Help | Advanced Search
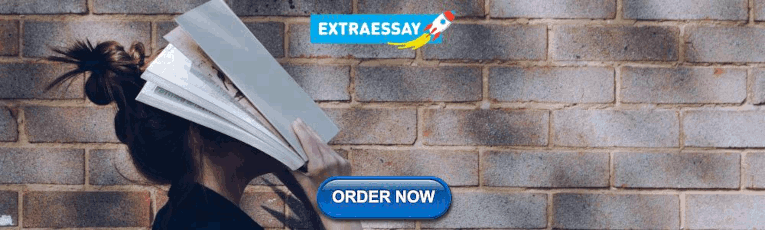
Electrical Engineering and Systems Science > Audio and Speech Processing
Title: speech dereverberation with a reverberation time shortening target.
Abstract: This work proposes a new learning target based on reverberation time shortening (RTS) for speech dereverberation. The learning target for dereverberation is usually set as the direct-path speech or optionally with some early reflections. This type of target suddenly truncates the reverberation, and thus it may not be suitable for network training. The proposed RTS target suppresses reverberation and meanwhile maintains the exponential decaying property of reverberation, which will ease the network training, and thus reduce signal distortion caused by the prediction error. Moreover, this work experimentally study to adapt our previously proposed FullSubNet speech denoising network to speech dereverberation. Experiments show that RTS is a more suitable learning target than direct-path speech and early reflections, in terms of better suppressing reverberation and signal distortion. FullSubNet is able to achieve outstanding dereverberation performance.
Submission history
Access paper:.
- Other Formats
References & Citations
- Google Scholar
- Semantic Scholar
BibTeX formatted citation

Bibliographic and Citation Tools
Code, data and media associated with this article, recommenders and search tools.
- Institution
arXivLabs: experimental projects with community collaborators
arXivLabs is a framework that allows collaborators to develop and share new arXiv features directly on our website.
Both individuals and organizations that work with arXivLabs have embraced and accepted our values of openness, community, excellence, and user data privacy. arXiv is committed to these values and only works with partners that adhere to them.
Have an idea for a project that will add value for arXiv's community? Learn more about arXivLabs .
- Help & FAQ
Single- and multi-microphone speech dereverberation using spectral enhancement
- Signal Processing Systems
- Adaptive array signal processing
Research output : Thesis › Phd Thesis 1 (Research TU/e / Graduation TU/e)
This output contributes to the following UN Sustainable Development Goals (SDGs)
Access to Document
- 10.6100/IR627677
- 200710970 Final published version, 6.68 MB
Fingerprint
- Microphone Engineering 100%
- Enhancement Engineering 100%
- Reverberation Engineering 100%
- Acoustics Physics 64%
- Models Engineering 42%
- Model Physics 42%
- Estimator Engineering 35%
- Distance Physics 35%
T1 - Single- and multi-microphone speech dereverberation using spectral enhancement
AU - Habets, E.A.P.
N2 - In speech communication systems, such as voice-controlled systems, hands-free mobile telephones, and hearing aids, the received microphone signals are degraded by room reverberation, background noise, and other interferences. This signal degradation may lead to total unintelligibility of the speech and decreases the performance of automatic speech recognition systems. In the context of this work reverberation is the process of multi-path propagation of an acoustic sound from its source to one or more microphones. The received microphone signal generally consists of a direct sound, reflections that arrive shortly after the direct sound (commonly called early reverberation), and reflections that arrive after the early reverberation (commonly called late reverberation). Reverberant speech can be described as sounding distant with noticeable echo and colouration. These detrimental perceptual effects are primarily caused by late reverberation, and generally increase with increasing distance between the source and microphone. Conversely, early reverberations tend to improve the intelligibility of speech. In combination with the direct sound it is sometimes referred to as the early speech component. Reduction of the detrimental effects of reflections is evidently of considerable practical importance, and is the focus of this dissertation. More specifically the dissertation deals with dereverberation techniques, i.e., signal processing techniques to reduce the detrimental effects of reflections. In the dissertation, novel single- and multimicrophone speech dereverberation algorithms are developed that aim at the suppression of late reverberation, i.e., at estimation of the early speech component. This is done via so-called spectral enhancement techniques that require a specific measure of the late reverberant signal. This measure, called spectral variance, can be estimated directly from the received (possibly noisy) reverberant signal(s) using a statistical reverberation model and a limited amount of a priori knowledge about the acoustic channel(s) between the source and the microphone(s). In our work an existing single-channel statistical reverberation model serves as a starting point. The model is characterized by one parameter that depends on the acoustic characteristics of the environment. We show that the spectral variance estimator that is based on this model, can only be used when the source-microphone distance is larger than the so-called critical distance. This is, crudely speaking, the distance where the direct sound power is equal to the total reflective power. A generalization of the statistical reverberation model in which the direct sound is incorporated is developed. This model requires one additional parameter that is related to the ratio between the direct sound energy and the sound energy of all reflections. The generalized model is used to derive a novel spectral variance estimator. When the novel estimator is used for dereverberation rather than the existing estimator, and the source-microphone distance is smaller than the critical distance, the dereverberation performance is significantly increased. Single-microphone systems only exploit the temporal and spectral diversity of the received signal. Reverberation, of course, also induces spatial diversity. To additionally exploit this diversity, multiple microphones must be used, and their outputs must be combined by a suitable spatial processor such as the so-called delay and sum beamformer. It is not a priori evident whether spectral enhancement is best done before or after the spatial processor. For this reason we investigate both possibilities, as well as a merge of the spatial processor and the spectral enhancement technique. An advantage of the latter option is that the spectral variance estimator can be further improved. Our experiments show that the use of multiple microphones affords a significant improvement of the perceptual speech quality. The applicability of the theory developed in this dissertation is demonstrated using a hands-free