested patients could not afford quality hardware and internet connectivity, a barrier that was doubled by low quality and privacy when accessing publicly available internet connections. Furthermore, although some digitally interested patients had internet access, their urgent life circumstances left scarce opportunity to access digital health and develop digital health skills and confidence.
In our quantitative second phase, 31% (151/487) of the survey participants from Australian general practices were found to have never used a form of digital health. Survey participants were more likely to use digital health tools frequently when they also had a general digital interest and a digital health interest. Those who did not frequently access digital health were more likely to report difficulty affording the financial costs needed for digital access. The survey participants who frequently accessed digital health were more likely to have high eHealth literacy and high levels of patient empowerment.
Comparison With Prior Work
In terms of general digital health access, the finding that 31% (151/487) of the survey participants had never used one of the described forms of eHealth is in keeping with an Australian-based general digital participation study that found that approximately 9% of the participants were nonusers and 17% rarely engaged with the internet at all [ 34 ]. With regard to the digital health divide, another Australian-based digital health divide study found that increased age, living in a lower socioeconomic area, being Aboriginal or Torres Strait Islander, being male, and having no tertiary education were factors negatively associated with access to digital health services [ 17 ]. Their findings correspond to our findings that higher-frequency users of eHealth were associated with younger age, being female, living in an urban area, and being employed. Both studies reinforce the evidence of the digital health divide based on gender, age, and socioeconomic disadvantage in Australia.
With regard to digital health barriers, our findings provide expanded details on the range of digital health items and services that present a cost barrier to consumers. Affordability is a known factor in digital access and digital health access, and it is measured often by general self-report or relative expenditure on internet access to income [ 30 ]. Our study revealed the comprehensive list of relevant costs for patients. Our study also demonstrated factors of cost affordability beyond the dollar value of an item, as interviewees described the struggle of using slow public internet access without privacy features and the risks involved in buying a computer in installments. When we reflected on the complexity and detail of the cost barrier in our survey, participants demonstrated a clear association between cost and the frequency of digital health use. This suggests that a way to improve digital health access for some people is to improve the quality, security, and accessibility of public internet access options as well as to provide free or subsidized hardware, internet connection, and maintenance options for those in need, work that is being done by at least 1 digital inclusion charity in the United Kingdom [ 35 ].
Many studies recognize the factors of eHealth literacy and digital confidence for beneficial digital health access [ 36 ]. Our interviews demonstrated that some patients with socioeconomic disadvantage have low digital confidence, but that this is often underlined by a socially reinforced lifelong low self-confidence in their intellectual ability. In contrast, active users, regardless of other demographic factors, described themselves as innately proactive question askers. This was reinforced by our finding of a relationship between health care empowerment and the frequency of eHealth use. This suggests that while digital health education and eHealth literacy programs can improve access for some patients, broader and deeper long-term solutions addressing socioeconomic drivers of digital exclusion are needed to improve digital health access for some patients with socioeconomic disadvantage [ 8 ]. The deep permeation of socially enforced low self-confidence and lifelong poverty experienced by some interviewees demonstrate that the provision of free hardware and a class on digital health skills can be, for some, a superficial offering when the key underlying factor is persistent general socioeconomic inequality.
The digital health divide literature tends to identify the digital health divide, the factors and barriers that contribute to it, and the potential for it to widen if not specifically addressed [ 16 ]. Our findings have also identified the divide and the barriers, but what this study adds through our qualitative phase in particular is a description of the complex interaction of those barriers and the stepped nature of some of those barriers as part of the individual’s experience in trying to access digital health.
Strengths and Limitations
A key strength of this study is the use of a sequential exploratory mixed methods design. The initial qualitative phase guided a phenomenological exploration of digital health access experiences for patients with chronic disease and socioeconomic disadvantage. Our results in both study phases stem from the patients’ real-life experiences of digital health access. While some of our results echo the findings of other survey-based studies on general digital and digital health participation, our method revealed a greater depth and detail of some of these barriers, as demonstrated in how our findings compare to prior work.
As mentioned previously, the emphasis of this study on the qualitative first phase is a strength that helped describe the interactions between different barriers. The interviewees described their experiences as cumulative unequal stepped barriers rather than as producing a nonordered list of equal barriers. These findings expand on the known complexity of the issue of digital exclusion and add weight to the understanding that improving digital health access needs diverse, complex solutions [ 17 ]. There is no panacea for every individual’s digital health access, and thus, patient-centered digital health services, often guided by health professionals within the continuity of primary care, are also required to address the digital health divide [ 37 ].
While the sequential exploratory design is a strength of the study, it also created some limitations for the second quantitative phase. Our commitment to using the qualitative interview findings to inform the survey questions meant that we were unable to use previously validated scales for every question and that our results were less likely to lead to a normal distribution. This likely affected our ability to demonstrate significant associations for some barriers. We expect that further modeling is required to control for baseline characteristics and determine barrier patterns for different types of users.
One strength of this study is that the survey was administered to a broad population of Australian family medicine patients with diverse patterns of health via both paper-based and digital options. Many other digital health studies use solely digital surveys, which can affect the sample. However, we cannot draw conclusions from our survey about patients with chronic disease due to the limitations of the sample size for these subgroups.
Another sample-based limitation of this study was that our qualitative population did not include anyone aged from 18 to 24 years, despite multiple efforts to recruit. Future research will hopefully address this demographic more specifically.
While not strictly a limitation, we recognize that because this research was before COVID-19, it did not include questions about telehealth, which has become much more mainstream in recent years. The patients may also have changed their frequency of eHealth use because of COVID-19 and an increased reliance on digital services in general. Future work in this area or future versions of this survey should include telehealth and acknowledge the impact of COVID-19. However, the larger concept of the digital health divide exists before and after COVID-19, and in fact, our widespread increased reliance on digital services makes the digital divide an even more pressing issue [ 12 ].
Conclusions
The experience of digital health access across Australian primary care is highly variable and more difficult to access for those with socioeconomic disadvantage. While general digital interest, financial cost, and digital health literacy and empowerment are clear factors in digital health access in a broad primary care population, the digital health divide is also facilitated in part by a stepped series of complex and cumulative barriers.
Genuinely improving digital health access for 1 cohort or even 1 person requires a series of multiple different interventions tailored to specific sequential barriers. Given the rapid expansion of digital health during the global COVID-19 pandemic, attention to these issues is necessary if we are to avoid entrenching inequities in access to health care. Within primary care, patient-centered care that continues to recognize the complex individual needs of, and barriers facing, each patient should be a part of addressing the digital health divide.
Acknowledgments
The authors are thankful to the patients who shared their experiences with them via interview and survey completion. The authors are also very grateful to the general practices in the Australian Capital Territory and New South Wales who kindly gave their time and effort to help organize interviews, administer, and post surveys in the midst of the stress of day-to-day practice life and the bushfires of 2018-2019. The authors thank and acknowledge the creators of the eHealth Literacy Scale, the eHealth Literacy Questionnaire, the ICEpop Capability Measure for Adults, the Health Care Empowerment Inventory, the Patient-Doctor Relationship Questionnaire, the Chao continuity questionnaire, and the Southgate Institute for Health Society and Equity for their generosity in sharing their work with the authors [ 17 , 19 - 25 ]. This study would not have been possible without the support of the administrative team of the Academic Unit of General Practice. This project was funded by the Royal Australian College of General Practitioners (RACGP) through the RACGP Foundation IPN Medical Centres Grant, and the authors gratefully acknowledge their support.
Data Availability
The data sets generated during this study are not publicly available due to the nature of our original ethics approval but are available from the corresponding author on reasonable request.
Authors' Contributions
MAC acquired the funding, conceptualized the project, and organized interview recruitment. MAC and KB conducted interviews and analyzed the qualitative data. EAS, ER, and KD contributed to project planning, supervision and qualitative data analysis. MAC, KB and KO wrote the survey and planned quantitative data analysis. MAC and KB recruited practices for survey administration. KO and KB conducted the quantitative data analysis. MAC and KO, with KB drafted the paper. EAS, ER, and KD helped with reviewing and editing the paper.
Conflicts of Interest
None declared.
Phase 1 interview guide.
Phase 2 survey: eHealth and digital divide.
Phase 2 participant characteristics by frequency of eHealth use.
- Eysenbach G. What is e-health? J Med Internet Res. 2001;3(2):E20. [ FREE Full text ] [ CrossRef ] [ Medline ]
- Iyawa GE, Herselman M, Botha A. Digital health innovation ecosystems: from systematic literature review to conceptual framework. Procedia Comput Sci. 2016;100:244-252. [ FREE Full text ] [ CrossRef ]
- Berrouiguet S, Baca-García E, Brandt S, Walter M, Courtet P. Fundamentals for future mobile-health (mHealth): a systematic review of mobile phone and web-based text messaging in mental health. J Med Internet Res. Jun 10, 2016;18(6):e135. [ FREE Full text ] [ CrossRef ] [ Medline ]
- Shen H, van der Kleij RM, van der Boog PJ, Chang X, Chavannes NH. Electronic health self-management interventions for patients with chronic kidney disease: systematic review of quantitative and qualitative evidence. J Med Internet Res. Nov 05, 2019;21(11):e12384. [ FREE Full text ] [ CrossRef ] [ Medline ]
- Australia's health 2018. Australian Institute of Health and Welfare. 2018. URL: https://www.aihw.gov.au/reports/australias-health/australias-health-2018/contents/table-of-contents [accessed 2024-04-04]
- Australian Institute of Health and Welfare. Chronic Diseases and Associated Risk Factors in Australia, 2006. Canberra, Australia. Australian Institute of Health and Welfare; 2006.
