- Online Students
- Faculty & Staff
- African American/Black
- Asian, Pacific Islander & Desi American
- DACA & Undocumented Students
- First Generation
- Hispanic/Latinx
- Indigenous/Native/First Nations
- International
- Justice Impacted
- Middle Eastern/North African/Arab-American
- Neurodivergent
- Students With Disabilities
- Liberal Arts
- Social Sciences
- Create a Resume / Cover Letter
- Earn Certifications & Badges
- Expand Your Network / Mentor
- Negotiate an Offer
- Prepare for an Interview
- Explore Your Interests / Self Assessment
- Prepare for Graduate School
- Explore Internships
- Search for a Job

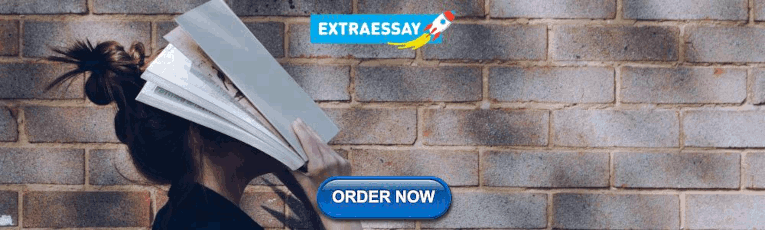
How to Research and Write Using Generative AI Tools
- Share This: Share How to Research and Write Using Generative AI Tools on Facebook Share How to Research and Write Using Generative AI Tools on LinkedIn Share How to Research and Write Using Generative AI Tools on X
Instructor: Dave Birss
You’ve probably already heard about ChatGPT, but did you know it can make you better at your job? Join instructor Dave Birss for a crash course in generative AI and learn how to get started with prompt engineering for ChatGPT and other AI chatbots to upskill as a researcher and a writer.
Dave shows you how to create effective prompts that deliver high-quality, task-relevant results. Get an overview of some of the key considerations of working with generative AI with hands-on, practical strategies to improve your research and writing. Find out how to summarize complex information, view subjects from multiple perspectives, build user personas and strategic models, analyze writing style, outline ideas, and generate new content. By the end of this course, you’ll be ready to leverage the power of ChatGPT and other chatbots to deliver more consistent writing outcomes every time.
Note: This course was created by Dave Birss. We are pleased to host this training in our library.
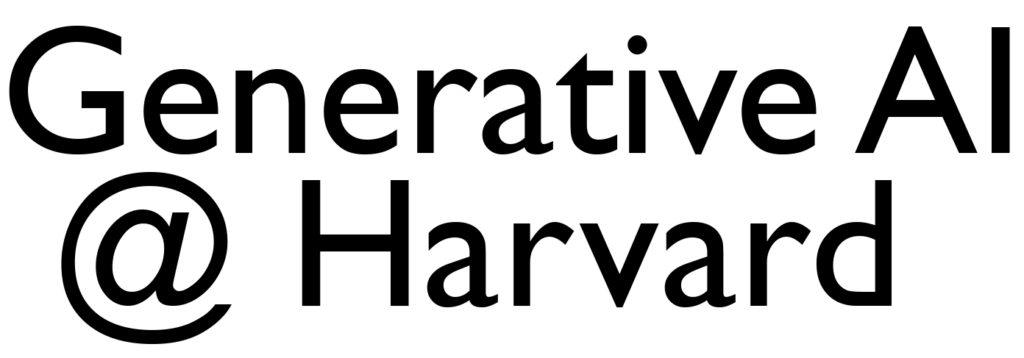
Research with Generative AI
Resources for scholars and researchers
Generative AI (GenAI) technologies offer new opportunities to advance research and scholarship. This resource page aims to provide Harvard researchers and scholars with basic guidance, information on available resources, and contacts. The content will be regularly updated as these technologies continue to evolve. Your feedback is welcome.
Leading the way
Harvard’s researchers are making strides not only on generative AI, but the larger world of artificial intelligence and its applications. Learn more about key efforts.
The Kempner Institute
The Kempner Institute is dedicated to revealing the foundations of intelligence in both natural and artificial contexts, and to leveraging these findings to develop groundbreaking technologies.
Harvard Data Science Initiative
The Harvard Data Science Initiative is dedicated to understanding the many dimensions of data science and propelling it forward.
More AI @ Harvard
Generative AI is only part of the fascinating world of artificial intelligence. Explore Harvard’s groundbreaking and cross-disciplinary academic work in AI.
funding opportunity
GenAI Research Program/ Summer Funding for Harvard College Students 2024
The Office of the Vice Provost for Research, in partnership with the Office of Undergraduate Research and Fellowships, is pleased to offer an opportunity for collaborative research projects related to Generative AI between Harvard faculty and undergraduate students over the summer of 2024.
Learn more and apply
Frequently asked questions
Can i use generative ai to write and/or develop research papers.
Academic publishers have a range of policies on the use of AI in research papers. In some cases, publishers may prohibit the use of AI for certain aspects of paper development. You should review the specific policies of the target publisher to determine what is permitted.
Here is a sampling of policies available online:
- JAMA and the JAMA Network
- Springer Nature
How should AI-generated content be cited in research papers?
Guidance will likely develop as AI systems evolve, but some leading style guides have offered recommendations:
- The Chicago Manual of Style
- MLA Style Guide
Should I disclose the use of generative AI in a research paper?
Yes. Most academic publishers require researchers using AI tools to document this use in the methods or acknowledgements sections of their papers. You should review the specific guidelines of the target publisher to determine what is required.
Can I use AI in writing grant applications?
You should review the specific policies of potential funders to determine if the use of AI is permitted. For its part, the National Institutes of Health (NIH) advises caution : “If you use an AI tool to help write your application, you also do so at your own risk,” as these tools may inadvertently introduce issues associated with research misconduct, such as plagiarism or fabrication.
Can I use AI in the peer review process?
Many funders have not yet published policies on the use of AI in the peer review process. However, the National Institutes of Health (NIH) has prohibited such use “for analyzing and formulating peer review critiques for grant applications and R&D contract proposals.” You should carefully review the specific policies of funders to determine their stance on the use of AI
Are there AI safety concerns or potential risks I should be aware of?
Yes. Some of the primary safety issues and risks include the following:
- Bias and discrimination: The potential for AI systems to exhibit unfair or discriminatory behavior.
- Misinformation, impersonation, and manipulation: The risk of AI systems disseminating false or misleading information, or being used to deceive or manipulate individuals.
- Research and IP compliance: The necessity for AI systems to adhere to legal and ethical guidelines when utilizing proprietary information or conducting research.
- Security vulnerabilities: The susceptibility of AI systems to hacking or unauthorized access.
- Unpredictability: The difficulty in predicting the behavior or outcomes of AI systems.
- Overreliance: The risk of relying excessively on AI systems without considering their limitations or potential errors.
See Initial guidelines for the use of Generative AI tools at Harvard for more information.
- Initial guidelines for the use of Generative AI tools at Harvard
Generative AI tools
- Explore Tools Available to the Harvard Community
- System Prompt Library
- Request API Access
- Request a Vendor Risk Assessment
- Questions? Contact HUIT
Copyright and intellectual property
- Copyright and Fair Use: A Guide for the Harvard Community
- Copyright Advisory Program
- Intellectual Property Policy
- Protecting Intellectual Property
Data security and privacy
- Harvard Information Security and Data Privacy
- Data Security Levels – Research Data Examples
- Privacy Policies and Guidelines
Research support
- University Research Computing and Data (RCD) Services
- Research Administration and Compliance
- Research Computing
- Research Data and Scholarship
- Faculty engaged in AI research
- Centers and initiatives engaged in AI research
- Degree and other education programs in AI
Thank you for visiting nature.com. You are using a browser version with limited support for CSS. To obtain the best experience, we recommend you use a more up to date browser (or turn off compatibility mode in Internet Explorer). In the meantime, to ensure continued support, we are displaying the site without styles and JavaScript.
- View all journals
- Explore content
- About the journal
- Publish with us
- Sign up for alerts
- Published: 18 March 2024
Techniques for supercharging academic writing with generative AI
- Zhicheng Lin ORCID: orcid.org/0000-0002-6864-6559 1
Nature Biomedical Engineering ( 2024 ) Cite this article
2242 Accesses
60 Altmetric
Metrics details
- Developing world
Generalist large language models can elevate the quality and efficiency of academic writing.
This is a preview of subscription content, access via your institution
Access options
Access Nature and 54 other Nature Portfolio journals
Get Nature+, our best-value online-access subscription
24,99 € / 30 days
cancel any time
Subscribe to this journal
Receive 12 digital issues and online access to articles
92,52 € per year
only 7,71 € per issue
Buy this article
- Purchase on Springer Link
- Instant access to full article PDF
Prices may be subject to local taxes which are calculated during checkout
Amano, T. et al. PLoS Biol. 21 , e3002184 (2023).
Article CAS PubMed PubMed Central Google Scholar
Lin, Z. & Li, N. Perspect. Psychol. Sci. 18 , 358–377 (2023).
Article ADS PubMed Google Scholar
Lin, Z. R. Soc. Open Sci. 10 , 230658 (2023).
Article ADS PubMed PubMed Central Google Scholar
Birhane, A., Kasirzadeh, A., Leslie, D. & Wachter, S. Nat. Rev. Phys. 5 , 277–280 (2023).
Article Google Scholar
Thirunavukarasu, A. J. et al. Nat. Med. 29 , 1930–1940 (2023).
Article CAS PubMed Google Scholar
Lin, Z. Nat. Hum. Behav . https://doi.org/10.1038/s41562-024-01847-2 (in the press).
Milano, S., McGrane, J. A. & Leonelli, S. Nat. Mach. Intell. 5 , 333–334 (2023).
White, A. D. Nat. Rev. Chem. 7 , 457–458 (2023).
Golan, R., Reddy, R., Muthigi, A. & Ramasamy, R. Nat. Rev. Urol. 20 , 327–328 (2023).
Article PubMed Google Scholar
Casal, J. E. & Kessler, M. Res. Meth. Appl. Linguist. 2 , 100068 (2023).
Lin, Z. Preprint at https://arxiv.org/abs/2401.15284 (2024).
Wang, H. et al. Nature 620 , 47–60 (2023).
Article ADS CAS PubMed Google Scholar
Dergaa, I., Chamari, K., Zmijewski, P. & Ben Saad, H. Biol. Sport 40 , 615–622 (2023).
Article PubMed PubMed Central Google Scholar
Hwang, S. I. et al. Korean J. Radiol. 24 , 952–959 (2023).
Bell, S. BMC Med. 21 , 334 (2023).
Nazari, N., Shabbir, M. S. & Setiawan, R. Heliyon 7 , e07014 (2021).
Yan, D. Educ. Inf. Technol. 28 , 13943–13967 (2023).
Semrl, N. et al. Hum. Reprod. 38 , 2281–2288 (2023).
Chamba, N., Knapen, J. H. & Black, D. Nat. Astron. 6 , 1015–1020 (2022).
Article ADS Google Scholar
Nat. Biomed. Eng . 2 , 53 (2018).
Croxson, P. L., Neeley, L. & Schiller, D. Nat. Hum. Behav. 5 , 1466–1468 (2021).
Luna, R. E. Nat. Rev. Mol. Cell Biol. 21 , 653–654 (2020).
Merow, C., Serra-Diaz, J. M., Enquist, B. J. & Wilson, A. M. Nat. Ecol. Evol. 7 , 960–962 (2023).
Yurkewicz, K. Nat. Rev. Mater. 7 , 673–674 (2022).
King, A. A. J. Manag. Sci. Rep . https://doi.org/10.1177/27550311231187068 (2023).
Patriotta, G. J. Manag. Stud. 54 , 747–759 (2017).
Gernsbacher, M. A. Adv. Meth. Pract. Psych. 1 , 403–414 (2018).
Google Scholar
Lin, Z. Trends Cogn. Sci. 82 , 85–88 (2024).
Lin, Z. Preprint at https://doi.org/10.31234/osf.io/s6h58 (2023).
Generative AI in Scholarly Communications: Ethical and Practical Guidelines for the Use of Generative AI in the Publication Process (STM, 2023).
Download references
Acknowledgements
The writing of this Comment was supported by the National Key R&D Program of China STI2030 Major Projects (2021ZD0204200), the National Natural Science Foundation of China (32071045) and the Shenzhen Fundamental Research Program (JCYJ20210324134603010). The author used GPT-4 ( https://chat.openai.com ) and Claude ( https://claude.ai ) alongside prompts from Box 1 to help write earlier versions of the text and to edit it. The text was then developmentally edited by the journal’s Chief Editor with basic-editing and structural-editing assistance from Claude, and checked by the author.
Author information
Authors and affiliations.
University of Science and Technology of China, Hefei, China
Zhicheng Lin
You can also search for this author in PubMed Google Scholar
Corresponding author
Correspondence to Zhicheng Lin .
Ethics declarations
Competing interests.
The author declares no competing interests.
Peer review
Peer review information.
Nature Biomedical Engineering thanks Serge Horbach and the other, anonymous, reviewer(s) for their contribution to the peer review of this work.
Rights and permissions
Reprints and permissions
About this article
Cite this article.
Lin, Z. Techniques for supercharging academic writing with generative AI. Nat. Biomed. Eng (2024). https://doi.org/10.1038/s41551-024-01185-8
Download citation
Published : 18 March 2024
DOI : https://doi.org/10.1038/s41551-024-01185-8
Share this article
Anyone you share the following link with will be able to read this content:
Sorry, a shareable link is not currently available for this article.
Provided by the Springer Nature SharedIt content-sharing initiative
Quick links
- Explore articles by subject
- Guide to authors
- Editorial policies
Sign up for the Nature Briefing newsletter — what matters in science, free to your inbox daily.


- Open Search Box
- Toggle side widget area
- No menu assigned!
QUICK LINKS
Using generative ai for scientific research, a quick user’s guide.
(Last updated: 5/03/2024)
If you’re just getting started with using generative AI (GenAI) in your research, start here.
This guide includes frequently asked questions and shows how GenAI can be used throughout the entire research process, based on published guidelines from journals, funding agencies, professional societies, and our own assessment of GenAI’s benefits and risks.
GenAI is a rapidly evolving technology, and we will update this guide as new information becomes available. Suggestions for improvements or additions? Email [email protected]. We look forward to developing this guide collaboratively with our research community.
Technical Guides for Using Generative AI

GenAI in Coding
This quick-start guide helps researchers with little programming experience learn coding with an AI assistant’s help, assuming a chosen programming language like Python, JavaScript, or C++.

Using ChatGPT’s ‘Data Analysis’
Explore how to use ChatGPT 4’s ‘Data Analysis’ feature effectively. This guide covers code organization, error checking, data visualization, and language translation, maximizing the likelihood of accurate and efficient results.

Using Custom GPTs in ChatGPT 4
Explore how to use ChatGPT 4’s ‘Custom GPT’ feature effectively, which allow users to create custom versions of ChatGPT for specific tasks.
Using Generative AI for Writing
Can i use generative ai to write research papers.
The default stance on using generative AI for writing research papers should generally be NO, particularly for creative contributions, due to issues around authorship, copyright, and plagiarism. However, generative AI can be beneficial for editorial assistance, provided you are aware of what is acceptable at your target publication venue.
Generating text and images for publications in scientific journals raises issues of authorship, copyright and plagiarism, many of which are still unresolved. Therefore, this is a very controversial area and many journals and research conferences are updating their policies. If you want to do this, please read very carefully the guidelines for authors of your target journal.
Here are a few examples of new authorship guidelines.
- Springer Nature journals prohibit the use of generative AI to generate images for manuscripts; texts generated by LLM should be well documented, and AI is not granted authorship.
- Science journals require full disclosure for the use of generative AI to generate text; generative AI-generated images and multimedia can be used only with explicit permission of their editors. AI is not granted authorship.
- JAMA and the JAMA network journals do not allow generative AI to be listed as authors. However, generative AI generated content or assistance in writing / editing are allowed in manuscripts but should be reported in the manuscript.
- Elsevier permits the use of AI tools to enhance text readability but not creating or altering scientific content. Authors should provide full disclosure of the use of AI. It prohibits the use of AI to generate or alter images, unless this is part of the research method. AI authorship is not allowed.
- IEEE mandates disclosure of all AI-generated content in submissions, exceptI for editing and grammar enhancement.
- The International Conference on Machine Learning prohibits content generated by generative AI, unless it is part of the research study being described.
While direct generation of content by generative AI is problematic, its role in the earlier stages of writing can be advantageous. For instance, non-native English speakers may use generative AI to refine the language of their writing. Generative AI can also serve as a tool for providing feedback on writing, similar to a copy editor’s role, by improving voice, argument, and structure. This utility is distinct from using AI for direct writing. As long as the human author assumes full responsibility for the final content, such editing help from generative AI is increasingly being recognized as acceptable in most disciplines where language is not the primary scholarly contribution. However, conservative editorial policies at some venues may limit the use of such techniques in the short term.
Can I use generative AI to write grants?
This should be undertaken only with an understanding of the risks involved. The bottom line is that the investigator is signing off on the proposal and is promising to do the work if funded, and so has to take responsibility for every part of the proposal content, even if generative AI assisted in some parts.
The reasoning is similar to that for writing papers, as discussed above, except that there usually will not be copyright and plagiarism issues. Also, not many funding agencies have well-developed policies as yet in this regard.
For example, although the National Institutes of Health (NIH) does not specifically prohibit the use of generative AI to write grants (they do prohibit use of generative AI technology in the peer review process), they state that an author assumes the risk of using an AI tool to help write an application, noting “[…] when we receive a grant application, it is our understanding that it is the original idea proposed by the institution and their affiliated research team.” If AI generated text includes plagiarism, fabricated citations or falsified information, the NIH “will take appropriate actions to address the non-compliance.” ( Source.)
Similarly, the National Science Foundation (NSF), in its notice dated December 14, 2023, emphasizes the use of generative AI in grant proposal preparation and the merit review process. While NSF acknowledges the potential benefits of AI in enhancing productivity and creativity, it imposes strict guidelines to safeguard the integrity and confidentiality of proposals.
The DOE requires authors to verify any citations suggested by generative AI, due to potential inaccuracies, and does not allow AI-based chatbots like ChatGPT to be credited as authors or co-authors.
Reviewers are prohibited from uploading proposal content to non-approved AI tools, and proposers are encouraged to disclose the extent and manner of AI usage in their proposals. The NSF stresses that any breach in confidentiality or authenticity, especially through unauthorized disclosure via AI, could lead to legal liabilities and erosion of trust in the agency. (Source.)
Can I use generative AI to help me when I write a literature review section for my paper?
Generative AI can offer multiple advantages. Generative AI can help you summarize a particular paper, so this saves you time and enables you to cover a much larger number of publications in the limited time you have. Generative AI can also help you summarize literature around certain research questions by searching through many papers.
However, you should consider a number of factors that may impact how much you can trust such reviews.
- When generative AI encounters a request that it lacks information / knowledge about, sometimes it “makes up” an answer. This “AI hallucination” is well documented and probably many of us have experienced it. You are responsible for verifying the summaries that generative AI gives you.
- Unlike human researchers, generative AI does not have the ability to evaluate the quality of the published work. Therefore, it will indiscriminately include publications of varying quality, perhaps also many studies that cannot be reproduced.
- A generative AI model has a knowledge cutoff date, so newer publications after the cutoff date will not be included in the responses that it gives you.
- Other types of inaccuracies. Generative AI’s effectiveness is based on the training datasets. Even though enormous amounts of training data are now used for generative AI models, there is still no guarantee that the training is unbiased.
Also, please do keep in mind all the limitations discussed above regarding the use of generative AI to assist in writing research papers. Subject to those limitations, this seems to be a reasonable thing to do.
Can I use generative AI to write non-technical summaries, create presentations, and translate my work?
Generative AI can be beneficial for summarizing or translating your work, especially with its ability to adjust the tone of a text, making it easier to create brief but complete summaries that suit different types of readers. Several advanced generative AI models are designed specifically to transform scientific manuscripts into presentations.
However, you should be sure that, while using generative AI to summarize, present, or translate your work, you don’t input confidential information to generative AI. You should also always verify that summaries, presentations and translations created by generative AI accurately represent your work. When using generative AI for translation, it could be challenging if you are not proficient in both languages involved and you need to consult with a fluent speaker for verification. Also note that not all generative AI models are explicitly designed for translation tasks. Therefore, you should explore and identify the most suitable generative AI model that aligns with your specific translation needs.
Using Generative AI to Improve Productivity
Can i use generative ai to review grant proposals or review papers.
No, you should not do this. The National Institutes of Health recently announced that it prohibits the use of generative AI to analyze and formulate critiques of grant proposals . This not only applies to generative AI systems that are publicly available, but also to systems hosted locally (such as a university’s own generative AI), as long as data may be shared with multiple individuals. The main rationale is that this would constitute a breach of confidentiality, which is essential in the grant review process. To use generative AI tools to evaluate and summarize grant proposals, or even let it edit critiques, one would need to feed to the AI system “substantial, privileged, and detailed information.” When we don’t know how the AI system will save, share or use the information that it is fed, we should not feed it such information.
Furthermore, expert review relies upon subject matter expertise, which a generative AI system could not be relied upon to have. So, it is unlikely that generative AI will produce a reliable and high-quality review.
For these reasons, we don’t recommend that you use generative AI for reviewing grant proposals or papers, even if the relevant publication venue or funding agency, unlike NIH, has not issued explicit guidance.
Can I use generative AI to write letters of support?
Generative AI can, in some situations, be useful to help you draft a letter, or edit your draft and to help you adopt a certain tone. We are not aware of any explicit rules against this. However, please keep in mind the following:
- You are still fully responsible for everything in the letter because you are still the author.
- You should consider the issue of confidentiality. Is there confidential information in the letter? If so, generative AI should not “know” it, because, again, we do not know for sure what it does with the information that users feed it.
- Texts written by GPT tend to sound very generic. This is not good for letters of support, whose value may depend on their providing very specific information, and recommendations, about the subject of the letter. You still need to ensure that the letter is what you feel comfortable sending and will convey to the reader the same level of support to the subject of the letter if you’d write it yourself.
How can I use generative AI as a Brainstorming Partner in My Research?
Generative AI can serve as effective brainstorming partners in research. These systems can – when used appropriately – help generate a variety of ideas, perspectives, and potential solutions, particularly useful during the initial stages of research planning. For instance, a researcher can input their basic research concept into the AI system and receive suggestions on experimental approaches, potential methodologies, or alternative research questions. An example prompt may be:
“Analyze recent research on memory consolidation and the influence of emotions on learning and recall. Based on this analysis, generate new hypotheses for potential studies investigating neurobiological mechanisms.”
However, AI-generated ideas must be critically evaluated. While AI can offer diverse insights, these are based on existing data and may not always be novel or contextually appropriate. Researchers should use these suggestions as a starting point for further development rather than as definitive solutions.
Using Generative AI for Data Generation and Analysis
Can i use generative ai to write code.
Yes, provided you can read code! Generative AI can indeed output computer programs. But, just as in the case of text, it is possible you get code that is good-looking but erroneous. To the extent that it is often easier to read code than to write it, you may be better off using generative AI to write code for you. We provide a guide on generating, editing and reviewing code using ChatGPT 4.0 here and a coding tutorial using local software such as GitHub copilot here .
This applies not just to computer programs, but also to databases. You can have generative AI write code for you in SQL to manage and to query databases. In fact, in many cases, you could even do some minimal debugging just by running the code/query on known instances and checking to make sure you get the right answers. While basic tests like these can catch many errors, remember that there is no guarantee your program will work on complex examples just because it worked on simple ones.
Can I use generative AI for data analysis and visualization?
Yes. Generative AI models have been constantly improved to carry out data analysis and visualization. We provide some examples of data analysis and visualizations using ChatGPT 4.0 here .
Can I use generative AI as a substitute for human participants in surveys?
Using generative AI as a substitute for human participants in surveys is not advisable due to significant concerns regarding construct validity. Generative AI, while adept at processing and generating data, cannot authentically replicate the nuances of human behavior and opinions that are the purpose of surveying humans in research.
However, generative AI can be valuable in the preliminary stages of survey design. It can assist in testing the clarity and structure of survey questions, helping address ambiguity and effectively capture the intended information. This application leverages AI’s capability to process language and simulate varied responses, providing insights into how questions may be interpreted by a diverse audience. In short, while generative AI’s use as a direct replacement for human survey participants is not recommended due to validity concerns, its role in enhancing survey design and testing is a viable and beneficial application.
Can generative AI be used for labeling data?
Generative AI can be employed for labeling, such as categorizing text and images. This application can streamline processes that are traditionally time-consuming and labor-intensive for human judges. However, the reliability of AI in these tasks requires careful consideration and validation on a case-by-case basis.
The key concern with AI-based judgment in labeling is its dependence on the quality and bias of training data. AI systems might replicate any inherent biases present in their training datasets, leading to skewed or inaccurate labeling. Researchers must validate the AI’s performance – comparing output with human-labeled benchmarks to ensure accuracy and impartiality.
Can I use generative AI to review data for errors and biases?
Yes! Generative AI can serve as a supplementary tool in the process of data quality assurance, assisting in the identification of errors, inconsistencies, or biases in datasets. Its capability to process extensive data rapidly enables it to spot potential issues that might be missed in manual reviews. Researchers should use Generative AI as one component of a broader data review strategy. It’s essential to corroborate AI-detected anomalies with manual checks and expert assessments.
Reporting the Use of Generative AI
How do i cite contents created or assisted by generative ai.
You used generative AI in the course of writing a research paper. How do you give it credit? And how do you inform the reader of your paper about its use?
Generative AI should not be listed as a co-author, but its use must be noted in the paper, including appropriate detail, e.g. about specific prompts and responses. The Committee on Publication Ethics has a succinct and incisive analysis .
The use of generative AI should be disclosed in the paper, along with a description of the places and manners of use. Typically, such disclosures will be in a “Methods” section of the paper, if it has one. If you rely on generative AI output, you should cite it, just as you would cite a web page look up or a personal communication. Keep in mind that some conversation identifiers may be local to your account, and hence not useful to your reader. Good citation style recommendations have been suggested by the American Psychological Association (APA) and the Chicago Manual of Style .
How do I report the use of generative AI models in a paper?
We provide recommendations on reporting the use of generative AI in research here .
Considerations for Choosing Generative AI Models
How do i decide which generative ai to use in research.
The most important factor is which generative AI system (what data, what model, what computing requirements) fits well with your research questions. In addition, there are some general considerations.
Open source . “Open source” describes software that is published alongside the source code for use and exploration by anyone. This is a consideration because most generative AI models are not developed locally by the researchers themselves (as opposed to the usual Machine Learning models). Open-source generative AIs, as well generative AI systems trained with publicly accessible data, can be advantageous for researchers who would like to fine tune generative AI models, scrutinize the security and functionality of the system, and improve explainability and interpretability of the models.
Accuracy and precision . When outputs of a generative AI can be verified (for example, if it is used in data analytics), you can gauge the efficacy of a generative AI by its precision and accuracy.
Cost. Some models require subscriptions to APIs (application programming interfaces) for research use. Other models may be able to be integrated locally, but also come with integration costs and potentially ongoing costs for maintenance and updates. When selecting otherwise free models, you might need to cover the cost for an expert to set up and maintain the model.
Can I customize generative AI models?
Yes. Some commercial generative AI developers now provide ways for users to easily customize the models, provide their own data and documents to fine tune the models, and specify the styles of model outputs. See our Custom GPT guide for more details.
What uniquely generative AI issues should I consider when I adopt generative AI in my research?
The nature of generative AI gives rise to a number of considerations that the entire research community is trying to grapple with. Transparency and accountability about the generative AI’s operations and decision making processes can be difficult when you operate a closed-source system.
We invite you to think about the following carefully, and be aware that many other issues might arise.
Data privacy concerns. Data privacy is more complicated with generative AI when using cloud-based services, as users don’t know for certain what happens to their input data and whether it could be retained for training future AI models. One way to circumvent these privacy concerns is to use locally-deployed generative AI models that run entirely on your own hardware and do not send data back to the AI provider. An example is Nvidia ChatRTX .
Bias in data. Bias in data, and consequently bias in the AI system’s output, could be a major issue because generative AI is trained on large datasets that you usually can’t access or assess, and may inadvertently learn and reproduce biases, stereotypes, and majority views present in these data. Moreover, many generative AI models are trained with overwhelmingly English texts, Western images and other types of data. Non-Western or non-English speaking cultures, as well as work by minorities and non-English speakers are seriously underrepresented in the training data. Thus, the results created by generative AI are definitely culturally biased. This should be a major consideration when assessing whether generative AI is suitable for your research.
AI hallucination. generative AI can produce outputs that are factually inaccurate or entirely incorrect, uncorroborated, nonsensical or fabricated. These phenomena are dubbed “hallucinations”. Therefore, it is essential for you to verify generative AI-generated output with reliable and credible sources.
Plagiarism. generative AI can only generate new contents based on, or drawn from, the data that it is trained on. Therefore, there is a likelihood that they will produce outputs that are similar to the training data, even to the point of being regarded as plagiarism if the similarity is too high. As such, you should confirm (e.g. by using plagiarism detection tools) that generative AI outputs are not plagiarized but instead “learned” from various sources in the manner humans learn without plagiarizing.
Prompt Engineering. The advent of generative AI has created a new human activity – prompt engineering – because the quality of generative AI responses is heavily influenced by the user input or ‘prompt’. There are courses dedicated to this concept. However, you will need to experiment with how to craft prompts that are clear, specific and appropriately structured so that generative AI will generate the output with the desired style, quality and purpose.
Knowledge Cutoff Date. Many generative AI models are trained on data up to a specific date, and are therefore unaware of any events or information produced beyond that. For example, if a generative AI is trained on data up to March 2019, they would be unaware of COVID-19 and the impact it had on humanity, or who is the current monarch of Britain. You need to know the cutoff date of the generative AI model that you use in order to assess what research questions are appropriate for its use.
Model Continuity . When you use generative AI models developed by external entities / vendors, you need to consider the possibility that one day the vendor might discontinue the model. This might have a big impact on the reproducibility of your research.
Security . As with any computer or online system, a generative AI system is susceptible to security breaches and attacks. We have already mentioned the issue of confidentiality and privacy as you input information or give prompts to the system. But malicious attacks could be a bigger threat. For example, a new type of attack, prompt injection, deliberately feeds harmful or malicious contents into the system to manipulate the results that it generates for users. generative AI developers are designing processes and technical solutions against such risks (for example, see OpenAI’s GPT4 System Card and disallowed usage policy . But as a user, you also need to be aware what is at risk, follow guidelines of your local IT providers, and do due diligence with the results that a generative AI creates for you.
Lack of Standardized Evaluations : The AI Index Report 2024 found that leading developers test their models against different responsible AI benchmarks, making it challenging to systematically compare the risks and limitations of AI models. Be wary when models tout confidence in certain evaluation measures, as the measures may not have been fully tested.
Related Resources
Additional reading.
Many recommendations, guidelines and comments are out there regarding the use of Generative AI in research and in other lines of work. Here are a few examples.
- Best Practices for Using AI When Writing Scientific Manuscripts: Caution, Care, and Consideration: Creative Science Depends on It. Jullian M. Buriak, et al. ACS Nano (2023)
- Science journals set new authorship guidelines for AI-generated text . Jennifer Harker. National Institute of Environmental Health Sciences (2023)
- NIH prohibits the use of Generative AI in peer review . (2023)
- Fighting reviewer fatigue or amplifying bias? Considerations and recommendations for use of ChatGPT and other large language models in scholarly peer review. Mohammad Hosseini and Serge P J M Horbach. Research integrity and peer review (2023)
- Nonhuman “Authors” and Implications for the Integrity of Scientific Publication and Medical Knowledge . Annette Flanagin et al, JAMA (2023)
- 2024 AI Index Report . Stanford University (2024)
For more content including manuscripts, use of generative AI in research and more – see our generative AI resource page .
Sign Up for the MIDAS Newsletter