communication system. Since hands-free systems are often used in a noisy and reverberant environment, the received microphone signal does not only contain the desired signal but also interferences such as room reverberation that is caused by the desired source, background noise, and a far-end echo signal that results from a sound that is produced by the loudspeaker. Usually an acoustic echo canceller is used to cancel the far-end echo. Additionally a post-processor is used to suppress background noise and residual echo, i.e., echo which could not be cancelled by the echo canceller. In this work a novel structure and post-processor for an acoustic echo canceller are developed. The post-processor suppresses late reverberation caused by the desired source, residual echo, and background noise. The late reverberation and late residual echo are estimated using the generalized statistical reverberation model. Experimental results convincingly demonstrate the benefits of the proposed system for suppressing late reverberation, residual echo and background noise. The proposed structure and post-processor have a low computational complexity, a highly modular structure, can be seamlessly integrated into existing hands-free communication systems, and affords a significant increase of the listening comfort and speech intelligibility.
AB - In speech communication systems, such as voice-controlled systems, hands-free mobile telephones, and hearing aids, the received microphone signals are degraded by room reverberation, background noise, and other interferences. This signal degradation may lead to total unintelligibility of the speech and decreases the performance of automatic speech recognition systems. In the context of this work reverberation is the process of multi-path propagation of an acoustic sound from its source to one or more microphones. The received microphone signal generally consists of a direct sound, reflections that arrive shortly after the direct sound (commonly called early reverberation), and reflections that arrive after the early reverberation (commonly called late reverberation). Reverberant speech can be described as sounding distant with noticeable echo and colouration. These detrimental perceptual effects are primarily caused by late reverberation, and generally increase with increasing distance between the source and microphone. Conversely, early reverberations tend to improve the intelligibility of speech. In combination with the direct sound it is sometimes referred to as the early speech component. Reduction of the detrimental effects of reflections is evidently of considerable practical importance, and is the focus of this dissertation. More specifically the dissertation deals with dereverberation techniques, i.e., signal processing techniques to reduce the detrimental effects of reflections. In the dissertation, novel single- and multimicrophone speech dereverberation algorithms are developed that aim at the suppression of late reverberation, i.e., at estimation of the early speech component. This is done via so-called spectral enhancement techniques that require a specific measure of the late reverberant signal. This measure, called spectral variance, can be estimated directly from the received (possibly noisy) reverberant signal(s) using a statistical reverberation model and a limited amount of a priori knowledge about the acoustic channel(s) between the source and the microphone(s). In our work an existing single-channel statistical reverberation model serves as a starting point. The model is characterized by one parameter that depends on the acoustic characteristics of the environment. We show that the spectral variance estimator that is based on this model, can only be used when the source-microphone distance is larger than the so-called critical distance. This is, crudely speaking, the distance where the direct sound power is equal to the total reflective power. A generalization of the statistical reverberation model in which the direct sound is incorporated is developed. This model requires one additional parameter that is related to the ratio between the direct sound energy and the sound energy of all reflections. The generalized model is used to derive a novel spectral variance estimator. When the novel estimator is used for dereverberation rather than the existing estimator, and the source-microphone distance is smaller than the critical distance, the dereverberation performance is significantly increased. Single-microphone systems only exploit the temporal and spectral diversity of the received signal. Reverberation, of course, also induces spatial diversity. To additionally exploit this diversity, multiple microphones must be used, and their outputs must be combined by a suitable spatial processor such as the so-called delay and sum beamformer. It is not a priori evident whether spectral enhancement is best done before or after the spatial processor. For this reason we investigate both possibilities, as well as a merge of the spatial processor and the spectral enhancement technique. An advantage of the latter option is that the spectral variance estimator can be further improved. Our experiments show that the use of multiple microphones affords a significant improvement of the perceptual speech quality. The applicability of the theory developed in this dissertation is demonstrated using a hands-free communication system. Since hands-free systems are often used in a noisy and reverberant environment, the received microphone signal does not only contain the desired signal but also interferences such as room reverberation that is caused by the desired source, background noise, and a far-end echo signal that results from a sound that is produced by the loudspeaker. Usually an acoustic echo canceller is used to cancel the far-end echo. Additionally a post-processor is used to suppress background noise and residual echo, i.e., echo which could not be cancelled by the echo canceller. In this work a novel structure and post-processor for an acoustic echo canceller are developed. The post-processor suppresses late reverberation caused by the desired source, residual echo, and background noise. The late reverberation and late residual echo are estimated using the generalized statistical reverberation model. Experimental results convincingly demonstrate the benefits of the proposed system for suppressing late reverberation, residual echo and background noise. The proposed structure and post-processor have a low computational complexity, a highly modular structure, can be seamlessly integrated into existing hands-free communication systems, and affords a significant increase of the listening comfort and speech intelligibility.