- Hart JT. The inverse care law. The Lancet. Feb 27, 1971;297(7696):405-412. [ CrossRef ]
- Davies AR, Honeyman M, Gann B. Addressing the digital inverse care law in the time of COVID-19: potential for digital technology to exacerbate or mitigate health inequalities. J Med Internet Res. Apr 07, 2021;23(4):e21726. [ FREE Full text ] [ CrossRef ] [ Medline ]
- Choi NG, Dinitto DM. The digital divide among low-income homebound older adults: internet use patterns, eHealth literacy, and attitudes toward computer/internet use. J Med Internet Res. May 02, 2013;15(5):e93. [ FREE Full text ] [ CrossRef ] [ Medline ]
- Household use of information technology. Australian Bureau of Statistics. 2018. URL: https://tinyurl.com/4efm6u92 [accessed 2024-03-24]
- Kontos E, Blake KD, Chou WY, Prestin A. Predictors of eHealth usage: insights on the digital divide from the health information national trends survey 2012. J Med Internet Res. Jul 16, 2014;16(7):e172. [ FREE Full text ] [ CrossRef ] [ Medline ]
- Litchfield I, Shukla D, Greenfield S. Impact of COVID-19 on the digital divide: a rapid review. BMJ Open. Oct 12, 2021;11(10):e053440. [ FREE Full text ] [ CrossRef ] [ Medline ]
- Butler DC, Joshy G, Douglas KA, Sayeed MS, Welsh J, Douglas A, et al. Changes in general practice use and costs with COVID-19 and telehealth initiatives: analysis of Australian whole-population linked data. Br J Gen Pract. Apr 27, 2023;73(730):e364-e373. [ FREE Full text ] [ CrossRef ] [ Medline ]
- Arsenijevic J, Tummers L, Bosma N. Adherence to electronic health tools among vulnerable groups: systematic literature review and meta-analysis. J Med Internet Res. Feb 06, 2020;22(2):e11613. [ FREE Full text ] [ CrossRef ] [ Medline ]
- Kontos EZ, Bennett GG, Viswanath K. Barriers and facilitators to home computer and internet use among urban novice computer users of low socioeconomic position. J Med Internet Res. Oct 22, 2007;9(4):e31. [ FREE Full text ] [ CrossRef ] [ Medline ]
- Latulippe K, Hamel C, Giroux D. Social health inequalities and eHealth: a literature review with qualitative synthesis of theoretical and empirical studies. J Med Internet Res. Apr 27, 2017;19(4):e136. [ FREE Full text ] [ CrossRef ] [ Medline ]
- Foley K, Freeman T, Ward P, Lawler A, Osborne R, Fisher M. Exploring access to, use of and benefits from population-oriented digital health services in Australia. Health Promot Int. Aug 30, 2021;36(4):1105-1115. [ CrossRef ] [ Medline ]
- Cresswell JW, Plano Clark VL. Designing and Conducting Mixed Methods Research. Thousand Oaks, CA. SAGE Publications; 2007.
- Tappen RM, Cooley ME, Luckmann R, Panday S. Digital health information disparities in older adults: a mixed methods study. J Racial Ethn Health Disparities. Feb 2022;9(1):82-92. [ FREE Full text ] [ CrossRef ] [ Medline ]
- Who can get a card. Services Australia. URL: https://www.servicesaustralia.gov.au/who-can-get-health-care-card?context=21981 [accessed 2023-11-03]
- Levesque JF, Harris MF, Russell G. Patient-centred access to health care: conceptualising access at the interface of health systems and populations. Int J Equity Health. Mar 11, 2013;12:18. [ FREE Full text ] [ CrossRef ] [ Medline ]
- Bryant A, Charmaz K. The SAGE Handbook of Grounded Theory, Paperback Edition. Thousand Oaks, CA. SAGE Publications; 2010.
- Johnson MO, Rose CD, Dilworth SE, Neilands TB. Advances in the conceptualization and measurement of health care empowerment: development and validation of the health care empowerment inventory. PLoS One. 2012;7(9):e45692. [ FREE Full text ] [ CrossRef ] [ Medline ]
- Norman CD, Skinner HA. eHEALS: the eHealth literacy scale. J Med Internet Res. Nov 14, 2006;8(4):e27. [ FREE Full text ] [ CrossRef ] [ Medline ]
- Kayser L, Karnoe A, Furstrand D, Batterham R, Christensen KB, Elsworth G, et al. A multidimensional tool based on the eHealth literacy framework: development and initial validity testing of the eHealth Literacy Questionnaire (eHLQ). J Med Internet Res. Feb 12, 2018;20(2):e36. [ FREE Full text ] [ CrossRef ] [ Medline ]
- Standards. Australian Bureau of Statistics. URL: https://www.abs.gov.au/statistics/standards [accessed 2024-04-04]
- Al-Janabi H, Flynn TN, Coast J. Development of a self-report measure of capability wellbeing for adults: the ICECAP-A. Qual Life Res. Feb 2012;21(1):167-176. [ FREE Full text ] [ CrossRef ] [ Medline ]
- Van der Feltz-Cornelis CM, Van Oppen P, Van Marwijk HW, De Beurs E, Van Dyck R. A patient-doctor relationship questionnaire (PDRQ-9) in primary care: development and psychometric evaluation. Gen Hosp Psychiatry. 2004;26(2):115-120. [ CrossRef ] [ Medline ]
- Chao J. Continuity of care: incorporating patient perceptions. Fam Med. 1988;20(5):333-337. [ Medline ]
- Wilson CK, Thomas J, Barraket J. Measuring digital inequality in Australia: the Australian digital inclusion index. JTDE. Jun 30, 2019;7(2):102-120. [ CrossRef ]
- Digital participation: a view of Australia's online behaviours. Australia Post. Jul 2017. URL: https://auspost.com.au/content/dam/auspost_corp/media/documents/white-paper-digital-inclusion.pdf [accessed 2024-04-04]
- Poli A, Kelfve S, Motel-Klingebiel A. A research tool for measuring non-participation of older people in research on digital health. BMC Public Health. Nov 08, 2019;19(1):1487. [ FREE Full text ] [ CrossRef ] [ Medline ]
- Cohen J. Statistical Power Analysis for the Behavioral Sciences Second Edition. London, UK. Routledge; 1988.
- Borg K, Smith L. Digital inclusion and online behaviour: five typologies of Australian internet users. Behav Inf Technol. Feb 15, 2018;37(4):367-380. [ CrossRef ]
- Mathers A, Richardson J, Vincent S, Joseph C, Stone E. Good Things Foundation COVID-19 response report. Good Things Foundation. 2020. URL: https://tinyurl.com/2peu3kak [accessed 2024-04-04]
- Norman CD, Skinner HA. eHealth literacy: essential skills for consumer health in a networked world. J Med Internet Res. Jun 16, 2006;8(2):e9. [ FREE Full text ] [ CrossRef ] [ Medline ]
- Neves AL, Burgers J. Digital technologies in primary care: implications for patient care and future research. Eur J Gen Pract. Dec 11, 2022;28(1):203-208. [ FREE Full text ] [ CrossRef ] [ Medline ]
Abbreviations
Edited by T Leung; submitted 03.07.23; peer-reviewed by T Freeman, H Shen; comments to author 16.08.23; revised version received 30.11.23; accepted 31.01.24; published 11.04.24.
©Melinda Ada Choy, Kathleen O'Brien, Katelyn Barnes, Elizabeth Ann Sturgiss, Elizabeth Rieger, Kirsty Douglas. Originally published in the Journal of Medical Internet Research (https://www.jmir.org), 11.04.2024.
This is an open-access article distributed under the terms of the Creative Commons Attribution License (https://creativecommons.org/licenses/by/4.0/), which permits unrestricted use, distribution, and reproduction in any medium, provided the original work, first published in the Journal of Medical Internet Research, is properly cited. The complete bibliographic information, a link to the original publication on https://www.jmir.org/, as well as this copyright and license information must be included.

An official website of the United States government
The .gov means it’s official. Federal government websites often end in .gov or .mil. Before sharing sensitive information, make sure you’re on a federal government site.
The site is secure. The https:// ensures that you are connecting to the official website and that any information you provide is encrypted and transmitted securely.
- Publications
- Account settings
Preview improvements coming to the PMC website in October 2024. Learn More or Try it out now .
- Advanced Search
- Journal List
- JRSM Short Rep
- v.4(6); 2013 Jun
Using mixed methods in health research
Shema tariq.
1 School of Health Sciences, City University London, EC1A 7QN, London, UK
Jenny Woodman
2 MRC Centre of Epidemiology for Child Health, UCL Institute of Child Health, WC1N 1EH, London, UK
Mixed methods research is the use of quantitative and qualitative methods in a single study or series of studies. It is an emergent methodology which is increasingly used by health researchers, especially within health services research. There is a growing literature on the theory, design and critical appraisal of mixed methods research. However, there are few papers that summarize this methodological approach for health practitioners who wish to conduct or critically engage with mixed methods studies. The objective of this paper is to provide an accessible introduction to mixed methods for clinicians and researchers unfamiliar with this approach. We present a synthesis of key methodological literature on mixed methods research, with examples from our own work and that of others, to illustrate the practical applications of this approach within health research. We summarize definitions of mixed methods research, the value of this approach, key aspects of study design and analysis, and discuss the potential challenges of combining quantitative and qualitative methods and data. One of the key challenges within mixed methods research is the successful integration of quantitative and qualitative data during analysis and interpretation. However, the integration of different types of data can generate insights into a research question, resulting in enriched understanding of complex health research problems.
Introduction
Mixed methods research is the use of quantitative and qualitative methods in one study. Research is often dichotomized as quantitative or qualitative. Quantitative research, such as clinical trials or observational studies, generates numerical data. On the other hand qualitative approaches tend to generate non-numerical data, using methods such as semi-structured interviews, focus group discussions and participant observation. Historically, quantitative methods have dominated health research. However, qualitative methods have been increasingly accepted by the health research community in the past two decades, with a rise in publication of qualitative studies. 1 As the value of qualitative approaches has been recognized, there has been a growing interest in combining qualitative and quantitative methods. A recent review of health services research within England has shown an increase in the proportion of studies classified as mixed methods from 17% in the mid-1990s to 30% in the early 2000s. 2 In this paper, we present a synthesis of key literature on mixed methods research, with examples from our own work and that of others to illustrate the practical applications of this approach. This paper is aimed at health researchers and practitioners who are new to the field of mixed methods research and may only have experience of either quantitative or qualitative approaches and methodologies. We wish to provide these readers with an accessible introduction to the increasingly popular methodology of mixed methods research. We hope this will help readers to consider whether their research questions might best be answered by a mixed methods study design, and to engage critically with health research that uses this approach.
