Get science-backed answers as you write with Paperpal's Research feature
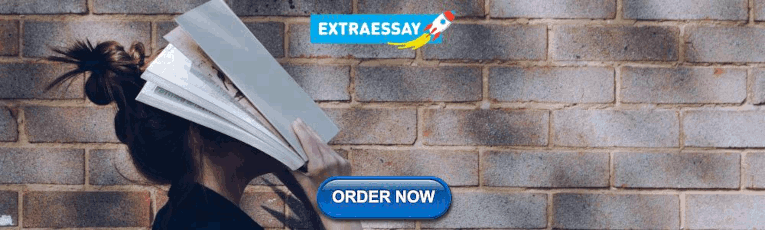
How to Write a Research Paper Introduction (with Examples)
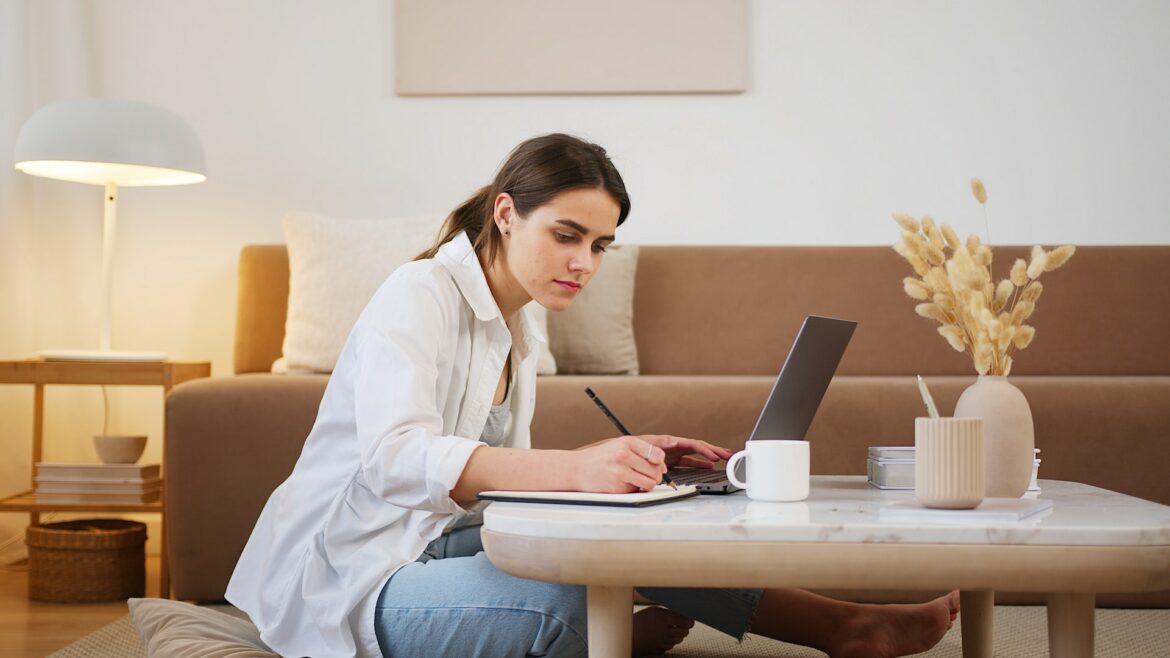
The research paper introduction section, along with the Title and Abstract, can be considered the face of any research paper. The following article is intended to guide you in organizing and writing the research paper introduction for a quality academic article or dissertation.
The research paper introduction aims to present the topic to the reader. A study will only be accepted for publishing if you can ascertain that the available literature cannot answer your research question. So it is important to ensure that you have read important studies on that particular topic, especially those within the last five to ten years, and that they are properly referenced in this section. 1 What should be included in the research paper introduction is decided by what you want to tell readers about the reason behind the research and how you plan to fill the knowledge gap. The best research paper introduction provides a systemic review of existing work and demonstrates additional work that needs to be done. It needs to be brief, captivating, and well-referenced; a well-drafted research paper introduction will help the researcher win half the battle.
The introduction for a research paper is where you set up your topic and approach for the reader. It has several key goals:
- Present your research topic
- Capture reader interest
- Summarize existing research
- Position your own approach
- Define your specific research problem and problem statement
- Highlight the novelty and contributions of the study
- Give an overview of the paper’s structure
The research paper introduction can vary in size and structure depending on whether your paper presents the results of original empirical research or is a review paper. Some research paper introduction examples are only half a page while others are a few pages long. In many cases, the introduction will be shorter than all of the other sections of your paper; its length depends on the size of your paper as a whole.
- Break through writer’s block. Write your research paper introduction with Paperpal Copilot
Table of Contents
What is the introduction for a research paper, why is the introduction important in a research paper, craft a compelling introduction section with paperpal. try now, 1. introduce the research topic:, 2. determine a research niche:, 3. place your research within the research niche:, craft accurate research paper introductions with paperpal. start writing now, frequently asked questions on research paper introduction, key points to remember.
The introduction in a research paper is placed at the beginning to guide the reader from a broad subject area to the specific topic that your research addresses. They present the following information to the reader
- Scope: The topic covered in the research paper
- Context: Background of your topic
- Importance: Why your research matters in that particular area of research and the industry problem that can be targeted
The research paper introduction conveys a lot of information and can be considered an essential roadmap for the rest of your paper. A good introduction for a research paper is important for the following reasons:
- It stimulates your reader’s interest: A good introduction section can make your readers want to read your paper by capturing their interest. It informs the reader what they are going to learn and helps determine if the topic is of interest to them.
- It helps the reader understand the research background: Without a clear introduction, your readers may feel confused and even struggle when reading your paper. A good research paper introduction will prepare them for the in-depth research to come. It provides you the opportunity to engage with the readers and demonstrate your knowledge and authority on the specific topic.
- It explains why your research paper is worth reading: Your introduction can convey a lot of information to your readers. It introduces the topic, why the topic is important, and how you plan to proceed with your research.
- It helps guide the reader through the rest of the paper: The research paper introduction gives the reader a sense of the nature of the information that will support your arguments and the general organization of the paragraphs that will follow. It offers an overview of what to expect when reading the main body of your paper.
What are the parts of introduction in the research?
A good research paper introduction section should comprise three main elements: 2
- What is known: This sets the stage for your research. It informs the readers of what is known on the subject.
- What is lacking: This is aimed at justifying the reason for carrying out your research. This could involve investigating a new concept or method or building upon previous research.
- What you aim to do: This part briefly states the objectives of your research and its major contributions. Your detailed hypothesis will also form a part of this section.
How to write a research paper introduction?
The first step in writing the research paper introduction is to inform the reader what your topic is and why it’s interesting or important. This is generally accomplished with a strong opening statement. The second step involves establishing the kinds of research that have been done and ending with limitations or gaps in the research that you intend to address. Finally, the research paper introduction clarifies how your own research fits in and what problem it addresses. If your research involved testing hypotheses, these should be stated along with your research question. The hypothesis should be presented in the past tense since it will have been tested by the time you are writing the research paper introduction.
The following key points, with examples, can guide you when writing the research paper introduction section:
- Highlight the importance of the research field or topic
- Describe the background of the topic
- Present an overview of current research on the topic
Example: The inclusion of experiential and competency-based learning has benefitted electronics engineering education. Industry partnerships provide an excellent alternative for students wanting to engage in solving real-world challenges. Industry-academia participation has grown in recent years due to the need for skilled engineers with practical training and specialized expertise. However, from the educational perspective, many activities are needed to incorporate sustainable development goals into the university curricula and consolidate learning innovation in universities.
- Reveal a gap in existing research or oppose an existing assumption
- Formulate the research question
Example: There have been plausible efforts to integrate educational activities in higher education electronics engineering programs. However, very few studies have considered using educational research methods for performance evaluation of competency-based higher engineering education, with a focus on technical and or transversal skills. To remedy the current need for evaluating competencies in STEM fields and providing sustainable development goals in engineering education, in this study, a comparison was drawn between study groups without and with industry partners.
- State the purpose of your study
- Highlight the key characteristics of your study
- Describe important results
- Highlight the novelty of the study.
- Offer a brief overview of the structure of the paper.
Example: The study evaluates the main competency needed in the applied electronics course, which is a fundamental core subject for many electronics engineering undergraduate programs. We compared two groups, without and with an industrial partner, that offered real-world projects to solve during the semester. This comparison can help determine significant differences in both groups in terms of developing subject competency and achieving sustainable development goals.
Write a Research Paper Introduction in Minutes with Paperpal
Paperpal Copilot is a generative AI-powered academic writing assistant. It’s trained on millions of published scholarly articles and over 20 years of STM experience. Paperpal Copilot helps authors write better and faster with:
- Real-time writing suggestions
- In-depth checks for language and grammar correction
- Paraphrasing to add variety, ensure academic tone, and trim text to meet journal limits
With Paperpal Copilot, create a research paper introduction effortlessly. In this step-by-step guide, we’ll walk you through how Paperpal transforms your initial ideas into a polished and publication-ready introduction.
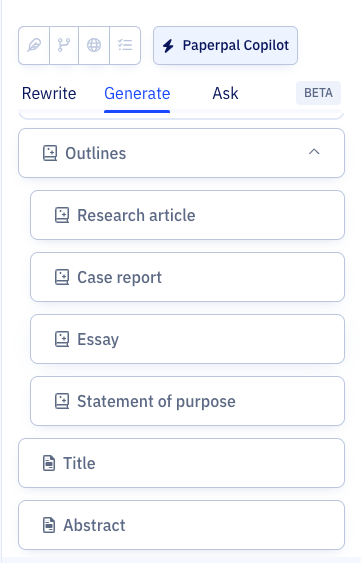
How to use Paperpal to write the Introduction section
Step 1: Sign up on Paperpal and click on the Copilot feature, under this choose Outlines > Research Article > Introduction
Step 2: Add your unstructured notes or initial draft, whether in English or another language, to Paperpal, which is to be used as the base for your content.
Step 3: Fill in the specifics, such as your field of study, brief description or details you want to include, which will help the AI generate the outline for your Introduction.
Step 4: Use this outline and sentence suggestions to develop your content, adding citations where needed and modifying it to align with your specific research focus.
Step 5: Turn to Paperpal’s granular language checks to refine your content, tailor it to reflect your personal writing style, and ensure it effectively conveys your message.
You can use the same process to develop each section of your article, and finally your research paper in half the time and without any of the stress.
The purpose of the research paper introduction is to introduce the reader to the problem definition, justify the need for the study, and describe the main theme of the study. The aim is to gain the reader’s attention by providing them with necessary background information and establishing the main purpose and direction of the research.
The length of the research paper introduction can vary across journals and disciplines. While there are no strict word limits for writing the research paper introduction, an ideal length would be one page, with a maximum of 400 words over 1-4 paragraphs. Generally, it is one of the shorter sections of the paper as the reader is assumed to have at least a reasonable knowledge about the topic. 2 For example, for a study evaluating the role of building design in ensuring fire safety, there is no need to discuss definitions and nature of fire in the introduction; you could start by commenting upon the existing practices for fire safety and how your study will add to the existing knowledge and practice.
When deciding what to include in the research paper introduction, the rest of the paper should also be considered. The aim is to introduce the reader smoothly to the topic and facilitate an easy read without much dependency on external sources. 3 Below is a list of elements you can include to prepare a research paper introduction outline and follow it when you are writing the research paper introduction. Topic introduction: This can include key definitions and a brief history of the topic. Research context and background: Offer the readers some general information and then narrow it down to specific aspects. Details of the research you conducted: A brief literature review can be included to support your arguments or line of thought. Rationale for the study: This establishes the relevance of your study and establishes its importance. Importance of your research: The main contributions are highlighted to help establish the novelty of your study Research hypothesis: Introduce your research question and propose an expected outcome. Organization of the paper: Include a short paragraph of 3-4 sentences that highlights your plan for the entire paper
Cite only works that are most relevant to your topic; as a general rule, you can include one to three. Note that readers want to see evidence of original thinking. So it is better to avoid using too many references as it does not leave much room for your personal standpoint to shine through. Citations in your research paper introduction support the key points, and the number of citations depend on the subject matter and the point discussed. If the research paper introduction is too long or overflowing with citations, it is better to cite a few review articles rather than the individual articles summarized in the review. A good point to remember when citing research papers in the introduction section is to include at least one-third of the references in the introduction.
The literature review plays a significant role in the research paper introduction section. A good literature review accomplishes the following: Introduces the topic – Establishes the study’s significance – Provides an overview of the relevant literature – Provides context for the study using literature – Identifies knowledge gaps However, remember to avoid making the following mistakes when writing a research paper introduction: Do not use studies from the literature review to aggressively support your research Avoid direct quoting Do not allow literature review to be the focus of this section. Instead, the literature review should only aid in setting a foundation for the manuscript.
Remember the following key points for writing a good research paper introduction: 4
- Avoid stuffing too much general information: Avoid including what an average reader would know and include only that information related to the problem being addressed in the research paper introduction. For example, when describing a comparative study of non-traditional methods for mechanical design optimization, information related to the traditional methods and differences between traditional and non-traditional methods would not be relevant. In this case, the introduction for the research paper should begin with the state-of-the-art non-traditional methods and methods to evaluate the efficiency of newly developed algorithms.
- Avoid packing too many references: Cite only the required works in your research paper introduction. The other works can be included in the discussion section to strengthen your findings.
- Avoid extensive criticism of previous studies: Avoid being overly critical of earlier studies while setting the rationale for your study. A better place for this would be the Discussion section, where you can highlight the advantages of your method.
- Avoid describing conclusions of the study: When writing a research paper introduction remember not to include the findings of your study. The aim is to let the readers know what question is being answered. The actual answer should only be given in the Results and Discussion section.
To summarize, the research paper introduction section should be brief yet informative. It should convince the reader the need to conduct the study and motivate him to read further. If you’re feeling stuck or unsure, choose trusted AI academic writing assistants like Paperpal to effortlessly craft your research paper introduction and other sections of your research article.
1. Jawaid, S. A., & Jawaid, M. (2019). How to write introduction and discussion. Saudi Journal of Anaesthesia, 13(Suppl 1), S18.
2. Dewan, P., & Gupta, P. (2016). Writing the title, abstract and introduction: Looks matter!. Indian pediatrics, 53, 235-241.
3. Cetin, S., & Hackam, D. J. (2005). An approach to the writing of a scientific Manuscript1. Journal of Surgical Research, 128(2), 165-167.
4. Bavdekar, S. B. (2015). Writing introduction: Laying the foundations of a research paper. Journal of the Association of Physicians of India, 63(7), 44-6.
Paperpal is a comprehensive AI writing toolkit that helps students and researchers achieve 2x the writing in half the time. It leverages 21+ years of STM experience and insights from millions of research articles to provide in-depth academic writing, language editing, and submission readiness support to help you write better, faster.
Get accurate academic translations, rewriting support, grammar checks, vocabulary suggestions, and generative AI assistance that delivers human precision at machine speed. Try for free or upgrade to Paperpal Prime starting at US$19 a month to access premium features, including consistency, plagiarism, and 30+ submission readiness checks to help you succeed.
Experience the future of academic writing – Sign up to Paperpal and start writing for free!
Related Reads:
- Scientific Writing Style Guides Explained
- 5 Reasons for Rejection After Peer Review
- Ethical Research Practices For Research with Human Subjects
- 8 Most Effective Ways to Increase Motivation for Thesis Writing
Practice vs. Practise: Learn the Difference
Academic paraphrasing: why paperpal’s rewrite should be your first choice , you may also like, ai in education: it’s time to change the..., is it ethical to use ai-generated abstracts without..., what are journal guidelines on using generative ai..., quillbot review: features, pricing, and free alternatives, what is an academic paper types and elements , should you use ai tools like chatgpt for..., publish research papers: 9 steps for successful publications , what are the different types of research papers, how to make translating academic papers less challenging, self-plagiarism in research: what it is and how....
- USC Libraries
- Research Guides
Organizing Your Social Sciences Research Paper
- 4. The Introduction
- Purpose of Guide
- Design Flaws to Avoid
- Independent and Dependent Variables
- Glossary of Research Terms
- Reading Research Effectively
- Narrowing a Topic Idea
- Broadening a Topic Idea
- Extending the Timeliness of a Topic Idea
- Academic Writing Style
- Choosing a Title
- Making an Outline
- Paragraph Development
- Research Process Video Series
- Executive Summary
- The C.A.R.S. Model
- Background Information
- The Research Problem/Question
- Theoretical Framework
- Citation Tracking
- Content Alert Services
- Evaluating Sources
- Primary Sources
- Secondary Sources
- Tiertiary Sources
- Scholarly vs. Popular Publications
- Qualitative Methods
- Quantitative Methods
- Insiderness
- Using Non-Textual Elements
- Limitations of the Study
- Common Grammar Mistakes
- Writing Concisely
- Avoiding Plagiarism
- Footnotes or Endnotes?
- Further Readings
- Generative AI and Writing
- USC Libraries Tutorials and Other Guides
- Bibliography
The introduction leads the reader from a general subject area to a particular topic of inquiry. It establishes the scope, context, and significance of the research being conducted by summarizing current understanding and background information about the topic, stating the purpose of the work in the form of the research problem supported by a hypothesis or a set of questions, explaining briefly the methodological approach used to examine the research problem, highlighting the potential outcomes your study can reveal, and outlining the remaining structure and organization of the paper.
Key Elements of the Research Proposal. Prepared under the direction of the Superintendent and by the 2010 Curriculum Design and Writing Team. Baltimore County Public Schools.
Importance of a Good Introduction
Think of the introduction as a mental road map that must answer for the reader these four questions:
- What was I studying?
- Why was this topic important to investigate?
- What did we know about this topic before I did this study?
- How will this study advance new knowledge or new ways of understanding?
According to Reyes, there are three overarching goals of a good introduction: 1) ensure that you summarize prior studies about the topic in a manner that lays a foundation for understanding the research problem; 2) explain how your study specifically addresses gaps in the literature, insufficient consideration of the topic, or other deficiency in the literature; and, 3) note the broader theoretical, empirical, and/or policy contributions and implications of your research.
A well-written introduction is important because, quite simply, you never get a second chance to make a good first impression. The opening paragraphs of your paper will provide your readers with their initial impressions about the logic of your argument, your writing style, the overall quality of your research, and, ultimately, the validity of your findings and conclusions. A vague, disorganized, or error-filled introduction will create a negative impression, whereas, a concise, engaging, and well-written introduction will lead your readers to think highly of your analytical skills, your writing style, and your research approach. All introductions should conclude with a brief paragraph that describes the organization of the rest of the paper.
Hirano, Eliana. “Research Article Introductions in English for Specific Purposes: A Comparison between Brazilian, Portuguese, and English.” English for Specific Purposes 28 (October 2009): 240-250; Samraj, B. “Introductions in Research Articles: Variations Across Disciplines.” English for Specific Purposes 21 (2002): 1–17; Introductions. The Writing Center. University of North Carolina; “Writing Introductions.” In Good Essay Writing: A Social Sciences Guide. Peter Redman. 4th edition. (London: Sage, 2011), pp. 63-70; Reyes, Victoria. Demystifying the Journal Article. Inside Higher Education.
Structure and Writing Style
I. Structure and Approach
The introduction is the broad beginning of the paper that answers three important questions for the reader:
- What is this?
- Why should I read it?
- What do you want me to think about / consider doing / react to?
Think of the structure of the introduction as an inverted triangle of information that lays a foundation for understanding the research problem. Organize the information so as to present the more general aspects of the topic early in the introduction, then narrow your analysis to more specific topical information that provides context, finally arriving at your research problem and the rationale for studying it [often written as a series of key questions to be addressed or framed as a hypothesis or set of assumptions to be tested] and, whenever possible, a description of the potential outcomes your study can reveal.
These are general phases associated with writing an introduction: 1. Establish an area to research by:
- Highlighting the importance of the topic, and/or
- Making general statements about the topic, and/or
- Presenting an overview on current research on the subject.
2. Identify a research niche by:
- Opposing an existing assumption, and/or
- Revealing a gap in existing research, and/or
- Formulating a research question or problem, and/or
- Continuing a disciplinary tradition.
3. Place your research within the research niche by:
- Stating the intent of your study,
- Outlining the key characteristics of your study,
- Describing important results, and
- Giving a brief overview of the structure of the paper.
NOTE: It is often useful to review the introduction late in the writing process. This is appropriate because outcomes are unknown until you've completed the study. After you complete writing the body of the paper, go back and review introductory descriptions of the structure of the paper, the method of data gathering, the reporting and analysis of results, and the conclusion. Reviewing and, if necessary, rewriting the introduction ensures that it correctly matches the overall structure of your final paper.
II. Delimitations of the Study
Delimitations refer to those characteristics that limit the scope and define the conceptual boundaries of your research . This is determined by the conscious exclusionary and inclusionary decisions you make about how to investigate the research problem. In other words, not only should you tell the reader what it is you are studying and why, but you must also acknowledge why you rejected alternative approaches that could have been used to examine the topic.
Obviously, the first limiting step was the choice of research problem itself. However, implicit are other, related problems that could have been chosen but were rejected. These should be noted in the conclusion of your introduction. For example, a delimitating statement could read, "Although many factors can be understood to impact the likelihood young people will vote, this study will focus on socioeconomic factors related to the need to work full-time while in school." The point is not to document every possible delimiting factor, but to highlight why previously researched issues related to the topic were not addressed.
Examples of delimitating choices would be:
- The key aims and objectives of your study,
- The research questions that you address,
- The variables of interest [i.e., the various factors and features of the phenomenon being studied],
- The method(s) of investigation,
- The time period your study covers, and
- Any relevant alternative theoretical frameworks that could have been adopted.
Review each of these decisions. Not only do you clearly establish what you intend to accomplish in your research, but you should also include a declaration of what the study does not intend to cover. In the latter case, your exclusionary decisions should be based upon criteria understood as, "not interesting"; "not directly relevant"; “too problematic because..."; "not feasible," and the like. Make this reasoning explicit!
NOTE: Delimitations refer to the initial choices made about the broader, overall design of your study and should not be confused with documenting the limitations of your study discovered after the research has been completed.
ANOTHER NOTE : Do not view delimitating statements as admitting to an inherent failing or shortcoming in your research. They are an accepted element of academic writing intended to keep the reader focused on the research problem by explicitly defining the conceptual boundaries and scope of your study. It addresses any critical questions in the reader's mind of, "Why the hell didn't the author examine this?"
III. The Narrative Flow
Issues to keep in mind that will help the narrative flow in your introduction :
- Your introduction should clearly identify the subject area of interest . A simple strategy to follow is to use key words from your title in the first few sentences of the introduction. This will help focus the introduction on the topic at the appropriate level and ensures that you get to the subject matter quickly without losing focus, or discussing information that is too general.
- Establish context by providing a brief and balanced review of the pertinent published literature that is available on the subject. The key is to summarize for the reader what is known about the specific research problem before you did your analysis. This part of your introduction should not represent a comprehensive literature review--that comes next. It consists of a general review of the important, foundational research literature [with citations] that establishes a foundation for understanding key elements of the research problem. See the drop-down menu under this tab for " Background Information " regarding types of contexts.
- Clearly state the hypothesis that you investigated . When you are first learning to write in this format it is okay, and actually preferable, to use a past statement like, "The purpose of this study was to...." or "We investigated three possible mechanisms to explain the...."
- Why did you choose this kind of research study or design? Provide a clear statement of the rationale for your approach to the problem studied. This will usually follow your statement of purpose in the last paragraph of the introduction.
IV. Engaging the Reader
A research problem in the social sciences can come across as dry and uninteresting to anyone unfamiliar with the topic . Therefore, one of the goals of your introduction is to make readers want to read your paper. Here are several strategies you can use to grab the reader's attention:
- Open with a compelling story . Almost all research problems in the social sciences, no matter how obscure or esoteric , are really about the lives of people. Telling a story that humanizes an issue can help illuminate the significance of the problem and help the reader empathize with those affected by the condition being studied.
- Include a strong quotation or a vivid, perhaps unexpected, anecdote . During your review of the literature, make note of any quotes or anecdotes that grab your attention because they can used in your introduction to highlight the research problem in a captivating way.
- Pose a provocative or thought-provoking question . Your research problem should be framed by a set of questions to be addressed or hypotheses to be tested. However, a provocative question can be presented in the beginning of your introduction that challenges an existing assumption or compels the reader to consider an alternative viewpoint that helps establish the significance of your study.
- Describe a puzzling scenario or incongruity . This involves highlighting an interesting quandary concerning the research problem or describing contradictory findings from prior studies about a topic. Posing what is essentially an unresolved intellectual riddle about the problem can engage the reader's interest in the study.
- Cite a stirring example or case study that illustrates why the research problem is important . Draw upon the findings of others to demonstrate the significance of the problem and to describe how your study builds upon or offers alternatives ways of investigating this prior research.
NOTE: It is important that you choose only one of the suggested strategies for engaging your readers. This avoids giving an impression that your paper is more flash than substance and does not distract from the substance of your study.
Freedman, Leora and Jerry Plotnick. Introductions and Conclusions. University College Writing Centre. University of Toronto; Introduction. The Structure, Format, Content, and Style of a Journal-Style Scientific Paper. Department of Biology. Bates College; Introductions. The Writing Center. University of North Carolina; Introductions. The Writer’s Handbook. Writing Center. University of Wisconsin, Madison; Introductions, Body Paragraphs, and Conclusions for an Argument Paper. The Writing Lab and The OWL. Purdue University; “Writing Introductions.” In Good Essay Writing: A Social Sciences Guide . Peter Redman. 4th edition. (London: Sage, 2011), pp. 63-70; Resources for Writers: Introduction Strategies. Program in Writing and Humanistic Studies. Massachusetts Institute of Technology; Sharpling, Gerald. Writing an Introduction. Centre for Applied Linguistics, University of Warwick; Samraj, B. “Introductions in Research Articles: Variations Across Disciplines.” English for Specific Purposes 21 (2002): 1–17; Swales, John and Christine B. Feak. Academic Writing for Graduate Students: Essential Skills and Tasks . 2nd edition. Ann Arbor, MI: University of Michigan Press, 2004 ; Writing Your Introduction. Department of English Writing Guide. George Mason University.
Writing Tip
Avoid the "Dictionary" Introduction
Giving the dictionary definition of words related to the research problem may appear appropriate because it is important to define specific terminology that readers may be unfamiliar with. However, anyone can look a word up in the dictionary and a general dictionary is not a particularly authoritative source because it doesn't take into account the context of your topic and doesn't offer particularly detailed information. Also, placed in the context of a particular discipline, a term or concept may have a different meaning than what is found in a general dictionary. If you feel that you must seek out an authoritative definition, use a subject specific dictionary or encyclopedia [e.g., if you are a sociology student, search for dictionaries of sociology]. A good database for obtaining definitive definitions of concepts or terms is Credo Reference .
Saba, Robert. The College Research Paper. Florida International University; Introductions. The Writing Center. University of North Carolina.
Another Writing Tip
When Do I Begin?
A common question asked at the start of any paper is, "Where should I begin?" An equally important question to ask yourself is, "When do I begin?" Research problems in the social sciences rarely rest in isolation from history. Therefore, it is important to lay a foundation for understanding the historical context underpinning the research problem. However, this information should be brief and succinct and begin at a point in time that illustrates the study's overall importance. For example, a study that investigates coffee cultivation and export in West Africa as a key stimulus for local economic growth needs to describe the beginning of exporting coffee in the region and establishing why economic growth is important. You do not need to give a long historical explanation about coffee exports in Africa. If a research problem requires a substantial exploration of the historical context, do this in the literature review section. In your introduction, make note of this as part of the "roadmap" [see below] that you use to describe the organization of your paper.
Introductions. The Writing Center. University of North Carolina; “Writing Introductions.” In Good Essay Writing: A Social Sciences Guide . Peter Redman. 4th edition. (London: Sage, 2011), pp. 63-70.
Yet Another Writing Tip
Always End with a Roadmap
The final paragraph or sentences of your introduction should forecast your main arguments and conclusions and provide a brief description of the rest of the paper [the "roadmap"] that let's the reader know where you are going and what to expect. A roadmap is important because it helps the reader place the research problem within the context of their own perspectives about the topic. In addition, concluding your introduction with an explicit roadmap tells the reader that you have a clear understanding of the structural purpose of your paper. In this way, the roadmap acts as a type of promise to yourself and to your readers that you will follow a consistent and coherent approach to addressing the topic of inquiry. Refer to it often to help keep your writing focused and organized.
Cassuto, Leonard. “On the Dissertation: How to Write the Introduction.” The Chronicle of Higher Education , May 28, 2018; Radich, Michael. A Student's Guide to Writing in East Asian Studies . (Cambridge, MA: Harvard University Writing n. d.), pp. 35-37.
- << Previous: Executive Summary
- Next: The C.A.R.S. Model >>
- Last Updated: Apr 5, 2024 1:38 PM
- URL: https://libguides.usc.edu/writingguide
- Privacy Policy
Buy Me a Coffee

Home » Research Paper – Structure, Examples and Writing Guide
Research Paper – Structure, Examples and Writing Guide
Table of Contents

Research Paper
Definition:
Research Paper is a written document that presents the author’s original research, analysis, and interpretation of a specific topic or issue.
It is typically based on Empirical Evidence, and may involve qualitative or quantitative research methods, or a combination of both. The purpose of a research paper is to contribute new knowledge or insights to a particular field of study, and to demonstrate the author’s understanding of the existing literature and theories related to the topic.
Structure of Research Paper
The structure of a research paper typically follows a standard format, consisting of several sections that convey specific information about the research study. The following is a detailed explanation of the structure of a research paper:
The title page contains the title of the paper, the name(s) of the author(s), and the affiliation(s) of the author(s). It also includes the date of submission and possibly, the name of the journal or conference where the paper is to be published.
The abstract is a brief summary of the research paper, typically ranging from 100 to 250 words. It should include the research question, the methods used, the key findings, and the implications of the results. The abstract should be written in a concise and clear manner to allow readers to quickly grasp the essence of the research.
Introduction
The introduction section of a research paper provides background information about the research problem, the research question, and the research objectives. It also outlines the significance of the research, the research gap that it aims to fill, and the approach taken to address the research question. Finally, the introduction section ends with a clear statement of the research hypothesis or research question.
Literature Review
The literature review section of a research paper provides an overview of the existing literature on the topic of study. It includes a critical analysis and synthesis of the literature, highlighting the key concepts, themes, and debates. The literature review should also demonstrate the research gap and how the current study seeks to address it.
The methods section of a research paper describes the research design, the sample selection, the data collection and analysis procedures, and the statistical methods used to analyze the data. This section should provide sufficient detail for other researchers to replicate the study.
The results section presents the findings of the research, using tables, graphs, and figures to illustrate the data. The findings should be presented in a clear and concise manner, with reference to the research question and hypothesis.
The discussion section of a research paper interprets the findings and discusses their implications for the research question, the literature review, and the field of study. It should also address the limitations of the study and suggest future research directions.
The conclusion section summarizes the main findings of the study, restates the research question and hypothesis, and provides a final reflection on the significance of the research.
The references section provides a list of all the sources cited in the paper, following a specific citation style such as APA, MLA or Chicago.
How to Write Research Paper
You can write Research Paper by the following guide:
- Choose a Topic: The first step is to select a topic that interests you and is relevant to your field of study. Brainstorm ideas and narrow down to a research question that is specific and researchable.
- Conduct a Literature Review: The literature review helps you identify the gap in the existing research and provides a basis for your research question. It also helps you to develop a theoretical framework and research hypothesis.
- Develop a Thesis Statement : The thesis statement is the main argument of your research paper. It should be clear, concise and specific to your research question.
- Plan your Research: Develop a research plan that outlines the methods, data sources, and data analysis procedures. This will help you to collect and analyze data effectively.
- Collect and Analyze Data: Collect data using various methods such as surveys, interviews, observations, or experiments. Analyze data using statistical tools or other qualitative methods.
- Organize your Paper : Organize your paper into sections such as Introduction, Literature Review, Methods, Results, Discussion, and Conclusion. Ensure that each section is coherent and follows a logical flow.
- Write your Paper : Start by writing the introduction, followed by the literature review, methods, results, discussion, and conclusion. Ensure that your writing is clear, concise, and follows the required formatting and citation styles.
- Edit and Proofread your Paper: Review your paper for grammar and spelling errors, and ensure that it is well-structured and easy to read. Ask someone else to review your paper to get feedback and suggestions for improvement.
- Cite your Sources: Ensure that you properly cite all sources used in your research paper. This is essential for giving credit to the original authors and avoiding plagiarism.
Research Paper Example
Note : The below example research paper is for illustrative purposes only and is not an actual research paper. Actual research papers may have different structures, contents, and formats depending on the field of study, research question, data collection and analysis methods, and other factors. Students should always consult with their professors or supervisors for specific guidelines and expectations for their research papers.
Research Paper Example sample for Students:
Title: The Impact of Social Media on Mental Health among Young Adults
Abstract: This study aims to investigate the impact of social media use on the mental health of young adults. A literature review was conducted to examine the existing research on the topic. A survey was then administered to 200 university students to collect data on their social media use, mental health status, and perceived impact of social media on their mental health. The results showed that social media use is positively associated with depression, anxiety, and stress. The study also found that social comparison, cyberbullying, and FOMO (Fear of Missing Out) are significant predictors of mental health problems among young adults.
Introduction: Social media has become an integral part of modern life, particularly among young adults. While social media has many benefits, including increased communication and social connectivity, it has also been associated with negative outcomes, such as addiction, cyberbullying, and mental health problems. This study aims to investigate the impact of social media use on the mental health of young adults.
Literature Review: The literature review highlights the existing research on the impact of social media use on mental health. The review shows that social media use is associated with depression, anxiety, stress, and other mental health problems. The review also identifies the factors that contribute to the negative impact of social media, including social comparison, cyberbullying, and FOMO.
Methods : A survey was administered to 200 university students to collect data on their social media use, mental health status, and perceived impact of social media on their mental health. The survey included questions on social media use, mental health status (measured using the DASS-21), and perceived impact of social media on their mental health. Data were analyzed using descriptive statistics and regression analysis.
Results : The results showed that social media use is positively associated with depression, anxiety, and stress. The study also found that social comparison, cyberbullying, and FOMO are significant predictors of mental health problems among young adults.
Discussion : The study’s findings suggest that social media use has a negative impact on the mental health of young adults. The study highlights the need for interventions that address the factors contributing to the negative impact of social media, such as social comparison, cyberbullying, and FOMO.
Conclusion : In conclusion, social media use has a significant impact on the mental health of young adults. The study’s findings underscore the need for interventions that promote healthy social media use and address the negative outcomes associated with social media use. Future research can explore the effectiveness of interventions aimed at reducing the negative impact of social media on mental health. Additionally, longitudinal studies can investigate the long-term effects of social media use on mental health.
Limitations : The study has some limitations, including the use of self-report measures and a cross-sectional design. The use of self-report measures may result in biased responses, and a cross-sectional design limits the ability to establish causality.
Implications: The study’s findings have implications for mental health professionals, educators, and policymakers. Mental health professionals can use the findings to develop interventions that address the negative impact of social media use on mental health. Educators can incorporate social media literacy into their curriculum to promote healthy social media use among young adults. Policymakers can use the findings to develop policies that protect young adults from the negative outcomes associated with social media use.
References :
- Twenge, J. M., & Campbell, W. K. (2019). Associations between screen time and lower psychological well-being among children and adolescents: Evidence from a population-based study. Preventive medicine reports, 15, 100918.
- Primack, B. A., Shensa, A., Escobar-Viera, C. G., Barrett, E. L., Sidani, J. E., Colditz, J. B., … & James, A. E. (2017). Use of multiple social media platforms and symptoms of depression and anxiety: A nationally-representative study among US young adults. Computers in Human Behavior, 69, 1-9.
- Van der Meer, T. G., & Verhoeven, J. W. (2017). Social media and its impact on academic performance of students. Journal of Information Technology Education: Research, 16, 383-398.
Appendix : The survey used in this study is provided below.
Social Media and Mental Health Survey
- How often do you use social media per day?
- Less than 30 minutes
- 30 minutes to 1 hour
- 1 to 2 hours
- 2 to 4 hours
- More than 4 hours
- Which social media platforms do you use?
- Others (Please specify)
- How often do you experience the following on social media?
- Social comparison (comparing yourself to others)
- Cyberbullying
- Fear of Missing Out (FOMO)
- Have you ever experienced any of the following mental health problems in the past month?
- Do you think social media use has a positive or negative impact on your mental health?
- Very positive
- Somewhat positive
- Somewhat negative
- Very negative
- In your opinion, which factors contribute to the negative impact of social media on mental health?
- Social comparison
- In your opinion, what interventions could be effective in reducing the negative impact of social media on mental health?
- Education on healthy social media use
- Counseling for mental health problems caused by social media
- Social media detox programs
- Regulation of social media use
Thank you for your participation!
Applications of Research Paper
Research papers have several applications in various fields, including:
- Advancing knowledge: Research papers contribute to the advancement of knowledge by generating new insights, theories, and findings that can inform future research and practice. They help to answer important questions, clarify existing knowledge, and identify areas that require further investigation.
- Informing policy: Research papers can inform policy decisions by providing evidence-based recommendations for policymakers. They can help to identify gaps in current policies, evaluate the effectiveness of interventions, and inform the development of new policies and regulations.
- Improving practice: Research papers can improve practice by providing evidence-based guidance for professionals in various fields, including medicine, education, business, and psychology. They can inform the development of best practices, guidelines, and standards of care that can improve outcomes for individuals and organizations.
- Educating students : Research papers are often used as teaching tools in universities and colleges to educate students about research methods, data analysis, and academic writing. They help students to develop critical thinking skills, research skills, and communication skills that are essential for success in many careers.
- Fostering collaboration: Research papers can foster collaboration among researchers, practitioners, and policymakers by providing a platform for sharing knowledge and ideas. They can facilitate interdisciplinary collaborations and partnerships that can lead to innovative solutions to complex problems.
When to Write Research Paper
Research papers are typically written when a person has completed a research project or when they have conducted a study and have obtained data or findings that they want to share with the academic or professional community. Research papers are usually written in academic settings, such as universities, but they can also be written in professional settings, such as research organizations, government agencies, or private companies.
Here are some common situations where a person might need to write a research paper:
- For academic purposes: Students in universities and colleges are often required to write research papers as part of their coursework, particularly in the social sciences, natural sciences, and humanities. Writing research papers helps students to develop research skills, critical thinking skills, and academic writing skills.
- For publication: Researchers often write research papers to publish their findings in academic journals or to present their work at academic conferences. Publishing research papers is an important way to disseminate research findings to the academic community and to establish oneself as an expert in a particular field.
- To inform policy or practice : Researchers may write research papers to inform policy decisions or to improve practice in various fields. Research findings can be used to inform the development of policies, guidelines, and best practices that can improve outcomes for individuals and organizations.
- To share new insights or ideas: Researchers may write research papers to share new insights or ideas with the academic or professional community. They may present new theories, propose new research methods, or challenge existing paradigms in their field.
Purpose of Research Paper
The purpose of a research paper is to present the results of a study or investigation in a clear, concise, and structured manner. Research papers are written to communicate new knowledge, ideas, or findings to a specific audience, such as researchers, scholars, practitioners, or policymakers. The primary purposes of a research paper are:
- To contribute to the body of knowledge : Research papers aim to add new knowledge or insights to a particular field or discipline. They do this by reporting the results of empirical studies, reviewing and synthesizing existing literature, proposing new theories, or providing new perspectives on a topic.
- To inform or persuade: Research papers are written to inform or persuade the reader about a particular issue, topic, or phenomenon. They present evidence and arguments to support their claims and seek to persuade the reader of the validity of their findings or recommendations.
- To advance the field: Research papers seek to advance the field or discipline by identifying gaps in knowledge, proposing new research questions or approaches, or challenging existing assumptions or paradigms. They aim to contribute to ongoing debates and discussions within a field and to stimulate further research and inquiry.
- To demonstrate research skills: Research papers demonstrate the author’s research skills, including their ability to design and conduct a study, collect and analyze data, and interpret and communicate findings. They also demonstrate the author’s ability to critically evaluate existing literature, synthesize information from multiple sources, and write in a clear and structured manner.
Characteristics of Research Paper
Research papers have several characteristics that distinguish them from other forms of academic or professional writing. Here are some common characteristics of research papers:
- Evidence-based: Research papers are based on empirical evidence, which is collected through rigorous research methods such as experiments, surveys, observations, or interviews. They rely on objective data and facts to support their claims and conclusions.
- Structured and organized: Research papers have a clear and logical structure, with sections such as introduction, literature review, methods, results, discussion, and conclusion. They are organized in a way that helps the reader to follow the argument and understand the findings.
- Formal and objective: Research papers are written in a formal and objective tone, with an emphasis on clarity, precision, and accuracy. They avoid subjective language or personal opinions and instead rely on objective data and analysis to support their arguments.
- Citations and references: Research papers include citations and references to acknowledge the sources of information and ideas used in the paper. They use a specific citation style, such as APA, MLA, or Chicago, to ensure consistency and accuracy.
- Peer-reviewed: Research papers are often peer-reviewed, which means they are evaluated by other experts in the field before they are published. Peer-review ensures that the research is of high quality, meets ethical standards, and contributes to the advancement of knowledge in the field.
- Objective and unbiased: Research papers strive to be objective and unbiased in their presentation of the findings. They avoid personal biases or preconceptions and instead rely on the data and analysis to draw conclusions.
Advantages of Research Paper
Research papers have many advantages, both for the individual researcher and for the broader academic and professional community. Here are some advantages of research papers:
- Contribution to knowledge: Research papers contribute to the body of knowledge in a particular field or discipline. They add new information, insights, and perspectives to existing literature and help advance the understanding of a particular phenomenon or issue.
- Opportunity for intellectual growth: Research papers provide an opportunity for intellectual growth for the researcher. They require critical thinking, problem-solving, and creativity, which can help develop the researcher’s skills and knowledge.
- Career advancement: Research papers can help advance the researcher’s career by demonstrating their expertise and contributions to the field. They can also lead to new research opportunities, collaborations, and funding.
- Academic recognition: Research papers can lead to academic recognition in the form of awards, grants, or invitations to speak at conferences or events. They can also contribute to the researcher’s reputation and standing in the field.
- Impact on policy and practice: Research papers can have a significant impact on policy and practice. They can inform policy decisions, guide practice, and lead to changes in laws, regulations, or procedures.
- Advancement of society: Research papers can contribute to the advancement of society by addressing important issues, identifying solutions to problems, and promoting social justice and equality.
Limitations of Research Paper
Research papers also have some limitations that should be considered when interpreting their findings or implications. Here are some common limitations of research papers:
- Limited generalizability: Research findings may not be generalizable to other populations, settings, or contexts. Studies often use specific samples or conditions that may not reflect the broader population or real-world situations.
- Potential for bias : Research papers may be biased due to factors such as sample selection, measurement errors, or researcher biases. It is important to evaluate the quality of the research design and methods used to ensure that the findings are valid and reliable.
- Ethical concerns: Research papers may raise ethical concerns, such as the use of vulnerable populations or invasive procedures. Researchers must adhere to ethical guidelines and obtain informed consent from participants to ensure that the research is conducted in a responsible and respectful manner.
- Limitations of methodology: Research papers may be limited by the methodology used to collect and analyze data. For example, certain research methods may not capture the complexity or nuance of a particular phenomenon, or may not be appropriate for certain research questions.
- Publication bias: Research papers may be subject to publication bias, where positive or significant findings are more likely to be published than negative or non-significant findings. This can skew the overall findings of a particular area of research.
- Time and resource constraints: Research papers may be limited by time and resource constraints, which can affect the quality and scope of the research. Researchers may not have access to certain data or resources, or may be unable to conduct long-term studies due to practical limitations.
About the author
Muhammad Hassan
Researcher, Academic Writer, Web developer
You may also like

How to Cite Research Paper – All Formats and...

Data Collection – Methods Types and Examples

Delimitations in Research – Types, Examples and...

Research Paper Format – Types, Examples and...

Research Process – Steps, Examples and Tips

Research Design – Types, Methods and Examples
How to Write an Introduction for a Research Paper
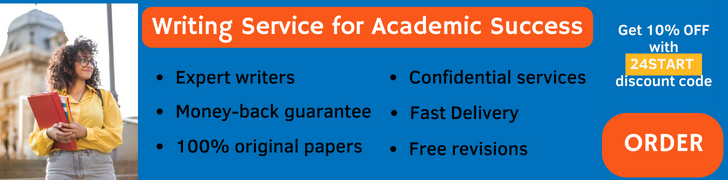
How to write an introduction for a research paper? Eventually (and with practice) all writers will develop their own strategy for writing the perfect introduction for a research paper. Once you are comfortable with writing, you will probably find your own, but coming up with a good strategy can be tough for beginning writers.
The Purpose of an Introduction
Your opening paragraphs, phrases for introducing thesis statements, research paper introduction examples, using the introduction to map out your research paper.
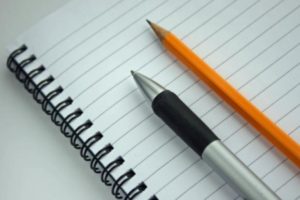
Academic Writing, Editing, Proofreading, And Problem Solving Services
Get 10% off with 24start discount code.
- First write your thesis.Your thesis should state the main idea in specific terms.
- After you have a working thesis, tackle the body of your paper before you write the rest of the introduction. Each paragraph in the body should explore one specific topic that proves, or summarizes your thesis. Writing is a thinking process. Once you have worked your way through that process by writing the body of the paper, you will have an intimate understanding of how you are supporting your thesis. After you have written the body paragraphs, go back and rewrite your thesis to make it more specific and to connect it to the topics you addressed in the body paragraph.
- Revise your introduction several times, saving each revision. Be sure your introduction previews the topics you are presenting in your paper. One way of doing this is to use keywords from the topic sentences in each paragraph to introduce, or preview, the topics in your introduction.This “preview” will give your reader a context for understanding how you will make your case.
- Experiment by taking different approaches to your thesis with every revision you make. Play with the language in the introduction. Strike a new tone. Go back and compare versions. Then pick the one that works most effectively with the body of your research paper.
- Do not try to pack everything you want to say into your introduction. Just as your introduction should not be too short, it should also not be too long. Your introduction should be about the same length as any other paragraph in your research paper. Let the content—what you have to say—dictate the length.
The first page of your research paper should draw the reader into the text. It is the paper’s most important page and, alas, often the worst written. There are two culprits here and effective ways to cope with both of them.
First, the writer is usually straining too hard to say something terribly BIG and IMPORTANT about the thesis topic. The goal is worthy, but the aim is unrealistically high. The result is often a muddle of vague platitudes rather than a crisp, compelling introduction to the thesis. Want a familiar example? Listen to most graduation speakers. Their goal couldn’t be loftier: to say what education means and to tell an entire football stadium how to live the rest of their lives. The results are usually an avalanche of clichés and sodden prose.
The second culprit is bad timing. The opening and concluding paragraphs are usually written late in the game, after the rest of the thesis is finished and polished. There’s nothing wrong with writing these sections last. It’s usually the right approach since you need to know exactly what you are saying in the substantive middle sections of the thesis before you can introduce them effectively or draw together your findings. But having waited to write the opening and closing sections, you need to review and edit them several times to catch up. Otherwise, you’ll putting the most jagged prose in the most tender spots. Edit and polish your opening paragraphs with extra care. They should draw readers into the paper.
After you’ve done some extra polishing, I suggest a simple test for the introductory section. As an experiment, chop off the first few paragraphs. Let the paper begin on, say, paragraph 2 or even page 2. If you don’t lose much, or actually gain in clarity and pace, then you’ve got a problem.
There are two solutions. One is to start at this new spot, further into the text. After all, that’s where you finally gain traction on your subject. That works best in some cases, and we occasionally suggest it. The alternative, of course, is to write a new opening that doesn’t flop around, saying nothing.
What makes a good opening? Actually, they come in several flavors. One is an intriguing story about your topic. Another is a brief, compelling quote. When you run across them during your reading, set them aside for later use. Don’t be deterred from using them because they “don’t seem academic enough.” They’re fine as long as the rest of the paper doesn’t sound like you did your research in People magazine. The third, and most common, way to begin is by stating your main questions, followed by a brief comment about why they matter.
Whichever opening you choose, it should engage your readers and coax them to continue. Having done that, you should give them a general overview of the project—the main issues you will cover, the material you will use, and your thesis statement (that is, your basic approach to the topic). Finally, at the end of the introductory section, give your readers a brief road map, showing how the paper will unfold. How you do that depends on your topic but here are some general suggestions for phrase choice that may help:
- This analysis will provide …
- This paper analyzes the relationship between …
- This paper presents an analysis of …
- This paper will argue that …
- This topic supports the argument that…
- Research supports the opinion that …
- This paper supports the opinion that …
- An interpretation of the facts indicates …
- The results of this experiment show …
- The results of this research show …
Comparisons/Contrasts
- A comparison will show that …
- By contrasting the results,we see that …
- This paper examines the advantages and disadvantages of …
Definitions/Classifications
- This paper will provide a guide for categorizing the following:…
- This paper provides a definition of …
- This paper explores the meaning of …
- This paper will discuss the implications of …
- A discussion of this topic reveals …
- The following discussion will focus on …
Description
- This report describes…
- This report will illustrate…
- This paper provides an illustration of …
Process/Experimentation
- This paper will identify the reasons behind…
- The results of the experiment show …
- The process revealed that …
- This paper theorizes…
- This paper presents the theory that …
- In theory, this indicates that …
Quotes, anecdotes, questions, examples, and broad statements—all of them can used successfully to write an introduction for a research paper. It’s instructive to see them in action, in the hands of skilled academic writers.
Let’s begin with David M. Kennedy’s superb history, Freedom from Fear: The American People in Depression and War, 1929–1945 . Kennedy begins each chapter with a quote, followed by his text. The quote above chapter 1 shows President Hoover speaking in 1928 about America’s golden future. The text below it begins with the stock market collapse of 1929. It is a riveting account of just how wrong Hoover was. The text about the Depression is stronger because it contrasts so starkly with the optimistic quotation.
“We in America today are nearer the final triumph over poverty than ever before in the history of any land.”—Herbert Hoover, August 11, 1928 Like an earthquake, the stock market crash of October 1929 cracked startlingly across the United States, the herald of a crisis that was to shake the American way of life to its foundations. The events of the ensuing decade opened a fissure across the landscape of American history no less gaping than that opened by the volley on Lexington Common in April 1775 or by the bombardment of Sumter on another April four score and six years later. The ratcheting ticker machines in the autumn of 1929 did not merely record avalanching stock prices. In time they came also to symbolize the end of an era. (David M. Kennedy, Freedom from Fear: The American People in Depression and War, 1929–1945 . New York: Oxford University Press, 1999, p. 10)
Kennedy has exciting, wrenching material to work with. John Mueller faces the exact opposite problem. In Retreat from Doomsday: The Obsolescence of Major War , he is trying to explain why Great Powers have suddenly stopped fighting each other. For centuries they made war on each other with devastating regularity, killing millions in the process. But now, Mueller thinks, they have not just paused; they have stopped permanently. He is literally trying to explain why “nothing is happening now.” That may be an exciting topic intellectually, it may have great practical significance, but “nothing happened” is not a very promising subject for an exciting opening paragraph. Mueller manages to make it exciting and, at the same time, shows why it matters so much. Here’s his opening, aptly entitled “History’s Greatest Nonevent”:
On May 15, 1984, the major countries of the developed world had managed to remain at peace with each other for the longest continuous stretch of time since the days of the Roman Empire. If a significant battle in a war had been fought on that day, the press would have bristled with it. As usual, however, a landmark crossing in the history of peace caused no stir: the most prominent story in the New York Times that day concerned the saga of a manicurist, a machinist, and a cleaning woman who had just won a big Lotto contest. This book seeks to develop an explanation for what is probably the greatest nonevent in human history. (John Mueller, Retreat from Doomsday: The Obsolescence of Major War . New York: Basic Books, 1989, p. 3)
In the space of a few sentences, Mueller sets up his puzzle and reveals its profound human significance. At the same time, he shows just how easy it is to miss this milestone in the buzz of daily events. Notice how concretely he does that. He doesn’t just say that the New York Times ignored this record setting peace. He offers telling details about what they covered instead: “a manicurist, a machinist, and a cleaning woman who had just won a big Lotto contest.” Likewise, David Kennedy immediately entangles us in concrete events: the stunning stock market crash of 1929. These are powerful openings that capture readers’ interests, establish puzzles, and launch narratives.
Sociologist James Coleman begins in a completely different way, by posing the basic questions he will study. His ambitious book, Foundations of Social Theory , develops a comprehensive theory of social life, so it is entirely appropriate for him to begin with some major questions. But he could just as easily have begun with a compelling story or anecdote. He includes many of them elsewhere in his book. His choice for the opening, though, is to state his major themes plainly and frame them as a paradox. Sociologists, he says, are interested in aggregate behavior—how people act in groups, organizations, or large numbers—yet they mostly examine individuals:
A central problem in social science is that of accounting for the function of some kind of social system. Yet in most social research, observations are not made on the system as a whole, but on some part of it. In fact, the natural unit of observation is the individual person… This has led to a widening gap between theory and research… (James S. Coleman, Foundations of Social Theory . Cambridge, MA: Harvard University Press, 1990, pp. 1–2)
After expanding on this point, Coleman explains that he will not try to remedy the problem by looking solely at groups or aggregate-level data. That’s a false solution, he says, because aggregates don’t act; individuals do. So the real problem is to show the links between individual actions and aggregate outcomes, between the micro and the macro.
The major problem for explanations of system behavior based on actions and orientations at a level below that of the system [in this case, on individual-level actions] is that of moving from the lower level to the system level. This has been called the micro-to-macro problem, and it is pervasive throughout the social sciences. (Coleman, Foundations of Social Theory , p. 6)
Explaining how to deal with this “micro-to-macro problem” is the central issue of Coleman’s book, and he announces it at the beginning.
Coleman’s theory-driven opening stands at the opposite end of the spectrum from engaging stories or anecdotes, which are designed to lure the reader into the narrative and ease the path to a more analytic treatment later in the text. Take, for example, the opening sentences of Robert L. Herbert’s sweeping study Impressionism: Art, Leisure, and Parisian Society : “When Henry Tuckerman came to Paris in 1867, one of the thousands of Americans attracted there by the huge international exposition, he was bowled over by the extraordinary changes since his previous visit twenty years before.” (Robert L. Herbert, Impressionism: Art, Leisure, and Parisian Society . New Haven, CT: Yale University Press, 1988, p. 1.) Herbert fills in the evocative details to set the stage for his analysis of the emerging Impressionist art movement and its connection to Parisian society and leisure in this period.
David Bromwich writes about Wordsworth, a poet so familiar to students of English literature that it is hard to see him afresh, before his great achievements, when he was just a young outsider starting to write. To draw us into Wordsworth’s early work, Bromwich wants us to set aside our entrenched images of the famous mature poet and see him as he was in the 1790s, as a beginning writer on the margins of society. He accomplishes this ambitious task in the opening sentences of Disowned by Memory: Wordsworth’s Poetry of the 1790s :
Wordsworth turned to poetry after the revolution to remind himself that he was still a human being. It was a curious solution, to a difficulty many would not have felt. The whole interest of his predicament is that he did feel it. Yet Wordsworth is now so established an eminence—his name so firmly fixed with readers as a moralist of self-trust emanating from complete self-security—that it may seem perverse to imagine him as a criminal seeking expiation. Still, that is a picture we get from The Borderers and, at a longer distance, from “Tintern Abbey.” (David Bromwich, Disowned by Memory: Wordsworth’s Poetry of the 1790s . Chicago: University of Chicago Press, 1998, p. 1)
That’s a wonderful opening! Look at how much Bromwich accomplishes in just a few words. He not only prepares the way for analyzing Wordsworth’s early poetry; he juxtaposes the anguished young man who wrote it to the self-confident, distinguished figure he became—the eminent man we can’t help remembering as we read his early poetry.
Let us highlight a couple of other points in this passage because they illustrate some intelligent writing choices. First, look at the odd comma in this sentence: “It was a curious solution, to a difficulty many would not have felt.” Any standard grammar book would say that comma is wrong and should be omitted. Why did Bromwich insert it? Because he’s a fine writer, thinking of his sentence rhythm and the point he wants to make. The comma does exactly what it should. It makes us pause, breaking the sentence into two parts, each with an interesting point. One is that Wordsworth felt a difficulty others would not have; the other is that he solved it in a distinctive way. It would be easy for readers to glide over this double message, so Bromwich has inserted a speed bump to slow us down. Most of the time, you should follow grammatical rules, like those about commas, but you should bend them when it serves a good purpose. That’s what the writer does here.
The second small point is the phrase “after the revolution” in the first sentence: “Wordsworth turned to poetry after the revolution to remind himself that he was still a human being.” Why doesn’t Bromwich say “after the French Revolution”? Because he has judged his book’s audience. He is writing for specialists who already know which revolution is reverberating through English life in the 1790s. It is the French Revolution, not the earlier loss of the American colonies. If Bromwich were writing for a much broader audience—say, the New York Times Book Review—he would probably insert the extra word to avoid confusion.
The message “Know your audience” applies to all writers. Don’t talk down to them by assuming they can’t get dressed in the morning. Don’t strut around showing off your book learnin’ by tossing in arcane facts and esoteric language for its own sake. Neither will win over readers.
Bromwich, Herbert, and Coleman open their works in different ways, but their choices work well for their different texts. Your task is to decide what kind of opening will work best for yours. Don’t let that happen by default, by grabbing the first idea you happen upon. Consider a couple of different ways of opening your thesis and then choose the one you prefer. Give yourself some options, think them over, then make an informed choice.
Whether you begin with a story, puzzle, or broad statement, the next part of the introduction should pose your main questions and establish your argument. This is your thesis statement—your viewpoint along with the supporting reasons and evidence. It should be articulated plainly so readers understand full well what your paper is about and what it will argue.
After that, give your readers a road map of what’s to come. That’s normally done at the end of the introductory section (or, in a book, at the end of the introductory chapter). Here’s John J. Mearsheimer presenting such a road map in The Tragedy of Great Power Politics . He not only tells us the order of upcoming chapters, he explains why he’s chosen that order and which chapters are most important:
The Plan of the Book The rest of the chapters in this book are concerned mainly with answering the six big questions about power which I identified earlier. Chapter 2, which is probably the most important chapter in the book, lays out my theory of why states compete for power and why they pursue hegemony. In Chapters 3 and 4, I define power and explain how to measure it. I do this in order to lay the groundwork for testing my theory… (John J. Mearsheimer, The Tragedy of Great Power Politics . New York: W. W. Norton, 2001, p. 27)
As this excerpt makes clear, Mearsheimer has already laid out his “six big questions” in the introduction. Now he’s showing us the path ahead, the path to answering those questions.
At the end of the introduction, give your readers a road map of what’s to come. Tell them what the upcoming sections will be and why they are arranged in this particular order.
After having written your introduction it’s time to move to the biggest part: body of a research paper.
Back to How To Write A Research Paper .
ORDER HIGH QUALITY CUSTOM PAPER

