Have a language expert improve your writing
Run a free plagiarism check in 10 minutes, generate accurate citations for free.
- Knowledge Base
Methodology
- Cross-Sectional Study | Definition, Uses & Examples
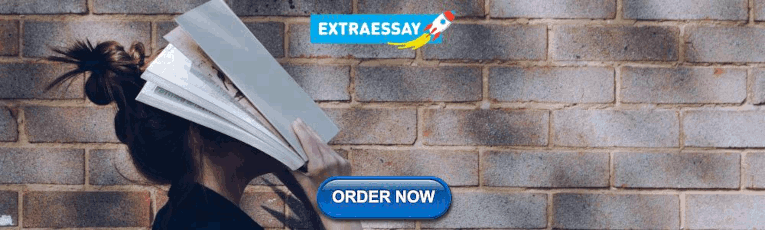
Cross-Sectional Study | Definition, Uses & Examples
Published on May 8, 2020 by Lauren Thomas . Revised on June 22, 2023.
A cross-sectional study is a type of research design in which you collect data from many different individuals at a single point in time. In cross-sectional research, you observe variables without influencing them.
Researchers in economics, psychology, medicine, epidemiology, and the other social sciences all make use of cross-sectional studies in their work. For example, epidemiologists who are interested in the current prevalence of a disease in a certain subset of the population might use a cross-sectional design to gather and analyze the relevant data.
Table of contents
Cross-sectional vs longitudinal studies, when to use a cross-sectional design, how to perform a cross-sectional study, advantages and disadvantages of cross-sectional studies, other interesting articles, frequently asked questions about cross-sectional studies.
The opposite of a cross-sectional study is a longitudinal study . While cross-sectional studies collect data from many subjects at a single point in time, longitudinal studies collect data repeatedly from the same subjects over time, often focusing on a smaller group of individuals that are connected by a common trait.
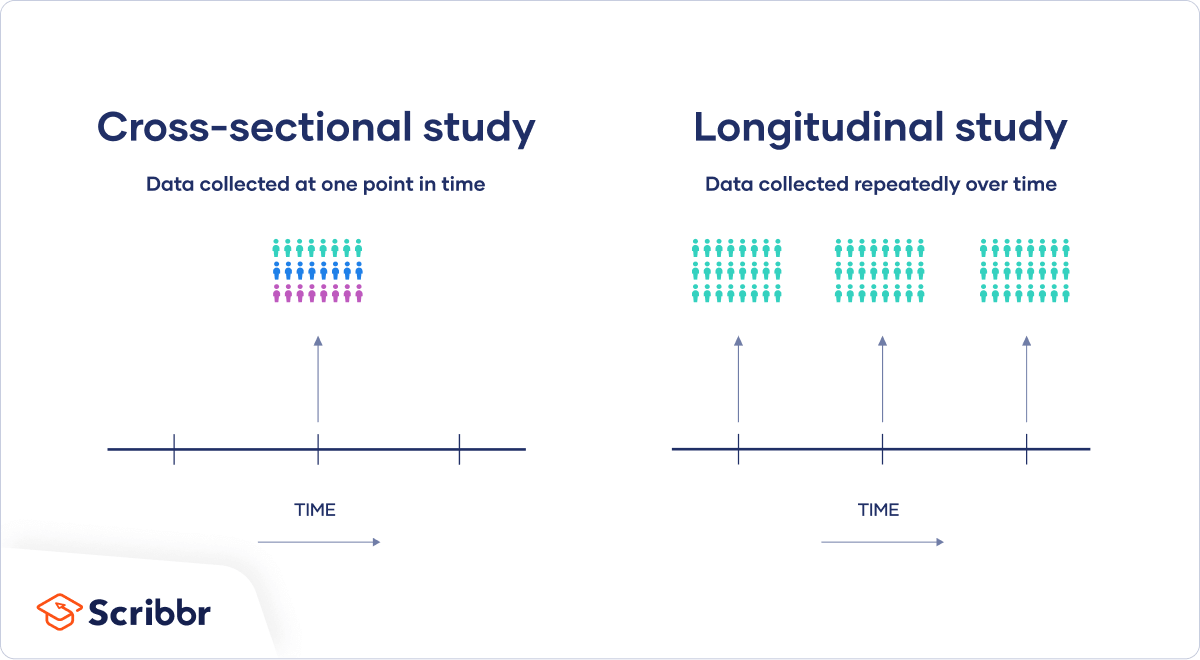
Both types are useful for answering different kinds of research questions . A cross-sectional study is a cheap and easy way to gather initial data and identify correlations that can then be investigated further in a longitudinal study.
Here's why students love Scribbr's proofreading services
Discover proofreading & editing
When you want to examine the prevalence of some outcome at a certain moment in time, a cross-sectional study is the best choice.
Sometimes a cross-sectional study is the best choice for practical reasons – for instance, if you only have the time or money to collect cross-sectional data, or if the only data you can find to answer your research question was gathered at a single point in time.
As cross-sectional studies are cheaper and less time-consuming than many other types of study, they allow you to easily collect data that can be used as a basis for further research.
Descriptive vs analytical studies
Cross-sectional studies can be used for both analytical and descriptive purposes:
- An analytical study tries to answer how or why a certain outcome might occur.