The authors each independently carried out a narrative literature review and met to discuss findings. Literature was identified via searches of PubMed, Google and Google Scholar, and hand-searches of the Journal of Mixed Methods Research, with relevant publications selected after discussion. An important consideration was that papers either had a methodological focus or contained a detailed description of their mixed methods design. For PubMed and Google searches, similar terms were used. For example, the PubMed strategy consisted of title and abstract searches for: ((mixed methods) OR ((mixed OR (qualitative AND quantitative)) AND methods)). We also drew upon recommendations from mixed methods conferences and seminars, and reference lists from key publications.
What is mixed methods research?
The most widely accepted definition of mixed methods research is research that ‘focuses on collecting, analysing, and mixing both quantitative and qualitative data in a single study or a series of studies’. 3 Central to the definition is the use of both quantitative and qualitative methods in one study (or a series of connected studies). Separate quantitative and qualitative studies addressing the same research question independently would not be considered ‘mixed methods’ as there would be no integration of approaches at the design, analysis or presentation stage. A recent innovation in mixed methods research is the mixed methods systematic review, which sets out to systematically appraise both quantitative and qualitative literature on a subject area and then synthesize the findings.
Why are mixed methods approaches used?
The underlying assumption of mixed methods research is that it can address some research questions more comprehensively than by using either quantitative or qualitative methods alone. 3 Questions that profit most from a mixed methods design tend to be broad and complex, with multiple facets that may each be best explored by quantitative or qualitative methods. See Boxes 1 and and2 2 for examples from our own work.
Examples of authors’ mixed methods research – JW.
Examples of authors’ mixed methods research – ST.
Usually, quantitative research is associated with a positivist stance and a belief that reality that can be measured and observed objectively. Most commonly, it sets out to test an a priori hypothesis and is therefore conventionally described as ‘deductive’. Strengths of quantitative research include its procedures to minimize confounding and its potential to generate generalizable findings if based on samples that are both large enough and representative. It remains the dominant paradigm in health research. However, this deductive approach is less suited to generating hypotheses about how or why things are happening, or explaining complex social or cultural phenomena.
Qualitative research most often comes from an interpretive framework and is usually informed by the belief that there are multiple realities shaped by personal viewpoints, context and meaning. In-depth qualitative research aims to provide a rich description of views, beliefs and meaning. It also tends to acknowledge the role of researcher and context in shaping and producing the data. Qualitative approaches are described as ‘inductive’ as questions are often open-ended with the analysis allowing hypotheses to emerge from data. High-quality qualitative research can generate robust theory that is applicable to contexts outside of the study area in question, helping to guide practitioners and policy-makers. 8 However, for research that aims to directly impact on policy and practice, the findings of qualitative research can be limited by the small sample sizes that are necessary for in-depth exploratory work and the consequent lack of generalizabilty.
Mixed methods research therefore has the potential to harness the strengths and counterbalance the weaknesses of both approaches and can be especially powerful when addressing complex, multifaceted issues such as health services interventions 9 and living with chronic illness. 10
There are many reasons why researchers choose to combine quantitative and qualitative methods in a study. 11 , 12 We list some common reasons below, using a hypothetical research question about adolescents’ adherence to anticonvulsant medication to illustrate real world applications.
- Complementarity: Using data obtained by one method to illustrate results from another. An example of this would be a survey of adolescents with epilepsy demonstrating poor levels of adherence. Semi-structured interviews with a sub-group of those surveyed may allow us to explore barriers to adherence.
- Development: Using results from one method to develop or inform the use of the other method. A focus group conducted with a group of adolescents with epilepsy may identify mobile phone technology as a potentially important tool in adherence support. We could then develop a mobile phone ‘app’ that reminds patients to take their medication and conduct an intervention study to assess its impact on adherence levels.
- Initiation: Using results from different methods specifically to look for areas of incongruence in order to generate new insights. An illustration of this would be a study exploring the discrepancy between reported adherence in clinic consultations and actual medication adherence. A review of case notes may find adherence levels of over 90% in a clinic population; however, semi-structured interviews with peer researchers may reveal lower levels of adherence and barriers to open discussion with clinicians.
- Expansion: Setting out to examine different aspects of a research question, where each aspect warrants different methods. We may wish to conduct a study that explores adherence more broadly. A large-scale survey of adolescents with epilepsy would provide information on adherence levels and associations whilst interviews and focus groups may allow us to engage with individual experiences of chronic illness and medication in adolescence.
- Triangulation: Using data obtained by both methods to corroborate findings. For example, we could conduct a clinical study measuring drug levels in individuals and documenting self-reported adherence. Qualitative methods such as video diaries may confirm adherence levels.
To this list we would also add political commitment. That is to say, researchers may recognize, and wish to deploy, the strengths of quantitative research in producing generalizable results but may also be committed to representing the voice of participants in their work.
Whatever the reasons for mixing methods, it is important that authors present these explicitly as it allows us to assess if a mixed methods study design is appropriate for answering the research question. 3 , 13
How is mixed methods research conducted?
When embarking on a mixed methods research project it is important to consider:
- the methods that will be used;
- the priority of the methods;
- the sequence in which the methods are to be used.
A wide variety of methods exists by which to collect both quantitative and qualitative data. Both the research question and the data required will be the main determinants of the methods used. To a lesser extent, the choice of methods may be influenced by feasibility, the research team’s skills and experience and time constraints.
Priority of methods relates to the emphasis placed on each method in the study. For instance, the study may be predominantly a quantitative study with a small qualitative component, or vice versa. Alternatively, both quantitative and qualitative methods and data may have equal weighting. The emphasis given to each component of the study will be driven mainly by the research question, the skills of the research team and feasibility.
Finally, researchers must decide when each method is to be used in the study. For instance a team may choose to start with a quantitative phase followed by a qualitative phase, or vice versa. Some studies use both quantitative and qualitative methods concurrently. Again the choice of when to use each method is largely dependent on the research question.
The priority and sequence of mixing methods have been elaborated in a typology of mixed methods research models. See Table 1 for typology and specific examples.
Examples of studies using mixed methods.
How is data analysed in a mixed methods project?
The most important, and perhaps most difficult, aspect of mixed methods research is integrating the qualitative and quantitative data. One approach is to analyse the two data types separately and to then undertake a second stage of analysis where the data and findings from both studies are compared, contrasted and combined. 19 The quantitative and qualitative data are kept analytically distinct and are analysed using techniques usually associated with that type of data; for example, statistical techniques could be used to analyse survey data whilst thematic analysis may be used to analyse interview data. In this approach, the integrity of each data is preserved whilst also capitalizing on the potential for enhanced understanding from combining the two data and sets of findings.
Another approach to mixed methods data analysis is the integrative strategy. 20 Rather than keeping the datasets separate, one type of data may be transformed into another type. That is to say that qualitative data may be turned into quantitative data (‘quantitizing’) or quantitative data may be converted into qualitative data (‘qualitizing’). 21 The former is probably the most common method of this type of integrated analysis. Quantitative transformation is achieved by the numerical coding of qualitative data to create variables that may relate to themes or constructs, allowing statements such as ‘six of 10 participants spoke of the financial barriers to accessing health care’. These data can then be combined with the quantitative dataset and analysed together. Transforming quantitative data into qualitative data is less common. An example of this is the development of narrative psychological ‘types’ from numerical data obtained by questionnaires. 22
Potential challenges in conducting mixed methods research
Despite its considerable strengths as an approach, mixed methods research can present researchers with challenges. 23 , 24
Firstly, combining methodologies has sometimes been seen as problematic because of the view that quantitative and qualitative belong to separate and incompatible paradigms. In this context, paradigms are the set of practices and beliefs held by an academic community at a given point in time. 25 Researchers subscribing to this view argue that it is neither possible nor desirable to combine quantitative and qualitative methods in a study as they represent essentially different and conflicting ways of viewing the world and how we collect information about it. 8 Other researchers take a more pragmatic view, believing that concerns about the incommensurability of worldviews can be set aside if the combination of quantitative and qualitative methods addresses the research question effectively. This pragmatic view informs much applied mixed methods research in health services or policy. 8
Secondly, combining two methods in one study can be time consuming and requires experience and skills in both quantitative and qualitative methods. This can mean, in reality, that a mixed methods project requires a team rather than a lone researcher in order to conduct the study rigorously and within the specified time frame. However, it is important that a team comprising members from different disciplines work well together, rather than becoming compartmentalized. 26 We believe that a project leader with experience in both quantitative and qualitative methods can act as an important bridge in a mixed methods team.
Thirdly, achieving true integration of the different types of data can be difficult. We have suggested various analytic strategies above but this can be hard to achieve as it requires innovative thinking to move between different types of data and make meaningful links between them. It is therefore important to reflect on the results of a study and ask if your understanding has been enriched by the combination of different types of data. If this is not the case then integration may not have occurred sufficiently. 23
Finally, many researchers cite the difficulty in presenting the results of mixed methods study as a barrier to conducting this type of research. 23 Researchers may decide to present their quantitative and qualitative data separately for different audiences. This strategy may involve a decision to publish additional work focusing on the interpretations and conclusions which come from comparing and contrasting findings from the different data types. See Box 1 for an example of this type of publication strategy. Many journals in the medical sciences have a distinct methodological base and relatively restrictive word limits which may preclude the publication of complex, mixed methods studies. However, as the number of mixed methods studies increases in the health research literature we would expect researchers to feel more confident in the presentation of this type of work.
Many of the areas we explore in health are complex and multifaceted. Mixed methods research (combining quantitative and qualitative methods in one study) is an innovative and increasingly popular way of addressing these complexities. Although mixed methods research presents some challenges, in much the same way as every methodology does, this approach provides the research team with a wider range of tools at their disposal in order to answer a question. We believe that the production and integration of different types of data and the combination of skill sets in a team can generate insights into a research question, resulting in enriched understanding.