Copyright © 2020 The Regents of the University of Michigan
- U-M Gateway
- U-M Non-discrimination Statement
- Governance and Advisory Boards
- Annual Highlights
- Newsletters
- Research Pillars
- MIDAS Funded Projects
- Generative AI
- AI in Science Program
- Michigan Data Science Fellows
- Graduate Certificate
- Summer Academies
- Future Leaders Summit
- Summer Camp
- Other Programs
- Mini-Symposia
- 2024 ADSA Annual Meeting
- Past Events
- Academic Partnerships
- Data Science for Social Good
- Working Groups
- Staff Collective for Data Science
- NLP+CSS 201
- Student Organizations Council
- Projects for Students
- Generative AI Resources
- Reproducibility Hub
- Research Datasets
- Drug Discovery IUCRC

Generative Artificial Intelligence
- What is Generative AI?
- When to Use AI for Research
- How to Use AI for Research
- Evaluating Generative AI Tools
- Citing Generative AI Tools
- Examining an AI Chatbot Hallucination
Accuracy of AI

This image was created with Dall-e 2 using the prompt: "a robot hallucinating"
Generative AI is it is only as good as the sources it pulls from and it can hallucinate information.
Treat your results from AI with caution.

Using AI for Research
To use AI effectively for research one of the first things you should ask is what are you trying to accomplish and be intentional about the type of tool you use. You may even want to consider if AI is the best sort of tool for what you are looking for.
There are lots of different AI tools. See [different page for a list of different AI research assistants and what they do].
Prompt Engineering for a Research Question
This section will focus on writing prompts for chat based AI tools like ChatGPT, GoogleBard, or Microsoft Bing's chatbot.
Key Terminology
Prompt Engineering is the intentional design of prompts given to artificial intelligence tools so that the response it gives you are helpful.
Prompt Patterns are different structures you can use to engineer AI prompts
Prompt Pattern: Persona
How to create the prompt.
- Act as a certain persona (e.g. "imagine you are an anthropologist"
- You can give additional context, such as what the persona is trying to accomplish
Here's an example of how to apply this in your research
Prompt: Imagine you are an anthropologist. What are keywords and terms you would likely use if you were writing a paper about the formation of social hierarchies in societies.
This prompt will give a list of different search terms you can try to find relevant papers on your topic
See how ChatGPT responded to a persona pattern prompt: https://chat.openai.com/share/8fa6b79a-0a30-4775-bc3f-96f7f8304c8d Compare this to a similar question but asked with the persona being a not specific about the persona who is doing the research: https://chat.openai.com/share/4917eed8-75fc-4e89-81a4-9db328639af1
Prompt Pattern: Simulated Interaction
Instead of asking ChatGPT questions, have it ask questions to you
- Explain to ChatGPT what the interaction will look like (I will prompt you, then I want you to ask me a question based on that prompt).
- You can do this as one-off questions or more in-depth.
Prompt: I want to write a psychology paper about memory but I am not sure what direction to go with my specific topic. Help me come up with a starting research question by asking me questions about what aspects of memory I am interested in. When you have enough information to write a research topic, stop asking questions and write the research question. Ask me one question at a time and wait for my response.
This prompt will make ChatGPT act as a conversation partner to help you think through decisions about narrowing down your research topic.
See how ChatGPT responded to a simulated interaction prompt: https://chat.openai.com/share/ffe72f48-799f-427e-b9dd-bce5ecea6996
Prompt Pattern: Schedule Maker
Tell ChatGPT what you are trying to accomplish and ask it to create a schedule to keep you on task
Prompt: I have three hours tonight I can work on research for my paper on social media's impact on presidential elections. By the end of those three hours I want to have found two peer-reviewed sources and created an annotated bibliography of those sources. Can you create a schedule of what I should do between 7pm and 10 pm to accomplish those things on time?
This prompt will give you a schedule of what to work on during a set amount of time and also breaks down research into concrete steps
See how ChatGPT responded to a prompt to create a schedule; https://chat.openai.com/share/3b867579-d62a-4736-b0d7-de26284cd061
- << Previous: When to Use AI for Research
- Next: Evaluating Generative AI Tools >>
- Last Updated: Apr 5, 2024 10:58 AM
- URL: https://davidson.libguides.com/generative-ai
Mailing Address : Davidson College - E.H. Little Library, 209 Ridge Road, Box 5000, Davidson, NC 28035

Generative AI in Academic Writing
What this handout is about.
You’ve likely heard of AI tools such as ChatGPT, Google Bard, Microsoft Bing, or others by now. These tools fall under a broad, encompassing term called generative AI that describes technology that can create new text, images, sounds, video, etc. based on information and examples drawn from the internet. In this handout, we will focus on potential uses and pitfalls of generative AI tools that generate text.
Before we begin: Stay tuned to your instructor
Instructors’ opinions on the use of AI tools may vary dramatically from one class to the next, so don’t assume that all of your instructors will think alike on this topic. Consult each syllabus for guidance or requirements related to the use of AI tools. If you have questions about if/how/when it may be appropriate to use generative AI in your coursework, be sure to seek input from your instructor before you turn something in for a grade. You are always 100% responsible for whatever writing you chose to turn in to an instructor, so it pays to inquire early.
Note that when your instructors authorize the use of generative AI tools, they will likely assume that these tools may help you think and write—not think or write for you. Keep that principle in mind when you are drafting and revising your assignments. You can maintain your academic integrity and employ the tools with the same high ethical standards and source use practices that you use in any piece of academic writing.
What is generative AI, and how does it work?
Generative AI is an artificial intelligence tool that allows users to ask it questions or make requests and receive quick written responses. It uses Large Language Models (LLMs) to analyze vast amounts of textual data to determine patterns in words and phrases. Detecting patterns allows LLMs to predict what words may follow other words and to transform the content of its corpus (the textual data) into new sentences that respond to the questions or requests. Using complex neural network models, LLMs generate writing that mimics human intelligence and varied writing styles.
The textual data used to train the LLM has been scraped from the internet, though it is unclear exactly which sources have been included in the corpus for each AI tool. As you can imagine, the internet has a vast array of content of variable quality and utility, and generative AI does not distinguish between accurate/inaccurate or biased/unbiased information. It can also recombine accurate source information in ways that generate inaccurate statements, so it’s important to be discerning when you use these tools and to carefully digest what’s generated for you. That said, the AI tools may spark ideas, save you time, offer models, and help you improve your writing skills. Just plan to bring your critical thinking skills to bear as you begin to experiment with and explore AI tools.
As you explore the world of generative AI tools, note that there are both free and paid versions. Some require you to create an account, while others don’t. Whatever tools you experiment with, take the time to read the terms before you proceed, especially the terms about how they will use your personal data and prompt history.
In order to generate responses from AI tools, you start by asking a question or making a request, called a “prompt.” Prompting is akin to putting words into a browser’s search bar, but you can make much more sophisticated requests from AI tools with a little practice. Just as you learned to use Google or other search engines by using keywords or strings, you will need to experiment with how you can extract responses from generative AI tools. You can experiment with brief prompts and with prompts that include as much information as possible, like information about the goal, the context, and the constraints.
You could experiment with some fun requests like “Create an itinerary for a trip to a North Carolina beach.” You may then refine your prompt to “Create an itinerary for a relaxing weekend at Topsail Beach and include restaurant recommendations” or “Create an itinerary for a summer weekend at Topsail Beach for teenagers who hate water sports.” You can experiment with style by refining the prompt to “Rephrase the itinerary in the style of a sailor shanty.” Look carefully at the results for each version of the prompt to see how your changes have shaped the answers.
The more you experiment with generative AI for fun, the more knowledgeable and prepared you will be to use the tool responsibly if you have occasion to use it for your academic work. Here are some ways you might experiment with generative AI tools when drafting or exploring a topic for a paper.
Potential uses
Brainstorming/exploring the instructor’s prompt Generative AI can help spark ideas or categories for brainstorming. You could try taking key words from your topic and asking questions about these ideas or concepts. As you narrow in on a topic, you can ask more specific or in-depth questions.
Based on the answers that you get from the AI tool, you may identify some topics, ideas, or areas you are interested in researching further. At this point, you can start exploring credible academic sources, visit your instructor’s office hours to discuss topic directions, meet with a research librarian for search strategies, etc.
Generating outlines AI tools can generate outlines of writing project timelines, slide presentations, and a variety of writing tasks. You can revise the prompt to generate several versions of the outlines that include, exclude, and prioritize different information. Analyze the output to spark your own thinking about how you’d like to structure the draft you’re working on.
Models of genres or types of writing If you are uncertain how to approach a new format or type of writing, an AI tool may quickly generate an example that may inform how you develop your draft. For example, you may never have written—a literature review, a cover letter for an internship, or an abstract for a research project. With good prompting, an AI tool may show you what type of written product you are aiming to develop, including typical components of that genre and examples. You can analyze the output for the sequence of information to help you get a sense of the structure of that genre, but be cautious about relying on the actual information (see pitfalls below). You can use what you learn about the structures to develop drafts with your own content.
Summarizing longer texts You can put longer texts into the AI tool and ask for a summary of the key points. You can use the summary as a guide to orient you to the text. After reading the summary, you can read the full text to analyze how the author has shaped the argument, to get the important details, and to capture important points that the tool may have omitted from the summary.
Editing/refining AI tools can help you improve your text at the sentence level. While sometimes simplistic, AI-generated text is generally free of grammatical errors. You can insert text you have written into an AI tool and ask it to check for grammatical errors or offer sentence level improvements. If this draft will be turned into your instructor, be sure to check your instructor’s policies on using AI for coursework.
As an extension of editing and revising, you may be curious about what AI can tell you about your own writing. For example, after asking AI tools to fix grammatical and punctuation errors in your text, compare your original and the AI edited version side-by-side. What do you notice about the changes that were made? Can you identify patterns in these changes? Do you agree with the changes that were made? Did AI make your writing more clear? Did it remove your unique voice? Writing is always a series of choices you make. Just because AI suggests a change, doesn’t mean you need to make it, but understanding why it was suggested may help you take a different perspective on your writing.
Translation You can prompt generative AI tools to translate text or audio into different languages for you. But similar to tools like Google Translate, these translations are not considered completely “fluent.” Generative AI can struggle with things like idiomatic phrases, context, and degree of formality.
Transactional communication Academic writing can often involve transactional communication—messages that move the writing project forward. AI tools can quickly generate drafts of polite emails to professors or classmates, meeting agendas, project timelines, event promotions, etc. Review each of the results and refine them appropriately for your audiences and purposes.
Potential pitfalls
Information may be false AI tools derive their responses by reassembling language in their data sets, most of which has been culled from the internet. As you learned long ago, not everything you read on the internet is true, so it follows that not everything culled and reassembled from the internet is true either. Beware of clearly written, but factually inaccurate or misleading responses from AI tools. Additionally, while they can appear to be “thinking,” they are literally assembling language–without human intelligence. They can produce information that seems plausible, but is in fact partly or entirely fabricated or fictional. The tendency for AI tools to invent information is sometimes referred to as “hallucinating.”
Citations and quotes may be invented AI responses may include citations (especially if you prompt them to do so), but beware. While the citations may seem reasonable and look correctly formatted, they may, in fact, not exist or be incorrect. For example, the tools may invent an author, produce a book title that doesn’t exist or incorrectly attribute language to an author who didn’t write the quote or wrote something quite different. Your instructors are conversant in the fields you are writing about and may readily identify these errors. Generative AI tools are not authoritative sources.
Responses may contain biases Again, AI tools are drawing from vast swaths of language from their data sets–and everything and anything has been said there. Accordingly, the tools mimic and repeat distortions in ideas on any topic in which bias easily enters in. Consider and look for biases in responses generated by AI tools.
You risk violating academic integrity standards When you prompt an AI tool, you may often receive a coherent, well written—and sometimes tempting—response. Unless you have received explicit, written guidance from an instructor on use of AI generated text, do not assume it is okay to copy and paste or paraphrase that language into your text—maybe at all. See your instructor’s syllabus and consult with them about how they authorize the use of AI tools and how they expect you to include citations for any content generated by the tool. The AI tools should help you to think and write, not think or write for you. You may find yourself violating the honor code if you are not thoughtful or careful in your use of any AI generated material.
The tools consume personal or private information (text or images) Do not input anything you prefer not to have widely shared into an AI generator. The tools take whatever you put in to a prompt and incorporate it into its systems for others to use.
Your ideas may be changed unacceptably When asked to paraphrase or polish a piece of writing, the tools can change the meaning. Be discerning and thorough in reviewing any generated responses to ensure the meaning captures and aligns with your own understanding.
A final note
Would you like to learn more about using AI in academic writing? Take a look at the modules in Carolina AI Literacy . Acquainting yourself with these tools may be important as your thinking and writing skills grow. While these tools are new and still under development, they may be essential tools for you to understand in your current academic life and in your career after you leave the university. Beginning to experiment with and develop an understanding of the tools at this stage may serve you well along the way.
Note: This tip sheet was created in July 2023. Generative AI technology is evolving quickly. We will update the document as the technology and university landscapes change.
You may reproduce it for non-commercial use if you use the entire handout and attribute the source: The Writing Center, University of North Carolina at Chapel Hill
Make a Gift
Generative AI Can Supercharge Your Academic Research
Explore more.
- Artificial Intelligence
- Perspectives
C onducting relevant scholarly research can be a struggle. Educators must employ innovative research methods, carefully analyze complex data, and then master the art of writing clearly, all while keeping the interest of a broad audience in mind.
Generative AI is revolutionizing this sometimes tedious aspect of academia by providing sophisticated tools to help educators navigate and elevate their research. But there are concerns, too. AI’s capabilities are rapidly expanding into areas that were once considered exclusive to humans, like creativity and ingenuity. This could lead to improved productivity, but it also raises questions about originality, data manipulation, and credibility in research. With a simple prompt, AI can easily generate falsified datasets, mimic others’ research, and avoid plagiarism detection.
As someone who uses generative AI in my daily work, both in academia and beyond, I have spent a lot of time thinking about these potential benefits and challenges—from my popular video to the symposium I organized this year, both of which discuss the impact of AI on research careers. While AI can excel in certain tasks, it still cannot replicate the passion and individuality that motivate educators; however, what it can do is help spark our genius.
Below, I offer several ways AI can inspire your research, elevating the way you brainstorm, analyze data, verify findings, and shape your academic papers.
AI’s potential impact on research, while transformative, does heighten ethical and existential concerns about originality and academic credibility. In addition to scrutiny around data manipulation and idea plagiarism, educators using AI may face questions about the style, or even the value, of their research.
However, what truly matters in academic research is not the tools used, but educators’ approach in arriving at their findings. Transparency, integrity, intellectual curiosity, and a willingness to question and challenge one’s previous beliefs and actions should underpin this approach.
Despite potentially compounding these issues, generative AI can also play a pivotal role in addressing them. For instance, a significant problem in research is the reliance on patterns and correlations without understanding the “why” behind them. We can now ask AI to help us understand causality and mechanisms that are most likely. For example, one could inquire, “ What are the causal explanations behind these correlations? What are the primary factors contributing to spurious correlations in this data? How can we design tests to limit spurious correlations? ”
AI has the potential to revolutionize research validation, ensuring the reliability of findings and bolstering the scientific community’s credibility. AI’s ability to process massive amounts of data efficiently makes it ideal for generating replication studies. Instructions such as “ Suggest a replication study design and provide detailed instructions for independent replication ,” or “ Provide precise guidance for configuring a chatbot to independently replicate these research findings ” can guide educators in replicating and verifying study results.
ChatGPT-4 , OpenAI’s latest and paid version of the large language model (LLM), plays a vital role in enhancing my daily research process; it has the capacity to write, create graphics, analyze data, and browse the internet, seemingly as a human would. Rather than using predefined prompts, I conduct generative AI research in a natural and conversational manner, using prompts that are highly specific to the context.
To use ChatGPT-4 as a valuable resource for brainstorming, I ask it prompts such as, “ I am thinking about [insert topic], but this is not a very novel idea. Can you help me find innovative papers and research from the last 10 years that has discussed [insert topic]? ” and “ What current topics are being discussed in the business press? ” or “ Can you create a table of methods that have and have not been used related to [insert topic] in recent management research? ”
The goal is not to have a single sufficient prompt, but to hone the AI’s output into robust and reliable results, validating each step along the way as a good scholar would. Perhaps the AI sparks an idea that I can then pursue, or perhaps it does not help me at all. But sometimes just asking the questions furthers my own process of getting “unstuck” with hard research problems.
There is still a lot of work to be done after using these prompts, but having an AI research companion helps me quickly get to a better answer. For example, the prompt “ Explore uncharted areas in organizational behavior and strategy research ” led to the discovery of promising niches for future research projects. You might think that this will result in redundant projects, but all you have to do is write, “ I don’t like that, suggest more novel ideas ” or “ I like the second point, suggest 10 ideas related to it and make them more unique ” to come up with some interesting projects.
2. Use AI to gather and analyze data
Although the AI is far from perfect, iterative feedback can help its output become more robust and valuable. It is like an intelligent sounding board that adds clarity to your own ideas. I do not necessarily have a set of similar prompts that I always use to gather data, but I have been able to leverage ChatGPT-4’s capabilities to assist in programming tasks, including writing and debugging code in various programming languages.
Additionally, I have used ChatGPT-4 to craft programs designed for web scraping and data extraction. The tool generates code snippets that are easy to understand and helps find and fix errors, which makes it useful for these tasks. Prior to AI, I would spend far more time debugging software programs than I did writing. Now, I simply ask, “ What is the best way to collect data on [insert topic]? What is the best software to use for this? Can you help get that data? How do I build the code to get this data? What is the best way to analyze this data? If you were a skeptical reviewer, what would you also control for with this analysis? ”
“While the initial results may not be on point, starting from scratch without AI is still more difficult.”
When the AI generates poor responses, I ask, “ That did not work. Here is my code, can you help me find the problem? Can you help me debug this code? Why did that not work? ” or “ No, that is incorrect. Can you suggest two alternative ways to generate the result? ” There have been many occasions when the AI suggests that data will exist; however, like inspiration in the absence of AI, the data is not practically accessible or useful upon further examination. In those situations, I write, “ That data is too difficult to get, can you suggest good substitutes? ” or “ That is not real data, can you suggest more novel data or a data source where I can find the proper data? ”
While the initial results may not be on point, starting from scratch without AI is still more difficult. By incorporating AI into this data gathering and analysis process, researchers can gain valuable insights and solve difficult problems that often have ambiguous and equivocal solutions. For instance, learning how to program more succinctly or think of different data sources can help discovery. It also makes the process much less frustrating and more effective.
3. Use AI to help verify your findings and enhance transparency
AI tools can document the evolution of research ideas, effectively serving as a digital audit trail. This trail is a detailed record of a research process, including queries, critical decision points, alternative hypotheses, and refinements throughout the entire research study creation process. One of the most significant benefits of maintaining a digital audit trail is the ability to provide clear and traceable evidence of the research process. This transparency adds credibility to research findings by demonstrating the methodical steps taken to reach conclusions.
For example, when I was writing some code to download data from an external server, I asked, “ Can you find any bugs or flaws in this software program? ” and “ What will the software program’s output be? ” One of the problems I ran into was that the code was inefficient and required too much memory, taking several days to complete. When I asked, “ Could you write it in simpler and more efficient code? ” the generated code provided an alternative method for increasing data efficiency, significantly reducing the time it took.
“Prior to AI, I would spend far more time debugging software programs than I did writing.”
What excites me the most is the possibility of making it easier for other researchers to replicate what I did. Because writing up these iterations takes time, many researchers skip this step. With generative AI, we can ask it to simplify many of these steps so that others can understand them. For example, I might ask the following:
Can you write summarized notations of this program or of the previous steps so that others can understand what I did here?
Can we reproduce these findings using a different statistical technique?
Can you generate a point-by-point summary diary of what I did in the previous month from this calendar?
Can you create a step-by-step representation of the workflow I used in this study?
Can you help generate an appendix of the parameters, tests, and configuration settings for this analysis?
In terms of qualitative data, I might ask, “ Can you identify places in this text where this idea was discussed? Please put it in an easy-to-understand table ” or “ Can you find text that would negate these findings? What conditions do you believe generated these counterfactual examples? ”
You could even request that the AI create a database of all the prompts you gave it in order for it to generate the results and data. With the advent of AI-generated images and videos, we may soon be able to ask it to generate simple video instructions for recreating the findings or to highlight key moments in a screen recording of researchers performing their analyses. This not only aids validation but also improves the overall reliability and credibility of the research. Furthermore, because researchers incur little cost in terms of time and resources, such demands for video instructions may eventually be quite reasonable.
4. Use AI to predict and then parse reviewer feedback
I try to anticipate reviewer concerns before submitting research papers by asking the AI, “ As a skeptical reviewer who is inclined to reject papers, what potential flaws in my paper do you see? How can I minimize those flaws? ” The results help me think through areas where my logic or analysis may be flawed, and what I might want to refine before submitting my paper to a skeptical scientific audience. The early detection of problems in a competitive scientific arena with high time pressure can be effective and time saving.
Once I receive reviewer feedback, I also like to use ChatGPT to better understand what reviewers expect of me as an author. I’ll ask, “ Help me identify key points in this review, listing them from the easiest and quickest comments to address, up to the most challenging and time-consuming reviewer comments. ” It’s surprising how much more enjoyable the review process becomes once I have a more holistic understanding of what the reviewer or editor is asking.
Balancing AI’s strengths and weaknesses to improve academic research
As educators, we must learn to coexist and co-create with these technological tools. LLMs have the potential to accelerate and improve research, resulting in ground-breaking ideas that push the limits of current possibilities.
But we must be careful. When used incorrectly, AI can speed up the process of achieving surface-level learning outcomes at the expense of a deeper understanding. Educators should approach generative AI with skepticism and curiosity, like they would with any other promising tool.
AI can also democratize research by making it accessible to people of all abilities and levels of expertise. This only makes our human essence—passions, interests, and complexities—even more important. After all, AI might be great at certain tasks, but the one thing it can’t take away is what makes you, well, you.
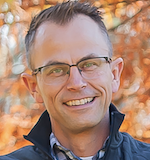
David Maslach is an associate professor at Florida State University specializing in organizational learning and innovation. He holds a PhD from the Ivey School of Business and serves on multiple academic journal boards. Maslach is also the founder of the R3ciprocity Project , a platform that provides solutions and hope to the global research community.
Related Articles
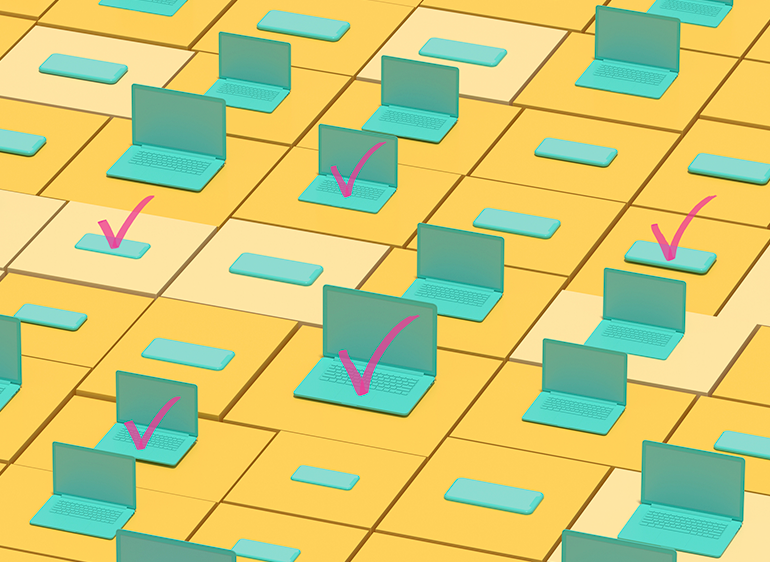
We use cookies to understand how you use our site and to improve your experience, including personalizing content. Learn More . By continuing to use our site, you accept our use of cookies and revised Privacy Policy .
- USC Libraries
- Research Guides
Organizing Your Social Sciences Research Paper
Generative ai and writing.
- Purpose of Guide
- Design Flaws to Avoid
- Independent and Dependent Variables
- Glossary of Research Terms
- Reading Research Effectively
- Narrowing a Topic Idea
- Broadening a Topic Idea
- Extending the Timeliness of a Topic Idea
- Academic Writing Style
- Applying Critical Thinking
- Choosing a Title
- Making an Outline
- Paragraph Development
- Research Process Video Series
- Executive Summary
- The C.A.R.S. Model
- Background Information
- The Research Problem/Question
- Theoretical Framework
- Citation Tracking
- Content Alert Services
- Evaluating Sources
- Primary Sources
- Secondary Sources
- Tiertiary Sources
- Scholarly vs. Popular Publications
- Qualitative Methods
- Quantitative Methods
- Insiderness
- Using Non-Textual Elements
- Limitations of the Study
- Common Grammar Mistakes
- Writing Concisely
- Avoiding Plagiarism
- Footnotes or Endnotes?
- Further Readings
- USC Libraries Tutorials and Other Guides
- Bibliography
Research Writing and Generative AI Large Language Models
A rapidly evolving phenomenon impacting higher education is the availability of generative artificial intelligence systems [such as Chat Generative Pre-trained Transformer or ChatGPT]. These systems have been developed from scanning text from millions of books, web sites, and other sources to enable algorithms within the system to learn patterns in how words and sentences are constructed. This allows the platforms to respond to a broad range of questions and prompts, generate stories, compose essays, create lists, and more. Generative AI systems are not actually thinking or understanding like a human, but they are good at mimicking written text based on what it has learned from the sources of input data used to build and enhance its artificial intelligence algorithms, protocols, and standards.
As such, generative AI systems [a.k.a., “Large Language Models”] have emerged , depending on one’s perspective, as either a threat or an opportunity in how faculty create or modify class assignments and how students approach the task of writing a college-level research paper. We are in the early stages of understanding how LLMs may impact learning outcomes associated with information literacy, i.e., fluency in effectively applying the skills needed to effectively identify, gather, organize, critically evaluate, interpret, and report information. However, before this is fully understood, Large Language Models w ill continue to improve and become more sophisticated, as will academic integrity detection programs used to identify AI generated text in student papers.
When assigned to write a research paper, it is up to your professor if using ChatGTP is permitted or not. Some professors embrace using these systems as part of an in-class writing exercise to help understand their limitations, while others will warn against its use because of their current defects and biases. That said, the future of information seeking using LLMs means that the intellectual spaces associated with research and writing will likely collapse into a single online environment in which students will be able to perform in-depth searches for information connected to the Libraries' many electronic resources.
As LLMs quickly become more sophisticated, here are some potential ways generative artificial intelligence programs could facilitate organizing and writing your social sciences research paper:
- Explore a Topic – develop a research problem related to the questions you have about a general subject of inquiry.
- Formulate Ideas – obtain background information and explore ways to place the research problem within specific contexts .
- Zero in on Specific Research Questions and Related Sub-questions – create a query-based framework for how to investigate the research problem.
- Locate Sources to Answer those Questions – begin the initial search for sources concerning your research questions.
- Obtain Summaries of Sources – build a synopsis of the sources to help determine their relevance to the research questions underpinning the problem.
- Outline and Structure an Argument – present information that assists in formulating an argument or an explanation for a stated position.
- Draft and Iterate on a Final Essay – create a final essay based on a process of repeating the action of text generation on the results of each prior action [i.e., ask follow up questions to build on or clarify initial results].
Despite their power to create text, generative AI systems are far from perfect and their ability to “answer” questions can be misleading, deceiving, or outright false. Described below are some current problems adapted from an essay written by Bernard Marr at Forbes Magazine and reiterated by researchers studying LLMs and writing. These issues focus on problems with using ChatGPT, but they are applicable to any current Large Language Model program .
- Not Connected to the Internet . Although the generative AI systems may appear to possess a significant amount of information, most LLM’s are currently not mining the Internet for that information [note that this is changing quickly. For example, an AI chatbot feature is now embedded into Microsoft’s Bing search engine, but you'll probably need to pay for this feature in the future]. Without a connection to the Internet, LLMs cannot provide real-time information about a topic. As a result, the scope of research is limited and any new developments in a particular field of study will not be included in the responses. In addition, the LLMs can only accept input in text format. Therefore, other forms of knowledge such as videos, web sites, audio recordings, or images, are excluded as part of the inquiry prompts.
- The Time-consuming Consequences of AI Generated Hallucinations . If proofreading AI generated text results in discovering nonsensical information or an invalid list of scholarly sources [e.g., the title of a book is not in the library catalog or found anywhere online], you obviously must correct these errors before handing in your paper. The challenge is that you have to replace nonsensical or false statements with accurate information and you must support any AI generated declarative statements [e.g., "Integrated reading strategies are widely beneficial for children in middle school"] with citations to valid academic research that supports this argument . This requires reviewing the literature to locate real sources and real information, which is time consuming and challenging if you didn't actually compose the text. And, of course, if your professor asks you to show what page in a book or journal article you got the information from to support a generated statement of fact, well, that's a problem. Given this, ChatGPT and other systems should be viewed as a help tool and never a shortcut to actually doing the work of investigating a research problem.
- Trouble Generating Long-form, Structured Content . ChatGPT and other systems are inadequate at producing long-form content that follows a particular structure, format, or narrative flow. The models are capable of creating coherent and grammatically correct text and, as a result, they are currently best suited for generating shorter pieces of content like summaries of topics, bullet point lists, or brief explanations. However, they are poor at creating a comprehensive, coherent, and well-structured college-level research paper.
- Limitations in Handling Multiple Tasks . Generative AI systems perform best when given a single task or objective to focus on. If you ask LLMs to perform multiple tasks at the same time [e.g., a question that includes multiple sub-questions], the models struggle to prioritize them, which will lead to a decrease in the accuracy and reliability of the results.
- Biased Responses . This is important to understand. While ChatGPT and other systems are trained on a large set of text data, that data has not been widely shared so that it can be reviewed and critically analyzed. You can ask the systems what sources they are using, but any responses can not be independently verified. Therefore, it is not possible to identify any hidden biases or prejudices that exist within the data [i.e., it doesn't cite its sources]. This means the LLM may generate responses that are biased, discriminatory, or inappropriate in certain contexts .
- Accuracy Problems or Grammatical Issues . The sensitivity to typographical errors, grammatical errors, and misspellings is currently very limited in LLMs. The models may produce responses that are technically correct, but they may not be entirely accurate in terms of context or relevance. This limitation can be particularly challenging when processing complex or specialized information where accuracy and precision are essential. Given this, never take the information that is generated at face value; always proofread and verify the results!
As they currently exist, ChatGPT and other Large Language Models truly are artificial in their intelligence. They cannot express thoughts, feelings, or other affective constructs that help a reader intimately engage with the author's written words; the output contains text, but the systems are incapable of producing creative expressions or thoughts, such as, conveying the idea of willful deception and other narrative devices that you might find in a poem or song lyric. Although creative devices, such as metaphors, idioms, imagery or subtleties in narrative rhythm, style, or voice, are rarely used in academic writing, it does illustrate that personalizing the way you present your research [e.g., sharing a personal story relating to the significance of the topic or being asked to write a reflective paper ] cannot be generated artificially.
Ethical Considerations
In the end, the ethical choice of whether to use ChatGTP or similar platforms to help write your research paper is up to you; it’s an introspective negotiation between you and your conscience. As noted by Bjork (2023) and others, though, it is important to keep in mind the overarching ethical problems related to the use of LLMs. These include:
- LLMs Do Not Understand the Meaning of Words . Without meaning as a guide, these systems use algorithms that rely on formulating context clues, stylistic structures, writing forms, linguistic patterns, and word frequency in determining how to respond to queries. This functionality means that, by default, LLMs perpetuate dominant modes of writing and language use while minimizing or hiding less common ones. As a result,...
- LLMs Prioritize Standard American English . White English-speaking men have dominated most writing-intensive sectors of the knowledge economy, such as, journalism, law, politics, medicine, academia, and perhaps most importantly, computer programming. As a result, writers and speakers of African American, Indigenous English, and other sociolinguistic dialects that use forms of language with its own grammar, lexicon, slang, and history of resistance within the dominant culture, are penalized and shamed for writing as they speak. The default functionality and outputs of LLMs, therefore, can privilege forms of English writing developed primarily by the dominant culture.
- LLMs Do Not Protect User Privacy . ChatGPT and other platforms record and retain the entire content of your conversations with the systems. This means any information you enter, including personal information or, for example, any documents you ask the systems to revise is retained and cannot be removed. Although the American Data Privacy and Protection Act was being considered within the 117th Congress, there is no federal privacy law that regulates how these for-profit companies can store, use, or possibly sell information entered into their platforms. Given this, it is highly recommended that personal information should never be included in any queries.
NOTE : If your professor allows you to use generative AI programs or you decide on your own to use an LLM for a writing assignment, then this fact should be cited in your research paper, just as any other source of information used to write your paper should be acknowledged. Why? Because unlike grammar or citation tools, such as Grammarly or Citation Machine that correct text you've already written, generative AI programs are creating new content that is not in your own words. Currently, the American Psychological Association (APA), Modern Language Association (MLA) and the Chicago Manual of Style provide recommendations on how to cite generated text.
ANOTHER NOTE : LLMs have significant deficiencies that still require attention to thorough proofreading and source verification, an ability to discern quality information from misleading, false, irrelevant, or even made up information, a capacity to interpret and critically analyze what you have found, and the skills required to extrapolate meaning from the research your have conducted. For help with any or all of these elements of college-level research and writing, you should still contact a librarian for help.
YET ANOTHER NOTE : Researchers are finding early evidence that suggests over-reliance on ChatGPT and other LLM platforms for even the simplest writing task may, over time, undermine confidence in a student's own writing ability. Just like getting better at giving a class presentation or working on a group project, good writing is an acquired skill that can only be improved upon through the act of doing; the more you write, the more comfortable and confident you become expressing your own ideas, opinions, and judgements applied to the problem you have researched. Substituting LLMs with your own voice can inhibit your growth as a writer, so give yourself room to write creatively and with confidence by accepting LLMs as a tool rather than a definitive source of text.
For more information about Generative AI platforms and guidance on their ethical use in an academic setting, review the USC Libraries' Using Generative AI in Research guide for students and faculty. For an introduction to the limitations and potential pitfalls generative AI text generators applied to law, GO HERE .
Introduction to ChatGPT for Library Professionals. Mike Jones and Curtis Fletcher. USC Libraries, Library Forum, May 18, 2023; Aikins, Ross and Albert Kuo. “What Students Said About the Spring of ChatGPT.” Inside Higher Education , September 3, 2023; Baugh, John. “Linguistic Profiling across International Geopolitical Landscapes.” 152 Dædalus (Summer 2023): 167-177; ChatGPT. Library, Wesleyan University; Bjork, Collin. "ChatGPT Threatens Language Diversity." The Conversation , February 9, 2023; Understanding AI Writing Tools and their Uses for Teaching and Learning at UC Berkeley . Center for Teaching & Learning, University of California, Berkeley; Ellis, Amanda R., and Emily Slade. "A New Era of Learning: Considerations for ChatGPT as a Tool to Enhance Statistics and Data Science Education." Journal of Statistics and Data Science Education 31 (2023): 1-10; Ray, Partha Pratim. “ChatGPT: A Comprehensive Review on Background, Applications, Key Challenges, Bias, Ethics, Limitations and Future Scope.” Internet of Things and Cyber-Physical Systems (2023); Uzun, Levent. "ChatGPT and Academic Integrity Concerns: Detecting Artificial Intelligence Generated Content." Language Education and Technology 3, no. 1 (2023); Lund, Brady D. Et al. “ChatGPT and a New Academic Reality: Artificial Intelligence Written Research Papers and the Ethics of the Large Language Models in Scholarly Publishing.” Journal of the Association for Information Science and Technology 74 (February 2023): 570–581; Rasul, Tareq et al. "The Role of ChatGPT in Higher Education: Benefits, Challenges, and Future Research Directions.” Journal of Applied Learning and Teaching 6 (2023); Rudolph, Jürgen, Samson Tan, and Shannon Tan. "ChatGPT: Bullshit Spewer or the End of Traditional Assessments in Higher Education?" Journal of Applied Learning and Teaching 6, no. 1 (2023): 342-362; Marr, Bernard. “The Top 10 Limitations Of ChatGPT.” Forbes (March 3, 2023): https://www.forbes.com/sites/bernardmarr/2023/03/03/the-top-10-limitations-of-chatgpt/?sh=41ae78e8f355; Thinking about ChatGPT? Academic Integrity at UBC, Office of the Provost and Vice-President Academic, University of British Columbia.
- << Previous: Further Readings
- Next: Acknowledgments >>
- Last Updated: May 20, 2024 8:18 AM
- URL: https://libguides.usc.edu/writingguide
Libraries | Research Guides
Using ai tools in your research, about chatgpt and generative ai tools, example: chatgpt and google bard, creating effective prompts.
- Academic Integrity & AI
- Machine Learning for Research
- Add'tl Reading for Librarians & Faculty
Instruction Librarian

