Have a language expert improve your writing
Run a free plagiarism check in 10 minutes, generate accurate citations for free.
- Knowledge Base
Methodology
- Systematic Review | Definition, Example, & Guide
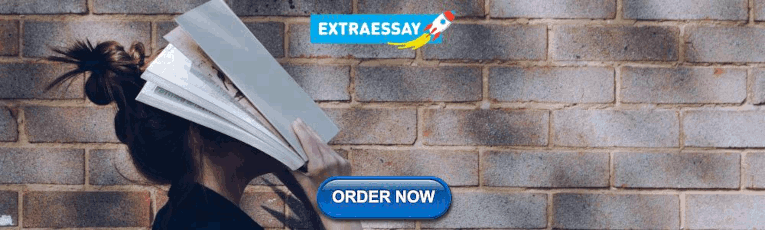
Systematic Review | Definition, Example & Guide
Published on June 15, 2022 by Shaun Turney . Revised on November 20, 2023.
A systematic review is a type of review that uses repeatable methods to find, select, and synthesize all available evidence. It answers a clearly formulated research question and explicitly states the methods used to arrive at the answer.
They answered the question “What is the effectiveness of probiotics in reducing eczema symptoms and improving quality of life in patients with eczema?”
In this context, a probiotic is a health product that contains live microorganisms and is taken by mouth. Eczema is a common skin condition that causes red, itchy skin.
Table of contents
What is a systematic review, systematic review vs. meta-analysis, systematic review vs. literature review, systematic review vs. scoping review, when to conduct a systematic review, pros and cons of systematic reviews, step-by-step example of a systematic review, other interesting articles, frequently asked questions about systematic reviews.
A review is an overview of the research that’s already been completed on a topic.
What makes a systematic review different from other types of reviews is that the research methods are designed to reduce bias . The methods are repeatable, and the approach is formal and systematic:
- Formulate a research question
- Develop a protocol
- Search for all relevant studies
- Apply the selection criteria
- Extract the data
- Synthesize the data
- Write and publish a report
Although multiple sets of guidelines exist, the Cochrane Handbook for Systematic Reviews is among the most widely used. It provides detailed guidelines on how to complete each step of the systematic review process.
Systematic reviews are most commonly used in medical and public health research, but they can also be found in other disciplines.
Systematic reviews typically answer their research question by synthesizing all available evidence and evaluating the quality of the evidence. Synthesizing means bringing together different information to tell a single, cohesive story. The synthesis can be narrative ( qualitative ), quantitative , or both.
Here's why students love Scribbr's proofreading services
Discover proofreading & editing
Systematic reviews often quantitatively synthesize the evidence using a meta-analysis . A meta-analysis is a statistical analysis, not a type of review.
A meta-analysis is a technique to synthesize results from multiple studies. It’s a statistical analysis that combines the results of two or more studies, usually to estimate an effect size .
A literature review is a type of review that uses a less systematic and formal approach than a systematic review. Typically, an expert in a topic will qualitatively summarize and evaluate previous work, without using a formal, explicit method.
Although literature reviews are often less time-consuming and can be insightful or helpful, they have a higher risk of bias and are less transparent than systematic reviews.
Similar to a systematic review, a scoping review is a type of review that tries to minimize bias by using transparent and repeatable methods.
However, a scoping review isn’t a type of systematic review. The most important difference is the goal: rather than answering a specific question, a scoping review explores a topic. The researcher tries to identify the main concepts, theories, and evidence, as well as gaps in the current research.
Sometimes scoping reviews are an exploratory preparation step for a systematic review, and sometimes they are a standalone project.
Prevent plagiarism. Run a free check.
A systematic review is a good choice of review if you want to answer a question about the effectiveness of an intervention , such as a medical treatment.
To conduct a systematic review, you’ll need the following:
- A precise question , usually about the effectiveness of an intervention. The question needs to be about a topic that’s previously been studied by multiple researchers. If there’s no previous research, there’s nothing to review.
- If you’re doing a systematic review on your own (e.g., for a research paper or thesis ), you should take appropriate measures to ensure the validity and reliability of your research.
- Access to databases and journal archives. Often, your educational institution provides you with access.
- Time. A professional systematic review is a time-consuming process: it will take the lead author about six months of full-time work. If you’re a student, you should narrow the scope of your systematic review and stick to a tight schedule.
- Bibliographic, word-processing, spreadsheet, and statistical software . For example, you could use EndNote, Microsoft Word, Excel, and SPSS.
A systematic review has many pros .
- They minimize research bias by considering all available evidence and evaluating each study for bias.
- Their methods are transparent , so they can be scrutinized by others.
- They’re thorough : they summarize all available evidence.
- They can be replicated and updated by others.
Systematic reviews also have a few cons .
- They’re time-consuming .
- They’re narrow in scope : they only answer the precise research question.
The 7 steps for conducting a systematic review are explained with an example.
Step 1: Formulate a research question
Formulating the research question is probably the most important step of a systematic review. A clear research question will:
- Allow you to more effectively communicate your research to other researchers and practitioners
- Guide your decisions as you plan and conduct your systematic review
A good research question for a systematic review has four components, which you can remember with the acronym PICO :
- Population(s) or problem(s)
- Intervention(s)
- Comparison(s)
You can rearrange these four components to write your research question:
- What is the effectiveness of I versus C for O in P ?
Sometimes, you may want to include a fifth component, the type of study design . In this case, the acronym is PICOT .
- Type of study design(s)
- The population of patients with eczema
- The intervention of probiotics
- In comparison to no treatment, placebo , or non-probiotic treatment
- The outcome of changes in participant-, parent-, and doctor-rated symptoms of eczema and quality of life
- Randomized control trials, a type of study design
Their research question was:
- What is the effectiveness of probiotics versus no treatment, a placebo, or a non-probiotic treatment for reducing eczema symptoms and improving quality of life in patients with eczema?
Step 2: Develop a protocol
A protocol is a document that contains your research plan for the systematic review. This is an important step because having a plan allows you to work more efficiently and reduces bias.
Your protocol should include the following components:
- Background information : Provide the context of the research question, including why it’s important.
- Research objective (s) : Rephrase your research question as an objective.
- Selection criteria: State how you’ll decide which studies to include or exclude from your review.
- Search strategy: Discuss your plan for finding studies.
- Analysis: Explain what information you’ll collect from the studies and how you’ll synthesize the data.
If you’re a professional seeking to publish your review, it’s a good idea to bring together an advisory committee . This is a group of about six people who have experience in the topic you’re researching. They can help you make decisions about your protocol.
It’s highly recommended to register your protocol. Registering your protocol means submitting it to a database such as PROSPERO or ClinicalTrials.gov .
Step 3: Search for all relevant studies
Searching for relevant studies is the most time-consuming step of a systematic review.
To reduce bias, it’s important to search for relevant studies very thoroughly. Your strategy will depend on your field and your research question, but sources generally fall into these four categories:
- Databases: Search multiple databases of peer-reviewed literature, such as PubMed or Scopus . Think carefully about how to phrase your search terms and include multiple synonyms of each word. Use Boolean operators if relevant.
- Handsearching: In addition to searching the primary sources using databases, you’ll also need to search manually. One strategy is to scan relevant journals or conference proceedings. Another strategy is to scan the reference lists of relevant studies.
- Gray literature: Gray literature includes documents produced by governments, universities, and other institutions that aren’t published by traditional publishers. Graduate student theses are an important type of gray literature, which you can search using the Networked Digital Library of Theses and Dissertations (NDLTD) . In medicine, clinical trial registries are another important type of gray literature.
- Experts: Contact experts in the field to ask if they have unpublished studies that should be included in your review.
At this stage of your review, you won’t read the articles yet. Simply save any potentially relevant citations using bibliographic software, such as Scribbr’s APA or MLA Generator .
- Databases: EMBASE, PsycINFO, AMED, LILACS, and ISI Web of Science
- Handsearch: Conference proceedings and reference lists of articles
- Gray literature: The Cochrane Library, the metaRegister of Controlled Trials, and the Ongoing Skin Trials Register
- Experts: Authors of unpublished registered trials, pharmaceutical companies, and manufacturers of probiotics
Step 4: Apply the selection criteria
Applying the selection criteria is a three-person job. Two of you will independently read the studies and decide which to include in your review based on the selection criteria you established in your protocol . The third person’s job is to break any ties.
To increase inter-rater reliability , ensure that everyone thoroughly understands the selection criteria before you begin.
If you’re writing a systematic review as a student for an assignment, you might not have a team. In this case, you’ll have to apply the selection criteria on your own; you can mention this as a limitation in your paper’s discussion.
You should apply the selection criteria in two phases:
- Based on the titles and abstracts : Decide whether each article potentially meets the selection criteria based on the information provided in the abstracts.
- Based on the full texts: Download the articles that weren’t excluded during the first phase. If an article isn’t available online or through your library, you may need to contact the authors to ask for a copy. Read the articles and decide which articles meet the selection criteria.
It’s very important to keep a meticulous record of why you included or excluded each article. When the selection process is complete, you can summarize what you did using a PRISMA flow diagram .
Next, Boyle and colleagues found the full texts for each of the remaining studies. Boyle and Tang read through the articles to decide if any more studies needed to be excluded based on the selection criteria.
When Boyle and Tang disagreed about whether a study should be excluded, they discussed it with Varigos until the three researchers came to an agreement.
Step 5: Extract the data
Extracting the data means collecting information from the selected studies in a systematic way. There are two types of information you need to collect from each study:
- Information about the study’s methods and results . The exact information will depend on your research question, but it might include the year, study design , sample size, context, research findings , and conclusions. If any data are missing, you’ll need to contact the study’s authors.
- Your judgment of the quality of the evidence, including risk of bias .
You should collect this information using forms. You can find sample forms in The Registry of Methods and Tools for Evidence-Informed Decision Making and the Grading of Recommendations, Assessment, Development and Evaluations Working Group .
Extracting the data is also a three-person job. Two people should do this step independently, and the third person will resolve any disagreements.
They also collected data about possible sources of bias, such as how the study participants were randomized into the control and treatment groups.
Step 6: Synthesize the data
Synthesizing the data means bringing together the information you collected into a single, cohesive story. There are two main approaches to synthesizing the data:
- Narrative ( qualitative ): Summarize the information in words. You’ll need to discuss the studies and assess their overall quality.
- Quantitative : Use statistical methods to summarize and compare data from different studies. The most common quantitative approach is a meta-analysis , which allows you to combine results from multiple studies into a summary result.
Generally, you should use both approaches together whenever possible. If you don’t have enough data, or the data from different studies aren’t comparable, then you can take just a narrative approach. However, you should justify why a quantitative approach wasn’t possible.
Boyle and colleagues also divided the studies into subgroups, such as studies about babies, children, and adults, and analyzed the effect sizes within each group.
Step 7: Write and publish a report
The purpose of writing a systematic review article is to share the answer to your research question and explain how you arrived at this answer.
Your article should include the following sections:
- Abstract : A summary of the review
- Introduction : Including the rationale and objectives
- Methods : Including the selection criteria, search method, data extraction method, and synthesis method
- Results : Including results of the search and selection process, study characteristics, risk of bias in the studies, and synthesis results
- Discussion : Including interpretation of the results and limitations of the review
- Conclusion : The answer to your research question and implications for practice, policy, or research
To verify that your report includes everything it needs, you can use the PRISMA checklist .
Once your report is written, you can publish it in a systematic review database, such as the Cochrane Database of Systematic Reviews , and/or in a peer-reviewed journal.
In their report, Boyle and colleagues concluded that probiotics cannot be recommended for reducing eczema symptoms or improving quality of life in patients with eczema. Note Generative AI tools like ChatGPT can be useful at various stages of the writing and research process and can help you to write your systematic review. However, we strongly advise against trying to pass AI-generated text off as your own work.
If you want to know more about statistics , methodology , or research bias , make sure to check out some of our other articles with explanations and examples.
- Student’s t -distribution
- Normal distribution
- Null and Alternative Hypotheses
- Chi square tests
- Confidence interval
- Quartiles & Quantiles
- Cluster sampling
- Stratified sampling
- Data cleansing
- Reproducibility vs Replicability
- Peer review
- Prospective cohort study
Research bias
- Implicit bias
- Cognitive bias
- Placebo effect
- Hawthorne effect
- Hindsight bias
- Affect heuristic
- Social desirability bias
A literature review is a survey of scholarly sources (such as books, journal articles, and theses) related to a specific topic or research question .
It is often written as part of a thesis, dissertation , or research paper , in order to situate your work in relation to existing knowledge.
A literature review is a survey of credible sources on a topic, often used in dissertations , theses, and research papers . Literature reviews give an overview of knowledge on a subject, helping you identify relevant theories and methods, as well as gaps in existing research. Literature reviews are set up similarly to other academic texts , with an introduction , a main body, and a conclusion .
An annotated bibliography is a list of source references that has a short description (called an annotation ) for each of the sources. It is often assigned as part of the research process for a paper .
A systematic review is secondary research because it uses existing research. You don’t collect new data yourself.
Cite this Scribbr article
If you want to cite this source, you can copy and paste the citation or click the “Cite this Scribbr article” button to automatically add the citation to our free Citation Generator.
Turney, S. (2023, November 20). Systematic Review | Definition, Example & Guide. Scribbr. Retrieved March 21, 2024, from https://www.scribbr.com/methodology/systematic-review/
Is this article helpful?
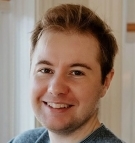
Shaun Turney
Other students also liked, how to write a literature review | guide, examples, & templates, how to write a research proposal | examples & templates, what is critical thinking | definition & examples, "i thought ai proofreading was useless but..".
I've been using Scribbr for years now and I know it's a service that won't disappoint. It does a good job spotting mistakes”
Log in using your username and password
- Search More Search for this keyword Advanced search
- Latest content
- Supplements
- BMJ Journals More You are viewing from: Google Indexer
You are here
- Volume 4, Issue Suppl 1
- Synthesising quantitative evidence in systematic reviews of complex health interventions
- Article Text
- Article info
- Citation Tools
- Rapid Responses
- Article metrics

- Julian P T Higgins 1 ,
- José A López-López 1 ,
- Betsy J Becker 2 ,
- Sarah R Davies 1 ,
- Sarah Dawson 1 ,
- Jeremy M Grimshaw 3 , 4 ,
- Luke A McGuinness 1 ,
- Theresa H M Moore 1 , 5 ,
- Eva A Rehfuess 6 ,
- James Thomas 7 ,
- Deborah M Caldwell 1
- 1 Population Health Sciences , Bristol Medical School, University of Bristol , Bristol , UK
- 2 Department of Educational Psychology and Learning Systems, College of Education , Florida State University , Tallahassee , Florida , USA
- 3 Clinical Epidemiology Program , Ottawa Hospital Research Institute, The Ottawa Hospital , Ottawa , Ontario , Canada
- 4 Department of Medicine , University of Ottawa , Ottawa , Ontario , Canada
- 5 NIHR Collaboration for Leadership in Applied Health Care (CLAHRC) West , University Hospitals Bristol NHS Foundation Trust , Bristol , UK
- 6 Institute for Medical Information Processing , Biometry and Epidemiology, Pettenkofer School of Public Health, LMU Munich , Munich , Germany
- 7 EPPI-Centre, Department of Social Science , University College London , London , UK
- Correspondence to Professor Julian P T Higgins; julian.higgins{at}bristol.ac.uk
Public health and health service interventions are typically complex: they are multifaceted, with impacts at multiple levels and on multiple stakeholders. Systematic reviews evaluating the effects of complex health interventions can be challenging to conduct. This paper is part of a special series of papers considering these challenges particularly in the context of WHO guideline development. We outline established and innovative methods for synthesising quantitative evidence within a systematic review of a complex intervention, including considerations of the complexity of the system into which the intervention is introduced. We describe methods in three broad areas: non-quantitative approaches, including tabulation, narrative and graphical approaches; standard meta-analysis methods, including meta-regression to investigate study-level moderators of effect; and advanced synthesis methods, in which models allow exploration of intervention components, investigation of both moderators and mediators, examination of mechanisms, and exploration of complexities of the system. We offer guidance on the choice of approach that might be taken by people collating evidence in support of guideline development, and emphasise that the appropriate methods will depend on the purpose of the synthesis, the similarity of the studies included in the review, the level of detail available from the studies, the nature of the results reported in the studies, the expertise of the synthesis team and the resources available.
- meta-analysis
- complex interventions
- systematic reviews
- guideline development
Data availability statement
No additional data are available.
This is an open access article distributed under the terms of the Creative Commons Attribution IGO License ( CC BY NC 3.0 IGO ), which permits use, distribution,and reproduction in any medium, provided the original work is properly cited. In any reproduction of this article there should not be any suggestion that WHO or this article endorse any specific organization or products. The use of the WHO logo is not permitted. This notice should be preserved along with the article’s original URL.Disclaimer: The author is a staff member of the World Health Organization. The author alone is responsible for the views expressed in this publication and they do not necessarily represent the views, decisions or policies of the World Health Organization.
https://doi.org/10.1136/bmjgh-2018-000858
Statistics from Altmetric.com
Request permissions.
If you wish to reuse any or all of this article please use the link below which will take you to the Copyright Clearance Center’s RightsLink service. You will be able to get a quick price and instant permission to reuse the content in many different ways.
Summary box
Quantitative syntheses of studies on the effects of complex health interventions face high diversity across studies and limitations in the data available.
Statistical and non-statistical approaches are available for tackling intervention complexity in a synthesis of quantitative data in the context of a systematic review.
Appropriate methods will depend on the purpose of the synthesis, the number and similarity of studies included in the review, the level of detail available from the studies, the nature of the results reported in the studies, the expertise of the synthesis team and the resources available.
We offer considerations for selecting methods for synthesis of quantitative data to address important types of questions about the effects of complex interventions.
Public health and health service interventions are typically complex. They are usually multifaceted, with impacts at multiple levels and on multiple stakeholders. Also, the systems within which they are implemented may change and adapt to enhance or dampen their impact. 1 Quantitative syntheses ('meta-analyses’) of studies of complex interventions seek to integrate quantitative findings across multiple studies to achieve a coherent message greater than the sum of their parts. Interest is growing on how the standard systematic review and meta-analysis toolkit can be enhanced to address complexity of interventions and their impact. 2 A recent report from the Agency for Healthcare Research and Quality and a series of papers in the Journal of Clinical Epidemiology provide useful background on some of the challenges. 3–6
This paper is part of a series to explore the implications of complexity for systematic reviews and guideline development, commissioned by WHO. 7 Clearly, and as covered by other papers in this series, guideline development encompasses the consideration of many different aspects, 8 such as intervention effectiveness, economic considerations, acceptability 9 or certainty of evidence, 10 and requires the integration of different types of quantitative as well as qualitative evidence. 11 12 This paper is specifically concerned with methods available for the synthesis of quantitative results in the context of a systematic review on the effects of a complex intervention. We aim to point those collating evidence in support of guideline development to methodological approaches that will help them integrate the quantitative evidence they identify. A summary of how these methods link to many of the types of complexity encountered is provided in table 1 , based on the examples provided in a table from an earlier paper in the series. 1 An annotated list of the methods we cover is provided in table 2 .
- View inline
Quantitative synthesis possibilities to address aspects of complexity
Quantitative graphical and synthesis approaches mentioned in the paper, with their main strengths and weaknesses in the context of complex interventions
We begin by reiterating the importance of starting with meaningful research questions and an awareness of the purpose of the synthesis and any relevant background knowledge. An important issue in systematic reviews of complex interventions is that data available for synthesis are often extremely limited, due to small numbers of relevant studies and limitations in how these studies are conducted and their results are reported. Furthermore, it is uncommon for two studies to evaluate exactly the same intervention, in part because of the interventions’ inherent complexity. Thus, each study may be designed to provide information on a unique context or a novel intervention approach. Outcomes may be measured in different ways and at different time points. We therefore discuss possible approaches when data are highly limited or highly heterogeneous, including the use of graphical approaches to present very basic summary results. We then discuss statistical approaches for combining results and for understanding the implications of various kinds of complexity.
In several places we draw on an example of a review undertaken to inform a recent WHO guideline on protecting, promoting and supporting breast feeding. 13 The review seeks to determine the effects of interventions to promote breast feeding delivered in five types of settings (health services, home, community, workplace, policy context or a combination of settings). 8 The included interventions were predominantly multicomponent, and were implemented in complex systems across multiple contexts. The review included 195 studies, including many from low-income and middle-income countries, and concluded that interventions should be delivered in a combination of settings to achieve high breastfeeding rates.
The importance of the research question
The starting point in any synthesis of quantitative evidence is a clear purpose. The input of stakeholders is critical to ensure that questions are framed appropriately, addressing issues important to those commissioning, delivering and affected by the intervention. Detailed discussion of the development of research questions is provided in an earlier paper in the series, 1 and a subsequent paper explains the importance of taking context into account. 9 The first of these papers describes two possible perspectives. A complex interventions perspective emphasises the complexities involved in conceptualising, specifying and implementing the intervention per se, including the array of possibly interacting components and the behaviours required to implement it. A complex systems perspective emphasises the complexity of the systems into which the intervention is introduced, including possible interactions between the intervention and the system, interactions between individuals within the system and how the whole system responds to the intervention.
The simplest purpose of a systematic review is to determine whether a particular type of complex intervention (or class of interventions) is effective compared with a ‘usual practice’ alternative. The familiar PICO framework is helpful for framing the review: 14 in the PICO framework, a broad research question about effectiveness is uniquely specified by describing the participants (‘P’, including the setting and prevailing conditions) to which the intervention is to be applied; the intervention (‘I’) and comparator (‘C’) of interest, and the outcomes (‘O’, including their time course) that might be impacted by the intervention. In the breastfeeding review, the primary synthesis approach was to combine all available studies, irrespective of setting, and perform separate meta-analyses for different outcomes. 15
More useful than a review that asks ‘does a complex intervention work?’ is one that determines the situations in which a complex intervention has a larger or smaller effect. Indeed, research questions targeted by syntheses in the presence of complexity often dissect one or more of the PICO elements to explore how intervention effects vary both within and across studies (ie, treating the PICO elements as ‘moderators’). For instance, analyses may explore variation across participants, settings and prevailing conditions (including context); or across interventions (including different intervention components that may be present or absent in different studies); or across outcomes (including different outcome measures, at different levels of the system and at different time points) on which effects of the intervention occur. In addition, there may be interest in how aspects of the underlying system or the intervention itself mediate the effects, or in the role of intermediate outcomes on the pathway from intervention to impact. 16 In the breastfeeding review, interest moved from the overall effects across interventions to investigations of how effects varied by such factors as intervention delivery setting, high-income versus low-income country, and urban versus rural setting. 15
The role of logic models to inform a synthesis
An earlier paper describes the benefits of using system-based logic models to characterise a priori theories about how the system operates. 1 These provide a useful starting point for most syntheses since they encourage consideration of all aspects of complexity in relation to the intervention or the system (or both). They can help identify important mediators and moderators, and inform decisions about what aspects of the intervention and system need to be addressed in the synthesis. As an example, a protocol for a review of the health effects of environmental interventions to reduce the consumption of sugar-sweetened beverages included a system-based logic model, detailing how the characteristics of the beverages, and the physiological characteristics and psychological characteristics of individuals, are thought to impact on outcomes such as weight gain and cardiovascular disease. 17 The logic model informs the selection of outcomes and the general plans for synthesis of the findings of included studies. However, system-based models do not usually include details of how implementation of an intervention into the system is likely to affect subsequent outcomes. They therefore have a limited role in informing syntheses that seek to explain mechanisms of action.
A quantitative synthesis may draw on a specific proposed framework for how an intervention might work; these are sometimes referred to as process-orientated logic models, and may be strongly driven by qualitative research evidence. 12 They represent causal processes, describing what components or aspects of an intervention are thought to impact on what behaviours and actions, and what the further consequences of these impacts are likely to be. 18 They may encompass mediators of effect and moderators of effect. A synthesis may simply adopt the proposed causal model at face value and attempt to quantify the relationships described therein. Where more than one possible causal model is available, a synthesis may explore which of the models is better supported by the data, for example, by examining the evidence for specific links within the model or by identifying a statistical model that corresponds to the overall causal model. 18 19
A systematic review on community-level interventions for improving access to food in low-income and middle-income countries was based on a logic model that depicts how interventions might lead to improved health status. 20 The model includes direct effects, such as increased financial resources of individuals and decreased food prices; intermediate effects, such as increased quantity of food available and increase in intake; and main outcomes of interest, such as nutritional status and health indicators. The planned statistical synthesis, however, was to tackle these one at a time.
Considering the types of studies available
Studies of the effects of complex interventions may be randomised or non-randomised, and often involve clustering of participants within social or organisational units. Randomised trials, if sufficiently large, provide the most convincing evidence about the effects of interventions because randomisation should result in intervention and comparator groups with similar distributions of both observed and unobserved baseline characteristics. However, randomised trials of complex interventions may be difficult or impossible to undertake, or may be performed only in specific contexts, yielding results that are not generalisable. Non-randomised study designs include so-called ‘quasi-experiments’ and may be longitudinal studies, including interrupted time series and before-after studies, with or without a control group. Non-randomised studies are at greater risk of bias, sometimes substantially so, although may be undertaken in contexts that are more relevant to decision making. Analyses of non-randomised studies often use statistical controls for confounders to account for differences between intervention groups, and challenges are introduced when different sets of confounders are used in different studies. 21 22
Randomised trials and non-randomised studies might both be included in a review, and analysts may have to decide whether to combine these in one synthesis, and whether to combine results from different types of non-randomised studies in a single analysis. Studies may differ in two ways: by answering different questions, or by answering similar questions with different risks of bias. The research questions must be sufficiently similar and the studies sufficiently free of bias for a synthesis to be meaningful. In the breastfeeding review, randomised, quasi-experimental and observational studies were combined; no evidence suggested that the effects differed across designs. 15 In practice, many methodologists generally recommend against combining randomised with non-randomised studies. 23
Preparing for a quantitative synthesis
Before undertaking a quantitative synthesis of complex interventions, it can be helpful to begin the synthesis non-quantitatively, looking at patterns and characteristics of the data identified. Systematic tabulation of information is recommended, and this might be informed by a prespecified logic model. The most established framework for non-quantitative synthesis is that proposed by Popay et al . 24 The Cochrane Consumers and Communication group succinctly summarise the process as an 'investigation of the similarities and the differences between the findings of different studies, as well as exploration of patterns in the data’. 25 Another useful framework was described by Petticrew and Roberts. 26 They identify three stages in the initial narrative synthesis: (1) Organisation of studies into logical categories, the structure of which will depend on the purpose of the synthesis, possibly relating to study design, outcome or intervention types. (2) Within-study analysis, involving the description of findings within each study. (3) Cross-study synthesis, in which variations in study characteristics and potential biases are integrated and the range of effects described. Aspects of this process are likely to be implemented in any systematic review, even when a detailed quantitative synthesis is undertaken.
In some circumstances the available data are too diverse, too non-quantitative or too sparse for a quantitative synthesis to be meaningful even if it is possible. The best that can be achieved in many reviews of complex interventions is a non-quantitative synthesis following the guidance given in the above frameworks.
Options when effect size estimates cannot be obtained or studies are too diverse to combine
Graphical approaches.
Graphical displays can be very valuable to illustrate patterns in results of studies. 27 We illustrate some options in figure 1 . Forest plots are the standard illustration of the results of multiple studies (see figure 1 , panel A), but require a similar effect size estimate from each study. For studies of complex interventions, the diversity of approaches to the intervention, the context, 1 evaluation approaches and reporting differences can lead to considerable variation across studies in what results are available. Some novel graphical approaches have been proposed for such situations. A recent development is the albatross plot, which plots p values against sample sizes, with approximate effect-size contours superimposed (see figure 1 , panel B). 28 The contours are computed from the p values and sample sizes, based on an assumption about the type of analysis that would have given rise to the p values. Although these plots are designed for situations when effect size estimates are not available, the contours can be used to infer approximate effect sizes from studies that are analysed and reported in highly diverse ways. Such an advantage may prove to be a disadvantage, however, if the contours are overinterpreted.
- Download figure
- Open in new tab
- Download powerpoint
Example graphical displays of data from a review of interventions to promote breast feeding, for the outcome of continued breast feeding up to 23 months. 15 Panel A: Forest plot for relative risk (RR) estimates from each study. Panel B: Albatross plot of p value against sample size (effect contours drawn for risk ratios assuming a baseline risk of 0.15; sample sizes and baseline risks extracted from the original papers by the current authors); Panel C: Harvest plot (heights reflect design: randomised trials (tall), quasi-experimental studies (medium), observational studies (short); bar shading reflects follow-up: longest follow-up (black) to shortest follow-up (light grey) or no information (white)). Panel D: Bubble plot (bubble sizes and colours reflect design: randomised trials (large, green), quasi-experimental studies (medium, red), observational studies (small, blue); precision defined as inverse of the SE of each effect estimate (derived from the CIs); categories are: “Potential Harm”: RR <0.8; “No Effect”: RRs between 0.8 and 1.25; “Potential Benefit”: RR >1.25 and CI includes RR=1; “Benefit”: RR >1.25 and CI excludes RR=1).
Harvest plots have been proposed by Ogilvie et al as a graphical extension of a vote counting approach to synthesis (see figure 1 , panel C). 29 However, approaches based on vote counting of statistically significant results have been criticised on the basis of their poor statistical properties, and because statistical significance is an outdated and unhelpful notion. 30 The harvest plot is a matrix of small illustrations, with different outcome domains defining rows and different qualitative conclusions (negative effect, no effect, positive effect) defining columns. Each study is represented by a bar that is positioned according to its measured outcome and qualitative conclusion. Bar heights and shadings can depict features of the study, such as objectivity of the outcome measure, suitability of the study design and study quality. 29 31 A similar idea to the harvest plot is the effect direction plot proposed by Thomson and Thomas. 32
A device to plot the findings from a large and complex collection of evidence is a bubble plot (see figure 1 , panel D). A bubble plot illustrates the direction of each finding (or whether the finding was unclear) on a horizontal scale, using a vertical scale to indicate the volume of evidence, and with bubble sizes to indicate some measure of credibility of each finding. Such an approach can also depict findings of collections of studies rather than individual studies, and was used successfully, for example, to summarise findings from a review of systematic reviews of the effects of acupuncture on various indications for pain. 33
Statistical methods not based on effect size estimates
We have mentioned that a frequent problem is that standard meta-analysis methods cannot be used because data are not available in a similar format from every study. In general, the core principles of meta-analysis can be applied even in this situation, as is highlighted in the Cochrane Handbook , by addressing the questions: ‘What is the direction of effect?’; 'What is the size of effect?’; ‘Is the effect consistent across studies?’; and 'What is the strength of evidence for the effect?’. 34
Alternatives to the estimation of effect sizes could be used more often than they are in practice, allowing some basic statistical inferences despite diversely reported results. The most fundamental analysis is to test the overall null hypothesis of no effect in any of the studies. Such a test can be undertaken using only minimally reported information from each study. At its simplest, a binomial test can be performed using only the direction of effect observed in each study, irrespective of its CI or statistical significance. 35 Where exact p values are available as well as the direction of effect, a more powerful test can be performed by combining these using, for example, Fisher’s combination of p values. 36 It is important that these p values are computed appropriately, however, accounting for clustering or matching of participants within the studies. Rejecting the null model based on such tests provides no information about the magnitude of the effect, providing information only on whether at least one study shows an effect is present, and if so, its direction. 37
Standard synthesis methods
Meta-analysis for overall effect.
Probably the most familiar approach to meta-analysis is that of estimating a single summary effect across similar studies. This simple approach lends itself to the use of forest plots to display the results of individual studies as well as syntheses, as illustrated for the breastfeeding studies in figure 1 (panel A). This analysis addresses the broad question of whether evidence from a collection of studies supports an impact of the complex intervention of interest, and requires that every study makes a comparison of a relevant intervention against a similar alternative. In the context of complex interventions, this is described by Caldwell and Welton as the ‘lumping’ approach, 38 and by Guise et al as the ‘holistic’ approach. 5 6 One key limitation of the simple approach is that it requires similar types of data from each study. A second limitation is that the meta-analysis result may have limited relevance when the studies are diverse in their characteristics. Fixed-effect models, for instance, are unlikely to be appropriate for complex interventions because they ignore between-studies variability in underlying effect sizes. Results based on random-effects models will need to be interpreted by acknowledging the spread of effects across studies, for example, using prediction intervals. 39
A common problem when undertaking a simple meta-analysis is that individual studies may report many effect sizes that are correlated with each other, for example, if multiple outcomes are measured, or the same outcome variable is measured at several time points. Numerous approaches are available for dealing with such multiplicity, including multivariate meta-analysis, multilevel modelling, and strategies for selecting effect sizes. 40 A very simple strategy that has been used in systematic reviews of complex interventions is to take the median effect size within each study, and to summarise these using the median of these effect sizes across studies. 41
Exploring heterogeneity
Diversity in the types of participants (and contexts), interventions and outcomes are key to understanding sources of complexity. 9 Many of these important sources of heterogeneity are most usefully examined—to the extent that they can reliably be understood—using standard approaches for understanding variability across studies, such as subgroup analyses and meta-regression.
A simple strategy to explore heterogeneity is to estimate the overall effect separately for different levels of a factor using subgroup analyses (referring to subgrouping studies rather than participants). 42 As an example, McFadden et al conducted a systematic review and meta-analysis of 73 studies of support for healthy breastfeeding mothers with healthy term babies. 43 They calculated separate average effects for interventions delivered by a health professional, a lay supporter or with mixed support, and found that the effect on cessation of exclusive breast feeding at up to 6 months was greater for lay support compared with professionals or mixed support (p=0.02). Guise et al provide several ways of grouping studies according to their interventions, for example, grouping studies by key components, by function or by theory. 5 6
Meta-regression provides a flexible generalisation to subgroup analyses, whereby study-level covariates are included in a regression model using effect size estimates as the dependent variable. 44 45 Both continuous and categorical covariates can be included in such models; with a single categorical covariate, the approach is essentially equivalent to subgroup analyses. Meta-regression with continuous covariates in theory allows the extrapolation of relationships to contexts that were not examined in any of the studies, but this should generally be avoided. For example, if the effect of an interventional approach appears to increase as the size of the group to which it is applied decreases, this does not mean that it will work even better when applied to a single individual. More generally, the mathematical form of the relationship modelled in a meta-regression requires careful selection. Most often a linear relationship is assumed, but a linear relationship does not permit step changes such as might occur if an interventional approach requires a particular level of some feature of the underlying system before it has an effect.
Several texts provide guidance for using subgroup analysis and meta-regression in a general context 45 46 and for complex interventions. 3 4 47 In principle, many aspects of complexity in interventions can be addressed using these strategies, to create an understanding of the ‘response surface’. 48–50 However, in practice, the number of studies is often too small for reliable conclusions to be drawn. In general, subgroup analysis and meta-regression are fraught with dangers associated with having few studies, many sources of variation across study features and confounding of these features with each other as well as with other, often unobserved, variables. It is therefore important to prespecify a small number of plausible sources of diversity so as to reduce the danger of reaching spurious conclusions based on study characteristics that correlate with the effects of the interventions but are not the cause of the variation. The ability of statistical analyses to identify true sources of heterogeneity will depend on the number of studies, the sizes of the studies and the true differences between effects in studies with different characteristics.
Synthesis methods for understanding components of the intervention
When interventions comprise distinct components, it is attractive to separate out the individual effects of these components. 51 Meta-regression can be used for this, using covariates to code the presence of particular features in each intervention implementation. As an example, Blakemore et al analysed 39 intervention comparisons from 33 independent studies aiming to reduce urgent healthcare use in adults with asthma. 52 Effect size estimates were coded according to components used in the interventions, and the authors found that multicomponent interventions including skills training, education and relapse prevention appeared particularly effective. In another example, of interventions to support family caregivers of people with Alzheimer’s disease, 53 the authors used methods for decomposing complex interventions proposed by Czaja et al , 54 and created covariates that reduced the complexity of the interventions to a small number of features about the intensity of the interventions. More sophisticated models for examining components have been described by Welton et al , 55 Ivers et al 56 and Madan et al . 57
A component-level approach may be useful when there is a need to disentangle the ‘active ingredients’ of an intervention, for example, when adapting an existing intervention for a new setting. However, components-based approaches require assumptions, such as whether individual components are additive or interact with each other. Furthermore, the effects of components can be difficult to estimate if they are used only in particular contexts or populations, or are strongly correlated with use of other components. An alternative approach is to treat each combination of components as a separate intervention. These separate interventions might then be compared in a single analysis using network meta-analysis. A network meta-analysis combines results from studies comparing two or more of a larger set of interventions, using indirect comparisons via common comparators to rank-order all interventions. 47 58 59 As an example, Achana et al examined the effectiveness of safety interventions on the uptake of three poisoning prevention practices in households with children. Each singular combination of intervention components was defined as a separate intervention in the network. 60 Network meta-analysis may also be useful when there is a need to compare multiple interventions to answer an ‘in principle’ question of which intervention is most effective. Consideration of the main goals of the synthesis will help those aiming to prepare guidelines to decide which of these approaches is most appropriate to their needs.
A case study exploring components is provided in box 1 , and an illustration is provided in figure 2 . The component-based analysis approach can be likened to a factorial trial, in that it attempts to separate out the effects of individual components of the complex interventions, and the network meta-analysis approach can be likened to a multiarm trial approach, where each complex intervention in the set of studies is a different arm in the trial. 47 Deciding between the two approaches can leave the analyst caught between the need to ‘split’ components to reflect complexity (and minimise heterogeneity) and ‘lump’ to make an analysis feasible. Both approaches can be used to examine other features of interventions, including interventions designed for delivery at different levels. For example, a review of the effects of interventions for children exposed to domestic violence and abuse included studies of interventions targeted at children alone, parents alone, children and parents together, and parents and children separately. 61 A network meta-analysis approach was taken to the synthesis, with the people targeted by the intervention used as a distinguishing feature of the interventions included in the network.
Example of understanding components of psychosocial interventions for coronary heart disease
Welton et al reanalysed data from a Cochrane review 89 of randomised controlled trials assessing the effects of psychological interventions on mortality and morbidity reduction for people with coronary heart disease. 55 The Cochrane review focused on the effectiveness of any psychological intervention compared with usual care, and found evidence that psychological interventions reduced non-fatal reinfarctions and depression and anxiety symptoms. The Cochrane review authors highlighted the large heterogeneity among interventions as an important limitation of their review.
Welton et al were interested in the effects of the different intervention components. They classified interventions according to which of five key components were included: educational, behavioural, cognitive, relaxation and psychosocial support ( figure 2 ). Their reanalysis examined the effect of each component in three different ways: (1) An additive model assuming no interactions between components. (2) A two-factor interaction model, allowing for interactions between pairs of components. (3) A network meta-analysis, defining each combination of components as a separate intervention, therefore allowing for full interaction between components. Results suggested that interventions with behavioural components were effective in reducing the odds of all-cause mortality and non-fatal myocardial infarction, and that interventions with behavioural and/or cognitive components were effective for reducing depressive symptoms.
Intervention components in the studies integrated by Welton et al (a sample of 18 from 56 active treatment arms). EDU, educational component; BEH, behavioural component; COG, cognitive component; REL, relaxation component; SUP, psychosocial support component.
A common limitation when implementing these quantitative methods in the context of complex interventions is that replication of the same intervention in two or more studies is rare. Qualitative comparative analysis (QCA) might overcome this problem, being designed to address the ’small N; many variables’ problem. 62 QCA involves: (1) Identifying theoretically driven thresholds for determining intervention success or failure. (2) Creating a 'truth table’, which takes the form of a matrix, cross-tabulating all possible combinations of conditions (eg, participant and intervention characteristics) against each study and its associated outcomes. (3) Using Boolean algebra to eliminate redundant conditions and to identify configurations of conditions that are necessary and/or sufficient to trigger intervention success or failure. QCA can usefully complement quantitative integration, sometimes in the context of synthesising diverse types of evidence.
Synthesis methods for understanding mechanisms of action
An alternative purpose of a synthesis is to gain insight into the mechanisms of action behind an intervention, to inform its generalisability or applicability to a particular context. Such syntheses of quantitative data may complement syntheses of qualitative data, 11 and the two forms might be integrated. 12 Logic models, or theories of action, are important to motivate investigations of mechanism. The synthesis is likely to focus on intermediate outcomes reflecting intervention processes, and on mediators of effect (factors that influence how the intervention affects an outcome measure). Two possibilities for analysis are to use these intermediate measurements as predictors of main outcomes using meta-regression methods, 63 or to use multivariate meta-analysis to model the intermediate and main outcomes simultaneously, exploiting and estimating the correlations between them. 64 65 If the synthesis suggests that hypothesised chains of outcomes hold, this lends weight to the theoretical model underlying the hypothesis.
An approach to synthesis closely identified with this category of interventions is model-driven meta-analysis, in which different sources of evidence are integrated within a causal path model akin to a directed acyclic graph. A model-driven meta-analysis is an explanatory analysis. 66 It attempts to go further than a standard meta-analysis or meta-regression to explore how and why an intervention works, for whom it works, and which aspects of the intervention (factors) are driving overall effect. Such syntheses have been described in frequentist 19 67–70 and Bayesian 71 72 frameworks and are variously known as model-driven meta-analysis, linked meta-analysis, meta-mediation analysis and meta-analysis of structural equation models. In their simplest form, standard meta-analyses estimate a summary correlation independently for each pair of variables in the model. The approach is inherently multivariate, requiring the estimation of multiple correlations (which, if obtained from a single study, are also not independent). 73–75 Each study is likely to contribute fragments of the correlation matrix. A summary correlation matrix, combined either by fixed-effects or random-effects methods, then serves as the input for subsequent analysis via a standardised regression or structural equation model.