- Resources Home 🏠
- Try SciSpace Copilot
- Search research papers
- Add Copilot Extension
- Try AI Detector
- Try Paraphraser
- Try Citation Generator
- April Papers
- June Papers
- July Papers

How to Write an Introduction for a Research Paper
Table of Contents
Writing an introduction for a research paper is a critical element of your paper, but it can seem challenging to encapsulate enormous amount of information into a concise form. The introduction of your research paper sets the tone for your research and provides the context for your study. In this article, we will guide you through the process of writing an effective introduction that grabs the reader's attention and captures the essence of your research paper.
Understanding the Purpose of a Research Paper Introduction
The introduction acts as a road map for your research paper, guiding the reader through the main ideas and arguments. The purpose of the introduction is to present your research topic to the readers and provide a rationale for why your study is relevant. It helps the reader locate your research and its relevance in the broader field of related scientific explorations. Additionally, the introduction should inform the reader about the objectives and scope of your study, giving them an overview of what to expect in the paper. By including a comprehensive introduction, you establish your credibility as an author and convince the reader that your research is worth their time and attention.
Key Elements to Include in Your Introduction
When writing your research paper introduction, there are several key elements you should include to ensure it is comprehensive and informative.
- A hook or attention-grabbing statement to capture the reader's interest. It can be a thought-provoking question, a surprising statistic, or a compelling anecdote that relates to your research topic.
- A brief overview of the research topic and its significance. By highlighting the gap in existing knowledge or the problem your research aims to address, you create a compelling case for the relevance of your study.
- A clear research question or problem statement. This serves as the foundation of your research and guides the reader in understanding the unique focus of your study. It should be concise, specific, and clearly articulated.
- An outline of the paper's structure and main arguments, to help the readers navigate through the paper with ease.
Preparing to Write Your Introduction
Before diving into writing your introduction, it is essential to prepare adequately. This involves 3 important steps:
- Conducting Preliminary Research: Immerse yourself in the existing literature to develop a clear research question and position your study within the academic discourse.
- Identifying Your Thesis Statement: Define a specific, focused, and debatable thesis statement, serving as a roadmap for your paper.
- Considering Broader Context: Reflect on the significance of your research within your field, understanding its potential impact and contribution.
By engaging in these preparatory steps, you can ensure that your introduction is well-informed, focused, and sets the stage for a compelling research paper.
Structuring Your Introduction
Now that you have prepared yourself to tackle the introduction, it's time to structure it effectively. A well-structured introduction will engage the reader from the beginning and provide a logical flow to your research paper.
Starting with a Hook
Begin your introduction with an attention-grabbing hook that captivates the reader's interest. This hook serves as a way to make your introduction more engaging and compelling. For example, if you are writing a research paper on the impact of climate change on biodiversity, you could start your introduction with a statistic about the number of species that have gone extinct due to climate change. This will immediately grab the reader's attention and make them realize the urgency and importance of the topic.
Introducing Your Topic
Provide a brief overview, which should give the reader a general understanding of the subject matter and its significance. Explain the importance of the topic and its relevance to the field. This will help the reader understand why your research is significant and why they should continue reading. Continuing with the example of climate change and biodiversity, you could explain how climate change is one of the greatest threats to global biodiversity, how it affects ecosystems, and the potential consequences for both wildlife and human populations. By providing this context, you are setting the stage for the rest of your research paper and helping the reader understand the importance of your study.
Presenting Your Thesis Statement
The thesis statement should directly address your research question and provide a preview of the main arguments or findings discussed in your paper. Make sure your thesis statement is clear, concise, and well-supported by the evidence you will present in your research paper. By presenting a strong and focused thesis statement, you are providing the reader with the information they could anticipate in your research paper. This will help them understand the purpose and scope of your study and will make them more inclined to continue reading.
Writing Techniques for an Effective Introduction
When crafting an introduction, it is crucial to pay attention to the finer details that can elevate your writing to the next level. By utilizing specific writing techniques, you can captivate your readers and draw them into your research journey.
Using Clear and Concise Language
One of the most important writing techniques to employ in your introduction is the use of clear and concise language. By choosing your words carefully, you can effectively convey your ideas to the reader. It is essential to avoid using jargon or complex terminology that may confuse or alienate your audience. Instead, focus on communicating your research in a straightforward manner to ensure that your introduction is accessible to both experts in your field and those who may be new to the topic. This approach allows you to engage a broader audience and make your research more inclusive.
Establishing the Relevance of Your Research
One way to establish the relevance of your research is by highlighting how it fills a gap in the existing literature. Explain how your study addresses a significant research question that has not been adequately explored. By doing this, you demonstrate that your research is not only unique but also contributes to the broader knowledge in your field. Furthermore, it is important to emphasize the potential impact of your research. Whether it is advancing scientific understanding, informing policy decisions, or improving practical applications, make it clear to the reader how your study can make a difference.
By employing these two writing techniques in your introduction, you can effectively engage your readers. Take your time to craft an introduction that is both informative and captivating, leaving your readers eager to delve deeper into your research.
Revising and Polishing Your Introduction
Once you have written your introduction, it is crucial to revise and polish it to ensure that it effectively sets the stage for your research paper.
Self-Editing Techniques
Review your introduction for clarity, coherence, and logical flow. Ensure each paragraph introduces a new idea or argument with smooth transitions.
Check for grammatical errors, spelling mistakes, and awkward sentence structures.
Ensure that your introduction aligns with the overall tone and style of your research paper.
Seeking Feedback for Improvement
Consider seeking feedback from peers, colleagues, or your instructor. They can provide valuable insights and suggestions for improving your introduction. Be open to constructive criticism and use it to refine your introduction and make it more compelling for the reader.
Writing an introduction for a research paper requires careful thought and planning. By understanding the purpose of the introduction, preparing adequately, structuring effectively, and employing writing techniques, you can create an engaging and informative introduction for your research. Remember to revise and polish your introduction to ensure that it accurately represents the main ideas and arguments in your research paper. With a well-crafted introduction, you will capture the reader's attention and keep them inclined to your paper.
Suggested Reads
ResearchGPT: A Custom GPT for Researchers and Scientists Best Academic Search Engines [2023] How To Humanize AI Text In Scientific Articles Elevate Your Writing Game With AI Grammar Checker Tools
You might also like

AI for Meta Analysis — A Comprehensive Guide
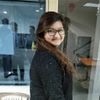
Cybersecurity in Higher Education: Safeguarding Students and Faculty Data

How To Write An Argumentative Essay
How to write an effective introduction for your research paper
Last updated
20 January 2024
Reviewed by
However, the introduction is a vital element of your research paper. It helps the reader decide whether your paper is worth their time. As such, it's worth taking your time to get it right.
In this article, we'll tell you everything you need to know about writing an effective introduction for your research paper.
- The importance of an introduction in research papers
The primary purpose of an introduction is to provide an overview of your paper. This lets readers gauge whether they want to continue reading or not. The introduction should provide a meaningful roadmap of your research to help them make this decision. It should let readers know whether the information they're interested in is likely to be found in the pages that follow.
Aside from providing readers with information about the content of your paper, the introduction also sets the tone. It shows readers the style of language they can expect, which can further help them to decide how far to read.
When you take into account both of these roles that an introduction plays, it becomes clear that crafting an engaging introduction is the best way to get your paper read more widely. First impressions count, and the introduction provides that impression to readers.
- The optimum length for a research paper introduction
While there's no magic formula to determine exactly how long a research paper introduction should be, there are a few guidelines. Some variables that impact the ideal introduction length include:
Field of study
Complexity of the topic
Specific requirements of the course or publication
A commonly recommended length of a research paper introduction is around 10% of the total paper’s length. So, a ten-page paper has a one-page introduction. If the topic is complex, it may require more background to craft a compelling intro. Humanities papers tend to have longer introductions than those of the hard sciences.
The best way to craft an introduction of the right length is to focus on clarity and conciseness. Tell the reader only what is necessary to set up your research. An introduction edited down with this goal in mind should end up at an acceptable length.
- Evaluating successful research paper introductions
A good way to gauge how to create a great introduction is by looking at examples from across your field. The most influential and well-regarded papers should provide some insights into what makes a good introduction.
Dissecting examples: what works and why
We can make some general assumptions by looking at common elements of a good introduction, regardless of the field of research.
A common structure is to start with a broad context, and then narrow that down to specific research questions or hypotheses. This creates a funnel that establishes the scope and relevance.
The most effective introductions are careful about the assumptions they make regarding reader knowledge. By clearly defining key terms and concepts instead of assuming the reader is familiar with them, these introductions set a more solid foundation for understanding.
To pull in the reader and make that all-important good first impression, excellent research paper introductions will often incorporate a compelling narrative or some striking fact that grabs the reader's attention.
Finally, good introductions provide clear citations from past research to back up the claims they're making. In the case of argumentative papers or essays (those that take a stance on a topic or issue), a strong thesis statement compels the reader to continue reading.
Common pitfalls to avoid in research paper introductions
You can also learn what not to do by looking at other research papers. Many authors have made mistakes you can learn from.
We've talked about the need to be clear and concise. Many introductions fail at this; they're verbose, vague, or otherwise fail to convey the research problem or hypothesis efficiently. This often comes in the form of an overemphasis on background information, which obscures the main research focus.
Ensure your introduction provides the proper emphasis and excitement around your research and its significance. Otherwise, fewer people will want to read more about it.
- Crafting a compelling introduction for a research paper
Let’s take a look at the steps required to craft an introduction that pulls readers in and compels them to learn more about your research.
Step 1: Capturing interest and setting the scene
To capture the reader's interest immediately, begin your introduction with a compelling question, a surprising fact, a provocative quote, or some other mechanism that will hook readers and pull them further into the paper.
As they continue reading, the introduction should contextualize your research within the current field, showing readers its relevance and importance. Clarify any essential terms that will help them better understand what you're saying. This keeps the fundamentals of your research accessible to all readers from all backgrounds.
Step 2: Building a solid foundation with background information
Including background information in your introduction serves two major purposes:
It helps to clarify the topic for the reader
It establishes the depth of your research
The approach you take when conveying this information depends on the type of paper.
For argumentative papers, you'll want to develop engaging background narratives. These should provide context for the argument you'll be presenting.
For empirical papers, highlighting past research is the key. Often, there will be some questions that weren't answered in those past papers. If your paper is focused on those areas, those papers make ideal candidates for you to discuss and critique in your introduction.
Step 3: Pinpointing the research challenge
To capture the attention of the reader, you need to explain what research challenges you'll be discussing.
For argumentative papers, this involves articulating why the argument you'll be making is important. What is its relevance to current discussions or problems? What is the potential impact of people accepting or rejecting your argument?
For empirical papers, explain how your research is addressing a gap in existing knowledge. What new insights or contributions will your research bring to your field?
Step 4: Clarifying your research aims and objectives
We mentioned earlier that the introduction to a research paper can serve as a roadmap for what's within. We've also frequently discussed the need for clarity. This step addresses both of these.
When writing an argumentative paper, craft a thesis statement with impact. Clearly articulate what your position is and the main points you intend to present. This will map out for the reader exactly what they'll get from reading the rest.
For empirical papers, focus on formulating precise research questions and hypotheses. Directly link them to the gaps or issues you've identified in existing research to show the reader the precise direction your research paper will take.
Step 5: Sketching the blueprint of your study
Continue building a roadmap for your readers by designing a structured outline for the paper. Guide the reader through your research journey, explaining what the different sections will contain and their relationship to one another.
This outline should flow seamlessly as you move from section to section. Creating this outline early can also help guide the creation of the paper itself, resulting in a final product that's better organized. In doing so, you'll craft a paper where each section flows intuitively from the next.
Step 6: Integrating your research question
To avoid letting your research question get lost in background information or clarifications, craft your introduction in such a way that the research question resonates throughout. The research question should clearly address a gap in existing knowledge or offer a new perspective on an existing problem.
Tell users your research question explicitly but also remember to frequently come back to it. When providing context or clarification, point out how it relates to the research question. This keeps your focus where it needs to be and prevents the topic of the paper from becoming under-emphasized.
Step 7: Establishing the scope and limitations
So far, we've talked mostly about what's in the paper and how to convey that information to readers. The opposite is also important. Information that's outside the scope of your paper should be made clear to the reader in the introduction so their expectations for what is to follow are set appropriately.
Similarly, be honest and upfront about the limitations of the study. Any constraints in methodology, data, or how far your findings can be generalized should be fully communicated in the introduction.
Step 8: Concluding the introduction with a promise
The final few lines of the introduction are your last chance to convince people to continue reading the rest of the paper. Here is where you should make it very clear what benefit they'll get from doing so. What topics will be covered? What questions will be answered? Make it clear what they will get for continuing.
By providing a quick recap of the key points contained in the introduction in its final lines and properly setting the stage for what follows in the rest of the paper, you refocus the reader's attention on the topic of your research and guide them to read more.
- Research paper introduction best practices
Following the steps above will give you a compelling introduction that hits on all the key points an introduction should have. Some more tips and tricks can make an introduction even more polished.
As you follow the steps above, keep the following tips in mind.
Set the right tone and style
Like every piece of writing, a research paper should be written for the audience. That is to say, it should match the tone and style that your academic discipline and target audience expect. This is typically a formal and academic tone, though the degree of formality varies by field.
Kno w the audience
The perfect introduction balances clarity with conciseness. The amount of clarification required for a given topic depends greatly on the target audience. Knowing who will be reading your paper will guide you in determining how much background information is required.
Adopt the CARS (create a research space) model
The CARS model is a helpful tool for structuring introductions. This structure has three parts. The beginning of the introduction establishes the general research area. Next, relevant literature is reviewed and critiqued. The final section outlines the purpose of your study as it relates to the previous parts.
Master the art of funneling
The CARS method is one example of a well-funneled introduction. These start broadly and then slowly narrow down to your specific research problem. It provides a nice narrative flow that provides the right information at the right time. If you stray from the CARS model, try to retain this same type of funneling.
Incorporate narrative element
People read research papers largely to be informed. But to inform the reader, you have to hold their attention. A narrative style, particularly in the introduction, is a great way to do that. This can be a compelling story, an intriguing question, or a description of a real-world problem.
Write the introduction last
By writing the introduction after the rest of the paper, you'll have a better idea of what your research entails and how the paper is structured. This prevents the common problem of writing something in the introduction and then forgetting to include it in the paper. It also means anything particularly exciting in the paper isn’t neglected in the intro.
Get started today
Go from raw data to valuable insights with a flexible research platform
Editor’s picks
Last updated: 21 December 2023
Last updated: 16 December 2023
Last updated: 6 October 2023
Last updated: 5 March 2024
Last updated: 25 November 2023
Last updated: 15 February 2024
Last updated: 11 March 2024
Last updated: 12 December 2023
Last updated: 6 March 2024
Last updated: 10 April 2023
Last updated: 20 December 2023
Latest articles
Related topics, log in or sign up.
Get started for free
How to Write a Research Paper Introduction
Learn how to write a research paper introduction with expert guidance.
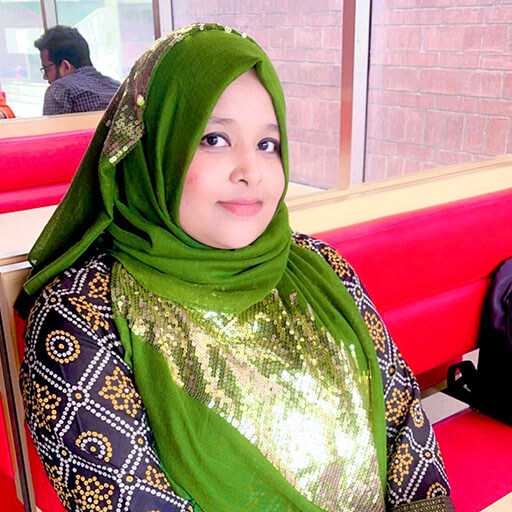
Last updated on Mar 13th, 2024
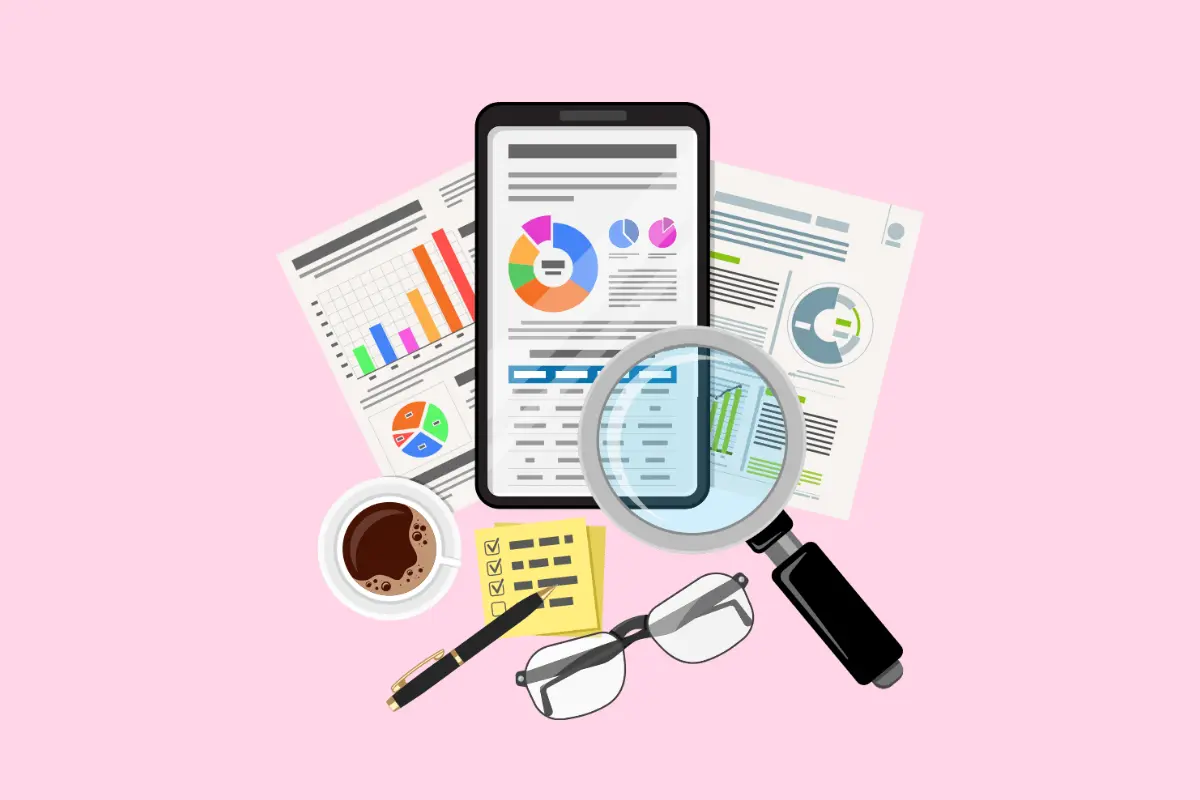
When you click on affiliate links on QuillMuse.com and make a purchase, you won’t pay a penny more, but we’ll get a small commission—this helps us keep up with publishing valuable content on QuillMuse. Read More .
Table of Contents
We write different types of papers for academic and professional reasons. Research paper is one of the most important papers and it is different from other papers. There are different types of rules for writing a research paper , the first part is the introduction. Through this article, we will try to tell you how to write an introduction for a research paper beautifully.
Introduction
Before starting to write any papers, especially research papers one should know how to write a research paper introduction. The introduction is intended to guide the reader from a general subject to a specific area of study. It establishes the context of the research being conducted by summarizing current understanding and background information on the topic, stating the purpose of the work in the form of a thesis, question, or research problem, Briefly explaining your rationale, your methodological approach, highlight the potential findings your research may reveal, and describe the remaining structure of the paper.
A well-written introduction is imperative since, essentially, you never get a second chance to form a great first impression. The opening passage of your paper will give your audience their introductory impression, almost the rationale of your contention, your composing style, the general quality of your investigation, and, eventually, the legitimacy of your discoveries and conclusions. A vague, disorganized, or error-filled introduction will create a negative impression on the readers. While a brief, engaging, and well-written introduction will begin your readers off considering profoundly your expository abilities, your writing style, and your research approach.
Tips for Writng an Introduction in Research Paper
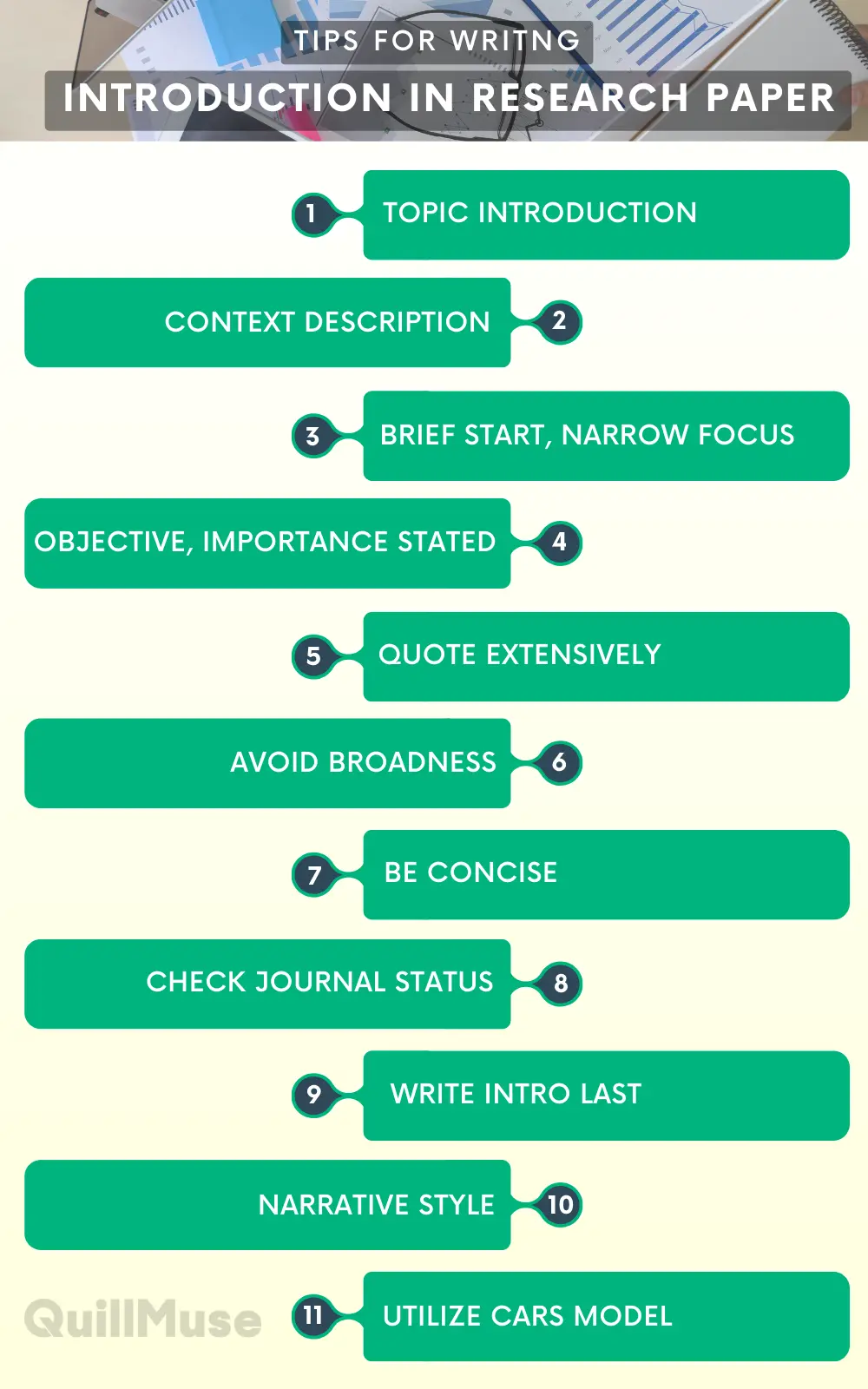
Introduce your topic
This is a significant part of how to write an introduction for a research paper. The first task of the introduction is to tell the reader what your topic is and why it is interesting or important. This is usually done with a strong opening hook.
A hook is a strong opening sentence that conveys relevance to your topic. Think of an interesting fact or statistic, a powerful statement, a question, or a brief anecdote that will make readers wonder about your topic.
Describe the context
This introduction varies depending on your approach to your writing. In a more argumentative article, you will explore the general context here. In a more empirical paper, this is a great place to review previous research and determine how your research fits together.
Start briefly, and narrow down
The first thing of a research paper introduction is, to briefly describe your broad parts of research, then narrow in on your specific focus. This will help position your research topic within a broader field, making the work accessible to a wider audience than just experts in your field.
A common mistake when writing a research paper introduction is trying to fit everything in at once. Instead, pace yourself and present each piece of information in the most logical order the reader can understand. Typically, this means starting with the big picture and then gradually getting more specific with the details.
For your research paper introduction, you should first present an overview of the topic and then focus on your specific paper. This “funnel” structure naturally includes all the necessary parts of what should be included in a research paper introduction, from context to appropriate or research gaps and finally to relevance.
State Objective and Importance
Papers abandoned because they “do not demonstrate the importance of the topic” or “lack a clear motivation” often miss this point. Say what you want to achieve and why your readers should want to know whether you achieved it or not.
Quote generously
Once you have focused on the specific topic of your research, you should detail the latest and most relevant literature related to your research. Your literature review should be comprehensive but not too long. Remember, you are not writing a review. If you find your introduction is too long or has too many citations, a possible solution is to cite journal articles, rather than cite all of the individual articles that have been summarised in the journal.
Do not Keep it broad
Try to avoid lengthy introductions. A good target is between 500 and 1,000 words, although checking the magazine’s guidelines and back issues will provide the clearest guidance.
The introduction is not lengthy or detailed; rather, they are initiating actions. Introductions are best when they get to the point: save the details in the body of the document, where they belong.
The most important point of a research paper introduction is that they are clear and easy to understand. Writing at length can be distracting and even make your point harder to understand, so cut out unnecessary words and try to express things in simple terms that everyone understands. understandable.
Check journal condition
Many journals have specific assertions in their author instructions. For example, a maximum of one word may be stated, or instructions may require specific content, such as a supposition statement or a summary of your key findings.
Write the introduction to your research paper at the last moment
Your introduction may appear first in a research paper, but the general advice is to wait to write it until everything else has been written. This makes it easier for you to summarize your article because at this point you know everything you’re going to say. This also eliminates the urge to include everything in the introduction because you don’t want to forget anything.
Additionally, it is especially helpful to write an introduction after your research paper is finished. The introduction and conclusion of a research paper have similar topics and often reflect the structure of each topic. Writing the conclusion is also generally easier thanks to the pace created by writing the rest of the paper, and the conclusion can guide you in writing the introduction.
Make your introduction narrative style
Although not always appropriate for formal writing, using a narrative style in the introduction of your research paper can do a lot to engage readers and engage them emotionally. A 2016 study found that in some articles, using narrative strategies improved how often they were cited in other articles. Narrative style involves making the paper more personal to appeal to the reader’s emotions.
- Use first-person pronouns (I, we, my, our) to show that you are the narrator expressing emotions and feelings in the text setting up the scene.
- Describe the times and locations of important events to help readers visualize them.
- Appeal to the reader’s morality, sympathy, or urgency as a persuasive tactic. Again, this style will not be appropriate for all research paper introductions, especially those devoted to scientific research.
However, for more informal research papers and especially essays, this style can make your writing more interesting or at least interesting, perfect for making readers excited right from the beginning of the article.
Use the CARS model
British scientist John Swales developed a method called the CARS model to “generate a search space” in the introduction. Although intended for scientific papers, this simple three-step structure can be used to outline the introduction to any research paper.
Explain the background of your topic, including previous research. Explain that information is lacking in your topic area or that current research is incomplete.
Explain how your research “fills in” missing information about your topic.
the research findings and providing an overview of the structure of the rest of the paper, although this does not apply to all research papers, especially those Unofficial documents.
Six Essential Elements of How to Write a Research Paper Introduction
1. topic overview.
Start with a general overview of your topic. Refine your outline until you address the specific topic of your article. Next, mention any questions or concerns you have about the case. Note that you will address these in the article.
2. Previous research
Your introduction is the perfect place to review other findings about your topic. Includes both old and modern scholars. This general information shows that you are aware of previous research. It also presents previous findings to those who may not have that expertise.
3. A justification for your article
Explain why your topic needs to be discussed now. If possible, connect it to current issues. Additionally, you can point out problems with old theories or reveal gaps in current research. No matter how you do it, a good reason will keep readers interested and demonstrate why they should read the rest of your article.
4. Describe the method you used
Tell about your processes to make your writing more trustworthy. Identify your goals and the questions you will answer. Reveal how you conducted the research and describe how you measured the results. Also, explain why you made the important choices.
5. A thesis statement
Your main introduction should end with a thesis statement. This statement summarises the ideas that will run throughout your entire research paper. It must be simple and clear.
6. An outline
It is an adequate idea of how to write an introduction for a research paper.
The introduction usually ends with an overview. Your layout should quickly present what you plan to cover in the following sections. Think of it as a road map, guiding readers to the end of your article.
What is the purpose of the introduction in a research paper, and why is it considered crucial?
The purpose of the introduction in a research paper is to guide the reader from a general subject to a specific area of study. It establishes the context of the research by summarizing current understanding, stating the purpose of the work, explaining the rationale and methodological approach, highlighting potential findings, and describing the paper’s structure. It’s considered crucial because it forms the reader’s first impression and sets the tone for the rest of the paper.
How can I effectively use a hook to engage readers in my research paper introduction?
Using a hook, such as an interesting fact, a powerful statement, a question, or a brief anecdote, can effectively engage readers in your research paper introduction. A hook captures the reader’s attention and makes them curious about your topic, encouraging them to continue reading.
How long the introduction should be in a research paper?
While there’s no strict word count, a good target for a research paper introduction is between 500 and 1,000 words, although you should check the specific guidelines provided by the journal you’re submitting to. It’s recommended to write the introduction after the rest of the paper has been completed. This way, you have a comprehensive understanding of your research, making it easier to summarize and guide your readers effectively.
Conclusion
These are the important tips and tricks on how to write an introduction for a research paper properly. If you maintain these rules we believe that you will be able to write an excellent introduction in your research paper.
How we've reviewed this article
Our content is thoroughly researched and fact-checked using reputable sources. While we aim for precision, we encourage independent verification for complete confidence.
We keep our articles up-to-date regularly to ensure accuracy and relevance as new information becomes available.
- Current Version
- Mar 13th, 2024
- Oct 14th, 2023
Share this article
I found the step-by-step guide really helpful. Looking forward to implementing these tips in my next academic endeavor. Thanks for sharing!
Thank you for your positive feedback! We’re thrilled to hear that you found the guide helpful. If you have any more questions or need further assistance with your research paper, feel free to ask. Best of luck with your academic endeavors!
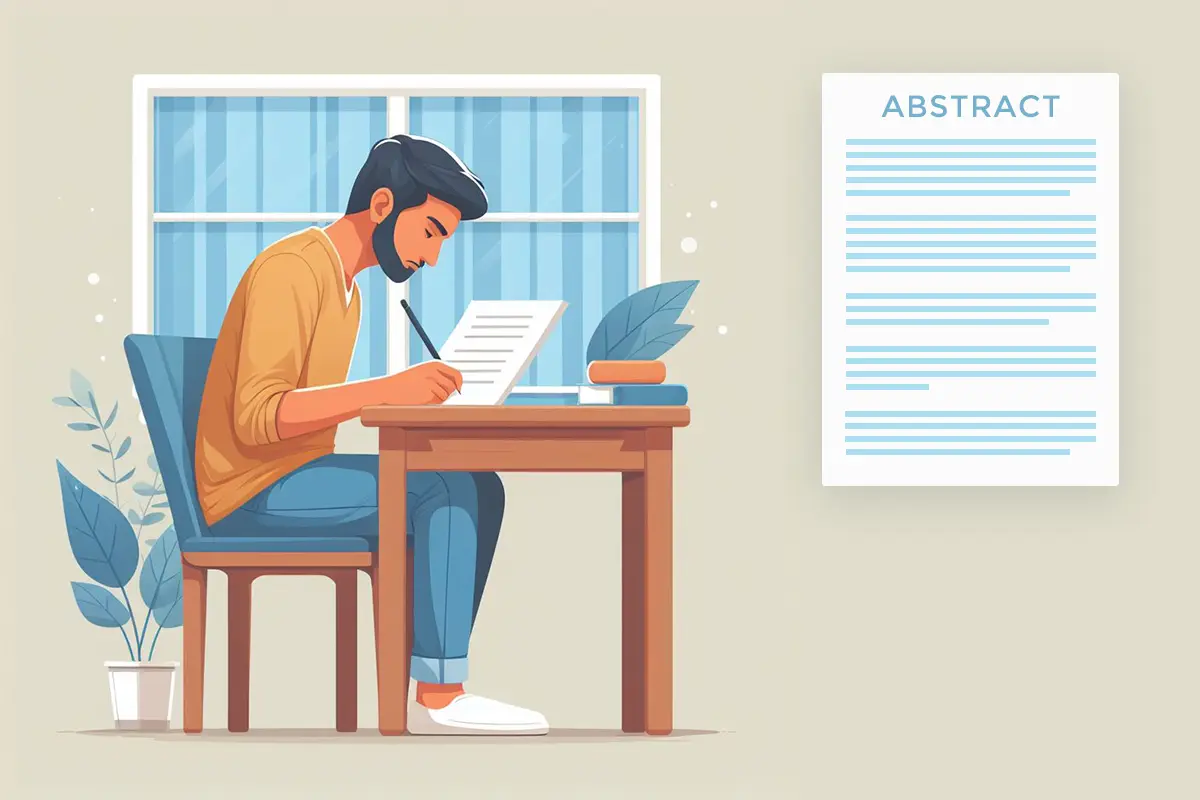
How to Write an Abstract for a Research Paper | 4 Examples
Abstract writing is essential for researchers looking to communicate the substance of their work concisely. In this comprehensive guide, we’ll systematically explore the process of writing a compelling abstract. From defining its purpose to exploring formatting requirements, understanding the key components and strategies ensures the effective communication of a research
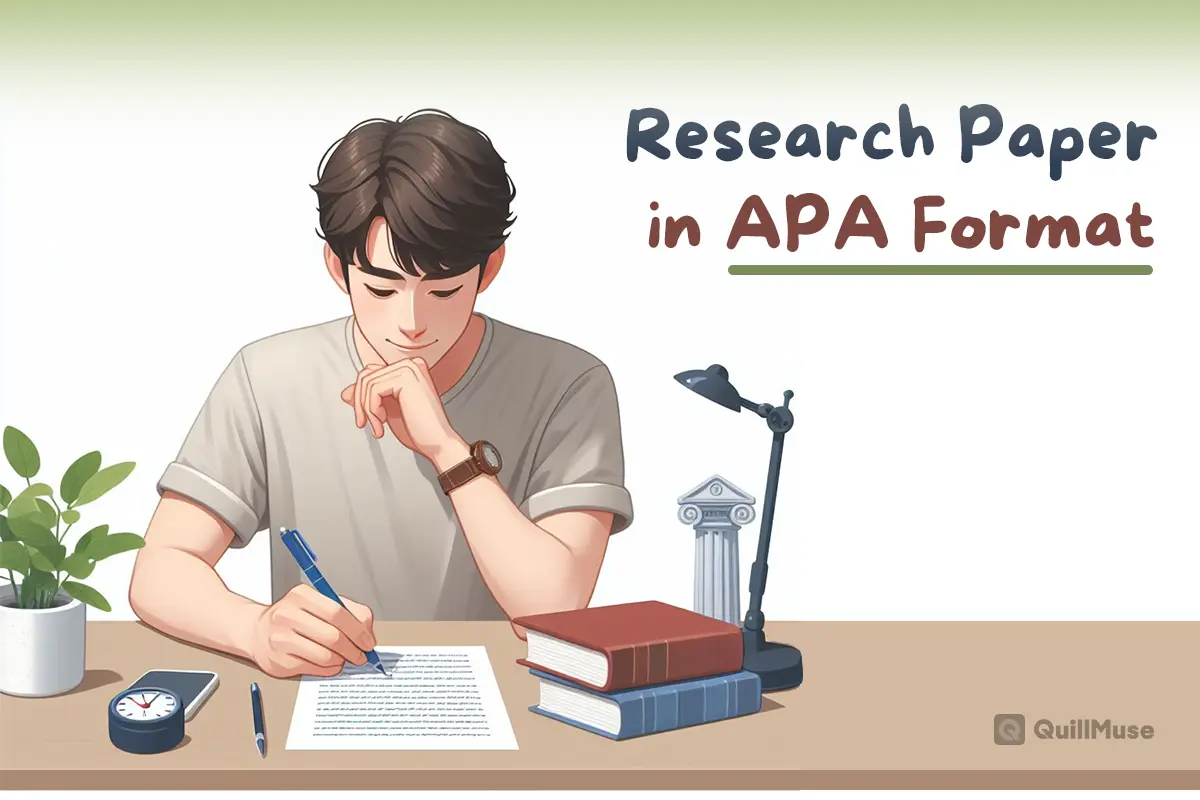
How to Write a Research Paper in APA Format with Examples
I visited a reputable university, where I found that lots of students were excited to learn how to write a research paper. They also want to learn the APA research paper format and outline. Every academic course has an APA research paper outline that is useful for writing research papers.
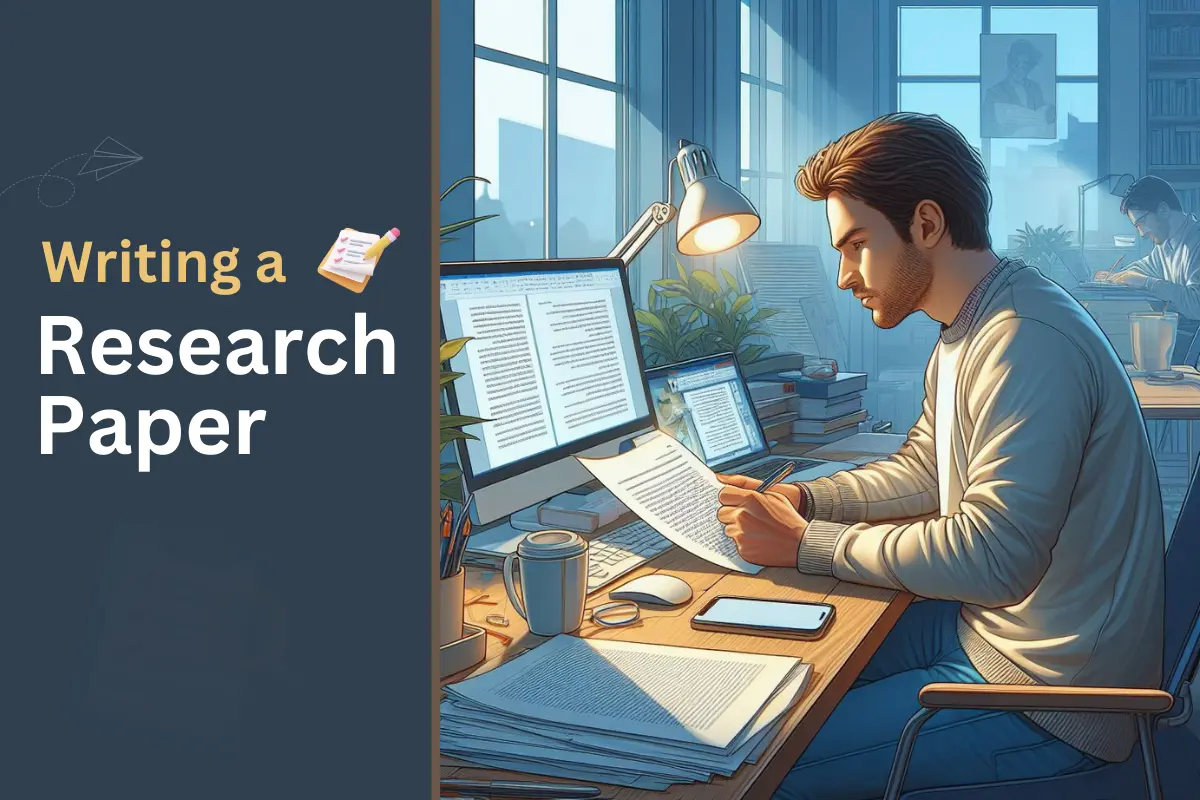
How to Write a Research Paper
A research paper is a kind of paper that is different from other papers. Especially a research paper needed for academic life. The main purpose of the research paper is to deliver new knowledge from researchers’ critical thinking. To learn how to write a research paper, you need to know
Report this article
Let us know if you notice any incorrect information about this article or if it was copied from others. We will take action against this article ASAP.
- Profile Page
- Edit Profile
- Add New Post
Read our Content Writing Guide .
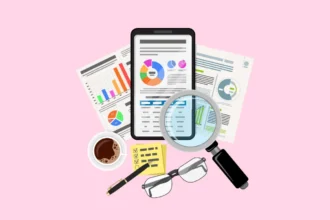

Introductions
What this handout is about.
This handout will explain the functions of introductions, offer strategies for creating effective introductions, and provide some examples of less effective introductions to avoid.
The role of introductions
Introductions and conclusions can be the most difficult parts of papers to write. Usually when you sit down to respond to an assignment, you have at least some sense of what you want to say in the body of your paper. You might have chosen a few examples you want to use or have an idea that will help you answer the main question of your assignment; these sections, therefore, may not be as hard to write. And it’s fine to write them first! But in your final draft, these middle parts of the paper can’t just come out of thin air; they need to be introduced and concluded in a way that makes sense to your reader.
Your introduction and conclusion act as bridges that transport your readers from their own lives into the “place” of your analysis. If your readers pick up your paper about education in the autobiography of Frederick Douglass, for example, they need a transition to help them leave behind the world of Chapel Hill, television, e-mail, and The Daily Tar Heel and to help them temporarily enter the world of nineteenth-century American slavery. By providing an introduction that helps your readers make a transition between their own world and the issues you will be writing about, you give your readers the tools they need to get into your topic and care about what you are saying. Similarly, once you’ve hooked your readers with the introduction and offered evidence to prove your thesis, your conclusion can provide a bridge to help your readers make the transition back to their daily lives. (See our handout on conclusions .)
Note that what constitutes a good introduction may vary widely based on the kind of paper you are writing and the academic discipline in which you are writing it. If you are uncertain what kind of introduction is expected, ask your instructor.
Why bother writing a good introduction?
You never get a second chance to make a first impression. The opening paragraph of your paper will provide your readers with their initial impressions of your argument, your writing style, and the overall quality of your work. A vague, disorganized, error-filled, off-the-wall, or boring introduction will probably create a negative impression. On the other hand, a concise, engaging, and well-written introduction will start your readers off thinking highly of you, your analytical skills, your writing, and your paper.
Your introduction is an important road map for the rest of your paper. Your introduction conveys a lot of information to your readers. You can let them know what your topic is, why it is important, and how you plan to proceed with your discussion. In many academic disciplines, your introduction should contain a thesis that will assert your main argument. Your introduction should also give the reader a sense of the kinds of information you will use to make that argument and the general organization of the paragraphs and pages that will follow. After reading your introduction, your readers should not have any major surprises in store when they read the main body of your paper.
Ideally, your introduction will make your readers want to read your paper. The introduction should capture your readers’ interest, making them want to read the rest of your paper. Opening with a compelling story, an interesting question, or a vivid example can get your readers to see why your topic matters and serve as an invitation for them to join you for an engaging intellectual conversation (remember, though, that these strategies may not be suitable for all papers and disciplines).
Strategies for writing an effective introduction
Start by thinking about the question (or questions) you are trying to answer. Your entire essay will be a response to this question, and your introduction is the first step toward that end. Your direct answer to the assigned question will be your thesis, and your thesis will likely be included in your introduction, so it is a good idea to use the question as a jumping off point. Imagine that you are assigned the following question:
Drawing on the Narrative of the Life of Frederick Douglass , discuss the relationship between education and slavery in 19th-century America. Consider the following: How did white control of education reinforce slavery? How did Douglass and other enslaved African Americans view education while they endured slavery? And what role did education play in the acquisition of freedom? Most importantly, consider the degree to which education was or was not a major force for social change with regard to slavery.
You will probably refer back to your assignment extensively as you prepare your complete essay, and the prompt itself can also give you some clues about how to approach the introduction. Notice that it starts with a broad statement and then narrows to focus on specific questions from the book. One strategy might be to use a similar model in your own introduction—start off with a big picture sentence or two and then focus in on the details of your argument about Douglass. Of course, a different approach could also be very successful, but looking at the way the professor set up the question can sometimes give you some ideas for how you might answer it. (See our handout on understanding assignments for additional information on the hidden clues in assignments.)
Decide how general or broad your opening should be. Keep in mind that even a “big picture” opening needs to be clearly related to your topic; an opening sentence that said “Human beings, more than any other creatures on earth, are capable of learning” would be too broad for our sample assignment about slavery and education. If you have ever used Google Maps or similar programs, that experience can provide a helpful way of thinking about how broad your opening should be. Imagine that you’re researching Chapel Hill. If what you want to find out is whether Chapel Hill is at roughly the same latitude as Rome, it might make sense to hit that little “minus” sign on the online map until it has zoomed all the way out and you can see the whole globe. If you’re trying to figure out how to get from Chapel Hill to Wrightsville Beach, it might make more sense to zoom in to the level where you can see most of North Carolina (but not the rest of the world, or even the rest of the United States). And if you are looking for the intersection of Ridge Road and Manning Drive so that you can find the Writing Center’s main office, you may need to zoom all the way in. The question you are asking determines how “broad” your view should be. In the sample assignment above, the questions are probably at the “state” or “city” level of generality. When writing, you need to place your ideas in context—but that context doesn’t generally have to be as big as the whole galaxy!
Try writing your introduction last. You may think that you have to write your introduction first, but that isn’t necessarily true, and it isn’t always the most effective way to craft a good introduction. You may find that you don’t know precisely what you are going to argue at the beginning of the writing process. It is perfectly fine to start out thinking that you want to argue a particular point but wind up arguing something slightly or even dramatically different by the time you’ve written most of the paper. The writing process can be an important way to organize your ideas, think through complicated issues, refine your thoughts, and develop a sophisticated argument. However, an introduction written at the beginning of that discovery process will not necessarily reflect what you wind up with at the end. You will need to revise your paper to make sure that the introduction, all of the evidence, and the conclusion reflect the argument you intend. Sometimes it’s easiest to just write up all of your evidence first and then write the introduction last—that way you can be sure that the introduction will match the body of the paper.
Don’t be afraid to write a tentative introduction first and then change it later. Some people find that they need to write some kind of introduction in order to get the writing process started. That’s fine, but if you are one of those people, be sure to return to your initial introduction later and rewrite if necessary.
Open with something that will draw readers in. Consider these options (remembering that they may not be suitable for all kinds of papers):
- an intriguing example —for example, Douglass writes about a mistress who initially teaches him but then ceases her instruction as she learns more about slavery.
- a provocative quotation that is closely related to your argument —for example, Douglass writes that “education and slavery were incompatible with each other.” (Quotes from famous people, inspirational quotes, etc. may not work well for an academic paper; in this example, the quote is from the author himself.)
- a puzzling scenario —for example, Frederick Douglass says of slaves that “[N]othing has been left undone to cripple their intellects, darken their minds, debase their moral nature, obliterate all traces of their relationship to mankind; and yet how wonderfully they have sustained the mighty load of a most frightful bondage, under which they have been groaning for centuries!” Douglass clearly asserts that slave owners went to great lengths to destroy the mental capacities of slaves, yet his own life story proves that these efforts could be unsuccessful.
- a vivid and perhaps unexpected anecdote —for example, “Learning about slavery in the American history course at Frederick Douglass High School, students studied the work slaves did, the impact of slavery on their families, and the rules that governed their lives. We didn’t discuss education, however, until one student, Mary, raised her hand and asked, ‘But when did they go to school?’ That modern high school students could not conceive of an American childhood devoid of formal education speaks volumes about the centrality of education to American youth today and also suggests the significance of the deprivation of education in past generations.”
- a thought-provoking question —for example, given all of the freedoms that were denied enslaved individuals in the American South, why does Frederick Douglass focus his attentions so squarely on education and literacy?
Pay special attention to your first sentence. Start off on the right foot with your readers by making sure that the first sentence actually says something useful and that it does so in an interesting and polished way.
How to evaluate your introduction draft
Ask a friend to read your introduction and then tell you what he or she expects the paper will discuss, what kinds of evidence the paper will use, and what the tone of the paper will be. If your friend is able to predict the rest of your paper accurately, you probably have a good introduction.
Five kinds of less effective introductions
1. The placeholder introduction. When you don’t have much to say on a given topic, it is easy to create this kind of introduction. Essentially, this kind of weaker introduction contains several sentences that are vague and don’t really say much. They exist just to take up the “introduction space” in your paper. If you had something more effective to say, you would probably say it, but in the meantime this paragraph is just a place holder.
Example: Slavery was one of the greatest tragedies in American history. There were many different aspects of slavery. Each created different kinds of problems for enslaved people.
2. The restated question introduction. Restating the question can sometimes be an effective strategy, but it can be easy to stop at JUST restating the question instead of offering a more specific, interesting introduction to your paper. The professor or teaching assistant wrote your question and will be reading many essays in response to it—he or she does not need to read a whole paragraph that simply restates the question.
Example: The Narrative of the Life of Frederick Douglass discusses the relationship between education and slavery in 19th century America, showing how white control of education reinforced slavery and how Douglass and other enslaved African Americans viewed education while they endured. Moreover, the book discusses the role that education played in the acquisition of freedom. Education was a major force for social change with regard to slavery.
3. The Webster’s Dictionary introduction. This introduction begins by giving the dictionary definition of one or more of the words in the assigned question. Anyone can look a word up in the dictionary and copy down what Webster says. If you want to open with a discussion of an important term, it may be far more interesting for you (and your reader) if you develop your own definition of the term in the specific context of your class and assignment. You may also be able to use a definition from one of the sources you’ve been reading for class. Also recognize that the dictionary is also not a particularly authoritative work—it doesn’t take into account the context of your course and doesn’t offer particularly detailed information. If you feel that you must seek out an authority, try to find one that is very relevant and specific. Perhaps a quotation from a source reading might prove better? Dictionary introductions are also ineffective simply because they are so overused. Instructors may see a great many papers that begin in this way, greatly decreasing the dramatic impact that any one of those papers will have.
Example: Webster’s dictionary defines slavery as “the state of being a slave,” as “the practice of owning slaves,” and as “a condition of hard work and subjection.”
4. The “dawn of man” introduction. This kind of introduction generally makes broad, sweeping statements about the relevance of this topic since the beginning of time, throughout the world, etc. It is usually very general (similar to the placeholder introduction) and fails to connect to the thesis. It may employ cliches—the phrases “the dawn of man” and “throughout human history” are examples, and it’s hard to imagine a time when starting with one of these would work. Instructors often find them extremely annoying.
Example: Since the dawn of man, slavery has been a problem in human history.
5. The book report introduction. This introduction is what you had to do for your elementary school book reports. It gives the name and author of the book you are writing about, tells what the book is about, and offers other basic facts about the book. You might resort to this sort of introduction when you are trying to fill space because it’s a familiar, comfortable format. It is ineffective because it offers details that your reader probably already knows and that are irrelevant to the thesis.
Example: Frederick Douglass wrote his autobiography, Narrative of the Life of Frederick Douglass, An American Slave , in the 1840s. It was published in 1986 by Penguin Books. In it, he tells the story of his life.
And now for the conclusion…
Writing an effective introduction can be tough. Try playing around with several different options and choose the one that ends up sounding best to you!
Just as your introduction helps readers make the transition to your topic, your conclusion needs to help them return to their daily lives–but with a lasting sense of how what they have just read is useful or meaningful. Check out our handout on conclusions for tips on ending your paper as effectively as you began it!
Works consulted
We consulted these works while writing this handout. This is not a comprehensive list of resources on the handout’s topic, and we encourage you to do your own research to find additional publications. Please do not use this list as a model for the format of your own reference list, as it may not match the citation style you are using. For guidance on formatting citations, please see the UNC Libraries citation tutorial . We revise these tips periodically and welcome feedback.
Douglass, Frederick. 1995. Narrative of the Life of Frederick Douglass, an American Slave, Written by Himself . New York: Dover.
You may reproduce it for non-commercial use if you use the entire handout and attribute the source: The Writing Center, University of North Carolina at Chapel Hill
Make a Gift
Microsoft 365 Life Hacks > Writing > How to write an introduction for a research paper
How to write an introduction for a research paper
Beginnings are hard. Beginning a research paper is no exception. Many students—and pros—struggle with how to write an introduction for a research paper.
This short guide will describe the purpose of a research paper introduction and how to create a good one.