What is ChatGPT? How does it work?
ChatGPT 3.5 is a highly sophisticated "large language model," a tool that can generate human-like text based on context and past conversations. ChatGPT is built on large amount of "training material" found on the internet from pre-2021. While it can answer questions, generate images, and write fluently, ChatGPT 3.5:
Is not an encyclopedia of knowledge or a database of information
Is not connected to the internet (unlike some other generative AI tools)
Is not a discrete work by an author - your prompt can generate different information each time
Is not unbiased or neutral, as bias is integrated into the training materials
ChatGPT can, however:
Assist with creative writing tasks, including brainstorming, composing an outline, grammar checks, generate research questions
Provide generic writing or knowledge that may lack specific details or accuracy
Write code, analyze data
There are thousands of large language models, and while some are based on OpenAI, many use a smaller subset or entirely different training material. We will try to keep this page up to date with new tools that add additional features.
- "What is ChatGPT Doing... and Why Does it Work?" by Stephen Wolphram February 14, 2023
- Northwestern access to Microsoft CoPilot (Bing AI, ChatGPT 4) Copilot, Microsoft’s large language model chatbot, is now available to all Northwestern students, faculty, and staff. The AI-powered chatbot is integrated into the Bing search engine and designed to understand complex and natural language queries. The result is summarized responses drawn from across the web, including links to resources. Users can safely use the tool knowing that data is stored securely in Northwestern’s Microsoft tenant, but Microsoft does not use it for product improvement or to train other AI models.
- Claude AI Claude 2's training data includes updates and information from 2022 and early 2023.
- Google Gemini Help with writing, planning, learning and more, "integrated with Google apps you already know and love." Advanced version available for a monthly fee.
- Hugging Face Database of LLMs and AI tools that includes models, datasets, spaces, and categorizes LLMs based on whether they generate images, text, audio, or combinations thereof.
How can you use LLMs?
Fall 2023 examples: Text-based Generative AI tools like ChatGPT can be useful for you when you are brainstorming topic ideas or trying to come up with keywords to use to search on a specific topic. Remember that these tools are not storehouses of knowledge: they are fluency-based text-language generators, and are using their training materials to deliver an answer.
- ChatGPT Limitations: Will generate incorrect information. May produce harmful instructions or biased content. Limited knowledge of world and events after 2021. Generates text based on probability, and will change its answers each time you ask a question.
ChatGPT cannot yet find specific peer-reviewed articles, but it can help you get ideas for how to search for scholarship. It can suggest keywords, search strings, and format citations.
Example : I asked ChatGPT for some keywords to search for sources on the Conquest of Mexico. ChatGPT generated the following list, which is mostly fine until about the 15th option:
Here are some keywords that you could use to research the conquest of Mexico (notes on results in red ):
Hernán Cortés, Aztecs, Montezuma II, Spanish Conquest, Tenochtitlan, Indigenous peoples of Mexico, Conquistadors, New Spain, Mesoamerica, Nahuatl language, Mexica, Tlaxcala, Bernal Diaz del Castillo, La Malinche, (less-than-accurate keywords begin here): Francisco Pizarro (best known for expeditions that led to conquest of Peru - not Mexico), Inca Empire (The primary indigenous empire of Peru), Conquest of Peru, Colonialism (too broad), Imperialism (too broad), Indigenous resistance (too broad)
You can prompt ChatGPT to be more specific in one or more areas, but note that the same will happen - a mix of good ideas, wrong ideas, and overly broad suggestions.
In addition to ChatGPT, there are other AI tools that would be useful, and offer similar assistance with brainstorming.
- Google Bard
I used the same prompt in Google Bard. At this time (fall 2023), its results were a little more accurate.
Here are some keyword phrases you can use to find scholarship about the conquest of Mexico:
- Spanish conquest of Mexico, Hernán Cortés, Aztec Empire, Mexica, Tenochtitlan, La Noche Triste, Malinche, Tlaxcala, Cuauhtemoc, Montezuma II, Bernal Díaz del Castillo, Francisco López de Gómara, Sahagún, Bernardino de
Additionally, Google Bard offered me the following advice: "You can also use more specific phrases, such as:
- The role of disease in the conquest of Mexico, The impact of the conquest of Mexico on the Aztec people, The legacy of the conquest of Mexico
When searching for scholarship, it is more helpful to use databases of work by academic publishers, such as JSTOR, Project MUSE, and EBSCOhost. These databases will allow you to search for articles and books that have been published in scholarly journals and by academic presses.
Here are some additional tips for finding scholarship:
- Read the introductions and conclusions of articles and books to get a sense of the main arguments being made.
- Pay attention to the footnotes and bibliographies to find other sources that you can consult.
- Be aware of the different perspectives - there is often no single "correct" interpretation of an event.
- "The CLEAR path: A framework for enhancing information literacy through prompt engineering" Journal of Academic Librarianship 49(4): July 2023. Article outlines 5 core principles of prompt engineering: Concise, Logical, Explicit, Adaptive, and Reflective.
Generative AI at Northwestern
- Northwestern AI AI@NU is a community of researchers, educators, and students across Northwestern advancing artificial intelligence in theory and practice. Includes research, collaborations, education, news & events.
- Generative AI tools and the Impact on Teaching and Learning The Office of the Provost hosted a webinar on March 8, 2023 titled "Generative AI and Its Impacts on Higher Education: A Conversation with Northwestern Faculty." Recording in clips available!
- Exploring the Pedagogical Possibilities of Generative AI A starting point for exploring the possibilities and avoiding the pitfalls of teaching in a world of AI tools, by the Searle Center for Advancing Learning and Teaching.
- Generative AI in the Northwestern Classroom Northwestern IT Teaching and Learning Technologies and the Searle Center for Advancing Learning and Teaching hosted all Northwestern instructors for a week of workshops on using Gen AI tools in the classroom.
- Generative AI and IT at NU Guidelines on the use of AI from NU IT.
- Next: Academic Integrity & AI >>
- Last Updated: Apr 4, 2024 2:53 PM
- URL: https://libguides.northwestern.edu/ai-tools-research

Generative AI: Supporting AI Literacy, Research, and Publishing
Further reading.
- Generative AI Tools
- Defining Artificial Intelligence
- Considerations for Using Generative AI
- Prompt Engineering
- Evaluating AI Tools & Outputs
- Citing Generative AI Contributions
- Ethics & Artificial Intelligence
- Researching with AI
- Publishing with AI
Interested in Using ChatGPT for Your Research and Creation?
There's been a lot of hype and fear about generative AI tools like ChatGPT. If you're interested in links from other libraries on using these tools for research and creating your projects, check out the following guides!
- Artificial Intelligence (U Calgary)
- Artificial Intelligence Tools for Research and Writing (Texas Tech)
- AI-Based Literature Review Tools (TAMU)
- ChatGPT and other AI tools (UMinn)
- Artificial Intelligence (U Adelaide)
- ChatGPT & AI in Higher Education (FIU)
- Artificial Intelligence (UF)
In November 2022, OpenAI took the tech world by storm when it launched ChatGPT, an artificial intelligence tool that has the ability to generate content by virtue of user prompts. Powered by copious data scraped from the open web and coupled with robust algorithms, ChatGPT prompted a symphony of technology experts, faculty, students, and everyday users who simultaneously embraced and decried this emerging technology.
This guide is designed to help faculty and students learn more about Generative AI, including the myriad Generative AI tools, recommendations for building AI literacy, how to leverage Generative AI ethically within research, and guidelines for publishing using content generated by Generative AI.

ChatGPT self-portrait, as visualized using ChatGPT and DALL-E
- 2023 EDUCAUSE Horizon Report This report profiles key trends and emerging technologies and practices shaping the future of teaching and learning, and envisions a number of scenarios and implications for that future. It is based on the perspectives and expertise of a global panel of leaders from across the higher education landscape. This report includes both Generative AI and AI-enabled applications for predictive and personal learning as technologies that will highly impact higher education.
- Next: Generative AI Tools >>
- Last Updated: May 14, 2024 11:55 AM
- URL: https://guides.libraries.wm.edu/GenerativeAI
- Harvard Library
- Research Guides
- Faculty of Arts & Sciences Libraries
Artificial Intelligence for Research and Scholarship
- Generative AI Tools
- AI for Research
- AI and Citation
- Generative AI Literacy
Applications
- Harvard AI Information
- Related Resources
- Definitions
- Chat GPT (Open AI): Very fast. Best used for content creation and basic coding queries.
- Claude (Anthropic): High content input/output limits. Best used for complex reasoning, creativity and coding.
Images:
- Adobe Firefly
Search and Discovery:
- Gemini (formerly Bard)
Productivity and Literature:
- Research Rabbit
- << Previous: Generative AI Literacy
- Next: Harvard AI Information >>
Except where otherwise noted, this work is subject to a Creative Commons Attribution 4.0 International License , which allows anyone to share and adapt our material as long as proper attribution is given. For details and exceptions, see the Harvard Library Copyright Policy ©2021 Presidents and Fellows of Harvard College.
Artificial Intelligence (Generative) Resources
Ai research tools, additional ai tools.
- How to Craft Prompts
- Research Resources on AI
- Latest News on AI
- Ethics & AI
- Citing Generative AI
- AI, Authorship, & Copyright
- Campus Resources and Policies
About This Table
The resources described in the table represent an incomplete list of tools specifically geared towards exploring and synthesizing research. As generative AI becomes more integrated in online search tools , even the very early stages of research and topic development could incorporate AI. If you have any questions about using these tools for your research, please Email a Librarian .
AI tools for research can help you to discover new sources for your literature review or research assignment. These tools will synthesize information from large databases of scholarly output with the aim of finding the most relevant articles and saving researchers' time. As with our research databases or any other search tool, however, it's important not to rely on one tool for all of your research, as you will risk missing important information on your topic of interest.
Georgetown University's Center for New Designs in Learning and Scholarship (CNDLS) offers a list of additional AI tools with a range of different purposes including visual design, writing, time management, and more.
Ithaka S+R has created a Generative AI Product Tracker which lists tools by their primary purposes and includes pricing information, as well as updates on the tools' features and limitations.
- << Previous: Home
- Next: How to Craft Prompts >>
- Last Updated: May 20, 2024 9:30 AM
- URL: https://guides.library.georgetown.edu/ai