An example is provided in box 2 . The model in figure 3 postulates that the effect of ‘Dietary adherence’ on ‘Diabetes complications’ is not direct but is mediated by ‘Metabolic control’. 76 The potential for model-driven meta-analysis to incorporate such indirect effects also allows for mediating effects to be explicitly tested and in so doing allows the meta-analyst to identify and explore the mechanisms underpinning a complex intervention. 77
Theoretical diabetes care model (adapted from Brown et al 68 ).
Example of a model-driven meta-analysis for type 2 diabetes
Brown et al present a model-driven meta-analysis of correlational research on psychological and motivational predictors of diabetes outcomes, with medication and dietary adherence factors as mediators. 76 In a linked methodological paper, they present the a priori theoretical model on which their analysis is based. 68 The model is simplified in figure 3 , and summarised for the dietary adherence pathway only. The aim of their full analysis was to determine the predictive relationships among psychological factors and motivational factors on metabolic control and body mass index (BMI), and the role of behavioural factors as possible mediators of the associations among the psychological and motivational factors and metabolic control and BMI outcomes.
The analysis is based on a comprehensive systematic review. Due to the number of variables in their full model, 775 individual correlational or predictive studies reported across 739 research papers met eligibility criteria. Correlations between each pair of variables in the model were summarised using an overall average correlation, and homogeneity assessed. Multivariate analyses were used to estimate a combined correlation matrix. These results were used, in turn, to estimate path coefficients for the predictive model and their standard errors. For the simplified model illustrated here, the results suggested that coping and self-efficacy were strongly related to dietary adherence, which was strongly related to improved glycaemic control and, in turn, a reduction in diabetic complications.
Synthesis approaches for understanding complexities of the system
Syntheses may seek to address complexities of the system to understand either the impact of the system on the effects of the intervention or the effects of the intervention on the system. This may start by modelling the salient features of the system’s dynamics, rather than focusing on interventions. Subgroup analysis and meta-regression are useful approaches for investigating the extent to which an intervention’s effects depend on baseline features of the system, including aspects of the context. Sophisticated meta-regression models might investigate multiple baseline features, using similar approaches to the component-based meta-analyses described earlier. Specifically, aspects of context or population characteristics can be regarded as ‘components’ of the system into which the intervention is introduced, and similar statistical modelling strategies used to isolate effects of individual factors, or interactions between them.
When interventions act at multiple levels, it may be important to understand the effects at these different levels. Outcomes may be measured at different levels (eg, at patient, clinician and clinical practice levels) and analysed separately. Qualitative research plays a particularly important role in identifying the outcomes that should be assessed through quantitative synthesis. 12 Care is needed to ensure that the unit of analysis issues are addressed. For example, if clinics are the unit of randomisation, then outcomes measured at the clinic level can be analysed using standard methods, whereas outcomes measured at the level of the patient within the clinic would need to account for clustering. In fact, multiple dependencies may arise in such data, when patients receive care in small groups. Detailed investigations of effect at different levels, including interactions between the levels, would lend themselves to multilevel (hierarchical) models for synthesis. Unfortunately, individual participant data at all levels of the hierarchy are needed for such analyses.
Model-based approaches also offer possibilities for addressing complex systems; these include economic models, mathematical models and systems science methods generally. 78–80 Broadly speaking, these provide mathematical representations of logic models, and analyses may involve incorporation of empirical data (eg, from systematic reviews), computer simulation, direct computation or a mixture of these. Multiparameter evidence synthesis methods might be used. 81 82 Approaches include models to represent systems (eg, systems dynamics models) and approaches that simulate individuals within the system (eg, agent-based models). 79 Models can be particularly useful when empirical evidence does not address all important considerations, such as ‘real-world’ contexts, long-term effects, non-linear effects and complexities such as feedback loops and threshold effects. An example of a model-based approach to synthesis is provided in box 3 . The challenge when adopting these approaches is often in the identification of system components, and accurately estimating causes and effects (and uncertainties). There are few examples of the use of these analytical tools in systematic reviews, but they may be useful when the focus of analysis is on understanding the causes of complexity in a given system rather than on the impact of an intervention.
Example of a mathematical modelling approach for soft drinks industry levy
Briggs et al examined the potential impact of a soft drinks levy in the UK, considering possible different types of response to the levy by industry. 90 Various scenarios were posited, with effects on health outcomes informed by empirical data from randomised trials and cohort studies of association between sugar intake and body weight, diabetes and dental caries. Figure 4 provides a simple characterisation of how the empirical data were fed into the model. Inputs into the model included levels of consumption of various types of drinks (by age and sex), volume of drinks sales, and baseline levels of obesity, diabetes and dental caries (by age and sex). The authors concluded that health gains would be greatest if industry reacted by reformulating their products to include less sugar.
Simplified version of the conceptual model used by Briggs et al ( a dapted from Briggs et al 90 ).
Considerations of bias and relevance
It is always important to consider the extent to which (1) The findings from each study have internal validity, particularly for non-randomised studies which are typically at higher risk of bias. (2) Studies may have been conducted but not reported because of unexciting findings. (3) Each study is applicable to the purposes of the review, that is, has external validity (or ‘directness’), in the language of the Grading of Recommendations Assessment, Development and Evaluation (GRADE) Working Group. 83 At minimum, internal and external validity should be examined and reported, and the risk of publication bias assessed, and these can be achieved through the GRADE framework. 10 With sufficient studies, information collected might be used in meta-regression analyses to evaluate empirically whether studies with and without specific sources of bias or indirectness differ in their results.
It may be desirable to learn about a specific setting, intervention type or outcome measure more directly than others. For example, to inform a decision for a low-income setting, emphasis should be placed on results of studies performed in low-income countries. One option is to restrict the synthesis to these studies. An alternative is to model the dependence of an intervention’s effect on some feature(s) related to the income setting, and extract predictions from the model that are most relevant to the setting of interest. This latter approach makes fuller use of available data, but relies on stronger assumptions.
Often, however, the accumulated studies are too few or too disparate to draw conclusions about the impact of bias or relevance. On rare occasions, syntheses might implement formal adjustments of individual study results for likely biases. Such adjustments may be made by imposing prior distributions to depict the magnitude and direction of any biases believed to exist. 84 85 The choice of a prior distribution may be informed by formal assessments of risk of bias, by expert judgement, or possibly by empirical data from meta-epidemiological studies of biases in randomised and/or non-randomised studies. 86 For example, Wolf et al implemented a prior distribution based on findings of a meta-epidemiological study 87 to adjust for lack of blinding in studies of interventions to improve quality of point-of-use water sources in low-income and middle-income settings. 88 Unfortunately, empirical evidence of bias is mostly limited to clinical trials, is weak for trials of public health and social care interventions, and is largely non-existent for non-randomised studies.
Our review of quantitative synthesis methods for evaluating the effects of complex interventions has outlined many possible approaches that might be considered by those collating evidence in support of guideline development. We have described three broad categories: (1) Non-quantitative methods, including tabulation, narrative and graphical approaches. (2) Standard meta-analysis methods, including meta-regression to investigate study-level moderators of effect. (3) More advanced synthesis methods, in which models allow exploration of intervention components, investigation of both moderators and mediators, examination of mechanisms, and exploration of complexities of the system.
The choice among these approaches will depend on the purpose of the synthesis, the similarity of the studies included in the review, the level of detail available from the studies, the nature of the results reported in the studies, the expertise of the synthesis team, and the resources available. Clearly the advanced methods require more expertise and resources than the simpler methods. Furthermore, they require a greater level of detail and typically a sizeable evidence base. We therefore expect them to be used seldomly; our aim here is largely to articulate what they can achieve so that they can be adopted when they are appropriate. Notably, the choice among these approaches will also depend on the extent to which guideline developers and users at global, national or local levels understand and are willing to base their decisions on different methods. Where possible, it will thus be important to involve concerned stakeholders during the early stages of the systematic review process to ensure the relevance of its findings.
Complexity is common in the evaluation of public health interventions at individual, organisational or community levels. To help systematic review and guideline development teams decide how to address this complexity in syntheses of quantitative evidence, we summarise considerations and methods in tables 1 and 2 . We close with the important remark that quantitative synthesis is not always a desirable feature of a systematic review. Whereas some sophisticated methods are available to deal with a variety of complex problems, on many occasions—perhaps even the majority in practice—the studies may be too different from each other, too weak in design or have data too sparse, for statistical methods to provide insight beyond a commentary on what evidence has been identified.
Acknowledgments
The authors thank the following for helpful comments on earlier drafts of the paper: Philippa Easterbrook, Matthias Egger, Anayda Portela, Susan L Norris, Mark Petticrew.
- Petticrew M ,
- Thomas J , et al
- Anderson L ,
- Elder R , et al
- Rehfuess E ,
- Noyes J , et al
- Viswanathan M , et al
- World Health Organization
- Rehfuess EA ,
- Stratil JM ,
- Scheel IB , et al
- Flemming K , et al
- Montgomery P ,
- Movsisyan A ,
- Grant SP , et al
- Flemming K ,
- Garside R , et al
- Moore G , et al
- Richardson WS ,
- Wilson MC ,
- Nishikawa J , et al
- Chowdhury R ,
- Sankar MJ , et al
- Collins D ,
- Johnson K ,
- von Philipsborn P ,
- Burns J , et al
- Schoonees A ,
- Becker BJ ,
- Duvendack M , et al
- Reeves BC ,
- Higgins JPT , et al
- Roberts H ,
- Ryan R , Cochrane Consumers and Communication Review Group
- Anzures-Cabrera J ,
- Harrison S ,
- Martin RM , et al
- Ogilvie D ,
- Petticrew M , et al
- Sterne JA ,
- Davey Smith G
- Crowther M ,
- Avenell A ,
- MacLennan G , et al
- Thomson HJ ,
- Taylor SL ,
- Higgins JPT ,
- Bushman BJ ,
- Caldwell DM ,
- Thompson SG ,
- Spiegelhalter DJ
- López-López JA ,
- Lipsey MW , et al
- Grimshaw JM ,
- Thomas RE ,
- Borenstein M ,
- McFadden A ,
- Renfrew MJ , et al
- van Houwelingen HC ,
- Arends LR ,
- Melendez-Torres GJ ,
- Ioannidis JP ,
- Steckelberg A ,
- Richter B , et al
- Presseau J ,
- Newham JJ , et al
- Blakemore A ,
- Dickens C ,
- Anderson R , et al
- Schulz R , et al
- Lee CC , et al
- Welton NJ ,
- Adamopoulos E , et al
- Tricco AC ,
- Trikalinos TA , et al
- Aveyard P , et al
- Calderbank-Batista T
- Achana FA ,
- Cooper NJ ,
- Bujkiewicz S , et al
- Howarth E ,
- Moore THM ,
- Welton NJ , et al
- O’Mara-Eves A ,
- Thompson SG
- Jackson D ,
- Raudenbush SW ,
- García AA , et al
- Cheung MW ,
- Watson SI ,
- Stewart GB ,
- Mengersen K ,
- García AA ,
- Brown A , et al
- Liu H , et al
- Johnson L ,
- Althaus C , et al
- Stamatakis KA
- Greenwood-Lee J ,
- Nettel-Aguirre A , et al
- Caldwell D , et al
- Colbourn T ,
- Asseburg C ,
- Bojke L , et al
- Guyatt GH ,
- Oxman AD , GRADE Working Group
- Turner RM ,
- Spiegelhalter DJ ,
- Smith GCS , et al
- Carlin JB , et al
- Schulz KF , et al
- Savović J ,
- Altman DG , et al
- Prüss-Ustün A ,
- Cumming O , et al
- Bennett P ,
- West R , et al
- Briggs ADM ,
- Mytton OT ,
- Kehlbacher A , et al
Handling editor Soumyadeep Bhaumik
Contributors JPTH co-led the project, conceived the paper, led discussions and wrote the first draft. JAL-L undertook analyses, contributed to discussions and contributed to writing the manuscript. BJB drafted material on mechanisms, contributed to discussions and contributed extensively to writing the manuscript. SRD screened and categorised the results of the literature searches, collated examples and contributed to discussions. SD undertook searches to identify relevant literature and contributed to discussions. JMG contributed to discussions and commented critically on drafts. LAM undertook analyses, contributed to discussions and commented critically on drafts. THMM contributed examples, contributed to discussions and commented critically on drafts. EAR and JT contributed to discussions and commented critically on drafts. DMC co-led the project, contributed to discussions and drafted extensive parts of the paper. All authors approved the final version of the manuscript.
Funding Funding provided by the World Health Organization Department of Maternal, Newborn, Child and Adolescent Health through grants received from the United States Agency for International Development and the Norwegian Agency for Development Cooperation. JPTH was funded in part by Medical Research Council (MRC) grant MR/M025209/1, by the MRC Integrative Epidemiology Unit at the University of Bristol (MC_UU_12013/9) and by the MRC ConDuCT-II Hub (Collaboration and innovation for Difficult and Complex randomised controlled Trials In Invasive procedures – MR/K025643/1). BJB was funded in part by grant DRL-1252338 from the US National Science Foundation (NSF). JMG holds a Canada Research Chair in Health Knowledge Transfer and Uptake. LAM is funded by a National Institute for Health Research (NIHR) Systematic Review Fellowship (RM-SR-2016-07 26). THMM was funded by the NIHR Collaboration for Leadership in Applied Health Research and Care West (NIHR CLAHRC West). JT is supported by the NIHR Collaboration for Leadership in Applied Health Research and Care North Thames at Bart’s Health NHS Trust. DMC was funded in part by NIHR grant PHR 15/49/08 and by the Centre for the Development and Evaluation of Complex Interventions for Public Health Improvement (DECIPHer –MR/KO232331/1).
Disclaimer The views expressed are those of the authors and not necessarily those of the CRC program, the MRC, the NSF, the NHS, the NIHR or the UK Department of Health.
Competing interests JMG reports personal fees from the Campbell Collaboration. EAR reports being a Methods Editor with Cochrane Public Health.
Patient consent Not required.
Provenance and peer review Not commissioned; externally peer reviewed.
Read the full text or download the PDF:
Have a language expert improve your writing
Run a free plagiarism check in 10 minutes, automatically generate references for free.
- Knowledge Base
- Methodology
- Systematic Review | Definition, Examples & Guide
Systematic Review | Definition, Examples & Guide
Published on 15 June 2022 by Shaun Turney . Revised on 17 October 2022.
A systematic review is a type of review that uses repeatable methods to find, select, and synthesise all available evidence. It answers a clearly formulated research question and explicitly states the methods used to arrive at the answer.
They answered the question ‘What is the effectiveness of probiotics in reducing eczema symptoms and improving quality of life in patients with eczema?’
In this context, a probiotic is a health product that contains live microorganisms and is taken by mouth. Eczema is a common skin condition that causes red, itchy skin.
Table of contents
What is a systematic review, systematic review vs meta-analysis, systematic review vs literature review, systematic review vs scoping review, when to conduct a systematic review, pros and cons of systematic reviews, step-by-step example of a systematic review, frequently asked questions about systematic reviews.
A review is an overview of the research that’s already been completed on a topic.
What makes a systematic review different from other types of reviews is that the research methods are designed to reduce research bias . The methods are repeatable , and the approach is formal and systematic:
- Formulate a research question
- Develop a protocol
- Search for all relevant studies
- Apply the selection criteria
- Extract the data
- Synthesise the data
- Write and publish a report
Although multiple sets of guidelines exist, the Cochrane Handbook for Systematic Reviews is among the most widely used. It provides detailed guidelines on how to complete each step of the systematic review process.
Systematic reviews are most commonly used in medical and public health research, but they can also be found in other disciplines.
Systematic reviews typically answer their research question by synthesising all available evidence and evaluating the quality of the evidence. Synthesising means bringing together different information to tell a single, cohesive story. The synthesis can be narrative ( qualitative ), quantitative , or both.
Prevent plagiarism, run a free check.
Systematic reviews often quantitatively synthesise the evidence using a meta-analysis . A meta-analysis is a statistical analysis, not a type of review.
A meta-analysis is a technique to synthesise results from multiple studies. It’s a statistical analysis that combines the results of two or more studies, usually to estimate an effect size .
A literature review is a type of review that uses a less systematic and formal approach than a systematic review. Typically, an expert in a topic will qualitatively summarise and evaluate previous work, without using a formal, explicit method.
Although literature reviews are often less time-consuming and can be insightful or helpful, they have a higher risk of bias and are less transparent than systematic reviews.
Similar to a systematic review, a scoping review is a type of review that tries to minimise bias by using transparent and repeatable methods.
However, a scoping review isn’t a type of systematic review. The most important difference is the goal: rather than answering a specific question, a scoping review explores a topic. The researcher tries to identify the main concepts, theories, and evidence, as well as gaps in the current research.
Sometimes scoping reviews are an exploratory preparation step for a systematic review, and sometimes they are a standalone project.
A systematic review is a good choice of review if you want to answer a question about the effectiveness of an intervention , such as a medical treatment.
To conduct a systematic review, you’ll need the following:
- A precise question , usually about the effectiveness of an intervention. The question needs to be about a topic that’s previously been studied by multiple researchers. If there’s no previous research, there’s nothing to review.
- If you’re doing a systematic review on your own (e.g., for a research paper or thesis), you should take appropriate measures to ensure the validity and reliability of your research.
- Access to databases and journal archives. Often, your educational institution provides you with access.
- Time. A professional systematic review is a time-consuming process: it will take the lead author about six months of full-time work. If you’re a student, you should narrow the scope of your systematic review and stick to a tight schedule.
- Bibliographic, word-processing, spreadsheet, and statistical software . For example, you could use EndNote, Microsoft Word, Excel, and SPSS.
A systematic review has many pros .
- They minimise research b ias by considering all available evidence and evaluating each study for bias.
- Their methods are transparent , so they can be scrutinised by others.
- They’re thorough : they summarise all available evidence.
- They can be replicated and updated by others.
Systematic reviews also have a few cons .
- They’re time-consuming .
- They’re narrow in scope : they only answer the precise research question.
The 7 steps for conducting a systematic review are explained with an example.
Step 1: Formulate a research question
Formulating the research question is probably the most important step of a systematic review. A clear research question will:
- Allow you to more effectively communicate your research to other researchers and practitioners
- Guide your decisions as you plan and conduct your systematic review
A good research question for a systematic review has four components, which you can remember with the acronym PICO :
- Population(s) or problem(s)
- Intervention(s)
- Comparison(s)
You can rearrange these four components to write your research question:
- What is the effectiveness of I versus C for O in P ?
Sometimes, you may want to include a fourth component, the type of study design . In this case, the acronym is PICOT .
- Type of study design(s)
- The population of patients with eczema
- The intervention of probiotics
- In comparison to no treatment, placebo , or non-probiotic treatment
- The outcome of changes in participant-, parent-, and doctor-rated symptoms of eczema and quality of life
- Randomised control trials, a type of study design
Their research question was:
- What is the effectiveness of probiotics versus no treatment, a placebo, or a non-probiotic treatment for reducing eczema symptoms and improving quality of life in patients with eczema?
Step 2: Develop a protocol
A protocol is a document that contains your research plan for the systematic review. This is an important step because having a plan allows you to work more efficiently and reduces bias.
Your protocol should include the following components:
- Background information : Provide the context of the research question, including why it’s important.
- Research objective(s) : Rephrase your research question as an objective.
- Selection criteria: State how you’ll decide which studies to include or exclude from your review.
- Search strategy: Discuss your plan for finding studies.
- Analysis: Explain what information you’ll collect from the studies and how you’ll synthesise the data.
If you’re a professional seeking to publish your review, it’s a good idea to bring together an advisory committee . This is a group of about six people who have experience in the topic you’re researching. They can help you make decisions about your protocol.
It’s highly recommended to register your protocol. Registering your protocol means submitting it to a database such as PROSPERO or ClinicalTrials.gov .
Step 3: Search for all relevant studies
Searching for relevant studies is the most time-consuming step of a systematic review.
To reduce bias, it’s important to search for relevant studies very thoroughly. Your strategy will depend on your field and your research question, but sources generally fall into these four categories:
- Databases: Search multiple databases of peer-reviewed literature, such as PubMed or Scopus . Think carefully about how to phrase your search terms and include multiple synonyms of each word. Use Boolean operators if relevant.
- Handsearching: In addition to searching the primary sources using databases, you’ll also need to search manually. One strategy is to scan relevant journals or conference proceedings. Another strategy is to scan the reference lists of relevant studies.
- Grey literature: Grey literature includes documents produced by governments, universities, and other institutions that aren’t published by traditional publishers. Graduate student theses are an important type of grey literature, which you can search using the Networked Digital Library of Theses and Dissertations (NDLTD) . In medicine, clinical trial registries are another important type of grey literature.
- Experts: Contact experts in the field to ask if they have unpublished studies that should be included in your review.
At this stage of your review, you won’t read the articles yet. Simply save any potentially relevant citations using bibliographic software, such as Scribbr’s APA or MLA Generator .
- Databases: EMBASE, PsycINFO, AMED, LILACS, and ISI Web of Science
- Handsearch: Conference proceedings and reference lists of articles
- Grey literature: The Cochrane Library, the metaRegister of Controlled Trials, and the Ongoing Skin Trials Register
- Experts: Authors of unpublished registered trials, pharmaceutical companies, and manufacturers of probiotics
Step 4: Apply the selection criteria
Applying the selection criteria is a three-person job. Two of you will independently read the studies and decide which to include in your review based on the selection criteria you established in your protocol . The third person’s job is to break any ties.
To increase inter-rater reliability , ensure that everyone thoroughly understands the selection criteria before you begin.
If you’re writing a systematic review as a student for an assignment, you might not have a team. In this case, you’ll have to apply the selection criteria on your own; you can mention this as a limitation in your paper’s discussion.
You should apply the selection criteria in two phases:
- Based on the titles and abstracts : Decide whether each article potentially meets the selection criteria based on the information provided in the abstracts.
- Based on the full texts: Download the articles that weren’t excluded during the first phase. If an article isn’t available online or through your library, you may need to contact the authors to ask for a copy. Read the articles and decide which articles meet the selection criteria.
It’s very important to keep a meticulous record of why you included or excluded each article. When the selection process is complete, you can summarise what you did using a PRISMA flow diagram .
Next, Boyle and colleagues found the full texts for each of the remaining studies. Boyle and Tang read through the articles to decide if any more studies needed to be excluded based on the selection criteria.
When Boyle and Tang disagreed about whether a study should be excluded, they discussed it with Varigos until the three researchers came to an agreement.
Step 5: Extract the data
Extracting the data means collecting information from the selected studies in a systematic way. There are two types of information you need to collect from each study:
- Information about the study’s methods and results . The exact information will depend on your research question, but it might include the year, study design , sample size, context, research findings , and conclusions. If any data are missing, you’ll need to contact the study’s authors.
- Your judgement of the quality of the evidence, including risk of bias .
You should collect this information using forms. You can find sample forms in The Registry of Methods and Tools for Evidence-Informed Decision Making and the Grading of Recommendations, Assessment, Development and Evaluations Working Group .
Extracting the data is also a three-person job. Two people should do this step independently, and the third person will resolve any disagreements.
They also collected data about possible sources of bias, such as how the study participants were randomised into the control and treatment groups.
Step 6: Synthesise the data
Synthesising the data means bringing together the information you collected into a single, cohesive story. There are two main approaches to synthesising the data:
- Narrative ( qualitative ): Summarise the information in words. You’ll need to discuss the studies and assess their overall quality.
- Quantitative : Use statistical methods to summarise and compare data from different studies. The most common quantitative approach is a meta-analysis , which allows you to combine results from multiple studies into a summary result.
Generally, you should use both approaches together whenever possible. If you don’t have enough data, or the data from different studies aren’t comparable, then you can take just a narrative approach. However, you should justify why a quantitative approach wasn’t possible.
Boyle and colleagues also divided the studies into subgroups, such as studies about babies, children, and adults, and analysed the effect sizes within each group.
Step 7: Write and publish a report
The purpose of writing a systematic review article is to share the answer to your research question and explain how you arrived at this answer.
Your article should include the following sections:
- Abstract : A summary of the review
- Introduction : Including the rationale and objectives
- Methods : Including the selection criteria, search method, data extraction method, and synthesis method
- Results : Including results of the search and selection process, study characteristics, risk of bias in the studies, and synthesis results
- Discussion : Including interpretation of the results and limitations of the review
- Conclusion : The answer to your research question and implications for practice, policy, or research
To verify that your report includes everything it needs, you can use the PRISMA checklist .
Once your report is written, you can publish it in a systematic review database, such as the Cochrane Database of Systematic Reviews , and/or in a peer-reviewed journal.
A systematic review is secondary research because it uses existing research. You don’t collect new data yourself.
A literature review is a survey of scholarly sources (such as books, journal articles, and theses) related to a specific topic or research question .
It is often written as part of a dissertation , thesis, research paper , or proposal .
There are several reasons to conduct a literature review at the beginning of a research project:
- To familiarise yourself with the current state of knowledge on your topic
- To ensure that you’re not just repeating what others have already done
- To identify gaps in knowledge and unresolved problems that your research can address
- To develop your theoretical framework and methodology
- To provide an overview of the key findings and debates on the topic
Writing the literature review shows your reader how your work relates to existing research and what new insights it will contribute.
Cite this Scribbr article
If you want to cite this source, you can copy and paste the citation or click the ‘Cite this Scribbr article’ button to automatically add the citation to our free Reference Generator.
Turney, S. (2022, October 17). Systematic Review | Definition, Examples & Guide. Scribbr. Retrieved 20 March 2024, from https://www.scribbr.co.uk/research-methods/systematic-reviews/
Is this article helpful?
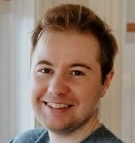
Shaun Turney
Other students also liked, what is a literature review | guide, template, & examples, exploratory research | definition, guide, & examples, what is peer review | types & examples.
Systematic Reviews
- What is a Systematic Review?
A systematic review is an evidence synthesis that uses explicit, reproducible methods to perform a comprehensive literature search and critical appraisal of individual studies and that uses appropriate statistical techniques to combine these valid studies.
Key Characteristics of a Systematic Review:
Generally, systematic reviews must have:
- a clearly stated set of objectives with pre-defined eligibility criteria for studies
- an explicit, reproducible methodology
- a systematic search that attempts to identify all studies that would meet the eligibility criteria
- an assessment of the validity of the findings of the included studies, for example through the assessment of the risk of bias
- a systematic presentation, and synthesis, of the characteristics and findings of the included studies.
A meta-analysis is a systematic review that uses quantitative methods to synthesize and summarize the pooled data from included studies.
Additional Information
- How-to Books
- Beyond Health Sciences
- Cochrane Handbook For Systematic Reviews of Interventions Provides guidance to authors for the preparation of Cochrane Intervention reviews. Chapter 6 covers searching for reviews.
- Systematic Reviews: CRD’s Guidance for Undertaking Reviews in Health Care From The University of York Centre for Reviews and Dissemination: Provides practical guidance for undertaking evidence synthesis based on a thorough understanding of systematic review methodology. It presents the core principles of systematic reviewing, and in complementary chapters, highlights issues that are specific to reviews of clinical tests, public health interventions, adverse effects, and economic evaluations.
- Cornell, Sytematic Reviews and Evidence Synthesis Beyond the Health Sciences Video series geared for librarians but very informative about searching outside medicine.
- << Previous: Getting Started
- Next: Levels of Evidence >>
- Getting Started
- Levels of Evidence
- Locating Systematic Reviews
- Searching Systematically
- Developing Answerable Questions
- Identifying Synonyms & Related Terms
- Using Truncation and Wildcards
- Identifying Search Limits/Exclusion Criteria
- Keyword vs. Subject Searching
- Where to Search
- Search Filters
- Sensitivity vs. Precision
- Core Databases
- Other Databases
- Clinical Trial Registries
- Conference Presentations
- Databases Indexing Grey Literature
- Web Searching
- Handsearching
- Citation Indexes
- Documenting the Search Process
- Managing your Review
Research Support
- Last Updated: Feb 29, 2024 3:16 PM
- URL: https://guides.library.ucdavis.edu/systematic-reviews

About Systematic Reviews
Are Systematic Reviews Qualitative or Quantitative?
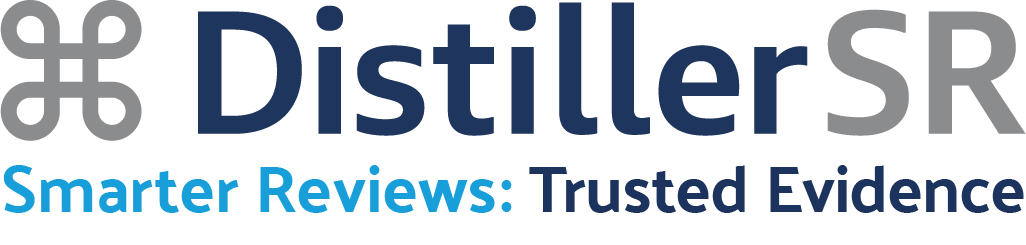
Automate every stage of your literature review to produce evidence-based research faster and more accurately.
A systematic review is designed to be transparent and replicable. Therefore, systematic reviews are considered reliable tools in scientific research and clinical practice. They synthesize the results using multiple primary studies by using strategies that minimize bias and random errors. Depending on the research question and the objectives of the research, the reviews can either be qualitative or quantitative. Qualitative reviews deal with understanding concepts, thoughts, or experiences. Quantitative reviews are employed when researchers want to test or confirm a hypothesis or theory. Let’s look at some of the differences between these two types of reviews.
To learn more about how long it takes to do a systematic review , you can check out the link to our full article on the topic.
Differences between Qualitative and Quantitative Reviews
The differences lie in the scope of the research, the methodology followed, and the type of questions they attempt to answer. Some of these differences include:
Research Questions
As mentioned earlier qualitative reviews attempt to answer open-ended research questions to understand or formulate hypotheses. This type of research is used to gather in-depth insights into new topics. Quantitative reviews, on the other hand, test or confirm existing hypotheses. This type of research is used to establish generalizable facts about a topic.
Type of Sample Data
The data collected for both types of research differ significantly. For qualitative research, data is collected as words using observations, interviews, and interactions with study subjects or from literature reviews. Quantitative studies collect data as numbers, usually from a larger sample size.
Data Collection Methods
To collect data as words for a qualitative study, researchers can employ tools such as interviews, recorded observations, focused groups, videos, or by collecting literature reviews on the same subject. For quantitative studies, data from primary sources is collected as numbers using rating scales and counting frequencies. The data for these studies can also be collected as measurements of variables from a well-designed experiment carried out under pre-defined, monitored conditions.
Data Analysis Methods
Data by itself cannot prove or demonstrate anything unless it is analyzed. Qualitative data is more challenging to analyze than quantitative data. A few different approaches to analyzing qualitative data include content analysis, thematic analysis, and discourse analysis. The goal of all of these approaches is to carefully analyze textual data to identify patterns, themes, and the meaning of words or phrases.
Quantitative data, since it is in the form of numbers, is analyzed using simple math or statistical methods. There are several software programs that can be used for mathematical and statistical analysis of numerical data.
Presentation of Results
Learn more about distillersr.
(Article continues below)
Final Takeaway – Qualitative or Quantitative?
3 reasons to connect.
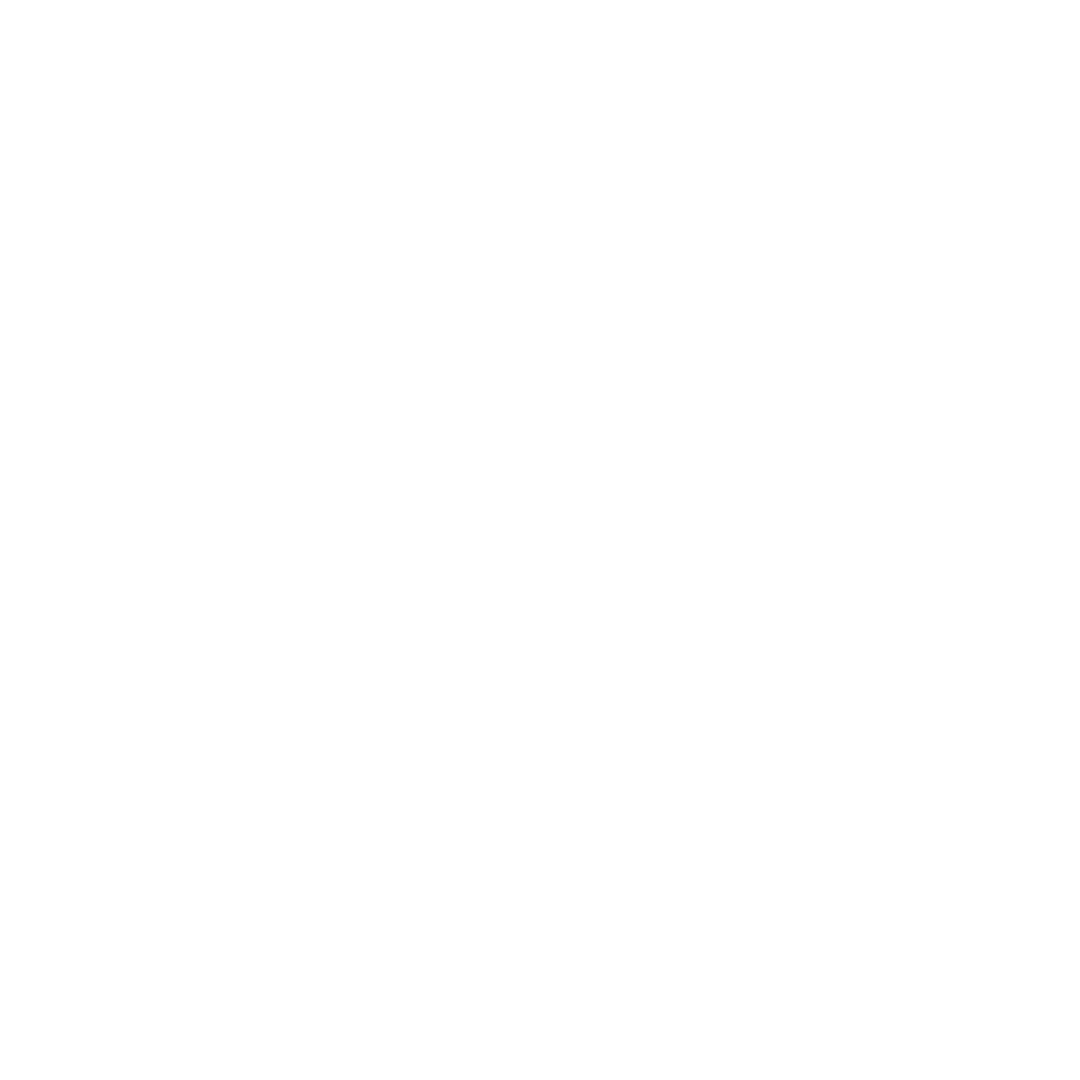
- Quantitative vs. Qualitative Research
Research can be quantitative or qualitative or both:
- A quantitative systematic review will include studies that have numerical data.
- A qualitative systematic review derives data from observation, interviews, or verbal interactions and focuses on the meanings and interpretations of the participants. It may include focus groups, interviews, observations and diaries.
Video source: UniversityNow: Quantitative vs. Qualitative Research
For more information on searching for qualitative evidence see:
Booth, A. (2016). Searching for qualitative research for inclusion in systematic reviews: A structured methodological review. Systematic Reviews, 5 (1), 1–23. https://doi.org/10.1186/S13643-016-0249-X/TABLES/5
- << Previous: Study Types & Terminology
- Next: Critical Appraisal of Studies >>
- Types of Questions
- Key Features and Limitations
- Is a Systematic Review Right for Your Research?
- Integrative Review
- Scoping Review
- Rapid Review
- Meta-Analysis/Meta-Synthesis
- Selecting a Review Type
- Reducing Bias
- Guidelines for Student Researchers
- Training Resources
- Register Your Protocol
- Handbooks & Manuals
- Reporting Guidelines
- PRESS 2015 Guidelines
- Search Strategies
- Selected Databases
- Grey Literature
- Handsearching
- Citation Searching
- Study Types & Terminology
- Critical Appraisal of Studies
- Broad Functionality Programs & Tools
- Search Strategy Tools
- Deduplication Tools
- CItation Screening
- Critical Appraisal Tools
- Quality Assessment/Risk of Bias Tools
- Data Collection/Extraction
- Meta Analysis Tools
- Books on Systematic Reviews
- Finding Systematic Review Articles in the Databases
- Systematic Review Journals
- More Resources
- Evidence-Based Practice Research in Nursing
- Citation Management Programs
- Last Updated: Mar 20, 2024 2:16 PM
- URL: https://libguides.adelphi.edu/Systematic_Reviews

- Duke NetID Login
- 919.660.1100
- Duke Health Badge: 24-hour access
- Accounts & Access
- Databases, Journals & Books
- Request & Reserve
- Training & Consulting
- Request Articles & Books
- Renew Online
- Reserve Spaces
- Reserve a Locker
- Study & Meeting Rooms
- Course Reserves
- Digital Health Device Collection
- Pay Fines/Fees
- Recommend a Purchase
- Access From Off Campus
- Building Access
- Computers & Equipment
- Wifi Access
- My Accounts
- Mobile Apps
- Known Access Issues
- Report an Access Issue
- All Databases
- Article Databases
- Basic Sciences
- Clinical Sciences
- Dissertations & Theses
- Drugs, Chemicals & Toxicology
- Grants & Funding
- Interprofessional Education
- Non-Medical Databases
- Search for E-Journals
- Search for Print & E-Journals
- Search for E-Books
- Search for Print & E-Books
- E-Book Collections
- Biostatistics
- Global Health
- MBS Program
- Medical Students
- MMCi Program
- Occupational Therapy
- Path Asst Program
- Physical Therapy
- Researchers
- Community Partners
Conducting Research
- Archival & Historical Research
- Black History at Duke Health
- Data Analytics & Viz Software
- Data: Find and Share
- Evidence-Based Practice
- NIH Public Access Policy Compliance
- Publication Metrics
- Qualitative Research
- Searching Animal Alternatives
Systematic Reviews
- Test Instruments
Using Databases
- JCR Impact Factors
- Web of Science
Finding & Accessing
- COVID-19: Core Clinical Resources
- Health Literacy
- Health Statistics & Data
- Library Orientation
Writing & Citing
- Creating Links
- Getting Published
- Reference Mgmt
- Scientific Writing
Meet a Librarian
- Request a Consultation
- Find Your Liaisons
- Register for a Class
- Request a Class
- Self-Paced Learning
Search Services
- Literature Search
- Systematic Review
- Animal Alternatives (IACUC)
- Research Impact
Citation Mgmt
- Other Software
Scholarly Communications
- About Scholarly Communications
- Publish Your Work
- Measure Your Research Impact
- Engage in Open Science
- Libraries and Publishers
- Directions & Maps
- Floor Plans
Library Updates
- Annual Snapshot
- Conference Presentations
- Contact Information
- Gifts & Donations
- What is a Systematic Review?
Types of Reviews
- Manuals and Reporting Guidelines
- Our Service
- 1. Assemble Your Team
- 2. Develop a Research Question
- 3. Write and Register a Protocol
- 4. Search the Evidence
- 5. Screen Results
- 6. Assess for Quality and Bias
- 7. Extract the Data
- 8. Write the Review
- Additional Resources
- Finding Full-Text Articles
Review Typologies
There are many types of evidence synthesis projects, including systematic reviews as well as others. The selection of review type is wholly dependent on the research question. Not all research questions are well-suited for systematic reviews.
- Review Typologies (from LITR-EX) This site explores different review methodologies such as, systematic, scoping, realist, narrative, state of the art, meta-ethnography, critical, and integrative reviews. The LITR-EX site has a health professions education focus, but the advice and information is widely applicable.
Review the table to peruse review types and associated methodologies. Librarians can also help your team determine which review type might be appropriate for your project.
Reproduced from Grant, M. J. and Booth, A. (2009), A typology of reviews: an analysis of 14 review types and associated methodologies. Health Information & Libraries Journal, 26: 91-108. doi:10.1111/j.1471-1842.2009.00848.x
- << Previous: What is a Systematic Review?
- Next: Manuals and Reporting Guidelines >>
- Last Updated: Mar 20, 2024 2:21 PM
- URL: https://guides.mclibrary.duke.edu/sysreview
- Duke Health
- Duke University
- Duke Libraries
- Medical Center Archives
- Duke Directory
- Seeley G. Mudd Building
- 10 Searle Drive
- [email protected]
- Open access
- Published: 15 December 2015
Qualitative and mixed methods in systematic reviews
- David Gough 1
Systematic Reviews volume 4 , Article number: 181 ( 2015 ) Cite this article
22k Accesses
46 Citations
23 Altmetric
Metrics details
Expanding the range of methods of systematic review
The logic of systematic reviews is very simple. We use transparent rigorous approaches to undertake primary research, and so we should do the same in bringing together studies to describe what has been studied (a research map) or to integrate the findings of the different studies to answer a research question (a research synthesis). We should not really need to use the term ‘systematic’ as it should be assumed that researchers are using and reporting systematic methods in all of their research, whether primary or secondary. Despite the universality of this logic, systematic reviews (maps and syntheses) are much better known in health research and for answering questions of the effectiveness of interventions (what works). Systematic reviews addressing other sorts of questions have been around for many years, as in, for example, meta ethnography [ 1 ] and other forms of conceptual synthesis [ 2 ], but only recently has there been a major increase in the use of systematic review approaches to answer other sorts of research questions.