U2 - 10.6100/IR627677
DO - 10.6100/IR627677
M3 - Phd Thesis 1 (Research TU/e / Graduation TU/e)
SN - 978-90-386-1544-8
PB - Technische Universiteit Eindhoven
CY - Eindhoven
- Study resources
- Calendar - Graduate
- Calendar - Undergraduate
- Class schedules
- Class cancellations
- Course registration
- Important academic dates
- More academic resources
- Campus services
- IT services
- Job opportunities
- Mental health support
- Student Service Centre (Birks)
- Calendar of events
- Latest news
- Media Relations
- Faculties, Schools & Colleges
- Arts and Science
- Gina Cody School of Engineering and Computer Science
- John Molson School of Business
- School of Graduate Studies
- All Schools, Colleges & Departments
- Directories
Spectrum Research Repository
- How to Deposit an Article
- How to Deposit an Article with a DOI
- Spectrum Deposit Checklist
- How to Prepare a Thesis for Deposit
- How to Deposit a Thesis
- How to Deposit a Research Creation Thesis
- How to Prepare a Graduate Project (Non-thesis) for Deposit
- How to Deposit a Graduate Project (Non-thesis)
- by Department
- by Document Type
- Spectrum and ORCID
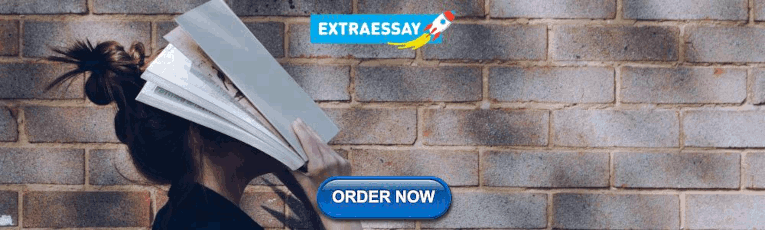
Speech Dereverberation Based on Multi-Channel Linear Prediction
Pu, Xinrui (2017) Speech Dereverberation Based on Multi-Channel Linear Prediction. Masters thesis, Concordia University.
Room reverberation can severely degrade the auditory quality and intelligibility of the speech signals received by distant microphones in an enclosed environment. In recent years, various dereverberation algorithms have been developed to tackle this problem, such as beamforming and inverse filtering of the room transfer function. However, this kind of methods relies heavily on the precise estimation of either the direction of arrival (DOA) or room acoustic characteristics. Thus, their performance is very much limited. A more promising category of dereverberation algorithms has been developed based on multi-channel linear predictor (MCLP). This idea was first proposed in time domain where speech signal is highly correlated in a short period of time. To ensure a good suppression of the reverberation, the prediction filter length is required to be longer than the reverberation time. As a result, the complexity of this algorithm is often unacceptable because of large covariance matrix calculation. To overcome this disadvantage, this thesis focuses on the MCLP dereverberation methods performed in the short-time Fourier transform (STFT) domain. Recently, the weighted prediction error (WPE) algorithm has been developed and widely applied to speech dereverberation. In WPE algorithm, MCLP is used in the STFT domain to estimate the late reverberation components from previous frames of the reverberant speech. The enhanced speech is obtained by subtracting the late reverberation from the reverberant speech. Each STFT coefficient is assumed to be independent and obeys Gaussian distribution. A maximum likelihood (ML) problem is formulated in each frequency bin to calculate the predictor coefficients. In this thesis, the original WPE algorithm is improved in two aspects. First, two advanced statistical models, generalized Gaussian distribution (GGD) and Laplacian distribution, are employed instead of the classic Gaussian distribution. Both of them are shown to give better modeling of the histogram of the clean speech. Second, we focus on improving the estimation of the variances of the STFT coefficients of the desired signal. In the original WPE algorithm, the variances are estimated in each frequency bin independently without considering the cross-frequency correlation. Thus, we integrate the nonnegative matrix factorization (NMF) into the WPE algorithm to refine the estimation of the variances and hence obtain a better dereverberation performance. Another category of MCLP based dereverberation algorithm has been proposed in literature by exploiting the sparsity of the STFT coefficients of the desired signal for calculating the predictor coefficients. In this thesis, we also investigate an efficient algorithm based on the maximization of the group sparsity of desired signal using mixed norms. Inspired by the idea of sparse linear predictor (SLP), we propose to include a sparse constraint for the predictor coefficients in order to further improve the dereverberation performance. A weighting parameter is also introduced to achieve a trade-off between the sparsity of the desired signal and the predictor coefficients. Computer simulation of the proposed dereverberation algorithms is conducted. Our experimental results show that the proposed algorithms can significantly improve the quality of reverberant speech signal under different reverberation times. Subjective evaluation also gives a more intuitive demonstration of the enhanced speech intelligibility. Performance comparison also shows that our algorithms outperform some of the state-of-the-art dereverberation techniques.
