
An official website of the United States government
The .gov means it’s official. Federal government websites often end in .gov or .mil. Before sharing sensitive information, make sure you’re on a federal government site.
The site is secure. The https:// ensures that you are connecting to the official website and that any information you provide is encrypted and transmitted securely.
- Publications
- Account settings
Preview improvements coming to the PMC website in October 2024. Learn More or Try it out now .
- Advanced Search
- Journal List
- Curr Ther Res Clin Exp
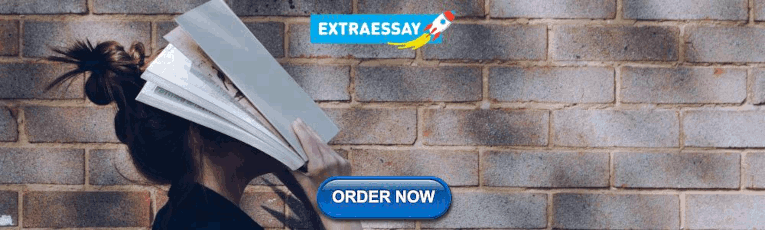
Improving Risk Assessment in Clinical Trials: Toward a Systematic Risk-Based Monitoring Approach
Firas fneish.
1 Institute of Cell Biology and Biophysics, Department of Biostatistics, Leibniz University Hannover, 30419 Hannover, Germany
Frank Schaarschmidt
Gerhard fortwengel.
2 Faculty III Media Information and Design, Hochschule Hannover, 30539 Hannover, Germany
Regulatory authorities have encouraged the usage of a monitoring (RBM) system in clinical trials before trial initiation for detection of potential risks and inclusion of a mitigation plan in the monitoring strategy. Several RBM tools were developed after the International Council for Harmonization gave sponsors the flexibility to initiate an approach to enhance quality management in a clinical trial. However, various studies have demonstrated the need for improvement of the available RBM tools as each does not provide a comprehensive overview of the characteristics, focus, and application.
This research lays out a rationale for a risk methodology assessment (RMA) within the RBM system. The core purpose of RMA is to deliver a scientifically based evaluation and decision of any potential risk in a clinical trial. Thereby, a monitoring plan can be developed to elude prior identified risk outcome.
To demonstrate RMA's theoretical approach in practice, a Shiny web application (R Foundation for Statistical Computing) was designed to describe the assessment process of risk analysis and visualization tools that eventually aid in focusing monitoring activities.
RMA focuses on the identification of an individual risk and visualizes its weight on the trial. The scoring algorithm of the presented approach computes the assessment of the individual risk in a radar plot and computes the overall score of the trial. Moreover, RMA's novelty lies in its ability to decrease biased decision making during risk assessment by categorizing risk influence and detectability; a characteristic pivotal to serve RBM in assessing risks, and in contributing to a better understanding in the monitoring technique necessary for developing a functional monitoring plan.
Future research should focus on validating the power of RMAs to demonstrate its efficiency. This would facilitate the process of characterizing the strengths and weaknesses of RMA in practice.
Introduction
Clinical trials are conventionally monitored by source data verification that is costly, requires ample resources, and exhibits several limitations. 1 , 2 The International Council for Harmonization (ICH) has provided sponsors with the flexibility to initiate a novel approach called risk-based monitoring (RBM) to enhance quality management in a clinical trial. 3 Regulatory authorities such as European Medicines Agency (EMA) define RBM as a systematic process that involves identification, assessment, controlling, communicating, and reviewing the risks in a clinical trial before its initiation. 4 With this methodology, not only would the occurrence of the assessed risk be prevented, but it would also minimize onsite monitoring duties to some extent. Following the ICH recommendation for approach utilization, several RBM tools were developed. The available RBM tools have been identified and summarized based on their structural approaches, similarities, and differences. 5 Additionally, noncommercial RBM tools were compared in their application on real clinical trial protocols to assess the overall risk level of each protocol by each tool; furthermore, each noncommercial RBM tool was compared directly with the Transcelerate RBM tool (commonly accepted as the standard in pharmaceutical industry) to investigate the risk category and risk coverage in each. 6
These studies reveal distinct approaches employed by the available RBM tools to assess a certain risk, demonstrate the unique assessment of each RBM Tool to the same clinical trial protocol, and exhibit the different risks investigated within each RBM tool. The Food and Drug Administration (FDA) encourages all clinical trials regardless of the phase to implement RBM. Currently a standardized RBM approach for clinical trials is lacking, 7 which presents a challenge to implement RBM by the industry. 8 Our objective is to fill the gap by presenting a systematic risk analysis in clinical trials to standardize RBM. To have an efficient RBM tool, a potent risk assessment has to be performed first. For this reason, we propose a novel methodology and a robust algorithm to assess any risk in a clinical trial. The methodology can be implemented on any clinical trial regardless of the phase and complexity. Moreover, the algorithm aids the assessor in the decision-making process of monitoring technique needed and monitoring level required during the development of the monitoring plan.
Risk Identification Process
The quality feature of an RBM system entails risk assessment of a study and a mitigation plan that details a monitoring strategy for the concerned trial. However, the crucial question arising is how to define a certain risk.
The presence of varying risk criteria covered and examined within the risk assessment by each RBM tool suggests the need to restructure the definition of a certain risk. A risk is defined as the unsolicited outcome of a certain process. Any event that is likely to have a negative influence on the trial should be counted as a risk. The identified risk must be assessed through its influence on the safety of the human participant, trial integrity, the chance of its occurrence, and the ease by which it can be detected. Several systems such as Delphi 9 or SWOT analysis 10 can be oriented toward identifying risks in clinical trials. The Delphi method is a process that utilizes a questionnaire circulated among experts such as clinical research associates, statisticians, clinical investigators, sponsors, and any member involved in a clinical trial stage. 9 SWOT analysis is yet another strategy that aids organizations to pinpoint strengths, weaknesses, opportunities, and threats to a business or a project planning, in this case a clinical trial. 10 The application of both methods is simple, and their outcome is highly dependent on the diverse groups involved. 11 Another approach is utilizing risk summaries from monitoring reports of completed clinical trials; however, it is unlikely to access those reports as they are only accessible by the sponsors. 11
An Ideal RBM System
Clinical trial sponsors along with the involved clinical trial members are responsible for guaranteeing the safety and well-being of the human participants, their rights, and the data quality. 12 The regulatory authorities require sponsors to ensure proper monitoring during the initiation and progress of a clinical trial. 13 RBM is expected to be an imperative tool in guiding the sponsor to identify and mitigate risks. 14 Similarly, EMA's reflection article concerning risk-based management demonstrates that a risk-based approach is needed to enhance quality management of clinical trials. 15 To date, FDA's guidance on RBM approach is divided into 3 parts, the detection of critical data and processes, the risk assessment categorization tool, and developing an appropriate monitoring plan following the risk-based approach. 16 Such a revolutionized technology played a huge role in achieving RBM in the field of mitigation monitoring techniques developed as remote monitoring. 17 The focus of any mitigation plan is shaped by the outcome of a risk assessment. Although 100% source data verification can certainly be reduced by the available mitigation plans, it does not reflect the focus of the personnel carrying out onsite monitoring activities, as the FDA entailed. 16
Proposed Risk Methodology Assessment in Clinical Trials
An RBM tool that covers risks in any clinical trial including a monitoring plan of appropriate technique is still missing. 18 Additionally, there still exists ambiguity in the assessment methodology behind a certain risk. In this study we propose a novel risk methodology assessment (RMA) that enables the user to visualize the assessment of individual or overall risks present in a specific trial. RMA follows the concept of failure mode and effect analysis, specifically a systematic failure mode and effect analysis. 19 The focus is on system-related deficiencies in which hazards are identified, studied, and prevented.
The fundamental process is to initially focus on the most common faults detected in previous trials. For this reason, the RMA approach includes the frequent findings detected by Good Clinical Practice- Inspectors Working Group (EMA GCP-IWG) report. 20 The EMA GCP-IWG objective is to harmonize and coordinate GCP activities in the European Union. The annual report, which emphasizes GCP practice in the European Union, can be used as a reference for risk identification. The report sheds light on the number of inspections done routinely and non-routinely to active clinical trial sites and reports deficiencies detected in the trials.
Our article follows the recommendation of the ICH to favor risk based monitoring by providing a methodology of risk assessment that evaluates the occurrence likelihood of a risk, summarizes the extent of monitoring required with the help of a radar plot-based visualization of said risk and hence aids in the decision making of the mitigation step to be put forth. RMA does not suggest a prevention strategy due to the miscellaneous outcome of a certain risk in an individual trial. For instance, a risk associated with investigational medicinal products in a Phase I trial might have a higher impact than a Phase III trial. The anticipation step and the overall mitigation plan should be developed by the stakeholders responsible for the planning procedure. The FDA specifically highlights the sponsors’ responsibility to have a mitigation approach for defined risks irrespective of the implemented risk assessment technique. 14 Figure 1 shows RMA's approach to identify, assess, and form a mitigation plan.

Flowchart of risk methodology assessment (RMA) risk assessment process before and after trial initiation. This flowchart shows the methodological approach of risk-based monitoring (RBM). Following risk identification, each risk is evaluated and assigned a mitigation technique. Following the assessment, stakeholders develop the monitoring plan based on the assessment. The assessment must be repeated if any amendments were established to the protocol or when unidentified faults are discovered.
Theoretical Implementation of RMA Methodology
Each clinical trial is based on an explicit study protocol outlining the study end point(s), study procedures, medical investigations, and so on, which necessitate appropriate consideration during risk identification. The results presented by the GCP-IWG annual report signify the definite complications that a monitoring team can detect during a routine site visit. For this reason, the identification process of potential risks could be derived from GCP-IWG report as a starting point. Accordingly, a risk assessment should reflect the detected faults as risks that must be assessed before trial initiation.
A risk assessment system should consist of components in which a risk is identified, assessed, visualized for its monitoring level, and classified into the type of monitoring required. The assessment process is classified based on the FDA's recommendation of impact, probability, and detectability. 7 Nonetheless it does not indicate standards each category should be assessed on. It is left to the stakeholders to decide the appropriate decision process. In the presented methodology we propose defined standards required for impact and detectability measurements.
According to the ICH-GCP guidelines, 21 monitoring is conducted to ensure the well-being/safety of participants, the reliability of data and compliance with GCP/protocol guidelines. A risk that does not affect at least 1 of these criteria must not be deliberated as a risk that can be covered by RBM monitoring. The individual criteria should be differentially weighted based on the critical aspect resulting from each separately. For instance, a risk affecting the well-being/safety of participants alone will have a higher impact than a risk affecting GCP/protocol compliance. The detectability and probability should be assessed by the stakeholders based on their decision process. However, probability is weighed based on the likelihood of a risk occurrence and detectability is evaluated based on the monitoring detection technique either as remote monitoring or onsite monitoring. We propose a score measure for the category of each criterion ( Table 1 ).
Risk assessment criteria. Following risk identification, each risk is evaluated based on the category it impacts, the probability of risk occurring, and the monitoring technique required for detection.
GCP = Good clinical practice.
Scoring Method
The scoring algorithm of RMA allows the stakeholders a unique prospect to visualize the risk size and quantify it. The goal of risk communication is to guide the stakeholders in the risk assessment in a transparent manner and to assist them in the decision plan to mitigate its occurrence by an effective measure. 22 Visual representation can help stakeholders observe the assessment of the risk and understand its needed monitoring level. The visualization process can be achieved by radar charts as they enhance comparisons of quality measurements. 23
With the defined scaling system, the area would reflect the extent of how critical a risk is, which subsequently hints to the extent of monitoring required. The larger the area, the more monitoring is required; however, it does not reflect the type of monitoring technique needed as this must be decided by the stakeholders themselves ( Figure 2 ). Following the assessment, a monitoring technique should be assigned. According to regulatory agencies, the main techniques can either be traditional onsite monitoring, remote monitoring, or a combination of both.

The area under the radar chart. This figure shows the total area of the radar chart. Each area of the subtriangles is calculated based on the conventional formula.
Area Under the Radar Chart
The aim of radar chart is to present multivariate data, the main advantage is to translate the data to a meaningful sense. The area under the radar is equivalent to the cumulative area of the separate triangles ( Figure 2 ). The area under the radar chart is then reported as a percentage of the maximum score possible.
Each area is detected by the sides of the respective triangle input
Practical Implementation of RMA
A shiny web application was formed to illustrate the theoretical approach of RMA. The application includes risks that could be assessed and visualized under the radar plot ( Figure 3 ).

The individual risk assessment presented by the radar chart. This figure shows the criteria of risk assessment that should be completed by the assessor. The individual risk is assessed by its impact, probability, and detectability. Accordingly, the total area of the risk is presented by the radar chart.
Following the assessment of the individual risks, the input scores provided by the assessor and the subsequent score areas are documented. The following process can aid stakeholders in comparing the assessment report with monitoring reports after trial initiation to get a better understanding of the faults/weaknesses and strengths of the performed assessment ( Figure 4 ).

Assessment score of each individual risk with corresponding input. This figure shows the documentation of the individual risks assessed with its input criteria score and the computed overall score.
The score of the distinct risks assessed allows stakeholders to distinguish high score risks that necessitate more extensive monitoring in the monitoring plan ( Figure 5 a). Consequently, based on the profile input of each risk ( Figure 5 b) and its relation to the threshold for maximum score, represented by dashed lines, stakeholders can decide on the extent of monitoring visits/checks required in the monitoring plan. Finally, an overview of the sum of risks to be monitored by each technique ( Figure 5 c) imparts a clearer understanding of the type of monitoring plan needed, which is highly essential in the application of RBM.

Risk methodology assessment. (A) Overall scores (area under the radar) of each risk. (B) Risks based on the input of the assessment; red, green, and blue points are compared with their respectively colored dashed lines representing the maximum score. (C) Overall counts of risks covered by each monitoring technique.
The assessment process should repeated as soon as amendments are made to the trial protocol or when identifying new risks during monitoring process after trial initiation. This would require the stakeholders to conduct a new risk assessment to engage a proper mitigation action in the monitoring plan. It is essential to act on a new identified risk to understand its direct effect on the overall score of the risk assessment as a whole and on the monitoring technique required to prevent its occurrence.
Generally, the monitoring activities of the clinical research coordinator/monitoring team should focus on the requirements, responsibilities, and hazards that can carry potential liabilities to the trial assurances. The final assessment report will stipulate the potential risks to be monitored and frequency of monitoring needed.
Because RBM is becoming a principle stage in clinical trials, 24 both RMA's strategy and approach have the potential to improve data quality and reduce clinical costs. Undoubtedly, the risk assessment within other RBM systems can also identify certain risks; however, the assessment methodology of the individual risk criteria is either not reported or vague. As for their systems, they are fixed on prespecified risks lacking the ability of tallying new ones. For this reason, RMA's scoring system provides a means to facilitate confirmation of a certain risk and assess its outcome measure. Additionally, it incorporates flexibility in directly, including an additional risk area in the assessment report. Finally, once the entire risk assessment is completed, risks could be grouped based on the monitoring technique to assist the stakeholders in the trial monitoring plan development. The established method can be considered a primary step toward a practical monitoring guidance in which a monitoring plan form will be based on different risks in a trial, individual process, and required monitoring. 25
The innovative approach of RBM will facilitate establishment of adequate and focused monitoring activities, reduce 100% source data verification activities, and enhance the quality of the trial and patient safety. 26 This goal should be clearly communicated to stakeholders and clinical trials to prevent misconceptions among clinical research coordinators regarding RBM's outcome in increasing workload, a concern that has been previously reported, despite its capacity to do the opposite. RBM is a continued improvement process that requires all stakeholders and clinical trial staff to initiate the risk assessment before and during the trial period. An effective monitoring plan can only be achieved after a successful implementation of RBM. 27 We believe the RMA approach can aid stakeholders in distinguishing and evaluating any potential risk. Future investigation should focus on validating the power of RMAs to demonstrate efficiency in practice.
RMA could be further developed to software that utilizes existing data to forecast a certain risk outcome and provide a mitigation plan based on the risk score. Further work is required to achieve the desired prediction. Classification models may be employed to predict the existence of a specific risk and measure its individual score; however, numerous factors such as data quality and model fit variability require consideration during the utilization of such models. 28 Artificial intelligence algorithms should be the next phase of any risk assessment. Transparent risk methodologies such as RMA should be made available to both regulatory authorities and the public. The prospect of being able to estimate a risk outcome and potential mitigation serves as a continuous incentive for future research. We believe the efficiency of RBM has been well established and proven; yet the ultimate design of RBM development will be a challenge for us for years.
Acknowledgments
The authors thank the journal for its editorial support and the anonymous referees for their numerous constructive comments toward improving our manuscript.
Shinyapp & Code
The shinyapp was coded using R software (R Foundation for Statistical Computing) and is platform independent; specifically, an interactive hypertext markup language document is produced using Rmarkdown runtime shiny. The Rmarkdown shiny syntax is deployed to shinyapps server in which it preserves the functionality of the code. The syntax is available on Github at https://github.com/firasfneish/Risk-Methodology-Assessment . Project home page: https://firasfneish.shinyapps.io/Risk_Based_Monitoring_Methodology/
Author Contributions
F. Fneish designed and conceptualized the methodology of the tool, programmed the shinyapp, and drafted the manuscript for intellectual content. F. Schaarschmidt, critically reviewed the manuscript. G. Fortwengel designed and conceptualized the methodology of the tool and critically reviewed the manuscript. The authors approved the final version of the manuscript.
Conflicts of Interest

Welcome to the Harvard Center for Risk Analysis
The Harvard Center for Risk Analysis (HCRA) is a multidisciplinary group of faculty, research staff, students, and visiting scholars who work together to improve decisions about environmental health and other risks. We conduct state-of-the-art research, educate the next generation of leaders in risk analysis and related disciplines, and encourage public discourse about risk topics. To learn more about HCRA, please contact any member of our faculty or research staff below or click here to join our email list.
Risk Analysis Special Issue on “Risk Assessment, Economic Evaluation, and Decisions”

A paper from the issue, “ Mortality Benefits and Control Costs of Improving Air Quality in Mexico City – the Case of Heavy Duty Diesel Vehicles ,” co-authored by Dr. Evans, Leonora Rojas-Bracho , Dr. Hammitt, and Douglas Dockery, received the Society for Risk Analysis 2021 Best Paper Award .
Benefit-Cost Analysis, Valuing Statistical Lives, and COVID-19
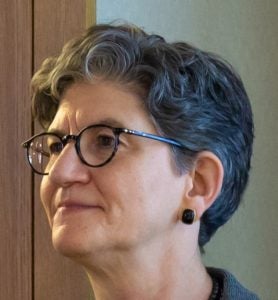
James K. Hammitt , Professor of Economics and Decision Sciences
Joel Schwartz , Professor of Environmental Epidemiology
Core Faculty and Affiliates
John Evans , Adjunct Professor of Environmental Health
Elsie Sunderland , Gordon McKay Professor of Environmental Chemistry
Lisa A. Robinson , Senior Research Scientist
Katherine von Stackelberg , Research Scientist
Jonathan B. Wiener , Duke University
Visiting Scholars
Eva Tène, Toulouse School of Economics (2020)
Rebecca McDonald, University of Birmingham (2019)
Danae Arroyos-Calvera, University of Birmingham (2019)
Nathalie de Marcellis-Warin, Polytechnique Montreal (2015-2016)
Daniel Herrera, Toulouse School of Economics (2014)
Emmanuelle Lavaine, Toulouse School of Economics (2014)
Damian Tago, Toulouse School of Economics (2014)
Tuba Tuncel, Toulouse School of Economics (2014)
News from the School
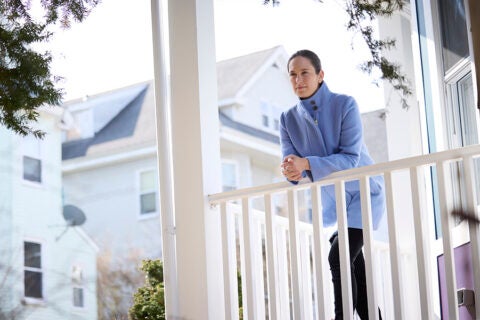
Bethany Kotlar, PhD '24, studies how children fare when they're born to incarcerated mothers
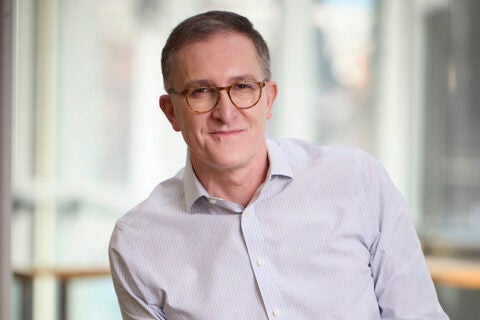
Soccer, truffles, and exclamation points: Dean Baccarelli shares his story
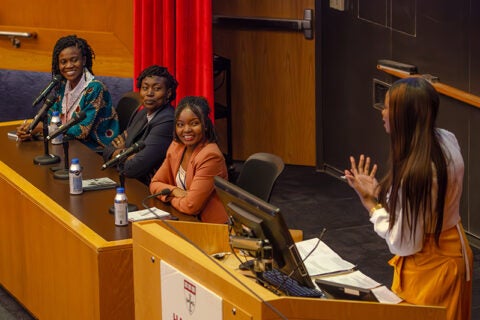
Health care transformation in Africa highlighted at conference
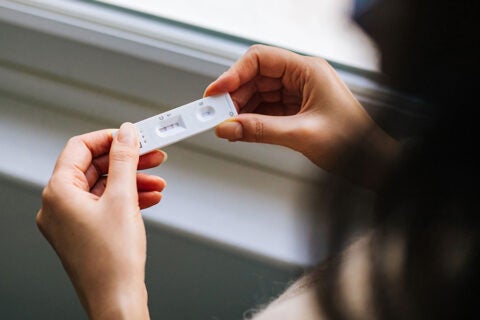
COVID, four years in
Log in using your username and password
- Search More Search for this keyword Advanced search
- Latest content
- Current issue
- JME Commentaries
- BMJ Journals More You are viewing from: Google Indexer
You are here
- Volume 47, Issue 5
- Process of risk assessment by research ethics committees: foundations, shortcomings and open questions
- Article Text
- Article info
- Citation Tools
- Rapid Responses
- Article metrics

- http://orcid.org/0000-0002-1953-9909 Pranab Rudra ,
- Christian Lenk
- Institute of the History, Philosophy and Ethics of Medicine , Ulm University , Ulm , Baden-Württemberg , Germany
- Correspondence to Pranab Rudra, Institute of the History, Philosophy and Ethics of Medicine, Ulm University, Ulm, Baden-Württemberg 89073, Germany; rudrapranab{at}gmail.com
Risks and burdens in the study participation, as well as an adequate risk-benefit balance, are key concepts for the evaluation of clinical studies by research ethics committees (RECs). An adequate assessment and continuous monitoring to ensure compliance of risks and burdens in clinical trials have long been described as a central task in research ethics. However, there is currently no uniform and solid theoretical approach to risk assessment by RECs. Regulatory standards of research ethics such as the Declaration of Helsinki provide only minimal guidance on how risk decisions are considered. Due to discrepancies in the existing literature and guidance documents, adequate risk assessment by RECs remains to be elusive. In this article, we address current definitions of risk and present our own concept of aggregate risk definition. Moreover, we highlight the concept of benefit, the standard of reasonableness with respect to ethics literature and different approaches of risk-benefit assessment. In order to present a comprehensive theoretical approach of risk assessment by RECs, further understanding of the definitions of risk may improve adequate decision-making tasks by RECs. To improve the process of risk assessment by RECs, a dynamic framework will be illustrated, showing step-by-step risk assessment functions. This approach may be a promising tool to ensure adequacy in risk assessment by RECs.
- research ethics
- clinical ethics
- clinical trials
- ethics committees/consultation
- technology/risk assessment
https://doi.org/10.1136/medethics-2019-105595
Statistics from Altmetric.com
Request permissions.
If you wish to reuse any or all of this article please use the link below which will take you to the Copyright Clearance Center’s RightsLink service. You will be able to get a quick price and instant permission to reuse the content in many different ways.
Introduction
The concept of risk within the clinical research community is considered to be a central element and is frequently mentioned in scientific publications, guidelines and regulations. 1–8 Over the last two decades, increased globalisation of clinical research paved its path to the increased expansion of clinical trials. 9 Although regulatory documents for every clinical research addressing benefit and harm comparison are helpful, there is no uniform methodology inherent on how research ethics committees (RECs) should use the heterogeneous information on risks and burdens gathered from clinical studies. Lacking, however, is a comprehensive and systematic framework for risk-benefit evaluations based on the relevant literature and on guidance documents. As a result, the role of RECs in risk evaluation and monitoring is not clearly understood, particularly in terms of the ongoing clinical trial. This issue is highlighted by Rid and Wendler: “The regulations seem to leave those charged with assessing the risks and potential benefits of medical research with only their own intuitions to guide them. However, while normative judgment is a crucial part of ensuring that medical research is ethical, these assessments should be based on a systematic evaluation of the risks and benefits posed by individual research studies, not based on mere intuition regarding which procedures and studies are, and are not, excessively risky”. 2 This critical description of the framework of risk assessment by RECs is supported by a number of further publications. 5 10–12 On the one hand, research ethics looks back at a long and fruitful discussion concerning the criteria of risk assessment. On the other hand, there are still uncertainties on how to use these criteria in the field of clinical trials in a regular and systematic manner in order achieve adequate risk monitoring and patient protection.
The importance of a rigid risk assessment and monitoring in clinical trials was dramatically demonstrated by a Dutch study in pregnant women that had to be terminated after fatal results during summer 2018. Due to a decision of the Data Safety and Monitoring Board of the Dutch Strider Trial, the risk-benefit ratio of the trial was regarded no longer acceptable. The data revealed that 17 infants born to women given the drug sildenafil (Viagra) had lung problems, and 11 of those infants died, in comparison to 3 infants with lung problems in the placebo group, where no infants died. 13 The study’s goal was to find an effective treatment against fetal growth restriction, where the published study design of the drug sildenafil proved to be effective and successful in a number of animal and preclinical studies. 14 In case an ongoing trial fails to show a significant beneficial effect or shows a signal of harm, RECs may decide to stop the trial and allow detailed review and validation of the findings before any further exposure of the given drug occurs. 15 The dramatic chain of events that took place in the Dutch study stands as an alarming example of possible weaknesses of monitoring and constant risk-benefit assessment in clinical trials. Reports of the deaths of research subjects raise serious concerns regarding the system and processes by which the participant’s safety is monitored.
This article considers three especially contested areas of research risk. First, we will review the concept of risk and benefits used in the relevant literature with respect to clinical practice (see section ‘Understanding risks and benefits in clinical studies’). Second, we will review the term ‘reasonable risk’ using different evaluation procedures that RECs may rely on when weighing the risks and benefits of medical research (see section ‘Reasonable risks as a common standard for clinical research’). Finally, we illustrate a new approach for risk assessment in clinical trials, which can be applied to the different study phases with respect to different kinds of risks at different time points (see section ‘Process of risk assessment’). We argue that such a comprehensive model may assist RECs and physicians to improve the monitoring of clinical trials as well as patient safety. In this article, we do not focus exclusively on pharmacological studies, but want to present concepts usable for all kinds of clinical studies.
Understanding risks and benefits in clinical studies
Regarding the international regulation and monitoring of clinical research, we find a well-defined system for the classification of pharmaceutical risks and medical devices, but no comparable provisions in other fields (eg, other interventional studies in medicine, surgery or psychology). This leaves out subjective and emotional burdens as well as additional physical or social risks for patients and study participants. At present, it is an open question, how the pharmacological understanding of risk can be complemented by risks and burdens of additional research procedures such as performing biopsies, spinal taps or experimental surgical interventions.
A systematic literature research review 3 5 16–19 reflects a widely endorsed definition of risk—a product formula for possible harm that may occur, expressed as chance (probability), and severity (magnitude) of the envisioned harm, denoting to the formula
In the economic sciences, it is widely accepted that the second factor, ‘severity of damage’, can be adequately described as the amount of financial damage (eg, the damage of a house, the loss of a car or the compensation for an injury). Evidently, such a concept of risk is in the end not very well suited for medicine and research ethics. As King and Churchill explain, ‘[b]oth potential benefits and risks of harm should always be examined and characterized, to the extent possible, according to their nature, magnitude (size and duration), and likelihood’. 20
Based on this definition, we propose an extended concept of risk and benefit and a comprehensive understanding of risk which does not only cover pharmacological risks and burdens in clinical trials. We define the extended clinical risk as the product of the frequency, the kind of harm and the magnitude (size and duration) of the expected side effect ( Box 1 , (2)). For example, it could be known that a drug causes in the case of 1%–10% of the treated patients impaired vision to a severe degree, but that this is a transient condition. Such a description would be adequate from a medical ethics’ perspective. The frequency of risk and benefit can be deduced from the former experience with a therapeutic intervention. In the case of common medical interventions, the rate of side effects is often published in medical guidelines.
Definitions of risk, types of risk and types of benefit
Economic concept of risk: risk=frequency×severity of damage
Example: risk of dying in a car crash=10 deaths p.a./100 000 vehicles=0.0001%
Extended concept of risk and benefit (King and Churchill 2008): risk=frequency×[(kind of harm+magnitude (size+duration)] benefit=frequency×[kind of benefit+magnitude (size+duration)]
Examples:risk=1%–10% of treated patients×(impaired vision+severe+transient) benefit=10% of treated patients×(6 months higher life expectancy+median quality of life )
Comprehensive understanding of risk: The overall risk and burden of study participation consists in the aggregate of partial risks and burdens (drug risk, additional physical risks/burdens, social risk, psychological risk/burden)
Additionally, to develop a comprehensive understanding of burden and risk in a clinical study, we come to the definition of comprehensive risk in Box 1 , (3). The overall risk and burden in a clinical study is then the sum of partial risks and burden in four different areas ( figure 1 ): first, the drug risk, which contains adverse events and side effects. Second, there are the burdens of study measurements like additional X-rays or spinal taps. Third, genetic examinations can also include some social risks, and the same is true for psychiatric or psychological examinations which strongly intrude into the privacy sphere of the participants. Finally, there exist psychological risks and burdens, for example, when psychiatric patients are questioned about sexual abuse in their childhood.
- Download figure
- Open in new tab
- Download powerpoint
Different types of risk in clinical studies.
In general, this is contrasted with three forms of benefit ( figure 2 ): first, the direct clinical benefit, that is, when the patient directly profits from a new therapy in a study. Second, the group benefit, that is, when the patient herself or himself does not directly profit from the intervention, but future patients having the same disease, and third the scientific benefit, that is, to provide generalisable knowledge which is normally produced in all studies of basic research. 20 These three forms of benefit are also incorporated in the Declaration of Helsinki and are connected to the acceptability of risk, that is, larger forms of risk are only acceptable when study participants have the chance to realise a direct clinic benefit. When the study participation bears only the chance for a group benefit, only minor risk is seen as acceptable (Art. 16–18). 4 From our point of view, with these definitions and descriptions of benefit and risk, research ethics has the necessary concepts and terminology to carry out an adequate benefit-risk assessment, over and above the reductionist understanding of pharmacological risk alone.
Different types of benefit in clinical studies.
Reasonable risks as a common standard for clinical research
International conventions and guidelines, as well as national law, usually demand an ‘adequate’ risk assessment and a ‘weighing’ of risks and benefits for the individual participant. From the participant’s point of view, it has to be determined, that in her or his situation, the probability and amount of harm in a clinical trial is acceptable or appropriate and does not exceed defined ethical, legal and medical thresholds (eg, pain, fear or toxicity). 21 The amount of risk for a special intervention (ie, the application of a new drug or an innovative surgical intervention) is often difficult to determine even for medical experts and has to be concluded—among other sources—from detailed statistical analysis and the available literature. A clinical trial has the aim to show the superiority or at least the non-inferiority between two or more different and prima facie equivalent medical interventions (the so-called clinical equipoise). When dealing with patient populations, risks are compared with those of the existing standard of care, which may itself involve a great deal of risk and the level of risk tolerance may therefore be quite high; when no such effective treatment or procedure exists, the benefits and risks of an innovative treatment approach are often compared with placebo. In this context, a comparable problem exists in the case of placebo use in a clinical trial when study participants are at risk of disease progression due to the placement in the placebo group. 22 23 However, there are currently no clearly defined criteria and procedures on how this should be organised. Therefore, the implementation of risk evaluation for RECs still remains a challenge. In the international research ethics literature, there are especially three approaches that discuss possible and adequate forms of risk assessment:
Weijer and Miller wanted to make RECs’ decisions more understandable and objective, and therefore suggested component analysis in the evaluation of clinical research. The component analysis divides the individual research interventions included in a given study into two groups: therapeutic and non-therapeutic. The approach involves an independent risk assessment of each research intervention or procedure involved in a study (eg, drawing blood, performing CT scan, taking liver biopsy). 24 25 According to Weijer, therapeutic procedures are justified in terms of the clinical benefits of the participants, that is, clinical equipoise must be met. In contrast, non-therapeutic procedures are regarded as justified when the procedure in the study includes knowledge gain (ie, administered solely for the purpose of answering scientific questions and the potential to advance society’s interests) provided that the risk is acceptable and does not or only slightly exceed risks of daily life.
In response to Weijer’s component analysis, Wendler and Miller developed the ‘net risks test’ that assesses the risk-benefit profile of each intervention by comparing its risks with the potential clinical benefits and then comparing them with existing alternatives. 26
The direct benefit assessment is used in risk assessment by RECs, which is derived from the US guidance documents for medical research on humans. 27 This assessment instrument offers a possibility to avoid the therapeutic and non-therapeutic distinction and takes the direct clinical benefits of a study for the participants as the deciding criterion for ethical considerations.
Concerning the three shortly described approaches, it catches one’s eye, that they do not give a clear and substantial definition of ‘reasonable risk’. The criteria are always relative or proportional to possible clinical or scientific benefits. Therefore, these instruments do not give a direct answer of what is meant by a ‘reasonable’, ‘justified’ or ‘balanced’ relation of risk and benefit. Rather, they can be regarded as decision aids for experts with longstanding experience in the field. It remains an open question, whether such approaches can ensure a homogeneous level of regulation based on similar decisions from different RECs and in the assessment of different clinical trials. Additionally, especially the Belmont Report and the different versions of the Declaration of Helsinki take up a stance regarding an adequate risk assessment. The different aspects of risk assessment in clinical research studies from the Declaration of Helsinki are compiled in table 1 .
- View inline
Criteria for risk assessment in clinical studies according to the Declaration of Helsinki
A closer look at these criteria of risk assessment in table 1 reveals that there are in general two different kinds of ‘reasonable risk’: first, we find a relative or proportional understanding of risk in the majority of passages in the cited documents. This suggests that it is asked or stated that an existing risk might be acceptable in comparison to the clinical benefit for the concerned patient, the benefit for society or in comparison with existing alternatives ( table 1 , (1–3)). We can call this a proportional understanding of risk, because it results in the point of view that the existing risk must be proportional to an aspired good or benefit. For example, when the patient suffers from only a minor disturbance of health and well-being, it would be irrational to accept major risks and burdens for the treatment of this condition. However, in the case of severe or untreatable diseases, it is rational to accept larger risks and burdens in the treatment of the disease. Such an understanding of risk seems to be adequate for medical research, but maybe produces further questions in some situations, for example, when patients suffer from a potentially lethal disease with no existing treatment options. Where is then the limit in such a relative understanding of risk and what is acceptable for such patients in the research for new therapies? The proportional perception of risk comes here to the conclusion, that also the sacrifice of important patient interests could be acceptable in such cases. From the ethical point of view, such a result is a dilemma of benefit-risk assessment (for further problems with the ‘weighing’ of risks and benefits, see King and Churchill 20 ). Given that such patients are an especially vulnerable group in research ethics, alternative approaches would be necessary to solve this dilemma.
Second, one can notice an absolute or definite understanding of ‘reasonable risk’ in the quotations number 4 and 5 from the Declaration of Helsinki ( table 1 , (4 and 5)). The first quotation number 4 refers to vulnerable patients who have no direct clinical benefit when participating in a research project. Art. 28 of the Declaration of Helsinki claims that in such cases only ‘minimal risk and minimal burden’ is acceptable. This indicates an absolute understanding of risk. Quotation number 5 refers to placebo studies and studies where standard treatment is withheld. According to the Declaration ‘additional risks of serious or irreversible harm’ must be excluded in such cases, which indicates an absolute understanding of risk as well. Apart from these two articles, the relative or proportional understanding of risk is seen as adequate or reasonable. The term absolute represents a predetermined or fixed risk level to ensure clinical safety for the participants. For example, exposing a group of children or individuals to the burdens of study participation (ie, an additional blood sampling) would not violate the requirements of patient protection when the study has the potential to promote the health of this patient group with minimal risk and burden. In such cases, Kopelman proposes an upper limit for risk, which ‘(a) represents no more than a minimal risk for children with conditions who are enrolled in studies and (b) be no more than a minor increase over minimal risk for healthy children were they enrolled’. 28 This constitutes an absolute standard of risk which is applied to children and other vulnerable groups.
Process of risk assessment
It remains unclear whether the models proposed in the literature are already used by RECs when making decisions in interdisciplinary consultations. The common risk-benefit approaches, namely component analysis, net risk test and direct benefit assessment do not give a complete account of risk-benefit assessment of medical research. 2 18 24–26 Most importantly, none of them accounts for risk identification on a continuous basis throughout the clinical trial. An ideal risk assessment process should start at the protocol design so mitigation can be built into the protocol and monitoring documents.
In the following, we describe the different steps in the assessment procedure of RECs, as it is portrayed in figure 3 . So far, the internal process of risk assessment has not been adequately described in relation to the different components of clinical trials (initiation phase, start of trial, conduct, reporting, end of trial). Our description refers to the processes as they are currently practised by RECs and other responsible authorities (eg, the respective national body responsible for the market approval of new drugs, ie, the Food and Drug Administration in the USA, the Medicines and Healthcare products Regulatory Agency in the UK and the Federal Institute for Drugs and Medical Devices in Germany). Additionally, the description includes the proposal of the extended concept of risk and benefit (see section ‘Understanding risks and benefits in clinical studies’) and a systematic order of REC risk assessment at different points in time in the course of the clinical trial. Therefore, the description represents a normative or idealised risk assessment procedure for RECs.
The process of risk assessment by research ethics committees. DSURs, Development Safety Update Report; EMA, European Medicines Agency; SAEs, Serious Adverse Events; SUSARs, Suspected Unexpected Serious Adverse Reaction; t1, Evaluation of Risk before Trial Start; t2-t3, Reporting in the Course of the Trial; t4, Foreseen End of Trial.
The process begins with the initiation phase of the clinical trial ( figure 3 , (1)) and the submission of the study protocol together with clinical and preclinical data and further documents to the official authorities and to the responsible RECs. In the assessment of the clinical trial by the REC, risks are identified and compared ( figure 3 , (2)) with standard treatment (insofar there is an established standard treatment). To come to a comprehensive understanding of risk, as described above, the different types of risk ( figure 1 ) have to be examined. It follows ( figure 3 , (3)) the proof of the risk classification, that is, what is to date known regarding the frequency of risk. The frequency at which the risk occurs is an important factor in classifying risk according to its weight. The European Medicines Agency guidelines classify drug risks according to the likelihood and severity of adverse events (ranging from very common to very rare). The risk-benefit assessment demands knowledge and a proper understanding of setting priorities in terms of risk identification and classification ( figure 3 , (4)). Considerations such as what can go wrong or the probability of a negative outcome occurring or trial subject’s safety, well-being and rights are all part of the assessment process. Different criteria of risk-benefit assessment profile of clinical trials, drug trials, first-in-human use, children, vulnerable groups and individuals according to Declaration of Helsinki are taken into account. 29
In summary, the concerned REC and the respective national body assess the information for a well-founded risk-benefit balance and take possible steps to reduce the risk to an acceptable level ( figure 3 , (5)). Once the right risk-benefit balance has been determined, the authorities finally decide on whether the clinical trial is allowed to start ( figure 3 , (5 and 6)). The regular reports of expected and unexpected adverse events ( figure 3 , (7)) during the trials are used to classify the risks appropriately (ranging from mild risk to the death of a participant). According to the international drug regulations, adverse events in drug trials have to be regularly communicated to the RECs and the responsible authorities. By systematic documentation of the adverse events, RECs gain insight into the existing risks and burdens in a clinical trial. This is complemented by annual reports of the researchers regarding the safety of the trial. In the course of the continued risk observation and assessment, the question arises, whether the original risk-benefit assessment at the trial start (t1) was accurate in comparison with the later on reported types and level of risk (t2–t3) ( figure 3 , (8)). At the present point of time, it is not defined in the Declaration of Helsinki or other documents (at least in the European Union) how much effort RECs should invest into this task of risk monitoring in clinical trials. However, as the above-mentioned example of the Strider Trial shows, this task is of extreme importance. In case of a fundamental deviation from the previously expected risk level and an excess of severe adverse events, the responsible authorities have the possibility of a termination of the clinical trial ( figure 3 , (9)). Otherwise, the trial reaches its foreseen end as it was defined in the study protocol ( figure 3 , (10)).
From our point of view, this systematic approach to risk assessment in clinical trials can facilitate better and more informed decision-making and make an important contribution to a steady and continuous improvement of patient safety. Although the approach presented here is an evolving framework, the concept is expected to benefit the RECs how and to what degree of transparency the information is shared. One should also consider that numerous values ground risk judgements and that a more comprehensive approach towards risk assessment will be more sensitive to the different needs and burdens of study participants. Additionally, a more systematic approach can strengthen the coherence of REC decisions and therefore the trust between researchers and the institution of the REC as such. Such an approach could also identify remaining elements of uncertainty in the concept of a research project, where more assertive measures from the REC could be needed. It is, however, in the responsibility of all involved parties to best contribute to the delivery of an effective risk assessment in research studies.
The task of risk-benefit assessment by RECs is a complex endeavour. To better understand this assessment, we took initiative to explore the literature definition and reasonableness of risk in clinical studies. We summarised how authors from ethics literature perceive risk and benefit definitions. A key part of the assessment process is to understand what reasonable risk means. Generally, with the term ‘reasonable risk’ we understand a proportionality of risks and benefits while for vulnerable groups there should only be minimal risks with the exclusion of any irreversible risks. In any case, a systematic approach where the definition and reasonableness of risk are uniform and its heterogeneity addressed promises to reduce unreliable decisions made by RECs. A model system to articulate the process of risk has been proposed here which provides a compelling dynamic foundation for a framework for assessing risk in clinical studies. The model may prove effective for continuous risk assessment in different clinical studies while maintaining a ‘homogenous approach’ by the RECs. The appropriate conduct of the risk assessment process suggested here may ensure step-by-step compliance with regulations and can prevent—in the given limits of risk assessment—major unjustified risks to participants in the study. When following the systematic framework, the RECs will be able to indicate any amendments related to risks and can, therefore, respond to them accordingly, timely, accurately and effectively.
To sum up, by looking at the theoretical aspects of risk in all dimensions, in particular, definition and reasonableness, we can better identify the problems of discrepancies and strategise consolidated solutions of risk assessment. Following the described process of risk assessment would be fruitful for RECs to conflate ethical considerations and risk-benefit tasks, thereby improving the functioning and the ethical consultation of RECs. As described above, the aim of a responsible risk-benefit assessment cannot be reached by only punctual and intuitive activities. Instead, a steady and systematic approach by RECs is needed, particularly in improving ongoing or intermediate clinical trials and patient safety. Next to that, our description of the domain of risk assessment in clinical trials shows that there should be more efforts for the establishment of absolute risk thresholds, for example, in the case of specific diseases (ie, cancer research, dementia research). This could lead to a more homogeneous risk assessment between different RECs, also in international comparison. Such an approach may be extremely important especially in the case of patients with severe and incurable diseases, where up to now a proportionate approach for risk assessment is not possible.
Acknowledgments
The authors would like to thank the two anonymous reviewers for their helpful comments, which have been incorporated into this article.
- Van Ness PH ,
- National Commission
- World Medical Association Declaration of Helsinki
- Kimmelman J
- EU Expert Group
- ↵ Regulation (EU) NO 536/2014 of the European Parliament and of the Council: clinical trials on medicinal products for human use, and repealing directive 2001/20/EC 2014 .
- Grinnell F ,
- Sadler JZ ,
- McNamara V , et al
- Emanuel EJ ,
- Alfirevic Z , et al
- Ganzevoort W ,
- Palmboom GG ,
- Willems DL ,
- Janssen NBAT , et al
- Engel-Glatter S
- Lantos JD ,
- Wendler D ,
- Septimus E , et al
- Canadian Institutes of Health Research, Natural Sciences and Engineering Research Council of Canada, and Social Sciences and Humanities Research Council of Canada
- Churchill L
- Emanuel E ,
- Kopelman LM
- European Commission
Contributors All authors contributed significantly to this article.
Funding This paper was written with support from the Medical Faculty of Ulm University.
Competing interests None declared.
Provenance and peer review Not commissioned; externally peer reviewed.
Read the full text or download the PDF:
Other content recommended for you.
- Eliminating the daily life risks standard from the definition of minimal risk D B Resnik, Journal of Medical Ethics, 2005
- Collaborative risk assessment in secure and forensic mental health settings in the UK Sarah Markham, General Psychiatry, 2020
- Intergenerational monitoring in clinical trials of germline gene editing Bryan Cwik, Journal of Medical Ethics, 2019
- Ambiguous articles in new EU Regulation may lead to exploitation of vulnerable research subjects Anna Eva Westra, Journal of Medical Ethics, 2016
- Acceptable risks and burdens for children in research without direct benefit: a systematic analysis of the decisions made by the Dutch Central Committee Anna E Westra et al., Journal of Medical Ethics, 2010
- Improving the Helsinki Declaration's guidance on research in incompetent subjects Anna Eva Westra et al., Journal of Medical Ethics, 2013
- Grants and Contracts, JIM 61-2 Amanda Beaini, Journal of Investigative Medicine, 2015
- Can you afford to make an inappropriate high-volume switch? Malcolm B R Partridge et al., European Journal of Hospital Pharmacy: Science and Practice, 2013
- How do electronic risk assessment tools affect the communication and understanding of diagnostic uncertainty in the primary care consultation? A systematic review and thematic synthesis Alex Burns et al., BMJ Open, 2022
- The views of public and clinician stakeholders on risk assessment tools for post-stroke dementia: a qualitative study Eugene Tang et al., BMJ Open, 2019