DECLARATIONS
Competing interests.
None declared
This work was funded by the Medical Research Council (MRC) [grant number: G0701648 to ST], and the MRC with the Economic and Social Research Council (ESRC) [grant number: G0800112 to JW]
Ethical approval
No ethical approval was required for this work
Contributorship
This work was conceived by both ST and JW who each carried out an independent literature review and collaborated on the structure and content of this report. ST wrote the manuscript with revisions and editing done by JW
Acknowledgements
We thank Professors Jonathan Elford and Ruth Gilbert for their comments on draft manuscripts
This article was submitted by the authors and peer reviewed by Geoffrey Harding
- Search This Site All UCSD Sites Faculty/Staff Search Term
- 3rd Week Enrollment
- Undergraduate Statistics
- Graduate Statistics
- Undergraduate Surveys
- Graduate Surveys
- Diversity Statistics
- Decision Support
Equity Analytics
Equity research & analytics.
Our team supports UC San Diego's Strategic Plan and the Strategic Plan for Inclusive Excellence through:
Creating Accountability Through Data
We facilitate the use of data to build meaning-making in collaboration with context-knowledgeable others. We achieve this by embedding our work into processes and partnerships focused on improving structures, policies, and practices to support our campus-wide commitment to Inclusive Excellence . Currently, the Equity Research & Analytics Team provides data for several key accountability processes, including the Campus-Wide Strategic Plan for Inclusive Excellence Accountability Process and the American Association for the Advancement of Science STEMM Equity Initiative , also known as SEA Change.
Mixed Methods & Theoretically Informed Research
We embrace sociological, educational, organizational change, and other bodies of scholarly research & theory to inform and contextualize our work and engage multiple methods to enable us to answer pressing questions with thoroughness and rigor to drive institutional action forward. An example of this work includes our 2022 report, co-authored with the Director of the Office for Faculty Diversity and Inclusion Mardestinee Perez, Supporting UC San Diego’s Academic Employees: Findings and Checklists for Change from the Academics@UCSD™ Survey and Roundtables .
Collaborative Partnerships Around Data & Research
The work of the Equity Research & Analytics Team would not be possible without our fantastic partners! Internal to Institutional Research, the Equity Research & Analytics Team partners closely with the Student Success Research & Analytics Team, Administrative Operations Research Team, and individual contributors to support data-informed change. The Equity Research & Analytics Team also partners with data & research experts across General Campus, the Health Sciences, and the Health System to ensure the information used to drive change is both accurate and appropriately contextualized. Our Team embraces the Executive Vice Chancellor's Collective Impact approach and operates under the motto that we can "make tremendous progress on UC San Diego’s strategic priorities by working together more intentionally."
Providing Educational Opportunities and Developing Community to Support Equity-Minded Data Use & Assessment Practices
The Equity Research & Analytics Team works in partnership with other units and experts to provide educational opportunities to increase equity-mindedness in the data, research, and assessment space. This includes providing support around developing an equity-mindedness approach to units undergoing program review, through trainings with Erin Espaldon, the Director of the Student Success Research & Analytics Team. We also work closely with other units that share our philosophies about equity-mindedness and student-centeredness such as Vice Chancellor for Student Affairs Assessment, Evaluation, and Organizational Development to create staff and faculty development opportunities, such as our multi-part equity-minded assessment cycle course, which will be offered for a third time with UC Santa Cruz in Fall 2023.
Providing Thought Partnership Around Equity-Minded, Privacy-Oriented, and Ethical Data Use
"It's important to remember that behind every data point is a daughter, a mother, a sister—a person with hopes and dreams ." - Melinda Gates
Have a language expert improve your writing
Run a free plagiarism check in 10 minutes, automatically generate references for free.
- Knowledge Base
- Methodology
- Mixed Methods Research | Definition, Guide, & Examples
Mixed Methods Research | Definition, Guide, & Examples
Published on 4 April 2022 by Tegan George . Revised on 25 October 2022.
Mixed methods research combines elements of quantitative research and qualitative research in order to answer your research question . Mixed methods can help you gain a more complete picture than a standalone quantitative or qualitative study, as it integrates benefits of both methods.
Mixed methods research is often used in the behavioral, health, and social sciences, especially in multidisciplinary settings and complex situational or societal research.
- To what extent does the frequency of traffic accidents ( quantitative ) reflect cyclist perceptions of road safety ( qualitative ) in Amsterdam?
- How do student perceptions of their school environment ( qualitative ) relate to differences in test scores ( quantitative ) ?
- How do interviews about job satisfaction at Company X ( qualitative ) help explain year-over-year sales performance and other KPIs ( quantitative ) ?
- How can voter and non-voter beliefs about democracy ( qualitative ) help explain election turnout patterns ( quantitative ) in Town X?
- How do average hospital salary measurements over time (quantitative) help to explain nurse testimonials about job satisfaction (qualitative) ?
Table of contents
When to use mixed methods research, mixed methods research designs, benefits of mixed methods research, disadvantages of mixed methods research, frequently asked questions about mixed methods research.
Mixed methods research may be the right choice if your research process suggests that quantitative or qualitative data alone will not sufficiently answer your research question. There are several common reasons for using mixed methods research:
- Generalisability : Qualitative research usually has a smaller sample size , and thus is not generalisable . In mixed methods research, this comparative weakness is mitigated by the comparative strength of ‘large N’, externally valid quantitative research.
- Contextualisation: Mixing methods allows you to put findings in context and add richer detail to your conclusions. Using qualitative data to illustrate quantitative findings can help ‘put meat on the bones’ of your analysis.
- Credibility: Using different methods to collect data on the same subject can make your results more credible. If the qualitative and quantitative data converge, this strengthens the validity of your conclusions. This process is called triangulation .
As you formulate your research question , try to directly address how qualitative and quantitative methods will be combined in your study. If your research question can be sufficiently answered via standalone quantitative or qualitative analysis, a mixed methods approach may not be the right fit.
Keep in mind that mixed methods research doesn’t just mean collecting both types of data; you need to carefully consider the relationship between the two and how you’ll integrate them into coherent conclusions. Mixed methods can be very challenging to put into practice, so it’s a less common choice than standalone qualitative or qualitative research.
Prevent plagiarism, run a free check.
There are different types of mixed methods research designs . The differences between them relate to the aim of the research, the timing of the data collection , and the importance given to each data type.
As you design your mixed methods study, also keep in mind:
- Your research approach ( inductive vs deductive )
- Your research questions
- What kind of data is already available for you to use
- What kind of data you’re able to collect yourself.
Here are a few of the most common mixed methods designs.
Convergent parallel
In a convergent parallel design, you collect quantitative and qualitative data at the same time and analyse them separately. After both analyses are complete, compare your results to draw overall conclusions.
- On the qualitative side, you analyse cyclist complaints via the city’s database and on social media to find out which areas are perceived as dangerous and why.
- On the quantitative side, you analyse accident reports in the city’s database to find out how frequently accidents occur in different areas of the city.
In an embedded design, you collect and analyse both types of data at the same time, but within a larger quantitative or qualitative design. One type of data is secondary to the other.
This is a good approach to take if you have limited time or resources. You can use an embedded design to strengthen or supplement your conclusions from the primary type of research design.
Explanatory sequential
In an explanatory sequential design, your quantitative data collection and analysis occurs first, followed by qualitative data collection and analysis.
You should use this design if you think your qualitative data will explain and contextualise your quantitative findings.
Exploratory sequential
In an exploratory sequential design, qualitative data collection and analysis occurs first, followed by quantitative data collection and analysis.
You can use this design to first explore initial questions and develop hypotheses. Then you can use the quantitative data to test or confirm your qualitative findings.
‘Best of both worlds’ analysis
Combining the two types of data means you benefit from both the detailed, contextualised insights of qualitative data and the generalisable, externally valid insights of quantitative data. The strengths of one type of data often mitigate the weaknesses of the other.
For example, solely quantitative studies often struggle to incorporate the lived experiences of your participants, so adding qualitative data deepens and enriches your quantitative results.
Solely qualitative studies are often not very generalisable, only reflecting the experiences of your participants, so adding quantitative data can validate your qualitative findings.
Method flexibility
Mixed methods are less tied to disciplines and established research paradigms. They offer more flexibility in designing your research, allowing you to combine aspects of different types of studies to distill the most informative results.
Mixed methods research can also combine theory generation and hypothesis testing within a single study, which is unusual for standalone qualitative or quantitative studies.
Mixed methods research is very labour-intensive. Collecting, analysing, and synthesising two types of data into one research product takes a lot of time and effort, and often involves interdisciplinary teams of researchers rather than individuals. For this reason, mixed methods research has the potential to cost much more than standalone studies.
Differing or conflicting results
If your analysis yields conflicting results, it can be very challenging to know how to interpret them in a mixed methods study. If the quantitative and qualitative results do not agree or you are concerned you may have confounding variables , it can be unclear how to proceed.
Quantitative research deals with numbers and statistics, while qualitative research deals with words and meanings.
Quantitative methods allow you to test a hypothesis by systematically collecting and analysing data, while qualitative methods allow you to explore ideas and experiences in depth.
In mixed methods research , you use both qualitative and quantitative data collection and analysis methods to answer your research question .
Data collection is the systematic process by which observations or measurements are gathered in research. It is used in many different contexts by academics, governments, businesses, and other organisations.
Triangulation in research means using multiple datasets, methods, theories and/or investigators to address a research question. It’s a research strategy that can help you enhance the validity and credibility of your findings.
Triangulation is mainly used in qualitative research , but it’s also commonly applied in quantitative research . Mixed methods research always uses triangulation.
These are four of the most common mixed methods designs :
- Convergent parallel: Quantitative and qualitative data are collected at the same time and analysed separately. After both analyses are complete, compare your results to draw overall conclusions.
- Embedded: Quantitative and qualitative data are collected at the same time, but within a larger quantitative or qualitative design. One type of data is secondary to the other.
- Explanatory sequential: Quantitative data is collected and analysed first, followed by qualitative data. You can use this design if you think your qualitative data will explain and contextualise your quantitative findings.