Use of generative AI tools to support learning
- Ethical use
- What to think about
- Using generative AI tools
- Academic reading
- Academic writing and presentation skills
- Supporting your learning
- Selecting the right tool
- Further resources
Part of what a university education teaches is certain academic skills, such as assimilating information, constructing an evidence-based argument and expressing your thoughts in clear, coherent prose.
AI tools cannot replace human critical thinking or the development of scholarly evidence-based arguments and subject knowledge that forms the basis of your university education.
You can make use of generative AI tools (e.g. ChatGPT, Claude, Bing Chat and Google Bard) in developing your academic skills to support your studies. Your ongoing critical appraisal of outputs by reviewing them for accuracy will maximise the potential for AI outputs to be a useful additional tool to support you in your studies.
In some instances academic staff, departments and colleges may give more detailed guidance on how they expect AI tools to be used (or not used) for different tasks or on specific assignments. You should always follow the guidance of your tutors, supervisors and department or faculty.
Ethical use of generative AI tools
Your ethical use of AI tools to support your learning is paramount to ensure you uphold the standards of academic rigour and academic integrity expected of you as a student studying at Oxford.
Students using AI during their studies must learn and practise the same academic skills of note-taking and clear attribution which are safeguards against plagiarism, ensuring clear differentiation of their own work from any text or material derived from any AI tools. Unauthorised use of AI falls under the plagiarism regulations and would be subject to academic penalties in summative assessments.
Where the use of generative AI in preparing work for examination has been authorised by the department, faculty or programme, you should give clear acknowledgment of how it has been used in your work.
The advice in this guide is in line with relevant university policies, which have been updated to provide examples of unauthorised uses of generative AI tools. Guidance on plagiarism is available from the Study skills and training webpages. The policy on the Use of Third Party Proof-readers is also relevant.
Five things to think about when using generative AI tools
- How can generative AI tools be useful in supporting your learning?
- How can generative AI tools be useful in developing your academic skills?
- How (and when) can you draw on AI outputs (knowing that different generative AI tools provide different outputs and that the same tool with the same prompts can produce different outputs)?
- How can you manage the risk of false information and fabrication?
- How can you ensure you maintain good academic practice?
Six tips to keep in mind when using generative AI tools
- Always cross-check AI generated outputs against established sources to verify accuracy and identify erroneous information.
- Give significant contextual information when asking questions or prompts and ask several follow-up questions to refine responses.
- Use personae in your prompts e.g. “I am an undergraduate student who is revising for a first-year calculus exam”.
- Give examples of the kind of responses you want.
- AI tools are not good at calculations so use other established tools, calculators, Excel or Mathematica.
- Do not share sensitive personal data such as financial details or passwords with AI tools. Avoid sharing your own or others intellectual property such as patents, trademarks, designs, sensitive information, or content created by others into any AI tools.
Five ideas for academic reading
Generative AI tools may be useful in supporting you to develop your academic reading skills. However, generative AI may, in some cases, undermine development of your academic reading skills (e.g., asking an AI tool to summarise an article rather than undertaking the task yourself).
Here are five ways you can use AI to support your academic reading, suggestions for how you might provide useful prompts, what some of the limitations are of the AI generated outputs, and how to engage critically with them to augment your learning.
- When reading a paper ask for a table of key terms or outline key points in the paper. Do this yourself before asking AI to do this and compare your terms or points. The AI tool can help you build a cognitive scaffolding of your reading of a paper but you cannot rely on it, so ensure you read the paper yourself.
- Ask AI to generate thought-provoking questions based on article content. You can develop your own understanding of an article by answering the questions asked. You could also use the questions to develop your own questions in relation to the article to deepen your learning.
- Ask AI to translate sections into another language. You can ask AI to translate text into your own language. Be sure to check the accuracy of the translation and that no significant meaning has been lost in translation. This could support your understanding of the article as you review the translation.
- Compare your own summary of a paper with one written by AI. AI can be a useful tool for providing a summary and supporting your reading of academic papers. Comparing your own understanding of the paper with an AI output can be a useful approach to developing your critical reading skills – both by recognising things you may have missed, and by giving you an opportunity to critique the AI output.
- Critically review all AI outputs. Ensure you critically review all AI outputs for accuracy to support your learning, and verify the outputs against other sources, e.g. can you locate all references and are they accurate to the text, and are the definitions correct?
Suggestions of prompts to try:
- You are an expert in [subject] and an educator who is good at giving great explanations to beginners. Make a table of the key concepts needed to understand the content of this paper.
- Give me a list of 20 key terms in this paper and break it into five categories.
- Rephrase this definition as a list of bullet points to help me understand it step by step.
- Make a list of propositions in this text in the format “X is a type of Y”, “W is caused by X”, “A explains B”. Put it into a table with three columns.
- Rephrase this sentence in simpler language that a non-expert can understand. You can break it into multiple sentences, if needed.
Five ideas for academic writing and presentation skills
Generative AI tools can be useful in developing your academic writing skills and providing initial feedback on them, translating between different styles and critiquing writing. AI tools cannot replace the need for you to develop these skills through teaching and independent learning.
Here are five ways you can use AI to develop and get feedback on your academic writing, suggestions for how you might provide useful prompts, what some of the limitations are of the AI generated outputs, and how to engage critically with them to augment your learning.
- Examples of writing in different styles and genres. AI can provide examples of many different written outputs. You may find this useful in identifying different styles of writing, appropriate to different tasks.
- Feedback on your writing. AI can provide rapid feedback on your writing, and this can be helpful for improving it, e.g. in relation to grammar and structure. AI cannot provide feedback nuanced to the rigour and expectations of academic writing in your specific subject so it cannot replace tutors’ feedback grounded in the context of your discipline. Be sure that you proof-read your own work, as this is an essential skill in academic writing, and do not use generative AI tools to make material changes to work in draft.
- Help you get started in writing. AI can be useful in overcoming writer’s block by providing some inspiration or points to consider when you are about to start writing.
- Suggesting ideas for graphics, images and visuals. AI can be useful in suggesting how you might present information in graphics, images and visuals to move beyond text-based presentation of information.
- Critically review all AI outputs. Ensure you critically review all AI outputs for accuracy and verify the outputs against other sources, e.g. can you locate all references and are they accurate to the text, and are the definitions accurate?
- Here is a paragraph I wrote. Rewrite it as a list of bullet points with the key arguments I made.
- I am a student of [subject]. I wrote this as an example of academic writing. Give me feedback on where I could improve. Focus on clarity, academic language and grammar.
- Suggest some practice exercises I can use to improve my weak points.
Five ideas for supporting your learning
Generative AI tools can be useful in supporting your academic studies.
Here are five ways you can use AI to support your studies, suggestions for how you might provide useful prompts and what some of the limitations are of the AI generated outputs. Be sure to verify any AI outputs against other established sources to ensure their accuracy, and cross-check to confirm your understanding.
- Preparing for lectures. You can ask for key concepts related to the topic of the lecture and use the lecture to compare this with your initial understanding.
- Engaging with new or complex topics. You can ask AI for explanations to help you develop a better understanding for yourself. For example, you can ask for alternative explanations of a topic or analogies from different perspectives.
- Organising your notes. Generative AI tools can be used to convert your notes into structures that are easier for you to review. You can ask for a table of key concepts, facts or figures organised by different categories. Timelines, workflow summaries, outlines you can convert into mind maps or mnemonic devices can be generated to help you remember facts. Remember, even if AI looks like it is copying things from your text, it is actually generating the new version from scratch using your text as context, so you must review all outputs for accuracy.
- Enhancing your language study. You can use AI to improve your language learning. You can ask to have a conversation at a certain level on a specific subject, receive feedback on your conversation, or generate sample texts for practice. Always ensure you cross-check the outputs against other sources as outputs in some languages may contain basic grammatical errors. If working with long texts, for languages other than English, the volume of text you can translate or work with will be much shorter. Some languages with non-Latin alphabet (like Arabic) may only be able to fit 1/5th or even less than a language like French. Many languages not well represented on the internet may only work very partially with generative AI.
- Developing your coding skills. You can use AI to develop your coding skills. Write code as you do normally – writing in (functional) chunks and testing the chunks for the right functionality yourself. You could then compare these chunks with a given AI tool. You may find it useful to ask your tutor which AI tools are more appropriate for coding. As with all AI outputs it is not perfect, and any code needs to be run to check that it functions as expected. Common errors are: the code may produce an error message, the description of the code or suggestions for improvement are inaccurate, the code is completely incorrect, or uses features not present in the language or the code generates references to outdated or non-existent libraries. It would not be appropriate to use AI to write a code, or to support the writing of a code, on which your coding skills will be assessed.
- I’m going to a lecture on [subject]. Give me a list of 20 key concepts I need to be prepared for. Break them into five categories and explain how they relate to each other. You are an expert in [subject] and always explain things in a way that is easy to understand for a student at [level]. Make a table of these terms with four columns. 1. Term, 2. Definition, 3. Category, 4. Related terms. Make a series of statements using these terms that will reveal the relationships between them. For example, working memory is a type of ….
- As an expert in [subject]. Here’s an explanation I was given of [concept]. Can you suggest some alternative explanations of [concept] to help me learn. I am a [level] student at university and have already taken [classes in…].
- You are an expert in [subject]. Here is a definition I was given of [term]. Can you give me five concrete examples that illustrate the definition. I want to be able to apply them in practice. For each example, specify exactly how it illustrates the definition.
- Here are my notes from class. Make me flashcards to help me study. Each flashcard should have the term on one side, and a brief explanation on the other. Use my explanations where available. If you have suggestions for alternatives, put them in italics so that I know what is in my words.
- You are a shop assistant in a bakery in Germany. I am a beginner student and I’ve come in to buy some bread. Let’s have a conversation in German. You start by greeting me and asking me what I want. You will pause and give me a chance to answer. Use simple language and always respond even if I say something using the wrong term. Do not use any English until I tell you to. Then make a table of all the things I said with three columns: 1. What I said, 2. What I should have said in correct German. 3. What error I made and how to correct it.
- I want to write code to do [describe task]. As an experienced programmer and a coach of new coders, suggest the best way to help me get started. Suggest the best language, coding environment, and dependencies. My level is [describe level].
Five tips for selecting the right generative AI tools for the task
There is a huge range of generative AI tools available, e.g. Microsoft Bing Chat, ChatGPT, Google Bard, Claude. Some of the tools are freely available and some with costs that bring extra features such as being able to work with large volumes of text.
- You will find it useful to try a few different AI tools and be aware that different tools will give different outputs using the same prompts and a tool will also generate different outputs to the same prompt.
- You will get different responses using the same prompts from the same AI tool. AI outputs are not repeatable, and all tools can generate outputs that can contain inaccuracies and fabrications.
- You could spend a lot of your study time trying out different AI tools. Be careful to manage your exploration of tools alongside managing your time.
- AI tools may draw on data that can be months or years out of date and whilst outputs seem plausible, they may contain errors and/or reflect biases from the original data, e.g. Western perspectives are overly represented.
- AI tools will not replace the need for you to develop your own knowledge and skills as an independent learner.
Further resources
- Guidance on plagiarism
- Policy on the Use of Third Party Proof-readers
Systems & Services
Access Student Self Service
- Student Self Service
- Self Service guide
- Registration guide
- Libraries search
- OXCORT - see TMS
- GSS - see Student Self Service
- The Careers Service
- Oxford University Sport
- Online store
- Gardens, Libraries and Museums
- Researchers Skills Toolkit
- LinkedIn Learning (formerly Lynda.com)
- Access Guide
- Lecture Lists
- Exam Papers (OXAM)
- Oxford Talks
Latest student news

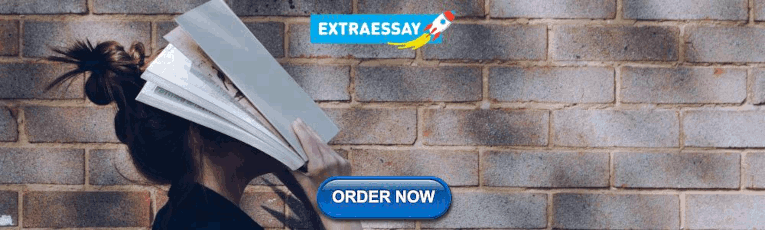
CAN'T FIND WHAT YOU'RE LOOKING FOR?
Try our extensive database of FAQs or submit your own question...
Ask a question
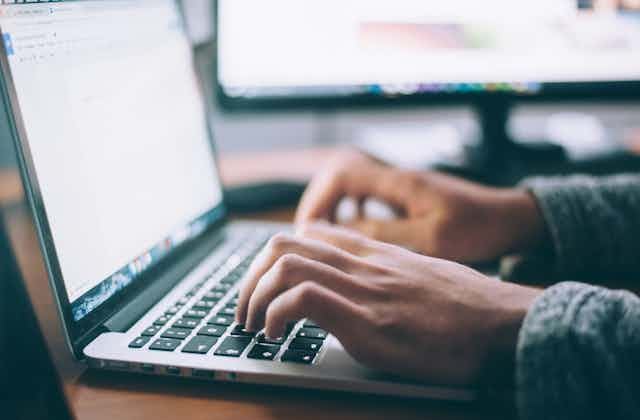
AI-assisted writing is quietly booming in academic journals. Here’s why that’s OK
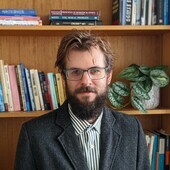
Lecturer in Bioethics, Monash University & Honorary fellow, Melbourne Law School, Monash University
Disclosure statement
Julian Koplin does not work for, consult, own shares in or receive funding from any company or organisation that would benefit from this article, and has disclosed no relevant affiliations beyond their academic appointment.
Monash University provides funding as a founding partner of The Conversation AU.
View all partners
If you search Google Scholar for the phrase “ as an AI language model ”, you’ll find plenty of AI research literature and also some rather suspicious results. For example, one paper on agricultural technology says:
As an AI language model, I don’t have direct access to current research articles or studies. However, I can provide you with an overview of some recent trends and advancements …
Obvious gaffes like this aren’t the only signs that researchers are increasingly turning to generative AI tools when writing up their research. A recent study examined the frequency of certain words in academic writing (such as “commendable”, “meticulously” and “intricate”), and found they became far more common after the launch of ChatGPT – so much so that 1% of all journal articles published in 2023 may have contained AI-generated text.
(Why do AI models overuse these words? There is speculation it’s because they are more common in English as spoken in Nigeria, where key elements of model training often occur.)
The aforementioned study also looks at preliminary data from 2024, which indicates that AI writing assistance is only becoming more common. Is this a crisis for modern scholarship, or a boon for academic productivity?
Who should take credit for AI writing?
Many people are worried by the use of AI in academic papers. Indeed, the practice has been described as “ contaminating ” scholarly literature.
Some argue that using AI output amounts to plagiarism. If your ideas are copy-pasted from ChatGPT, it is questionable whether you really deserve credit for them.
But there are important differences between “plagiarising” text authored by humans and text authored by AI. Those who plagiarise humans’ work receive credit for ideas that ought to have gone to the original author.
By contrast, it is debatable whether AI systems like ChatGPT can have ideas, let alone deserve credit for them. An AI tool is more like your phone’s autocomplete function than a human researcher.
The question of bias
Another worry is that AI outputs might be biased in ways that could seep into the scholarly record. Infamously, older language models tended to portray people who are female, black and/or gay in distinctly unflattering ways, compared with people who are male, white and/or straight.
This kind of bias is less pronounced in the current version of ChatGPT.
However, other studies have found a different kind of bias in ChatGPT and other large language models : a tendency to reflect a left-liberal political ideology.
Any such bias could subtly distort scholarly writing produced using these tools.
The hallucination problem
The most serious worry relates to a well-known limitation of generative AI systems: that they often make serious mistakes.
For example, when I asked ChatGPT-4 to generate an ASCII image of a mushroom, it provided me with the following output.
It then confidently told me I could use this image of a “mushroom” for my own purposes.
These kinds of overconfident mistakes have been referred to as “ AI hallucinations ” and “ AI bullshit ”. While it is easy to spot that the above ASCII image looks nothing like a mushroom (and quite a bit like a snail), it may be much harder to identify any mistakes ChatGPT makes when surveying scientific literature or describing the state of a philosophical debate.
Unlike (most) humans, AI systems are fundamentally unconcerned with the truth of what they say. If used carelessly, their hallucinations could corrupt the scholarly record.
Should AI-produced text be banned?
One response to the rise of text generators has been to ban them outright. For example, Science – one of the world’s most influential academic journals – disallows any use of AI-generated text .
I see two problems with this approach.
The first problem is a practical one: current tools for detecting AI-generated text are highly unreliable. This includes the detector created by ChatGPT’s own developers, which was taken offline after it was found to have only a 26% accuracy rate (and a 9% false positive rate ). Humans also make mistakes when assessing whether something was written by AI.
It is also possible to circumvent AI text detectors. Online communities are actively exploring how to prompt ChatGPT in ways that allow the user to evade detection. Human users can also superficially rewrite AI outputs, effectively scrubbing away the traces of AI (like its overuse of the words “commendable”, “meticulously” and “intricate”).
The second problem is that banning generative AI outright prevents us from realising these technologies’ benefits. Used well, generative AI can boost academic productivity by streamlining the writing process. In this way, it could help further human knowledge. Ideally, we should try to reap these benefits while avoiding the problems.
The problem is poor quality control, not AI
The most serious problem with AI is the risk of introducing unnoticed errors, leading to sloppy scholarship. Instead of banning AI, we should try to ensure that mistaken, implausible or biased claims cannot make it onto the academic record.
After all, humans can also produce writing with serious errors, and mechanisms such as peer review often fail to prevent its publication.
We need to get better at ensuring academic papers are free from serious mistakes, regardless of whether these mistakes are caused by careless use of AI or sloppy human scholarship. Not only is this more achievable than policing AI usage, it will improve the standards of academic research as a whole.
This would be (as ChatGPT might say) a commendable and meticulously intricate solution.
- Artificial intelligence (AI)
- Academic journals
- Academic publishing
- Hallucinations
- Scholarly publishing
- Academic writing
- Large language models
- Generative AI

Lecturer / Senior Lecturer - Marketing

Case Management Specialist

Assistant Editor - 1 year cadetship

Executive Dean, Faculty of Health

Lecturer/Senior Lecturer, Earth System Science (School of Science)
University Libraries University of Nevada, Reno
- Skill Guides
- Subject Guides
Generative AI for Research: AI Research Tools
- Writing Prompts
- AI & Source Evaluation
- AI Research Tools
- AI & Copyright
AI Tools for Finding, Tracking, and Summarizing Research Literature
Many of the tools below can be used in research to assist with finding, tracking, and summarizing sources. These tools are either free or offer free versions that allow users to access a certain number of searches, summaries, or other features on a monthly or other basis.
- Bing Chat Provides responses to questions that contain web references. The search option can be used along with chat to ask questions about the content in individual webpages. For use in the Edge browser.
- Connected Papers Starting with a "seed paper," users can create graphs and lists of articles based on citations in order to visualize topical connections and identify seminal papers. Sources come from the Semantic Scholar database.
- Consensus Provides answers to questions using information drawn from research papers, with associated article summaries, snapshots of articles, and citation help. Sources come from the Semantic Scholar database.
- Elicit Provides answers to questions using information synthesized from scholarly sources, in addition to article summaries. Also allows users to upload and dissect article PDFs. Sources come from the Semantic Scholar database.
- Explainpaper Allows users to upload an article PDF, highlight a passage, and receive an explanation in simpler language along with related web resources. Users can also ask follow-up questions regarding the passage.
- Paper Digest Provides users with lists of sources and summaries in response to a search. Users can also use the Text Rewriter to wikify, paraphrase, questionfy, or summarize a passage.
- Perplexity Acting like a cross between ChatGPT and Google, it returns narrative answers to a query with cited web sources. It also allows users to ask follow-up questions.
- Research Rabbit Starting with a "seed paper," users can explore and visualize connections among papers/authors. Sources come from Semantic Scholar and PubMed.
- Semantic Scholar A free, AI-powered academic search engine with more than 200 million papers covering all disciplines. Allows users to search for research papers, save them to a library, read TLDR (Too Long; Didn't Read) summaries, ask questions of research papers, cite them, find related papers, see citation counts, and set up content alerts.
- << Previous: AI & Source Evaluation
- Next: AI & Copyright >>
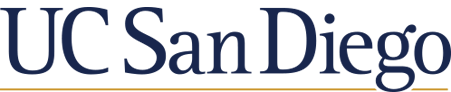
- Research & Collections
- Borrow & Request
- Computing & Technology

Generative Artificial Intelligence: Using Generative AI Tools
- What is Generative Artificial Intelligence?
- Challenges and Possibilities of Generative AI
- AI and Academic Integrity
- Copyright & Legal Considerations
- How to Cite AI
- Using Generative AI Tools
- Additional Resources
Academic Integrity Reminder
Utilizing GenAI tools effectively involves choosing the right tool for the task at hand, crafting prompts that generate the output you want/expect, AND using the tools ethically and with integrity. While this section of the guide focuses on crafting prompts and evaluating and choosing specific tools, be sure to fact-check any AI-generated content and sources you plan to use in the work you share with others and/or publish. Students should only use these tools within the guidelines established by instructors and the Academic Integrity Office . Employees should follow guidelines established by their employer, whether that is UC San Diego or another entity. Make sure to document/cite the parts of the work created by the GenAI tool. Do not include anyone's Personal Identifiable Information (PII) in your prompts, whether it is your own or someone else's.
- Academic Integrity Office - Artificial Intelligence & Academic Integrity
- AI at UC San Diego: Guidance for Staff
- UC Presidential Working Group on Artificial Intelligence Final Report (October 2021): Recommendations to Guide the University of California’s Artificial Intelligence Strategy
- AI Essentials at UC San Diego (UC Learning Training) Interested in getting up to speed on Generative AI terms and techniques? Take the following training now available in UC Learning. Course Title: AI Essentials at UC San Diego Duration: Approximately 20-40 minutes Access: UCSD Staff
- AI Essentials for All Audiences UC San Diego training module reframed for a general audience, no login required
- Everyday I AI - Short Example Uses of AI video playlist (UCSD OSI) A collection of example uses and tips for generative AI tools. This series uses the UC San Diego tool "TritonGPT" but the prompting tips and examples apply to many AI tools.
How to Write Prompts
Learn Prompting defines "prompt engineering" as "the process of communicating effectively with an AI to achieve desired results ."
Using GenAI tools effectively requires that the user know the right questions to ask, and how to phrase them for the best results. Vague or generic questions generate vague or generic results. (In other words, garbage in, garbage out.)
Tips for crafting prompts to get the best results from chatbots:
- R eflective
- Be specific about the format of the output - number of words or paragraphs, writing style or tone, reading level, formatting such as bullet points, a table, html, css, etc.
- Instruct the chatbot to take on an expert role: for example, "act as a mathematician" or "take on the role of a professional news blogger" or "I want you to act as a Linux terminal."
- Provide the chatbot with examples or a reference text.
- Split complex tasks into simpler subtasks.
- Beware hallucinations, or the chatbot confidently stating incorrect or made up information. If you are unfamiliar with the topic, check the chatbot's work. Treat the chatbot like a brand new assistant who is eager to please but doesn't know what they don't know. ChatGPT and other chatbots are notorious for making up citations to sources that simply do not exist. Microsoft Copilot (Bing Chat), Google Search Generative Experience, or Perplexity may be better choices because they provide links to the websites they claim as information sources .
- Learn Prompting online course/ebook The definitive guide to prompt engineering. Extensive but easy-to-read online guide to crafting prompts. Each chapter is only a few paragraphs and includes illustrations and hands-on examples. Great as a reference - does not need to be read all at once or sequentially.
- Brex's Prompt Engineering Guide
- AI-Based Literature Review Tools: Approaches to Write or Fine Tune Prompts (Texas A&M University Libraries guide) Thorough overview with examples of different types of prompts. Serves as a good one-page cheatsheet to Learn Prompting . more... less... -Action prompts -5W+H prompts -Prompts for a specific context -Visual generation prompts -Prompts that pose multiple queries -Act-as-an-expert prompts -Style prompts -Prompts that incorporate a quotation -Relationship prompts -Dividing a complex concept into subset prompts -Summarize/synthesize a reference prompts -Zero shot, one shot & few shot prompts
- GPT best practices (OpenAI) OpenAI's guide to strategies and tactics for getting better results from GPTs offers six strategies for getting better results more... less... 1. Write clear instructions 2. Provide reference text 3. Split complex tasks into simpler subtasks 4. Give GPTs time to "think" 5. Use external tools (feed them the output of those tools) 6. Test changes systematically
- Quick Guide: AI Prompt Engineering infographic (by Jeri Hurd, Branksome Hall Asia)
- Get the Best From ChatGPT With These Golden Prompts (The New York Times: On Tech: A.I.)
- Generative AI Is Only as Good as the Prompt You Give It (Inside Higher Ed, 4/26/2023)
- Prompt Engineering for ChatGPT (Coursera course)
- Awesome ChatGPT Prompts (GitHub repository) Collection of pre-written prompts.
- AI Prompt Generator (Coefficient) Generates a ChatGPT prompt based on choices in dropdown menus.
Comparing the AI Chatbots
Many tech reviewers have published comparisons between popular AI chatbots, but the landscape changes rapidly.
This guide primarily features free and freemium tools. We recommend experimenting with these options before investing in any paid subscriptions. Unless you need a feature that is unique to another tool, we also highly recommend choosing chatbots that connect to the internet and provide links so that you can verify their claims.
Microsoft Copilot (also known as Bing)
- Microsoft Copilot (Bing Chat) Chatbot powered by Microsoft's proprietary Prometheus , which combines GPT-4 (which also powers the subscription version of ChatGPT) and Bing web search. Copilot's Designer is powered by DALL-E 3. Copilot can help users with a variety of tasks, such as writing code, generating text, creating graphic art from text descriptions, and finding information on the web. As of early 2024, Copilot is considered the best free alternative to a paid ChatGPT subscription.
- What is Copilot, and how can you use it? (Microsoft Guide)
- Copilot: AI prompt writing 101 (Microsoft Guide)
- Enhance online research with Copilot’s AI-powered search features (Microsoft Guide)
- Art and creative writing Copilot AI showcase (Microsoft Guide)
- What can Copilot’s AI text generator do?
- Microsoft Copilot "secret" prompt generator for Microsoft Edge
- Copilot Cheat Sheet (Formerly Bing Chat): Complete Guide for 2024 (Tech Republic, 1/25/2024)
Google Gemini (formerly known as Bard)
- Google Gemini
- Gemini Apps Help: Use the Gemini web app How to start a conversation and edit prompts.
- Google Gemini Cheat Sheet (Formerly Google Bard): What Is Google Gemini, and How Does It Work? (Tech Republic, 2/22/2024)
- Google's Gemini Advanced: Tasting Notes and Implications (One Useful Thing, 2/8/2024)
- Perplexity Perplexity AI is an AI-powered search engine and chatbot that is designed to provide accurate and comprehensive answers to user queries. By searching the web in real-time, it offers up-to-date information on a wide range of topics. It is particularly notable as the only chatbot with an "Academic" Focus setting.
- Getting Started: What you need to know to get started with Perplexity
- Prompting Tips and Examples on Perplexity
- Claude Claude is an "AI assistant created by Anthropic to be helpful, harmless, and honest." Claude is known for having a particularly large memory and can summarize large documents or multiple pdfs. Claude currently cannot search the internet or provide links to its sources.
Meta's popular, open source Llama model powers many different tools. Meta AI's assistant is available as a stand-alone app and infused throughout Meta's products like Facebook and Instagram. The assistant in Facebook, Instagram, and WhatsApp messengers is designed to provide personalized and empathetic responses, understand context and nuances of human conversations, and offer creative solutions and ideas.
- Use Meta AI in chats on Messenger (Facebook Help Center)
- Introducing new AI experiences from Meta (Meta)
- About AIs from Meta (WhatsApp)
- Poe Poe by Quora is a unique platform that gives users access to multiple AI chatbots in one place. Includes its own GPT-3.5-turbo assistant, Claude, GPTs, LLaMa and Code LLaMa, and Gemini, Gemma, and PaLM, Mistral, Mixtral, and large collection of other models. Also includes a variety of specialized bots and image generators, including StableDiffusionXL and Playground.
- TritonGPT TritonGPT is a UC San Diego-specific chatbot powered by Llama 2, an open-source model that is optimized for dialogue. TritonGPT is hosted at the San Diego Supercomputer Center in a secure environment and is built to answer a range of questions about UC San Diego. TritonGPT is in pilot mode and only available to users who complete the AI Essentials at UC San Diego Training and sign up via the interest form.
- Prompt Engineering in TritonGPT (Canva slides) more... less... - Use the START model to craft basic prompts: S ituation T ask A ction R eferences T one - Use the EDIT model to refine prompts E xpand D irect I nvestigate T rim - Use CAPITALIZATION to emphasize parts of the prompt that have greater weight. - Use the word ONLY to focus on key elements of your prompt. -- The model understands first, second, and last but may not understand positionality like third or fifth. The model is trained for language and is less reliable for mathematical prompts in general.
Test other chatbots
- Groq Groq is a powerful AI chatbot that utilizes an LPU Inference Engine and is known for its speedy responses. Utilizes Gemma, Llama, and Mixtral models.
- Hugging Chat Try new and popular open source models hosted on Hugging Face
- Perplexity Labs Playground Try an ever-growing variety of open source models hosted by Perplexity
- Awesome-LLM GitHub repo of featuring curated list of papers about large language models, frameworks for LLM training, tools to deploy LLM, courses and tutorials about LLM and all publicly available LLM checkpoints and APIs:
- Chatbot Arena Created by researchers at UC San Diego and UC Berkeley, Chatbot Arena is a benchmark platform for large language models (LLMs) that features anonymous, randomized battles in a crowdsourced manner. more... less... Chatbot Arena: Benchmarking LLMs in the Wild with Elo Ratings by: Lianmin Zheng*, Ying Sheng*, Wei-Lin Chiang, Hao Zhang, Joseph E. Gonzalez, Ion Stoica, May 03, 2023
- Open LLM Leaderboard The Open LLM Leaderboard aims to track, rank and evaluate LLMs and chatbots as they are released. Anyone from the community can submit a model for automated evaluation on the GPU cluster.
If you are working on a project that requires citations, you want to confirm the generative AI chatbot's information sources, or you are seeking information newer than would be included in the chatbot's large language model (LLM), you will want to use a generative AI tool with internet search capabilities like Microsoft Copilot (Bing Chat) or Google SGE (Search Generative Experience).
- Google SGE (Search Generative Experience) Google Search Generative Experience (SGE) is an AI-driven feature that generates interactive AI snippets to provide users with accurate and relevant answers to their queries, along with links to corroborating sources AI generated summary. Also provides the standard Google search results links below the AI summary. Join the waitlist in Google Labs.
- You.com "AI chatbot to search the web"
Citation & Research Assistance Tools
- Consensus Consensus is a search engine that uses AI to extract and distill findings from peer-reviewed sources. Subject matter coverage ranges from medical research and physics to social sciences and economics. Utilizes the Semantic Scholar dataset.
- Elicit Helps automate research workflows, like creating literature reviews, brainstorming, summarization, and text classification. Utilizes the Semantic Scholar dataset. Moving to a paid-only model.
- Research Rabbit Provide a seed article that allows you to retrieve recommended papers, visualize networks of papers and authors, and get alerts about additional relevant research. It integrates with Zotero and allows for collaborative research sharing.
- Semantic Scholar A project at the Allen Institute for AI, it indexes over 200 million academic papers sourced from publisher partnerships, data providers, and web crawls.