Conducting Risk-Benefit Assessments and Determining Level of IRB Review
Regulatory background.
Investigators should understand the concept of minimizing risk when designing research and conduct a risk-benefit assessment to determine the level of IRB review of the research. In the protocol application the Investigator should:
- Assess potential risks and discomforts associated with each intervention or research procedure;
- Estimate the probability that a given harm may occur and its severity;
- Explain measures that will be taken to prevent and minimize potential risks and discomforts;
- Describe the benefits that may accrue directly to subjects; and
- Discuss and the potential societal benefits that may be expected from the research.
Risks to subjects who participate in research should be justified by the anticipated benefits to the subject or society. This requirement is found in all codes of research ethics, and is a central requirement in the Federal regulations ( 45 CFR 46.111 and 21 CFR 56.111 ). Two of the required criteria for granting IRB approval of the research are:
- Risks to subjects are minimized by using procedures which are consistent with sound research design and which do not unnecessarily expose subjects to risk, and whenever appropriate, by using procedures already being performed on the subjects for diagnostic or treatment purposes.
- Risks to subjects are reasonable in relation to anticipated benefits, if any, to subjects, and the importance of the knowledge that may reasonably be expected to result. In evaluating risks and benefits, the IRB Committee will consider only those risks and benefits that may result from the research , as distinguished from risks and benefits of therapies subjects would receive even if not participating in the research.
Definitions
Benefit: A helpful or good effect, something intended to help, promote or enhance well-being; an advantage.
Risk: The probability of harm or injury (physical, psychological, social, or economic) occurring as a result of participation in a research study. Both the probability and magnitude of possible harm may vary from minimal to significant.
Minimal Risk: A risk is minimal when “the probability and magnitude of harm or discomfort anticipated in the proposed research are not greater in and of themselves than those ordinarily encountered in daily life of the general population or during the performance of routine physical or psychological examinations or tests .” Examples of procedures that typically are considered no more than minimal risk include: collection of blood or saliva, moderate exercise, medical record chart reviews, quality of life questionnaires and focus groups. See Expedited review categories for a complete listing.
Minimal Risk for Research involving Prisoners: The definition of minimal risk for research involving prisoners differs somewhat from that given for non-institutionalized adults. A risk is minimal when, "the probability and magnitude of physical or psychological harm that is normally encountered in the daily lives, or in the routine medical, dental or psychological examinations of healthy persons ."
Privacy: Privacy is about people and their sense of being in control of others access to them or to information about themselves.
Confidentiality: Confidentiality is about how identifiable, private information that has been disclosed to others is used and stored. People share private information in the context of research with the expectation that it be kept confidential and will not be divulged except in ways that have been agreed upon.
Types of Risks to Research Subjects
Physical Harms: Medical research often involves exposure to pain, discomfort, or injury from invasive medical procedures, or harm from possible side effects of drugs, devices or new procedures. All of these should be considered "risks" for purposes of IRB review.
- Some medical research is designed only to measure the effects of therapeutic or diagnostic procedures applied in the course of caring for an illness. Such research may not entail any significant risks beyond those presented by medically indicated interventions.
- Research designed to evaluate new drugs, devices or procedures typically present more than minimal risk and involve risks that are unforeseeable that could cause serious or disabling injuries.
Psychological Harms: Participation in research may result in undesired changes in thought processes and emotion (e.g., episodes of depression, confusion, feelings of stress, guilt, and loss of self-esteem). Most psychological risks are minimal or transitory, but some research has the potential for causing serious psychological harm.
- Stress and feelings of guilt or embarrassment may arise from thinking or talking about one's own behavior or attitudes on sensitive topics such as drug use, sexual preferences, selfishness, and violence.
- Stress may be induced when the researchers manipulate the subjects' environment to observe their behaviors and reactions. The possibility of psychological harm is heightened when behavioral research involves an element of deception.
Social and Economic Harms: Some losses of privacy and breaches of confidentiality may result in embarrassment within one's business or social group, loss of employment, or criminal prosecution.
- Areas of particular sensitivity involve information regarding alcohol or drug abuse, mental illness, illegal activities, and sexual behavior.
- Some social and behavioral research may yield information about individuals that could be considered stigmatizing to individual subjects or groups of subjects. (e.g., as actual or potential carriers of a gene; individuals prone to alcoholism). Confidentiality safeguards must be strong in these instances.
- Participation in research may result in additional actual costs to individuals. Any anticipated costs to research participants should be described to prospective subjects during the consent process.
Privacy Risks: Loss of privacy in the research context usually involves either covert observation or participant observation of behavior that the subjects consider private. It can also involve access and use of private information about the subjects. The IRB must make two determinations:
- Is the loss of privacy involved acceptable in light of the subjects' reasonable expectations of privacy in the situation under study; and
- Is the research question of sufficient importance to justify the intrusion?
Breach of Confidentiality Risks: Absolutely confidentiality cannot be guaranteed and is always a potential risk of participation in research. A breach of confidentiality is sometimes confused with loss of privacy, but it is a different risk. Loss of privacy concerns access to private information about a person or to a person's body or behavior without consent; confidentiality of data concerns safeguarding information that has been given voluntarily by one person to another. It is important to recognize that a breach of confidentiality may result in psychological harm to individuals (embarrassment, guilt, stress, etc.) or in social harm.
Conducting Risk-Benefit Assessments
Role of the Investigator: When designing research studies, investigators are responsible for conducting an initial risk-benefit assessment using the steps outlined in the diagram below.
Role of the IRB: The IRB ultimately is responsible for evaluating the potential risks and weighing the probability of the risk occurring and the magnitude of harm that may result. It must then judge whether the anticipated benefit, either of new knowledge or of improved health for the research subjects, justifies asking any person to undertake the risks. The IRB cannot approve research in which the risks are judged unreasonable in relation to the anticipated benefits. The IRB must:
- Identify the risks associated with the research, as distinguished from the risks of therapies the subjects would receive even if not participating in research;
- Determine that the risks will be minimized to the extent possible;
- Identify the probable benefits to be derived from the research;
- Determine that the risks are reasonable in relation to be benefits to subjects, if any, and the importance of the knowledge to be gained; and
- Assure that potential subjects will be provided with an accurate and fair description (during consent) of the risks or discomforts and the anticipated benefits.
Diagram 1: Steps for Conducting a Risk-Benefit Assessment

Ways to Minimize Risk
- Provide complete information in the protocol regarding the experimental design and the scientific rationale underlying the proposed research, including the results of previous animal and human studies.
- Assemble a research team with sufficient expertise and experience to conduct the research.
- Ensure that the projected sample size is sufficient to yield useful results.
- Collect data from conventional (standard) procedures to avoid unnecessary risk, particularly for invasive or risky procedures (e.g., spinal taps, cardiac catheterization).
- Incorporate adequate safeguards into the research design such as an appropriate data safety monitoring plan, the presence of trained personnel who can respond to emergencies.
- Store data in such a way that it is impossible to connect research data directly to the individuals from whom or about the data pertain; limit access to key codes and store separately from the data.
- Incorporate procedures to protect the confidentiality of the data (e.g., encryption, codes, and passwords) and follow UCLA IRB guidelines on Data Security in Research .
Levels of IRB Review
Exempt research.
Although the category is called "exempt," this type of research does require IRB review and registration. The exempt registration process is much less rigorous than an expedited or full-committee review. To qualify, research must fall into 8 federally-defined exempt categories. These categories present the lowest amount of risk to potential subjects because, generally speaking, they involve either collection of anonymous or publicly-available data, or conduct of the least potentially-harmful research experiments. For additional information see OHRPP Exempt Guidance .
- Anonymous surveys or interviews
- Passive observation of public behavior without collection of identifiers
- Retrospective chart reviews with no recording of identifiers
- Analyses of discarded pathological specimens without identifiers
Expedited Research
To qualify for an expedited review, research must be no more than minimal risk and fall into nine (9) federally-defined expedited categories. These categories involve collection of samples and data in a manner that is not anonymous and that involves no more than minimal risk to subjects. For additional information see OHRPP Expedited Guidance .
- Surveys and interviews with collection of identifiers
- Collection of biological specimens (e.g., hair, saliva) for research by noninvasive means
- Collection of blood samples from healthy volunteers
- Studies of existing pathological specimens with identifiers
Full Board Research
Proposed human subject research that does not fall into either the exempt or expedited review categories must be submitted for full committee review. This is the most rigorous level of review and, accordingly, is used for research projects that present greater than minimal risk to subjects. The majority of biomedical protocols submitted to the IRB require full Committee review. For additional information see OHRPP Full Board Guidance .
- Clinical investigations of drugs and devices
- Studies involving invasive medical procedures or diagnostics
- Longitudinal interviews about illegal behavior or drug abuse
- Treatment interventions for suicidal ideation and behavior
Regulations and References
- DHHS 45 CFR 46.110
- DHHS 45 CFR 46.111(a)(1-2)
- FDA 21 CFR 56.110
- FDA 21 CFR 56.111(a)(1-2)
- OHRP IRB Guidebook, Chapter 3: Basic IRB Review, Section A, Risk/Benefit Analysis
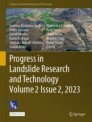
Progress in Landslide Research and Technology, Volume 2 Issue 2, 2023 pp 323–340 Cite as
A Review of the Disaster Risk Assessment Perspectives
- Dayan Munasinghe 15 ,
- Terrance Fernando 16 ,
- Kaushal Keraminiyage 16 &
- Asiri Karunawardena 15
- Open Access
- First Online: 29 December 2023
1045 Accesses
Part of the book series: Progress in Landslide Research and Technology ((PLRT))
Researchers have explored different risk assessment approaches from the perspectives of different disciplines to capture urban risks, resulting in many risk assessment frameworks. In these frameworks, the risk environment is analysed using different quantitative and qualitative assessment methods, such as fuzzy set, probability theory, and evidence theory. While each approach has contributed to risk assessment, they suffer from a lack of consensus in defining and measuring the impact of risk in an urban environment.
Therefore, the study aims to conduct a literature survey to consolidate a common set of risk assessment perspectives and approaches for measuring these risks.
A structured review was carried out to achieve the aim of this research. The research question used for conducting the literature review was “What approaches are being used to define and measure the impact of hazard risks in an urban environment?”. The PICO (Population, Intervention, Compression Intervention, and Outcome) method was used to generate the search string for the literature review by considering the keywords in the research question. Initially, 206 research papers were selected through a search strategy, and by applying a screening method, 119 research articles were selected for the detailed review. The Nvivo software was supported for the review purpose; then, a mind map was developed, integrating all the risk assessment perspectives.
Risk assessments were summarised by considering the various researchers’ perspectives. Thirty-four risk perspectives were identified through the literature, and a mind map was developed to understand the connectivity. This mind map was converted into a network diagram, and future requirements of risk perspectives were identified based on the risk assessment network diagram. According to the analysis, risk communication, risk treatment, critical curve, judgment curve, and risk matrix could be identified as future research areas. The risk reduction measuring strategies were identified by considering the feedback loop of the network diagram. Thus, 14 risk reduction strategies could be identified through the analysis.
The risk assessment frameworks focused on holistic approaches, but most research studies did not adequately follow the risk perspectives. Therefore, research gaps were identified in the risk assessment process, and the areas were highlighted as state-of-the-art to conduct future research studies. The feedback loops of the network diagram emphasised the risk reduction strategies, which could be further researched through application to a case study.
- Risk assessment
- Risk perspective
- Risk reduction strategies
- Risk communication
Download chapter PDF
1 Introduction
Disaster Risk Assessment (DRA) is a fundamental activity necessary for establishing a clear understanding of local risks to implement appropriate interventions for risk reduction activities and build resilient urban environments. Therefore, there are many international efforts to encourage governments to implement DRA to create sustainable cities and communities through partnerships (Nations U 2015b ). For example, many disaster risk reduction action plans such as the International Decade for Natural Disaster Reduction (Nations 1989 ), the Yokohama Strategy and Plan of Action for a Safer World, the Hyogo Framework for Action (HFA) (2005–2015), Sendai Framework for Disaster Risk Reduction (2015–2030) are calling for governments to focus on disaster risk reduction against climate-induced disasters (Nations U 2015a ). In this context, the Sendai Framework calls for strengthening the scientific capacities to develop or apply DRA methodologies and frameworks to assess vulnerabilities, exposure, and disaster risks for various hazards (Nations U 2015b ). Such risk assessment frameworks need to take a systemic view of DRA within urban environments by considering the interdependencies of various subsystems and help city governors fully understand the cascading impact of hazards across various subsystems and domains (Hasani 2014 ).
Due to the complex relationships that exist among the risk assessment variables, researchers have explored different risk assessment approaches, resulting in many risk assessment frameworks (Khazai et al. 2014 ; Yeganeh and Sabri 2014 ; Zlatanova et al. 2014 ; Munasinghe and Wijegunarathne 2015 ; Torre et al. 2019 ; Huang et al. 2020 ). In practice, different institutions and countries have used different risk management frameworks for assessing disaster risks in their local contexts.
A risk management framework is a guided process on risk management principles, policies, and practices developed more systematically by various institutions. Pedro Basabe ( 2018 ) has divided these frameworks into four categories: (1) Generic Principles, guidelines, and methods, (2) Applied principles for governmental organisations, (3) Intergovernmental framework, and (4) National Institutionalised Framework. The examples of category one frameworks on “Generic principles” include ISO31000 and IRGC risk frameworks which are purely theoretical frameworks. In contrast, OECD and UNECE risk assessment frameworks are category two frameworks on “Applied principles for governmental organisations”, which are focused on a specific thematic area (OECD 2012 ). For example, the OECD methodology, developed by G20 countries, focused on assessing the financial risk status of a country (OECD 2012 ). On the other hand, the Sendai Framework, introduced by UNISDR, is an example of a category three risk framework on “Intergovernmental risk management”, which proposes seven targets and four priorities that are aimed at supporting the reduction of existing and new disaster threats (UNDRR 2015 ). In contrast, the National Risk Register, prepared by the UK cabinet office, describes the national risk reduction approaches aligned to the National Institutionalised framework type, which comes under category four (Cabinet-Office-UK 2020 ).
Even though the risk frameworks are different, these frameworks possess common characteristics since they all focus on risk, vulnerabilities, and their causes and consequences. They focus on hazards, existing linkages to development, risk governance and regulations (Pedro Basabe 2018 ). Therefore, it is essential to understand these frameworks’ common characteristics and terminologies to establish a holistic risk assessment approach (Hasani et al. 2014 ).
In these frameworks, the analysis of the risk environment is carried out by using different quantitative and qualitative assessment methods. Some examples of methods used in risk assessment include possibility theory, fuzzy set, probability theory, and evidence theory (Giorgini 2009 ). Aksha et al. ( 2020 ) have identified various limitations in these current multi-hazard risk assessment approaches, which include: a lack of standard definitions for multi-hazard risk, lack of a holistic approach for integrating risks from different hazards, unavailability of intensive data required for risk assessment, confinement of risk assessments within disciplinary boundaries, and the inherent uniqueness of each place generating geographically specific hazard conditions and outcomes.
Therefore, this study aims to understand how the risk assessment characteristics are discussed in various research studies in the urban environment. It aims to answer the question “What approaches are being used to define and measure the impact of hazard risks in an urban environment?”
2 Literature Survey
This section discusses the literature review approach used to gather relevant research papers to explore the research question. The PICO method was used to generate the search string by considering the keywords in the research question for identifying the relevant literature. The PICO represents four groups: Population, Intervention, Compression intervention, and Outcome. Table 1 presents the initial and extended keywords identified for the literature review.
The keywords were connected with “AND” or “OR” operations. The “OR” connection was used to connect within the group, and the “AND” connection was used to connect between the groups. The following literature searching statement was used to search the research papers that match with keywords, abstract and title of the research papers in the Scopus and the Web of Science databases. Further, eight reports were collected from intergovernmental organisational websites. A total number of 206 research articles were selected for screening.
Literature search statement: (‘Urban’ OR ‘Urban Infrastructure’ OR ‘Urban Development’ OR ‘Urban design*’ OR ‘Urban Plan*’ OR City OR Town) AND (Hazard OR Risk OR ‘Cascading effect’ OR Propagation OR ‘Cascading failure OR ‘Chain Reaction’ Or ‘System thinking’ OR ‘System Dynamics’ OR interdependence*) AND (Resilience OR Impact OR Disaster OR Perturbation OR Damage OR Failure) AND (Model* OR Theory* OR Method* OR Formulas OR Index OR Eq. OR Approach OR Simulation).
Figure 1 shows the PRISMA method of screening the literature records for the study. The following inclusion criteria were used to select the most relevant papers for this study, which are most relevant to generate risk propagation modelling: consider only the research papers published after 1999 in English; consider papers only from the key subjects areas Engineering, Environmental science, Physics, Mathematics, and Social Science, which are more relevant with the research scope. This process resulted in 123 papers for further consideration. After reading the abstracts, only 119 research papers were selected for the full paper review process.
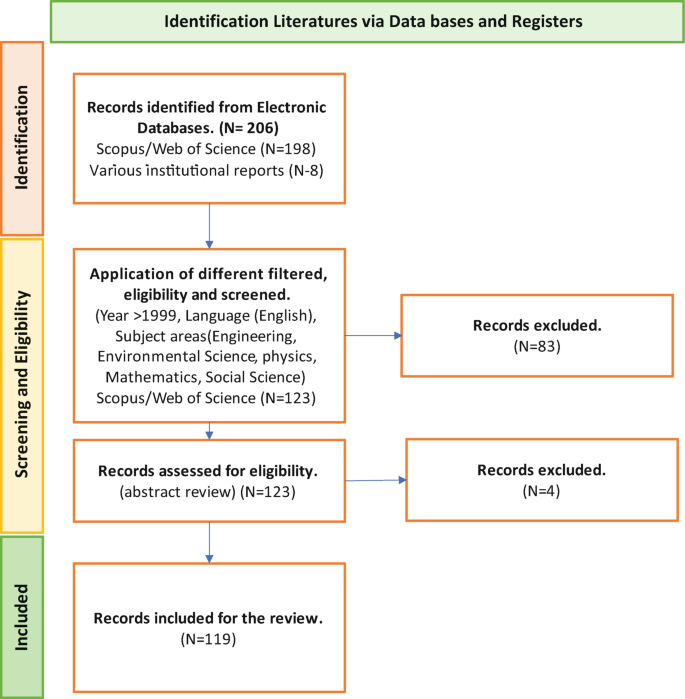
Stages in PRISMA review as carried out in the study
2.1 Detailed Review Process
A systematic/ mapping review approach was selected because of mapping the existing characteristics on a network diagram. Accordingly, each research paper was reviewed to identify key risk assessment characteristics and make a network diagram. The Nvivo12 Pro software was used to support the literature review (Sweet 2014 ), and cases/nodes were created for each risk character. Figure 2 shows different risk management perspectives that were addressed in the chosen research papers in this study.
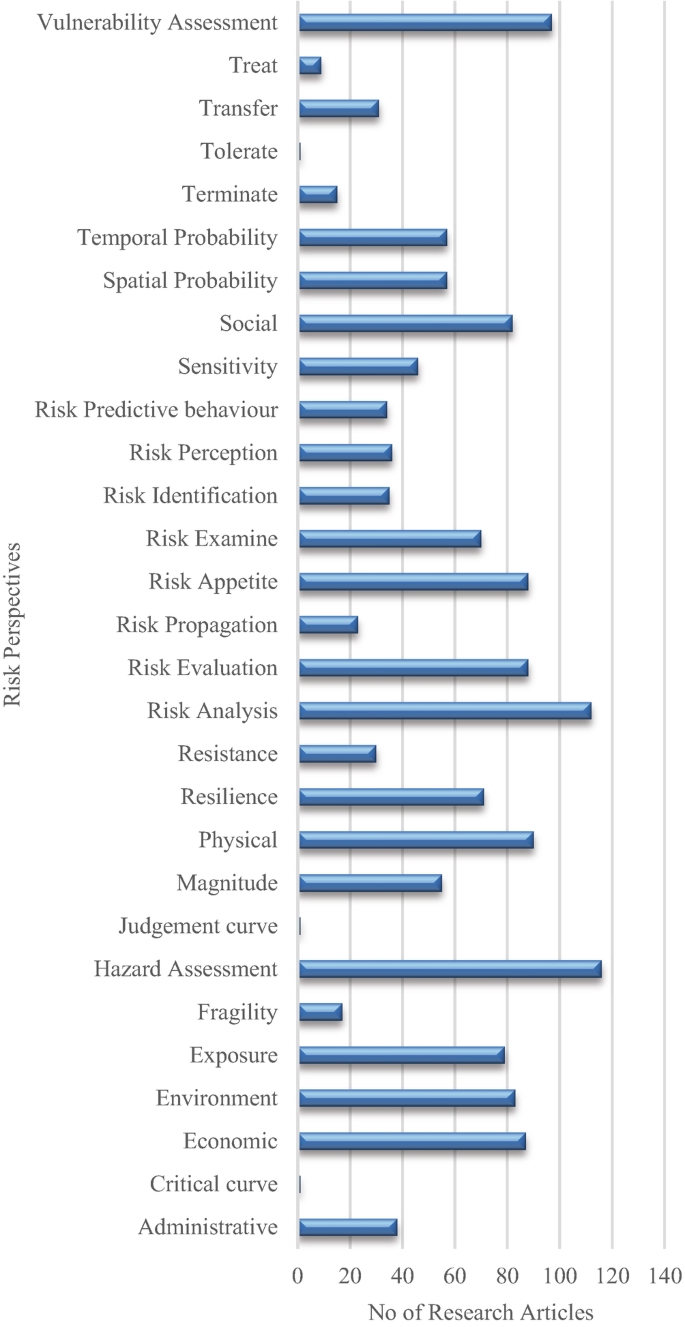
Number of research papers categorised under each risk characteristic
2.2 DRA Characteristics and Percentage of Research Interests
According to the review, 94% of the research papers discussed the risk analysis approaches, and in there, hazard assessments (97%); vulnerability assessment (82%); exposures (66%); risk propagation (19%); and risk perception (30%) were discussed. Although risk appetite was discussed in 74% of the research papers, this aspect was not discussed in detail. For example, the key elements of risk appetite, such as risk thresholds, risk tolerance, and critical or judgment values, were only found in 1% of research studies. The following section discusses the risk analysis and risk analysis methods in detail.
3 Risk Assessment
The risk assessment is an important study in risk management and is conducted to assess the risk status of the community. According to Zlatanova et al. ( 2014 ), risk management is comprised of four major phases; (1) risk identification, (2) risk evaluation, (3) choice and implementation of risk reduction measures and instruments, and (4) monitoring and maintenance of acceptable risk. Within this context of risk management, the first two phases (risk identification and risk evaluation) belong to the risk assessment. However, it is evident that there are many ways to define the key risk assessment activities. For example, ISO-Guide73 ( 2009 ) has defined risk assessment activities as risk understanding, analysis, and evaluation.
In contrast, Corominas et al. ( 2013 ) have proposed risk analysis and risk evaluation as the key phases in risk assessment, where the key activities of risk analysis are hazard identification, hazard assessment, inventory of elements at risk and exposure, vulnerability assessment, and risk estimation. In this phase, hazard identification is the initial step for gaining insights into any threat, uncertainty, vulnerability, and unexpected event that can become a source or trigger for risk to materialise (Tran et al. 2018 ). Risk evaluation is the stage at which values and judgments enter the decision process, explicitly or implicitly, including considerations of the importance of the estimated risks and the associated social, environmental, and economic consequences to identify a range of alternatives for managing the risks (Corominas et al. 2013 ).
Risk Evaluation is considered an essential step in the risk assessment process to guide how to improve the current system to face future risks. At this step, values and judgments enter the decision-making process, explicitly or implicitly, to consider the severity of the estimated risks and the associated social, environmental, and economic consequences and to identify a range of alternatives for managing the risks (Corominas et al. 2013 ).
3.1 Risk Analysis
There is no exact definition for risk, which varies from discipline to discipline (Stock and Wentworth 2019 ; Aksha et al. 2020 ). According to the generic principles, risk analysis is a process of understanding the nature of risk and deciding on the level of risk to provide a basis for risk evaluation and decisions about risk treatment (ISO-Guide73 2009 ). Typically, risk analysis is conducted by considering three variables: hazard, vulnerability, and exposure (Gallopín 2006 ; Bibi et al. 2018 ; Huang et al. 2020 ; Zhou et al. 2020 ).
According to the literature, the risk analysis includes parameters such as consequences, probability of occurrence, hazard, exposure, vulnerability, capacity, triggering factors, cycle incubations, perturbation, range of alternatives, uncertainty, unexpected events, threat and perception (Gallopín 2006 ; Corominas et al. 2013 ; Zlatanova et al. 2014 ; Izquierdo-Horna and Kahhat 2018 ; Tran et al. 2018 ; Huang et al. 2020 ; Zhou et al. 2020 ). The following subsections discuss the connections between the risk analysis variables in detail.
3.2 Risk Analysis Methods
Different risk assessment formulas are used in different assessments, and in general, the risk is considered a function of hazard, vulnerability, and exposure. This section discusses the representation of the risk values to support decision-making. However, Corominas et al. ( 2013 ) have developed a formula for landslide risk assessment by subcategorisation of hazard based on its characteristics of magnitude, spatial probability, and temporal probability. His formula and expression can be described as follows.
where R is the risk due to the occurrence of a landslide of magnitude M i on an element at risk located at a distance X from the landslide source, P(M i ) is the probability of occurrence of a landslide of magnitude Mi, P(X j |M i ) is the probability of the landslide reaching a point located at a distance X from the landslide source with an intensity j, P(T|X j ) is the probability of the element being at the point X at the time of occurrence of the landslide, V ij is the vulnerability of the element to a landslide of magnitude i and intensity j, and C is the monitory value of the element at risk (Corominas et al. 2013 ). Here, the magnitude defines the event’s size when a risk materialises, sometimes referred to as the ‘severity’ of the event, is compared with the impact or consequences of the risk materialising (Hopkin 2010 ). Also, the intensity is expressed by considering the propagation mechanism—for example, the landslide intensity coupled with its volume or the kinetic energy (Corominas et al. 2013 ).
Different theories and approaches are used for different hazard analyses. Mathematical and statistical analysis, quantitative methods, Multi-Criteria Decision Analysis, system dynamic method and uncertainty methods are the most common methodologies to assess disaster risk (Yeganeh and Sabri 2014 ). These theories and approaches could be categorised into four types; (1) Stochastic programming approach, where variables are considered here as random variables with defined probability distributions; (2) Fuzzy programming approach, which considers variables as fuzzy numbers; (3) Stochastic dynamic programming which considers random variables distributed in all areas of multi-stage decision making; (4) Robust optimisation, which finds the optimum result for a given set of input data to an actual scenario (Yeganeh and Sabri 2014 ). Yeganeh and Sabri ( 2014 ) have identified four common techniques that could be used for developing weights for each variable: ranking method, rating method, pairwise comparison method, and trade-off analysis method & comparing method.
Most of the risk assessment has mainly considered the physical features of settlements. However, some studies address the valuation of human life in risk assessments. For example, Zhou et al. ( 2020 ) have considered the ‘Value of Statistical Life (V‘L)’ and calculated risk using the following equation.
Here, direct loss (Loss(I) is calculated by using direct economic loss (LDE (I)) and the loss of VSL(LVSL(I)). The evaluation method of VSL calculates risk as a human capital approach or willingness-to-pay approach (Zhou et al. 2020 ).
The presentations of the risk values are also studied in this survey. Lee et al. ( 2015 ) described that risk values should be classified into zero (0) to one (1) range for easy classification purposes or assigned unique names for different risk classes according to their probabilities and level of perturbations. For example, based on the risk levels, the following names are assigned: Medusa, Cassandra, Pandora, Pythia, Cyclops, and Damocles (Stock and Wentworth 2019 ). A risk matrix is a standard method for representing risk values in most risk assessments (Hopkin 2010 ; Corominas et al. 2013 ). In this method, a plot is generated by individually considering the expected losses against the probability of occurrence of natural hazards. These individual risk matrices could be integrated into the total risk matrix for a specific area. Figure 3 shows the risk matrix diagram, which is a graph considering two dimensions impact and likelihood.
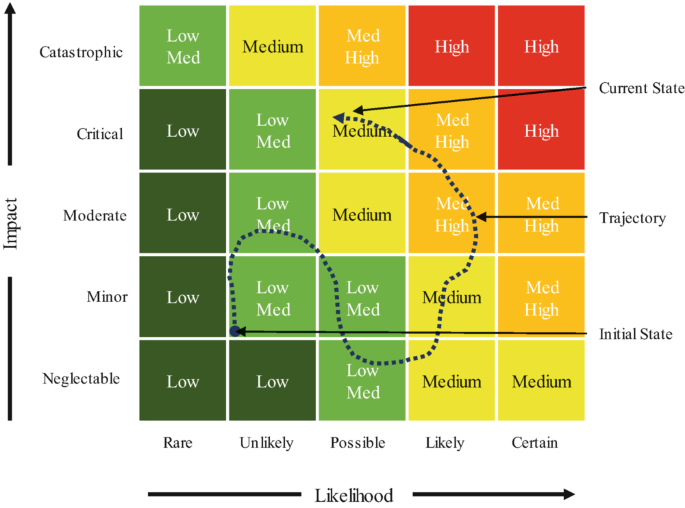
Risk matrix and trajectory curve
Trajectory trend curves can be used to monitor risk values with various criteria, and they can be overlayed with the risk matrix to see the risk value variations with identified variables. This method has been used by Gallopín ( 2006 ) to monitor social resilience values as the ability of groups or communities to cope with external stresses and disturbances due to social, political, and environmental change. Figure 3 illustrates the trajectory trends curve with an initial state, trajectories, and current state. This method has linked with a risk matrix.
3.3 Risk Evaluation Methods
Risk evaluation is a significant step in risk assessment and is discussed in the risk management frameworks. The ISO-Guide73 ( 2009 ) defines risk evaluation as comparing risk analysis results with risk criteria to determine risk appetite. This risk evaluation assists the risk treatment decisions. For example, gathering the stakeholders’ perceptions on risk status can be an evaluation. Kellens et al. ( 2013 ) have described risk perception as a subjective risk assessment that can assess the attendees’ perception of risk status on an identified perturbation. For example, Xu et al. ( 2020 ) have identified that the new communication (media) systems help to increase the community perception level in terms of understanding of risks (magnitude and frequency levels). Therefore, it is required to find literature on risk perception methods that could be used for risk assessments.
3.4 Risk Perception
Risk perception is a complex process that encompasses both cognitive (e.g., likelihood, knowledge) and affective (e.g., feelings, perceived control) aspects (Kellens et al. 2013 ). Two methods are used for risk perception studies: the Psychometric Paradigm and the heuristics approaches (Kellens et al. 2011 , 2013 ). The psychometric Paradigm is an influential and popular theoretical framework in risk perception which attempts to quantify personal risk perception and attitudes through a structured questionnaire. Heuristics, or simple and efficient rules of thumb, are often used to simplify complex problems and make decisions without using all of their cognitive capacities. The prominent approaches to examine risk perceptions are Expectancy Valence Approaches (EV) and Applications of Contingent Valuation Methods (CVM).
Expectancy Valence Approaches (EV) : This approach focuses on the explanation of people’s adaptive behaviour, which can predict by using their valences for different outcomes like a desire to protect oneself, instrumentalities of their performance of actions like installation of flood barriers, and experience of previous successful performances on risk reduction. The concepts are based on psychological theories, and a few other methods are linked with the EV, which could be described as follows.
Protection Motivation Theory (PMT): This tool helps analyse the threat and coping appraisal of a person’s perception. Three constructs are defined as a tool to predict the coping appraisal: response efficiency, self-efficiency, and response cost (Kellens et al. 2013 ). Two constants for predicting the threat appraisal are perceived probability and perceived consequence (Bubeck et al. 2012 ). Bubeck et al. ( 2012 ) have discussed the usability of PMT for flood risk management, emphasising that these aspects should receive greater attention in risk communication policies.
RCSA model (Risk, Coping and Social Appraisal): This model has three appraisals, Risk appraisal (includes a perception of severity and perception of probability), Coping Appraisal (includes self-efficiency and response-efficiency), and Social Appraisal (includes community identification and perceived norms). This tool is used to understand the farmers’ drought adaptation practices and concludes that the tool is a better predictor of adaptation intention than a strictly demographic model (Truelove et al. 2015 ).
Protective Action Decision Model (PADM): This tool is closely like the PMT and is mainly applied for earthquake hazards. Here, efficiency attributes are protecting people, protecting property, and protecting utilities.
Motivation Intention Volition Model (MIV): “Motivation results from perceived risk but may be hampered by a lack of perceived personal responsibility and tendencies to avoid or suppress the perceived threat. A person’s intention to adopt hazard adjustments is further influenced by perceived response and self-efficacy. The violation phase intentions are turned into actions depending on the situational barriers encountered.” (Kellens et al. 2013 )
Risk Information Seeking and Processing Model (RISP): this tool expresses the construct of insufficient information defined as the gap between a person’s current knowledge and his/her knowledge threshold.
Applications of Contingent Valuation Methods (CVM): This economical approach estimates people’s perceptions. This method is focused on people’s willingness to pay (Kellens et al. 2013 ). The technique has been used to analyse earthquake disasters in Taiwan by Chen et al. ( 2020 ). The study shows that people tend to have greater risk perceptions of future hazard risk and less willingness to pay for retrofitting their houses after a severe disaster. Similarly, people with higher education and a better occupation status may be more willing to pay than others to adopt adaptation behaviour.
In addition, there are non-theoretical approaches to examine the risk perceptions which are based on; (1) awareness (or consciousness, e.g., “Are you aware that you live in a flood-prone area?”); (2) affect (or worry, fear, concern, e.g., “Do you feel personally endangered by a flood?”); (3) likelihood (or probability, e.g., “What do you think about the chances of a flood in your neighbourhood within the next ten years?”); (4) impact (or consequences, vulnerability, e.g., “Rate the following statement: A flood will have fatal consequences for my family and me”); and (5) cause (or origin, e.g., “Can you indicate the cause of the flood risk in your neighbourhood?”) (Kellens et al. 2013 ). A perception study has been conducted to assess the acceptability of flood risk in Japan’s Toki River basin in Japan. This study identified that public facilities and services perception loss due to floods increases flood risk’s public acceptability (Zhai and Ikeda 2008 ).
3.5 Risk Predictive Behaviour
Understanding the existing risk and predicting its behaviour are essential to determine the risk reduction approaches (Jemec Auflič et al. 2018 ). Therefore, understanding the probability of hazard occurrence at the hazard assessment stage is significant, and it involves stakeholder and community engagements to identify the possible hazards (Jemec Auflič et al. 2018 ). Risk perception techniques are required to capture the existing and possible neighbourhood hazards (Kellens et al. 2013 ).
The training and knowledge-sharing sessions are required to conduct frequently to upgrade the existing knowledge of the community and stakeholders to capture the hazard impacts. For example, the Learning-by-doing approach for community awareness of the landslide proved more effective in community awareness programmes (Jemec Auflič et al. 2018 ). However, the effectiveness of community awareness activities depended on the social classes, impact experience, and affect-length of residence in hazardous areas (Kellens et al. 2013 ).
Hypothesis or scenarios are required to investigate the community risk by changing various parameters (Xu et al. 2020 ). Then, the damage assessment (possible) is conducted by considering the hypothesised events and impact on the properties (Xu et al. 2020 ). Based on the outputs, the risk acceptance is discussed by referring to the cost-benefit or the consequences of the damage assessment (Zhai and Ikeda 2008 ; Stock and Wentworth 2019 ). The emergency planning activities are determined based on these risk acceptance priorities (Stock and Wentworth 2019 ).
The worst-case and the most-probable scenarios play a significant role in emergency planning (Stock and Wentworth 2019 ). Governments should communicate the most-probable scenario to the public, and community engagement may help validate the scenario outputs by acquiring geographic and physical information (Stock and Wentworth 2019 ). The reasonable worst-case scenario can represent the challenge to the community but is not necessarily informative for businesses and the public. However, it should be considered in the risk assessment (Stock and Wentworth 2019 ).
3.6 Risk Appetite
Risk evaluation gives some idea of the risk mitigation options, and risk appetite is for identifying risk capacities related to a settlement. Hopkin ( 2010 ) has discussed risk appetite as a technique to identify threshold levels in each risk category; Risk accepts, adopts, adapts, and avoids. The judgement and critical lines could be identified based on the levels of risk management activities (Hopkin 2010 ). Both lines are plotted on the risk matrix, and the judgement line represents the margin between the comfort and cautious zones. If the risk level belongs to the comfort zone, the dominant response will be Risk Tolerate, and if the risk level belongs to the cautious zone, the dominant response will be either Risk Transfer or Risk Treat (Hopkin 2010 ). The critical line of risk is the Cautious and Concerned zone’s margin. The dominant response will be risk Terminate if the risk level belongs to the concerned zone (Hopkin 2010 ).
4 Discussion
4.1 disaster risk assessment dynamic connections.
Risk assessment characteristics were summarised in the previous sections, and a conceptual diagram was developed for a better presentation. Accordingly, the risk assessment is comprised of three activities: identification, analysis, and evaluation. The risk analysis has three major components: Hazard, Vulnerability, and Exposure assessment. Gallopín ( 2006 ) has identified the relationship between each component of hazard, vulnerability, and exposure of the human settlements. Vulnerable elements are the internal processes of human settlements, and hazards or perturbations are the external processes of human settlements.
According to the literature review, hazard assessment has four major elements: spatial probability, temporal probability, magnitude, and resistances. The vulnerability assessment consists of physical, social, economic, environmental, governance, resilience, sensitivity, and fragility. The risk evaluation assessment divides into two: risk perception and risk appetite (through a risk matrix). Risk perceptions assess people’s perception of known perturbation, and it has two elements: risk examination and risk prediction behaviour. Risk appetite is how people assess the limits and bounds of the risk status. It is a matrix of risk-treating options (Risk tolerance, risk transfer, risk treatment, and risk termination), which is influenced by risk judgment and critical curves. All these concepts were connected as a system in Fig. 4 to illustrate the risk perspectives needed in risk assessment.
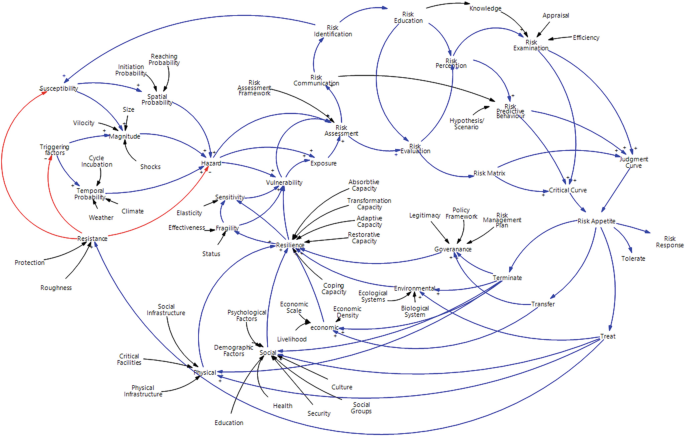
Risk perspectives connections (a mind map)
4.2 Risk Reduction Strategies
Figure 4 shows the risk perspectives identified through the literature review. Accordingly, a stock-flow diagram was developed to understand the balancing and reinforced loops. The negative (Red) and positive (Blue) connections were also identified in the loops. These loops guide the development of risk-reduction strategies. Consequently, 14 strategies were identified by analysing the balancing loops, connected to the risks, in the network, as shown in Fig. 5 .
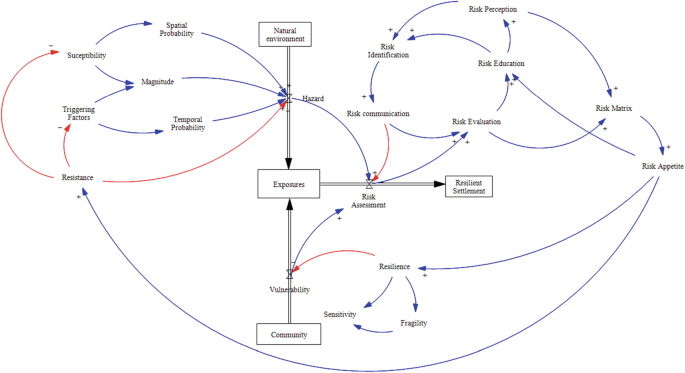
Stock-flow diagram for risk assessment perspective
The following section proposes strategies that can be used to reduce the risks by analysing the identified loops.
Update the risk education programs with recent risk evaluation experiences ( Risk → Risk Assessment → Risk Education → Risk Identification → Risk Communication → Risk)—This balancing loop suggests that by introducing risk education programs, updated with the outcomes of recent disaster assessments, will lead to effective risk identification and communication, and consequently reducing overall local risks on the community.
Update community risk perception to enhance their coping capacities. (Risk → Risk Assessment → Risk Education → Risk Perception → Risk Identification → Risk Communication → Risk)—This balancing loop indicates that it is necessary to frequently measure the risk perception of the community, using the tools discussed under the risk perception section, and introduce community activities that can help them to gain an accurate understanding of their local risks. Such activities can make the community an active stakeholder in risk reduction and communication.
Risk education programmes should be informed through evidence-based approaches. ( Risk → Risk Assessment → Risk Matrix → Risk Appetite → Risk Education → Risk Identification → Risk communication → Risk)—A thorough analysis should be conducted to identify the risks and how to control them through risk appetite. The outcome of this approach should play a major role in educating the communities on why certain risk management decisions are being made. These approaches should be communicated to the communities.
Bring the community as an active participant in reducing local risks to safeguard their livelihoods ( Risk → Risk Assessment →Risk Matrix → Risk Appetite → Risk Education → Risk Perception → Risk Identification → Risk communication → Risk)—This balancing loop suggests that by bringing communities as an active participant in the whole risk management process to reduce the local risks and to protect their livelihoods. The risk-management actions should be practised and absorbed into their livelihood by providing appropriate training.
Improve the resilience capacities to reduce vulnerabilities ( Risk → Risk Assessment → Risk Matrix → Risk Appetite → Resilience → Vulnerability → Risk)—The actions carried out within the risk appetite will enhance resilience capacities (anticipatory, absorptive, restorative, transformative, coping) of various dimensions (physical, social, economic, environmental, and governance) hence reducing the vulnerabilities in the settlements for various hazards.
Deploy community-based approaches to defining and addressing resilience and vulnerabilities. (Risk → Risk Assessment → Risk Education → Risk Perception → Risk Matrix → Risk Appetite → Resilience → Vulnerability → Risk)—Risk perception of the community influences the local risk appetite for choosing appropriate interventions for enhancing community resilience and reducing community vulnerabilities. Therefore, communities’ risk knowledge and risk perception can influence their attitude towards investment in various risk reduction activities, such as the implementation of building codes and the construction of retaining walls.
Promote ecosystem management approaches for reducing hazards ( Risk → Risk Assessment → Risk Matrix → Risk Appetite → Resistance → Hazard → Risk)—This balancing loop suggests that risk appetite measures influence resistance to hazards. Therefore, ecosystem management approaches, including structural or non-structural methods, must be introduced for building resistance. As an example, the introduction of appropriate native plants as a nature-based solution can help build resistance to hazards.
Communicate the importance of ecosystem management in mitigating hazards. ( Risk → Risk Assessment → Risk Education → Risk Perception → Risk Matrix → Risk Appetite → Resistance → Hazard → Risk)—The local community should be aware of the importance of ecosystem management for their livelihood through risk education. For example, communities should understand how deforestation in mountainous slopes can lead to potential landslides. The community must practice such risk-reduction approaches to mitigate or reduce hazard occurrences.
Promote ecosystem restoration programs at susceptible locations. ( Risk → Risk Assessment → Risk Matrix → Risk Appetite → Resistance → Susceptibility → Spatial Probability → Hazard → Risk)—The susceptible hazard locations can quickly reach the threshold to trigger a hazard. Therefore, these locations should be thoroughly investigated and introduce nature-based mitigation solutions. For example, SABO dams can be created to control the debris flow and protect the surrounding areas from overflowing water.
Implement a community-led approach to hazard Mitigation at critical locations. ( Risk → Risk Assessment → Risk Education → Risk Perception → Risk Matrix → Risk Appetite → Resistance → Susceptibility → Spatial Probability→ Hazard)—The local knowledge of risk perception should be used to identify critical susceptible hazard locations and introduce structural or non-structural mitigation solutions to control the hazard magnitude.
Prioritise ecosystem solutions at locations which can trigger hazards of high magnitude. ( Risk → Risk Assessment → Risk Matrix → Risk Appetite → Resistance → Susceptibility → Magnitude → Hazard)—It is important to Identify and mitigate the hazard with high magnitudes, by introducing structural or non-structural solutions. Here hazard mitigation is concerned with reducing or eliminating the triggering factors that lead to a high-magnitude hazard. For example, groundwater levels in the landslide-prone area have significantly influenced the triggering of landslides. Therefore, horizontal drains can be applied to remove the excess water on the slope to reduce the magnitude of the triggering factors.
Deploy community-based Hazard Mitigation applications for reducing hazard magnitudes (Risk → Risk Assessment → Risk Education → Risk Perception → Risk Matrix → Risk Appetite → Resistance → Susceptibility → Magnitude → Hazard)—Some measure leads to reducing the hazard magnitude or slowing down the event. For example, flood weirs and SABO dams were constructed to control the flood water and debris flow speeds. This structural measure leads to reducing the hazard magnitude and reducing impacts.
Communities can understand the hazard triggering factors’ temporal variations: ( Risk → Risk Assessment → Risk Matrix → Risk Appetite → Resistance → Triggering Factors → Temporal Probability → Hazard →Risk)—The strategy focuses on understanding the triggering factor’s frequencies. Accordingly, the community can take disaster preparedness actions if they know the triggering factors’ changes. For example, rainfall is a triggering factor for landslides, and the community should be aware of the frequency of rainfall changes with time.
Implement hazard mitigation applications to reduce hazard frequencies by communities: ( Risk → Risk Assessment → Risk Education → Risk Perception → Risk Matrix → Risk Appetite → Resistance → Triggering Factors → Temporal Probability → Hazard →Risk)—This strategy focuses on reducing hazard frequencies by application of mitigation measures. For example, soil erosions occur due to rapid water flow from mountainous areas. However, the forest areas act as water sponges, gradually releasing the water to the downslope areas. Therefore, it helps to reduce the hazard frequencies in the downslope areas.
Selecting appropriate strategies for risk reduction is challenging in disaster risk assessment. The application of risk reduction strategies is discussed in the risk response stage, which needs to be more focused in the research study.
4.3 Future Research Requirements
The mind map was converted into a risk perspective network diagram by establishing the connections between the variables, and identified literature percentage values could be assigned as the node weight. The edge distance was considered a constant value equal to one. A graph was developed using the Gephi Software, and analysis of the network parameters aligned risk perspectives. The discussion was based on the above analysis.
The graph structure with various risk perspectives presented in Fig. 4 was analysed using the following three tests to identify the key risk perspectives that should be further researched: (1) degree of nodes, (2) closeness centrality, and (3) eigenvector centrality.
Test 1 : The degree of a node represents how many connections it has to other nodes in a graph. The higher the degree, a node has more influence on other nodes. Figure 6 shows the degree of nodes of all the risk perspectives. In this figure, the size of the node represents the degree of the node (the larger the circle higher the degree), and the colour variation represents how well it is researched (the darker the colour, the higher the number of research publications). These two variables are illustrated in Fig. 7 as a bar chart, with blue bars representing the degree of nodes (Degree) and red bars representing the research density(Node_w). This analysis assumes that nodes with a higher degree of nodes but with fewer research publications need further research.
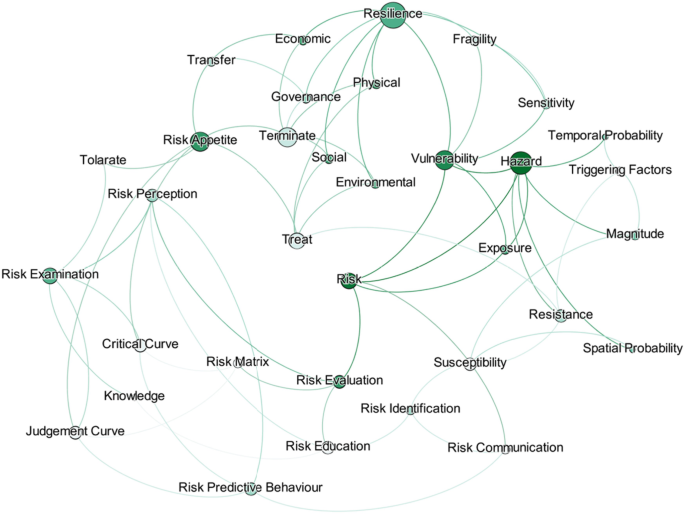
Test 1 results based on the degree of nodes and research density
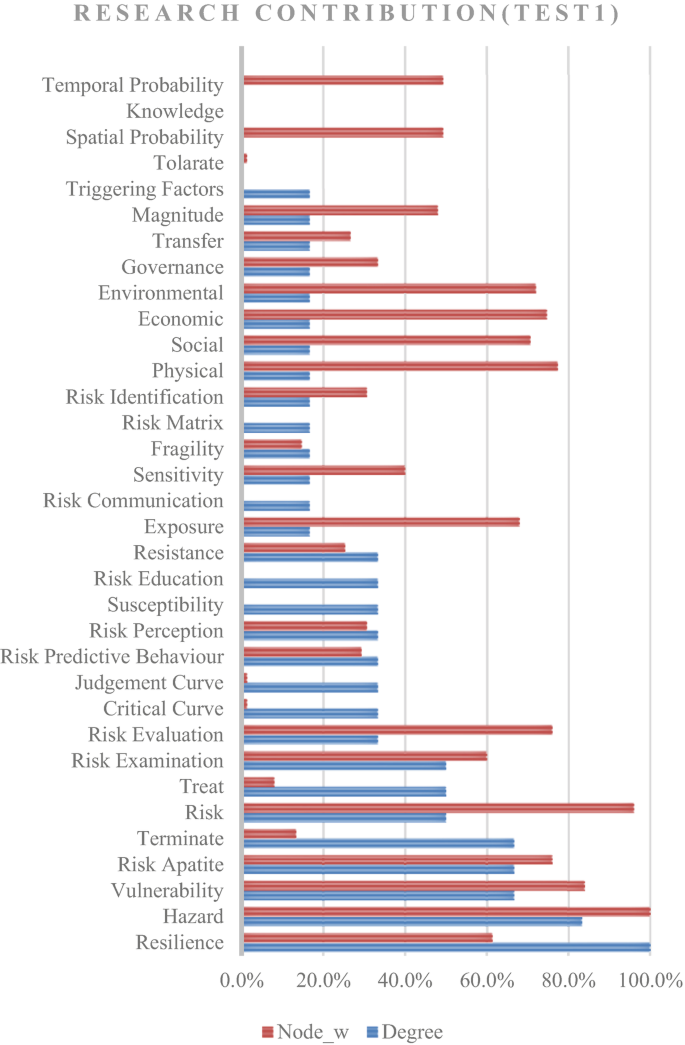
Test 1 a results as a bar chart
This analysis shows that resilience has the highest degree of node value, and hazard has the highest research publications. It is clear that further research is required in the areas such as Resilience, Risk treatment, risk termination, susceptibility, Risk education, and risk Predictive behaviour.
Test 2: The closeness centrality of the network was assessed to understand the most central risk perspective of the risk assessment. Nodes with the highest closeness centrality are considered as important and significant in a graph. Accordingly, a network graph was developed representing the node size as closeness centrality (the larger the node higher the closeness centrality) and colour density representing research density (the darker the colour, the higher the number of research publications), as shown in Fig. 8 . These two variables are illustrated in Fig. 9 as a bar chart, red bars representing the closeness centrality and blue bars representing the research density. This analysis assumes that nodes with higher closeness centrality but with fewer research publications need further research.
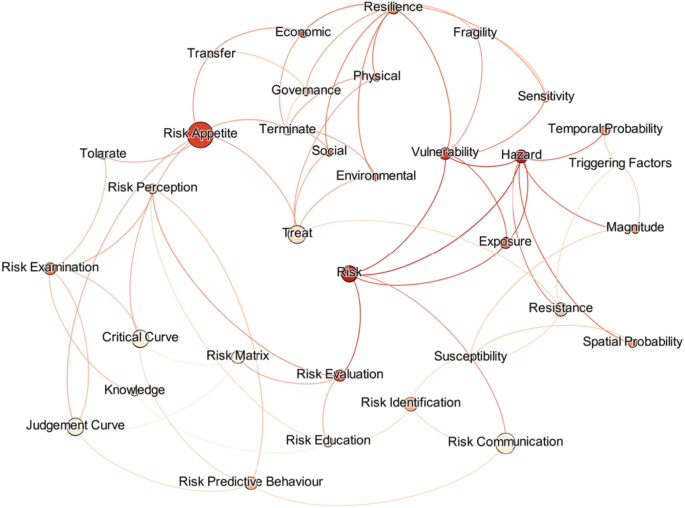
Test2 results based on closeness centrality and research density
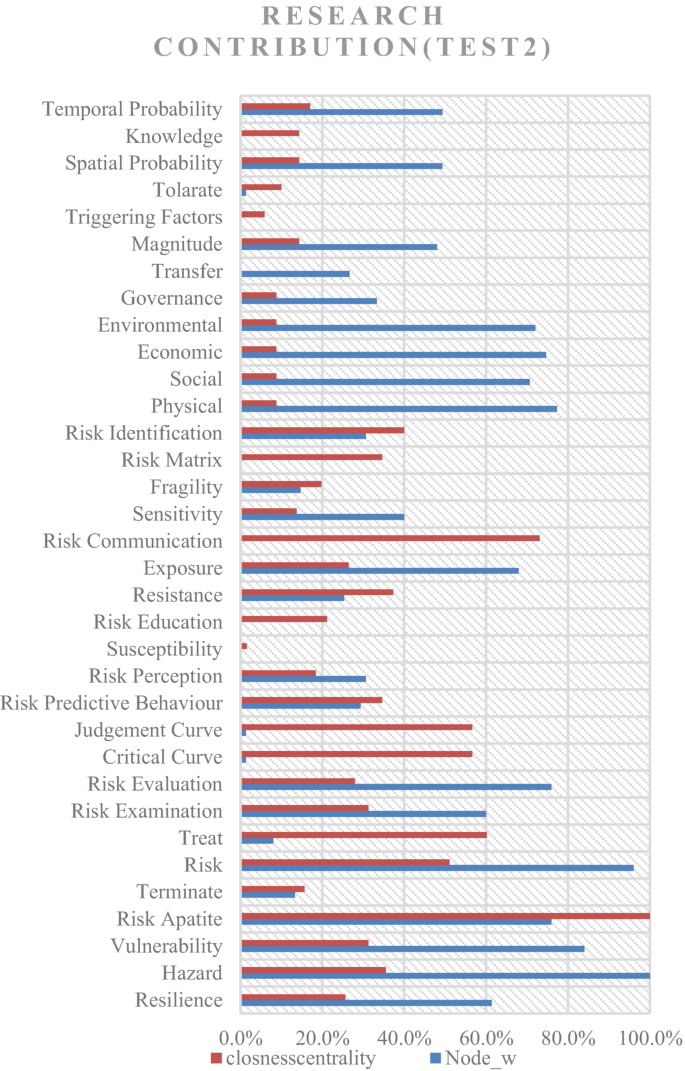
Test 2 result as a bar chart
Figure 8 and 9 indicate that the risk appetite has the highest closeness centrality value of the network and highlights the need for further research in risk perspectives such as risk appetite, risk communication, risk treatment, critical curves, judgment curves, and risk identification.
Test 3: Eigenvector centrality was assessed to understand the common nodes in the shortest path that have been adequately researched. Accordingly, a network graph was used to analyse this test.
Figure 10 shows the integrated network diagram of risk perspective density and eigenvector centrality. The significance of eigenvector centrality lies in its ability to capture the idea of “prominence” within a network. Nodes with high eigenvector centrality are not only well-connected but are also connected to other highly central nodes, making them influential within the network. Therefore, the eigenvector centrality of each node was calculated to identify the most influential risk perspectives. Figure 10 shows eigenvector centrality (the larger the node, the higher the eigenvector centrality) and colour density representing research density (the darker the colour, the higher the number of research publications). These two variables are illustrated in Fig. 11 as a bar chart, the blue bars representing the eigenvector centrality and the red bars representing the research density. This analysis assumes that nodes with higher eigenvector centrality but fewer research publications need further research.
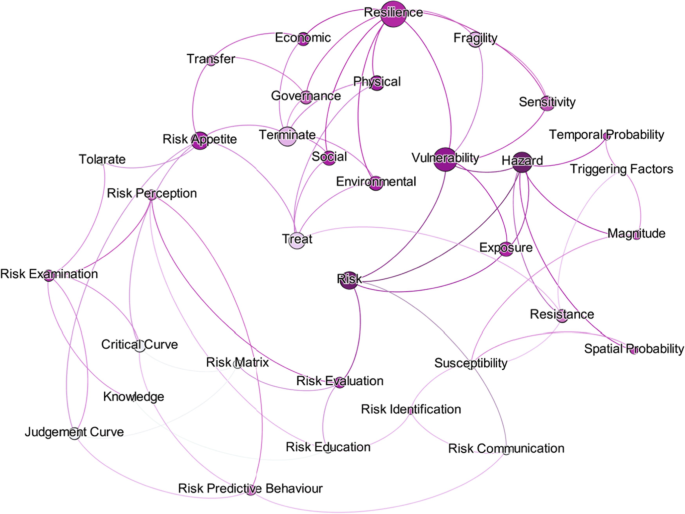
Test3 results based on eigenvector centrality and research density
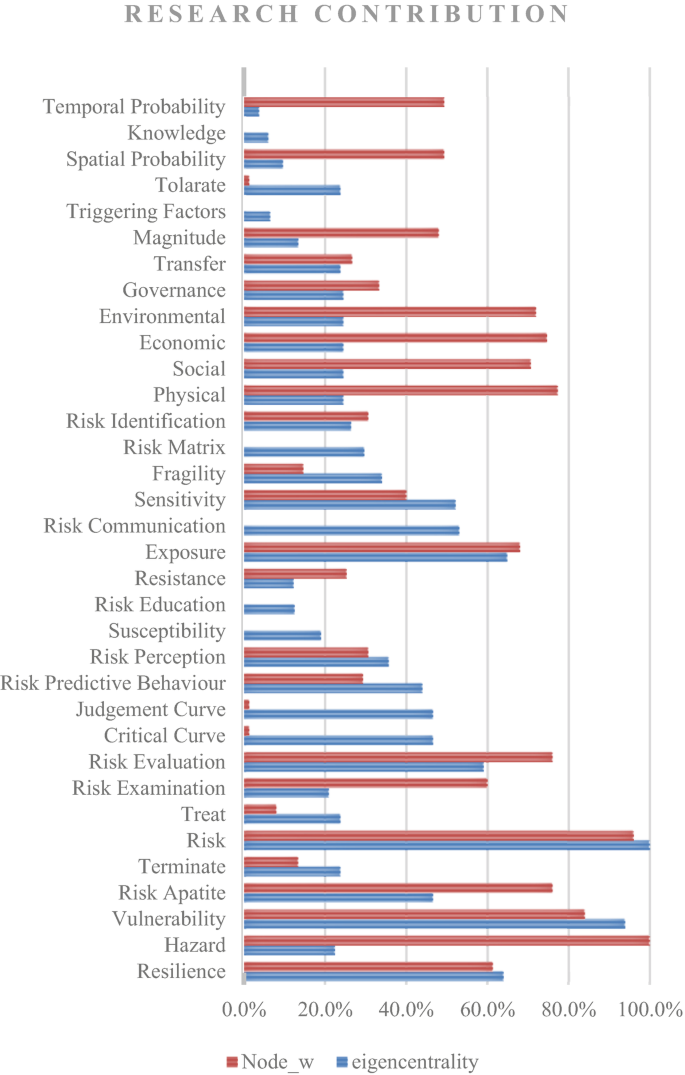
Test 3 results as a bar chart
The analysis of Figs. 10 and 11 shows that risk perspectives such as vulnerability, resilience, risk communication, and risk matrix need further research.