Repository Staff Only: item control page

Downloads per month over past year
View more statistics
Speech Dereverberation Using Statistical Reverberation Models
Cite this chapter.
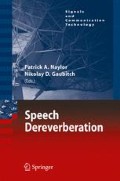
- Emanuël A. P. Habets 2
Part of the book series: Signals and Commmunication Technology ((SCT))
1618 Accesses
18 Citations
In speech communication systems, such as voice-controlled systems, hands-free mobile telephones and hearing aids, the received microphone signals are degraded by room reverberation, ambient noise and other interferences. This signal degradation can decrease the fidelity and intelligibility of speech and the word recognition rate of automatic speech recognition systems.
The reverberation process is often described using deterministic models that depend on a large number of unknown parameters. These parameters are often difficult to estimate blindly and are dependent on the exact spatial position of the source and receiver. In recently emerged speech dereverberation methods, which are feasible in practice, the reverberation process is described using a statistical model. This model depends on smaller number of parameters such as the reverberation time of the enclosure, which can be assumed to be independent of the spatial location of the source and receiver. This model can be utilized to estimate the spectral variance of part of the reverberant signal component. Together with an estimate of the spectral variance of the ambient noise, this estimate can then be used to enhance the observed noisy and reverberant speech.
In this chapter we provide a brief overview of dereverberation methods. We then describe single and multiple microphone algorithms that are able to jointly suppress reverberation and ambient noise. Finally, experimental results demonstrate the beneficial use of the algorithms developed.
This is a preview of subscription content, log in via an institution to check access.
Access this chapter
- Available as PDF
- Read on any device
- Instant download
- Own it forever
- Compact, lightweight edition
- Dispatched in 3 to 5 business days
- Free shipping worldwide - see info
- Durable hardcover edition
Tax calculation will be finalised at checkout
Purchases are for personal use only
Institutional subscriptions
Unable to display preview. Download preview PDF.
Abramson, A., Cohen, I.: Markov-switching GARCH model and application to speech enhancement in subbands. In: Proc. Int. Workshop Acoust. Echo Noise Control (IWAENC), pp. 1–4. Paris, France (2006)
Google Scholar
Abramson, A., Cohen, I.: Recursive supervised estimation of a Markov-switching GARCH process in the short-time Fourier transform domain. IEEE Trans. Signal Process. 55 (7), 3227–3238 (2007)
Article MathSciNet Google Scholar
Accardi, A.J., Cox, R.V.: A modular approach to speech enhancement with an application to speech coding. In: Proc. IEEE Int. Conf. on Acoustics, Speech and Signal Processing (ICASSP), vol. 1, pp. 201–204 (1999)
Allen, J.B.: Effects of small room reverberation on subjective preference. J. Acoust. Soc. Am. 71 (S1), S5 (1982)
Article Google Scholar
Allen, J.B., Berkley, D.A.: Image method for efficiently simulating small-room acoustics. J. Acoust. Soc. Am. 65 (4), 943–950 (1979)
Benesty, J., Makino, S., Chen, J. (eds.): Speech Enhancement. Springer (2005)
Benesty, J., Sondhi, M.M., Huang, Y. (eds.): Springer Handbook of Speech Processing. Springer (2007)
Berouti, M., Schwartz, R., Makhoul, J.: Enhancement of speech corrupted by acoustic noise. In: Proc. IEEE Int. Conf. on Acoustics, Speech and Signal Processing (ICASSP), vol. 4, pp. 208–211 (1979)
Boll, S.F.: Suppression of acoustic noise in speech using spectral subtraction. IEEE Trans. Acoust., Speech, Signal Process. ASSP-27 (2), 113–120 (1979)
Bolt, R.H., MacDonald, A.D.: Theory of speech masking by reverberation. J. Acoust. Soc. Am. 21 , 577–580 (1949)
Burshtein, D., Gannot, S.: Speech enhancement using a mixture-maximum model. IEEE Trans. Speech Audio Process. 10 (6), 341351 (2002)
Cappe, O.: Elimination of the musical noise phenomenon with the Ephraim and Malah noise suppressor. IEEE Trans. Speech Audio Process. 2 (2), 345–349 (1994). DOI 10.1109/89. 279283
Cohen, I.: Optimal speech enhancement under signal presence uncertainty using log-spectral amplitude estimator. IEEE Signal Process. Lett. 9 (4), 113–116 (2002)