There are probably several reasons for this broadening of approach. One may be that the increased awareness of systematic reviews has made people consider the possibilities for all areas of research. A second related factor may be that more training and funding resources have become available and increased the capacity to undertake such varied review work.
A third reason could be that some of the initial anxieties about systematic reviews have subsided. Initially, there were concerns that their use was being promoted by a new managerialism where reviews, particularly effectiveness reviews, were being used to promote particular ideological and theoretical assumptions and to indirectly control research agendas. However, others like me believe that explicit methods should be used to enable transparency of perspectives driving research and to open up access to and participation in research agendas and priority setting [ 3 ] as illustrated, for example, by the James Lind Alliance (see http://www.jla.nihr.ac.uk/ ).
A fourth possible reason for the development of new approaches is that effectiveness reviews have themselves broadened. Some ‘what works’ reviews can be open to criticism for only testing a ‘black box’ hypothesis of what works with little theorizing or any logic model about why any such hypothesis should be true and the mechanisms involved in such processes. There is now more concern to develop theory and to test how variables combine and interact. In primary research, qualitative strategies are advised prior to undertaking experimental trials [ 4 , 5 ] and similar approaches are being advocated to address complexity in reviews [ 6 ], in order to ask questions and use methods that address theories and processes that enable an understanding of both impact and context.
This Special Issue of Systematic Reviews Journal is providing a focus for these new methods of review whether these use qualitative review methods on their own or mixed together with more quantitative approaches. We are linking together with the sister journal Trials for this Special Issue as there is a similar interest in what qualitative approaches can and should contribute to primary research using experimentally controlled trials (see Trials Special Issue editorial by Claire Snowdon).
Dimensions of difference in reviews
Developing the range of methods to address different questions for review creates a challenge in describing and understanding such methods. There are many names and brands for the new methods which may or may not withstand the changes of historical time, but another way to comprehend the changes and new developments is to consider the dimensions on which the approaches to review differ [ 7 , 8 ].
One important distinction is the research question being asked and the associated paradigm underlying the method used to address this question. Research assumes a particular theoretical position and then gathers data within this conceptual lens. In some cases, this is a very specific hypothesis that is then tested empirically, and sometimes, the research is more exploratory and iterative with concepts being emergent and constructed during the research process. This distinction is often labelled as quantitative or positivist versus qualitative or constructionist. However, this can be confusing as much research taking a ‘quantitative’ perspective does not have the necessary numeric data to analyse. Even if it does have such data, this might be explored for emergent properties. Similarly, research taking a ‘qualitative’ perspective may include implicit quantitative themes in terms of the extent of different qualitative findings reported by a study.
Sandelowski and colleagues’ solution is to consider the analytic activity and whether this aggregates (adds up) or configures (arranges) the data [ 9 ]. In a randomized controlled trial and an effectiveness review of such studies, the main analysis is the aggregation of data using a priori non-emergent strategies with little iteration. However, there may also be post hoc analysis that is more exploratory in arranging (configuring) data to identify patterns as in, for example, meta regression or qualitative comparative analysis aiming to identify the active ingredients of effective interventions [ 10 ]. Similarly, qualitative primary research or reviews of such research are predominantly exploring emergent patterns and developing concepts iteratively, yet there may be some aggregation of data to make statements of generalizations of extent.
Even where the analysis is predominantly configuration, there can be a wide variation in the dimensions of difference of iteration of theories and concepts. In thematic synthesis [ 11 ], there may be few presumptions about the concepts that will be configured. In meta ethnography which can be richer in theory, there may be theoretical assumptions underlying the review question framing the analysis. In framework synthesis, there is an explicit conceptual framework that is iteratively developed and changed through the review process [ 12 , 13 ].
In addition to the variation in question, degree of configuration, complexity of theory, and iteration are many other dimensions of difference between reviews. Some of these differences follow on from the research questions being asked and the research paradigm being used such as in the approach to searching (exhaustive or based on exploration or saturation) and the appraisal of the quality and relevance of included studies (based more on risk of bias or more on meaning). Others include the extent that reviews have a broad question, depth of analysis, and the extent of resultant ‘work done’ in terms of progressing a field of inquiry [ 7 , 8 ].
Mixed methods reviews
As one reason for the growth in qualitative synthesis is what they can add to quantitative reviews, it is not surprising that there is also growing interest in mixed methods reviews. This reflects similar developments in primary research in mixing methods to examine the relationship between theory and empirical data which is of course the cornerstone of much research. But, both primary and secondary mixed methods research also face similar challenges in examining complex questions at different levels of analysis and of combining research findings investigated in different ways and may be based on very different epistemological assumptions [ 14 , 15 ].
Some mixed methods approaches are convergent in that they integrate different data and methods of analysis together at the same time [ 16 , 17 ]. Convergent systematic reviews could be described as having broad inclusion criteria (or two or more different sets of criteria) for methods of primary studies and have special methods for the synthesis of the resultant variation in data. Other reviews (and also primary mixed methods studies) are sequences of sub-reviews in that one sub-study using one research paradigm is followed by another sub-study with a different research paradigm. In other words, a qualitative synthesis might be used to explore the findings of a prior quantitative synthesis or vice versa [ 16 , 17 ].
An example of a predominantly aggregative sub-review followed by a configuring sub-review is the EPPI-Centre’s mixed methods review of barriers to healthy eating [ 18 ]. A sub-review on the effectiveness of public health interventions showed a modest effect size. A configuring review of studies of children and young people’s understanding and views about eating provided evidence that the public health interventions did not take good account of such user views research, and that the interventions most closely aligned to the user views were the most effective. The already mentioned qualitative comparative analysis to identify the active ingredients within interventions leading to impact could also be considered a qualitative configuring investigation of an existing quantitative aggregative review [ 10 ].
An example of a predominantly configurative review followed by an aggregative review is realist synthesis. Realist reviews examine the evidence in support of mid-range theories [ 19 ] with a first stage of a configuring review of what is proposed by the theory or proposal (what would need to be in place and what casual pathways would have to be effective for the outcomes proposed by the theory to be supported?) and a second stage searching for empirical evidence to test for those necessary conditions and effectiveness of the pathways. The empirical testing does not however use a standard ‘what works’ a priori methods approach but rather a more iterative seeking out of evidence that confirms or undermines the theory being evaluated [ 20 ].
Although sequential mixed methods approaches are considered to be sub-parts of one larger study, they could be separate studies as part of a long-term strategic approach to studying an issue. We tend to see both primary studies and reviews as one-off events, yet reviews are a way of examining what we know and what more we want to know as a strategic approach to studying an issue over time. If we are in favour of mixing paradigms of research to enable multiple levels and perspectives and mixing of theory development and empirical evaluation, then we are really seeking mixed methods research strategies rather than simply mixed methods studies and reviews.
Noblit G. Hare RD: meta-ethnography: synthesizing qualitative studies. Newbury Park NY: Sage Publications; 1988.
Google Scholar
Barnett-Page E, Thomas J. Methods for the synthesis of qualitative research: a critical review. BMC Med Res Methodol. 2009;9:59.
Article PubMed PubMed Central Google Scholar
Gough D, Elbourne D. Systematic research synthesis to inform policy, practice and democratic debate. Soc Pol Soc. 2002;2002:1.
Moore GF, Audrey S, Barker M, Bond L, Bonell C, Hardeman W, et al. Process evaluation of complex interventions: Medical Research Council guidance 2015. BMJ. 2015;350:h1258
Candy B, Jone L, King M, Oliver S. Using qualitative evidence to help understand complex palliative care interventions: a novel evidence synthesis approach. BMJ Support Palliat Care. 2014;4:Supp A41–A42.
Article Google Scholar
Noyes J, Gough D, Lewin S, Mayhew A, Michie S, Pantoja T, et al. A research and development agenda for systematic reviews that ask complex questions about complex interventions. J Clin Epidemiol. 2013;66:11.
Gough D, Oliver S, Thomas J. Introduction to systematic reviews. London: Sage; 2012.
Gough D, Thomas J, Oliver S. Clarifying differences between review designs and methods. Syst Rev. 2012;1:28.
Sandelowski M, Voils CJ, Leeman J, Crandlee JL. Mapping the mixed methods-mixed research synthesis terrain. J Mix Methods Res. 2012;6:4.
Thomas J, O’Mara-Eves A, Brunton G. Using qualitative comparative analysis (QCA) in systematic reviews of complex interventions: a worked example. Syst Rev. 2014;3:67.
Thomas J, Harden A. Methods for the thematic synthesis of qualitative research in systematic reviews. BMC Med Res Methodol. 2008;8:45.
Oliver S, Rees R, Clarke-Jones L, Milne R, Oakley AR, Gabbay J, et al. A multidimensional conceptual framework for analysing public involvement in health services research. Health Exp. 2008;11:72–84.
Booth A, Carroll C. How to build up the actionable knowledge base: the role of ‘best fit’ framework synthesis for studies of improvement in healthcare. BMJ Qual Saf. 2015. 2014-003642.
Brannen J. Mixed methods research: a discussion paper. NCRM Methods Review Papers, 2006. NCRM/005.
Creswell J. Mapping the developing landscape of mixed methods research. In: Teddlie C, Tashakkori A, editors. SAGE handbook of mixed methods in social & behavioral research. New York: Sage; 2011.
Morse JM. Principles of mixed method and multi-method research design. In: Teddlie C, Tashakkori A, editors. Handbook of mixed methods in social and behavioural research. London: Sage; 2003.
Pluye P, Hong QN. Combining the power of stories and the power of numbers: mixed methods research and mixed studies reviews. Annu Rev Public Health. 2014;35:29–45.
Harden A, Thomas J. Mixed methods and systematic reviews: examples and emerging issues. In: Tashakkori A, Teddlie C, editors. Handbook of mixed methods in the social and behavioral sciences. 2nd ed. London: Sage; 2010. p. 749–74.
Chapter Google Scholar
Pawson R. Evidenced-based policy: a realist perspective. London: Sage; 2006.
Book Google Scholar
Gough D. Meta-narrative and realist reviews: guidance, rules, publication standards and quality appraisal. BMC Med. 2013;11:22.
Download references
Author information
Authors and affiliations.
EPPI-Centre, Social Science Research Unit, University College London, London, WC1H 0NR, UK
David Gough
You can also search for this author in PubMed Google Scholar
Corresponding author
Correspondence to David Gough .
Additional information
Competing interests.
The author is a writer and researcher in this area. The author declares that he has no other competing interests.
Rights and permissions
Open Access This article is distributed under the terms of the Creative Commons Attribution 4.0 International License ( http://creativecommons.org/licenses/by/4.0/ ), which permits unrestricted use, distribution, and reproduction in any medium, provided you give appropriate credit to the original author(s) and the source, provide a link to the Creative Commons license, and indicate if changes were made. The Creative Commons Public Domain Dedication waiver ( http://creativecommons.org/publicdomain/zero/1.0/ ) applies to the data made available in this article, unless otherwise stated.
Reprints and permissions
About this article
Cite this article.
Gough, D. Qualitative and mixed methods in systematic reviews. Syst Rev 4 , 181 (2015). https://doi.org/10.1186/s13643-015-0151-y
Download citation
Received : 13 October 2015
Accepted : 29 October 2015
Published : 15 December 2015
DOI : https://doi.org/10.1186/s13643-015-0151-y
Share this article
Anyone you share the following link with will be able to read this content:
Sorry, a shareable link is not currently available for this article.
Provided by the Springer Nature SharedIt content-sharing initiative
Systematic Reviews
ISSN: 2046-4053
- Submission enquiries: Access here and click Contact Us
- General enquiries: [email protected]
- Open access
- Published: 18 March 2024
A mixed methods analysis of the medication review intervention centered around the use of the ‘Systematic Tool to Reduce Inappropriate Prescribing’ Assistant (STRIPA) in Swiss primary care practices
- Katharina Tabea Jungo 1 , 13 ,
- Michael J. Deml 2 ,
- Fabian Schalbetter 1 ,
- Jeanne Moor 1 , 3 ,
- Martin Feller 1 ,
- Renata Vidonscky Lüthold 1 , 12 ,
- Johanna Alida Corlina Huibers 4 ,
- Bastiaan Theodoor Gerard Marie Sallevelt 5 ,
- Michiel C Meulendijk 6 ,
- Marco Spruit 6 , 7 , 8 ,
- Matthias Schwenkglenks 9 , 10 , 11 ,
- Nicolas Rodondi 1 , 3 &
- Sven Streit 1
BMC Health Services Research volume 24 , Article number: 350 ( 2024 ) Cite this article
1585 Accesses
1 Altmetric
Metrics details
Electronic clinical decision support systems (eCDSS), such as the ‘Systematic Tool to Reduce Inappropriate Prescribing’ Assistant (STRIPA), have become promising tools for assisting general practitioners (GPs) with conducting medication reviews in older adults. Little is known about how GPs perceive eCDSS-assisted recommendations for pharmacotherapy optimization. The aim of this study was to explore the implementation of a medication review intervention centered around STRIPA in the ‘Optimising PharmacoTherapy In the multimorbid elderly in primary CAre’ (OPTICA) trial.
We used an explanatory mixed methods design combining quantitative and qualitative data. First, quantitative data about the acceptance and implementation of eCDSS-generated recommendations from GPs ( n = 21) and their patients ( n = 160) in the OPTICA intervention group were collected. Then, semi-structured qualitative interviews were conducted with GPs from the OPTICA intervention group ( n = 8), and interview data were analyzed through thematic analysis.
In quantitative findings, GPs reported averages of 13 min spent per patient preparing the eCDSS, 10 min performing medication reviews, and 5 min discussing prescribing recommendations with patients. On average, out of the mean generated 3.7 recommendations (SD=1.8). One recommendation to stop or start a medication was reported to be implemented per patient in the intervention group (SD=1.2). Overall, GPs found the STRIPA useful and acceptable. They particularly appreciated its ability to generate recommendations based on large amounts of patient information. During qualitative interviews, GPs reported the main reasons for limited implementation of STRIPA were related to problems with data sourcing (e.g., incomplete data imports), preparation of the eCDSS (e.g., time expenditure for updating and adapting information), its functionality (e.g., technical problems downloading PDF recommendation reports), and appropriateness of recommendations.
Conclusions
Qualitative findings help explain the relatively low implementation of recommendations demonstrated by quantitative findings, but also show GPs’ overall acceptance of STRIPA. Our results provide crucial insights for adapting STRIPA to make it more suitable for regular use in future primary care settings (e.g., necessity to improve data imports).
Trial registration
Clinicaltrials.gov NCT03724539, date of first registration: 29/10/2018.
Peer Review reports
Globally the proportion of adults with multimorbidity has increased in past decades [ 1 , 2 ]. More than 50% of older adults aged ≥ 65 years have several chronic conditions [ 3 ]. The coexistence of ≥ 2 chronic conditions is commonly referred to as multimorbidity [ 4 ]. Multimorbidity is usually accompanied by polypharmacy, which can be defined as the concurrent, regular intake of ≥ 5 medications [ 5 ]. The higher the number of medications used, the more likely older adults are to have potentially inappropriate polypharmacy, which not only consists of the use of inappropriate medications, but also prescribing omissions [ 6 , 7 , 8 , 9 , 10 ]. The use of potentially inappropriate medications, highly prevalent in older adults with multimorbidity and polypharmacy [ 11 ], is associated with an increased risk of adverse drug events, falls, and cognitive decline in older adults [ 12 , 13 , 14 , 15 , 16 ]. This in turn is associated with increased health services use, such as hospitalizations or emergency department visits, and higher healthcare costs. Hence, optimizing medication use of older adults with multimorbidity and polypharmacy is a crucial task.
However, performing medication reviews is time-consuming and can be challenging, especially in a context in which time allocated to treating individual patients is short, as is commonly the case in primary care settings, and large amounts of patient information need to be processed (e.g., medications, diagnoses, lab values, patient preferences). Considering new possibilities available through the digital revolution, electronic clinical decision support systems (eCDSS) can be a useful tool for supporting healthcare professionals, when performing medication reviews. eCDSS are software-based tools, able of managing large amounts of data and designed to be a direct aid to clinical decision making [ 17 ]. They are capable of matching information, such as evidence-based clinical recommendations (e.g., guidelines), with patient information and can thereby generate patient-specific recommendations.
One such eCDSS is the ‘Systematic Tool to Reduce Inappropriate Prescribing’ Assistant (STRIPA). It is based on the algorithms of the ‘Screening Tool to Alert doctors to Right Treatment’ (START) and ‘Screening Tool of Older Person’s Prescriptions’ (STOPP) version 2 [ 18 ]. The STOPP/START criteria are the most widely used and extensively studied explicit screening tool to detect potentially inappropriate prescribing in older patients in Europe [ 19 , 20 ]. While the STOPP criteria highlight situations of potentially inappropriate medication use (e.g., overprescribing, drug-drug interactions, drug-disease interactions, incorrect dosages), the START criteria indicate potential prescribing omissions. The STRIPA generates patient-specific recommendations, based on the STOPP and START criteria, by considering medication lists, diagnoses, and selected lab values [ 21 ]. It is thus a promising tool for optimizing pharmacotherapy in older adults and has been tested in two clinical trials to determine if its use can improve clinical outcomes (e.g., European multicenter hospital-based OPERAM trial in Switzerland, the Netherlands, Belgium and Ireland [ 22 , 23 ], OPTICA trial in Swiss primary care settings [ 24 , 25 , 26 ].
The use of eCDSS has been shown to be beneficial for certain medication-related outcomes, such as reductions of medication errors, improvements in prescribing quality and decreases in the use of potentially inappropriate medications, which in turn leads to increased medication safety [ 27 , 28 , 29 ]. However, the evidence supporting the use of eCDSS largely focuses on hospital settings and results are mixed for primary care settings [ 30 ]. More specifically, current evidence shows high variability in the effectiveness and implementation of such tools in primary care settings and reports implementation challenges (e.g., time-consuming data entry, alert fatigue) [ 31 , 32 , 33 , 34 ]. Such documented problems related to implementing these tools can be hypothesized to have negatively influenced the impact of their use. Consequently, studying eCDSS implementation in primary care settings is crucial, as this will influence the future development of effective implementation strategies. In this context, the present study aimed to explore the implementation of the medication review intervention centered on the use of the STRIPA during the ‘Optimising PharmacoTherapy In the multimorbid elderly in primary CAre’ (OPTICA) trial conducted in Swiss primary care settings by using an explanatory mixed-methods approach. Our goal was to analyze the number of prescribing recommendations generated and implemented, the time expenditure for performing the intervention, and the key themes emerging from interviewing general practitioners (GPs) about their use of the intervention.
This research was embedded in the OPTICA trial [ 26 ], a cluster randomized controlled trial in Swiss primary care practices conducted by an interdisciplinary and interprofessional team (e.g., GPs, epidemiologists, etc.). The main goal of this trial was to investigate whether the use of a structured medication review intervention centered around the use of an eCDSS, namely the ‘Systematic Tool to Reduce Inappropriate Prescribing’ Assistant (STRIPA), helps to improve the medication appropriateness and reduce prescribing omissions in older multimorbid adults with polypharmacy compared to a medication discussion between GPs and patients [ 24 , 25 , 26 ]. The details of the trial protocol and the baseline characteristics of study participants have previously been reported [ 24 , 25 ]. Fig 1 provides an overview of the different steps of the intervention. In addition to detecting potential overuse, underuse, and misuse of drugs, STRIPA generated prescribing recommendations to prevent drug-drug interactions and inappropriate dosages, by combining both implicit and explicitly tools to improve appropriate prescribing [ 21 ]. The version of the STRIPA used for the OPTICA trial had been adapted for use in primary care settings from the STRIPA version used in the OPERAM trial conducted in four European countries, in which the medication review intervention was done during hospitalization [ 22 , 23 , 35 ]. The data on medications, coded diagnoses, laboratory values, and vital signs originating from the electronic health records (EHR) of participating GPs and their patients were imported into the STRIPA by the study team after they were obtained from the ‘Family Medicine ICPC-Research using Electronic Medical Records’ (FIRE) EHR database [ 36 ]. Trial participants were ≥ 65 years old, had ≥ 3 chronic conditions, regularly used ≥ 5 medications and were followed-up for 12 months. In the intervention arm GPs used the STRIPA to perform a medication review and engaged in shared-decision making with patients. Trial results were inconclusive on whether the medication review intervention centered around the use of an eCDSS led to an improvement in medication appropriateness or a reduction in prescribing omissions at 12 months compared to a medication discussion in line with usual care (without medication review). Nevertheless, the intervention was safely delivered without causing any harm to patients and led to the implementation of several prescribing recommendations [ 26 ].
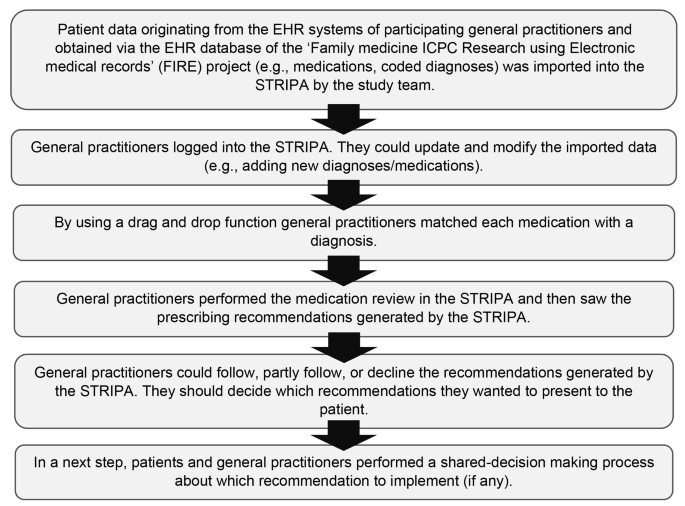
Schema of the six steps of the OPTICA study intervention using the ‘Systematic Tool to Reduce Inappropriate Prescribing’ (STRIP) assistant. Adapted from: Jungo et al. [ 24 ]
Study design
In this sub-study, we used a mixed methods design in which we combined information collected from participating GPs on the prescribing recommendations generated and implemented and semi-structured interviews with GPs from the OPTICA intervention group. In an explanatory approach, we first collected quantitative data, which we sought to subsequently further explain and understand through qualitative methods [ 37 ]. We reported the findings of this study according to the CRISP statement [ 38 ].
Participants
In both the quantitative and qualitative part of the research project, the study participants were the GPs who were randomly assigned to the intervention arm of the OPTICA trial ( n = 21).
Data collection
Quantitative component.
Since during the trial all GPs from the OPTICA intervention group had access to the medication review intervention centered around STRIPA and were asked to perform it with their recruited patients, we invited all of them to report information on the use of the intervention in the REDCap study database. This covered the number of generated and the implemented prescribing recommendations, which are relevant outcomes to study the implementation of a medication review intervention. In addition, GPs had the option of providing free text responses on why they did not implement any prescribing recommendations. KTJ verified the entries in REDCap and completed them with information available in STRIPA. The following variables were collected for each recommendation generated: name of the recommendation, type of the recommendation, whether the recommendation was presented to the patient, and (if applicable) whether the recommendation was implemented. Furthermore, GPs directly reported the time used to prepare and conduct the medication review as well as the time spent on the shared decision-making with the patient. Quantitative data were collected between May 2019 and February 2020.
Qualitative component
We performed semi-structured interviews with a purposive sample of intervention group GPs who had been included in the OPTICA study. Interviews were conducted by FS in Swiss German and transcribed verbatim to High German. The interview guide included questions related to GPs’ attitudes towards treating older adults with multimorbidity and polypharmacy, the conduct of the medication review intervention tested during the OPTICA trial, and GPs’ general attitudes towards the use of eCDSS for optimizing prescribing practices (Appendix 1 in the Supporting Material). Preliminary quantitative data were used to inform the interview guide (e.g., quantitative findings about the implementation of prescribing recommendations and the use of the eCDSS, such as “We saw that it took around 40 minutes to prepare and perform the intervention. How does that compare to your experience during the trial when conducting the intervention?”), so that GPs could provide information on their perspective. Interviews were audio-recorded and transcribed into text for analysis. Interviews were conducted between October 2019 and February 2020.
Data analysis
We described participant baseline characteristics and performed descriptive analyses. We calculated the total number of recommendations generated per study participant in the OPTICA intervention arm. We then calculated the number of recommendations physicians reported to have discussed with patients and the number implemented after shared decision-making. In addition, we calculated the average time spent on preparing and conducting medication reviews and the average time of shared decision-making consultations. Since some variables were non-normally distributed (visual test), we present mean (standard deviation) and median (interquartile range). We performed all analyses with Stata 15.1 (StataCorp, College Station, TX, USA) [ 39 ].
We analyzed the qualitative data with thematic analysis , which is a commonly used tool to identify and analyze patterns in qualitative data [ 40 ]. We used a mix of deductive and inductive coding, with deductive coding allowing us to expand on specific findings from the quantitative results and inductive coding allowing us to interpret any surprising findings we had not expected. Three of the investigators (KTJ, MJD, FS) contributed to the identification of themes. Consensus was reached by discussing the themes that were independently identified. In addition, we used the Framework method by Gale et al. to structure our analyses [ 41 ]. We used the software TamsAnalyzer to code and organize qualitative data into meaningful themes [ 42 ].
Baseline characteristics
There were a total of 21 GPs and 160 of their patients in the intervention group. Table 1 provides baseline characteristics of the GPs and patients in the OPTICA intervention group.
Table 2 shows the expenditure of time, per patient, for the preparation of the STRIPA, the conduct of the medication review intervention, as well as the duration of discussion with the patient. We observed that the drag/drop function to assign drugs to medical conditions in the STRIPA had been used for 133 out of the 160 patients in the intervention group, by 20 of the 21 GPs. GPs in the intervention group conducted a mean of 6 medication reviews (median = 7). For the 133 patients, a minimum of one prescribing recommendation had been generated for 130 patients (97.7%). A total of 704 prescribing recommendations had been generated for patients in the intervention group [ 26 ]. For the 133 patients, an average of 3.7 STOPP/START recommendations (SD 1.8, range: 0–11, median = 3, IQR = 2–5) was generated by STRIPA per patient. The mean number of STOPP recommendations generated by STRIPA was 2.3 (SD 1.3, range: 0–7, median = 2, IQR = 1–3) per patient and the mean number of generated START recommendations was 1.3 (SD 1.2, range: 0–6, median = 1, IQR = 1–2). For 53 patients in the intervention group, 10 of the GPs provided information on the implementation of prescribing recommendations. For 31 out of the 53 patients (58.5%) at least one prescribing recommendation was reported to have been implemented. On average, 1 recommendation to stop or start a medication was reported as implemented per patient (SD = 1.2, median = 1, IQR = 0–2). The most common reasons why GPs reported not implementing the prescribing recommendations were: beliefs that current prescriptions were beneficial for patients, recommendations were not suitable for patients, and bad experiences with previous medication changes.
Quantitative findings
Qualitative findings.
Overall, semi-structured interviews were conducted with 8 of the 21 GPs randomized to the intervention group. The qualitative results allowed us to focus more specifically on GP perspectives on, and experiences with, STRIPA and to support our understanding of the limited implementation documented in the quantitative findings (e.g., significant time expenditure and limited implementation of prescribing recommendations). GPs generally appreciated the fact that the STRIPA was able to manage a large amount of data and to generate different types of prescribing recommendations, such as discontinuing or initiating medications. Despite this general appreciation, we identified the following themes as being barriers for GPs for STRIPA use: length of time for STRIPA preparation, problems with data sources, and poor data quality, sub-optimal functionality, limited recommendation practicability, and problems related to the implementation of recommendations.
Preparation
Most GPs mentioned that the coding of diagnoses (to ICPC-2) in their EHR systems was a time-consuming and cumbersome task because most did not routinely use it prior to the beginning of the trial. GPs found the expenditure of time to prepare the STRIPA, including the coding of diagnoses, too high. For instance, one GP (male, 57 years) stated, “ I was a little overwhelmed by the administrative burden ”. It also became clear that the lengthy time expenditure involved in preparing the STRIPA would be a limiting factor for the tool’s future use: “if time expenditure remains that high, the STRIPA has no chance of being used in clinical practice ” (GP, male, 44 years). It was also stated that this long preparation time would not have made it possible for GPs to use the tool during the consultations with patients present.
Data import
Another major theme involved sub-optimal completeness of data imported from EHR systems to web-based STRIPA, which created additional work for GPs. Problems with data imports were multifaceted. First, not all information needed for STRIPA use was systematically captured in EHR systems and fully exported to the FIRE project database. For instance, this concerned unstructured information in text fields and lab values for which the FIRE team did not yet standardize imports into their database. Second, there was a time lag of up to a couple of weeks, because as explained above, data were transferred via data exports from the physicians' EHR systems to the FIRE project database and then back to the STRIPA. This required data to be updated and verified once they were in the STRIPA. Overall, GPs expressed that this time-consuming data updating and correcting was a limiting factor for future use of the STRIPA: “I had to capture quite a lot of information by hand, and that is of course terribly tedious and time-consuming and thus not suitable for daily practice ” (GP, male, 44 years Footnote 1 ). Some GPs mentioned how they would have appreciated an automated data transfer from the EHR system used in their GP office to the STRIPA, as this would have facilitated their use of the tool.
Functions and features
Overall, GPs reported to be satisfied with the functions and features of the STRIPA. For instance, GPs appreciated STRIPA’s ability to incorporate a wide variety of values into analysis (i.e., different lab values, medication lists, diagnoses, vital signs), which they would not have been able to do manually. Further, GPs described how they appreciated the varied types of prescribing recommendations, since this highlighted different types of prescribing-related problems. However, not all GPs thought the tool was intuitive to use. Further, some GPs reported technical problems when using the tool (e.g., long buffering when loading a new page or the next step of the analyses, problems with downloading PDF reports). GPs also noted a learning effect (e.g., after getting to know the tool, GPs were able to perform the subsequent reviews faster).
GPs’ perceptions of the suitability and practicability of recommendations
GPs reported being satisfied with the overall quality of recommendations. However, GPs emphasized that recommendations were not always suitable, practicable or clinically relevant. First, due to the above-mentioned problems with data imports, recommendations were sometimes not applicable for patients. For example, there may have been valid reasons why certain medications were prescribed at certain doses, and these reasons were not captured in the STRIPA. Second, recommendations were sometimes not suitable because of the seasonality of recommendation (i.e., influenza vaccine: most GPs used the STRIPA in spring 2019, which did not correspond to the influenza vaccination season). Furthermore, in the EHR systems GPs usually did not list the influenza vaccine to the regular medications used by their patients, which is why the recommendation to vaccinate appeared, irrespective of whether the patient had been vaccinated in the past fall. Third, in some cases, the STRIPA could not use all information provided (e.g., it did not capture that some medications had several active ingredients). In some instances, GPs reported not implementing certain recommendations as they did not believe that these recommendations would change patient health-status or well-being.
Further, some recommendations were perceived as too basic and therefore not useful for experienced GPs. One GP put it like this: “ Some of the information provided is not necessary for an experienced general practitioner ” (GP, male, 44 years). In some instances, the STRIPA generated prescribing recommendations that were already known to the GPs but had deliberately not been implemented for specific reasons, such as patient preferences. Another GP explicitly stated that he had wished for more “courageous” recommendations, which would have gone beyond the “evident” recommendations and would have challenged his previous prescribing decisions. GPs, however, also emphasized how the generation of only few recommendation for some of patients confirmed their prescribing decisions and work as physicians: “ I was happy, that the medication was not questioned in general. Otherwise, I would have had to doubt the quality of my work ” (GP, male, 44 years). The recommendations, or rather the lack thereof, was perceived as a confirmation of quality work by some GPs.
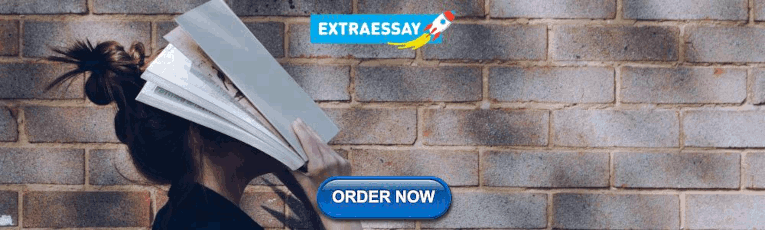
Implementation of prescribing recommendations
The implementation of prescribing recommendations generated by the STRIPA was one of the themes that was discussed during the interviews. In general, GPs confirmed the relatively low implementation rate with only a fraction of recommendations being implemented, which is in line with our first step’s quantitative findings. However, interviews showed differences between GPs in terms of how many recommendations they reported having implemented. Because the STRIPA sometimes did not capture all nuances of patient health status, GPs often had valid reasons to reject generated recommendations. Consequently, only a small percentage of recommendations was presented to and discussed with patients. One GP, however, also told us that while he was not able to implement many recommendations directly, seeing them with the tool helped him to become aware of potential prescribing problems. With regards to the implementation of recommendations that they deemed feasible, some GPs reported challenges when respect to presentation to patients. One GP expressed it like this: “You have to be careful not to make yourself ‘lower’ than you are as a doctor. You should radiate a certain competence and not give the impression ‘I need a computer to help me treat you.’ Otherwise, it’ll be too complicated ” (male, 44 years).
Finally, the overall impressions of GPs were that the STRIPA was a potentially useful tool, but that its functionality was not ideal for regular use in clinical practice. For instance, a GP (male, 57 years) said, “ The STRIPA is actually very useful, even in the way in which it works right now, but it is too complex for everyday use. ” Another GP (male, 44 years) echoed this sentiment, “ If the STRIPA wants to get a chance, it has to run a lot smarter ,” meaning that data entry should be fully automated. Overall, while some GPs stated that their expectations were met, others stated that they were disappointed by the tool.
This mixed-methods study set out to explore the conduct of a medication review intervention centered around the use of the STRIPA in a real-life clinical setting during the OPTICA trial, a cluster-randomized controlled trial conducted in Swiss primary care settings. Our quantitative findings show that the expenditure of time for the preparation and use of the STRIPA as well as for the discussion of the recommendations generated was substantial, which may have limited the overall implementation of the intervention. Further, a small percentage of recommendations generated by the tool were presented to patients and implemented. The qualitative part of the study helped to explain the quantitative findings and showed that the main reasons for limited implementation of the STRIPA were related to problems with the data source, preparation of the eCDSS and its functionality, as well as the practicability of generated prescribing recommendations.
Time factor
Both our quantitative and qualitative findings showed substantial time expenditures were required to prepare STRIPA, to run analyses and to discuss recommendations with patients. This finding is in line with the results from a process evaluation of a deprescribing intervention based on an eCDSS, in which GPs mainly reported retrieving additional information for the use of the tool to be time-consuming and inconvenient [ 32 ]. A previous study on the efficiency of medication reviews performed with the STRIPA showed that the time expenditure declined as professionals gained more experience (e.g., from around 20 to around 10 min per review) [ 43 ]. We unfortunately do not have any data to make comparisons about the time needed for medication review based on the STRIPA to other medication reviews performed by the same GPs in our sample.
Data handling
Another major implementation challenge that we observed involved problems with data imports and the cumbersome nature of manual data entry, which was partially needed to add or update missing or incorrect information. In the OPTICA trial, the purpose of using data from electronic health records was to facilitate data entry for GPs. Despite this, most GPs reported that they had to spend a relatively large amount of time to manually update and add information as shown by the quantitative data (e.g., code diagnoses, update medication lists due to frequent changes in older multimorbid patients). In most cases, this was due to time lags following latest exports to the FIRE project database, which may have rendered an update necessary. There were also issues because not all data from the physicians’ electronic health record systems could be exported to FIRE (e.g., unstructured text information or certain lab values collected with different measurement units in different reference laboratories) and because different EHR systems exported data differently (e.g., reporting of medications and diagnoses at every encounter vs. reporting only when changes are made in the record). Some GPs criticized “missing information” in the data that had been imported into the STRIPA from their electronic health records programs via the FIRE project database. This may have resulted from GPs not knowing how data exported to the FIRE project were structured (i.e., that they were limited to selected values, or that data had to figure in the EHR system for a certain amount of time before inclusion in an export, which is why last-minute updates before an export may not have been captured).
Another main barrier to the use of the STRIPA, which was shown by the quantitative findings and explained by the qualitative findings, was the relatively low implementation rate of recommendations generated by the tool. These findings are similar to previous ones from trials testing an eCDSS based on the STOPP/START criteria in hospital settings [ 23 , 44 , 45 ], one of which showed that 15% of all prescribing recommendations were implemented and the other one showed that 62% of patients had had ≥ 1 recommendation successfully implemented 2 months post-recommendation. Additionally, previous research on the usability of eCDSS-assisted de-prescribing found that 32% of GPs reported not having implemented any recommendation [ 33 ]. Interestingly, there seemed to be a wide variability between different GPs in previous studies. For instance, researchers found that while some GPs implemented nearly all generated recommendations, others implemented few or none [ 32 ]. While there is limited data about this in our study due to the small sample size, our findings suggest variability between GPs with regards to the implementation of prescribing recommendations (with the mean number of recommendations implementing ranging from 0.3 to 2.3). Furthermore, previous research has shown that more experienced healthcare professionals were more likely to disregard and reject recommendations [ 46 ]. Of note, a low implementation rate based upon generated recommendations is not necessarily bad; GPs may have had valid reasons for not implementing recommendations (e.g., recommendation not being appropriate for the patient, etc.), and it is not expected that every single prescribing recommendation should be implemented. A critical review of prescribing recommendations generated by eCDSS by clinicians is always required, as these tools can support clinicians but not replace their clinical judgment.
The reasons for implementation problems reported in the literature were similar to what we found in our qualitative analysis [ 32 , 33 ]. First, the eCDSS did not capture all relevant patient-specific information, which is why some recommendations were not appropriate. This aligns with findings from the OPERAM trial, which had tested the STRIPA in hospital settings across four European countries and during which the medication review intervention was done during hospitalization [ 45 ]. Second, there were difficulties in implementing recommendations when prescribing decisions had been made by other medical specialists. Third, GPs’ or patients’ hesitancy toward medication changes can be major barriers to implementing recommendations. This is also reflected in the findings from the OPERAM trial, which found that the main reason for not implementing a recommendation was patients’ reluctance to change their medication use [ 45 , 47 ]. These challenges need to be considered when further developing eCDSS. Despite the potentially low immediate implementation of recommendations, research shows that the use of eCDSS can be a useful tool to start reflections and discussions about patient medication use [ 48 ]. Hence, eCDSS-based interventions can positively influence GPs’ prescribing behaviors, as GPs have reported an increased awareness of prescribing problems after using a CDSS [ 33 ].
Even though some GPs reported a learning effect when performing the medication review using the STRIPA, we retrospectively assume that an average of 6 medication reviews may not have been enough to benefit from this learning effect. Performing such a small number of medication reviews using the STRIPA may not have allowed GPs to incorporate the use of the tool in their workflow in an efficient manner. Fragmented workflows are a commonly reported problem linked to the use of eCDSS, as these tools are often designed without considering the human information processing and behaviors [ 46 ]. While providing assistance to participating GPs during the study intervention, our study team noticed that the computer literacy differed between participating GPs. We assume that this influenced the STRIPA use during the trial. Consequently, working on better integrating the use of the STRIPA into the routine clinical practice of GPs and adapting it to computer literacy levels of individual GPs may be crucial for a successful implementation of eCDSS in primary care settings.
Willingness to use eCDSS
Our findings showed that overall GPs would be willing to use eCDSS, such as the STRIPA, for medication review if the above-mentioned issues were addressed. This openness to using eCDSS is reflected in previous research [ 32 ]. In one study, 65% of respondents mentioned that they would be willing to use eCDSS in routine practice if the CDSS was integrated into their EHR system [ 33 ]. In addition to this, there would have to be minimal data entry so that the additional expenditure of time for using a tool would be as short as possible. Further, it is necessary that algorithms behind eCDSS must regularly be updated (e.g., with latest guidelines) [ 48 ]. Finally, our research clearly shows that simply providing new eCDSS to GPs is not sufficient and does not automatically translate into implementation of prescribing recommendations. GPs need to be supported with communication strategies on how to conduct shared decision-making with patients and strategies on how to overcome their own barriers to inappropriate prescribing.
Overall, qualitative findings suggest that GPs were dissatisfied with reoccurring problems when using the STRIPA (e.g., problems with data entry, generation of recommendations that GPs did not deem useful). Consequently, apart from solving technical issues and improving data imports, it will be crucial to work on presenting recommendations in a way that is perceived as useful by GPs. This is crucial, because instead of GPs focusing their energy on discarding non-useful recommendations, they should be able to focus on other potentially useful recommendations for prescribing decisions with older adults with multimorbidity and polypharmacy.
Need for interoperable electronic health record systems in Swiss primary care settings
Direct, fully automated imports from the physicians’ EHR systems into the STRIPA would not have been technically feasible due to the multiple different EHR software providers used in the Swiss German language region of Switzerland. It thus made sense to collaborate with the FIRE project, as this was the best available option operationalizing EHR data for a clinical trial with an eCDSS in Switzerland. This mixed-methods study, however, shows this approach’s limitations. This should be a wake-up call for Swiss software developers to implement industrial standards allowing different EHR systems to be compatible with one another (e.g., feed data from one software into another, combine data from different software). In the future, this would allow easier use of eCDSS, such as the STRIPA. In addition, efforts should be made to make the coding of ICPC-2 diagnoses more common in Swiss primary care settings. At the moment, diagnostic coding is not commonly done in routine care, which affects the feasibility of implementing tools like the STRIPA.