- Exploratory sequential: Qualitative data is collected and analysed first, followed by quantitative data. You can use this design if you think the quantitative data will confirm or validate your qualitative findings.
Cite this Scribbr article
If you want to cite this source, you can copy and paste the citation or click the ‘Cite this Scribbr article’ button to automatically add the citation to our free Reference Generator.
George, T. (2022, October 25). Mixed Methods Research | Definition, Guide, & Examples. Scribbr. Retrieved 9 April 2024, from https://www.scribbr.co.uk/research-methods/mixed-methods/
Is this article helpful?
Tegan George
- News & Media
- Chemical Biology
- Computational Biology
- Ecosystem Science
- Cancer Biology
- Exposure Science & Pathogen Biology
- Metabolic Inflammatory Diseases
- Advanced Metabolomics
- Mass Spectrometry-Based Measurement Technologies
- Spatial and Single-Cell Proteomics
- Structural Biology
- Biofuels & Bioproducts
- Human Microbiome
- Soil Microbiome
- Synthetic Biology
- Computational Chemistry
- Chemical Separations
- Chemical Physics
- Atmospheric Aerosols
- Human-Earth System Interactions
- Modeling Earth Systems
- Coastal Science
- Plant Science
- Subsurface Science
- Terrestrial Aquatics
- Materials in Extreme Environments
- Precision Materials by Design
- Science of Interfaces
- Friction Stir Welding & Processing
- Dark Matter
- Flavor Physics
- Fusion Energy Science
- Neutrino Physics
- Quantum Information Sciences
- Emergency Response
- AGM Program
- Tools and Capabilities
- Grid Architecture
- Grid Cybersecurity
- Grid Energy Storage
- Earth System Modeling
- Energy System Modeling
- Transmission
- Distribution
- Appliance and Equipment Standards
- Building Energy Codes
- Advanced Building Controls
- Advanced Lighting
- Building-Grid Integration
- Building and Grid Modeling
- Commercial Buildings
- Federal Performance Optimization
- Resilience and Security
- Grid Resilience and Decarbonization
- Building America Solution Center
- Energy Efficient Technology Integration
- Home Energy Score
- Electrochemical Energy Storage
- Flexible Loads and Generation
- Grid Integration, Controls, and Architecture
- Regulation, Policy, and Valuation
- Science Supporting Energy Storage
- Chemical Energy Storage
- Waste Processing
- Radiation Measurement
- Environmental Remediation
- Subsurface Energy Systems
- Carbon Capture
- Carbon Storage
- Carbon Utilization
- Advanced Hydrocarbon Conversion
- Fuel Cycle Research
- Advanced Reactors
- Reactor Operations
- Reactor Licensing
- Solar Energy
- Wind Resource Characterization
- Wildlife and Wind
- Community Values and Ocean Co-Use
- Wind Systems Integration
- Wind Data Management
- Distributed Wind
- Energy Equity & Health
- Environmental Monitoring for Marine Energy
- Marine Biofouling and Corrosion
- Marine Energy Resource Characterization
- Testing for Marine Energy
- The Blue Economy
- Environmental Performance of Hydropower
- Hydropower Cybersecurity and Digitalization
- Hydropower and the Electric Grid
- Materials Science for Hydropower
- Pumped Storage Hydropower
- Water + Hydropower Planning
- Grid Integration of Renewable Energy
- Geothermal Energy
- Algal Biofuels
- Aviation Biofuels
- Waste-to-Energy and Products
- Hydrogen & Fuel Cells
- Emission Control
- Energy-Efficient Mobility Systems
- Lightweight Materials
- Vehicle Electrification
- Vehicle Grid Integration
- Discovery and Insight
- Proactive Defense
- Trusted Systems
- Nuclear Material Science
- Radiological & Nuclear Detection
- Nuclear Forensics
- Ultra-Sensitive Nuclear Measurements
- Nuclear Explosion Monitoring
- Global Nuclear & Radiological Security
- Disaster Recovery
- Global Collaborations
- Legislative and Regulatory Analysis
- Technical Training
- Additive Manufacturing
- Deployed Technologies
- Rapid Prototyping
- Systems Engineering
- 5G Security
- RF Signal Detection & Exploitation
- Climate Security
- Internet of Things
- Maritime Security
- Contraband Detection
- Pathogen Science & Detection
- Explosives Detection
- Threat-Agnostic Biodefense
- Lab Objectives
- Publications & Reports
- Featured Research
- Artificial Intelligence
- Graph and Data Analytics
- Software Engineering
- Computational Mathematics & Statistics
- High-Performance Computing
- Visual Analytics
- Diversity, Equity, Inclusion & Accessibility
- Lab Leadership
- Lab Fellows
- Staff Accomplishments
- Undergraduate Students
- Graduate Students
- Post-graduate Students
- University Faculty
- University Partnerships
- K-12 Educators and Students
- STEM Workforce Development
- STEM Outreach
- Meet the Team
- Internships
- Regional Impact
- Philanthropy
- Volunteering
- Available Technologies
- Industry Partnerships
- Licensing & Technology Transfer
- Entrepreneurial Leave
- Atmospheric Radiation Measurement User Facility
- Electricity Infrastructure Operations Center
- Energy Sciences Center
- Environmental Molecular Sciences Laboratory
- Grid Storage Launchpad
- Institute for Integrated Catalysis
- Interdiction Technology and Integration Laboratory
- PNNL Portland Research Center
- PNNL Seattle Research Center
- PNNL-Sequim (Marine and Coastal Research)
- Radiochemical Processing Laboratory
- Shallow Underground Laboratory
How to Identify Good Coupling Methods With Error Analysis
An error analysis framework for evaluating and developing process coupling methods for complex computational models
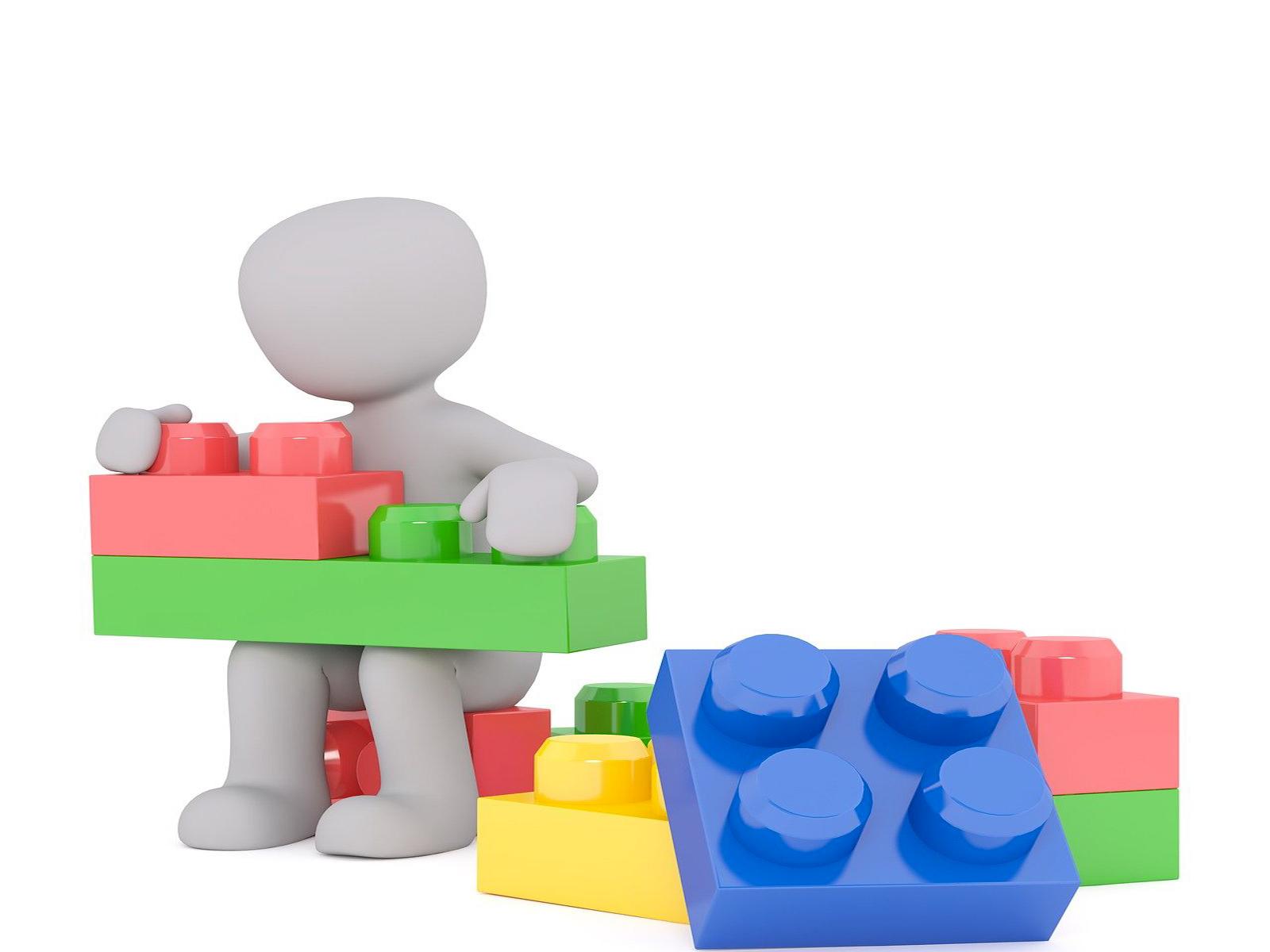
Researchers introduced a framework that helps scientists evaluate methods for coupling different processes in complex computational models, combining mathematical analysis with an intuitive interpretation.
(Image by Peggy_Marco | Pixabay )
The Science
The simulation of complex systems like the global atmosphere requires coupling of many physics processes. These include, for example, atmospheric motions, cloud and rain formation processes, as well as pollutant transport and transformation. Atmospheric models typically couple these processes by adopting methods found in earlier models or textbooks, but the implications of the methods and their impacts on the simulation results are often unclear. This study introduces a mathematically rigorous analysis framework that provides insight into the features and implications of coupling method choices. As an example, the framework is applied to the Energy Exascale Earth System Model version 1 (E3SMv1) to evaluate two coupling methods used for aerosols.