Research requirement assessment
According to this analysis, risk perspectives such as risk communication, risk treatment, critical curve, judgment curve, and risk matrix could be identified as areas requiring future research.
5 Conclusion
This research paper focused on understanding the risk assessment perspectives discussed by various researchers in the disaster risk domain. Accordingly, a systematic literature review was conducted to identify the literature, and a detailed review was conducted with NVIVO software’s support. As a result, 34 risk assessment perspectives were identified and plotted as a network diagram for better representation. Accordingly, the identified risk perspectives were discussed in the initial section of the research paper.
A stock-flow diagram was developed by considering the network diagram, which helps to generate the overall risk reduction strategies. Here, the stock flow diagram helped to identify the balancing and reinforcing loops to determine the risk reduction measures. Accordingly, 14 balancing loops were identified and analysed to propose risk reduction strategies.
Furthermore, the study analysed future research needs by analysing the importance of each risk perspective and the number of publications against each risk perspective. Consequently, the network diagram was further evaluated by conducting three tests on the network (degree of nodes, closeness centrality and eigenvector centrality). The average results of these three tests show that further research is required in areas such as risk communication, risk matrix, and risk treatment.
The research outputs will be validated in the SATREPS case study locations specified in the landslide risk management in Sri Lanka. A project objective refers to strengthening risk communication to reduce landslide risk. Therefore, the community’s and stakeholder’s perceptions will be evaluated concerning the stock-flow diagram. The identified actions will be implemented under the purview of the National Building Research Organisation.
Aksha SK, Resler LM, Juran L, Carstensen LW (2020) A geospatial analysis of multi-hazard risk in Dharan, Nepal. Geomat Nat Haz Risk 11(1):88–111
Article Google Scholar
Bibi T, Nawaz F, Abdul Rahman A, Azahari Razak K, Latif A (2018) Flood risk assessment of river Kabul and SWAT catchment area: district Charsadda, Pakistan. International archives of the photogrammetry, Remote Sensing and Spatial Information Sciences - ISPRS Archives
Google Scholar
Bubeck P, Botzen WJ, Aerts JC (2012) A review of risk perceptions and other factors that influence flood mitigation behavior. Risk Anal 32(9):1481–1495
Cabinet-Office-UK (2020) National Risk Register 2020
Chen T-L, Chao T-Y, Cheng H-T (2020) Exploring the changes in risk perceptions and adaptation behaviors based on various socioeconomic characteristics before and after earthquake disasters – a case study in Taiwan. Nat Hazards Earth Syst Sci 20(9):2433–2446
Corominas J, van Westen C, Frattini P, Cascini L, Malet JP, Fotopoulou S, Catani F, Van Den Eeckhaut M, Mavrouli O, Agliardi F, Pitilakis K, Winter MG, Pastor M, Ferlisi S, Tofani V, Hervás J, Smith JT (2013) Recommendations for the quantitative analysis of landslide risk. Bull Eng Geol Environ 73(2):209–263
Gallopín GC (2006) Linkages between vulnerability, resilience, and adaptive capacity. Glob Environ Chang 16(3):293–303
Giorgini P (2009) Tropos: risk analysis
Hasani S, El-Haddadeh R, Aktas E (2014) A disaster severity assessment decision support tool for reducing the risk of failure in response operations. WIT Transactions on Information and Communication Technologies
Hopkin P (2010) Fundamentals of risk management: understanding evaluating, and implementing effective risk management. The Institute of Risk Management
Huang X, Li Y, Guo Y, Zheng D, Qi M (2020) Assessing urban risk to extreme heat in China. Sustainability 12(7)
ISO-Guide73 (2009) ISO guide 73–2009 risk management vocabulary. ISO
Izquierdo-Horna L, Kahhat R (2018) Methodological framework to integrate social and physical vulnerability in the prevention of seismic risk. WIT Trans Eng Sci
Jemec Auflič M, Kumelj Š, Peternel T, Jež J (2018) Understanding of landslide risk through learning by doing: case study of Koroška Bela community, Slovenia. Landslides 16(9):1681–1690
Kellens W, Terpstra T, De Maeyer P (2013) Perception and communication of flood risks: a systematic review of empirical research. Risk Anal 33(1):24–49
Kellens W, Zaalberg R, Neutens T, Vanneuville W, De Maeyer P (2011) An analysis of the public perception of flood risk on the Belgian coast. Risk Anal 31(7):1055–1068
Khazai B, Kunz-Plapp T, Büscher C, Wegner A (2014) VuWiki: an ontology-based semantic wiki for vulnerability assessments. Int J Disaster Risk Sci 5(1):55–73
Lee T-L, Chen C-H, Pai T-Y, Wu R-S (2015) Development of a meteorological risk map for disaster mitigation and management in the Chishan Basin, Taiwan. Sustainability 7(1):962–987
Munasinghe D, Wijegunarathne E (2015) Vulnerability assessment methods for land subsidence prone areas -a case study: Matale municipal council area. NBRO research symposium 2015. Colombo, NBRO
Nations U (1989) Resolutions adopted on the reports of the second committee, United Nations: 161–162
Nations U (2015a) Sendai framework for disaster risk reduction 2015–2030
Nations U (2015b) Transforming our world: the 2030 agenda for sustainable development
OECD (2012) Disaster risk assessment and risk financing
Pedro Basabe (2018) Comparing and contrasting approaches to risk governance. IRGC
Stock M, Wentworth J (2019) Evaluating UK natural hazards: the national risk assessment, Parliamentary Office of Science and Technology (POST)
Sweet L (2014) Using NVivo and EndNote for literature reviews
Torre DMG, d Cruz PKA, Jose RP, Gatdula NB, Blanco AC (2019) Geospatial assessment of vulnerabilities of croplands to flooding risks: a case study of philippine river basins. International archives of the photogrammetry, remote sensing and spatial information sciences, XLII-4/W19: 173–180
Tran TH, Dobrovnik M, Kummer S (2018) Supply chain risk assessment: a content analysis-based literature review. Int J Logistics Syst Manage 31(4):562–591
Truelove HB, Carrico AR, Thabrew L (2015) A socio-psychological model for analyzing climate change adaptation: a case study of Sri Lankan paddy farmers. Glob Environ Chang 31:85–97
UNDRR (2015) Sendai Framework for Disaster Risk Reduction 2015–2030 from https://www.undrr.org/publication/sendai-framework-disaster-risk-reduction-2015-2030
Xu D, Zhuang L, Deng X, Qing C, Yong Z (2020) Media exposure, disaster experience, and risk perception of rural households in earthquake-stricken areas: evidence from rural China. Int J Environ Res Public Health 17(9)
Yeganeh N, Sabri S (2014) Flood vulnerability assessment in Iskandar Malaysia using multi-criteria evaluation and fuzzy logic. Res J Appl Sci Eng Technol 8(16):1794–1806
Zhai G, Ikeda S (2008) Empirical analysis of Japanese flood risk acceptability within multi-risk context. Nat Hazards Earth Syst Sci 8(5):1049–1066
Zhou S, Zhai G, Shi Y, Lu Y (2020) Urban seismic risk assessment by integrating direct economic loss and loss of statistical life: an empirical study in Xiamen, China. Int J Environ Res Public Health 17(21):1–21
Zlatanova S, Ghawana T, Kaur A, Neuvel JMM (2014) Integrated flood disaster management and spatial information: case studies of Netherlands and India. International Archives of the Photogrammetry, Remote Sensing and Spatial Information Sciences - ISPRS Archives
Download references
Acknowledgment
This research was conducted as part of a Ph.D. study under the TRANSCEND project at the University of Salford, UK. Also, we would like to extend our appreciation to the SATREPS project team for their constant support, guidance, and encouragement throughout our research journey. Their expertise, timely feedback, and commitment to excellence have been instrumental in shaping the outcomes of our study.
Author information
Authors and affiliations.
National Building Research Organisation, Jaffna, Sri Lanka
Dayan Munasinghe & Asiri Karunawardena
University of Salford, School of Science, Engineering, and Environment, Manchester, UK
Terrance Fernando & Kaushal Keraminiyage
You can also search for this author in PubMed Google Scholar
Editor information
Editors and affiliations.
Institute of Geography, Natl Autonomous University of Mexico, Ciudad De Mexico, Estado de México, Mexico
Irasema Alcántara-Ayala
Faculty of Civil Engineering, University of Rijeka, Rijeka, Croatia
Željko Arbanas
Geological Survey of Canada, Vancouver, BC, Canada
David Huntley
International Consortium on Landslides, Kyoto, Japan
Kazuo Konagai
Fac. Mining, Geology and Petroleum Eng., University of Zagreb, Zagreb, Croatia
Snježana Mihalić Arbanas
Faculty of Civil & Geodetic Engineering, University of Ljubljana, Ljubljana, Slovenia
Matjaž Mikoš
Amrita Cent. for Wireless Networks, App., Amrita School of Sustainable Development, Amritapuri, India
Maneesha V. Ramesh
Kyoji Sassa
National Institute of Maritime, Port and Aviation Technology, Port and Airport Research Institute, Yokosuka, Japan
Shinji Sassa
Engineering Faculty, China University of Geoscience, Wuhan, China
Huiming Tang
Civil & Environmental Engineering, California State University, Fullerton, Fullerton, CA, USA
Binod Tiwari
Rights and permissions
Open Access This chapter is licensed under the terms of the Creative Commons Attribution 4.0 International License ( http://creativecommons.org/licenses/by/4.0/ ), which permits use, sharing, adaptation, distribution and reproduction in any medium or format, as long as you give appropriate credit to the original author(s) and the source, provide a link to the Creative Commons license and indicate if changes were made.
The images or other third party material in this chapter are included in the chapter's Creative Commons license, unless indicated otherwise in a credit line to the material. If material is not included in the chapter's Creative Commons license and your intended use is not permitted by statutory regulation or exceeds the permitted use, you will need to obtain permission directly from the copyright holder.
Reprints and permissions
Copyright information
© 2023 The Author(s)
About this chapter
Cite this chapter.
Munasinghe, D., Fernando, T., Keraminiyage, K., Karunawardena, A. (2023). A Review of the Disaster Risk Assessment Perspectives. In: Alcántara-Ayala, I., et al. Progress in Landslide Research and Technology, Volume 2 Issue 2, 2023. Progress in Landslide Research and Technology. Springer, Cham. https://doi.org/10.1007/978-3-031-44296-4_18
Download citation
DOI : https://doi.org/10.1007/978-3-031-44296-4_18
Published : 29 December 2023
Publisher Name : Springer, Cham
Print ISBN : 978-3-031-44295-7
Online ISBN : 978-3-031-44296-4
eBook Packages : Earth and Environmental Science Earth and Environmental Science (R0)
Share this chapter
Anyone you share the following link with will be able to read this content:
Sorry, a shareable link is not currently available for this article.
Provided by the Springer Nature SharedIt content-sharing initiative
- Publish with us
Policies and ethics
- Find a journal
- Track your research
- Search Menu
- Browse content in Arts and Humanities
- Browse content in Archaeology
- Anglo-Saxon and Medieval Archaeology
- Archaeological Methodology and Techniques
- Archaeology by Region
- Archaeology of Religion
- Archaeology of Trade and Exchange
- Biblical Archaeology
- Contemporary and Public Archaeology
- Environmental Archaeology
- Historical Archaeology
- History and Theory of Archaeology
- Industrial Archaeology
- Landscape Archaeology
- Mortuary Archaeology
- Prehistoric Archaeology
- Underwater Archaeology
- Urban Archaeology
- Zooarchaeology
- Browse content in Architecture
- Architectural Structure and Design
- History of Architecture
- Residential and Domestic Buildings
- Theory of Architecture
- Browse content in Art
- Art Subjects and Themes
- History of Art
- Industrial and Commercial Art
- Theory of Art
- Biographical Studies
- Byzantine Studies
- Browse content in Classical Studies
- Classical History
- Classical Philosophy
- Classical Mythology
- Classical Literature
- Classical Reception
- Classical Art and Architecture
- Classical Oratory and Rhetoric
- Greek and Roman Epigraphy
- Greek and Roman Law
- Greek and Roman Archaeology
- Greek and Roman Papyrology
- Late Antiquity
- Religion in the Ancient World
- Digital Humanities
- Browse content in History
- Colonialism and Imperialism
- Diplomatic History
- Environmental History
- Genealogy, Heraldry, Names, and Honours
- Genocide and Ethnic Cleansing
- Historical Geography
- History by Period
- History of Agriculture
- History of Education
- History of Emotions
- History of Gender and Sexuality
- Industrial History
- Intellectual History
- International History
- Labour History
- Legal and Constitutional History
- Local and Family History
- Maritime History
- Military History
- National Liberation and Post-Colonialism
- Oral History
- Political History
- Public History
- Regional and National History
- Revolutions and Rebellions
- Slavery and Abolition of Slavery
- Social and Cultural History
- Theory, Methods, and Historiography
- Urban History
- World History
- Browse content in Language Teaching and Learning
- Language Learning (Specific Skills)
- Language Teaching Theory and Methods
- Browse content in Linguistics
- Applied Linguistics
- Cognitive Linguistics
- Computational Linguistics
- Forensic Linguistics
- Grammar, Syntax and Morphology
- Historical and Diachronic Linguistics
- History of English
- Language Acquisition
- Language Variation
- Language Families
- Language Evolution
- Language Reference
- Lexicography
- Linguistic Theories
- Linguistic Typology
- Linguistic Anthropology
- Phonetics and Phonology
- Psycholinguistics
- Sociolinguistics
- Translation and Interpretation
- Writing Systems
- Browse content in Literature
- Bibliography
- Children's Literature Studies
- Literary Studies (Asian)
- Literary Studies (European)
- Literary Studies (Eco-criticism)
- Literary Studies (Modernism)
- Literary Studies (Romanticism)
- Literary Studies (American)
- Literary Studies - World
- Literary Studies (1500 to 1800)
- Literary Studies (19th Century)
- Literary Studies (20th Century onwards)
- Literary Studies (African American Literature)
- Literary Studies (British and Irish)
- Literary Studies (Early and Medieval)
- Literary Studies (Fiction, Novelists, and Prose Writers)
- Literary Studies (Gender Studies)
- Literary Studies (Graphic Novels)
- Literary Studies (History of the Book)
- Literary Studies (Plays and Playwrights)
- Literary Studies (Poetry and Poets)
- Literary Studies (Postcolonial Literature)
- Literary Studies (Queer Studies)
- Literary Studies (Science Fiction)
- Literary Studies (Travel Literature)
- Literary Studies (War Literature)
- Literary Studies (Women's Writing)
- Literary Theory and Cultural Studies
- Mythology and Folklore
- Shakespeare Studies and Criticism
- Browse content in Media Studies
- Browse content in Music
- Applied Music
- Dance and Music
- Ethics in Music
- Ethnomusicology
- Gender and Sexuality in Music
- Medicine and Music
- Music Cultures
- Music and Religion
- Music and Culture
- Music and Media
- Music Education and Pedagogy
- Music Theory and Analysis
- Musical Scores, Lyrics, and Libretti
- Musical Structures, Styles, and Techniques
- Musicology and Music History
- Performance Practice and Studies
- Race and Ethnicity in Music
- Sound Studies
- Browse content in Performing Arts
- Browse content in Philosophy
- Aesthetics and Philosophy of Art
- Epistemology
- Feminist Philosophy
- History of Western Philosophy
- Metaphysics
- Moral Philosophy
- Non-Western Philosophy
- Philosophy of Science
- Philosophy of Action
- Philosophy of Law
- Philosophy of Religion
- Philosophy of Language
- Philosophy of Mind
- Philosophy of Perception
- Philosophy of Mathematics and Logic
- Practical Ethics
- Social and Political Philosophy
- Browse content in Religion
- Biblical Studies
- Christianity
- East Asian Religions
- History of Religion
- Judaism and Jewish Studies
- Qumran Studies
- Religion and Education
- Religion and Health
- Religion and Politics
- Religion and Science
- Religion and Law
- Religion and Art, Literature, and Music
- Religious Studies
- Browse content in Society and Culture
- Cookery, Food, and Drink
- Cultural Studies
- Customs and Traditions
- Ethical Issues and Debates
- Hobbies, Games, Arts and Crafts
- Lifestyle, Home, and Garden
- Natural world, Country Life, and Pets
- Popular Beliefs and Controversial Knowledge
- Sports and Outdoor Recreation
- Technology and Society
- Travel and Holiday
- Visual Culture
- Browse content in Law
- Arbitration
- Browse content in Company and Commercial Law
- Commercial Law
- Company Law
- Browse content in Comparative Law
- Systems of Law
- Competition Law
- Browse content in Constitutional and Administrative Law
- Government Powers
- Judicial Review
- Local Government Law
- Military and Defence Law
- Parliamentary and Legislative Practice
- Construction Law
- Contract Law
- Browse content in Criminal Law
- Criminal Procedure
- Criminal Evidence Law
- Sentencing and Punishment
- Employment and Labour Law
- Environment and Energy Law
- Browse content in Financial Law
- Banking Law
- Insolvency Law
- History of Law
- Human Rights and Immigration
- Intellectual Property Law
- Browse content in International Law
- Private International Law and Conflict of Laws
- Public International Law
- IT and Communications Law
- Jurisprudence and Philosophy of Law
- Law and Politics
- Law and Society
- Browse content in Legal System and Practice
- Courts and Procedure
- Legal Skills and Practice
- Primary Sources of Law
- Regulation of Legal Profession
- Medical and Healthcare Law
- Browse content in Policing
- Criminal Investigation and Detection
- Police and Security Services
- Police Procedure and Law
- Police Regional Planning
- Browse content in Property Law
- Personal Property Law
- Study and Revision
- Terrorism and National Security Law
- Browse content in Trusts Law
- Wills and Probate or Succession
- Browse content in Medicine and Health
- Browse content in Allied Health Professions
- Arts Therapies
- Clinical Science
- Dietetics and Nutrition
- Occupational Therapy
- Operating Department Practice
- Physiotherapy
- Radiography
- Speech and Language Therapy
- Browse content in Anaesthetics
- General Anaesthesia
- Neuroanaesthesia
- Browse content in Clinical Medicine
- Acute Medicine
- Cardiovascular Medicine
- Clinical Genetics
- Clinical Pharmacology and Therapeutics
- Dermatology
- Endocrinology and Diabetes
- Gastroenterology
- Genito-urinary Medicine
- Geriatric Medicine
- Infectious Diseases
- Medical Oncology
- Medical Toxicology
- Pain Medicine
- Palliative Medicine
- Rehabilitation Medicine
- Respiratory Medicine and Pulmonology
- Rheumatology
- Sleep Medicine
- Sports and Exercise Medicine
- Clinical Neuroscience
- Community Medical Services
- Critical Care
- Emergency Medicine
- Forensic Medicine
- Haematology
- History of Medicine
- Browse content in Medical Dentistry
- Oral and Maxillofacial Surgery
- Paediatric Dentistry
- Restorative Dentistry and Orthodontics
- Surgical Dentistry
- Medical Ethics
- Browse content in Medical Skills
- Clinical Skills
- Communication Skills
- Nursing Skills
- Surgical Skills
- Medical Statistics and Methodology
- Browse content in Neurology
- Clinical Neurophysiology
- Neuropathology
- Nursing Studies
- Browse content in Obstetrics and Gynaecology
- Gynaecology
- Occupational Medicine
- Ophthalmology
- Otolaryngology (ENT)
- Browse content in Paediatrics
- Neonatology
- Browse content in Pathology
- Chemical Pathology
- Clinical Cytogenetics and Molecular Genetics
- Histopathology
- Medical Microbiology and Virology
- Patient Education and Information
- Browse content in Pharmacology
- Psychopharmacology
- Browse content in Popular Health
- Caring for Others
- Complementary and Alternative Medicine
- Self-help and Personal Development
- Browse content in Preclinical Medicine
- Cell Biology
- Molecular Biology and Genetics
- Reproduction, Growth and Development
- Primary Care
- Professional Development in Medicine
- Browse content in Psychiatry
- Addiction Medicine
- Child and Adolescent Psychiatry
- Forensic Psychiatry
- Learning Disabilities
- Old Age Psychiatry
- Psychotherapy
- Browse content in Public Health and Epidemiology
- Epidemiology
- Public Health
- Browse content in Radiology
- Clinical Radiology
- Interventional Radiology
- Nuclear Medicine
- Radiation Oncology
- Reproductive Medicine
- Browse content in Surgery
- Cardiothoracic Surgery
- Gastro-intestinal and Colorectal Surgery
- General Surgery
- Neurosurgery
- Paediatric Surgery
- Peri-operative Care
- Plastic and Reconstructive Surgery
- Surgical Oncology
- Transplant Surgery
- Trauma and Orthopaedic Surgery
- Vascular Surgery
- Browse content in Science and Mathematics
- Browse content in Biological Sciences
- Aquatic Biology
- Biochemistry
- Bioinformatics and Computational Biology
- Developmental Biology
- Ecology and Conservation
- Evolutionary Biology
- Genetics and Genomics
- Microbiology
- Molecular and Cell Biology
- Natural History
- Plant Sciences and Forestry
- Research Methods in Life Sciences
- Structural Biology
- Systems Biology
- Zoology and Animal Sciences
- Browse content in Chemistry
- Analytical Chemistry
- Computational Chemistry
- Crystallography
- Environmental Chemistry
- Industrial Chemistry
- Inorganic Chemistry
- Materials Chemistry
- Medicinal Chemistry
- Mineralogy and Gems
- Organic Chemistry
- Physical Chemistry
- Polymer Chemistry
- Study and Communication Skills in Chemistry
- Theoretical Chemistry
- Browse content in Computer Science
- Artificial Intelligence
- Computer Architecture and Logic Design
- Game Studies
- Human-Computer Interaction
- Mathematical Theory of Computation
- Programming Languages
- Software Engineering
- Systems Analysis and Design
- Virtual Reality
- Browse content in Computing
- Business Applications
- Computer Security
- Computer Games
- Computer Networking and Communications
- Digital Lifestyle
- Graphical and Digital Media Applications
- Operating Systems
- Browse content in Earth Sciences and Geography
- Atmospheric Sciences
- Environmental Geography
- Geology and the Lithosphere
- Maps and Map-making
- Meteorology and Climatology
- Oceanography and Hydrology
- Palaeontology
- Physical Geography and Topography
- Regional Geography
- Soil Science
- Urban Geography
- Browse content in Engineering and Technology
- Agriculture and Farming
- Biological Engineering
- Civil Engineering, Surveying, and Building
- Electronics and Communications Engineering
- Energy Technology
- Engineering (General)
- Environmental Science, Engineering, and Technology
- History of Engineering and Technology
- Mechanical Engineering and Materials
- Technology of Industrial Chemistry
- Transport Technology and Trades
- Browse content in Environmental Science
- Applied Ecology (Environmental Science)
- Conservation of the Environment (Environmental Science)
- Environmental Sustainability
- Environmentalist Thought and Ideology (Environmental Science)
- Management of Land and Natural Resources (Environmental Science)
- Natural Disasters (Environmental Science)
- Nuclear Issues (Environmental Science)
- Pollution and Threats to the Environment (Environmental Science)
- Social Impact of Environmental Issues (Environmental Science)
- History of Science and Technology
- Browse content in Materials Science
- Ceramics and Glasses
- Composite Materials
- Metals, Alloying, and Corrosion
- Nanotechnology
- Browse content in Mathematics
- Applied Mathematics
- Biomathematics and Statistics
- History of Mathematics
- Mathematical Education
- Mathematical Finance
- Mathematical Analysis
- Numerical and Computational Mathematics
- Probability and Statistics
- Pure Mathematics
- Browse content in Neuroscience
- Cognition and Behavioural Neuroscience
- Development of the Nervous System
- Disorders of the Nervous System
- History of Neuroscience
- Invertebrate Neurobiology
- Molecular and Cellular Systems
- Neuroendocrinology and Autonomic Nervous System
- Neuroscientific Techniques
- Sensory and Motor Systems
- Browse content in Physics
- Astronomy and Astrophysics
- Atomic, Molecular, and Optical Physics
- Biological and Medical Physics
- Classical Mechanics
- Computational Physics
- Condensed Matter Physics
- Electromagnetism, Optics, and Acoustics
- History of Physics
- Mathematical and Statistical Physics
- Measurement Science
- Nuclear Physics
- Particles and Fields
- Plasma Physics
- Quantum Physics
- Relativity and Gravitation
- Semiconductor and Mesoscopic Physics
- Browse content in Psychology
- Affective Sciences
- Clinical Psychology
- Cognitive Neuroscience
- Cognitive Psychology
- Criminal and Forensic Psychology
- Developmental Psychology
- Educational Psychology
- Evolutionary Psychology
- Health Psychology
- History and Systems in Psychology
- Music Psychology
- Neuropsychology
- Organizational Psychology
- Psychological Assessment and Testing
- Psychology of Human-Technology Interaction
- Psychology Professional Development and Training
- Research Methods in Psychology
- Social Psychology
- Browse content in Social Sciences
- Browse content in Anthropology
- Anthropology of Religion
- Human Evolution
- Medical Anthropology
- Physical Anthropology
- Regional Anthropology
- Social and Cultural Anthropology
- Theory and Practice of Anthropology
- Browse content in Business and Management
- Business Strategy
- Business History
- Business Ethics
- Business and Government
- Business and Technology
- Business and the Environment
- Comparative Management
- Corporate Governance
- Corporate Social Responsibility
- Entrepreneurship
- Health Management
- Human Resource Management
- Industrial and Employment Relations
- Industry Studies
- Information and Communication Technologies
- International Business
- Knowledge Management
- Management and Management Techniques
- Operations Management
- Organizational Theory and Behaviour
- Pensions and Pension Management
- Public and Nonprofit Management
- Strategic Management
- Supply Chain Management
- Browse content in Criminology and Criminal Justice
- Criminal Justice
- Criminology
- Forms of Crime
- International and Comparative Criminology
- Youth Violence and Juvenile Justice
- Development Studies
- Browse content in Economics
- Agricultural, Environmental, and Natural Resource Economics
- Asian Economics
- Behavioural Finance
- Behavioural Economics and Neuroeconomics
- Econometrics and Mathematical Economics
- Economic Systems
- Economic Methodology
- Economic History
- Economic Development and Growth
- Financial Markets
- Financial Institutions and Services
- General Economics and Teaching
- Health, Education, and Welfare
- History of Economic Thought
- International Economics
- Labour and Demographic Economics
- Law and Economics
- Macroeconomics and Monetary Economics
- Microeconomics
- Public Economics
- Urban, Rural, and Regional Economics
- Welfare Economics
- Browse content in Education
- Adult Education and Continuous Learning
- Care and Counselling of Students
- Early Childhood and Elementary Education
- Educational Equipment and Technology
- Educational Strategies and Policy
- Higher and Further Education
- Organization and Management of Education
- Philosophy and Theory of Education
- Schools Studies
- Secondary Education
- Teaching of a Specific Subject
- Teaching of Specific Groups and Special Educational Needs
- Teaching Skills and Techniques
- Browse content in Environment
- Applied Ecology (Social Science)
- Climate Change
- Conservation of the Environment (Social Science)
- Environmentalist Thought and Ideology (Social Science)
- Natural Disasters (Environment)
- Social Impact of Environmental Issues (Social Science)
- Browse content in Human Geography
- Cultural Geography
- Economic Geography
- Political Geography
- Browse content in Interdisciplinary Studies
- Communication Studies
- Museums, Libraries, and Information Sciences
- Browse content in Politics
- African Politics
- Asian Politics
- Chinese Politics
- Comparative Politics
- Conflict Politics
- Elections and Electoral Studies
- Environmental Politics
- European Union
- Foreign Policy
- Gender and Politics
- Human Rights and Politics
- Indian Politics
- International Relations
- International Organization (Politics)
- International Political Economy
- Irish Politics
- Latin American Politics
- Middle Eastern Politics
- Political Methodology
- Political Communication
- Political Philosophy
- Political Sociology
- Political Theory
- Political Behaviour
- Political Economy
- Political Institutions
- Politics and Law
- Public Administration
- Public Policy
- Quantitative Political Methodology
- Regional Political Studies
- Russian Politics
- Security Studies
- State and Local Government
- UK Politics
- US Politics
- Browse content in Regional and Area Studies
- African Studies
- Asian Studies
- East Asian Studies
- Japanese Studies
- Latin American Studies
- Middle Eastern Studies
- Native American Studies
- Scottish Studies
- Browse content in Research and Information
- Research Methods
- Browse content in Social Work
- Addictions and Substance Misuse
- Adoption and Fostering
- Care of the Elderly
- Child and Adolescent Social Work
- Couple and Family Social Work
- Developmental and Physical Disabilities Social Work
- Direct Practice and Clinical Social Work
- Emergency Services
- Human Behaviour and the Social Environment
- International and Global Issues in Social Work
- Mental and Behavioural Health
- Social Justice and Human Rights
- Social Policy and Advocacy
- Social Work and Crime and Justice
- Social Work Macro Practice
- Social Work Practice Settings
- Social Work Research and Evidence-based Practice
- Welfare and Benefit Systems
- Browse content in Sociology
- Childhood Studies
- Community Development
- Comparative and Historical Sociology
- Economic Sociology
- Gender and Sexuality
- Gerontology and Ageing
- Health, Illness, and Medicine
- Marriage and the Family
- Migration Studies
- Occupations, Professions, and Work
- Organizations
- Population and Demography
- Race and Ethnicity
- Social Theory
- Social Movements and Social Change
- Social Research and Statistics
- Social Stratification, Inequality, and Mobility
- Sociology of Religion
- Sociology of Education
- Sport and Leisure
- Urban and Rural Studies
- Browse content in Warfare and Defence
- Defence Strategy, Planning, and Research
- Land Forces and Warfare
- Military Administration
- Military Life and Institutions
- Naval Forces and Warfare
- Other Warfare and Defence Issues
- Peace Studies and Conflict Resolution
- Weapons and Equipment