Increasingly digitalized healthcare systems and readily available health data will allow the widespread use of eCDSS in the future. However, digitalization alone will not provide a sufficient basis for eCDSS to be used efficiently. Clinical practice and research must address the shortcomings identified in our research and in previous studies. In particular, approaches need to be developed to better integrate eCDSS into clinical workflows in primary care settings. Furthermore, EHR systems must become more interoperable for eCDSS to be effectively integrated into clinical workflows, so that data from different sources can be used reliably. If these challenges are successfully addressed, eCDSS can become a useful tool supporting physicians in primary care settings for optimizing prescribing practices.
Strengths & limitations
The combined analyses of both quantitative and qualitative data allowed for better data triangulation and strengthened our findings. However, this mixed methods study has several limitations. First, since there were problems when generating PDF reports at the end of the STRIPA use, we had to retrospectively collect information on the prescribing recommendations by manually exporting them from the STRIPA. This came with the downside that we could only see which recommendations were generated, but not which ones had been accepted by GPs. This is why we had to rely on self-reported information from GPs regarding their acceptance of prescribing recommendations. Second, despite sending multiple reminders to GPs, we were faced with a small sample size and significant amount of missing quantitative data, as only 7 out of 21 GPs reported information about implementing prescribing recommendations, and only 8 out of 21 GPs agreed to be interviewed. Further, the sample of GPs mostly consisted of male GPs, which, in addition to the small sample size, could have limited the generalizability of findings. Next, we would like to acknowledge that the GPs who agreed to participate in the OPTICA trial and the qualitative interview were likely not representative of all GPs practicing in Swiss primary care settings. Finally, we did not consider patient perspectives on the conduct of the medication review intervention, which represents an important opportunity for future studies.
Overall, GPs found the STRIPA useful, particularly due to its ability to generate recommendations based on large amounts of data. During the OPTICA trial, however, general practitioners only discussed and implemented a fraction of the recommendations generated by the STRIPA. Issues related to the STRIPA’s usability, general practitioners’ high expectations about the tool’s functionalities, data management, and time expenditure involved with preparing the STRIPA for analysis were important barriers described during semi-structured interviews. The qualitative findings help explain the low acceptance and implementation rate of the recommendations. Due to a learning effect, a decline in the expenditure of time needed to perform medication reviews with the STRIPA would be expected if GPs continued to use this tool more regularly and with more patients. In its current form, it is unlikely that the STRIPA will be implemented more broadly. Our results, however, are crucial for designing and adapting eCDSS like STRIPA in a meaningful way to make them more feasible and acceptable to providers and more suitable for regular use in primary care settings on a larger scale, as this will become increasingly possible in the context of digitalized healthcare systems.
Data availability
We will make the data for this study available to other researchers upon request. The data will be made available for scientific research purposes, after the proposed analysis plan has been approved. Data and documentation will be made available through a secure file exchange platform after approval of the proposal. In addition, a data transfer agreement must be signed (which defines obligations that the data requester must adhere to regarding privacy and data handling). Deidentified participant data limited to the data used for the proposed project will be made available, along with a data dictionary and annotated case report forms. For data access, please contact the corresponding author.
Several GPs were male and 44 years old at the time of the interview.
Roig JJ, Souza D, Oliveras-Fabregas A, Minobes-Molina E, Cancela MdC, Galbany-Estragués P. Trends of multimorbidity in 15 European countries: a population-based study in community-dwelling adults aged 50 and over. Research Square; 2020.
Chowdhury SR, Chandra Das D, Sunna TC, Beyene J, Hossain A. Global and regional prevalence of multimorbidity in the adult population in community settings: a systematic review and meta-analysis. EClinicalMedicine. 2023;57:101860.
Article PubMed PubMed Central Google Scholar
Marengoni A, Angleman S, Melis R, Mangialasche F, Karp A, Garmen A, et al. Aging with multimorbidity: a systematic review of the literature. Ageing Res Rev. 2011;10(4):430–9.
Article PubMed Google Scholar
Johnston MC, Crilly M, Black C, Prescott GJ, Mercer SW. Defining and measuring multimorbidity: a systematic review of systematic reviews. Eur J Public Health. 2019;29(1):182–9.
Masnoon N, Shakib S, Kalisch-Ellett L, Caughey GE. What is polypharmacy? A systematic review of definitions. BMC Geriatr. 2017;17(1):230.
Bazargan M, Smith JL, King EO. Potentially inappropriate medication use among hypertensive older African-American adults. BMC Geriatr. 2018;18(1):238.
Simões PA, Santiago LM, Maurício K, Simões JA. Prevalence of potentially inappropriate medication in the older Adult Population within Primary Care in Portugal: a nationwide cross-sectional study. Patient Prefer Adherence. 2019;13:1569–76.
Roux B, Sirois C, Simard M, Gagnon ME, Laroche ML. Potentially inappropriate medications in older adults: a population-based cohort study. Fam Pract. 2020;37(2):173–9.
PubMed Google Scholar
Nothelle SK, Sharma R, Oakes A, Jackson M, Segal JB. Factors associated with potentially inappropriate medication use in community-dwelling older adults in the United States: a systematic review. Int J Pharm Pract. 2019;27(5):408–23.
Kuijpers MA, van Marum RJ, Egberts AC, Jansen PA. Relationship between polypharmacy and underprescribing. Br J Clin Pharmacol. 2008;65(1):130–3.
Jungo KT, Streit S, Lauffenburger JC. Utilization and Spending on Potentially Inappropriate Medications by US Older Adults with Multiple Chronic Conditions using Multiple Medications. Arch Gerontol Geriatr. 2021;93:104326. https://doi.org/10.1016/j.archger.2020.104326 .
Xing XX, Zhu C, Liang HY, Wang K, Chu YQ, Zhao LB, et al. Associations between potentially inappropriate medications and adverse Health outcomes in the Elderly: a systematic review and Meta-analysis. Ann Pharmacother. 2019;53(10):1005–19.
Masumoto S, Sato M, Maeno T, Ichinohe Y, Maeno T. Potentially inappropriate medications with polypharmacy increase the risk of falls in older Japanese patients: 1-year prospective cohort study. Geriatr Gerontol Int. 2018;18(7):1064–70.
Koyama A, Steinman M, Ensrud K, Hillier TA, Yaffe K. Long-term cognitive and functional effects of potentially inappropriate medications in older women. The journals of gerontology Series A, Biological sciences and medical sciences. 2014;69(4):423–9.
Liew TM, Lee CS, Goh Shawn KL, Chang ZY. Potentially inappropriate prescribing among older persons: a Meta-analysis of Observational studies. Annals Family Med. 2019;17(3):257–66.
Article Google Scholar
Fabbietti P, Ruggiero C, Sganga F, Fusco S, Mammarella F, Barbini N, et al. Effects of hyperpolypharmacy and potentially inappropriate medications (PIMs) on functional decline in older patients discharged from acute care hospitals. Arch Gerontol Geriatr. 2018;77:158–62.
Hernandez G, Garin O, Dima AL, Pont A, Martí Pastor M, Alonso J, et al. EuroQol (EQ-5D-5L) validity in assessing the quality of life in adults with Asthma: cross-sectional study. J Med Internet Res. 2019;21(1):e10178.
Huibers CJA, Sallevelt BTGM, de Groot DA, Boer MJ, van Campen JPCM, Davids CJ, et al. Conversion of STOPP/START version 2 into coded algorithms for software implementation: a multidisciplinary consensus procedure. Int J Med Informatics. 2019;125:110–7.
O’Mahony D, O’Sullivan D, Byrne S, O’Connor MN, Ryan C, Gallagher P. STOPP/START criteria for potentially inappropriate prescribing in older people: version 2. Age Ageing. 2015;44(2):213–8.
Alshammari H, Al-Saeed E, Ahmed Z, Aslanpour Z. Reviewing potentially inappropriate medication in hospitalized patients over 65 using Explicit Criteria: a systematic literature review. Drug Healthc Patient Saf. 2021;13:183–210.
Drenth-van Maanen AC, Leendertse AJ, Jansen PAF, Knol W, Keijsers C, Meulendijk MC, et al. The systematic Tool to reduce Inappropriate Prescribing (STRIP): combining implicit and explicit prescribing tools to improve appropriate prescribing. J Eval Clin Pract. 2018;24(2):317–22.
Adam L, Moutzouri E, Baumgartner C, Loewe AL, Feller M, M’Rabet-Bensalah K, et al. Rationale and design of OPtimising thERapy to prevent avoidable hospital admissions in Multimorbid older people (OPERAM): a cluster randomised controlled trial. BMJ Open. 2019;9(6):e026769.
PubMed PubMed Central Google Scholar
Blum MR, Sallevelt BTGM, Spinewine A, O’Mahony D, Moutzouri E, Feller M, et al. Optimizing therapy to prevent Avoidable Hospital admissions in Multimorbid older adults (OPERAM): cluster randomised controlled trial. BMJ. 2021;374:n1585.
Jungo KT, Rozsnyai Z, Mantelli S, Floriani C, Löwe AL, Lindemann F, et al. Optimising PharmacoTherapy in the multimorbid elderly in primary CAre’ (OPTICA) to improve medication appropriateness: study protocol of a cluster randomised controlled trial. BMJ open. 2019;9(9):e031080.
Jungo KT, Meier R, Valeri F, Schwab N, Schneider C, Reeve E, et al. Baseline characteristics and comparability of older multimorbid patients with polypharmacy and general practitioners participating in a randomized controlled primary care trial. BMC Fam Pract. 2021;22(1):123.
Jungo KT, Ansorg AK, Floriani C, Rozsnyai Z, Schwab N, Meier R, et al. Optimising prescribing in older adults with multimorbidity and polypharmacy in primary care (OPTICA): cluster randomised clinical trial. BMJ. 2023;381:e074054.
Jia P, Zhang L, Chen J, Zhao P, Zhang M. The effects of clinical decision support systems on Medication Safety: an overview. PLoS ONE. 2016;11(12):e0167683–e.
Reis WC, Bonetti AF, Bottacin WE, Reis AS Jr., Souza TT, Pontarolo R, et al. Impact on process results of clinical decision support systems (CDSSs) applied to medication use: overview of systematic reviews. Pharm Pract. 2017;15(4):1036.
Google Scholar
Monteiro L, Maricoto T, Solha I, Ribeiro-Vaz I, Martins C, Monteiro-Soares M. Reducing potentially inappropriate prescriptions for older patients using computerized decision support tools: systematic review. J Med Internet Res. 2019;21(11):e15385.
Scott IA, Pillans PI, Barras M, Morris C. Using EMR-enabled computerized decision support systems to reduce prescribing of potentially inappropriate medications: a narrative review. Therapeutic Adv drug Saf. 2018;9(9):559–73.
Bryan C, Boren SA. The use and effectiveness of electronic clinical decision support tools in the ambulatory/primary care setting: a systematic review of the literature. Inform Prim Care. 2008;16(2):79–91.
Rieckert A, Sommerauer C, Krumeich A, Sönnichsen A. Reduction of inappropriate medication in older populations by electronic decision support (the PRIMA-eDS study): a qualitative study of practical implementation in primary care. BMC Fam Pract. 2018;19(1):110.
Rieckert A, Teichmann AL, Drewelow E, Kriechmayr C, Piccoliori G, Woodham A, et al. Reduction of inappropriate medication in older populations by electronic decision support (the PRIMA-eDS project): a survey of general practitioners’ experiences. J Am Med Inf Association: JAMIA. 2019;26(11):1323–32.
Bell H, Garfield S, Khosla S, Patel C, Franklin BD. Mixed methods study of medication-related decision support alerts experienced during electronic prescribing for inpatients at an English hospital. Eur J Hosp Pharmacy: Sci Pract. 2019;26(6):318–22.
Crowley EK, Sallevelt B, Huibers CJA, Murphy KD, Spruit M, Shen Z, et al. Intervention protocol: OPtimising thERapy to prevent avoidable hospital admission in the multi-morbid elderly (OPERAM): a structured medication review with support of a computerised decision support system. BMC Health Serv Res. 2020;20(1):220.
Chmiel C, Bhend H, Senn O, Zoller M, Rosemann T. The FIRE project: a milestone for research in primary care in Switzerland. Swiss Med Wkly. 2011;140:w13142.
Creswell J, Plano Clark V. Designing and conducting mixed methods research. Los Angeles: SAGE; 2011.
Phillips WR, Sturgiss E, Glasziou P, Hartman TCo, Orkin AM, Prathivadi P et al. Improving the Reporting of Primary Care Research: Consensus Reporting Items for Studies in Primary Care—the CRISP Statement. The Annals of Family Medicine. 2023:3029.
StataCorp. Stata Statistical Software: Release 17 College Station. TX: StataCorp LLC; 2021.
Braun V, Clarke V. Using thematic analysis in psychology. Qualitative Res Psychol. 2006;3(2):77–101.
Gale NK, Heath G, Cameron E, Rashid S, Redwood S. Using the framework method for the analysis of qualitative data in multi-disciplinary health research. BMC Med Res Methodol. 2013;13(1):117.
Weinstein M. TAMS Analyzer 4.0 [Computer software] 2010 [Available from: https://tamsys.sourceforge.io/osxtams/docs/basic/TA%20User%20Guide.pdf].
Meulendijk MC, Spruit MR, Willeboordse F, Numans ME, Brinkkemper S, Knol W, et al. Efficiency of clinical decision support systems improves with experience. J Med Syst. 2016;40(4):76.
O’Mahony D, Gudmundsson A, Soiza RL, Petrovic M, Jose Cruz-Jentoft A, Cherubini A, et al. Prevention of adverse drug reactions in hospitalized older patients with multi-morbidity and polypharmacy: the SENATOR* randomized controlled clinical trial. Age Ageing. 2020;49(4):605–14.
Sallevelt BTGM, Huibers CJA, Heij JMJO, Egberts TCG, van Puijenbroek EP, Shen Z, et al. Frequency and Acceptance of clinical decision support system-generated STOPP/START signals for hospitalised older patients with polypharmacy and Multimorbidity. Drugs Aging. 2022;39(1):59–73.
Sutton RT, Pincock D, Baumgart DC, Sadowski DC, Fedorak RN, Kroeker KI. An overview of clinical decision support systems: benefits, risks, and strategies for success. Npj Digit Med. 2020;3(1):17.
Huibers CJA, Sallevelt B, Heij J, O’Mahony D, Rodondi N, Dalleur O, et al. Hospital physicians’ and older patients’ agreement with individualised STOPP/START-based medication optimisation recommendations in a clinical trial setting. Eur Geriatr Med. 2022;13(3):541–52.
Article CAS PubMed PubMed Central Google Scholar
Peiris DP, Joshi R, Webster RJ, Groenestein P, Usherwood TP, Heeley E, et al. An electronic clinical decision support tool to assist primary care providers in cardiovascular disease risk management: development and mixed methods evaluation. J Med Internet Res. 2009;11(4):e51.
Download references
Acknowledgements
The authors would like to thank the general practitioners participating in the OPTICA trial for participating in this research, in particular those who were in the intervention group and provided the information for this implementation evaluation. Thanks go to the CTU Bern for their support in conducting the OPTICA trial. KTJ is funded by a Postdoc.Mobility Fellowship from the Swiss National Science Foundation (P500PM_206728). KTJ was a member of the Junior Investigator Intensive Program of the US Deprescribing Research Network, which is funded by the National Institute on Aging (R24AG064025).
This work was funded by the Swiss National Science Foundation, within the framework of the National Research Programme 74 (NRP74) under contract number 407440_167465 (to SS and NR).
Author information
Authors and affiliations.
Institute of Primary Health Care (BIHAM), University of Bern, Bern, Switzerland
Katharina Tabea Jungo, Fabian Schalbetter, Jeanne Moor, Martin Feller, Renata Vidonscky Lüthold, Nicolas Rodondi & Sven Streit
Institute of Sociological Research, University of Geneva, Geneva, Switzerland
Michael J. Deml
Department of General Internal Medicine, Inselspital, Bern University Hospital, University of Bern, Bern, Switzerland
Jeanne Moor & Nicolas Rodondi
Geriatrics, Department of Geriatric Medicine, University Medical Center Utrecht, Utrecht University, Utrecht, The Netherlands
Johanna Alida Corlina Huibers
Department of Clinical Pharmacy, University Medical Center Utrecht, Utrecht, Utrecht, The Netherlands
Bastiaan Theodoor Gerard Marie Sallevelt
Public Health and Primary Care (PHEG), Leiden University Medical Center, Leiden University, Leiden, Netherlands
Michiel C Meulendijk & Marco Spruit
Leiden Institute of Advanced Computer Science (LIACS), Faculty of Science, Leiden University, Leiden, Netherlands
Marco Spruit
Department of Information and Computing Sciences, Utrecht University, Utrecht, Netherlands
Health Economics Facility, Department of Public Health, University of Basel, Basel, Switzerland
Matthias Schwenkglenks
Institute of Pharmaceutical Medicine (ECPM), University of Basel, Basel, Switzerland
Epidemiology, Biostatistics and Prevention Institute (EBPI), University of Zurich, Zurich, Switzerland
Graduate School for Health Sciences, University of Bern, Bern, Switzerland
Renata Vidonscky Lüthold
Center for Healthcare Delivery Sciences (C4HDS), Division of Pharmacoepidemiology and Pharmacoeconomics, Department of Medicine, Brigham and Women’s Hospital and Harvard Medical School, Boston, United States
Katharina Tabea Jungo
You can also search for this author in PubMed Google Scholar
Contributions
KTJ, MJD, and SS designed the mixed-methods implementation study. KTJ and FS acquired the qualitative data. KTJ, FS, and MJD analyzed the qualitative data. KTJ, MSp, MSch, NR, SS acquired the quantitative data. KTJ analyzed the quantitative data. KTJ drafted the first draft of the manuscript with help from MJD and SS. All authors (KTJ, MJD, FS, JM, MF, RL, CJAH, BTGMS, MCM, MSp, MSch, NR, SS) reviewed and edited the manuscript and approved the final version.
Corresponding author
Correspondence to Katharina Tabea Jungo .
Ethics declarations
Ethical approval and consent to participate.
The ethics committee of the canton of Bern (Switzerland) and the Swiss regulatory authority (Swissmedic) approved the study protocol of the OPTICA trial (BASEC ID: 2018–00914) including the conduct of this mixed-methods evaluation. All study participants provided informed consent to participate in the trial. All methods were performed in accordance with the relevant guidelines and regulations (e.g., declaration of Helsinki).
Consent for publication
Not required.
Competing interests
The authors declare no competing interests.
Additional information
Publisher’s note.
Springer Nature remains neutral with regard to jurisdictional claims in published maps and institutional affiliations.
Electronic supplementary material
Below is the link to the electronic supplementary material.
Supplementary Material 1
Rights and permissions.
Open Access This article is licensed under a Creative Commons Attribution 4.0 International License, which permits use, sharing, adaptation, distribution and reproduction in any medium or format, as long as you give appropriate credit to the original author(s) and the source, provide a link to the Creative Commons licence, and indicate if changes were made. The images or other third party material in this article are included in the article’s Creative Commons licence, unless indicated otherwise in a credit line to the material. If material is not included in the article’s Creative Commons licence and your intended use is not permitted by statutory regulation or exceeds the permitted use, you will need to obtain permission directly from the copyright holder. To view a copy of this licence, visit http://creativecommons.org/licenses/by/4.0/ . The Creative Commons Public Domain Dedication waiver ( http://creativecommons.org/publicdomain/zero/1.0/ ) applies to the data made available in this article, unless otherwise stated in a credit line to the data.
Reprints and permissions
About this article
Cite this article.
Jungo, K.T., Deml, M.J., Schalbetter, F. et al. A mixed methods analysis of the medication review intervention centered around the use of the ‘Systematic Tool to Reduce Inappropriate Prescribing’ Assistant (STRIPA) in Swiss primary care practices. BMC Health Serv Res 24 , 350 (2024). https://doi.org/10.1186/s12913-024-10773-y
Download citation
Received : 15 August 2023
Accepted : 23 February 2024
Published : 18 March 2024
DOI : https://doi.org/10.1186/s12913-024-10773-y
Share this article
Anyone you share the following link with will be able to read this content:
Sorry, a shareable link is not currently available for this article.
Provided by the Springer Nature SharedIt content-sharing initiative
- Multimorbidity
- Polypharmacy
- Primary care
- Medication optimization
- Electronic clinical decision support system
- Mixed methods research
BMC Health Services Research
ISSN: 1472-6963
- General enquiries: [email protected]
Empowering education development through AIGC: A systematic literature review
- Published: 29 February 2024
Cite this article
- Xiaojiao Chen 1 ,
- Zhebing Hu 2 &
- Chengliang Wang ORCID: orcid.org/0000-0003-2208-3508 3
507 Accesses
Explore all metrics
As an exemplary representative of AIGC products, ChatGPT has ushered in new possibilities for the field of education. Leveraging its robust text generation and comprehension capabilities, it has had a revolutionary impact on pedagogy, learning experiences, personalized education and other aspects. However, to date, there has been no comprehensive review of AIGC technology’s application in education. In light of this gap, this study employs a systematic literature review and selects 134 relevant publications on AIGC’s educational application from 4 databases: EBSCO, EI Compendex, Scopus, and Web of Science. The study aims to explore the macro development status and future trends in AIGC’s educational application. The following findings emerge: 1) In the AIGC’s educational application field, the United States is the most active country. Theoretical research dominates the research types in this domain; 2) Research on AIGC’s educational application is primarily published in journals and academic conferences in the fields of educational technology and medicine; 3) Research topics primarily focus on five themes: AIGC technology performance assessment, AIGC technology instructional application, AIGC technology enhancing learning outcomes, AIGC technology educational application’s Advantages and Disadvantages analysis, and AIGC technology educational application prospects. 4) Through Grounded Theory, the study delves into the core advantages and potential risks of AIGC’s educational application, deconstructing the scenarios and logic of AIGC’s educational application. 5) Based on a review of existing literature, the study provides valuable future agendas from both theoretical and practical application perspectives. Discussing the future research agenda contributes to clarifying key issues related to the integration of AI and education, promoting more intelligent, effective, and sustainable educational methods and tools, which is of great significance for advancing innovation and development in the field of education.
This is a preview of subscription content, log in via an institution to check access.
Access this article
Price includes VAT (Russian Federation)
Instant access to the full article PDF.
Rent this article via DeepDyve
Institutional subscriptions
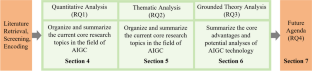
Data availability
The datasets (Coding results) generated during and/or analysed during the current study are available from the corresponding author on reasonable request.
Abdelghani, R., Wang, Y. H., Yuan, X., Wang, T., Lucas, P., Sauzéon, H., & Oudeyer, P. Y. (2023). Gpt-3-driven pedagogical agents to train children’s curious question-asking skills. International Journal of Artificial Intelligence in Education , 1–36. https://doi.org/10.1007/s40593-023-00340-7
Abdulai, A. F., & Hung, L. (2023). Will ChatGPT undermine ethical values in nursing education, research, and practice. Nursing Inquiry . e12556–e12556. https://doi.org/10.1111/nin.12556
Ahmed, S. K. (2023). The Impact of ChatGPT on the Nursing Profession: Revolutionizing Patient Care and Education. Annals of Biomedical Engineering , 1–2. https://doi.org/10.1007/s10439-023-03262-6
Albeshri, A., & Thayananthan, V. (2018). Analytical techniques for decision making on information security for big data breaches. International Journal of Information Technology & Decision Making, 17 (02), 527–545. https://doi.org/10.1142/S0219622017500432
Article Google Scholar
Allen, B., Dreyer, K., Stibolt Jr, R., Agarwal, S., Coombs, L., Treml, C., ... & Wald, C. (2021). Evaluation and real-world performance monitoring of artificial intelligence models in clinical practice: Try it, buy it, check it. Journal of the American College of Radiology , 18 (11), 1489–1496. https://doi.org/10.1016/j.jacr.2021.08.022
Alnaqbi, N. M., & Fouda, W. (2023). Exploring the role of ChatGPT and social media in enhancing student evaluation of teaching styles in higher education using neutrosophic sets. International Journal of Neutrosophic Science, 20 (4), 181–190. https://doi.org/10.1111/nin.12556
Alqahtani, T., Badreldin, H. A., Alrashed, M., Alshaya, A. I., Alghamdi, S. S., bin Saleh, K., ... & Albekairy, A. M. (2023). The emergent role of artificial intelligence, natural learning processing, and large language models in higher education and research. Research in Social and Administrative Pharmacy. https://doi.org/10.1016/j.sapharm.2023.05.016
Ancillai, C., Sabatini, A., Gatti, M., & Perna, A. (2023). Digital technology and business model innovation: A systematic literature review and future research agenda. Technological Forecasting and Social Change, 188 , 122307. https://doi.org/10.1016/j.techfore.2022.122307
Banić, B., Konecki, M., & Konecki, M. (2023, May). Pair Programming Education Aided by ChatGPT. In 2023 46th MIPRO ICT and Electronics Convention (MIPRO) (pp. 911–915). IEEE.
Busch, F., Adams, L. C., & Bressem, K. K. (2023). Biomedical ethical aspects towards the implementation of artificial intelligence in medical education. Medical Science Educator., 33 , 1007–1012. https://doi.org/10.1007/s40670-023-01815-x
Article PubMed PubMed Central Google Scholar
Chang, C.-Y., Kuo, S.-Y., & Hwang, G.-H. (2022). Chatbot-facilitated nursing education: Incorporating a knowledge-based Chatbot system into a nursing training program. Educational Technology & Society , 25 (1), 15–27. Retrieved December 19, 2023, from https://www.jstor.org/stable/48647027
Charmaz, K., & Thornberg, R. (2021). The pursuit of quality in grounded theory. Qualitative Research in Psychology, 18 (3), 305–327. https://doi.org/10.1080/14780887.2020.1780357
Choi, E. P. H., Lee, J. J., Ho, M. H., Kwok, J. Y. Y., & Lok, K. Y. W. (2023). Chatting or cheating? The impacts of ChatGPT and other artificial intelligence language models on nurse education. Nurse Education Today, 125 , 105796–105796. https://doi.org/10.1016/j.nedt.2023.105796
Article PubMed Google Scholar
Cooper, G. (2023). Examining science education in chatgpt: An exploratory study of generative artificial intelligence. Journal of Science Education and Technology, 32 (3), 444–452. https://doi.org/10.1007/s10956-023-10039-y
Article ADS Google Scholar
Cross, J., Robinson, R., Devaraju, S., Vaughans, A., Hood, R., Kayalackakom, T., ... & Robinson, R. E. (2023). Transforming medical education: Assessing the integration of ChatGPT into faculty workflows at a Caribbean medical school. Cureus , 15 (7). https://doi.org/10.7759/cureus.41399
Currie, G. M. (2023, May). Academic integrity and artificial intelligence: Is ChatGPT hype, hero or heresy? In Seminars in Nuclear Medicine. WB Saunders. https://doi.org/10.1053/j.semnuclmed.2023.04.008
Das, D., Kumar, N., Longjam, L. A., Sinha, R., Roy, A. D., Mondal, H., & Gupta, P. (2023). Assessing the capability of ChatGPT in answering first-and second-order knowledge questions on microbiology as per competency-based medical education curriculum. Cureus , 15 (3). https://doi.org/10.7759/cureus.36034
Deacon, B., Laufer, M., & Schäfer, L. O. (2023). Infusing educational technologies in the heart of the university-a systematic literature review from an organisational perspective. British Journal of Educational Technology, 54 (2), 441–466. https://doi.org/10.1111/bjet.13277
Deeley, S. J. (2018). Using technology to facilitate effective assessment for learning and feedback in higher education. Assessment & Evaluation in Higher Education, 43 (3), 439–448. https://doi.org/10.1080/02602938.2017.1356906
Deng, X., & Yu, Z. (2023). A meta-analysis and systematic review of the effect of chatbot technology use in sustainable education. Sustainability, 15 (4), 2940. https://doi.org/10.3390/su15042940
Diekemper, R. L., Ireland, B. K., & Merz, L. R. (2015). Development of the documentation and appraisal review tool for systematic reviews. World Journal of Meta-Analysis, 3 (3), 142–150. https://doi.org/10.13105/wjma.v3.i3.142
Engel, A., & Coll, C. (2022). Hybrid teaching and learning environments to promote personalized learning. RIED-Revista Iberoamericana de Educacion a Distancia , 225–242. https://doi.org/10.5944/ried.25.1.31489
Escotet, M. Á. (2023). The optimistic future of Artificial Intelligence in higher education. Prospects, 1–10. https://doi.org/10.1007/s11125-023-09642-z
Esplugas, M. (2023). The use of artificial intelligence (AI) to enhance academic communication, education and research: A balanced approach. Journal of Hand Surgery (European Volume) , 48 (8), 819–822. https://doi.org/10.1177/17531934231185746
Extance, A. (2023). ChatGPT has entered the classroom: How LLMs could transform education. Nature, 623 , 474–477. https://doi.org/10.1038/d41586-023-03507-3
Article CAS PubMed ADS Google Scholar
Foroughi, B., Senali, M. G., Iranmanesh, M., Khanfar, A., Ghobakhloo, M., Annamalai, N., & Naghmeh-Abbaspour, B. (2023). Determinants of Intention to Use ChatGPT for Educational Purposes: Findings from PLS-SEM and fsQCA. International Journal of Human-Computer Interaction , 1–20. https://doi.org/10.1080/10447318.2023.2226495
Gaur, A., & Kumar, M. (2018). A systematic approach to conducting review studies: An assessment of content analysis in 25 years of IB research. Journal of World Business, 53 (2), 280–289. https://doi.org/10.1016/j.jwb.2017.11.003
Ghorbani, M., Bahaghighat, M., Xin, Q., & Özen, F. (2020). ConvLSTMConv network: A deep learning approach for sentiment analysis in cloud computing. Journal of Cloud Computing, 9 (1), 1–12. https://doi.org/10.1186/s13677-020-00162-1
Glaser, B., & Strauss, A. (2017). Discovery of grounded theory: Strategies for qualitative research . Routledge https://doi.org/10.1016/j.jwb.2017.11.003
Gough, D., Oliver, S., & Thomas, J. (Eds.). (2017). An introduction to systematic reviews . Sage https://doi.org/10.5124/jkma.2014.57.1.49
Grant, N., & Metz, C. (2022). A new chat bot is a ‘code red’ for Google's search business, The New York Times. Available at: https://www.nytimes.com/2022/12/21/technology/ai-chatgpt-google-search.html . Accessed 19 Dec 2023
Hadi, M. S., & Junor, R. S. (2022). Speaking to devices: Can we use Google assistant to Foster Students' speaking skills? Journal of Languages and Language Teaching, 10 (4), 570–578. https://doi.org/10.33394/jollt.v10i4.5808
Heng, J. J., Teo, D. B., & Tan, L. F. (2023). The impact of Chat Generative Pre-trained Transformer (ChatGPT) on medical education. Postgraduate Medical Journal , qgad058. https://doi.org/10.1093/postmj/qgad058
Ho, W., & Lee, D. (2023). Enhancing engineering education in the roblox metaverse: Utilizing chatgpt for game development for electrical machine course. International Journal on Advanced Science, Engineering & Information Technology , 13 (3). https://doi.org/10.18517/ijaseit.13.3.18458
Holmes, W., & Kay, J. (2023, June). AI in education. Coming of age? The community voice. In International conference on artificial intelligence in education (pp. 85–90). Springer Nature Switzerland.
Google Scholar
Hsu, Y. C., & Ching, Y. H. (2023). Generative Artificial Intelligence in Education, Part One: the Dynamic Frontier. TechTrends , 1–5. https://doi.org/10.1007/s11528-023-00863-9
Hwang, G. J., & Chang, C. Y. (2021). A review of opportunities and challenges of chatbots in education. Interactive Learning Environments , 1–14. https://doi.org/10.1080/10494820.2021.1952615
Jalil, S., Rafi, S., LaToza, T. D., Moran, K., & Lam, W. (2023, April). Chatgpt and software testing education: Promises & perils. In 2023 IEEE International Conference on Software Testing, Verification and Validation Workshops (ICSTW) (pp. 4130–4137). IEEE.
Jeon, J., & Lee, S. (2023). Large language models in education: A focus on the complementary relationship between human teachers and ChatGPT. Education and Information Technologies , 1–20. https://doi.org/10.1007/s10639-023-11834-1
Jing, Y., Wang, C., Chen, Y., Wang, H., Yu, T., & Shadiev, R. (2023). Bibliometric mapping techniques in educational technology research: A systematic literature review. Education and Information Technologies , 1–29. https://doi.org/10.1007/s10639-023-12178-6
Johnson, R. B., & Onwuegbuzie, A. J. (2004). Mixed methods research: A research paradigm whose time has come. Educational Researcher, 33 (7), 14–26. https://doi.org/10.3102/0013189X033007014
Karabacak, M., Ozkara, B. B., Margetis, K., Wintermark, M., & Bisdas, S. (2023). The advent of generative language models in medical education. JMIR Medical Education, 9 , e48163. https://doi.org/10.2196/48163
Kasneci, E., Seßler, K., Küchemann, S., Bannert, M., Dementieva, D., Fischer, F., … Kasneci, G. (2023). ChatGPT for good? On opportunities and challenges of large language models for education. Learning and Individual Differences, 103 , 102274. https://doi.org/10.1016/j.lindif.2023.102274
Kepuska, V., & Bohouta, G. (2018, January). Next-generation of virtual personal assistants (microsoft cortana, apple siri, amazon alexa and google home). In 2018 IEEE 8th annual computing and communication workshop and conference (CCWC) (pp. 99–103). IEEE.
Kerneža, M. (2023). Fundamental And Basic Cognitive Skills Required For Teachers To Effectively Use Chatbots In Education. In Science And Technology Education: New Developments And Innovations (pp. 99–110). Scientia Socialis, UAB.
Kılıçkaya, F. (2020). Using a chatbot, Replika, to practice writing through conversations in L2 English: A Case study. In New Technological applications for foreign and second language learning and teaching (pp. 221–238). IGI Global. https://doi.org/10.4018/978-1-7998-2591-3.ch011
Killian, C. M., Marttinen, R., Howley, D., Sargent, J., & Jones, E. M. (2023). “Knock, Knock... Who’s There?” ChatGPT and Artificial Intelligence-Powered Large Language Models: Reflections on Potential Impacts Within Health and Physical Education Teacher Education. Journal of Teaching in Physical Education , 1 (aop), 1–5. https://doi.org/10.1123/jtpe.2023-0058
Kohnke, L. (2022). A pedagogical Chatbot: A supplemental language learning Tool. RELC Journal , 00336882211067054. https://doi.org/10.1177/00336882211067054
Kung, T. H., Cheatham, M., Medenilla, A., Sillos, C., De Leon, L., Elepaño, C., ... & Tseng, V. (2023). Performance of ChatGPT on USMLE: Potential for AI-assisted medical education using large language models. PLoS Digital Health, 2 (2), e0000198. https://doi.org/10.1371/journal.pdig.0000198
Lee, D., & Yeo, S. (2022). Developing an AI-based chatbot for practicing responsive teaching in mathematics. Computers & Education, 191 , 104646. https://doi.org/10.1016/j.compedu.2022.104646
Lee, L. W., Dabirian, A., McCarthy, I. P., & Kietzmann, J. (2020). Making sense of text: Artificial intelligence-enabled content analysis. European Journal of Marketing, 54 (3), 615–644. https://doi.org/10.1108/EJM-02-2019-0219
Li, L., Ma, Z., Fan, L., Lee, S., Yu, H., & Hemphill, L. (2023). ChatGPT in education: A discourse analysis of worries and concerns on social media. arXiv preprint arXiv :2305.02201. https://doi.org/10.48550/arXiv.2305.02201
Lim, W. M., Gunasekara, A., Pallant, J. L., Pallant, J. I., & Pechenkina, E. (2023). Generative AI and the future of education: Ragnarök or reformation? A paradoxical perspective from management educators. The International Journal of Management Education, 21 (2), 100790. https://doi.org/10.1016/j.ijme.2023.100790
Lin, T. J., & Lan, Y. J. (2015). Language learning in virtual reality environments: Past, present, and future. Journal of Educational Technology & Society, 18 (4), 486–497. Retrieved December 19, 2023, from http://www.jstor.org/stable/jeductechsoci.18.4.486
Lodge, J. M., Thompson, K., & Corrin, L. (2023). Mapping out a research agenda for generative artificial intelligence in tertiary education. Australasian Journal of Educational Technology, 39 (1), 1–8. https://doi.org/10.14742/ajet.8695
Luo, H., Li, G., Feng, Q., Yang, Y., & Zuo, M. (2021). Virtual reality in K-12 and higher education: A systematic review of the literature from 2000 to 2019. Journal of Computer Assisted Learning, 37 (3), 887–901. https://doi.org/10.1111/jcal.12538
Mariani, M. M., Hashemi, N., & Wirtz, J. (2023). Artificial intelligence empowered conversational agents: A systematic literature review and research agenda. Journal of Business Research, 161 , 113838. https://doi.org/10.1016/j.jbusres.2023.113838
Mohamed, A. M. (2023). Exploring the potential of an AI-based Chatbot (ChatGPT) in enhancing English as a Foreign Language (EFL) teaching: perceptions of EFL Faculty Members. Education and Information Technologies, 1–23. https://doi.org/10.1007/s10639-023-11917-z
Mohammad, B., Supti, T., Alzubaidi, M., Shah, H., Alam, T., Shah, Z., & Househ, M. (2023). The pros and cons of using ChatGPT in medical education: A scoping review. Student Health Technology Information, 305 , 644–647. https://doi.org/10.3233/SHTI230580
Moher, D., Liberati, A., Tetzlaff, J., Altman, D. G., & PRISMA Group. (2009). Preferred reporting items for systematic reviews and meta-analyses: The PRISMA statement. Annals of Internal Medicine, 151 , 264–269. https://doi.org/10.7326/0003-4819-151-4-200908180-00135
Mokmin, N. A. M., & Ibrahim, N. A. (2021). The evaluation of chatbot as a tool for health literacy education among undergraduate students. Education and Information Technologies, 26 (5), 6033–6049. https://doi.org/10.1007/s10639-021-10542-y
Patel, N., Nagpal, P., Shah, T., Sharma, A., Malvi, S., & Lomas, D. (2023). Improving mathematics assessment readability: Do large language models help? Journal of Computer Assisted Learning, 39 (3), 804–822. https://doi.org/10.1111/jcal.12776
Paul, J., Lim, W. M., O’Cass, A., Hao, A. W., & Bresciani, S. (2021). Scientific procedures and rationales for systematic literature reviews (SPAR-4-SLR). International Journal of Consumer Studies, 45 (4), O1–O16. https://doi.org/10.1111/ijcs.12695
Pentina, I., Xie, T., Hancock, T., & Bailey, A. (2023). Consumer–machine relationships in the age of artificial intelligence: Systematic literature review and research directions. Psychology & Marketing, 40 (8), 1593–1614. https://doi.org/10.1002/mar.21853
Pereira, R., Reis, A., Barroso, J., Sousa, J., & Pinto, T. (2022). Virtual assistants applications in education. In International conference on technology and innovation in learning, teaching and education (pp. 468–480). Springer Nature Switzerland.
Pinto, A. S., Abreu, A., Costa, E., & Paiva, J. (2023). How Machine Learning (ML) is transforming higher education: A systematic literature review. Journal of Information Systems Engineering and Management, 8 (2). https://doi.org/10.55267/iadt.07.13227
Prikshat, V., Islam, M., Patel, P., Malik, A., Budhwar, P., & Gupta, S. (2023). AI-augmented HRM: Literature review and a proposed multilevel framework for future research. Technological Forecasting and Social Change, 193 , 122645. https://doi.org/10.1016/j.techfore.2023.122645
Radianti, J., Majchrzak, T. A., Fromm, J., & Wohlgenannt, I. (2020). A systematic review of immersive virtual reality applications for higher education: Design elements, lessons learned, and research agenda. Computers & Education, 147 , 103778. https://doi.org/10.1016/j.compedu.2019.103778
Rahimzadeh, V., Kostick-Quenet, K., Blumenthal Barby, J., & McGuire, A. L. (2023). Ethics education for healthcare professionals in the era of chatGPT and other large language models: Do we still need it?. The American Journal of Bioethics , 1–11. https://doi.org/10.1080/15265161.2023.2233358
Rahman, M. M., & Watanobe, Y. (2023). ChatGPT for education and research: Opportunities, threats, and strategies. Applied Sciences, 13 (9), 5783. https://doi.org/10.3390/app13095783
Article CAS Google Scholar
Rasul, T., Nair, S., Kalendra, D., Robin, M., de Oliveira Santini, F., Ladeira, W. J., ... & Heathcote, L. (2023). The role of ChatGPT in higher education: Benefits, challenges, and future research directions. Journal of Applied Learning and Teaching, 6 (1). https://doi.org/10.37074/jalt.2023.6.1
Sallam, M. (2023a). ChatGPT utility in healthcare education, research, and practice: systematic review on the promising perspectives and valid concerns. In Healthcare (Vol. 11, No. 6, p. 887). MDPI. https://doi.org/10.3390/healthcare11060887
Sallam, M. (2023b). ChatGPT utility in healthcare education, research, and practice: Systematic review on the promising perspectives and valid concerns. Healthcare, 11 (6), 887. https://doi.org/10.3390/healthcare11060887
Sánchez-Ruiz, L. M., Moll-López, S., Nuñez-Pérez, A., Moraño-Fernández, J. A., & Vega-Fleitas, E. (2023). ChatGPT challenges blended learning methodologies in engineering education: A case study in mathematics. Applied Sciences, 13 (10), 6039. https://doi.org/10.3390/app13106039
Sandu, N., & Gide, E. (2019). Adoption of AI-Chatbots to enhance student learning experience in higher education in India. In 2019 18th International Conference on Information Technology Based Higher Education and Training (ITHET) (pp. 1–5). IEEE.