- ChatGPT and Bing Chat Generative AI Legal Research Guide (University of Arizona Law Library)
Summary Tools
- Don’t Use A.I. to Cheat in School. It’s Better for Studying.(New York Times On Tech: AI)
- ChatPDF "Chat with any PDF"
- Docalysis Docalysis is an AI tool that allows you to chat with your PDF files and get answers to your questions within seconds.
- Explainpaper "Upload a paper, highlight confusing text, get an explanation."
- Glasp YouTube Summary with ChatGPT Generate ChatGPT summaries of YouTube video transcripts. Extension for Chrome and Safari.
- Google NotebookLM Create notebooks of up to 20 sources including text, Google Docs, and pdfs and then ask them questions. Requires a personal Google account and activation via Google Labs .
- Humata "ChatGPT for all your files"
Text Generators & Writing Assistants
- Using AI Language Models (Hallmark University Library) Examples of prompts to improve your outline, vocabulary, grammar, and overall essay.
GPT-powered tools
- Copilot (Bing) Compose Embedded in Microsoft Edge browser in Copilot settings. May not be available for enterprise accounts.
- Canva Magic Write Powered by OpenAI's GPT-3. Free tier only offers a one-time allotment of 25 Magic Write generated documents.
- Using Canva Magic Design to create a presentation
Google-powered tools
- Google Gemini for Workspace (formerly Duet AI) Embedded in Google Workspace apps such as Google Docs and Gmail. Users with personal accounts can apply to join the tester program in Google Labs.
- Get started with Google Workspace Labs Google Workspace Labs is a trusted tester program for personal account users to try new AI features. Activates features in Google Docs, Gmail, Google Slides, Google Sheets, Google Meet, Google Drive, Keep
- Gemini for Google Workspace Help Center
- Gemini for Google Workspace cheat sheet How use use Help Me Write in Google Docs and Gmail; Help Me Organize in Google Sheets; and image creation in Google Slides.
Other AI-powered tools to improve your writing. Students take note: please make sure that you are using these in accordance with your instructor's rules on use of generative AI and the Academic Integrity Office's guidelines. If you have questions or concerns, please consult your instructor first.
- DeepL Write: AI-powered writing companion "Type or paste text to see ideas for improvement." DeepL Write is an AI-powered writing companion that can help you write clearly, precisely, and with ease in English or German.
- Hemingway Editor "It's like a spellchecker, but for style." Hemingway Editor is a writing app that helps you improve your writing by highlighting and suggesting corrections for common issues, such as long sentences, passive voice, and grammar errors.
- Grammarly "Work with an AI writing partner that helps you find the words you need"
- Quillbot QuillBot was created as a full-sentence thesaurus to help students and professionals write more confidently and quickly.
- WordTune Wordtune is an AI-powered writing tool that helps you write better and faster by suggesting alternative ways to express your ideas, tone, and voice.
Image and Media Generators
Most AI image generating sites operate on a freemium model where users are allotted a set number of credits. For many tools, the credits reset daily or monthly, but some tools provide only a one-time allotment. Users who pay for accounts can generally unlock more credits and/or premium features. We do not recommend paying for any sites without further research.
- How to Use A.I. to Edit and Generate Stunning Photos (The New York Times: On Tech: A.I.)
- How to create effective prompts for AI image generation
- How to Write AI Art Prompts [Examples + Templates] (HootSuite, 7/5/2023)
OpenAI / DALL·E
DALL-E is OpenAI's text-to-image model. DALL-E 3 is included in a ChatGPT Plus subscription or available for free via Copilot Design.
- Copilot Designer (Bing Image Generator) Text-to-image generator powered by OpenAI's DALL·E 3. Free with a Microsoft account. Bing suggests the following template to get started: adjective + noun + verb + image/art style. Also see Image prompting 101 (Microsoft Guide)
- DALL·E 2 OpenAI's freemium text-to-image generator. No longer free for new subscribers. Copilot Designer / Bing Image Creator is a free alternative.
- Adobe Firefly AI tools for text to image, generative fill, text effects, generative recolor, 3D to image, and extend image (change the aspect ratio). Free plan provides 25 credits per month. Also included in Adobe Creative Cloud products including Express, Illustrator, Photoshop, and Stock.
- Imagine with Meta AI Meta's image generation site that provides access to their Emu image-synthesis model, which was trained on 1.1 billion publicly visible Facebook and Instagram images Requires a Meta account, which can be connected to a Facebook or Instagram account. Also available via Messenger in messages by typing /imagine and then the text-to-image prompt.
Stable Diffusion and SD-powered tools
Stable Diffusion is a family of popular open source text-to-image models created by Stability AI. Anyone can download and run the code on their own PC, and a plethora of generative AI sites have incorporated Stable Diffusion code into their tools in both its original form and modified through low-ranking adaptation (LoRA), an AI training technique that helps to fine-tune the models.
- Leonardo.AI Robust tool that bills itself as a free alternative to Midjourney. Includes an assortment of both general and finely-tuned Leonardo, Stable Diffusion, and member-created models, each crafted for different types of content generation. Free users have a daily allowance of 150 Tokens. How to Write Great Text-to-Image Prompts
- Mage.Space Mage.Space AI is an AI art generator that offers several Stable Diffusion models and various features like text-to-image, image-to-image, and image-to-GIF conversions. The tool has a slightly complex interface, but it offers various image creation and editing features. The free plan includes Unlimited creations with SDXL, Stable Diffusion v1.5, or Stable Diffusion v2.1. Paid plans unlock additional models.
- NightCafe NightCafe bills itself as an AI Art Generator with a focus on community and fun. Features SDXL, SD1.5, and other models plus a large collection of community fine-tuned models. Users can log in and collect 5 free credits per day. Users can also participate in AI Art Challenges and interact with a community of other AI Art enthusiasts.
- Playground AI Playground AI is another popular and user-friendly site for generating images with Stable Diffusion XL (SDXL) and Playground's own models. Users can generate 100 free images per day.
- Dezgo Dezgo is a free site where users can generate an image from a text description, powered by Stable Diffusion models. You can use the free version without registering but there are limitations such as rate limits, image resolution and image quality (capped sampling steps).
- Civitai Civitai is a free, open-source, generative AI platform that allows users to share and use AI image-generating models.
- TensorArt Site where users can share and use AI image-generating models, similar to Civitai. Users can generate 100 free images per day.
- Lexica Lexica is an image generation tool that utilizes Stable Diffusion 1.5 and Lexica's own Aperture models. Also includes a search engine to search images that have already been generated.
- Stable Diffusion Online Despite its name, Stable Diffusion Online is not associated with StabilityAI and some users have raised questions about the site, especially its paid version. Has a nice interface for choosing artistic style. 10 free credits reset daily. more... less... Image styles: Cinematic, Analog Film, Animation, Comic Book, Craft Clay, Fantasy Art, Line Art, Cyberpunk, Photograph, Pixel Art, Graffiti, Game GTA, Papercraft Paper, 3D Character, Baroque, Caricature, Colored Pencil, Doodle Art, Futurism, Impressionism, Rococo, Sketch, Surrealism, Sticker Designs
- Canva: Text to Image Powered by Stable Diffusion. Free Canva users have 50 lifetime credits, while Canva Pro members have 500 credits per month.
- Canva: Magic Design Canva tool to automatically generate templates based on uploaded image or entered text prompt. Canva can also generate a 10-slide presentation deck, complete with images, based on entered text.
- StabilityAI's Stable Diffusion 2.1 Demo (Hugging Face) Free, no-frills version of Stable Diffusion from its creator.
- DreamStudio by StabilityAI Official web app from the creator of Stable Diffusion. Users are given 25 free credits (~125 images), which do not renew, and then purchase additional credits as needed. DreamStudio prompt guide more... less... Styles: Enhance, Anime, Photographic, Digital art, Comic book, Fantasy art, Analog film, Neon punk, Isometric, Low poly, Origami, Line art, Craft clay, Cinematic, 3D model, Pixel art
Popular free tools
- Craiyon Completely free text-to-image generator. Formerly called DALL·E mini but never associated with DALL·E or OpenAI. Designed by developer Boris Dayma.
- Deep Dream Generator AI image generator with its own models. Free users can amass 20 energy points, which regenerate 1-2 per hour. Standard images use 4 energy points. Free users are limited to 3 images generated with PRO models per day.
- Dream by WOMBO Text-to-image generator. Free version generates one image at a time; paid version generates four options. Images are editable with additional text prompts.
- Ideogram Ideogram is an AI image generator that claims to have solved a problem that plagues AI image generators -- the ability to generate coherent text within an image.
- Modyfi Modyfi is a collaborative, AI-native, web-based design and image creation platform that combines the design and image making tools with AI-powered creative technology
- NeuralBlender NeuralBlender is currently revamping with some unique AI features. During this transition period payment is disabled and NeuralBlender can be used for free.
- Midjourney Midjourney is a self-funded, independent research lab whose text-to-image generator creates the highest quality digital art. Currently considered one of the best, if not the best, image generator. There is currently no free tier for new users and prompts must be entered via Discord. 8 Tips For Writing Effective Midjourney Prompts .
Music Generators
- AIVA AIVA (Artificial Intelligence Virtual Artist) is an AI-powered music generator that composes original and personalized soundtracks for various projects, such as videos, games, and movies. By leveraging advanced algorithms and deep learning, AIVA can create music in different styles and genres, offering users a range of presets and the ability to edit soundtracks.
- MusicGen This is the demo for MusicGen, Meta's music generating tool.
- Soundful Soundful is an AI-powered music generator platform that enables creators to produce unique, royalty-free tracks with just a click of a button. The software uses complex algorithms to create customizable compositions across various genres and moods, catering to musicians, content creators, and casual listeners alike.
- Stable Audio Stability AI's music creator
Transcription & Captioning
- Transcribe in Office 365/Word
- Google Docs Voice Typing Tools > Voice typing
- Kaltura/MediaSpace
Code Generation
- Awesome-Code-AI A crowd-sourced list of AI-assisted coding tools.
- Quickstart for GitHub Copilot GitHub Copilot is free to use for verified students, teachers, and maintainers of popular open source projects. If you are not a student, teacher, or maintainer of a popular open source project, you can try GitHub Copilot for free with a one-time 30-day trial.
- GitHub Student Developer Pack
- Open AI Codex
- Six tips for better coding with ChatGPT (Nature, 6/3/2023)
AI-powered Tools Directories
- AI Tools Directory Includes AI Tools for Librarians tab. Complements Laura Abbene's AI Powered Tools & Resources document.
- Futurepedia AI Tools Directory
- The Rundown AI's Tools Database Companion to The Rundown AI newsletter.
- There's an AI for That (sponsored by the TeamSmart ChatGPT Chrome Extension)
- All Things AI "A curated directory of the latest AI tools & services"
- AI Valley "The Latest Source of AI Tools & Prompts"
- << Previous: How to Cite AI
- Next: Additional Resources >>
- Last Updated: May 17, 2024 11:58 AM
- URL: https://ucsd.libguides.com/AI
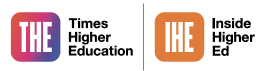
The (AI) sky isn’t falling
Students using generative AI to write their essays is a problem, but it isn’t a crisis, writes Christopher Hallenbrook. We have the tools to tackle the issue of artificial intelligence