Rethinking Risk Assessment: The MacArthur Study of Mental Disorder and Violence
- Cite Icon Cite
- Permissions Icon Permissions
The presumed link between mental disorder and violence has been the driving force behind mental health law and policy for centuries. Legislatures, courts, and the public have come to expect that mental health professionals will protect them from violent acts by persons with mental disorders. Yet for three decades research has shown that clinicians’ unaided assessments of “dangerousness” are barely better than chance. Rethinking Risk Assessment: The MacArthur Study of Mental Disorder and Violence tells the story of a pioneering investigation that challenges preconceptions about the frequency and nature of violence among persons with mental disorders, and suggests an innovative approach to predicting its occurrence. The authors of this massive project—the largest ever undertaken on the topic—demonstrate how clinicians can use a “decision tree” to identify groups of patients at very low and very high risk for violence. This dramatic new finding, and its implications for the every day clinical practice of risk assessment and risk management, is thoroughly described in this remarkable and long-anticipated volume. Taken to heart, its message will change the way clinicians, judges, and others who must deal with persons who are mentally ill and may be violent will do their work.
Signed in as
Institutional accounts.
- Google Scholar Indexing
- GoogleCrawler [DO NOT DELETE]
Personal account
- Sign in with email/username & password
- Get email alerts
- Save searches
- Purchase content
- Activate your purchase/trial code
Institutional access
- Sign in with a library card Sign in with username/password Recommend to your librarian
- Institutional account management
- Get help with access
Access to content on Oxford Academic is often provided through institutional subscriptions and purchases. If you are a member of an institution with an active account, you may be able to access content in one of the following ways:
IP based access
Typically, access is provided across an institutional network to a range of IP addresses. This authentication occurs automatically, and it is not possible to sign out of an IP authenticated account.
Sign in through your institution
Choose this option to get remote access when outside your institution. Shibboleth/Open Athens technology is used to provide single sign-on between your institution’s website and Oxford Academic.
- Click Sign in through your institution.
- Select your institution from the list provided, which will take you to your institution's website to sign in.
- When on the institution site, please use the credentials provided by your institution. Do not use an Oxford Academic personal account.
- Following successful sign in, you will be returned to Oxford Academic.
If your institution is not listed or you cannot sign in to your institution’s website, please contact your librarian or administrator.
Sign in with a library card
Enter your library card number to sign in. If you cannot sign in, please contact your librarian.
Society Members
Society member access to a journal is achieved in one of the following ways:
Sign in through society site
Many societies offer single sign-on between the society website and Oxford Academic. If you see ‘Sign in through society site’ in the sign in pane within a journal:
- Click Sign in through society site.
- When on the society site, please use the credentials provided by that society. Do not use an Oxford Academic personal account.
If you do not have a society account or have forgotten your username or password, please contact your society.
Sign in using a personal account
Some societies use Oxford Academic personal accounts to provide access to their members. See below.
A personal account can be used to get email alerts, save searches, purchase content, and activate subscriptions.
Some societies use Oxford Academic personal accounts to provide access to their members.
Viewing your signed in accounts
Click the account icon in the top right to:
- View your signed in personal account and access account management features.
- View the institutional accounts that are providing access.
Signed in but can't access content
Oxford Academic is home to a wide variety of products. The institutional subscription may not cover the content that you are trying to access. If you believe you should have access to that content, please contact your librarian.
For librarians and administrators, your personal account also provides access to institutional account management. Here you will find options to view and activate subscriptions, manage institutional settings and access options, access usage statistics, and more.
Our books are available by subscription or purchase to libraries and institutions.
- About Oxford Academic
- Publish journals with us
- University press partners
- What we publish
- New features
- Open access
- Rights and permissions
- Accessibility
- Advertising
- Media enquiries
- Oxford University Press
- Oxford Languages
- University of Oxford
Oxford University Press is a department of the University of Oxford. It furthers the University's objective of excellence in research, scholarship, and education by publishing worldwide
- Copyright © 2024 Oxford University Press
- Cookie settings
- Cookie policy
- Privacy policy
- Legal notice
This Feature Is Available To Subscribers Only
Sign In or Create an Account
This PDF is available to Subscribers Only
For full access to this pdf, sign in to an existing account, or purchase an annual subscription.
The Essex website uses cookies. By continuing to browse the site you are consenting to their use. Please visit our cookie policy to find out which cookies we use and why. View cookie policy.
Research risk assessment
It's the responsibility of the principal investigators (PI) and researchers to identify reasonably foreseeable risks associated with their research and control the risks so far as is reasonably practicable.
All participants and research assistants have the right to expect protection from physical, psychological, social, legal and economic harm at all times during an investigation. Certain research may also present reputational, legal and / or economic risks to the University.
As part of the ethical approval process for research involving human participants you are required to identify potential risks associated with your research and the action you will take to mitigate risk. You may be asked to submit your risk assessment.
The risk assessment process is a careful examination of what could cause harm, who/what could be harmed and how. It will help you to determine what risk control measures are needed and whether you are doing enough.
Risk assessment responsibility
The PI and researchers need to take responsibility for all assessments associated with their projects. Occasionally you may need research workers or students to risk assess an aspect of the work and you will need to check the assessments are adequate and sign them off.
Risk assessors need to be competent and you’ll need to ensure they have adequate training and resource to do the assessments. There is risk assessment training available and help and advice help and advice help and advice from your Health and Safety Advisory Service link advisor and safety specialists (for health and safety risks), or the REO Research Governance team for other risks. In some cases, the hazards are so unique to the research that the PI and their team might be the only people who know the work well enough to make valid judgements about the risk and justify their conclusions.
Risk assessment process
The risk assessment process is a careful examination of what could cause harm, who/what could be harmed and how. It will help you to determine what risk control measures are needed and whether you are doing enough.
To simplify the process you can use the health and safety risk assessment templates, risk estimation tool and guidance for all risks associated with your research project. Please refer to the research risk estimation guidance under how to carry out a risk assessment below to assist you.
Research risks
Typical risks that need to be considered as part of research ethics are:
- Social risks: disclosures that could affect participants standing in the community, in their family, and their job.
- Legal risks: activities that could result in the participant, researchers and / or University committing an offence; activities that might lead to a participant disclosing criminal activity to a researcher which would necessitate reporting to enforcement authorities; activities that could result in a civil claim for compensation.
- Economic harm: financial harm to participant, researcher and / or University through disclosure or other event.
- Reputational risk: damage to public perception of University or the University/researchers’ reputation in the eyes of funders, the research community and / or the general public.
- Safeguarding risks: Risk to young people, vulnerable adults and / or researcher from improper behaviour, abuse or exploitation. Risk to researcher of being in a comprising situation, in which there might be accusations of improper behaviour.
- Health and safety risks: risks of harm to health, physical injury or psychological harm to participants or the researcher. Further information on health and safety risks is given below.
Health and safety risks
The potential hazards and risks in research can be many and varied. You will need to be competent and familiar with the work or know where to obtain expert advice to ensure you have identified reasonably foreseeable risks. Here are some common research hazards and risks:
- Location hazards Location hazards Location hazards and risks are associated with where the research is carried out. For example: fire; visiting or working in participant’s homes; working in remote locations and in high crime areas; overseas travel; hot, cold or extreme weather conditions; working on or by water. Also hazardous work locations, such as construction sites, confined spaces, roofs or laboratories. For overseas travel, you will need to check country / city specific information, travel health requirements and consider emergency arrangements as part of your research planning, by following the University’s overseas travel health and safety standard .
- Activity hazards Activity hazards Activity hazards and risks associated with the tasks carried out. For example: potentially mentally harmful activities; distressing and stressful work and content; driving; tripping, or slipping; falling from height; physically demanding work; lifting, carrying, pushing and pulling loads; night time and weekend working.
- Machinery and equipment Machinery and equipment Machinery and equipment . For example: ergonomic hazards, including computer workstations and equipment; contact with electricity; contact with moving, rotating, ejecting or cutting parts in machinery and instruments; accidental release of energy from machines and instruments.
- Chemicals and other hazardous substances . The use, production, storage, waste, transportation and accidental release of chemicals and hazardous substances; flammable, dangerous and explosive substances; asphyxiating gases; allergens; biological agents, blood and blood products. You’ll need to gather information about the amount, frequency and duration of exposure and carry out a COSHH or DSEAR assessment which will inform whether you may need health surveillance for yourself and / or your research participants.
- Physical agents Physical agents Physical agents . For example: excessive noise exposure, hand-arm vibration and whole body vibration; ionising radiation; lasers; artificial optical radiation and electromagnetic fields. You’ll need to gather information about the amount, frequency and duration of exposure inform whether you may need health surveillance for yourself and / or your research participants.
When to carry out a risk assessment
Carrying out initial risk assessments as part of the planning process will help you identify whether existing resources and facilities are adequate to ensure risk control, or if the project needs to be altered accordingly. It will also help you to identify potential costs that need to be considered as part of the funding bid.
Once the project is approved, research specific risk assessments need to be carried out before work starts.
The research may need ethical approval if there is significant risk to participants, researchers or the University.
How to carry out a risk assessment
The University standard on risk assessments provides guidance, tips on getting it right, as well as resources and the forms to help you produce suitable and sufficient risk assessments and must be used.
- Risk assessment template (.dotx)
- Flow chart to research risk assessment (.pdf)
- Research risk assessment: Risk estimation tool (.pdf)
- Example of a Social Science research risk assessment (.pdf)
Refer to carrying out a risk assessment carrying out a risk assessment carrying out a risk assessment for step by step guidance.
Risk assessments must relate to the actual work and must be monitored by the PI. If there are significant changes to the activities, locations, equipment or substances used, the risk assessment will need to reviewed, updated and the old version archived. Risk assessments should also consider the end of projects, arrangements for waste disposal, equipment, controlled area decommission and emergencies.
Things to consider:
- The risks may be specialist in nature or general. Information can found from legislation, sector guidance, safety data sheets, manufacturers equipment information, research documents, forums and health and safety professionals.
- Practical research might involve less well-known hazards. Do you or your team have the expertise to assess the risk adequately? Do you know who to go to for expert advice?
- The capabilities, training, knowledge, skills and experience of the project team members. Are they competent or are there gaps?
- In fast changing research environments, is there a need to carry out dynamic risk assessments? Are they understood and recorded?
- The right personal protective equipment for the hazards identified and training in how to use it.
- Specific Occupational Health vaccinations, health surveillance and screening requirements identified and undertaken. With physical agents and substances you’ll need to make an informed decision about the amount, frequency and duration of exposure. If you need help with this contact HSAS.
- Associated activities: storage, transport/travel, cleaning, maintenance, foreseeable emergencies (eg spillages), decommissioning and disposal.
- The safe design, testing and maintenance of the facilities and equipment.
- Planned and preventative maintenance of general plant and specialist equipment.
These risk assessments relate to the actual work and must be monitored by the PI. If there are significant changes to the activities, locations, equipment or substances used, the risk assessment will need to reviewed, updated and the old version archived. Risk assessments should also consider the end of projects, arrangements for waste disposal, equipment and controlled area decommission and emergencies.
Training
If you would like training on risk assessment, please book onto one of our courses:
- Research Risk Assessment (for research staff and students in Humanities and Social Sciences)
- Research Risk Assessment (for research staff and students in Faculty of Science of Health only)
- Carrying out a risk assessment Carrying out a risk assessment Carrying out a risk assessment
- People especially at risk People especially at risk People especially at risk
- IOSH/USHA/UCEA guidance on managing health and safety in research (.pdf)
- Research governance: Ethical approval
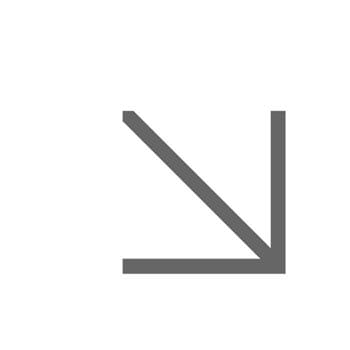
- For enquiries contact your Student Services Hub
- University of Essex
- Wivenhoe Park
- Colchester CO4 3SQ
- Accessibility
- Privacy and Cookie Policy
- IRB-SBS Home
- Contact IRB-SBS
- IRB SBS Staff Directory
- IRB SBS Board Members
- About the IRB-SBS
- CITI Training
- Education Events
- Virginia IRB Consortium
- IRB-SBS Learning Shots
- HRPP Education & Training
- Student Support
- Access iProtocol
- Getting Started
- iProtocol Question Guide
- iProtocol Management
- Protocol Review Process
- Certificate of Confidentiality
- Deception and/or Withholding Information from a Participant
- Ethnographic Research
- IRB-SBS 101
- IRB-SBS Glossary
- Participant Pools
- Paying Participants
- Research in an Educational Setting
- Research in an International Setting and/or Location
- Risk-Sensitive Populations
- Student Researchers and Faculty Sponsors
- Study Funding and the IRB
Understanding Risk in Research
- Vulnerable Participants
- IRB-SBS PAM & Ed
- Federal Regulations
- Ethical Principals
- Partner Offices
- Determining Human Subjects Research
- Determining HSR or SBS