Cohen, I.: Noise spectrum estimation in adverse environments: Improved minima controlled recursive averaging. IEEE Trans. Speech Audio Process. 11 (5), 466–475 (2003). DOI 10. 1109/TSA.2003.811544
Cohen, I.: From volatility modeling of financial time-series to stochastic modeling and enhancement of speech signals. In: J. Benesty, S. Makino, J. Chen (eds.) Speech Enhancement, chap. 5, pp. 97–114. Springer (2005)
Cohen, I.: Speech spectral modeling and enhancement based on autoregressive conditional heteroscedasticity models. Signal Processing 86 (4), 698–709 (2006)
Article MATH Google Scholar
Cohen, I., Gannot, S.: Spectral enhancement methods. In: Benesty et al. [7], chap. 45. Part H
Cohen, I., Gannot, S., Berdugo, B.: An integrated real-time beamforming and post filtering system for nonstationary noise environments. EURASIP J. on App. Signal Process. 11 , 1064–1073 (2003)
Cox, T.J., Li, F., Darlington, P.: Extracting room reverberation time from speech using artificial neural networks. J. Audio Eng. Soc. 49 (4), 219–230 (2001)
Crochiere, R.E., Rabiner, L.R.: Multirate Digital Signal Processing. Prentice-Hall (1983)
Delcroix, M., Hikichi, T., Miyoshi, M.: Precise dereverberation using multichannel linear prediction. IEEE Trans. Audio, Speech, Lang. Process. 15 (2), 430–440 (2007)
Deller, J.R., Proakis, J.G., Hansen, J.H.L.: Discrete-Time Processing of Speech Signals. New York: MacMillan (1993)
Ephraim, Y., Cohen, I.: Recent advancements in speech enhancement. In: R.C. Dorf (ed.) The Electrical Engineering Handbook, Circuits, Signals, and Speech and Image Processing, third edn. CRC Press (2006)
Ephraim, Y., Lev-Ari, H., Roberts, W.J.J.: A brief survey of speech enhancement. In: The Electronic Handbook, second edn. CRC Press (2005)
Ephraim, Y., Malah, D.: Speech enhancement using a minimum-mean square error short-time spectral amplitude estimator. IEEE Trans. Acoust., Speech, Signal Process. 32 (6), 1109–1121 (1984)
Ephraim, Y., Malah, D.: Speech enhancement using a minimum mean-square error logspectral amplitude estimator. IEEE Trans. Acoust., Speech, Signal Process. 33 (2), 443–445 (1985)
Gannot, S., Cohen, I.: Adaptive beamforming and postfiltering. In: Benesty et al. [7], chap. 48
Gannot, S., Moonen, M.: Subspace methods for multimicrophone speech dereverberation. EURASIP J. on App. Signal Process. 2003 (11), 1074–1090 (2003)
Gaubitch, N.D., Naylor, P.A.: Analysis of the dereverberation performance of microphone arrays. In: Proc. Int. Workshop Acoust. Echo Noise Control (IWAENC) (2005)
Gaubitch, N.D., Naylor, P.A., Ward, D.B.: On the use of linear prediction for dereverberation of speech. In: Proc. Int. Workshop Acoust. Echo Noise Control (IWAENC), pp. 99–102 (2003)
Goh, Z., Tan, K.C., Tan, T.G.: Postprocessing method for suppressing musical noise generated by spectral subtraction. IEEE Trans. Speech Audio Process. 6 (3), 287–292 (1998). DOI 10.1109/89.668822
Griebel, S.M., Brandstein, M.S.: Wavelet transform extrema clustering for multi-channel speech dereverberation. In: Proc. Int. Workshop Acoust. Echo Noise Control (IWAENC), pp. 52–55. Pocono Manor, Pennsylvania (1999)
Gürelli, M.I., Nikias, C.L.: EVAM: An eigenvector-based algorithm for multichannel blind deconvolution of input colored signals. IEEE Trans. Signal Process. 43 (1), 134–149 (1995)
Gustafsson, S., Martin, R., Jax, P., Vary, P.: A psychoacoustic approach to combined acoustic echo cancellation and noise reduction. IEEE Trans. Speech Audio Process. 10 (5), 245–256 (2002)
Gustafsson, S., Martin, R., Vary, P.: Combined acoustic echo control and noise reduction for hands-free telephony. Signal Processing 64 (1), 21–32 (1998)
Gustafsson, S., Nordholm, S., Claesson, I.: Spectral subtraction using reduced delay convolution and adaptive averaging. IEEE Trans. Speech Audio Process. 9 (8), 799–807 (2001)
Habets, E.A.P.: Multi-channel speech dereverberation based on a statistical model of late reverberation. In: Proc. IEEE Int. Conf. on Acoustics, Speech and Signal Processing (ICASSP), pp. 173–176. Philadelphia, USA (2005)
Habets, E.A.P.: Speech dereverberation based on a statistical model of late reverberation using a linear microphone array. In: Proc. Joint Workshop on Hands-Free Speech Communication and Microphone Arrays (HSCMA), pp. d7–d8. Piscataway, NJ, USA (2005)