Schmulian, A., & Coetzee, S. A. (2019). Students’ experience of team assessment with immediate feedback in a large accounting class. Assessment & Evaluation in Higher Education, 44 (4), 516–532. https://doi.org/10.1080/02602938.2018.1522295
Seetharaman, R. (2023). Revolutionizing medical education: Can ChatGPT boost subjective learning and expression? Journal of Medical Systems, 47 (1), 1–4. https://doi.org/10.1007/s10916-023-01957-w
Sharma, M., & Sharma, S. (2023). A holistic approach to remote patient monitoring, fueled by ChatGPT and Metaverse technology: The future of nursing education. Nurse Education Today, 131 , 105972. https://doi.org/10.1016/j.nedt.2023.105972
Shlonsky, A., Noonan, E., Littell, J. H., & Montgomery, P. (2011). The role of systematic reviews and the Campbell collaboration in the realization of evidence-informed practice. Clinical Social Work Journal, 39 , 362–368. https://doi.org/10.1007/s10615-010-0307-0
Shoja, M. M., Van de Ridder, J. M., & Rajput, V. (2023). The emerging role of generative artificial intelligence in medical education, research, and practice. Cureus, 15 (6), e40883. https://doi.org/10.7759/cureus.40883
Siegle, D. (2023). A role for ChatGPT and AI in gifted education. Gifted Child Today, 46 (3), 211–219. https://doi.org/10.1177/10762175231168443
Smith, A., Hachen, S., Schleifer, R., Bhugra, D., Buadze, A., & Liebrenz, M. (2023). Old dog, new tricks? Exploring the potential functionalities of ChatGPT in supporting educational methods in social psychiatry. International Journal of Social Psychiatry . https://doi.org/10.1177/0020764023117845
Strzelecki, A. (2023). To use or not to use ChatGPT in higher education? A study of students’ acceptance and use of technology. Interactive Learning Environments , 1–14. https://doi.org/10.1080/10494820.2023.2209881
Tam, W., Huynh, T., Tang, A., Luong, S., Khatri, Y., & Zhou, W. (2023). Nursing education in the age of artificial intelligence powered Chatbots (AI-Chatbots): Are we ready yet? Nurse Education Today, 129 , 105917. https://doi.org/10.1016/j.nedt.2023.105917
Teel, Z. A., Wang, T., & Lund, B. (2023). ChatGPT conundrums: Probing plagiarism and parroting problems in higher education practices. College & Research Libraries News, 84 (6), 205. https://doi.org/10.5860/crln.84.6.205
Tlili, A., Shehata, B., Adarkwah, M. A., Bozkurt, A., Hickey, D. T., Huang, R., & Agyemang, B. (2023). What if the devil is my guardian angel: ChatGPT as a case study of using chatbots in education. Smart Learning Environments, 10 (1), 15. https://doi.org/10.1186/s40561-023-00237-x
Tsang, R. (2023). Practical applications of ChatGPT in undergraduate medical education. Journal of Medical Education and Curricular Development , 10 . https://doi.org/10.1177/23821205231178449
Yan, D. (2023). Impact of ChatGPT on learners in a L2 writing practicum: An exploratory investigation. Education and Information Technologies, 1–25. https://doi.org/10.1007/s10639-023-11742-4
Zhang, R., Zou, D., & Cheng, G. (2023a). A review of chatbot-assisted learning: pedagogical approaches, implementations, factors leading to effectiveness, theories, and future directions. Interactive Learning Environments , 1–29. https://doi.org/10.1080/10494820.2023.2202704
Zhang, S., Shan, C., Lee, J. S. Y., Che, S., & Kim, J. H. (2023b). Effect of chatbot-assisted language learning: A meta-analysis. Education and Information Technologies , 1–21. https://doi.org/10.1007/s10639-023-11805-6
Zhu, C., Sun, M., Luo, J., Li, T., & Wang, M. (2023). How to harness the potential of ChatGPT in education? Knowledge Management & E-Learning, 15 (2), 133. https://doi.org/10.34105/j.kmel.2023.15.008
Download references
Author information
Authors and affiliations.
College of Educational Science and Technology, Zhejiang University of Technology, Hangzhou, China
Xiaojiao Chen
College of Foreign Languages, Zhejiang University of Technology, Hangzhou, China
Department of Education Information Technology, Faculty of Education, East China Normal University, Shanghai, China
Chengliang Wang
You can also search for this author in PubMed Google Scholar
Corresponding author
Correspondence to Chengliang Wang .
Ethics declarations
Conflict of interest.
During the research, the authors indicate that no commercial or financial ties that may be regarded a possible conflict of interest existed.
Additional information
Publisher’s note.
Springer Nature remains neutral with regard to jurisdictional claims in published maps and institutional affiliations.
Rights and permissions
Springer Nature or its licensor (e.g. a society or other partner) holds exclusive rights to this article under a publishing agreement with the author(s) or other rightsholder(s); author self-archiving of the accepted manuscript version of this article is solely governed by the terms of such publishing agreement and applicable law.
Reprints and permissions
About this article
Chen, X., Hu, Z. & Wang, C. Empowering education development through AIGC: A systematic literature review. Educ Inf Technol (2024). https://doi.org/10.1007/s10639-024-12549-7
Download citation
Received : 19 October 2023
Accepted : 05 February 2024
Published : 29 February 2024
DOI : https://doi.org/10.1007/s10639-024-12549-7
Share this article
Anyone you share the following link with will be able to read this content:
Sorry, a shareable link is not currently available for this article.
Provided by the Springer Nature SharedIt content-sharing initiative
- Artificial intelligence generated content
- Artificial intelligence
- Systematic literature review
- Educational technology
- Find a journal
- Publish with us
- Track your research
- Open access
- Published: 07 July 2023
Exploring the use of social network interventions for adults with mental health difficulties: a systematic review and narrative synthesis
- Helen Brooks ORCID: orcid.org/0000-0002-2157-0200 1 ,
- Angela Devereux-Fitzgerald 1 ,
- Laura Richmond 1 , 2 ,
- Neil Caton 3 ,
- Mary Gemma Cherry 4 , 5 ,
- Penny Bee 1 ,
- Karina Lovell 1 , 6 ,
- James Downs 7 na1 ,
- Bethan Mair Edwards 8 na1 ,
- Ivaylo Vassilev 9 ,
- Laura Bush 10 &
- Anne Rogers 9
BMC Psychiatry volume 23 , Article number: 486 ( 2023 ) Cite this article
1911 Accesses
1 Citations
4 Altmetric
Metrics details
People with mental health difficulties often experience social isolation. The importance of interventions to enhance social networks and reduce this isolation is increasingly being recognised. However, the literature has not yet been systematically reviewed with regards to how these are best used. This narrative synthesis aimed to investigate the role of social network interventions for people with mental health difficulties and identify barriers and facilitators to effective delivery. This was undertaken with a view to understanding how social network interventions might work best in the mental health field.
Systematic searches using combinations of synonyms for mental health difficulties and social network interventions were undertaken across 7 databases (MEDLINE, Embase, PsycINFO, CINAHL, Cochrane Library, Web of Science) and 2 grey literature databases (EThoS and OpenGrey) from their inception to October 2021. We included studies reporting primary qualitative and quantitative data from all study types relating to the use of social network interventions for people with mental health difficulties. The quality of included studies was assessed using the Mixed Methods Appraisal Tool. Data were extracted and synthesised narratively.
The review included 54 studies, reporting data from 6,249 participants. Social network interventions were generally beneficial for people with mental health difficulties but heterogeneity in intervention type, implementation and evaluation made it difficult to draw definitive conclusions. Interventions worked best when they (1) were personalised to individual needs, interests and health, (2) were delivered outside formal health services and (3) provided the opportunity to engage in authentic valued activities. Several barriers to access were identified which, without careful consideration could exacerbate existing health inequalities. Further research is required to fully understand condition-specific barriers which may limit access to, and efficacy of, interventions.
Conclusions
Strategies for improving social networks for people with mental health difficulties should focus on supporting engagement with personalised and supported social activities outside of formal mental health services. To optimise access and uptake, accessibility barriers should be carefully considered within implementation contexts and equality, diversity and inclusion should be prioritised in intervention design, delivery and evaluation and in future research.
Peer Review reports
Mental health difficulties are increasingly globally and are one of the primary drivers of disability worldwide [ 1 , 2 ]. In the UK alone, 3.3 million adults in the United Kingdom (UK) were referred to mental health services between 2020 and 2021 [ 3 ]. More disability-adjusted life years are lost to mental health difficulties than to any other health condition in the UK, including cancer and heart disease, with considerable economic, societal and individual cost [ 4 ]. Adults with severe and/or enduring mental health difficulties, such as schizophrenia and bipolar disorder, face additional challenges; they are at greater risk of multiple physical health comorbidities, and have a 15-20-year shorter life expectancy than the general population [ 5 , 6 ]. Optimising the effectiveness and reach of mental health support for these people is essential to ensure high-quality care whilst minimising pressures on already-stretched NHS resources.
Community engagement and social connections can support people living with mental health difficulties in the community, sometimes preventing the need for the involvement of formal health service provision and providing support for recovery post-discharge [ 7 ]. Community engagement is often used as a proxy measure of community integration which is considered a fundamental aspect of recovery from mental health difficulties [ 8 ]. Evidence suggests that both close and distal social network support are associated with community integration [ 9 ]. However, recent research suggests both individual and wider barriers to community engagement [ 10 ]. This highlights the potential value of interventions designed specifically to mitigate both the individual level barriers such as physical and psychological capabilities and social barriers which reduce access to suitable community resources [ 10 ].
Social networks Footnote 1 , social connectivity and engagement in valued activities have multiple benefits for people with severe and/or enduring mental health difficulties and associated benefits for the services and people that support them. They enhance recovery and self-management, promote engagement with community-based support and extend the availability of heterogenous support for the secondary prevention of mental health difficulties, with potential to reduce direct healthcare costs [ 7 , 12 , 13 , 14 , 15 , 16 ]. It is theorised that formal and informal social support, interpersonal contact, and mobilisation of resources enhance individual coping strategies and functional support [ 17 , 18 ], thereby providing protection from stress and improving daily self-management of mental health difficulties [ 19 , 20 , 21 ]. In turn, social activity can increase the size and quality of an individual’s social network [ 22 ], further sustaining and enhancing social connectivity and well-being promotion [ 14 ].
The usefulness of social networks is contingent on the availability of requisite knowledge, understanding and willingness to provide help within networks. These are not always present, available or acceptable to individuals [ 23 ]. People with mental health difficulties tend to have smaller, less diverse networks of poorer quality and configuration, and tend to rely heavily on support from family members or health professionals [ 24 , 25 ]. Social network availability and configuration varies depending on the severity of mental health difficulties and availability of resource [ 26 ].
Interventions designed to improve people’s social networks by connecting them with meaningful and valued activities, people, and places, can extend access to support, thus aiding and sustaining recovery [ 25 ]. These interventions can be effective in optimising social connections for people with mental health difficulties [ 12 ]. It is important to note that social network interventions include those that strive to modify the composition or size of social networks by adding new members and those that seek to bring together existing network members to modify existing links to enhance the functional quality of a network. The former includes linking individuals to new activities or social situations where new network connections can be made [ 27 ] whilst the latter often take the form of network meetings which dependent on an individual’s personal situation bring together relevant network members (family, friends and other supporters) in order to optimise the consistency and connectedness of network support [ 28 ]. However, specific attention needs to be paid to implementation of these types of interventions because previous research in other fields suggest variability in uptake of network interventions, fluctuating capacity of organisations to deliver such interventions and organisational cultures which do not allow for sustainable implementation [ 29 , 30 ].
To fully translate social network interventions into mainstream mental health services and optimise their use, we must first ascertain how effective, acceptable and feasible existing interventions are, and understand their mechanisms of effect [ 12 ]. A recent systematic review examining the effectiveness, acceptability, feasibility and cost-effectiveness of existing interventions concluded that extant literature is in its infancy, but suggested that social network interventions which connect and support people to engage in social activities may be acceptable, economically viable and effective [ 12 ]. The aim of this review is to build on these findings by providing a critical overview of how social network interventions might work best for adults with mental health difficulties. We used systematic review methods to critically answer the following questions: for people with mental health difficulties, (i) what social network interventions work best and for whom; and (ii) what are the optimal conditions for implementing social network interventions?
Preferred Reporting Items for Systematic Reviews and Meta-Analysis (PRISMA) guidance [ 31 ] informed the methods and reporting of this systematic review and narrative synthesis, and the protocol is available from: https://www.crd.york.ac.uk/prospero/display_record.php?ID=CRD42020206490 .
Eligibility
Published journal articles or dissertations reporting primary data on the use of interventions designed specifically to improve and/or measure social network quantity or quality for people with mental health difficulties were included in this review. Review articles were excluded but reference lists of identified reviews were checked for potentially relevant articles. Only studies with a sample mean age ≥ 18 years and a minimum of 75% of participants with primary diagnosis of mental health difficulties (self-report or physician defined) were included.
No restrictions were imposed in relation to language or date of publication. Non-English language articles were screened for eligibility by native speakers affiliated with the research team. Papers where the sample held a primary diagnosis of substance misuse, autism spectrum disorders, dementia, attention deficit hyperactivity disorder (ADHD), or cognitive impairment were excluded. Also excluded were dyadic interventions or individual-level interventions such as purely social skills/cognition programmes. Table 1 displays full inclusion and exclusion criteria.
Search strategy
The following seven databases were searched in August 2020 with searches updated in October 2021: Medline, Embase, PsycINFO, CINAHL, Cochrane Library, and Web of Science. Published reviews and literature on social network interventions informed the search strategy which was agreed with the wider authorship team and was subject to a Peer Review of Electronic Search Strategies (PRESS) review by an expert librarian [ 32 ]. Search terms were organised using the first two components of the Population, Intervention, Comparator, Outcome (PICO) framework (Population: People with a diagnosis of mental health difficulties or self-reported emotional distress and Intervention: Social network) and were intentionally broad to maximise search returns (see Additional File 1 for an example search syntax).
To reduce publication bias, grey literature sites such as EThoS and OpenGrey were also searched. We also contacted authors of possibly eligible conference abstracts for full manuscripts where these were not readily available and examined identified review articles and book chapters for relevant literature.
Data extraction
The data management software Covidence ( http://www.covidence.org ) was used to aid the data selection and extraction process. After duplicates were removed, titles and abstracts of identified studies were screened independently by two reviewers, and conflicts were resolved by a third reviewer in line with the inclusion/exclusion criteria (See Table 1 ). Full text reviews of potential papers were undertaken by the team, with two reviewers independently screening each paper and conflicts resolved by consensus.
Standardised data extraction forms were created in Excel and used to extract data from all eligible papers by seven team members (HB, ADF, LR, NC, JD and BME), including both academic and patient and public involvement (PPI) researchers. Extracted data included social network measures (where applicable), factors of context, mechanisms and outcomes, acceptability and standard demographics. Interventions were categorised into broad groups for the purposes of analysis by one member of the research team (HB) and checked for accuracy by another (MGC). For qualitative data, both raw data quotations and author interpretations were extracted where applicable and identified as such. A second reviewer from those outlined above (HB and ADF) cross-checked 30% of extracted data from each member of the review team for accuracy.
Quality appraisal
The Mixed Methods Appraisal Tool (MMAT) [ 33 ] was used to assess the quality of the included papers, applicable to the broad range of research arising in this review [ 34 ]. Each full-text paper was quality assessed by one reviewer in parallel with data extraction with 10% of quality appraisals cross-checked for accuracy. Disagreements were resolved through consensus.
Data synthesis
Meta-analysis of the quantitative data was not possible due to the heterogeneity of included studies. Consequently, a narrative synthesis was undertaken following the stages outlined in the Guidance on the Conduct of Narrative Synthesis in Systematic Reviews [ 35 ]. Data were collated and textual summaries of study characteristics were produced via data extraction spreadsheets (including intervention content, study design, participants, recruitment and delivery). Qualitative data from qualitative and mixed methods studies were explored inductively using aspects of thematic synthesis: a thematic framework was developed consisting of themes which were refined, merged, split or created, as necessary, with analysis of each study [ 36 ]. Constant comparison was used to translate concepts between studies [ 37 , 38 ]. Verification of findings were provided by a second researcher and verified by the wider team. The thematic framework was also applied to the quantitative data from quantitative studies and mixed-methods studies, which aided with the visual grouping of patterns across the whole data set. Quality appraisals were used to assess the robustness of the thematic analysis by removing papers of the lowest quality from each theme to consider their impact on overall presentation. No themes needed to be revised following this process so references were added back into the synthesis [ 35 ]. Data from all included studies were also grouped on aspects of context such as delivery setting and approach, diagnosis and significance of results [ 35 ]. Analytical themes were inferred from the material inherent in the descriptive findings and, together with the patterns apparent across the whole data set formed the narrative synthesis. This synthesis allowed interpretation of the concepts arising in this review beyond the primary findings of individual papers.
As shown in Fig. 1 , searches identified 22,367 potentially relevant studies, resulting in 19,575 unique citations after de-duplication. The full texts of 841 studies were reviewed for relevance, resulting in the inclusion of 54 unique papers. Of these, 17 were randomised controlled trials (RCTs), 12 were quantitative studies of other designs (‘other quantitative studies’), 13 studies were qualitative, and 12 used mixed methods. Studies were conducted in the following countries: UK (n = 25), United States of America (n = 8), Australia (n = 5), China, India, Ireland, Italy, Netherlands, Sweden and Canada (all n = 2), Denmark and Hungary (n = 1). For more information on included studies, see Additional File 2 and Additional File 3 for a completed PRISMA checklist.
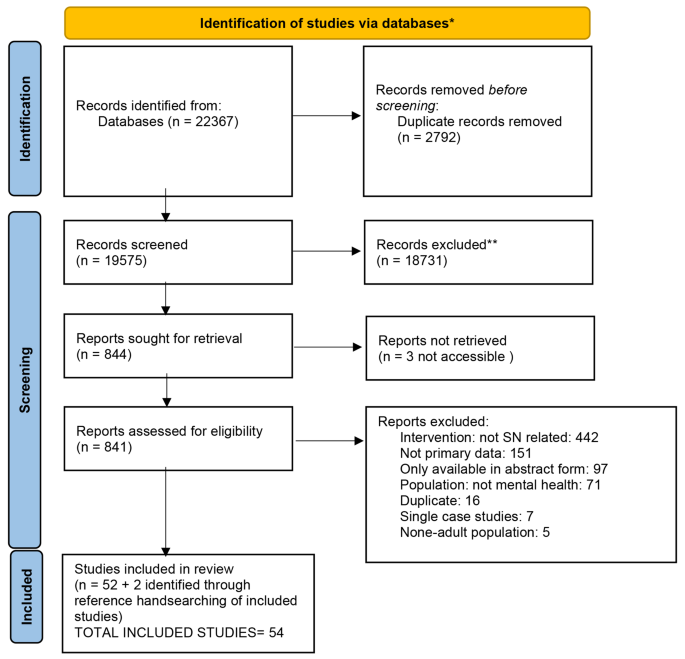
PRISMA 2020 flow diagram
There was a total of 6,249 participants recruited across the included studies with an average age of 47.42 years and broadly equivalent numbers of males and females. Most (21/54) studies recruited participants with mixed forms of mental health difficulties and emotional distress. The remaining studies included only participants with the following diagnoses or self-reported difficulties Footnote 2 :
Psychosis and/or schizophrenia (n = 12).
Serious and/or long-term mental health difficulties (n = 10).
Depression (n = 4).
Mild to moderate mental health difficulties (n = 2).
DSM AXIS 1 disorders (e.g. anxiety disorders, such as panic disorder, social anxiety disorder, and post-traumatic stress disorder) (n = 2).
Psychotic and affective disorders (n = 1).
Eating disorder (n = 1).
PTSD and depression (n = 1).
For more information on participants in included studies, see Additional File 2 .
The 54 included studies reported on 51 unique interventions which were broadly categorised into five types. The most commonly reported interventions were those that supported community or social activities (25/51). These included 13 interventions that supported access to existing community resources and activities, 3 football interventions, 5 horticulture or nature-based interventions, 3 arts-based intervention and one which involved closed group social activities. The second most included type of intervention (13/51) was intensive or enhanced community treatment. These were mostly assertive community treatment (n = 3), case management approaches (n = 3) and specialised community treatment teams (n = 2). There was also one reported example of each of the following: day centre, community club, social recreation team, occupational therapy and rehabilitation specialised services. There were 7 peer support group interventions within included studies and 3 one-to-one interventions (behavioural activation, cognitive behavioural therapy and peer-led recovery). Three interventions were classified as other and these included 2 action research approaches and an enhanced sheltered accommodation project. Please see Table S1 in the additional files for additional detail on included interventions.
23/54 intervention activities were additional to statutory provision and delivered externally to statutory services, 9 were additional but were delivered within health services, 1 was a combination of both internally and externally delivered activities and 21 were designed to enhance existing provision.
There was minimal description of formal patient and public involvement (PPI) in the included studies, with notable few exceptions (n = 10; [ 39 , 40 , 41 , 42 , 43 , 44 , 45 , 46 , 47 , 48 ]). PPI activities included participatory approaches such as Photovoice [ 39 ], co-production activities [ 47 ], peer researchers/facilitators [ 40 , 41 , 42 , 44 ] and inclusion of advisory groups or public advisors [ 43 , 45 , 46 , 48 ].
Quality assessment
Details of the quality assessment of included studies is found in Additional File 2 which includes assessments for each type of study. Quality assessments and main methodological weaknesses for each type of study are summarised in Table 2 .
Review question 1: what type of social network interventions work best and for whom?
Of the 17 RCTs, 12 other quantitative and 12 mixed-methods studies included, 9 RCTs [ 49 , 50 , 51 , 52 , 53 , 54 , 55 , 56 , 57 ], 3 other quantitative studies [ 58 , 59 , 60 ] and 1 mixed-methods study [ 61 ] used included a formal quantitative measure of social network size or quality. Of these, 7 provided evidence of statistically significant improvements to social networks post intervention [ 49 , 51 , 53 , 55 , 56 , 58 , 59 ] and others demonstrated improvements which favoured the intervention group but did not reach statistical significance [ 52 , 54 , 62 ]. Just over half (5/9) of the RCTs examined the effectiveness of interventions using aspects of supported socialisation [ 53 , 54 , 56 , 59 , 62 ], highlighting the potential value of these types of interventions for people with mental health difficulties. The follow up periods for RCTs ranged from 3 months to 24 months and effect sizes were generally small to moderate when compared to usual care – ranging from 0.39 to 0.65 [ 12 ].
A range of statistically significant improvements in other outcomes following intervention were reported across the included studies. These included mental health symptomatology [ 44 , 51 , 55 , 63 , 64 , 65 , 66 , 67 ], general health [ 68 ], social anxiety [ 69 ], social support, social capital and satisfaction with aspects social relationships [ 43 , 49 , 50 , 70 ], distress [ 50 ], general and social functioning/engagement [ 43 , 44 , 54 , 63 , 64 , 65 , 71 , 72 , 73 , 74 ], occupational functioning [ 75 ], structured activity levels [ 76 ], loneliness [ 43 , 54 , 64 , 69 ], relatedness and social inclusion [ 42 , 44 ], sense of belonging [ 69 ], self-esteem [ 43 ], quality of life [ 43 , 72 ], wellbeing [ 42 , 64 , 77 ], treatment adherence [ 72 ], service use [ 44 , 52 ] and satisfaction with care [ 52 , 72 ]. Of these 24 studies, 20 provided information on follow-up periods which were on average 9 months ranging from 2 to 18 months. See Additional File 2 .
It was not possible from included studies to draw definitive conclusions about the groups of people for whom these interventions work best due to the heterogeneity of participants in included studies. However, 8 of the 28 studies that demonstrated significant improvements in outcomes evaluated the effectiveness of interventions for people with schizophrenia and/or psychosis. Significant improvements at follow-up across studies were also associated with:
Being female [ 57 , 58 , 63 ].
Being married [ 43 , 58 ].
Living with a spouse or partner [ 43 ].
Completing A-levels [ 57 ].
Fewer negative symptoms [ 57 ].
Larger network at baseline [ 57 ].
Better baseline functioning [ 63 ].
Greater distress from positive symptoms [ 51 ].
Longer duration of illness [ 51 ].
People who demonstrated improvement in other outcomes [ 56 ].
Undertaking more social activities [ 51 , 65 ].
Having a better clinical prognosis [ 56 ].
What are the optimal conditions for the implementation of social network interventions?
Synthesis of data from qualitative and mixed-methods studies identified a range of barriers and facilitators to implementing social network interventions which are presented in Table 3 (Individual level barriers and facilitators) and Table 4 (Provider/agender level barriers and facilitators). Overarching themes identified during the narrative synthesis relating to optimal conditions for implementation and how interventions are thought to bring about changes in outcomes are presented below with supporting quotes presented in Table 5 .
Bridging the gap – the fundamental role of facilitation
Facilitators played a central role in the successful implementation of social network interventions for all types of mental health difficulties. A facilitator could support the initiation of social activity through personalised discussions about activity options and going along to activities with an individual until they had developed sufficient skills, knowledge and confidence to undertake activities on their own [ 39 , 40 , 42 , 46 , 48 , 62 , 67 , 78 , 79 , 80 , 81 , 82 ]. To do this well, facilitators needed to have sufficient local knowledge, empathy and engagement skills [ 83 , 84 ]. The development of interpersonal trust and provision of suitable options for an individual to consider were considered key to successful facilitation [ 41 , 84 ]. Other requirements included being non-judgemental, approachable, friendly, and having a basic understanding of mental health difficulties [ 41 , 42 , 48 , 62 , 66 , 67 , 74 , 82 , 85 ]. A mutual understanding and respect of roles and boundaries was also crucial to successful facilitation [ 79 ].
Participants described how facilitators needed to maintain a delicate balance between providing support to engage with new social activities and promoting independence to ensure future sustainability [ 39 , 46 , 83 ]. Facilitators could support the uptake of social activities by providing structured programmes with sufficient flexibility to overcome individual barriers to accessing local activities [ 40 ]. Studies also highlighted the need for adequate training and supervision for facilitators in advance of programmes starting and for consideration to be given to the end of interventions when contact with the facilitator ended. Sufficient facilitator relationships coming to an end and for consideration of how benefits would be sustained once the programme ended [ 67 , 79 , 81 ].
Whilst there wasequivalence in the quantitative effectiveness data in relation to peer versus non-peer facilitators [ 12 ], qualitative data identified particular strengths of peer facilitators in relation to having shared experiences and having opportunities to model behaviours. Peer facilitators were seen to provide hope for the future as an example of someone who had recovered from a mental health difficulty, and also to reduce the imbalance of power between facilitator and service user, which improved their relationship [ 41 , 79 ].
Social network interventions could benefit facilitators and host organisations by increasing knowledge about, and access to community infrastructure which provided s ongoing support to service users. Additionally, professionals were able to develop more in-depth understanding of individual service users during such interventions, which could improve understanding about individual triggers of distress and relapse [ 67 , 79 ].
My voice, my choice, my pace – the need for flexibility and valuing individual differences
Social network interventions worked best when service users felt they could choose activities within interventions which mirrored their own interests [ 46 , 48 , 67 ]. This improved uptake and engagement with social activities particularly when users felt that their voices were being heard and their choices considered [ 39 , 40 , 83 , 84 ].
Acknowledgement of individual differences and allowing people to be who they are whilst providing gentle encouragement appeared to increase engagement with valued activities [ 46 , 62 , 79 , 86 ]. Participants, particularly those with serious and/or enduring mental health difficulties, experienced increased motivation for, and enjoyment of, self-selected activities [ 79 , 82 , 86 ]. Participants described such activities as evoking a sense of fun and spontaneity which helped them to be playful and self-expressive [ 77 , 79 , 87 ] as well as to laugh and be adventurous [ 41 ]. Engagement with valued activities was seen as empowering and participants expressed an increased desire for future engagement, feeling as though they were seen as a person rather than an ‘illness’ [ 42 , 62 , 88 ]. Participation was optimised if space was provided to allow people to try different activities and ascertain what was most enjoyable for them. This allowed people to become familiar with, and embedded into, intervention locations [ 48 , 66 , 74 ].
Participants described the impact that their mental health and other external circumstances could have on their ability or readiness to engage with social network interventions. Studies recommended flexibility in implementation to mitigate against this [ 67 , 77 , 78 , 80 , 82 ]. Interventions worked best when participants felt that they could be honest in relation to their own boundaries/capabilities [ 80 ] and when they could be left alone when they needed to be [ 46 ]. This flexibility and acceptance of individual situations meant people felt their own needs, choices and health were being adequately considered, which allowed them to push themselves further than they might have thought possible [ 46 , 80 ]. It also appeared to contribute to a sense of agency and control over their own participation which was deemed important for successful engagement with social network interventions [ 46 , 82 ]. Not having individual needs met through a lack of flexibility could result in withdrawal from intervention activities [ 81 ].
Another key feature of successful social network interventions was allowing participants to progress at their own pace, one that was manageable given their individual circumstances [ 62 , 79 , 82 , 85 , 89 ]. Any pressure to move faster than this or at another’s pace was viewed as a potential barrier to these types of interventions. One study with people with serious mental health difficulties found that those who engaged with social activities independently were more consistent and committed in their engagement, and this was attributed to the ability to go at their own pace [ 78 ].
Similarly, social network interventions should not be seen as a quick fix or panacea for people with mental health difficulties. What is experienced as valuable and beneficial for one person is likely to be different for another and individual preferences may change over time. These types of interventions need to be personalised to individuals to ensure they meet people’s needs and that expectations for engagement are realistic for the individual [ 46 , 67 , 79 ]. It was recognised, within included studies, that not everyone would be able to engage with social network interventions, and this should be factored in from the outset and a flexible approach undertaken [ 79 , 80 ]. Flexibility in delivery also incorporated the ability to include the wider family, friends and other supporters in intervention activities where appropriate and desired [ 46 ].
Social building blocks – rebuilding or acquiring social resources and skills and making connections with others
Social network interventions were considered to work best when they enabled individuals to build on existing or develop new skills whilst also being supported to make connections with others [ 45 , 83 , 86 ]. This applied at an individual level (self-esteem, self-efficacy, resilience, social skills, self-management) and social network level (quantity and quality of new and existing social networks) [ 39 , 41 , 42 , 45 , 46 , 48 , 77 , 78 , 81 , 82 , 83 , 87 , 88 , 89 ]. Individual-level improvements were considered necessary in order to realise benefits from social network interventions [ 84 ]. Such benefits could be conferred formally through didactic sessions or naturally through group interactions [ 46 , 48 ].
Benefits could impact on other wider aspects of everyday life including health and employment [ 40 , 41 , 62 , 84 , 87 ] as well as having ripple effects on friends and family through the sharing of knowledge about social and cultural activities in local areas [ 78 ].
A range of potential mechanisms through which interventions were thought to bring about benefits were identified. Social network interventions provided the opportunities for distraction, allowing attendees to clear their minds which promoted self-reflection and the ability to process negative thoughts through engagement with valued activities [ 42 , 62 , 82 , 86 ]. This led to calmer states which enabled cognitive and social skills to develop or be re-established [ 62 , 86 ].
Social acceptance through connectedness with the local community helped individuals to see themselves in a more positive light, reminding them of their own strengths whilst challenging previously-held beliefs about what they could and could not do [ 48 , 77 , 80 , 82 ]. One study found that that the use of humour around previously shame-inducing situations could support people to disidentify with negative identities and increase their sense of belonging [ 80 ]. This, combined with undertaking new or re-engaging with lost skills and pursuits, could engender a sense of pride and hope for the future [ 41 , 45 , 46 , 77 , 79 , 89 ]. Studies also highlighted how connections made during intervention activities were considered to reduce the intensity of interactions within existing networks thereby improving social interactions more generally [ 45 ].
Participants described a virtuous cycle whereby participating in social network interventions developed skills and capabilities to support social connectedness, which in turn stimulated a sense of purpose, renewed interest in the world and desire for further social engagement and participation [ 39 , 41 , 46 , 62 , 77 , 78 , 81 , 83 , 86 ].
However, such benefits were not seen in all those who accessed social network interventions [ 40 ]. Involvement in interventions which were considered too challenging or encouraged downward social comparison had little impact on individual or social network outcomes [ 40 ].
The importance of a positive and safe space to support community integration
Participants expressed a strong desire to reduce social isolation and valued interventions that promoted community integration [ 42 , 77 , 87 ]. A key factor in the success of social network interventions was the context in which the intervention was delivered. Social network interventions were considered more likely to be successful if delivered in community venues external to formal health services. Those delivered in group settings were experienced as less intimidating as there was less pressure to make one-to-one connections [ 80 ].
Successful participation in real world activities was highly valued and indeed necessary for participants to benefit from social network interventions [ 39 , 83 ]. Participants felt that interventions should be integrated into local communities and provide an access point to resources rather than further segregating people with mental health difficulties [ 80 , 86 ]. However, such interactions could be challenging due to concerns about stigmatisation and previous negative experiences; facilitation or support to mitigate this was identified as imperative across studies [ 39 , 48 , 77 , 78 , 80 ]. This was particularly important at the early stages of involvement before trust and belonging had developed [ 80 ]. Engagement in shared activities that were not overburdensome (e.g. sport, games, shopping) helped to develop community relationships and overcome initial doubts and concerns [ 39 ].
Community engagement in non-judgemental settings had a range of benefits including increased community integration and improved connection to society more generally. These appeared particularly salient for those with serious mental health difficulties [ 78 , 86 , 89 ]. They also fostered the development of transferable skills that were easily integrated into everyday life and provided connections to wider society beyond the health care system [ 66 , 78 , 79 , 82 , 83 , 84 ]. It was considered important to foster connections with people in the community who understood but did not necessarily have direct experience of mental health difficulties so that the focus of interactions was on shared interests or hobbies rather than ‘illnesses’ [ 40 , 42 , 48 , 62 , 84 ]. Self-selected, reciprocal and naturally occurring social connections were highly valued and considered more likely to occur outside of formal mental health settings [ 41 , 42 , 46 , 81 , 85 , 86 ]. Participants also valued opportunities to help others and give back to the community [ 40 ].
Participants’ feeling safe, relaxed and accepted during intervention activities was considered instrumental to successful implementation of social network interventions. This was supported, where necessary, by home visits, particularly prior to community engagement [ 39 , 40 , 41 , 46 , 48 , 79 , 80 ]. These were more easily arranged for interventions in non-statutory settings, and particularly for nature or arts-based activities [ 42 , 48 , 62 , 74 , 77 , 78 ]. Outdoor interventions were generally considered to be naturally restorative, calm, peaceful and safe which facilitated social interactions [ 48 , 74 , 86 ].
The need for available, accessible and sustainable activities
The availability of appropriate community resources for supported socialisation interventions and those interventions led by the third sector was raised as a challenge to the implementation of social network interventions in included studies [ 39 , 83 ]. Funding for third sector activities was often precarious which meant that activities stopped with little notice. This was hard for intervention facilitators to keep abreast of and could be demotivating for participants [ 39 ]. Adequate staff training in relation to awareness of such activities locally and optimal ways to connect people to them was raised as a key facilitator to success.
Lack of accessibility to intervention activities was also highlighted as a barrier to intervention success. Issues included lack of funding for transport and access [ 39 , 46 , 67 , 84 ], gender inaccessibility within activities [ 40 , 41 , 77 ], inflexibility which reduced accessibility for those with work or caring responsibilities [ 67 , 78 , 79 , 89 ], stigma [ 41 ], lack of support for social anxiety and amotivation [ 42 , 66 , 67 , 77 , 79 , 80 , 82 ], social barriers, such as social norms and stereotypes [ 41 ], language barriers and low literacy [ 67 , 77 ], rurality [ 67 ], and safety concerns created by location or timing of activities (e.g. at night) [ 78 ]. These barriers were particularly pertinent for participants who lacked family support to attend groups [ 41 ].
Insufficient consideration of accessibility issues could exacerbate health inequalities and meant that participants felt unable to realise their own potential for social connectedness [ 39 , 84 ]. An example of particularly accessible environments was public allotments, which were considered widely available, inexpensive and inclusive settings, and as a result involvement was easier to maintain post intervention [ 86 ]. The provision of stipends was found to be a useful way to mitigate financial barriers [ 78 ].
Concerns were raised about the sustainability of certain activities and the potential impact of this on intervention participants [ 83 ]. Several studies highlighted harms caused by ending interventions without adequate consideration of how activities would be sustained [ 79 , 87 ]. Most documented sustainability was attributed to participants’ planning for maintenance both within and outside their own networks [ 42 , 78 , 82 ]. This was considered particularly useful when facilitated as part of the intervention itself [ 46 , 79 ], or where ongoing post-intervention engagement with activities or individuals was supported [ 62 , 82 , 87 ].
This systematic review and narrative synthesis aimed to identify and synthesise current evidence pertaining to the use of social network interventions for people with mental health difficulties, with a view to understanding their effectiveness, and the conditions in which these interventions might work best. Collectively, data from the 54 included studies demonstrated the utility of these types of interventions for people with mental health difficulties in terms of improving social networks and other health and social outcomes. Studies included a breadth of data and range of implementation and evaluation methods that lacked an explicit focus on context and outcome relationships. This made it difficult to draw definitive conclusion about what types of interventions work best for whom. However, we were able to identify conditions in which interventions can be optimally implemented.
In line with previous research, data supported the potential utility of interventions which focussed on supporting socialisation for people with mental health difficulties [ 12 , 90 , 91 ]. Most (21/54) studies included people with a range of mental health diagnoses. The remainder included participants with diagnoses of schizophrenia and/or psychosis (n = 12) or serious and/or enduring mental health difficulties (n = 10) with lesser attention given to other diagnoses. As a result, it was not possible to ascertain whom social network interventions worked best for. Encouragingly, participants demonstrated a strong desire for interventions which reduced social isolation and promoted community integration, suggesting high levels of acceptability across mental health conditions. Despite this, it is unlikely that social network interventions are a panacea, with the qualitative studies demonstrating the need to consider individual readiness for intervention participation and to ensure that interventions are sufficiently personalised to individual needs, preferences, heath, and access requirements [ 39 , 83 ].
Factors that affected the implementation of social network interventions mirrored and extended those identified in the physical health field [ 23 , 92 ]. In the current review, greater salience was given to the value of freedom, choice and personalisation within intervention activities, the need for individuals to be heard and progress at their own pace, and safe and non-judgemental spaces for intervention activities. Participants were more likely to raise concerns about stigma relating to mental health or past negative experiences with community organisations, which may relate to differences in the experience of mental health difficulties when compared to physical health difficulties [ 93 ]. Specific requirements relating to mental health and appropriate facilitation in this regard suggests a need for mental health specific training for intervention facilitators. Factors affecting the implementation of social network interventions appeared broadly applicable across mental health conditions and nuances in identified barriers and facilitators for people with specific diagnoses or severity were not discernible. Future research is required to ascertain whether there are condition-specific challenges to accessing social network interventions so that strategies to mitigate these can be developed.
This manuscript adds to existing literature by demonstrating the complexity of implementing social network interventions in the mental health field and identifying a range of access- related barriers which can hinder engagement. Failure to adequately consider the context in which an intervention will be delivered can exacerbate existing health inequalities by reducing access to potentially effective interventions [ 6 ]. This was evidenced across included studies; participants who were female, white, educated, married and had stronger baseline social networks and functioning were the most likely to access and benefit from these types of interventions [ 43 , 57 , 63 ]. This highlights the need for pre-implementation preparation to fully understand local delivery contexts and the needs of all those who might benefit from such interventions [ 94 ]. It is notable that only 2/54 included studies involved transgender participants and no included studies recorded the sexual orientation of study participants or considered neurodiversity. There were also limited numbers of identified facilitators in the included studies which related to issues of diversity and inclusion (Tables 3 and 4 ). This supports wider calls for prioritisation of equality, diversity and inclusion in the design, delivery and evaluation of social network interventions in future research in order to maximise intervention benefits [ 95 ]. This could be facilitated through co-production activities with those from diverse backgrounds and who represent or have insight into these communities.
This review identified a range of facilitators and barriers to implementing social network interventions in the mental health field potentially identifying a fundamental set of requirements as well as more bespoke requirements specific to type of need (Tables 3 and 4 ). Concomitantly, it highlighted the need to consider both downstream and upstream factors relating to implementation (i.e., individual motivation, capabilities, and opportunities and social and organisation level capacity). There was a particular tension between the sustainability of intervention activities and meaningful outcomes for participants. Consideration should therefore be given to how interventions are delivered (e.g. length of engagement time and potential enhanced role of facilitators) and the need to prioritise valued resources/activities that are sustainable [ 23 ].