Christopher R. Hallenbrook

You may also like
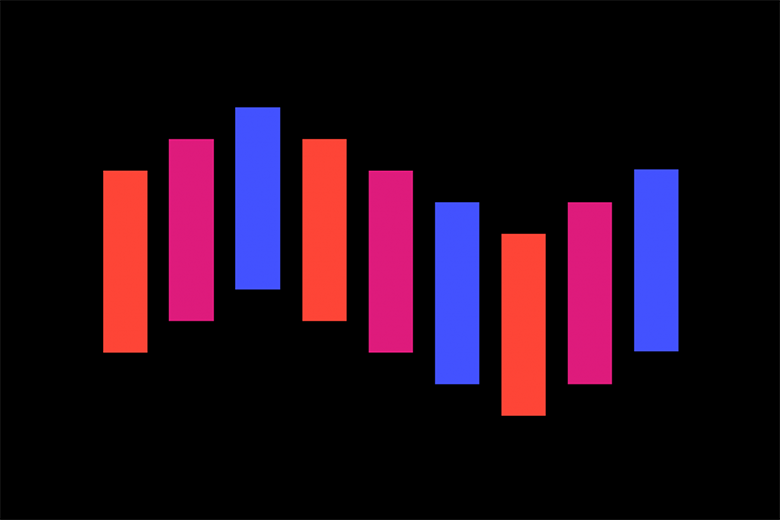
Popular resources
.css-1txxx8u{overflow:hidden;max-height:81px;text-indent:0px;} Rather than restrict the use of AI, embrace the challenge
Emotions and learning: what role do emotions play in how and why students learn, leveraging llms to assess soft skills in lifelong learning, how hard can it be testing ai detection tools, a diy guide to starting your own journal.
In January, the literary world was rocked by the news that novelist Rie Qudan had used ChatGPT to write 5 per cent of her novel that won Japan’s prestigious Akutagawa Prize. The consternation over this revelation mirrored the conversations that have been taking place in academia since ChatGPT was launched in late 2022. Discussions and academic essays since that time have consistently spoken of a new wave of cheating on campus, one we are powerless to prevent.
While this reaction is understandable, I disagree with it. Students using AI to write their essays is a problem, but it isn’t a crisis. We have the tools to tackle the issue.
AI is easy to spot
In most cases AI writing can be easily recognised. If you ask multipart questions, as I do, ChatGPT defaults to using section headings for each component. When I grade a paper that has six section headings in a three- to five-page paper (something I have experienced), I see a red flag. ChatGPT’s vocabulary reinforces this impression. Its word choice does not align with how most undergraduates write. I’ve never seen a student call Publius a “collective pseudonym” in a paper about The Federalist Papers , but ChatGPT frequently does. AI is quick to discuss the “ethical foundations of governance”, “intrinsic equilibrium” and other terms that are rare in undergraduate writing if you haven’t used the terms in class. Certainly, some students do use such vocabulary.
One must be careful and know one’s students. In-class discussions and short response papers can help you get a feel for how your students talk and write. Worst-case scenario, a one-to-one discussion of the paper with the student goes a long way. I’ve asked students to explain what they meant by a certain term. The answer “I don’t know” tells you what you need to know about whether or not they used AI.
- Resource collection: AI transformers like ChatGPT are here, so what next?
- Rather than restrict the use of AI, let’s embrace the challenge it offers
- AI did not disturb assessment – it just made our mistakes visible
Even when you can’t identify AI writing so readily, you will likely fail the paper on its merits anyway. I’ve found ChatGPT will frequently engage with the topic but will write around the question. The answer is related to what I asked about but doesn’t answer my question. By missing the question, making its points in brief and not using the textual evidence that I instruct students to include (but I don’t put that instruction in the question itself), ChatGPT produces an essay that omits the most essential elements that I grade on. So even if I miss that the essay was AI generated, I’m still going to give it a poor grade.
The summary is ‘dead and buried’
Careful consideration and structuring of essay prompts also reduce the risk of students getting AI-written work past you. A simple summary of concepts is easy for ChatGPT. Even deep questions of political theory have enough written on them for ChatGPT to rapidly produce a quality summary. Summaries were never the most pedagogically sound take-home essay assignment; now they are dead and buried.
Creativity in how we ask students to analyse and apply concepts makes it much harder for ChatGPT to answer our questions. When I was an undergraduate student, my mentor framed all his questions as “in what manner and to what extent” can something be said to be true. That framework invites nuance, forces students to define their terms and can be used to create less-written-about topics.
Similarly, when responding to prompts asking about theories of democratic representation, ChatGPT can effectively summarise the beliefs of Publius, the anti-federalist Brutus or Malcolm X on the nature of representation, but it struggles to answer: “Can Professor Hallenbrook properly represent Carson? Why or why not? Draw on the ideas of thinkers we have read in class to justify your answer.” In fact, it doesn’t always recognise that by “Carson”, I am referring to the city where I teach, not a person. By not specifying which thinkers, ChatGPT has to pick its own and in my practice runs with this prompt, it used almost exclusively thinkers I had not taught in my American political thought class.
Ask ChatGPT first, then set the essay topic
I select my phrasing after putting different versions of the question through ChatGPT. Running your prompt through ChatGPT before you assign it will both let you know if you’ve successfully created a question that the generative AI will struggle with and give you a feel for the tells in its approach that will let you know if a student tries to use it. I’d recommend running the prompt multiple times to see different versions of an AI answer and make note of the tells. It is a touch more prep time but totally worth it. After all, we should be continually re-examining our prompts anyway.
So, yes, ChatGPT is a potential problem. But it is not insurmountable. As with plagiarism, some uses may escape our detection. But through attention to detail and careful design of our assignments, we can make it harder for students to use ChatGPT to write their papers effectively and easier to spot it when they do.
Christopher R. Hallenbrook is assistant professor of political science and chair of the general education committee at California State University, Dominguez Hills.
If you would like advice and insight from academics and university staff delivered direct to your inbox each week, sign up for the Campus newsletter .
Rather than restrict the use of AI, embrace the challenge
Let’s think about assessments and ai in a different way, how students’ genai skills affect assignment instructions, how not to land a job in academia, contextual learning: linking learning to the real world, three steps to unearth the hidden curriculum of networking.
Register for free
and unlock a host of features on the THE site
10 Powerful AI Tools for Academic Research
- Serra Ardem
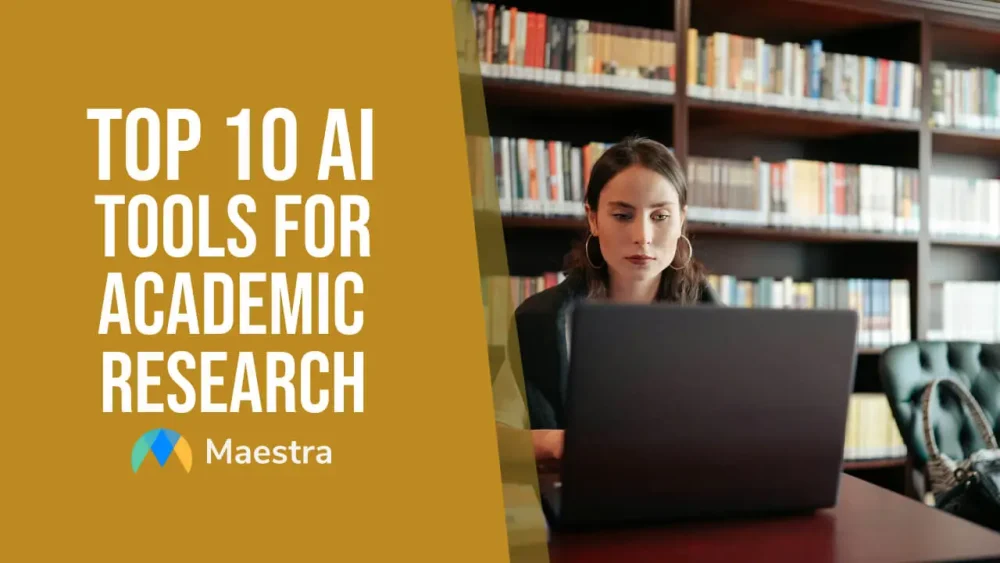
AI is no longer science fiction, but a powerful ally in the academic realm. With AI by their side, researchers can free themselves from the burden of tedious tasks, and push the boundaries of knowledge. However, they must use AI carefully and ethically, as these practices introduce new considerations regarding data integrity, bias mitigation, and the preservation of academic rigor.
In this blog, we will:
- Highlight the increasing role of AI in academic research
- List 10 best AI tools for academic research, with a focus on each one’s strengths
- Share 5 best practices on how to use AI tools for academic research
Let’s dig in…
The Role of AI in Academic Research
AI tools for academic research hold immense potential, as they can analyze massive datasets and identify complex patterns. These tools can assist in generating new research questions and hypotheses, navigate mountains of academic literature to find relevant information, and automate tedious tasks like data entry.
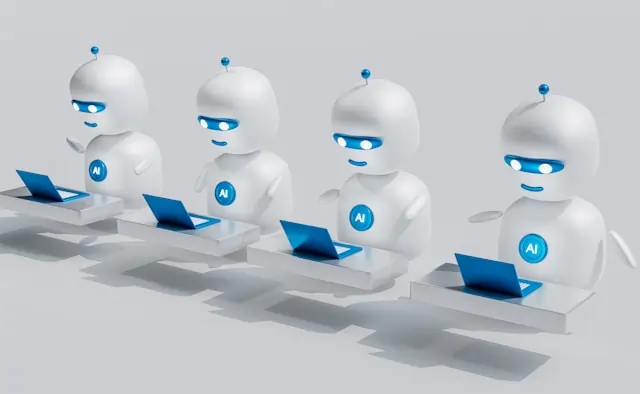
Let’s take a look at the benefits AI tools offer for academic research:
- Supercharged literature reviews: AI can sift through vast amounts of academic literature, and pinpoint relevant studies with far greater speed and accuracy than manual searches.
- Accelerated data analysis: AI tools can rapidly analyze large datasets and uncover intricate insights that might otherwise be overlooked, or time-consuming to identify manually.
- Enhanced research quality: Helping with grammar checking, citation formatting, and data visualization, AI tools can lead to a more polished and impactful final product.
- Automation of repetitive tasks: By automating routine tasks, AI can save researchers time and effort, allowing them to focus on more intellectually demanding tasks of their research.
- Predictive modeling and forecasting: AI algorithms can develop predictive models and forecasts, aiding researchers in making informed decisions and projections in various fields.
- Cross-disciplinary collaboration: AI fosters collaboration between researchers from different disciplines by facilitating communication through shared data analysis and interpretation.
Now let’s move on to our list of 10 powerful AI tools for academic research, which you can refer to for a streamlined, refined workflow. From formulating research questions to organizing findings, these tools can offer solutions for every step of your research.
1. HyperWrite
For: hypothesis generation
HyperWrite’s Research Hypothesis Generator is perfect for students and academic researchers who want to formulate clear and concise hypotheses. All you have to do is enter your research topic and objectives into the provided fields, and then the tool will let its AI generate a testable hypothesis. You can review the generated hypothesis, make any necessary edits, and use it to guide your research process.
Pricing: You can have a limited free trial, but need to choose at least the Premium Plan for additional access. See more on pricing here .
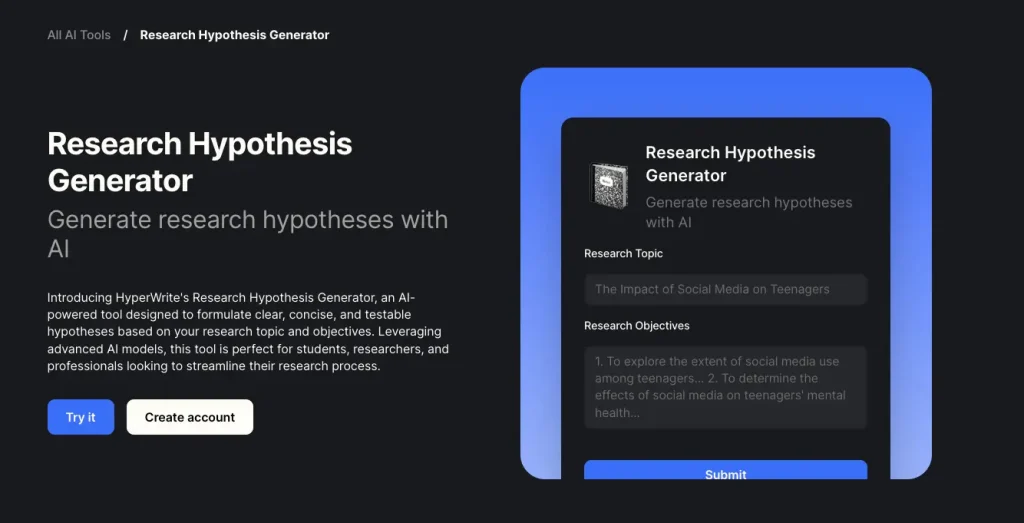
2. Semantic Scholar
For: literature review and management
With over 200 million academic papers sourced, Semantic Scholar is one of the best AI tools for literature review. Mainly, it helps researchers to understand a paper at a glance. You can scan papers faster with the TLDRs (Too Long; Didn’t Read), or generate your own questions about the paper for the AI to answer. You can also organize papers in your own library, and get AI-powered paper recommendations for further research.
Pricing: free
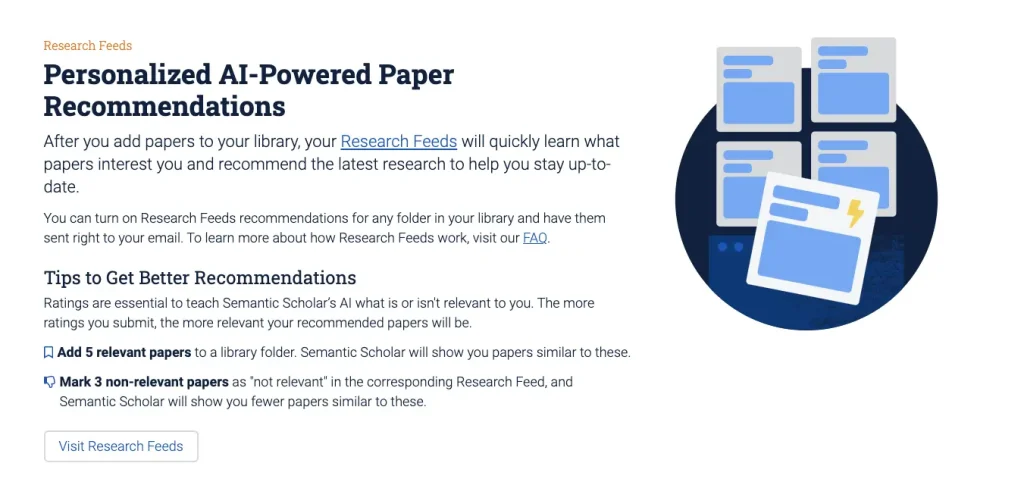
For: summarizing papers
Apparently, Elicit is a huge booster as its users save up to 5 hours per week. With a database of 125 million papers, the tool will enable you to get one-sentence, abstract AI summaries, and extract details from a paper into an organized table. You can also find common themes and concepts across many papers. Keep in mind that Elicit works best with empirical domains that involve experiments and concrete results, like biomedicine and machine learning.
Pricing: Free plan offers 5,000 credits one time. See more on pricing here .
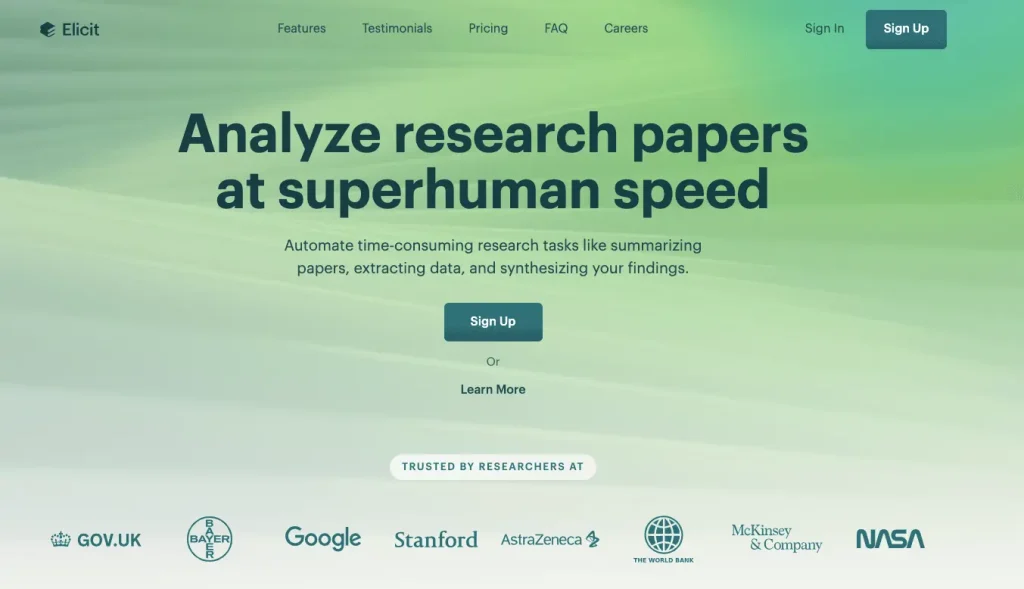
For: transcribing interviews
Supporting 125+ languages, Maestra’s interview transcription software will save you from the tedious task of manual transcription so you can dedicate more time to analyzing and interpreting your research data. Just upload your audio or video file to the tool, select the audio language, and click “Submit”. Maestra will convert your interview into text instantly, and with very high accuracy. You can always use the tool’s built-in text editor to make changes, and Maestra Teams to collaborate with fellow researchers on the transcript.
Pricing: With the “Pay As You Go” plan, you can pay for the amount of work done. See more on pricing here .
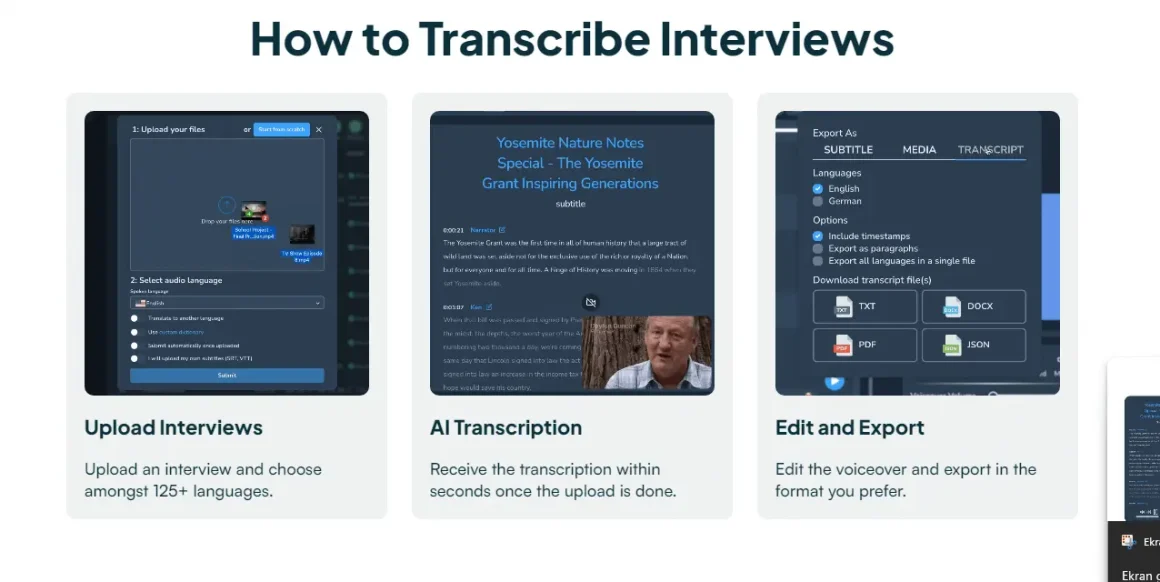
5. ATLAS.ti
For: qualitative data analysis
Whether you’re working with interview transcripts, focus group discussions, or open-ended surveys, ATLAS.ti provides a set of tools to help you extract meaningful insights from your data. You can analyze texts to uncover hidden patterns embedded in responses, or create a visualization of terms that appear most often in your research. Plus, features like sentiment analysis can identify emotional undercurrents within your data.
Pricing: Offers a variety of licenses for different purposes. See more on pricing here .
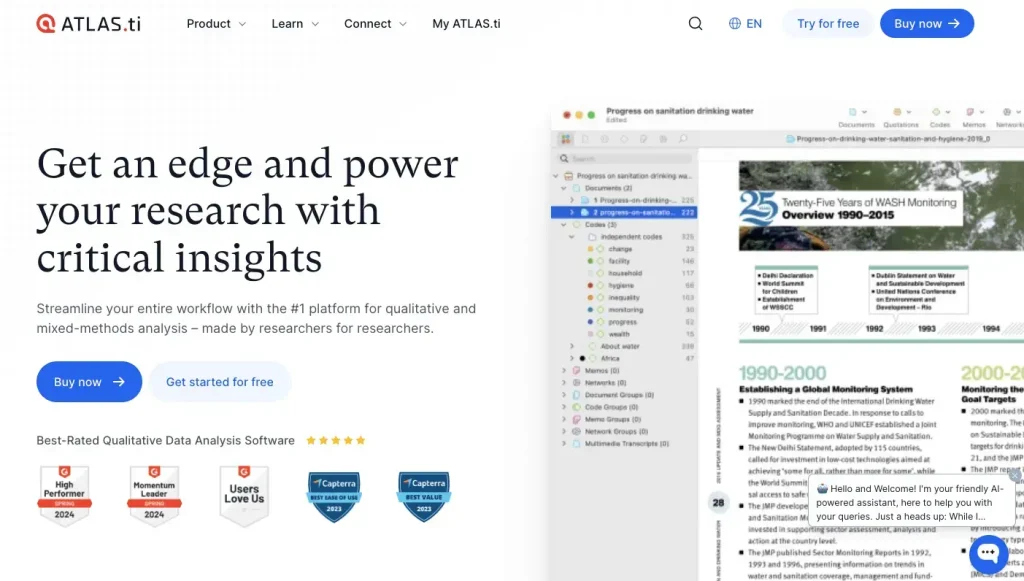
6. Power BI
For: quantitative data analysis
Microsoft’s Power BI offers AI Insights to consolidate data from various sources, analyze trends, and create interactive dashboards. One feature is “Natural Language Query”, where you can directly type your question and get quick insights about your data. Two other important features are “Anomaly Detection”, which can detect unexpected patterns, and “Decomposition Tree”, which can be utilized for root cause analysis.
Pricing: Included in a free account for Microsoft Fabric Preview. See more on pricing here .
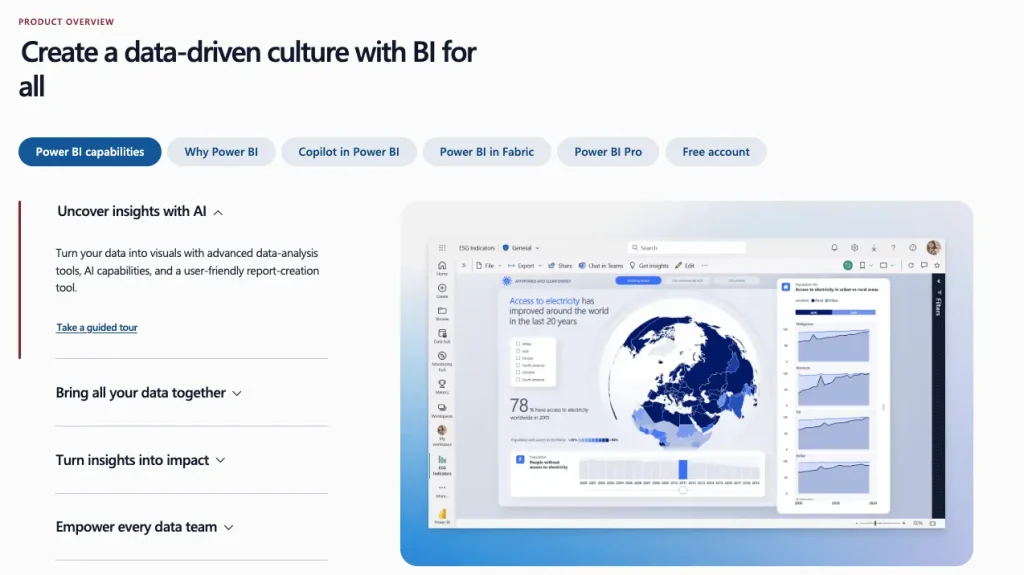
7. Paperpal
For: writing research papers
As a popular AI writing assistant for academic papers, Paperpal is trained and built on 20+ years of scholarly knowledge. You can generate outlines, titles, abstracts, and keywords to kickstart your writing and structure your research effectively. With its ability to understand academic context, the tool can also come up with subject-specific language suggestions, and trim your paper to meet journal limits.
Pricing: Free plan offers 5 uses of AI features per day. See more on pricing here .
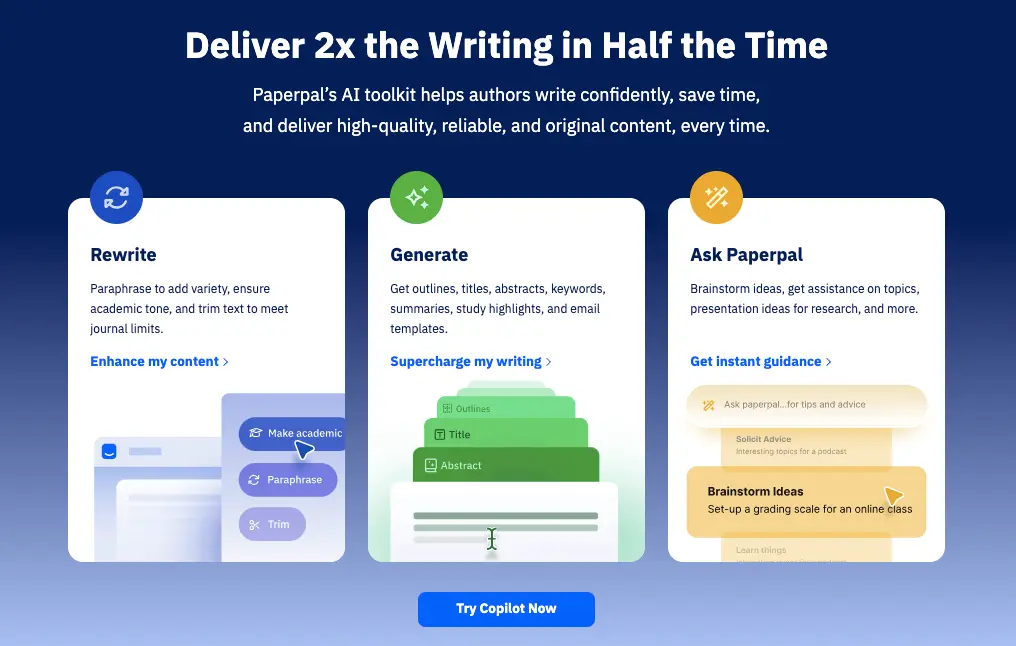
For: proofreading
With Scribbr’s AI Proofreader by your side, you can make your academic writing more clear and easy to read. The tool will first scan your document to catch mistakes. Then it will fix grammatical, spelling and punctuation errors while also suggesting fluency corrections. It is really easy to use (you can apply or reject corrections with 1-click), and works directly in a DOCX file.
Pricing: The free version gives a report of your issues but does not correct them. See more on pricing here .
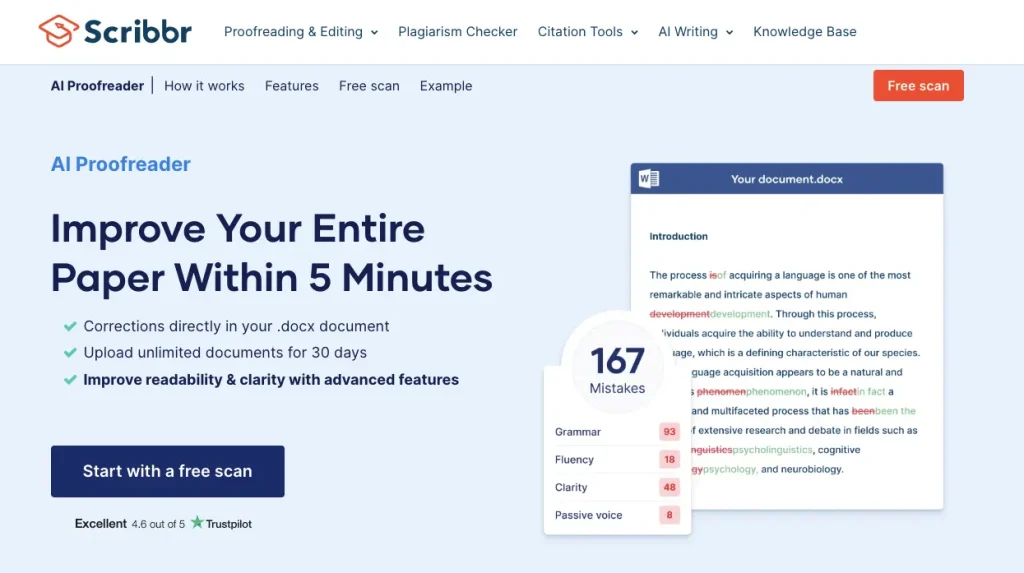
9. Quillbot
For: detecting AI-generated content
Want to make sure your research paper does not include AI-generated content? Quillbot’s AI Detector can identify certain indicators like repetitive words, awkward phrases, and an unnatural flow. It’ll then show a percentage representing the amount of AI-generated content within your text. The tool has a very user-friendly interface, and you can have an unlimited number of checks.
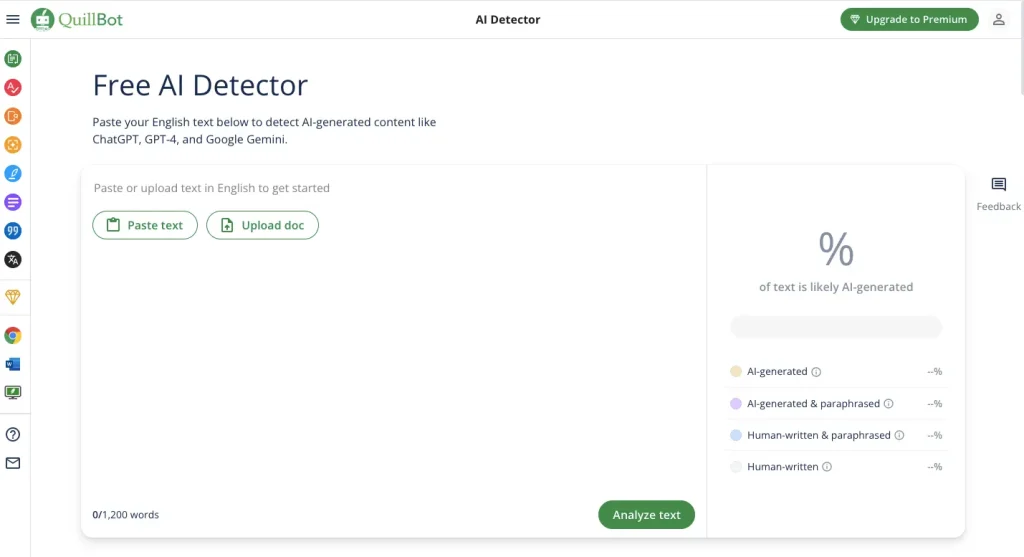
10. Lateral
For: organizing documents
Lateral will help you keep everything in one place and easily find what you’re looking for.
With auto-generated tables, you can keep track of all your findings and never lose a reference. Plus, Lateral uses its own machine learning technology (LIP API) to make content suggestions. With its “AI-Powered Concepts” feature, you can name a Concept, and the tool will recommend relevant text across all your papers.
Pricing: Free version offers 500 Page Credits one-time. See more on pricing here .
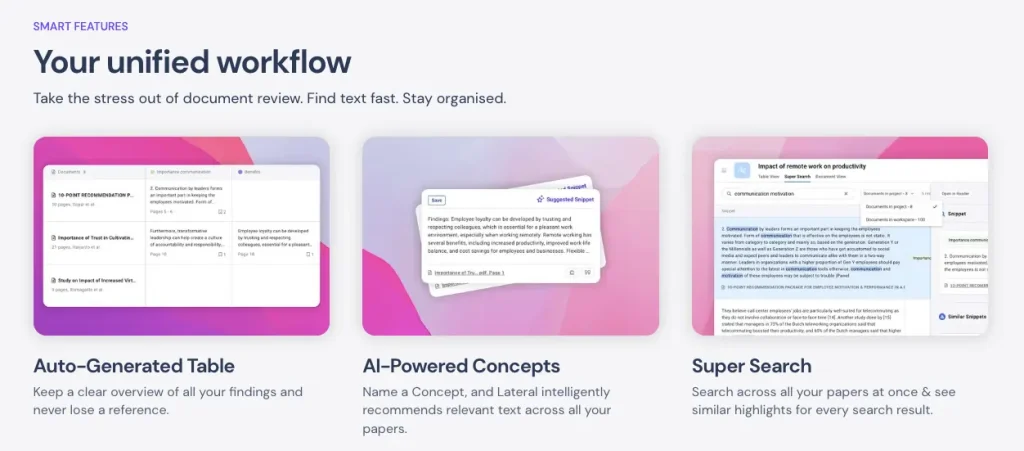
How to Use AI Tools for Research: 5 Best Practices
Before we conclude our blog, we want to list 5 best practices to adopt when using AI tools for academic research. They will ensure you’re getting the most out of AI technology in your academic pursuits while maintaining ethical standards in your work.
- Always remember that AI is an enhancer, not a replacement. While it can excel at tasks like literature review and data analysis, it cannot replicate the critical thinking and creativity that define strong research. Researchers should leverage AI for repetitive tasks, but dedicate their own expertise to interpret results and draw conclusions.
- Verify results. Don’t take AI for granted. Yes, it can be incredibly efficient, but results still require validation to prevent misleading or inaccurate results. Review them thoroughly to ensure they align with your research goals and existing knowledge in the field.
- Guard yourself against bias. AI tools for academic research are trained on existing data, which can contain social biases. You must critically evaluate the underlying assumptions used by the AI model, and ask if they are valid or relevant to your research question. You can also minimize bias by incorporating data from various sources that represent diverse perspectives and demographics.
- Embrace open science. Sharing your AI workflow and findings can inspire others, leading to innovative applications of AI tools. Open science also promotes responsible AI development in research, as it fosters transparency and collaboration among scholars.
- Stay informed about the developments in the field. AI tools for academic research are constantly evolving, and your work can benefit from the recent advancements. You can follow numerous blogs and newsletters in the area ( The Rundown AI is a great one) , join online communities, or participate in workshops and training programs. Moreover, you can connect with AI researchers whose work aligns with your research interests.
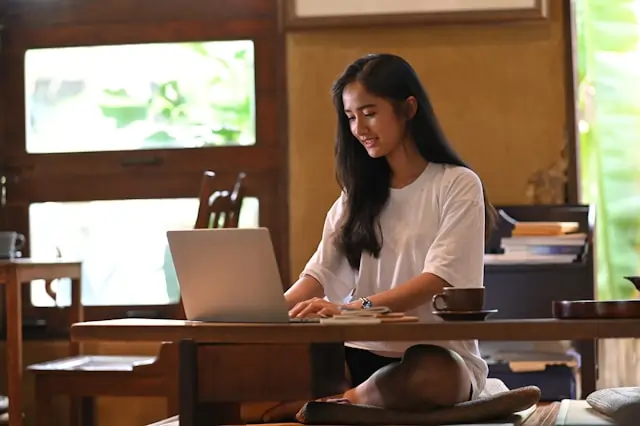
Frequently Asked Questions
Is chatgpt good for academic research.
ChatGPT can be a valuable tool for supporting your academic research, but it has limitations. You can use it for brainstorming and idea generation, identifying relevant resources, or drafting text. However, ChatGPT can’t guarantee the information it provides is entirely accurate or unbiased. In short, you can use it as a starting point, but never rely solely on its output.
Can I use AI for my thesis?
Yes, but it shouldn’t replace your own work. It can help you identify research gaps, formulate a strong thesis statement, and synthesize existing knowledge to support your argument. You can always reach out to your advisor and discuss how you plan to use AI tools for academic research .
Can AI write review articles?
AI can analyze vast amounts of information and summarize research papers much faster than humans, which can be a big time-saver in the literature review stage. Yet it can struggle with critical thinking and adding its own analysis to the review. Plus, AI-generated text can lack the originality and unique voice that a human writer brings to a review.
Can professors detect AI writing?
Yes, they can detect AI writing in several ways. Software programs like Turnitin’s AI Writing Detection can analyze text for signs of AI generation. Furthermore, experienced professors who have read many student papers can often develop a gut feeling about whether a paper was written by a human or machine. However, highly sophisticated AI may be harder to detect than more basic versions.
Can I do a PhD in artificial intelligence?
Yes, you can pursue a PhD in artificial intelligence or a related field such as computer science, machine learning, or data science. Many universities worldwide offer programs where you can delve deep into specific areas like natural language processing, computer vision, and AI ethics. Overall, pursuing a PhD in AI can lead to exciting opportunities in academia, industry research labs, and tech companies.
This blog shared 10 powerful AI tools for academic research, and highlighted each tool’s specific function and strengths. It also explained the increasing role of AI in academia, and listed 5 best practices on how to adopt AI research tools ethically.
AI tools hold potential for even greater integration and impact on research. They are likely to become more interconnected, which can lead to groundbreaking discoveries at the intersection of seemingly disparate fields. Yet, as AI becomes more powerful, ethical concerns like bias and fairness will need to be addressed. In short, AI tools for academic research should be utilized carefully, with a keen awareness of their capabilities and limitations.