What is an introduction for a research paper?
Introductions to research papers do a lot of work.
It may seem obvious, but introductions are always placed at the beginning of a paper. They guide your reader from a general subject area to the narrow topic that your paper covers. They also explain your paper’s:
- Scope: The topic you’ll be covering
- Context: The background of your topic
- Importance: Why your research matters in the context of an industry or the world
Your introduction will cover a lot of ground. However, it will only be half of a page to a few pages long. The length depends on the size of your paper as a whole. In many cases, the introduction will be shorter than all of the other sections of your paper.

Write with Confidence using Editor
Elevate your writing with real-time, intelligent assistance
Why is an introduction vital to a research paper?
The introduction to your research paper isn’t just important. It’s critical.
Your readers don’t know what your research paper is about from the title. That’s where your introduction comes in. A good introduction will:
- Help your reader understand your topic’s background
- Explain why your research paper is worth reading
- Offer a guide for navigating the rest of the piece
- Pique your reader’s interest
Without a clear introduction, your readers will struggle. They may feel confused when they start reading your paper. They might even give up entirely. Your introduction will ground them and prepare them for the in-depth research to come.
What should you include in an introduction for a research paper?
Research paper introductions are always unique. After all, research is original by definition. However, they often contain six essential items. These are:
- An overview of the topic. Start with a general overview of your topic. Narrow the overview until you address your paper’s specific subject. Then, mention questions or concerns you had about the case. Note that you will address them in the publication.
- Prior research. Your introduction is the place to review other conclusions on your topic. Include both older scholars and modern scholars. This background information shows that you are aware of prior research. It also introduces past findings to those who might not have that expertise.
- A rationale for your paper. Explain why your topic needs to be addressed right now. If applicable, connect it to current issues. Additionally, you can show a problem with former theories or reveal a gap in current research. No matter how you do it, a good rationale will interest your readers and demonstrate why they must read the rest of your paper.
- Describe the methodology you used. Recount your processes to make your paper more credible. Lay out your goal and the questions you will address. Reveal how you conducted research and describe how you measured results. Moreover, explain why you made key choices.
- A thesis statement. Your main introduction should end with a thesis statement. This statement summarizes the ideas that will run through your entire research article. It should be straightforward and clear.
- An outline. Introductions often conclude with an outline. Your layout should quickly review what you intend to cover in the following sections. Think of it as a roadmap, guiding your reader to the end of your paper.
These six items are emphasized more or less, depending on your field. For example, a physics research paper might emphasize methodology. An English journal article might highlight the overview.
Three tips for writing your introduction
We don’t just want you to learn how to write an introduction for a research paper. We want you to learn how to make it shine.
There are three things you can do that will make it easier to write a great introduction. You can:
- Write your introduction last. An introduction summarizes all of the things you’ve learned from your research. While it can feel good to get your preface done quickly, you should write the rest of your paper first. Then, you’ll find it easy to create a clear overview.
- Include a strong quotation or story upfront. You want your paper to be full of substance. But that doesn’t mean it should feel boring or flat. Add a relevant quotation or surprising anecdote to the beginning of your introduction. This technique will pique the interest of your reader and leave them wanting more.
- Be concise. Research papers cover complex topics. To help your readers, try to write as clearly as possible. Use concise sentences. Check for confusing grammar or syntax . Read your introduction out loud to catch awkward phrases. Before you finish your paper, be sure to proofread, too. Mistakes can seem unprofessional.

Get started with Microsoft 365
It’s the Office you know, plus the tools to help you work better together, so you can get more done—anytime, anywhere.
Topics in this article
More articles like this one.

What is independent publishing?
Avoid the hassle of shopping your book around to publishing houses. Publish your book independently and understand the benefits it provides for your as an author.

What are literary tropes?
Engage your audience with literary tropes. Learn about different types of literary tropes, like metaphors and oxymorons, to elevate your writing.

What are genre tropes?
Your favorite genres are filled with unifying tropes that can define them or are meant to be subverted.

What is literary fiction?
Define literary fiction and learn what sets it apart from genre fiction.
Everything you need to achieve more in less time
Get powerful productivity and security apps with Microsoft 365

Explore Other Categories
How to Write the Introduction to a Scientific Paper?
- Open Access
- First Online: 24 October 2021
Cite this chapter
You have full access to this open access chapter
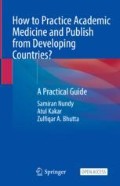
- Samiran Nundy 4 ,
- Atul Kakar 5 &
- Zulfiqar A. Bhutta 6
61k Accesses
145 Altmetric
An Introduction to a scientific paper familiarizes the reader with the background of the issue at hand. It must reflect why the issue is topical and its current importance in the vast sea of research being done globally. It lays the foundation of biomedical writing and is the first portion of an article according to the IMRAD pattern ( I ntroduction, M ethodology, R esults, a nd D iscussion) [1].
I once had a professor tell a class that he sifted through our pile of essays, glancing at the titles and introductions, looking for something that grabbed his attention. Everything else went to the bottom of the pile to be read last, when he was tired and probably grumpy from all the marking. Don’t get put at the bottom of the pile, he said. Anonymous
You have full access to this open access chapter, Download chapter PDF
Similar content being viewed by others
The Introduction Section
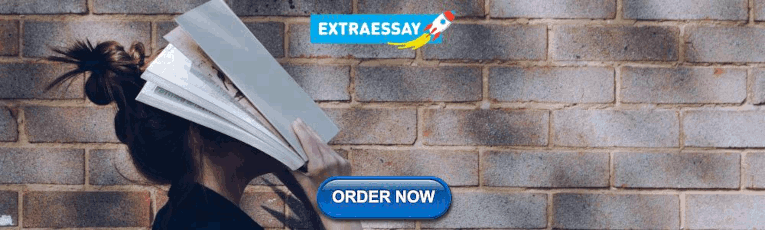
Abstract and Keywords
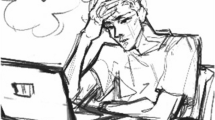
Writing and publishing a scientific paper
Fritz Scholz
1 What is the Importance of an Introduction?
An Introduction to a scientific paper familiarizes the reader with the background of the issue at hand. It must reflect why the issue is topical and its current importance in the vast sea of research being done globally. It lays the foundation of biomedical writing and is the first portion of an article according to the IMRAD pattern ( I ntroduction, M ethodology, R esults, a nd D iscussion) [ 1 ].
It provides the flavour of the article and many authors have used phrases to describe it for example—'like a gate of the city’ [ 2 ], ‘the beginning is half of the whole’ [ 3 ], ‘an introduction is not just wrestling with words to fit the facts, but it also strongly modulated by perception of the anticipated reactions of peer colleagues’, [ 4 ] and ‘an introduction is like the trailer to a movie’. A good introduction helps captivate the reader early.
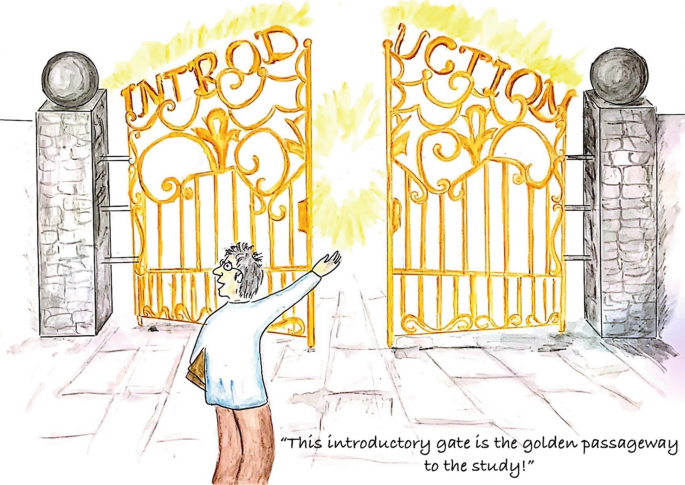
2 What Are the Principles of Writing a Good Introduction?
A good introduction will ‘sell’ an article to a journal editor, reviewer, and finally to a reader [ 3 ]. It should contain the following information [ 5 , 6 ]:
The known—The background scientific data
The unknown—Gaps in the current knowledge
Research hypothesis or question
Methodologies used for the study
The known consist of citations from a review of the literature whereas the unknown is the new work to be undertaken. This part should address how your work is the required missing piece of the puzzle.
3 What Are the Models of Writing an Introduction?
The Problem-solving model
First described by Swales et al. in 1979, in this model the writer should identify the ‘problem’ in the research, address the ‘solution’ and also write about ‘the criteria for evaluating the problem’ [ 7 , 8 ].
The CARS model that stands for C reating A R esearch S pace [ 9 , 10 ].
The two important components of this model are:
Establishing a territory (situation)
Establishing a niche (problem)
Occupying a niche (the solution)
In this popular model, one can add a fourth point, i.e., a conclusion [ 10 ].
4 What Is Establishing a Territory?
This includes: [ 9 ]
Stating the general topic and providing some background about it.
Providing a brief and relevant review of the literature related to the topic.
Adding a paragraph on the scope of the topic including the need for your study.
5 What Is Establishing a Niche?
Establishing a niche includes:
Stating the importance of the problem.
Outlining the current situation regarding the problem citing both global and national data.
Evaluating the current situation (advantages/ disadvantages).
Identifying the gaps.
Emphasizing the importance of the proposed research and how the gaps will be addressed.
Stating the research problem/ questions.
Stating the hypotheses briefly.
Figure 17.1 depicts how the introduction needs to be written. A scientific paper should have an introduction in the form of an inverted pyramid. The writer should start with the general information about the topic and subsequently narrow it down to the specific topic-related introduction.
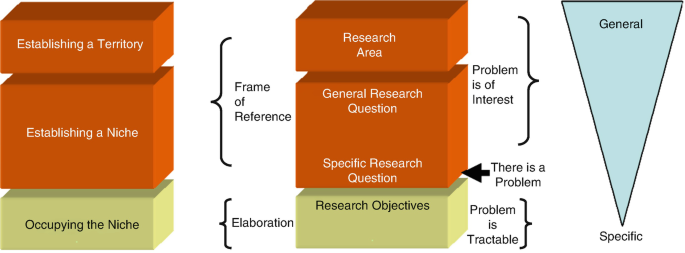
Flow of ideas from the general to the specific
6 What Does Occupying a Niche Mean?
This is the third portion of the introduction and defines the rationale of the research and states the research question. If this is missing the reviewers will not understand the logic for publication and is a common reason for rejection [ 11 , 12 ]. An example of this is given below:
Till date, no study has been done to see the effectiveness of a mesh alone or the effectiveness of double suturing along with a mesh in the closure of an umbilical hernia regarding the incidence of failure. So, the present study is aimed at comparing the effectiveness of a mesh alone versus the double suturing technique along with a mesh.
7 How Long Should the Introduction Be?
For a project protocol, the introduction should be about 1–2 pages long and for a thesis it should be 3–5 pages in a double-spaced typed setting. For a scientific paper it should be less than 10–15% of the total length of the manuscript [ 13 , 14 ].
8 How Many References Should an Introduction Have?
All sections in a scientific manuscript except the conclusion should contain references. It has been suggested that an introduction should have four or five or at the most one-third of the references in the whole paper [ 15 ].
9 What Are the Important Points Which Should be not Missed in an Introduction?
An introduction paves the way forward for the subsequent sections of the article. Frequently well-planned studies are rejected by journals during review because of the simple reason that the authors failed to clarify the data in this section to justify the study [ 16 , 17 ]. Thus, the existing gap in knowledge should be clearly brought out in this section (Fig. 17.2 ).
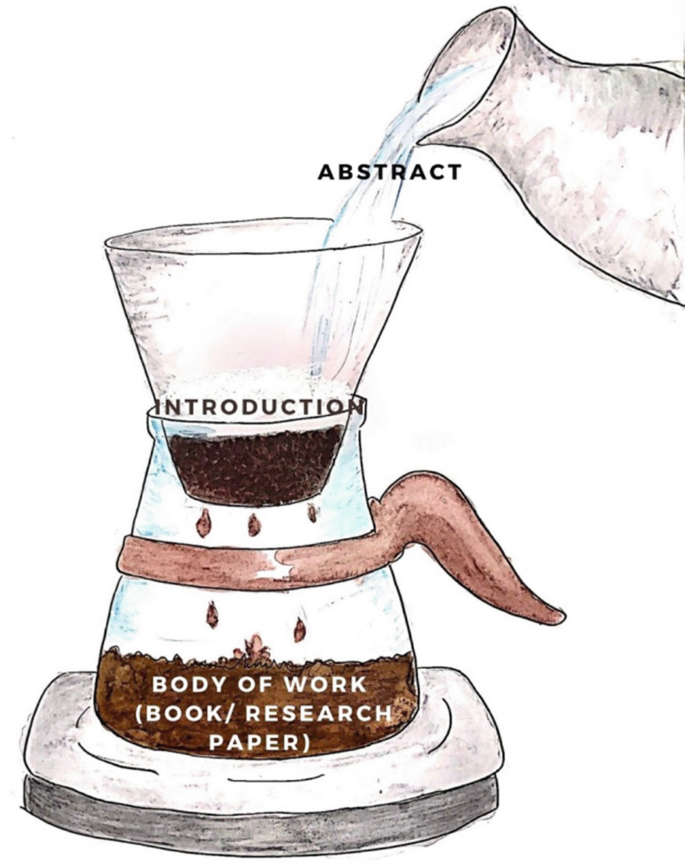
How should the abstract, introduction, and discussion look
The following points are important to consider:
The introduction should be written in simple sentences and in the present tense.
Many of the terms will be introduced in this section for the first time and these will require abbreviations to be used later.
The references in this section should be to papers published in quality journals (e.g., having a high impact factor).
The aims, problems, and hypotheses should be clearly mentioned.
Start with a generalization on the topic and go on to specific information relevant to your research.
10 Example of an Introduction
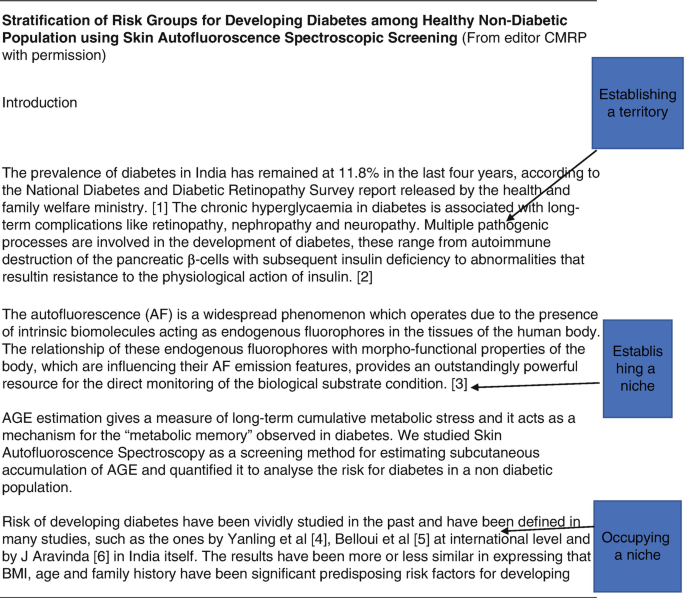
11 Conclusions
An Introduction is a brief account of what the study is about. It should be short, crisp, and complete.
It has to move from a general to a specific research topic and must include the need for the present study.
The Introduction should include data from a literature search, i.e., what is already known about this subject and progress to what we hope to add to this knowledge.
Moore A. What’s in a discussion section? Exploiting 2-dimensionality in the online world. Bioassays. 2016;38(12):1185.
Article Google Scholar
Annesley TM. The discussion section: your closing argument. Clin Chem. 2010;56(11):1671–4.
Article CAS Google Scholar
Bavdekar SB. Writing the discussion section: describing the significance of the study findings. J Assoc Physicians India. 2015;63(11):40–2.
PubMed Google Scholar
Foote M. The proof of the pudding: how to report results and write a good discussion. Chest. 2009;135(3):866–8.
Kearney MH. The discussion section tells us where we are. Res Nurs Health. 2017;40(4):289–91.
Ghasemi A, Bahadoran Z, Mirmiran P, Hosseinpanah F, Shiva N, Zadeh-Vakili A. The principles of biomedical scientific writing: discussion. Int J Endocrinol Metab. 2019;17(3):e95415.
Swales JM, Feak CB. Academic writing for graduate students: essential tasks and skills. Ann Arbor, MI: University of Michigan Press; 2004.
Google Scholar
Colombo M, Bucher L, Sprenger J. Determinants of judgments of explanatory power: credibility, generality, and statistical relevance. Front Psychol. 2017;8:1430.
Mozayan MR, Allami H, Fazilatfar AM. Metadiscourse features in medical research articles: subdisciplinary and paradigmatic influences in English and Persian. Res Appl Ling. 2018;9(1):83–104.
Hyland K. Metadiscourse: mapping interactions in academic writing. Nordic J English Stud. 2010;9(2):125.
Hill AB. The environment and disease: association or causation? Proc Royal Soc Med. 2016;58(5):295–300.
Alpert JS. Practicing medicine in Plato’s cave. Am J Med. 2006;119(6):455–6.
Walsh K. Discussing discursive discussions. Med Educ. 2016;50(12):1269–70.
Polit DF, Beck CT. Generalization in quantitative and qualitative research: myths and strategies. Int J Nurs Stud. 2010;47(11):1451–8.
Jawaid SA, Jawaid M. How to write introduction and discussion. Saudi J Anaesth. 2019;13(Suppl 1):S18–9.
Jawaid SA, Baig M. How to write an original article. In: Jawaid SA, Jawaid M, editors. Scientific writing: a guide to the art of medical writing and scientific publishing. Karachi: Published by Med-Print Services; 2018. p. 135–50.
Hall GM, editor. How to write a paper. London: BMJ Books, BMJ Publishing Group; 2003. Structure of a scientific paper. p. 1–5.
Download references
Author information
Authors and affiliations.
Department of Surgical Gastroenterology and Liver Transplantation, Sir Ganga Ram Hospital, New Delhi, India
Samiran Nundy
Department of Internal Medicine, Sir Ganga Ram Hospital, New Delhi, India
Institute for Global Health and Development, The Aga Khan University, South Central Asia, East Africa and United Kingdom, Karachi, Pakistan
Zulfiqar A. Bhutta
You can also search for this author in PubMed Google Scholar
Rights and permissions
Open Access This chapter is licensed under the terms of the Creative Commons Attribution 4.0 International License ( http://creativecommons.org/licenses/by/4.0/ ), which permits use, sharing, adaptation, distribution and reproduction in any medium or format, as long as you give appropriate credit to the original author(s) and the source, provide a link to the Creative Commons license and indicate if changes were made.
The images or other third party material in this chapter are included in the chapter's Creative Commons license, unless indicated otherwise in a credit line to the material. If material is not included in the chapter's Creative Commons license and your intended use is not permitted by statutory regulation or exceeds the permitted use, you will need to obtain permission directly from the copyright holder.
Reprints and permissions
Copyright information
© 2022 The Author(s)
About this chapter
Nundy, S., Kakar, A., Bhutta, Z.A. (2022). How to Write the Introduction to a Scientific Paper?. In: How to Practice Academic Medicine and Publish from Developing Countries?. Springer, Singapore. https://doi.org/10.1007/978-981-16-5248-6_17
Download citation
DOI : https://doi.org/10.1007/978-981-16-5248-6_17
Published : 24 October 2021
Publisher Name : Springer, Singapore
Print ISBN : 978-981-16-5247-9
Online ISBN : 978-981-16-5248-6
eBook Packages : Medicine Medicine (R0)
Share this chapter
Anyone you share the following link with will be able to read this content:
Sorry, a shareable link is not currently available for this article.
Provided by the Springer Nature SharedIt content-sharing initiative
- Publish with us
Policies and ethics
- Find a journal
- Track your research

Organizing Academic Research Papers: 4. The Introduction
- Purpose of Guide
- Design Flaws to Avoid
- Glossary of Research Terms
- Narrowing a Topic Idea
- Broadening a Topic Idea
- Extending the Timeliness of a Topic Idea
- Academic Writing Style
- Choosing a Title
- Making an Outline
- Paragraph Development
- Executive Summary
- Background Information
- The Research Problem/Question
- Theoretical Framework
- Citation Tracking
- Content Alert Services
- Evaluating Sources
- Primary Sources
- Secondary Sources
- Tertiary Sources
- What Is Scholarly vs. Popular?
- Qualitative Methods
- Quantitative Methods
- Using Non-Textual Elements
- Limitations of the Study
- Common Grammar Mistakes
- Avoiding Plagiarism
- Footnotes or Endnotes?
- Further Readings
- Annotated Bibliography
- Dealing with Nervousness
- Using Visual Aids
- Grading Someone Else's Paper
- How to Manage Group Projects
- Multiple Book Review Essay
- Reviewing Collected Essays
- About Informed Consent
- Writing Field Notes
- Writing a Policy Memo
- Writing a Research Proposal
- Acknowledgements
The introduction serves the purpose of leading the reader from a general subject area to a particular field of research. It establishes the context of the research being conducted by summarizing current understanding and background information about the topic, stating the purpose of the work in the form of the hypothesis, question, or research problem, briefly explaining your rationale, methodological approach, highlighting the potential outcomes your study can reveal, and describing the remaining structure of the paper.
Key Elements of the Research Proposal. Prepared under the direction of the Superintendent and by the 2010 Curriculum Design and Writing Team. Baltimore County Public Schools.
Importance of a Good Introduction
Think of the introduction as a mental road map that must answer for the reader these four questions:
- What was I studying?
- Why was this topic important to investigate?
- What did we know about this topic before I did this study?
- How will this study advance our knowledge?
A well-written introduction is important because, quite simply, you never get a second chance to make a good first impression. The opening paragraph of your paper will provide your readers with their initial impressions about the logic of your argument, your writing style, the overall quality of your research, and, ultimately, the validity of your findings and conclusions. A vague, disorganized, or error-filled introduction will create a negative impression, whereas, a concise, engaging, and well-written introduction will start your readers off thinking highly of your analytical skills, your writing style, and your research approach.
Introductions . The Writing Center. University of North Carolina.
Structure and Writing Style
I. Structure and Approach
The introduction is the broad beginning of the paper that answers three important questions for the reader:
- What is this?
- Why am I reading it?
- What do you want me to think about / consider doing / react to?
Think of the structure of the introduction as an inverted triangle of information. Organize the information so as to present the more general aspects of the topic early in the introduction, then narrow toward the more specific topical information that provides context, finally arriving at your statement of purpose and rationale and, whenever possible, the potential outcomes your study can reveal.
These are general phases associated with writing an introduction:
- Highlighting the importance of the topic, and/or
- Making general statements about the topic, and/or
- Presenting an overview on current research on the subject.
- Opposing an existing assumption, and/or
- Revealing a gap in existing research, and/or
- Formulating a research question or problem, and/or
- Continuing a disciplinary tradition.
- Stating the intent of your study,
- Outlining the key characteristics of your study,
- Describing important results, and
- Giving a brief overview of the structure of the paper.
NOTE: Even though the introduction is the first main section of a research paper, it is often useful to finish the introduction very late in the writing process because the structure of the paper, the reporting and analysis of results, and the conclusion will have been completed and it ensures that your introduction matches the overall structure of your paper.
II. Delimitations of the Study
Delimitations refer to those characteristics that limit the scope and define the conceptual boundaries of your study . This is determined by the conscious exclusionary and inclusionary decisions you make about how to investigate the research problem. In other words, not only should you tell the reader what it is you are studying and why, but you must also acknowledge why you rejected alternative approaches that could have been used to examine the research problem.
Obviously, the first limiting step was the choice of research problem itself. However, implicit are other, related problems that could have been chosen but were rejected. These should be noted in the conclusion of your introduction.
Examples of delimitating choices would be:
- The key aims and objectives of your study,
- The research questions that you address,
- The variables of interest [i.e., the various factors and features of the phenomenon being studied],
- The method(s) of investigation, and
- Any relevant alternative theoretical frameworks that could have been adopted.
Review each of these decisions. You need to not only clearly establish what you intend to accomplish, but to also include a declaration of what the study does not intend to cover. In the latter case, your exclusionary decisions should be based upon criteria stated as, "not interesting"; "not directly relevant"; “too problematic because..."; "not feasible," and the like. Make this reasoning explicit!
NOTE: Delimitations refer to the initial choices made about the broader, overall design of your study and should not be confused with documenting the limitations of your study discovered after the research has been completed.
III. The Narrative Flow
Issues to keep in mind that will help the narrative flow in your introduction :
- Your introduction should clearly identify the subject area of interest . A simple strategy to follow is to use key words from your title in the first few sentences of the introduction. This will help focus the introduction on the topic at the appropriate level and ensures that you get to the primary subject matter quickly without losing focus, or discussing information that is too general.
- Establish context by providing a brief and balanced review of the pertinent published literature that is available on the subject. The key is to summarize for the reader what is known about the specific research problem before you did your analysis. This part of your introduction should not represent a comprehensive literature review but consists of a general review of the important, foundational research literature (with citations) that lays a foundation for understanding key elements of the research problem. See the drop-down tab for "Background Information" for types of contexts.
- Clearly state the hypothesis that you investigated . When you are first learning to write in this format it is okay, and actually preferable, to use a past statement like, "The purpose of this study was to...." or "We investigated three possible mechanisms to explain the...."
- Why did you choose this kind of research study or design? Provide a clear statement of the rationale for your approach to the problem studied. This will usually follow your statement of purpose in the last paragraph of the introduction.
IV. Engaging the Reader
The overarching goal of your introduction is to make your readers want to read your paper. The introduction should grab your reader's attention. Strategies for doing this can be to:
- Open with a compelling story,
- Include a strong quotation or a vivid, perhaps unexpected anecdote,
- Pose a provocative or thought-provoking question,
- Describe a puzzling scenario or incongruity, or
- Cite a stirring example or case study that illustrates why the research problem is important.
NOTE: Only choose one strategy for engaging your readers; avoid giving an impression that your paper is more flash than substance.
Freedman, Leora and Jerry Plotnick. Introductions and Conclusions . University College Writing Centre. University of Toronto; Introduction . The Structure, Format, Content, and Style of a Journal-Style Scientific Paper. Department of Biology. Bates College; Introductions . The Writing Center. University of North Carolina; Introductions . The Writer’s Handbook. Writing Center. University of Wisconsin, Madison; Introductions, Body Paragraphs, and Conclusions for an Argument Paper. The Writing Lab and The OWL. Purdue University; Resources for Writers: Introduction Strategies . Program in Writing and Humanistic Studies. Massachusetts Institute of Technology; Sharpling, Gerald. Writing an Introduction . Centre for Applied Linguistics, University of Warwick; Writing Your Introduction. Department of English Writing Guide. George Mason University.
Writing Tip
Avoid the "Dictionary" Introduction
Giving the dictionary definition of words related to the research problem may appear appropriate because it is important to define specific words or phrases with which readers may be unfamiliar. However, anyone can look a word up in the dictionary and a general dictionary is not a particularly authoritative source. It doesn't take into account the context of your topic and doesn't offer particularly detailed information. Also, placed in the context of a particular discipline, a term may have a different meaning than what is found in a general dictionary. If you feel that you must seek out an authoritative definition, try to find one that is from subject specific dictionaries or encyclopedias [e.g., if you are a sociology student, search for dictionaries of sociology].
Saba, Robert. The College Research Paper . Florida International University; Introductions . The Writing Center. University of North Carolina.
Another Writing Tip
When Do I Begin?
A common question asked at the start of any paper is, "where should I begin?" An equally important question to ask yourself is, "When do I begin?" Research problems in the social sciences rarely rest in isolation from the history of the issue being investigated. It is, therefore, important to lay a foundation for understanding the historical context underpinning the research problem. However, this information should be brief and succinct and begin at a point in time that best informs the reader of study's overall importance. For example, a study about coffee cultivation and export in West Africa as a key stimulus for local economic growth needs to describe the beginning of exporting coffee in the region and establishing why economic growth is important. You do not need to give a long historical explanation about coffee exportation in Africa. If a research problem demands a substantial exploration of historical context, do this in the literature review section; note in the introduction as part of your "roadmap" [see below] that you covering this in the literature review.
Yet Another Writing Tip
Always End with a Roadmap
The final paragraph or sentences of your introduction should forecast your main arguments and conclusions and provide a description of the rest of the paper [a "roadmap"] that let's the reader know where you are going and what to expect.
- << Previous: Executive Summary
- Next: Background Information >>
- Last Updated: Jul 18, 2023 11:58 AM
- URL: https://library.sacredheart.edu/c.php?g=29803
- QuickSearch
- Library Catalog
- Databases A-Z
- Publication Finder
- Course Reserves
- Citation Linker
- Digital Commons
- Our Website
Research Support
- Ask a Librarian
- Appointments
- Interlibrary Loan (ILL)
- Research Guides
- Databases by Subject
- Citation Help
Using the Library
- Reserve a Group Study Room
- Renew Books
- Honors Study Rooms
- Off-Campus Access
- Library Policies
- Library Technology
User Information
- Grad Students
- Online Students
- COVID-19 Updates
- Staff Directory
- News & Announcements
- Library Newsletter
My Accounts
- Interlibrary Loan
- Staff Site Login