Habets, E.A.P.: Single- and multi-microphone speech dereverberation using spectral enhancement. Ph.D. thesis, Technische Universiteit Eindhoven (2007)
Habets, E.A.P., Cohen, I., Gannot, S.: MMSE log spectral amplitude estimator for multiple interferences. In: Proc. Int. Workshop Acoust. Echo Noise Control (IWAENC), pp. 1–4. Paris, France (2006)
Habets, E.A.P., Cohen, I., Gannot, S., Sommen, P.C.W.: Joint dereverberation and residual echo suppression of speech signals in noisy environments. IEEE Trans. Audio, Speech, Lang. Process. 16 (8), 1433–1451 (2008)
Habets, E.A.P., Gannot, S., Cohen, I.: Dual-microphone speech dereverberation in a noisy environment. In: Proc. IEEE Int. Symposium on Signal Processing and Information Technology (ISSPIT), pp. 651–655. Vancouver, Canada (2006)
Haykin, S.: Blind Deconvolution, fourth edn. Prentice-Hall Information and System Sciences. Prentice-Hall (1994)
Hopgood, J.: Nonstationary signal processing with application to reverberation cancellation in acoustic environments. Ph.D. thesis, Cambridge University (2001)
Huang, Y., Benesty, J.: A class of frequency-domain adaptive approaches to blind multichannel identification. IEEE Trans. Signal Process. 51 (1), 11–24 (2003)
Jetzt, J.J.: Critical distance measurement of rooms from the sound energy spectral response. J. Acoust. Soc. Am. 65 (5), 1204–1211 (1979)
Jot, J.M., Cerveau, L., Warusfel, O.: Analysis and synthesis of room reverberation based on a statistical time-frequency model. In: Proc. Audio Eng. Soc. Convention (1997)
Kuttruff, H.: Room Acoustics, 4th edn. Taylor & Frances (2000)
Lebart, K., Boucher, J.M., Denbigh, P.N.: A new method based on spectral subtraction for speech dereverberation. Acta Acoustica 87 , 359–366 (2001)
Lim, J.S., Oppenheim, A.V.: Enhancement and bandwidth compression of noisy speech. Proc. IEEE 67 (12), 1586–1604 (1979)
Lindsey, G., Breen, A., Nevard, S.: SPAR’s archivable actual-word databases. Technical report, University College London (1987)
Loizou, P.C.: Speech enhancement based on perceptually motivated Bayesian estimators of the magnitude spectrum. IEEE Trans. Speech Audio Process. 13 (5), 857–869 (2005). DOI 10.1109/TSA.2005.851929
Löllmann, H.W., Vary, P.: Estimation of the reverberation time in noisy environments. In: Proc. Int. Workshop Acoust. Echo Noise Control (IWAENC), pp. 1–4 (2008)
Martin, R.: Noise power spectral density estimation based on optimal smoothing and minimum statistics. IEEE Trans. Speech Audio Process. 9 , 504–512 (2001). DOI 10.1109/89.928915
Martin, R.: Speech enhancement based on minimum mean-square error estimation and supergaussian priors. IEEE Trans. Speech Audio Process. 13 (5), 845–856 (2005). DOI 10.1109/TSA.2005.851927
Miyoshi, M., Kaneda, Y.: Inverse filtering of room acoustics. IEEE Trans. Acoust., Speech, Signal Process. 36 (2), 145–152 (1988)
Nábˇelek, A.K., Letowski, T.R., Tucker, F.M.: Reverberant overlap- and self-masking in consonant identification. J. Acoust. Soc. Am. 86 (4), 1259–1265 (1989)
Nábˇelek, A.K., Mason, D.: Effect of noise and reverberation on binaural and monaural word identification by subjects with various audiograms. J. Speech Hear. Res. 24 , 375–383 (1981)
Peterson, P.M.: Simulating the response of multiple microphones to a single acoustic source in a reverberant room. J. Acoust. Soc. Am. 80 (5), 1527–1529 (1986)
Peutz, V.M.A.: Articulation loss of consonants as a criterion for speech transmission in a room. J. Audio Eng. Soc. 19 (11), 915–919 (1971)
Polack, J.D.: La transmission de l’énergie sonore dans les salles. Ph.D. thesis, Université du Maine, La Mans, France (1988)
Polack, J.D.: Playing billiards in the concert hall: the mathematical foundations of geometrical room acoustics. Appl. Acoust. 38 (2), 235–244 (1993)
Radlovi´c, B.D., Kennedy, R.A.: Nonminimum-phase equalization and its subjective importance in room acoustics. IEEE Trans. Speech Audio Process. 8 (6), 728–737 (2000)
Ratnam, R., Jones, D.L., Wheeler, B.C., O’Brien, Jr., W.D., Lansing, C.R., Feng, A.S.: Blind estimation of reverberation time. J. Acoust. Soc. Am. 114 (5), 2877–2892 (2003)
Sabine, W.C.: Collected Papers on acoustics (Originally 1921). Peninsula Publishing (1993)
Schroeder, M.R.: Statistical parameters of the frequency response curves of large rooms. J. Audio Eng. Soc. 35 , 299–306 (1954)
MathSciNet Google Scholar
Schroeder, M.R.: Frequency correlation functions of frequency responses in rooms. J. Acoust. Soc. Am. 34 (12), 1819–1823 (1962)