The framework enables scientists to evaluate impacts of coupling method choices without implementing all possible methods or deriving lengthy mathematical expressions tailored to each method’s details. Furthermore, the framework provides an accuracy assessment for each interacting process. This can help avoid incidental error cancelation and increase the confidence in estimating the overall accuracy of a numerical solution.
The need to choose, update, and evaluate coupling methods during the development of global atmospheric models for weather, climate, and Earth system prediction prompted researchers to launch this study. Many of the existing mathematical analyses focused on details of specific physics problems and numerical models; some analyses were more general but produced mathematical expressions that were hard to interpret by physical scientists. This study introduces an analysis framework that is general and intuitive to use. The framework ignores some details of the mathematical formulation and numerical algorithms used for each interacting process and focuses on coupling issues. The study starts by describing two fundamental sources of numerical error as two processes are coupled. It then demonstrates how these insights can be applied through simple arithmetic to derive numerical errors in multi-process problems. Two coupling methods used for the aerosol life cycles in E3SMv1 are analyzed as a concrete example of applying the tool.
Hui Wan, [email protected] , coauthor at Pacific Northwest National Laboratory and project principal investigator
Christopher J. Vogl, [email protected] , corresponding author at Lawrence Livermore National Laboratory
Carol S. Woodward, [email protected] , coauthor at Lawrence Livermore National Laboratory
The study was supported by the DOE Office of Science’s Advanced Scientific Computing Research program and Biological and Environmental Research program, under the Scientific Discovery through Advanced Computing (SciDAC) program, via a partnership in Earth system model development. The computational resources used in this study were provided by the National Energy Research Scientific Computing Center, a U.S. Department of Energy (DOE) Office of Science user facility located at Lawrence Berkeley National Laboratory, and by the Compy supercomputer operated for DOE by Pacific Northwest National Laboratory.
Published: April 9, 2024
C.J. Vogl, H. Wan, C.S. Woodward, Q.M. Bui, “ Numerical coupling of aerosol emissions, dry removal, and turbulent mixing in the E3SM Atmosphere Model version 1 (EAMv1) – Part 2: A semi-discrete error analysis framework for assessing coupling schemes .” Geoscientific Model Development 17, 3, 1409-1428 (2024). [DOI: 10.5194/gmd-17-1409-2024]
Research topics
- Frontiers in Medicine
- Healthcare Professions Education
- Research Topics
Impact of Technology on Human Behaviors in Medical Professions Education
Total Downloads
Total Views and Downloads
About this Research Topic
Human behaviors are essential in understanding how individuals engage in medical science academic activities. Healthcare systems across the globe have witnessed a significant shift in recent years by integrating technology in innovating new methods and practices to improve educational practices. Therefore, ...
Keywords : healthcare education, medical education, teachers’ behavior, students’ behavior, human behavior, technology in medical sciences, program development, curriculum development, teacher and student performance
Important Note : All contributions to this Research Topic must be within the scope of the section and journal to which they are submitted, as defined in their mission statements. Frontiers reserves the right to guide an out-of-scope manuscript to a more suitable section or journal at any stage of peer review.
Topic Editors
Topic coordinators, recent articles, submission deadlines, participating journals.
Manuscripts can be submitted to this Research Topic via the following journals:
total views
- Demographics
No records found
total views article views downloads topic views
Top countries
Top referring sites, about frontiers research topics.
With their unique mixes of varied contributions from Original Research to Review Articles, Research Topics unify the most influential researchers, the latest key findings and historical advances in a hot research area! Find out more on how to host your own Frontiers Research Topic or contribute to one as an author.
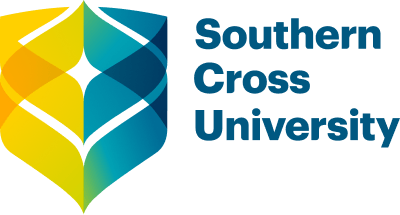
Main Navigation
/prod01/channel_8/media/scu-dep/current-students/images/Coffs-harbour_student-group_20220616_33.jpg)
- Accept offer and enrol
- Current Students
Personalise your experience
Did you mean..., diploma of arts and social sciences, art/science collaboration wins waterhouse natural science art prize, unit of study hlth6007 health research project a (2025).
Future students: T: 1800 626 481 E: Email your enquiry here
Current students: Contact: Faculty of Health
Students studying at an education collaboration: Please contact your relevant institution
updated - DO NOT REMOVE THIS LINE 6:05 AM on Fri, 12 April
Show me unit information for year
Unit snapshot.
PG Coursework Unit
Credit points
Faculty & college.
Faculty of Health
Unit description
Learners develop a proposed research project, from conception of an idea to preparation of a study protocol. Learners discover how to formulate a research question, reviewing the literature, critically appraise the literature, develop a research justification statement, and define the parameters of a research project (including the aims, objectives, design, outcomes, data collection methods and data analysis procedures). Learners also realise how to prepare a study protocol that may be suitable for publication in a peer-reviewed journal.
Unit content
- Research project management
- Establishing a clear, succinct research question
- Reviewing and appraising the literature and preparing a justification statement
- Developing a research protocol
- Data collection methods
- Data analysis procedures
Availabilities
Learning outcomes.
Unit Learning Outcomes express learning achievement in terms of what a student should know, understand and be able to do on completion of a unit. These outcomes are aligned with the graduate attributes . The unit learning outcomes and graduate attributes are also the basis of evaluating prior learning.
On completion of this unit, students should be able to:
apply the principles and strategies for effective research project management to a planned research project
develop a clear and succinct research question and/or hypothesis
compose a well-supported, current and persuasive research justification statement
formulate a comprehensive research protocol outlining data collection and analysis procedures aligned to project research question and/or hypothesis
Teaching and assessment
Online (term), prescribed learning resources.
- Prescribed text information is not currently available.
- Prescribed resources/equipment information is not currently available.
Prescribed Learning Resources may change in future Teaching Periods.
Fee information
Commonwealth Supported courses For information regarding Student Contribution Amounts please visit the Student Contribution Amounts .
Fee paying courses For postgraduate or undergraduate full-fee paying courses please check Domestic Postgraduate Fees OR Domestic Undergraduate Fees .
International
Please check the international course and fee list to determine the relevant fees.
Courses that offer this unit
Master of osteopathic medicine (2024), master of osteopathic medicine (2025), master of advanced naturopathic medicine (2025), master of advanced naturopathic medicine (2024), master of lifestyle medicine (2024), master of lifestyle medicine (2025), master of naturopathic medicine (2025), master of naturopathic medicine (2024), any questions we'd love to help.
Read our research on: Gun Policy | International Conflict | Election 2024
Regions & Countries
7 facts about americans and taxes.
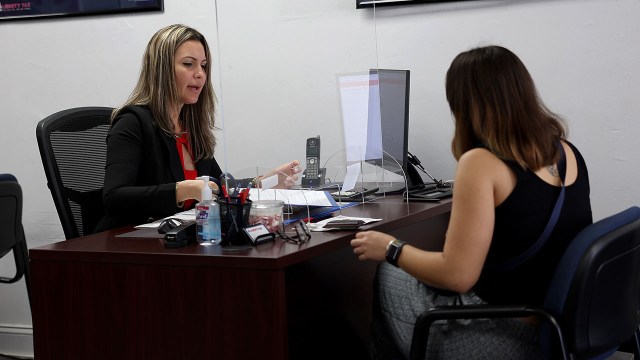
Spring reliably brings a whirlwind of number-crunching and form-filing as Americans finish their tax returns. Altogether, the IRS expects to process more than 160 million individual and business tax returns this season.
Ahead of Tax Day on April 15, here are seven facts about Americans and federal taxes, drawn from Pew Research Center surveys and analyses of federal data.
Ahead of Tax Day 2024, Pew Research Center sought to understand Americans’ views of the federal tax system and outline some of its features.
The public opinion data in this analysis comes from Pew Research Center surveys. Links to these surveys, including details about their methodologies, are available in the text.
The external data comes from the U.S. Office of Management and Budget and the IRS Data Book . Data is reported by fiscal year, which for the federal government begins Oct. 1 and ends Sept. 30. For example, fiscal 2024 began Oct. 1, 2023, and ends Sept. 30, 2024.
A majority of Americans feel that corporations and wealthy people don’t pay their fair share in taxes, according to a Center survey from spring 2023 . About six-in-ten U.S. adults say they’re bothered a lot by the feeling that some corporations (61%) and some wealthy people (60%) don’t pay their fair share.
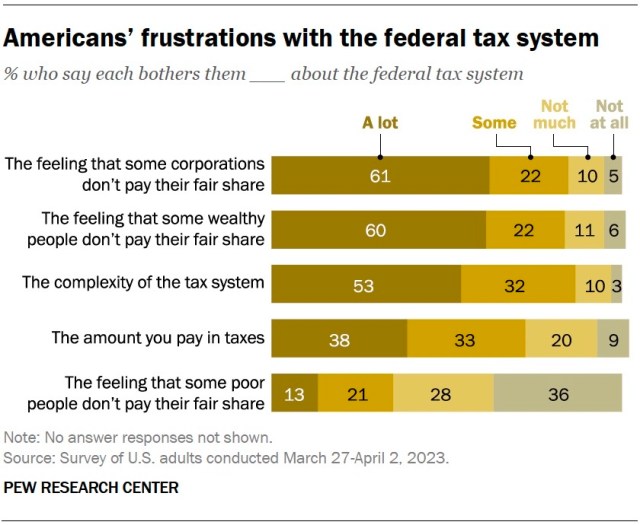
Democrats are far more likely than Republicans to feel this way. Among Democrats and Democratic-leaning independents, about three-quarters say they’re bothered a lot by the feeling that some corporations (77%) and some wealthy people (77%) don’t pay their fair share. Much smaller shares of Republicans and GOP leaners share these views (46% say this about corporations and 43% about the wealthy).