FIND US ON
- If you are writing in a new discipline, you should always make sure to ask about conventions and expectations for introductions, just as you would for any other aspect of the essay. For example, while it may be acceptable to write a two-paragraph (or longer) introduction for your papers in some courses, instructors in other disciplines, such as those in some Government courses, may expect a shorter introduction that includes a preview of the argument that will follow.
- In some disciplines (Government, Economics, and others), it’s common to offer an overview in the introduction of what points you will make in your essay. In other disciplines, you will not be expected to provide this overview in your introduction.
- Avoid writing a very general opening sentence. While it may be true that “Since the dawn of time, people have been telling love stories,” it won’t help you explain what’s interesting about your topic.
- Avoid writing a “funnel” introduction in which you begin with a very broad statement about a topic and move to a narrow statement about that topic. Broad generalizations about a topic will not add to your readers’ understanding of your specific essay topic.
- Avoid beginning with a dictionary definition of a term or concept you will be writing about. If the concept is complicated or unfamiliar to your readers, you will need to define it in detail later in your essay. If it’s not complicated, you can assume your readers already know the definition.
- Avoid offering too much detail in your introduction that a reader could better understand later in the paper.
- picture_as_pdf Introductions
Have a language expert improve your writing
Run a free plagiarism check in 10 minutes, generate accurate citations for free.
- Knowledge Base
- How to write an essay introduction | 4 steps & examples
How to Write an Essay Introduction | 4 Steps & Examples
Published on February 4, 2019 by Shona McCombes . Revised on July 23, 2023.
A good introduction paragraph is an essential part of any academic essay . It sets up your argument and tells the reader what to expect.
The main goals of an introduction are to:
- Catch your reader’s attention.
- Give background on your topic.
- Present your thesis statement —the central point of your essay.
This introduction example is taken from our interactive essay example on the history of Braille.
The invention of Braille was a major turning point in the history of disability. The writing system of raised dots used by visually impaired people was developed by Louis Braille in nineteenth-century France. In a society that did not value disabled people in general, blindness was particularly stigmatized, and lack of access to reading and writing was a significant barrier to social participation. The idea of tactile reading was not entirely new, but existing methods based on sighted systems were difficult to learn and use. As the first writing system designed for blind people’s needs, Braille was a groundbreaking new accessibility tool. It not only provided practical benefits, but also helped change the cultural status of blindness. This essay begins by discussing the situation of blind people in nineteenth-century Europe. It then describes the invention of Braille and the gradual process of its acceptance within blind education. Subsequently, it explores the wide-ranging effects of this invention on blind people’s social and cultural lives.
Instantly correct all language mistakes in your text
Upload your document to correct all your mistakes in minutes
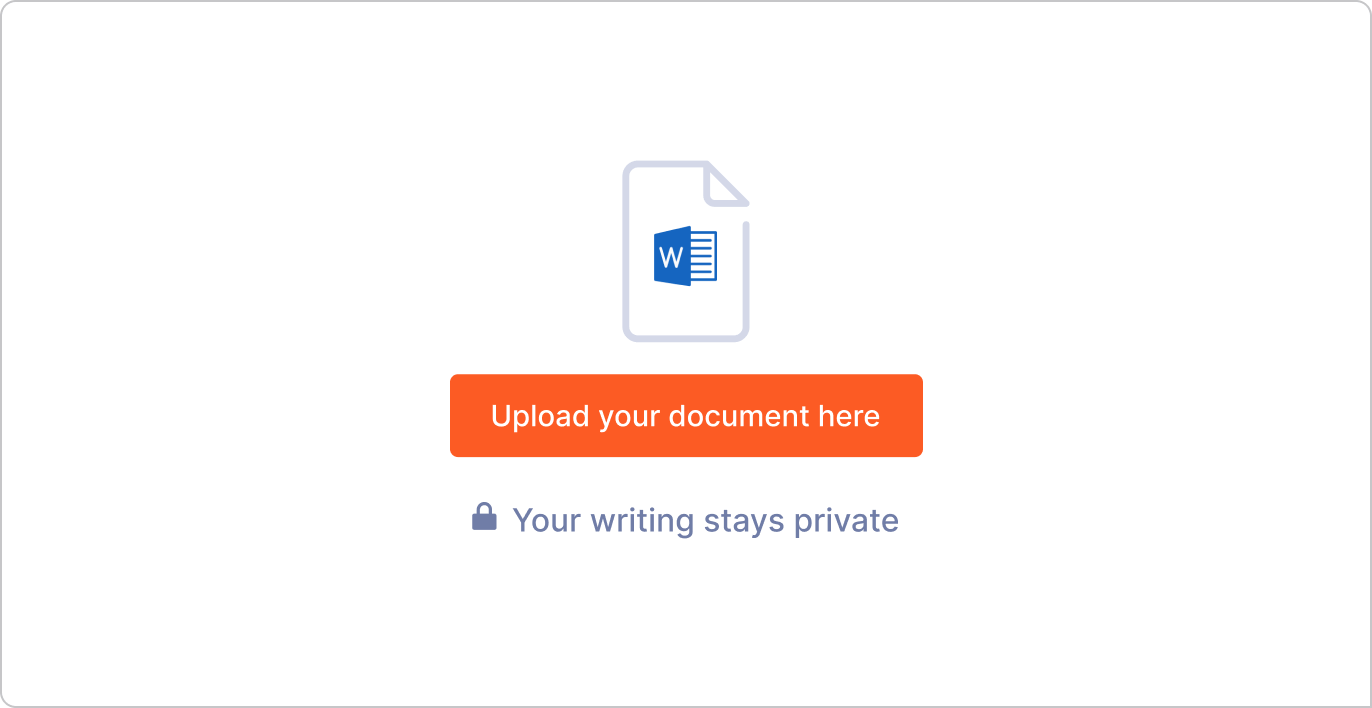
Table of contents
Step 1: hook your reader, step 2: give background information, step 3: present your thesis statement, step 4: map your essay’s structure, step 5: check and revise, more examples of essay introductions, other interesting articles, frequently asked questions about the essay introduction.
Your first sentence sets the tone for the whole essay, so spend some time on writing an effective hook.
Avoid long, dense sentences—start with something clear, concise and catchy that will spark your reader’s curiosity.
The hook should lead the reader into your essay, giving a sense of the topic you’re writing about and why it’s interesting. Avoid overly broad claims or plain statements of fact.
Examples: Writing a good hook
Take a look at these examples of weak hooks and learn how to improve them.
- Braille was an extremely important invention.
- The invention of Braille was a major turning point in the history of disability.
The first sentence is a dry fact; the second sentence is more interesting, making a bold claim about exactly why the topic is important.
- The internet is defined as “a global computer network providing a variety of information and communication facilities.”
- The spread of the internet has had a world-changing effect, not least on the world of education.
Avoid using a dictionary definition as your hook, especially if it’s an obvious term that everyone knows. The improved example here is still broad, but it gives us a much clearer sense of what the essay will be about.
- Mary Shelley’s Frankenstein is a famous book from the nineteenth century.
- Mary Shelley’s Frankenstein is often read as a crude cautionary tale about the dangers of scientific advancement.
Instead of just stating a fact that the reader already knows, the improved hook here tells us about the mainstream interpretation of the book, implying that this essay will offer a different interpretation.
Receive feedback on language, structure, and formatting
Professional editors proofread and edit your paper by focusing on:
- Academic style
- Vague sentences
- Style consistency
See an example
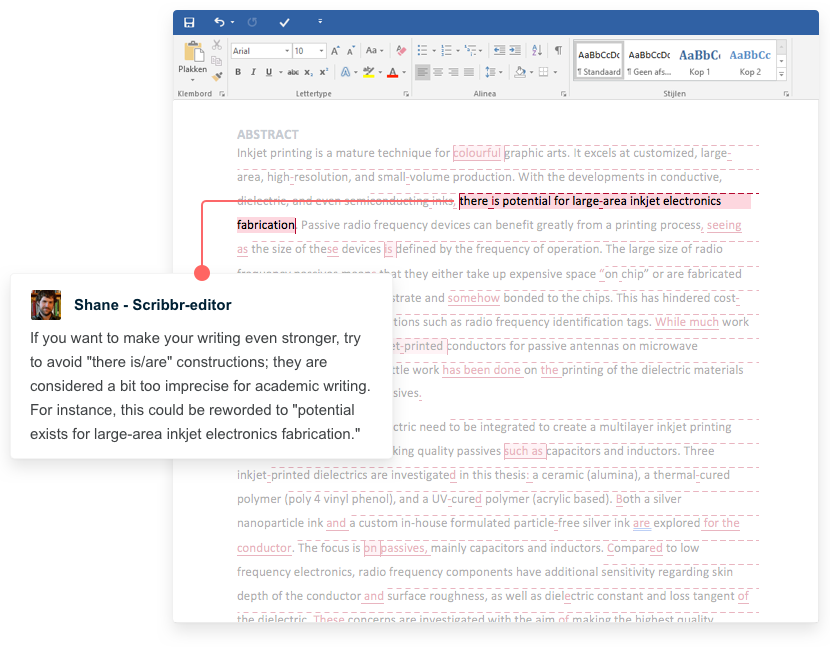
Next, give your reader the context they need to understand your topic and argument. Depending on the subject of your essay, this might include:
- Historical, geographical, or social context
- An outline of the debate you’re addressing
- A summary of relevant theories or research about the topic
- Definitions of key terms
The information here should be broad but clearly focused and relevant to your argument. Don’t give too much detail—you can mention points that you will return to later, but save your evidence and interpretation for the main body of the essay.
How much space you need for background depends on your topic and the scope of your essay. In our Braille example, we take a few sentences to introduce the topic and sketch the social context that the essay will address:
Now it’s time to narrow your focus and show exactly what you want to say about the topic. This is your thesis statement —a sentence or two that sums up your overall argument.
This is the most important part of your introduction. A good thesis isn’t just a statement of fact, but a claim that requires evidence and explanation.
The goal is to clearly convey your own position in a debate or your central point about a topic.
Particularly in longer essays, it’s helpful to end the introduction by signposting what will be covered in each part. Keep it concise and give your reader a clear sense of the direction your argument will take.
As you research and write, your argument might change focus or direction as you learn more.
For this reason, it’s often a good idea to wait until later in the writing process before you write the introduction paragraph—it can even be the very last thing you write.
When you’ve finished writing the essay body and conclusion , you should return to the introduction and check that it matches the content of the essay.
It’s especially important to make sure your thesis statement accurately represents what you do in the essay. If your argument has gone in a different direction than planned, tweak your thesis statement to match what you actually say.
To polish your writing, you can use something like a paraphrasing tool .
You can use the checklist below to make sure your introduction does everything it’s supposed to.
Checklist: Essay introduction
My first sentence is engaging and relevant.
I have introduced the topic with necessary background information.
I have defined any important terms.
My thesis statement clearly presents my main point or argument.
Everything in the introduction is relevant to the main body of the essay.
You have a strong introduction - now make sure the rest of your essay is just as good.
- Argumentative
- Literary analysis
This introduction to an argumentative essay sets up the debate about the internet and education, and then clearly states the position the essay will argue for.
The spread of the internet has had a world-changing effect, not least on the world of education. The use of the internet in academic contexts is on the rise, and its role in learning is hotly debated. For many teachers who did not grow up with this technology, its effects seem alarming and potentially harmful. This concern, while understandable, is misguided. The negatives of internet use are outweighed by its critical benefits for students and educators—as a uniquely comprehensive and accessible information source; a means of exposure to and engagement with different perspectives; and a highly flexible learning environment.
This introduction to a short expository essay leads into the topic (the invention of the printing press) and states the main point the essay will explain (the effect of this invention on European society).
In many ways, the invention of the printing press marked the end of the Middle Ages. The medieval period in Europe is often remembered as a time of intellectual and political stagnation. Prior to the Renaissance, the average person had very limited access to books and was unlikely to be literate. The invention of the printing press in the 15th century allowed for much less restricted circulation of information in Europe, paving the way for the Reformation.
This introduction to a literary analysis essay , about Mary Shelley’s Frankenstein , starts by describing a simplistic popular view of the story, and then states how the author will give a more complex analysis of the text’s literary devices.
Mary Shelley’s Frankenstein is often read as a crude cautionary tale. Arguably the first science fiction novel, its plot can be read as a warning about the dangers of scientific advancement unrestrained by ethical considerations. In this reading, and in popular culture representations of the character as a “mad scientist”, Victor Frankenstein represents the callous, arrogant ambition of modern science. However, far from providing a stable image of the character, Shelley uses shifting narrative perspectives to gradually transform our impression of Frankenstein, portraying him in an increasingly negative light as the novel goes on. While he initially appears to be a naive but sympathetic idealist, after the creature’s narrative Frankenstein begins to resemble—even in his own telling—the thoughtlessly cruel figure the creature represents him as.
If you want to know more about AI tools , college essays , or fallacies make sure to check out some of our other articles with explanations and examples or go directly to our tools!
- Ad hominem fallacy
- Post hoc fallacy
- Appeal to authority fallacy
- False cause fallacy
- Sunk cost fallacy
College essays
- Choosing Essay Topic
- Write a College Essay
- Write a Diversity Essay
- College Essay Format & Structure
- Comparing and Contrasting in an Essay
(AI) Tools
- Grammar Checker
- Paraphrasing Tool
- Text Summarizer
- AI Detector
- Plagiarism Checker
- Citation Generator
Your essay introduction should include three main things, in this order:
- An opening hook to catch the reader’s attention.
- Relevant background information that the reader needs to know.
- A thesis statement that presents your main point or argument.
The length of each part depends on the length and complexity of your essay .
The “hook” is the first sentence of your essay introduction . It should lead the reader into your essay, giving a sense of why it’s interesting.
To write a good hook, avoid overly broad statements or long, dense sentences. Try to start with something clear, concise and catchy that will spark your reader’s curiosity.
A thesis statement is a sentence that sums up the central point of your paper or essay . Everything else you write should relate to this key idea.
The thesis statement is essential in any academic essay or research paper for two main reasons:
- It gives your writing direction and focus.
- It gives the reader a concise summary of your main point.
Without a clear thesis statement, an essay can end up rambling and unfocused, leaving your reader unsure of exactly what you want to say.
The structure of an essay is divided into an introduction that presents your topic and thesis statement , a body containing your in-depth analysis and arguments, and a conclusion wrapping up your ideas.
The structure of the body is flexible, but you should always spend some time thinking about how you can organize your essay to best serve your ideas.
Cite this Scribbr article
If you want to cite this source, you can copy and paste the citation or click the “Cite this Scribbr article” button to automatically add the citation to our free Citation Generator.
McCombes, S. (2023, July 23). How to Write an Essay Introduction | 4 Steps & Examples. Scribbr. Retrieved April 8, 2024, from https://www.scribbr.com/academic-essay/introduction/
Is this article helpful?
Shona McCombes
Other students also liked, how to write a thesis statement | 4 steps & examples, academic paragraph structure | step-by-step guide & examples, how to conclude an essay | interactive example, what is your plagiarism score.
Thank you for visiting nature.com. You are using a browser version with limited support for CSS. To obtain the best experience, we recommend you use a more up to date browser (or turn off compatibility mode in Internet Explorer). In the meantime, to ensure continued support, we are displaying the site without styles and JavaScript.
- View all journals
- My Account Login
- Explore content
- About the journal
- Publish with us
- Sign up for alerts
- Open access
- Published: 26 March 2024
Predicting and improving complex beer flavor through machine learning
- Michiel Schreurs ORCID: orcid.org/0000-0002-9449-5619 1 , 2 , 3 na1 ,
- Supinya Piampongsant 1 , 2 , 3 na1 ,
- Miguel Roncoroni ORCID: orcid.org/0000-0001-7461-1427 1 , 2 , 3 na1 ,
- Lloyd Cool ORCID: orcid.org/0000-0001-9936-3124 1 , 2 , 3 , 4 ,
- Beatriz Herrera-Malaver ORCID: orcid.org/0000-0002-5096-9974 1 , 2 , 3 ,
- Christophe Vanderaa ORCID: orcid.org/0000-0001-7443-5427 4 ,
- Florian A. Theßeling 1 , 2 , 3 ,
- Łukasz Kreft ORCID: orcid.org/0000-0001-7620-4657 5 ,
- Alexander Botzki ORCID: orcid.org/0000-0001-6691-4233 5 ,
- Philippe Malcorps 6 ,
- Luk Daenen 6 ,
- Tom Wenseleers ORCID: orcid.org/0000-0002-1434-861X 4 &
- Kevin J. Verstrepen ORCID: orcid.org/0000-0002-3077-6219 1 , 2 , 3
Nature Communications volume 15 , Article number: 2368 ( 2024 ) Cite this article
50k Accesses
851 Altmetric
Metrics details
- Chemical engineering
- Gas chromatography
- Machine learning
- Metabolomics
- Taste receptors
The perception and appreciation of food flavor depends on many interacting chemical compounds and external factors, and therefore proves challenging to understand and predict. Here, we combine extensive chemical and sensory analyses of 250 different beers to train machine learning models that allow predicting flavor and consumer appreciation. For each beer, we measure over 200 chemical properties, perform quantitative descriptive sensory analysis with a trained tasting panel and map data from over 180,000 consumer reviews to train 10 different machine learning models. The best-performing algorithm, Gradient Boosting, yields models that significantly outperform predictions based on conventional statistics and accurately predict complex food features and consumer appreciation from chemical profiles. Model dissection allows identifying specific and unexpected compounds as drivers of beer flavor and appreciation. Adding these compounds results in variants of commercial alcoholic and non-alcoholic beers with improved consumer appreciation. Together, our study reveals how big data and machine learning uncover complex links between food chemistry, flavor and consumer perception, and lays the foundation to develop novel, tailored foods with superior flavors.
Similar content being viewed by others
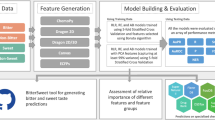
BitterSweet: Building machine learning models for predicting the bitter and sweet taste of small molecules
Rudraksh Tuwani, Somin Wadhwa & Ganesh Bagler
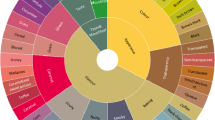
Sensory lexicon and aroma volatiles analysis of brewing malt
Xiaoxia Su, Miao Yu, … Tianyi Du
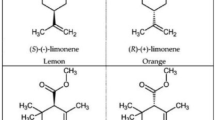
Predicting odor from molecular structure: a multi-label classification approach
Kushagra Saini & Venkatnarayan Ramanathan
Introduction
Predicting and understanding food perception and appreciation is one of the major challenges in food science. Accurate modeling of food flavor and appreciation could yield important opportunities for both producers and consumers, including quality control, product fingerprinting, counterfeit detection, spoilage detection, and the development of new products and product combinations (food pairing) 1 , 2 , 3 , 4 , 5 , 6 . Accurate models for flavor and consumer appreciation would contribute greatly to our scientific understanding of how humans perceive and appreciate flavor. Moreover, accurate predictive models would also facilitate and standardize existing food assessment methods and could supplement or replace assessments by trained and consumer tasting panels, which are variable, expensive and time-consuming 7 , 8 , 9 . Lastly, apart from providing objective, quantitative, accurate and contextual information that can help producers, models can also guide consumers in understanding their personal preferences 10 .
Despite the myriad of applications, predicting food flavor and appreciation from its chemical properties remains a largely elusive goal in sensory science, especially for complex food and beverages 11 , 12 . A key obstacle is the immense number of flavor-active chemicals underlying food flavor. Flavor compounds can vary widely in chemical structure and concentration, making them technically challenging and labor-intensive to quantify, even in the face of innovations in metabolomics, such as non-targeted metabolic fingerprinting 13 , 14 . Moreover, sensory analysis is perhaps even more complicated. Flavor perception is highly complex, resulting from hundreds of different molecules interacting at the physiochemical and sensorial level. Sensory perception is often non-linear, characterized by complex and concentration-dependent synergistic and antagonistic effects 15 , 16 , 17 , 18 , 19 , 20 , 21 that are further convoluted by the genetics, environment, culture and psychology of consumers 22 , 23 , 24 . Perceived flavor is therefore difficult to measure, with problems of sensitivity, accuracy, and reproducibility that can only be resolved by gathering sufficiently large datasets 25 . Trained tasting panels are considered the prime source of quality sensory data, but require meticulous training, are low throughput and high cost. Public databases containing consumer reviews of food products could provide a valuable alternative, especially for studying appreciation scores, which do not require formal training 25 . Public databases offer the advantage of amassing large amounts of data, increasing the statistical power to identify potential drivers of appreciation. However, public datasets suffer from biases, including a bias in the volunteers that contribute to the database, as well as confounding factors such as price, cult status and psychological conformity towards previous ratings of the product.
Classical multivariate statistics and machine learning methods have been used to predict flavor of specific compounds by, for example, linking structural properties of a compound to its potential biological activities or linking concentrations of specific compounds to sensory profiles 1 , 26 . Importantly, most previous studies focused on predicting organoleptic properties of single compounds (often based on their chemical structure) 27 , 28 , 29 , 30 , 31 , 32 , 33 , thus ignoring the fact that these compounds are present in a complex matrix in food or beverages and excluding complex interactions between compounds. Moreover, the classical statistics commonly used in sensory science 34 , 35 , 36 , 37 , 38 , 39 require a large sample size and sufficient variance amongst predictors to create accurate models. They are not fit for studying an extensive set of hundreds of interacting flavor compounds, since they are sensitive to outliers, have a high tendency to overfit and are less suited for non-linear and discontinuous relationships 40 .
In this study, we combine extensive chemical analyses and sensory data of a set of different commercial beers with machine learning approaches to develop models that predict taste, smell, mouthfeel and appreciation from compound concentrations. Beer is particularly suited to model the relationship between chemistry, flavor and appreciation. First, beer is a complex product, consisting of thousands of flavor compounds that partake in complex sensory interactions 41 , 42 , 43 . This chemical diversity arises from the raw materials (malt, yeast, hops, water and spices) and biochemical conversions during the brewing process (kilning, mashing, boiling, fermentation, maturation and aging) 44 , 45 . Second, the advent of the internet saw beer consumers embrace online review platforms, such as RateBeer (ZX Ventures, Anheuser-Busch InBev SA/NV) and BeerAdvocate (Next Glass, inc.). In this way, the beer community provides massive data sets of beer flavor and appreciation scores, creating extraordinarily large sensory databases to complement the analyses of our professional sensory panel. Specifically, we characterize over 200 chemical properties of 250 commercial beers, spread across 22 beer styles, and link these to the descriptive sensory profiling data of a 16-person in-house trained tasting panel and data acquired from over 180,000 public consumer reviews. These unique and extensive datasets enable us to train a suite of machine learning models to predict flavor and appreciation from a beer’s chemical profile. Dissection of the best-performing models allows us to pinpoint specific compounds as potential drivers of beer flavor and appreciation. Follow-up experiments confirm the importance of these compounds and ultimately allow us to significantly improve the flavor and appreciation of selected commercial beers. Together, our study represents a significant step towards understanding complex flavors and reinforces the value of machine learning to develop and refine complex foods. In this way, it represents a stepping stone for further computer-aided food engineering applications 46 .
To generate a comprehensive dataset on beer flavor, we selected 250 commercial Belgian beers across 22 different beer styles (Supplementary Fig. S1 ). Beers with ≤ 4.2% alcohol by volume (ABV) were classified as non-alcoholic and low-alcoholic. Blonds and Tripels constitute a significant portion of the dataset (12.4% and 11.2%, respectively) reflecting their presence on the Belgian beer market and the heterogeneity of beers within these styles. By contrast, lager beers are less diverse and dominated by a handful of brands. Rare styles such as Brut or Faro make up only a small fraction of the dataset (2% and 1%, respectively) because fewer of these beers are produced and because they are dominated by distinct characteristics in terms of flavor and chemical composition.
Extensive analysis identifies relationships between chemical compounds in beer
For each beer, we measured 226 different chemical properties, including common brewing parameters such as alcohol content, iso-alpha acids, pH, sugar concentration 47 , and over 200 flavor compounds (Methods, Supplementary Table S1 ). A large portion (37.2%) are terpenoids arising from hopping, responsible for herbal and fruity flavors 16 , 48 . A second major category are yeast metabolites, such as esters and alcohols, that result in fruity and solvent notes 48 , 49 , 50 . Other measured compounds are primarily derived from malt, or other microbes such as non- Saccharomyces yeasts and bacteria (‘wild flora’). Compounds that arise from spices or staling are labeled under ‘Others’. Five attributes (caloric value, total acids and total ester, hop aroma and sulfur compounds) are calculated from multiple individually measured compounds.
As a first step in identifying relationships between chemical properties, we determined correlations between the concentrations of the compounds (Fig. 1 , upper panel, Supplementary Data 1 and 2 , and Supplementary Fig. S2 . For the sake of clarity, only a subset of the measured compounds is shown in Fig. 1 ). Compounds of the same origin typically show a positive correlation, while absence of correlation hints at parameters varying independently. For example, the hop aroma compounds citronellol, and alpha-terpineol show moderate correlations with each other (Spearman’s rho=0.39 and 0.57), but not with the bittering hop component iso-alpha acids (Spearman’s rho=0.16 and −0.07). This illustrates how brewers can independently modify hop aroma and bitterness by selecting hop varieties and dosage time. If hops are added early in the boiling phase, chemical conversions increase bitterness while aromas evaporate, conversely, late addition of hops preserves aroma but limits bitterness 51 . Similarly, hop-derived iso-alpha acids show a strong anti-correlation with lactic acid and acetic acid, likely reflecting growth inhibition of lactic acid and acetic acid bacteria, or the consequent use of fewer hops in sour beer styles, such as West Flanders ales and Fruit beers, that rely on these bacteria for their distinct flavors 52 . Finally, yeast-derived esters (ethyl acetate, ethyl decanoate, ethyl hexanoate, ethyl octanoate) and alcohols (ethanol, isoamyl alcohol, isobutanol, and glycerol), correlate with Spearman coefficients above 0.5, suggesting that these secondary metabolites are correlated with the yeast genetic background and/or fermentation parameters and may be difficult to influence individually, although the choice of yeast strain may offer some control 53 .
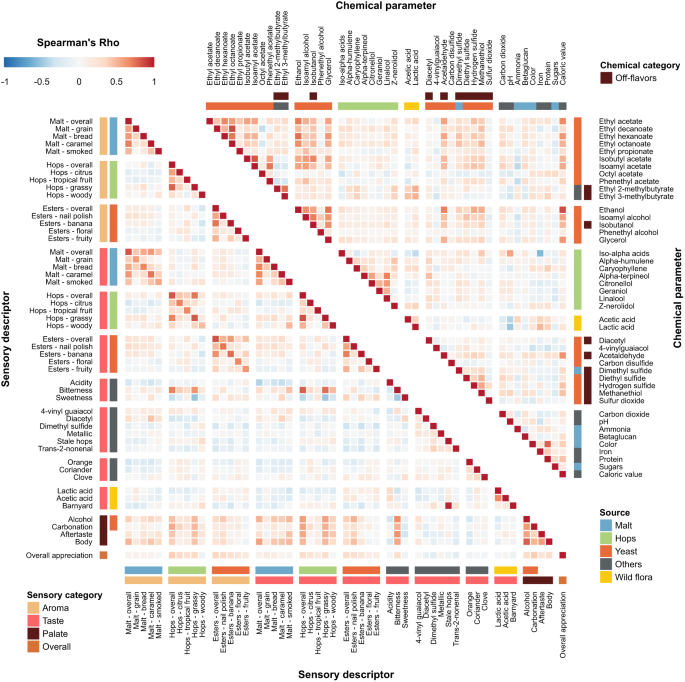
Spearman rank correlations are shown. Descriptors are grouped according to their origin (malt (blue), hops (green), yeast (red), wild flora (yellow), Others (black)), and sensory aspect (aroma, taste, palate, and overall appreciation). Please note that for the chemical compounds, for the sake of clarity, only a subset of the total number of measured compounds is shown, with an emphasis on the key compounds for each source. For more details, see the main text and Methods section. Chemical data can be found in Supplementary Data 1 , correlations between all chemical compounds are depicted in Supplementary Fig. S2 and correlation values can be found in Supplementary Data 2 . See Supplementary Data 4 for sensory panel assessments and Supplementary Data 5 for correlation values between all sensory descriptors.
Interestingly, different beer styles show distinct patterns for some flavor compounds (Supplementary Fig. S3 ). These observations agree with expectations for key beer styles, and serve as a control for our measurements. For instance, Stouts generally show high values for color (darker), while hoppy beers contain elevated levels of iso-alpha acids, compounds associated with bitter hop taste. Acetic and lactic acid are not prevalent in most beers, with notable exceptions such as Kriek, Lambic, Faro, West Flanders ales and Flanders Old Brown, which use acid-producing bacteria ( Lactobacillus and Pediococcus ) or unconventional yeast ( Brettanomyces ) 54 , 55 . Glycerol, ethanol and esters show similar distributions across all beer styles, reflecting their common origin as products of yeast metabolism during fermentation 45 , 53 . Finally, low/no-alcohol beers contain low concentrations of glycerol and esters. This is in line with the production process for most of the low/no-alcohol beers in our dataset, which are produced through limiting fermentation or by stripping away alcohol via evaporation or dialysis, with both methods having the unintended side-effect of reducing the amount of flavor compounds in the final beer 56 , 57 .
Besides expected associations, our data also reveals less trivial associations between beer styles and specific parameters. For example, geraniol and citronellol, two monoterpenoids responsible for citrus, floral and rose flavors and characteristic of Citra hops, are found in relatively high amounts in Christmas, Saison, and Brett/co-fermented beers, where they may originate from terpenoid-rich spices such as coriander seeds instead of hops 58 .
Tasting panel assessments reveal sensorial relationships in beer
To assess the sensory profile of each beer, a trained tasting panel evaluated each of the 250 beers for 50 sensory attributes, including different hop, malt and yeast flavors, off-flavors and spices. Panelists used a tasting sheet (Supplementary Data 3 ) to score the different attributes. Panel consistency was evaluated by repeating 12 samples across different sessions and performing ANOVA. In 95% of cases no significant difference was found across sessions ( p > 0.05), indicating good panel consistency (Supplementary Table S2 ).
Aroma and taste perception reported by the trained panel are often linked (Fig. 1 , bottom left panel and Supplementary Data 4 and 5 ), with high correlations between hops aroma and taste (Spearman’s rho=0.83). Bitter taste was found to correlate with hop aroma and taste in general (Spearman’s rho=0.80 and 0.69), and particularly with “grassy” noble hops (Spearman’s rho=0.75). Barnyard flavor, most often associated with sour beers, is identified together with stale hops (Spearman’s rho=0.97) that are used in these beers. Lactic and acetic acid, which often co-occur, are correlated (Spearman’s rho=0.66). Interestingly, sweetness and bitterness are anti-correlated (Spearman’s rho = −0.48), confirming the hypothesis that they mask each other 59 , 60 . Beer body is highly correlated with alcohol (Spearman’s rho = 0.79), and overall appreciation is found to correlate with multiple aspects that describe beer mouthfeel (alcohol, carbonation; Spearman’s rho= 0.32, 0.39), as well as with hop and ester aroma intensity (Spearman’s rho=0.39 and 0.35).
Similar to the chemical analyses, sensorial analyses confirmed typical features of specific beer styles (Supplementary Fig. S4 ). For example, sour beers (Faro, Flanders Old Brown, Fruit beer, Kriek, Lambic, West Flanders ale) were rated acidic, with flavors of both acetic and lactic acid. Hoppy beers were found to be bitter and showed hop-associated aromas like citrus and tropical fruit. Malt taste is most detected among scotch, stout/porters, and strong ales, while low/no-alcohol beers, which often have a reputation for being ‘worty’ (reminiscent of unfermented, sweet malt extract) appear in the middle. Unsurprisingly, hop aromas are most strongly detected among hoppy beers. Like its chemical counterpart (Supplementary Fig. S3 ), acidity shows a right-skewed distribution, with the most acidic beers being Krieks, Lambics, and West Flanders ales.
Tasting panel assessments of specific flavors correlate with chemical composition
We find that the concentrations of several chemical compounds strongly correlate with specific aroma or taste, as evaluated by the tasting panel (Fig. 2 , Supplementary Fig. S5 , Supplementary Data 6 ). In some cases, these correlations confirm expectations and serve as a useful control for data quality. For example, iso-alpha acids, the bittering compounds in hops, strongly correlate with bitterness (Spearman’s rho=0.68), while ethanol and glycerol correlate with tasters’ perceptions of alcohol and body, the mouthfeel sensation of fullness (Spearman’s rho=0.82/0.62 and 0.72/0.57 respectively) and darker color from roasted malts is a good indication of malt perception (Spearman’s rho=0.54).
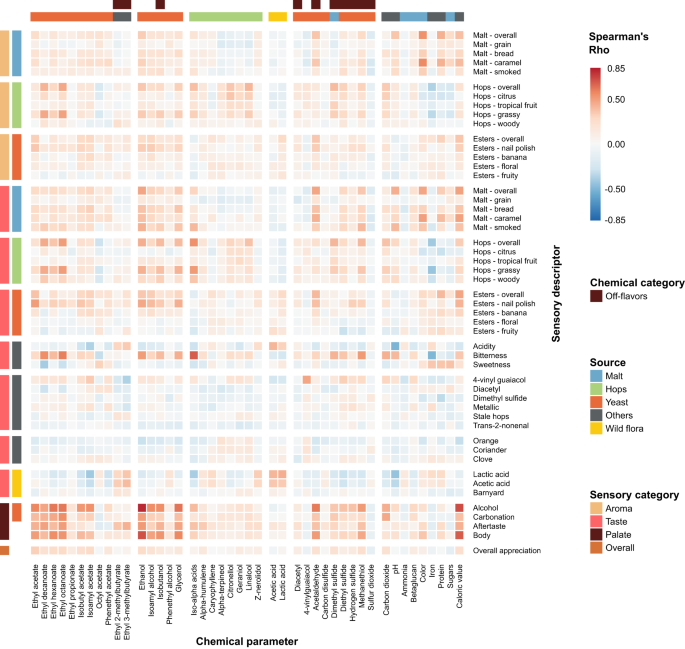
Heatmap colors indicate Spearman’s Rho. Axes are organized according to sensory categories (aroma, taste, mouthfeel, overall), chemical categories and chemical sources in beer (malt (blue), hops (green), yeast (red), wild flora (yellow), Others (black)). See Supplementary Data 6 for all correlation values.
Interestingly, for some relationships between chemical compounds and perceived flavor, correlations are weaker than expected. For example, the rose-smelling phenethyl acetate only weakly correlates with floral aroma. This hints at more complex relationships and interactions between compounds and suggests a need for a more complex model than simple correlations. Lastly, we uncovered unexpected correlations. For instance, the esters ethyl decanoate and ethyl octanoate appear to correlate slightly with hop perception and bitterness, possibly due to their fruity flavor. Iron is anti-correlated with hop aromas and bitterness, most likely because it is also anti-correlated with iso-alpha acids. This could be a sign of metal chelation of hop acids 61 , given that our analyses measure unbound hop acids and total iron content, or could result from the higher iron content in dark and Fruit beers, which typically have less hoppy and bitter flavors 62 .
Public consumer reviews complement expert panel data
To complement and expand the sensory data of our trained tasting panel, we collected 180,000 reviews of our 250 beers from the online consumer review platform RateBeer. This provided numerical scores for beer appearance, aroma, taste, palate, overall quality as well as the average overall score.
Public datasets are known to suffer from biases, such as price, cult status and psychological conformity towards previous ratings of a product. For example, prices correlate with appreciation scores for these online consumer reviews (rho=0.49, Supplementary Fig. S6 ), but not for our trained tasting panel (rho=0.19). This suggests that prices affect consumer appreciation, which has been reported in wine 63 , while blind tastings are unaffected. Moreover, we observe that some beer styles, like lagers and non-alcoholic beers, generally receive lower scores, reflecting that online reviewers are mostly beer aficionados with a preference for specialty beers over lager beers. In general, we find a modest correlation between our trained panel’s overall appreciation score and the online consumer appreciation scores (Fig. 3 , rho=0.29). Apart from the aforementioned biases in the online datasets, serving temperature, sample freshness and surroundings, which are all tightly controlled during the tasting panel sessions, can vary tremendously across online consumers and can further contribute to (among others, appreciation) differences between the two categories of tasters. Importantly, in contrast to the overall appreciation scores, for many sensory aspects the results from the professional panel correlated well with results obtained from RateBeer reviews. Correlations were highest for features that are relatively easy to recognize even for untrained tasters, like bitterness, sweetness, alcohol and malt aroma (Fig. 3 and below).
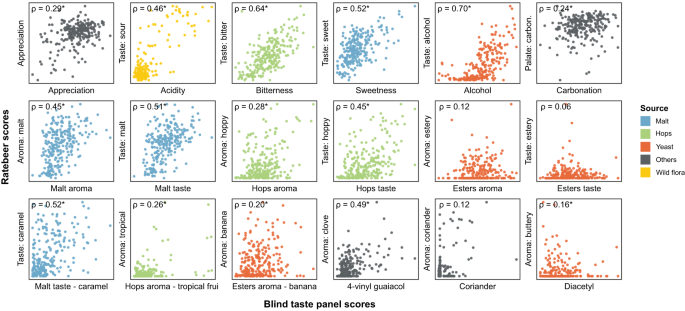
RateBeer text mining results can be found in Supplementary Data 7 . Rho values shown are Spearman correlation values, with asterisks indicating significant correlations ( p < 0.05, two-sided). All p values were smaller than 0.001, except for Esters aroma (0.0553), Esters taste (0.3275), Esters aroma—banana (0.0019), Coriander (0.0508) and Diacetyl (0.0134).
Besides collecting consumer appreciation from these online reviews, we developed automated text analysis tools to gather additional data from review texts (Supplementary Data 7 ). Processing review texts on the RateBeer database yielded comparable results to the scores given by the trained panel for many common sensory aspects, including acidity, bitterness, sweetness, alcohol, malt, and hop tastes (Fig. 3 ). This is in line with what would be expected, since these attributes require less training for accurate assessment and are less influenced by environmental factors such as temperature, serving glass and odors in the environment. Consumer reviews also correlate well with our trained panel for 4-vinyl guaiacol, a compound associated with a very characteristic aroma. By contrast, correlations for more specific aromas like ester, coriander or diacetyl are underrepresented in the online reviews, underscoring the importance of using a trained tasting panel and standardized tasting sheets with explicit factors to be scored for evaluating specific aspects of a beer. Taken together, our results suggest that public reviews are trustworthy for some, but not all, flavor features and can complement or substitute taste panel data for these sensory aspects.
Models can predict beer sensory profiles from chemical data
The rich datasets of chemical analyses, tasting panel assessments and public reviews gathered in the first part of this study provided us with a unique opportunity to develop predictive models that link chemical data to sensorial features. Given the complexity of beer flavor, basic statistical tools such as correlations or linear regression may not always be the most suitable for making accurate predictions. Instead, we applied different machine learning models that can model both simple linear and complex interactive relationships. Specifically, we constructed a set of regression models to predict (a) trained panel scores for beer flavor and quality and (b) public reviews’ appreciation scores from beer chemical profiles. We trained and tested 10 different models (Methods), 3 linear regression-based models (simple linear regression with first-order interactions (LR), lasso regression with first-order interactions (Lasso), partial least squares regressor (PLSR)), 5 decision tree models (AdaBoost regressor (ABR), extra trees (ET), gradient boosting regressor (GBR), random forest (RF) and XGBoost regressor (XGBR)), 1 support vector regression (SVR), and 1 artificial neural network (ANN) model.
To compare the performance of our machine learning models, the dataset was randomly split into a training and test set, stratified by beer style. After a model was trained on data in the training set, its performance was evaluated on its ability to predict the test dataset obtained from multi-output models (based on the coefficient of determination, see Methods). Additionally, individual-attribute models were ranked per descriptor and the average rank was calculated, as proposed by Korneva et al. 64 . Importantly, both ways of evaluating the models’ performance agreed in general. Performance of the different models varied (Table 1 ). It should be noted that all models perform better at predicting RateBeer results than results from our trained tasting panel. One reason could be that sensory data is inherently variable, and this variability is averaged out with the large number of public reviews from RateBeer. Additionally, all tree-based models perform better at predicting taste than aroma. Linear models (LR) performed particularly poorly, with negative R 2 values, due to severe overfitting (training set R 2 = 1). Overfitting is a common issue in linear models with many parameters and limited samples, especially with interaction terms further amplifying the number of parameters. L1 regularization (Lasso) successfully overcomes this overfitting, out-competing multiple tree-based models on the RateBeer dataset. Similarly, the dimensionality reduction of PLSR avoids overfitting and improves performance, to some extent. Still, tree-based models (ABR, ET, GBR, RF and XGBR) show the best performance, out-competing the linear models (LR, Lasso, PLSR) commonly used in sensory science 65 .
GBR models showed the best overall performance in predicting sensory responses from chemical information, with R 2 values up to 0.75 depending on the predicted sensory feature (Supplementary Table S4 ). The GBR models predict consumer appreciation (RateBeer) better than our trained panel’s appreciation (R 2 value of 0.67 compared to R 2 value of 0.09) (Supplementary Table S3 and Supplementary Table S4 ). ANN models showed intermediate performance, likely because neural networks typically perform best with larger datasets 66 . The SVR shows intermediate performance, mostly due to the weak predictions of specific attributes that lower the overall performance (Supplementary Table S4 ).
Model dissection identifies specific, unexpected compounds as drivers of consumer appreciation
Next, we leveraged our models to infer important contributors to sensory perception and consumer appreciation. Consumer preference is a crucial sensory aspects, because a product that shows low consumer appreciation scores often does not succeed commercially 25 . Additionally, the requirement for a large number of representative evaluators makes consumer trials one of the more costly and time-consuming aspects of product development. Hence, a model for predicting chemical drivers of overall appreciation would be a welcome addition to the available toolbox for food development and optimization.
Since GBR models on our RateBeer dataset showed the best overall performance, we focused on these models. Specifically, we used two approaches to identify important contributors. First, rankings of the most important predictors for each sensorial trait in the GBR models were obtained based on impurity-based feature importance (mean decrease in impurity). High-ranked parameters were hypothesized to be either the true causal chemical properties underlying the trait, to correlate with the actual causal properties, or to take part in sensory interactions affecting the trait 67 (Fig. 4A ). In a second approach, we used SHAP 68 to determine which parameters contributed most to the model for making predictions of consumer appreciation (Fig. 4B ). SHAP calculates parameter contributions to model predictions on a per-sample basis, which can be aggregated into an importance score.
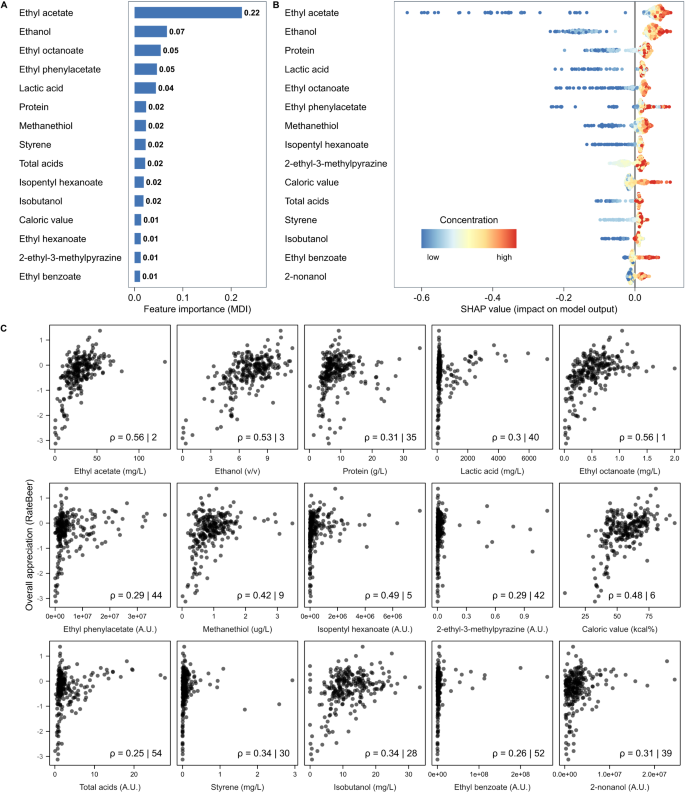
A The impurity-based feature importance (mean deviance in impurity, MDI) calculated from the Gradient Boosting Regression (GBR) model predicting RateBeer appreciation scores. The top 15 highest ranked chemical properties are shown. B SHAP summary plot for the top 15 parameters contributing to our GBR model. Each point on the graph represents a sample from our dataset. The color represents the concentration of that parameter, with bluer colors representing low values and redder colors representing higher values. Greater absolute values on the horizontal axis indicate a higher impact of the parameter on the prediction of the model. C Spearman correlations between the 15 most important chemical properties and consumer overall appreciation. Numbers indicate the Spearman Rho correlation coefficient, and the rank of this correlation compared to all other correlations. The top 15 important compounds were determined using SHAP (panel B).
Both approaches identified ethyl acetate as the most predictive parameter for beer appreciation (Fig. 4 ). Ethyl acetate is the most abundant ester in beer with a typical ‘fruity’, ‘solvent’ and ‘alcoholic’ flavor, but is often considered less important than other esters like isoamyl acetate. The second most important parameter identified by SHAP is ethanol, the most abundant beer compound after water. Apart from directly contributing to beer flavor and mouthfeel, ethanol drastically influences the physical properties of beer, dictating how easily volatile compounds escape the beer matrix to contribute to beer aroma 69 . Importantly, it should also be noted that the importance of ethanol for appreciation is likely inflated by the very low appreciation scores of non-alcoholic beers (Supplementary Fig. S4 ). Despite not often being considered a driver of beer appreciation, protein level also ranks highly in both approaches, possibly due to its effect on mouthfeel and body 70 . Lactic acid, which contributes to the tart taste of sour beers, is the fourth most important parameter identified by SHAP, possibly due to the generally high appreciation of sour beers in our dataset.
Interestingly, some of the most important predictive parameters for our model are not well-established as beer flavors or are even commonly regarded as being negative for beer quality. For example, our models identify methanethiol and ethyl phenyl acetate, an ester commonly linked to beer staling 71 , as a key factor contributing to beer appreciation. Although there is no doubt that high concentrations of these compounds are considered unpleasant, the positive effects of modest concentrations are not yet known 72 , 73 .
To compare our approach to conventional statistics, we evaluated how well the 15 most important SHAP-derived parameters correlate with consumer appreciation (Fig. 4C ). Interestingly, only 6 of the properties derived by SHAP rank amongst the top 15 most correlated parameters. For some chemical compounds, the correlations are so low that they would have likely been considered unimportant. For example, lactic acid, the fourth most important parameter, shows a bimodal distribution for appreciation, with sour beers forming a separate cluster, that is missed entirely by the Spearman correlation. Additionally, the correlation plots reveal outliers, emphasizing the need for robust analysis tools. Together, this highlights the need for alternative models, like the Gradient Boosting model, that better grasp the complexity of (beer) flavor.
Finally, to observe the relationships between these chemical properties and their predicted targets, partial dependence plots were constructed for the six most important predictors of consumer appreciation 74 , 75 , 76 (Supplementary Fig. S7 ). One-way partial dependence plots show how a change in concentration affects the predicted appreciation. These plots reveal an important limitation of our models: appreciation predictions remain constant at ever-increasing concentrations. This implies that once a threshold concentration is reached, further increasing the concentration does not affect appreciation. This is false, as it is well-documented that certain compounds become unpleasant at high concentrations, including ethyl acetate (‘nail polish’) 77 and methanethiol (‘sulfury’ and ‘rotten cabbage’) 78 . The inability of our models to grasp that flavor compounds have optimal levels, above which they become negative, is a consequence of working with commercial beer brands where (off-)flavors are rarely too high to negatively impact the product. The two-way partial dependence plots show how changing the concentration of two compounds influences predicted appreciation, visualizing their interactions (Supplementary Fig. S7 ). In our case, the top 5 parameters are dominated by additive or synergistic interactions, with high concentrations for both compounds resulting in the highest predicted appreciation.
To assess the robustness of our best-performing models and model predictions, we performed 100 iterations of the GBR, RF and ET models. In general, all iterations of the models yielded similar performance (Supplementary Fig. S8 ). Moreover, the main predictors (including the top predictors ethanol and ethyl acetate) remained virtually the same, especially for GBR and RF. For the iterations of the ET model, we did observe more variation in the top predictors, which is likely a consequence of the model’s inherent random architecture in combination with co-correlations between certain predictors. However, even in this case, several of the top predictors (ethanol and ethyl acetate) remain unchanged, although their rank in importance changes (Supplementary Fig. S8 ).
Next, we investigated if a combination of RateBeer and trained panel data into one consolidated dataset would lead to stronger models, under the hypothesis that such a model would suffer less from bias in the datasets. A GBR model was trained to predict appreciation on the combined dataset. This model underperformed compared to the RateBeer model, both in the native case and when including a dataset identifier (R 2 = 0.67, 0.26 and 0.42 respectively). For the latter, the dataset identifier is the most important feature (Supplementary Fig. S9 ), while most of the feature importance remains unchanged, with ethyl acetate and ethanol ranking highest, like in the original model trained only on RateBeer data. It seems that the large variation in the panel dataset introduces noise, weakening the models’ performances and reliability. In addition, it seems reasonable to assume that both datasets are fundamentally different, with the panel dataset obtained by blind tastings by a trained professional panel.
Lastly, we evaluated whether beer style identifiers would further enhance the model’s performance. A GBR model was trained with parameters that explicitly encoded the styles of the samples. This did not improve model performance (R2 = 0.66 with style information vs R2 = 0.67). The most important chemical features are consistent with the model trained without style information (eg. ethanol and ethyl acetate), and with the exception of the most preferred (strong ale) and least preferred (low/no-alcohol) styles, none of the styles were among the most important features (Supplementary Fig. S9 , Supplementary Table S5 and S6 ). This is likely due to a combination of style-specific chemical signatures, such as iso-alpha acids and lactic acid, that implicitly convey style information to the original models, as well as the low number of samples belonging to some styles, making it difficult for the model to learn style-specific patterns. Moreover, beer styles are not rigorously defined, with some styles overlapping in features and some beers being misattributed to a specific style, all of which leads to more noise in models that use style parameters.