Schroeder, M.R.: Integrated-impulse method measuring sound decay without using impulses. J. Acoust. Soc. Am. 66 (2), 497–500 (1979)
Schroeder, M.R.: The “schroeder frequency” revisited. J. Acoust. Soc. Am. 99 (5), 3240–3241 (1996). DOI 10.1121/1.414868
Sim, B.L., Tong, Y.C., Chang, J.S., Tan, C.T.: A parametric formulation of the generalized spectral subtraction method. IEEE Trans. Speech Audio Process. 6 (4), 328–337 (1998)
Steinberg, J.C.: Effects of distortion upon the recognition of speech sounds. J. Acoust. Soc. Am. 1 , 35–35 (1929)
Takata, Y., Nábˇelek, A.K.: English consonant recognition in noise and in reverberation by Japanese and American listeners. J. Acoust. Soc. Am. 88 , 663–666 (1990)
Talantzis, F., Ward, D.B.: Robustness of multichannel equalization in an acoustic reverberant environment. J. Acoust. Soc. Am. 114 (2), 833–841 (2003)
Tashev, I., Malvar, H.S.: A new beamformer design algorithm for microphone arrays. In: Proc. IEEE Int. Conf. on Acoustics, Speech and Signal Processing (ICASSP), vol. 3, pp. iii/101–iii/104 (2005)
Varga, A., Steeneken, H.J.M.: Assessment for automatic speech recognition II: NOISEX-92: a database and an experiment to study the effect of additive noise on speech recognition systems. Speech Communication 3 (3), 247–251 (1993). DOI 10.1016/0167--6393(93)90095--3
Wexler, J., Raz, S.: Discrete Gabor expansions. Signal Processing 21 (3), 207–220 (1990)
Wolfe, P.J., Godsill, S.J.: Efficient alternatives to the Ephraim and Malah suppression rule for audio signal enhancement. EURASIP J. on App. Signal Process. 2003 (10), 1043–1051 (2003)
Yegnanarayana, B., Satyanarayana, P.: Enhancement of reverberant speech using LP residual signal. IEEE Trans. Speech Audio Process. 8 (3), 267–281 (2000)
Download references
Author information
Authors and affiliations.
Imperial College London, London, UK
Emanuël A. P. Habets
You can also search for this author in PubMed Google Scholar
Editor information
Editors and affiliations.
Department of Electrical and Electronic Engineering, Imperial College London, Exhibition Road, SW7 2AZ, London, UK
Patrick A. Naylor & Nikolay D. Gaubitch &
Rights and permissions
Reprints and permissions
Copyright information
© 2010 Springer-Verlag London Limited
About this chapter
Habets, E. (2010). Speech Dereverberation Using Statistical Reverberation Models. In: Naylor, P., Gaubitch, N. (eds) Speech Dereverberation. Signals and Commmunication Technology. Springer, London. https://doi.org/10.1007/978-1-84996-056-4_3
Download citation
DOI : https://doi.org/10.1007/978-1-84996-056-4_3
Publisher Name : Springer, London
Print ISBN : 978-1-84996-055-7
Online ISBN : 978-1-84996-056-4
eBook Packages : Engineering Engineering (R0)
Share this chapter
Anyone you share the following link with will be able to read this content:
Sorry, a shareable link is not currently available for this article.
Provided by the Springer Nature SharedIt content-sharing initiative
- Publish with us
Policies and ethics
- Find a journal
- Track your research
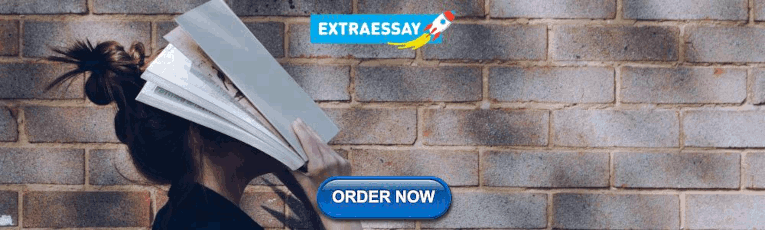
IMAGES
VIDEO
COMMENTS
Following the speech enhancement method in [19], the time-frequency (T-F) representation, the spectrogram, can be treated as an image. Consequentially, the enhancement task becomes an image-to-image transformation. Treating the re-verberant speech as an image has two major advantages. First, speech spectrograms exhibit typical patterns (e.g ...
The main objective of the thesis is to implement a framework to improve the ASR results for a distant, multi-channel recording corrupted by reverberation and background noise, and performs better than existing C-NMF based methods in objec-tive measures, such as cepstral distance and speech-to-reverberation modulation energy ratio (SRMR). Expand.
First, we extend deep learning based magnitude-domain single-channel speech dereverberation to the complex domain for phase estimation. where Sq(t, f) ∈ C are the complex STFT values of the direct-path signal of the target speaker captured by a reference mi-. P crophone q at time t and frequency f, (f; q) ×1 c ∈ C is the relative transfer ...
In this thesis, we focus on robust dereverberation methods for online processing as required in real-time speech communication systems. To achieve dereverberation, two main aspects can be ...