Assessing risk in a research study is one of the primary responsibilities of an IRB and one of its most controversial tasks. By nature, studying human beings is a complicated process because the subject matter itself is complicated. The level of risk can vary because of many factors including: the population included in the study, the situations encountered by the participants, and/ or the experience of the researcher or team. Two studies may appear similar but a few factors could make one inherently more risky than the other.
This section describes what a researcher needs to consider when developing a protocol as well as the risk analysis conducted by an IRB board member. This section does not cover ever scenario nor is it meant to be all inclusive; if you have a specific question about the risks in your study, please contact our office for further guidance.
Section Topics
- Open access
- Published: 27 January 2024
The methodology of quantitative risk assessment studies
- Maxime Rigaud 1 ,
- Jurgen Buekers 2 ,
- Jos Bessems 2 ,
- Xavier Basagaña 3 , 4 , 5 ,
- Sandrine Mathy 6 ,
- Mark Nieuwenhuijsen 3 , 4 , 5 &
- Rémy Slama 1
Environmental Health volume 23 , Article number: 13 ( 2024 ) Cite this article
1507 Accesses
Metrics details
Once an external factor has been deemed likely to influence human health and a dose response function is available, an assessment of its health impact or that of policies aimed at influencing this and possibly other factors in a specific population can be obtained through a quantitative risk assessment, or health impact assessment (HIA) study. The health impact is usually expressed as a number of disease cases or disability-adjusted life-years (DALYs) attributable to or expected from the exposure or policy. We review the methodology of quantitative risk assessment studies based on human data. The main steps of such studies include definition of counterfactual scenarios related to the exposure or policy, exposure(s) assessment, quantification of risks (usually relying on literature-based dose response functions), possibly economic assessment, followed by uncertainty analyses. We discuss issues and make recommendations relative to the accuracy and geographic scale at which factors are assessed, which can strongly influence the study results. If several factors are considered simultaneously, then correlation, mutual influences and possibly synergy between them should be taken into account. Gaps or issues in the methodology of quantitative risk assessment studies include 1) proposing a formal approach to the quantitative handling of the level of evidence regarding each exposure-health pair (essential to consider emerging factors); 2) contrasting risk assessment based on human dose–response functions with that relying on toxicological data; 3) clarification of terminology of health impact assessment and human-based risk assessment studies, which are actually very similar, and 4) other technical issues related to the simultaneous consideration of several factors, in particular when they are causally linked.
Peer Review reports
Introduction
The main aims of environmental health research are i) to identify positive or negative determinants of health-related states (environmental factors in a broad sense encompassing physical, chemical, social, behavioral, systemic factors); ii) to understand the mechanisms underlying the effects of these factors; iii) to quantify the corresponding population impact, which can either be a burden or benefit. This quantification can be done e.g., in terms of number of deaths or disease cases or of healthy years of life lost attributable to the factor or set of factors; and iv) to identify interventions (which can be of all natures and act e.g., on the body, behaviors, knowledge, representations, values, the social, physical and chemical environments, the economy) allowing to limit this impact and preserve or improve the health of populations and limit health inequalities.
In the classical view of the 1983 Redbook of the US National Research Council on risk assessment [ 1 , 2 ], aim i) corresponds to hazard identification and aim iii) to risk assessment. Aim iv) is usually seen as being tackled by health impact assessment (HIA) studies, or analytical HIA studies [ 3 ] but as we will discuss (see "Issues related to terminology" below, last section), from a methodological point of view, the approaches used to tackle aims iii) and iv) are essentially similar. We will therefore use (quantitative) risk assessment to point to studies filling specifically the aim of steps iii) (quantification of population impact of existing factors) and iv) (which corresponds to the quantification of the expected impact of hypothetical policies or interventions). The overall aim of quantitative risk assessment as broadly defined can be described as the quantification of the population impact of any type of factor, exposure, policy or program, hypothesized or already present.
Risk assessment studies typically allow to answer questions such as “How many cases of these diseases are attributable (yesterday/today/tomorrow) to this (exposure) factor or policy?”, or “How many disease cases would be avoided today/in the future if this (exposure) factor was/had been brought to a certain level, or if this policy was/had been implemented, all other things not influenced by this factor or policy being kept identical?”. These questions relate to the consequences of interventions (more precisely about the comparison of counterfactual situations), and not about associations or effects (i.e., hazards, for example: can exposure to this factor cause liver cancer?), as is typical for epidemiological study designs such as cohorts and case–control studies. Such measures of associations, or dose–response functions, are essential but not sufficient to assess the risk. Indeed, dose–response functions alone generally do not allow providing a relevant hierarchy of the disease burden, or impact, associated with each factor: the impact can be higher for an exposure with a mild dose–response function curve than for an exposure with a steep dose–response function, if the first exposure is more frequent than the latter exposure.
For many, if not all risk factors that influence the occurrence of a health-related event, it is not possible to identify if the occurrence of this event in a given subject has been caused by the risk factor; indeed, disease causes generally do not leave a specific (unambiguous) signature in the body, even for strong associations such as the induction of lung cancer by active tobacco smoking. Therefore, one cannot add up cases identified from death certificates or other routine medical data to estimate the disease or mortality burden attributable to an exposure or policy. Similarly, before-after observational studies can be used to document quantitatively health changes in a given population, following e.g., a heat wave, a major air pollution episode [ 4 ], a decrease in air pollution following the temporary closure of an industrial site, an abrupt change in regulation [ 5 ] or an event such as the Olympic Games. However, they are no general solution here; indeed, they may allow to identify a hazard but they are limited to documenting observed (factual) changes and not to studying other (counterfactual) scenarios.
Consequently, one has to rely on more indirect – modelling – approaches. This can be done combining knowledge about the overall frequency of the health parameter considered in the specific population under study, about the distribution of exposure and about dose–response function(s) associated with the factor(s), typically stemming from long-term studies such as cohorts (or animal studies in the case of animal-based risk assessment approaches). Risk assessment studies are related to three research and activity streams: that of the epidemiological concept of population attributable fraction, or etiologic fraction, dating back from the mid-twentieth century [ 6 , 7 , 8 ], that of chemical risk assessment derived from toxicological studies [ 9 ], and the practice of environmental impact assessment in relation to a planned policy or project, in which the consideration of the health impacts possibly induced by the planned policy or project has become more frequent, in addition to the consideration of its environmental impacts [ 3 , 10 ].
These quantitative risk assessment studies contribute to integrating and translating knowledge generated from environmental health research in a form more relevant for policy making. They can be used to define or compare risk management strategies or projects, policies, infrastructures of various kinds with possible health consequences (Fig. 1 ). The risk assessment step can be followed by (or include) an economic assessment step, in which the estimated health impact is translated into an economic cost, providing an economic assessment of the impact of the factor or policy considered.
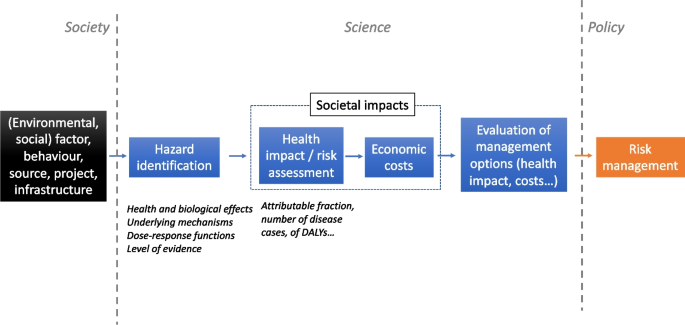
Position of quantitative risk assessment in the process of risk characterization and management. Risk assessment can be used to assess the impacts of the “factors” considered (leftmost box) and of policies aiming at managing and limiting their impacts. Adapted from [ 11 ]
Many risk assessment studies have been conducted in relation to atmospheric pollutants, urban policies, metals, tobacco smoke, alcohol consumption, dietary factors, access to clean water. Although many chemicals are likely to influence health, the consideration of chemical exposures in (human-based) risk assessment studies appears relatively limited [ 12 , 13 ]. The most recent worldwide environmental burden of disease assessment coordinated by the IHME (Institute for Health Metrics and Evaluation, Seattle, USA) considered 87 risk factors and combinations of risk factors, including air pollutants, non-optimal temperatures, lead, unsafe water, sanitation and handwashing, but no other chemical factors except those considered in an occupational setting [ 14 ]. However, the production volume of synthetic chemicals is still increasing and is expected to triple in 2050 compared to 2010 [ 15 ], and widespread human exposure is documented by biomonitoring studies [ 16 , 17 ].
Several reviews about risk assessment and HIA studies have been published [ 10 , 18 , 19 , 20 , 21 , 22 , 23 ]. For example, Harris-Roxas et al. provided a narrative of the historical origins of health impact assessment, strengths and opportunities [ 20 ], Nieuwenhuijsen [ 21 ] reviewed issues related to the participatory nature of HIA studies, while Briggs [ 19 ] provided a conceptual framework for integrated environmental health impact assessment. With the exception of issues related to the challenging concepts of etiologic fraction/excess cases [ 7 , 8 ], very few reviews focused on methodological issues related to the technicalities of the execution of the assessment itself, while, with the development of more refined approaches to assess exposures, the identification of a growing number of hazards through toxicological and biomarker-based epidemiological studies or epidemiological studies based on fine-scale (e.g., air pollution) modeling, there is a need to review options and strategies related to input data and handling of uncertainties at each step of risk assessment studies.
We therefore aimed to perform a literature review (see [ 24 ]) of the methodology of quantitative risk assessment studies, discussing sequentially each step posterior to issue framing [ 19 ]. Qualitative health impact assessment approaches and those based on animal (toxicological) dose–response functions were not considered, with the exception of a few points illustrating in which respect the latter diverge from assessments based on human dose–response functions. We conclude by summarizing the identified methodological gaps, in particular related to the handling of emerging factors with partial data, and issues related to terminology.
Key issues and options at each step
Overall methodology of quantitative risk assessment studies.
The main technical steps of quantitative risk assessment, include:
Definition/identification of the factor(s) (environmental factors/infrastructure/plan/policy) considered;
Definition of the study area and study population;
Description of the counterfactual situations compared and of the study period (may be merged with step 1);
Assessment/description of “exposures” in the study population under each counterfactual scenario;
Identification of the hazards (health outcomes) induced by the factors considered and of the corresponding dose–response functions and level of evidence;
Assessment of “baseline” (usually, current) disease frequency or of the DALYs attributable to the health outcomes considered, if needed;
Quantification of health risk or impact (e.g., in number of disease cases or DALYs);
Quantification of the social and economic impacts;
Uncertainty analysis;
Reporting/communication.
Note that some reviews [ 19 , 25 ] include preparatory or organizational steps, which are not detailed here. Public involvement (not discussed here) can be present at virtually each of these steps. The steps of identification of the exposures, outcomes, area and population considered (numbered 1–3 above) and protocol definition are sometimes referred to as “scoping” step. Note also that the order of some steps is somewhat arbitrary. For example step 2 may come first.
Not all quantitative risk assessment studies follow all of these steps in practice, depending on their scope and the chosen approach. In practice, some studies may stop before completing the risk assessment step – for example many “risk assessment” exercises conducted by national agencies actually only correspond to the identification of hazards associated with the exposure or policy considered without quantification of the corresponding risk, e.g., because robust dose–response functions are lacking (see Sect. " Issues related to terminology " for further discussion). In case a policy is indeed implemented, a “policy implementation phase”, monitoring the implementation of the policy and possibly its actual impact, is sometimes added.
We will review steps 2 to 9, corresponding to the “appraisal steps” in the WHO terminology [ 25 ].
These steps are depicted in Fig. 2 . We first consider the simple case of a single environmental factor for which exposure levels are available or can be assessed (exposure being here understood in its strict meaning of the contact of an environmental factor with the human body). From the knowledge about exposure in the population under study and various other essential pieces of information detailed below, an estimation of the corresponding health impact will be provided, and generally compared to the impact under an alternative (or counterfactual) scenario (e.g., corresponding to the same population in which exposure is set to zero or another reference value, sometimes called TMREL, or Theoretical Minimum Risk Exposure Level, see Selection of the target scenario(s) – exposure levels). This health impact may be positive or negative, restricted to a specific symptom or disease, or consider several diseases or integrated measures of health such as Disability Adjusted Life Years (DALYs), which integrate years lost due to both death and disability.
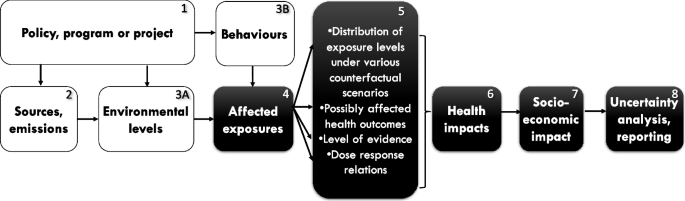
Overview of the main steps of risk assessment studies. The starting point of the study (or the counterfactual scenarios) can be formulated in terms of policy, program, project (1), environmental emissions (2), environmental level (3A), behavior (3B), human exposure (4)
Numerous variations around this basic scheme exist: one can start upstream of human exposure (box 4 in Fig. 2 ), for example from the environmental level of the factor (e.g., the amount of contamination of food by metals or the average atmospheric concentration of particulate matter [ 26 ], box 3A), further upstream, considering a source of potentially harmful or beneficial factors (e.g., a factory that emits several pollutants, the presence of green space [ 27 ]; box 2), or a behavior, possibly influencing exposures, such as burning incense, use of tanning cabins, use of electronic screens or cigarette smoking [ 28 ] (box 3B), or a policy/infrastructure or foreseen societal or environmental change (box 1), such as the ban of coal burning or of inefficient woodstoves in a specific area [ 29 ] or of flavored tobacco [ 30 ], the building of a school, or the temperature changes expected from climate change a few decades ahead [ 31 ], this policy, infrastructure or environmental or societal change being either real or hypothetical. In this latter case, some assessment (e.g., though modelling) of the variations of all the chemical, physical, psychosocial factors that may change as a result of the policy may be required, if it is not already available, e.g. as a result of a pre-existing environmental impact assessment study. One can also start downstream of exposure(s), in particular from a body dose or from the organ dose or the excreted level of an exposure biomarker. Depending on the starting point, specific data and modelling may be required, typically to translate the information about this starting point (say, a behavior or the presence of a factory) into an exposure metric that can be translated into a health risk, which will generally be the exposure metric for which reliable dose–response functions exist. Downstream of the health risk assessment, one may wish to also consider economic and other societal impacts.
Compared situations
Principle of counterfactual comparisons.
To estimate the impact of a given factor or policy, one needs to subtract the number of disease cases expected under the hypothesis that the policy is present or the factor(s) has a given distribution in the study population from the number of cases expected in the situation assuming that the policy is absent or different, or that the factor(s) has a different distribution. Either of these situations may correspond to reality as observed at a given time point. For this estimation to be relevant (i.e., for it to correspond to an estimation of the causal effect of the “change” implemented), the comparison has to be done in the same population and at the same time point. Otherwise, trends in the health outcome unrelated to the considered policy or factor may exist and bias the comparison between both situations. As an illustration, comparing the number of air pollution-related diseases in 10 years, after a road infrastructure has been built, with the situation today, in the absence of the infrastructure, would not distinguish the impact on health of changes in the traffic fleet in time from the impact of the infrastructure per se. In the terminology of causal inference theory, this corresponds to considering counterfactual situations. The reliance on counterfactual thinking is a basis for causal inference: the causal effect of a factor A corresponds to the difference between the situation in the presence of A and a (counterfactual) situation in which everything is similar with the exception that A has been removed [ 32 , 33 ]. Note that the epidemiological concept of population attributable fraction developed before the application of counterfactual thinking to the health field, with the possible consequence that the counterfactual scenarios are not always explicit when estimating population attributable fractions.
The comparison can apply to the past, the current period, or to another period in the future. Therefore, the two main options are:
Counterfactual approach at the current period or in the past: The number of disease cases (or healthy years lost because of specific diseases or another health metric) at the current time t 0 in a hypothetical (“counterfactual”) world similar to the current world but for the fact that the factor is absent, or altered, or the policy has been implemented, is compared to the number of disease cases (or healthy years lost or another health metric) in the real world considered at the same time t 0 ; t 0 needs not to be to the time when the study is performed but can correspond to some time point in the past. We group the situations corresponding to t 0 corresponding to current or past situations because in principle real data on exposures and health can be accessed for the default scenario. See e.g., examples in [ 34 , 35 ].
Counterfactual approach with future scenarios: Here, the number of disease cases (or healthy years lost because of specific diseases or another health metric) at a specific time t 1 in the future in the study population in which the factor has been removed or altered, or the policy has been implemented, is compared to what would be observed at the same time assuming a specific reference scenario [ 29 , 36 , 37 ]. Time t 1 might correspond to the time when the planned policy is expected to have been implemented, or some time later when a new stationary state is assumed to have been reached.
t 0 and t 1 are usually not time points but time periods over which impacts are summed; they may typically correspond to a one-year period, but may also correspond to a long duration, which may correspond to the life expectancy of the planned infrastructure, in which case the risk assessment may be repeated during each year of the study period to allow integration of the impact and possibly infrastructure or policy costs over this period. This is in particular relevant if the costs and benefits vary over time (the costs being possibly borne in the beginning and the positive impacts reaped after a longer duration). Such an integration allows to provide a relevant average of the yearly impacts and costs. An example is the assessment of the health, economic impact and cost of measures to limit air pollution over the 2017–2045 period, assuming that the measures have been implemented at the start of the study period [ 29 ].
The two options above may actually be combined, by providing estimates of the situation at time t 1 in the future under various scenarios, together with an estimate providing to another earlier time t 0 such as the current time. As an illustration, Martinez-Solanas et al. estimated the impact of non-optimal (both cold and warm) temperatures at the end of the twenty-first century under three greenhouse gas emission scenarios, but also at the historical period (1971–2005), allowing both to compare two possible futures with different levels of action against greenhouse gas emissions, as well as to compare the current situations with some possible futures [ 31 ].
Note that the first situation typically corresponds to attributable fraction calculations [ 7 ] done on the basis of the measure of association (e.g., a relative risk) estimated in an epidemiological study, using the exposure in the population from which the relative risk has been estimated. Also note that the observational study equivalent to the second situation corresponds to what is called the difference-in-differences approach [ 38 ]; in this observational approach, in order to estimate the impact of a real intervention, a community or group experiencing an intervention is compared to itself before the intervention, while another group that did not experience the intervention to correct this before-after comparison for temporal trends in the health outcome of interest).
A specificity of the second (“future”) option relates to the temporal evolutions of the study area. These possibly include changes in the demography (age structure and hence also possibly raw disease risk, as the incidence of most diseases varies with age), in specific disease risk factors besides the one in focus and also possibly regarding the dose response function, in particular for health outcomes very sensitive to societal changes, such as mortality. Such evolutions may be difficult to predict, in particular over periods of several decades or more. Illustrations include studies of long-term effects of ozone depletion [ 37 ] or of climate change, in which sociodemographic changes as well as societal adaptation to high temperatures [ 39 ] are expected.
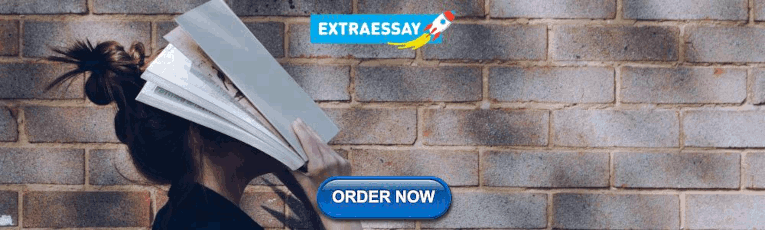
Selection of the target scenario(s) – exposure levels
The scenarios correspond to the counterfactual situations that one wishes to compare to answer the study aim. One scenario will typically correspond to the current or baseline situation (if one is interested in the effect of a factor present today), or to the extension over time of the current situation, the so-called “business as usual” scenario, if the question asked pertains to a policy or infrastructure that would be implemented or built in the future. The alternative scenario(s) will correspond to the hypothetical situation in which the policy considered has been implemented (the policy being e.g., the construction of an infrastructure, a change in urban design, or a lowering of the pollution levels, if the study aims at quantifying the impact of a specific exposure). Of course, several counterfactual scenarios can be considered and compared (see examples in Table 1 or [ 29 ]).
An essential question here, for actions on one or several specific factors, relates to the targeted levels (or distribution) of the factor. In the case of a study aiming to quantify the current impact of a factor that has monotonic effects on all diseases considered, the counterfactual situation in which no one is exposed to the factor can be considered. This would correspond to the ban of the substance or the behavior considered, assuming that the substance is not persistent in the body or the environment, and that the compliance to the regulation is perfect. Other scenarios are however worth considering in specific situations; in particular, one may wish to consider levels strictly higher than zero as an alternative to the current situation if the factor corresponds to a substance persistent in the body or the environment (so that a ban would not lead to the immediate disappearance of the pollutant, as is the case for DDT [dichlorodiphenyltrichloroethane] or PCBs [polychlorinated biphenyls]), if it has both human and natural sources (as is the case for particulate matter, which are also emitted by volcanic eruptions) or if exposure to the factor does not have a monotonic association with disease occurrence (as is the case for outdoor temperature, exposure to sun radiations or level of essential elements in the body). The methodology of the Global burden of disease (GBD) project coordinated by IHME refers to a Theoretical Minimum Risk Exposure Level (TMREL) defined as the exposure level that “minimizes risk at the population level, or […] captures the maximum attributable burden” [ 14 ]. Alternatives exist, such as considering a feasible minimum (which may however require a specific approach in order to be rigorously identified), or specific existing guideline levels (e.g., WHO air quality guidelines [ 41 ]).
In the case of particulate matter (PM), studies in the early 2000s typically used WHO PM guideline value (then 10 µg/m 3 for PM 2.5 ) as the target, which then seemed a remote target – although this value did not correspond to a “no-effect level”, which has not been evidenced for PM 2.5 . Today, as exposures in many Western cities decreased close or below 10 µg/m 3 , lower reference values are often chosen, such as the 2021 WHO guideline value of 5 µg/m 3 , the lowest observed value, or the fifth percentile of the values observed across the cities of the country (which, in the case of PM, will lead to very different reference values in India and Canada, making between-country comparisons difficult), or an estimate of the levels that would be observed in the absence of anthropogenic source of PM. Since the “target” value has of course generally a very large impact on estimates, it is crucial for it to be explicitly quoted when summarizing the study results. Mueller et al. [ 36 ] give the example of a policy that would simultaneously target noise, air pollution, green space and heat exposure, as well as physical activity, taking for each exposure factor levels internationally recommended (in general by WHO) as counterfactual scenario (see Figure S 1 ).
Considering environmental and societal side effects of policies
Ideally, the counterfactual scenarios should consider possible side effects and remote consequences of the considered intervention. For example, a risk assessment of a ban of bisphenol A (totally or for specific uses) could consider several counterfactual scenarios, one in which the consumer products containing bisphenol A are simply banned (possibly trying to consider the societal cost of such a ban if the products product a benefit to society), and others in which bisphenol A is replaced by other compounds, including some that also have possible adverse effects, such as bisphenol S. Studies on the expected impacts of climate change mitigation strategies, such as the limitation of fossil fuels use, may consider health effects due to the expected long-term improvement in climate, but also those related to changes in air pollution levels possibly resulting from the limitation of fossil fuels use, and also the possible consequences of increases in physical activity if this limitation of fossil fuels is expected to be followed by shifts from individual cars to other more active modes of transportation.
Study area and population
The study area should be coherent with the policy or factor considered, trying to include the whole population likely to undergo positive or negative impacts from the factor or policy. Its choice should also take into account the entity (institution, community, administration, population…) able to take a decision regarding this policy. Choosing an area larger than that targeted by a policy makes sense, as it may allow to consider unplanned effects on the surrounding areas (for example, in the case of a policy planning to ban the most polluting vehicles from a city, an increase in the traffic of these vehicles in the surrounding cities), and to provide estimates specific to various sub-areas, which are also relevant because sometimes the exact area concerned with a possible policy is not always decided a priori – in which case the study may help making this decision. However, one should also keep in mind that considering a study area larger than that in which the policy will be implemented may entail possible dilution effects – i.e., the impact may appear lower than it is actually in the population targeted by the policy, if expressed on a multiplicative scale, that is, as a change in the proportion of deaths or DALYs in the area. When considering a policy decided at the city level, estimating the health impact in the city and possibly the surrounding ones is for example relevant; for a European policy, one may consider the whole Europe, or a (possibly random) group of regions if limiting the size of the study population limits bias, costs or uncertainties, which is not always the case for risk assessment studies contrarily to field studies.
In addition to factors related to health (see Assessment of disease frequency) and to exposure to the factor or policy considered (such as possibly fine-scale data on population density), it is usually relevant to collect information on population size, sociodemographic (documenting e.g., age and social-category distribution) and behavioral factors. Although seldom done in practice in the context of risk assessment studies, it is worth considering conducting a specific survey to document specific characteristics of the study population not available in administrative or health databases. For example, if the intervention may as a side effect impact physical activity (which has a non-linear relation to health), it is useful to document the distribution of physical activity in the population.
Exposure (risk factors) assessment
Identification of exposures.
In the simple case of a risk assessment study considering a single pre-identified factor A, before assessing the impact (Health impact), one has to:
assess the expected exposure to factor A in the study population under the considered counterfactual scenarios (see Exposure assessment tools – general considerations to Reliance on exposure biomarkers);
identify all health endpoints and possibly biological parameters H 1 , H 2 … H i that can or could be influenced by A (see Identifying all health-related events possibly influenced by the considered exposures);
assess the level of evidence regarding the possible effect of A on H 1 , of A on H 2 … H i (see Estimating the strength of evidence about the effect of factors on health);
assess the incidence of the health endpoints in the considered population, and the dose response function of all exposure-outcome pairs (A, H 1 ), (A, H 2 )… (A, H i ) (see Exposure-response functions).
As described in Fig. 2 , the level of intervention can correspond to either the emission of factor A (e.g., what a chemical plant is allowed to emit on a yearly basis, box 1), its concentration in a milieu (e.g., air, water, food, box 3A), human exposure to A (referring, strictly speaking, to the contact of humans with the factor, integrating both the duration of the contact and the level of the compound, box 4); A may also correspond to a behavior (e.g., having sexual intercourse without using condoms, box 3B). We will here refer to all of these situations with the same simplifying terminology of exposure in the loose sense, keeping in mind that this differs from its strict definition given above, and that depending on the situation, one may have to do some modelling to translate the intervention into a metric compatible with the exposure response function to be used (see Exposure assessment tools – general considerations below).
If the starting point of the study is now a family of factors (e.g., endocrine disruptors, or environmental factors, as in the case of Environmental burden of disease assessments), then one may first have to list/identify which factors fall under this definition. This step can in practice be challenging and may require a specific technical study.
If the study aims at assessing the impact of a policy, one has to first analyze if and how the policy may translate in terms of environmental (understood in the broad sense) impacts – that is, identify which chemical, physical and social factors A 1 , A 2 … A j may be influenced by the policy, and quantify the expected amplitude of the variations in these factors once the policy has been implemented. For example, if one aims at estimating the impact of banning a fraction of gasoline- and diesel-powered cars or trucks from an area, then one will generally have to rely on atmospheric dispersion models to estimate which changes in specific atmospheric pollutants will be induced by this ban, in addition to consider other consequences of the ban, e.g. related to physical activity. This actually corresponds to a study in itself (an environmental and social impact assessment), which may be already available, possibly because of legal requirements. One would then have to perform the three steps listed above (see end of Study area and population) for each of the factors A 1 , A 2 … A j , influenced by the policy, which may imply to assess and synthesize the evidence regarding a large number of exposure-outcome pairs (A i , H j ).
The consideration of several risk factors has implications in the way the health impact is estimated, which are discussed in Consideration of multiple risks factors below.
Exposure assessment tools – general considerations
Whatever the starting point of the study (i.e., the targeted intervention or factor), the estimation of the health impact should ideally rely on some estimate of the exposure metric coherent with the dose–response function considered (see Exposure-response functions below), which should itself be chosen to minimize the uncertainties and bias in the final risk estimate. If, for example, the evaluated intervention corresponds to the closing of a plant emitting hazardous gases, one could attempt estimating the spatial distribution of the air concentration of the corresponding gases in the area surrounding the plant, averaged over a relevant time period, to convert this spatial distribution into an estimate of population exposure taking into account the spatial distribution of density of the target population (e.g., general population or any specific subgroup) in the study area, and possibly any relevant information on the time–space activity budget of the local populations, and then estimate the population risk from this estimated distribution of population exposure and a dose–response function chosen in coherence with this exposure metric. Similarly, if the intervention aims at changing a behavior such as having unprotected sexual intercourse, one would ideally need to obtain estimates of such behaviors before and after the hypothetical intervention, to provide an estimate of the incidence of a sexually transmitted disease.
All the tools of exposure science and etiological epidemiological studies can in principle be used to assess exposures, from environmental models to questionnaires, dosimeters and biomarkers, keeping in mind that they differ in terms of resolution, accuracy, cost, potential for bias…
As risk assessment studies are expected to provide an estimate relevant in a given population, representativeness of the group in which exposure is assessed with respect to this target population is a desired feature, contrarily to etiological epidemiological studies, for which representativeness is usually not required [ 42 ]. For this reason, “simpler” rather than more accurate and cumbersome approaches to exposure assessment than those used in etiological studies may be preferred, since the later, although more accurate at the individual level, may entail selection bias and thus have in some instances more limited validity at the population level. Consequently, environmental models or data, which may be developed without always requiring contact with the population, are very frequently used in risk assessment studies of environmental factors; we discuss below issues related to the spatial resolution of such models without entering into details of models development and validity not specific to risk assessment studies [ 43 ]; for behaviors, questionnaires may be used, while for many chemical exposures, data from biomarkers or exposure models may be preferred.
In addition to issues related to the validity of the exposure metric itself, errors may arise because of participation (selection) bias induced by the selection of the population for which this metric is available (e.g., answering a questionnaire or providing biospecimens to assess exposure biomarkers). Simply identifying a representative sampling base may be challenging in some areas if no relevant public database exists or if access cannot be granted; even if such a relevant sampling base exists, a participation rate of 100% cannot be expected, and refusing to participate can a priori be expected to be associated with sociodemographic characteristics and possibly with the exposure of interest. Tools such as reweighing approaches may be used to limit the impact of such selection bias on the estimated exposure distribution.
Again, whatever the approach used, the estimate of exposure provided should be coherent with the hazard identified and the dose response function to be used in the following steps (i.e., a dose–response function based on yearly exposure averages should not be combined with a weekly estimate of exposure).
Issues related to the assessment of environmental factors through questionnaires
Questionnaires may be used to assess behaviors, such as the frequency of use of specific modes of transportation (which would be relevant if one aims to quantify the impact of a policy entailing a shift in the share of specific modes of transportation), diet, smoking or physical activity and also psychosocial factors. Just like for environmental models, validation studies are particularly relevant to discuss and possibly quantify (see below, sensitivity analyses, Sensitivity and uncertainty analyses) any bias possibly induced by the questionnaire used, keeping in mind that the validity of questionnaires may be population-specific, as the validity of replies will depend on the social desirability of the considered behavior (for example, replies to questionnaires on alcohol consumption may be dependent on the social perception of alcohol consumption in the given cultural background), as well as evolve over time in a given society, limiting the validity of temporal comparisons.
When it comes to assessing exposure to specific chemical or physical factors, questionnaires may be very limited. Indeed, one is generally not aware of one’s own exposure to a chemical, in particular if exposure occurs through several routes (e.g., in the case of bisphenol A, PCBs, or specific pesticides), and cannot provide any quantitative estimate of her/his own exposure; additionally, one’s perception of exposures may be strongly influenced by social or psychological factors (e.g., one’s perception of the noxiousness of the factor, or the existence of a disease that one considers to be imputable to the considered exposure), which may bias the estimated impact (assuming that perception is not of interest by itself). As an illustration, a European study showed limited agreement of one’s self-declared exposure to traffic with an objective assessment of exposure, to extents that varied between countries [ 44 ]. The fact that questionnaires alone are typically limited to assess exposures to chemical and physical factors does not imply that they cannot be used in combination with other sources of information to provide a relevant exposure estimate (see Reliance on environmental models and surveys below). Moreover, questionnaires (including those relying on smartphone-based applications) are essential to assess behavioral factors such as dietary patterns, alcohol or tobacco consumption, physical exercise, transportation patterns, sexual activity… These often rely on data collected independently from the health impact assessment study, but there is no reason for investigators planning such a study not to envision an ad-hoc questionnaire survey.
Reliance on environmental models and surveys
In some areas, public authorities or other institutions developed “databases” regarding specific factors that may be a relevant source of information about the exposure of interest. These databases may be built from various sources and approaches. These include data on sources of specific hazards (e.g., maps on the location of pollution sources or on the chemical composition of cosmetics or other consumers’ products), environmental monitoring (e.g., databases on water contamination (typically based on measurements in the water distribution network, job-exposure matrices in an occupational setting or models), corresponding to boxes 2, 3A and 3B of Fig. 2 (the case of human biomonitoring is discussed specifically below). When available, databases on sources and environmental models and measurements have the advantage of being possibly representative of a specific milieu, in particular if they are based on environmental measurements or models, which can more easily be developed on a representative basis than measurements implying the participation of human subjects; they also have the advantage to rely on information often not known to the inhabitants of the study area (e.g., the level of contamination of the air or drinking water or food by specific compounds) and to possibly cover large spatial areas and extended temporal windows. They may provide source-specific information, which may be relevant if the health impact assessment study considers a possible intervention limited to a specific source of exposure; for example, atmospheric pollution dispersion models typically combine emissions from urban heating, traffic, industry… and may be used to predict environmental levels that would be observed assuming that emissions from one or several specific sources are decreased [ 29 ]. They possibly allow avoiding directly contacting individuals to collect information on their exposure, although in many situations it is actually relevant to combine such environmental models with questionnaire data: for example, questionnaires are essential to combine models or data on food contamination or dietary characteristics with individual information on food consumption patterns [ 45 ], models or measurements in drinking water benefit from information on water use (sources and amount of water drunk, frequency and temperature of baths and showers… [ 46 ], and atmospheric pollution models may be relevantly combined with individual information on time space activity, to integrate individual exposures across the places where each individual spends time [ 47 , 48 ]. Indeed, environmental models typically provide an estimate of an environmental level and not an estimate of personal exposure in the strict meaning. Other issues related to their use is that such models do not exist for all environmental factors and areas and that their spatial resolution may be limited.
Environmental models—Issues related to spatial scale
Indeed, the spatial resolutions of such models vary between models. In the case of atmospheric pollutants, early risk assessment studies typically relied on data from background monitoring stations, which cover a generally small fraction of territories and are sometimes distant by several kilometers one from another. In addition, background monitoring stations are by definition located in “background” sites, which are located far (typically, a couple hundred meters or more) from air pollution “hot spots” (see Fig. 3 ). On the one hand, relying on data from background monitoring stations may underestimate health impacts, as these background stations are not meant to represent people living or spending time close to air pollution hot spots such as industrial sources or high-traffic roads. On the other hand, stations located close to specific sources to monitor their activity are not meant to provide an estimate valid for a large area.
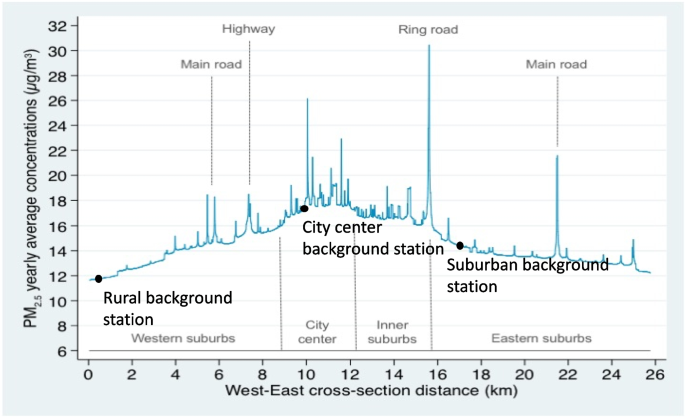
Cross-sectional variations of fine particulate matter (PM 2.5 ) throughout the urban area of Lyon, as estimated from a fine-scale dispersion model, and typical locations of background permanent monitoring stations (black circles). Adapted from [ 40 ]
In the last decades, models providing a finer-scale resolution, such as geostatistical models based on measurement campaigns in a large number of points, land-use regression or dispersion models were developed [ 49 ]. These have a much finer spatial resolution (Fig. 4 ). In a study in two French urban areas, assessing exposures from background monitoring stations entailed an underestimation of the mortality attributable to fine particulate matter by 10 to 20%, compared to fine-scale models with spatial resolutions taking into account variations in the pollutants’ concentration with a spatial scale of about 10 m [ 34 ]. Identifying the most relevant approach for risk assessment purposes is not straightforward. Even some fine-scale approaches may entail some error, as these may represent a “smoothed” version of the actual spatial contrasts in air pollution, and as smoothing typically entails a poor representation of the extreme values. These models with a very fine spatial resolution may be limited in terms of temporal resolution, which may be an issue for some health outcomes. Moreover and maybe counter-intuitively, relying on spatially very fine models may not be desirable in risk assessment studies in which there is no available data on the time–space activity of individuals. Indeed, if, in the absence of such individual time–space activity data, one has to assume that individuals are exposed at the concentration assessed at the location of their home address, then models that tend to smooth concentration over rather large areas may be more accurate for the purpose of risk assessment than models very fine spatially used ignoring the other places where individuals spend time.
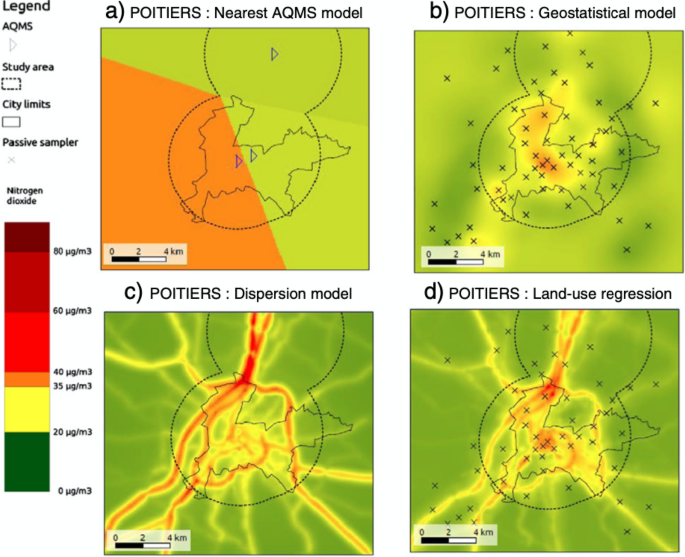
Spatial resolutions of various air pollution (nitrogen dioxide) exposure models developed in a middle size city. a Estimates based on permanent background monitoring stations; b geostatistical model relying on a fine-scale measurement campaign; c dispersion model taking into account emission and meteorological conditions; d Land-use regression model relying on the same measurement points as geostatistical model ( b ) [ 50 ]
Integrating environmental models with data on population density
As already mentioned, environmental models do not provide an estimate of the population exposure in the strict sense, if only because population density varies with space so that simply averaging the environmental levels over the study area, which gives the same weight to each location (and is equivalent to assume that the population is homogeneously distributed spatially across the study area) may poorly approximate population exposure. Getting closer to population exposure may imply to combine the estimated environmental level with data on population density (i.e., weighting concentrations with population density), which will allow considering the fact that the population is not evenly distributed in a given area. Kulhánová et al. [ 51 ] have illustrated these issues in a study of the lung cancer risk attributable to fine particulate matter exposure in France. Compared to a model that took into account PM 2.5 exposure at a 2 km resolution and population density, a model ignoring the spatial distribution of homes within each département (geographical units of 200,000 to one million inhabitants) underestimated the population attributable fraction by about one third; when the variations in population sizes between départements was ignored, so that one assumed that everyone in the country was exposed to the median level observed at the country level, the estimated population attributable fraction was divided by 3.6, compared to the original one taking into account population density and fine-scale air pollution data (see Table 2 ). A large part of this bias was due to ignoring population density.
Note that at this step, the environmental levels and population density data can be combined with other spatially referenced data, such as information on sociodemographic characteristics, as a way to provide an estimate of how exposure distribution varies across these sociodemographic characteristics.
Reliance on personal dosimeters
Exposure assessment may also rely on personal sensors and dosimeters [ 54 ]. Generally, these have the advantages to provide an estimate of exposure that does not rely on detailed data on the sources of the factor, which are not always available (for many chemicals whose sources are not always monitored, such as benzene and other volatile compounds or pesticides), not on a modeling of the dispersion of the factor from its sources to the human environment, contrarily to the approaches discussed above in 2.4.4 to 2.4.6. Since dosimeters are carried by individuals, they efficiently allow taking into account the variability in exposure due to people moving between different environment [ 47 ]. They also allow to capture indoor levels of the factor of interest, which is of importance (for factors whose levels indoors and outdoors differ, such as ozone, benzene, radiations, temperature, noise…) given that people spend the vast majority of time indoors, at least in Northern countries. This increased “spatial” resolution compared to the above-mentioned environmental models (which typically capture outdoor levels, and generally at one location only if the time space activity of the population is not assessed) generally comes at the cost of possible limitations in terms of temporal resolution. In particular, it may be cumbersome to assess long-term exposure (which may be toxicologically relevant for specific outcomes) using personal dosimeters, which are typically carried over short-term (typically, from a day to a week) periods; these measurement periods may be repeated over time to improve the accuracy of the assessment as a proxy of long-term exposure [ 55 ], as discussed below for exposure biomarkers. Dosimeters are particularly relevant for media-specific exposures or factors, such as atmospheric pollutants including particulate matter [ 56 , 57 ] or nitrogen oxides [ 47 , 58 ], benzene [ 59 , 60 ] and other volatile organic compounds [ 61 ], non-ionizing radiation such as ultra-violet [ 62 ] or ionizing radiation [ 63 ], temperature, noise [ 64 ]… Contrarily to environmental models, their use in the context of a health impact assessment study implies to recruit a population sample as representative of the target population as possible. Their use in risk assessment studies appears quite limited so far outside the occupational setting [ 61 ].
Reliance on exposure biomarkers
In the case of chemicals with multiple routes of exposure such as specific pesticides, which may be present in food, water and air, exposure biomarkers (the assessment of the compound or of its metabolite(s) in a tissue or fluid of the organism) may be a relevant approach. With the development of biomonitoring studies [ 65 , 66 , 67 ] and of cohorts collecting biospecimens [ 68 ], exposure biomarkers may be expected to be increasingly used in quantitative risk assessment studies related to chemicals.
Biomarkers study typically provide an estimate of the circulating or excreted level of the compound, which is not exposure in the strict sense but is related to it, while also depending on toxicokinetic factors, that typically vary between subjects [ 69 ]. Biomarkers integrate multiple routes of exposure, in that the level of a compound or its metabolites in a given body compartment will generally depend on the doses entering the body by ingestion, inhalation, dermal contact… (with many specificities according to the compound and tissue in which the metabolites are assessed). This may or may not be seen as an advantage, depending on the study aim, which may relate to exposure as a whole or to route-specific exposure (e.g., that due to food contamination). Considering these different routes is in principle possible via environmental models and measurements, but may be very cumbersome in terms of data collection and modeling work (consider for example a study on the impact of pesticides, which would have to estimate pesticide levels in water, possibly in the air, in food and to assess eating behaviors to try to reconstruct an individual’s exposure). A limitation of exposure biomarkers is related to the short half-life of many chemicals in the body, which implies that a spot biospecimen will generally be a poor proxy of long-term internal dose [ 70 ]. This is an issue for etiological studies, in which dose response functions assessed using a single biomarker in each subject are expected to suffer from bias towards the null [ 70 ]. This issue may also impact risk assessment studies. Indeed, even in the context of classical-type error, which may be hypothesized for biomarkers, although the population average exposure may not be biased when a spot measurement is done in each subject, the estimation of other features of the exposure distribution, such as variance and hence the estimation of specific exposure percentiles, is expected to be biased. Like questionnaire-based approaches, and generally to a larger extent, biomarker-based approach are dependent on individual’s participation and are therefore prone to selection biases; these may, again, be partly corrected if information on the factors associated with participation is available. Just like for all other approaches to assess exposures, behaviors and other health drivers, although rarely done, there is no reason beyond logistics and funding not to consider an ad-hoc biomonitoring survey as part of the HIA, on the contrary. We are not aware of specific quantitative evaluation of the bias associated with all the possible exposure assessment tools to a priori justify the choice of the exposure metric used in a given risk assessment study.
Exposure–response functions
Identifying all health-related events possibly influenced by the considered exposures.
For each factor (in the broad sense of chemical, physical, psychosocial, behavioral factor) primarily considered or identified at the previous stages as possibly influenced by the considered intervention, all health-related events that this factor might influence (the “health effects”, or hazards, a qualitative notion not to be mistaken with the quantitative health impacts) need to be identified. This identification should cover proximal and more remote effects, and positive (beneficial) as well as negative (detrimental) effects. For several environmental factors, the list of possible health effects may be long; for example, lead is a cause of neurological, nephrotoxic, cardiac, reproductive… effects, while particulate matter can affect cardiac, respiratory, metabolic and possibly reproductive and neurodevelopmental function [ 71 ]. Complex policies may entail numerous health consequences; for example, acting on traffic will affect air pollutants, but also noise, traffic accidents, greenhouse gas emissions, that may have long-term health effects (even if the corresponding impact may be limited, depending on the considered spatial scale). Even if the study does not provide a quantitative assessment of all the effects of a given exposure, identifying all of these effects is important. This identification of possible health effects should in principle rely on a systematic review that should encompass the human literature but also toxicology and possibly in vitro or in silico studies that may inform on mechanisms and point to specific health effects. Such an identification of all likely effects of the factor, change or intervention considered may rely on a recent well-conducted published review.
Estimating the strength of evidence about the effect of factors on health
The identification of each health effect possibly influenced by each considered factor should come with some assessment of the corresponding level of evidence. The assessment of the level of evidence evolved in the last decades from experts’ opinion to more formalized systematic reviews, possibly followed by meta-analyses and evidence integration approaches combining streams of evidence from various disciplines such as in silico data, in vivo and in vitro toxicology, environmental sciences, epidemiology… (see e.g., [ 72 , 73 ] or the chapter 6 of [ 74 ] for a presentation of these approaches). Given the sometimes very large effort required by the implementation of such approaches, in particular for factors about which a vast literature exists, it is relevant to rely on existing assessments of the level of evidence, whenever a recent one with a transparent and relevant methodology is available. If not, the time and effort required for this step should not be underestimated, so that a review of the literature from all relevant disciplines, experts from these disciplines can be gathered to synthesize and weight the evidence and provide an assessment on a pre-specified grading scale (e.g., in terms of probability of causation). In case several factors are considered, then the number of exposure outcome pairs considered can be very large. An example of the assessment of the strength of evidence about endocrine disruptors is provided in Trasande et al. [ 75 ] and in Table 3 .
Handling of the strength of evidence about the effect of environmental factors on health
In the past, a common practice was to only consider exposure-outcome pairs (A i , H j ) for which the strength of the evidence regarding the effect of A i on H j was very strong or deemed causal. Another common option is to focus on a specific a priori chosen health outcome induced by the exposure, acknowledging that other effects are ignored; for example, many studies quantified the impact of tobacco smoke on lung cancer only, while other effects, e.g., on cardiovascular diseases, are certain.
The obvious consequence of these practices is to bias the estimated impact of the exposure or policy, generally in the direction of an underestimation (assuming that all associations go in the same direction, e.g., a negative effect of exposures on health). This is obvious for the second option above, but is also true for the first one. This is because in some cases, the discarded exposure-outcome associations will eventually turn out to correspond to very likely effects, as research continues, while the symmetrical situation of an effect deemed very likely or certain becoming unlikely as research unfolds is arguably much rarer in practice [ 76 ].
Possible alternatives to only considering very likely exposure–response pairs include:
Considering all exposure-outcome pairs for which the estimated level of evidence is above a certain level (e.g., “likely association” or above, keeping in mind that diverse approaches are used to obtain these causality gradings and that various scales of evidence are used by various institutions), and estimating the corresponding impacts of these likely effects just like for the exposure-outcome pairs with a very high level of evidence. A special case consists in considering all exposure-outcome pairs for which there is at least “some evidence” of an association; in this case, there is potential for an overestimation of the overall impact (again, assuming that all associations go in the same direction);
Performing sensitivity (additional) analyses considering exposure-outcome pairs with decreasing levels of evidence, and report the estimated impact of the exposure or policy considering only very likely effects, as well as the impact estimated considering also likely effects and suspected effects;
Considering all exposure-outcome pairs for which the estimated level of evidence is above a certain level in the risk assessment and summing their impacts, weighing the sum by a weight increasing with the level of evidence of the corresponding effect, so that in the overall impact more weight is given to impacts from exposure-outcome pairs with a high level of evidence and less to the less likely ones.
Many studies more or less explicitly correspond to approach a). For example, the GBD methodology currently focuses on exposure-outcome pairs for which there is “convincing or probable evidence” [ 14 ]. An example of approach c), which is an intermediate one between the two first alternatives above, is a study of the cost of exposure to endocrine disruptors in the European Union [ 12 , 75 ]. In this study, the health impact (and corresponding cost) attributable to exposure to each considered endocrine disruptors has been assessed using a Monte-Carlo approach in which, at each simulation run, the impact of a given exposure-outcome pair was possibly set to zero, according to the estimated probability of causation (with more likely effects being less often set to zero), and the overall impact was estimated averaging over many simulation runs and summed across exposure-outcome pairs. For example, in the case of a dose–response function of an exposure-outcome pair for which the strength of evidence had been rated to be about 50%, then the corresponding attributable cases were taken into consideration in only half of the simulation runs [ 75 ]. This approach seems relevant if a large number of factors is considered and if one assumes that the literature is not systematically biased. Indeed, if twenty factors are considered and the evidence is properly evaluated, then, assuming that the weight of evidence is estimated to be 50% for all factors, one may expect that eventually 10 of these factors turn out to really have an impact, so that counting half of the effect of the twenty factors may fall closer to the true impact (corresponding to that of ten factors) than if all twenty factors are ignored because the strength of evidence is not high enough. Note that the assumption regarding the fact that the literature is not biased can be debated for environmental factors, as for many factors, the weight of evidence typically tends to increase over time, rather than vary up or down randomly, and as a literature review of environmental alerts concluded that “false alarms” tended to be very rare in environmental health [ 76 ]. This would rather support not restricting the risk assessment to “certain” exposure-outcome associations, and also consider those with a lower level of evidence, possibly taking the level of evidence in the estimation as described above in c). Considering associations with less than certain level of evidence also allows to quantify the possible impact of suspected hazards, which is relevant to prioritize environmental factors for which research efforts should be dedicated [ 77 ]. In practice, the ability to implement the approaches depends on the availability of relevant data on exposures (which can be collected in the context of the risk assessment if not already available), exposure response functions (which may be very long and cumbersome to obtain if they are not already available) and baseline population health; this means that whatever the option chosen to handle the weight of evidence regarding each exposure-health outcome pair, the list of effectively considered pairs may be further restricted because of these issues related to data availability; this is expected to bias the health impact estimate (see Assessing the impact of policies versus the impact of exposures for further discussion). Transparency on all possibly affected outcomes is anyway warranted, even if not all of them can eventually be incorporated in the estimated overall impact.
Exposure to chemical, physical and behavioral factors is now generally assessed on quantitative scales, and the expected impact of policies or plans on environmental and societal factor can often be translated in terms of quantitative variations in drivers of health. The ERF provides an estimate of the effect (positive or detrimental) associated with the exposure at each level (the terms of dose, concentration or exposure response function (DRF, CRF, ERF) or curve are here used synonymously). Issues related to the ERF relate to the validity of its assessment, including the slope and shape and to its applicability to the study population.
In the context of a risk assessment study, contrarily to the estimation of exposure which may in some cases be done ad hoc, it is generally not realistic to expect to generate from scratch a new ERF (whose estimation may require the follow-up of large populations over periods of years or decades) so that one has to rely on external ERFs. If no ERF is available, then one may either 1) try to derive an ERF from existing toxicological (animal) studies [ 78 ], if any or 2) perform a qualitative HIA. If several ERFs are available, then performing a meta-analysis of these ERFs to obtain a more robust one should be considered. This step may be done on the basis of the systematic review possibly performed to assess the level of evidence (see Estimating the strength of evidence about the effect of factors on health above).
We will here assume that some ERFs (or relative risks or any equivalent measure of association) are available. The choice of the exposure response function may have large influences, as illustrated by a review of air pollution HIAs illustrating that the estimated health impact of fine particulate matter in Europe varies by a ratio of two when switching between exposure–response functions based on two large studies [ 79 ] (see also Table 2 ). Three dimensions to consider are those of the sample size from which the ERF has been estimated, the potential for bias of the ERF, e.g., in relation to the adjustment factors considered, and that of its applicability to the study population. Researchers are typically left with the choice between an ERF estimated on a local (or nearby) population, which possibly relies on a small population (hence a large variance), or more global estimates, such as those from meta-analyses, which may be more robust (based on larger populations) but ignore possible differences in sensitivity between the local population and the rest of the world (and therefore are possibly biased). This can be seen as an illustration of the classical bias-variance trade-off. In an ideal situation in which many studies providing an ERF are available, one would characterize the potential for bias of each individual study (evidence evaluation, see e.g., chapter 5 in [ 74 ]) and then perform meta-analyses, in which the poor-quality studies would be discarded if they tend to produce different ERF estimates than the studies with better quality. The potential for heterogeneity in the ERF across populations and geographical areas should also be characterized (see Fig. 5 ), allowing to decide whether it appears more relevant to derive the ERF from a small group of studies in settings very similar to the setting of the HIA, or from a larger group of studies covering a wider diversity of settings.
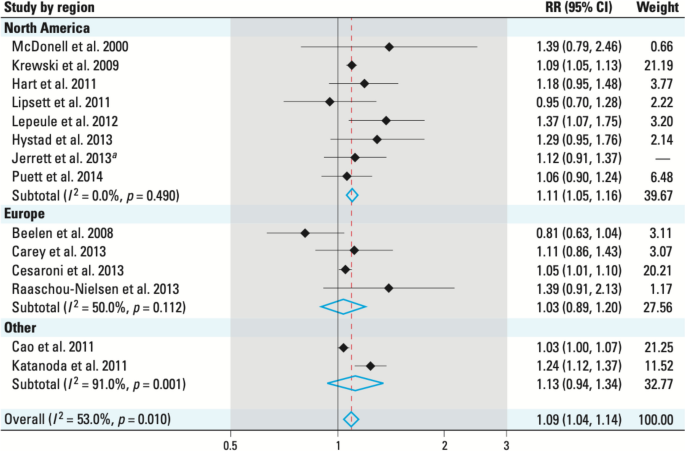
Meta-analysis of the relative-risk (RR) of lung cancer associated with PM 2.5 exposure, by region [ 53 ]
It may be relevant to also consider other factors, such as the range of exposures in the studies from which the ERF is based, trying to base the ERF on studies with an exposure range similar to that of the population in which the risk assessment is conducted, and avoiding to extrapolate ERFs outside the exposure levels where the bulk of the original data lie. In the case of risk assessment studies focusing on factors of other nature, such as social or behavioral factors, for which the hypothesis of heterogeneity in sensitivity across large areas is more likely, the meta-analysis may not be the preferred option.
The concept underlying the exposure assessment in the study from which the exposure response function is based should be similar to that used in the risk assessment study. For example, if “exposure” in the study from which the exposure–response curve originates corresponds to lead environmental level, then it is generally not advised to rely on lead biomarkers (which assess the levels circulating in the body and not environmental levels) in the risk assessment study; the length of the exposure window should also be similar, as this length generally influences the estimated exposure variability. If both entities differ, then in some cases it may be possible to convert one into the other, using a formula derived from a study in which both entities have been assessed in the same population, or using toxicokinetic modelling. Contrarily to what is sometimes said, this requirement of a similarity of exposure concepts does not imply that the specific approaches used to assess exposures need to be identical. The measurement approaches can differ, provided one is not biased with respect to the other. For example, if the exposure considered is fine particulate matter and the exposure–response function stems from a cohort study in which exposure was assessed relying on permanent monitoring station, then a dispersion model could in principle be used to assess fine particulate matter levels in the risk assessment study. In this example, both the permanent monitoring stations and the dispersion models provide an estimate of the same entity (the environmental level), and since etiologic studies relying on permanent monitoring stations are not expected to be strongly biased compared to studies using environmental models with a finer scale such as a dispersion model (assuming that the concept of Berkson error [ 80 ] applies), the exposure–response function stemming from the study using monitoring stations is in expectation similar to the one that would have been obtained if a dispersion model had been used instead to assess exposure.
Non-linear exposure–response functions
The studied factor may have non-linear associations with the health outcome considered on a given (e.g., additive or multiplicative) scale. Note that such deviations from linearity are not always investigated in etiological studies (possibly for reasons related to limited statistical power). As an illustration, a 2001 review on the ERF of physical activity effects on mortality indicates that only 17 of the 44 studies conducted a test of linear trend [ 81 ]. A more recent and robust review does a meta-analysis on studies with larger population samples and finds a better fit for the curve y =—x 0.25 , with steeper effect of moderate, as opposed to higher, physical activity on mortality [ 82 ].
Non-linear dose–response functions are all the more likely when the underlying mechanisms of action are complex and as the range of exposure values increases. Ignoring this non-linear relation can significantly impact the estimated risk [ 83 ], hence potentially misestimating the risks or benefits of the change, depending on the distribution of the exposure in the population.
Non-linear ERFs have been exhibited for several risk factors. This is the case for temperature and air pollution effects on mortality in particular, and also effects of physical activity on health (Fig. 6 ); they may also be expected e.g., for endocrine disruptors [ 84 ].
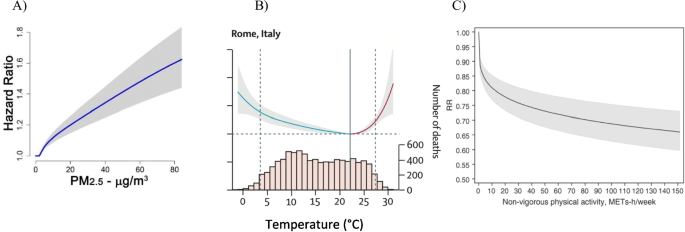
Illustration of non-linear exposure response functions: A ) Fine particulate matter and mortality [ 85 ]; B ) Temperature and mortality in Rome [ 86 ], C ) Physical activity and cardiovascular events [ 87 ]. MET: Metabolic equivalents: RR: Relative risk
Note that many risk assessment studies, at least from a certain period in the past, used to assume the existence of thresholds (hence, a non-linear dose–response) in the non-carcinogenic health effects of chemicals, and a lack of threshold of carcinogenic effects. There is to our knowledge no toxicological justification to such general claims. The “threshold” model can be seen as being related to the misconception that the NOAEL (no observed adverse effect level) estimated in some regulatory toxicology studies corresponds to a true “no effect” exposure level. In fact, a NOAEL generally corresponds to a level with an effect, whose size depends in particular on the number of animals used in the experiment aiming to estimate the NOAEL [ 88 ].
Assessment of disease frequency
The ideal situation corresponds to that of an area where a register (or any other system allowing an exhaustive assessment of new disease cases on a representative basis) exists. Such registers exist in many countries for cancers but, outside Scandinavian countries, are rarer for other diseases. Just like for the case of the assessment of exposures, tools typically used in etiologic cohort studies may provide a relevant estimate of the disease frequency, with the same caveat as above, namely that etiologic studies are rarely representative of a given area, which would be a limitation if the disease frequency obtained in such a study is to be used in a risk assessment exercise. Alternatively, one can rely directly on estimates of the disease burden, such as those provided by the Global burden of disease project ( https://www.healthdata.org/results/gbd_summaries/2019 ).
The disease frequency can correspond to different entities, generally related to incidence (the number of cases appearing during a given time period in a population) or prevalence (the number of cases present at a given time point, whatever the time when the disease started). In principle, incidence should be targeted. The entity used to assess disease frequency should be coherent with the measure of association (the exposure–response function) chosen. For example, a hazard rate stemming from a cohort study (or an incident case control study) assesses the change in the disease hazard (the strength of apparition of new cases) and needs to be combined with a measure of incidence and not prevalence.
- Health impact
Concepts of impact
The health impact (or risk) is the core estimate of a risk assessment study. It is a challenging notion, both from a conceptual and estimation perspective, not to mention issues related to the use of this expression with possibly different meanings across scientific and public health communities. When it comes to the human-derived risk assessment studies discussed here, the core product corresponds to notions close to the epidemiologic notion of attributable fraction. Following Greenland [ 8 ], we shall remind that this expression covers different concepts: the etiologic fraction, the excess fraction, the incidence density fraction, to which one shall add the expected (healthy) years of life lost.
The excess fraction corresponds to the proportion of cases that would not have become a case during the study period in the absence of exposure, while the etiologic fraction includes these excess cases as well as the cases due to exposure that would also have occurred during the study period in the absence of exposure, but at a later time point during this period. These two fractions can strongly differ because the etiologic fraction includes, in addition to the “excess cases”, the cases which would have happened also in the absence of exposure, but for which exposure made the incidence happen earlier than if the subject had not been exposed. These cases for which exposure simply made the case happen earlier in the study period may correspond to a large fraction of cases for complex diseases (as opposed to diseases with a simpler etiology such as infectious diseases), and their number increases with the duration of the study period. This is illustrated with the extreme example of a study of a factor increasing mortality or any other inevitable outcome: if the study period is very long, so that all members of the considered population are dead at the end of this period, then the excess fraction will become zero (because everyone eventually dies, even in the absence of the considered exposure)(see for example Fig. 2.6c in [ 89 ]), while the etiologic fraction may be non-null if the exposure does influence mortality [ 8 ]. For this reason, some advise not to use the excess fraction as a metrics, or the similar yearly number of avoided cases in a population [ 89 ]. Although this metric is indeed limited when it comes to estimating a meaningful health impact (that may be used to quantify an economic impact), when comparing the impact of various factors, it is possible that in many common situations the ranking of risk factors is preserved across metrics. In any case, it is of course crucial to only compare exposures in terms of impact assessed using exactly the same metric. Although in principle more relevant, the estimation of the etiologic fraction requires specific biological knowledge or hypotheses [ 8 ].
The incidence density fraction, defined as (ID E+ -ID E- )/ID E+ , where ID E+ (respectively, ID E- ) is the incidence in the exposed (respectively, unexposed) group, has different interpretations, depending on whether one relies on instantaneous or average incidence densities [ 8 ]. Estimating attributable life-years (or healthy life-years) associated with the exposure or policy may appear as a relevant option for many public health purposes [ 8 ] and should be attempted. One reason is that this metric does not suffer from the above-mentioned limitation related to the fact that, since everyone eventually dies (the deaths are postponed, not avoided), the long-term gain expressed as a total number of deaths avoided from the reduction of exposure to a harmful environmental factor will appear much smaller than could be thought at first sight when considering the number of deaths avoided during a given year. The number of avoided life-years, by depending both on the number of deaths postponed each year and on the delay in their occurrence induced by the environmental change, takes into account the two dimensions of the problem. The same goes for the change in life expectancy [ 89 ]. Note that a given number of (healthy) life-years lost may translate into very different impacts on life expectancy, depending on how the life-years lost are distributed in the population (something that cannot be determined without strong assumptions). As an illustration, the UK committee on the medical effects of air pollution (COMEAP) concluded that anthropogenic fine particulate matter (PM 2.5 ) at the level observed in 2008 in the UK was associated with an effect on mortality equivalent to nearly 29,000 deaths at typical ages in 2008, and that, depending on how this burden is spread in the whole population, this might correspond to impacts on life expectancy ranging from 6 months (if the air pollution effects was distributed across all deaths) to 11.5 years (if PM 2.5 were only implied in 29,000 deaths) [ 89 ].
The risk estimation relies on a more or less sophisticated combination of the exposure distribution under each counterfactual scenario with the ERF and with an estimate of the baseline disease frequency in the considered population, whether explicitly or hidden in the externally available disease burden. This estimation is repeated under each of counterfactual scenario, and the risk difference between the targeted and “baseline” scenario is computed. In practice, several ways to estimate the risk are used, which are not all strictly valid in theory. The main types of approaches correspond to:
PAF-based formulas: An analytical (formula-based) estimation of a “population attributable fraction” associated with the exposure, multiplied either by the incidence of the disease or by an externally available estimate of the disease burden in the population (i.e., the impact of the disease in the considered population irrespective of all its possible causes, typically, expressed in DALYs);
Person-years method: A simulation of the whole population in which new disease cases occur each year during the course of the study period under various counterfactual scenarios, from which attributable cases, differences in life expectancy, DALYs and other specific measures of risk can be estimated. This approach has the advantage of allowing to take into account the dynamics between death rates, population size and age structure [ 89 ].
Note that alternative approaches exist, such as compartmental models (generally, but not exclusively, used for infectious diseases) or approaches based on individual, as opposed to population-based modeling, such as microsimulations (see e.g. Mueller et al. [ 90 ] for a review). Compartmental models assume that subjects switch between mutually exclusive states (e.g., susceptible, infected, recovered, dead, for an infectious disease) and model the trajectory of each individual from the population across these states via deterministic or probabilistic approaches. They are particularly relevant to model the impact of interventions that may influence infectious diseases, and will not be detailed here (see [ 91 ] for an example). The lifetable (or person-years) approach mentioned below can in a way be seen as a particular example of compartmental models.
As already mentioned, one general issue relates to the consistency between the various metrics used; for example, the data on baseline disease frequency need to correspond to an estimate of disease incidence if the exposure–response function is derived from an etiologic study assessing the occurrence of new disease cases, such as a cohort or incident case-controls study.
The “PAF-based formula” approach can be illustrated taking the simple example in which a binary exposure level is changed from 1 to 0 (e.g., all smokers stop smoking) in the counterfactual situation and in which the overall burden associated with the disease, assumed to correspond to a dichotomous outcome (with/without disease) is available. The health impact is generally estimated in two steps, the first one corresponding to the estimation of the PAF, which, in a situation without confounding, is defined as:
Where Y corresponds to the disease, P(Y = 1) is the proportion of subjects developing the disease in the study period and X is the exposure of interest, with X = 0 corresponding to non-exposed subjects. Equivalently, the PAF can be defined as:
Were R e and R u are the risks of disease in the exposed and unexposed subgroups, respectively.
Note that the assumption about the lack of confounding can be conveniently ignored by relying on the structural causal modeling framework [ 92 ] and the do operator:
where do(X = 0) refers to a situation in which X is set to 0 through a minimally invasive intervention (in the terminology of Pearl [ 92 ]), all other variables possibly influencing X remaining constant; of course, the “reference” value X = 0 can be replaced by any other value or distribution, in the case of an exposure with more than two categories.
Coming back to the situation in which X is binary, the PAF is generally estimated relying on Levin’s formula, which can be derived from the previous ones:
Where P is the prevalence of exposure in the population, or P(X = 1), RR the relative risk associated with exposure (assumed here to be dichotomous). (Note that Rockhill et al. [ 6 ] explain that this formula is not valid in the context of confounding. This is true when one applies the formula ( 4 ) in the study population from which the RR is estimated but not, as in a risk assessment exercise, if one uses (4) to estimate the attributable fraction in a given population using a RR assumed to be unbiased estimated from another population.
The health impact is then estimated by combining the estimated PAF with the burden of the considered disease, BD, in the study population, generally available or approximated from external sources (or estimated via an ad-hoc study):
The unit of BD (e.g., deaths, DALYs, etc.) defines the unit of measure of the health impact.
In the case of a categorical assessment of exposure, the health impact is estimated as above in each exposure category, after which the overall impact is estimated by summing over all exposure levels. If exposure is continuous, formula ( 1 ) above is generalized by integrating the PAF over all exposure levels as shown here:
Where m is the maximal exposure level, P S1 is the observed distribution of exposure level (or baseline scenario), P S2 the distribution of exposure under the counterfactual hypothesis (which may correspond to a uniform distribution with all subjects at zero if zero is the targeted level) and RR the exposure–response function, with RR(x) providing the relative risk when exposure is x.
If the health parameter Y is continuous (e.g., blood pressure, birth weight…), then the impact of X on the average value of Y can be estimated as:
Where β corresponds to the exposure–response function describing the average value of the outcome Y as a function of the outcome. In the case of a binary exposure with prevalence P, the right-hand side of this formula simplifies to β x P. This value can be multiplied by the population size if one wants to express the impact in terms of units of Y (e.g., IQ points) due to the exposure in the population as a whole.
The person-years approach consists in simulating the cohorts corresponding to each of the considered counterfactual scenarios throughout the study period, with new disease cases appearing each year. It has several key advantages over the formula-based approach, including: 1) to make all assumptions more explicit; 2) to avoid issues related to the estimation of the expected number of cases [ 7 , 93 ], since the number of subjects still at risk in each cohort is explicitly estimated; 3) to be more flexible in coping with various exposures simultaneously, assuming various correlation structures between them, with scenarios implying gradual changes in exposure over time, considering sociodemographic changes in the study population, without having to work out an analytical solution. The cost of this approach is that it is generally much more complex to implement and compute.
The estimation needs to be repeated for the other health outcomes identified at the hazard identification step (Identification of exposures above) as being possibly influenced by the considered factor. It can also be repeated for other factors, as we now discuss.
Consideration of multiple risks factors
If several factors are considered (e.g., because one is interested in a prespecified set of exposures, or because the policy evaluated is expected to influence several physical, chemical, psychosocial factors), the estimation needs to be repeated for each of these factors, at least those for which the level of evidence regarding effects on one health outcome are above the selected level, if any (see Handling of the strength of evidence about the effect of environmental factors on health). A central issue here relates to the situation in which two or more of these factors can influence the same health outcome. Indeed, care is required to acknowledge the fact that the fraction of cases of a specific disease attributable to different risk factors can generally not be summed. This is a consequence of the multiplicative nature of risk and of the multifactorial nature of most diseases [ 94 ]; moreover, care is needed to consider possible relations, and in particular correlation, effect measure modifications or mediation between risk factors.
Again, the PAF-based formula and the person-years method can be used when considering several factors influencing the same health outcome, with the latter being more flexible. Regarding the former approach, if population attributable fractions have been estimated for each of the R risk factors influencing the considered outcome, and under some hypotheses (see below), then these can be aggregated with formula ( 6 ):
Where PAF r is the population attributable risk fraction associated with risk factor r estimated independently from the other risk factors. This approach makes strong assumption: that all risk factors act independently (in particular, that no effect of one factor is to some extent mediated by another factor, or modified by another factor) and are not correlated. Note that this formula is identical to that used in toxicology for the so-called case of independent action [ 95 ]. Under these assumptions, if two independent factors have attributable fraction of the cases of 40 and 50% respectively, then their joint action corresponds to an attributable fraction of 70% (40% plus 50% of the remaining 60%).
Some of these assumptions may not hold in real situations.
A first issue relates to the situation in which exposures to the considered factors (say, x 1 and x 2 ) are correlated. Formula ( 8 ) assumes that the fraction of cases attributable to B is the same in the whole population and in the population from which factor A has been removed (independent action), which does not hold if A and B are correlated because then the prevalence of exposure to B is not the same in the two populations. From this it appears that information on the relations between exposures to the considered factors (here, x 1 and x 2 ) in the study population is required to estimate the fraction of cases attributable to x 1 and x 2 . Specifically, their joint distribution P(x 1 , x 2 ) needs to be considered in the PAF estimation, as described in Ezzati et al. [ 96 ]. Formula ( 6 ) can be adapted by replacing each integral by:
this implies of course that information on the joint (and not only marginal) distribution of all relevant factors is available or collected in the population in which the risk assessment is conducted. Biomonitoring and exposome studies, provided they assessed multiple exposures simultaneously in the same participants, allow providing such a joint distribution [ 97 ]. Equation ( 9 ) assumes that the risk for a given combination (x 1 , x 2 ) of exposures X 1 and X 2 is the product of the relative risks associated with X 1 and X 2 , corresponding to a hypothesis of lack of effect measure modification of X 1 by X 2 .
If now there is evidence of effect measure modification (sometimes termed interaction) between risk factors then in principle RR 1 (x 1 ).RR 2 (x 2 ) in Eq. ( 9 ) should be replaced by RR(x 1 ,x 2 ), that is the relative risk function describing the joint effect of x 1 and x 2 , which can incorporate a different relative risk associated with x 1 at each given value of x 2 . To our knowledge, there are currently few examples of risk factors and outcomes for which this function is accurately characterized and available.
Another option to handle it is to consider different ERFs in different population strata; again, one needs information on the joint distribution of all relevant factors, as well as stratum-specific relative risks.
Another (non-exclusive) situation is that of mediation effects [ 98 ]. Consider the case of a disease D (say, lung cancer), influenced by several risk factors including active smoking (A) and green space exposure (B), the effect of which being partly mediated by changes in air pollution levels (C). Figure 7 provides a possible model of the assumed relations between A, B, C and D. Let us assume that one is interested in estimating the overall impact of factors A, B, C on D, that is, the number (or the fraction) of cases of disease D that would be avoided if A, B, and C all had “optimal” levels. The estimated impacts of B (improving green space exposure) and C (getting rid of air pollution) cannot be considered as being independent because a part of the effect of B is included in the effect of C (a mediation issue). Estimates of the share of the effect (in the sense of measure of association) of B on D that is not mediated by C (but that may be mediated by other factors not considered here, such as an increase in physical activity), termed the natural direct effect, can be provided by mediation analysis techniques [ 98 ]. This natural direct effect of B on the disease risk is by construction independent of the effect of C on disease risk, so that the corresponding attributable fractions can then be estimated and combined using formula ( 8 ) above. In the Global Burden Disease methodology, for each pair of risk factors that share an outcome, the fraction of the risk mediated through the other factor is estimated using mediation analysis techniques, if the relevant studies (in which B, C, D are altogether estimated) are available. A concern here (besides the usual assumptions required by mediation analysis [ 98 ]) relates to the transposability from one area to the other of such mediation analyses; for example, the change in air pollution level following a change in green space surface may be influenced by the local share of traffic-related air pollution among the total of the emissions of air pollutants from all sources, which may vary across areas.
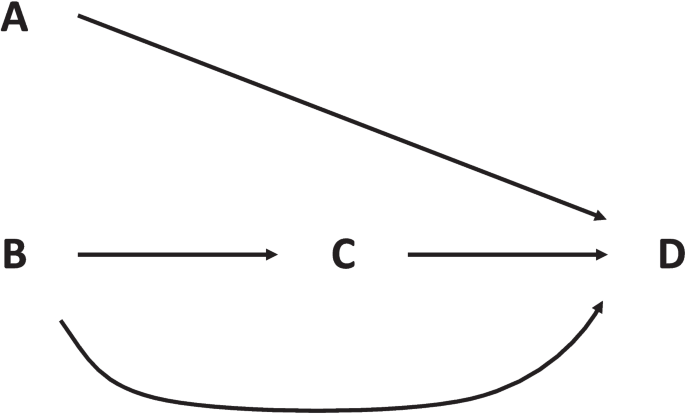
Causal diagram summarizing the causal relations between hypothetical risk factors ( A , B and C ) and a disease D . Here, A and B are assumed to independently affect the probability of disease, while a part of the effect of B on D is mediated by C
In the case of a continuous health outcome, one option to estimate the joint impact of several factors, which assumes a lack of synergy (or of departure from additivity) is to sum the average changes in the outcome attributed to each exposure to obtain an estimate of the impact of the combined exposure.
Cessation lag
The cessation lag can be defined as the time lag between the implementation of the considered intervention and the consequent change in hazard rate. It is meant to take into account the fact that for most (chronic) clinical endpoints, the effect of changes in (external) risk factors does not manifest fully immediately. The COMEAP study of particulate matter impacts on mortality [ 89 ] provides an illustration of the impact of various cessation lags (see also Fig. 8 ). Such a cessation lag can be implemented in studies relying on person-year approaches. Whether a cessation lag needs to be considered depends on the availability of knowledge about when a change in exposure will start influencing the considered health outcomes, as well as on the question asked: if for example one is interested in knowing how health is likely to vary in the short and mid-term if one managed to implement a given intervention now (or in a near future), then considering a cessation lag is relevant; if the question of interest is the more theoretical one of quantifying how much better the population health would be today if a given exposure or set of exposure was absent (or if a specific intervention had been implemented a long time ago), then cessation lags might be ignored.
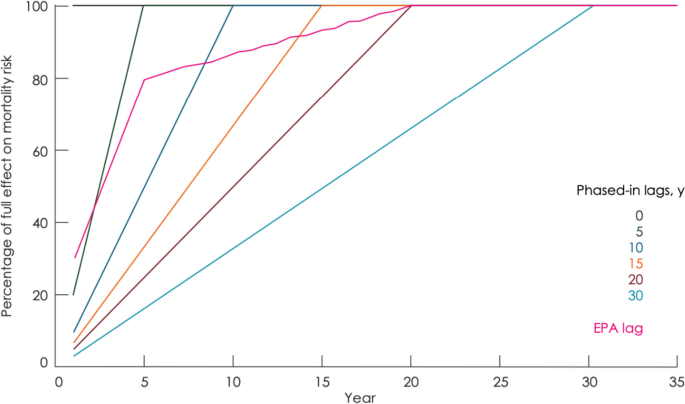
Illustration of possible cessation lags (in years) considered in the estimation of the impact of fine particulate matter exposure on mortality [ 89 ]. The first year of the intervention implementation is designated as year one
Socio-economic impact of attributable health effects
One may wish to go beyond the health impact estimates. Economic analysis can further inform choices between different project or policy alternatives considering dimensions beyond health. Such an analysis can be limited to quantifying the costs of implementation of the considered scenarios or policies (e.g., those required to induce a reduction of exposure to a specific factor), and relate these costs to the health benefits expressed in non-monetary terms, such as DALYs (corresponding to a cost–benefit analysis). Beyond this, cost–benefit analyses have the advantage of allowing to compare the costs and monetized health and non-health benefits. Such an analysis is more complete and may be more meaningful for decision-makers than one ignoring the costs of implementation of each of the considered alternatives.
The benefits include the monetization of health benefits, which takes into account both tangible and intangible costs. The tangible costs refer both to direct costs, in particular the costs to the health system (costs of specific treatments for each pathology: drugs, consultation, hospitalization, etc.) and indirect costs linked to absenteeism and the resulting loss of productivity. The intangible costs refer to the inclusion in the economic analysis of the loss of well-being due to anxiety, discomfort, sadness or the restriction of leisure or domestic activities due. These are therefore non-market costs for which it is necessary to reveal their value through survey methods or analysis of the behavior implicitly attributed to them. For example, Ready et al. [ 99 ] and Chilton et al. [ 100 ] valued the willingness to pay to avoid an air pollution-related morbidity episode. Among these intangible costs, the economic valuation of mortality is a delicate step from an ethical point of view in the absence of consensual values. It is generally based on the monetary valuation of a statistical life or a year of life lost. In recent years, the most popular approach in the economic literature to determining the statistical value of a human life is the willingness-to-pay approach. The statistical value of a human life is thus approximated by the amount of money a society is willing to pay to reduce the risk exposure of each of its members. This literature shows that the statistical value of a human life depends on characteristics such as age at death, time between exposure and death (i.e. latency), and nature of the underlying risk [ 101 , 102 ]. Empirical assessments have provided a range of values generally between €0.7 and €10 million per human life. The other approach used is that of revealed preferences, which is based on observed behavior: for example, the difference in wages between two branches of economic activity with different mortality risks.
The cost–benefit analysis, beyond the health benefits directly generated by the project or policy, can also integrate co-benefits not directly related to health. For example, Bouscasse et al. [ 29 ] considered the following co-benefits of measures to reduce fine particle pollution: the reduction of noise, the development of active mobility on health, which lead to health co-benefits, but also the reduction of greenhouse gas emissions, which includes benefits not related to health.
With regard to the evaluation of costs, it is necessary to define the scope: is it the cost of implementing the policy for the public authorities? Should the impact on household expenditure also be taken into account? This may be important, for example, in the case of measures aimed at reducing air pollution through actions on heating or transport, for which individuals carry a part of the cost. The assessment may also seek to quantify the impact on employment, for example, or on imports or exports of goods and equipment.
Finally, the time dimension is important in the implementation of a cost–benefit analysis. While costs usually occur at the beginning of the period (implementation of actions or policies, investments), benefits tend to occur later. Indeed, health benefits are generated over time depending i) on the speed of implementation of actions and of the progressiveness of the reduction of exposures, and ii) on the fact that health benefits may not be immediate following the reduction of this exposure (cessation lag).
The time lag between costs and benefits has another consequence in a cost–benefit analysis. We do not give the same value to €1 to be paid today or in several years, because of what economists call the preference for the present. This is introduced into cost–benefit analysis through a discount rate , which gives a present value to monetary flows in the future. The higher the discount rate used by the public authority or by the stakeholders, the lower the present value of benefits that occur later.
Sensitivity and uncertainty analyses
Sources of uncertainty in quantitative risk assessment studies.
Uncertainties exist at each step of risk assessment. For example, there may be uncertainties in the health outcomes influenced by the considered factor or policy, in the level of evidence relating the factor or policy with a given health outcome, in the corresponding dose–response function, in exposure distribution… We will here distinguish uncertainties due to the variability of the quantitative parameters considered in a risk assessment study (typically, in the dose–response function, but also possibly in parameters of higher dimension, such as the distribution of an exposure in the population) from more systemic uncertainties related to the model choice (sometimes termed systemic or epistemic uncertainties , e.g. related to the assumption that the risk factor(s) considered only influences a specific health outcome, possibly disregarding health effects not identified yet or for which a dose–response function is not available) [ 103 ]. A typology of sources of uncertainty in burden of diseases studies is presented in Knoll et al. [ 104 ]. We will focus here on uncertainty due to the variability in parameters, and touch upon systemic uncertainty in Sect. " Sensitivity and uncertainty analyses " below.
The consideration of the uncertainty related to variability typically implies to obtain an estimate of the uncertainties occurring at each step of the study, in order to combine these uncertainties (uncertainty propagation, or error propagation) and try providing an estimate of the resulting uncertainty on the overall study results.
Estimating the impact of uncertainties
In the simple case of a single source of uncertainty, the translation of this uncertainty on the overall results is in principle relatively straightforward; for example, if one only considers uncertainty on a dose response function expressed using a relative risk and (simplistically) assumes that this uncertainty is conveyed by the confidence interval of the relative risk, then the estimation of the health impact can be repeated using the limits of the confidence interval instead of the point estimate of the relative risk (of course, the confidence interval usually only conveys uncertainties related to the population sampling, more specifically to random error related to sampling.
However, there are multiple sources of uncertainty beyond those related to sampling error. Indeed, in the classical view of epidemiology (developed for measures of association), the uncertainty due to variability can be seen as having a random and a systemic component; only the former is easily estimated, while the estimation of bias requires quantitative bias assessment methods [ 105 ] that are seldom applied. In particular, sources of uncertainty related to exposure measurement error, to the assessment of disease frequency, possibly confounding bias or uncertainties in the shape of the exposure response function and the existence and shape of cessation lag, are not conveyed by the confidence interval of the relative risk and are worth considering – but rarely taken into account.
If one tries to simultaneously take into account several sources of uncertainty, then more complex approaches are required to propagate the uncertainty down to the impact estimate. Although analytical approaches (such as the delta-method) may be applicable in relatively simple situations, one more general approach corresponds to Monte-Carlo simulations. Monte-Carlo simulations rely on the principle of repeating a large number of times the health impact estimation, letting all underlying parameters (relative risks, exposure distribution, possibly the number of factors influencing the health outcome and the outcomes that they influence, if there are uncertainties at this level…) vary across realistic values [ 106 , 107 ]. They allow providing an estimate of the distribution of the possible values of the health impact. This requires knowledge or assumptions on the likely distribution of each parameter considered in the study. If such an approach is implemented, then authors will be able to report a distribution of the most likely value of the health impact or cost. The results will for example be conveyed in a way such as: “Taken all identified sources of uncertainty into account, there is a 90% probability that the number of deaths attributable to factor A in the area is above 10, a 50% chance that it is above 30 and a 10% chance that it is above 50 cases” (with of course specific explanations for a non-scientific audience). Alternative approaches to Monte-Carlo simulations also exist, in particular in the context of Bayesian modeling [ 108 ]. Provided relevant data are available, this framework can in principle accommodate both the uncertainty related to variability, but also systemic uncertainty [ 109 ].
In the absence of formal consideration of the systemic uncertainty in the uncertainty analysis, it remains essential for the investigators to state their model’s assumptions and limitations, including in particular the impacts related to specific risk factors or health outcomes that could not be taken into account in the quantitative assessment (see also 3.3 below).
Quantitative assessment studies are at the interplay between scientific, policy and legal issues; contrarily to what the deceptively simple epidemiological concept and formula of the “population attributable fraction” may let think [ 7 , 8 ], their implementation and interpretation is very challenging.
We have reviewed some of the possible approaches and issues at each step of risk assessment studies. We have made the choice not to discuss the steps of problem framing, study reporting, and issues related to population participation, which are presented elsewhere [ 19 , 21 ]. In the absence of broad methodological studies (e.g., via simulation approaches) in this field, we acknowledge that some of the choices we have done in presenting methods carry some amount of subjectivity and encourage the development of studies to quantitatively assess bias and trade-offs in this area to help investigators make more informed choices with regards to the methodological options. Such simulation studies could e.g., be used to select the most efficient approach to assess exposures in a given context.
To conclude, we will touch upon issues related to the terminology of risk assessment and HIA studies, the distinction between human-derived and animal-derived (toxicological) risk assessment studies, and research needs.
Issues related to terminology
Studies aiming at characterizing the health and societal impact of policies or environmental factors are riddled with many different terminologies and acronyms. This diversity of acronyms encompasses some real differences in aims or methodology, but is also due to the convergence of various research and application streams. Indeed, as already mentioned, these studies originate from the epidemiological research stream related to the concept of population attributable fraction, which dates back to the 1950s [ 7 ], from the development of legal requirements for environmental impact assessment before the development of new policies, plans or programs (which progressively also encompassed health issues), and from the applied stream of chemical risk assessment based on “regulatory toxicology” approaches and the risk assessment logic outlined in the USA National Research Council originally published in 1983 and also known as the “red book [ 1 , 2 ]. Three key expressions are used: 1) burden of disease; 2) risk assessment; 3) health impact assessment.
Burden of disease studies generally correspond to an assessment of the risk (e.g., in terms of attributable cases or DALYs) associated with a given disease in human populations, without referring to an exposure possibly causing the disease or to a policy aiming at limiting its impact. However, when used in relation with a factor or family of factors, then only the risk (or disease burden) associated with this factor is considered (e.g., in “environmental burden of disease”), so that there is no essential distinction anymore with what we have discussed here. In practice, health impact assessment is often used in relation with a policy or intervention likely to affect health, while environmental burden of disease is often used in the absence of explicit consideration of a policy or intervention (see below).
Regarding health impact assessment, a difficulty arises from the fact that much of the theory and examples of HIA studies has been published in the grey literature [ 10 ]. The term has most often been used to assess the potential impact of a public policy, plan, program or project not yet implemented [ 3 ]. HIA is defined by WHO as “a combination of procedures, methods and tools by which a policy, program or project may be judged as to its potential effects on the health of a population, and the distribution of those effects within the population”. In addition, the consideration of inequalities (i.e., considering the distribution of risk within a population rather than only its mean value) has been put forward as an essential part of HIAs, at least in principle [ 110 ]. Several distinctions exist within the field of HIAs, which refer to various notions and dimensions [ 10 , 110 , 111 ]. Some of these notions are at different levels and hence not mutually exclusive (for example some distinctions refer to the way health is conceptualized, other to the qualitative or quantitative nature of the study, others to the level of participation of the considered population), making it difficult to suggest a simple and unified terminology. A distinction between “broad focus” HIA studies, in which “a holistic model of health is used, democratic values and community participation are paramount and in which quantification of health impacts is rarely attempted” [ 10 ], and “tight focus” HIAs, based on epidemiology and toxicology and tending towards measurement and quantification, is sometimes done [ 10 ]. “Analytical HIA” is sometimes used synonymously to these “tight focus” HIAs. Harris-Roxas and Harris also quote distinctions such as between quantitative and qualitative HIAs, to those relying on “tight” or “broad” definitions of health, to HIAs of projects or policies [ 111 ]. What we have reviewed here is close (if not equivalent) to these “analytical”, “tight focus” or “quantitative” HIAs.
Such quantitative HIAs typically aim at answering a question about the future (“how is the planned policy expected to affect the health of the concerned population?”) while many risk assessment studies aim at answering a question about the present, and are sometimes presented as considering a single factor at a time (typically, “how many lung cancer cases would be avoided in this city if WHO air pollution guidelines were respected?”). We come back to these apparent differences in the Sect. " Assessing the impact of policies versus the impact of exposures ".
As already stated, in practice, some HIA or “risk assessment” exercises fall short of providing a quantitative estimate of the risk, e.g., because of a lack of relevant dose response functions or support to collect missing information; they may for example stop at the step of hazard identification. It is, in a way, what happens with animal-based risk assessment studies.
Animal-based risk assessment studies
It is important to recall that in addition to the approach based on human-derived dose–response functions that we described above exists a whole stream of research and applied studies relying on animal-based dose–response functions and so-called toxicological reference values. The core approach of the benchmark dose (BMDL) consists in identifying an exposure level corresponding to an effect considered small in an animal model (say, a 5% decrease in organ weight or 5% increase in the frequency of a disease) [ 112 ]. The lower confidence bound of the benchmark dose is then divided by an uncertainty factor (typically, 100), to take into account between-species and within-species differences in sensitivity, and this value is used as a “daily tolerable dose”, or compared to the exposure distribution in a specific human population. Therefore, this approach aims at identifying a range of doses under which, under certain assumptions, there would be no “appreciable adverse health effects” of exposure. The comparison of the estimated daily tolerable dose with the exposure distribution in the considered population allows to identify if a substantial share of the population is above this daily tolerable dose, and thus finds itself at exposure levels that cannot be deemed safe (a qualitative rather than quantitative statement about risk). For this reason, these risk assessment approaches based on animal-derived reference values or dose–response functions rather correspond to safety assessment : they can allow to state that, given its exposure distribution, a given human population is “safe”, or unlikely to suffer appreciable adverse health effects, with respect to a specific exposure (or set of exposures if the approach is used in the context of mixtures of exposures) and do not strictly correspond to risk assessment as we defined it, i.e., the estimation of a number of disease cases (risks) attributable to an exposure in a population. To limit ambiguity, it might be relevant either to use the expression “safety assessment” when referring to the so-called risk assessment studies relying on animal derived toxicological reference values, or to distinguish “animal-based risk assessment” (which implies between-species extrapolation) from “human-based risk assessment” (which does not).
Assessing the impact of policies versus the impact of exposures
The factors influencing health considered in risk assessment studies range from single behaviors (e.g., smoking, physical activity), to physical and chemical factors such as particulate matter [ 35 , 113 ] or other environmental exposures including lead or families of factors such as endocrine disruptors [ 12 , 75 ]; these can be considered at various scales, from the neighborhood, region, country or at the planetary scale (e.g. as done by the Global Burden of Disease Studies, or by a study considering different ozone layer depletion scenarios [ 37 ]). In addition, as described above, the formalism of risk assessment studies used for a single exposure can be extended to the case of two or more exposures, at least under certain assumptions. Consequently, if one is interested in a project (e.g., the building of a road infrastructure, a factory) or a policy (regulating a behavior such as smoking, alcohol consumption, speed limit on highways, frequency of social contacts or exposure to a chemical or set of chemical factors, or consisting in taxes aiming at modifying exposures or behaviors), and if it is possible to provide a quantitative estimate of the expected changes in the factors affecting health impacted by this project or policy, then the methodology of risk assessment as described above can be used to provide an estimation of the impact of this project or policy. Symmetrically, evaluating the impact of a factor, such as an atmospheric pollutant, implies, as we have seen, to compare a given situation (usually, the current one or a future of this situation assuming a “business as usual” scenario) with a counterfactual situation (a hypothetical present in which a behavior or an exposure has been altered at the population level) that can be seen as resulting from a policy or an infrastructure. For example, assessing the risk associated with air pollution exposure implies to consider a counterfactual level (e.g., the WHO air pollution guidelines); the estimated risk is then identical to the health gain expected from a policy that would allow to lower air pollution from the current situation down to this guideline value (see Fig. 9 for an illustration). In other words, assessing ex-ante the impact of a hypothetical policy or infrastructure (what is sometimes termed health impact assessment) boils down to evaluating the health impact of its immediate consequences relevant for health (a set of factors); and assessing the impact of one or several behavioral, social or environmental factors (for which the expression risk assessment is usually reserved) is equivalent to considering the impact of a policy that would alter this or these factor(s). There may be some differences in implementation between the two specific questions (e.g., one may want to assume that it takes a few years for air pollution to reach the target value in the case of the evaluation of a policy, while an estimation of the current impact only requires to compare two “parallel worlds” with distinct air pollution levels), but these are not always considered and can be seen as minor technical differences. For these reasons, there are no essential differences in quantitatively assessing the effect of a single factor, of several factors, or of a policy or project. Recognizing this similarity in design of risk assessment and analytical/quantitative HIAs may allow to bring more clarity in the methodology and terminology; in particular, it may be relevant to adopt a unified terminology allowing to point to the differences that bear strong consequences, such as whether the study relies on human-based dose response functions (as illustrated here) or on dose–response functions derived from animal models.
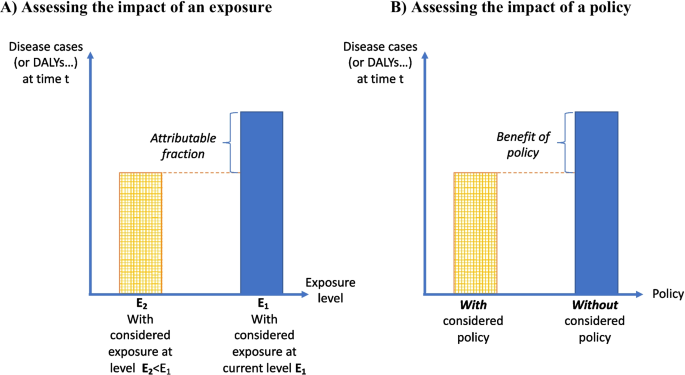
Illustration of the similitude of the principles of risk assessment of an exposure ( A ) and of a policy or program ( B ). When considering an exposure ( A ), the fraction of disease cases attributable to a specific exposure (compared to a lower and theoretically achievable level) is estimated for time t (typically assumed to correspond to the current time). When considering a policy ( B ), the expected health benefit of the project or policy (consisting in changing the level of one or more environmental factors) is estimated, considering the population at the current time or at a later time t, comparing it to the situation without change. Both approaches can be seen as aiming to estimate the impact of a theoretical policy or intervention lowering (or, more generally, changing) the level of one or several environmental factors, compared to a reference situation considered at the same time period
The perils of quantification: leaving emerging hazards by the roadside
Risk assessment studies imply many steps requiring a large amount of data; this is all the more true in the case of studies considering simultaneously multiple exposures, exposures with effects on multiple health endpoints (such as tobacco smoke, particulate matter, lead, physical activity…), or policies likely to influence several exposures or behaviors (such as a “zero pollution action plan”, as envisioned by the European Commission, or a set of actions to limit greenhouse gas emissions in multiple sectors). In some (probably not infrequent) cases, only a fraction of the data relevant for the risk assessment will be available or possibly available within a limited time frame. Researchers are then facing several non-exclusive options:
collect additional data (the scientifically rigorous approach); this may take a long time and be very expensive, since in many cases the missing data correspond to dose–response functions, which are typically generated by cohort studies. For example, in an ongoing exposome study conducted as part of ATHLETE project and considering 74 exposure-outcome pairs corresponding to effects with a level of evidence deemed likely or more than likely, a human dose–response function could be identified for only 70% of these possible effects (Rocabois et al., personal communication). Although working with high-quality data, even if this implies to delay the availability of the final results, is often the preferred option in science, such an option is problematic for health impact assessment studies, which often require to be conducted within a constrained time frame so that a decision about the planned policy or a possibly harmful exposure can be quickly taken, to bring potential health benefits to society or inform a legal process;
perform the study with the limited data available in the constrained time frame (imperfect but timely approach); in this case, it is possible that only a fraction of the impact of the exposure(s) or policy will be assessed (because several dose response functions corresponding to the effects of the exposure or policy are available) and that the quantified fraction will be estimated with large uncertainties;
perform a purely qualitative health impact assessment study (qualitative approach);
not to perform the study (“analysis paralysis”).
In many cases, option 2), consisting in moving along with the limited data available, will be preferred. The consequence may be that a fraction, which may be large, of the impact, will be ignored. Thus, because of their relative complexity, health impact assessment studies, which aim to make health impacts visible, may paradoxically leave a large fraction of this impact on the roadside. Impacts left on the road side will often correspond to “emerging” (newly identified factors, newly identified effects) risks. Under this imperfect but timely approach, it is essential not only to try to provide a quantification of the uncertainty around the quantified part (see above, Sect. " Sensitivity and uncertainty analyses "). It would also be relevant to attempt providing some estimate of the magnitude of what has obviously been left out (for example, the impact of a known exposure likely to affect an outcome for which no dose–response function is available), or at least to make the missing part (the “known unknown”) visible in some way.
Identified gaps
This review provided a general methodological framework for risk assessment studies and demonstrated their relevance to also consider the expected impact of policies and infrastructures, and therefore their closeness to health impact assessment studies; it illustrated recent development related to the diversity of approaches to assess factors at the individual levels (such as fine-scale environmental models and personal dosimeters), and the potentially strong impact of choices regarding exposure assessment tools, including the consideration of population density when environmental models are used. It also allowed to identify some gaps, challenges or pending issues in the methodology of risk assessment studies. These issues include 1) proposing a formal approach to the quantitative handling of the level of evidence regarding each exposure-health outcome pairs (see Handling of the strength of evidence about the effect of environmental factors on health); 2) more generally, develop more formal and if possible quantitative assessment of the health impacts not handled by a specific quantitative risk assessment study (the “know unknowns”); 3) confronting the approaches of risk assessment based on human dose–response function reviewed here with that relying on toxicological data; and 4) other technical issues related to the simultaneous consideration of several exposures (or of policies acting on health via changes in several environmental factors), in particular when some of these exposures are causally related.
Availability of data and materials
Not applicable.
National Research Council. Risk assessment in the federal government. Managing the process. Washington, DC: National Academy Press; 1983.
Google Scholar
National Research Council (U.S.). Committee on Risk Assessment of Hazardous Air Pollutants. Science and judgment in risk assessment. Washington, D.C: National Academy Press; 1994.
Cole BL, Fielding JE. Health impact assessment: a tool to help policy makers understand health beyond health care. Annu Rev Public Health. 2007;28:393–412.
Article Google Scholar
Bell ML, Davis DL. Reassessment of the lethal London fog of 1952: novel indicators of acute and chronic consequences of acute exposure to air pollution. Environ Health Perspect. 2001;109(Suppl 3):389–94.
Article CAS Google Scholar
Clancy L, Goodman P, Sinclair H, Dockery DW. Effect of air-pollution control on death rates in Dublin, Ireland: an intervention study. Lancet. 2002;360(9341):1210–4.
Rockhill B, Newman B, Weinberg C. Use and misuse of population attributable fractions. Am J Public Health. 1998;88(1):15–9.
Poole C. A history of the population attributable fraction and related measures. Ann Epidemiol. 2015;25(3):147–54.
Greenland S, Robins JM. Conceptual problems in the definition and interpretation of attributable fractions. Am J Epidemiol. 1988;128(6):1185–97.
Bobst S. History of risk assessment in toxicology. London, San Diego: Academic Press is an imprint of Elsevier; 2017.
Mindell JS, Boltong A, Forde I. A review of health impact assessment frameworks. Public Health. 2008;122(11):1177–87.
Slama R. Causes et conditions extérieures des maladies et de la santé. Paris: Collège de France/Fayard; 2022.
Book Google Scholar
Trasande L, Zoeller RT, Hass U, Kortenkamp A, Grandjean P, Myers JP, DiGangi J, Hunt PM, Rudel R, Sathyanarayana S, et al. Burden of disease and costs of exposure to endocrine disrupting chemicals in the European Union: an updated analysis. Andrology. 2016;4(4):565–72.
Shaffer RM, Sellers SP, Baker MG, de Buen Kalman R, Frostad J, Suter MK, Anenberg SC, Balbus J, Basu N, Bellinger DC, et al. Improving and expanding estimates of the global burden of disease due to environmental health risk factors. Environ Health Perspect. 2019;127(10):105001.
G. B. D. Risk Factors Collaborators. Global burden of 87 risk factors in 204 countries and territories, 1990–2019: a systematic analysis for the Global Burden of Disease Study 2019. Lancet. 2020;396(10258):1223–49.
OECD. OECD Environmental Outlook to 2050: the consequences of inaction. In: OECD Environmental Outlook. 2012.
Fillol C, Oleko A, Saoudi A, Zeghnoun A, Balicco A, Gane J, Rambaud L, Leblanc A, Gaudreau E, Marchand P, et al. Exposure of the French population to bisphenols, phthalates, parabens, glycol ethers, brominated flame retardants, and perfluorinated compounds in 2014–2016: results from the Esteban study. Environ Int. 2021;147:106340.
Lobo Vicente J, Ganzleben C, Gasol R, Marnane I, Gilles L, Buekers J, Bessems J, Colles A, Gerofke A, David M, et al. HBM4EU results support the chemicals’ strategy for sustainability and the zero-pollution action plan. Int J Hyg Environ Health. 2023;248:114111.
Lebret E. Integrated environmental health impact assessment for risk governance purposes; across what do we integrate? (INTARESE HEIMTSA). Int J Environ Res Public Health. 2015;13(1):71.
Briggs DJ. A framework for integrated environmental health impact assessment of systemic risks. Environ Health. 2008;7:61.
Harris-Roxas B, Viliani F, Bond A, Cave B, Divall M, Furu P, Harris P, Soeberg M, Wernham A, Winkler M. Health impact assessment: the state of the art. Impact Assess Proj Apprais. 2012;30(1):43–52.
Nieuwenhuijsen MJ, Khreis H, Verlinghieri E, Mueller N, Rojas-Rueda D. Participatory quantitative health impact assessment of urban and transport planning in cities: a review and research needs. Environ Int. 2017;103:61–72.
Brown SL. Quantitative risk assessment of environmental hazards. Annu Rev Public Health. 1985;6:247–67.
Goldstein BD. Advances in risk assessment and communication. Annu Rev Public Health. 2005;26:141–63.
Grant MJ, Booth A. A typology of reviews: an analysis of 14 review types and associated methodologies. Health Info Libr J. 2009;26(2):91–108.
Health impact assessment (HIA) tools and methods. https://www.who.int/tools/health-impact-assessments .
Kunzli N, Kaiser R, Medina S, Studnicka M, Chanel O, Filliger P, Herry M, Horak F Jr, Puybonnieux-Texier V, Quenel P, et al. Public-health impact of outdoor and traffic-related air pollution: a European assessment. Lancet. 2000;356(9232):795–801.
Barboza EP, Cirach M, Khomenko S, Iungman T, Mueller N, Barrera-Gomez J, Rojas-Rueda D, Kondo M, Nieuwenhuijsen M. Green space and mortality in European cities: a health impact assessment study. Lancet Planet Health. 2021;5(10):e718-730.
Lhachimi SK, Nusselder WJ, Smit HA, Baili P, Bennett K, Fernandez E, Kulik MC, Lobstein T, Pomerleau J, Boshuizen HC, et al. Potential health gains and health losses in eleven EU countries attainable through feasible prevalences of the life-style related risk factors alcohol, BMI, and smoking: a quantitative health impact assessment. BMC Public Health. 2016;16:734.
Bouscasse H, Gabet S, Kerneis G, Provent A, Rieux C, Ben Salem N, Dupont H, Troude F, Mathy S, Slama R. Designing local air pollution policies focusing on mobility and heating to avoid a targeted number of pollution-related deaths: Forward and backward approaches combining air pollution modeling, health impact assessment and cost-benefit analysis. Environ Int. 2022;159:107030.
Zeng Z, Cook AR, Chen JI, van der Eijk Y. Evaluating the public health impact of partial and full Tobacco flavour bans: a simulation study. Lancet Reg Health West Pac. 2022;21:100414.
Martínez-Solanas È, Quijal-Zamorano M, Achebak H, Petrova D, Robine JM, Herrmann FR, Rodó X, Ballester J. Projections of temperature-attributable mortality in Europe: a time series analysis of 147 contiguous regions in 16 countries. Lancet Planet Health. 2021;5(7):e446-454.
Pearl J, Glymour M, Jewell NP. Causal inference in statistics: a primer. Chichester: John Wiley & Sons Ltd; 2016. p. 1 online resource.
Hernan MA, Robins JM. Causal inference: what if. Boca Raton: Chapman & Hall/CRC; 2020.
Morelli X, Rieux C, Cyrys J, Forsberg B, Slama R. Air pollution, health and social deprivation: a fine-scale risk assessment. Environ Res. 2016;147:59–70.
Pascal M, Corso M, Chanel O, Declercq C, Badaloni C, Cesaroni G, Henschel S, Meister K, Haluza D, Martin-Olmedo P, et al. Aphekom - assessing the public health impacts of urban air pollution in 25 European cities: results of the Aphekom project. Sci Total Environ. 2013;449:390–400.
Mueller N, Rojas-Rueda D, Basagana X, Cirach M, Cole-Hunter T, Dadvand P, Donaire-Gonzalez D, Foraster M, Gascon M, Martinez D, et al. Urban and transport planning related exposures and mortality: a health impact assessment for cities. Environ Health Perspect. 2017;125(1):89–96.
van Dijk A, Slaper H, den Outer PN, Morgenstern O, Braesicke P, Pyle JA, Garny H, Stenke A, Dameris M, Kazantzidis A, et al. Skin cancer risks avoided by the Montreal protocol–worldwide modeling integrating coupled climate-chemistry models with a risk model for UV. Photochem Photobiol. 2013;89(1):234–46.
Dimick JB, Ryan AM. Methods for evaluating changes in health care policy: the difference-in-differences approach. JAMA. 2014;312(22):2401–2.
Petkova EP, Gasparrini A, Kinney PL. Heat and mortality in New York City since the beginning of the 20th century. Epidemiology. 2014;25(4):554–60.
Morelli X, Gabet S, Rieux C, Bouscasse H, Mathy S, Slama R. Which decreases in air pollution should be targeted to bring health and economic benefits and improve environmental justice? Environ Int. 2019;129:538–50.
What are the WHO air quality guidelines? https://www.who.int/news-room/feature-stories/detail/what-are-the-who-air-quality-guidelines .
Rothman KJ, Gallacher JE, Hatch EE. Why representativeness should be avoided. Int J Epidemiol. 2013;42(4):1012–4.
Nieuwenhuijsen M, Paustenbach D, Duarte-Davidson R. New developments in exposure assessment: the impact on the practice of health risk assessment and epidemiological studies. Environ Int. 2006;32(8):996–1009.
Heinrich J, Gehring U, Cyrys J, Brauer M, Hoek G, Fischer P, Bellander T, Brunekreef B. Exposure to traffic related air pollutants: self reported traffic intensity versus GIS modelled exposure. Occup Environ Med. 2005;62(8):517–23.
Traore T, Forhan A, Sirot V, Kadawathagedara M, Heude B, Hulin M, de Lauzon-Guillain B, Botton J, Charles MA, Crepet A. To which mixtures are French pregnant women mainly exposed? A combination of the second French total diet study with the EDEN and ELFE cohort studies. Food Chem Toxicol. 2018;111:310–28.
Villanueva CM, Cantor KP, Grimalt JO, Malats N, Silverman D, Tardon A, Garcia-Closas R, Serra C, Carrato A, Castano-Vinyals G, et al. Bladder cancer and exposure to water disinfection by-products through ingestion, bathing, showering, and swimming in pools. Am J Epidemiol. 2007;165(2):148–56.
Ouidir M, Giorgis-Allemand L, Lyon-Caen S, Morelli X, Cracowski C, Pontet S, Pin I, Lepeule J, Siroux V, Slama R. Estimation of exposure to atmospheric pollutants during pregnancy integrating space-time activity and indoor air levels: does it make a difference? Environ Int. 2015;84:161–73.
Woodward H, Schroeder A, de Nazelle A, Pain CC, Stettler MEJ, ApSimon H, Robins A, Linden PF. Do we need high temporal resolution modelling of exposure in urban areas? A test case. Sci Total Environ. 2023;885:163711.
de Hoogh K, Korek M, Vienneau D, Keuken M, Kukkonen J, Nieuwenhuijsen MJ, Badaloni C, Beelen R, Bolignano A, Cesaroni G, et al. Comparing land use regression and dispersion modelling to assess residential exposure to ambient air pollution for epidemiological studies. Environ Int. 2014;73:382–92.
Sellier Y, Galineau J, Hulin A, Caini F, Marquis N, Navel V, Bottagisi S, Giorgis-Allemand L, Jacquier C, Slama R, et al. Health effects of ambient air pollution: do different methods for estimating exposure lead to different resifts? Environ Int. 2014;66:165–73.
Kulhánová I, Morelli X, Le Tertre A, Loomis D, Charbotel B, Medina S, Ormsby J-N, Lepeule J, Slama R, Soerjomataram I. The fraction of lung cancer incidence attributable to fine particulate air pollution in France: impact of spatial resolution of air pollution models. Environ Int. 2018;121:1079–86.
Raaschou-Nielsen O, Andersen ZJ, Beelen R, Samoli E, Stafoggia M, Weinmayr G, Hoffmann B, Fischer P, Nieuwenhuijsen MJ, Brunekreef B, et al. Air pollution and lung cancer incidence in 17 European cohorts: prospective analyses from the European Study of Cohorts for Air Pollution Effects (ESCAPE). Lancet Oncol. 2013;14(9):813–22.
Hamra GB, Guha N, Cohen A, Laden F, Raaschou-Nielsen O, Samet JM, Vineis P, Forastiere F, Saldiva P, Yorifuji T, et al. Outdoor particulate matter exposure and lung cancer: a systematic review and meta-analysis. Environ Health Perspect. 2014;122(9):906–11.
Nieuwenhuijsen MJ, Donaire-Gonzalez D, Foraster M, Martinez D, Cisneros A. Using personal sensors to assess the exposome and acute health effects. Int J Environ Res Public Health. 2014;11(8):7805–19.
Brunekreef B, Noy D, Clausing P. Variability of exposure measurements in environmental epidemiology. Am J Epidemiol. 1987;125(5):892–8.
Avery CL, Mills KT, Williams R, McGraw KA, Poole C, Smith RL, Whitsel EA. Estimating error in using ambient PM2.5 concentrations as proxies for personal exposures: a review. Epidemiology. 2010;21(2):215–23.
Avery CL, Mills KT, Williams R, McGraw KA, Poole C, Smith RL, Whitsel EA. Estimating error in using residential outdoor PM2.5 concentrations as proxies for personal exposures: a meta-analysis. Environ Health Perspect. 2010;118(5):673–8.
Nethery E. From measures to models: predicting exposure to air pollution among pregnant women. MSc. Vancouver: The University of British Columbia; 2007.
Ballesta PP, Field RA, Connolly R, Cao N, Baeza Caracena A, De Saeger E. Population exposure to benzene: one day cross-sections in six European cities. Atmospheric Environ. 2006;40(18):3355–66.
Slama R, Thiebaugeorges O, Goua V, Aussel L, Sacco P, Bohet A, Forhan A, Ducot B, Annesi-Maesano I, Heinrich J, et al. Maternal personal exposure to airborne benzene and intrauterine growth. Environ Health Perspect. 2009;117(8):1313–21.
Li C, Li Q, Tong D, Wang Q, Wu M, Sun B, Su G, Tan L. Environmental impact and health risk assessment of volatile organic compound emissions during different seasons in Beijing. J Environ Sci (China). 2020;93:1–12.
Zhang R, Smit AK, Espinoza D, Allen M, Reyes-Marcelino G, Kimlin MG, Lo SN, Sharman AR, Law MH, Kanetsky PA, et al. Validation of self-reported sun exposure against electronic ultraviolet radiation dosimeters. Int J Epidemiol. 2022;52(1):324–8.
Kreuzer M, Heinrich J, Wolke G, Schaffrath Rosario A, Gerken M, Wellmann J, Keller G, Kreienbrock L, Wichmann HE. Residential radon and risk of lung cancer in Eastern Germany. Epidemiology. 2003;14(5):559–68.
Jacobs N, Roberts B, Reamer H, Mathis C, Gaffney S, Neitzel R. Noise exposures in different community settings measured by traditional dosimeter and smartphone app. Appl Acoust. 2020;167:107408.
Woodruff TJ, Zota AR, Schwartz JM. Environmental chemicals in pregnant women in the United States: NHANES 2003–2004. Environ Health Perspect. 2011;119(6):878–85.
Calafat AM, Ye X, Silva MJ, Kuklenyik Z, Needham LL. Human exposure assessment to environmental chemicals using biomonitoring. Int J Androl. 2006;29(1):166–71 discussion 181 – 165.
Dereumeaux C, Fillol C, Charles MA, Denys S. The French human biomonitoring program: first lessons from the perinatal component and future needs. Int J Hyg Environ Health. 2017;220(2 Pt A):64–70.
Haug LS, Sakhi AK, Cequier E, Casas M, Maitre L, Basagana X, Andrusaityte S, Chalkiadaki G, Chatzi L, Coen M, et al. In-utero and childhood chemical exposome in six European mother-child cohorts. Environ Int. 2018;121(Pt 1):751–63.
Weisskopf MG, Webster TF. Trade-offs of personal versus more proxy exposure measures in environmental epidemiology. Epidemiology. 2017;28(5):635–43.
Perrier F, Giorgis-Allemand L, Slama R, Philippat C. Within-subject pooling of biological samples to reduce exposure misclassification in biomarker-based studies. Epidemiology. 2016;27(3):378–88.
Flora G, Gupta D, Tiwari A. Toxicity of lead: a review with recent updates. Interdiscip Toxicol. 2012;5(2):47–58.
Hardy A, Benford D, Halldorsson T, Jeger MJ, Knutsen HK, More S, Naegeli H, Noteborn H, Ockleford C, Ricci A, et al. Guidance on the use of the weight of evidence approach in scientific assessments. EFSA J. 2017;15(8):e04971.
Martin P, Bladier C, Meek B, Bruyere O, Feinblatt E, Touvier M, Watier L, Makowski D. Weight of evidence for hazard identification: a critical review of the literature. Environ Health Perspect. 2018;126(7):076001.
National Research Council. Review of EPA’s Integrated Risk Information System (IRIS) process. Washington, D.C: National Academies Press; 2014.
Trasande L, Zoeller RT, Hass U, Kortenkamp A, Grandjean P, Myers JP, DiGangi J, Bellanger M, Hauser R, Legler J, et al. Estimating burden and disease costs of exposure to endocrine-disrupting chemicals in the European union. J Clin Endocrinol Metab. 2015;100(4):1245–55.
Hansen SF, von Krayer MP, Tickner JA. Categorizing mistaken false positives in regulation of human and environmental health. Risk Anal. 2007;27(1):255–69.
Grandjean P. Science for precautionary decision-making. In: Late lessons from early warnings: science, precaution, innovation. Edited by Gee D et al. Copenhagen: Published by European environment agency (EEA); 2013. p. 623–642. https://www.eea.europa.eu/publications/late-lessons-2 .
Pedeli X, Hoek G, Katsouyanni K. Risk assessment of diesel exhaust and lung cancer: combining human and animal studies after adjustment for biases in epidemiological studies. Environ Health. 2011;10:30.
Malmqvist E, Oudin A, Pascal M, Medina S. Choices behind numbers: a review of the major air pollution health impact assessments in Europe. Curr Environ Health Rep. 2018;5(1):34–43.
Heid IM, Kuchenhoff H, Miles J, Kreienbrock L, Wichmann HE. Two dimensions of measurement error: classical and Berkson error in residential radon exposure assessment. J Expo Anal Environ Epidemiol. 2004;14(5):365–77.
Lee IM, Skerrett PJ. Physical activity and all-cause mortality: what is the dose-response relation? Med Sci Sports Exerc. 2001;33(Supplement):S459–71.
Woodcock J, Franco OH, Orsini N, Roberts I. Non-vigorous physical activity and all-cause mortality: systematic review and meta-analysis of cohort studies. Int J Epidemiol. 2011;40(1):121–38.
Pope CA 3rd, Cropper M, Coggins J, Cohen A. Health benefits of air pollution abatement policy: role of the shape of the concentration-response function. J Air Waste Manag Assoc. 2015;65(5):516–22.
Vandenberg LN, Colborn T, Hayes TB, Heindel JJ, Jacobs DR Jr, Lee DH, Shioda T, Soto AM, vom Saal FS, Welshons WV, et al. Hormones and endocrine-disrupting chemicals: low-dose effects and nonmonotonic dose responses. Endocr Rev. 2012;33(3):378–455.
Burnett R, Chen H, Szyszkowicz M, Fann N, Hubbell B, Pope CA, Apte JS, Brauer M, Cohen A, Weichenthal S, et al. Global estimates of mortality associated with long-term exposure to outdoor fine particulate matter. Proc Natl Acad Sci. 2018;115(38):9592–7.
Gasparrini A, Guo Y, Hashizume M, Lavigne E, Zanobetti A, Schwartz J, Tobias A, Tong S, Rocklöv J, Forsberg B, et al. Mortality risk attributable to high and low ambient temperature: a multicountry observational study. Lancet. 2015;386(9991):369–75.
Sattelmair J, Pertman J, Ding EL, Kohl HW 3rd, Haskell W, Lee IM. Dose response between physical activity and risk of coronary heart disease: a meta-analysis. Circulation. 2011;124(7):789–95.
Slob W. Benchmark dose and the three rs. Part I. getting more information from the same number of animals. Crit Rev Toxicol. 2014;44(7):557–67.
COMEAP. The mortality effects of long-term exposure to particulate air pollution in the United Kingdom. In: COMEAP (Committee on the medical effects of air pollutants). 2010. p. 98.
Mueller N, Anderle R, Brachowicz N, Graziadei H, Lloyd SJ, de Sampaio Morais G, Sironi AP, Gibert K, Tonne C, Nieuwenhuijsen M, et al. Model choice for quantitative health impact assessment and modelling: an expert consultation and narrative literature review. Int J Health Policy Manag. 2023;12:7103.
Di Domenico L, Pullano G, Sabbatini CE, Boelle PY, Colizza V. Modelling safe protocols for reopening schools during the COVID-19 pandemic in France. Nat Commun. 2021;12(1):1073.
Pearl J. On the interpretation of do(x). J Causal Inference. 2019;7(1):20192002.
Berry G. The analysis of mortality by the subject-years method. Biometrics. 1983;39(1):173–84.
Rothman KJ, Greenland S, Lash TL. Modern epidemiology. 3rd ed. Philadelphia: Lippincott Williams & Wilkins; 2008.
Escher B, Braun G, Zarfl C. Exploring the concepts of concentration addition and independent action using a linear low-effect mixture model. Environ Toxicol Chem. 2020;39(12):2552–9.
Ezzati M, Vander Hoorn S, Rodgers A, Lopez AD, Mathers CD, Murray CJL. Estimates of global and regional potentil health gains from reducing muliple major risk factors. Lancet. 2003;362(9380):271–80.
Tamayo-Uria I, Maitre L, Thomsen C, Nieuwenhuijsen MJ, Chatzi L, Siroux V, Aasvang GM, Agier L, Andrusaityte S, Casas M, et al. The early-life exposome: description and patterns in six European countries. Environ Int. 2019;123:189–200.
VanderWeele TJ. Mediation analysis: a practitioner’s guide. Annu Rev Public Health. 2016;37(1):17–32.
Ready R, Navrud S, Day B, Dubourg R, Machado F, Mourato S, Spanninks F, Vazquez Rodriquez MX. Benefit transfer in Europe: how reliable are transfers between countries? Environ Resour Econ. 2004;29:67–82.
Chilton S, Covey J, Jones-Lee M, Loomes G, Metcalf H. Valuation of health benefits associated with reductions in air pollution. London: DEFRA; 2004.
Cropper M, Hammitt JK, Robinson LA. Valuing mortality risk reductions: progress and challenges. Annu Rev Resource Econ. 2011;3(1):313–36.
Dekker T, Brouwer R, Hofkes M, Moeltner K. The effect of risk context on the value of a statistical life: a Bayesian meta-model. Environ Resour Econ. 2011;49(4):597–624.
Aven T. Risk assessment and risk management: review of recent advances on their foundation. Eur J Oper Res. 2016;253(1):1–13.
Knol AB, Petersen AC, van der Sluijs JP, Lebret E. Dealing with uncertainties in environmental burden of disease assessment. Environ Health. 2009;8:21.
Fox MP, Lash TL. Quantitative bias analysis for study and grant planning. Ann Epidemiol. 2020;43:32–6.
Baccini M, Grisotto L, Catelan D, Consonni D, Bertazzi PA, Biggeri A. Commuting-adjusted short-term health impact assessment of airborne fine particles with uncertainty quantification via Monte Carlo simulation. Environ Health Perspect. 2015;123(1):27–33.
Burmaster DE, Anderson PD. Principles of good practice for the use of Monte Carlo techniques in human health and ecological risk assessments. Risk Anal. 1994;14(4):477–81.
Guo X, Ji J, Khan F, Ding L, Tong Q. A novel fuzzy dynamic Bayesian network for dynamic risk assessment and uncertainty propagation quantification in uncertainty environment. Saf Sci. 2021;141:105285.
Hu L, Kang R, Pan X, Zuo D. Uncertainty expression and propagation in the risk assessment of uncertain random system. IEEE Syst J. 2021;15(2):1604–15.
Cole BL, MacLeod KE, Spriggs R. Health impact assessment of transportation projects and policies: living up to aims of advancing population health and health equity? Annu Rev Public Health. 2019;40:305–18.
Harris-Roxas B, Harris E. Differing forms, differing purposes: a typology of health impact assessment. Environ Impact Assess Rev. 2011;31(4):396–403.
Hardy A, Benford D, Halldorsson T, Jeger MJ, Knutsen KH, More S, Mortensen A, Naegeli H, Noteborn H, Ockleford C, et al. Update: use of the benchmark dose approach in risk assessment. EFSA J. 2017;15(1):e04658.
Smith KR, Bruce N, Balakrishnan K, Adair-Rohani H, Balmes J, Chafe Z, Dherani M, Hosgood HD, Mehta S, Pope D, et al. Millions dead: how do we know and what does it mean? Methods used in the comparative risk assessment of household air pollution. Annu Rev Public Health. 2014;35:185–206.
Download references
The manuscript was conducted as part of HERA (Health Environment Research Agenda for Europe) project funded by Horizon Europe research and innovation programme from the DG Research of the European Commission (grant 825417).
Author information
Authors and affiliations.
Inserm, University of Grenoble Alpes, CNRS, IAB, Team of Environmental Epidemiology Applied to Reproduction and Respiratory Health, Grenoble, France
Maxime Rigaud & Rémy Slama
VITO, Flemish Institute for Technological Research, Unit Health, Mol, Belgium
Jurgen Buekers & Jos Bessems
ISGlobal, Barcelona, 08003, Spain
Xavier Basagaña & Mark Nieuwenhuijsen
Universitat Pompeu Fabra (UPF), Barcelona, 08003, Spain
CIBER Epidemiología y Salud Pública (CIBERESP), Madrid, 28029, Spain
CNRS, University Grenoble Alpes, INRAe, Grenoble INP, GAEL, Grenoble, France
Sandrine Mathy
You can also search for this author in PubMed Google Scholar
Contributions
R.S. and M.R. wrote the main manuscript text, with contributions from S.M., J. Be. and J. Bu.All authors reviewed the manuscript.
Corresponding author
Correspondence to Rémy Slama .
Ethics declarations
Competing interests.
The authors declare no competing interests.
Additional information
Publisher’s note.
Springer Nature remains neutral with regard to jurisdictional claims in published maps and institutional affiliations.
Supplementary Information
Additional file 1: figure s1..
Illustration of the structure of a health impact assessment tool allowing quantification of the number of deaths preventable through compliance with recommendations regarding physical activity, air pollution, noise, heat and access to green space [ 36 ].
Rights and permissions
Open Access This article is licensed under a Creative Commons Attribution 4.0 International License, which permits use, sharing, adaptation, distribution and reproduction in any medium or format, as long as you give appropriate credit to the original author(s) and the source, provide a link to the Creative Commons licence, and indicate if changes were made. The images or other third party material in this article are included in the article's Creative Commons licence, unless indicated otherwise in a credit line to the material. If material is not included in the article's Creative Commons licence and your intended use is not permitted by statutory regulation or exceeds the permitted use, you will need to obtain permission directly from the copyright holder. To view a copy of this licence, visit http://creativecommons.org/licenses/by/4.0/ . The Creative Commons Public Domain Dedication waiver ( http://creativecommons.org/publicdomain/zero/1.0/ ) applies to the data made available in this article, unless otherwise stated in a credit line to the data.
Reprints and permissions
About this article
Cite this article.
Rigaud, M., Buekers, J., Bessems, J. et al. The methodology of quantitative risk assessment studies. Environ Health 23 , 13 (2024). https://doi.org/10.1186/s12940-023-01039-x
Download citation
Received : 23 June 2023
Accepted : 05 December 2023
Published : 27 January 2024
DOI : https://doi.org/10.1186/s12940-023-01039-x
Share this article
Anyone you share the following link with will be able to read this content:
Sorry, a shareable link is not currently available for this article.
Provided by the Springer Nature SharedIt content-sharing initiative
- Dose–response
- Environment
Environmental Health
ISSN: 1476-069X
- General enquiries: [email protected]
Understanding Potential Risks for Human Subjects Research
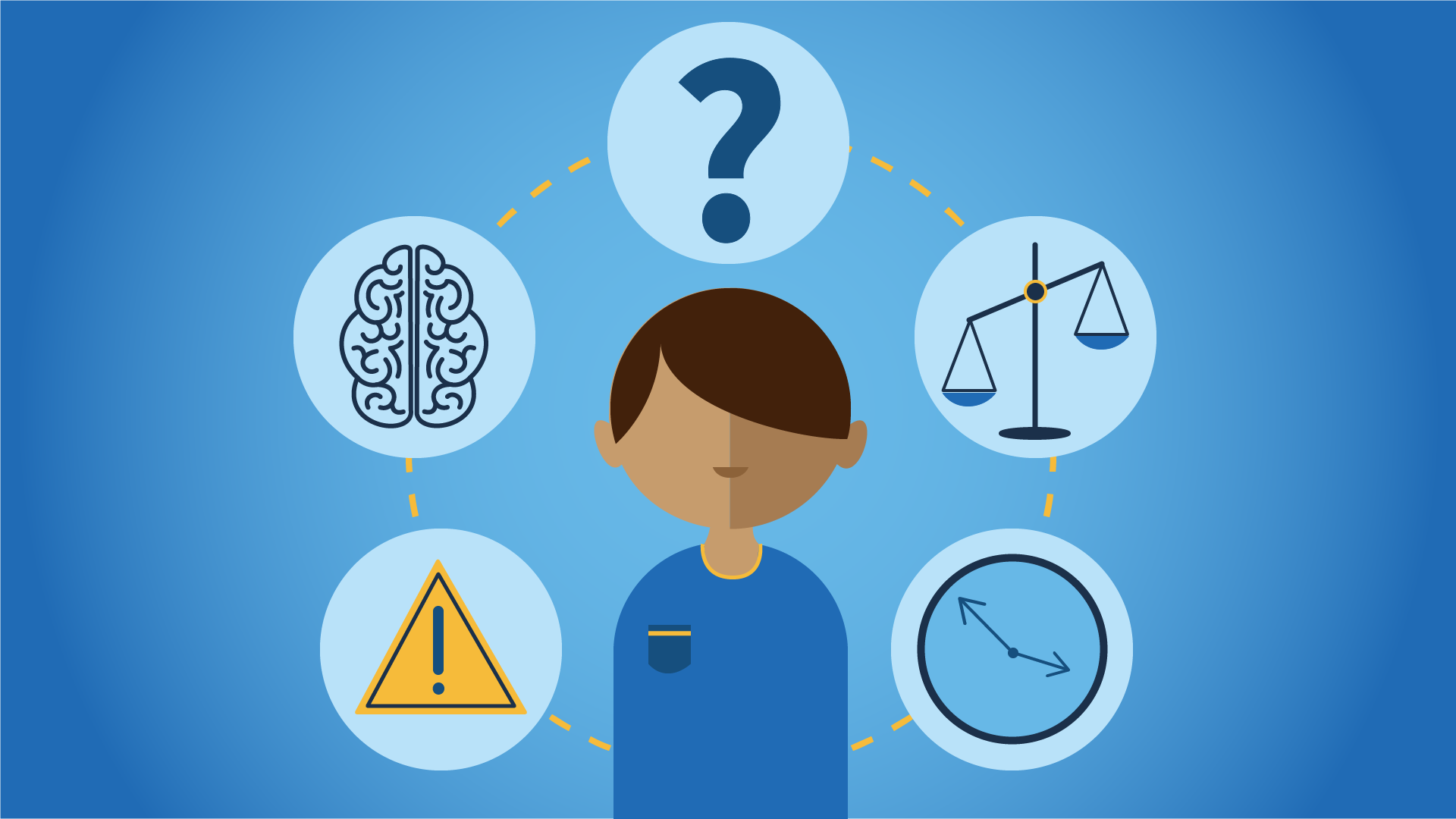
As part of their ethical duty to participants, all researchers are required to properly identify, minimize, and disclose any potential harm or discomforts that may come to participants. These harms and discomforts are labeled as “ risks, ” and should be minimized, according to the beneficence principle of the Belmont Report . Any risks that may potentially befall participants must be disclosed in the informed consent , and participants should be given the choice of whether or not to participate in research.
Additional risks may also be encountered in light of a catastrophic event (e.g., a natural disaster, epidemic, terrorist attack, pandemic, etc.). During these times, researchers with active protocols must review their study activities to identify and mitigate any new risk. For example, prior to the COVID-19 pandemic and depending on the topic, TC IRB typically considered in-person surveys with adults competent to consent as low risk. Under COVID-19 circumstances, however, studies with in-person components are now reviewed as higher risk, as person-to-person exposure is the most frequent route of transmission for infectious viruses. The IRB is required to assess the level of risk involved in a research study when making a determination for review requirements. Some items factoring into the IRB’s risk assessment include possible risk to a subject’s psychological wellbeing, or the risk to a subject if their confidentiality is compromised. The more risk involved in a study, the higher the level of review and the more human subject protections required by the IRB.
Examples of Potential Risks & Suggestions for Mitigation
Reviewing potential risks to participants requires a knowledge of various types of harm or discomfort that participants may encounter. The Teachers College research community is largely composed of behavioral and social researchers. The following examples and suggestions for minimizing risks are likely to be encountered in behavioral science research, though they may also extend to biomedical and other types of research as well.
Loss of Time : Researchers are required to include the estimated time of each study activity and the total time of completion on the informed consent (parent permission or assent form). Researchers should:
- Estimate study completion time based on pilot tests.
- Consider the reading level of participants (e.g., 3rd grade readers vs. post-secondary students) when calculating the time necessary to complete the study.
- Reasonably overestimate times to account for participants that may take longer.
- Prepare for potential time delays when using online software (e.g., Zoom ) or introducing new activities.
Traumatic Events: Recalling traumatic or distressing events can be uncomfortable, and in some cases, harmful to an individual. Traumatic events are defined as events that are shocking, scary, or dangerous (e.g., natural disasters, acts of violence, accidents, etc.). While it is not always possible to identify what types of questions may trigger participants, researchers should identify and disclose questions or activities pertaining to traumatic events. Researchers should:
- Disclose any topics, study activities, or questions that may be triggering in the informed consent. For example, if the study includes questions about a traumatic event (e.g., 9/11), provide a trigger warning such as “The next section will include questions that may be uncomfortable to you. This study is voluntary. You can choose to stop the study at any time or skip any question.”
- Monitor the participant during each study activity and after, if possible. While the participant may appear fine during a study, recalling traumatic events may lead to flashbacks, insomnia, trouble concentrating, or higher levels of anxiety, sadness, or anger for a prolonged period of time.
- Provide participants with a list of community resources, or offer free counseling services (if appropriate), should the need arise.
- Consider options such as recruiting a trained professional to be available to help debrief the participants if they start to experience symptoms of distress.
Unwanted Stimuli: Exposure to unwanted or distressing stimuli during a study’s activities may bring harm and discomfort. Participants should not be exposed to distressing stimuli (e.g., pornography, smoking, suicide) without first providing their clear consent. Researchers should:
- Know the risks associated with exposing participants to unwanted stimuli (e.g., increased sadness or irritability), and implement additional safeguards throughout their study, such as debriefing participants after the study activities are finished.
- Refrain from exposing high-risk groups to unwanted stimuli. For example, study activities including heavy drinking or intoxication should screen out recovering substance users.
Labeling: Participants unfamiliar with clinical terminology may begin to identify with disorders or personalities outlined in study measures. For example, someone taking a scale labeled “Depression Scale” may begin to identify as having depression without receiving a clinical diagnosis. Researchers should:
- Reduce participant confusion by appropriately and clearly naming scales or removing measure names altogether.
- Examine tiles of studies for potential bias and risk to participants.
Environmental Stimuli: Environmental stimuli, such as the research location, building layout, lighting, or external noise, is not always considered in the research design. However, some stimuli may be triggering for participants, and should be eliminated, if possible. Researchers should:
- Examine their research space for any environmental allergens or health issues, such as dust and flickering lights, prior to inviting participants.
- Remain mindful of possible food allergies (e.g., nuts, shellfish, gluten) when serving snacks or refreshments.
- Ensure that the space is safe, secure, hygienic , and, if applicable, private.
- Review the CDC's detailed instructions on how to clean and disinfect workspaces, along with their list of EPA-approved disinfectants .
Minor Emotional Risk: Typical common, but minor, risks include mental fatigue, embarrassment, discomfort, or frustration. Researchers should:
- Review their study activities from the perspective of the participant in order to determine if these emotions might be encountered. For example, a 5-minute survey on personal food preferences is unlikely to cause frustration. However, a study activity in which participants must solve complex mathematical equations in front of peers may cause embarrassment or frustration.
- Always disclose any anticipated distressing emotions in the consent form.
Participant Relationships: Putting strain on participants’ relationships (e.g., causing a fight between a dating couple) will likely cause harm or discomfort to a participant. Researchers should:
- Disclose study activities in the consent form that may cause relationship distress.
- Distinguish between study activities that may cause relationship distress (e.g., this study will examine household spending habits between partners) from unintentional relationship distress perpetuated in error by the researcher. In the latter’s case, the researcher should gauge the situation and take steps to ensure respect, participant autonomy, and care.
Exercise & Repetitive Movements : Studies involving exercise or movement have an inherent risk of physical injury to the participants. Researchers should:
- Disclose all activities at the beginning of the study that may cause physical discomfort.
- Evaluate or discuss the participants’ physical health and ability based on the study activity (e.g., able to lift 20 pounds without assistance).
- Gauge the health of the participant to engage in the activities. For example, does the participant have any underlying health conditions that might impact their ability to participate? (e.g., a study requiring intense cardio exertion might want to screen for asthmatic participants).
- Disclose health risks on the consent form.
Personal Information: A sking questions about private information such as income, health habits, illegal substance use, etc. may be distressing for participants. Inclusion of these types of questions should be clearly justified to the IRB. Researchers should:
- Clarify confidentiality policies.
- Disclose what identifiers may be disclosed, or are at risk of disclosure (e.g., during focus group sessions, researchers cannot guarantee confidentiality).
- Review Certificates of Confidentiality (CoC) for human subjects, if applicable.
Viral Illnesses, Spreads, or Pandemics: With the COVID-19 pandemic, person-to-person contact is the most frequent route of viral transmission. Researchers with in-person components in their study must evaluate study activities for points of contact. Exposure is at its highest when people are within approximately 6 feet of one another, and in close contact for 15 minutes or more. Shared spaces, equipment, and surfaces (e.g., office keyboards, writing utensils, etc.) can also be contaminated. To reduce the risk of infection, researchers should:
- Wash hands with soap and water for 20 seconds. If soap and water are not available, use a hand sanitizer that contains at least 60% alcohol.
- Avoid touching one’s face.
- Use face coverings when around other people.
- Cover coughs and sneezes.
- Throw used tissues in the trash.
- Practice social distancing, if possible.
- Clean and disinfect high-touch surfaces daily. This may include tables, doorknobs, light switches, countertops, handles, desks, phones, keyboards, toilets, faucets, and sinks.
- Restrict in-person interactions to ventilated areas, or outside when possible.
- Remain vigilant about taking the steps that reduce exposure.
Requirements for Mitigating Research Risks
When assessing risk and benefits in a study, the IRB can support researchers in determining if a risk is justifiable or should be removed. Generally, risks to participants must be minimized, and any risks in the study should be reasonable when compared to the benefits of the study.
The IRB also requires that each individual participant provides their informed consent. All consent must be documented and available for review if requested. If a participant is unable to provide their consent (e.g., a minor), their guardian must provide consent, and the participant’s assent should be sought. If a participant is likely to be vulnerable to coercion (e.g., prisoners, cognitively impaired persons, etc.), additional safeguards must be outlined in the IRB application. Additionally, researchers must justify the rationale for conducting their study with vulnerable populations as opposed to general populations (e.g., the study provides an intervention which fills a need for that specific population). For sample consent and application language, please visit our TC IRB Submission Document Templates & Samples guide.
In the informed consent, the privacy and confidentiality of the participants must be appropriately documented. TC IRB recommends that all data be de-identified prior to the start of analysis. Finally, the research plan must make provisions for the secure collection and monitoring of data (visit our Data Sharing, Requests, & Encryption for more information). TC IRB has worked closely with TC Information Technologies (IT) to support researchers working remotely with limited resources and technological capabilities. TC IT should be consulted as the first point of contact if data cannot be secured to the standards outlined in the data security plan.
For COVID-19 related information, risks, and preparation please visit here.
Institutional Review Board
Address: Russell Hall, Room 13
* Phone: 212-678-4105 * Email: [email protected]
Appointments are available by request . Make sure to have your IRB protocol number (e.g., 19-011) available. If you are unable to access any of the downloadable resources, please contact OASID via email [email protected] .
Thank you for visiting nature.com. You are using a browser version with limited support for CSS. To obtain the best experience, we recommend you use a more up to date browser (or turn off compatibility mode in Internet Explorer). In the meantime, to ensure continued support, we are displaying the site without styles and JavaScript.
- View all journals
- My Account Login
- Explore content
- About the journal
- Publish with us
- Sign up for alerts
- Open access
- Published: 22 March 2024
Determination and risk assessment of aflatoxin B1 in the kernel of imported raw hazelnuts from Eastern Azerbaijan Province of Iran
- Parnian Samimi 1 ,
- Ramin Aslani 1 ,
- Ebrahim Molaee-Aghaee 1 ,
- Parisa Sadighara 1 ,
- Nabi Shariatifar 1 ,
- Gholamreza Jahed Khaniki 1 ,
- Sibel Ozcakmak 2 &
- Zahra Reshadat 3
Scientific Reports volume 14 , Article number: 6864 ( 2024 ) Cite this article
286 Accesses
1 Altmetric
Metrics details
- Environmental sciences
- Microbiology
Aflatoxin B1 (AFB1) is widespread and seriously threatens public health worldwide. This study aimed to investigate AFB1 in imported hazelnut samples in northwest of Iran (Eastern Azerbaijan Province) using High-Performance Liquid Chromatography with a Fluorescent Detector (HPLC-FLD). In all tested samples AFB1 was detected. The mean concentration of AFB1 was 4.20 μg/kg and ranged from 3.145 to 8.13 μg/kg. All samples contained AFB1 levels within the maximum acceptable limit except for one sample. Furthermore, the human health risk assessment of AFB1 from consuming imported hazelnuts by Iranian children and adults was evaluated based on the margin of exposure (MoE) and quantitative liver cancer risk approaches. The MoE mean for children was 2529.76, while for adults, it was 8854.16, indicating a public health concern. The present study found that the risk of developing liver cancer among Iranian children was 0.11100736 per 100,000 people, and in the Iranian adult population was 0.0314496 cancers per 100,000 people. Since environmental conditions potentially affect aflatoxin levels in nuts, countries are advised to monitor aflatoxin contents in imported nuts, especially from countries with a conducive climate for mold growth.
Similar content being viewed by others
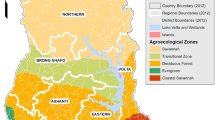
The occurrence of aflatoxins and human health risk estimations in randomly obtained maize from some markets in Ghana
Nii Korley Kortei, Theophilus Annan, … Paul Esua-Amoafo
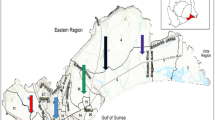
Aflatoxin M1 exposure in a fermented millet-based milk beverage ‘brukina’ and its cancer risk characterization in Greater Accra, Ghana
Nii Korley Kortei, Theophilus Annan, … Vincent Kyei-Baffour
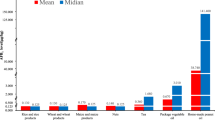
Probabilistic risk assessment of dietary exposure to aflatoxin B1 in Guangzhou, China
Weiwei Zhang, Yufei Liu, … Kuncai Chen
Introduction
Hazelnut ( Corylus avellana ) is a hard-peeled fruit that grows predominantly in the Mediterranean basin (temperate climates with high humidity and rainfall) and is internationally traded. It is estimated that approximately 1,200,000 tons of hazelnuts are produced annually worldwide, and the majority are produced in Turkey, Italy, Azerbaijan, the United States, Spain, Greece, Georgia, England, and Iran 1 , 2 . Hazelnuts are a rich source of squalane, protein, carbohydrates, vitamins, minerals, and unsaturated fatty acids. It has been established that hazelnut oil reduces blood cholesterol levels and hypertension side effects. In addition, 100 g of hazelnuts provide 600–650 cal 3 , 4 . Since hazelnuts contain various nutrients, they are susceptible to decay and mold growth when harvested, dried, and stored at high temperatures and humidity 4 , 5 .
The prevalence of molds is one of the main challenges in the nut industry. Besides causing spoilage of crops, molds also produce mycotoxin. Mycotoxins are secondary metabolites of mold and are classified as food contaminants 6 , 7 . Aflatoxins (AFs) are the most prevalent type of mycotoxin in hazelnuts, peanuts, pistachio nuts, almonds, Brazil nuts, and walnuts 8 , 9 . These metabolites pose significant risks to human and animal health and agricultural output, leading to substantial economic losses 10 , 11 . AFs are among the most hazardous and toxic mycotoxins, primarily produced by Aspergillus species, notably A. flavus and A. parasiticus . Aspergillus fungi are capable of releasing diverse enzymes, which allows them to thrive under a variety of environmental conditions. AFs are stable metabolites and remarkably resistant to different processes 9 , 12 , 13 .
Since 1960, more than 20 AFs have been identified, and based on lethal dose values (LD 50 ), aflatoxin B1 (AFB1) is the most toxic aflatoxin 14 . AFB1 is a genotoxic carcinogen linked to various diseases and complications, including acute hepatitis, liver cancer, immune and reproductive system disorders, and blood-forming stem cell dysfunction 3 , 15 .
Currently, numerous analytical techniques are available 16 , and previous researchers have utilized different methods to determine aflatoxin contents in foods, such as High-performance liquid chromatography (HPLC) 9 , 12 , Liquid Chromatography Coupled with Mass Spectrometry (LC–MS) 17 , Multidimensional Liquid Chromatography (2D-LC) 18 , Gas Chromatography (GC) 19 , Supercritical Fluid Chromatography (SFC) 20 , Enzyme-Linked Immunosorbent Assay–ELISA 21 , and UV–vis spectroscopic 22 , 23 .
Iran has an annual production of more than 50 thousand tons of hazelnuts and is among the top ten hazelnut producing countries. In recent years, the production of hazelnuts in Iran has decreased due to various reasons, including climate change, which has led to the annual import of a significant amount of this food item. Considering the high consumption of hazelnut kernels in Iran and the fact that no comprehensive study has been conducted on the aflatoxin concentration in imported hazelnuts in Iran. Therefore, the present study was carried out in order to determine the contents and assess the health risks of aflatoxin B1 in raw hazelnut kernels imported from Iran.
Materials and methods
Sample collection.
In total, 60 samples (considering double sampling, each 500 g) of imported raw hazelnut kernels without shell were collected from Eastern Azerbaijan Province market, Iran during 2022. All samples were kept in the glass container with the condition of sterility in refrigerator (4 °C) until analyses at the same day.
All chemicals were of analytical grade and were purchased from Merck Co., (Darmstadt, Germany).
Sample preparation and analysis
Immunoaffinity columns (IAC) were ordered from Neogen (Lansing, MI, USA). The AOAC Official Method 991.31 was employed to determine AFB1 hazelnut samples. Initially, 50 g of the hazelnut sample was ground with a blender, mixed with 5 g of NaCl from Merck (Darmstadt, Germany), and then placed into a suitable container. A digital scale with a sensitivity of 0.1 g was employed to weigh the samples. The sample containing NaCl was transferred to a mixing tank, and 50 mL of extracting solvent (MeOH from Merck (Darmstadt, Germany) and water in an 8:2 v/v ratio) was added to each sample and blended for 1 min. Then, the obtained solution was filtered via filter paper (Whatman No. 1). Afterward, 5 ml of the filtered solution was diluted with 35 ml of grade 3 water. The diluted solution was filtered again with microfiber filter paper, and 20 ml was applied to an HPLC immunoaffinity column. The immunoaffinity column was prepared utilizing antibodies of aflatoxins B and maintained at 25 °C until connecting to the tank. In the following step, 3 ml of PBS buffer (phosphate buffer saline) was dripped into the tank, and the solution flowed through the prepared immunoaffinity column at a rate of 2–3 ml per minute. The immunoaffinity column was washed with 20 ml (2 × 10 ml) of deionized water and dried with positive air pressure. The output mixture was collected in clean vials (HPLC-specific vials) after 375 L of methanol from Merck (Darmstadt, Germany) was passed through the column. The contents of the vials were diluted with 375 ml of deionized water, which was then stirred using a vortex (vacuum pump). The standard curve and calibration were prepared by injecting specific amounts of standard aflatoxins into the column before injection of the samples. The percent recovery of AFB1 was determined by injecting 200 μl of standard samples of AFB1 with a concentration of 5 ml into the HPLC (Alliance e2695, American Waters Company, fluorescence detector (FLR2475)) 9 , 12 , 24 .
Health risk assessment
Table 1 describes the details of the parameters used to compute the risk assessment caused by exposure to AFB1 from hazelnut consumption.
Estimation of daily intake
The estimated daily intake (EDI) of AFB1 was obtained based on the mean, minimum, and maximum concentrations of AFB1 in hazelnut samples for Iranian children and adults. The following equation was employed to compute the estimated daily intake (EDI) 25 , 26 , 27 , 28 :
where C is the concentration of AFB1, IR is the ingestion rate (0.32 g/day), and BW is the average body weight (20 kg for children and 70 kg for adults).
Risk characterization
Risk assessment was carried out with margin of exposure (MoE) approach recommended by EFSA 29 and quantitative liver cancer risk approach recommended by FAO and WHO 30 .
MoE is the proportion of a toxicological reference point to a dose that leads to a low but measurable response. BMDL10 is the lowest dose that is 95% assured to cause no more than 10% cancer incidence in rodents. MoE values lower than 10,000 are considered a concern from a public health viewpoint. The following equations were used to calculate the margin of exposure (MoE) 31 , 32 :
The quantitative liver cancer risk approach was used to evaluate the liver cancer risk induced by exposure to AFB1. The probability of developing liver cancer resulting from exposure to AFB1 is synergistically augmented by hepatitis B. This risk assessment considers both the carcinogenic potency for individuals infected with hepatitis B and those not infected with hepatitis B. For hepatitis B surface antigen-positive individuals (HBsAg + ) and hepatitis B surface antigen-negative individuals (HBsAg − ), the carcinogenic potency of AFB1 is 0.3 and 0.01 cancers/year/10 5 individuals per ng/kg bw/day. The prevalence of HBsAg + in Iran is 2.2% 33 . The cancer risk of AFB1 was esteemed using the following Eqs. 31 , 34 , 35 :
Hazelnuts were spiked with AFB1 to determine analytical accuracy and performance. The limit of detection (LOD) and limit of quantitation (LOQ) were 0.004 and 0.012 µg/kg, respectively. Also, the correlation coefficient (R 2 ) of the dependent matrix calibration plots was 0.998%, and the Y-line equation was y = 3E + 07x-1848. The recovery rate of AFB1 was 113.1%.
Figure 1 illustrates the levels of aflatoxin B1 measured in 30 hazelnut samples collected from the Eastern Azerbaijan market, Iran. AFB1 was found in all samples at different amounts. The concentrations of AFB1 in the analyzed hazelnut samples ranged from 3.145 to 8.13 μg/kg, and the Mean ± SD concentration was 4.20 ± 0.15 μg/kg. AFB1 levels found in the analyzed samples were lower than the maximum permissible tolerance (8 μg/kg) recommended by Iran's National Standard (INSO No. 53925, Food and feed- Maximum tolerated level of mycotoxins), except in one sample (sample 9). The European Commission 36 recommends a maximum acceptable level of AFB1 contamination in foods between 2 and 12 μg/kg, whereas the United States Food and Drug Administration (FDA) has established a limit of 20 μg/kg 9 , 37 .
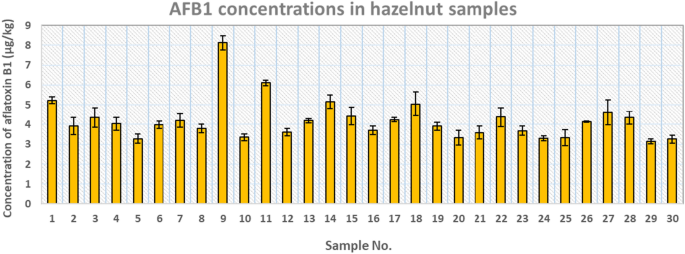
The concentration of aflatoxin B1 in 30 samples of hazelnut kernel.
As shown in Table 2 , the AFB1 concentration found in the current investigation was compared with those reported in the previous literature. Saffari et al. reported AFB1 levels in 100 samples of hazelnuts within Iran's acceptable standard, and the concentration of AFB1 was significantly lower than in the current results 38 . In the study by Gürses, the mean concentration of AFB1 in the hazelnut sample was 34.4 µg/kg and ranged from 1 to 113 µg/kg, which was higher than the present results 39 . In Turkey, Bircan et al. analyzed 2784 samples of dried Figs. (2643), pistachios (28), hazelnuts (80), peanuts (10), and paprikas (23). They reported that 57% of pistachio, 2.5% of hazelnut, 50% of peanut,11.8% of dried figs, and 83% of paprika contained total aflatoxin in the range of 2.31–63.11 µg/kg, 5.46–6.55 µg/kg, 0.75–26.36 µg/kg, 0.2–162.76 µg/kg, and 0.38–14.71 µg/kg, respectively. Total AF levels in all pistachio and hazelnut samples were higher than the regulatory limits of the EU (5 µg/kg for AFB1 and 10 µg/kg for total AFs). Moreover, hazelnut samples contained less aflatoxin than the present study 40 . Kabak measured the aflatoxins concentration in 300 samples of hazelnuts and dried figs using the HPLC-FLD method. The study found that 6 raw hazelnut kernel samples and 5 roasted hazelnut kernel samples were positive for total aflatoxin. AFs concentration ranged from 0.09 to 11.3 µg/kg in raw hazelnut kernel and from 0.17 to 11.2 µg/kg in roasted hazelnut kernel. Also, AFs level in 3 hazelnut samples exceeded the European maximum limits 41 .
The hazelnut hard shell is a good obstacle against fungal contamination. However, climatic and storage conditions can cause aflatoxin formation 47 . Acute exposure to aflatoxin may trigger abdominal pain, nausea, vomiting, and seizures, while chronic exposure can lead to hepatotoxicity, teratogenicity, and immunotoxicity. In developing countries, aflatoxin is one of the leading causes of hepatocellular carcinoma 48 .
Hazelnuts produced in different countries may contain varying amounts of AFs because the growth of Aspergillus species and the production of aflatoxin predominantly depend on environmental conditions. As reported in the study by Prelle et al. 66.7% of Turkish hazelnuts (mean contamination 0.33 mg/kg) and 35.9% of Italian hazelnuts (mean contamination 0.14 mg/kg) were contaminated with aflatoxin 17 . Furthermore, Ebrahimi et al. after conducting a global systematic review of aflatoxins contents in various nut samples, concluded that the average amounts of AFB1 in hazelnut samples from different countries were as follows: Italy (28.15 µg/kg) > Turkey (2.97 µg/kg) > Spain (0.51 µg/kg) 49 .
AF levels in hazelnuts can be affected by growing, harvesting, drying, and storing conditions 5 . Ozay et al. in a three-year study on factors influencing fungal and aflatoxin levels in Turkish hazelnuts during growth, harvest, drying, and storage, concluded that manual harvesting of ripe hazelnuts or harvesting hazelnuts into cloth by shaking the trees, utilizing jute rather than of nylon sacks, and mechanical drying technique would mitigate aflatoxin content in hazelnuts 36 . In regions that produce nuts traditionally, nuts are commonly dried out under the sunlight, thus becoming more exposed to environmental factors. In these regions, mechanical drying techniques can effectively contribute to preventing mold growth and AF contamination.
It has been determined that AFs concentration increases with the long-term storage of nuts. Also, damaged nuts are more susceptible to aflatoxin contamination 50 . For instance, the study by Bensassi et al. indicated that after 2 years of pistachio nuts storage in an extremely dry and aerated place, AFs contamination obviously occurred. Also, after 4 years of storage, the mean AFB1 concentration ranged from 2.7 to 12.7 µg/kg, beyond the maximum allowable limit. Hence, nuts should not be stored for long periods of time 51 . Furthermore, Gürses et al. found that controlling humidity and reducing the storage period minimizes aflatoxin formation in hazelnuts 50 .
The use of UV radiation, infrared ray roasting, cold plasmas, hot air, and citric acid are some of the most effective methods for mitigating AFs levels 3 , 52 , 53 . Furthermore, manual and mechanical separating, roasting, and membrane peeling can diminish AFs levels in hazelnuts. It seems that the majority of aflatoxin is concentrated in the membrane of the hazelnut (especially the inner membrane), and when membranes are peeled, 98% of the aflatoxin contaminant is removed 54 . The Özer study indicated AFB1 concentration in raw hazelnuts was 11.28 µg/kg, and after roasting, it decreased to 11.11 µg/kg. This amount was reduced to 0.23 µg/kg after peeling the membrane 55 . In contrast, Amiri et al. found no difference between raw and roasted hazelnuts regarding AFB1 amounts. In other words, if there is AF contamination, it is not reasonable to expect the unacceptable and higher levels of AFs to be reduced by processing and become acceptable. Quality assurance systems for nut industries are crucial to minimizing the growth of toxigenic mold and, thereby, the occurrence of mycotoxins, especially supplier qualifications 56 .
A risk assessment was conducted to evaluate the health effects of AFB1. Estimated daily intake (EDI) and margin of exposure (MoE) through hazelnut consumption by children and adults are presented in Table 3 . The EDI means of AFB1 was 0.0504 ng/kg bw/day (range 0.0672–0.13008 ng/kg bw/day) for children and 0.0192 ng/kg bw/day (range 0.0144–0.0371 ng/kg bw/day) for adults.
The MoE mean was 2529.76 for children and 8854.16 for adults. These results demonstrate a public health concern for Iranian children and adults associated with AFB1 exposure through imported hazelnut consumption. Similarly to the results of this study, the MoE values reported by Taghizadeh et al 45 . Blanco-Lizarazo et al 57 . and Leong et al 58 . were less than 10,000, which provides evidence of a public health concern. In contrast, MoE values reported by Renwick et al. were not more than 10,000 59 .
Figure 2 illustrates the quantitative liver cancer risk incidence caused by AFB1 exposure based on imported hazelnut consumption. The risk of liver cancer in the Iranian children population was estimated at 0.1100736 cancers/year/ per 10 5 individuals, and in the Iranian adult, it was 0.0314496 cancers/year/ per 10 5 individuals. Results indicate that children have a significantly higher risk of liver cancer than adults.
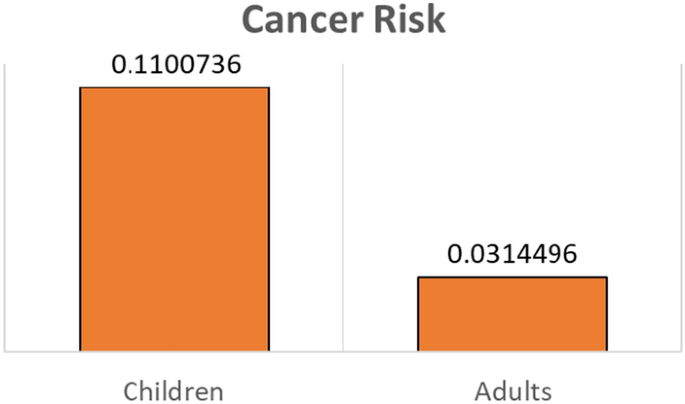
Liver cancer risk (cancers/year/ per 10 5 individuals) through hazelnut consumption by Iranian children and adults.
Andrade et al. reported cancer risk for consumers with a high consumption level, and the entire population was 0.3056 and 0.0753 cancers/year/ per 10 5 individuals, respectively 60 . Another investigation by Taghizadeh et al. revealed that risk of cancer in individuals who are positive for hepatitis B and those who are not positive for hepatitis B were 0.0001 and 0.0000034 cancers/year/ per 10 5 individuals, respectively 45 . Undoubtedly, AFB1 can cause a severe public health threat, and the prevention and management of this contaminant should be a top priority.
The current study focused on a major contaminant AFB1 in a main nut with a relatively high consumption in food industry and also by people followed by a risk assessment. However, due to limitations in comparison with some studies, a combined samples of imported and domestic cultivated hazelnuts and collected from different provinces could reveal a more comprehensive state for AFB1 condition.
The present investigation assessed AFB1 in imported hazelnuts into Iran. Except for one sample, all samples contained lower levels of AFB1 than the maximum permissible limit. The results obtained from margin of exposure risk assessment indicated a public health concern (MOE < 10,000 value). Due to the inherent properties of aflatoxins regarding their resistance to high temperatures, their complete removal from food matrices has faced significant challenges. It is recommended that aflatoxin levels in nuts are monitored constantly and accurately by appropriate government agencies.
Data availability
All data generated or analysed during this study are included in this published article.
Bacchetta, L., Aramini, M., Bernardini, C. & Rugini, E. In vitro propagation of traditional Italian hazelnut cultivars as a tool for the valorization and conservation of local genetic resources. HortScience 43 , 562–566 (2008).
Article Google Scholar
FAO. FAO statistical databases and data sets. Available from: http://faostat.fao.org (2012).
Sen, Y., Onal-Ulusoy, B. & Mutlu, M. Detoxification of hazelnuts by different cold plasmas and gamma irradiation treatments. Innov. Food Sci. Emerg. Technol. 54 , 252–259 (2019).
Article CAS Google Scholar
Valente, S. et al. Effect of drying temperatures and exposure times on Aspergillus flavus growth and aflatoxin production on artificially inoculated hazelnuts. J. Food Prot. 83 , 1241–1247 (2020).
Article CAS PubMed Google Scholar
Şengül, Ü. Comparing determination methods of detection and quantification limits for aflatoxin analysis in hazelnut. J. Food Drug Anal. 24 , 56–62 (2016).
Article PubMed Google Scholar
WHO. Mycotoxins. available; www.who.int/news-room/fact-sheets
van Egmond, H. P., Schothorst, R. C. & Jonker, M. A. Regulations relating to mycotoxins in food. Anal. Bioanal. Chem. 389 , 147–157 (2007).
Gelderblom, W. et al . in Food Safety Management 301–324 (Elsevier, 2014).
Bagheri Marzooni, B., Jahed Khaniki, G., Shariatifar, N. & Molaee-Aghaee, E. Assessment of aflatoxins in some pistachio cultivars supplied in bulk in Tehran food stores. Int. J. Environ. Anal. Chem. 17 , 1 (2022).
Zain, M. E. Impact of mycotoxins on humans and animals. J. Saudi Chem. Soc. 15 , 129–144 (2011).
Hassan, H. F. et al. Assessment of mycotoxins in cornflakes marketed in Lebanon. Sci. Rep. 13 , 20944. https://doi.org/10.1038/s41598-023-48172-8 (2023).
Article ADS CAS PubMed PubMed Central Google Scholar
Ahmadi, M., Jahed Khaniki, G., Shariatifar, N. & Molaee-Aghaee, E. Investigation of aflatoxins level in some packaged and bulk legumes collected from Tehran market of Iran. Int. J. Environ. Anal. Chem. 102 , 4804–4813. https://doi.org/10.1080/03067319.2020.1789614 (2022).
Ghanbari, R., et al. Biocontrol effect of Kluyveromyces lactis on aflatoxin expression and production in Aspergillus parasiticus . FEMS Microbiol. Letter. 366 , fnz114 (2019).
Kortei, N. K. et al. Exposure and risk characterizations of ochratoxins a and aflatoxins through maize (Zea mays) consumed in different agro-ecological zones of Ghana. Sci. Rep. 11 , 23339. https://doi.org/10.1038/s41598-021-02822-x (2021).
Larou, E. et al. High throughput cellular biosensor for the ultra-sensitive, ultra-rapid detection of aflatoxin M1. Food Control 29 , 208–212 (2013).
Schincaglia, A. et al. Current developments of analytical methodologies for aflatoxins’ determination in food during the last decade (2013–2022), with a particular focus on nuts and nut products. Foods. 12 (3), 527 (2023).
Article CAS PubMed PubMed Central Google Scholar
Prelle, A., Spadaro, D., Garibaldi, A. & Gullino, M. L. Aflatoxin monitoring in Italian hazelnut products by LC-MS. Food Addit. Contam. Part B 5 , 279–285 (2012).
Campone, L. et al. Determination of mycotoxins in beer by multi heart-cutting two-dimensional liquid chromatography tandem mass spectrometry method. Food Chem. 318 , 126496. https://doi.org/10.1016/j.foodchem.2020.126496 (2020).
Wacoo, A. P., Wendiro, D., Vuzi, P. C. & Hawumba, J. F. Methods for detection of aflatoxins in agricultural food crops. J. Appl. Chem. 2014 , 706291. https://doi.org/10.1155/2014/706291 (2014).
Engelhardt, H. & Haas, P. Possibilities and limitations of SFE in the extraction of aflatoxin B1 from food matrices. J. Chromatogr. Sci. 31 , 13–19 (1993).
Beyene, A. M., Du, X., Schrunk, E. D., Ensley, S. & Rumbeiha, W. K. High-performance liquid chromatography and enzyme-linked immunosorbent assay techniques for detection and quantification of aflatoxin B1 in feed samples: a comparative study. BMC Res. Notes 12 , 1–6 (2019).
Teichmann, B. & Krug, G. Spectrophotometric investigations on aflatoxin B1 and aflatoxin G1 (author’s transl). Arch. Geschwulstforsch 45 , 25–33 (1975).
CAS PubMed Google Scholar
Hojjati-Najafabadi, A. et al. A tramadol drug electrochemical sensor amplified by biosynthesized au nanoparticle using mentha aquatic extract and ionic liquid. Top. Catal. 65 , 587–594. https://doi.org/10.1007/s11244-021-01498-x (2022).
Truckness, M. W. AOAC Official Method 991.31, Aflatoxin in corn, raw peanuts, and peanut butter. J. AOAC Int. 49 , 22–24 (2000).
Google Scholar
Esmi, F. et al. Ochratoxin A in chamomile, black and green tea and human health risk assessment in Iranian population. J. Food Measur. Charact. 16 , 5066–5076 (2022).
Naghshbandi, B., Omrani, M. A., Jafari-Sales, A., Jalil, A. T. & Naghsh, N. Human health risk assessment and exposure evaluation by monte-carlo simulation method for aflatoxin M1 in widely consumed infant dried powder milk in Iran. J. Food Protect. 86 , 100047–100047 (2023).
Kortei, N. K. et al. The occurrence of aflatoxins and human health risk estimations in randomly obtained maize from some markets in Ghana. Sci. Rep. 11 , 4295. https://doi.org/10.1038/s41598-021-83751-7 (2021).
Khanniri, E. et al. Determination of heavy metals in municipal water network of Tehran, Iran: A health risk assessment with a focus on carcinogenicity. Int. J. Cancer Manag. https://doi.org/10.5812/ijcm-137240 (2023).
European Food Safety Authority (EFSA). Opinion of the Scientific Committee on a request from EFSA related to a harmonised approach for risk assessment of substances which are both genotoxic and carcinogenic. EFSA J. 3 (10), 282 (2005).
Joint, F., Additives, W. E. C. O. F. & Organization, W. H. in Safety evaluation of certain food additives and contaminants/prepared by the forty-ninth meeting of the Joint FAO/WHO Expert Committee on Food Additives (JEFCA).
Nugraha, A., Khotimah, K. & Rietjens, I. M. Risk assessment of aflatoxin B1 exposure from maize and peanut consumption in Indonesia using the margin of exposure and liver cancer risk estimation approaches. Food Chem. Toxicol. 113 , 134–144 (2018).
de Matos, C. J. et al. Aflatoxin M1 in Brazilian goat milk and health risk assessment. J. Environ. Sci. Health Part B 56 , 415–422 (2021).
Salehi-Vaziri M, Sadeghi F, Hashiani AA, Fesharaki MG, Alavian SM. Hepatitis B virus infection in the general population of Iran: an updated systematic review and meta-analysis. Hepatitis Monthly. 2016 16(4).
Udovicki, B. et al. Risk assessment of dietary exposure to aflatoxin B1 in Serbia. Food Chem .Toxicol. 151 , 112116 (2021).
Zhang, W. et al. Probabilistic risk assessment of dietary exposure to aflatoxin B1 in Guangzhou China. Sci. Rep. 10 , 7973 (2020).
Ozay, G., Seyhan, F., Pembeci, C., Saklar, S. & Yilmaz, A. Factors influencing fungal and aflatoxin levels in Turkish hazelnuts (Corylus avellana L.) during growth, harvest, drying and storage: A 3-year study. Food Addit. Contam. 25 , 209–218 (2008).
Chain, E. P. O. C. I. T. F. et al. Risk assessment of aflatoxins in food. Efsa J. 18 , e06040 (2020).
Saffari, E., Madani, M., Karbasizade, V. & Shakib, P. Detection of fungal and bacterial contamination of hazelnut and determination of aflatoxin B by HPLC method in Isfahan Iran. Curr. Med. Mycol. 7 , 1 (2021).
PubMed PubMed Central Google Scholar
Gürses, M. Mycoflora and aflatoxin content of hazelnuts, walnuts, peanuts, almonds and roasted chickpeas (LEBLEBI) sold in Turkey. Int. J. Food Proper. 9 , 395–399 (2006).
Bircan, C., Barringer, S. A., Ulken, U. & Pehlivan, R. Aflatoxin levels in dried figs, nuts and paprika for export from Turkey. Int. J. Food Sci. Technol. 43 , 1492–1498 (2008).
Kabak, B. Aflatoxins in hazelnuts and dried figs: Occurrence and exposure assessment. Food Chem. 211 , 8–16 (2016).
Wang, Y. et al. Occurrence and co-occurrence of mycotoxins in nuts and dried fruits from China. Food control 88 , 181–189 (2018).
Akbar, R. M. & Nasrin, A. Aflatoxin occurrence in nuts consumed in Arak Iran. Int. J. Food Nutr. Saf. 4 , 91–97 (2013).
Diella, G. et al. Aflatoxin contamination in nuts marketed in Italy: preliminary results. Ann. Ig. 30 , 401–409 (2018).
Taghizadeh, S. F., Rezaee, R., Badibostan, H. & Karimi, G. Aflatoxin B1 in walnuts: A probabilistic cancer risk assessment for Iranians. Toxicol. Environ. Chem. 102 , 506–519 (2020).
Asghar, M. A. et al. Incidence of aflatoxins contamination in dry fruits and edible nuts collected from Pakistan. Food Control 78 , 169–175 (2017).
Ekinci, R., Otağ, M. & Kadakal, Ç. Patulin & ergosterol: New quality parameters together with aflatoxins in hazelnuts. Food Chem. 150 , 17–21 (2014).
Dhakal, A. & Sbar, E. Aflatoxin toxicity. (2020).
Ebrahimi, A. et al. The prevalence of aflatoxins in different nut samples: A global systematic review and probabilistic risk assessment. AIMS Agric. Food 7 , 130–148 (2022).
Gürses, M., Erdoğan, A., Türkoğlu, H. & Sert, S. The effects of storage period and relative humidity on Tombul type hazelnut produced in Turkey. Pak. J. Biol. Sci. 4 , 858–860 (2001).
Bensassi, F., Rhouma, A., Ghrab, M., Bacha, H. & Rabeh Hajlaoui, M. Evaluation of cultivar susceptibility and storage periods towards aflatoxin B1 contamination on pistachio nuts. Mycotoxin Res. 26 , 199–203 (2010).
Siciliano, I., Dal Bello, B., Zeppa, G., Spadaro, D. & Gullino, M. L. Static hot air and infrared rays roasting are efficient methods for aflatoxin decontamination on hazelnuts. Toxins 9 , 72 (2017).
Article PubMed PubMed Central Google Scholar
Demirci, A. S., Tirpanci Sivri, G., Tunc, M. & Mutlu, S. Detoxification of unshelled hazelnut artificially contaminated with aflatoxins by gaseous ozone. J. Food Measur. Charact. 17 (4), 3515–3522 (2023).
Keskin, Z. S. & Gürsoy, N. Investıgatıon of natural mycoflora and aflatoxin formation in hazelnuts and products. Cumhuriyet Sci. J. 40 , 967–977 (2019).
Özer, H. Fındıklara uygulanan fiziksel ve ısıl süreçlerin aflatoksinler üzerine etkisi. (2009).
Kluczkovski, A. M. Fungal and mycotoxin problems in the nut industry. Curr. Opin. Food Sci. 29 , 56–63 (2019).
Blanco-Lizarazo, C. M., Gamboa-Marín, A., Vega, S., Jiménez-Rodríguez, L. P. & Sánchez, B. I. C. Assessment of dietary exposure to aflatoxin B1 from corn arepas in Colombia. Food Addit. Contam. Part A. 36 (7), 1109–1117 (2019).
Leong, Y.-H., Rosma, A., Latiff, A. A. & Ahmad, N. I. Exposure assessment and risk characterization of aflatoxin B 1 in Malaysia. Mycotoxin Res. 27 , 207–214 (2011).
Renwick, A., Leblanc, J. C. & Setzer, R. W. Application of the margin of exposure (MOE) approach to substances in food that are genotoxic and carcinogenic. Example: Leucomalachite green. Food Chem. Toxicol. 48 , S75–S80 (2010).
Andrade, P., de Mello, M. H., França, J. & Caldas, E. Aflatoxins in food products consumed in Brazil: a preliminary dietary risk assessment. Food Addit. Contam. Part A 30 , 127–136 (2013).
Download references
Acknowledgements
This work is a report on the food safety and hygiene division in the School of Public Health at Tehran University of Medical Sciences.
Author information
Authors and affiliations.
Division of Food Safety and Hygiene, Department of Environmental Health Engineering, School of Public Health, Tehran University of Medical Sciences, Tehran, Iran
Parnian Samimi, Ramin Aslani, Ebrahim Molaee-Aghaee, Parisa Sadighara, Nabi Shariatifar & Gholamreza Jahed Khaniki
Provincial Directorate of Agriculture and Livestock, Ministry of Agriculture and Forestry, Samsun, Türkiye
Sibel Ozcakmak
Department of Food Hygiene, Science and Research Branch, Islamic Azad University, Tehran, Iran
Zahra Reshadat
You can also search for this author in PubMed Google Scholar
Contributions
All the authors contributed to the completion of this article. Their individual contributions were as follows: Conceptualization: E.M.A. and P.S.; Investigation: P.S. and R.A.; Sample collection and analysis: P.S.; Writing—original draft preparation: R.A. and Z.R.; Writing—review and editing: E.M.A., P.S., N.S., G.J.K. and S.Ö.
Corresponding author
Correspondence to Ebrahim Molaee-Aghaee .
Ethics declarations
Competing interests.
The authors declare no competing interests.
Additional information
Publisher's note.
Springer Nature remains neutral with regard to jurisdictional claims in published maps and institutional affiliations.
Rights and permissions
Open Access This article is licensed under a Creative Commons Attribution 4.0 International License, which permits use, sharing, adaptation, distribution and reproduction in any medium or format, as long as you give appropriate credit to the original author(s) and the source, provide a link to the Creative Commons licence, and indicate if changes were made. The images or other third party material in this article are included in the article's Creative Commons licence, unless indicated otherwise in a credit line to the material. If material is not included in the article's Creative Commons licence and your intended use is not permitted by statutory regulation or exceeds the permitted use, you will need to obtain permission directly from the copyright holder. To view a copy of this licence, visit http://creativecommons.org/licenses/by/4.0/ .
Reprints and permissions
About this article
Cite this article.
Samimi, P., Aslani, R., Molaee-Aghaee, E. et al. Determination and risk assessment of aflatoxin B1 in the kernel of imported raw hazelnuts from Eastern Azerbaijan Province of Iran. Sci Rep 14 , 6864 (2024). https://doi.org/10.1038/s41598-024-57422-2
Download citation
Received : 10 December 2023
Accepted : 18 March 2024
Published : 22 March 2024
DOI : https://doi.org/10.1038/s41598-024-57422-2
Share this article
Anyone you share the following link with will be able to read this content:
Sorry, a shareable link is not currently available for this article.
Provided by the Springer Nature SharedIt content-sharing initiative
By submitting a comment you agree to abide by our Terms and Community Guidelines . If you find something abusive or that does not comply with our terms or guidelines please flag it as inappropriate.
Quick links
- Explore articles by subject
- Guide to authors
- Editorial policies
Sign up for the Nature Briefing newsletter — what matters in science, free to your inbox daily.