Model validation
To test if our predictive models give insight into beer appreciation, we set up experiments aimed at improving existing commercial beers. We specifically selected overall appreciation as the trait to be examined because of its complexity and commercial relevance. Beer flavor comprises a complex bouquet rather than single aromas and tastes 53 . Hence, adding a single compound to the extent that a difference is noticeable may lead to an unbalanced, artificial flavor. Therefore, we evaluated the effect of combinations of compounds. Because Blond beers represent the most extensive style in our dataset, we selected a beer from this style as the starting material for these experiments (Beer 64 in Supplementary Data 1 ).
In the first set of experiments, we adjusted the concentrations of compounds that made up the most important predictors of overall appreciation (ethyl acetate, ethanol, lactic acid, ethyl phenyl acetate) together with correlated compounds (ethyl hexanoate, isoamyl acetate, glycerol), bringing them up to 95 th percentile ethanol-normalized concentrations (Methods) within the Blond group (‘Spiked’ concentration in Fig. 5A ). Compared to controls, the spiked beers were found to have significantly improved overall appreciation among trained panelists, with panelist noting increased intensity of ester flavors, sweetness, alcohol, and body fullness (Fig. 5B ). To disentangle the contribution of ethanol to these results, a second experiment was performed without the addition of ethanol. This resulted in a similar outcome, including increased perception of alcohol and overall appreciation.
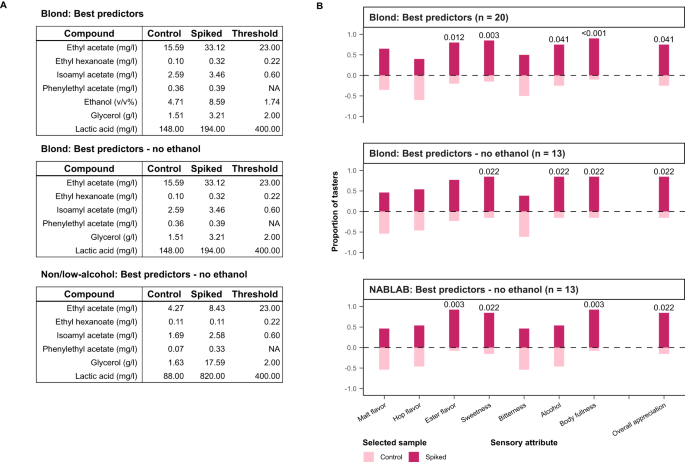
Adding the top chemical compounds, identified as best predictors of appreciation by our model, into poorly appreciated beers results in increased appreciation from our trained panel. Results of sensory tests between base beers and those spiked with compounds identified as the best predictors by the model. A Blond and Non/Low-alcohol (0.0% ABV) base beers were brought up to 95th-percentile ethanol-normalized concentrations within each style. B For each sensory attribute, tasters indicated the more intense sample and selected the sample they preferred. The numbers above the bars correspond to the p values that indicate significant changes in perceived flavor (two-sided binomial test: alpha 0.05, n = 20 or 13).
In a last experiment, we tested whether using the model’s predictions can boost the appreciation of a non-alcoholic beer (beer 223 in Supplementary Data 1 ). Again, the addition of a mixture of predicted compounds (omitting ethanol, in this case) resulted in a significant increase in appreciation, body, ester flavor and sweetness.
Predicting flavor and consumer appreciation from chemical composition is one of the ultimate goals of sensory science. A reliable, systematic and unbiased way to link chemical profiles to flavor and food appreciation would be a significant asset to the food and beverage industry. Such tools would substantially aid in quality control and recipe development, offer an efficient and cost-effective alternative to pilot studies and consumer trials and would ultimately allow food manufacturers to produce superior, tailor-made products that better meet the demands of specific consumer groups more efficiently.
A limited set of studies have previously tried, to varying degrees of success, to predict beer flavor and beer popularity based on (a limited set of) chemical compounds and flavors 79 , 80 . Current sensitive, high-throughput technologies allow measuring an unprecedented number of chemical compounds and properties in a large set of samples, yielding a dataset that can train models that help close the gaps between chemistry and flavor, even for a complex natural product like beer. To our knowledge, no previous research gathered data at this scale (250 samples, 226 chemical parameters, 50 sensory attributes and 5 consumer scores) to disentangle and validate the chemical aspects driving beer preference using various machine-learning techniques. We find that modern machine learning models outperform conventional statistical tools, such as correlations and linear models, and can successfully predict flavor appreciation from chemical composition. This could be attributed to the natural incorporation of interactions and non-linear or discontinuous effects in machine learning models, which are not easily grasped by the linear model architecture. While linear models and partial least squares regression represent the most widespread statistical approaches in sensory science, in part because they allow interpretation 65 , 81 , 82 , modern machine learning methods allow for building better predictive models while preserving the possibility to dissect and exploit the underlying patterns. Of the 10 different models we trained, tree-based models, such as our best performing GBR, showed the best overall performance in predicting sensory responses from chemical information, outcompeting artificial neural networks. This agrees with previous reports for models trained on tabular data 83 . Our results are in line with the findings of Colantonio et al. who also identified the gradient boosting architecture as performing best at predicting appreciation and flavor (of tomatoes and blueberries, in their specific study) 26 . Importantly, besides our larger experimental scale, we were able to directly confirm our models’ predictions in vivo.
Our study confirms that flavor compound concentration does not always correlate with perception, suggesting complex interactions that are often missed by more conventional statistics and simple models. Specifically, we find that tree-based algorithms may perform best in developing models that link complex food chemistry with aroma. Furthermore, we show that massive datasets of untrained consumer reviews provide a valuable source of data, that can complement or even replace trained tasting panels, especially for appreciation and basic flavors, such as sweetness and bitterness. This holds despite biases that are known to occur in such datasets, such as price or conformity bias. Moreover, GBR models predict taste better than aroma. This is likely because taste (e.g. bitterness) often directly relates to the corresponding chemical measurements (e.g., iso-alpha acids), whereas such a link is less clear for aromas, which often result from the interplay between multiple volatile compounds. We also find that our models are best at predicting acidity and alcohol, likely because there is a direct relation between the measured chemical compounds (acids and ethanol) and the corresponding perceived sensorial attribute (acidity and alcohol), and because even untrained consumers are generally able to recognize these flavors and aromas.
The predictions of our final models, trained on review data, hold even for blind tastings with small groups of trained tasters, as demonstrated by our ability to validate specific compounds as drivers of beer flavor and appreciation. Since adding a single compound to the extent of a noticeable difference may result in an unbalanced flavor profile, we specifically tested our identified key drivers as a combination of compounds. While this approach does not allow us to validate if a particular single compound would affect flavor and/or appreciation, our experiments do show that this combination of compounds increases consumer appreciation.
It is important to stress that, while it represents an important step forward, our approach still has several major limitations. A key weakness of the GBR model architecture is that amongst co-correlating variables, the largest main effect is consistently preferred for model building. As a result, co-correlating variables often have artificially low importance scores, both for impurity and SHAP-based methods, like we observed in the comparison to the more randomized Extra Trees models. This implies that chemicals identified as key drivers of a specific sensory feature by GBR might not be the true causative compounds, but rather co-correlate with the actual causative chemical. For example, the high importance of ethyl acetate could be (partially) attributed to the total ester content, ethanol or ethyl hexanoate (rho=0.77, rho=0.72 and rho=0.68), while ethyl phenylacetate could hide the importance of prenyl isobutyrate and ethyl benzoate (rho=0.77 and rho=0.76). Expanding our GBR model to include beer style as a parameter did not yield additional power or insight. This is likely due to style-specific chemical signatures, such as iso-alpha acids and lactic acid, that implicitly convey style information to the original model, as well as the smaller sample size per style, limiting the power to uncover style-specific patterns. This can be partly attributed to the curse of dimensionality, where the high number of parameters results in the models mainly incorporating single parameter effects, rather than complex interactions such as style-dependent effects 67 . A larger number of samples may overcome some of these limitations and offer more insight into style-specific effects. On the other hand, beer style is not a rigid scientific classification, and beers within one style often differ a lot, which further complicates the analysis of style as a model factor.
Our study is limited to beers from Belgian breweries. Although these beers cover a large portion of the beer styles available globally, some beer styles and consumer patterns may be missing, while other features might be overrepresented. For example, many Belgian ales exhibit yeast-driven flavor profiles, which is reflected in the chemical drivers of appreciation discovered by this study. In future work, expanding the scope to include diverse markets and beer styles could lead to the identification of even more drivers of appreciation and better models for special niche products that were not present in our beer set.
In addition to inherent limitations of GBR models, there are also some limitations associated with studying food aroma. Even if our chemical analyses measured most of the known aroma compounds, the total number of flavor compounds in complex foods like beer is still larger than the subset we were able to measure in this study. For example, hop-derived thiols, that influence flavor at very low concentrations, are notoriously difficult to measure in a high-throughput experiment. Moreover, consumer perception remains subjective and prone to biases that are difficult to avoid. It is also important to stress that the models are still immature and that more extensive datasets will be crucial for developing more complete models in the future. Besides more samples and parameters, our dataset does not include any demographic information about the tasters. Including such data could lead to better models that grasp external factors like age and culture. Another limitation is that our set of beers consists of high-quality end-products and lacks beers that are unfit for sale, which limits the current model in accurately predicting products that are appreciated very badly. Finally, while models could be readily applied in quality control, their use in sensory science and product development is restrained by their inability to discern causal relationships. Given that the models cannot distinguish compounds that genuinely drive consumer perception from those that merely correlate, validation experiments are essential to identify true causative compounds.
Despite the inherent limitations, dissection of our models enabled us to pinpoint specific molecules as potential drivers of beer aroma and consumer appreciation, including compounds that were unexpected and would not have been identified using standard approaches. Important drivers of beer appreciation uncovered by our models include protein levels, ethyl acetate, ethyl phenyl acetate and lactic acid. Currently, many brewers already use lactic acid to acidify their brewing water and ensure optimal pH for enzymatic activity during the mashing process. Our results suggest that adding lactic acid can also improve beer appreciation, although its individual effect remains to be tested. Interestingly, ethanol appears to be unnecessary to improve beer appreciation, both for blond beer and alcohol-free beer. Given the growing consumer interest in alcohol-free beer, with a predicted annual market growth of >7% 84 , it is relevant for brewers to know what compounds can further increase consumer appreciation of these beers. Hence, our model may readily provide avenues to further improve the flavor and consumer appreciation of both alcoholic and non-alcoholic beers, which is generally considered one of the key challenges for future beer production.
Whereas we see a direct implementation of our results for the development of superior alcohol-free beverages and other food products, our study can also serve as a stepping stone for the development of novel alcohol-containing beverages. We want to echo the growing body of scientific evidence for the negative effects of alcohol consumption, both on the individual level by the mutagenic, teratogenic and carcinogenic effects of ethanol 85 , 86 , as well as the burden on society caused by alcohol abuse and addiction. We encourage the use of our results for the production of healthier, tastier products, including novel and improved beverages with lower alcohol contents. Furthermore, we strongly discourage the use of these technologies to improve the appreciation or addictive properties of harmful substances.
The present work demonstrates that despite some important remaining hurdles, combining the latest developments in chemical analyses, sensory analysis and modern machine learning methods offers exciting avenues for food chemistry and engineering. Soon, these tools may provide solutions in quality control and recipe development, as well as new approaches to sensory science and flavor research.
Beer selection
250 commercial Belgian beers were selected to cover the broad diversity of beer styles and corresponding diversity in chemical composition and aroma. See Supplementary Fig. S1 .
Chemical dataset
Sample preparation.
Beers within their expiration date were purchased from commercial retailers. Samples were prepared in biological duplicates at room temperature, unless explicitly stated otherwise. Bottle pressure was measured with a manual pressure device (Steinfurth Mess-Systeme GmbH) and used to calculate CO 2 concentration. The beer was poured through two filter papers (Macherey-Nagel, 500713032 MN 713 ¼) to remove carbon dioxide and prevent spontaneous foaming. Samples were then prepared for measurements by targeted Headspace-Gas Chromatography-Flame Ionization Detector/Flame Photometric Detector (HS-GC-FID/FPD), Headspace-Solid Phase Microextraction-Gas Chromatography-Mass Spectrometry (HS-SPME-GC-MS), colorimetric analysis, enzymatic analysis, Near-Infrared (NIR) analysis, as described in the sections below. The mean values of biological duplicates are reported for each compound.
HS-GC-FID/FPD
HS-GC-FID/FPD (Shimadzu GC 2010 Plus) was used to measure higher alcohols, acetaldehyde, esters, 4-vinyl guaicol, and sulfur compounds. Each measurement comprised 5 ml of sample pipetted into a 20 ml glass vial containing 1.75 g NaCl (VWR, 27810.295). 100 µl of 2-heptanol (Sigma-Aldrich, H3003) (internal standard) solution in ethanol (Fisher Chemical, E/0650DF/C17) was added for a final concentration of 2.44 mg/L. Samples were flushed with nitrogen for 10 s, sealed with a silicone septum, stored at −80 °C and analyzed in batches of 20.
The GC was equipped with a DB-WAXetr column (length, 30 m; internal diameter, 0.32 mm; layer thickness, 0.50 µm; Agilent Technologies, Santa Clara, CA, USA) to the FID and an HP-5 column (length, 30 m; internal diameter, 0.25 mm; layer thickness, 0.25 µm; Agilent Technologies, Santa Clara, CA, USA) to the FPD. N 2 was used as the carrier gas. Samples were incubated for 20 min at 70 °C in the headspace autosampler (Flow rate, 35 cm/s; Injection volume, 1000 µL; Injection mode, split; Combi PAL autosampler, CTC analytics, Switzerland). The injector, FID and FPD temperatures were kept at 250 °C. The GC oven temperature was first held at 50 °C for 5 min and then allowed to rise to 80 °C at a rate of 5 °C/min, followed by a second ramp of 4 °C/min until 200 °C kept for 3 min and a final ramp of (4 °C/min) until 230 °C for 1 min. Results were analyzed with the GCSolution software version 2.4 (Shimadzu, Kyoto, Japan). The GC was calibrated with a 5% EtOH solution (VWR International) containing the volatiles under study (Supplementary Table S7 ).
HS-SPME-GC-MS
HS-SPME-GC-MS (Shimadzu GCMS-QP-2010 Ultra) was used to measure additional volatile compounds, mainly comprising terpenoids and esters. Samples were analyzed by HS-SPME using a triphase DVB/Carboxen/PDMS 50/30 μm SPME fiber (Supelco Co., Bellefonte, PA, USA) followed by gas chromatography (Thermo Fisher Scientific Trace 1300 series, USA) coupled to a mass spectrometer (Thermo Fisher Scientific ISQ series MS) equipped with a TriPlus RSH autosampler. 5 ml of degassed beer sample was placed in 20 ml vials containing 1.75 g NaCl (VWR, 27810.295). 5 µl internal standard mix was added, containing 2-heptanol (1 g/L) (Sigma-Aldrich, H3003), 4-fluorobenzaldehyde (1 g/L) (Sigma-Aldrich, 128376), 2,3-hexanedione (1 g/L) (Sigma-Aldrich, 144169) and guaiacol (1 g/L) (Sigma-Aldrich, W253200) in ethanol (Fisher Chemical, E/0650DF/C17). Each sample was incubated at 60 °C in the autosampler oven with constant agitation. After 5 min equilibration, the SPME fiber was exposed to the sample headspace for 30 min. The compounds trapped on the fiber were thermally desorbed in the injection port of the chromatograph by heating the fiber for 15 min at 270 °C.
The GC-MS was equipped with a low polarity RXi-5Sil MS column (length, 20 m; internal diameter, 0.18 mm; layer thickness, 0.18 µm; Restek, Bellefonte, PA, USA). Injection was performed in splitless mode at 320 °C, a split flow of 9 ml/min, a purge flow of 5 ml/min and an open valve time of 3 min. To obtain a pulsed injection, a programmed gas flow was used whereby the helium gas flow was set at 2.7 mL/min for 0.1 min, followed by a decrease in flow of 20 ml/min to the normal 0.9 mL/min. The temperature was first held at 30 °C for 3 min and then allowed to rise to 80 °C at a rate of 7 °C/min, followed by a second ramp of 2 °C/min till 125 °C and a final ramp of 8 °C/min with a final temperature of 270 °C.
Mass acquisition range was 33 to 550 amu at a scan rate of 5 scans/s. Electron impact ionization energy was 70 eV. The interface and ion source were kept at 275 °C and 250 °C, respectively. A mix of linear n-alkanes (from C7 to C40, Supelco Co.) was injected into the GC-MS under identical conditions to serve as external retention index markers. Identification and quantification of the compounds were performed using an in-house developed R script as described in Goelen et al. and Reher et al. 87 , 88 (for package information, see Supplementary Table S8 ). Briefly, chromatograms were analyzed using AMDIS (v2.71) 89 to separate overlapping peaks and obtain pure compound spectra. The NIST MS Search software (v2.0 g) in combination with the NIST2017, FFNSC3 and Adams4 libraries were used to manually identify the empirical spectra, taking into account the expected retention time. After background subtraction and correcting for retention time shifts between samples run on different days based on alkane ladders, compound elution profiles were extracted and integrated using a file with 284 target compounds of interest, which were either recovered in our identified AMDIS list of spectra or were known to occur in beer. Compound elution profiles were estimated for every peak in every chromatogram over a time-restricted window using weighted non-negative least square analysis after which peak areas were integrated 87 , 88 . Batch effect correction was performed by normalizing against the most stable internal standard compound, 4-fluorobenzaldehyde. Out of all 284 target compounds that were analyzed, 167 were visually judged to have reliable elution profiles and were used for final analysis.
Discrete photometric and enzymatic analysis
Discrete photometric and enzymatic analysis (Thermo Scientific TM Gallery TM Plus Beermaster Discrete Analyzer) was used to measure acetic acid, ammonia, beta-glucan, iso-alpha acids, color, sugars, glycerol, iron, pH, protein, and sulfite. 2 ml of sample volume was used for the analyses. Information regarding the reagents and standard solutions used for analyses and calibrations is included in Supplementary Table S7 and Supplementary Table S9 .
NIR analyses
NIR analysis (Anton Paar Alcolyzer Beer ME System) was used to measure ethanol. Measurements comprised 50 ml of sample, and a 10% EtOH solution was used for calibration.
Correlation calculations
Pairwise Spearman Rank correlations were calculated between all chemical properties.
Sensory dataset
Trained panel.
Our trained tasting panel consisted of volunteers who gave prior verbal informed consent. All compounds used for the validation experiment were of food-grade quality. The tasting sessions were approved by the Social and Societal Ethics Committee of the KU Leuven (G-2022-5677-R2(MAR)). All online reviewers agreed to the Terms and Conditions of the RateBeer website.
Sensory analysis was performed according to the American Society of Brewing Chemists (ASBC) Sensory Analysis Methods 90 . 30 volunteers were screened through a series of triangle tests. The sixteen most sensitive and consistent tasters were retained as taste panel members. The resulting panel was diverse in age [22–42, mean: 29], sex [56% male] and nationality [7 different countries]. The panel developed a consensus vocabulary to describe beer aroma, taste and mouthfeel. Panelists were trained to identify and score 50 different attributes, using a 7-point scale to rate attributes’ intensity. The scoring sheet is included as Supplementary Data 3 . Sensory assessments took place between 10–12 a.m. The beers were served in black-colored glasses. Per session, between 5 and 12 beers of the same style were tasted at 12 °C to 16 °C. Two reference beers were added to each set and indicated as ‘Reference 1 & 2’, allowing panel members to calibrate their ratings. Not all panelists were present at every tasting. Scores were scaled by standard deviation and mean-centered per taster. Values are represented as z-scores and clustered by Euclidean distance. Pairwise Spearman correlations were calculated between taste and aroma sensory attributes. Panel consistency was evaluated by repeating samples on different sessions and performing ANOVA to identify differences, using the ‘stats’ package (v4.2.2) in R (for package information, see Supplementary Table S8 ).
Online reviews from a public database
The ‘scrapy’ package in Python (v3.6) (for package information, see Supplementary Table S8 ). was used to collect 232,288 online reviews (mean=922, min=6, max=5343) from RateBeer, an online beer review database. Each review entry comprised 5 numerical scores (appearance, aroma, taste, palate and overall quality) and an optional review text. The total number of reviews per reviewer was collected separately. Numerical scores were scaled and centered per rater, and mean scores were calculated per beer.
For the review texts, the language was estimated using the packages ‘langdetect’ and ‘langid’ in Python. Reviews that were classified as English by both packages were kept. Reviewers with fewer than 100 entries overall were discarded. 181,025 reviews from >6000 reviewers from >40 countries remained. Text processing was done using the ‘nltk’ package in Python. Texts were corrected for slang and misspellings; proper nouns and rare words that are relevant to the beer context were specified and kept as-is (‘Chimay’,’Lambic’, etc.). A dictionary of semantically similar sensorial terms, for example ‘floral’ and ‘flower’, was created and collapsed together into one term. Words were stemmed and lemmatized to avoid identifying words such as ‘acid’ and ‘acidity’ as separate terms. Numbers and punctuation were removed.
Sentences from up to 50 randomly chosen reviews per beer were manually categorized according to the aspect of beer they describe (appearance, aroma, taste, palate, overall quality—not to be confused with the 5 numerical scores described above) or flagged as irrelevant if they contained no useful information. If a beer contained fewer than 50 reviews, all reviews were manually classified. This labeled data set was used to train a model that classified the rest of the sentences for all beers 91 . Sentences describing taste and aroma were extracted, and term frequency–inverse document frequency (TFIDF) was implemented to calculate enrichment scores for sensorial words per beer.
The sex of the tasting subject was not considered when building our sensory database. Instead, results from different panelists were averaged, both for our trained panel (56% male, 44% female) and the RateBeer reviews (70% male, 30% female for RateBeer as a whole).
Beer price collection and processing
Beer prices were collected from the following stores: Colruyt, Delhaize, Total Wine, BeerHawk, The Belgian Beer Shop, The Belgian Shop, and Beer of Belgium. Where applicable, prices were converted to Euros and normalized per liter. Spearman correlations were calculated between these prices and mean overall appreciation scores from RateBeer and the taste panel, respectively.
Pairwise Spearman Rank correlations were calculated between all sensory properties.
Machine learning models
Predictive modeling of sensory profiles from chemical data.
Regression models were constructed to predict (a) trained panel scores for beer flavors and quality from beer chemical profiles and (b) public reviews’ appreciation scores from beer chemical profiles. Z-scores were used to represent sensory attributes in both data sets. Chemical properties with log-normal distributions (Shapiro-Wilk test, p < 0.05 ) were log-transformed. Missing chemical measurements (0.1% of all data) were replaced with mean values per attribute. Observations from 250 beers were randomly separated into a training set (70%, 175 beers) and a test set (30%, 75 beers), stratified per beer style. Chemical measurements (p = 231) were normalized based on the training set average and standard deviation. In total, three linear regression-based models: linear regression with first-order interaction terms (LR), lasso regression with first-order interaction terms (Lasso) and partial least squares regression (PLSR); five decision tree models, Adaboost regressor (ABR), Extra Trees (ET), Gradient Boosting regressor (GBR), Random Forest (RF) and XGBoost regressor (XGBR); one support vector machine model (SVR) and one artificial neural network model (ANN) were trained. The models were implemented using the ‘scikit-learn’ package (v1.2.2) and ‘xgboost’ package (v1.7.3) in Python (v3.9.16). Models were trained, and hyperparameters optimized, using five-fold cross-validated grid search with the coefficient of determination (R 2 ) as the evaluation metric. The ANN (scikit-learn’s MLPRegressor) was optimized using Bayesian Tree-Structured Parzen Estimator optimization with the ‘Optuna’ Python package (v3.2.0). Individual models were trained per attribute, and a multi-output model was trained on all attributes simultaneously.
Model dissection
GBR was found to outperform other methods, resulting in models with the highest average R 2 values in both trained panel and public review data sets. Impurity-based rankings of the most important predictors for each predicted sensorial trait were obtained using the ‘scikit-learn’ package. To observe the relationships between these chemical properties and their predicted targets, partial dependence plots (PDP) were constructed for the six most important predictors of consumer appreciation 74 , 75 .
The ‘SHAP’ package in Python (v0.41.0) was implemented to provide an alternative ranking of predictor importance and to visualize the predictors’ effects as a function of their concentration 68 .
Validation of causal chemical properties
To validate the effects of the most important model features on predicted sensory attributes, beers were spiked with the chemical compounds identified by the models and descriptive sensory analyses were carried out according to the American Society of Brewing Chemists (ASBC) protocol 90 .
Compound spiking was done 30 min before tasting. Compounds were spiked into fresh beer bottles, that were immediately resealed and inverted three times. Fresh bottles of beer were opened for the same duration, resealed, and inverted thrice, to serve as controls. Pairs of spiked samples and controls were served simultaneously, chilled and in dark glasses as outlined in the Trained panel section above. Tasters were instructed to select the glass with the higher flavor intensity for each attribute (directional difference test 92 ) and to select the glass they prefer.
The final concentration after spiking was equal to the within-style average, after normalizing by ethanol concentration. This was done to ensure balanced flavor profiles in the final spiked beer. The same methods were applied to improve a non-alcoholic beer. Compounds were the following: ethyl acetate (Merck KGaA, W241415), ethyl hexanoate (Merck KGaA, W243906), isoamyl acetate (Merck KGaA, W205508), phenethyl acetate (Merck KGaA, W285706), ethanol (96%, Colruyt), glycerol (Merck KGaA, W252506), lactic acid (Merck KGaA, 261106).
Significant differences in preference or perceived intensity were determined by performing the two-sided binomial test on each attribute.
Reporting summary
Further information on research design is available in the Nature Portfolio Reporting Summary linked to this article.
Data availability
The data that support the findings of this work are available in the Supplementary Data files and have been deposited to Zenodo under accession code 10653704 93 . The RateBeer scores data are under restricted access, they are not publicly available as they are property of RateBeer (ZX Ventures, USA). Access can be obtained from the authors upon reasonable request and with permission of RateBeer (ZX Ventures, USA). Source data are provided with this paper.
Code availability
The code for training the machine learning models, analyzing the models, and generating the figures has been deposited to Zenodo under accession code 10653704 93 .
Tieman, D. et al. A chemical genetic roadmap to improved tomato flavor. Science 355 , 391–394 (2017).
Article ADS CAS PubMed Google Scholar
Plutowska, B. & Wardencki, W. Application of gas chromatography–olfactometry (GC–O) in analysis and quality assessment of alcoholic beverages – A review. Food Chem. 107 , 449–463 (2008).
Article CAS Google Scholar
Legin, A., Rudnitskaya, A., Seleznev, B. & Vlasov, Y. Electronic tongue for quality assessment of ethanol, vodka and eau-de-vie. Anal. Chim. Acta 534 , 129–135 (2005).
Loutfi, A., Coradeschi, S., Mani, G. K., Shankar, P. & Rayappan, J. B. B. Electronic noses for food quality: A review. J. Food Eng. 144 , 103–111 (2015).
Ahn, Y.-Y., Ahnert, S. E., Bagrow, J. P. & Barabási, A.-L. Flavor network and the principles of food pairing. Sci. Rep. 1 , 196 (2011).
Article CAS PubMed PubMed Central Google Scholar
Bartoshuk, L. M. & Klee, H. J. Better fruits and vegetables through sensory analysis. Curr. Biol. 23 , R374–R378 (2013).
Article CAS PubMed Google Scholar
Piggott, J. R. Design questions in sensory and consumer science. Food Qual. Prefer. 3293 , 217–220 (1995).
Article Google Scholar
Kermit, M. & Lengard, V. Assessing the performance of a sensory panel-panellist monitoring and tracking. J. Chemom. 19 , 154–161 (2005).
Cook, D. J., Hollowood, T. A., Linforth, R. S. T. & Taylor, A. J. Correlating instrumental measurements of texture and flavour release with human perception. Int. J. Food Sci. Technol. 40 , 631–641 (2005).
Chinchanachokchai, S., Thontirawong, P. & Chinchanachokchai, P. A tale of two recommender systems: The moderating role of consumer expertise on artificial intelligence based product recommendations. J. Retail. Consum. Serv. 61 , 1–12 (2021).
Ross, C. F. Sensory science at the human-machine interface. Trends Food Sci. Technol. 20 , 63–72 (2009).
Chambers, E. IV & Koppel, K. Associations of volatile compounds with sensory aroma and flavor: The complex nature of flavor. Molecules 18 , 4887–4905 (2013).
Pinu, F. R. Metabolomics—The new frontier in food safety and quality research. Food Res. Int. 72 , 80–81 (2015).
Danezis, G. P., Tsagkaris, A. S., Brusic, V. & Georgiou, C. A. Food authentication: state of the art and prospects. Curr. Opin. Food Sci. 10 , 22–31 (2016).
Shepherd, G. M. Smell images and the flavour system in the human brain. Nature 444 , 316–321 (2006).
Meilgaard, M. C. Prediction of flavor differences between beers from their chemical composition. J. Agric. Food Chem. 30 , 1009–1017 (1982).
Xu, L. et al. Widespread receptor-driven modulation in peripheral olfactory coding. Science 368 , eaaz5390 (2020).
Kupferschmidt, K. Following the flavor. Science 340 , 808–809 (2013).
Billesbølle, C. B. et al. Structural basis of odorant recognition by a human odorant receptor. Nature 615 , 742–749 (2023).
Article ADS PubMed PubMed Central Google Scholar
Smith, B. Perspective: Complexities of flavour. Nature 486 , S6–S6 (2012).
Pfister, P. et al. Odorant receptor inhibition is fundamental to odor encoding. Curr. Biol. 30 , 2574–2587 (2020).
Moskowitz, H. W., Kumaraiah, V., Sharma, K. N., Jacobs, H. L. & Sharma, S. D. Cross-cultural differences in simple taste preferences. Science 190 , 1217–1218 (1975).
Eriksson, N. et al. A genetic variant near olfactory receptor genes influences cilantro preference. Flavour 1 , 22 (2012).
Ferdenzi, C. et al. Variability of affective responses to odors: Culture, gender, and olfactory knowledge. Chem. Senses 38 , 175–186 (2013).
Article PubMed Google Scholar
Lawless, H. T. & Heymann, H. Sensory evaluation of food: Principles and practices. (Springer, New York, NY). https://doi.org/10.1007/978-1-4419-6488-5 (2010).
Colantonio, V. et al. Metabolomic selection for enhanced fruit flavor. Proc. Natl. Acad. Sci. 119 , e2115865119 (2022).
Fritz, F., Preissner, R. & Banerjee, P. VirtualTaste: a web server for the prediction of organoleptic properties of chemical compounds. Nucleic Acids Res 49 , W679–W684 (2021).
Tuwani, R., Wadhwa, S. & Bagler, G. BitterSweet: Building machine learning models for predicting the bitter and sweet taste of small molecules. Sci. Rep. 9 , 1–13 (2019).
Dagan-Wiener, A. et al. Bitter or not? BitterPredict, a tool for predicting taste from chemical structure. Sci. Rep. 7 , 1–13 (2017).
Pallante, L. et al. Toward a general and interpretable umami taste predictor using a multi-objective machine learning approach. Sci. Rep. 12 , 1–11 (2022).
Malavolta, M. et al. A survey on computational taste predictors. Eur. Food Res. Technol. 248 , 2215–2235 (2022).
Lee, B. K. et al. A principal odor map unifies diverse tasks in olfactory perception. Science 381 , 999–1006 (2023).
Mayhew, E. J. et al. Transport features predict if a molecule is odorous. Proc. Natl. Acad. Sci. 119 , e2116576119 (2022).
Niu, Y. et al. Sensory evaluation of the synergism among ester odorants in light aroma-type liquor by odor threshold, aroma intensity and flash GC electronic nose. Food Res. Int. 113 , 102–114 (2018).
Yu, P., Low, M. Y. & Zhou, W. Design of experiments and regression modelling in food flavour and sensory analysis: A review. Trends Food Sci. Technol. 71 , 202–215 (2018).
Oladokun, O. et al. The impact of hop bitter acid and polyphenol profiles on the perceived bitterness of beer. Food Chem. 205 , 212–220 (2016).
Linforth, R., Cabannes, M., Hewson, L., Yang, N. & Taylor, A. Effect of fat content on flavor delivery during consumption: An in vivo model. J. Agric. Food Chem. 58 , 6905–6911 (2010).
Guo, S., Na Jom, K. & Ge, Y. Influence of roasting condition on flavor profile of sunflower seeds: A flavoromics approach. Sci. Rep. 9 , 11295 (2019).
Ren, Q. et al. The changes of microbial community and flavor compound in the fermentation process of Chinese rice wine using Fagopyrum tataricum grain as feedstock. Sci. Rep. 9 , 3365 (2019).
Hastie, T., Friedman, J. & Tibshirani, R. The Elements of Statistical Learning. (Springer, New York, NY). https://doi.org/10.1007/978-0-387-21606-5 (2001).
Dietz, C., Cook, D., Huismann, M., Wilson, C. & Ford, R. The multisensory perception of hop essential oil: a review. J. Inst. Brew. 126 , 320–342 (2020).
CAS Google Scholar
Roncoroni, Miguel & Verstrepen, Kevin Joan. Belgian Beer: Tested and Tasted. (Lannoo, 2018).
Meilgaard, M. Flavor chemistry of beer: Part II: Flavor and threshold of 239 aroma volatiles. in (1975).
Bokulich, N. A. & Bamforth, C. W. The microbiology of malting and brewing. Microbiol. Mol. Biol. Rev. MMBR 77 , 157–172 (2013).
Dzialo, M. C., Park, R., Steensels, J., Lievens, B. & Verstrepen, K. J. Physiology, ecology and industrial applications of aroma formation in yeast. FEMS Microbiol. Rev. 41 , S95–S128 (2017).
Article PubMed PubMed Central Google Scholar
Datta, A. et al. Computer-aided food engineering. Nat. Food 3 , 894–904 (2022).
American Society of Brewing Chemists. Beer Methods. (American Society of Brewing Chemists, St. Paul, MN, U.S.A.).
Olaniran, A. O., Hiralal, L., Mokoena, M. P. & Pillay, B. Flavour-active volatile compounds in beer: production, regulation and control. J. Inst. Brew. 123 , 13–23 (2017).
Verstrepen, K. J. et al. Flavor-active esters: Adding fruitiness to beer. J. Biosci. Bioeng. 96 , 110–118 (2003).
Meilgaard, M. C. Flavour chemistry of beer. part I: flavour interaction between principal volatiles. Master Brew. Assoc. Am. Tech. Q 12 , 107–117 (1975).
Briggs, D. E., Boulton, C. A., Brookes, P. A. & Stevens, R. Brewing 227–254. (Woodhead Publishing). https://doi.org/10.1533/9781855739062.227 (2004).
Bossaert, S., Crauwels, S., De Rouck, G. & Lievens, B. The power of sour - A review: Old traditions, new opportunities. BrewingScience 72 , 78–88 (2019).
Google Scholar
Verstrepen, K. J. et al. Flavor active esters: Adding fruitiness to beer. J. Biosci. Bioeng. 96 , 110–118 (2003).
Snauwaert, I. et al. Microbial diversity and metabolite composition of Belgian red-brown acidic ales. Int. J. Food Microbiol. 221 , 1–11 (2016).
Spitaels, F. et al. The microbial diversity of traditional spontaneously fermented lambic beer. PLoS ONE 9 , e95384 (2014).
Blanco, C. A., Andrés-Iglesias, C. & Montero, O. Low-alcohol Beers: Flavor Compounds, Defects, and Improvement Strategies. Crit. Rev. Food Sci. Nutr. 56 , 1379–1388 (2016).
Jackowski, M. & Trusek, A. Non-Alcohol. beer Prod. – Overv. 20 , 32–38 (2018).
Takoi, K. et al. The contribution of geraniol metabolism to the citrus flavour of beer: Synergy of geraniol and β-citronellol under coexistence with excess linalool. J. Inst. Brew. 116 , 251–260 (2010).
Kroeze, J. H. & Bartoshuk, L. M. Bitterness suppression as revealed by split-tongue taste stimulation in humans. Physiol. Behav. 35 , 779–783 (1985).
Mennella, J. A. et al. A spoonful of sugar helps the medicine go down”: Bitter masking bysucrose among children and adults. Chem. Senses 40 , 17–25 (2015).
Wietstock, P., Kunz, T., Perreira, F. & Methner, F.-J. Metal chelation behavior of hop acids in buffered model systems. BrewingScience 69 , 56–63 (2016).
Sancho, D., Blanco, C. A., Caballero, I. & Pascual, A. Free iron in pale, dark and alcohol-free commercial lager beers. J. Sci. Food Agric. 91 , 1142–1147 (2011).
Rodrigues, H. & Parr, W. V. Contribution of cross-cultural studies to understanding wine appreciation: A review. Food Res. Int. 115 , 251–258 (2019).
Korneva, E. & Blockeel, H. Towards better evaluation of multi-target regression models. in ECML PKDD 2020 Workshops (eds. Koprinska, I. et al.) 353–362 (Springer International Publishing, Cham, 2020). https://doi.org/10.1007/978-3-030-65965-3_23 .
Gastón Ares. Mathematical and Statistical Methods in Food Science and Technology. (Wiley, 2013).
Grinsztajn, L., Oyallon, E. & Varoquaux, G. Why do tree-based models still outperform deep learning on tabular data? Preprint at http://arxiv.org/abs/2207.08815 (2022).
Gries, S. T. Statistics for Linguistics with R: A Practical Introduction. in Statistics for Linguistics with R (De Gruyter Mouton, 2021). https://doi.org/10.1515/9783110718256 .
Lundberg, S. M. et al. From local explanations to global understanding with explainable AI for trees. Nat. Mach. Intell. 2 , 56–67 (2020).
Ickes, C. M. & Cadwallader, K. R. Effects of ethanol on flavor perception in alcoholic beverages. Chemosens. Percept. 10 , 119–134 (2017).
Kato, M. et al. Influence of high molecular weight polypeptides on the mouthfeel of commercial beer. J. Inst. Brew. 127 , 27–40 (2021).
Wauters, R. et al. Novel Saccharomyces cerevisiae variants slow down the accumulation of staling aldehydes and improve beer shelf-life. Food Chem. 398 , 1–11 (2023).
Li, H., Jia, S. & Zhang, W. Rapid determination of low-level sulfur compounds in beer by headspace gas chromatography with a pulsed flame photometric detector. J. Am. Soc. Brew. Chem. 66 , 188–191 (2008).
Dercksen, A., Laurens, J., Torline, P., Axcell, B. C. & Rohwer, E. Quantitative analysis of volatile sulfur compounds in beer using a membrane extraction interface. J. Am. Soc. Brew. Chem. 54 , 228–233 (1996).
Molnar, C. Interpretable Machine Learning: A Guide for Making Black-Box Models Interpretable. (2020).
Zhao, Q. & Hastie, T. Causal interpretations of black-box models. J. Bus. Econ. Stat. Publ. Am. Stat. Assoc. 39 , 272–281 (2019).
Article MathSciNet Google Scholar
Hastie, T., Tibshirani, R. & Friedman, J. The Elements of Statistical Learning. (Springer, 2019).
Labrado, D. et al. Identification by NMR of key compounds present in beer distillates and residual phases after dealcoholization by vacuum distillation. J. Sci. Food Agric. 100 , 3971–3978 (2020).
Lusk, L. T., Kay, S. B., Porubcan, A. & Ryder, D. S. Key olfactory cues for beer oxidation. J. Am. Soc. Brew. Chem. 70 , 257–261 (2012).
Gonzalez Viejo, C., Torrico, D. D., Dunshea, F. R. & Fuentes, S. Development of artificial neural network models to assess beer acceptability based on sensory properties using a robotic pourer: A comparative model approach to achieve an artificial intelligence system. Beverages 5 , 33 (2019).
Gonzalez Viejo, C., Fuentes, S., Torrico, D. D., Godbole, A. & Dunshea, F. R. Chemical characterization of aromas in beer and their effect on consumers liking. Food Chem. 293 , 479–485 (2019).
Gilbert, J. L. et al. Identifying breeding priorities for blueberry flavor using biochemical, sensory, and genotype by environment analyses. PLOS ONE 10 , 1–21 (2015).
Goulet, C. et al. Role of an esterase in flavor volatile variation within the tomato clade. Proc. Natl. Acad. Sci. 109 , 19009–19014 (2012).
Article ADS CAS PubMed PubMed Central Google Scholar
Borisov, V. et al. Deep Neural Networks and Tabular Data: A Survey. IEEE Trans. Neural Netw. Learn. Syst. 1–21 https://doi.org/10.1109/TNNLS.2022.3229161 (2022).
Statista. Statista Consumer Market Outlook: Beer - Worldwide.
Seitz, H. K. & Stickel, F. Molecular mechanisms of alcoholmediated carcinogenesis. Nat. Rev. Cancer 7 , 599–612 (2007).
Voordeckers, K. et al. Ethanol exposure increases mutation rate through error-prone polymerases. Nat. Commun. 11 , 3664 (2020).
Goelen, T. et al. Bacterial phylogeny predicts volatile organic compound composition and olfactory response of an aphid parasitoid. Oikos 129 , 1415–1428 (2020).
Article ADS Google Scholar
Reher, T. et al. Evaluation of hop (Humulus lupulus) as a repellent for the management of Drosophila suzukii. Crop Prot. 124 , 104839 (2019).
Stein, S. E. An integrated method for spectrum extraction and compound identification from gas chromatography/mass spectrometry data. J. Am. Soc. Mass Spectrom. 10 , 770–781 (1999).
American Society of Brewing Chemists. Sensory Analysis Methods. (American Society of Brewing Chemists, St. Paul, MN, U.S.A., 1992).
McAuley, J., Leskovec, J. & Jurafsky, D. Learning Attitudes and Attributes from Multi-Aspect Reviews. Preprint at https://doi.org/10.48550/arXiv.1210.3926 (2012).
Meilgaard, M. C., Carr, B. T. & Carr, B. T. Sensory Evaluation Techniques. (CRC Press, Boca Raton). https://doi.org/10.1201/b16452 (2014).
Schreurs, M. et al. Data from: Predicting and improving complex beer flavor through machine learning. Zenodo https://doi.org/10.5281/zenodo.10653704 (2024).
Download references
Acknowledgements
We thank all lab members for their discussions and thank all tasting panel members for their contributions. Special thanks go out to Dr. Karin Voordeckers for her tremendous help in proofreading and improving the manuscript. M.S. was supported by a Baillet-Latour fellowship, L.C. acknowledges financial support from KU Leuven (C16/17/006), F.A.T. was supported by a PhD fellowship from FWO (1S08821N). Research in the lab of K.J.V. is supported by KU Leuven, FWO, VIB, VLAIO and the Brewing Science Serves Health Fund. Research in the lab of T.W. is supported by FWO (G.0A51.15) and KU Leuven (C16/17/006).
Author information
These authors contributed equally: Michiel Schreurs, Supinya Piampongsant, Miguel Roncoroni.
Authors and Affiliations
VIB—KU Leuven Center for Microbiology, Gaston Geenslaan 1, B-3001, Leuven, Belgium
Michiel Schreurs, Supinya Piampongsant, Miguel Roncoroni, Lloyd Cool, Beatriz Herrera-Malaver, Florian A. Theßeling & Kevin J. Verstrepen
CMPG Laboratory of Genetics and Genomics, KU Leuven, Gaston Geenslaan 1, B-3001, Leuven, Belgium
Leuven Institute for Beer Research (LIBR), Gaston Geenslaan 1, B-3001, Leuven, Belgium
Laboratory of Socioecology and Social Evolution, KU Leuven, Naamsestraat 59, B-3000, Leuven, Belgium
Lloyd Cool, Christophe Vanderaa & Tom Wenseleers
VIB Bioinformatics Core, VIB, Rijvisschestraat 120, B-9052, Ghent, Belgium
Łukasz Kreft & Alexander Botzki
AB InBev SA/NV, Brouwerijplein 1, B-3000, Leuven, Belgium
Philippe Malcorps & Luk Daenen
You can also search for this author in PubMed Google Scholar
Contributions
S.P., M.S. and K.J.V. conceived the experiments. S.P., M.S. and K.J.V. designed the experiments. S.P., M.S., M.R., B.H. and F.A.T. performed the experiments. S.P., M.S., L.C., C.V., L.K., A.B., P.M., L.D., T.W. and K.J.V. contributed analysis ideas. S.P., M.S., L.C., C.V., T.W. and K.J.V. analyzed the data. All authors contributed to writing the manuscript.
Corresponding author
Correspondence to Kevin J. Verstrepen .
Ethics declarations
Competing interests.
K.J.V. is affiliated with bar.on. The other authors declare no competing interests.
Peer review
Peer review information.
Nature Communications thanks Florian Bauer, Andrew John Macintosh and the other, anonymous, reviewer(s) for their contribution to the peer review of this work. A peer review file is available.
Additional information
Publisher’s note Springer Nature remains neutral with regard to jurisdictional claims in published maps and institutional affiliations.
Supplementary information
Supplementary information, peer review file, description of additional supplementary files, supplementary data 1, supplementary data 2, supplementary data 3, supplementary data 4, supplementary data 5, supplementary data 6, supplementary data 7, reporting summary, source data, source data, rights and permissions.
Open Access This article is licensed under a Creative Commons Attribution 4.0 International License, which permits use, sharing, adaptation, distribution and reproduction in any medium or format, as long as you give appropriate credit to the original author(s) and the source, provide a link to the Creative Commons licence, and indicate if changes were made. The images or other third party material in this article are included in the article’s Creative Commons licence, unless indicated otherwise in a credit line to the material. If material is not included in the article’s Creative Commons licence and your intended use is not permitted by statutory regulation or exceeds the permitted use, you will need to obtain permission directly from the copyright holder. To view a copy of this licence, visit http://creativecommons.org/licenses/by/4.0/ .
Reprints and permissions
About this article
Cite this article.
Schreurs, M., Piampongsant, S., Roncoroni, M. et al. Predicting and improving complex beer flavor through machine learning. Nat Commun 15 , 2368 (2024). https://doi.org/10.1038/s41467-024-46346-0
Download citation
Received : 30 October 2023
Accepted : 21 February 2024
Published : 26 March 2024
DOI : https://doi.org/10.1038/s41467-024-46346-0
Share this article
Anyone you share the following link with will be able to read this content:
Sorry, a shareable link is not currently available for this article.
Provided by the Springer Nature SharedIt content-sharing initiative
By submitting a comment you agree to abide by our Terms and Community Guidelines . If you find something abusive or that does not comply with our terms or guidelines please flag it as inappropriate.
Quick links
- Explore articles by subject
- Guide to authors
- Editorial policies
Sign up for the Nature Briefing: Translational Research newsletter — top stories in biotechnology, drug discovery and pharma.