About Serra Ardem
Serra Ardem is a freelance writer and editor based in Istanbul. For the last 8 years, she has been collaborating with brands and businesses to tell their unique story and develop their verbal identity.
Improving Writing Feedback for Struggling Writers: Generative AI to the Rescue?
- Original Paper
- Open access
- Published: 14 May 2024
Cite this article
You have full access to this open access article
- Anya S. Evmenova 1 ,
- Kelley Regan 1 ,
- Reagan Mergen 1 &
- Roba Hrisseh 1
162 Accesses
1 Altmetric
Explore all metrics
Generative AI has the potential to support teachers with writing instruction and feedback. The purpose of this study was to explore and compare feedback and data-based instructional suggestions from teachers and those generated by different AI tools. Essays from students with and without disabilities who struggled with writing and needed a technology-based writing intervention were analyzed. The essays were imported into two versions of ChatGPT using four different prompts, whereby eight sets of responses were generated. Inductive thematic analysis was used to explore the data sets. Findings indicated: (a) differences in responses between ChatGPT versions and prompts, (b) AI feedback on student writing did not reflect provided student characteristics (e.g., grade level or needs; disability; ELL status), and (c) ChatGPT’s responses to the essays aligned with teachers’ identified areas of needs and instructional decisions to some degree. Suggestions for increasing educator engagement with AI to enhance teaching writing is discussed.
Similar content being viewed by others
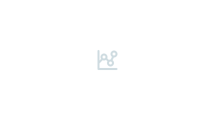
Students’ voices on generative AI: perceptions, benefits, and challenges in higher education
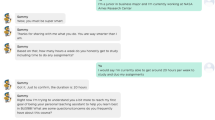
Artificial Intelligence (AI) Student Assistants in the Classroom: Designing Chatbots to Support Student Success
Examining science education in chatgpt: an exploratory study of generative artificial intelligence.
Avoid common mistakes on your manuscript.
The advances in Generative Artificial Intelligence (generative AI) have transformed the field of education introducing new ways to teach and learn. Its integration is fast growing in all areas of education, including special education (Marino et al., 2023 ). Generative AI has the potential to increase the inclusion of students with disabilities in general education by providing additional assistive supports (Garg and Sharma, 2020 ; Zdravkova, 2022 ). Specifically, large language models like the one used by a popular AI tool, ChatGPT (Chat Generative Pre-trained Transformer) can generate human-like responses to prompts, similar to a conversation. It can facilitate learning for students with and without high-incidence disabilities (e.g., learning disabilities, ADHD) who struggle with writing (Barbetta, 2023 ). While experts continue to investigate the future of writing in the ChatGPT era, it is evident that it will significantly alter writing instruction (Wilson, 2023 ). ChatGPT can support students in choosing a topic, brainstorming, outlining, drafting, soliciting feedback, revising, and proofreading (Trust et al., 2023 ). This tool may also be a helpful resource for teachers in providing feedback on students’ writing. Timely and quality feedback by ChatGPT can encourage the use of higher-level thinking skills while improving the writing process including the planning, writing, and reviewing phases of that process (Golinkoff & Wilson, 2023 ).
Writing Instruction and Feedback for Struggling Writers
The writing process may be challenging for some students for many reasons. For example, planning is the first step of writing, but many students don’t systematically brainstorm. Instead, they move directly into drafting their sentences which may, in turn, be disjointed and not effectively communicated (Evmenova & Regan, 2019 ). Students, particularly those with high-incidence disabilities may not produce text or compose limited text, struggling with content generation, vocabulary, and the organization of ideas (Chung et al., 2020 ). While multilinguism is an asset, we have observed similar challenges with writing among English Language Learners in our research (Hutchison et al., 2024 ). The cognitive demands needed for drafting a response leave many students at no capacity to then edit or revise their work (Graham et al., 2017 ). Therefore, teachers should provide scaffolds to break down the complex process of writing so that it is sequential and manageable, progressing from simple to more complex concepts and skills.
Instruction for struggling writers is typically characterized as systematic and explicit (Archer & Hughes, 2011 ; Hughes et al., 2018 ). In order to provide explicit instruction, teachers should be guided by ongoing student data. Specifically, special and general education teachers of writing should collaboratively, systematically, and continuously monitor and responsively adjust instruction based on student progress (Graham et al., 2014 ). Formative assessments of writing inform feedback that a teacher provides a learner. McLeskey et al., ( 2017 ) describes:
Effective feedback must be strategically delivered, and goal directed; feedback is most effective when the learner has a goal, and the feedback informs the learner regarding areas needing improvement and ways to improve performance… Teachers should provide ongoing feedback until learners reach their established learning goals. (p. 25)
Various formative assessments are available to guide feedback in writing, with rubrics being one frequently used method, which we will explore in the following section.
Supporting Writing by Struggling Writers
School-aged students are required to show progress towards mastery of writing independently in order to be successful at school, future work, and in their personal lives (Graham, 2019 ). Thus, educators continuously look for tools to increase and support learner agency and independence including in writing (Edyburn, 2021 ). Over the past decade, the authors have developed a digital tool to support learner autonomy, access, and independence during essay composition as part of a federally funded, design-based research project referred to as WEGO: Writing Effectively with Graphic Organizers (Evmenova et al., 2018–2023 ). This tool is a technology-based graphic organizer (or TBGO) that embeds numerous evidence-based strategies and universally designed supports for students as well as an analytic rubric for teachers to evaluate student products and providing feedback. A detailed description of the tool can be found elsewhere (students’ features: Evmenova et al., 2020a ; teachers’ features: Regan et al., 2021 ).
The TBGO was developed to support upper elementary and middle school students with and without high-incidence disabilities to compose multiple genres of writing including persuasive (Evmenova et al., 2016 ), argumentative (Boykin et al., 2019 ), and/or personal narrative writing (Rana, 2018 ). The TBGO has also been effectively used by English Language Learners (Day et al., 2023 ; Boykin et al., 2019 ). In addition, it includes a dashboard that allows a teacher or caregiver to personalize instruction: assign prompts and support features embedded in the TBGO. After the student has an opportunity to write independently, the teacher can engage in what we refer to as data-driven decision making (or DDDM; Park & Datnow, 2017 ; Reeves and Chiang, 2018 ).
Teachers’ DDDM
A common formative assessment of writing used in classrooms is a rubric. In order to facilitate the DDDM process within the TBGO, various data are collected by the tool and provided to teachers including final writing product, total time spent actively using the tool, video views and duration, text-to-speech use and duration, audio comments use and duration, transition words use, total number of words, number of attempts to finish. A teacher first evaluates those data as well as student’s writing using a 5-point rubric embedded in the teacher dashboard of the TBGO (a specific rubric is available at https://wego.gmu.edu ). Based on the rubric, a teacher identifies an area of need organized by phases of the writing process: Planning (select a prompt; select essay goal; select personal writing goal; brainstorm); Writing (identify your opinion, determine reasons, explain why or say more, add transition words, summarize, check your work); and Reviewing: Revise and Edit (word choice, grammar/spelling, punctuation, capitalization, evaluate). Then, a teacher provides specific instructional suggestions when the students’ score does not meet a threshold (e.g., content video models, modeling, specific practice activities). Once teachers select a targeted instructional move that is responsive to the identified area on the writing rubric, they record their instructional decision in the TBGO dashboard. The student’s work, the completed rubric, and the instructional decision is stored within the teacher dashboard. Recent investigations report that teachers positively perceive the ease and usability of the integrated digital rubric in the TBGO (see Regan et al., 2023a ; b ). Although promising, the teachers in those studies used DDDM with only a few students in their inclusive classes.
Efficient and Effective DDDM
The current version of the TBGO relies on teachers or caregivers to score student writing using an embedded rubric and to subsequently provide the student(s) with instructional feedback. In a classroom of twenty or more students, scoring individual essays and personalizing the next instructional move for each student is time consuming, and teachers may not regularly assess or interpret students’ writing abilities in the upper grades, especially (Graham et al., 2014 ; Kiuhara et al., 2009 ). Generative AI or chatbots are arguably leading candidates to consider when providing students with instructional feedback in a more time efficient manner (Office of Educational Technology, 2023 ). For example, automated essay scoring (AES) provides a holistic and analytic writing quality score of students’ writing and a description as to how the student can improve their writing. Recent research on classroom-based implementation of AES suggests its potential; but questions have been raised as to how teachers and students perceive the scores, and how it is used in classroom contexts (Li et al., 2015 ; Wilson et al., 2022 ). Other investigations remark on the efficiency and reliability among AES systems (Wilson & Andrada, 2016 ) and the consistency of scores with human raters (Shermis, 2014 ). More recently, a large-language model (specifically, GPT-3.5 version of ChatGPT) was prompted to rate secondary students’ argumentative essays and chatbot’s responses were compared to humans across five measures of feedback quality (see Steiss et al., 2023 ). Although GPT-3.5 included some inaccuracies in the feedback and the authors concluded that humans performed better than ChatGPT, the comparisons were remarkably close.
A greater understanding of what generative AI tools can do to support classroom teachers is needed. First, leveraging technology, with the use of automated systems, or logistical tools, can potentially improve working conditions for both general and special education teachers (Billingsley & Bettini, 2017 ; Johnson et al., 2012 ). Also, although educators see the benefits of AI and how it can be used to enhance educational services, there is urgent concern about the policies needed around its use and how it is ever evolving. For example, when writing this manuscript, GPT-4 evolved, but at a cost, this latter version may not be widely accessible for educators or students. With the fast adoption of AI, the Office of Educational Technology states that “it is imperative to address AI in education now to realize and mitigate emergent risks and tackle unintended consequences” (U.S. Department of Education, 2023 , p. 3). A first step in addressing AI in education is to understand what AI can do, and how its use supports or hinders student learning and teacher instruction. In this case, we focus on teachers’ writing instruction and feedback.
As we learn more about AI tools, it becomes obvious that AI literacy skills will need to be developed as part of digital skills by both teachers and students (Cohen, 2023 ). The importance of how we use chatbots, how we prompt them, and what parameters we use to direct the responses of chatbots becomes paramount.
Thus, the purpose of this study was to explore feedback and instructional suggestions generated by different AI tools when using prompts providing varying specificity (e.g., a generic 0–4 rating vs. analytic rubric provided) to help guide teachers of writing in their use of these tools. The purpose of including two versions of ChatGPT was not to criticize one and promote the other; but rather to understand and leverage their similarities and differences, given the same prompt. The research questions were:
RQ1: What is the difference between responses generated by GPT-3.5 and GPT-4 given prompts which provide varying specificity about students’ essays?
RQ2: What is the nature of the instructional suggestions provided by ChatGPT for students with and without disabilities and/or ELLs (aka struggling writers)?
RQ3: How does the formative feedback provided by GPT-3.5 and GPT-4 compare to the feedback provided by teachers when given the same rubric?
Data for this study were selected from a large intervention research study (led by the same authors) for a secondary data analysis. Specifically, while previous studies focused on the improvements in students’ writing outcomes (e.g., both quantity and quality of written essays) as well as explored how teachers provide feedback on students’ writing, the unique focus of this paper was on the use of AI to provide writing feedback (something we have not done before). The data included 34 persuasive student essays, a teacher’s completed analytic rubric evaluating the essay, and a teacher’s data-driven decisions with instructional feedback in the area of Writing and Reviewing (essays with the teachers’ DDDM in the area of Planning were excluded). We purposefully selected essays completed by students with various abilities and needs in different grade levels who struggled with writing and needed the TBGO intervention.
Participants
The 34 essays used in this study were written by 21 girls and 13 boys. Students ranged in age 8–13 and were in grades 3–7. The majority (59%) were White, 21% were Hispanic, 3% were African American, and 17% were other. Among the students, 41% were identified with high-incidence disabilities (learning disabilities, ADHD); 24% were English language learners (with a variety of primary languages); and 35% were struggling writers as reported by teachers. Teachers identified struggling writers as those who consistently demonstrated writing performance below grade level expectations (e.g., needing extra support with writing mechanics, cohesive and well-organized ideas).
Study Context
The data used in this study were collected in two separate settings: two inclusive classrooms in a suburban, private day school and an after-school program in a community center serving economically disadvantaged families. The same essay writing procedures were used in both settings. All students were first asked to write a persuasive opinion-based essay in response to one of two prompts validated by previous research (Regan et al., 2023b ). Examples of the prompts included:
Some students go to school on Saturday. Write an essay on whether or not students should go to school on Saturdays.
Some people believe kids your age should not have cell phones. Using specific details and examples to persuade someone of your opinion, argue whether or not kids your age should have cell phones.
After the pretest, students were introduced to the technology-based graphic organizer (TBGO) with embedded evidence-based strategies and supports. The instruction lasted 5–6 lessons. Then students were asked to use the TBGO to practice independent essay writing without any help from the teachers. As the TBGO is a Chrome-based web application and works on any device with a Chrome browser installed, each student used their own device/laptop and individual login credentials to access the TBGO. After completing the independent writing, teachers reviewed students’ products and completed the analytic rubric built into the TBGO’s teacher dashboard. They identified one primary area of need and determined an instructional decision that should take place in order to address the existing area of need. The instructional decisions included whole- and small-group activities (especially in those cases when multiple students demonstrated the same area of need); independent activities (including watching video models embedded within the TBGO); as well as individual teacher-student check-ins to discuss the area of need and future steps. A posttest with the TBGO and a delayed posttest without the TBGO were later administered. The essays used in the current study were from an independent writing phase since those included teachers’ DDDM. On average, essays had 133.44 ( SD = 57.21; range 32–224) total words written. The vast majority included such important persuasive essay elements such as a topic sentence introducing the opinion, distinct reasons, examples to explain the reasons, summary sentence, and transition words. While this provides some important context, the quantity and quality of students’ writing products is not the focus of the current study and is reported elsewhere (Boykin et al., 2019 ; Day et al., 2023 ; Evmenova et al., 2016 , 2020b ; Regan et al., 2018 , 2023b ).
Data Sources
The existing 34 essays were imported into two different versions of the ChatGPT generative AI: GPT-3.5 version of ChatGPT (free version) and GPT-4 (subscription version). Four different prompts were used in both ChatGPT versions (see Table 1 ). As can be seen in Table 1 , the different prompts included (1) using a specific analytic rubric (when a rubric from the TBGO was uploaded to ChatGPT); (2) asking for a generic 0–4 rating (without any additional specifics regarding scoring); (3) no rubric (asking to identify the area of need without any rubric); (4) no information (asking to provide generic feedback without any information about the student in the prompt). Each prompt type constituted its own GPT chat. Thus, eight sets of responses (or eight different chats) were generated by ChatGPT. A prompt tailored to include the student’s essay as well as the specific student characteristics and the essay topic when applicable (according to the prompt samples presented in Table 1 ) was pasted into the chat. After GPT had a chance to react and provide feedback, the next prompt was pasted into the same chat. Thus, each chat included a total of 34 prompts and 34 GPT outputs. Each chat was then saved and analyzed.
Data Analysis and Credibility
Inductive thematic analysis was used to explore how generative AI can be used to provide writing feedback and guide writing instruction for struggling writers (Guest et al., 2011 ). First, each set of ChatGPT responses (or each GPT chat) was analyzed individually, and reoccurring codes across responses were grouped into categories. The four members of the research team were randomly assigned to analyze two GPT sets each. Each member generated a list of codes and categories within a chat that were the shared with the team and discussed. During those discussions, the patterns within categories were compared across different sets to develop overarching themes in response to RQ1 and RQ2. The trustworthiness of findings was established by data triangulation across 34 writing samples and eight sets of feedback. Also, peer debriefing was used throughout the data analysis (Brantlinger et al., 2005 ).
To answer RQ3, frequencies were used to compare teachers’ and ChatGPT scores on the analytic rubric and suggested instructional decisions. First, two researchers independently compared teachers’ and ChatGPT scores and suggestions. Since the same language from the rubric was used to identify the area of need, the comparisons were rated as 0 = no match; 1 = match. For instructional suggestions, the scale was 0 = no match; 1 = match in concept, but not in specifics; and 2 = perfect match. Over 50% of comparisons were completed by two independent researchers. Interrater reliability was established using point-by-point agreement formula dividing the number of agreements by the total number of agreements plus disagreements and yielding 100% agreement.
RQ1: Differences in AI Responses
In effort to answer RQ1 and explore the differences between responses generated by different ChatGPT versions when given prompts with varying specificity, we analyzed eight sets of responses. While the purpose was not to compare the sets in effort to find which one is better, several patterns have been observed that can guide teachers in using ChatGPT as the starting point for generating writing feedback to their struggling writers. The following are the six overarching themes that emerged from this analysis.
Predictable Pattern of Response
As can be seen in Table 2 , all sets generated excessive amounts of feedback (average length: M = 383; SD = 109.7; range 258–581 words) and followed a consistent, formulaic, and predictable pattern of responses across all the writing samples. While the layout and headers used to organize the responses differed across different ChatGPT versions and prompts, the layout and headers were consistent within each set. That said, it was also observed in all ChatGPT sets that the organization and headings found in a response changed slightly towards the end of the run for the 34 writing samples. It is unclear whether this pattern change may happen after a certain number of entries (or writing samples in our case) were entered into the ChatGPT run or if this shift in pattern occurs randomly. Similarly, we also observed that the later responses seemed to be more concise and lacked details which were observed earlier in the same set.
Specific Analytic Rubric
Both GPT-3.5 and GPT-4 provided responses organized into nine categories matching those included in the uploaded rubric. Each category included 1–2 sentences of feedback along with a numerical rating on a 0–4 scale. An overall holistic score was also calculated at the end along with a summary of the student’s overall strengths and weaknesses.
Generic 0–4 Rating
For each writing sample, GPT-3.5 consistently included an evaluation of student writing using four criteria-based categories: Content, Organization, Language Use (punctuation, spelling, and grammar), and Development of Ideas. Two to three bullet points of feedback were listed under each category along with a numeric rating on a 0–4 scale for each. The scale was not defined or explained. An overall holistic score was totaled at the end along with a summary of feedback presented in a bulleted list.
GPT-4’s response to the first writing sample included a definition of what each point on the scale meant (e.g., 4 = writing is clear, well-organized, well-developed, with effectively chosen details and examples presented logically, and few to no errors in conventions). In all consecutive responses, an introductory paragraph identified an overall bold-faced score (0–4) and an overview of what the student did and did not demonstrate in the writing. The following areas of writing were discussed across essays: Organization, Development, Main Idea, Reasons, Examples, Coherence, and Grammar.
Each response in GPT-3.5 began with “One area of need is…” followed by two sentences including how to address the need. Areas of need for instruction identified by ChatGPT included a high frequency of subject-verb agreement as parts of sentence structure (topic sentence and supporting details), followed by transition words or phrases, spelling and grammar conventions, spelling and word choice, capitalization, and punctuation. The second part of the response, titled Instructional Suggestion, provided an instructional strategy for a teacher to use, followed by a model of a ‘revised’ essay using ideas from the student’s response.
GPT-4 provided four consistent parts. First, the response opened with a statement about what the student wrote, a positive affirmation, and an instructional area of writing that could be improved upon. Next, under a header of Instructional Suggestion was a brief description as to what the teacher should do. The third part was a bold-faced, numbered list of steps for implementing that suggestion with bulleted cues underneath. The final part of the response was a ‘revised’ paragraph using the student’s initial writing and addressing the area of need.
GPT-3.5 provided feedback organized in 9 to 11 bolded categories. The sections that were identical for every writing sample included Proofreading; Revising and Editing; Encourage Creativity; and Positive Reinforcement. The sections that were consistent but individualized for each writing sample were Clarity and Organization (including a topic/introductory sentence); Supporting Details; Sentence Structure and Grammar (primarily focus on sentence fragments, punctuation, and capitalization); Conclusion; Vocabulary and Word Choice. Feedback on spelling and transition words/phrases was offered either as separate categories or subsumed under others.
GPT-4’s response could be organized in 3 overarching groups: Positive Reinforcement (including specific praise, affirmation, and creativity); Areas for Improvement (content feedback including idea development; details; coherence; clarity and focus; concluding sentence; and technical feedback including sentence structure; punctuation; grammar; word choice); as well as Instructional Suggestions. A sample revised paragraph was offered at the end with an explanation as to how it showcased the offered suggestions.
Using Specific Language from the Rubric
Both Specific Analytic Rubric sets (using GPT-3.5 and GPT-4) referred exclusively to the uploaded rubric and provided feedback using specific language from the rubric. This included feedback across the nine categories built into the rubric (e.g., the writer clearly identified an opinion, the writer has determined three reasons that support his/her opinion, etc.). Also, both ChatGPT versions used descriptors from the rubric (0 = Try again; 1 = Keep trying; 2 = Almost there; 3 = Good job; 4 = Got it). However, GPT-3.5 did not use any explicit examples from the student’s writing within the feedback and used broad and general statements. GPT-4 referred to the specific content from the students’ writing samples and was more tailored, or individualized (e.g., There are some grammatical and spelling errors present, e.g., "are" instead of "our").
Identifying General, Broad Areas of Need
Feedback in all GPT-3.5 sets (regardless of the prompt) was characterized as using common phrases representing broad areas of need. These phrases were not specifically targeted or explicit. For example, the Generic Rating GPT-3.5 set included such common phrases as “The essay presents ideas and supports them with reasonable detail, but there's room for more depth and elaboration.” or “The content is well-structured and effectively conveys the main points.” Similarly, the No Rubric GPT-3.5 set identified instructional areas of need that were only broadly relevant to the students’ writing. For example, in several instances, our review questioned the prioritization of the writing area identified and if ChatGPT was overgeneralizing areas in need of improvement. Specifically, does two instances of using lowercase when it should be uppercase mean that capitalization should be prioritized over other essential features of writing? Finally, the No Info GPT-3.5 set also used common phrases to describe areas for improvement regardless of the writing sample. For example, there were no difference in ChatGPT’s feedback for a writing essay with eight complete, robust, well-written sentences vs. an incomplete paragraph with just two sentences indicating the lack of targeted and specific feedback.
No Rubric GPT-4 set would start with identifying a broad area of need (e.g., coherence, grammar, development, organization/development of ideas, attention to detail) followed by a more individualized and specific instructional suggestion (as discussed below). The authors acknowledge that this might be explained by the prompt language to identify one area of need.
Focusing on an Individualized, Specific Areas of Need
Like the Specific Analytic Rubric GPT-4 set, the Generic 0–4 Rating GPT-4 set and the No Info GPT-4 sets were observed to include more guidance for the student, drawing on specific areas of an essay to provide corrective feedback. For example, Generic Rating GPT-4 feedback noted, “We should also try to provide more specific examples or explanations for each reason. For example, you mentioned that students get tired – maybe you can explain more about how having some recess can help them feel less tired.” In turn, No Info GPT-4 included detailed feedback focused on specific areas of need such as encouraging more details and clarifications, cohesion and flow, capitalization, spelling, homophones, and punctuation (including avoiding run-on sentences and properly using commas). Word choice, contractions, and conjunctions were often mentioned offering specific revisions. Varying the length and structure of sentences was sometimes suggested for making the writing more engaging and readable.
Misaligned Feedback
While there were some occasional discrepancies in GPT-4 sets, all GPT-3.5 sets appeared to generate feedback that was more misaligned with writing samples. For example, in the Specific Analytic Rubric GPT-3.5 set, a “Good Job” score of 3 was given for the Summary sentence that read, “Moreover, …” and was not a complete sentence. Also, the Generic Rating GPT-3.5 set did not mention any misuse of capitalization despite numerous cases of such misuse. Subject-verb agreement was erroneously mentioned as an area of need for some writing samples for the No Rubric GPT-3.5 set, and then, not mentioned for those students’ writing in which this feedback would be relevant. In the No Info GPT-3.5 set, the topic or introductory sentence was always noted as a suggested area of improvement and a revised sentence was always provided. This was true for cases when a student:
was missing an opinion that aligned with the prompt
had an opinion but did not start it with words “I believe …” (e.g., “Kids should get more recess time.”); and
already had a strong introductory sentence (e.g., “I believe that school starts too early and should begin later in the morning.”).
Starting with Specific Praise/Positive Affirmation
While most ChatGPT feedback included some general praise and affirmation, Generic Rating GPT-4, No Rubric GPT-4, and No Info GPT-4 sets always started with specific positive reinforcement. Unique elements in each essay were praised including conveying personal experiences, having a clear stance or position, and including a variety of reasons, etc.
RQ2: Instructional Suggestions
Instructional suggestions based on the evaluation of student writing was a focus of RQ2. Although we expected the responses from prompts that included specific student characteristics to differentiate the instructional suggestions in some way, this was not the case. In fact, none of the sets provided explicit instructional suggestions aligned with students’ characteristics (e.g., grade, disability, ELL). First, the suggestions for improving the writing of a 3rd grader’s essay were not distinct from those suggestions provided in response to a 7th grader’s writing (in Generic Rating GPT-3.5 and No Rubric GPT-3.5 sets). Also, there were no remarkable differences in the vocabulary used in the feedback for a 3rd grader vs. a 7th grader (in Generic Rating GPT-4 set). Only one set (Generic Rating GPT-4) offered a personalized message in a student-friendly format (without any additional prompting to do so).
Second, student characteristics were merely acknowledged in some sets. For example, Specific Analytic Rubric GPT-3.5 and GPT-4 only noted those characteristics in the summary section at the end of the feedback (e.g., “This is a well-written persuasive essay by your 7th-grade student with ADHD”). This was also observed in responses from the Generic Rating GPT-4 set, as well. For example, “This feedback emphasizes both the strengths of the student’s writing and the areas where improvement can be made, offering encouragement and guidance that is particularly important for a student with ADHD.” Finally, the No Rubric GPT-4 set also gave a mere nod to the additional context (e.g., Given student characteristics…). Although rare, connecting student characteristics with instruction was observed here: “Students with ADHD often struggle with organizing their thoughts in a coherent manner, and the flow of ideas in this student’s paragraph seems a bit disjointed….” Students’ characteristics were not mentioned in any other sets in which student information was included in the prompt (Generic Rating GPT-3.5 and No Rubric GPT-3.5).
Below is the description of how specific, broad, or no instructional suggestions were included in the ChatGPT sets (see Table 2 ).
Specific Suggestions
Specific instructional suggestions were mentioned in Generic Rating GPT-4, No Rubric GPT-4, and No Info GPT-4 sets. At the end of responses for the Generic Rating GPT-4 set, ChatGPT encouraged the teacher to use self-regulatory instructional strategies with students, such as goal setting or self-evaluation. For example, “By involving the student in the refinement of their work and setting goals, you empower them to take ownership of their learning and progression.”
No Rubric GPT-4 responses used such headings as modeling, guided practice, feedback, and independent practice with bulleted ideas under each. The specific suggestions included practice, mini-instructional lessons, engaging activities, peer review, explicit instruction, sentence-building activities, peer review sentence starters, technology such as word processing and online games, the five W’s and How strategy (i.e., a writing strategy that helps students remember to include the answers to “who,” “what,” “where,” “when,” “why,” and “how” in their writing to make their writing complete and clear), a mnemonic referred to as PEE (i.e., Point, Explain, Elaborate; this mnemonic helps students ensure their writing is focused, well-supported, and thoroughly developed), a personal dictionary, interactive editing, and a graphic organizer or outline. When the latter was suggested to support the “coherence” or “development of ideas,” ChatGPT’s response sometimes provided a backwards planning model of what the student’s ideas would look like in an outline format.
Responses of the No Info GPT-4 set included specific and varied instructional suggestions organized by categories: Writing Exercises; Focused Practice; and Revision Work. Suggestions included mini lessons on sentence structure, transition workshops, details workshops, personal experience illustrations, developing ideas workshops, worksheets, grammar lessons, spelling activities, sentence expansion or completion, and editing practice.
Broad Instructional Suggestions