In terms of implications for health services, findings illustrated the importance of targeting people with lower levels of baseline social functioning and people with smaller social networks or networks of poorer quality at baseline [ 57 , 63 ]. Given the need for a safe and accessible venue for intervention delivery and the importance of the facilitator role, provision for someone to accompany people to activities, especially during early interactions should be included in intervention design [ 80 ]. The findings also lend support to recent calls to reorient mental health service provision and reduce the focus on individual psychopathology and one-to-one interactions with health professionals [ 96 , 97 ]. An alternative focus oncare provision through outreach work and engagement with community resources, to harness the collective value of social networks would potentially be of more value [ 98 ]. This review also highlights the need to prioritise third sector funding to provide suitable resources for people to access [ 29 ].
Strengths and limitations
This review benefits from comprehensive search strategies which incorporated both published and grey literature, the inclusion of papers published in languages other than English, and the rigour of screening and extraction processes. Hand-searching of relevant journals and included papers identified a further two papers to be included. Another strength was the inclusion of seven members of the review team who had lived experience of mental health difficulties and two members who had clinical experience of delivering mental health care. This enhanced the review in several ways: ensuring that search terms were inclusive and comprehensive; clarifying understanding of social network interventions; and enhancing contextualisation of implementation barriers and facilitators. The qualitative studies provided most learning in relation to the use of social network interventions for people with mental health difficulties. There is a need to further this research by testing these factors against outcomes through powered mechanistic trials.
Several limitations should also be considered. First, incorporation of two grey literature databases is unlikely to have fully addressed potential publication bias. Second, whilst attempts were made to integrate study quality into the narrative synthesis, the overall quality of included studies may have impacted on the synthesis presented. Finally, the review only included the views of participants in social network interventions in relation to perceived barriers and facilitators to implementation, and it may be that these participants were not fully aware of all the potential factors that impacted implementation. The review also did not include those who had a primary diagnosis of substance misuse, autism spectrum disorders, dementia, attention deficit hyperactivity disorder (ADHD), or cognitive impairment. These limitations should be carefully weighed against the feasibility of managing and synthesising manuscripts from a review strategy that was more inclusive.
Strategies for improving the social networks of people with mental health difficulties should focus on ensuring access to personalised and supported social activities outside of formal mental health services. To optimise access and uptake, accessibility barriers should also be carefully considered within implementation contexts, and equality, diversity and inclusion should be prioritised in intervention design, delivery and evaluation, as well as in future research in this area.
Data availability
All data generated or analysed during this study are included in this published article [and its supplementary information files].
Personally-meaningful communities, connections and ties which link people to relationships, resources and activities that may help to manage and optimise their mental health [ 11 ].
Terms are used that were included in the original paper.
Abbreviations
Attention deficit hyperactivity disorder
Mixed Methods Appraisal Tool
Population, intervention, comparatory, outcome
- Patient and public involvement
Peer Review of Electronic Search Strategies
Preferred Reporting Items for Systematic Reviews and Meta-Analysis
randomised control tried
United Kingdom
Kessler RC, Aguilar-Gaxiola S, Alonso J, Chatterji S, Lee S, Ormel J, et al. The global burden of mental disorders: an update from the WHO World Mental Health (WMH) surveys. Epidemiol Psichiatr Soc. 2009;18(1):23–33.
Article PubMed PubMed Central Google Scholar
Vos T, Allen C, Arora M, Barber RM, Bhutta ZA, Brown A, et al. Global, regional, and national incidence, prevalence, and years lived with disability for 310 diseases and injuries, 1990–2015: a systematic analysis for the global burden of Disease Study 2015. Lancet. 2016;388(10053):1545–602.
Article Google Scholar
Iacobucci G. England saw record 4.3 million referrals to mental health services in 2021. BMJ. 2022;376:o672.
Article PubMed Google Scholar
Ferrari AJ, Norman RE, Freedman G, Baxter AJ, Pirkis JE, Harris MG, et al. The burden attributable to mental and substance use disorders as risk factors for suicide: findings from the global burden of Disease Study 2010. PLoS ONE. 2014;9(4):e91936.
Chesney E, Goodwin GM, Fazel S. Risks of all-cause and suicide mortality in mental disorders: a meta-review. World psychiatry: official journal of the World Psychiatric Association (WPA). 2014;13(2):153–60.
Public Health England. Severe mental illness (SMI) and physical health inequalities: briefing. London: Public Health England; 2018.
Google Scholar
Salehi A, Ehrlich C, Kendall E, Sav A. Bonding and bridging social capital in the recovery of severe mental illness: a synthesis of qualitative research. J Ment Health. 2019;28(3):331–9.
Townley G, Kloos B, Wright PA. Understanding the experience of place: expanding methods to conceptualize and measure community integration of persons with serious mental illness. Health Place. 2009;15(2):520–31.
Townley G, Miller H, Kloos B. A little goes a long way: the impact of Distal Social Support on Community Integration and Recovery of individuals with Psychiatric Disabilities. Am J Community Psychol. 2013;52(1–2):84–96.
Baxter L, Burton A, Fancourt D. Community and cultural engagement for people with lived experience of mental health conditions: what are the barriers and enablers? BMC Psychol. 2022;10(1):71.
Vassilev I, Rogers A, Blickem C, Brooks H, Kapadia D, Kennedy A et al. Social Networks, the ‘work’ and work force of chronic illness Self-Management: a Survey Analysis of Personal Communities. PLoS ONE. 2013;8(4).
Brooks H, Devereux-Fitzgerald A, Richmond L, Bee P, Lovell K, Caton N et al. Assessing the effectiveness of social network interventions for adults with a diagnosis of mental health problems: a systematic review and narrative synthesis of impact. Soc Psychiatry Psychiatr Epidemiol. 2022.
Brooks HL, Bee P, Lovell K, Rogers A. Negotiating support from relationships and resources: a longitudinal study examining the role of personal support networks in the management of severe and enduring mental health problems. BMC Psychiatry. 2020;20(1):50.
Sweet D, Byng R, Webber M, Enki DG, Porter I, Larsen J, et al. Personal well-being networks, social capital and severe mental illness: exploratory study. Br J Psychiatry. 2018;212(5):308–17.
Evert H, Harvey C, Trauer T, Herrman H. The relationship between social networks and occupational and self-care functioning in people with psychosis. Soc Psychiatry Psychiatr Epidemiol. 2003;38(4):180–8.
Article CAS PubMed Google Scholar
Daker-White G, Rogers A. What is the potential for social networks and support to enhance future telehealth interventions for people with a diagnosis of schizophrenia: a critical interpretive synthesis. BMC Psychiatry. 2013;13.
Berkman LF, Glass T, Brissette I, Seeman TE. From social integration to health: Durkheim in the new millennium. Soc Sci Med. 2000;51(6):843–57.
Kawachi I, Berkman LF. Social ties and mental health. J Urban Health. 2001;78(3):458–67.
Article CAS PubMed PubMed Central Google Scholar
Pescosolido BA. Beyond rational choice: the Social Dynamics of how people seek help. Am J Sociol. 1992;97(4):1096–138.
Pescosolido BA. Illness careers and network ties: a conceptual model of utilization and compliance. In: Albrecht G, Levy J, editors. Advances in medical sociology. Greenwich, CT: JAI Press; 1991. pp. 161–84.
Pescosolido BA, Gardner CB, Lubell KM. How people get into mental health services: stories of choice, coercion and “muddling through” from “first-timers. Soc Sci Med. 1998;46(2):275–86.
Putnam RD, Leonardi R, Nanenetti R. Making democracy work: civic traditions in modern Italy. Princeton: Princeton University Press; 1993.
Vassilev I, Band R, Kennedy A, James E, Rogers A. The role of collective efficacy in long-term condition management: a metasynthesis. Health Soc Care Commun. 2019;27(5):e588–e603.
Perry BL, Pescosolido BA. Social Network Dynamics and Biographical Disruption: The Case of “First-Timers” with Mental Illness. American Journal of Sociology. 2012;118(1):134–75.
Albert M, Becker T, McCrone P, Thornicroft G. Social Networks and Mental Health Service Utilisation - a literature review. Int J Soc Psychiatry. 1998;44(4):248–66.
Rusca R, Onwuchekwa I-F, Kinane C, MacInnes D. Comparing the social networks of service users with long term mental health needs living in community with those in a general adult in-patient unit. Int J Soc Psychiatry. 2021:00207640211017590.
Brooks H, Devereux-Fitzgerald A, Richmond L, Caton N, Newton A, Downs J, et al. Adapting a social network intervention for use in secondary mental health services using a collaborative approach with service users, carers/supporters and health professionals in the United Kingdom. BMC Health Serv Res. 2022;22(1):1140.
Tracy EM, Biegel DE. Preparing Social Workers for Social Network Interventions in Mental Health Practice. J Teach Social Work. 1994;10(1–2):19–41.
Ellis J, Kinsella K, James E, Cheetham-Blake T, Lambrou M, Ciccognani A et al. Examining the optimal factors that promote implementation and sustainability of a network intervention to alleviate loneliness in community contexts. Health Soc Care Community. 2022.
Biegel DE, Tracy EM, Song L. Barriers to social network interventions with persons with severe and persistent mental illness: a survey of mental health case managers. Commun Ment Health J. 1995;31(4):335–49.
Article CAS Google Scholar
Page MJ, McKenzie JE, Bossuyt PM, Boutron I, Hoffmann TC, Mulrow CD, et al. The PRISMA 2020 statement: an updated guideline for reporting systematic reviews. BMJ. 2021;372:n71.
McGowan J, Sampson M, Salzwedel DM, Cogo E, Foerster V, Lefebvre C. PRESS peer review of electronic search strategies: 2015 Guideline Statement. J Clin Epidemiol. 2016;75:40–6.
Hong QN, Gonzalez-Reyes A, Pluye P. Improving the usefulness of a tool for appraising the quality of qualitative, quantitative and mixed methods studies, the mixed methods Appraisal Tool (MMAT). J Eval Clin Pract. 2018;24(3):459–67.
Needleman IG. A guide to systematic reviews. J Clin Periodontol. 2002;29(s3):6–9.
Popay J, Roberts H, Sowden A, Petticrew M, Arai L, Rodgers M, et al. Guidance on the Conduct of Narrative Synthesis in systematic reviews a product from the ESRC Methods Programme. Lancaster University.; 2006.
Thomas J, Harden A. Methods for the thematic synthesis of qualitative research in systematic reviews. BMC Med Res Methodol. 2008;8(1):45.
Glaser B, Strauss A. The Discovery of grounded theory: strategies for qualitative research. Mill Valley, CA: Sociology Press; 1967.
Glaser BG. The constant comparative method of qualitative analysis. Soc Probl. 1965;12(4):436–45.
Felton A, Arnold P, Fairbank S, Shaw T. Using focus groups and photography to evaluate experiences of social inclusion within rehabilitation adult mental health services. Mental Health Review Journal. 2009;14(3):13–22.
Friedrich B, Mason OJ. Qualitative evaluation of a football intervention for people with mental health problems in the north east of London. Ment Health Phys Act. 2018;15:132–8.
Mathias K, Singh P, Butcher N, Grills N, Srinivasan V, Kermode M. Promoting social inclusion for young people affected by psycho-social disability in India – a realist evaluation of a pilot intervention. Glob Public Health. 2019;14(12):1718–32.
Margrove KL, Heydinrych K, Secker J. Waiting list-controlled evaluation of a participatory arts course for people experiencing mental health problems. Perspect Public Health. 2013;133(1):28–35.
Petryshen PM, Hawkins JD, Fronchak TA. An evaluation of the social recreation component of a community mental health program. Psychiatr Rehabil J. 2001;24(3):293–8.
O’Connell MJ, Flanagan EH, Delphin-Rittmon ME, Davidson L. Enhancing outcomes for persons with co-occurring disorders through skills training and peer recovery support. J Mental Health. 2020;29(1):6–11.
Tarbet SF. Self-help and support groups as social network interventions: a comparison of two groups. Dissertation Abstracts International. 1985;46(2–B):631.
Hassan SM, Giebel C, Morasae EK, Rotheram C, Mathieson V, Ward D, et al. Social prescribing for people with mental health needs living in disadvantaged communities: the life rooms model. BMC Health Serv Res. 2020;20(1):19.
Webber M, Ngamaba K, Moran N, Pinfold V, Boehnke JR, Knapp M, et al. The implementation of connecting people in Community Mental Health Teams in England: a quasi-experimental study. Br J Social Work. 2021;51(3):1080–100.
Suto MJ, Smith S, Damiano N, Channe S. Participation in Community Gardening: sowing the seeds of Well-Being: Participation au jardinage communautaire: pour semer les graines du bien-être. Can J Occup Ther. 2021;88(2):142–52.
Calsyn RJ, Morse GA, Klinkenberg WD, Trusty ML, Allen G. The impact of assertive community treatment on the social relationships of people who are homeless and mentally ill. Commun Ment Health J. 1998;34(6):579–93.
Ammerman RT, Putnam FW, Altaye M, Teeters AR, Stevens J, Van Ginkel JB. Treatment of depressed mothers in home visiting: impact on psychological distress and social functioning. Child Abuse Negl. 2013;37(8):544–54.
Castelein S, Bruggeman R, van Busschbach J, van der Gaag M, Stant A, Knegtering H, et al. The effectiveness of peer support groups in psychosis: a randomized controlled trial. Acta psychiatrica Scandinavica. 2008;118(1):64–72.
Johnson S, Lamb D, Marston L, Osborn D, Mason O, Henderson C, et al. Peer-supported self-management for people discharged from a mental health crisis team: a randomised controlled trial. Lancet. 2018;392(10145):409–18.
Rivera JJ, Sullivan AM, Valenti SS. Adding consumer-providers to intensive case management: does it improve outcome? Psychiatric services (washington, DC). 2007;58(6):802–9.
Sheridan AJ, Drennan J, Coughlan B, O’Keeffe D, Frazer K, Kemple M, et al. Improving social functioning and reducing social isolation and loneliness among people with enduring mental illness: report of a randomised controlled trial of supported socialisation. Int J Soc Psychiatry. 2015;61(3):241–50.
Tempier R, Balbuena L, Garety P, Craig TJ. Does assertive community outreach improve social support? Results from the Lambeth Study of early-episode psychosis. Psychiatric Serv (Washington DC). 2012;63(3):216–22.
Terzian E, Tognoni G, Bracco R, De Ruggieri E, Ficociello RA, Mezzina R, et al. Social network intervention in patients with schizophrenia and marked social withdrawal: a randomized controlled study. Can J Psychiatry. 2013;58(11):622–31.
Thorup A, Petersen L, Jeppesen P, Øhlenschlaeger J, Christensen T, Krarup G, et al. Social network among young adults with first-episode schizophrenia spectrum disorders: results from the danish OPUS trial. Soc Psychiatry Psychiatr Epidemiol. 2006;41(10):761–70.
Becker T, Leese M, McCrone P, Clarkson P, Szmukler G, Thornicroft G. Impact of community mental health services on users’ social networks. PRiSM Psychos Study 7 Br J psychiatry. 1998;173:404–8.
CAS Google Scholar
Hacking S, Bates P. The inclusion web: a tool for person-centered planning and service evaluation. Mental Health Review Journal. 2008;13(2):4–15.
Segal SP, Holschuh J, EFFECTS OF SHELTERED CARE ENVIRONMENTS, AND RESIDENT CHARACTERISTICS ON THE DEVELOPMENT OF SOCIAL NETWORKS. Hosp Community Psychiatry. 1991;42(11):1125–31.
CAS PubMed PubMed Central Google Scholar
Mak WW, Chan RC, Pang IH, Chung NY, Yau SS, Tang JP. Effectiveness of Wellness Recovery Action Planning (WRAP) for chinese in Hong Kong. Am J Psychiatric Rehabilitation. 2016;19(3):235–51.
Howarth M, Rogers M, Withnell N, McQuarrie C. Growing spaces: an evaluation of the mental health recovery programme using mixed methods. J Res Nurs. 2018;23(6):476–89.
Chang WC, Kwong VW, Chan GH, Jim OT, Lau ES, Hui CL, et al. Prediction of functional remission in first-episode psychosis: 12-month follow-up of the randomized-controlled trial on extended early intervention in Hong Kong. Schizophr Res. 2016;173(1–2):79–83.
Haslam C, Cruwys T, Haslam S, Dingle G, Chang MX-L. GROUPS 4 HEALTH: evidence that a social-identity intervention that builds and strengthens social group membership improves mental health. J Affect Disord. 2016;194:188–95.
Mazzi F, Baccari F, Mungai F, Ciambellini M, Brescancin L, Starace F. Effectiveness of a social inclusion program in people with non-affective psychosis. BMC Psychiatry. 2018;18.
Kaltman S, de Mendoza AH, Serrano A, Gonzales FA. A Mental Health intervention strategy for Low-Income, trauma-exposed Latina Immigrants in Primary Care: a preliminary study. Am J Orthopsychiatry. 2016;86(3):345–54.
Chowdhary N, Anand A, Dimidjian S, Shinde S, Weobong B, Balaji M, et al. The healthy activity program lay counsellor delivered treatment for severe depression in India: systematic development and randomised evaluation. Br J Psychiatry. 2016;208(4):381–.
Aggar C, Thomas T, Gordon C, Bloomfield J, Baker J. Social Prescribing for individuals living with Mental Illness in an Australian Community setting: a pilot study. Commun Ment Health J. 2021;57(1):189–95.
Haslam C, Cruwys T, Chang MX, Bentley SV, Haslam S, Dingle GA, et al. GROUPS 4 HEALTH reduces loneliness and social anxiety in adults with psychological distress: findings from a randomized controlled trial. J Consult Clin Psychol. 2019;87(9):787–801.
Webber M, Morris D, Howarth S, Fendt-Newlin M, Treacy S, McCrone P. Effect of the connecting people intervention on Social Capital: a pilot study. Res Social Work Pract. 2019;29(5):483–94.
Gater R, Waheed W, Husain N, Tomenson B, Aseem S, Creed F. Social intervention for british pakistani women with depression: randomised controlled trial. Br J Psychiatry. 2010;197(3):227–33.
Garety PA, Craig TK, Dunn G, Fornells-Ambrojo M, Colbert S, Rahaman N, et al. Specialised care for early psychosis: symptoms, social functioning and patient satisfaction: randomised controlled trial. Br J Psychiatry. 2006;188:37–45.
Varga E, Endre S, Bugya T, Tenyi T, Herold R. Community-based psychosocial treatment has an impact on social processing and functional outcome in schizophrenia. Front Psychiatry. 2018;9(JUN).
Bragg R. Nature-based interventions for mental wellbeing and sustainable behaviour: the potential for green care in the. UK: University of Essex (United Kingdom); 2014.
Fitzgerald M. An evaluation of the impact of a social inclusion programme on occupational functioning for forensic service users. Br J Occup Therapy. 2011;74(10):465–72.
Fowler D, Hodgekins J, French P, Marshall M, Freemantle N, McCrone P, et al. Social recovery therapy in combination with early intervention services for enhancement of social recovery in patients with first-episode psychosis (SUPEREDEN3): a single-blind, randomised controlled trial. The lancet Psychiatry. 2018;5(1):41–50.
van de Venter E, Buller A. Arts on referral interventions: a mixed-methods study investigating factors associated with differential changes in mental well-being. J Public Health (Oxf). 2015;37(1):143–50.
Sheridan A, O’Keeffe D, Coughlan B, Frazer K, Drennan J, Kemple M. Friendship and money: a qualitative study of service users’ experiences of participating in a supported socialisation programme. Int J Soc Psychiatry. 2018;64(4):326–34.
Hanly F, Torrens-Witherow B, Warren N, Castle D, Phillipou A, Beveridge J et al. Peer mentoring for individuals with an eating disorder: a qualitative evaluation of a pilot program. J Eat Disorders. 2020;8(1).
Lund K, Argentzell E, Leufstadius C, Tjörnstrand C, Eklund M. Joining, belonging, and re-valuing: a process of meaning-making through group participation in a mental health lifestyle intervention. Scand J Occup Ther. 2019;26(1):55–68.
Bradshaw T, Haddock G. Is befriending by trained volunteers of value to people suffering from long-term mental illness? J Adv Nurs. 1998;27(4):713–20.
Snethen G, McCormick BP, Van Puymbroeck M. Community involvement, planning and coping skills: pilot outcomes of a recreational-therapy intervention for adults with schizophrenia. Disabil Rehabil. 2012;34(18):1575–84.
Bertotti M, Frostick C, Hutt P, Sohanpal R, Carnes D. A realist evaluation of social prescribing: an exploration into the context and mechanisms underpinning a pathway linking primary care with the voluntary sector. Prim Health Care Res Dev (Cambridge Univ Press / UK). 2018;19(3):232–45.
Hanlon P, Gray CM, Chng NR, Mercer SW. Does Self-Determination Theory help explain the impact of social prescribing? A qualitative analysis of patients’ experiences of the Glasgow ‘Deep-End’ Community Links Worker Intervention. Chronic Illness. 2019.
Abotsie G, Kingerlee R, Fisk A, Watts S, Cooke R, Woodley L, et al. The men’s wellbeing project: promoting the well-being and mental health of men. J Public Mental Health. 2020;19(2):179–89.
Fieldhouse J. The impact of an Allotment Group on Mental Health clients’ health, wellbeing and social networking. Br J Occup Therapy. 2003;66(7):286–96.
Darongkamas J, Scott H, Taylor E. Kick-starting men’s mental health: an evaluation of the effect of playing football on mental health service users’ well-being. Int J Mental Health Promotion. 2011;13(3):14–21.
Sexton D. Kirton companions, the clients assess: evaluating a community Mental Health Day facility. Br J Occup Therapy. 1992;55(11):414–8.
O’Brien L, Burls A, Townsend M, Ebden M. Volunteering in nature as a way of enabling people to reintegrate into society. Perspect Public Health. 2011;131(2):71–81.
Newlin M, Webber M, Morris D, Howarth S. Social participation interventions for adults with Mental Health problems: a review and narrative synthesis. Social Work Research. 2015;39(3):167–80.
Anderson K, Laxhman N, Priebe S. Can mental health interventions change social networks? A systematic review. BMC Psychiatry. 2015;15.
James E, Kennedy A, Vassilev I, Ellis J, Rogers A. Mediating engagement in a social network intervention for people living with a long-term condition: a qualitative study of the role of facilitation. Health Expect. 2020;23(3):681–90.
Brooks HL, Rogers A, Sanders C, Pilgrim D. Perceptions of recovery and prognosis from long-term conditions: the relevance of hope and imagined futures. Chronic Illn. 2014.
May CR, Johnson M, Finch T. Implementation, context and complexity. Implement Sci. 2016;11(1):141.
UK Research and Innovation (UKRI). Equality, diversity and inclusion in research and innovation: UK review. London: Advance HE; 2019.
Brooks H, Lovell K, Bee P, Fraser C, Molloy C, Rogers A. Implementing an intervention designed to enhance service user involvement in mental health care planning: a qualitative process evaluation. Soc Psychiatry Psychiatr Epidemiol. 2019;54(2):221–33.
Brooks H, Pilgrim D, Rogers A. Innovation in mental health services: what are the key components of success? Implement Sci. 2011;6:120.
Goering S. Rethinking disability: the social model of disability and chronic disease. Curr Rev Musculoskelet Med. 2015;8(2):134–8.
Download references
Acknowledgements
The authors would like to thank Alice Newton-Braithwaite for her helpful comments on the final version of the manuscript.
Funding for this project is through the Research for Patient Benefit (RfPB) Programme (Grant Reference Number PB-PG-0418-20011) via the National Institute for Health Research (NIHR). Views expressed within this article are those of the author(s) and not necessarily those of the Department of Health and Social Care or the NIHR.
Author information
James Downs and Bethan Mair Edwards are equal contributors.
Authors and Affiliations
Mental Health Research Group, Division of Nursing, Midwifery and Social Work, School of Health Sciences, Faculty of Biology, Medicine and Health, University of Manchester, Manchester Academic Health Science Centre, Manchester, M13 9PL, UK
Helen Brooks, Angela Devereux-Fitzgerald, Laura Richmond, Penny Bee & Karina Lovell
Department of Clinical, Education & Health Psychology, University College London, London, UK
Laura Richmond
Patient and Public Involvement Contributor, University of Manchester, Manchester, UK
Department of Primary Care and Mental Health, Institute of Population Health, University of Liverpool, Liverpool, UK
Mary Gemma Cherry
Linda McCartney Centre, Liverpool University Hospitals NHS Trust, Prescot St, Liverpool, UK
Greater Manchester Mental Health NHS Foundation Trust, Manchester, UK
Karina Lovell
Patient and Public Involvement Contributor, Cambridge, UK
James Downs
School of Health Sciences, University of Manchester, Manchester, UK
Bethan Mair Edwards
NIHR CLAHRC Wessex, Faculty of Health Sciences, University of Southampton, Southampton, UK
Ivaylo Vassilev & Anne Rogers
Public Contributor, Manchester, UK
You can also search for this author in PubMed Google Scholar
Contributions
HB, MGC, PB, KL, II and AR obtaining funding for the study, formulated the review questions and designed the review. HB, PB, ADF KL, LR, NC, JD, BME, LB undertook title and abstract and full-text screening, data extraction and quality appraisal. HB, ADF and MGC undertook the narrative analysis. HB led the writing of the manuscript with input from AR and MGC. All authors provided input into draft manuscripts and approved the final version for submission.
Corresponding author
Correspondence to Helen Brooks .
Ethics declarations
Ethics approval and consent to participate.
Not applicable.
Consent for publication
Competing interests.
The authors declare no competing interests.
Additional information
Publisher’s note.
Springer Nature remains neutral with regard to jurisdictional claims in published maps and institutional affiliations.
Electronic supplementary material
Below is the link to the electronic supplementary material.
Supplementary Material 1
Supplementary material 2, supplementary material 3, rights and permissions.
Open Access This article is licensed under a Creative Commons Attribution 4.0 International License, which permits use, sharing, adaptation, distribution and reproduction in any medium or format, as long as you give appropriate credit to the original author(s) and the source, provide a link to the Creative Commons licence, and indicate if changes were made. The images or other third party material in this article are included in the article’s Creative Commons licence, unless indicated otherwise in a credit line to the material. If material is not included in the article’s Creative Commons licence and your intended use is not permitted by statutory regulation or exceeds the permitted use, you will need to obtain permission directly from the copyright holder. To view a copy of this licence, visit http://creativecommons.org/licenses/by/4.0/ . The Creative Commons Public Domain Dedication waiver ( http://creativecommons.org/publicdomain/zero/1.0/ ) applies to the data made available in this article, unless otherwise stated in a credit line to the data.
Reprints and permissions
About this article
Cite this article.
Brooks, H., Devereux-Fitzgerald, A., Richmond, L. et al. Exploring the use of social network interventions for adults with mental health difficulties: a systematic review and narrative synthesis. BMC Psychiatry 23 , 486 (2023). https://doi.org/10.1186/s12888-023-04881-y
Download citation
Received : 31 August 2022
Accepted : 17 May 2023
Published : 07 July 2023
DOI : https://doi.org/10.1186/s12888-023-04881-y
Share this article
Anyone you share the following link with will be able to read this content:
Sorry, a shareable link is not currently available for this article.
Provided by the Springer Nature SharedIt content-sharing initiative
- Social networks
- Mental health
- Implementation
- Systematic review
BMC Psychiatry
ISSN: 1471-244X
- Submission enquiries: [email protected]
- General enquiries: [email protected]
The impact of COVID-19 on young people's mental health, wellbeing and routine from a European perspective: A co-produced qualitative systematic review
Affiliations.
- 1 NIHR Patient Safety Translational Research Centre, Institute of Global Health Innovation, Imperial College London, London, United Kingdom.
- 2 School of Public Health, Imperial College London, London, United Kingdom.
- 3 Centre for Health Policy, Institute of Global Health Innovation, Imperial College London, London, United Kingdom.
- 4 Liggins Institute, University of Auckland Waipapa Taumata Rau, Auckland, New Zealand.
- 5 Manchester Institute of Education, The University of Manchester, Manchester, United Kingdom.
- 6 School of Psychology, Liverpool John Moores University, Liverpool, United Kingdom.
- 7 Environmental Health Institute, Medicine Faculty, University of Lisbon, Lisbon, Portugal.
- 8 Newcastle Population Health Sciences Institute, Faculty of Medical Sciences, University of Newcastle, Newcastle upon Tyne, United Kingdom.
- PMID: 38507395
- PMCID: PMC10954119
- DOI: 10.1371/journal.pone.0299547
Background: The impact of the Covid-19 pandemic on young people's (YP) mental health has been mixed. Systematic reviews to date have focused predominantly on quantitative studies and lacked involvement from YP with lived experience of mental health difficulties. Therefore, our primary aim was to conduct a qualitative systematic review to examine the perceived impact of the Covid-19 pandemic on YP's (aged 10-24) mental health and wellbeing across Europe.
Methods and findings: We searched MEDLINE, PsycINFO, Embase, Web of Science, MEDRXIV, OSF preprints, Google, and voluntary sector websites for studies published from 1st January 2020 to 15th November 2022. European studies were included if they reported qualitative data that could be extracted on YP's (aged 10-24) own perspectives of their experiences of Covid-19 and related disruptions to their mental health and wellbeing. Screening, data extraction and appraisal was conducted independently in duplicate by researchers and YP with lived experience of mental health difficulties (co-researchers). Confidence was assessed using the Confidence in the Evidence from Reviews of Qualitative Research (CERQual) approach. We co-produced an adapted narrative thematic synthesis with co-researchers. This study is registered with PROSPERO, CRD42021251578. We found 82 publications and included 77 unique studies in our narrative synthesis. Most studies were from the UK (n = 50; 65%); and generated data during the first Covid-19 wave (March-May 2020; n = 33; 43%). Across the 79,491 participants, views, and experiences of YP minoritised by ethnicity and sexual orientation, and from marginalised or vulnerable YP were limited. Five synthesised themes were identified: negative impact of pandemic information and restrictions on wellbeing; education and learning on wellbeing; social connection to prevent loneliness and disconnection; emotional, lifestyle and behavioural changes; and mental health support. YP's mental health and wellbeing across Europe were reported to have fluctuated during the pandemic. Challenges were similar but coping strategies to manage the impact of these challenges on mental health varied across person, study, and country. Short-term impacts were related to the consequences of changing restrictions on social connection, day-to-day lifestyle, and education set-up. However, YP identified potential issues in these areas going forward, and therefore stressed the importance of ongoing long-term support in education, learning and mental health post-Covid-19.
Conclusions: Our findings map onto the complex picture seen from quantitative systematic reviews regarding the impact of Covid-19 on YP's mental health. The comparatively little qualitative data found in our review means there is an urgent need for more high-quality qualitative research outside of the UK and/or about the experiences of minoritised groups to ensure all voices are heard and everyone is getting the support they need following the pandemic. YP's voices need to be prioritised in decision-making processes on education, self-care strategies, and mental health and wellbeing, to drive impactful, meaningful policy changes in anticipation of a future systemic crisis.
Copyright: © 2024 Dewa et al. This is an open access article distributed under the terms of the Creative Commons Attribution License, which permits unrestricted use, distribution, and reproduction in any medium, provided the original author and source are credited.
Publication types
- COVID-19* / epidemiology
- Mental Health*
- Qualitative Research
Grants and funding

The Physical Health Trajectories of Young People with Neurodevelopmental Conditions: A Protocol for a Systematic Review of Longitudinal Studies.
- Find this author on Google Scholar
- Find this author on PubMed
- Search for this author on this site
- ORCID record for Naomi Wilson
- For correspondence: [email protected]
- Info/History
- Preview PDF
Introduction It is now widely acknowledged that without appropriate support, young people with neurodevelopmental conditions (NDCs) are at an increased risk of many of the social and psychiatric outcomes which are known to be key drivers of physical health inequalities. Despite this, until recently relatively little attention has been paid to their physical health trajectories. There is now emerging longitudinal evidence to suggest an association between specific NDCs in childhood or adolescence and certain physical long-term conditions (LTCs) in adulthood. However, to date this literature has never been comprehensively appraised. As a result, our understanding of all the future health risks that young people with NDCs may collectively be at risk of is limited and the factors which drive these adult health outcomes also remain obscure. Methods A search strategy has been developed in collaboration with two medical librarians and will be used to conduct systematic searches of Medline, Embase, APA PsycINFO, Cumulative Index to Nursing and Allied Health Literature, and Web Of Science. Prospective longitudinal studies exploring the association between three common NDCs in childhood or adolescence (i.e., ADHD, Autism, and Tic Disorders <18 years of age) and any physical LTC in adulthood (i.e., > 18 years of age) will be selected through title and abstract review, followed by a full-text review. Data extracted will include definition of exposure and outcome, mediators or moderators investigated, confounders adjusted for, and crude and adjusted effect estimates. Risk of bias assessment will be conducted. Results will be synthesized narratively and if the data allow, a meta-analysis will also be conducted. Ethics and dissemination Ethics approval is not applicable for this study since no original data will be collected. The results of the review will be widely disseminated locally, nationally, and internationally through peer-reviewed publication, adhering to the PRISMA statement, and conference presentations.
Competing Interest Statement
The authors have declared no competing interest.
Clinical Protocols
https://www.crd.york.ac.uk/prospero/display_record.php?ID=CRD42024516684
Funding Statement
This work was supported by the Wellcome Trust [223499/Z/21/Z].
Author Declarations
I confirm all relevant ethical guidelines have been followed, and any necessary IRB and/or ethics committee approvals have been obtained.
I confirm that all necessary patient/participant consent has been obtained and the appropriate institutional forms have been archived, and that any patient/participant/sample identifiers included were not known to anyone (e.g., hospital staff, patients or participants themselves) outside the research group so cannot be used to identify individuals.
I understand that all clinical trials and any other prospective interventional studies must be registered with an ICMJE-approved registry, such as ClinicalTrials.gov. I confirm that any such study reported in the manuscript has been registered and the trial registration ID is provided (note: if posting a prospective study registered retrospectively, please provide a statement in the trial ID field explaining why the study was not registered in advance).
I have followed all appropriate research reporting guidelines, such as any relevant EQUATOR Network research reporting checklist(s) and other pertinent material, if applicable.
Data Availability
No data was collected in the completion of this work.
View the discussion thread.
Thank you for your interest in spreading the word about medRxiv.
NOTE: Your email address is requested solely to identify you as the sender of this article.

Citation Manager Formats
- EndNote (tagged)
- EndNote 8 (xml)
- RefWorks Tagged
- Ref Manager
- Tweet Widget
- Facebook Like
- Google Plus One
Subject Area
- Psychiatry and Clinical Psychology
- Addiction Medicine (313)
- Allergy and Immunology (615)
- Anesthesia (157)
- Cardiovascular Medicine (2238)
- Dentistry and Oral Medicine (275)
- Dermatology (199)
- Emergency Medicine (367)
- Endocrinology (including Diabetes Mellitus and Metabolic Disease) (792)
- Epidemiology (11516)
- Forensic Medicine (10)
- Gastroenterology (674)
- Genetic and Genomic Medicine (3524)
- Geriatric Medicine (336)
- Health Economics (610)
- Health Informatics (2270)
- Health Policy (907)
- Health Systems and Quality Improvement (858)
- Hematology (332)
- HIV/AIDS (739)
- Infectious Diseases (except HIV/AIDS) (13103)
- Intensive Care and Critical Care Medicine (749)
- Medical Education (355)
- Medical Ethics (99)
- Nephrology (383)
- Neurology (3304)
- Nursing (189)
- Nutrition (502)
- Obstetrics and Gynecology (643)
- Occupational and Environmental Health (643)
- Oncology (1738)
- Ophthalmology (517)
- Orthopedics (206)
- Otolaryngology (283)
- Pain Medicine (220)
- Palliative Medicine (65)
- Pathology (433)
- Pediatrics (995)
- Pharmacology and Therapeutics (417)
- Primary Care Research (394)
- Psychiatry and Clinical Psychology (3029)
- Public and Global Health (5950)
- Radiology and Imaging (1211)
- Rehabilitation Medicine and Physical Therapy (710)
- Respiratory Medicine (803)
- Rheumatology (363)
- Sexual and Reproductive Health (343)
- Sports Medicine (307)
- Surgery (381)
- Toxicology (50)
- Transplantation (169)
- Urology (141)

- Ask a Librarian
Artificial Intelligence (AI)
Ai for systematic review.
- How to Cite AI Generated Content
- Prompt Design
- Resources for Educators
- Purdue AI Resources
- AI and Ethics
- Publisher Policies
- Selected Journals in AI
Various AI tools are invaluable throughout the systematic review or evidence synthesis process. While the consensus acknowledges the significant utility of AI tools across different review stages, it's imperative to grasp their inherent biases and weaknesses. Moreover, ethical considerations such as copyright and intellectual property must be at the forefront.
- Application ChatGPT in conducting systematic reviews and meta-analyses
- Are ChatGPT and large language models “the answer” to bringing us closer to systematic review automation?
- Artificial intelligence in systematic reviews: promising when appropriately used
- Harnessing the power of ChatGPT for automating systematic review process: methodology, case study, limitations, and future directions
- In-depth evaluation of machine learning methods for semi-automating article screening in a systematic review of mechanistic
- Tools to support the automation of systematic reviews: a scoping review
- The use of a large language model to create plain language summaries of evidence reviews in healthcare: A feasibility study
- Using artificial intelligence methods for systematic review in health sciences: A systematic review
AI Tools for Systematic Review
- DistillerSR Securely automate every stage of your literature review to produce evidence-based research faster, more accurately, and more transparently at scale.
- Rayyan A web-tool designed to help researchers working on systematic reviews, scoping reviews and other knowledge synthesis projects, by dramatically speeding up the process of screening and selecting studies.
- RobotReviewer A machine learning system aiming which aims to automate evidence synthesis.
- << Previous: AI Tools
- Next: How to Cite AI Generated Content >>
- Last Edited: Mar 21, 2024 1:34 PM
- URL: https://guides.lib.purdue.edu/ai
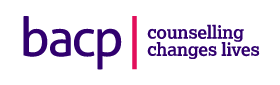
500 - Server error - Sorry, there is a problem.
Please try again later or contact us at [email protected] with details of what you were trying to do,
Return to the homepage
SYSTEMATIC REVIEW article
Promoting mental health in children and adolescents through digital technology: a systematic review and meta-analysis.
- Faculty of Artificial Intelligence in Education, Central China Normal University, Wuhan, China
Background: The increasing prevalence of mental health issues among children and adolescents has prompted a growing number of researchers and practitioners to explore digital technology interventions, which offer convenience, diversity, and proven effectiveness in addressing such problems. However, the existing literature reveals a significant gap in comprehensive reviews that consolidate findings and discuss the potential of digital technologies in enhancing mental health.
Methods: To clarify the latest research progress on digital technology to promote mental health in the past decade (2013–2023), we conducted two studies: a systematic review and meta-analysis. The systematic review is based on 59 empirical studies identified from three screening phases, with basic information, types of technologies, types of mental health issues as key points of analysis for synthesis and comparison. The meta-analysis is conducted with 10 qualified experimental studies to determine the overall effect size of digital technology interventions and possible moderating factors.
Results: The results revealed that (1) there is an upward trend in relevant research, comprising mostly experimental and quasi-experimental designs; (2) the common mental health issues include depression, anxiety, bullying, lack of social emotional competence, and mental issues related to COVID-19; (3) among the various technological interventions, mobile applications (apps) have been used most frequently in the diagnosis and treatment of mental issues, followed by virtual reality, serious games, and telemedicine services; and (4) the meta-analysis results indicated that digital technology interventions have a moderate and significant effect size ( g = 0.43) for promoting mental health.
Conclusion: Based on these findings, this study provides guidance for future practice and research on the promotion of adolescent mental health through digital technology.
Systematic review registration: https://inplasy.com/inplasy-2023-12-0004/ , doi: 10.37766/inplasy2023.12.0004 .
1 Introduction
In recent years, the mental health status of children and adolescents (6–18 years old) has been a matter of wide societal concern. The World Health Organization noted that one in seven adolescents suffers from mental issues, accounting for 13% of the global burden of disease in this age group ( World Health Organization, 2021 ). In particular, the emergence of COVID-19 has led to an increase in depression, anxiety, and other psychological symptoms ( Jones et al., 2021 ; Shah et al., 2021 ). There is thus an urgent need to monitor and diagnose the mental health of teenagers.
The development of digital technology has brought about profound socio-economic changes; it also provides new opportunities for mental health diagnosis and intervention ( Goodyear and Armour, 2018 ; Giovanelli et al., 2020 ). First, digital technology breaks the constraints of time and space. It not only provides adolescents with mental health services at a distance but also enables real-time behavioral monitoring for the timely acquisition of dynamic data on adolescents’ mental health ( Naslund et al., 2017 ). Second, due to the still-developing stage of mental health resource building, traditional intervention methods may not be able to meet the increasing demand for mental health services among children and adolescents ( Villarreal, 2018 ; Aschbrenner et al., 2019 ). In addition, as digital natives in the information age, adolescents have the ability to use digital technology proficiently, and social media, such as the internet, has long been integrated into all aspects of adolescents’ lives ( Uhlhaas and Torous, 2019 ). However, it is worth noting that excessive reliance on digital technology (e.g., internet and smartphone addiction) are also common triggers of mental problems among youth ( Wacks and Weinstein, 2021 ). Therefore, we must be aware of the risks posed by digital technology to better utilize it for promoting the mental health of young people.