- Original article
- Open access
- Published: 24 November 2021
Reducing maritime accidents in ships by tackling human error: a bibliometric review and research agenda
- Carine Dominguez-Péry ORCID: orcid.org/0000-0002-4288-6810 1 ,
- Lakshmi Narasimha Raju Vuddaraju 1 ,
- Isabelle Corbett-Etchevers 1 &
- Rana Tassabehji 2
Journal of Shipping and Trade volume 6 , Article number: 20 ( 2021 ) Cite this article
17k Accesses
15 Citations
1 Altmetric
Metrics details
Over the past decade the number of maritime transportation accidents has fallen. However, as shipping vessels continue to increase in size, one single incident, such as the oil spills from ‘super’ tankers, can have catastrophic and long-term consequences for marine ecosystems, the environment and local economies. Maritime transport accidents are complex and caused by a combination of events or processes that might ultimately result in the loss of human and marine life, and irreversible ecological, environmental and economic damage. Many studies point to direct or indirect human error as a major cause of maritime accidents, which raises many unanswered questions about the best way to prevent catastrophic human error in maritime contexts. This paper takes a first step towards addressing some of these questions by improving our understanding of upstream maritime accidents from an organisation science perspective—an area of research that is currently underdeveloped. This will provide new and relevant insights by both clarifying how ships can be described in terms of organisations and by considering them in a whole ecosystem and industry. A bibliometric review of extant literature of the causes of maritime accidents related to human error was conducted, and the findings revealed three main root causes of human and organisational error, namely, human resources and management, socio-technical Information Systems and Information Technologies, and individual/cognition-related errors. As a result of the bibliometric review, this paper identifies the gaps and limitations in the literature and proposes a research agenda to enhance our current understanding of the role of human error in maritime accidents. This research agenda proposes new organisational theory perspectives—including considering ships as organisations; types of organisations (highly reliable organisations or self-organised); complex systems and socio-technical systems theories for digitalised ships; the role of power; and developing dynamic safety capabilities for learning ships. By adopting different theoretical perspectives and adapting research methods from social and human sciences, scholars can advance human error in maritime transportation, which can ultimately contribute to addressing human errors and improving maritime transport safety for the wider benefit of the environment and societies ecologies and economies.
Introduction
The global shipping industry is responsible for transporting as much as 90% of world trade (SSR 2021 ). Over the past decade, improved ship design, technology, regulation and risk management systems have contributed to a 70% drop in reported shipping losses (SSR 2021 ). However, while the frequency of maritime accidents may be in decline, one single incident can have catastrophic and long-term consequences for marine ecosystems, the environment and local economies (Roberts et al. 2002 ). This is exacerbated further by the fact that maritime transportation vessels are increasing in size and the amounts of cargo on-board with them. For instance, in September 2019, Brazil’s north-eastern state of Bahia declared an emergency after an oil spill from the tanker Bouboulina contaminated kilometres of coastal beaches. In August 2020, Mauritius also declared a state of environmental emergency after the MV Wakashio ran aground at Pointe d’Esny, spilling oil into an area renowned as a sanctuary for rare wildlife. These types of accidents attract the attention of the media and heighten the concerns of people around the world, as images of the damage to marine wildlife and the environment are graphically visible.
Despite the ostensible fall in total reported losses, the number of accidents Footnote 1 , especially those related to passenger/car carrier vessels and ro-ros has increased, as has the number of reported casualties (SSR 2021 ). Therefore, this study's starting point was to understand further why maritime accidents with such wide-ranging consequences continue to occur.
Maritime transport accidents are complex (Guven-Koçak 2015 ) and caused by a combination of events or processes (Soares and Teixeira 2001 ) involving various actors that ultimately lead to disastrous consequences including loss of human and marine life and irreparable ecological, environmental and economic damage (Harrald et al. 1998 ). Apart from uncontrollable acts of God defined as ‘an extreme interruption with a natural cause (e.g. earthquake, storm, etc.)’ (Kristiansen 2005 :14), the literature consistently highlights human error (HE) as one of the main contributing factors in more than 85% of cases of maritime accidents (Acejo et al. 2018 ; Galieriková, 2019 ). Furthermore, experts estimate that 30–50% of oil spills are caused directly or indirectly by HE (Michel and Fingas 2016 ). Despite this, there is a surprising dearth of research in the management literature investigating HE in the maritime context (Berkowitz et al. 2019 ). This leads us to question the role of humans in the maritime transport ecosystem and ask: ‘ What is the current state-of-the-art research regarding human error as the main cause of maritime transportation accidents? How have researchers considered and framed human error? What research agenda is recommended to integrate the “human” further to avoid human error from an organisation science perspective, including team, organisational and collaborative networks/ecosystems?
This paper aims to address these questions by improving our understanding of maritime accidents and prevention from an organisational perspective, which is currently underdeveloped in organisation science. In order to achieve these objectives, a bibliometric review is conducted. The bibliometric review (BR) is a quantitative approach that uses co-citation analysis to visualise the literature in the field (van Oorschot et al. 2018 ). This reduces the reviewers’ subjectivity and bias and will generate a more systematic and encompassing picture of HE research in the field of maritime transportation.
The paper is organised as follows. The first part lays out the general context of the maritime transportation industry, the main causes of vessel accidents and the role of HE in maritime accidents. Then the five-step bibliometric review method adopted for this study is described. The findings are collated, analysed and discussed to provide a deeper understanding of what currently constitutes HE. Finally, a research agenda to investigate maritime accidents and HE from a socio-organisational perspective to prevent future accidents is proposed.
Accidents in maritime shipping
The maritime transportation industry’s distinct maritime culture is characterised by its global nature, working conditions, autonomy and complexity (Güven-Koçak 2015 ). The global nature of the shipping industry means worldwide competition is driving ship-owners to seek ever-increasing cost-efficiencies (Lützhöft et al. 2011 ). Maritime shipping is heavily influenced by the global economic, trade and environmental trends and were significantly impacted by the economic downturn in 2020 resulting from the COVID-19 pandemic. According to UNCTAD ( 2020 ), the total world fleet consists of 98,140 commercial ships over 100 gross tons (GT). Of these, the number of gas carriers, oil tankers, bulk carriers and container ships grew most rapidly over the year to 2020. Despite the advances in technology, processes, procedures, training and regulations, a total of 193 vessels exceeding 100 GT were lost over the 3 years from 2017, mainly through sinking (62%), grounding (15%), fire/explosion (10%), machinery damage/failure (6%) (SSR 2021 : 14). The type of cargo and size of vessel have a big impact on the extent and consequences of an accident at sea. Crude oil alone accounted for around 17–20% of total seaborne goods loaded between 2010 and 2019, and the amount of crude oil transported annually averages around 1,800 million metric tons (UNCTAD STAT 2019 ). In addition to the type of cargo, the increasing size of vessels can impact safety, effective fire prevention and salvage in the event of an accident (SSR 2021 ), highlighted so vividly by the recent case of the Ever Given ‘wedged’ in the Suez Canal (Guardian 2021 ).
Over the past 50 years, the size and capacity of vessels have increased by 1,500%, with the largest container ships now being as big as the largest oil tanker and bigger than the largest cruise ships (UNCTAD 2020 ). According to the ITOPF ( 2019 ), between 2010 and 2018, 91% of all oil spills resulted from 10 incidents, an increase from the previous decade where 75% of oil spills resulted from 10 incidents. Indeed, many studies identify collision/allision as a major cause of oil spill accidents in over half of the cases, most occurring while the vessels are underway or in open water (Eliopoulou and Papanikolaou 2007 ; Uğurlu et al. 2015). The catastrophic and often long-term human, economic and ecological consequences of accidents involving large vessels carrying increased volumes of highly toxic pollutants can be felt globally (ITOPF 2019 ; Chen et al. 2018 ). The focus of this study is to investigate human error (HE) in all types of maritime transportation, with a view to better understanding these errors in order to prevent future devastating accidents.
In addition to increasing the size of vessels, another very common way ship-owners reduce their fixed costs is by hiring multinational crews from developing countries or reducing the number of crew members on-board (Lützhöft et al. 2011 ). This often leads to de-prioritising employee training (Güven-Koçak 2015 ) and increased communication and comprehension problems between the multi-lingual and multi-cultural crew, who cannot effectively communicate with and understand one another. Crew members also inevitably transfer their cultural perspectives, stereotypes, and racial prejudices, leading to cultural tensions and strained relationships. These tensions are further exacerbated by long working hours, a noisy environment, a sense of isolation and loneliness, poor and often shared living conditions with little privacy, and the impossibility of getting away to enjoy free time alone (Güven-Koçak 2015 ). Living and working under such conditions for long periods can affect crew morale and raise stress levels, ultimately leading to fatigue, loss of concentration and focus, lower productivity (Alderton et al. 2004 ) and ultimately accidents.
Human error (HE) as the central cause of accidents
The complexity and lack of standardisation in maritime accident reporting often mean it is difficult and time-consuming to uncover detailed causal factors (Grech et al. 2002 ). Despite this, HE has been identified as one of the primary factors in over 75% of maritime accidents (Acejo et al. 2018 ; Celik and Cebi 2008 ). In an analysis of 177 maritime accident reports, Grech et al. ( 2002 ) found one aspect of HE – lack of situation awareness—to be a severe problem in the maritime domain. Specifically, ‘ shortcomings of the cognitive psychology paradigm of perception, cognition and projection of future events’ ( ibid. p.2), where HE resulted from a failure to anticipate future actions, a failure to correctly perceive information, a failure to correctly integrate or comprehend information and/or the system. In the context of advancing on-board digital systems, these human failings are particularly concerning as they suggest that as the crew become over-reliant on new technologies, the problems of situational awareness will grow considerably and have more of a negative impact on safety.
How have researchers considered and framed HE?
Having reviewed the literature, what is apparent is the different ways in which the concept of ‘human error’ is defined. ‘Human errors’ are the consequences focusing on individual actions leading to errors resulting from intentional actions (Reason 1990 ), a deviation from the performance of an action (Leveson 2011 ), a slip (Norman 1981 ) or a human disturbance that leads to an accident (Rasmussen 2000 ). For some, HE also includes organisational factors (Reason 1997 ; Dekker 2006 ). A selection of these definitions is summarised in Table 1 .
The definition of HE has evolved from being seen as a slip (Norman 1980 ) to a more complex interaction between people, tools and tasks in an organisational environment (Dekker 2002 ). How HE is defined is mainly dependent on the perspective of the discipline evaluating it. For instance, from the engineering discipline, HE is considered a set of causes that need to be tackled to avoid accidents. However, from the perspective of human factors and ergonomics (HFE), HE is more complex and includes aspects of organisational factors and has no systematic solutions to solve the causes. However, the terms are generally ill-defined with little distinction between them and are often used interchangeably.
In reviewing human factors that contribute to organisational accidents in shipping, Hetherington et al. ( 2006 ) developed a framework highlighting three areas common to accidents that can potentially improve shipping safety if moderated. These are (1) personnel issues (fatigue, stress, health, situation awareness, teamwork, decision-making, communication) which were immediate causes (2) organisational and management issues (safety culture) which were underlying causes, and (3) design issues (automation). As with all such studies, there are acknowledged limitations. In this case, there are only 20 studies, many of which lack measures of the impact of specific human behaviours on accidents. This, however, does not invalidate this study but rather highlights the need for more robust research in this complex area.
Researchers have used techniques such as Human Factor Analysis and Classification System (HFACS) and Fuzzy Analytical Hierarchy Process (FAHP) to further investigate causal links and weightings of HE in shipping accidents. For instance, operator failure due to lack of skills, misperception or error of judgement (Celik and Cebi 2008 ), fatigue and miscommunication more recently (Ung 2019 ; Yıldırım et al. 2017). These studies concluded that HE was one of the leading causes of shipping accidents. While these studies offer high level and general insights into the role of shipping, they do not sufficiently explain the role of HE in shipping accidents from an organisational or ecosystem perspective.
By applying a bibliometric review approach, this paper explores the literature in more depth to understand the themes related to the causes of maritime accidents and, more specifically, the aspects attributed to HE.
Methodology
A bibliometric review (BR) methodology was selected for this paper. It is a systematic approach consistent with the paper’s objective of presenting the state-of-the-art of published research on the causes of human error in maritime transportation accidents. Bibliometric reviews mobilise quantitative rather than qualitative techniques, reducing researcher subjectivity and bias, and are increasingly being used by scholars to map the development and structure of a scientific field (Zupic and Cater, 2015 ). They can combine co-citation analysis and bibliographic coupling to map the network of publications and arrive at distinct clusters of thematically related publications (van Oorschot et al. 2018 : 2). Bibliometric reviews also include other complementary analyses such as co-occurrence of keywords (where two or more keywords appear together in a document), co-word analysis (words that occur more frequently together with titles and abstracts) and co-citation of authors (Munim et al. 2020 ). The bibliometric review in this study followed the workflow process proposed by Zupic and Cater ( 2015 :5) summarised in Fig. 1 .
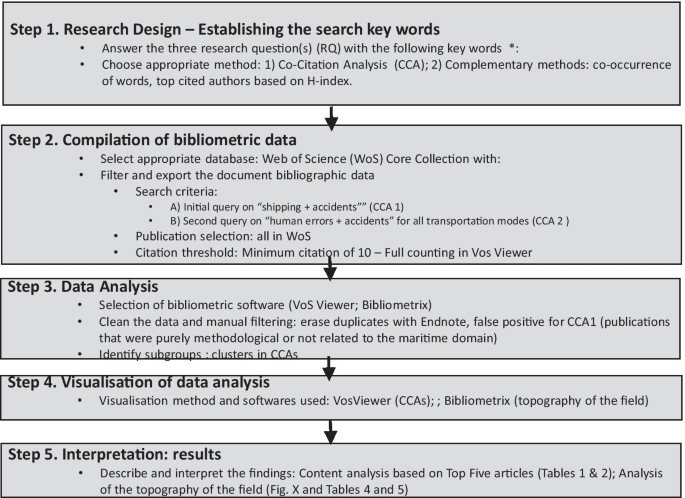
Bibliometric review workflow (adapted from Zupic and Cater ( 2015 :5))
Research design (Step 1) The initial broad review of the maritime transportation literature highlighted the important issues of ‘human error’ in maritime ship accidents. Therefore, the research question to direct this study, established at the outset of the paper, is, ‘ what is the current state-of-the-art of the research regarding HE as the main cause in maritime transportation accidents?’ In order to have a complete view of how this human dimension is handled in the field of maritime transportation, the appropriate methods selected were, (1) co-citation analysis to visualise the seminal publications related to these keywords (CCA); (2) the co-occurrence of words to complete the structuring of main topics to provide a topography of the field; and (3) top-cited authors based on the h-index analysis in order to further analyse the most recently developed topics and concepts.
Compilation of bibliometric data (Step 2) The Web of Science (WoS), which contains over 33,000 journals, including books, conference proceedings, data sets and patents dating back to 1900, was used as the core database for this bibliometric search. The WOS content is curated by experts and provides the data for Journal Impact Factor scores. The metadata and citation data are considered high quality and reliable (Haraldstad and Christophersen 2015 ) and, in line with other studies, is considered most appropriate for bibliometric reviews (Zupic and Cater 2015 ).
The initial search using the keywords “Shipping + Accidents” resulted in 1661 publications and was the basis for Stage 1 of the co-citation analysis. Several false positives were encountered. This is where ostensibly relevant articles that had keywords matching the search terms, on close reading, were found not to be related to the maritime domain and so were excluded. However, the seminal books that were most cited were included in the dataset. Articles that were purely about research methodologies with no relation to the maritime context were also excluded; for instance Yang et al. ( 2013 ) focuses only on fuzzy logic techniques and Saaty ( 1980 ) focuses only on the Analytic Hierarchy Process (AHP). This resulted in a total of 191 publications. The second search using the keywords “Accidents + Human Error” resulted in 2019 publications and was the basis for Stage 2 of the co-citation analysis (CCA). After filtering the data, this resulted in 225 articles.
For each search, a citation threshold was set at ten, which means that only documents that obtained at least ten local citations were included in the network. Furthermore, the entire counting method was used to select the articles for the CCAs. Any co-authored documents are counted and where ‘a link between [two authors] has a strength of 2 [this] indicates that both authors have co-authored two documents’ (Van Eck and Waltman 2013 , p.32).
Data analysis and visualisation (Step 3 and 4) To provide a complete bibliometric analysis, we used VosViewer software for the co-citation analysis (CCA) and Bibliometrix software for the bibliometric citation analysis (Munim et al. 2020 ) to identify the most influential articles, journals, authors and institutions. VosViewer software was used to generate a Co-Citation Analysis (CCA) of cited articles that were co-cited at least 10 times. Regarding CCAs, an overview of the major publications classified in clusters corresponding to seminal themes of interest-based on the dataset collated using the keywords “Shipping + Accidents” (CCA1) and “Accidents and Human Errors” (CCA2) was presented. These are further considered in the discussion section. Bibliometrix software provides a topography of the field with co-occurrence of keywords, a co-word analysis (Figs. 4 , 5 ). Finally, the top 20 authors resulting from the keywords are presented in Table 4 following Munim et al. ( 2020 ).
Interpretation (Step 5) At this stage, the researchers evaluated the top five papers of each cluster to interpret their content and were labelled according to the keywords (see Tables 2 , 3 ). The analysis of the CCA was supplemented with a topography of the field (analysis of top 20 authors for “Accidents + shipping” and “Accidents + Human error”) and discussed in the following section.
Discussion of findings
Co-citation analysis (cca 1): understanding shipping accidents.
The initial query using the keywords “Shipping + Accidents” was grouped into four clusters illustrated in Fig. 2 . Two clusters (A and C) focus on human error, whereas the other two (B and D) refer to engineering or other causes.
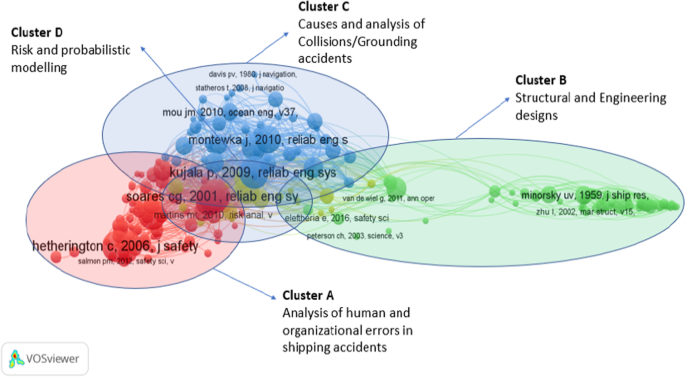
CCA clusters based on keywords “Shipping + Accidents”
Figure 2 presents the four main clusters identified in Stage 1 of the bibliometric review with CCA based on shipping accidents and illustrates the clusters with the most weight within the overall map based on the total articles per cluster and the average number of citations per article as summarised in Table 2 . Cluster A and C in Fig. 2 focus on finding and/or explaining the causes (with methods such as Root Cause Analysis) of HE. Cluster B deals with technical, engineering and other structural design issues, while Cluster D is related to risk and probabilistic modelling with mathematical models. As Clusters B and D were not related to HE, they are excluded from the analysis below.
Cluster A is labelled “ Analysis of Human and organisational errors in shipping accidents ”. It gathers 73 of the most cited co-cited references. Most research papers in this cluster describe and/or analyse the human error. In Table 6 (in the “ Appendix ”), the main themes were classified into three categories: Managerial and Human Resources, Socio-technical use and Individual and Cognitive approaches to explain, predict and/or prevent maritime shipping accidents. Cluster A contains the most significant proportion of references and overlaps extensively with Cluster C (Collision/Grounding accidents).
Cluster C is labelled “Collisions/Grounding accidents” . This cluster has 47 cited references and has extensive connections with Cluster A, which incorporates human and organisational errors as the leading causes of groundings and collisions. However, in Cluster D, HE is considered one of many other mathematical variables in risk models and algorithms; nevertheless, it overlooks the different dimensions that constitute HE (such as fatigue, organisation choices etc.).
This initial search confirms that HE is the central concern related to shipping accidents, highlighted in more than 63% of articles in Clusters A and C. To examine further the results of clusters A and C, all the articles were reviewed by the researchers concentrating on their titles and abstracts. These were classified into three main topics related to HE, namely (1) managerial and human resources, (2) socio-technical use, and (3) individual errors analysed with a cognitive approach. These categories are used to evaluate the literature identified in each of the respective clusters (A and C) and are summarised in Tables 6 and 7 in the “ Appendix ”.
Co-citation analysis (CCA2): understanding the role of “human error” in maritime accidents
In the second stage of the CCA process, another query using the keywords “Accidents + Human error” was conducted to refine our understanding of human error. A total of 225 articles resulted and were grouped into 5 clusters described in Table 3 and illustrated in Fig. 3 .
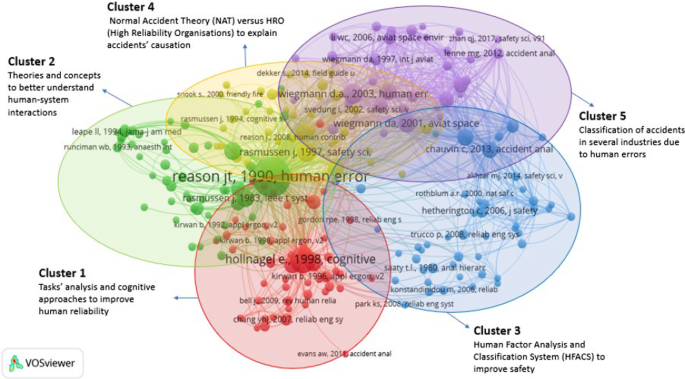
CCA clusters based on keywords “Accidents + Human error”
Cluster 1 focuses on an individual unit of analysis looking into tasks and cognitive reactions. Cluster 2 proposes the main theories around man–machine interactions (particularly Information Technologies and Systems) with the work of Reason ( 1990 ) linking all the other clusters. Cluster 4 adopts a more structural unit of analysis based on ship structures and illustrates the theoretical debate between Normal Accident Theory (NAT) and High-Reliability Organisations (HRO). Cluster 3 is centred in the Human Factor Analysis and Classification System (HFACS) related to safety, and Cluster 5 centres on identifying contributing factors and classifying accidents in several industries.
Cluster 1 was labelled Task analysis and cognitive approaches to improving human reliability . It gathers 51 co-cited references. Most papers propose methods or models to assess the risk of accidents to better predict them (Hollnagel 1998 ; Swain and Guttmann 1983 ; Shorrock and Kirwan 2002 ). Most research approaches adopt a cognitive understanding of HE (Hollnagel 1998 ; Shorrock and Kirwan 2002 ; Chang and Mosleh 2007 ). Kirwan ( 1994 ) focuses on tasks performed by humans as they interact with systems or technologies and the related risks. The top ten articles of this cluster are oriented toward improving human reliability.
Cluster 2 was labelled Theories and concepts to better understand human-system interactions . This has 51 co-cited references that are primarily dominated by the work of Reason, who proposed the theoretical integration of several previously independent literatures (Reason 1990 ). He further proposed two ways of modelling HE: using a person or a systems approach (Reason 2000 ). Other articles focus on socio-technical use, such as Rasmussen ( 1983 ), who develops theoretical backgrounds related to introducing information technology, digital computers and knowledge. Endsley ( 1995 ) discusses several methods to measure situation awareness, and Norman ( 1981 ) suggests a theory of action to avoid action slips.
Cluster 3 was labelled Human Factor Analysis and Classification System (HFACS) to improve safety. Cluster 3 gathers 48 co-cited references. Most papers investigate human error using the HFACS method to analyse multiple accidents (Celik and Cebi 2008 ; Chauvin et al. 2013 ; Chen et al. 2013 ). Chen et al. ( 2013 ) develop an HFACS dedicated to Maritime Accidents. Hetherington et al. ( 2006 ) raise the issue of aggregating the causal factors of HE within the maritime context, while Trucco et al. ( 2008 ) propose an innovative approach to integrate the human and organisational factors into risk analysis.
Cluster 4 was labelled Explaining accident causes using two theoretical approaches . Cluster 4 gathers 41 co-cited references. This cluster illustrates the theoretical debate between Normal Accident Theory (NAT) and High-Reliability Organisations (HRO) to explain the causes of accidents.
Cluster 5 was labelled Classification of accidents in several industries due to human error . Cluster 5 gathers 34 co-cited references with several classifications of accidents due to HE. Shappell and Wiegmann ( 1997 ) propose a taxonomy of unsafe operations. Reinach and Viale ( 2006 ) investigate six accidents, highlighting 36 probable contributing factors. Based on an analysis of 508 mining accidents, Patterson and Shappell ( 2010 ) classify main causations between operator error and system deficiencies
Overall, Fig. 3 identifies relevant literature tackling managerial and human resources issues. Clusters 3 and 5 adopt quantitative methods and provide statistics and factor weightings to describe the cause of accidents. Cluster 1 represents individual and cognitive issues with HFACS as the main method. Socio-technical issues are addressed in Clusters 2 and 4 but mainly with theoretical approaches coming from psychology, cognitive sciences and ergonomics.
Both CCA1 and CCA2 are complementary. Figure 2 of CCA1 (‘Shipping + Accidents’) provides the whole landscape of seminal publications (including papers and books) related to accidents in maritime transportation. Two clusters of CCA2 are more related to understanding human error in accidents (A and C). To go further, Fig. 3 with CCA2 ‘Accidents + Human error’ provides the seminal books and papers related to the analysis of what human error is, their causes and recommendations to cope with them, whatever the types of transportation. CCA1 is focused on maritime transportation, whereas CCA2 includes all types of transportation modes that tackle the HE question.
There is minimal overlap between CCA1 and CCA2. There are only two authors that belong both to CCA1 and CCA2. One is Reason for his seminal book on HE that is both co-cited in maritime and other transportation fields to study accidents. The other is Hetherington et al. ( 2006 ), who is one of the main cited papers to study HE in maritime.
This review highlights the limited understanding of HE and the lack of depth that would fully explain HE and on-board group behaviours, both from human resources and socio-technical perspectives.
Topography of the research field
To further develop the bibliometric review and compliment the co-citation analysis, the following section presents a topography of the field, following Munim et al.’s ( 2020 ) approach, which further maps the structure of the research themes related to the research keywords.
Topography of ‘shipping and accidents’ research
There has been a growing trend in the number of articles’ citations related to ‘Shipping and Accidents’, particularly over the last decade, illustrated in Fig. 4 . This suggests the growing interest and importance of this topic.
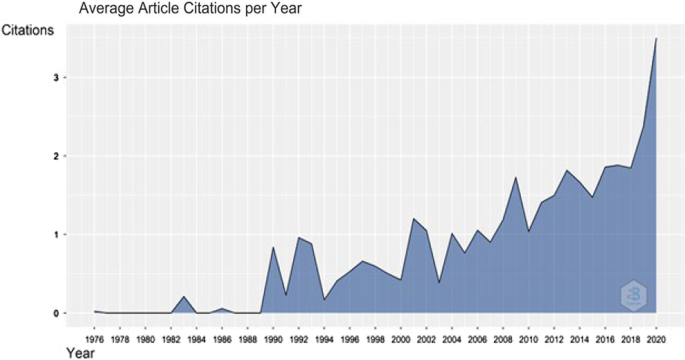
Average citations per year for the keywords “Shipping + Accidents”
To understand this trend in more depth, centrality and density measures of the main topics are calculated and presented visually in Fig. 5 . Centrality (Callon centrality) measures the strength of association between the keywords in one cluster with another cluster. Density (Callon density) measures the aggregate strength of the relationships between the keywords in the same cluster (Cobo et al., 2011 ).
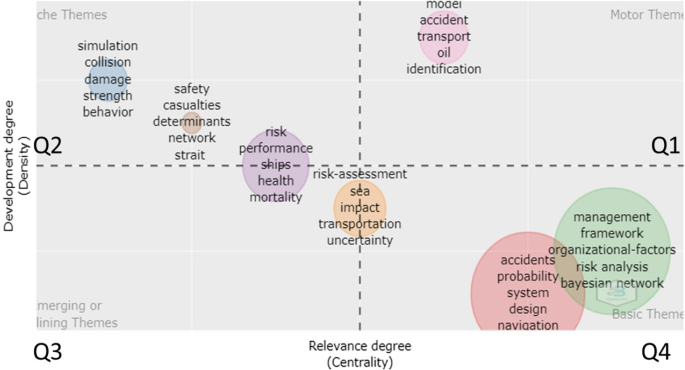
Thematic map with co-occurrence of keywords for ‘Shipping + Accidents’
Based on keyword co-occurrence centrality, the themes in quadrant Q1 (top right) called motor themes are topics that act as a bridge between other topics. The keywords in quadrant Q2 (top left) indicate highly developed or niche themes. The keywords in quadrant Q3 (bottom left) display emerging topics in a particular field. Finally, the keywords in quadrant Q4 (bottom right) indicate basic and transversal themes currently under development.
This thematic map shows that the most well developed and highly researched themes are related to models of accidents related to transportation, specifically in the context of oil spills and using identification systems such as AIS. In addition, the basic topics that are underdeveloped are related to frameworks, organisational factors, risk analysis and Bayesian networks, followed by probability of accidents related to design engineering.
The themes in the top right Q1 are fundamental to structure the research field. The keywords in the theme (model, accident, transport, oil, identification) are related to accident modelling, oil transportation and identification of risks. Q1 has strong connections with the keywords (sea, impact, transportation, uncertainty) (between Q2 and Q3). The cluster in Q1 is also connected with the themes of Q3 and Q4 regarding quantitative analysis of accidents and behavioural factors. The keywords in Q2 (simulation, damage, collision, strength) are research fields related to collision simulations and strength behaviour simulations of maritime structures. The other cluster (safety, casualties, determinants, network) has specialised themes; it is pretty isolated with strong internal ties but weak relations with other themes. The themes in Q4 (accident, probability, system, design, navigation) are related to a quantitative analysis of accidents, human error quantification and decision making (consistent with clusters B and D of CCA1). The other themes in Q4 (management, framework, organizational factors, risk analysis, Bayesian networks) are related to human and organizational factors related to the maritime industry and risk analysis using Bayesian networks (consistent with cluster C of CCA1). These themes have strong external ties with all other clusters (Fig. 6 ).
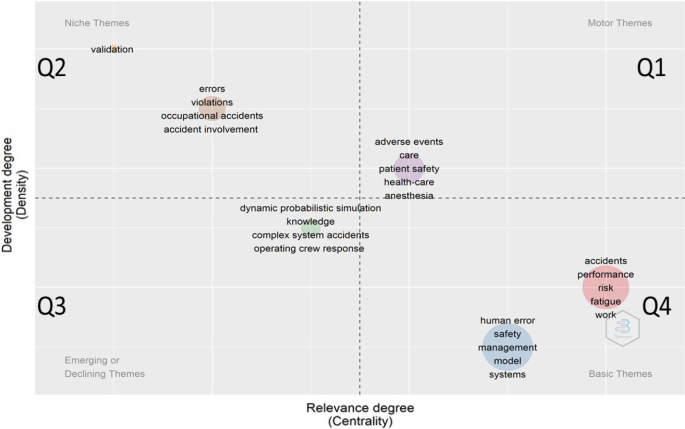
Thematic map with co-occurrence of keywords for “Human error + Accidents”
Topography of ‘human error + accidents’ research
In order to understand shipping accidents in more depth, the topography of the research related to the keywords ‘human error + accidents’ was also developed. While there were studies related to human error in other fields (such as medicine), only those related to the maritime sector are commented upon in this section.
The keywords in the Q4 are basic themes still in development with many external links but not necessarily strong with all the other clusters of Fig. 6 . On one side, the cluster related to (Accidents, performance, risk, fatigue, work) corresponds to the themes developed by the top 20 authors in marine technology and reliability engineering (See Table 4 ). On the other hand, the other cluster with keywords (human error, safety, management, models) is related to the themes developed by the top 20 authors in human factors and ergonomics (See Table 5 ). The clusters on the Q2 (errors, violations, occupational accidents, accidental involvement) are specialised and isolated themes.
As a conclusion, we can see that the keywords related to the understanding of human error with organisational insights (human error, safety, management systems, organisational factors, accidents, Bayesian network, performance, risks, fatigue and work) are promising fields of research as shown in Figs. 5 and 6 . Having highlighted the most interesting keywords and their co-occurrences, we further develop the literature by looking at the top-cited papers of the top 20 authors.
Focused literature review: shipping + accidents
To review the literature for both keywords “Shipping + Accidents” and then “Human error + Accidents”, the most cited papers of the top 20 authors highlighted by the Bibliometrix software (Table 4 ) were selected and analysed. Firstly, papers published before 2015 that were cited at least 40 times were selected; second, from 2015 to date (2021), the papers cited 15 or more times were included as they would highlight important and emerging topics. For the keywords “Shipping + Accidents”, this led to a comprehensive database of 222 articles. Table 4 below shows the top 20 authors according to their h-index provided by Bibliometrix.
All papers most cited and more recently published enter into one of the cluster labels of CCA1. Below we only focus on papers related to clusters A and C as they are related to HE in shipping accidents. Papers related to Cluster A split into two categories: first, some papers focus on the scope of HE from an individual cognitive approach with several methods: second, other papers adopt a monograph or historical approach to highlight human factors.
Firstly, Celik and Cebi ( 2008 ) develop a Human Factor Analysis and Classification System (HFACS) for HE in shipping accidents to improve group decision-making. In this model, the organisational influences are described as “big categories” (resource management, organisational climate and organisational processes). Supervision causes are described as inadequate or inappropriate. Finally, “communication, coordination and planning factors” are categorised as “personnel factors” and considered group-related activities. These models provide useful categories but do not fully describe how organisations act in a dynamic context.
Secondly, Graziano et al. ( 2016 ) propose a classification of HE taxonomy based on collision and grounding reports with four main categories: task errors, cognitive domain, technical equipment and performance. Interestingly, internal and external communication errors are highlighted as one key task; external communication includes communication between pilots, other vessels, tugs, VTS and on-shore. The main novelty of this paper is the description of the leading technical equipment which mediates HE, the most frequent being radars, followed by VHF and paper charts. All in all, we can infer from these categories of errors that they occur in situations where internal teams and/or external groups and stakeholders are involved.
Thirdly, Wu et al. ( 2017 ) propose a cognitive reliability and error analysis with evidential-based reasoning with original variables such as linguistic issues and incomplete information on-board. Akyuz and Celik ( 2014 ) similarly provide an HFCAS model combined with cognitive maps and highlight, in all categories of the model, the lack of knowledge or training as the major causes of accidents. They recommend studying ships in team contexts (including better diversity management on-board) and training them to adapt according to unexpected circumstances. In this paper, the recommendations are drawn on the necessity to adopt continuous learning, whatever the categories of HE.
Regarding monograph or historical approaches, Islam et al. ( 2017 ) develop a monograph for HE in operations maintenance useful for chief engineers and captains. Interestingly, the major causes of accidents come from deficiencies in knowledge (lack of experience) or insufficient training followed by seafarers' fatigue. Hansen developed several historical analyses of death on-board that enlarged and refined the human factors currently considered in studies. For instance, in their analysis, which covers the period between 1986 and 1993, Hansen and Pedersen ( 1996 ) concluded that the maritime workplace is a high risk where half of the deaths are due to the workplace and the lifestyle of seafarers. Hansen and Jensen ( 1998 ) undertook a unique study on the risks related to female seafarers and showed that major risks are due to their lifestyle (notably the consumption of alcohol and tobacco) and the fact that they “adopt the traditional male jobs at sea”. Roberts and Hansen ( 2002 ) highlighted several factors that concern both individuals (notably the age of the vessel as being one of the most important ones), several factors related to the working conditions (such as change of ship due to lost employment, daily routine duties, lifestyle) and the use of space on board (walking from one place to another, falling in docks when hazardous access and working practices are adopted). In a nutshell, most results of this cluster are oriented toward results aiming at facilitating decision-making but mostly at the level of individuals.
Complementary papers related to Cluster C are characterised by a diversity of research methods such as Bayesian networks (Hänninen and Kujala 2012 ), identification of events and processes of risks (Montewka et al. 2014b ), what-if analysis, association rules (Weng and Li 2019 ), scenario-event tree (Chai et al. 2017 ), binary logistic regressions (Weng and Yang 2015 ) and accident reports (Wróbel et al. 2017 ). They also sometimes develop research on specific ships such as ROPAX, cruise ships or tankers. Finally, they also propose tools or methods that improve safety: for instance, a ship collision alert system (Goerlandt et al. 2015 ) or a method for detecting possible near-miss ship collisions (Zhang et al. 2016 ).
This cluster provides interesting categorisations of human and organisational factors but always in “big categories” regarding the organisation of ships that remain static (except for Aps et al. 2015 ) and still mainly focused on “individuals” as units of analysis and not groups or networks. For instance, Hänninen and Kujala ( 2012 ) highlight the changing course in an encounter situation, the officer of the watch, the situation assessment, danger detection, personal conditions and other distractions (maintenance routines, fatigue, bridge view) as the main causes of accidents. Hänninen and Kujala ( 2014 ) integrate a new and interesting variable—the role of port state control in accidents—broadening the scope of study from the ship to her wider network. Regarding the automation and digitalisation of ships, Wróbel et al. ( 2017 ) provide one of the few analyses of the evolution of accidents with unmanned ships, arguing that if the number of navigational accidents falls, other types of accidents, such as fire on board, will increase with potentially worse consequences.
All in all, these pieces of research provide an interesting categorisation of the causes of HE. However, they all remain static pictures without providing a dynamic analysis, which would be a good basis for adaptive decision-making in specific contexts and building learning recommendations. Most studies still focus on individuals as units of analysis; few consider groups, and even fewer include the whole network of the ship. Research that includes “organisational factors” does not describe their workplaces nor the working conditions and routines on-board. Few studies recommend the necessity of a dynamic learning culture on-board offering ships the possibility to continuously adapt to the unexpected. This paper contends that these approaches will provide an in-depth understanding of the causes of accidents on ships, moving from a “technical structure” described through static categories to a real organisation with human beings on-board, able to adapt accordingly to their specific contexts. Finally, even though the digitalisation of ships is a reality, very few studies consider the use of technical tools as a cause of potential accidents.
Focused literature review: “human error + accidents”
The search keywords used led to papers related predominantly to transportation modes in aviation or rail. However, all papers related to maritime transportation that were cited 10 or more times were all included (see Table 5 ). The papers were reviewed to ensure a complete understanding of the content and themes within them. This led to a complete database of 241 articles.
The close analysis of the top 20 authors revealed three main academic disciplines that are currently structuring the field grouped as follows: (1) Human Factors and Ergonomics (HFE) on one side and (2) Marine Technology and Transportation Engineering (MTTE) and Reliability Engineering (RE) on the other side. HFE constitutes 11 top-cited authors Footnote 2 and publishes topics inspired from clusters 1, 2, 3 and 4 in CCA 1; (3) MTTE and RE consist of six authors Footnote 3 who publish on topics related to those in Clusters 3 and 5. The research of these authors is in the context of different modes of transportation (including maritime, rail, road, aviation) or other industries (health, mining, nuclear). Some authors are specialised in specific transportation modes—for instance, Shappell and Wiegmann in aviation, Mosleh in nuclear and Akyuz and Celik in maritime.
Our analysis highlights two main contributions of HFE:
Frameworks or models based on complex systems and sociotechnical systems theories (such as ACCIMAP, Human Factor Analysis and Classification System (HFACS), Systems Theoretic Accident Model and Processes (STAMP), Causal Analysis based on STAMP (CAST), Critical Path Analysis EAST, Functional Resonance Analysis Method (FRAM) to better assess risks based on taxonomies of human errors. Jenkins et al. ( 2017 ) and Hulme et al. ( 2019 ) propose a good synthesis and comparisons of them.
A diversity of industries and transportation modes can benefit or complement others (Banks et al. 2019 ; Grant et al. 2018 ; Hulme et al. 2019 ). There is, for instance, a historic move in the literature from research in the aviation industry that started to study the concept of situation awareness that is then applied to the maritime context. Indeed, Grant et al. ( 2018 ) recently proposed a generic accident causation model that could fit several industries using ‘systems thinking’.
There remain gaps and limitations in the HFE literature. For instance, the term HE does not sit easily with sociotechnical systems theories and concepts on which all these frameworks and models are based (Stanton et al. 2016 ), and specific phenomena such as the effects of communication and compounded information on performance are still under researched. Another limitation is the difficulty to model the different flows of information between separate teams (Jenkins et al. 2010 ). Furthermore, except for Harvey and Stanton ( 2014 ), there is still very little research focusing on the cognition of systems and large and distributed networks as units of analysis. An exception is Salmon et al. ( 2015 ) who study situation awareness at the level of systems. They present ten challenges for improving the understanding of interactions between social, technical and organisations, integrating the openness in systems, developing an understanding of what happens across boundaries (notably communication and coordination), culture, responsibility (with external pressure) and finally emerging behaviours (being more adaptive) and the ability to cope with changes. All these are still relevant and remain potentially fruitful areas for future research.
In the area of MTTE and RE overall, researchers tend to quantify HE in order to avoid researcher subjectivity using a range of methods such as fuzzy process on HFCAS (Celik and Cebi 2008 ), methods to set up the probabilities of human errors with the Error Producing Conditions (EPC) (Akyuz and Celik 2016 ) or weights related to causes (Akyuz et al. 2017 ) or the development of human error indexes (Khan et al. 2006 ). These methods are sometimes complemented by qualitative approaches such as the Why-because graphs of Chen et al. ( 2013 ). Furthermore, research in this field examines accidents in fine-grain looking at the specificities of different types of accidents, such as grounding (Akyuz and Celik 2015 ), fire (Akyuz et al., 2018 ), explosions (Baalisampang et al., 2018 ), offshore (Khan et al. 2016; Ren et al. 2008; Islam et al. 2017 ), and also different types of ships (Akyuz et al., 2017 ). To a lesser extent, there is also some research into the interactions between human and information systems (Mokhtari 2007 ).
However, similar to HFE, there are also gaps and limitations in the MTTE and RE literature that can provide an opportunity for future research. For example, much of the literature in this field, that highlighted that most current causes of HE relate to collective actions, is based on the modelling and analysis of cognitive and individual units of analysis (for instance, Akyuz and Celik 2014 ), which are mostly related to stress, fatigue, health except for Fan et al. ( 2018 ); Fan et al. ( 2018 ) mention the emotions of seafarers. Moreover, while Baalisampang et al. ( 2018 ) extended these individual factors to include elements such as knowledge, competencies, expectations, goals and attention, combined with workplaces factors (site and equipment design, work environment) and managerial factors (organisation of work, job design and information transfer), these are still not fully developed. Furthermore, when reviewing accident reports (for instance, Baalisampang et al. 2018 ), researchers do not address the lack of standardisation of these reports (Celik and Cebi 2008 ), which is a considerable limitation and an area for future work. Finally, as ships are becoming increasingly more automated, there are still very few studies investigating the on-board use of information systems and technologies and their interactions with the shore to improve communication and coordination.
All in all, this previous work has built a solid foundation for analysing HE to better prevent accidents. In the research agenda below, we propose how organisation and management sciences can bring new insights to advance human error research in maritime transportation.
Research agenda: propositions for studying human error in maritime accidents
Having evaluated the findings from the bibliometric review, it was clear that accidents are mainly explained from an engineering perspective. Human errors remain under-explored from organisational and network perspectives. In this section, five propositions for theoretically framing future research approaches are presented. Each of these theoretical management approaches can help improve our understanding of HE in the context of maritime accidents.
Ships as organisations: a novel perspective
The findings from this study revealed that the literature on maritime accidents has not fully conceptualised ships as organisations. Neither has it considered how these organisations behave according to the different temporalities in navigation. So, apart from individual and cognitive-based approaches, how can ships be conceptualised as organisations? Here, the conceptualisation of ships as temporary organisations generally follows navigational routines but, in cases of imminent accidents, develop crisis navigation routines.
From this perspective, merchant ships can be considered as organisations that go from point A to point B in order to deliver products. They are characterised by an organised (collective) course of action ‘aimed at evoking a non-routine process and/or completing a non-routine product’ (Packendorff 1995 ). Routines are defined as “repetitive, recognizable patterns of interdependent actions, carried out by multiple actors” (Feldman and Pentland 2003 ). The temporary time frame of the navigating crew is particularly relevant when considering safety management on-board. This is similar to project-based organisations characterised by a once-in-a-lifetime task with a predetermined delivery date, subject to performance goals and consisting of several complex and/or interdependent activities (Packendorff 1995 ).
Indeed, the analogy of merchant ships and temporary organisations is helpful to distinguish two types of temporalities: regular navigation and the period before an accident. When there are no accidents, the ship’s organisation and the environment are stable most of the time. The objectives of the ship are clear (to go from point A to point B), and actors behave according to a highly centralised and rational organisation that follows relatively standardised and shared routines (Degani and Wiener 1993 ), which we call ‘regular routine navigation’. This is empirically similar to formal quality management systems. However, during the period just before the accident (which can be short depending on the context), the crew and its network (notably for remote-controlled ships) try to make sense of the situation and adapt to it. Adopting a routine lens to study how routines cease or are transformed during an accident could be an interesting perspective yet not explored.
The transition between ‘regular routine navigation’ and ‘crisis routine navigation’ depends on the type of accident and can range from a few minutes to hours or days. During this transition time, which we term ‘crisis routine navigation’, actors on-board are aware of the imminence of the accident; behaviours on-board change due to uncertainty. As a result, there is an increase in stress (Sheridan 2008 ) that may lead to phenomena such as “out-of-the-loop” performance. This is characterised by actors’ failure to observe parameter changes and intervene when necessary, an over-reliance and absolute trust in information technology artefacts, a loss of situation awareness and finally, deterioration of an actor’s manual skills (Kaber and Endsley 1997 ). In such circumstances, both social cooperation modes and decision-making are affected. In the case of disaster management, resilience is critical. This is the system’s ability to anticipate and respond to anomalous circumstances to maintain safe functioning and recover and return to a stable equilibrium (Sheridan 2008 ; Normandin and Therrien 2016 ). Further research is needed to study ships as organisations that also include the specificities of their culture.
In the literature, as highlighted in Fig. 3 , the leading theory related to ships seen as organisations is the debate between High Reliable Organisations (HRO) and Normal Accident Theory (NAT). This controversy questions two domains, which raises new research questions: firstly, are there alternative theoretical models that can describe ships in practice? Second, with all the technologies and potential resources available today to secure ships, is it still relevant to consider the assumptions of NAT as reliable?
Ships: High Reliable Organisations (HRO) or self-organisations embedded in ecosystems?
Arguably, ships can be characterised as HRO and are perceived as one of the most highly centralised and rational types of transportation modes. Like the airline industry, maritime navigation has adopted standardised routines such as Cockpit Resource Management (CRM) implemented to provide checklist procedures that need to be accomplished by coordinated actions and communications between the captain and the other pilot(s) in a flight (Degani and Wiener 1993 ). According to the ‘high-reliability theory’, extremely safe operations are possible, even with extremely hazardous technologies, if appropriate organisational design and management techniques are followed (Sagan 1993 ).
However, accidents still do happen in HRO. Normal accident theory (NAT) presents a much more pessimistic prediction – specifically that ‘serious accidents with complex high technology systems are inevitable’ (Sagan 1993 :13). This empirical observation presents new research questions, such as, is the NAT still relevant today? Should we extend HRO theory to propose new concepts that would better describe ships as they function in real conditions? Could another way to manage resources and trade-off decisions concerning investments on ships avoid accidents? Has the maritime industry learnt from the aviation industry (International Air Transport Association congress of 1975) that it is machines that have to be adapted to human-beings and not the reverse (Clostermann 2017 :20)?
By applying Normal Accident Theory, ships can be considered to be an assemblage of components that are self-organised. From this perspective, we propose that ethnographic studies can better describe and shed light on working conditions on ships in real-life settings. From a theoretical perspective, we suggest exploring new concepts to study ships, notably in the case of imminent accidents. For instance, applying the concept of self-organisation of different maritime agents/stakeholders coordinating ports, ships and operations (Caschili and Medda 2012 ; Watson et al. 2021 ). More broadly, as ships are being increasingly managed remotely, this implies that their whole ecosystem and interactions with other stakeholders need to be considered in any future research. This includes the near network of shipping (incorporating the ship owner, insurances, port state control, VTS) and in a larger ecosystem representing the choices of the whole industry (flag ship, meta-organisations, countries that develop their marine policy).
Even though ships can be characterised as HRO, the proposition here is that their real organisational mode may be closer to self-organisation depending on the temporality of the accident. This is in direct opposition to the HRO view. The response to any accident is organisationally hierarchical and procedures officially documented according to quality management linked to the International Maritime Organisation (IMO) (Ismael 2011 ).
Digitalisation of ships and management of information systems
Many maritime vessels already use a range of information technologies (IT) and information systems (IS) with a host of different navigational equipment and sensors to assist them to navigate safely and efficiently, including Electronic Chart Display Information System (ECDIS) as a modern replacement for paper-based navigational charts, the Automatic Identification System (AIS) and radar (Radio Detection and Ranging) help improve situational awareness of other vessels and obstacles (Harti-Mokhtari et al., 2007). Furthermore, as Artificial Intelligence (AI) and machine learning develop at a pace, more vessels are using autonomous and semi-autonomous technologies that are monitored remotely from shore-based facilities requiring highly reliable and efficient communication channels (Hogg and Ghosh 2016 ).
These new technologies and other integrated bridge equipment mean that crew on-board ships increasingly rely on them. “Unlike in static situations where human–machine systems have complete control, in dynamic situations like navigation, changes occur rapidly giving only partial control to the operator” (Hoc 2000 : 835). This creates socio-technical systems that incorporate complex interactions between humans, machines and other environmental aspects (Baxter and Sommerville 2011 ). In this context, three main settings are particularly impacted by the socio-technical use of IT/IS, where human error can occur. Namely, IT/IS implementation, IT/IS use in navigation practice and IT/IS-based decision-making. For instance, the improper consideration of human–computer-interaction in the design of the technologies, the often ad-hoc way in which new and emerging technologies are implemented, and inadequate user training can all lead to inevitable human error (Lützhöft et al. 2011 ).
Similarly, the objectives of improving navigation safety are inextricably linked to a set of daily decisions taken by several interdependent actors on-board. This process is increasingly dependent on the diffusion and integration of data, information and knowledge between humans and technological devices in order to make decisions and take appropriate actions. Poor systems interfaces and improper allocation of functions to human and computer controllers can result in misinterpretation and misunderstanding of data and information being displayed, which leads to poor decision-making, degraded performance and ultimately accidents (Kaber and Endsley 1997 ).
Although ship systems are becoming increasingly well-equipped, technologically advanced and more reliable (Rothblum 2002 ), maritime accidents still happen. No technology is used in isolation, but rather the maritime system incorporates people, the environment (socio-technical and natural), and the organisation. In order to better understand the complexities, issues and problems, and how to avoid the repetition of accidents, all the different IT/IS technologies on-board a vessel must be considered holistically as part of the complex maritime ecosystem (Güven-Koçak, 2015 ; Watson et al. 2021 ). This digital transformation in the industry driven by new technologies such as AI and big data generates new operational challenges and risks such as cyber-attacks for the maritime sector that need further investigation (Munim et al. 2020 ).
One theoretical lens suggested continuing to develop complex systems and sociotechnical systems theories. Ships can be considered complex systems through this theoretical lens, both internally as an organisation and concerning their environment (Sovacool, 2008 ). These are large, tightly coupled systems (Perrow 1984 ) where socio-technical interdependencies (Thompson 1967 ) are high due to their complexity. Internally, a ship is a complex system involving a collection of crew members and the range of instruments and computer networks that support them. None of the crew possesses the complete plan or vision to navigate the ship. However, collectively they use information from the crew in conjunction with instrument observations and procedures to keep the vessel on the course (Ismael 2011 ). The more complicated the interdependence of systems and subsystems, the higher they become prone to failure due to their complexity, speed of interaction, tight coupling and limitations of their human operators and their designers (Sovacool 2008 ; Lützhöft et al. 2011 : 285). Consequently, from this perspective, ship-related maritime accidents can be characterised by a high level of complexity due to the interrelations of multiple and combined causes and the variability of contexts.
Orlikowski’s ( 1992 ) structuration theory, where technology is embedded with structure, can also offer insights into how human agents carry out their routines and the intervention that changes the relationship between human agents and organisational structure (Barley 1986 ) in the maritime context. Since technology is not always used by knowledgeable agents, this theoretical lens can explain how agents use these new technologies in their daily routines, and how they enact new structures or “technology-in-practice” (Orlikowski, 2000 ) to better understand human error.
De Vries ( 2017 ) is one of the few researchers in the maritime domain that showed how navigation safety of seagoing vessels can be improved through the socio-technical interaction of humans, technology, organisations and the environment drawing on Hollnagel et al.’s ( 2014 ) Functional Resonance Analysis Method (FRAM). Building on this work, De Vries and Bligård ( 2019 ) further demonstrated the benefits of applying a socio-technical systems perspective to influence navigation assistance assessment and design. Furthermore, they showed how discussions with stakeholders such as users, designers, managers, and regulators contributed to safe operations in the maritime context. However, these studies are few, and by applying a socio-technical perspective to the design of on-board systems to ensure they are compatible with and adapted to the human operator to improve performance (Brett et al. 2011 ) is a fruitful area of research for understanding and ultimately reducing human error in maritime transportation accidents.
As a consequence of these fast-paced technological developments, further research is needed on the interaction of ships within their broad and complex maritime ecosystems. These include but are not limited to the maritime environment, navigation and technologies, and the international organisations that frame, govern and regulate today’s shipping industry. This idea of improvement relies on developing standards in an industry that is more and more digitalised and interconnected (Watson et al. 2021 ). By improving our understanding of the maritime industry's emerging needs, which is partly considered self-organisations within an ecosystem, and partly tightly coupled with other systems, future accidents can be reduced.
Power Lens: a missing link
Organisations of all types, including ships and their ecosystems, are fundamentally underpinned by power relationships and issues. However, there is limited literature on this topic in the maritime context. At the level of the ship, a unique aspect of maritime culture is absolute autonomy and a strong power culture where the captain, known as “master under God”, is in full charge. While at sea, the captain has full authority over the ship, her occupants, and operations and is responsible for all safety issues (Güven-Koçak 2015 ), including final decisions and the responsibility related to accidents such as grounding. The captain and officers can exercise their judgement to make necessary decisions, such as changing routes, arrival ports or schedules.
With increasing links between the sea and the shore, communications between the ship-owner, who manages the ships from the shore, and the captain who stays on-board, may sometimes not be very effective. For example, in the Torrey Canyon oil tanker wrecked off the coast of Cornwall, this was initially attributed to several human errors. However, a more detailed examination identified management decisions ‘that put pressure on the captain’ and ‘equipment design issues’ related to activation of the autopilot mode (Harvey et al. 2013 ) as contributing factors to the disaster. Despite this, the literature hardly mentions in any depth communications issues between the vessels at sea and the shore and the pressure from the shore, in some cases due to trade-offs between security and profit that the captain and its crew experience. The few papers that deal with this issue mention “external pressure” as a factor without providing any details.
At the level of the ecosystem of ships, having multiple actors in this domain makes it difficult to legally assign responsibilities in the case of an accident. Empirical data suggests that diverging political interests stall proper investigation and prevention of similar accidents. Thus, the appearance of a mysterious oil spill on the north-east coast of Brazil in September 2019 is most probably linked to crude oil from Venezuela that was carried by the Greek-flagged ship Bouboulina (BBC 2019 ). There is strong evidence that the company, the captain and the vessel’s crew failed to communicate to authorities about the oil spill/release of the crude oil in the Atlantic Ocean.
The broad literature on power is diverse and complex, and its ramifications for the study of organisations have remained largely unexplored (Haugaard and Clegg 2012 ), especially in the maritime transportation sector. Indeed, power concerns the ways that social relationships shape capabilities, decisions and changes within organisations. Organisational power is bounded by the capacity of the decision-makers to gather and analyse complex data, which are often multi-dimensional and constrained by prior experiences, learning and knowledge (Haugaard and Clegg 2012 ). As such, the sources of power—reward, sanction, expertise, reference value and legitimacy—can also trigger conflict, especially when there is a divergence of objectives and strategies for achieving those objectives (Fulconis and Lissillour, 2021 ).
Of the few studies that examine power, Lissillour and Bonet Fernandez ( 2020 ) adopt a Bourdieusian perspective to understand the balance of power in the governance of the global maritime chain. They highlight the conflict of interest between the different global maritime stakeholders. In the context of human error and accidents, the maritime transportation stakeholders – which includes vessel owners, ship captains, classification authorities, insurers, customers and many others – often have differing and competing priorities between safety and economic interests. Often their strategies for managing these most effectively also diverge, leading to tensions and conflicts and ultimately trickle down to operational and human errors resulting in catastrophic accidents. Research should be developed to further understand the interactions among all the stakeholders at the level of the network of actors cooperating in the case of accidents, including the meta-organisations in this wider network (Berkowitz and Dumez 2016 ) acting to regulate the industry and sustain the oceans.
In the context of maritime transportation, there are several meta-organisations (Berkowitz and Dumez 2016 ) operating to regulate the industry with significant consequences on the collective actions of ships in their daily activities. More research is needed to build on Harvey et al. ( 2013 )'s work to further develop and mobilise the concept of meta-organisations. Other theoretical backgrounds, such as neo-institutionalism (DiMaggio and Powell, 1983 ), can shed light on potential isomorphism behaviours at the industry level. This can then be applied to the maritime context to explore how to better cope with accidents, reduce their often catastrophic consequences, and ultimately reduce them.
Since organisations are neither rational nor natural, the theories of power can translate practice to theory and highlight the phenomena of changing organisational practises (Haugaard and Clegg 2012 ). Thus, future studies could use the lens of power theories with human error in the maritime accident context at the centre of the analysis to better understand communication and coordination issues and the stakes and conflicts of interest of the power relationships between the different actors.
Developing dynamic safety capabilities for learning ships
In addition to more collaborative relationships, each ship and related stakeholders should develop their capacities to learn from the past to reduce future accidents. In this area, we propose to develop the concept of dynamic safety capability within the literature on learning organisations. Several streams of research have explored how organisations can learn from rare events such as crises or accidents. Developing alertness to weak cues in the environment is the first step for developing intelligence. Attentional triangulation (Rerup, 2009 ) combines three forms of attention – stability, coherence and vividness- for anticipating and preventing unexpected events. Previous studies have tended to base their analysis on the concept of situation awareness, mainly focusing on individuals (Hetherington et al. 2006 ). Very few studies have mobilised situation awareness through teams and systems (Stanton et al. 2015 ). Thus, dynamic capabilities can provide an interesting perspective for encompassing the previous concepts concerned with issues of adaptation and growth.