Meanwhile, about two-thirds of Americans (65%) support raising tax rates on large businesses and corporations, and a similar share (61%) support raising tax rates on households with annual incomes over $400,000. Democrats are much more likely than Republicans to say these tax rates should increase.
Just over half of U.S. adults feel they personally pay more than what is fair, considering what they get in return from the federal government, according to the same survey.
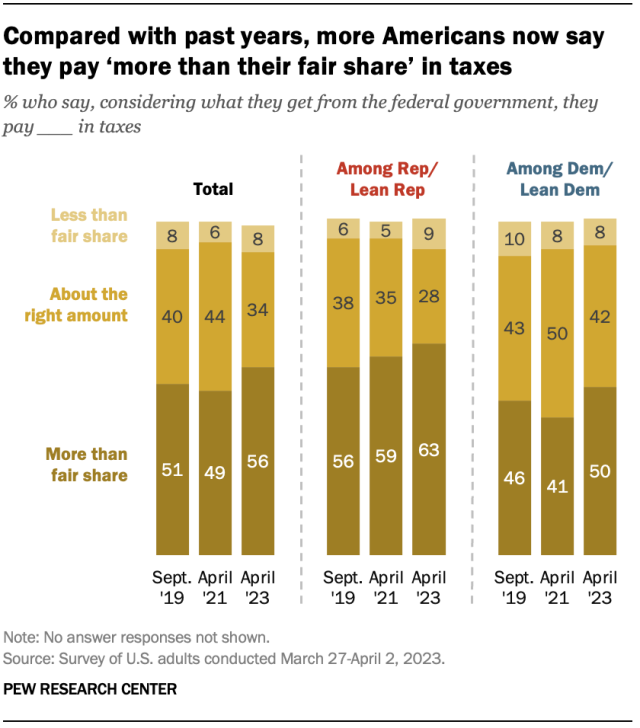
This sentiment has grown more widespread in recent years: 56% of Americans now say they pay more than their fair share in taxes, up from 49% in 2021. Roughly a third (34%) say they pay about the right amount, and 8% say they pay less than their fair share.
Republicans are more likely than Democrats to say they pay more than their fair share (63% vs. 50%), though the share of Democrats who feel this way has risen since 2021. (The share among Republicans is statistically unchanged from 2021.)
Many Americans are frustrated by the complexity of the federal tax system, according to the same survey. About half (53%) say its complexity bothers them a lot. Of the aspects of the federal tax system that we asked about, this was the top frustration among Republicans – 59% say it bothers them a lot, compared with 49% of Democrats.
Undeniably, the federal tax code is a massive document, and it has only gotten longer over time. The printed 2022 edition of the Internal Revenue Code clocks in at 4,192 pages, excluding front matter. Income tax law alone accounts for over half of those pages (2,544).
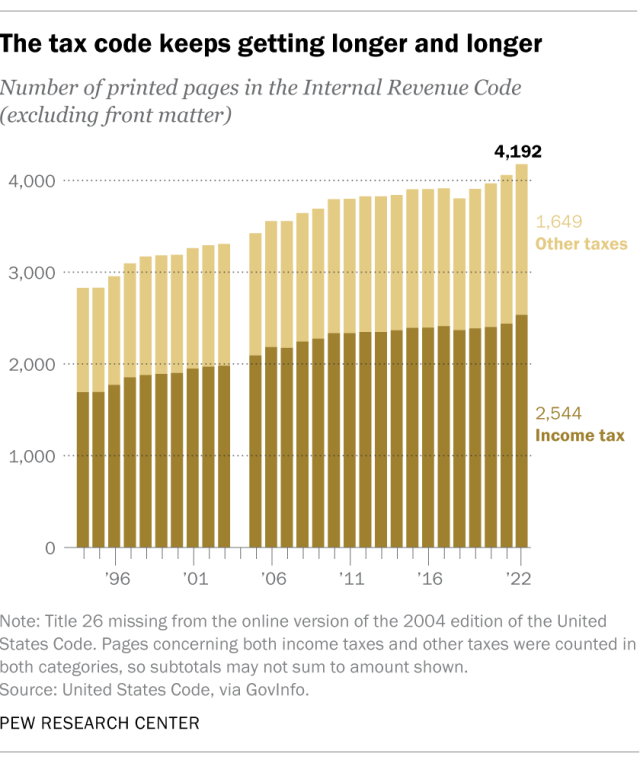
The public is divided in its views of the IRS. In a separate spring 2023 Center survey , 51% of Americans said they have an unfavorable opinion of the government tax agency, while 42% had a favorable view of the IRS. Still, of the 16 federal agencies and departments we asked about, the IRS was among the least popular on the list.
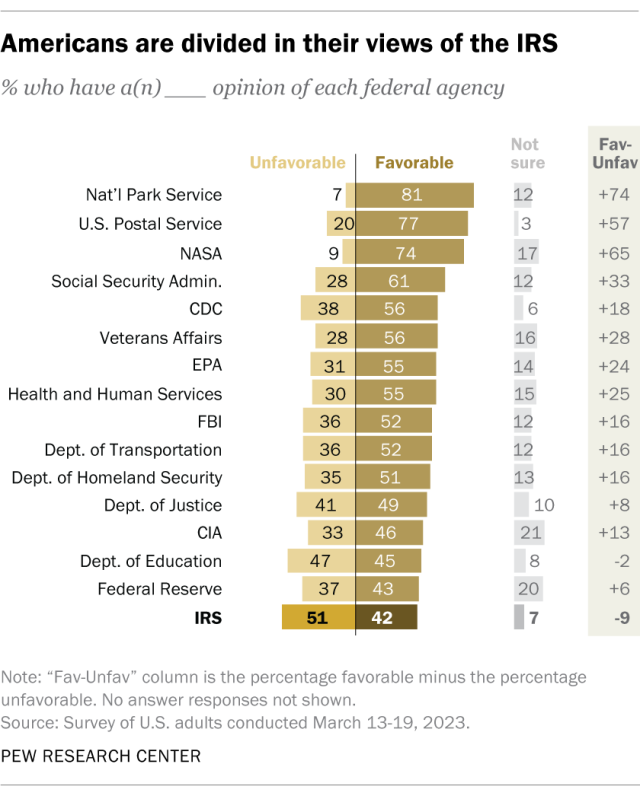
Views of the IRS differ greatly by party:
- Among Republicans, 29% have a favorable view and 64% have an unfavorable view.
- Among Democrats, it’s 53% favorable and 40% unfavorable.
On balance, Democrats offer much more positive opinions than Republicans when it comes to most of the federal agencies we asked about. Even so, the IRS ranks near the bottom of their list.
Individual income taxes are by far the government’s largest single source of revenue, according to estimates from the Office of Management and Budget (OMB).
The federal government expects to collect about $2.5 trillion in individual income taxes in fiscal year 2024. That accounts for nearly half (49%) of its total estimated receipts for the year. The next largest chunk comes from Social Security taxes (including those for disability and retirement programs), which are projected to pull in $1.2 trillion this fiscal year (24%).
By comparison, corporate income taxes are estimated to bring in $612.8 billion, or 12% of this fiscal year’s federal receipts. And excise taxes – which include things like transportation trust fund revenue and taxes on alcohol, tobacco and crude oil – are expected to come to $99.7 billion, or 2% of receipts.
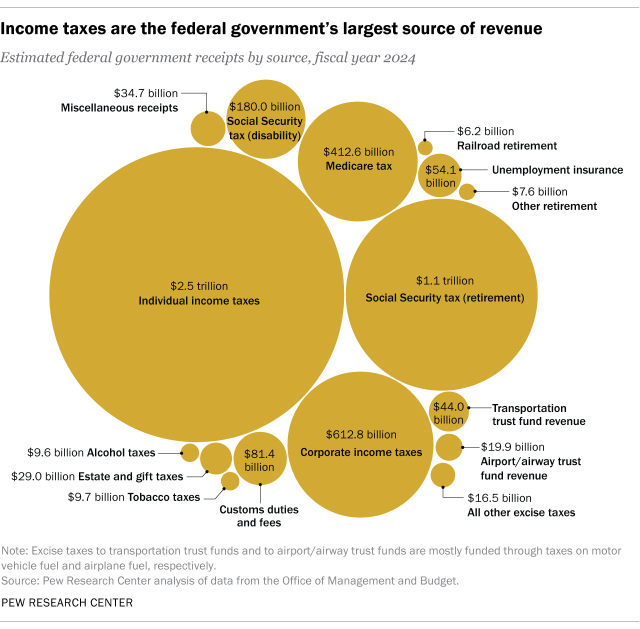
American tax dollars mostly go to social services. Human services – including education, health, Social Security, Medicare, income security and veterans benefits – together will account for 66% ($4.6 trillion) of federal government spending in fiscal 2024, according to OMB estimates.
An estimated 13% ($907.7 billion) will go toward defense spending. Another 13% ($888.6 billion) will repay net interest on government debt, and 10% ($726.9 billion) will fund all other functions, including energy, transportation, agriculture and more.
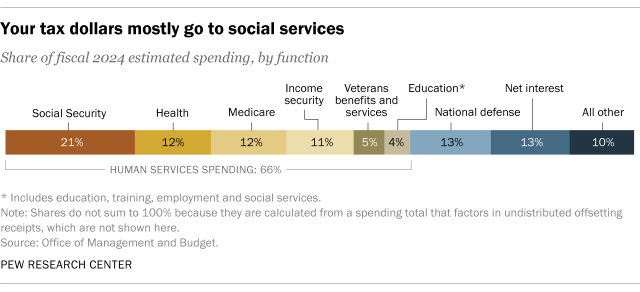
Related: 6 facts about Americans’ views of government spending and the deficit
The vast majority of Americans e-file their taxes, according to IRS data . In fiscal 2022, 150.6 million individual federal income tax returns were filed electronically, accounting for 94% of all individual filings that year.