Mental health, sometimes referred to as psychological health in the literature, encompasses three different perspectives: pathological orientation, positive orientation, and complete orientation ( Keyes, 2009 ). Pathological orientation refers to whether patients exhibit symptoms of mental issues, including internalized mental disorders (e.g., depression and anxiety) and behavioral dysfunctions (e.g., aggression, self-harm) as well as other mental illnesses. Studies have indicated that both internalizing and externalizing disorders belong to different dimensions of mental disorders ( Scott et al., 2020 ), and internalizing symptoms often occur simultaneously with externalizing behaviors ( Essau and de la Torre-Luque, 2023 ). The positive orientation suggests that mental health is a positive mental state, characterized by a person’s ability to fully participate in various activities and to express positive and negative emotions ( Kenny et al., 2016 ). The complete orientation integrates pathological and positive orientation ( Antaramian et al., 2010 ), suggesting that mental health means the absence of mental issues and the presence of subjective well-being ( Suldo and Shaffer, 2008 ). The development of social emotional abilities helps to promote subjective well-being for adolescents during social, emotional, and cognitive development ( Cejudo et al., 2019 ). Adolescents with mental health issues may thus exhibit pathological symptoms or lack of subjective well-being due to a lack of social emotional abilities. In this study, mental health is defined as a psychological state advocated by the complete orientation.
Promoting mental health using digital technology involves providing help through digital tools such as computers, tablets, or phones with internet-based programs ( Hollis et al., 2017 ). Currently, various digital technologies have been tested to address mental health issues in young individuals, including apps, video games, telemedicine, chatbots, and virtual reality (VR). However, the impact of digital technology interventions is affected by various factors ( Piers et al., 2023 ). Efficacy varies based on the kind of mental health issues. Individuals with mental illness related to COVID-19 may profit more from digital interventions than those experiencing depression and anxiety. Moreover, studies reveal that several mental health conditions in young people deteriorate with age, particularly anxiety and suicide attempts ( Tang et al., 2019 ). The impact of digital technology interventions may therefore differ depending on the adolescent’s age. Having psychological problems usually indicates that people are in an unhealthy mental state for a long time, so an enduring intervention may have greater efficacy than a short-term one. Earlier studies have also suggested that the outcomes of treatment are linked to its duration, with patients receiving long-term treatment experiencing better results ( Grist et al., 2019 ).
Although more digital technologies are being used to treat mental health issues, the most important clinical findings have come from strict randomized controlled trials ( Mohr et al., 2018 ). It is still unclear how these interventions affect long-term care or how they would function in real-world settings ( Folker et al., 2018 ). There is much relevant empirical research, but it is scattered, and there is a need for systematic reviews in this area. In previous studies about technology for mental health, Grist et al. (2019) analyzed how digital interventions affect teenagers with depression and anxiety, but their study only considered mental disorders, without considering other mental health issues. Cheng et al. (2019) examined serious games and their application of gamification elements to enhance mental health; however, they overlooked various technological approaches beyond serious games and did not give adequate consideration to the diverse types and features of technology. Eisenstadt et al. (2021) reviewed how mobile apps can help adults between 18 and 45 years of age improve their emotional regulation, mental health, and overall well-being; however, they did not investigate the potential benefits of apps for teenagers.
The present study reviews research from the past decade on digital technology for promoting adolescent mental health. A systematic literature review and meta-analysis are used to explore which types and features of technology can enhance mental health. We believe that the present study makes a meaningful contribution to scholarship because it is among the earliest to report on the impact of technology-enhanced mental health interventions and has revealed crucial influencing factors that merit careful consideration during both research and practical implementation. The following three research questions guided our systematic review and meta-analysis:
1. What is the current status of global research on digital technology for promoting children and adolescent mental health?
2. What digital technology characteristics support the development of mental health among children and adolescents?
3. How effective is digital technology in promoting the mental health of children and adolescents? What factors have an impact on the effectiveness of digital technology interventions?
2 Study 1: systematic literature review
2.1.1 study design.
This study used the systematic literature review method to analyze the relevant literature on the promotion of mental health through digital technology. It followed the Preferred Reporting Items for Systematic Reviews and Meta-Analysis statement for the selection and use of research methods. The protocol for this study was registered with INPLASY (2023120004). Standardized systematic review protocol is used to strictly identify, screen, analyze, and integrate literature ( Bearman et al., 2012 ). To clarify the research issues, systematic literature reviews typically comprise the following six key procedures: planning, literature search, literature assessment, data extraction, data synthesis, and review composition ( Lacey and Matheson, 2011 ).
2.1.2 Literature search
To access high-quality empirical research literature from the past decade, this study selected SCIE and SSCI index datasets from the Web of Science core database and Springer Link. Abstracts containing the English search terms “mental health or psychological health or psychological wellbeing” AND “technology or technological or technologies or digital media” AND “K-12 or teenager or children or adolescents or youth” were retrieved. The search period spanned from January 1, 2013, to July 1, 2023, and 1,032 studies were obtained. To ensure the relevance of the studies to the research question, the relevant inclusion and exclusion criteria were developed based on the 1,032 studies retrieved. The specific criteria are listed in Table 1 .
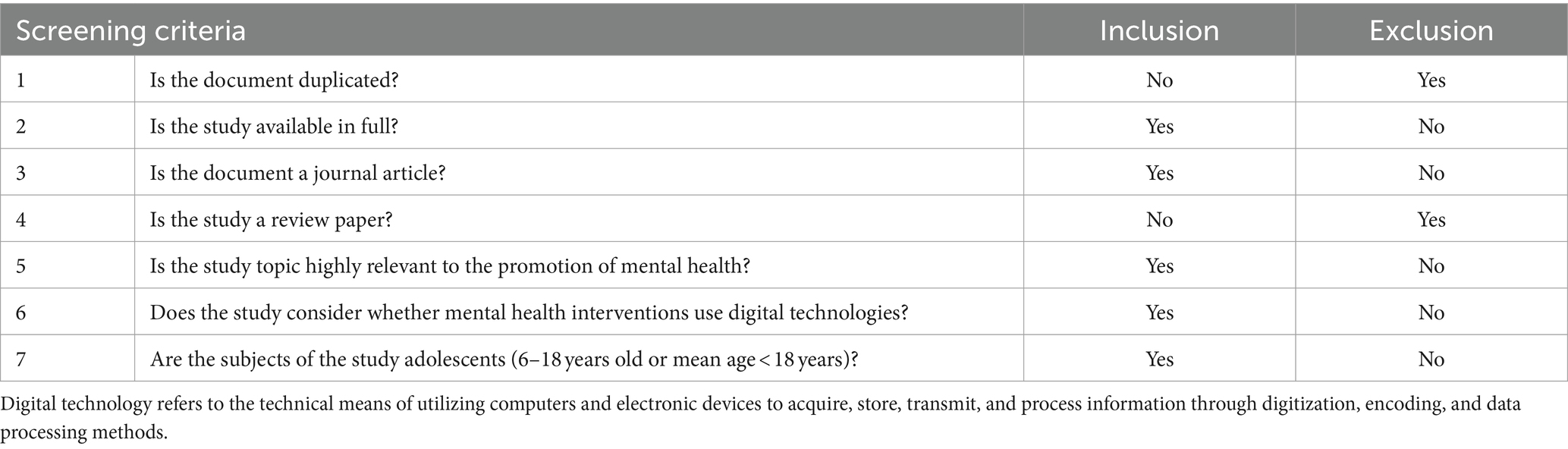
Table 1 . Literature screening criteria.
In this study, we followed a systematic literature review approach and screened the retrieved studies based on the above selection criteria. We conducted three rounds of screening and supplemented new studies through snowballing, ultimately including 59 effective sample documents. The specific process is shown in Figure 1 .
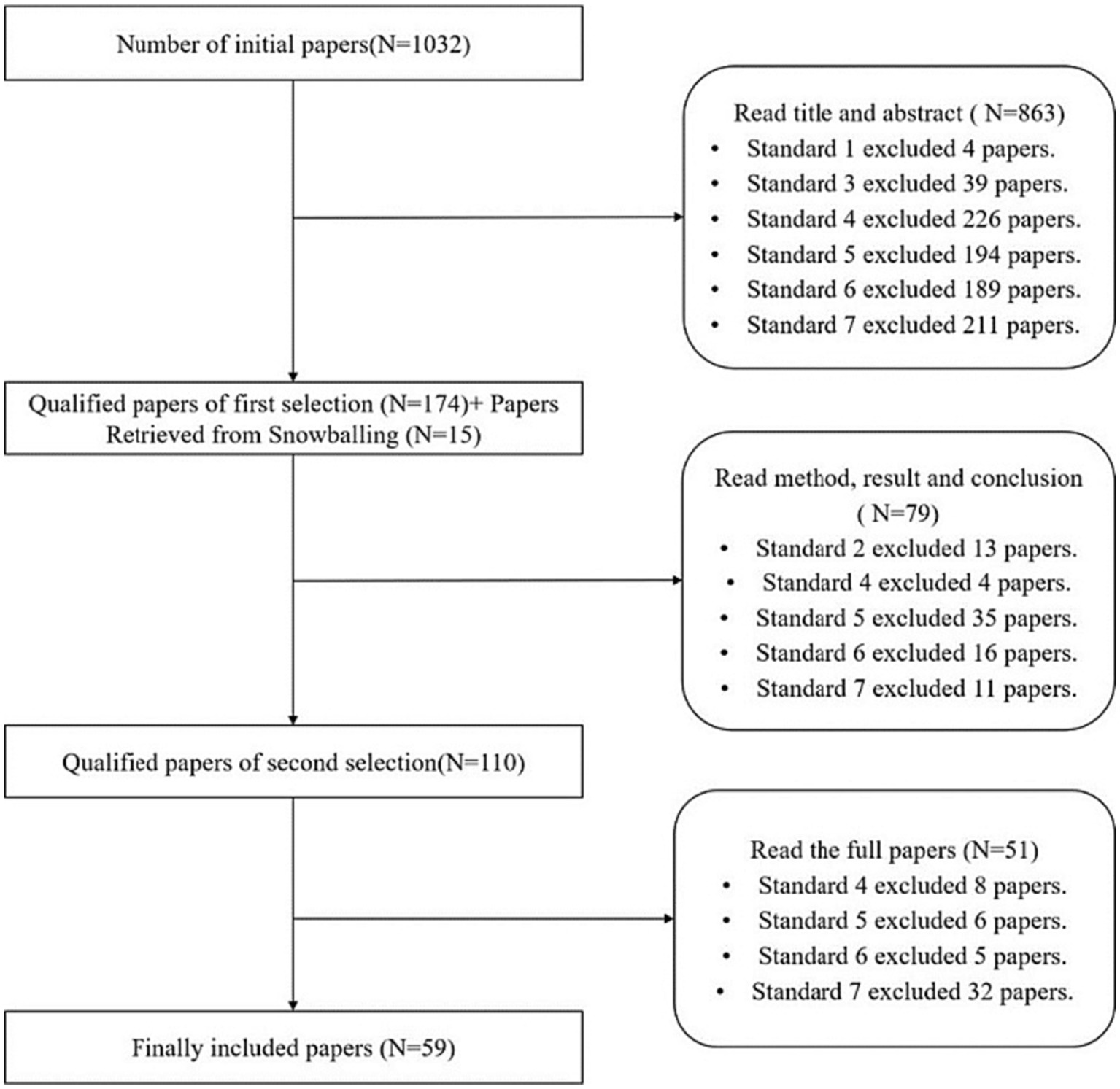
Figure 1 . Screening process and results.
2.1.3 Coding protocol
To extract key information from the included papers, we systematically analyzed 59 studies on the basis of reading the full text. Our coding protocol encompassed the following aspects: (a) basic information about the study, including the first author, publication year, publication region, study type, study object, and intervention duration; (b) the type of technology used in the study, including apps, chatbots, serious games, VR/AR, short messaging service (SMS), telemedicine services, and others; (c) mental health issues, including depression and anxiety, mental illness, bullying, lack of social and emotional competence, mental health issues caused by COVID-19, and other mental health issues; and (d) experimental data (mean, sample size, standard deviation or p -value, t -value, etc.). By capturing basic study information, we establish a foundation for comparing and contextualizing the selected studies. The type of technology used is crucial as it reflects the innovative approaches and their technical affordances. Mental health issues are the core focus that dictates the objectives of the technological interventions as well as their suitability and relevance. Experimental data provides quantifiable evidence to support the effectiveness claims and lays a foundation for the meta-analysis. Together, these four coding aspects offer a holistic view for a comprehensive understanding and analysis of the existing literature. The document coding was completed jointly by the researchers after confirming the coding rules and details through multiple rounds of negotiation. Problems arising in the coding process were intensively discussed to ensure consistency and accuracy of the coding.
2.2 Results and discussion
2.2.1 study and sample characteristics.
As shown in Figure 2 , in terms of the time of publication, the number of studies has gradually increased from 2013 to 2021 along with the development of digital technology. The proportion of studies published in the past 5 years (2019–2023) accounted for 76.3% of the total (45/59), with a peak in 2021 with 15 papers. Social isolation, school suspension, and reduced extracurricular activities caused by COVID-19 may exacerbate mental health issues among children and adolescents, which has attracted more researchers to explore the application of digital technology to mental health treatment.
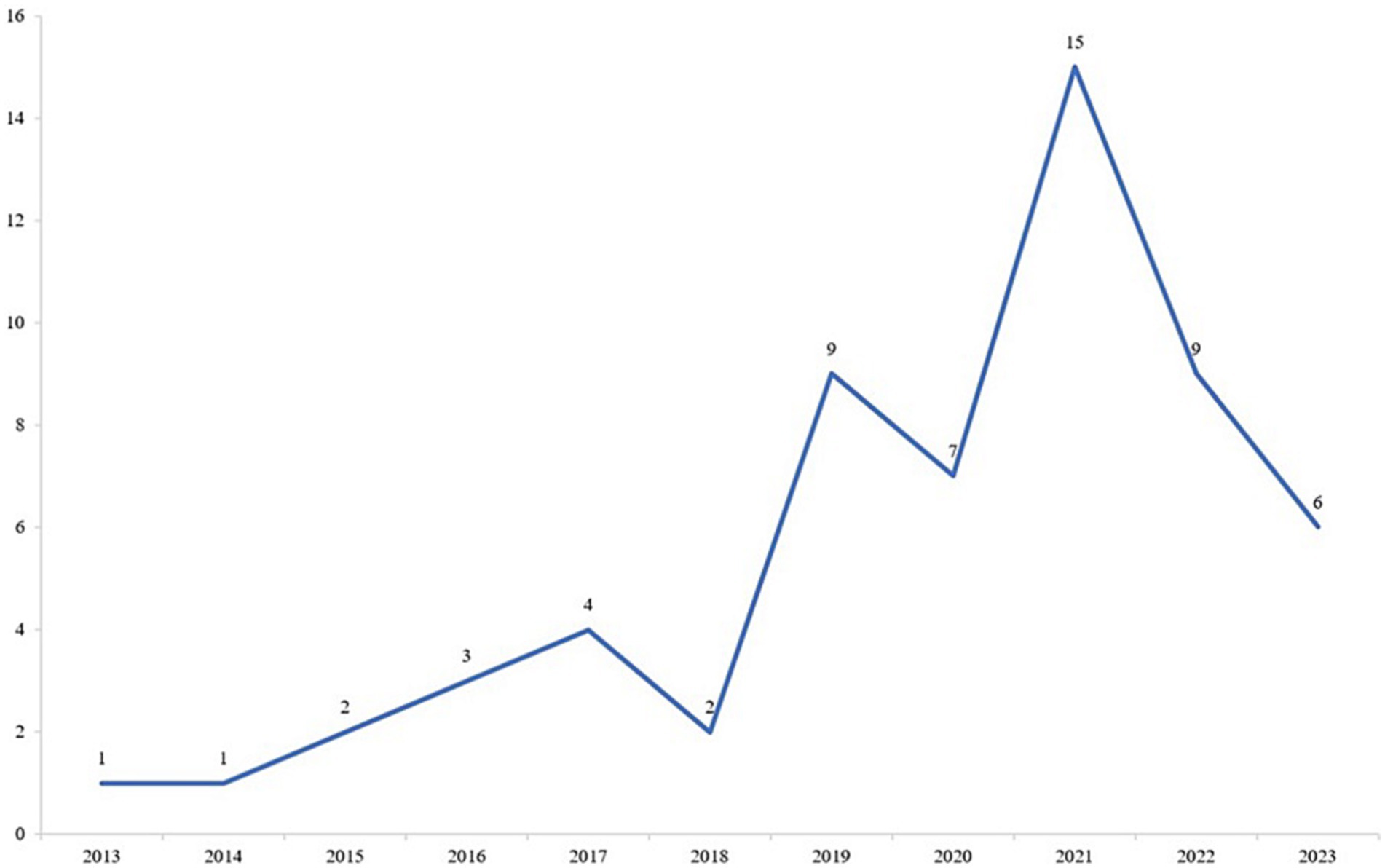
Figure 2 . Trend in the number of studies published in the past decade.
From the perspective of published journals, all of the studies were published in 41 kinds of journals, but two fields were clear leaders: 46 studies (77.97%) were published in medical journals, followed by psychological journals (13.56%). Table 2 shows the source distribution and types of the sample studies. Looking at the country of the first author, the largest number of articles came from the Americas, including the United States and Canada, accounting for 40.7%, followed by European countries, including the United Kingdom and Finland. Only one article came from the African region. In terms of the research types, experimental research was the main type, followed by mixed research, and the number of investigation- and design-based research was relatively small.
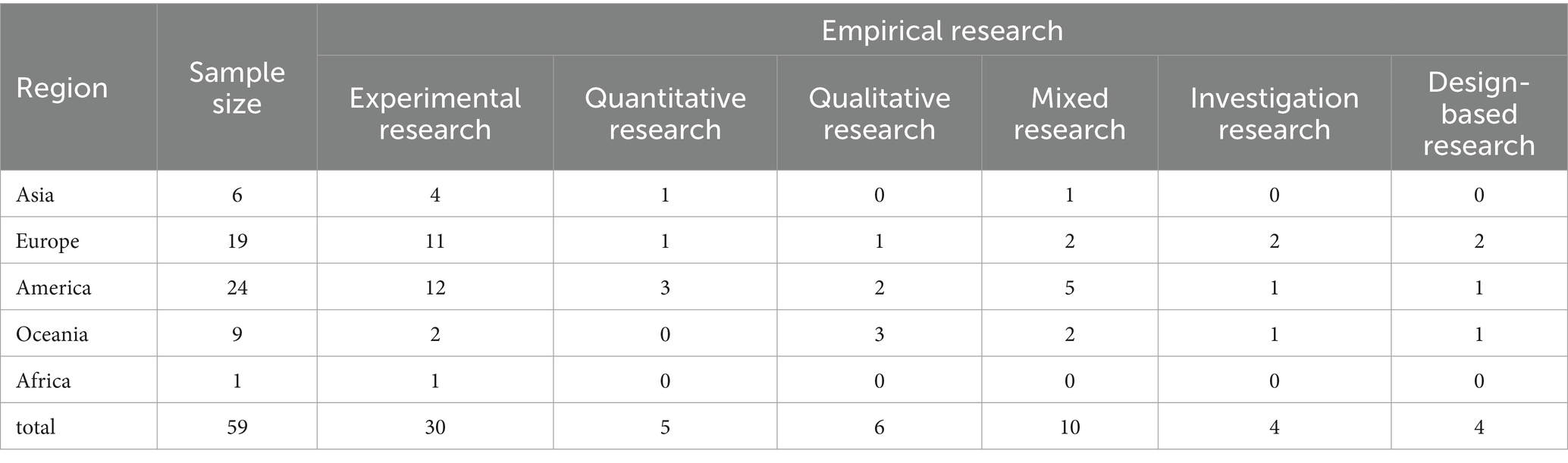
Table 2 . Coding results for sample studies.
Looking more specifically at the research objects, the age range varied from 6 to 18 years. Overall, adolescents aged 13–18 years received more attention, while only six articles considered the younger age group aged 6–12 years. In addition, by coding the sample size of the studies, we found that the quality and size of the studies varied, ranging from small pilot studies or case studies to large-scale cluster studies. For example, Orlowski et al. (2016) conducted a qualitative study on adolescents with experience of seeking help in mental health care institutions in rural Australia; in their study, 10 adolescents with an average age of 18 years were recruited for semi-structured interviews to determine their attitudes and views on the use of technology as a mental health care tool. Another large-scale, randomized controlled trial is planned to enroll 10,000 eighth graders to investigate whether cognitive behavioral therapy (CBT) provided by a smartphone app can prevent depression ( Werner-Seidler et al., 2020 ).
2.2.2 Mental health issues and technology interventions
Based on the coding results, we present the total number of studies that correspond to both mental health issues and technological interventions in Figure 3 . Our findings indicate that apps represent the most prevalent form of digital technology, particularly in addressing depression and anxiety. Telemedicine services also rank highly in terms of utilization. Contrarily, there are comparatively fewer apps involving virtual reality (VR), augmented reality (AR), chatbots, and serious games. Below, we delve into the specifics of digital technology application and its unique affordances, tailored to distinct mental health issues.
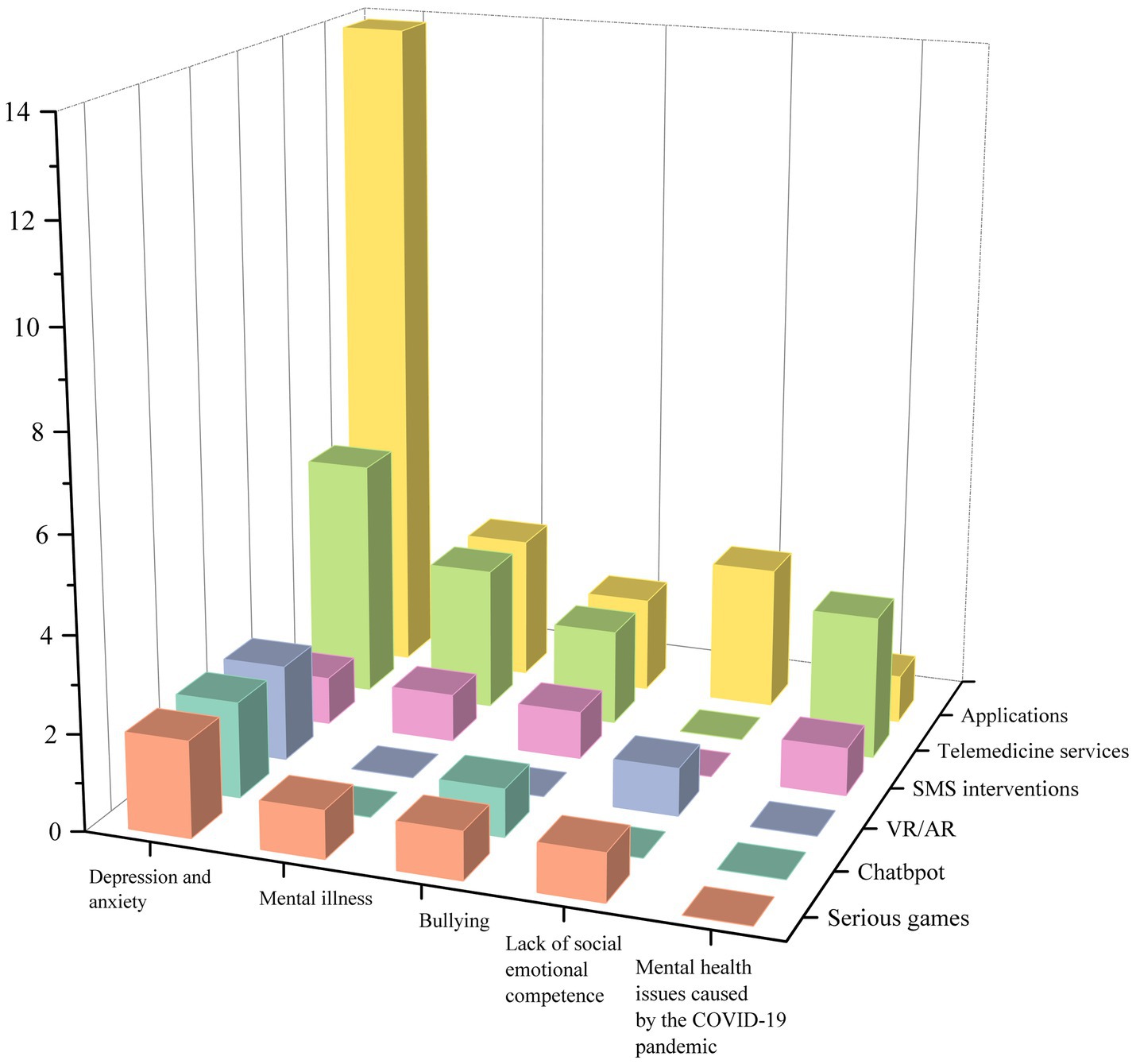
Figure 3 . Numbers of studies by mental health issues and technology interventions.
2.2.2.1 Depression and anxiety
Depression and anxiety in adolescents have become increasingly common, and their presence may signal the beginning of long-term mental health issues, with approximately one in five people experiencing a depressive episode before the age of 18 years ( Lewinsohn et al., 1993 ). This has a range of adverse consequences, including social dysfunction, substance abuse, and suicidal tendency. From the 59 articles considered here, 29 studies used digital technology to treat depression- and anxiety-related symptoms in adolescents. Among the many types of digital technology considered, 19 studies used apps or educational websites as intervention tools, accounting for 76%, followed by serious games, chatbots, and VR with two articles each.
Apps are a broad concept, but they typically refer to software that can be downloaded from app stores to mobile devices such as phones or tablets. Due to characteristics such as their clear structure, ease of use, accessibility, strong privacy, interactivity, and multi-modularity, apps and educational websites are commonly used as tools for technological interventions. For example, Gladstone et al. (2015) developed an interactive website called CATCH-IT to prevent depression in adolescents; the site includes 14 optional modules. The course design of each module applies educational design theories, such as attracting learners’ attention, reviewing content, enhancing memory, and maintaining transfer. Apps and websites can also combine CBT with digital technology. The theoretical framework of CBT is rooted in a core assumption that depression is caused and maintained by unhelpful cognitions and behaviors. Treatment thus focuses on improving the function of these areas by applying skill-based behavioral strategies ( Wenzel, 2017 ). Multiple studies have incorporated CBT’s emphasis on reducing cognitive errors and strengthening positive behavior into their designs by, for example, using fictional storylines to help participants correct irrational thought patterns during reflective tasks, thereby improving patients’ depression conditions ( Stasiak et al., 2014 ; Topooco et al., 2019 ; Neumer et al., 2021 ).
In addition to the intervention methods involving apps and websites, serious games have also become a prospect for treating depression due to their interesting and interactive characteristics. Low-intensity human support combined with smartphone games may potentially reduce the resource requirements of traditional face-to-face counseling. Games contain complete storylines and competitive and cooperative tasks between peers in the form of levels that encourage adolescents to reflect on quizzes at the end of each challenge ( Gonsalves et al., 2019 ). Game designs tend to use flow theory, which emphasizes the dynamic matching of game challenges and the user’s own skill level ( Csikszentmihalyi, 2014 ). During game design, it is necessary to provide users with an easy-to-use and interesting gaming experience, as well as appropriate difficulty challenges, clear rules and goals, and instant feedback, which will help them relax and relieve stress, concentrate on changing cognitive processes, and improve their mood.
Two articles also consider the use of chatbots in interventions. Chatbots act as a dialog agent ( Mariamo et al., 2021 ), which makes the intervention process more interactive. Establishing a relationship of trust between adolescents and chatbots may also help lead to better results in depression and anxiety treatment. Chatbot functions are typically integrated into apps ( Werner-Seidler et al., 2020 ) and tend to be developed as part of the program rather than as a separate technological tool.
In recent years, with the gradual marketization of head-mounted VR devices, VR technology has been increasingly applied to mental health interventions. Studies have shown that the effectiveness of VR apps is often attributed to the distraction created by immersive environments, which produce an illusion of being in a virtual world, thus reducing users’ awareness of painful stimuli in the real world ( Ahmadpour et al., 2020 ). In the treatment of depression and anxiety for adolescents, active distraction supported by VR can engage users in games or cognitive tasks to redirect their attention to virtual objects and away from negative stimuli. Studies have also shown that, in addition to providing immersion, VR should create a pleasant emotional experience (e.g., the thrill of riding a roller coaster) and embed narrative stories (e.g., adventure and exploration) to meet adolescents’ need for achievement ( Ahmadpour et al., 2019 ).
2.2.2.2 Mental illness
In this study, we define mental illness as neurological developmental problems other than depression and anxiety. Among the 59 reviewed articles, 10 were coded as mental illness, including obsessive-compulsive disorder, attention-deficit/hyperactivity disorder, conduct disorder, oppositional defiant disorder, personality disorder, drug addiction, bipolar disorder, and non-suicidal self-injury. For the treatment of mental illness, mobile apps based on CBT appeared twice in 10 articles, while other technology types included SMS intervention, serious games, remote video conferencing, and mobile sensing technology.
Similar to apps for treating depression and anxiety, adolescent patients believe that the apps have good usability and ease of use and can encourage them to share their thoughts, feelings, and behavioral information more openly and honestly while protecting their privacy ( Adams et al., 2021 ). However, due to the severe condition of patients with mental illness, the apps not only are used independently by patients but also serves as a bridge between therapists and patients. Therapists can thus closely monitor treatment progress through behavioral records, which can provide direct feedback to both patients and therapists ( Babiano-Espinosa et al., 2021 ).
SMS interventions send specific content text messages to patients. As a longitudinal intervention method, it is convenient, easy to operate, and low cost. For example, Owens and Charles (2016) sent text messages to adolescents with non-suicidal self-injury behaviors in an attempt to reduce their self-mutilation behaviors. The ultimate effect seemed to be unsatisfactory, as interventions for adolescents with self-mutilation behaviors may be better applied in schools and adolescents’ service agencies, which can help them control their self-mutilation behaviors in the early stages and prevent such behaviors from escalating.
There are also studies that have designed six serious games based on CBT frameworks to treat typical developmental disorders in adolescents, including attention-deficit/hyperactivity disorder, conduct disorder, and oppositional defiant disorder ( Ong et al., 2019 ). In the safe environment provided by the game world, the research subjects shape the behavior of the characters in the context through rule learning and task repetition, which allows them to master emotional management strategies and problem-solving skills. In addition to interventions, digital technology can also be used to evaluate treatment effectiveness and the type of disease. Orr et al. (2023) used mobile sensing technology and digital phenotyping to quantify people’s behavioral data in real time, thereby allowing diagnosis and evaluation of diseases.
2.2.2.3 Bullying
Bullying generally includes traditional bullying and cyberbullying. Traditional bullying usually manifests as direct physical violence or threats of abuse against victims, as well as indirect methods such as spreading rumors and social exclusion. Cyberbullying is defined as intentional harm to others through computers, mobile phones, and other electronic devices. Data show that, as of 2021, the proportion of adolescents who have experienced cyberbullying in the United States may be as high as 45.5% ( Patchin, 2021 ), which indicates that it has become a serious social problem. Among the nine articles on the topic of bullying and cyberbullying, three used SMS intervention methods, and two used mobile apps; chatbots, technology-supported courses, and CBT-based telemedicine services were also used in the mental health treatment for patients who had been bullied and cyberbullied.
The SMS intervention for bullying implemented personalized customization, and the automatic SMS content can be customized based on the subjects’ previous questionnaire or completed self-report status ( Ranney et al., 2019 ). The subjects are required to rate their feelings at the end of the day and report whether they were bullied that day. The psychotherapist then made adjustments based on their actual situation, and if necessary, the psychotherapist would also contact specific subjects to provide offline psychological counseling services ( Ranney et al., 2019 ). In addition to having similar functions as the SMS intervention ( Kutok et al., 2021 ), mobile apps can provide opportunities for personalized learning, where a variety of learning methods can be applied (e.g., providing therapist guidance, conducting meetings, and conducting family practice activities) to promote the acquisition of mental health skills ( Davidson et al., 2019 ). Furthermore, for adolescents, touchscreen learning, interactive games, and video demonstrations can enhance their enthusiasm for participating in the treatment process.
Chatbots with specific names and images were also used to guide research subjects through a series of online tasks in the form of conversations, including watching videos involving bullying and cyberbullying among adolescents, provoking self-reflection through questions and suggestions, and providing constructive strategic advice ( Gabrielli et al., 2020 ). Digital technology-supported courses and CBT-based telemedicine services both make full use of the convenience of technology, effectively addressing the time- and location-based limitations of traditional face-to-face treatment. Digital courses can be implemented on a large scale in schools through teacher training, and compared with professional medical services, such courses have a wider target audience and can play a scientific and preventive role in bullying and cyberbullying. Telemedicine services refer to the use of remote communication technology to provide psychological services ( Joint Task Force for the Development of Telepsychology Guidelines for Psychologists, 2013 ). For families with severely troubled adolescents, telemedicine allows parents and children to meet together, increasing the flexibility of timing, and one-on-one video services can help to build a closer relationship between patients and therapists.
2.2.2.4 Lack of social emotional competence
In research, social emotional competence typically refers to the development of emotional intelligence in adolescents ( De la Barrera et al., 2021 ), which also includes personal abilities (self-awareness and self-management), interpersonal relationships (social awareness and interpersonal skills), and cognitive abilities (responsible decision-making) ( Collaborative for Academic, Social, and Emotional Learning, 2020 ). It is an important indicator for measuring the mental health level of adolescents. People with positive social emotional intelligence are less likely to experience mental health issues such as depression, anxiety, and behavioral disorders. Using digital technology to promote social emotional development is becoming increasingly common, and in six intervention studies on social emotional competence, apps, serious games, VR technology, and SMS interventions were used.
The studies considered all emphasized the importance of interactive design in digital technology to enhance social and emotional skills, as interactive technology can increase students’ engagement, resulting in positive learning experiences. For example, Cherewick et al. (2021) designed a smartphone app that can be embedded with multimedia learning materials, allowing adolescents to watch social and emotional skill–related learning videos autonomously and complete topic reflection activities with family/peers after school. The app also has rich teaching interaction functions, allowing teachers to evaluate and share course and learning materials, which can provide pleasant learning experiences to students while also improving the flexibility of teaching. In addition to teacher–student interaction, another paper also mentioned the importance of human–computer interaction for developing social emotional competence. The fun and interactivity of the app are the key to attracting adolescents to download and use it, and it can also have a positive effect on improving students’ self-management and decision-making skills ( Kenny et al., 2016 ).
Unlike the treatment of depression and anxiety, the application of VR in the cultivation of social emotional competence not only relies on its highly immersive characteristics but also emphasizes the positive effects of multi-sensory experiences on emotional regulation. By utilizing various sensor devices and visualization devices, adolescents are provided with ideal visual, auditory, and tactile guidance and regulation, which can enhance their emotional regulation abilities and relieve psychological stress ( Wu et al., 2022 ). Existing studies have integrated dance and music into virtual scenes ( Liu et al., 2021 ), using virtual harmonic music therapy to allow users to relax physically and mentally while enjoying music, thereby reducing stress and anxiety. VR technology is also highly adaptable and generalizable, which can help in building diverse scenes that meet the psychological expectations of patients based on the characteristics of the different treatment objects.
2.2.2.5 Mental health issues caused by the COVID-19 pandemic
The global outbreak of COVID-19 created severe challenges for the mental health of adolescents. Factors such as lack of social contact, lack of personal space at home, separation from parents and relatives, and concerns about academics and the future have exacerbated mental health risks, leading to increased loneliness, pain, social isolation, mental disorders, and symptoms of anxiety, depression, and stress. The reports from five studies indicated that the COVID-19 pandemic has exacerbated mental health issues in adolescents. During the pandemic, technology—which is not limited by time and space—became the preferred method of treatment. Apps, remote health services, and online training courses were used in research. The apps were resource-oriented and evidence-based interventions that allowed patients to interact with therapists through remote conferencing and encouraged patients to self-reflect and express themselves after the conference to improve their mental condition ( Gómez-Restrepo et al., 2022 ). Remote health services combined CBT and dialectical behavior therapy with professional counselors engaging in online communication with patients for several weeks. This was in line with research that indicates that the establishment of a positive relationship between therapists and patients is the foundation for obtaining good effect ( Zepeda et al., 2021 ).
2.2.2.6 Other mental health issues
In addition to the common mental health issues mentioned above, there were also interventions mentioned in the literature for improving body image anxiety, mental issues caused by hospitalization, and reading disabilities through digital technology means. Due to its high-immersion and simulation characteristics, VR technology was selected for improving mental health issues such as loneliness, disconnection from peers, and academic anxiety caused by hospitalization ( Thabrew et al., 2022 ). Immersive VR experience technology used 360° panoramic live broadcast and VR headphones to enable hospitalized adolescents to indirectly participate in social activities through cameras in school or home environments, as well as to contact peers and teachers through methods such as text messages; such interventions are conducive to improving social inclusion, social connectivity, and happiness. Furthermore, two studies mentioned body image anxiety, especially targeting female audiences, and the research integrated body image CBT techniques into serious games and chatbots ( Mariamo et al., 2021 ; Matheson et al., 2021 ), using interesting interactive exploration and free dialog forms to help adolescents gain a correct understanding of body image and solve body image anxiety issues.
Another study used eye-tracking technology to treat children with reading disabilities ( Davidson et al., 2019 ). The researcher developed a reading evaluation platform called Lexplore, which used eye-tracking technology to monitor children’s eye movements when reading to determine the cognitive processes behind each child’s individual reading style and then design appropriate strategies to improve their reading difficulties.
3 Study 2: meta-analysis
To explore the effect of digital technology in promoting mental health, this study used a meta-analysis to assess 10 papers. It includes both experimental and quasi-experimental research studies. CMA3.0 (Comprehensive Meta-Analysis 3.0) was used, and the meta-analysis process consisted of five phases.
Phase 1: Literature screening, based on the prior stage of literature information coding. Relevant literature was filtered using the following criteria for meta-analysis: (a) the study must compare “technical intervention” and “traditional intervention”; (b) the study should report complete data that can generate the effect amount (e.g., average, sample size, standard deviation or t -values, p -values, etc.); and (c) the dependent variables in the study should contain at least one aspect of mental health.
Phase 2: Effect size calculation. In the case of a large sample size, there is little difference between Cohen’s d, Glass, and Hedges’ g values, but Cohen’s d can significantly overestimate the effect size for studies with a small sample ( Hedges, 1981 ). Therefore, Hedges’ g was used as the effect size indicator in this study.
Phase 3: Model selection. Meta-analyses include fixed- and random-effects models. Different models may produce different effect sizes. Due to the differences in sample size, experimental procedures, and methods among the initial studies included in the meta-analysis, the estimated average effect values may not be completely consistent with the true population effect values, which results in sample heterogeneity. This study used the method proposed by Borenstein et al. (2009) to establish fixed- and random-effects models to eliminate the influence of sample heterogeneity. When the heterogeneity test ( Q value) results were significant, the random-effects model was used; otherwise, the fixed-effects model was used.
Phase 4: Testing of main effects and moderating effects. Based on the selected model, a test of the main effects was conducted. Meanwhile, if heterogeneity was present, a test of moderating effects could be conducted.
Phase 5: Publication bias test. Publication bias is a common systematic error in meta-analyses and refers to a tendency for significantly significant research results to be more likely to be published than non-significant results. This study used a funnel plot to visually assess publication bias qualitatively and then further quantitatively assessed publication bias using Begg’s rank correlation method and the trim and fill method.
3.2 Results and discussion
3.2.1 inclusion and coding results.
For the studies that met the requirements of the meta-analysis, detailed classification was carried out based on the following variables one-by-one on the basis of the systematic review coding: (a) basic information (authors, year, sample size); (b) age stage, which is divided into three categories: primary school, junior high school, and senior high school; (c) mental health issues, including depression, bullying, and mental health issues caused by COVID-19; (d) technology type, including app, telemedicine, and chatbots; (e) intervention duration, coded as short-term for interventions less than a month and long-term for intervention that lasted more than a month; and (f) effect size. The coding results are shown in Table 3 .
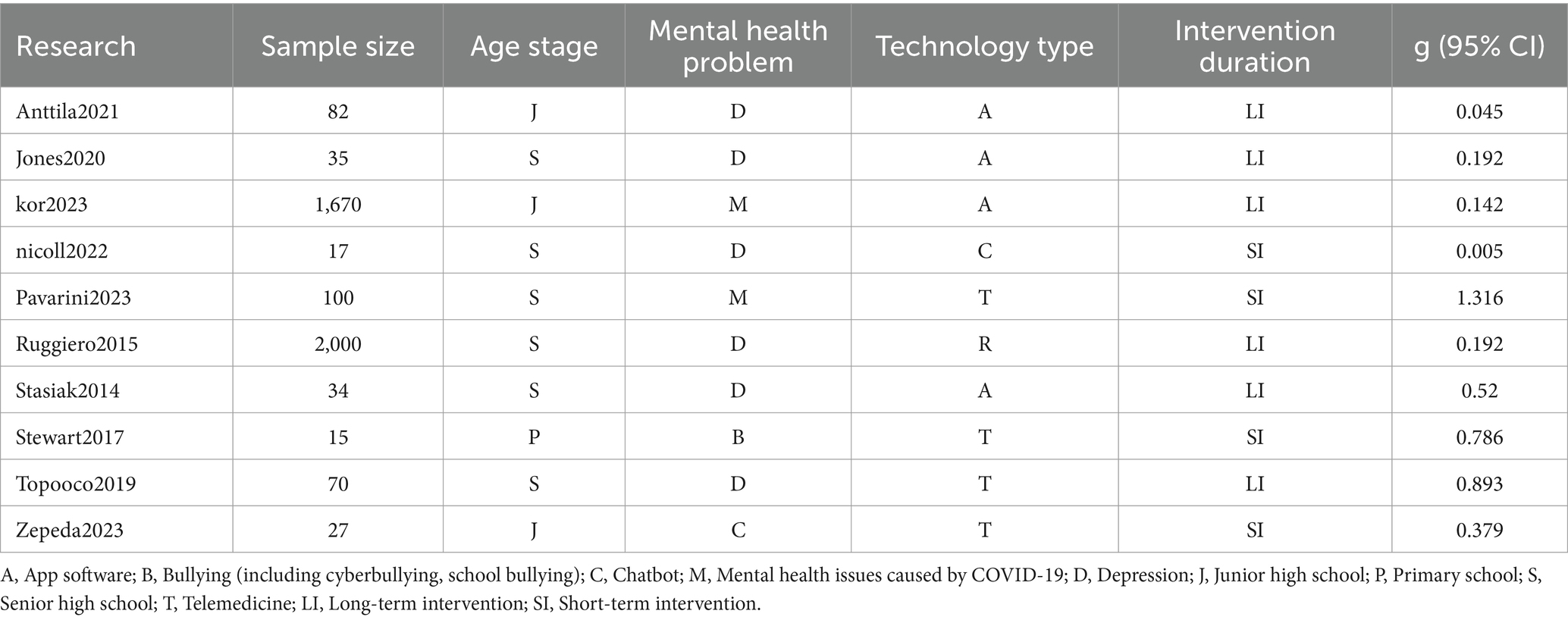
Table 3 . Research coding results included in meta-analysis.