Different kinds of dynamic capabilities have already been identified in the literature. A dynamic safety capability is an organisation’s capacity to “generate, reconfigure, and adapt organisational routines to sustain high levels of safety performance in organisations characterised by change and uncertainty” (Griffin et al. 2016 : 249). Dynamic safety capability relies on three processes of organisational learning. Experience is first accumulated through tacit learning from ongoing action and events. Then the tacit learning is articulated and shared through collective discussions and processes of sense-making. Finally, knowledge is formalised into regulatory procedures (Griffin et al. 2016 ). Since crises remain rare events, the authors suggest using the simulation of high-risk environments and their potential consequences to allowing participants to engage in sense-making and focus on team communication and coordination processes. This literature provides rich insights into the importance of developing the ability to share knowledge and learn. However, most of the disaster cases investigated by Griffin et al. ( 2016 ) dealt with stable organisations.
Further research could focus on the mechanisms, processes and related skills for developing a safety capability aboard extreme cases such as tankers. In such temporary organisations, a salient issue is the ability to share knowledge among highly dispersed teams in terms of role tasks. In addition, these teams that frequently change, have to manage the continuity of routines through periods of transitions. These organisations, partly similar to SMEs, have to develop a certain level of absorptive capacity (Benhayoun et al. 2020 ) to identify and capture the external information that comes from the ecosystem to support on-board decision-making. The temporality of ships, which partly prevents routines for learning from rare events, questions how they can become learning organisations. This raises new research questions such as: How could we reconcile ships being both temporary and learning organisations? What is the subculture that would allow ships to move from a culture of adjustment (Baumler et al. 2020 ) to become learning organisations?
Under the umbrella term of “human error”, the literature presents many different explanations for accidents, including flaws in structural and engineering designs, cognitive limits and organisational choices. Can all these causes be considered to be “human” errors? In principle, at some point, the causes of all accidents can be related to the “human”, but in providing such a vague catch-all term, the real issues fail to be identified and addressed. This paper suggests that research from the disciplines of human and social sciences, particularly organisation studies, can provide new and relevant insights by clarifying how ships can be described in terms of organisations and by considering them in a whole ecosystem and industry.
The main contributions of this paper are twofold. First, four thematic clusters were identified through a bibliometric review of the causes of maritime accidents related to human error. Among them, the analysis of human and organisational errors showed that the three main causes are related to human resources and management, socio-technical IT/IS, and individual and cognitive errors. A second search on “human error” highlighted five clusters that confirm these three main root causes and provide several references for each of them. Second, the paper provides a critical analysis of the papers published by the top 20 authors cited both for shipping accidents and human error. Finally, several theoretical concepts and propositions for future researchers and practitioners to help tackle the causes of human error in the context of maritime accidents were suggested.
The implications of this study are several. First, the proposed agenda for future researchers can advance the field of human error in the maritime transport context by providing different theoretical perspectives and adapting research methods from social and human sciences. Second, this study highlights the gap in our current understanding of the role of human error in maritime accidents, which can feed into curricula for the education and training of maritime cadets, seafarers and other personnel. Finally, by understanding these gaps, maritime organisations and stakeholders can implement policies that will embed human factors more specifically with the ultimate objective of improving safety in maritime transportation.
Availability of data and materials
All data generated and analysed during this study are available through Web of Science and are included in this published article (please see references).
In line with academic literature (Rothblum 2002 :1) this paper refers to accidents and distinguishes between accidents and incidents depending on the severity of damage. Insurance company reports (SSR 2021 ) refer to any damage no matter the severity (including sinking of a vessel) as an “incident”.
(N.A. Stanton; G.H. Walker; P.H. Seong; S.A. Shappell; D.A. Wiegmann; S.W.A. Dekker; W. Jung; M.G. Lenne; S. Nazir, P. Waterson and J. Kim).
(E. Akyuz; J. Wang; M. Celik; F. Khan; Mosleh; R. Abassi).
Acejo I, Sampson H, Turgo N, Ellis N, Tang L (2018) The causes of maritime accidents in the period 2002–2016, Seafarers International Research Centre (SIRC), Cardiff University, United Kingdom. Availalbe from http://orca.cf.ac.uk/117481/1/Sampson_The%20causes%20of%20maritime%20accidents%20in%20the%20period%202002-2016.pdf
Akyuz E, Celik M (2014) Utilisation of cognitive map in modelling human error in marine accident analysis and prevention. Saf Sci 70:19–28
Article Google Scholar
Akyuz E, Celik M (2015) Application of CREAM human reliability model to cargo loading process of LPG tankers. J Loss Prev Process Ind 34:39–48. https://doi.org/10.1016/j.jlp.2015.01.019
Akyuz E, Celik E (2016) A modified human reliability analysis for cargo operation in single point mooring (SPM) off-shore units. Appl Ocean Res 58:11–20. https://doi.org/10.1016/j.apor.2016.03.012
Akyuz E, Celik E, Celik M (2017) A practical application of human reliability assessment for operating procedures of the emergency fire pump at ship. Ships Offshore Struct 13(2):208–216. https://doi.org/10.1080/17445302.2017.1354658
Akyuz E, Celik M, Akgun I, Cicek K (2018) Prediction of human error probabilities in a critical marine engineering operation on-board chemical tanker ship: the case of ship bunkering. Saf Sci 110:102–109. https://doi.org/10.1016/j.ssci.2018.08.002
Alderton T, Bloor M, Kahveci E, Lane T, Sampson H, Zhao M, Wu B (2004) The global seafarer: living and working conditions in a globalized industry. International Labour Organization, Geneva
Google Scholar
Aps R, Fetissov M, Goerlandt F, Helferich J, Kopti M, Kujala P (2015) Towards STAMP based dynamic safety management of eco-socio-technical maritime transport system. Procedia Eng 128:64–73
Baalisampang T, Abbassi R, Garaniya V, Khan F, Dadashzadeh M (2018) Review and analysis of fire and explosion accidents in maritime transportation. Ocean Eng 158:350–366. https://doi.org/10.1016/j.oceaneng.2018.04.022
Banda OA, Goerlandt F, Montewka J, Kujala P (2015) A risk analysis of winter navigation in Finnish sea areas. Accid Anal Prev 79:100–116. https://doi.org/10.1016/j.aap.2015.03.024
Banks VA, Stanton NA, Plant KL (2019) Who is responsible for automated driving? A macro-level insight into automated driving in the United Kingdom using the Risk Management Framework and Social Network Analysis. Appl Ergonom 81:102904
Barley SR (1986) Technology as an occasion for structuring: evidence from observations of CT scanners and the social order of radiology departments. Adm Sci Q 31:78–108
Baumler R, De Klerk Y, Manuel ME, Carballo L (2020) A culture of adjustment – evaluating the implementation of the current maritime regulatory framework on rest and work hours. World Maritime University, Malmo
Book Google Scholar
Baxter G, Sommerville I (2011) Socio-technical systems: from design methods to systems engineering. Interact Comput 23(1):4–17
BBC (2019) ‘Brazil oil spill: where has it come from?’ (BBC News Online 1st November, 2019). https://www.bbc.com/news/world-latin-america-50223106
Benhayoun L, Le Dain MA, Dominguez-Péry C, Lyons AC (2020) SMEs embedded in collaborative innovation networks: how to measure their absorptive capacity? Technol Forecast Soc Change 159:120–196
Berkowitz H, Dumez H (2016) The concept of meta-organization: issues for management studies. Eur Manag Rev 13(2):149–156
Berkowitz H, Prideaux M, Lelong S, Frey F (2019) The urgency of sustainable ocean studies in management. M@n@gement 22(2):297–315
Brett BE, Rothblum AM, Lyle WA, Durgavich J, Sargent MG, Downer KF (2011) Predicting total system performance: the benefit of integrating human performance models. Proc Hum Fact Ergon Soc Annu Meet 55(1):2020–2024. https://doi.org/10.1177/1071181311551421
Caschili S, Medda FR (2012) A review of the maritime container shipping industry as a complex adaptive system. Interdiscip Descr Complex Syst INDECS 10(1):1–15
Celik M, Cebi S (2008) Analytical HFACS for investigating human errors in shipping accidents. Accid Anal Prev 41(1):66–75
Chai T, Weng J, De-qi X (2017) Development of a quantitative risk assessment model for ship collisions in fairways. Saf Sci 91:71–83
Chang YHJ, Mosleh A (2007) Cognitive modeling and dynamic probabilistic simulation of operating crew response to complex system accidents: part 1: overview of the IDAC model. Reliab Eng Syst Saf 92(8):997–1013. https://doi.org/10.1016/j.ress.2006.05.014
Chauvin C, Lardjane S, Morel G, Clostermann J-P, Langard B (2013) Human and organisational factors in maritime accidents: analysis of collisions at sea using the HFACS. Accid Anal Prev 59:26–37. https://doi.org/10.1016/j.aap.2013.05.006
Chen S-T, Wall A, Davies P, Yang Z, Wang J, Chou Y-H (2013) A Human and Organisational Factors (HOFs) analysis method for marine casualties using HFACS-Maritime Accidents (HFACS-MA). Saf Sci 60:105–114. https://doi.org/10.1016/j.ssci.2013.06.009
Chen J, Zhang W, Li S, Zhang F, Zhu Y, Huang X (2018) Identifying critical factors of oil spill in the tanker shipping industry worldwide. J Clean Prod 180:1–10. https://doi.org/10.1016/j.jclepro.2017.12.238
Clostermann J-P (2017) La conduite du navire marchand. Facteurs humains dans une activité à risques. InfoMer, Marines éditions. 3ème edition.
Cobo MJ, López-Herrera AG, Herrera-Viedma E, Herrera F (2011) Science mapping software tools: review, analysis, and cooperative study among tools. J Am Soc Inf Sci Technol 62(7):1382–1402
de Vries L (2017) Work as done? Understanding the practice of socio-technical work in the maritime domain. J Cogn Eng Decis Mak 11(3):270–295
de Vries L, Bligård LO (2019) Visualising safety: the potential for using socio-technical systems models in prospective safety assessment and design. Saf Sci 111:80–93
Degani A, Wiener EL (1993) Cockpit checklists: concepts, design, and use. Hum Factors 35(2):345–359
Dekker SW (2002) Reconstructing human contributions to accidents: the new view on error and performance. J Saf Res 33(3):371–385
Dekker S (2006) The field guide to understanding human error. Ashgate Publishing, Ltd., Farnham
DiMaggio PJ, Powell WW (1983) The iron cage revisited: institutional isomorphism and collective rationality in organizational fields. Am Sociol Rev 48:147–160
ElBardissi AW, Wiegmann DA, Dearani JA, Daly RC, Sundt TM (2007) Application of the human factors analysis and classification system methodology to the cardiovascular surgery operating room. Ann Thorac Surg 83(4):1412–1419. https://doi.org/10.1016/j.athoracsur.2006.11.002
Eliopoulou E, Papanikolaou A (2007) Casualty analysis of large tankers. J Mar Sci Technol 12(4):240–250. https://doi.org/10.1007/s00773-007-0255-8
Endsley MR (1995) Measurement of situation awareness in dynamic systems. Hum Factors J Hum Factors Ergon Soc 37(1):65–84. https://doi.org/10.1518/001872095779049499
Fan S, Zhang J, Blanco-Davis E, Yang Z, Wang J, Yan X (2018) Effects of seafarers’ emotion on human performance using bridge simulation. Ocean Eng 170:111–119
Feldman MS, Pentland BT (2003) Reconceptualizing organizational routines as a source of flexibility and change. Adm Sci Q 48(1):94–118
Fowler TG, Sørgård E (2000) Modeling ship transportation risk. Risk Anal 20(2):225–244. https://doi.org/10.1111/0272-4332.202022
Fulconis F, Lissillour R (2021) Toward a behavioral approach of international shipping: a study of the inter-organisational dynamics of maritime safety. J Shipping Trade 6(1):1–23
Galieriková A (2019) The human factor and maritime safety. Transp Res Procedia 40:1319–1326
Goerlandt F, Kujala P (2011) Traffic simulation based ship collision probability modeling. Reliab Eng Syst Saf 96(1):91–107
Goerlandt F, Montewka J (2015a) Maritime transportation risk analysis: review and analysis in light of some foundational issues. Reliab Eng Syst Saf 138:115–134. https://doi.org/10.1016/j.ress.2015.01.025
Goerlandt F, Montewka J (2015b) A framework for risk analysis of maritime transportation systems: a case study for oil spill from tankers in a ship–ship collision. Saf Sci 76:42–66. https://doi.org/10.1016/j.ssci.2015.02.009
Goerlandt F, Ståhlberg K, Kujala P (2012) Influence of impact scenario models on collision risk analysis. Ocean Eng 47:74–87. https://doi.org/10.1016/j.oceaneng.2012.03.006
Goerlandt F, Montewka J, Kuzmin V, Kujala P (2015) A risk-informed ship collision alert system: framework and application. Saf Sci 77:182–204
Grant E, Salmon PM, Stevens NJ, Goode N, Read GJ (2018) Back to the future: What do accident causation models tell us about accident prediction? Safety Sci 104:99–109
Graziano A, Teixeira AP, Soares CG (2016) Classification of human errors in grounding and collision accidents using the TRACEr taxonomy. Saf Sci 86:245–257
Grech MR, Horberry T, Smith A (2002) Human error in maritime operations: analyses of accident reports using the Leximancer tool. In: Proceedings of the human factors and ergonomics society annual meeting, vol 46(19). Sage Publications, Los Angeles, pp 1718–1721
Griffin MA, Cordery J, Soo C (2016) Dynamic safety capability: how organizations proactively change core safety systems. Organ Psychol Rev 6(3):248–272
Guardian (2021) 'Ever Given, the ship that blocked the Suez Canal, to be released after settlement agreed’ Reuters Online Mon 5 Jul 2021 00.10 BST https://www.theguardian.com/world/2021/jul/05/ever-given-ship-that-blocked-the-suez-canal-to-be-released-after-settlement-agreed
Güven-Koçak S (2015) Maritime informatics framework and literature survey-ecosystem perspective. In: Twenty-first American conference on information systems, Puerto Rico
Hänninen M, Kujala P (2012) Influences of variables on ship collision probability in a Bayesian belief network model. Reliab Eng Syst Saf 102:27–40
Hänninen M, Kujala P (2014) Bayesian network modeling of Port State Control inspection findings and ship accident involvement. Expert Syst Appl 41(4):1632–1646
Hansen HL, Jensen J (1998) Female seafarers adopt the high risk lifestyle of male seafarers. Occup Environ Med 55(1):49–51
Hansen HL, Pedersen G (1996) Influence of occupational accidents and deaths related to lifestyle on mortality among merchant seafarers. Int J Epidemiol 25(6):1237–1243
Haraldstad AMB, Christophersen E (2015) Literature searches and reference management. In: Laake P, Breien Benestad H, Reino B (eds) Research in medical and biological sciences. (Second edition), Academic Press, pp 125–165.
Harrald JR, Mazzuchi TA, Spahn J, Van Dorp R, Merrick J, Shrestha S, Grabowski M (1998) Using system simulation to model the impact of human error in a maritime system. Saf Sci 30(1):235–247. https://doi.org/10.1016/S0925-7535(98)00048-4
Harvey C, Stanton N, Zheng P (2013) Safety at sea: human factors aboard ship The Ergonomist, Issue 517, July, 2013. http://archived.ciehf.org/safety-at-sea-human-factors-aboard-ship/
Harvey C, Stanton NA (2014) Safety in system-of-systems: ten key challenges. Saf Sci 70:358–366
Haugaard M, Clegg SR (eds) (2012) Power and politics. Sage Publications
Hetherington C, Flin R, Mearns K (2006) Safety in shipping: the human element. J Safety Res 37(4):401–411. https://doi.org/10.1016/j.jsr.2006.04.007
Hoc JM (2000) From human–machine interaction to human–machine cooperation. Ergonomics 43(7):833–843
Hogg T, Ghosh S (2016) Autonomous merchant vessels: examination of factors that impact the effective implementation of unmanned ships. Aust J Marit Ocean Aff 8(3):206–222
Hollnagel E (1998) Cognitive reliability and error analysis method (CREAM). Elsevier , Amsterdam
Hollnagel E (2016) Barriers and accident prevention. Routledge , Milton Park
Hollnagel E, Alm H, Axelsson B, Ros A, Shamoun S, Cook R (2014) A FRAM (Functional Resonance Analysis Method) analysis of labour-and-delivery: locating risk in a complex system. International Forum on Quality and Safety in healthcare, Paris, France
Hulme A, Stanton NA, Walker GH, Waterson P, Salmon PM (2019) What do applications of systems thinking accident analysis methods tell us about accident causation? A systematic review of applications between 1990 and 2018. Saf Sci 117:164–183
Islam R, Yu H, Abbassi R, Garaniya V, Khan F (2017) Development of a monograph for human error likelihood assessment in marine operations. Saf Sci 91:33–39
Ismael JT (2011) Self-organization and self-governance. Philos Soc Sci 41(3):327–351
ITOPF (2019) Oil tanker spill statistics published. https://www.itopf.org/news-events/news/2019-oil-tanker-spill-statistics-published/ . Retrieved August 4, 2020
Jenkins DP, Salmon PM, Stanton NA, Walker GH (2010) A systemic approach to accident analysis: a case study of the Stockwell shooting. Ergonomics 53(1):1–17
Jenkins D, Salmon PS, Walker GH (2017) Event analysis of systemic team-work. Modelling command and control. CRC Press, Boca Raton, pp 49–118
Kaber DB, Endsley MR (1997) Out-of-the-loop performance problems and the use of intermediate levels of automation for improved control system functioning and safety. Process Saf Prog 16(3):editor126–131
Kaber DB, Endsley MR (1997) Out-of-the-loop performance problems and the use of intermediate levels of automation for improved control system functioning and safety. Process Saf Prog 16(3):126–131
Khan FI, Amyotte PR, DiMattia DG (2006) HEPI: A new tool for human error probability calculation for offshore operation. Saf Sci 44(4):313–334
Khan B, Khan F, Veitch B, Yang M (2018) An operational risk analysis tool to analyze marine transportation in Arctic waters. Reliab Eng Syst Saf 169:485–502. https://doi.org/10.1016/j.ress.2017.09.014
Kirwan B (1994) A guide to practical human reliability assessment. CRC Press , Boca Raton
Kristiansen S (2005) Maritime transportation: safety management and risk analysis, 1st edn. Routledge, Milton Park . https://doi.org/10.4324/978080473369
Kujala P, Hanninen M, Arola T, Ylitalo J (2009) Analysis of the marine traffic safety in the Gulf of Finland. Reliab Eng Syst Saf 94(8):1349–1357
Kum S, Sahin B (2015) A root cause analysis for Arctic Marine accidents from 1993 to 2011. Saf Sci 74:206–220. https://doi.org/10.1016/j.ssci.2014.12.010
Lenné MG, Salmon PM, Liu CC, Trotter M (2012) A systems approach to accident causation in mining: an application of the HFACS method. Accid Anal Prev 48:111–117. https://doi.org/10.1016/j.aap.2011.05.026
Leveson NG (2011) Applying systems thinking to analyze and learn from events. Saf Sci 49(1):55–64. https://doi.org/10.1016/j.ssci.2009.12.021
Li S, Meng Q, Qu X (2012) An overview of maritime waterway quantitative risk assessment models. Risk Anal 32(3):496–512. https://doi.org/10.1111/j.1539-6924.2011.01697.x
Lissillour R, Bonet Fernandez D (2020) The balance of power in the governance of the global maritime safety: the role of classification societies from a habitus perspective. Supply Chain Forum Int J. https://doi.org/10.1080/16258312.2020.1824533
Lützhöft M, Grech MR, Porathe T (2011) Information environment, fatigue, and culture in the maritime domain. Rev Hum Factors Ergon 7(1):280–322
Michel J, Fingas M (2016) Oil spills: causes, consequences, prevention and countermeasures. In: Fossil fuels: current status and future directions, pp 159–201
Minorsky UV (1959) An analysis of ship collisions with reference to nuclear power plants. J Ship Res 3(2):1–4
Mokhtari AH (2007) Impact of automatic identification system (AIS) on safety of marine navigation. Liverpool John Moores University, Liverpool
Montewka J, Hinz T, Kujala P, Matusiak J (2010) Probability modelling of vessel collisions. Reliab Eng Syst Saf 95(5):573–589
Montewka J, Ehlers S, Goerlandt F, Hinz T, Tabri K, Kujala P (2014a) A framework for risk assessment for maritime transportation systems: a case study for open sea collisions involving RoPax vessels. Reliab Eng Syst Saf 124(13):142–157
Montewka J, Goerlandt F, Kujala P (2014b) On a systematic perspective on risk for formal safety assessment (FSA). Reliab Eng Syst Saf 127:77–85
Munim ZH, Dushenko M, Jimenez VJ, Shakil MH, Imset M (2020) Big data and artificial intelligence in the maritime industry: a bibliometric review and future research directions. Marit Policy Manag 47(5):577–597
Norman DA (1980) Twelve issues for cognitive science. Cogn Sci 4(1):1–32
Norman DA (1981) Categorization of action slips. Psychol Rev 88(1):1–15. https://doi.org/10.1037//0033-295X.88.1.1
Normandin JM, Therrien MC (2016) Resilience factors reconciled with complexity: the dynamics of order and disorder. J Conting Crisis Manag 24(2):107–118
Orlikowski WJ (1992) The duality of technology: rethinking the concept of technology in organizations. Organ Sci 3(3):398–427
Orlikowski WJ (2000) Using technology and constituting structures: a practice lens for studying technology in organizations. Organ Sci 11(4):404–428
Packendorff J (1995) Inquiring into the temporary organization: new directions for project management research. Scand J Manag 11(4):319–333
Patterson JM, Shappell SA (2010) Operator error and system deficiencies: analysis of 508 mining incidents and accidents from Queensland, Australia using HFACS. Accid Anal Prev 42(4):1379–1385. https://doi.org/10.1016/j.aap.2010.02.018
Pedersen PT (2010) Review and application of ship collision and grounding analysis procedures. Mar Struct 23:241–262. https://doi.org/10.1016/j.marstruc.2010.05.001
Perrow C (1984) Normal Accidents: living with High-Risk Technologies. Basic Books, New York
Perrow C (1999) Normal accidents: living with high-risk technologies. Princeton University Press, Princeton
Rasmussen J (1983) Skills, rules, and knowledge; signals, signs, and symbols, and other distinctions in human performance models. IEEE Trans Syst Man Cybern SMC 13(3):257–266. https://doi.org/10.1109/TSMC.1983.6313160
Rasmussen J (1997) Risk management in a dynamic society: a modelling problem. Saf Sci 27(2–3):183–213. https://doi.org/10.1016/S0925-7535(97)00052-0
Rasmussen J (2000) Human factors in a dynamic information society: where are we heading? Ergonomics 43(7):869–879
Reason J (1990) Human error. Cambridge University Press , Cambridge
Reason J (1997) Managing the risks of organizational accidents. Routledge , Milton Park
Reason J (2000) Human error: models and management. BMJ 320(7237):768–770. https://doi.org/10.1136/bmj.320.7237.768
Reinach S, Viale A (2006) Application of a human error framework to conduct train accident/incident investigations. Accid Anal Prev 38(2):396–406. https://doi.org/10.1016/j.aap.2005.10.013
Rerup C (2009) Attentional triangulation: learning from unexpected rare crises. Organ Sci 20(5):876–893
Roberts SE, Hansen HL (2002) An analysis of the causes of mortality among seafarers in the British merchant fleet (1986–1995) and recommendations for their reduction. Occup Med 52(4):195–202
Roberts CM, McClean CJ, Veron JEN, Hawkins JP, Allen GR, McAllister DE, Mittermeier CG, Schueler FW, Spalding M, Wells F, Vynne C, Werner TB (2002) Marine biodiversity hotspots and conservation priorities for tropical reefs. Science 295(5558):1280–1284. https://doi.org/10.1126/science.1067728
Rothblum AM (2002) Keys to successful incident inquiry. In: Human factors in incident investigation and analysis, 2nd international workshop on human factors in offshore operations (HFW2002), Houston, TX
Saaty T (1980) The analytic hierarchy process (AHP) for decision making. In Kobe, Japan, pp 1–69
Sagan S (1993) The limits of safety: organizations, accidents, and nuclear weapons. Princeton University Press, Princeton
Salmon PM, Walker GH, Stanton NA (2015) Pilot error versus sociotechnical systems failure: a distributed situation awareness analysis of Air France 447. Theor Issues Ergon Sci 17(1):64–79. https://doi.org/10.1080/1463922x.2015.1106618
Shappell SA, Wiegmann DA (1997) A human error approach to accident investigation: the taxonomy of unsafe operations. Int J Aviat Psychol 7(4):269–291. https://doi.org/10.1207/s15327108ijap0704_2
Sheridan TB (2008) Risk, human error, and system resilience: fundamental ideas. Hum Factors 50(3):418–426
Shorrock ST, Kirwan B (2002) Development and application of a human error identification tool for air traffic control. Appl Ergon 33(4):319–336. https://doi.org/10.1016/S0003-6870(02)00010-8
Simonsen BC (1997) Mechanics of ship grounding. Department of Naval Architecture and Offshore Engineering, Milton Park, p 260
Soares CG, Teixeira AP (2001) Risk assessment in maritime transportation. Reliab Eng Syst Saf 74(3):299–309
Sovacool BK (2008) The costs of failure: a preliminary assessment of major energy accidents, 1907–2007. Energy Policy 36(5):1802–1820
SSR (2021) Safety and shipping review 2021—allianz global corporate & specialty (AGCS). https://www.agcs.allianz.com/news-and-insights/reports/shipping-safety.html
Stanton NA, Salmon PM, Walker GH (2015) Let the reader decide: a paradigm shift for situation awareness in sociotechnical systems. J Cogn Eng Decis Mak 9(1):44–50
Stanton NA, Plant KL, Roberts AP, Harvey C, Thomas TG (2016) Extending helicopter operations to meet future integrated transportation needs. Appl Ergon 53:364–373
Swain AD, Guttmann HE (1983) Handbook of human-reliability analysis with emphasis on nuclear power plant applications. Final report (NUREG/CR-1278; SAND-80–0200). Sandia National Labs., Albuquerque, NM (USA). Doi: https://doi.org/10.2172/5752058
Terndrup Pedersen P, Zhang S (1998) On Impact mechanics in ship collisions. Mar Struct 11(10):429–449. https://doi.org/10.1016/S0951-8339(99)00002-7
Thompson JD (1967) Organizations. Action: Social Science Bases of Administrative
Trucco P, Cagno E, Ruggeri F, Grande O (2008) A Bayesian Belief Network modelling of organisational factors in risk analysis: a case study in maritime transportation. Reliab Eng Syst Saf 93(6):845–856. https://doi.org/10.1016/j.ress.2007.03.035
Uğurlu Ö, Köse E, Yıldırım U, Yüksekyıldız E (2015a) Marine accident analysis for collision and grounding in oil tanker using FTA method. Marit Policy Manag 42(2):163–185. https://doi.org/10.1080/03088839.2013.856524
UNCTAD (2020) Review of Maritime Transport 2000. United Nations, Geneva
UNCTAD STAT (2019) World seaborne trade by types of cargo and by group of economies, annual. https://unctadstat.unctad.org/wds/TableViewer/tableView.aspx?ReportId=32363
Ung ST (2019) Evaluation of human error contribution to oil tanker collision using fault tree analysis and modified fuzzy Bayesian Network based CREAM. Ocean Eng 179:159–172
Van Eck NJ, Waltman L (2013) Vosviewer manual. Leiden: Univeristeit Leiden 1(1):1–53
van Oorschot JAWH, Hofman E, Halman JIM (2018) A bibliometric review of the innovation adoption literature. Technol Forecast Soc Change 134(2018):1–21
Wang G, Chen Y, Zhang H, Peng H (2002) Longitudinal strength of ships with accidental damages. Mar Struct 15(2):119–138. https://doi.org/10.1016/S0951-8339(01)00018-1
Watson R, Haraldson S, Lind M, Rygh T, Singh S, Voorspuij J, Ward R (2021) Foundations of maritime informatics. The World of Shipping. In: An international conference on maritime affairs, Portugal, January, 16
Weng J, Li G (2019) Exploring shipping accident contributory factors using association rules. J Transp Saf Secur 11(1):36–57
Weng J, Yang D (2015) Investigation of shipping accident injury severity and mortality. Accid Anal Prev 76:92–101
Woods DD, Johannesen LJ, Cook RI, Sarter NB (1994) Behind human error: cognitive systems, computers and hindsight. University of Dayton Research Institute, Dayton
Wróbel K, Montewka J, Kujala P (2017) Towards the assessment of potential impact of unmanned vessels on maritime transportation safety. Reliab Eng Syst Saf 165:155–169
Wu B, Yan X, Wang Y, Zhang D, Guedes Soares C (2017) Three-stage decision-making model under restricted conditions for emergency response to ships not under control. Risk Anal 37(12):2455–2474
Yang ZL, Bonsall S, Wall A, Wang J, Usman M (2013) A modified CREAM to human reliability quantification in marine engineering. Ocean Eng 58:293–303
Zhang W, Goerlandt F, Kujala P, Wang Y (2016) An advanced method for detecting possible near miss ship collisions from AIS data. Ocean Eng 124:141–156
Zupic I, Cater T (2015) Bibliometric methods in management and organization. Organ Res Methods 18(3):429–472
Download references
Acknowledgements
The authors of this paper wish to thank the Master 2 ARAMIS group 2019–2020, at the University of Grenoble-Alpes, for their exploratory thesis on the prevention of recurrence of oil spills.
This research has been developed owing to the IDEX-IRS funding of the University of Grenoble-Alpes (OCEAN project). This work is supported by the French National Research Agency in the framework of the "Investissements d’avenir” program (ANR-15-IDEX-02).
Author information
Authors and affiliations.
Grenoble INP*, CERAG, Univ. Grenoble Alpes, 38000, Grenoble, France
Carine Dominguez-Péry, Lakshmi Narasimha Raju Vuddaraju & Isabelle Corbett-Etchevers
Visiting Fellow at the University of Bath School of Management, Bath, UK
Rana Tassabehji
You can also search for this author in PubMed Google Scholar
Contributions
The order of the authors reflects their level of contribution to the paper. All authors read and approved the final manuscript.
Corresponding author
Correspondence to Carine Dominguez-Péry .
Ethics declarations
Competing interests.
The authors declare that they have no competing interests.
Additional information
Publisher's note.
Springer Nature remains neutral with regard to jurisdictional claims in published maps and institutional affiliations.
Rights and permissions
Open Access This article is licensed under a Creative Commons Attribution 4.0 International License, which permits use, sharing, adaptation, distribution and reproduction in any medium or format, as long as you give appropriate credit to the original author(s) and the source, provide a link to the Creative Commons licence, and indicate if changes were made. The images or other third party material in this article are included in the article's Creative Commons licence, unless indicated otherwise in a credit line to the material. If material is not included in the article's Creative Commons licence and your intended use is not permitted by statutory regulation or exceeds the permitted use, you will need to obtain permission directly from the copyright holder. To view a copy of this licence, visit http://creativecommons.org/licenses/by/4.0/ .
Reprints and permissions
About this article
Cite this article.
Dominguez-Péry, C., Vuddaraju, L.N.R., Corbett-Etchevers, I. et al. Reducing maritime accidents in ships by tackling human error: a bibliometric review and research agenda. J. shipp. trd. 6 , 20 (2021). https://doi.org/10.1186/s41072-021-00098-y
Download citation
Received : 22 March 2021
Accepted : 13 October 2021
Published : 24 November 2021
DOI : https://doi.org/10.1186/s41072-021-00098-y
Share this article
Anyone you share the following link with will be able to read this content:
Sorry, a shareable link is not currently available for this article.
Provided by the Springer Nature SharedIt content-sharing initiative
- Ship accident
- Human error
- Socio-technical use of information technologies
- Organisation
- Bibliometric review
- Open access
- Published: 27 December 2022
Global patterns of diversity and metabolism of microbial communities in deep-sea hydrothermal vent deposits
- Zhichao Zhou 1 na1 ,
- Emily St. John 2 na1 ,
- Karthik Anantharaman 1 &
- Anna-Louise Reysenbach 2
Microbiome volume 10 , Article number: 241 ( 2022 ) Cite this article
7254 Accesses
17 Citations
126 Altmetric
Metrics details
When deep-sea hydrothermal fluids mix with cold oxygenated fluids, minerals precipitate out of solution and form hydrothermal deposits. These actively venting deep-sea hydrothermal deposits support a rich diversity of thermophilic microorganisms which are involved in a range of carbon, sulfur, nitrogen, and hydrogen metabolisms. Global patterns of thermophilic microbial diversity in deep-sea hydrothermal ecosystems have illustrated the strong connectivity between geological processes and microbial colonization, but little is known about the genomic diversity and physiological potential of these novel taxa. Here we explore this genomic diversity in 42 metagenomes from four deep-sea hydrothermal vent fields and a deep-sea volcano collected from 2004 to 2018 and document their potential implications in biogeochemical cycles.
Our dataset represents 3635 metagenome-assembled genomes encompassing 511 novel and recently identified genera from deep-sea hydrothermal settings. Some of the novel bacterial (107) and archaeal genera (30) that were recently reported from the deep-sea Brothers volcano were also detected at the deep-sea hydrothermal vent fields, while 99 bacterial and 54 archaeal genera were endemic to the deep-sea Brothers volcano deposits. We report some of the first examples of medium- (≥ 50% complete, ≤ 10% contaminated) to high-quality (> 90% complete, < 5% contaminated) MAGs from phyla and families never previously identified, or poorly sampled, from deep-sea hydrothermal environments. We greatly expand the novel diversity of Thermoproteia, Patescibacteria (Candidate Phyla Radiation, CPR), and Chloroflexota found at deep-sea hydrothermal vents and identify a small sampling of two potentially novel phyla, designated JALSQH01 and JALWCF01. Metabolic pathway analysis of metagenomes provides insights into the prevalent carbon, nitrogen, sulfur, and hydrogen metabolic processes across all sites and illustrates sulfur and nitrogen metabolic “handoffs” in community interactions. We confirm that Campylobacteria and Gammaproteobacteria occupy similar ecological guilds but their prevalence in a particular site is driven by shifts in the geochemical environment.
Our study of globally distributed hydrothermal vent deposits provides a significant expansion of microbial genomic diversity associated with hydrothermal vent deposits and highlights the metabolic adaptation of taxonomic guilds. Collectively, our results illustrate the importance of comparative biodiversity studies in establishing patterns of shared phylogenetic diversity and physiological ecology, while providing many targets for enrichment and cultivation of novel and endemic taxa.
Video Abstract
Introduction
Actively venting deep-sea hydrothermal deposits at oceanic spreading centers and arc volcanoes support a high diversity of thermophilic microorganisms. Many of these microbes acquire metabolic energy from chemical disequilibria created by the mixing of reduced high-temperature endmember hydrothermal fluids with cold oxygenated seawater. Community analysis of deposits using the 16S rRNA gene has revealed a rich diversity of novel archaeal and bacterial taxa [ 1 , 2 , 3 , 4 ] where the community composition is strongly influenced by the abundance of redox reactive species in high-temperature vent fluids (e.g., [ 5 , 6 , 7 ]). The variations in the composition of endmember fluids, and in turn the microbial community composition at different vent fields, reflect the temperature and pressure of fluid-rock interaction, in addition to substrate composition and entrainment of magmatic volatiles. For example, along the Mid-Atlantic Ridge, methanogens are associated with deposits from H 2 -rich vents at Rainbow and are absent in H 2 -poor vents at Lucky Strike [ 3 ]. At the Eastern Lau Spreading Center (ELSC), similar to other back-arc basins, the hydrothermal fluids are generally quite variable depending on differences in inputs of acidic magmatic volatiles, contributions from the subducting slab, and proximity of island arc volcanoes. Such geochemical differences are imprinted in the diversity of microbial communities [ 3 , 4 ]. Similar complex community structure dynamics have also been recently reported for the communities of the submarine Brothers volcano on the Kermadec Arc [ 8 ].
While such global patterns of high-temperature microbial diversity in deep-sea hydrothermal systems have demonstrated geological drivers of microbial colonization, little is known about the genomic diversity and physiological potential of the many reported novel taxa. While a few metagenomic studies of hydrothermal fluids and sediments have provided a much greater understanding of the functional potential of these communities (e.g., [ 7 , 9 , 10 , 11 , 12 , 13 ]), the metagenomic analysis of deposits has been limited to a small number of samples (e.g., [ 14 , 15 , 16 ]). One exception is the study of about 16 deep-sea hydrothermal deposits from Brothers volcano, which resulted in 701 medium- and high-quality metagenome-assembled genomes (MAGs) [ 8 ]. Further, this study demonstrated that there were functionally distinct high-temperature communities associated with the volcano that could be explained through an understanding of the geological history and subsurface hydrologic regime of the volcano.
Here, we expand on the Brothers volcano study by exploring the genomic and functional diversity of hydrothermal deposits collected from deep-sea vents in the Pacific and Atlantic oceans. We greatly increase the number of novel high-quality assembled genomes from deep-sea vents, many of which are endemic to vents and do not have any representatives in culture yet. We also show that known important biogeochemical cycles in hydrothermal ecosystems are accomplished by the coordination of several taxa as metabolic handoffs, where in some cases different taxa accomplish similar functions in different environments, potentially providing functional redundancy in fluctuating conditions.
Results and discussion
Patterns of metagenomic diversity in deep-sea hydrothermal deposits.
We sequenced 42 metagenomes from 40 samples (38 hydrothermal vent deposit samples and two diffuse flow fluids) collected at deep-sea hydrothermal vents and a deep-sea volcano. These represent one of the largest global collections of metagenomes from such samples (Fig. S 1 , S 2 ). This study spans vent deposit collections from 2004 to 2018, from deep-sea hydrothermal vent fields in the north Atlantic (Mid-Atlantic Ridge, MAR), east and southwest Pacific (East Pacific Rise, EPR; Eastern Lau Spreading Center, ELSC), a sedimented hydrothermal system (Guaymas Basin, GB), and a deep-sea volcano (Brothers volcano, BV) (Table S 1 ).
In this study, de novo assembly of sequencing data and subsequent genome binning and curation (see the “ Methods ” section for details) resulted in 2983 bacterial and 652 archaeal draft metagenome-assembled genomes (MAGs with ≥ 50% completeness, Table S 2 ). Of these, ~ 21% were > 90% complete, with < 5% contamination, and ~ 36% contained a 16S rRNA gene fragment. The MAGs were initially characterized phylogenetically using the Genome Taxonomy Database Toolkit (GTDB-Tk) (Figs. 1 , 2 , and 3 , Data S 1 , S 2 , S 3 , S 4 , S 5 ) [ 17 ]. MAGs that could not be assigned to a known genus by GTDB-Tk were assigned to new genera using AAI with the recommended cutoffs in Konstantinidis et al. [ 18 ] (Table S 3 A, B). Shared phyla between most of the hydrothermal deposits (excluding samples from the highly acidic Brothers volcano sites, and the diffuse flow fluids) included the Halobacteriota (e.g., Archaeoglobaceae), Methanobacteriota (e.g., Thermococcaceae), Thermoproteota (e.g., Acidilobaceae, Pyrodictiaceae), Acidobacteriota, Aquificota (e.g., Aquificaceae), Bacteroidota (e.g., Flavobacteriaceae), Campylobacterota (e.g., Sulfurimonadaceae, Nautiliaceae, Hippeaceae), Chloroflexota, Deinococcota (e.g., Marinithermaceae), Desulfobacterota (e.g., Dissulfuribacteraceae, Thermodesulfobacteriaceae), Proteobacteria (e.g., Alphaproteobacteria, Gammaproteobacteria), and the Patescibacteria (Table S 4 ). Many of these phyla have only a few representatives in isolated cultures and point to the importance of combining enrichment cultivation strategies with metagenomic approaches to obtain additional insights into the physiological ecology of these core lineages.
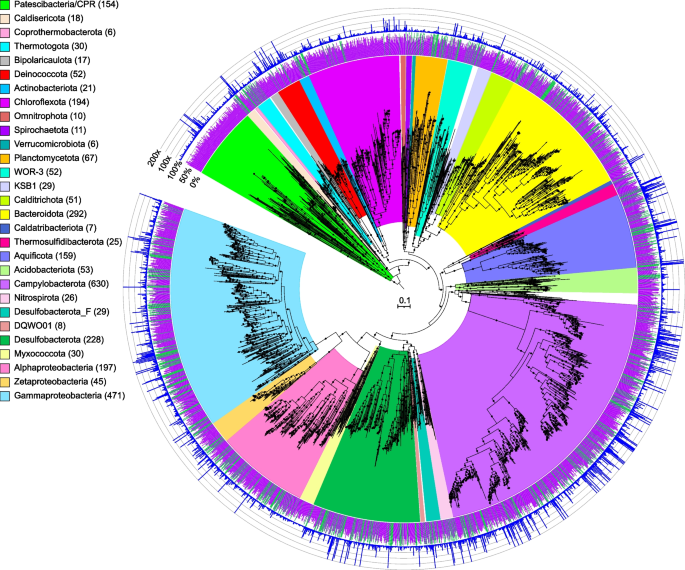
Maximum-likelihood phylogenomic tree of bacterial metagenome-assembled genomes, constructed using 120 bacterial marker genes in GTDB-Tk. Major taxonomic groups are highlighted, and the number of MAGs in each taxon is shown in parentheses. See Table S 2 for details. Bacterial lineages are shown at the phylum classification, except for the Proteobacteria which are split into their component classes. The inner ring displays quality (green: high quality, > 90% completion, < 5% contamination; purple: medium quality, ≥ 50% completion, ≤ 10% contamination), while the outer ring shows normalized read coverage up to 200x. The scale bar indicates 0.1 amino acid substitutions per site, and filled circles are shown for SH-like support values ≥ 80%. The tree was artificially rooted with the Patescibacteria using iTOL. The Newick format tree used to generate this figure is available in Data S 4 , and the formatted tree is available online at https://itol.embl.de/shared/alrlab
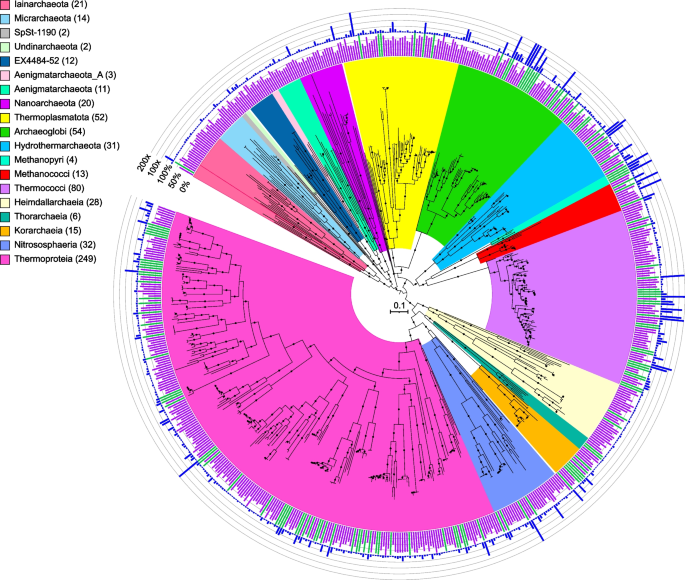
Maximum-likelihood phylogenomic reconstruction of deep-sea hydrothermal vent archaeal metagenome-assembled genomes generated in GTDB-Tk. The tree was generated with 122 archaeal marker genes. Taxa are shown at the phylum level, except for the Thermoproteota, Asgardarchaeota, Halobacteriota, and Methanobacteriota, shown at the class level. The number of MAGs in each highlighted taxon is shown in parentheses. See Table S 2 for details. Quality is shown on the inner ring (green: high quality, purple: medium quality, with one manually curated Nanoarchaeota MAG below the 50% completion threshold also displayed as medium quality), while the outer ring displays normalized read coverage up to 200x. SH-like support values ≥ 80% are indicated with filled circles, and the scale bar represents 0.1 amino acid substitutions per site. The tree was artificially rooted with the Iainarchaeota, Micrarchaeota, SpSt-1190, Undinarchaeota, Nanohaloarchaeota, EX4484-52, Aenigmarchaeota, Aenigmarchaeota_A, and Nanoarchaeota using iTOL. The tree used to create this figure is available in Newick format (Data S 5 ), and the formatted tree is publicly available on iTOL at https://itol.embl.de/shared/alrlab
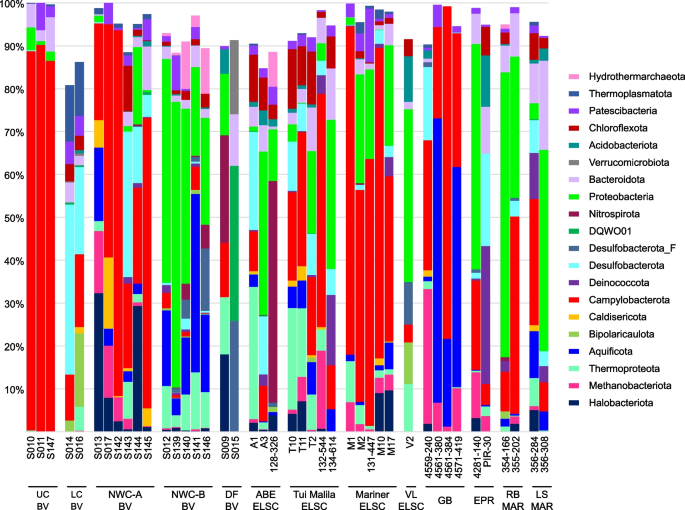
Relative abundance of MAG phyla, based on normalized read coverage. The phyla shown comprise ≥ 10% of the MAG relative abundance in at least one metagenomic assembly. Read coverage was normalized to 100 M reads per sample, and coverage values for MAGs were summed and expressed as a percent. UC, Upper Cone; LC, Lower Cone, NWC-A, Northwest Caldera Wall A; NWC-B, Northwest Caldera Wall B and Upper Caldera Wall; DF, diffuse flow; VL, Vai Lili; RB, Rainbow; LS, Lucky Strike
While shared taxa differed in relative abundance and distribution, observable differences in community structure between vent fields were somewhat limited in this study due to small sample numbers from some of the vent fields (two samples apiece from EPR; Rainbow, MAR; Lucky Strike, MAR), and the overall lower read depth of samples from these sites and a few other samples (Fig. S 3 ). Therefore, obtaining statistically robust community structure patterns using MAG phylogenetic diversity for the entire dataset was not possible. However, Reysenbach et al. [ 8 ] did show that if metagenomic sequencing is deep, assembled MAG diversity tracks 16S rRNA amplicon diversity structure. Extrapolating to this study, the Brothers volcano MAG diversity patterns were retained and confirmed the amplicon observations from Reysenbach et al. [ 8 ] (Fig. S 4 ), and in turn tracked the ELSC MAG community diversity (Fig. 4 A, B). For example, sites at Brothers volcano that were hypothesized to have some magmatic inputs were predicted to be more similar in community structure to the sites along the ELSC with greater magmatic inputs, such as Mariner. Several of the samples from the more acidic Mariner vent field were more closely aligned in MAG diversity structure to those of the acidic solfataric Upper Cone sites at Brothers. The MAG data also demonstrated that the Guaymas samples were quite unique, which is not surprising, given that Guaymas Basin is a sediment-hosted system where the hydrothermal fluid geochemistry is quite different from other basalt- or andesitic-hosted hydrothermal systems (e.g., higher pH, high organics, high ammonia and methane) [ 19 , 20 ].
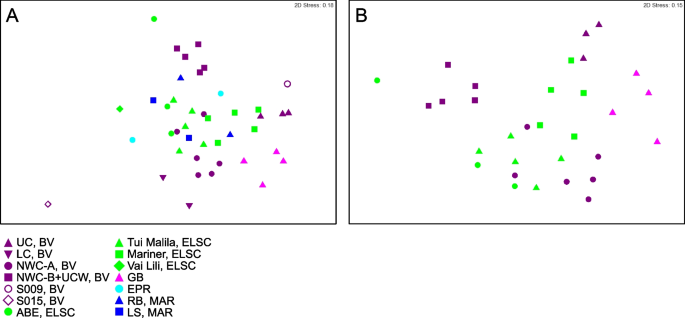
Non-metric multidimensional scaling (NMDS) plots showing taxonomic diversity of MAGs. Plots depict A all samples in this study and B a subset of the data, limited to locations with three or more samples. Plots were generated using Bray–Curtis matrices of the relative abundance of GTDB taxa, based on normalized read coverage of medium- and high-quality MAGs (Table S 4 ; set to 100 M reads and expressed as a percentage of MAG read coverage per sample). Points that are closer together in the plots represent a higher degree of similarity
Our dataset greatly broadens genomic diversity from deep-sea vents, by representing 511 novel and previously identified [ 8 ] genera, comprising 395 Bacteria and 116 Archaea. Notably, 52% (206) of these bacterial genera (Table S 3 A) and 72% (84) of archaeal genera (Table S 3 B) were found at Brothers volcano. Furthermore, 25% (99) of the recently identified bacterial genera and 47% (54) of the archaeal genera were unique to the Brothers volcano samples (Tables S 3 A, B), which further supports the understanding that this environment is a hotbed for novel microbial biodiversity, reflected in the volcano’s complex subsurface geology [ 8 ].
While many of these novel archaeal and bacterial genera were previously reported from Brothers volcano [ 8 ], we report them again here in the context of the new data of the four deep-sea hydrothermal vent environments and the new assemblies (1000 bp contig cutoff, used for Brothers volcano samples and ELSC 2015 samples) and iterative DAS Tool binning used for all our metagenomes. Our data support that of Reysenbach et al. [ 8 ], which used MetaBAT for assemblies (2000 bp contig cutoff) of the Brothers volcano metagenomes. Namely, we recovered approximately 202 novel bacterial genera and 83 new archaeal genera from Brothers volcano communities in Reysenbach et al. [ 8 ], well within the range detected in this analysis (viz. 206 and 84, respectively). In this study, using a lower contig cutoff allowed for the recovery of a much higher number of MAGs, but many are of lower quality with higher contig counts. For example, MAGs recovered in the Reysenbach et al. [ 8 ] study had an average of 254 contigs per MAG, with ~ 19% (135) of MAGs comprising 100 contigs or less. In contrast, only 7% (258) of MAGs in this current study had 100 contigs or less, and the average number of contigs per MAG was 511 (Table S 2 ). However, using the iterative binning approach provided advantages when resolving lineages of high microdiversity, such as in the Nautiliales, with the caveat of creating some MAGs with large collections of erroneous contigs that were poorly detected by CheckM, as they had very few associated marker genes (e.g., MAGs 4571-419_metabat1_scaf2bin.008, M10_maxbin2_scaf2bin.065; Fig. S 5 ). This points to the importance of carefully choosing assembly parameters depending on the ultimate goal of whether quality over quantity of MAGs is preferred for analyses of ecological patterns. Our data demonstrate, however, that overall patterns of MAG diversity are retained regardless of assembly techniques and parameters (Fig. S 4 ).
Furthermore, here we document some of the first examples of medium- to high-quality MAGs from phyla and classes never previously identified, or poorly sampled, from deep-sea hydrothermal environments. These include Thermoproteia, Patescibacteria (formerly Candidate Phyla Radiation, CPR), Chloroflexota, and a few MAGs representing two putative new bacterial phyla, JALSQH01 (3 MAGs) and JALWCF01 (13 MAGs) (Supplementary Discussion, Fig. S 6 , Table S 5 ). For example, with 249 MAGs belonging to the Thermoproteia (Table S 2 , Fig. S 7 ), we have significantly expanded the known diversity and genomes from this phylum. The importance of this group at deep-sea vents was first recognized through 16S rRNA amplicon studies, where the depth of sequencing highlighted that much of this novel thermophilic diversity had been overlooked (e.g., [3, 4]). Furthermore, it is now recognized that many members of this group have several introns in the 16S rRNA gene, which explains why they were missed in original clone library assessments and may be underestimated in amplicon sequencing [ 21 , 22 , 23 , 24 ]. For example, 24 MAGs were related to a recently described genus of the Thermoproteia, Zestosphaera (GTDB family NBVN01) [ 24 ]. This genus was first isolated from a hot spring in New Zealand but is clearly a common member of many deep-sea vent sites. Further, the discovery of a 16S rRNA gene related to Caldisphaera at deep-sea vents [ 25 ], previously only detected in terrestrial acidic solfataras, led to the isolation of related Thermoplasmata— Aciduliprofundum boonei —but the Caldisphaera escaped cultivation. Here we report several high-quality MAGs related to this genus (M2_metabat2_scaf2bin.319, 131-447_metabat1_scaf2bin.050, M1_metabat1_scaf2bin.025, S016_metabat2_scaf2bin.003). Additionally, we also recovered a genome from the Gearchaeales (S146_metabat1_scaf2bin.098), first discovered in iron-rich acidic mats in Yellowstone National Park [ 26 ], and members of the poorly sampled Ignicoccaceae, Ignisphaeraceae, and Thermofilaceae. While we identified several genomes from recently discovered archaeal lineages including the Micrarchaeota, Iainarchaeota, and Asgardarchaeota, we also recovered 15 MAGs belonging to the Korarchaeia, 14 of which comprise two putative novel genera, and one which is closely related to a MAG previously recovered from sediment in Guaymas Basin (Genbank accession DRBY00000000.1) [ 27 , 28 ]. Additionally, we recovered four MAGs from the Caldarchaeales that span two novel genera, one of which was recently proposed as Candidatus Benthortus lauensis [ 29 ] using a MAG generated from a previous assembly of the T2 metagenome (T2_175; Genbank accession JAHSRM000000000.1). MAGs belonging to this genus were identified at both Tui Malila, ELSC, and Brothers volcano (T2_metabat2_scaf2bin.284, S140_maxbin2_scaf2bin.281, S141_maxbin2_scaf2bin.262) with the Tui Malila MAG nearly identical (99.7% AAI similarity) to the described Cand . B. lauensis T2_175 MAG.
While within the Bacteria, the Gammaproteobacteria and Campylobacterota were by far the most highly represented bacterial genomes, there were other lineages for which we have very little if any data or cultures from deep-sea hydrothermal systems (Fig. 3 , Fig. S 7 ). Two such groups are the Patescibacteria and Chloroflexota, with 154 and 194 MAGs respectively.
Patescibacteria and Chloroflexota are diverse and abundant members of deep-sea hydrothermal vent deposits
The Patescibacteria/Candidate Phyla Radiation (CPR) encompasses a phylogenetically diverse branch within the bacterial tree of life that is poorly understood and rarely documented in deep-sea hydrothermal systems. Originally, the CPR was proposed to include several phylum-level lineages [ 30 ], but the entire group was later reclassified by GTDB as a single phylum, Patescibacteria [ 31 ]. Members of the Patescibacteria have been well-characterized in terrestrial soils, sediments, and groundwater [ 32 , 33 , 34 , 35 , 36 , 37 ], and in the mammalian oral cavity [ 38 , 39 , 40 ]. Several 16S rRNA gene and metagenomic studies have also identified members of the Patescibacteria from deep-sea vents, including EPR, MAR, ELSC, and Guaymas Basin [ 3 , 4 , 12 , 15 , 41 , 42 , 43 ], from Suiyo Seamount [ 44 ], and the Santorini submarine volcano [ 45 ], further supporting the widespread distribution of this metabolically diverse phylum.
Our study adds 56 novel genera based on AAI and GTDB classifications to the Patescibacteria phylum. These include large clades within the Gracilibacteria (10 new genera), representatives within the Microgenomatia (9 novel genera), Dojkabacteria (10 new genera), and several clades in the Paceibacteria (13 new genera) (Fig. 5 A, B , Fig. S 8 ). The Gracilibacteria and Paceibacteria were overall the most prevalent lineages of Patescibacteria in the samples but had contrasting distributions across vents (Fig. 5 B). In general, when the Gracilibacteria were prevalent, the Paceibacteria appeared to be a minor component or not present, and vice versa. In particular, the Gracilibacteria MAGs were often associated with the acidic sites such as the Upper Cone at Brothers volcano (S011, S147), and the Mariner vent fields, and in the early colonization experiment from Guaymas Basin (Supplementary Discussion). This may suggest that Gracilibacteria function as early colonizers and are associated with turbulent ephemeral environments as observed previously in oil seeps [ 46 ]. Continued investigation into the ecology, evolution, and host association patterns of these groups, however, may shed more light on these distribution differences.
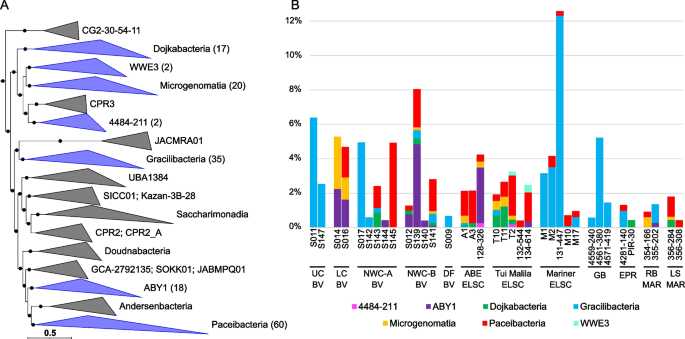
Phylogenomic placement and relative abundance of Patescibacteria MAGs, displayed at the class rank. A Blue clades in the maximum-likelihood phylogenomic tree contain MAGs from this study, with the number of MAGs shown in parentheses. The scale bar shows 0.5 substitutions per amino acid, and filled circles indicate SH-like support (≥ 80%). B Relative abundance of Patescibacteria MAGs was calculated using normalized read coverage for MAGs in each assembly (set to 100 M reads and expressed as a percentage of MAG read coverage per sample)
Consistent with previous studies [ 30 , 34 ], many of the recovered Patescibacteria MAGs had very small genomes (often ~ 1 MB or smaller; Table S 2 ) with highly reduced metabolic potential, often lacking detectable genes for synthesis of fatty acids, nucleotides, and most amino acids (Table S 6 ). Gene patterns also suggested that many of the organisms are obligate anaerobes, lacking aerobic respiration, and that they likely form symbiotic or parasitic associations with other microbes, as has been shown for Patescibacteria cultivated thus far from the Absconditabacterales and Saccharibacteria [ 39 , 40 , 47 , 48 ].
We recovered several MAGs from Mariner, Guaymas Basin, and Brothers volcano that were related to the parasitic Cand . Vampirococcus lugosii [ 47 ] and Cand . Absconditicoccus praedator [ 48 ]. In order to explore if our MAGs had any hints of a parasitic lifestyle, we searched for some of the large putative cell-surface proteins identified in the genomes of Cand . V. lugosii [ 47 ] and Cand . A. praedator [ 48 ]. Using a local BlastP of nine of the longest genes found in Cand . V. lugosii, we recovered high-confidence homologs ( E -value = 0) for alpha-2 macroglobulin genes in several MAGs from the Abscontitabacterales (based on search of Cand . V. lugosii protein MBS8121711.1), which may be involved in protecting parasites against host defense proteases [ 47 ]. We also recovered homologs for PKD-repeat containing proteins (MBS8122536.1; E -value = 0), which are likely involved in protein–protein interactions [ 47 ]. Previous analysis of Cand . V. lugosii found these giant proteins are likely membrane-localized, suggesting they may potentially play a role in host/symbiont interactions. Additionally, we identified these long proteins from Cand . V. lugosii elsewhere in the Gracilibacteria MAGs. For example, putative homologs of the PKD repeat containing protein (MBS8122536.1), a hypothetical protein (MBS8121701.1), and the alpha-2 macroglobulin (MBS8121711.1) were identified in multiple other orders of the class Gracilibacteria ( E -value ≤ 1E − 25). The alpha-2 macroglobulin was also identified in the very distantly related Paceibacteria, and a single putative homolog of the alpha-2 macroglobulin was found in a MAG belonging to the class WWE3 (134-614_metabat1_scaf2bin.084; E -value ≤ 1E − 24).
While the Patescibacteria likely rely on symbiotic or parasitic relationships, members of the Chloroflexota phylum are diverse and metabolically flexible organisms, capable of thriving in a wide variety of geochemical niches. Chloroflexota are abundant and widely distributed in a variety of environments, including terrestrial soils, sediments and groundwater, freshwater, pelagic oceans, and the marine subseafloor and sediments [ 49 , 50 , 51 , 52 , 53 , 54 , 55 ], and hydrothermal settings such as Guaymas Basin [ 11 ] and Brothers submarine volcano [ 8 ]. Genomic evidence suggests that Chloroflexota are associated with important metabolisms in the carbon cycle, including fermentation, carbon fixation, acetogenesis, and the utilization of sugars, polymers, fatty acids, organic acids, and other organic carbon compounds [ 50 , 51 , 54 ].
Here we add to the growing evidence that the Chloroflexota are diverse and metabolically versatile members of deep-sea hydrothermal vent communities. We recovered a total of 194 Chloroflexota MAGs spanning 12 orders (GTDB taxonomy), which included 22 novel genera. Of these novel genera, 14 were identified at Brothers volcano and 6 were unique to the Brothers volcano samples (Table S 3 A). Based on read coverage, Chloroflexota MAGs were in high relative abundance (≥ 7%) in several samples from the ELSC, namely, from Tui Malila and ABE, and in one NW Caldera Wall sample from Brothers volcano (Table S 4 ). To further explore the metabolic potential of Chloroflexota in hydrothermal vent communities, we focused our analyses on ≥ 80%-completeness MAGs (≥ 80% completeness, n = 58) distributed in 6 orders: Caldilineales, Promineofilales, Anaerolineales, Ardenticatenales, B4-G1, and SBR1031 (Fig. 6 , Table S 7 A).
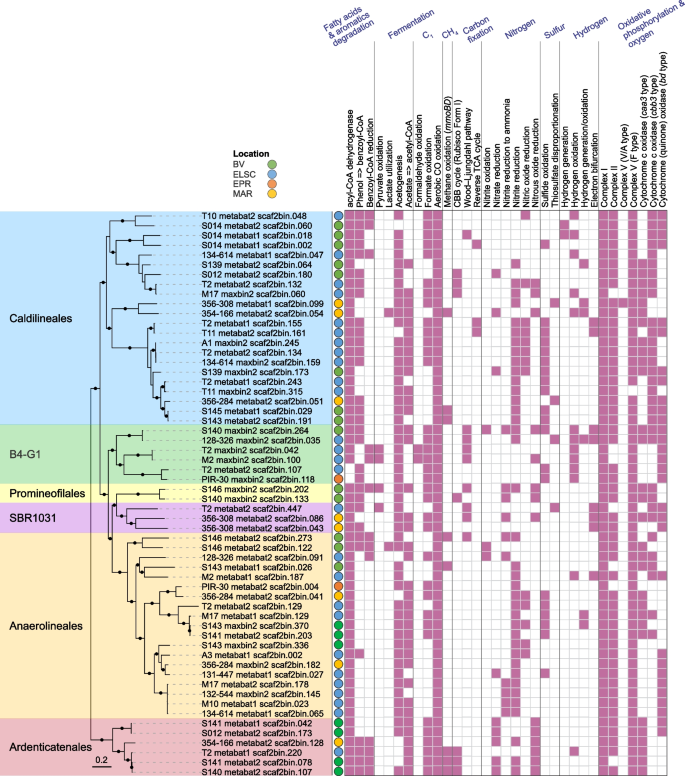
Phylogenetic tree of 58 ≥ 80%-completeness Chloroflexota MAGs with predicted functional capabilities. Nodes with ultrafast bootstrap support values ≥ 90% are shown with filled circles, and the scale bar shows 0.2 substitutions per site. One genome from the GTDB r202 database (GTDB accession GB_GCA_007123655.1) was used to re-root the tree. Hydrothermal vent fields: Brothers volcano (green), Eastern Lau Spreading Center (blue), East Pacific Rise (orange), Mid Atlantic Ridge (yellow)
The majority (≥ 75%) of the ≥ 80%-completeness Chloroflexota MAGs encoded marker genes involved in several processes previously associated with the Chloroflexota (Table S 7 B), including fatty acid degradation [ 50 , 55 ], formate oxidation [ 56 ], aerobic CO oxidation [ 57 ], and selenate reduction [ 53 ]. Except for the Anaerolineales, over 66% of the MAGs in the other five orders had the capacity for degradation of aromatic compounds, as previously reported for Chloroflexota from the marine subsurface [ 51 ]. While some MAGs had the potential for substrate-level phosphorylation through acetate formation, most of the MAGs contained pathways for oxidative phosphorylation and oxygen metabolism [ 50 , 51 ]. The Wood–Ljungdahl pathway, the CBB cycle based on a Form I Rubisco, and the reverse TCA cycle were detected in some of the MAGs [ 50 , 51 ]. Soluble methane monooxygenase genes, a metabolic potential recently also detected in a Chloroflexota MAG from the arctic [ 58 ], were identified in a total of eight of our MAGs from the orders Caldilineales, Anaerolineales, and Ardenticatenales.
Although the primary metabolic potential of the hydrothermal vent-associated Chloroflexota was in carbon cycling, we did, however, observe minor evidence for their roles in nitrogen and sulfur cycling (Fig. 6 , Table S 7 ). About 22% of the MAGs (with ≥ 80% completeness) encoded capacities for sulfide oxidation, as previously reported for members of this group, e.g., Chloroflexus spp. [ 59 , 60 ]. The potential to disproportionate thiosulfate was also observed in a few MAGs. Further, thermophilic Chloroflexota grown in an enrichment culture from Yellowstone National Park were shown to oxidize nitrite. A few of our MAGs encoded genes involved in nitrite oxidation [ 61 ], while a larger proportion of the MAGs encoded genes for nitrite or nitric oxide reduction. None of the MAGs encoded complete pathways for entire sulfur oxidation or denitrification, suggesting that Chloroflexota in these environments may be associated with metabolic handoffs involving other community members (see below).
Metabolic and functional diversity in deep-sea hydrothermal vent deposits
In order to explore the metabolic and functional diversity associated with our MAGs, we utilized functional assignment results in tandem with the corresponding MAG relative abundance (Table S 8 ). In general, genes involved in carbon, nitrogen, sulfur, and hydrogen metabolism were prevalent and shared across all hydrothermal systems in this study (Figs. 7 and 8 ). While heterotrophy, autotrophy, and mixotrophy potential were identified in all samples, 47.1% of the MAGs (by count) exhibited potential for carbon fixation. Marker genes associated with five different carbon fixation pathways were identified in the MAGs, namely, the Calvin-Benson-Bassham (CBB) cycle (form I or form II Rubisco), the 3-hydroxypropionate/4-hydroxybutyrate cycle, the dicarboxylate/4-hydroxybutyrate cycle, the reverse TCA cycle, and the Wood–Ljungdahl pathway (Figs. 7 and 8 ). Marker gene presence also suggested the potential for widespread heterotrophic metabolism of peptides, polysaccharides, nucleotides, and lipids, and fermentation via acetogenesis (Figs. 7 and 8 ).
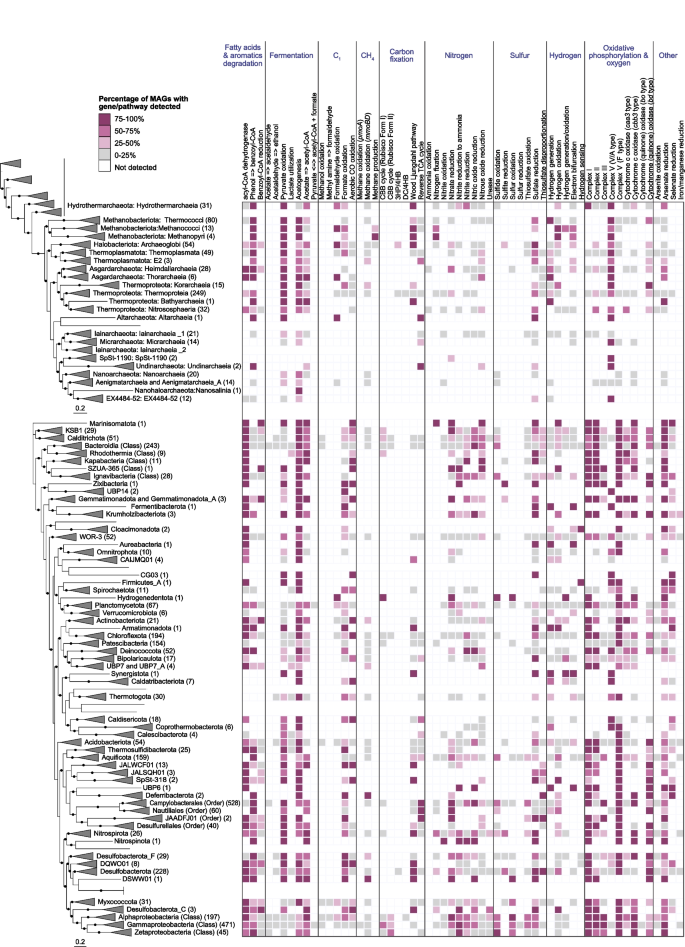
Core metabolic gene presence across phylogenetic clusters in deep-sea hydrothermal vent deposits. The number of MAGs in each clade is shown in parentheses, and MAGs belonging to unclassified lineages or falling outside their corresponding phylogenetic cluster due to unstable tree topology are shown without names. In instances where a phylum was not recovered as a monophyletic lineage within the tree (e.g., Iainarchaeia), MAG count and gene distribution for the entire phylum is only shown on one of the branches. Unless otherwise indicated, archaeal clades are shown at the class level, while bacterial clades are shown at the phylum level. Nodes with ultrafast bootstrap support ≥ 90% are shown with filled circles, and scale bars indicating 0.2 amino acid substitutions per site are provided for both archaeal and bacterial trees. Detailed metabolic gene presence information can be found in Table S 9
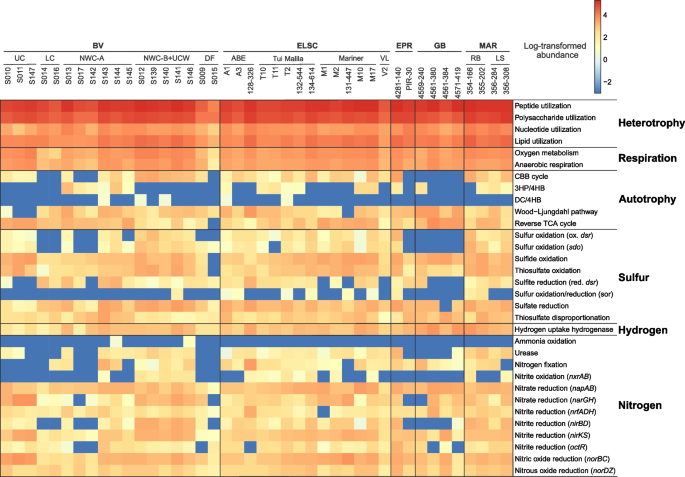
Heatmap displaying the metabolic potential for each metagenome. Within each metagenomic dataset, functional abundance values were calculated as described in the methods. Functional abundances were then log-transformed, with abundance values equal to zero replaced by 10 −3 to avoid negative infinite values
Genes involved in nitrogen fixation, denitrification, and nitrite oxidation were identified across the different hydrothermal sites, yet the potential for anaerobic or aerobic ammonia oxidation was rarely detected (Fig. 8 ). The absence of ammonia oxidation is not totally surprising, since ammonia is in very low to undetectable concentrations in deep-sea hydrothermal fluids, with the exception of sediment-hosted hydrothermal areas like at Guaymas Basin [ 19 , 20 ]. In these sedimented hydrothermal systems, aerobic and anaerobic ammonia oxidation are key processes within the sediments and hydrothermal plumes [ 62 , 63 , 64 , 65 ], but they may not be as important in the hydrothermal deposits. Our data also expands the importance of nitrogen fixation from the first detection at deep-sea vents in Methanocaldococcus [ 66 ] to a greater diversity of hydrothermal Bacteria and Archaea.
Given the importance of sulfur cycling in deep-sea hydrothermal systems [ 67 , 68 , 69 ], it is not surprising that genes associated with elemental sulfur, sulfide, and thiosulfate oxidation; sulfate reduction; and thiosulfate disproportionation were widely distributed in MAGs from different hydrothermal samples and were associated with diverse taxonomic guilds (Figs. 7 and 8 ). Based on metabolic gene distribution statistics (Table S 9 ), the potential for sulfur oxidation was identified in 16% of the MAGs (577), primarily in members of the Alphaproteobacteria and Gammaproteobacteria. Genes associated with sulfide oxidation were identified in 34% of the MAGs (1216), including members of the Bacteroidia, Campylobacteria, Alphaproteobacteria, and Gammaproteobacteria. Thiosulfate oxidation genes were detected in 23% of the MAGs (836), largely comprised of the Campylobacteria, Alphaproteobacteria, and Gammaproteobacteria, while 14% of the MAGs (522) encoded genes for thiosulfate disproportionation, including the classes Bacteroidia and Campylobacteria and the phylum Desulfobacterota. The potential for dissimilatory sulfite reduction was identified in 6% of the MAGs (220) distributed across ten bacterial and archaeal phyla, namely Halobacteriota (class Archaeoglobi), Bacteroidota (class Kapabacteria), Campylobacterota (class Campylobacterales), Zixibacteria, Gemmatimonadota, Acidobacteriota, Nitrospirota, Desulfobacterota, Desulfobacterota_F, and Myxococcota.
Hydrogen is highly variable in hydrothermal fluids, with some of the highest concentrations in geothermal systems hosted by ultramafic rocks, such as the Rainbow hydrothermal vent field [ 3 ], or in sediment-hosted regions like Guaymas basin [ 70 ]. In these systems, methanogens and sulfate reducers are prevalent hydrogen consumers [ 3 , 71 , 72 , 73 , 74 ], although a wide variety of other heterotrophs and autotrophs can also derive energy from hydrogen oxidation [ 72 ]. Hydrogenase enzymes are responsible for mediating hydrogen oxidation in microbial populations but are also involved in a variety of other functions, including hydrogen evolution, electron bifurcation, and hydrogen sensing [ 75 ]. Approximately 27% of the MAGs in this study (974) encoded for at least one hydrogenase gene for hydrogen oxidation, and the MAGs were predominantly associated with the classes Campylobacteria, Bacteroidia, Gammaproteobacteria, and the phylum Desulfobacterota (Figs. 7 and 8 , Table S 9 ). In several cases (132 MAGs), hydrogenase genes co-occurred with genes involved in the oxidation of reduced sulfur species (sulfide, elemental sulfur, sulfite, or thiosulfate). This is not surprising, given that the capability to oxidize both sulfur and hydrogen has been shown in multiple isolates, including members of the Campylobacteria [ 76 , 77 , 78 ] and Aquificae (e.g., [ 79 , 80 ]).
Metabolic handoffs are a central feature of community interactions in deep-sea hydrothermal vent deposits