An official website of the United States government
Here's how you know
Official websites use .gov A .gov website belongs to an official government organization in the United States.
Secure .gov websites use HTTPS A lock ( ) or https:// means you’ve safely connected to the .gov website. Share sensitive information only on official, secure websites.
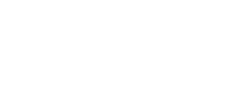
- Digg
Latest Earthquakes | Chat Share Social Media
New publication on study on antibiotic resistance in well water
Congratulations to Laboratory for Infectious Disease and the Environment (LIDE) Biologist Joel Stokdyk who is a coauthor on a recent paper about antibiotic resistance in well water.
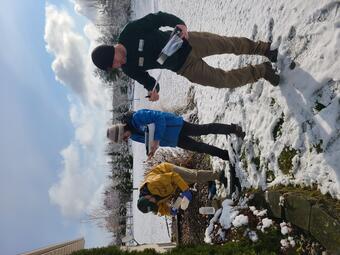
Antibiotic resistance is a global public health challenge with well-known environmental dimensions, but quantitative analyses of the roles played by various natural environments in transmission of antibiotic resistance are lacking, particularly for drinking water.
A new paper was recently published, Quantitative microbial risk assessment for ingestion of antibiotic resistance genes from private wells contaminated by human and livestock fecal sources .
This study assesses risk of ingestion for several antibiotic resistance genes (ARGs) and the class 1 integron gene ( intI1 ) in drinking water from private wells in a rural area of northeast Wisconsin, United States. Results allow comparison of drinking water as an exposure route for antibiotic resistance relative to other routes like food and recreational water. They also enable a comparison of the importance of human versus livestock fecal sources in the study area. Our study demonstrates the previously unrecognized importance of untreated rural drinking water as an exposure route for antibiotic resistance and identifies bovine fecal material as an important exposure factor in the study setting.
This study is part of a research program about private well water quality in Northeast Wisconsin's vulnerable aquifer, and, more generally, vulnerable groundwater across Wisconsin. Related previous publications such as Microbial source tracking and land use associations for antibiotic resistance genes in private wells influenced by human and livestock fecal sources , and Sources and Risk Factors for Nitrate and Microbial Contamination of Private Household Wells in the Fractured Dolomite Aquifer of Northeastern Wisconsin study groundwater contamination, sources of contamination, factors for contamination, and (with the recent field work) forecasting when contamination is most likely.
These studies aren't done in isolation and are part of a broader effort to understand and improve well water quality for Northeast Wisconsin residents.
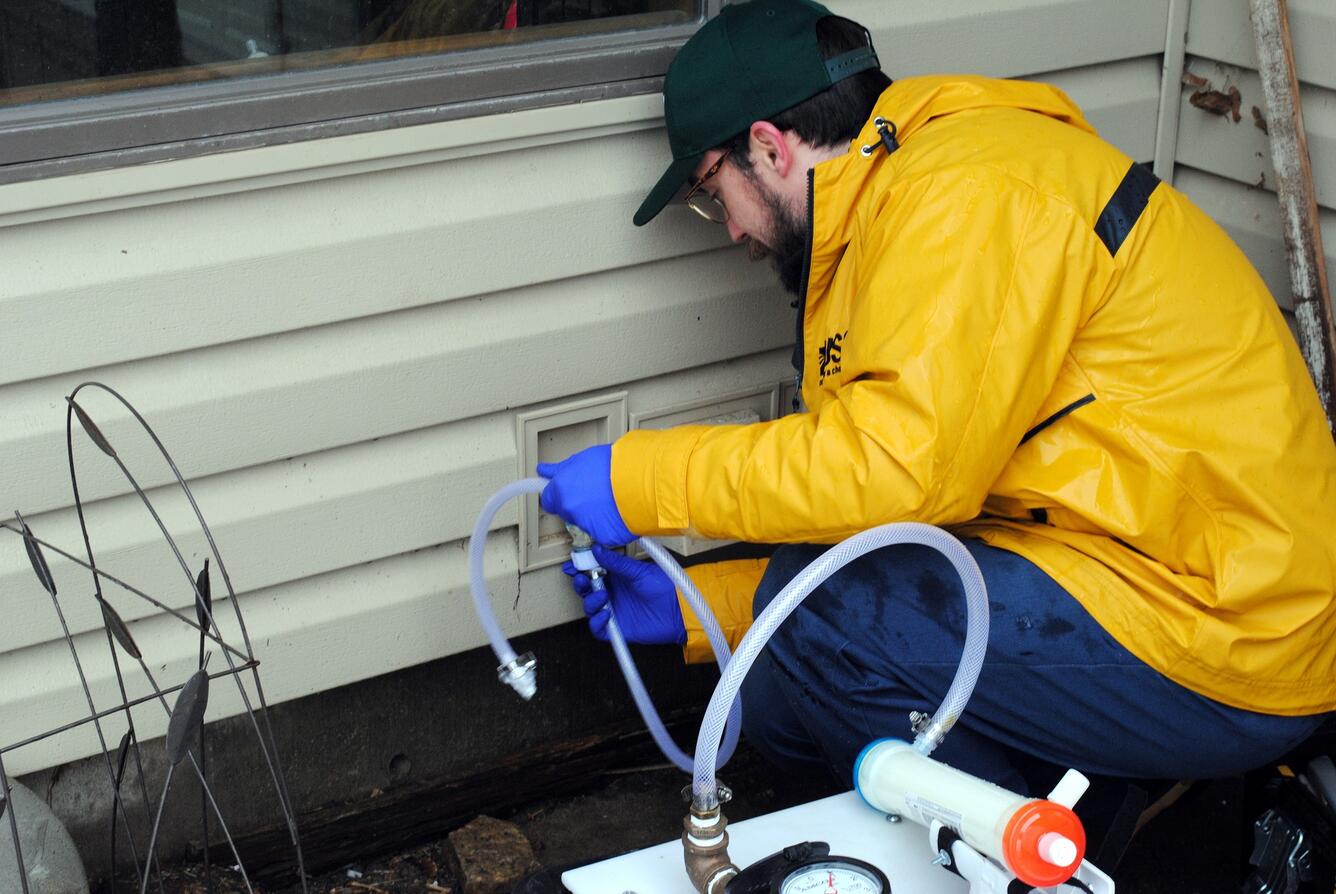
Related Content
- Publications
Quantitative microbial risk assessment for ingestion of antibiotic resistance genes from private wells contaminated by human and livestock fecal sources
Microbial source tracking and land use associations for antibiotic resistance genes in private wells influenced by human and livestock fecal sources, sources and risk factors for nitrate and microbial contamination of private household wells in the fractured dolomite aquifer of northeastern wisconsin, get our news.
These items are in the RSS feed format (Really Simple Syndication) based on categories such as topics, locations, and more. You can install and RSS reader browser extension, software, or use a third-party service to receive immediate news updates depending on the feed that you have added. If you click the feed links below, they may look strange because they are simply XML code. An RSS reader can easily read this code and push out a notification to you when something new is posted to our site.
Laboratory for Infectious Disease and the Environment News
Upper Midwest Water Science Center News
Study: Combining info on genes, gut bacteria enhances early disease detection
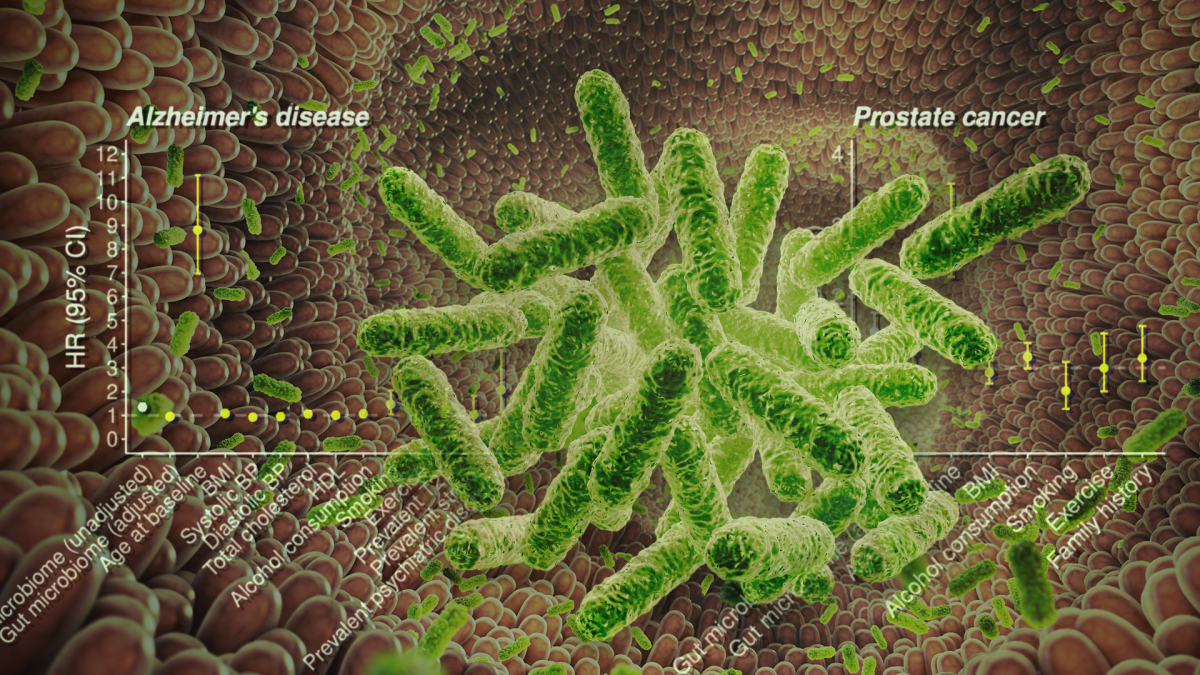
A new study highlights improvements in estimating the risk of common diseases when data from the body's gut microbiome is combined with genetic and other risk factors. Graphic by Jason Drees
Identifying those at highest risk for developing common chronic diseases like heart disease, diabetes, Alzheimer’s disease and cancer is a core priority for preventive medicine. By catching elevated risk early, physicians can implement lifestyle interventions, closer monitoring and targeted therapies to potentially delay or even prevent disease onset and progression.
Traditional risk assessment models based solely on factors like age, family history and standard clinical measurements often fail to capture the full scope of an individual's inherited and biological susceptibility.
A new study demonstrates the power of integrating cutting-edge microbiome analysis into disease risk prediction models, paving the way for more personalized and effective preventive strategies.
Arizona State University's Qiyun Zhu is part of an international research team, spearheaded by Yang Liu at the University of Cambridge, delving into how microbiomics can refine disease risk metrics.
Microbiomics examines the complex relationships between a host and its microorganisms, including bacteria, viruses and fungi, to discern their effects on health, disease progression and general wellness.
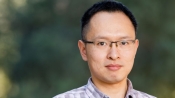
Zhu is a researcher with the Biodesign Center for Fundamental and Applied Microbiomics and an assistant professor with ASU’s School of Life Sciences .
The study was a collaborative effort involving experts from institutions worldwide, including the University of Cambridge, Baker Heart and Diabetes Institute, Finnish Institute for Health and Welfare, and University of California San Diego. This diverse team combined their expertise in genomics, microbiomics, epidemiology and computational biology to drive the innovative research forward.
The study appears in the current issue of the journal Nature Aging.
Diagnostic challenges
Early detection of many diseases remains crucial for successful therapy. Despite significant advances in disease discovery, early diagnosis for many common ailments remains an inexact science. But research into the body’s vast composition of gut microbial cultures is offering new insights into disease susceptibility.
The gut microbiome serves as an enormous reservoir of microbial life. These complex communities of microorganisms can act synergistically, performing a variety of tasks ranging from digestive activities and weight management to changes in the brain believed to influence behavior.
By combining three complementary components — clinical risk factors, polygenic risk score (a number that indicates a person's inherited risk for developing a particular medical condition) and gut microbiome analysis — the researchers were able to develop integrated predictive models that outperformed any single component alone in forecasting disease risk over long-term follow-up.
The new study gathers health data from more than 5,670 adults, conducted in collaboration by the Baker Heart and Diabetes Institute, the University of Cambridge and the Finnish Institute of Health and Welfare.
Conventional disease risk assessment typically relies on factors like age, sex, body mass index, blood pressure, cholesterol levels and blood glucose measurements. By leveraging powerful new data streams combining polygenic risk scores with analysis of gut microbial composition, predictive models are enhanced, improving physicians' ability to pinpoint those at elevated risk and tailor prevention strategies to their unique biological profile.
In this study, researchers investigated the individual and combined predictive performance of polygenic risk scores, the gut microbiome and traditional risk factors for development of future coronary artery disease, Type 2 diabetes, Alzheimer's disease and prostate cancer over a median of ~18 years of follow-up using a population survey known as FINRISK.
Multi-pronged analysis
Multi-omics refers to the integration and analysis of data from multiple fields to gain a more comprehensive understanding of biological systems and processes. This approach aims to provide a holistic view of how genetic, transcriptomic, proteomic and metabolomic levels interact within an organism, offering insights into complex biological questions and diseases that cannot be understood through single-omics studies alone.
Multi-omic technologies have uncovered new biomarkers for a variety of common age-related diseases. If individuals at elevated risk for these prevalent chronic illnesses can be flagged early through predictive modeling that incorporates multi-omic biomarkers, preventive interventions could then be applied to high-risk groups before disease onset or progression.
Early preventive strategies like lifestyle modifications, increased monitoring, preventive medications or therapies targeting specific biological mechanisms could potentially delay or even avoid the manifestation of disease symptoms and complications.
As multi-omic technologies continue advancing, their integration into robust predictive models holds promise for ushering in an era of precision prevention where personalized preventive plans can be tailored based on each individual's unique biomolecular profile and risk landscape.
"Multiomics combined with machine learning is revolutionizing how we diagnose diseases and predict disease risks,” Zhu says. “Microbiomics is one critical ‘omics’ among these.”
The new study demonstrates the potential of integrating diverse multi-omic data, including gut microbial composition, to advance the understanding of the development and prediction of diseases. Challenges remain in properly incorporating the diverse data to provide the best risk analyses and improve treatment options.
More Science and technology
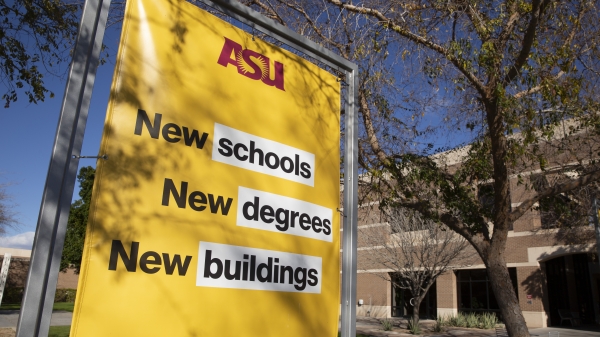
New engineering degrees at ASU aim to open pathways, empower engineering expertise
It doesn’t take an extensive internet search to discover that engineering has become one of the most rapidly and broadly expanding STEM fields. Engineering has been on an upswing in recent years,…
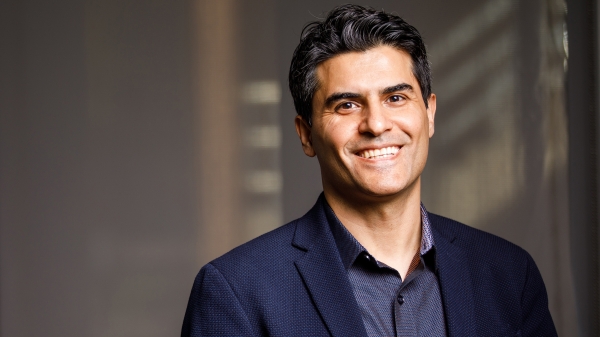
ASU researcher's microscale tech is chipping away at cancer, organ failure and neurological disease
Arizona State University’s Mehdi Nikkhah, a biomedical engineer in Arizona State University's School of Biological Health Systems Engineering and a member of the Personalized Diagnostics Faculty at…
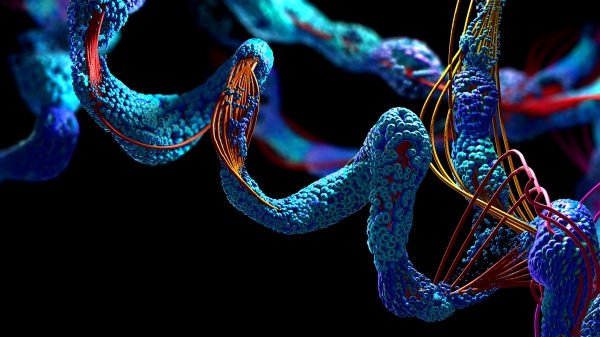
ASU Regents Professor inducted into 2024 class of AIMBE College of Fellows
The American Institute for Medical and Biological Engineering (AIMBE) has announced the induction of Regents Professor Petra Fromme, director of the Biodesign Center for Applied Structural Discovery…
- Skip to main content
- Skip to FDA Search
- Skip to in this section menu
- Skip to footer links