An Overview of Speech Dereverberation 135. wherey(n) is the reverberant speech,h(n) is the RIR function, andx(n)isthe dean speech. Eliminating the influence of convolution is the main task of speech dereverberation. According to whether RIR needs to be estimated, algorithms using the sta- tistical acoustic model can be divided into two ...
A. Enhancement and dereverberation For speech enhancement, Xu et. al. [24] devised a mapping from noisy speech to clean speech using a supervised neural network. In a similar manner, ideal ratio mask based neural mappings [25] have been explored for speech separation tasks. On the dereverberation front, Zhao et. al. proposed an LSTM
This work proposes a new learning target based on reverberation time shortening (RTS) for speech dereverberation. The learning target for dereverberation is usually set as the direct-path speech or optionally with some early reflections. This type of target suddenly truncates the reverberation, and thus it may not be suitable for network training. The proposed RTS target suppresses ...
where y(n) is the reverberant speech, h(n) is the RIR function, and x(n) is the dean speech.Eliminating the influence of convolution is the main task of speech dereverberation. According to whether RIR needs to be estimated, algorithms using the statistical acoustic model can be divided into two categories: reverberation cancellation and reverberation suppression, they are discussed separately ...
This thesis focuses on the MCLP dereverberation methods performed in the short-time Fourier transform (STFT) domain and investigates an efficient algorithm based on the maximization of the group sparsity of desired signal using mixed norms. Room reverberation can severely degrade the auditory quality and intelligibility of the speech signals received by distant microphones in an enclosed ...
Speech Dereverberation Based on Multi-Channel Linear Prediction Xinrui Pu A Thesis in The Department of Electrical and Computer Engineering Presented in Partial Fulfillment of the Requirements for the Degree of Master of Applied Science Concordia University
Abstract. Speech interaction technology is becoming increasingly popular in practical voice-driven applications. However, due to the inferences caused by reverberation in real-world environments ...
About this book. Speech Dereverberation gathers together an overview, a mathematical formulation of the problem and the state-of-the-art solutions for dereverberation. Speech Dereverberation presents current approaches to the problem of reverberation. It provides a review of topics in room acoustics and also describes performance measures for ...
The block diagram of the proposed speech dereverberation method based on the SS and spectral line enhancement is shown in Fig. 1.There are nine modules in the proposed scheme, including windowing & short-time Fourier transform (STFT), reverberation time (RT) estimation, late reverberation estimation, SS gain computation, invert STFT, voice activity detection (VAD), energy attenuation, spectral ...
The goal is to analyze each type of algorithms' advantages and disadvantages and provide the necessary background to the readers who are going to devote themselves to making progress in this area. Speech dereverberation is an important preprocessing step in speech signal processing, aims at improving the sound quality by canceling or suppressing the effect of reverb.
tion deals with dereverberation techniques, i.e., signal processing techniques to reduce the detrimental effects of reflections. In the dissertation, novel single- and multi-microphone speech dereverberation algorithms are developed that aim at the suppres-sion of late reverberation, i.e., at estimation of the early speech component. This is
Single Channel Speech Dereverberation by Arnau Torrent Florensa Master ofScience thesis Project period: February 2005 - June 2005 Report Number: 18-05.Supervisors: Name (TV/e): Dr.ir. P.C.W. Sommenenir. E.A.P. Habets Name Universitat Politecnica de Catalunya The Department ofElectrical Engineering of the Eindhoven University ofTechnology accepts no
Doire CSJ (2016) Single-channel enhancement of speech corrupted by reverberation and noise. Ph.D. Thesis, Imperial College, London, UK ... Mitianoudis Nikolaos (2019) A novel scheme for single-channel speech dereverberation. Acoustics 1:711-725. Article Google Scholar Schroeder MR (1965) Apparatus for suppressing noise and distortion in ...
In the dissertation, novel single- and multimicrophone speech dereverberation algorithms are developed that aim at the suppression of late reverberation, i.e., at estimation of the early speech component. ... M3 - Phd Thesis 1 (Research TU/e / Graduation TU/e) SN - 978-90-386-1544-8. PB - Technische Universiteit Eindhoven. CY - Eindhoven. ER -
This paper introduces a distributed speech dereverberation method that emphasizes low computational complexity at each node. Specifically, we leverage the distributed adaptive node-specific signal estimation (DANSE) algorithm within the multichannel linear prediction (MCLP) process. This approach empowers each node to perform local operations ...
Room reverberation can severely degrade the auditory quality and intelligibility of the speech signals received by distant microphones in an enclosed environment. In recent years, various dereverberation algorithms have been developed to tackle this problem, such as beamforming and inverse filtering of the room transfer function. However, this kind of methods relies heavily on the precise ...
The objective of the thesis is to find an approach to dereverberate the reverberated signals which degrade the quality of a speech signal. This thesis is proposed to find out the way in which we can improve the single channel speech dereverberation using both blind and non blind approaches.
Abstract. In speech communication systems, such as voice-controlled systems, hands-free mobile telephones and hearing aids, the received microphone signals are degraded by room reverberation, ambient noise and other interferences. This signal degradation can decrease the fidelity and intelligibility of speech and the word recognition rate of ...