The microbial communities at deep-sea hydrothermal vents are shaped by a wide variety of complex interactions, including symbiosis, syntrophy, commensalism, cross-feeding, and metabolic handoffs [ 11 , 12 , 81 , 82 , 83 ]. While many of the MAGs encode genes associated with different biogeochemical cycles, as expected, the genes for a complex functional pathway often were not localized in a single MAG, but instead distributed across several MAGs. This is likened to “metabolic handoffs” where the interaction between different organisms produces pathway intermediates, enabling community members to perform downstream reactions in the metabolic pathway. For example, metagenomic analysis of a subsurface aquifer environment suggested that metabolic handoffs are commonly utilized in key biogeochemical pathways such as sulfide oxidation and denitrification [ 37 ]. Genes for sulfide oxidation were identified in all the deep-sea hydrothermal vent sites in this study, but few MAGs encoded genes for the entire three-step pathway. A much larger proportion of the MAGs, however, contained genes for a single step in sulfur oxidation (Fig. 9 ), consistent with a metabolic handoff scenario. Similar patterns were also observed for sulfate reduction and denitrification (Fig. 9 ). Additionally, the genes for individual steps in sulfide oxidation were often found coupled with at least one gene from the denitrification pathway, which may increase the thermodynamic favorability of both pathways. Furthermore, one or more denitrification genes co-occurred with sulfide oxidation genes in 1113 MAGs, with elemental sulfur oxidation genes in 485 MAGs and with sulfite oxidation genes in 1025 MAGs (Table S 9 ). We recognize that some of these observations may be attributed to the incompleteness of the MAGs; however, our observations are in line with similar findings from other environments such as the terrestrial subsurface [ 37 ].
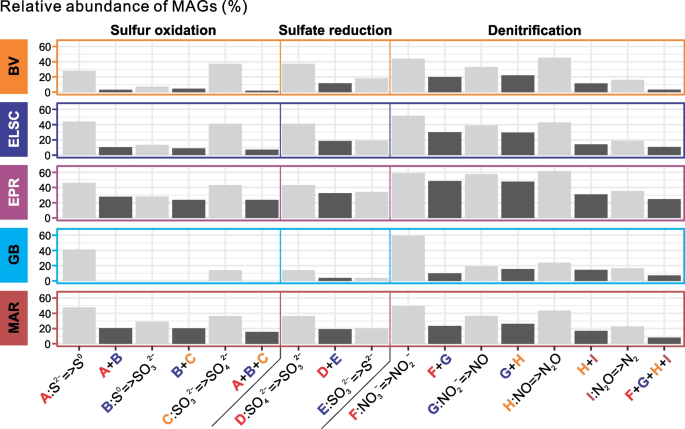
Bar plots showing the sequential steps of sulfur oxidation, denitrification, and sulfate reduction. Bar height indicates the percent relative abundance of MAGs in each metagenome with genes for a particular function(s), averaged across hydrothermal vent sites
Conserved microbial functions are mediated by different taxa at different hydrothermal vent systems
Previous analyses of deep-sea hydrothermal environments and global oceans have pointed to widespread functional redundancy in microbial communities [ 8 , 12 , 84 , 85 ], with similar metabolic potential identified across taxonomically diverse samples. For example, a study of Guaymas Basin metagenome-assembled genomes suggested that many functional genes could be identified across multiple distinct taxa [ 12 ]. In our study, members of the Campylobacteria and Gammaproteobacteria were present in almost all samples, yet showed contrasting patterns of abundance (Fig. 10 ). These lineages can perform several of the same functional processes including oxidation of reduced sulfur species [ 86 ], denitrification [ 87 , 88 , 89 ], and carbon fixation [ 90 , 91 , 92 , 93 ]. This can be partially explained by ecophysiological and growth differences between the groups, which are selected for by the different geochemical profiles at the various vent sites. For example, studies have suggested that Campylobacteria tend to favor higher sulfide conditions but have a broader range of oxygen tolerance than the Gammaproteobacteria, while Gammaproteobacteria tend to inhabit a narrower range of higher oxygen and lower sulfide [ 16 , 86 , 90 , 94 ]. It is therefore not surprising that the Campylobacteria were more prevalent at several of the acidic and more turbulent sites, such as at the Upper Cone, Brothers volcano, and in early colonized samples from a thermocouple array at Guaymas Basin (Table S 4 , Supplementary Discussion). Patwardhan et al. [ 95 ] also showed that Campylobacteria were early colonizers of shallow marine vents followed by Gammaproteobacteria, and their differential colonization could be linked to sulfide, oxygen, and temporal differences.
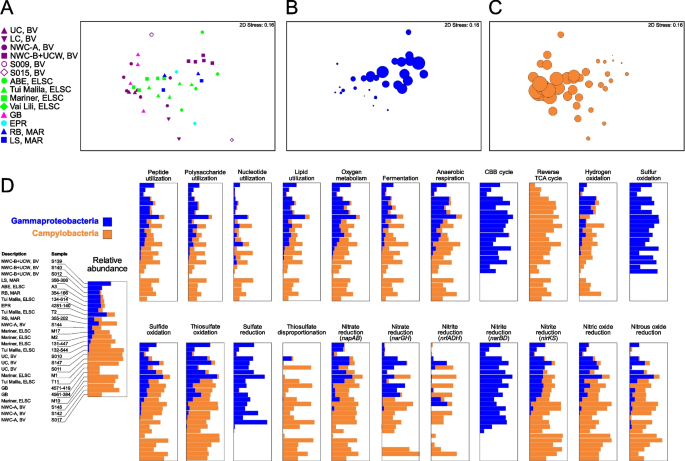
Comparative taxonomic and functional gene abundance of the Campylobacteria and Gammaproteobacteria. NMDS plots were generated using a Bray–Curtis matrix of relative MAG abundance, based on GTDB-assigned taxonomy at the class level. Plots are shown for A all sample sites, and for all sample sites with bubbles proportional to the relative abundance of B Gammaproteobacteria and C Campylobacteria. D Comparative functional distribution is also shown for the Gammaproteobacteria and Campylobacteria for the 26 samples that had a summed relative abundance of both Gammaproteobacteria and Campylobacteria of ≥ 30%. The 22 functions depicted were selected as the Gammaproteobacteria and Campylobacteria accounted for an average of ≥ 20% of the total abundance for each function across the metagenomes
The covariation of the Campylobacteria and Gammaproteobacteria in our data also coincided with genes for key functional processes associated with these taxa (Fig. 10 ). Thus, the overall ecological function contributed by the Campylobacteria and Gammaproteobacteria to the community at all sites was similar, but carried out by either one, viz., same guild different taxa. For example, relative gene abundance of individual functions tracked the relative abundance of Campylobacteria and Gammaproteobacteria for 15 of 22 broadly distributed functions, including heterotrophy associated with various organic carbon compounds, respiration of oxygen and nitrogen compounds, and oxidation of reduced sulfur compounds. However, genes for some functions were exclusively represented by either group (Fig. 10 , Table S 10 ). For example, marker genes for formaldehyde oxidation, urea utilization, and elemental sulfur oxidation were found in the Gammaproteobacteria but were hardly detected in Campylobacteria, while genes associated with thiosulfate disproportionation were attributed almost exclusively to Campylobacteria (Fig. 10 , Table S 10 ). In some cases, metabolic analysis also suggested that both Campylobacteria and Gammaproteobacteria had similar metabolic capabilities but encoded different pathways for the same functions. For example, consistent with a previously observed but non-ubiquitous trend [ 90 , 91 , 92 , 93 ], Campylobacteria mostly encoded genes for the rTCA cycle while the Gammaproteobacteria encoded genes for the CBB cycle. Both taxa also showed the potential for nitrite reduction to ammonia, with more nrfADH genes identified in the Campylobacteria and nirBD only found in the Gammaproteobacteria.
Conclusions
From a comparative metagenomic analysis of 38 deep-sea hydrothermal deposits from multiple globally distributed sites, we provide insights into the shared vent-specific lineages and greatly expand the genomic representation of core taxa that have very few, if any, examples in cultivation. Furthermore, we document many novel high-quality assembled genomes that were originally only identified from deep-sea vents as 16S rRNA genes. This study sheds light on the metabolic potential and physiological ecology of such taxa. We show that overall, the different communities share similar functions, but differences in the environmental geochemistry between sites select distinct taxonomic guilds. Further, metabolic handoffs in communities provide functional interdependency between populations achieving efficient energy and substrate transformation, while functional redundancy confers higher ecosystem resiliency to perturbations and geochemical fluctuations. In summary, this study provides an integrated view of the genomic diversity and potential functional interactions within high-temperature deep-sea hydrothermal deposits and has implications on their biogeochemical significance in mediating energy and substrate transformations in hydrothermal environments.
Sample collection, DNA extraction, and sequencing
High-temperature, actively venting deep-sea hydrothermal deposits, a diffuse flow sample, and a water sample were collected from Brothers volcano (2018), the Eastern Lau Spreading Center (2005 and 2015), Guaymas Basin (2009), the Mid-Atlantic Ridge (2008), and the East Pacific Rise (2004 and 2006) as previously described (Flores et al., 2012a, Reysenbach et al., 2020). Expedition details, including identification numbers, research vessels, and submersibles utilized for sampling, are described in Table S 1 . Samples were processed [ 4 ] and DNA extraction was performed as previously described [ 4 , 8 , 25 , 96 ].
Thermocouple array from Guaymas Basin
The thermocouple array experimental setup from Guaymas Basin in 2009 is described in Teske et al. [ 20 ].
Metagenomic assembling and binning
Reads from Brothers volcano and ELSC (2015) were quality-filtered using FastQC v.0.11.8 ( https://www.bioinformatics.babraham.ac.uk/projects/fastqc/ ) and de novo assembled using metaSPAdes v.3.12.0 [ 97 ] with the settings “-k 21,33,55,77,99,127 -m 400 –meta”. Reads from ELSC (2005), MAR, EPR, and Guaymas Basin were assembled by the Department of Energy, Joint Genome Institute (JGI) using metaSPAdes v.3.11.1 with the settings “-k 33,55,77,99,127 –only-assembler –meta”. Individual assemblies were generated for each metagenomic dataset. MetaWRAP v.1.2.2 [ 98 ] was used to generate metagenome-assembled genomes (MAGs) from each assembly with the settings “–metabat2 –metabat1 –maxbin2”. DAS Tool v.1.0 [ 99 ] was then applied to screen the three sets of MAGs generated by MetaWRAP, resulting in consensus MAGs with a minimum scaffold length of 1000 bp.
Metagenome-assembled genome curation and quality assessment
CheckM v.1.0.7 [ 100 ] was used to assess MAG quality and screen for the presence of 16S rRNA genes. Erroneous SSU genes were then removed using RefineM v.0.0.20 [ 101 ], which was also used to identify and remove outlier scaffolds with abnormal coverage, tetranucleotide signals, and GC patterns from highly contaminated MAGs. GTDB-Tk v.1.5.0, data release 202 [ 17 ], was used to assign taxonomy to each MAG with default settings. SSU sequences from each MAG were then re-parsed and annotated by SINA v.1.2.11 [ 102 ]. Scaffolds containing 16S rRNA gene sequences inconsistent with GTDB taxonomic classifications were deemed contaminants and were removed. Selected MAGs were then further refined and manually inspected by VizBin v.1.0.0 [ 103 ]. Final MAGs had an estimated ≥ 50% genome completion and ≤ 10% contamination, with completeness and contamination rounded to the nearest whole number.
Iterative Nanoarchaeota MAG curation
As a case study, two MAGs assigned to the Nanoarchaeota (4571-419_metabat1_scaf2bin.008, M10_maxbin2_scaf2bin.065) were iteratively curated, demonstrating that the original MAGs generated by DAS Tool contained large quantities of contaminant contigs that were not recognized by CheckM, given the low abundance of marker genes. Each MAG was visualized using the Anvi’o v.7.1 interactive interface [ 104 ], where contigs were divided into subsets based on clustering patterns in Anvi’o. Contigs in each cluster were assigned a putative taxonomy using the Contig Annotation Tool (CAT) [ 105 ]. Clusters containing most of the contigs assigned to the Nanoarchaeota were repeatedly sub-sampled and screened using the CAT pipeline until no meaningful correspondence between clustering patterns and assigned taxonomy could be identified (Fig. S 5 ). Contigs in the final clusters were then removed if CAT definitively assigned them to a taxonomic group outside the Nanoarchaeota, while contigs assigned to the Nanoarchaeota and unclassified higher ranks were retained. A third Nanoarchaeota MAG (4281-140_maxbin2_scaf2bin.078) was also identified, but attempted curation using the above workflow revealed the presence of extensive contamination, with only a very small subset of scaffolds confidently assigned to the Nanoarchaeota. CAT analysis of a putative Nanoarchaeota MAG (JGI Bin ID 3300028417_39) separately assembled from the same read set by the JGI as part of the Genomes from Earth’s Microbiomes project [ 106 ] also showed very few contigs assigned to the DPANN superphylum and extensive bacterial contamination, suggesting that this particular read set may represent a challenge for commonly utilized binning algorithms. Given the extensive contamination and difficulty identifying a valid Nanoarchaeota MAG of significant size, the 4281-140_maxbin2_scaf2bin.078 was excluded from the MAG dataset submitted to Genbank, so as to avoid contaminating the public database with erroneous information. However, the MAG was included in functional and relative abundance calculations.
MAG characterization and annotation
Open reading frames (ORFs) were predicted by Prodigal v.2.6.3 [ 107 ] with the parameter “-p meta”. ORFs were then annotated by KOfam [ 108 ] and custom HMM profiles within METABOLIC v.4.0 [ 109 ] and eggNOG-emapper v.2.1.2 [ 110 ] with default settings. Transfer RNAs were predicted using tRNAscan-SE 2.0 using the general tRNA model [ 111 ]. Genomic properties, including genome coverage, genome and 16S rRNA taxonomy, tRNAs, genome completeness, and scaffold parameters, were parsed from results that were calculated by CheckM, tRNAscan-SE 2.0, and METABOLIC. Relative genome coverages were normalized by setting each metagenomic dataset size as 100 M paired-end reads.
Prior to detailed metabolic analysis, open reading frames from the Gracilibacteria orders BD1-5 and Absconditabacterales, which are known to use genetic code 25 (e.g., [ 47 , 48 , 112 , 113 ]), were re-called using Prodigal v.2.6.3 as implemented in Prokka v.1.14.6 [ 114 ]. An additional MAG from the Gracilibacteria order GCA-2401425 (4559-240_metabat1_scaf2bin.085) was also processed using genetic code 25. Currently, the only other genome in GTDB order GCA-2401425 (Genbank accession NVTB00000000.1) [ 115 ] is publicly available in Genbank with ORFs generated using genetic code 11. However, comparative analysis of our GCA-2401425 MAG showed that ORFs called with genetic code 11 were truncated, with an average length of approximately 85 amino acids, while those called with genetic code 25 averaged 277 amino acids in length. ORFs from two additional MAGs from the Paceibacteria (A3_metabat2_scaf2bin.333 and S145_metabat2_scaf2bin.004) were also re-generated in Prokka using genetic code 11. Open reading frames were then annotated in GhostKoala [ 116 ].
Phylogenomic inference
For archaeal phylogenomic tree construction, a concatenated multiple sequence alignment (MSA) was generated in GTDB-Tk using 122 archaeal marker genes (2991 sequences, 5124 columns) [ 17 ]. IQ-TREE v.1.6.9 [ 117 ] was used to reconstruct the tree with the settings “-m MFP -bb 1000 -redo -mset WAG,LG,JTT,Dayhoff -mrate E,I,G,I + G -mfreq FU -wbtl” (Data S 1 ). The bacterial phylogenomic tree was constructed in a similar manner, using a concatenated MSA of 120 bacterial GTDB marker genes [ 17 ]. For each GTDB bacterial phylum, no more than 15 reference genomes from the GTDB r202 database were used (4248 sequences, 5037 columns; Data S 2 ). Additionally, a second bacterial phylogenomic tree was inferred from the same MSA using FastTree v.2.1.8 (WAG, + gamma, SH support; Data S 3 ) [ 118 ]. Additional MSAs solely using MAGs from this study were generated for the Archaea (122 marker genes) and Bacteria (120 marker genes) using the GTDB-Tk identify and align commands [ 17 ]. FastTree v.2.1.10 (parameter: –gamma) was used to infer the phylogenomic trees, as implemented in GTDB-Tk (Data S 4 , S 5 ; formatted trees available online at https://itol.embl.de/shared/alrlab ).
A tree was constructed in GTDB-Tk (parameter: –gamma) using MAGs assigned to the Patescibacteria, along with recently described Cand . Vampirococcus lugosii [ 47 ] and Cand . Absconditicoccus praedator [ 48 ], and the GTDB r202 bacterial tree-building dataset. A phylogenomic tree of the Chloroflexota was also generated by extracting a concatenated MSA of Chlorofexota MAGs from the entire bacterial MSA. IQ-TREE v.2.1.4 [ 119 ] was used to reconstruct the tree with the settings “-m TESTMERGE -bb 1000 -bnni”. An outgroup genome (GCA_007123655.1) was added to reroot the phylogenomic tree. Final trees were visualized using Interactive Tree of Life (iTOL) v.6 [ 120 ].
Taxonomic assignment
Initial taxonomy was assigned to each MAG using the GTDB-Tk classify pipeline. In rare instances where there were discrepancies between the class-level (Archaea) or phylum-level taxonomy (Bacteria) assigned by GTDB-Tk and phylogenetic tree topology, we deferred to tree topology. In the Bacteria, topological taxonomic assignments were only used if confirmed by both trees. MAGs that were not assigned to a known genus by GTDB-Tk were compared to their closest relatives in this study using average amino acid identity (AAI) matrices generated in CompareM v.0.1.2 ( https://github.com/dparks1134/CompareM ). MAGs were assigned to novel genera using cutoffs provided by Konstantinidis et al. [ 18 ], and MAGs assigned the taxonomic status “unclassified” were automatically assigned to a novel genus.
Trophic and energy metabolism analysis
Functional genes were first characterized by METABOLIC [ 109 ]. Additional peptide utilization genes were characterized using the MEROPS database release 12.3 [ 121 ], and additional polysaccharide utilization genes were identified using dbCAN2 (2020–04-08) and the CAZy (2021–05-31) database [ 122 , 123 ]. Cellular localization of peptidases/inhibitors, gene calls identified by the CAZy database, and predicted extracellular nucleases were verified using PSORTb v.3.0 [ 124 ]. Functional annotations for protein, polysaccharide, nucleic acid, and lipid utilization were derived in part from previous publications [ 125 , 126 ]. Iron cycling genes and hydrogenase genes were characterized based on HMMs directly obtained or indirectly parsed from FeGenie [ 127 ] and HydDB [ 75 ].
For each of these trophic and energy metabolisms, the number of functional gene calls in each genome was calculated using two different scenarios: (1) the presence of any marker gene in the complex/pathway was treated as the presence of the whole function (indicated as C), and the highest number of gene calls for an individual gene in the complex was taken to be the number of pathway “hits” in the MAG. (2) Stand-alone genes that were not part of a large complex or functional pathway (indicated as A) were treated as individual accumulative gene calls for their particular function. In specific cases, marker genes were manually verified using phylogenetic trees and by inspecting operon arrangements (see below). To calculate functional abundance, all genomes were included in the analysis. Functional abundance was then calculated by multiplying normalized genome coverage (100 M reads/sample) by the number of functional gene calls for each sample. For visualization, functional abundance was then log-transformed and used to generate heatmaps with the R package pheatmap v.1.0.12 (settings: clustering_method = ward.D2). Combined functional heatmaps were also generated by summing values within larger functional groups.
To avoid potential mis-annotation by the automated methods described above, phylogenetic trees were constructed to validate predicted protein sequences for dissimilatory sulfite reductase (Dsr; Fig. S 9 ), methyl-coenzyme M reductase subunit alpha (McrA; Fig. S 10 ), and sulfur dioxygenase (Sdo; Fig. S 11 ). Based on current understanding, two metabolic directions are possible for the Dsr protein: reductive Dsr, which catalyzes the reduction of sulfite to sulfide, and oxidative (or reverse) Dsr, which converts elemental sulfur oxidation to sulfite [ 128 ]. Paired DsrAB proteins were first identified in all MAGs using in-house Perl scripts. In cases where Dsr subunits were duplicated, one set of paired DsrAB proteins was manually selected. A concatenated protein alignment was then generated for DsrAB proteins from the MAGs and reference sequences using MAFFT v.7.310 [ 129 ], and the alignment was trimmed using trimAl v.1.4.rev15 [ 130 ] with the parameter “-gt 0.25”. A phylogenetic tree was then constructed in IQ-TREE with settings “-m MFP -bb 1000 -redo -mset WAG,LG,JTT,Dayhoff -mrate E,I,G,I + G -mfreq FU -wbtl” (Fig. S 9 ). Reductive and oxidative DsrAB proteins were identified based on placement in the phylogenetic tree.
Predicted proteins for McrA were first identified using the TIGR03256 HMM. Presumed false gene calls were then manually removed, including those identified in bacterial MAGs and non-methanogenic/anaerobic methanotrophic archaeal MAGs with high sequence coverage. An alignment was constructed in MAFFT v.7.310 [ 129 ] using the remaining McrA protein sequences, together with reference genes recovered from methanogens, anaerobic methanotrophs, and short-chain alkane oxidizing Archaea from the Bathyarchaeia, Helarchaeales, Syntrophoarchaeum and Polytropus [ 11 , 12 , 131 , 132 ]. Alignment trimming and phylogenetic tree inference were performed as described above.
Sulfur dioxygenase (Sdo) proteins were predicted using the “sulfur_dioxygenase_sdo” HMM [ 109 ]. Alignment, trimming, and construction of the phylogeny were performed as described above. Positive Sdo calls were identified using two conserved amino acid residues (Asp196 and Asn244 of hETHE1, NCBI accession NP_055112) that are specific to Sdo in comparison with other metallo-β-lactamase superfamily members [ 133 ].
Statistical analysis
The relative abundance of MAGs in this study was calculated for each sample using normalized read coverage (set to 100 M reads) expressed as a percentage. Bray–Curtis similarity matrices were then generated from relative abundance data at various taxonomic ranks, and nonmetric multidimensional scaling (NMDS) plots were generated from the matrices using PRIMER v.6.1.13 [ 134 ].
Availability of data and materials
Metagenome reads are publicly available in the Sequence Read Archive (Table S 1 ), and MAGs generated in this study are available in NCBI Genbank (BioProject PRJNA821212, Table S 2 ).
Nakagawa S, Takai K, Inagaki F, Chiba H, Ishibashi JI, Kataoka S, et al. Variability in microbial community and venting chemistry in a sediment-hosted backarc hydrothermal system: impacts of subseafloor phase-separation. FEMS Microbiol Ecol. 2005;54:141–55.
Article CAS Google Scholar
Nunoura T, Takai K. Comparison of microbial communities associated with phase-separation- induced hydrothermal fluids at the Yonaguni Knoll IV hydrothermal field, the Southern Okinawa Trough. FEMS Microbiol Ecol. 2009;67:351–70.
Flores GE, Campbell JH, Kirshtein JD, Meneghin J, Podar M, Steinberg JI, et al. Microbial community structure of hydrothermal deposits from geochemically different vent fields along the Mid-Atlantic Ridge. Environ Microbiol. 2011;13:2158–71.
Flores GE, Shakya M, Meneghin J, Yang ZK, Seewald JS, Geoff Wheat C, et al. Inter-field variability in the microbial communities of hydrothermal vent deposits from a back-arc basin. Geobiology. 2012;10:333–46.
Dahle H, Økland I, Thorseth IH, Pederesen RB, Steen IH. Energy landscapes shape microbial communities in hydrothermal systems on the Arctic Mid-Ocean Ridge. ISME J. 2015;9:1593–606.
Dahle H, Le Moine BS, Baumberger T, Stokke R, Pedersen RB, Thorseth IH, et al. Energy landscapes in hydrothermal chimneys shape distributions of primary producers. Front Microbiol. 2018;9:1570.
Article Google Scholar
Fortunato CS, Larson B, Butterfield DA, Huber JA. Spatially distinct, temporally stable microbial populations mediate biogeochemical cycling at and below the seafloor in hydrothermal vent fluids. Environ Microbiol. 2018;20:769–84.
Reysenbach A-L, St. John E, Meneghin J, Flores GE, Podar M, Dombrowski N, et al. Complex subsurface hydrothermal fluid mixing at a submarine arc volcano supports distinct and highly diverse microbial communities. Proc Natl Acad Sci U S A. 2020;117:32627–38.
Reveillaud J, Reddington E, McDermott J, Algar C, Meyer JL, Sylva S, et al. Subseafloor microbial communities in hydrogen-rich vent fluids from hydrothermal systems along the Mid-Cayman Rise. Environ Microbiol. 2016;18:1970–87.
Anderson RE, Reveillaud J, Reddington E, Delmont TO, Eren AM, McDermott JM, et al. Genomic variation in microbial populations inhabiting the marine subseafloor at deep-sea hydrothermal vents. Nat Commun. 2017;8:1114.
Dombrowski N, Seitz KW, Teske AP, Baker BJ. Genomic insights into potential interdependencies in microbial hydrocarbon and nutrient cycling in hydrothermal sediments. Microbiome. 2017;5:106.
Dombrowski N, Teske AP, Baker BJ. Expansive microbial metabolic versatility and biodiversity in dynamic Guaymas Basin hydrothermal sediments. Nat Commun. 2018;9:4999.
Ramírez GA, McKay LJ, Fields MW, Buckley A, Mortera C, Hensen C, et al. The Guaymas Basin subseafloor sedimentary archaeome reflects complex environmental histories. iScience. 2020;23:101459.
Xie W, Wang F, Guo L, Chen Z, Sievert SM, Meng J, et al. Comparative metagenomics of microbial communities inhabiting deep-sea hydrothermal vent chimneys with contrasting chemistries. ISME J. 2011;5:414–26.
Hou J, Sievert SM, Wang Y, Seewald JS, Natarajan VP, Wang F, et al. Microbial succession during the transition from active to inactive stages of deep-sea hydrothermal vent sulfide chimneys. Microbiome. 2020;8:102.
Meier DV, Pjevac P, Bach W, Hourdez S, Girguis PR, Vidoudez C, et al. Niche partitioning of diverse sulfur-oxidizing bacteria at hydrothermal vents. ISME J. 2017;11:1545–58.
Chaumeil P-A, Mussig AJ, Hugenholtz P, Parks DH. GTDB-Tk: a toolkit to classify genomes with the Genome Taxonomy Database. Bioinformatics. 2020;36:1925–7.
CAS Google Scholar
Konstantinidis KT, Rosselló-Móra R, Amann R. Uncultivated microbes in need of their own taxonomy. ISME J. 2017;11:2399–406.
Von Damm KL, Edmond JM, Measures CI, Grant B. Chemistry of submarine hydrothermal solutions at Guaymas Basin, Gulf of California. Geochim Cosmochim Acta. 1985;49:2221–37.
Teske A, de Beer D, McKay LJ, Tivey MK, Biddle JF, Hoer D, et al. The Guaymas Basin hiking guide to hydrothermal mounds, chimneys, and microbial mats: complex seafloor expressions of subsurface hydrothermal circulation. Front Microbiol. 2016;7:75.
Burggraf S, Larsen N, Woese CR, Stetter KO. An intron within the 16S ribosomal RNA gene of the archaeon Pyrobaculum aerophilum . Proc Natl Acad Sci U S A. 1993;90:2547–50.
Nomura N, Morinaga Y, Kogishi T, Kim E-J, Sako Y, Uchida A. Heterogeneous yet similar introns reside in identical positions of the rRNA genes in natural isolates of the archaeon Aeropyrum pernix . Gene. 2002;295:43–50.
Jay ZJ, Inskeep WP. The distribution, diversity, and importance of 16S rRNA gene introns in the order Thermoproteales. Biol Direct. 2015;10:35.
St. John E, Liu Y, Podar M, Stott MB, Meneghin J, Chen Z, et al. A new symbiotic nanoarchaeote ( Candidatus Nanoclepta minutus) and its host ( Zestosphaera tikiterensis gen. nov., sp. Nov.) from a New Zealand hot spring. Syst Appl Microbiol. 2019;42:94–106.
Reysenbach A-L, Liu Y, Banta AB, Beveridge TJ, Kirshtein JD, Schouten S, et al. A ubiquitous thermoacidophilic archaeon from deep-sea hydrothermal vents. Nature. 2006;442:444–7.
Kozubal MA, Romine M, Jennings RD, Jay ZJ, Tringe SG, Rusch DB, et al. Geoarchaeota: a new candidate phylum in the Archaea from high-temperature acidic iron mats in Yellowstone National Park. ISME J. 2013;7:622–34.
McKay L, Klokman VW, Mendlovitz HP, Larowe DE, Hoer DR, Albert D, et al. Thermal and geochemical influences on microbial biogeography in the hydrothermal sediments of Guaymas Basin, Gulf of California. Environ Microbiol Rep. 2016;8:150–61.
Zhou Z, Liu Y, Xu W, Pan J, Luo Z-H, Li M. Genome- and community-level interaction insights into carbon utilization and element cycling functions of Hydrothermarchaeota in hydrothermal sediment. mSystems. 2020;5:e00795-19.
Buessecker S, Palmer M, Lai D, Dimapilis J, Mayali X, Mosier D, et al. An essential role for tungsten in the ecology and evolution of a previously uncultivated lineage of anaerobic, thermophilic Archaea. Nat Commun. 2022;13:3773.
Hug LA, Baker BJ, Anantharaman K, Brown CT, Probst AJ, Castelle CJ, et al. A new view of the tree of life. Nat Microbiol. 2016;1:16048.
Parks DH, Chuvochina M, Waite DW, Rinke C, Skarshewski A, Chaumeil P-A, et al. A standardized bacterial taxonomy based on genome phylogeny substantially revises the tree of life. Nat Biotechnol. 2018;36:996–1004.
Luef B, Frischkorn KR, Wrighton KC, Holman H-YN, Birarda G, Thomas BC, et al. Diverse uncultivated ultra-small bacterial cells in groundwater. Nat Commun. 2015;6:6372.
Kantor RS, Wrighton KC, Handley KM, Sharon I, Hug LA, Castelle CJ, et al. Small genomes and sparse metabolisms of sediment-associated bacteria from four candidate phyla. MBio. 2013;4:e00708-e713.
Castelle CJ, Brown CT, Anantharaman K, Probst AJ, Huang RH, Banfield JF. Biosynthetic capacity, metabolic variety and unusual biology in the CPR and DPANN radiations. Nat Rev Microbiol. 2018;16:629–45.
Tian R, Ning D, He Z, Zhang P, Spencer SJ, Gao S, et al. Small and mighty: adaptation of superphylum Patescibacteria to groundwater environment drives their genome simplicity. Microbiome. 2020;8:51.
Lemos LN, Manoharan L, Mendes LW, Venturini AM, Pylro VS, Tsai SM. Metagenome assembled-genomes reveal similar functional profiles of CPR/Patescibacteria phyla in soils. Environ Microbiol Rep. 2020;12:651–5.
Anantharaman K, Brown CT, Hug LA, Sharon I, Castelle CJ, Probst AJ, et al. Thousands of microbial genomes shed light on interconnected biogeochemical processes in an aquifer system. Nat Commun. 2016;7:13219.
McLean JS, Bor B, Kerns KA, Liu Q, To TT, Solden L, et al. Acquisition and adaptation of ultra-small parasitic reduced genome Bacteria to mammalian hosts. Cell Rep. 2020;32:107939.
Cross KL, Campbell JH, Balachandran M, Campbell AG, Cooper SJ, Griffen A, et al. Targeted isolation and cultivation of uncultivated bacteria by reverse genomics. Nat Biotechnol. 2019;37:1314–21.
He X, McLean JS, Edlund A, Yooseph S, Hall AP, Liu S-Y, et al. Cultivation of a human-associated TM7 phylotype reveals a reduced genome and epibiotic parasitic lifestyle. Proc Natl Acad Sci U S A. 2015;112:244–9.
Rinke C, Schwientek P, Sczyrba A, Ivanova NN, Anderson IJ, Cheng J-F, et al. Insights into the phylogeny and coding potential of microbial dark matter. Nature. 2013;499:431–7.
Anantharaman K, Breier JA, Dick GJ. Metagenomic resolution of microbial functions in deep-sea hydrothermal plumes across the Eastern Lau Spreading Center. ISME J. 2016;10:225–39.
Beam JP, Becraft ED, Brown JM, Schulz F, Jarett JK, Bezuidt O, et al. Ancestral absence of electron transport chains in Patescibacteria and DPANN. Front Microbiol. 2020;11:1848.
Kato S, Nakawake M, Kita J, Yamanaka T, Utsumi M, Okamura K, et al. Characteristics of microbial communities in crustal fluids in a deep-sea hydrothermal field of the Suiyo Seamount. Front Microbiol. 2013;4:85.
Oulas A, Polymenakou PN, Seshadri R, Tripp HJ, Mandalakis M, Paez-Espino AD, et al. Metagenomic investigation of the geologically unique Hellenic Volcanic Arc reveals a distinctive ecosystem with unexpected physiology. Environ Microbiol. 2016;18:1122–36.
Sieber CMK, Paul BG, Castelle CJ, Hu P, Tringe SG, Valentine DL, et al. Unusual metabolism and hypervariation in the genome of a gracilibacterium (BD1-5) from an oil-degrading community. MBio. 2019;10:e02128-e2219.
Moreira D, Zivanovic Y, López-Archilla AI, Iniesto M, López-García P. Reductive evolution and unique predatory mode in the CPR bacterium Vampirococcus lugosii . Nat Commun. 2021;12:2454.
Yakimov MM, Merkel AY, Gaisin VA, Pilhofer M, Messina E, Hallsworth JE, et al. Cultivation of a vampire: ‘ Candidatus Absconditicoccus praedator’. Environ Microbiol. 2022;24:30–49.
Coutinho FH, von Meijenfeldt FAB, Walter JM, Haro-Moreno JM, Lopéz-Pérez M, van Verk MC, et al. Ecogenomics and metabolic potential of the South Atlantic Ocean microbiome. Sci Total Environ. 2021;765:142758.
Hug LA, Castelle CJ, Wrighton KC, Thomas BC, Sharon I, Frischkorn KR, et al. Community genomic analyses constrain the distribution of metabolic traits across the Chloroflexi phylum and indicate roles in sediment carbon cycling. Microbiome. 2013;1:22.
Fincker M, Huber JA, Orphan VJ, Rappé MS, Teske A, Spormann AM. Metabolic strategies of marine subseafloor Chloroflexi inferred from genome reconstructions. Environ Microbiol. 2020;22:3188–204.
Fullerton H, Moyer CL. Comparative single-cell genomics of Chloroflexi from the Okinawa Trough deep-subsurface biosphere. Appl Environ Microbiol. 2016;82:3000–8.
Nuppunen-Puputti M, Kietäväinen R, Raulio M, Soro A, Purkamo L, Kukkonen I, et al. Epilithic microbial community functionality in deep oligotrophic continental bedrock. Front Microbiol. 2022;13:826048.
West-Roberts JA, Matheus-Carnevali PB, Schoelmerich MC, Al-Shayeb B, Thomas AD, Sharrar A, et al. The Chloroflexi supergroup is metabolically diverse and representatives have novel genes for non-photosynthesis based CO 2 fixation. BioRxiv. https://doi.org/10.1101/2021.08.23.457424 .
Liu R, Wei X, Song W, Wang L, Cao J, Wu J, et al. Novel Chloroflexi genomes from the deepest ocean reveal metabolic strategies for the adaptation to deep-sea habitats. Microbiome. 2022;10:75.
McGonigle JM, Lang SQ, Brazelton WJ. Genomic evidence for formate metabolism by Chloroflexi as the key to unlocking deep carbon in Lost City microbial ecosystems. Appl Environ Microbiol. 2020;86:e02583-e2619.
Islam ZF, Cordero PRF, Feng J, Chen Y-J, Bay SK, Jirapanjawat T, et al. Two Chloroflexi classes independently evolved the ability to persist on atmospheric hydrogen and carbon monoxide. ISME J. 2019;13:1801–13.
Altshuler I, Raymond-Bouchard I, Magnuson E, Tremblay J, Greer CW, Whyte LG. Unique high Arctic methane metabolizing community revealed through in situ 13 CH 4- DNA-SIP enrichment in concert with genome binning. Sci Rep. 2022;12:1160.
Madigan MT, Brock TD. Photosynthetic sulfide oxidation by Chloroflexus aurantiacus , a filamentous, photosynthetic, gliding bacterium. J Bacteriol. 1975;122:782–4.
Kawai S, Martinez JN, Lichtenberg M, Trampe E, Kühl M, Tank M, et al. In-situ metatranscriptomic analyses reveal the metabolic flexibility of the thermophilic anoxygenic photosynthetic bacterium Chloroflexus aggregans in a hot spring Cyanobacteria-dominated microbial mat. Microorganisms. 2021;9:652.
Spieck E, Spohn M, Wendt K, Bock E, Shively J, Frank J, et al. Extremophilic nitrite-oxidizing Chloroflexi from Yellowstone hot springs. ISME J. 2020;14:364–79.
Baker BJ, Lesniewski RA, Dick GJ. Genome-enabled transcriptomics reveals archaeal populations that drive nitrification in a deep-sea hydrothermal plume. ISME J. 2012;6:2269–79.
Engelen B, Nguyen T, Heyerhoff B, Kalenborn S, Sydow K, Tabai H, et al. Microbial communities of hydrothermal Guaymas Basin surficial sediment profiled at 2 millimeter-scale resolution. Front Microbiol. 2021;12:710881.
Beman JM, Popp BN, Francis CA. Molecular and biogeochemical evidence for ammonia oxidation by marine Crenarchaeota in the Gulf of California. ISME J. 2008;2:429–41.
Speth DR, Yu FB, Connon SA, Lim S, Magyar JS, Peña-Salinas ME, et al. Microbial communities of Auka hydrothermal sediments shed light on vent biogeography and the evolutionary history of thermophily. ISME J. 2022;16:1750–64.
Mehta MP, Baross JA. Nitrogen fixation at 92°C by a hydrothermal vent archaeon. Science. 2006;314:1783–6.
Dick GJ. The microbiomes of deep-sea hydrothermal vents: distributed globally, shaped locally. Nat Rev Microbiol. 2019;17:271–83.
Frank KL, Rogers DR, Olins HC, Vidoudez C, Girguis PR. Characterizing the distribution and rates of microbial sulfate reduction at Middle Valley hydrothermal vents. ISME J. 2013;7:1391–401.
Zhou Z, Tran PQ, Adams AM, Kieft K, Breier JA, Sinha RK, et al. The sulfur cycle connects microbiomes and biogeochemistry in deep-sea hydrothermal plumes. BioRxiv. https://doi.org/10.1101/2022.06.02.494589 .
Von Damm KL, Parker CM, Zierenberg RA, Lilley MD, Olson EJ, Clague DA, et al. The Escanaba Trough, Gorda Ridge hydrothermal system: temporal stability and subseafloor complexity. Geochim Cosmochim Acta. 2005;69:4971–84.
Dhillon A, Lever M, Lloyd KG, Albert DB, Sogin ML, Teske A. Methanogen diversity evidenced by molecular characterization of methyl coenzyme M reductase A ( mcrA ) genes in hydrothermal sediments of the Guaymas Basin. Appl Environ Microbiol. 2005;71:4592–601.
Adam N, Perner M. Microbially mediated hydrogen cycling in deep-sea hydrothermal vents. Front Microbiol. 2018;9:2873.
Lever MA, Teske AP. Diversity of methane-cycling Archaea in hydrothermal sediment investigated by general and group-specific PCR primers. Appl Environ Microbiol. 2015;81:1426–41.
Teske A, Wegener G, Chanton JP, White D, MacGregor B, Hoer D, et al. Microbial communities under distinct thermal and geochemical regimes in axial and off-axis sediments of Guaymas Basin. Front Microbiol. 2021;12:633649.
Søndergaard D, Pedersen CNS, Greening C. HydDB: a web tool for hydrogenase classification and analysis. Sci Rep. 2016;6:34212.
Kodama Y, Watanabe K. Sulfuricurvum kujiense gen. nov., sp. nov., a facultatively anaerobic, chemolithoautotrophic, sulfur-oxidizing bacterium isolated from an underground crude-oil storage cavity. Int J Syst Evol Microbiol. 2004;54:2297–300.
Takai K, Inagaki F, Nakagawa S, Hirayama H, Nunoura T, Sako Y, et al. Isolation and phylogenetic diversity of members of previously uncultivated ε-Proteobacteria in deep-sea hydrothermal fields. FEMS Microbiol Lett. 2003;218:167–74.
Takai K, Suzuki M, Nakagawa S, Miyazaki M, Suzuki Y, Inagaki F, et al. Sulfurimonas paralvinellae sp. nov., a novel mesophilic, hydrogen- and sulfur-oxidizing chemolithoautotroph within the Epsilonproteobacteria isolated from a deep-sea hydrothermal vent polychaete nest, reclassification of Thiomicrospira denitrificans as Sulfurimonas denitrificans comb. nov. and emended description of the genus Sulfurimonas . Int J Syst Evol Microbiol. 2006;56:1725–33.
Caldwell SL, Liu Y, Ferrera I, Beveridge T, Reysenbach A-L. Thermocrinis minervae sp. nov., a hydrogen- and sulfur-oxidizing, thermophilic member of the Aquificales from a Costa Rican terrestrial hot spring. Int J Syst Evol Microbiol. 2010;60:338–43.
Götz D, Banta A, Beveridge TJ, Rushdi AI, Simoneit BRT, Reysenbach A-L. Persephonella marina gen. nov., sp. nov. and Persephonella guaymasensis sp. nov., two novel, thermophilic, hydrogen-oxidizing microaerophiles from deep-sea hydrothermal vents. Int J Syst Evol Microbiol. 2002;52:1349–59.
Google Scholar
Topçuoglu BD, Stewart LC, Morrison HG, Butterfield DA, Huber JA, Holden JF. Hydrogen limitation and syntrophic growth among natural assemblages of thermophilic methanogens at deep-sea hydrothermal vents. Front Microbiol. 2016;7:1240.
Webster NS. Cooperation, communication, and co-evolution: grand challenges in microbial symbiosis research. Front Microbiol. 2014;5:164.
Petersen JM, Zielinski FU, Pape T, Seifert R, Moraru C, Amann R, et al. Hydrogen is an energy source for hydrothermal vent symbioses. Nature. 2011;476:176–80.
Galambos D, Anderson RE, Reveillaud J, Huber JA. Genome-resolved metagenomics and metatranscriptomics reveal niche differentiation in functionally redundant microbial communities at deep-sea hydrothermal vents. Environ Microbiol. 2019;21:4395–410.
Louca S, Parfrey LW, Doebeli M. Decoupling function and taxonomy in the global ocean microbiome. Science. 2016;353:1272–7.
Yamamoto M, Takai K. Sulfur metabolisms in Epsilon- and Gamma-proteobacteria in deep-sea hydrothermal fields. Front Microbiol. 2011;2:192.
Giovannelli D, Chung M, Staley J, Starovoytov V, Le Bris N, Vetriani C. Sulfurovum riftiae sp. nov., a mesophilic, thiosulfate-oxidizing, nitrate-reducing chemolithoautotrophic epsilonproteobacterium isolated from the tube of the deep-sea hydrothermal vent polychaete Riftia pachyptila . Int J Syst Evol Microbiol. 2016;66:2697–701.
Mori K, Yamaguchi K, Hanada S. Sulfurovum denitrificans sp. nov., an obligately chemolithoautotrophic sulfur-oxidizing epsilonproteobacterium isolated from a hydrothermal field. Int J Syst Evol Microbiol. 2018;68:2183–7.
Nakagawa S, Takai K, Inagaki F, Horikoshi K, Sako Y. Nitratiruptor tergarcus gen. nov., sp. nov. and Nitratifractor salsuginis gen. nov., sp. nov., nitrate-reducing chemolithoautotrophs of the ε-Proteobacteria isolated from a deep-sea hydrothermal system in the Mid-Okinawa Trough. Int J Syst Evol Microbiol. 2005;55:925–33.
Assié A, Leisch N, Meier DV, Gruber-Vodicka H, Tegetmeyer HE, Meyerdierks A, et al. Horizontal acquisition of a patchwork Calvin cycle by symbiotic and free-living Campylobacterota (formerly Epsilonproteobacteria). ISME J. 2020;14:104–22.
Berg IA. Ecological aspects of the distribution of different autotrophic CO 2 fixation pathways. Appl Environ Microbiol. 2011;77:1925–36.
Markert S, Arndt C, Felbeck H, Becher D, Sievert SM, Hügler M, et al. Physiological proteomics of the uncultured endosymbiont of Riftia pachyptila . Science. 2007;315:247–50.
Waite DW, Vanwonterghem I, Rinke C, Parks DH, Zhang Y, Takai K, et al. Comparative genomic analysis of the class Epsilonproteobacteria and proposed reclassification to Epsilonbacteraeota (phyl. nov.). Front Microbiol. 2017;8:682.
Macalady JL, Dattagupta S, Schaperdoth I, Jones DS, Druschel GK, Eastman D. Niche differentiation among sulfur-oxidizing bacterial populations in cave waters. ISME J. 2008;2:590–601.
Patwardhan S, Foustoukos DI, Giovannelli D, Yücel M, Vetriani C. Ecological succession of sulfur-oxidizing Epsilon- and Gammaproteobacteria during colonization of a shallow-water gas vent. Front Microbiol. 2018;9:2970.
Flores GE, Wagner ID, Liu Y, Reysenbach A-L. Distribution, abundance, and diversity patterns of the thermoacidophilic “deep-sea hydrothermal vent Euryarchaeota 2.” Front Microbiol. 2012;3:47.
Nurk S, Meleshko D, Korobeynikov A, Pevzner PA. MetaSPAdes: a new versatile metagenomic assembler. Genome Res. 2017;27:824–34.
Uritskiy GV, DiRuggiero J, Taylor J. MetaWRAP – a flexible pipeline for genome-resolved metagenomic data analysis. Microbiome. 2018;6:158.
Sieber CMK, Probst AJ, Sharrar A, Thomas BC, Hess M, Tringe SG, et al. Recovery of genomes from metagenomes via a dereplication, aggregation and scoring strategy. Nat Microbiol. 2018;3:836–43.
Parks DH, Imelfort M, Skennerton CT, Hugenholtz P, Tyson GW. CheckM: assessing the quality of microbial genomes recovered from isolates, single cells, and metagenomes. Genome Res. 2015;25:1043–55.
Parks DH, Rinke C, Chuvochina M, Chaumeil P-A, Woodcroft BJ, Evans PN, et al. Recovery of nearly 8,000 metagenome-assembled genomes substantially expands the tree of life. Nat Microbiol. 2017;2:1533–42.
Pruesse E, Peplies J, Glöckner FO. SINA: accurate high-throughput multiple sequence alignment of ribosomal RNA genes. Bioinformatics. 2012;28:1823–9.
Laczny CC, Sternal T, Plugaru V, Gawron P, Atashpendar A, Margossian HH, et al. VizBin - an application for reference-independent visualization and human-augmented binning of metagenomic data. Microbiome. 2015;3:1.
Eren AM, Kiefl E, Shaiber A, Veseli I, Miller SE, Schechter MS, et al. Community-led, integrated, reproducible multi-omics with Anvi’o. Nat Microbiol. 2021;6:3–6.
von Meijenfeldt FAB, Arkhipova K, Cambuy DD, Coutinho FH, Dutilh BE. Robust taxonomic classification of uncharted microbial sequences and bins with CAT and BAT. Genome Biol. 2019;20:217.
Nayfach S, Roux S, Seshadri R, Udwary D, Varghese N, Schulz F, et al. A genomic catalog of Earth’s microbiomes. Nat Biotechnol. 2021;39:499–509.
Hyatt D, Chen G-L, LoCascio PF, Land ML, Larimer FW, Hauser LJ. Prodigal: prokaryotic gene recognition and translation initiation site identification. BMC Bioinformatics. 2010;11:119.
Aramaki T, Blanc-Mathieu R, Endo H, Ohkubo K, Kanehisa M, Goto S, et al. KofamKOALA: KEGG ortholog assignment based on profile HMM and adaptive score threshold. Bioinformatics. 2020;36:2251–2.
Zhou Z, Tran PQ, Breister AM, Liu Y, Kieft K, Cowley ES, et al. METABOLIC: high-throughput profiling of microbial genomes for functional traits, metabolism, biogeochemistry, and community-scale functional networks. Microbiome. 2022;10:33.
Huerta-Cepas J, Szklarczyk D, Heller D, Hernández-Plaza A, Forslund SK, Cook H, et al. EggNOG 5.0: a hierarchical, functionally and phylogenetically annotated orthology resource based on 5090 organisms and 2502 viruses. Nucleic Acids Res. 2019;47:D309-14.
Chan PP, Lowe TM. tRNAscan-SE: searching for tRNA genes in genomic sequences. Methods Mol Biol. 2019;1962:1–14.
Campbell JH, O’Donoghue P, Campbell AG, Schwientek P, Sczyrba A, Woyke T, et al. UGA is an additional glycine codon in uncultured SR1 Bacteria from the human microbiota. Proc Natl Acad Sci U S A. 2013;110:5540–5.
Hanke A, Hamann E, Sharma R, Geelhoed JS, Hargesheimer T, Kraft B, et al. Recoding of the stop codon UGA to glycine by a BD1-5/SN-2 bacterium and niche partitioning between Alpha- and Gammaproteobacteria in a tidal sediment microbial community naturally selected in a laboratory chemostat. Front Microbiol. 2014;5:231.
Seemann T. Prokka: rapid prokaryotic genome annotation. Bioinformatics. 2014;30:2068–9.
Tully BJ, Wheat CG, Glazer BT, Huber JA. A dynamic microbial community with high functional redundancy inhabits the cold, oxic subseafloor aquifer. ISME J. 2018;12:1–16.
Kanehisa M, Sato Y, Morishima K. BlastKOALA and GhostKOALA: KEGG tools for functional characterization of genome and metagenome sequences. J Mol Biol. 2016;428:726–31.
Nguyen L-T, Schmidt HA, von Haeseler A, Minh BQ. IQ-TREE: a fast and effective stochastic algorithm for estimating maximum-likelihood phylogenies. Mol Biol Evol. 2015;32:268–74.
Price MN, Dehal PS, Arkin AP. FastTree 2 – approximately maximum-likelihood trees for large alignments. PLoS ONE. 2010;5:e9490.
Minh BQ, Schmidt HA, Chernomor O, Schrempf D, Woodhams MD, von Haeseler A, et al. IQ-TREE 2: new models and efficient methods for phylogenetic inference in the genomic era. Mol Biol Evol. 2020;37:1530–4.
Letunic I, Bork P. Interactive tree of life (iTOL) v5: an online tool for phylogenetic tree display and annotation. Nucleic Acids Res. 2021;49:W293–6.
Rawlings ND, Barrett AJ, Thomas PD, Huang X, Bateman A, Finn RD. The MEROPS database of proteolytic enzymes, their substrates and inhibitors in 2017 and a comparison with peptidases in the PANTHER database. Nucleic Acids Res. 2018;46:D624–32.
Zhang H, Yohe T, Huang L, Entwistle S, Wu P, Yang Z, et al. DbCAN2: a meta server for automated carbohydrate-active enzyme annotation. Nucleic Acids Res. 2018;46:W95-101.
Drula E, Garron M-L, Dogan S, Lombard V, Henrissat B, Terrapon N. The carbohydrate-active enzyme database: functions and literature. Nucleic Acids Res. 2022;50:D571–7.
Yu NY, Wagner JR, Laird MR, Melli G, Rey S, Lo R, et al. PSORTb 3.0: improved protein subcellular localization prediction with refined localization subcategories and predictive capabilities for all prokaryotes. Bioinformatics. 2010;26:1608–15.
Shaffer M, Borton MA, McGivern BB, Zayed AA, La Rosa SL, Solden LM, et al. DRAM for distilling microbial metabolism to automate the curation of microbiome function. Nucleic Acids Res. 2020;48:8883–900.
Pérez Castro S, Borton MA, Regan K, Hrabe de Angelis I, Wrighton KC, Teske AP, et al. Degradation of biological macromolecules supports uncultured microbial populations in Guaymas Basin hydrothermal sediments. ISME J. 2021;15:3480–97.
Garber AI, Nealson KH, Okamoto A, McAllister SM, Chan CS, Barco RA, et al. FeGenie: a comprehensive tool for the identification of iron genes and iron gene neighborhoods in genome and metagenome assemblies. Front Microbiol. 2020;11:37.
Anantharaman K, Hausmann B, Jungbluth SP, Kantor RS, Lavy A, Warren LA, et al. Expanded diversity of microbial groups that shape the dissimilatory sulfur cycle. ISME J. 2018;12:1715–28.
Katoh K, Standley DM. MAFFT multiple sequence alignment software version 7: improvements in performance and usability. Mol Biol Evol. 2013;30:772–80.
Capella-Gutiérrez S, Silla-Martínez JM, Gabaldón T. trimAl: a tool for automated alignment trimming in large-scale phylogenetic analyses. Bioinformatics. 2009;25:1972–3.
Seitz KW, Dombrowski N, Eme L, Spang A, Lombard J, Sieber JR, et al. Asgard Archaea capable of anaerobic hydrocarbon cycling. Nat Commun. 2019;10:1822.
Boyd JA, Jungbluth SP, Leu AO, Evans PN, Woodcroft BJ, Chadwick GL, et al. Divergent methyl-coenzyme M reductase genes in a deep-subseafloor Archaeoglobi. ISME J. 2019;13:1269–79.
Liu H, Xin Y, Xun L. Distribution, diversity, and activities of sulfur dioxygenases in heterotrophic bacteria. Appl Environ Microbiol. 2014;80:1799–806.
Clarke KR, Gorley RN. Primer V6: user manual - tutorial. Plymouth: Plymouth Marine Laboratory; 2006.
Download references
Acknowledgements
We thank the crew of the R/V Roger Revelle , R/V Atlantis , R/V Thomas G. Thompson , HOV Alvin , and the ROV Jason for assistance in collecting the samples. Many thanks to the many students who over the years helped extract the DNA, to Nicole Wagner and Jennifer Meneghin for initial bioinformatic analysis assistance, and to MK Tivey for thoughtful comments on the manuscript.
This work was funded by the US-National Science Foundation grants OCE-0728391, OCE-0937404, OCE-1558795 to A-L.R, and OCE-2049478 and DBI-2047598 to K.A. We thank the Department of Energy Joint Genome Institute (Community Science Program award 339, lead Peter Girguis) for sequencing several of the samples.
Author information
Zhichao Zhou and Emily St. John contributed equally to this work.
Authors and Affiliations
Department of Bacteriology, University of Wisconsin-Madison, Madison, WI, 53706, USA
Zhichao Zhou & Karthik Anantharaman
Center for Life in Extreme Environments, Biology Department, Portland State University, Portland, OR, 97201, USA
Emily St. John & Anna-Louise Reysenbach
You can also search for this author in PubMed Google Scholar
Contributions
A-L.R conceived of the study, collected and processed the samples, and wrote the manuscript; Z.Z. and E.S.J. did the bioinformatic processing and data analysis and generated the figures and tables; K.A. assisted in project conception and data analysis; and all authors read, reviewed, edited, and approved the final manuscript.
Corresponding authors
Correspondence to Karthik Anantharaman or Anna-Louise Reysenbach .
Ethics declarations
Ethics approval and consent to participate.
Not applicable.
Consent for publication
Competing interests.
The authors declare that they have no competing interests.
Additional information
Publisher’s note.
Springer Nature remains neutral with regard to jurisdictional claims in published maps and institutional affiliations.
Supplementary Information
Additional file 1: fig. s1..
Geographic distribution of deep-sea hydrothermal vent sampling locations. The number of samples collected in each region is shown with n values. Fig. S2. Deep-sea hydrothermal vent photographs from ELSC, EPR, MAR and Guaymas Basin. Fig. S3. Comparison between the number of medium- to high-quality MAGs recovered in each metagenomic assembly and the number of reads that passed quality control measures. Metagenomic assemblies are ordered by (A) increasing MAG count and (B) increasing read count. Fig. S4. NMDS plot showing the taxonomic diversity of Brothers volcano MAGs, based on normalized relative abundance. Clustering patterns show a high degree of similarity to NMDS plot clustering previously reported in Reysenbach et al., 2020. Fig. S5. Anvi’o plot showing the cluster of scaffolds (blue) predominantly corresponding to the Nanoarchaeota in M10_maxbin2_scaf2bin.065. Analysis with CAT revealed three additional contaminating scaffolds which were removed, bringing the final scaffold count to 149, with an estimated 47% completion by CheckM. Scaffold clusters that were removed (pink; 972 scaffolds) were largely assigned to taxonomic groups outside the Nanoarchaeota by CAT and had a low number of marker genes, as estimated by CheckM (6.99% completion, 0.29% contamination). Fig. S6. Predicted cell metabolism diagrams for the putative new phyla (A) JALSQH01 (3 MAGs) and (B) JALWCF01 (13 MAGs). Functions (F) and modules (M) were identified using METABOLIC (Table S5). Solid lines indicate the presence of a module or function, while dashed lines and a “p” in parentheses indicate that a module or function was only present sporadically (<50% of MAGs). Modules and functions not identified in any MAGs are shown with dashed lines and gray labels. Fig. S7. Normalized relative abundance of GTDB classes, expressed as a percentage. Classes depicted comprise ≥16% of the relative MAG abundance in at least one assembly. Fig. S8. Maximum-likelihood GTDB-Tk concatenated protein tree showing members of the Patescibacteria, used to generate Fig. 5A. Lineages outside the Patescibacteria are shown as a collapsed triangle, and MAGs from this study are indicated in bold type. Filled circles represent SH-like branch support (0.8-1.0), and the scale bar shows 0.5 substitutions per amino acid. Fig. S9. Concatenated dissimilatory sulfite reductase (DsrAB) protein phylogenetic tree. Only the nodes with ultrafast bootstrap (UFBoot) support values over 90% were labeled with black dots. This tree included both reductive DsrAB (for reductive dissimilatory sulfite reduction to sulfide) and oxidative DsrAB (for dissimilatory sulfur oxidation to sulfite). For collapsed clades in the oxidative DsrAB clade (labeled in blue), the DsrAB call numbers and DsrAB-containing MAG numbers were labeled in square brackets. The total number for both reductive DsrAB calls and reductive DsrAB-containing MAG numbers and oxidative DsrAB calls and oxidative DsrAB-containing MAG numbers were labeled accordingly on the side of the tree. Note that one genome can have multiple paired DsrAB calls. Fig. S10. Phylogenetic protein tree of methyl coenzyme M reductase subunit alpha (McrA). Ultrafast bootstrap support values (>90%) are shown with filled circles. Clades comprised of predicted butane oxidation (Butane clade), X-alkane oxidation (X-alkane clade) and anaerobic methanotrophy-associated (ANME-1 and -2) McrA amino acid sequences are highlighted, and the three predicted McrA sequences from the Archaeoglobi are shown in red. Fig. S11. Sdo (sulfur dioxygenase) phylogenetic protein tree. Only the nodes with ultrafast bootstrap (UFBoot) support values over 90% were labeled with black dots. The positive Sdo sequences that were checked by two conservative amino acid residues were labeled yellow in the tree. Three positive Sdo clades (including ETHE1, Sdo, and Blh) were labeled yellow; the numbers of positive Sdo sequences, non-Sdo sequences, and Sdo reference sequences were labeled accordingly. Other unannotated clades and non-Sdo clades (including metallo-beta-lactamase, GloB1, and GloB2) all contained non-Sdo sequences. Fig. S12. Relative abundance of GTDB-assigned MAG taxa at Guaymas Basin. Abundances are shown (A) for all taxa at the genus level, and (B) for the Archaea at the order level, using read coverage normalized to 100M reads per sample and expressed as a percentage of MAG reads per sample. Relative abundances were averaged for the two samples from the six-day thermocouple array (4561-380 and 4561-384).
Additional file 2: Table S1.
Sample metadata including location, year, research vessel, number of metagenome reads and accession numbers. Table S2. MAG genome properties, accession numbers and taxonomic classifications. Taxonomy was assigned using GTDB-Tk, and mis-classified MAGs were taxonomically re-assigned at the phylum level (Bacteria) and class level (Archaea) using curated archaeal and bacterial phylogenetic trees. Genome quality statistics are based on completion and contamination (high quality, >90% completion, <5% contamination; medium quality, ≥50% completion, ≤10% contamination). Average contamination was 4.02%. Table S3. Average amino acid identity (AAI) matrices for the (A) Bacteria and (B) Archaea. Matrices are grouped by GTDB taxonomy and include MAGs that could not be assigned to a known genus by GTDB-Tk. Details are provided which recently identified MAGs were from Brothers volcano hydrothermal deposits. Table S4. Relative abundance of GTDB taxa by site, based on read coverage of MAGs normalized to 100M reads per sample. MAG coverage for each site was summed and expressed as a percent. Table S5. METABOLIC-G results for JALSQH01 (3 MAGs) and JALWCF01 (13 MAGs). In the summary rows for JALSQH01 and JALWCF01, functions and modules are listed as “present” if identified in ≥50% of all MAGs, “partially present” if found in <50% of the MAGs, and “absent” if undetected in the MAGs. Table S6. Selected functional genes found in Patescibacteria MAGs, based on annotation with GhostKOALA. KEGG module numbers are shown in parentheses. Table S7. Functional genes identified in selected > 80%-completeness MAGs from the Chloroflexota. (A) Genes are marked as present (1; green highlight) or not detected (0) in individual MAGs. (B) The proportion of > 80%-completeness MAGs in six GTDB orders that encode functional genes is also shown, with proportions ≥50% highlighted in green. Table S8. Identification and distribution of functional genes in this study. (A) The HMMs, MEROPS peptidases, and CAZymes used to identify functional genes. Gene call numbers were calculated using the component (C) or accumulative (A) methods described in the methods. Genes requiring manual validation (M) are indicated. (B) Functional gene abundance, calculated as described in the methods. Table S9. Percentage of MAGs in phylogenetic clusters that encode core metabolic genes. Unless otherwise indicated, Archaea are shown at the class level, and Bacteria are shown at the phylum level. Genes were detected using METABOLIC, with additional validation steps for oxidative and reductive Dsr, Sdo, PmoA and McrA. Table S10. Comparative (A) relative abundance and (B) functional gene abundance for the Gammaproteobacteria and Campylobacteria, used to generate Fig. 10.
Additional file 3: Data S1.
Newick format archaeal concatenated protein phylogenetic tree, including both MAGs and GTDB reference genomes.
Additional file 4: DataS2.
Newick file ofbacterial concatenated protein phylogenetic tree including MAGs and GTDBreference genomes, generated using IQ-TREE.
Additional file 5: Data S3.
Concatenated protein phylogenetic tree of bacterial MAGs and GTDB reference genomes, generated with FastTree (Newick format).
Additional file 6:Data S4.
MAG-only bacterialconcatenated phylogenetic protein tree in Newick format.
Additional file 7: Data S5.
Concatenated protein phylogeny of archaeal MAGs in Newick format.
Additional file 8.
Supplementary Discussion.
Rights and permissions
Open Access This article is licensed under a Creative Commons Attribution 4.0 International License, which permits use, sharing, adaptation, distribution and reproduction in any medium or format, as long as you give appropriate credit to the original author(s) and the source, provide a link to the Creative Commons licence, and indicate if changes were made. The images or other third party material in this article are included in the article's Creative Commons licence, unless indicated otherwise in a credit line to the material. If material is not included in the article's Creative Commons licence and your intended use is not permitted by statutory regulation or exceeds the permitted use, you will need to obtain permission directly from the copyright holder. To view a copy of this licence, visit http://creativecommons.org/licenses/by/4.0/ . The Creative Commons Public Domain Dedication waiver ( http://creativecommons.org/publicdomain/zero/1.0/ ) applies to the data made available in this article, unless otherwise stated in a credit line to the data.
Reprints and permissions
About this article
Cite this article.
Zhou, Z., St. John, E., Anantharaman, K. et al. Global patterns of diversity and metabolism of microbial communities in deep-sea hydrothermal vent deposits. Microbiome 10 , 241 (2022). https://doi.org/10.1186/s40168-022-01424-7
Download citation
Received : 05 August 2022
Accepted : 11 November 2022
Published : 27 December 2022
DOI : https://doi.org/10.1186/s40168-022-01424-7
Share this article
Anyone you share the following link with will be able to read this content:
Sorry, a shareable link is not currently available for this article.
Provided by the Springer Nature SharedIt content-sharing initiative
- Thermophiles
- Deep-sea hydrothermal vents
- Metagenomics
ISSN: 2049-2618
- General enquiries: [email protected]
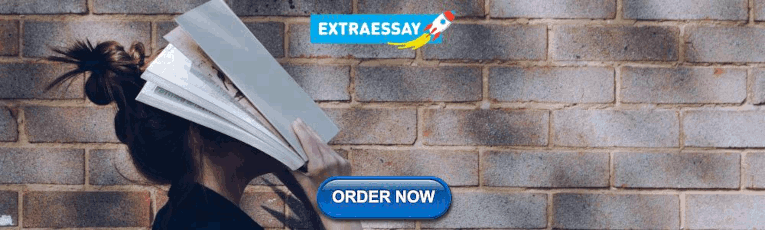
COMMENTS
The research paper introduction section, along with the Title and Abstract, can be considered the face of any research paper. The following article is intended to guide you in organizing and writing the research paper introduction for a quality academic article or dissertation. The research paper introduction aims to present the topic to the ...
Table of contents. Step 1: Introduce your topic. Step 2: Describe the background. Step 3: Establish your research problem. Step 4: Specify your objective (s) Step 5: Map out your paper. Research paper introduction examples. Frequently asked questions about the research paper introduction.
Research paper introduction is the first section of a research paper that provides an overview of the study, its purpose, and the research question(s) or hypothesis(es) being investigated. It typically includes background information about the topic, a review of previous research in the field, and a statement of the research objectives. The ...
The introduction leads the reader from a general subject area to a particular topic of inquiry. It establishes the scope, context, and significance of the research being conducted by summarizing current understanding and background information about the topic, stating the purpose of the work in the form of the research problem supported by a hypothesis or a set of questions, explaining briefly ...
The introduction section of a research paper provides background information about the research problem, the research question, and the research objectives. ... Research papers are typically written when a person has completed a research project or when they have conducted a study and have obtained data or findings that they want to share with ...
The introduction to a research paper presents your topic, provides background, and details your research problem. 3477. Writing a Research Paper Conclusion | Step-by-Step Guide The conclusion of a research paper restates the research problem, summarizes your arguments or findings, and discusses the implications. 863.
After you've done some extra polishing, I suggest a simple test for the introductory section. As an experiment, chop off the first few paragraphs. Let the paper begin on, say, paragraph 2 or even page 2. If you don't lose much, or actually gain in clarity and pace, then you've got a problem. There are two solutions.
When writing your research paper introduction, there are several key elements you should include to ensure it is comprehensive and informative. A hook or attention-grabbing statement to capture the reader's interest. It can be a thought-provoking question, a surprising statistic, or a compelling anecdote that relates to your research topic.
Overview of the structure. To help guide your reader, end your introduction with an outline of the structure of the thesis or dissertation to follow. Share a brief summary of each chapter, clearly showing how each contributes to your central aims. However, be careful to keep this overview concise: 1-2 sentences should be enough.
Step 2: Building a solid foundation with background information. Including background information in your introduction serves two major purposes: It helps to clarify the topic for the reader. It establishes the depth of your research. The approach you take when conveying this information depends on the type of paper.
The purpose of the introduction in a research paper is to guide the reader from a general subject to a specific area of study. It establishes the context of the research by summarizing current understanding, stating the purpose of the work, explaining the rationale and methodological approach, highlighting potential findings, and describing the paper's structure.
1. The placeholder introduction. When you don't have much to say on a given topic, it is easy to create this kind of introduction. Essentially, this kind of weaker introduction contains several sentences that are vague and don't really say much. They exist just to take up the "introduction space" in your paper.
Narrow the overview until you address your paper's specific subject. Then, mention questions or concerns you had about the case. Note that you will address them in the publication. Prior research. Your introduction is the place to review other conclusions on your topic. Include both older scholars and modern scholars.
A scientific paper should have an introduction in the form of an inverted pyramid. The writer should start with the general information about the topic and subsequently narrow it down to the specific topic-related introduction. Fig. 17.1. Flow of ideas from the general to the specific. Full size image.
The introduction serves the purpose of leading the reader from a general subject area to a particular field of research. It establishes the context of the research being conducted by summarizing current understanding and background information about the topic, stating the purpose of the work in the form of the hypothesis, question, or research problem, briefly explaining your rationale ...
The introduction to an academic essay will generally present an analytical question or problem and then offer an answer to that question (the thesis). ... When you're writing a paper based on your own research, you will need to provide more context about the sources you're going to discuss. If you're not sure how much you can assume your ...
Hannah, a writer and editor since 2017, specializes in clear and concise academic and business writing. She has mentored countless scholars and companies in writing authoritative and engaging content. A great research paper introduction starts with a catchy hook and ends with a road map for the research. At every step, QuillBot can help.
1: Introduction to the Research Process. 3. Report and Argument Research Papers. All research papers require an exploration of the work of other scholars on the chosen topic. The type of research paper you write will depend on your research question . and your treatment of the information that you gather. There are two basic types of research ...
The thesis is generally the narrowest part and last sentence of the introduction, and conveys your position, the essence of your argument or idea. See our handout on Writing a Thesis Statement for more. The roadmap Not all academic papers include a roadmap, but many do. Usually following the thesis, a roadmap is a
The key thing is. to guide the reader into your topic and situate your ideas. Step 2: Describe the background. This part of the introduction differs depending on what approach your paper is ...
The Federalist Papers were a series of essays written by Alexander Hamilton, James Madison, and John Jay under the pen name "Publius." ... Bibliographic and Research Instruction Librarian, Law Library of Congress. Created: May 3, 2019. Last Updated: August 13, 2019. Introduction The Federalist Papers were a series of eighty-five essays urging ...
Step 1: Hook your reader. Step 2: Give background information. Step 3: Present your thesis statement. Step 4: Map your essay's structure. Step 5: Check and revise. More examples of essay introductions. Other interesting articles. Frequently asked questions about the essay introduction.
The perception and appreciation of food flavor depends on many interacting chemical compounds and external factors, and therefore proves challenging to understand and predict. Here, we combine ...
Introduction. The global shipping industry is responsible for transporting as much as 90% of world trade (SSR 2021). Over the past decade, ... This paper suggests that research from the disciplines of human and social sciences, particularly organisation studies, can provide new and relevant insights by clarifying how ships can be described in ...
Feature papers represent the most advanced research with significant potential for high impact in the field. A Feature Paper should be a substantial original Article that involves several techniques or approaches, provides an outlook for future research directions and describes possible research applications. ... Introduction. In the context of ...
Background When deep-sea hydrothermal fluids mix with cold oxygenated fluids, minerals precipitate out of solution and form hydrothermal deposits. These actively venting deep-sea hydrothermal deposits support a rich diversity of thermophilic microorganisms which are involved in a range of carbon, sulfur, nitrogen, and hydrogen metabolisms. Global patterns of thermophilic microbial diversity in ...