Primarily broad instructional suggestions were offered in the Generic Rating GPT-3.5 and No Rubric GPT-3.5 sets. For example, Generic Rating GPT-3.5 responses had a section with a bulleted list of actionable, instructional suggestions. Each began with a verb (i.e., Work on…; Encourage the student to…; Practice…). It was also not clear if these suggestions were presented in any order of instructional priority. Also, the items included broad ideas that aligned with the student essays but may or may not have aligned with the lowest rated category of writing. Examples of largely vague and broad instructional suggestions recycled throughout the responses in the No Rubric GPT-3.5 set including: “use different types of sentences,” “teach basic spelling rules,” and “use appropriate punctuation.”
Revised Essay
The following three ChatGPT sets included responses with a revised student essay along with a brief explanation of how it was better (even though a revision was not requested in the prompt): No Rubric GPT-3.5, No Rubric GPT-4, and No Info GPT-4 sets. We considered that a model of writing, revised for improvement, was a broad instructional strategy. This is one of many excellent strategies for teaching writing, however, the revisions were often characterized by sophisticated vocabulary and complex elaborations. For example, a student wrote, “To illustrate, when students are hungry it’s hard for them to listen.” And ChatGPT elevated the sentence with, “To illustrate, when students are hungry, it's hard for them to listen because their minds may be preoccupied with thoughts of food.” Whereas the latter sentence is a well-crafted model for the student, this revision arguably loses the student’s voice and tone.
No Instructional Suggestions
No explicit instructional suggestions were included in the responses for Specific Analytic Rubric GPT-3.5, No Info GPT-3.5, and Specific Analytic Rubric GPT-4 sets. The reader was only reminded to provide feedback in a constructive and supportive manner and encourage students to ask questions and seek clarifications on any offered suggestions. While this is logical for both Specific Analytic rubric sets (not asking for instructional suggestions in the prompt), it is surprising for the No Info GPT-3.5 set (which asked for feedback and instructional suggestions).
RQ3: Comparisons Between Teachers and ChatGPT
In response to RQ3, we compared a real teachers’ data-based decision-making (DDDM), including the score and the instructional decision, to the scores generated in the Specific Analytic Rubric GPT-3.5 and Specific Analytic Rubric GPT-4 sets for students’ essays ( N = 34). The first rubric category scored with a 2 or below was considered the area of need for writing instruction.
GPT-3.5 matched the teacher’s recommendation for the area of writing need 17.6% of the time. For example, the teacher identified Word Selection as the area of need (e.g., high use of repeated words and lacking sensory words) and GPT-3.5 noted the same area of need (e.g., there is some repetition and awkward phrasing). When comparing teacher versus ChatGPTs instructional decisions, there was no perfect match; however, 26.5% were coded as a partial match. For example, both the teacher and GPT-3.5 suggested an instructional activity of modeling how to write a summary sentence.
GPT-4 matched the teacher’s recommendation for the area of writing need 23.5% of the time. Similarly, when comparing the teacher versus ChatGPT’s instructional decisions, 47.1% were coded as a partial match for instruction.
Discussion and Practical Implications
Since the end of 2022 when it debuted, school leaders and teachers of writing have been grappling with what ChatGPT means for writing instruction. Its ability to generate essays from a simple request or to correct writing samples is making an impact on the classroom experience for students with and without disabilities and it is reshaping how teachers assess student writing (Marino et al., 2023 ; Trust et al., 2023 ; Wilson, 2023 ). However, teachers may have limited knowledge of how AI works and poor self-efficacy for using AI in the classroom to support their pedagogical decision making (Chiu et al., 2023 ). It is imperative to ensure that teachers receive professional development to facilitate the effective and efficient use of AI. There are more questions than answers currently, especially for its application by students struggling with academics.
The purpose of this investigation was to explore the application of ChatGPT chatbot for teachers of writing. Specifically, we used different versions of ChatGPT (GPT-3.5 – free and GPT-4 – subscription) and purposefully different types of prompts, providing limited or more information about the student characteristics and the topic of their writing. Essentially, we asked ChatGPT to evaluate an authentic student’s writing, identify the area(s) of need, and provide instructional suggestion(s) for addressing the problematic area(s) in that individual writing sample. We then compared AI-generated feedback to that completed by humans.
The findings indicate the possibilities and limitations of ChatGPT for evaluating student writing, interpreting a teacher-developed rubric, and providing instructional strategies.
Our finding is that, generally, ChatGPT can follow purposeful prompts, interpret and score using a criterion-based rubric when provided, create its own criteria for evaluating student writing, effectively revise student essay writing, celebrate what students do well in their writing, paraphrase student essay ideas, draft outlines of a student’s completed essay, and provide formative feedback in broad and specific areas along different stages of the writing process. Moreover, the response is immediate. These findings are consistent with previous investigations of ChatGPT and the assessment of student writing (Steiss et al., 2023 ). However, teachers need to consider the following points before relying on ChatGPT to provide feedback to their struggling writers.
In the ChatGPT sets which included no contextual information, the responses included more feedback.
All sets generated excessive amounts of feedback about student writing with no delineation of the next clear instructional move a teacher should attend to. So, ChatGPT may work as a great starting point, but teachers will need to go through the response to prioritize and design their instruction. Sifting through information for relevance can be time consuming and may even warrant a teacher verifying the content further.
Additionally, if students relied directly on ChatGPT, without any vetting from a teacher about the content, they too may be overwhelmed by the amount of feedback given to modify their writing or they may even be provided with erroneous feedback.
All GPT-3.5 sets identified broad areas of writing that needed improvement and frequently used common phrases such as grammar, organization/development of ideas, and attention to detail. In addition, this feedback was more often misaligned with students’ writing. This observation is worrisome since GPT-3.5 version of ChatGPT is free and highly accessible, making it likely the preferred AI tool for classroom educators.
Most GPT-4 sets (except one) generated more specific and individualized feedback about student writing. The specific feedback included in the generated outputs were much lengthier and would take much more time for a teacher to review than GPT-3.5 responses.
All sets identified multiple areas of need and when included in the responses, there were multiple instructional suggestions. Even the No Rubric sets, which explicitly prompted ChatGPT to focus on just one area of instructional need and one suggestion, included much more in the responses. This finding reiterates that we are still learning about AI literacy and the language we need to use to communicate effectively.
Both GPT-3.5 and GPT-4 allowed the upload of a researcher-developed analytic rubric and moreover, interpreted the performance criteria, rating scale, and indicators. ChatGPT also used the rubric’s specific language when providing its evaluation of the student writing.
No tailored feedback or specific suggestions were contextualized when prompts included varying ages, grade levels, or various student abilities and needs. Further research is needed to determine the types of AI literacy prompts or the contextual information that ChatGPT needs to address the particular needs of an individual child. Specially designed instruction, the heart of special education, should be tailored to a particular student (Sayeski et al., 2023 ).
Low agreement reported between the rubric scores and instructional suggestions made by teachers and those generated by ChatGPT does not necessarily mean that ChatGPT’s feedback is incorrect. One explanation for the difference may be that teachers provide targeted and individualized instruction using multiple forms of data and critical information to make instructional decisions. This includes their own professional judgement and knowledge about how each students’ backgrounds, culture, and language may influence student performance (McLeskey et al., 2017 ).
Limitations
This study is an initial exploration. There are several limitations that need to be taken into consideration. First and foremost, the four prompts were designed to present the chatbots with varying levels of details and student information to consider when providing feedback about a student’s writing sample. For example, Specific Analytic Rubric prompt asked the chatbot to assess students’ writing using an uploaded rubric, while No Rubric prompt asked to identify one area of need for the student’s writing and offer one instructional suggestion to address it. In addition to providing the chatbots with varying information, we also used varying language throughout the prompts when seeking feedback and suggestions (e.g., “Identify areas of need for this student’s writing”; “Identify one area of need … and offer one instructional suggestion”; “what feedback and instructional suggestions…”). Chatbots are clearly sensitive to the word choices made; thus, a consistency of the language in prompts should be considered for any future investigations that aim at prompt comparison. The purpose of this work was not to compare the four prompts in effort to find the best possible one. We also were not looking specifically for the feedback that could be shared with students as is (even though some versions generated such feedback without additional prompting). Instead, we were trying to explore how the output might differ depending on the prompts with differing level of detail. So, some of the reported difference are logical. We also did not prompt the ChatGPT any further, which would most likely result in refined feedback and/or suggestions. There is an infinite number of prompts that we could have used in this analysis. In fact, a new field of prompt engineering is emerging right in front of our eyes as we learn to design inputs for generative AI tools that would produce optimal outputs. Further investigations of various prompts to feed ChatGPT are needed. Our hope is that this paper will inspire teacher to spend some time exploring different tools and prompts in effort to find the most appropriate output depending on their context and their students’ needs.
Also, there was a limited numbers of essays from each specific group of learners (e.g., certain age/grade, specific disability categories and other characteristics). While we reported meaningful findings for this initial exploratory analysis, future research should include writing products from more homogeneous groups. Finally, teachers’ DDDM was accomplished by evaluating a completed graphic organizer, while ChatGPT feedback was provided based on the final student essay copied and pasted from the TBGO. Future research should consider new features of generative AI tools (e.g., Chat GPT’s new image analysis feature) where an image of a completed graphic organizer can be uploaded and analyzed.
This study offers examples for how to potentially incorporate AI effectively and efficiently into writing instruction. High quality special education teachers are reflective about their practice, use a variety of assistive and instructional technologies to promote student learning, and regularly monitor student progress with individualized assessment strategies. It seems very likely that teachers will adopt the capabilities of generative AI tools. With ongoing development and enhancements, AI technology is certain to become an integral component of classroom instruction. However, given the limitations of ChatGPT identified in this study, teacher-led instruction and decision making is still needed to personalize and individualize specialized instruction. Engaging with the technology more and building familiarity of what it can do to improve student learning and teacher practice is warranted.
Archer, A. L., & Hughes, C. A. (2011). Explicit instruction: Effective and efficient teaching . Guilford press.
Google Scholar
Barbetta, P. M. (2023). Remedial and compensatory writing technologies for middle school students with learning disabilities and their classmates in inclusive classrooms. Preventing School Failure: Alternative Education for Children and Youth . https://doi.org/10.1080/1045988X.2023.2259837
Article Google Scholar
Boykin, A., Evmenova, A. S., Regan, K., & Mastropieri, M. (2019). The impact of a computer-based graphic organizer with embedded self-regulated learning strategies on the argumentative writing of students in inclusive cross-curricula settings. Computers & Education, 137 , 78–90. https://doi.org/10.1016/j.compedu.2019.03.008
Billingsley, B., & Bettini, E. (2017). Improving special education teacher quality and effectiveness. In J. M. Kauffman, D. P. Hallahan, & P. C. Pullen (Eds.), Handbook of special education (2nd ed., pp. 501-520). Boston: Taylor & Francis.
Brantlinger, E., Jimenez, R., Klinger, J., Pugach, M., & Richardson, V. (2005). Qualitative studies in special education. Exceptional Children, 71 (2), 195–207. https://doi.org/10.1177/001440290507100205
Garg, S., & Sharma, S. (2020). Impact of artificial intelligence in special need education to promote inclusive pedagogy. International Journal of Information and Education Technology, 10 (7), 523–527. https://doi.org/10.18178/ijiet.2020.10.7.1418
Chiu, T. K. F., Xia, Q., Zhou, X., Chai, C. S., & Cheng, M. (2023). Systematic literature review on opportunities, challenges, and future research recommendations. Computers and Education: Artificial Intelligence, 4 , 1–15. https://doi.org/10.1010/j.caeai.2022.100118
Chung, P. J., Patel, D. R., & Nizami, I. (2020). Disorder of written expression and dysgraphia: Definition, diagnosis, and management. Translational Pediatrics, 9 (1), 46–54. https://doi.org/10.21037/tp.2019.11.01
Cohen, Z. (2023). Moving beyond Google: Why ChatGPT is the search engine of the future [Blog Post]. Retrieved from https://thecorecollaborative.com/moving-beyond-google-why-chatgpt-is-the-search-engine-of-the-future/ . Accessed 1 Nov 2023
Day, J., Regan, K., Evmenova, A. S., Verbiest, C., Hutchison, A., & Gafurov, B. (2023). The resilience of students and teachers using a virtual writing intervention during COVID-19. Reading & Writing Quarterly, 39 (5), 390–412. https://doi.org/10.1080/10573569.2022.2124562
Edyburn, D. (2021). Universal usability and Universal Design for Learning. Intervention in School and Clinic, 56 (5), 310–315. https://doi.org/10.1177/1053451220963082
Evmenova, A. S., & Regan, K. (2019). Supporting the writing process with technology for students with disabilities. Intervention in School and Clinic, 55 (2), 78–87. https://doi.org/10.1177/1053451219837636
Evmenova, A. S., Regan, K., Boykin, A., Good, K., Hughes, M. D., MacVittie, N. P., Sacco, D., Ahn, S. Y., & Chirinos, D. S. (2016). Emphasizing planning for essay writing with a computer-based graphic organizer. Exceptional Children, 82 (2), 170–191. https://doi.org/10.1177/0014402915591697
Evmenova, A. S., Regan, K., & Hutchison, A. (2018-2023). W EGO RIITE: Writing ef iciently with graphic organizers – responsive instruction while implementing technology ef ectively (Project No. H327S180004) [Grant]. Technology and media services for individuals with disabilities: Stepping-up technology implementation grant, office of special education.
Evmenova, A. S., Regan, K., & Hutchison, A. (2020a). AT for writing: Technology-based graphic organizers with embedded supports. TEACHING Exceptional Children , 52 (4), 266–269. https://doi.org/10.1177/0040059920907571
Evmenova, A. S., Regan, K., Ahn, S. Y., & Good, K. (2020b). Teacher implementation of a technology-based intervention for writing. Learning Disabilities: A Contemporary Journal, 18 (1), 27–47. https://www.ldw-ldcj.org/
Golinkoff, R. M., & Wilson, J. (2023). ChatGPT is a wake-up call to revamp how we teach writing. [Opinion]. Retrieved from https://www.inquirer.com/opinion/commentary/chatgpt-ban-ai-education-writing-critical-thinking-20230202.html . Accessed 1 Nov 2023
Graham, S. (2019). Changing how writing is taught. Review of Research in Education, 43 (1), 277–303. https://doi.org/10.3102/0091732X18821125
Graham, S., Capizzi, A., Harris, K. R., Hebert, M., & Morphy, P. (2014). Teaching writing to middle school students: A national survey. Reading and Writing, 27 , 1015–1042. https://doi.org/10.1007/s11145-013-9495-7
Graham, S., Collins, A. A., & Rigby-Wills, H. (2017). Writing characteristics of students with learning disabilities and typically achieving peers: A meta-analysis. Exceptional Children, 83 (2), 199–218. https://doi.org/10.1177/001440291666407
Guest, G., MacQueen, K. M., & Namey, E. E. (2011). Applied thematic analysis . SAGE Publications.
Hughes C. A., Riccomini P. J., & Morris J. R. (2018). Use explicit instruction. In High leverage practices for inclusive classrooms (pp. 215–236). Routledge. https://doi.org/10.4324/9781315176093 .
Hutchison, A., Evmenova, A. S., Regan, K., & Gafurov, B. (2024). Click, see, do: Using digital scaffolding to support persuasive writing instruction for emerging bilingual learners. Reading Teacher . https://doi.org/10.1002/trtr.2310
Johnson S. M., Kraft M. A., & Papay J. P. (2012). How context matters in high-need schools: The effects of teachers’ working conditions on their professional satisfaction and their students’ achievement. Teachers College Record , 114 , 1–39.
Kiuhara, S. A., Graham, S., & Hawken, L. S. (2009). Teaching writing to highschool students. Journal of Educational Psychology, 101 (1), 136–160. https://doi.org/10.1037/a0013097
Li, J., Link, S., & Hegelheimer, V. (2015). Rethinking the role of automated writing evaluation (AWE) feedback in ESL writing instruction. Journal of Second Language Writing, 27 , 1–18. https://doi.org/10.1016/j.jslw.2014.10.004
Marino, M. T., Vasquez, E., Dieker, L., Basham, J., & Blackorby, J. (2023). The future of artificial intelligence in special education technology. Journal of Special Education Technology, 38 (3), 404–416. https://doi.org/10.1177/01626434231165977
McLeskey, J., Barringer, M.-D., Billingsley, B., Brownell, M., Jackson, D., Kennedy, M., Lewis, T., Maheady, L., Rodriguez, J., Scheeler, M. C., Winn, J., & Ziegler, D. (2017). High-leverage practices in special education . Council for Exceptional Children & CEEDAR Center.
Office of Educational Technology (2023). Artificial intelligence and the future of teaching and learning: Insights and recommendations. Retrieved from https://tech.ed.gov/files/2023/05/ai-future-of-teaching-and-learning-report.pdf . Accessed 1 Nov 2023
Park, V., & Datnow, A. (2017). Ability grouping and differentiated instruction in an era of data-driven decision making. American Journal of Education, 123 (2), 281–306.
Rana, S. (2018). The impact of a computer-based graphic organizer with embedded technology features on the personal narrative writing of upper elementary students with high-incidence disabilities (Publication No. 13420322) [Doctoral dissertation, George Mason University]. ProQuest Dissertation Publishing.
Reeves, T. D., & Chiang, J.-L. (2018). Online interventions to promote teacher data-driven decision making: Optimizing design to maximize impact. Studies in Educational Evaluation, 59 , 256–269. https://doi.org/10.1016/j.stueduc.2018.09.006
Regan, K., Evmenova, A. S., Good, K., Leggit, A, Ahn, S., Gafurov, G., & Mastropieri, M. (2018). Persuasive writing with mobile-based graphic organizers in inclusive classrooms across the curriculum. Journal of Special Education Technology, 33 (1), 3–14. https://doi.org/10.1177/0162643417727292
Regan, K., Evmenova, A. S., Hutchison, A., Day, J., Stephens, M., Verbiest, C., & Gufarov, B. (2021). Steps for success: Making instructional decisions for students’ essay writing. TEACHING Exceptional Children , 54 (3), 202–212. https://doi.org/10.1177/00400599211001085
Regan, K., Evmenova, A. S., & Hutchison, A. (2023a). Specially designed assessment of writing to individualize instruction for students. In K. L. Write, & T. S. Hodges (Eds.), Assessing disciplinary writing in both research and practice (pp. 29–56). IGI Global. https://doi.org/10.4018/978-1-6684-8262-9
Regan, K., Evmenova, A. S., Mergen, R., Verbiest, C., Hutchison, A., Murnan, R., Field, S., & Gafurov, B. (2023b). Exploring the feasibility of virtual professional development to support teachers in making data-based decisions for improving student writing. Learning Disabilities Research & Practice , 38 (1), 40–56. https://doi.org/10.1111/ldrp.12301
Sayeski, K. L., Reno, E. A., & Thoele, J. M. (2023). Specially designed instruction: Operationalizing the delivery of special education services. Exceptionality, 31 (3), 198–210. https://doi.org/10.1080/09362835.2022.2158087
Shermis, M. D. (2014). State-of-the-art automated essay scoring: Competition, results, and future directions from a United States demonstration. Assessing Writing, 20 , 53–76. https://doi.org/10.1016/j.asw.2013.04.001
Steiss, J., Tate, T., Graham, S., Cruz, J., Hevert, M., Wang, J., Moon, Y., Tseng, W., & Warschauer, M. (2023). Comparing the quality of human and ChatGPT feedback on students’ writing. Retrieved from https://osf.io/preprints/edarxiv/ty3em/ . Accessed 1 Nov 2023
Trust, T., Whalen, J., & Mouza, C. (2023). Editorial: ChatGPT: Challenges, opportunities, and implications for teacher education. Contemporary Issues in Technology and Teacher Education, 23 (1), 1–23.
U.S. Department of Education. (2023). Office of Educational Technology, Artificial Intelligence and Future of Teaching and Learning: Insights and Recommendations , Washington, DC.
Wilson, J., & Andrada, G. N. (2016). Using automated feedback to improve writing quality: Opportunities and challenges. In Y. Rosen, S. Ferrara, & M. Mosharraf (Eds.), Handbook of research on technology tools for real-world skill development (pp. 678–703). IGI Global.
Wilson, J., Myers, M. C., & Potter, A. (2022). Investigating the promise of automated writing evaluation for supporting formative writing assessment at scale. Assessment in Education: Principles, Policy, & Practice, 29 (1), 1–17. https://doi.org/10.1080/0969594X.2022.2025762
Wilson, J. (2023). Writing without thinking? There’s a place for ChatGPT – if used properly [Guest Commentary]. Retrieved from https://www.baltimoresun.com/opinion/op-ed/bs-ed-op-0206-chatgpt-tool-20230203-mydxfitujjegndnjwwen4s4x7m-story.html . Accessed 1 Nov 2023
Zdravkova, K. (2022). The potential of artificial intelligence for assistive technology in education. In M. Ivanović, A. Klašnja-Milićević, L. C. Jain (Eds) Handbook on intelligent techniques in the educational process. Learning and analytics in intelligent systems (vol 29). Springer. https://doi.org/10.1007/978-3-031-04662-9_4 .
Download references
This research was supported by the U.S. Department of Education, Office of Special Education Programs [award number: H327S180004]. The views expressed herein do not necessarily represent the positions or policies of the Department of Education. No official endorsement by the U.S. Department of Education of any product, commodity, service, or enterprise mentioned in this publication is intended or should be inferred.
Author information
Authors and affiliations.
George Mason University, 4400 University Dr. MS 1F2, Fairfax, VA, 22030, USA
Anya S. Evmenova, Kelley Regan, Reagan Mergen & Roba Hrisseh
You can also search for this author in PubMed Google Scholar
Corresponding author
Correspondence to Anya S. Evmenova .
Ethics declarations
Research involving human participants.
All the procedures in this study were evaluated and approved by the Institutional Research Board. All authors have complied with the ethical standards in the treatment of our participants.
Informed Consent
Informed parental consent and student assent were obtained for all individual participants in the study.
Conflict of Interest
There is no known conflict of interest to declare.
Additional information
Publisher's note.
Springer Nature remains neutral with regard to jurisdictional claims in published maps and institutional affiliations.
Rights and permissions
Open Access This article is licensed under a Creative Commons Attribution 4.0 International License, which permits use, sharing, adaptation, distribution and reproduction in any medium or format, as long as you give appropriate credit to the original author(s) and the source, provide a link to the Creative Commons licence, and indicate if changes were made. The images or other third party material in this article are included in the article's Creative Commons licence, unless indicated otherwise in a credit line to the material. If material is not included in the article's Creative Commons licence and your intended use is not permitted by statutory regulation or exceeds the permitted use, you will need to obtain permission directly from the copyright holder. To view a copy of this licence, visit http://creativecommons.org/licenses/by/4.0/ .
Reprints and permissions
About this article
Evmenova, A.S., Regan, K., Mergen, R. et al. Improving Writing Feedback for Struggling Writers: Generative AI to the Rescue?. TechTrends (2024). https://doi.org/10.1007/s11528-024-00965-y
Download citation
Accepted : 19 April 2024
Published : 14 May 2024
DOI : https://doi.org/10.1007/s11528-024-00965-y
Share this article
Anyone you share the following link with will be able to read this content:
Sorry, a shareable link is not currently available for this article.
Provided by the Springer Nature SharedIt content-sharing initiative
- Data-based decision-making
- Generative AI
- Essay feedback
- Struggling writers
- Students with and without high-incidence disabilities
- Writing instruction
- Find a journal
- Publish with us
- Track your research
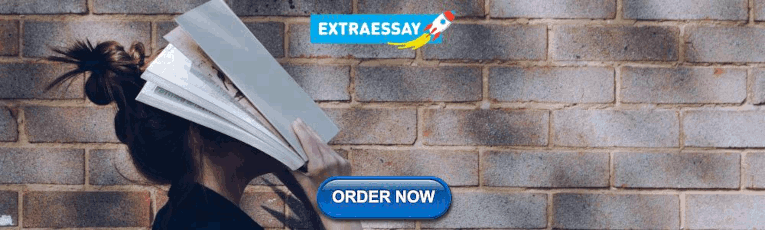
IMAGES
VIDEO
COMMENTS
How to Research and Write Using Generative AI Tools With Dave Birss Liked by 11,612 users. Duration: 1h 15m Skill level: Beginner Released: 3/15/2023. Start my 1-month free trial ...
Generative AI can be a good tool to use, and learning how to use it skillfully and ethically will be an asset to you in your academic journey and beyond. Use the tips below to master using *ChatGPT (or any other generative AI tool) for research and writing. *Use of the term "ChatGPT" in the information below applies to any generative AI program.
Get an overview of some of the key considerations of working with generative AI with hands-on, practical strategies to improve your research and writing. Find out how to summarize complex information, view subjects from multiple perspectives, build user personas and strategic models, analyze writing style, outline ideas, and generate new content.
Generative AI (GenAI) technologies offer new opportunities to advance research and scholarship. This resource page aims to provide Harvard researchers and scholars with basic guidance, information on available resources, and contacts. The content will be regularly updated as these technologies continue to evolve.
AI can make writing faster and better, enabling greater focus on content and ideas — thereby reducing communication costs and enhancing creativity and quality 9, 13, 14, 15. There are two ...
Many recommendations, guidelines and comments are out there regarding the use of Generative AI in research and in other lines of work. Here are a few examples. Best Practices for Using AI When Writing Scientific Manuscripts: Caution, Care, and Consideration: Creative Science Depends on It. Jullian M. Buriak, et al. ACS Nano (2023)
To use AI effectively for research one of the first things you should ask is what are you trying to accomplish and be intentional about the type of tool you use. You may even want to consider if AI is the best sort of tool for what you are looking for. There are lots of different AI tools.
These tools fall under a broad, encompassing term called generative AI that describes technology that can create new text, images, sounds, video, etc. based on information and examples drawn from the internet. In this handout, we will focus on potential uses and pitfalls of generative AI tools that generate text.
1. Use AI to help you brainstorm. ChatGPT-4, OpenAI's latest and paid version of the large language model (LLM), plays a vital role in enhancing my daily research process; it has the capacity to write, create graphics, analyze data, and browse the internet, seemingly as a human would.Rather than using predefined prompts, I conduct generative AI research in a natural and conversational manner ...
Generative AI r efers to a subset of artificial intelligence that focuses on creating new, original content rather than analyzing or classifying existing. data. It involves training models to ...
When you understand how a generative AI tool can fit into your process, you can use it to create better work. After watching this video, you'll be able to start working with ChatGPT effectively.
After watching this video, you will be able to articulate the benefits of using AI in your normal writing/research workflows. By understanding that AI is a great tool rather than a threat to your ...
Research Writing and Generative AI Large Language Models. ... Given this, ChatGPT and other systems should be viewed as a help tool and never a shortcut to actually doing the work of investigating a research problem. Trouble Generating Long-form, Structured Content. ChatGPT and other systems are inadequate at producing long-form content that ...
Fall 2023 examples: Text-based Generative AI tools like ChatGPT can be useful for you when you are brainstorming topic ideas or trying to come up with keywords to use to search on a specific topic. Remember that these tools are not storehouses of knowledge: they are fluency-based text-language generators, and are using their training materials ...
In recent years, Generative Artificial Intelligence (AI) has emerged as a transformative tool for. researchers and writers, revoluti onizing the way knowledge is acquired and content is produced ...
Artificial intelligence (AI) refers to the ability of computer software to perform advanced reasoning and problem-solving tasks. Generative AI refers to software tools that can create new content such as text, images, audio, and video. AI technologies have been around for some time, often operating in the background of many online and ...
This guide is designed to help faculty and students learn more about Generative AI, including the myriad Generative AI tools, recommendations for building AI literacy, how to leverage Generative AI ethically within research, and guidelines for publishing using content generated by Generative AI.
Finding Research Gaps: The Detective's Lens. AI-powered engines like Powerdrill simplify the arduous task of finding gaps in existing literature by helping to highlight overlooked or ...
Chat GPT (Open AI): Very fast. Best used for content creation and basic coding queries. Best used for content creation and basic coding queries. Claude (Anthropic): High content input/output limits.
The resources described in the table represent an incomplete list of tools specifically geared towards exploring and synthesizing research. As generative AI becomes more integrated in online search tools, even the very early stages of research and topic development could incorporate AI.If you have any questions about using these tools for your research, please Email a Librarian.
Generative AI tools can be useful in supporting your academic studies. Here are five ways you can use AI to support your studies, suggestions for how you might provide useful prompts and what some of the limitations are of the AI generated outputs. Be sure to verify any AI outputs against other established sources to ensure their accuracy, and ...
Any such bias could subtly distort scholarly writing produced using these tools. The hallucination problem The most serious worry relates to a well-known limitation of generative AI systems: that ...
AI Tools for Finding, Tracking, and Summarizing Research Literature. Many of the tools below can be used in research to assist with finding, tracking, and summarizing sources. These tools are either free or offer free versions that allow users to access a certain number of searches, summaries, or other features on a monthly or other basis. Bing ...
Other AI-powered tools to improve your writing. Students take note: please make sure that you are using these in accordance with your instructor's rules on use of generative AI and the Academic Integrity Office's guidelines. If you have questions or concerns, please consult your instructor first.
We have the tools to tackle the issue of artificial intelligence. Students using generative AI to write their essays is a problem, but it isn't a crisis, writes Christopher Hallenbrook. ... THE podcast: how to use generative AI in your teaching and research. Original essays written in seconds: how 'transformers' will change assessment ...
A new study by Ithaka S+R seeks to gain insight into the technology's potential to transform the production of academic scholarship. The rapid rise of generative artificial intelligence (AI) has confronted the scholarly publishing world with the potential risks and benefits of using the new technology in the production of academic research and writing.
The trick is that the neural networks we use as generative models have a number of parameters significantly smaller than the amount of data we train them on, so the models are forced to discover and efficiently internalize the essence of the data in order to generate it. Generative models have many short-term applications. But in the long run ...
No, this policy refers to generative AI and AI-assisted technologies, such as Large Language Models, when they are used to create original content for publication. This policy does not prevent the use of AI and AI-assisted tools in formal research design or research methods. We recognize that the use of such technology is common in many fields.
5. ATLAS.ti. For: qualitative data analysis Whether you're working with interview transcripts, focus group discussions, or open-ended surveys, ATLAS.ti provides a set of tools to help you extract meaningful insights from your data. You can analyze texts to uncover hidden patterns embedded in responses, or create a visualization of terms that appear most often in your research.
Generative AI has the potential to support teachers with writing instruction and feedback. The purpose of this study was to explore and compare feedback and data-based instructional suggestions from teachers and those generated by different AI tools. Essays from students with and without disabilities who struggled with writing and needed a technology-based writing intervention were analyzed ...