The .gov means it’s official. Federal government websites often end in .gov or .mil. Before sharing sensitive information, make sure you're on a federal government site.
The site is secure. The https:// ensures that you are connecting to the official website and that any information you provide is encrypted and transmitted securely.
U.S. Food and Drug Administration
- Search
- Menu
- Guidance & Regulation (Food and Dietary Supplements)
- Retail Food Protection
Retail Food Risk Factor Study
Retail Food Risk Factor Study Toolkit
FDA’s National Retail Food Team designed the following resources to help state, local, territorial, and tribal jurisdictions complete a risk factor study as part of Standard 9 - Program Assessment of the Voluntary National Retail Food Regulatory Program Standards .
Completion of a risk factor study and implementation of a focused public health intervention can decrease the occurrence of foodborne illness risk factors and protect food served at retail.
- Risk Factor Study Overview and Guidance Document (PDF)
- Sample Size Statistics Guide (PDF)
- Sample Size Calculator (PDF)
- Food Code Reference Crosswalk (PDF)
- Study Checklist (PDF)
- Sample Restaurant Data Collection Form (PDF)
- Sample Retail Food Store Data Collection Form (PDF)
- Marking Instructions (PDF)
Additionally, Retail Food Specialists have other resources they can customize to assist jurisdictions with study design, training, and data analysis, including workshops and our risk factor study database.
For more information, contact your Retail Food Specialist .
Get regular FDA email updates delivered on this topic to your inbox.
Log in using your username and password
- Search More Search for this keyword Advanced search
- Latest content
- Current issue
- For authors
- New editors
- BMJ Journals More You are viewing from: Google Indexer
You are here
- Volume 58, Issue 7
- Association between change in cardiorespiratory fitness and prostate cancer incidence and mortality in 57 652 Swedish men
- Article Text
- Article info
- Citation Tools
- Rapid Responses
- Article metrics