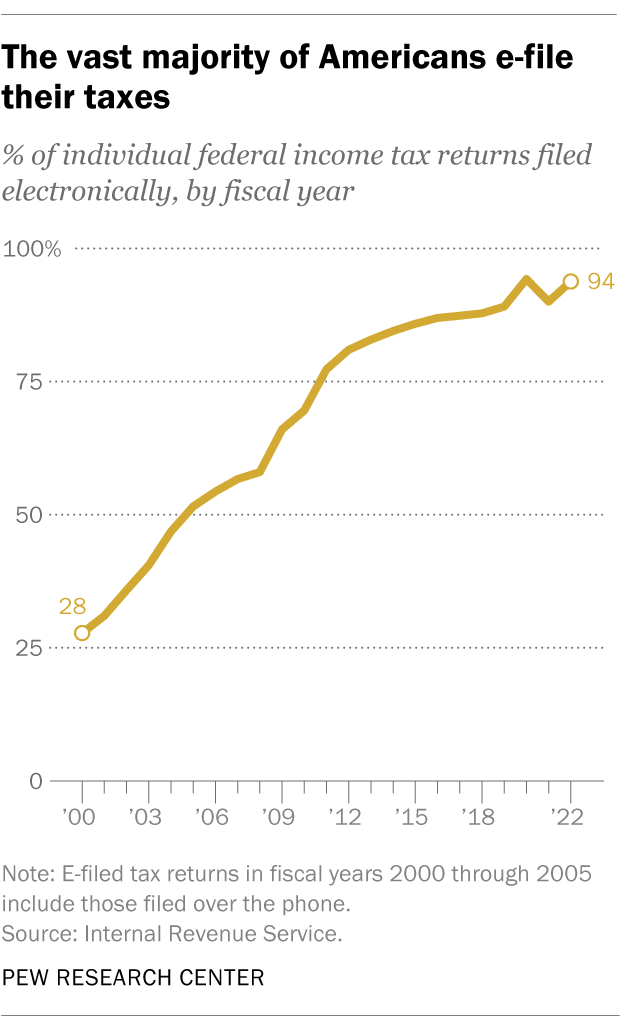
Unsurprisingly, e-filing has become more popular since the turn of the century. Fiscal 2000, the earliest year for which comparable data is available, saw 35.4 million individual income tax returns filed electronically (including those filed over the phone). These accounted for just 28% of individual filings that year.
By fiscal 2005, more than half of individual income tax returns (52%) were filed electronically.
Note: This is an update combining information from two posts originally published in 2014 and 2015.
Sign up for our weekly newsletter
Fresh data delivered Saturday mornings
Top tax frustrations for Americans: The feeling that some corporations, wealthy people don’t pay fair share
Growing partisan divide over fairness of the nation’s tax system, public has mixed expectations for new tax law, most popular.
About Pew Research Center Pew Research Center is a nonpartisan fact tank that informs the public about the issues, attitudes and trends shaping the world. It conducts public opinion polling, demographic research, media content analysis and other empirical social science research. Pew Research Center does not take policy positions. It is a subsidiary of The Pew Charitable Trusts .
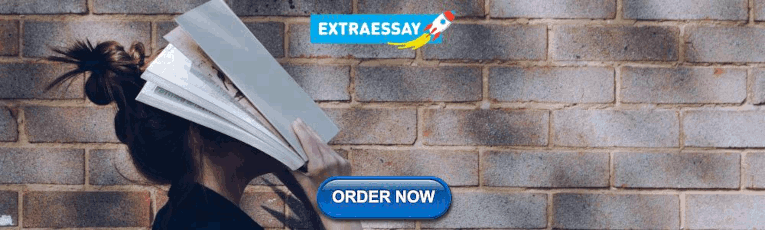
IMAGES
VIDEO
COMMENTS
However, discussion has begun concerning the use of mixed methods questions in studies and also how to design them (see Creswell & Plano Clark, 2007; Tashakkori & Creswell, 2007). A strong mixed methods study should start with a mixed methods research question, to shape the methods and the overall design of a study. Because a mixed methods
Mixed methods research combines elements of quantitative research and qualitative research in order to answer your research question. Mixed methods can help you gain a more complete picture than a standalone quantitative or qualitative study, as it integrates benefits of both methods. Mixed methods research is often used in the behavioral ...
A mixed methods research design is an approach to collecting and analyzing both qualitative and quantitative data in a single study. Mixed methods designs allow for method flexibility and can provide differing and even conflicting results. Examples of mixed methods research designs include convergent parallel, explanatory sequential, and ...
We systematically reviewed the literature in the field of instructional design to understand how studies framed research questions. We adapted the Brereton et al. (2007) three phases including plan and review as phase one, conduct review as phase two, and document review as phase three (Figure 1). We found the steps listed in the model ...
Mixed methods approaches allows researchers to use a diversity of methods, combining inductive and deductive thinking, and offsetting limitations of exclusively quantitative and qualitative research through a complementary approach that maximizes strengths of each data type and facilitates a more comprehensive understanding of health issues and ...
Mixed‐methods research, or multi‐strategy designs, 1 can be defined as 'the collection, analysis and integration of both qualitative and quantitative data in a single study': 2 semi‐structured interviews and workplace measures (e.g. attendance data) might be undertaken concurrently to gain a multifaceted perspective on a particular ...
Proponents of mixed methods research suggest that the purist view, that quantitative and qualitative approaches cannot be merged, poses a threat to the advancement of science (Onwuegbuzie and Leech, 2005) and that while epistemological and ontological commitments may be associated with certain research methods, the connections are not necessary ...
Combining methods in social scientific research has recently gained momentum through a research strand called Mixed Methods Research (MMR). This approach, which explicitly aims to offer a framework for combining methods, has rapidly spread through the social and behavioural sciences, and this article offers an analysis of the approach from a field theoretical perspective. After a brief outline ...
Quantitative dominant [or quantitatively driven] mixed methods research is the type of mixed research in which one relies on a quantitative, postpositivist view of the research process, while concurrently recognizing that the addition of qualitative data and approaches are likely to benefit most research projects. (p.
Philosophy in mixed methods research: Mixed methods researchers use and often make explicit diverse philosophical positions. These positions often are referred to as dialectal stances that bridge postpositivist and social constructivist worldviews, pragmatic perspectives, and transformative perspectives (Greene, 2007). For example,
SPIDER is a search strategy for finding research to answer a mixed-method qualitative research question.. Sample: Sample is similar to Patient/Population of PICO.This is the group of people you are interesting in studying qualitatively. For example, in the qualitative research question, "What are the barriers felt by clinicians that lead to the reluctance to use EBP in practice?", S = clinicians
Abstract. Mixed methods research is becoming increasingly popular and is widely acknowledged as a means of achieving a more complex understanding of research problems. Combining both the in-depth, contextual views of qualitative research with the broader generalizations of larger population quantitative approaches, mixed methods research can be ...
Linking Research Questions to Mixed Methods Data Analysis Procedures 1 . Abstract . The purpose of this paper is to discuss the development of research questions in mixed methods studies. First, we discuss the ways that the goal of the study, the research objective(s), and the research purpose shape the formation of research questions.
Flexibility: Mixed methods research is flexible, allowing researchers to adapt the research design and methods as needed to best address the research question. Validity: Mixed methods research can increase the validity of the research by using multiple methods to measure the same concept. Generalizability: Mixed methods research can improve the ...
Guidelines for Conducting Mixed-methods Research: An Extension and Illustration. This paper extends the guidelines of Venkatesh et al. (2013) for mixed-methods research by identifying and integrating variations in mixed- methods research and develops a decision tree to map the flow and relationship among the design strategies.
Mixed methods research combines qualitative and quantitative data to provide a comprehensive analysis of research questions. In data analytics, this approach can be particularly powerful, offering ...
Mixed methods research is a popular approach used to understand persistent and complex problems related to quality and safety, such as reasons why interventions are not implemented as intended or explaining differential outcomes. However, the quality and rigour of mixed methods research proposals and publications often miss opportunities for integration, which is the core of mixed methods.
This study provides an overview of the shifting epistemological terrain regarding methodological approaches to the research process in the field of communication and discusses how a mixed-methods design could improve methods comprehension in undergraduate education. The authors describe how they incorporated a mixed-methods project into the curriculum and discuss the student experience of ...
Objective: This study aims to provide comprehensive primary mixed methods data on the patient experience of barriers to digital health access, with a focus on the digital health divide. Methods: We applied an exploratory mixed methods design to ensure that our survey was primarily shaped by the experiences of our interviewees.
Summary. Mixed methods research is the use of quantitative and qualitative methods in a single study or series of studies. It is an emergent methodology which is increasingly used by health researchers, especially within health services research. There is a growing literature on the theory, design and critical appraisal of mixed methods research.
Mixed Methods & Theoretically Informed Research. We embrace sociological, educational, organizational change, and other bodies of scholarly research & theory to inform and contextualize our work and engage multiple methods to enable us to answer pressing questions with thoroughness and rigor to drive institutional action forward.
Mixed methods research combines elements of quantitative research and qualitative research in order to answer your research question. Mixed methods can help you gain a more complete picture than a standalone quantitative or qualitative study, as it integrates benefits of both methods. Mixed methods research is often used in the behavioral ...
This study introduces a mathematically rigorous analysis framework that provides insight into the features and implications of coupling method choices. As an example, the framework is applied to the Energy Exascale Earth System Model version 1 (E3SMv1) to evaluate two coupling methods used for aerosols. The Impact
Human behaviors are essential in understanding how individuals engage in medical science academic activities. Healthcare systems across the globe have witnessed a significant shift in recent years by integrating technology in innovating new methods and practices to improve educational practices. Therefore, pedagogical practices in medical sciences are expected to be consistent with the current ...
Learners develop a proposed research project, from conception of an idea to preparation of a study protocol. Learners discover how to formulate a research question, reviewing the literature, critically appraise the literature, develop a research justification statement, and define the parameters of a research project (including the aims, objectives, design, outcomes, data collection methods ...
This sentiment has grown more widespread in recent years: 56% of Americans now say they pay more than their fair share in taxes, up from 49% in 2021. Roughly a third (34%) say they pay about the right amount, and 8% say they pay less than their fair share. Republicans are more likely than Democrats to say they pay more than their fair share (63 ...