3.2.2 The overall effect of digital technology on mental health outcomes
According to the results of the heterogeneity test in Table 4 , the Q test is significant ( p < 0.001), which indicates that there is significant heterogeneity among the samples. The random-effects model was therefore selected as the more reasonable option. The pooled effect size is 0.43. According to the criteria proposed by Cohen (1992) , 0.2, 0.5, and 0.8 are considered the boundaries of small, medium, and large effect sizes, respectively. It can be seen that the effect size for the promotion of mental health by digital technology is moderate and significant. At the same time, the lower limit of the 95% confidence interval is greater than 0 for each study, which indicates that the probability of the effect size being caused by chance is very small. In addition, the I 2 value is 78.164, which indicates that the heterogeneity between studies is high. Important moderating variables therefore may exist ( Higgins and Green, 2008 ), and additional moderating effect tests need to be conducted.

Table 4 . Overall effect of technology on mental health.
3.2.3 Moderating effect test
Moderating effect tests were conducted on four variables: age stage, mental health issues, technology type, and intervention duration. As shown in Table 5 , among the four moderating variables, only the age stage has a significant moderating effect ( p < 0.05). In particular, the effect size is the largest for the primary school stage, followed by the senior high school stage with a moderate promoting effect. In addition, although the effect size for the junior high school stage is small, it is still significant, which may be related to the limited number of studies considering this population. The results also indicate that the moderating effects of mental health issues, technology type, and intervention duration are not significant. However, it can be seen that digital technology methods have the largest effect size for treating psychological problems caused by COVID-19, while compared with apps and chatbots, remote medical services can achieve better effects. In terms of treatment duration, the effect size for short-term interventions is greater than that for long-term interventions.
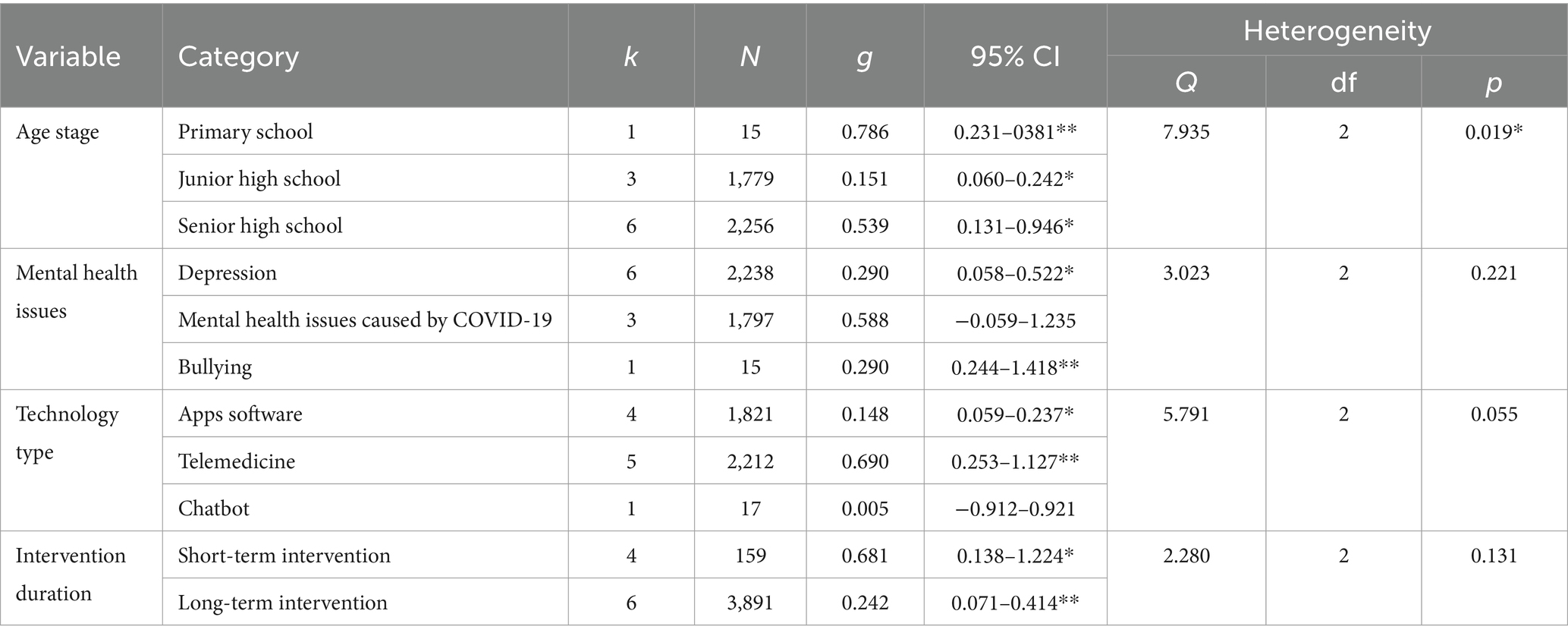
Table 5 . Regulatory effect test of technology (random-effect model).
3.2.4 Publication bias test
This study used funnel plots, Begg’s test, and the trim and fill method for the publication bias test. As shown in Figure 4 , the distribution of effect values in the study shows uneven and asymmetric distribution on both sides of the mean effect value, which initially suggests the possibility of publication bias. Begg’s test was thus used for further testing. Begg’s test is a method of quantitatively identifying bias using a rank correlation test, and it applies to studies with a small sample. The result of Begg’s test shows that t = 0.267, p = 0.283, Z = 1.01 < 1.96, which indicates that there is no obvious publication bias. Finally, the censoring method was used to censor the literature on both sides of the effect value, and this revealed that the effect value was still significant. In summary, there is negligible publication bias.
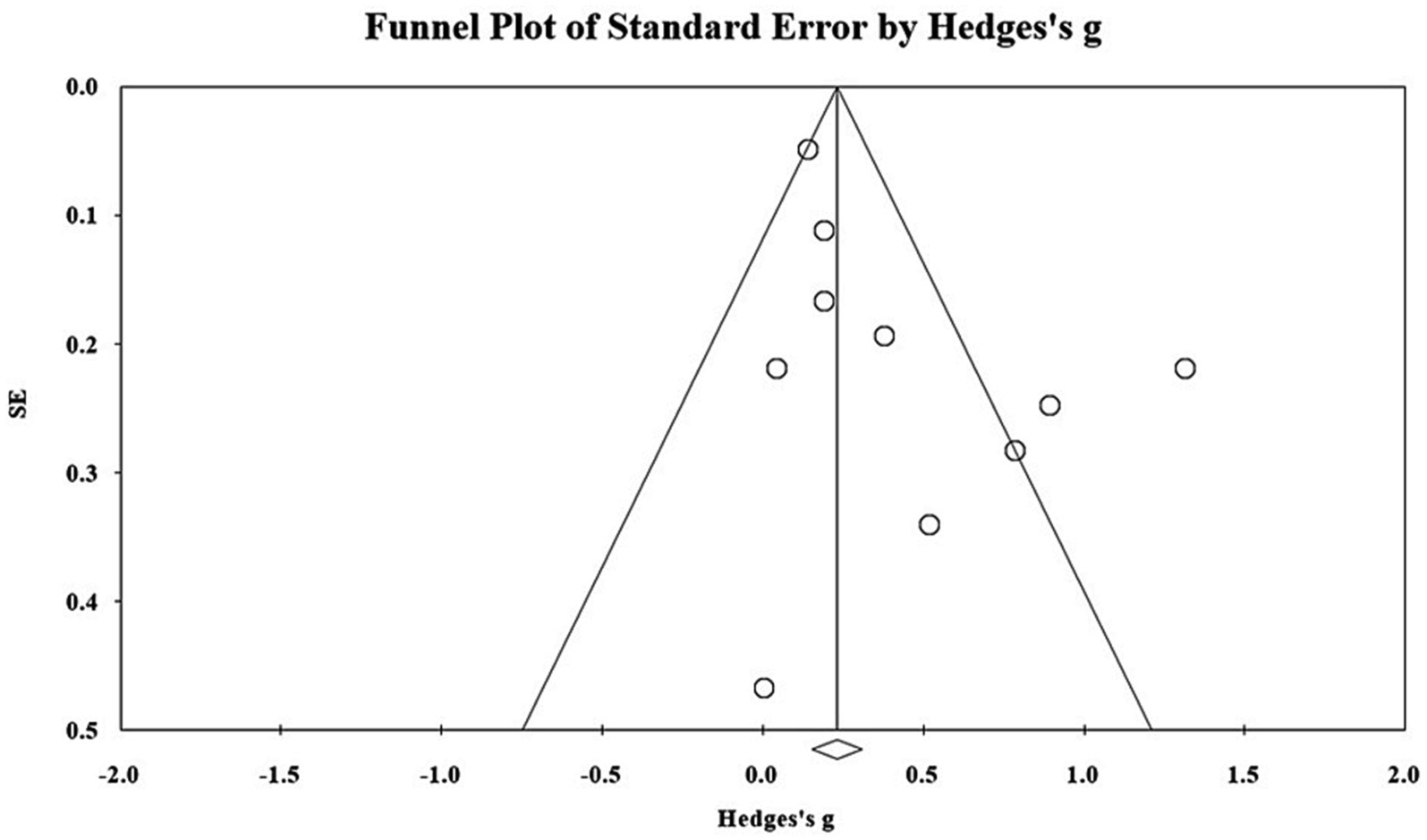
Figure 4 . Distributions of effect sizes for mental health treatment outcomes.
4 Conclusion and implications
4.1 summary of key findings.
This study made a systematic review and meta-analysis of 59 studies on digital technology promoting adolescents’ mental health over the past decade. Based on the investigation of current research, the types and characteristics of the commonly used technology interventions for different mental health issues were analyzed, and the actual effects and potential regulatory variables of digital technology in promoting mental health were investigated in the meta-analysis. The main findings are outlined below.
• Over the past decade, especially between 2013 and 2021, the number of studies on digital technology promoting adolescents’ mental health has generally shown an upward trend, with nearly 80% of the literature being published in medical journals.
• Digital technology is most commonly used to intervene in the mental health issues of adolescents aged 13–18 years, and children in the younger age group (6–12 years old) receive relatively less attention.
• Depression and anxiety disorders are the mental health issues that received the most research attention, followed by obsessive-compulsive disorder, attention-deficit hyperactivity disorder, conduct disorder, and other mental illnesses. There were also studies on, in decreasing order of the number of studies, bullying, social emotional competence deficiency, and mental health issues caused by COVID-19, dyslexia, and adolescent body image anxiety.
• Apps with convenience, ease of use, interactivity, and remote communication were most commonly used to treat mental health issues. Serious games, remote health services, and text message intervention were less often used, and only three studies used VR, which is difficult to realize for mental health treatment.
• Digital technology plays a significant role in promoting the treatment of mental health issues of adolescents, especially in primary and senior high school.
4.2 Interpretation and insights
The findings of this study highlight the nuanced role played by digital technology in promoting mental health for children and adolescents. While technology has broadened the scope of mental health interventions with innovative apps and programs, it should be viewed as a complement to traditional face-to-face approaches, not a replacement ( Aguilera, 2015 ), as they cannot replicate the personal connection and empathy provided by a trained mental health professional. Moreover, different technologies vary in effectiveness for specific mental health issues, emphasizing the need for careful evaluation of their benefits and limitations. For instance, virtual reality, cognitive behavioral therapy apps, and online support platforms have shown promise for in addressing depression and anxiety, but their effects vary depending on individual needs and contexts, suggesting the non-uniform efficacy of digital technologies across mental health conditions.
Furthermore, this study also draws attention to the limited incorporation of digital technology in mental health education, especially among children aged 6 to 12. Given the significance of this developmental stage, where emotional management, relationships, and mental health knowledge are crucial, innovative digital approaches that draw upon the unique affordances of mobile apps, online courses, and virtual reality are warranted to deliver interactive and personalized learning experiences. Nevertheless, this innovation poses challenges and risks, including addiction to virtual environments and a reduction in social activities, which can also negatively impact the mental health of youth ( Taylor et al., 2020 ). Therefore, striking a balance between harnessing technology’s potential and mitigating its risks is essential, emphasizing the need for responsible and targeted use of digital tools in mental healthcare and education.
4.3 Implication for practice and future research
Based on the results of the systematic review and meta-analysis, this study puts forward the relevant implications for practice and research. First, for mental health education service personnel, we suggest that the first step is to fully utilize the characteristics of digital technology and select the most appropriate digital intervention tools for different mental health issues. For example, apps are more suitable for the treatment of depression, anxiety, and mental illnesses. When facing adolescents who have been bullied, text message interventions may be a good choice. In addition, serious games and VR could play a greater role in developing adolescents’ social emotional competence.
Second, for mental health counselors or school mental health workers, it is necessary to consider learner characteristics and intervention duration, among other factors. In contrast to previous research results ( Tang et al., 2019 ), we found that the regulating effect of age was significant, so therapists need to implement personalized technical interventions for adolescents at different age stages. Short-term interventions seem to induce a greater effect size, so lengthy interventions should be avoided, as they are more likely to cause marginal effect and develop technical immunity for the youth population.
Third, for technology intervention developers, it is important to recognize that not all practitioners (e.g., psychologists, therapists) are technology savvy. In the process of designing mental health apps and VR interventions, it is necessary to provide sufficient technical support, such as instructional manuals and tutorial videos, to reduce the potential digital divide. It is also essential to arrange for appropriate technical personnel to provide safeguard services and training continuously, ensuring the personal safety and cybersecurity of practitioners and patients during intervention sessions.
For researchers, we suggest that, first, more empirical studies are needed to report first-hand experimental results. Most of the existing studies only described the experimental scheme and lacked key research results. It is hoped that future research will report the results as comprehensively as possible to improve the credibility and reliability of meta-analytical results. Second, the number of studies on moderating effects in the meta-analysis was relatively small. For example, there was only one study on the primary school population. Future research needs to focus on people who have paid less attention to existing studies and thus enhance the understanding of technology interventions in mental health. Finally, there have been few studies that analyze cost-effectiveness, which is key to determining whether technical interventions can be normalized and sustainable. Future studies need to conduct sufficient investigation and report on the cost-effectiveness of digital technology interventions, including the development and maintenance costs of VR ( Kraft, 2020 ).
Data availability statement
The raw data supporting the conclusions of this article will be made available by the authors, without undue reservation.
Author contributions
TC: Data curation, Formal analysis, Investigation, Visualization, Writing – original draft. JO: Formal analysis, Investigation, Writing – original draft. GL: Formal analysis, Writing – review & editing. HL: Conceptualization, Methodology, Supervision, Writing – review & editing.
The author(s) declare that no financial support was received for the research, authorship, and/or publication of this article.
Conflict of interest
The authors declare that the research was conducted in the absence of any commercial or financial relationships that could be construed as a potential conflict of interest.
Publisher’s note
All claims expressed in this article are solely those of the authors and do not necessarily represent those of their affiliated organizations, or those of the publisher, the editors and the reviewers. Any product that may be evaluated in this article, or claim that may be made by its manufacturer, is not guaranteed or endorsed by the publisher.
Adams, Z., Grant, M., Hupp, S., Scott, T., Feagans, A., Phillips, M. L., et al. (2021). Acceptability of an mHealth app for youth with substance use and mental health needs: iterative, mixed methods design. JMIR Form. Res. 5:e30268. doi: 10.2196/30268
PubMed Abstract | Crossref Full Text | Google Scholar
Aguilera, A. (2015). Digital technology and mental health interventions: opportunities and challenges. Arbor 191:a210. doi: 10.3989/arbor.2015.771n1012
Crossref Full Text | Google Scholar
Ahmadpour, N., Randall, H., Choksi, H., Gao, A., Vaughan, C., and Poronnik, P. (2019). Virtual reality interventions for acute and chronic pain management. Int. J. Biochem. Cell Biol. 114:105568. doi: 10.1016/j.biocel.2019.105568
Ahmadpour, N., Weatherall, A. D., Menezes, M., Yoo, S., Hong, H., and Wong, G. (2020). Synthesizing multiple stakeholder perspectives on using virtual reality to improve the periprocedural experience in children and adolescents: survey study. J. Med. Internet Res. 22:e19752. doi: 10.2196/19752
Antaramian, S. P., Huebner, E. S., Hills, K. J., and Valois, R. F. (2010). A dual-factor model of mental health: toward a more comprehensive understanding of youth functioning. Am. J. Orthopsychiatry 80, 462–472. doi: 10.1111/j.1939-0025.2010.01049.x
Aschbrenner, K. A., Naslund, J. A., Tomlinson, E. F., Kinney, A., Pratt, S. I., and Brunette, M. F. (2019). Adolescents' use of digital technologies and preferences for mobile health coaching in public mental health settings. Front. Public Health 7:178. doi: 10.3389/fpubh.2019.00178
Babiano-Espinosa, L., Wolters, L. H., Weidle, B., Compton, S. N., Lydersen, S., and Skokauskas, N. (2021). Acceptability and feasibility of enhanced cognitive behavioral therapy (eCBT) for children and adolescents with obsessive-compulsive disorder. Child Adolesc. Psychiatry Ment. Health 15:47. doi: 10.1186/s13034-021-00400-7
Bearman, M., Smith, C. D., Carbone, A., Slade, S., Baik, C., Hughes-Warrington, M., et al. (2012). Systematic review methodology in higher education. High. Educ. Res. Dev. 31, 625–640. doi: 10.1080/07294360.2012.702735
Borenstein, M., Hedges, L. V., Higgins, J. P. T., and Rothstein, H. R. (2009). Introduction to meta-analysis Wiley.
Google Scholar
Cejudo, J., López-Delgado, M. L., and Losada, L. (2019). Effectiveness of the videogame “Spock” for the improvement of the emotional intelligence on psychosocial adjustment in adolescents. Comput. Hum. Behav. 101, 380–386. doi: 10.1016/j.chb.2018.09.028
Cheng, V. W. S., Davenport, T., Johnson, D., Vella, K., and Hickie, I. B. (2019). Gamification in apps and technologies for improving mental health and well-being: systematic review. JMIR Mental Health 6:e13717. doi: 10.2196/13717
Cherewick, M., Lebu, S., Su, C., Richards, L., Njau, P. F., and Dahl, R. E. (2021). Study protocol of a distance learning intervention to support social emotional learning and identity development for adolescents using interactive mobile technology. Front. Public Health 9:623283. doi: 10.3389/fpubh.2021.623283
Cohen, J. (1992). A power primer. Psychol. Bull. 112, 155–159. doi: 10.1037/0033-2909.112.1.155
Collaborative for Academic, Social, and Emotional Learning (2020), What is the CASEL framework? A framework creates a foundation for applying evidence-based SEL strategies to your community. Available at: https://casel.org/fundamentals-of-sel/what-is-the-casel-framework/
Csikszentmihalyi, M. (2014). “Learning, “flow,” and happiness” in Applications of flow in human development and education (Dordrecht: Springer), 153–172.
Davidson, T. M., Bunnell, B. E., Saunders, B. E., Hanson, R. F., Danielson, C. K., Cook, D., et al. (2019). Pilot evaluation of a tablet-based application to improve quality of care in child mental health treatment. Behav. Ther. 50, 367–379. doi: 10.1016/j.beth.2018.07.005
De la Barrera, U., Postigo-Zegarra, S., Mónaco, E., Gil-Gómez, J.-A., and Montoya-Castilla, I. (2021). Serious game to promote socioemotional learning and mental health (emoTIC): a study protocol for randomised controlled trial. BMJ Open 11:e052491. doi: 10.1136/bmjopen-2021-052491
Eisenstadt, M., Liverpool, S., Infanti, E., Ciuvat, R. M., and Carlsson, C. (2021). Mobile apps that promote emotion regulation, positive mental health, and well-being in the general population: systematic review and meta-analysis. JMIR Mental Health 8:e31170. doi: 10.2196/31170
Essau, C. A., and de la Torre-Luque, A. (2023). Comorbidity between internalising and externalising disorders among adolescents: symptom connectivity features and psychosocial outcome. Child Psychiatry Hum. Dev. 54, 493–507. doi: 10.1007/s10578-021-01264-w
Folker, A. P., Mathiasen, K., Lauridsen, S. M., Stenderup, E., Dozeman, E., and Folker, M. P. (2018). Implementing internet-delivered cognitive behavior therapy for common mental health disorders: a comparative case study of implementation challenges perceived by therapists and managers in five European internet services. Internet Interv. 11, 60–70. doi: 10.1016/j.invent.2018.02.001
Gabrielli, S., Rizzi, S., Carbone, S., and Donisi, V. (2020). A chatbot-based coaching intervention for adolescents to promote life skills: pilot study. JMIR Hum. Factors 7:e16762. doi: 10.2196/16762
Giovanelli, A., Ozer, E. M., and Dahl, R. E. (2020). Leveraging technology to improve health in adolescence: a developmental science perspective. J. Adolesc. Health 67, S7–S13. doi: 10.1016/j.jadohealth.2020.02.020
Gladstone, T. G., Marko-Holguin, M., Rothberg, P., Nidetz, J., Diehl, A., DeFrino, D. T., et al. (2015). An internet-based adolescent depression preventive intervention: study protocol for a randomized control trial. Trials 16:203. doi: 10.1186/s13063-015-0705-2
Gómez-Restrepo, C., Sarmiento-Suárez, M. J., Alba-Saavedra, M., Bird, V. J., Priebe, S., and van Loggerenberg, F. (2022). Adapting DIALOG+ in a school setting-a tool to support well-being and resilience in adolescents living in postconflict areas during the COVID-19 pandemic: protocol for a cluster randomized exploratory study. JMIR Res. Protoc. 11:e40286. doi: 10.2196/40286
Gonsalves, P. P., Hodgson, E. S., Kumar, A., Aurora, T., Chandak, Y., Sharma, R., et al. (2019). Design and development of the "POD adventures" smartphone game: a blended problem-solving intervention for adolescent mental health in India. Front. Public Health 7:238. doi: 10.3389/fpubh.2019.00238
Goodyear, V. A., and Armour, K. M. (2018). Young people’s perspectives on and experiences of health-related social media, apps, and wearable health devices. Soc. Sci. 7:137. doi: 10.3390/socsci7080137
Grist, R., Croker, A., Denne, M., and Stallard, P. (2019). Technology delivered interventions for depression and anxiety in children and adolescents: a systematic review and meta-analysis. Clin. Child. Fam. Psychol. Rev. 22, 147–171. doi: 10.1007/s10567-018-0271-8
Hedges, L. V. (1981). Distribution theory for glass's estimator of effect size and related estimators. J. Educ. Stat. 6, 107–128. doi: 10.3102/10769986006002107
Higgins, J. P., and Green, S. (2008). Cochrane handbook for systematic reviews of interventions: Cochrane book series . Hoboken, New Jersey: Wiley.
Hollis, C., Falconer, C. J., Martin, J. L., Whittington, C., Stockton, S., Glazebrook, C., et al. (2017). Annual research review: digital health interventions for children and young people with mental health problems – a systematic and meta-review. J. Child Psychol. Psychiatry 58, 474–503. doi: 10.1111/jcpp.12663
Joint Task Force for the Development of Telepsychology Guidelines for Psychologists (2013). Guidelines for the practice of telepsychology. Am. Psychol. 68, 791–800. doi: 10.1037/a0035001
Jones, E. A. K., Mitra, A. K., and Bhuiyan, A. R. (2021). Impact of COVID-19 on mental health in adolescents: a systematic review. Int. J. Environ. Res. Public Health 18:2470. doi: 10.3390/ijerph18052470
Kenny, R., Dooley, B., and Fitzgerald, A. (2016). Developing mental health mobile apps: exploring adolescents’ perspectives. Health Informatics J. 22, 265–275. doi: 10.1177/1460458214555041
Keyes, C. L. M. (2009). “Toward a science of mental health” in The Oxford handbook of positive psychology (Oxford: Oxford University Press), 88–96.
Kraft, M. A. (2020). Interpreting effect sizes of education interventions. Educ. Res. 49, 241–253. doi: 10.3102/0013189x20912798
Kutok, E. R., Dunsiger, S., Patena, J. V., Nugent, N. R., Riese, A., Rosen, R. K., et al. (2021). A cyberbullying media-based prevention intervention for adolescents on instagram: pilot randomized controlled trial. JMIR Mental Health 8:e26029. doi: 10.2196/26029
Lacey, F. M., and Matheson, L. (2011). Doing your literature review: Traditional and systematic techniques . London: Sage.
Lewinsohn, P. M., Hops, H., Roberts, R. E., Seeley, J. R., and Andrews, J. A. (1993). Adolescent psychopathology: I. Prevalence and incidence of depression and other DSM-III—R disorders in high school students. J. Abnorm. Psychol. 102, 133–144. doi: 10.1037/0021-843x.102.1.133
Liu, T.-C., Lin, Y.-C., Wang, T.-N., Yeh, S.-C., and Kalyuga, S. (2021). Studying the effect of redundancy in a virtual reality classroom. Educ. Technol. Res. Dev. 69, 1183–1200. doi: 10.1007/s11423-021-09991-6
Mariamo, A., Temcheff, C. E., Léger, P.-M., Senecal, S., and Lau, M. A. (2021). Emotional reactions and likelihood of response to questions designed for a mental health chatbot among adolescents: experimental study. JMIR Hum. Factors 8:e24343. doi: 10.2196/24343
Matheson, E. L., Smith, H. G., Amaral, A. C. S., Meireles, J. F. F., Almeida, M. C., Mora, G., et al. (2021). Improving body image at scale among Brazilian adolescents: study protocol for the co-creation and randomised trial evaluation of a chatbot intervention. BMC Public Health 21:2135. doi: 10.1186/s12889-021-12129-1
Mohr, D. C., Riper, H., and Schueller, S. M. (2018). A solution-focused research approach to achieve an implementable revolution in digital mental health. JAMA Psychiatry 75, 113–114. doi: 10.1001/jamapsychiatry.2017.3838
Naslund, J. A., Aschbrenner, K. A., Kim, S. J., McHugo, G. J., Unützer, J., Bartels, S. J., et al. (2017). Health behavior models for informing digital technology interventions for individuals with mental illness. Psychiatr. Rehabil. J. 40, 325–335. doi: 10.1037/prj0000246
Neumer, S.-P., Patras, J., Holen, S., Lisøy, C., Askeland, A. L., Haug, I. M., et al. (2021). Study protocol of a factorial trial ECHO: optimizing a group-based school intervention for children with emotional problems. BMC Psychol. 9:97. doi: 10.1186/s40359-021-00581-y
Ong, J. G., Lim-Ashworth, N. S., Ooi, Y. P., Boon, J. S., Ang, R. P., Goh, D. H., et al. (2019). An interactive mobile app game to address aggression (regnatales): pilot quantitative study. JMIR Ser Games 7:e13242. doi: 10.2196/13242
Orlowski, S., Lawn, S., Antezana, G., Venning, A., Winsall, M., Bidargaddi, N., et al. (2016). A rural youth consumer perspective of technology to enhance face-to-face mental health services. J. Child Fam. Stud. 25, 3066–3075. doi: 10.1007/s10826-016-0472-z
Orr, M., MacLeod, L., Bagnell, A., McGrath, P., Wozney, L., and Meier, S. (2023). The comfort of adolescent patients and their parents with mobile sensing and digital phenotyping. Comput. Hum. Behav. 140:107603. doi: 10.1016/j.chb.2022.107603
Owens, C., and Charles, N. (2016). Implementation of a text-messaging intervention for adolescents who self-harm (TeenTEXT): a feasibility study using normalisation process theory. Child Adolesc. Psychiatry Ment. Health 10:14. doi: 10.1186/s13034-016-0101-z
Patchin, J. W. (2021). 2021 Cyberbullying Data. Available at: https://cyberbullying.org/2021-cyberbullying-data
Piers, R., Williams, J. M., and Sharpe, H. (2023). Review: can digital mental health interventions bridge the ‘digital divide’ for socioeconomically and digitally marginalised youth? A systematic review. Child Adolesc. Ment. Health 28, 90–104. doi: 10.1111/camh.12620
Ranney, M. L., Patena, J. V., Dunsiger, S., Spirito, A., Cunningham, R. M., Boyer, E., et al. (2019). A technology-augmented intervention to prevent peer violence and depressive symptoms among at-risk emergency department adolescents: protocol for a randomized control trial. Contemp. Clin. Trials 82, 106–114. doi: 10.1016/j.cct.2019.05.009
Scott, L. N., Victor, S. E., Kaufman, E. A., Beeney, J. E., Byrd, A. L., Vine, V., et al. (2020). Affective dynamics across internalizing and externalizing dimensions of psychopathology. Clin. Psychol. Sci. 8, 412–427. doi: 10.1177/2167702619898802
Shah, S. M. A., Mohammad, D., Qureshi, M. F. H., Abbas, M. Z., and Aleem, S. (2021). Prevalence, psychological responses and associated correlates of depression, anxiety and stress in a global population, during the coronavirus disease (COVID-19) pandemic. Community Ment. Health J. 57, 101–110. doi: 10.1007/s10597-020-00728-y
Stasiak, K., Hatcher, S., Frampton, C., and Merry, S. N. (2014). A pilot double blind randomized placebo controlled trial of a prototype computer-based cognitive behavioural therapy program for adolescents with symptoms of depression. Behav. Cogn. Psychother. 42, 385–401. doi: 10.1017/s1352465812001087
Suldo, S. M., and Shaffer, E. J. (2008). Looking beyond psychopathology: the dual-factor model of mental health in youth. School Psychol. Rev. 37, 52–68. doi: 10.1080/02796015.2008.12087908
Tang, X., Tang, S., Ren, Z., and Wong, D. F. K. (2019). Prevalence of depressive symptoms among adolescents in secondary school in mainland China: a systematic review and meta-analysis. J. Affect. Disord. 245, 498–507. doi: 10.1016/j.jad.2018.11.043
Taylor, C. B., Ruzek, J. I., Fitzsimmons-Craft, E. E., Sadeh-Sharvit, S., Topooco, N., Weissman, R. S., et al. (2020). Using digital technology to reduce the prevalence of mental health disorders in populations: time for a new approach. J. Med. Internet Res. 22:e17493. doi: 10.1177/2167702619859336
Thabrew, H., Chubb, L. A., Kumar, H., and Fouché, C. (2022). Immersive reality experience technology for reducing social isolation and improving social connectedness and well-being of children and young people who are hospitalized: open trial. JMIR Pediatr. Parent. 5:e29164. doi: 10.2196/29164
Topooco, N., Byléhn, S., Dahlström Nysäter, E., Holmlund, J., Lindegaard, J., Johansson, S., et al. (2019). Evaluating the efficacy of internet-delivered cognitive behavioral therapy blended with synchronous chat sessions to treat adolescent depression: randomized controlled trial. J. Med. Internet Res. 21:e13393. doi: 10.2196/13393
Uhlhaas, P., and Torous, J. (2019). Digital tools for youth mental health. NPJ Digit. Med. 2:104. doi: 10.1038/s41746-019-0181-2
Villarreal, V. (2018). Mental health collaboration: a survey of practicing school psychologists. J. Appl. Sch. Psychol. 34, 1–17. doi: 10.1080/15377903.2017.1328626
Wacks, Y., and Weinstein, A. M. (2021). Excessive smartphone use is associated with health problems in adolescents and young adults. Front. Psych. 12:669042. doi: 10.3389/fpsyt.2021.669042
Wenzel, A. (2017). Basic strategies of cognitive behavioral therapy. Psychiatr. Clin. North Am. 40, 597–609. doi: 10.1016/j.psc.2017.07.001
Werner-Seidler, A., Huckvale, K., Larsen, M. E., Calear, A. L., Maston, K., Johnston, L., et al. (2020). A trial protocol for the effectiveness of digital interventions for preventing depression in adolescents: the future proofing study. Trials 21:2. doi: 10.1186/s13063-019-3901-7
World Health Organization (2021). Mental health of adolescents. Available at: https://www.who.int/news-room/fact-sheets/detail/adolescent-mental-health (Accessed December 14, 2023).
Wu, B., Zheng, C., and Huang, B. (2022). Influence of science education on mental health of adolescents based on virtual reality. Front. Psychol. 13:895196. doi: 10.3389/fpsyg.2022.895196
Zepeda, M., Deighton, S., Markova, V., Madsen, J., and Racine, N. (2021). iCOPE with COVID-19: A brief telemental health intervention for children and adolescents during the COVID-19 pandemic. PsyArXiv . doi: 10.31234/osf.io/jk32s
Keywords: children and adolescents, digital technology, systematic literature review, meta-analysis, mental health issues
Citation: Chen T, Ou J, Li G and Luo H (2024) Promoting mental health in children and adolescents through digital technology: a systematic review and meta-analysis. Front. Psychol . 15:1356554. doi: 10.3389/fpsyg.2024.1356554
Received: 15 December 2023; Accepted: 29 February 2024; Published: 12 March 2024.
Reviewed by:
Copyright © 2024 Chen, Ou, Li and Luo. This is an open-access article distributed under the terms of the Creative Commons Attribution License (CC BY) . The use, distribution or reproduction in other forums is permitted, provided the original author(s) and the copyright owner(s) are credited and that the original publication in this journal is cited, in accordance with accepted academic practice. No use, distribution or reproduction is permitted which does not comply with these terms.
*Correspondence: Heng Luo, [email protected]
Disclaimer: All claims expressed in this article are solely those of the authors and do not necessarily represent those of their affiliated organizations, or those of the publisher, the editors and the reviewers. Any product that may be evaluated in this article or claim that may be made by its manufacturer is not guaranteed or endorsed by the publisher.
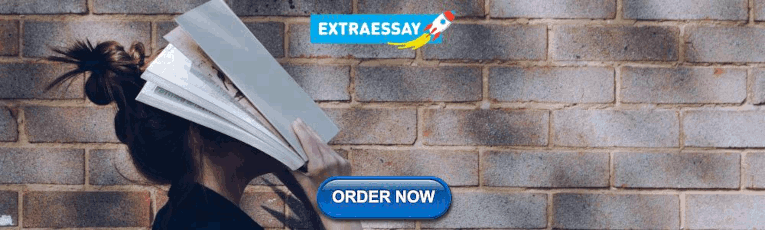
IMAGES
VIDEO
COMMENTS
The best reviews synthesize studies to draw broad theoretical conclusions about what a literature means, linking theory to evidence and evidence to theory. This guide describes how to plan, conduct, organize, and present a systematic review of quantitative (meta-analysis) or qualitative (narrative review, meta-synthesis) information.
A review is an overview of the research that's already been completed on a topic. What makes a systematic review different from other types of reviews is that the research methods are designed to reduce bias. The methods are repeatable, and the approach is formal and systematic: Formulate a research question. Develop a protocol.
A systematic review aims to bring evidence together to answer a pre-defined research question. This involves the identification of all primary research relevant to the defined review question, the critical appraisal of this research, and the synthesis of the findings.13 Systematic reviews may combine data from different.
We outline established and innovative methods for synthesising quantitative evidence within a systematic review of a complex intervention, including considerations of the complexity of the system into which the intervention is introduced. ... LAM is funded by a National Institute for Health Research (NIHR) Systematic Review Fellowship (RM-SR ...
Quantitative systematic reviews can, therefore, also be narrative, where the results of the combined studies are synthesized descriptively. Both of these methods alone or in combination are able to summarize existing research on a particular topic. ... Using bibliographic software to appraise and code data in educational systematic review ...
Published on 15 June 2022 by Shaun Turney . Revised on 17 October 2022. A systematic review is a type of review that uses repeatable methods to find, select, and synthesise all available evidence. It answers a clearly formulated research question and explicitly states the methods used to arrive at the answer.
Effects of E-learning in a continuing education context on nursing care: Systematic review of systematic qualitative, quantitative, and mixed-studies reviews. Journal of Medical Internet Research , 21(10), e15118.
The purpose of our systematic literature review is twofold: (a) to understand how critical approaches to quantitative inquiry emerged as a new paradigm within quantitative methods and (b) whether there is any distinction between quantitative criticalism, QuantCrit, and critical quantitative inquiries or simply interchangeable wordplay.
an explicit, reproducible methodology. a systematic search that attempts to identify all studies that would meet the eligibility criteria. an assessment of the validity of the findings of the included studies, for example through the assessment of the risk of bias. a systematic presentation, and synthesis, of the characteristics and findings of ...
Systematic review (SR), a way of evidence generation, is a synthesis of primary scientific evidence, which summarizes the best evidence on a specific clinical question using a transparent, a priori protocol driven approach. ... In quantitative research, the aim is usually to establish changes in the other variable (experimental studies), and/or ...
Therefore, systematic reviews are considered reliable tools in scientific research and clinical practice. They synthesize the results using multiple primary studies by using strategies that minimize bias and random errors. Depending on the research question and the objectives of the research, the reviews can either be qualitative or quantitative.
A quantitative systematic review will include studies that have numerical data. A qualitative systematic review derives data from observation, interviews, or verbal interactions and focuses on the meanings and interpretations of the participants. ... Booth, A. (2016). Searching for qualitative research for inclusion in systematic reviews: A ...
Mixed studies review/mixed methods review. Refers to any combination of methods where one significant component is a literature review (usually systematic). Within a review context it refers to a combination of review approaches for example combining quantitative with qualitative research or outcome with process studies
Mixed methods reviews. As one reason for the growth in qualitative synthesis is what they can add to quantitative reviews, it is not surprising that there is also growing interest in mixed methods reviews. This reflects similar developments in primary research in mixing methods to examine the relationship between theory and empirical data which ...
This research was embedded in the OPTICA trial [], a cluster randomized controlled trial in Swiss primary care practices conducted by an interdisciplinary and interprofessional team (e.g., GPs, epidemiologists, etc.).The main goal of this trial was to investigate whether the use of a structured medication review intervention centered around the use of an eCDSS, namely the 'Systematic Tool to ...
Literature review is an essential feature of academic research. Fundamentally, knowledge advancement must be built on prior existing work. To push the knowledge frontier, we must know where the frontier is. By reviewing relevant literature, we understand the breadth and depth of the existing body of work and identify gaps to explore.
The research questions of this systematic review are designed to synthesize the existing findings on mental health conditions in survivors of the Anfal Genocide. ... E. B. (2006). Sex differences in trauma and posttraumatic stress disorder: A quantitative review of 25 years of research. Psychological Bulletin, 132(6), 959-992. https://doi.org ...
In terms of quantitative research methods, many existing studies employed experimental research. For example, scholars used ChatGPT as a subject and conducted quantitative research by comparing its writing abilities with other learners in the experiment. ... ChatGPT utility in healthcare education, research, and practice: Systematic review on ...
We included studies reporting primary qualitative and quantitative data from all study types relating to the use of social network interventions for people with mental health difficulties. ... Harden A. Methods for the thematic synthesis of qualitative research in systematic reviews. BMC Med Res Methodol. 2008;8(1):45. Article PubMed PubMed ...
Conclusions: Our findings map onto the complex picture seen from quantitative systematic reviews regarding the impact of Covid-19 on YP's mental health. The comparatively little qualitative data found in our review means there is an urgent need for more high-quality qualitative research outside of the UK and/or about the experiences of ...
Prospective longitudinal studies exploring the association between three common NDCs in childhood or adolescence (i.e., ADHD, Autism, and Tic Disorders <18 years of age) and any physical LTC in adulthood (i.e., > 18 years of age) will be selected through title and abstract review, followed by a full-text review.
Securely automate every stage of your literature review to produce evidence-based research faster, more accurately, and more transparently at scale. Rayyan A web-tool designed to help researchers working on systematic reviews, scoping reviews and other knowledge synthesis projects, by dramatically speeding up the process of screening and ...
Research relating to counselling military service personnel 2009 (pdf 0.3MB) Older people. Counselling older people: A summary of the literature 2010 (pdf 0.3MB) Psychological interventions for carers of people with dementia: a systematic review of quantitative and qualitative evidence 2012
It followed the Preferred Reporting Items for Systematic Reviews and Meta-Analysis statement for the selection and use of research methods. The protocol for this study was registered with INPLASY (2023120004). Standardized systematic review protocol is used to strictly identify, screen, analyze, and integrate literature (Bearman et al., 2012).