- http://orcid.org/0000-0002-5140-9098 Kate A Bolam 1 ,
- Emil Bojsen-Møller 1 ,
- Peter Wallin 2 ,
- Sofia Paulsson 2 ,
- Magnus Lindwall 1 , 3 ,
- http://orcid.org/0000-0002-5617-9076 Helene Rundqvist 4 ,
- http://orcid.org/0000-0002-3901-7833 Elin Ekblom-Bak 1
- 1 Department of Physical Activity and Health , Swedish School of Sport and Health Sciences GIH , Stockholm , Sweden
- 2 Research Department , HPI Health Profile Institute , Stockholm , Sweden
- 3 Department of Psychology , University of Gothenburg , Goteborg , Sweden
- 4 Department of Laboratory Medicine , Karolinska Institutet , Stockholm , Sweden
- Correspondence to Dr Kate A Bolam, Department of Physical Activity and Health, Swedish School of Sport and Health Sciences GIH, Stockholm 114 33, Sweden; kate.bolam{at}gih.se
Objectives To examine the associations between changes in cardiorespiratory fitness (CRF) in adulthood and prostate cancer incidence and mortality.
Methods In this prospective study, men who completed an occupational health profile assessment including at least two valid submaximal CRF tests, performed on a cycle ergometer, were included in the study. Data on prostate cancer incidence and mortality were derived from national registers. HRs and CIs were calculated using Cox proportional hazard regression with inverse probability treatment weights of time-varying covariates.
Results During a mean follow-up time of 6.7 years (SD 4.9), 592 (1%) of the 57 652 men were diagnosed with prostate cancer, and 46 (0.08%) died with prostate cancer as the primary cause of death. An increase in absolute CRF (as % of L/min) was associated with a reduced risk of prostate cancer incidence (HR 0.98, 95% CI 0.96 to 0.99) but not mortality, in the fully adjusted model. When participants were grouped as having increased (+3%), stable (±3%) or decreased (−3%) CRF, those with increased fitness also had a reduced risk of prostate cancer incidence compared with those with decreased fitness (HR 0.65, 95% CI 0.49 to 0.86), in the fully adjusted model.
Conclusion In this study of employed Swedish men, change in CRF was inversely associated with risk of prostate cancer incidence, but not mortality. Change in CRF appears to be important for reducing the risk of prostate cancer.
- Physical fitness
Data availability statement
Data may be obtained from a third party and are not publicly available. The data underlying the findings in this study are currently not publicly available as the original ethical approval application and the informed consent form did not include such direct, free access to the data. Data are stored by and can be requested from the HPI Health Profile Institute at [email protected].
This is an open access article distributed in accordance with the Creative Commons Attribution Non Commercial (CC BY-NC 4.0) license, which permits others to distribute, remix, adapt, build upon this work non-commercially, and license their derivative works on different terms, provided the original work is properly cited, appropriate credit is given, any changes made indicated, and the use is non-commercial. See: http://creativecommons.org/licenses/by-nc/4.0/ .
https://doi.org/10.1136/bjsports-2023-107007
Statistics from Altmetric.com
Request permissions.
If you wish to reuse any or all of this article please use the link below which will take you to the Copyright Clearance Center’s RightsLink service. You will be able to get a quick price and instant permission to reuse the content in many different ways.
WHAT IS ALREADY KNOWN ON THIS TOPIC
Cardiorespiratory fitness (CRF) is associated with the risk of being diagnosed with, or dying from, certain cancer types.
The associations between CRF measured at one time point and risk of prostate cancer incidence are contradictory, which may be the result of the influence of higher prostate cancer screening rates in men with higher fitness.
WHAT THIS STUDY ADDS
Our results suggest that increases in CRF are inversely associated with risk of prostate cancer incidence, but not mortality.
The results highlight the importance of CRF for prostate cancer risk, which has been challenging to determine with single timepoint studies.
HOW THIS STUDY MIGHT AFFECT RESEARCH, PRACTICE OR POLICY
Improvements in CRF in adult men should be encouraged and may reduce the risk of prostate cancer.
Introduction
Unlike other common cancers such as breast, colon and lung cancer, there are relatively few preventable risk factors with strong evidence for reducing prostate cancer risk. Aside from developmental factors, being diagnosed with overweight or obesity are the main risk factors for developing advanced prostate cancer, but insufficient evidence exists to extend this conclusion to non-advanced prostate cancer. 1
While there are well-established relationships between physical activity levels and the incidence and mortality of an increasing number of cancer types, the evidence for prostate cancer is not conclusive. 2 Several studies have reported a decreased risk of prostate cancer incidence, while others have reported an increased risk of prostate cancer for those with higher physical activity levels. 2 Fewer still have investigated the relationships between cardiorespiratory fitness (CRF) and prostate cancer incidence and mortality risk. Local and systemic inflammation is associated with increased risk of (advanced) prostate cancer; therefore, there is an increasing focus on understanding the role of CRF on cancer risk because of the favourable associations between CRF and systemic inflammation. 3 Additionally, CRF has shown to have desirable associations with abdominal obesity, 4 dyslipidaemia 5 and insulin sensitivity. 6 In the studies that have investigated this relationship between CRF and cancer, the findings generally mirror those conflicting findings in studies of physical activity and cancer risk. 7–11 The tendency for men with higher CRF to also have higher prostate cancer screening participation rates may be an influencing factor to explain these inconsistent findings. 7 10
Examining the association between change in CRF over two time points and prostate cancer incidence and mortality risk could contribute to our understanding of the role of CRF as a risk factor. To date, only four smaller studies have investigated the association between change in CRF or physical activity and cancer incidence or mortality. 12–15 Despite the known differences in mechanisms behind both the development of different cancers and the role CRF may play in reducing the risk of cancer, none of these studies examined either incidence or mortality by specific cancer type. To better understand the relationship between CRF and prostate cancer incidence and mortality, and to contribute to cancer-specific prevention recommendations, it is essential to conduct analyses by cancer type. Therefore, the aim of the current study was to determine if there were any associations between changes in CRF and prostate cancer incidence and mortality.
In this prospective cohort study, data were drawn from the health profile assessment (HPA) database, managed by the HPI Health Profile Institute (HPI, Stockholm Sweden). Participation in the HPA was optional, usually offered to all employees working at an associated company or organisation and conducted by an occupational and health service with no financial cost to the employees. HPI was contracted by the occupational health services and responsible for the development and standardisation of the method, education of data collection staff, and administration of the central database. Each HPA consisted of a questionnaire assessing physical activity, lifestyle, perceived health, measurement of body mass and height, and a submaximal ergometer CRF test.
From October 1982 until December 2019 (month of follow-up of outcome data in national registers) data from a total of 181 673 men (aged 18 years and older) were stored in the central HPA database. People were included if they had data for all covariates included in the full analyses (age, education level, body mass index (BMI) and smoking), and at least two valid CRF tests from two separate health assessments, 11 months or more apart. For participants who had more than two tests, only their first and last tests were included in the analyses. Participants were excluded if they had already been diagnosed with prostate cancer before the second health assessment, and only men were included in the study population.
Assessment of CRF
The standardised Åstrand test, a submaximal cycle ergometer test, was used to assess the study exposure variable CRF as estimated VO 2max . 16–18 Previously, the Åstrand test has been validated against directly measured VO 2max during a maximal treadmill test to exhaustion in an adult population. The validation study demonstrated a marginal and insignificant mean difference of −0.07 L/min (95% CI −0.21 to 0.06) in VO 2max between the Åstrand test and VO 2max measured directly. The SE of estimate was 0.48 L/min and the coefficient of variation was 18.1%, which align with validations of other submaximal VO 2max tests. 16 Participants were requested to refrain from the following before the test: vigorous activity the day before, consuming a heavy meal 3 hours before, smoking/snuff use 1 hour before and avoiding stress directly before test. Change in CRF was expressed as absolute (L/min) and relative (mL/kg/min) per year and divided into groups based on if their absolute CRF increased by more than 3% (increase), decreased by more than 3% (decrease) or remained within ±3% (stable). In addition, we divided participants into tertials according to their baseline relative CRF, resulting in three equally sized groups: low CRF (<32.4 mL/kg/min), moderate CRF (32.4–40.7 mL/kg/min) and high CRF (>40.7 mL/kg/min). In this stratified analysis the individual change in absolute CRF was standardised to the mean and SD of the baseline relative CRF category group the individual belonged to, in order to minimise the regression toward the mean effect of the groups.
Prostate cancer incidence and mortality
The Swedish National Hospital and National Cause of Death registries 19 were used to draw data on prostate cancer diagnosis and mortality. This data was linked to the HPA database by linking each participant’s unique Swedish personal identification number. For the incidence analyses, all participants were followed from the date of the last HPA to the date of their prostate cancer diagnosis, death (of any cause) or until 31 December 2019. For the mortality analyses, all participants were followed from the date of their second HPA to either the date of death from prostate cancer, death from any cause, or until 31 December 2019. The ICD-10 code used to identify prostate cancer was C61.
Assessment of covariates
Covariates were included based on current evidence of their potential to influence the relationship between CRF and prostate cancer incidence or mortality. Highest level of education attained at the time of the HPA (defined as length of education <9 years to postgraduate education) was obtained from Statistics Sweden by linking the participant personal identity number. Smoking at the time of the HPA was self-reported using the following statements: I smoke … with the options: At least 20 cig/day, 11–19 cig/day, 1–10 cig/day, occasionally or never. Body mass was assessed at the HPA with a calibrated scale in light-weight clothing to the nearest 0.5 kg. Height was assessed at the HPA using a wall mounted stadiometer and measured to the nearest 0.5 cm. BMI (kg/m 2 ) was subsequently calculated.
Patient and public involvement
We did not involve patients or the public in the design or while conducting our research. In addition to scientific journal articles, the results of the study will be disseminated to the public through online print media and with the cancer community through the Swedish Cancer Society.
Equity, diversity and inclusion statement
Our study did not purposefully exclude people based on their race, ethnicity or socioeconomic level. Information on race, ethnicity or socioeconomic level was not included in the database. The project group included four women and three men and was led by a woman. Researchers involved are from all career levels from postdoctoral researcher to professor. All researchers reside in Stockholm, Sweden.
Statistical analyses
Outliers that lay −20% and 20% (L/min/year) from the mean were removed (n=1319) to minimise influence of measurement error, as this level of change per year over several years is extremely rare and unlikely. Associations between CRF at baseline and at follow-up and prostate cancer incidence and mortality were analysed using Cox proportional hazard regression models. Associations between changes in CRF and prostate cancer incidence and mortality were analysed using Cox proportional hazard regression models with inverse probability treatment weights of time-varying confounders such as age, BMI and smoking. Non-time varying confounders (education, baseline CRF and year of last HPA test) were adjusted for conventionally. For visualisation purposes, restricted cubic splines with knots at 5th, 50th and 95th percentile were performed for % change in absolute CRF with 0% change as the reference.
Four models were created with an increasing number of variables for each model. Model 1 included only baseline CRF. Model 2 additionally included age, education and year of the last HPA test. Model 3 additionally included BMI, and model 4 further included smoking. Model 5 ( online supplemental file ) additionally included adjustment for self-reported physical activity. Baseline CRF was not adjusted for in the one timepoint analysis (baseline and follow-up associations) or in the subanalysis stratified by baseline CRF.
Supplemental material
In the subgroup analyses, Cox proportional hazard modelling with inverse probability treatment weight of time-varying confounders was used to examine the relationships between the groups, stratified by direction of change in absolute fitness (stable, decrease and increase) and baseline relative CRF (low, moderate and high), and prostate cancer incidence and mortality. For all models the proportional hazard assumption was checked using scaled Schoenfeld residuals. To investigate if change in absolute CRF were associated with change in self-reported physical activity, a linear regression weighted for age, and adjusted for education, and the year of the last HPA test was performed.
Sensitivity analysis to address potential reverse causality was performed by dropping incident cases that occurred ≤2 years after the last HPA test for the main analysis and the grouped (increase, decrease and stable) analysis.
All statistical analyses were performed using R Studio, V.4.2.1 (2022-06-23). 20 The IPW package 21 was used to create inverse probability treatment weights and the survival 22 package was used to create Cox proportional hazard regression models. The splines, 20 ggplot2 23 and survminer 24 package were used to visualise the restricted cubic splines. Conduct and presentation of statistical analyses in this study are in accordance with the CHecklist for statistical Assessment of Medical Papers) statement. 25
Among the 181 673 men, 58 971 met the criteria of having undertaken a minimum of two tests spaced at least 11 months apart. We excluded 1319 individuals due to a CRF decrease or increase of over 20% per year ( figure 1 ). The analytic sample consisted of 57 652 men, with a mean age of 41.4 years (range 18–79 years) and an average BMI of 26.0 kg/m 2 (SD 3.6) at the first HPA ( table 1 ). Of the 57 652 men, 592 (1%) were diagnosed with prostate cancer, while 46 (0.08%) died of prostate cancer as the primary cause of death. At baseline, the sample’s mean relative and absolute CRF were 37.4 mL/kg/min and 3.12 L/min, respectively. From the first to the second HPA relative CRF decreased by 0.27 mL/kg/min (SD 2.2), while absolute CRF decreased by 0.01 L/min (SD 0.17), on average. The time between the tests was on average 4.9 years (SD 3.6). The mean follow-up time for the incidence analyses was 6.7 years (SD 4.9) from the last HPA test.
- Download figure
- Open in new tab
- Download powerpoint
Flow chart of participant inclusion.
- View inline
Participant descriptive characteristics, all, and grouped by changes in and baseline cardiorespiratory fitness
There was no association between baseline CRF or CRF at last test and the risk of prostate cancer incidence, when the models were adjusted for relevant covariates ( online supplemental table s1 ). Only in the unadjusted model 1 was there an inverse association between CRF both at baseline and at last test and the risk of incidence prostate cancer.
Percentage change in absolute CRF (L/min) was inversely associated with risk of being diagnosed with prostate cancer ( figure 2 and table 2 ) and remained significant after adjusting for all covariates (baseline CRF, age, education level, year of test, BMI, smoking) ( table 2 ) and physical activity ( online supplemental table s2 ). In comparison, per cent change in relative CRF (mL/kg/min) was associated with prostate cancer incidence only for model 1 (adjusted for baseline CRF).
Restricted cubic splines of the Cox proportional model examining the association between % change in cardiorespiratory fitness and incidence of prostate cancer. Model 1: adjusted for baseline fitness. Model 2: adjusted for baseline fitness, age, education and year of last test. Model 3: adjusted for baseline fitness, age, education, year of last test and body mass index. Model 4: adjusted for baseline fitness, age, education, year of last test, body mass index and smoking. BMI, body mass index; VO 2max , estimated maximal oxygen consumption.
HRs with 95% CIs for the association between change in cardiorespiratory fitness and prostate cancer incidence and mortality
When participants were grouped by those with increased (+3%), stable (±3%) or decreased (−3%) absolute CRF, those with increased CRF had significantly lower cancer incidence risk compared with those who held their CRF stable ( figure 3 ). The associations remained significant after adjusting for the covariates in all models. In a sensitivity analysis, individuals who were diagnosed with prostate cancer in the 2 years following the last HPA were excluded (n=103). The sensitivity analyses showed that the association between absolute CRF and prostate cancer risk remained significant (see online supplemental table s3 ). However, in the directional analysis, comparing the stable group to the decreased and increased group, models 2 and 3 were no longer significant.
Cox proportional HRs with 95% CIs showing decrease (−3%) compared with stable (±3%) and increase (+3%). Model 1: adjusted for baseline fitness. Model 2: adjusted for baseline fitness, age, education and year of last test. Model 3: adjusted for baseline fitness, age, education, year of last test and body mass index. Model 4: adjusted for baseline fitness, age, education, year of last test, body mass index and smoking. *p<0.05; **p<0.01; ***p<0.001.
Figure 4 shows the results from subgroup analyses stratified by baseline relative CRF using group-standardised absolute fitness change. For the group with moderate baseline CRF (32.4–40.7 mL/kg/min), every SD increase in absolute CRF (L/min) reduced the risk of prostate cancer incidence by 16% for model 1. Significant associations remained even after adjusting for covariates in models 2 to 4. None of the models yielded significant associations for the low and high fitness groups. Even when using the percentage change in absolute fitness to analyse the data, the results remained consistent with the standardised analysis. However, in the case of the high fitness group, the least adjusted model (model 1) did show a significant inverse association with prostate cancer incidence, but this association was attenuated when adjusting for covariates (see online supplemental table s4 ).
Cox proportional HRs with 95% CIs for the association between change in cardiorespiratory fitness and incidence of prostate cancer within low, moderate and high baseline cardiorespiratory fitness groups. Model 1: adjusted for baseline fitness. Model 2: adjusted for baseline fitness, age, education and year of last test. Model 3: adjusted for baseline fitness, age, education, year of last test, and body mass index. Model 4: adjusted for baseline fitness, age, education, year of last test, body mass index and smoking. *p<0.05; **p<0.01; ***p<0.001.
Change in absolute CRF was positively associated with changes in self-reported physical activity (see online supplemental table s5 ). Only 46 prostate cancer deaths were observed and changes in CRF were not associated with prostate cancer mortality ( table 2 ).
This is the largest study to examine the relationships between change in CRF and cancer incidence and mortality, and the first study to examine change in CRF specifically on prostate cancer incidence and mortality. The main finding of this study of over 57 000 men were that change in CRF (% L/min) was inversely associated with the risk of prostate cancer incidence and remained significant despite adjusting for relevant covariates. Subsequently, individuals with an annual increase in absolute CRF by 3% or more had a significantly lower risk of being diagnosed with cancer compared with individuals whose CRF was stable. When the participants were grouped by baseline CRF, the association between change in absolute CRF and prostate cancer incidence was only significant for the group with moderate baseline CRF. Changes in both absolute and relative CRF were not associated with prostate cancer mortality.
The findings in the present study contribute significantly to our knowledge of the relationship between CRF and prostate cancer as it is the first study to investigate change in CRF rather than CRF at a single time point, and to focus on prostate cancer specifically. Contrary to other cancer types, in the studies that have examined the relationship between CRF at one time point and prostate cancer incidence, the majority have reported an increased risk of prostate cancer incidence with increasing CRF, however there are studies that have reported the opposite. 2 7–10 While the reason for these positive associations may not be due to a ‘true’ increased risk for those with higher CRF, but in part due to the influence of higher prostate cancer screening rates in men with higher CRF. This cannot be confirmed in the current analyses due to lack of information on screening rates. 2
Analyses showed a reduced risk for prostate cancer incidence for those who increased their absolute CRF by more than 3%. In context, meta-analyses in adults have shown a person’s CRF can be improved by up to 16% in exercise interventions shorter than 1 year in duration. 26 27 In the only other study to investigate change in CRF and cancer incidence, Robsahm et al 14 reported similar findings to the current study, that in comparison to a decrease in CRF (>5%), an increase in CRF (>5%) was associated with 19% lower cancer incidence. 14
When the full group was stratified by baseline CRF, a reduced risk for prostate cancer incidence (−15%) was seen only in those with moderate CRF. While we can only speculate on the reason for this, it may be that improving one’s CRF from an already high baseline does not confer any discernible additional benefits. It may also be that the increases in CRF in people with low CRF were not sufficient to reach a potential threshold needed to contribute to lowering their risk of prostate cancer. In addition, residual confounding may account for the lack of associations. There is a possibility that the observed change in both the high and low groups may, in part, be a result of a regression to the mean. This means that individuals with initially extreme values, whether high or low, are more likely to have less extreme values on subsequent tests, due to random variation, rather than a change in their underlying fitness level. Consequently, it is plausible to suggest that only individuals in the moderate fitness group have the potential to show changes in both directions. This group’s fitness level may change, in a manner that is more reflective of actual change in their fitness, without the influence of regression to the mean.
Change in CRF was not associated with prostate cancer mortality in the current study. While no study has investigated the association between change in CRF and prostate cancer mortality, three studies have investigated the associations between CRF and cancer mortality in general. 13–15 These studies in men found that higher CRF was associated with a 16%–30% lower risk of cancer mortality. Only 46 prostate cancer-related deaths were observed in the present study, and therefore the analyses may not have been sufficiently powered to detect any associations. There is also considerable competing mortality risk from other chronic diseases, such as cardiovascular and metabolic disease, associated with prostate cancer treatment side effects. 28 It would therefore be of interest to examine the associations between CRF and mortality from these diseases in men diagnosed with prostate cancer.
The strengths of this study are the large sample size, the possibility to adjust for relevant covariates and the access to the national registry. Importantly, the study focused on a specific cancer type ensuring prostate specific conclusions. A novelty of the study was the objective measurement of CRF at two timepoints using standardised methodology.
Clinical implications
Where previous cancer prevention recommendations have focused on physical activity, the findings of this study provide further clarity on the associations between CRF and cancer incidence in an area of research with conflicting results. Although highly complex in nature, these investigations that aim to understand potential mechanisms behind the beneficial role of physical activity for preventing cancer will lead to more targeted prevention recommendations. The results of this study highlight the important role of supporting the general public to increase their CRF or aim to reach moderate fitness levels.
Limitations
While measuring VO 2max directly during a maximal protocol with spirometry is the gold standard, it was not possible in this study. The submaximal protocol used in this study has however been reported to produce valid and reliable estimations compared with directly measured VO 2max . 16 Furthermore, the submaximal test used has not been validated in change studies, and this must be taken into consideration when translating the findings from this study. It is also important to note that there is a significant genetic component to both an individual’s CRF levels and cancer risk, which must be considered when interpreting the results. Furthermore, the recruited group were employed and therefore findings may not be generalisable to populations outside of this demographic, particularly people who are unemployed. Unfortunately, information on race or ethnicity was not recorded in the current data set, therefore it was not possible to conduct analyses among the different groups. In the present study, two time points were used to capture changes in CRF. It has been put forward that at least three time points are needed to establish the true trajectory of change. 29 However, as relatively few individuals in the current study had data for three or more time points, we decided to include those with at least two time points. Finally, while there is an established link between systemic inflammation and advanced prostate cancer, the mechanism between less aggressive or localised prostate tumours is not fully understood and warrants future research.
Conclusions
In this large study of Swedish men, change in CRF was inversely associated with risk of prostate cancer incidence, but not prostate cancer mortality. Participants whose CRF increased by more than 3% had a significantly lower risk of prostate cancer incidence.
Ethics statements
Patient consent for publication.
Not applicable.
Ethics approval
The study was approved by the Stockholm Ethics Review Board (Dnr 2015/1864-31/2, 2016/9-32 and 2019-05711), and adhered to the Declaration of Helsinki.
- McMichael AJ
- Shephard RJ
- Church TS ,
- Milani RV , et al
- Grandes G ,
- Sanchez A , et al
- Sarzynski MA ,
- Lee D-C , et al
- Solomon TPJ ,
- Karstoft K , et al
- Hébert JR , et al
- Stattin P ,
- Brooks JD , et al
- Kunutsor SK ,
- Voutilainen A ,
- Laukkanen JA
- Reiter-Brennan C ,
- Al-Mallah MH , et al
- Ahmadi MN ,
- Hamer M , et al
- Imboden MT ,
- Harber MP ,
- Whaley MH , et al
- Robsahm TE ,
- Sandvik L , et al
- Hand GA , et al
- Björkman F ,
- Ekblom-Bak E ,
- Ekblom Ö , et al
- Hellenius M-L , et al
- Brooke HL ,
- Talbäck M ,
- Hörnblad J , et al
- R Core Team
- van der Wal WM ,
- Therneau TM
- Wickham H ,
- Henry L , et al
- Kassambara A ,
- Kosinski M ,
- Biecek P , et al
- Mansournia MA ,
- Collins GS ,
- Nielsen RO , et al
- Gibson CA ,
- Tran ZV , et al
- Scribbans TD ,
- Hankinson PB , et al
- Riihimäki M ,
- Thomsen H ,
- Brandt A , et al
- Curran PJ ,
Supplementary materials
Supplementary data.
This web only file has been produced by the BMJ Publishing Group from an electronic file supplied by the author(s) and has not been edited for content.
- Data supplement 1
KAB and EB-M are joint first authors.
Twitter @katebolam
Contributors All authors have contributed with the conception and design of the work. KAB and EB-M contributed equally to this paper and share joint first authorship. SP contributed to the acquisition of the data. EB-M contributed by conducting the analyses and he and KAB interpreted the data. KAB and EB-M drafted the work, while all authors have revised the work and contributed with intellectual content. EE-B and EB-M monitored adherence to the design and statistical analyses. All authors had full access to all the data in the study and take responsibility for the integrity of the data and the accuracy of the data analyses. All authors have approved the final version of the paper to be published and agreed to be accountable for all aspects of the work in ensuring that questions related to the accuracy or integrity of any part of the work were appropriately investigated and resolved. E-EB was responsible for the overall content of the paper as the guarantor.
Funding This study was funded by the Swedish Cancer Society, ref. 21 1837 Pj.
Competing interests None declared.
Patient and public involvement We did not involve patients or the public in the design or while conducting our research. In addition to scientific journal articles, the results of the study will be disseminated to the public through online print media and with the cancer community through the Swedish Cancer Society.
Provenance and peer review Not commissioned; externally peer reviewed.
Supplemental material This content has been supplied by the author(s). It has not been vetted by BMJ Publishing Group Limited (BMJ) and may not have been peer-reviewed. Any opinions or recommendations discussed are solely those of the author(s) and are not endorsed by BMJ. BMJ disclaims all liability and responsibility arising from any reliance placed on the content. Where the content includes any translated material, BMJ does not warrant the accuracy and reliability of the translations (including but not limited to local regulations, clinical guidelines, terminology, drug names and drug dosages), and is not responsible for any error and/or omissions arising from translation and adaptation or otherwise.
Read the full text or download the PDF:
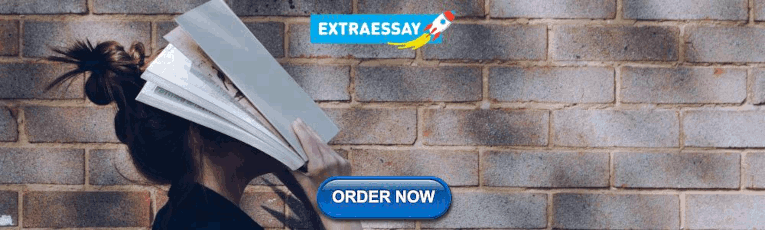
IMAGES
VIDEO
COMMENTS
In this study we propose a novel risk methodology assessment (RMA) that enables the user to visualize the assessment of individual or overall risks present in a specific trial. RMA follows the concept of failure mode and effect analysis, specifically a systematic failure mode and effect analysis. 19 The focus is on system-related deficiencies ...
The risk field has two main tasks, (I) to use risk assessments and risk management to study and treat the risk of specific activities (for example the operation of an offshore installation or an investment), and (II) to perform generic risk research and development, related to concepts, theories, frameworks, approaches, principles, methods and ...
Risk assessment and management was established as a scientific field some 30-40 years ago. Principles and methods were developed for how to conceptualise, assess and manage risk. These principles ...
The Harvard Center for Risk Analysis (HCRA) is a multidisciplinary group of faculty, research staff, students, and visiting scholars who work together to improve decisions about environmental health and other risks. We conduct state-of-the-art research, educate the next generation of leaders in risk analysis and related disciplines, and ...
For instance, Viljoen et al. (Citation 2018) reviewed studies evaluating the translation of risk assessment into risk management plans and concluded that there was often a weak link between the assessment and risk management plan. Firstly, in 22 of the 34 studies addressing adherence to the risk principle, the match was moderate, and in 5 ...
Conduct a risk assessment and vulnerability study to determine the risk factors. Determine the exposure factor (EF), which is the percentage of asset loss caused by the identified threat. Based on the risk factors determined in the value of tangible or intangible assets under risk, determine the SLE, which equals the asset value multiplied by ...
Risks and burdens in the study participation, as well as an adequate risk-benefit balance, are key concepts for the evaluation of clinical studies by research ethics committees (RECs). An adequate assessment and continuous monitoring to ensure compliance of risks and burdens in clinical trials have long been described as a central task in research ethics.
Conducting Risk-Benefit Assessments. Role of the Investigator: When designing research studies, investigators are responsible for conducting an initial risk-benefit assessment using the steps outlined in the diagram below. Role of the IRB: The IRB ultimately is responsible for evaluating the potential risks and weighing the probability of the risk occurring and the magnitude of harm that may ...
The risk assessment frameworks focused on holistic approaches, but most research studies did not adequately follow the risk perspectives. Therefore, research gaps were identified in the risk assessment process, and the areas were highlighted as state-of-the-art to conduct future research studies. ... The risk assessment is an important study in ...
Yet for three decades research has shown that clinicians' unaided assessments of "dangerousness" are barely better than chance. Rethinking Risk Assessment: The MacArthur Study of Mental Disorder and Violence tells the story of a pioneering investigation that challenges preconceptions about the frequency and nature of violence among ...
Risk Assessment • Is normally done for the entire study but can also be done: For a component of the study. For example: Modification of written consent for one population in the study Minors (child) determination Revision and whether it can be reviewed expedited 7 Risk Assessment for Devices • This is a different risk assessment for
Room 4S.6.2. Telephone: 01206 872944. Email: [email protected]. Telephone: 01206 874847. Email: [email protected]. Principal investigators and researchers must identify reasonably foreseeable risks associated with their research and control the risks so far as is reasonably practicable.
The level of risk can vary because of many factors including: the population included in the study, the situations encountered by the participants, and/ or the experience of the researcher or team. Two studies may appear similar but a few factors could make one inherently more risky than the other. This section describes what a researcher needs ...
Risk assessment studies are related to three research and activity streams: that of the epidemiological concept of population attributable fraction, or etiologic fraction, dating back from the mid-twentieth century [6,7,8], that of chemical risk assessment derived from toxicological studies , and the practice of environmental impact assessment ...
Under COVID-19 circumstances, however, studies with in-person components are now reviewed as higher risk, as person-to-person exposure is the most frequent route of transmission for infectious viruses. The IRB is required to assess the level of risk involved in a research study when making a determination for review requirements.
1 Define your research objectives. Before you start assessing risk, you need to have a clear idea of what you want to achieve with your research, and how you will measure your success. Your ...
According to the findings of Onay and Öztürk (2018), the most studied topics in credit risk-related research are "statistical methods and accuracy" and "new determinants of creditworthiness."Moreover, Louzada et al. (2016) discovered that the most common objective of the papers related to credit scoring was the suggestion of new techniques.
This finding has yet to be reasonably disputed in CCT studies and is an invaluable consideration for subsequent risk management (Bjørk & Hamilton, Citation 2011; Chaffey et al., ... Therefore, research that explores the risk assessment processes of nurses by gathering data in a group context may augment the findings of this study. Further ...
A risk assessment is a systematic process performed by a competent person which involves identifying, analyzing, and controlling hazards and risks present in a situation or a place. This decision-making tool aims to determine which measures should be put in place in order to eliminate or control those risks, as well as specify which of them ...
The present study found that the risk of developing liver cancer among Iranian children was 0.11100736 per 100,000 people, and in the Iranian adult population was 0.0314496 cancers per 100,000 ...
A new paper was recently published, Quantitative microbial risk assessment for ingestion of antibiotic resistance genes from private wells contaminated by human and livestock fecal sources. This study assesses risk of ingestion for several antibiotic resistance genes (ARGs) and the class 1 integron gene ( intI1) in drinking water from private ...
A new study highlights improvements in estimating the risk of common diseases when data from the body's gut microbiome is combined with genetic and other risk factors. Graphic by Jason Drees. Identifying those at highest risk for developing common chronic diseases like heart disease, diabetes, Alzheimer's disease and cancer is a core priority ...
FDA's National Retail Food Team designed the following resources to help state, local, territorial, and tribal jurisdictions complete a risk factor study as part of Standard 9 - Program ...
Shear wave velocity (Vs) has significant applications in geoengineering investigations. With the ongoing rise in carbon capture and storage (CCS) initiatives, the role of Vs in monitoring the CO2 sequestration sites is escalating. Although many studies have been conducted to assess CCS-induced risks, no inclusive research has been conducted integrating those investigations. This study strives ...
Objectives To examine the associations between changes in cardiorespiratory fitness (CRF) in adulthood and prostate cancer incidence and mortality. Methods In this prospective study, men who completed an occupational health profile assessment including at least two valid submaximal CRF tests, performed on a cycle ergometer, were included in the study. Data on prostate cancer incidence and ...