- A descriptive study only summarizes said outcome using descriptive statistics.
To implement a cross-sectional study, you can rely on data assembled by another source or collect your own. Governments often make cross-sectional datasets freely available online.
Prominent examples include the censuses of several countries like the US or France , which survey a cross-sectional snapshot of the country’s residents on important measures. International organizations like the World Health Organization or the World Bank also provide access to cross-sectional datasets on their websites.
However, these datasets are often aggregated to a regional level, which may prevent the investigation of certain research questions. You will also be restricted to whichever variables the original researchers decided to study.
If you want to choose the variables in your study and analyze your data on an individual level, you can collect your own data using research methods such as surveys . It’s important to carefully design your questions and choose your sample .
Like any research design , cross-sectional studies have various benefits and drawbacks.
- Because you only collect data at a single point in time, cross-sectional studies are relatively cheap and less time-consuming than other types of research.
- Cross-sectional studies allow you to collect data from a large pool of subjects and compare differences between groups.
- Cross-sectional studies capture a specific moment in time. National censuses, for instance, provide a snapshot of conditions in that country at that time.
Disadvantages
- It is difficult to establish cause-and-effect relationships using cross-sectional studies, since they only represent a one-time measurement of both the alleged cause and effect.
- Since cross-sectional studies only study a single moment in time, they cannot be used to analyze behavior over a period of time or establish long-term trends.
- The timing of the cross-sectional snapshot may be unrepresentative of behavior of the group as a whole. For instance, imagine you are looking at the impact of psychotherapy on an illness like depression. If the depressed individuals in your sample began therapy shortly before the data collection, then it might appear that therapy causes depression even if it is effective in the long term.
Receive feedback on language, structure, and formatting
Professional editors proofread and edit your paper by focusing on:
- Academic style
- Vague sentences
- Style consistency
See an example
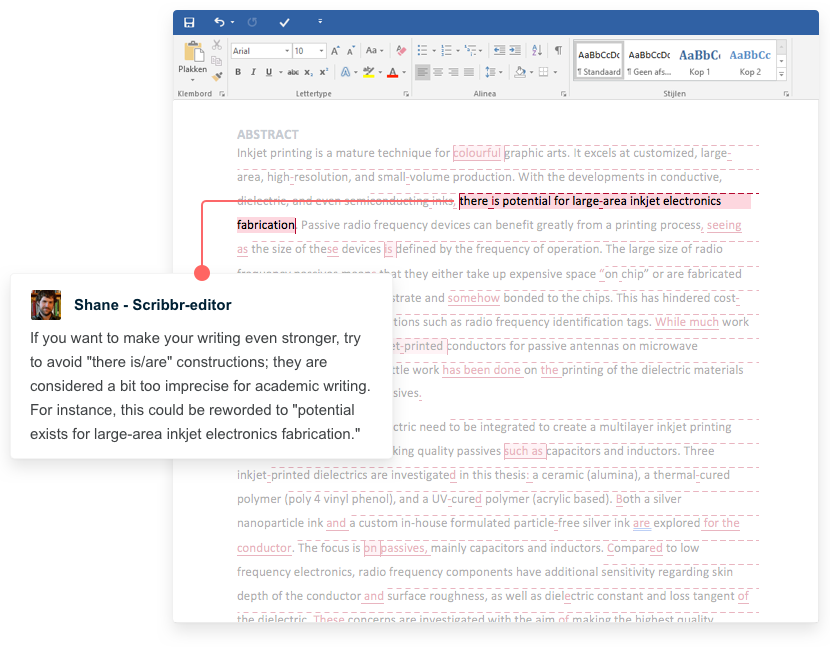
If you want to know more about statistics , methodology , or research bias , make sure to check out some of our other articles with explanations and examples.
- Normal distribution
- Degrees of freedom
- Null hypothesis
- Discourse analysis
- Control groups
- Mixed methods research
- Non-probability sampling
- Quantitative research
- Ecological validity
Research bias
- Rosenthal effect
- Implicit bias
- Cognitive bias
- Selection bias
- Negativity bias
- Status quo bias
Longitudinal studies and cross-sectional studies are two different types of research design . In a cross-sectional study you collect data from a population at a specific point in time; in a longitudinal study you repeatedly collect data from the same sample over an extended period of time.
Cross-sectional studies are less expensive and time-consuming than many other types of study. They can provide useful insights into a population’s characteristics and identify correlations for further research.
Sometimes only cross-sectional data is available for analysis; other times your research question may only require a cross-sectional study to answer it.
Cross-sectional studies cannot establish a cause-and-effect relationship or analyze behavior over a period of time. To investigate cause and effect, you need to do a longitudinal study or an experimental study .
Cite this Scribbr article
If you want to cite this source, you can copy and paste the citation or click the “Cite this Scribbr article” button to automatically add the citation to our free Citation Generator.
Thomas, L. (2023, June 22). Cross-Sectional Study | Definition, Uses & Examples. Scribbr. Retrieved April 15, 2024, from https://www.scribbr.com/methodology/cross-sectional-study/
Is this article helpful?
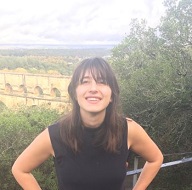
Lauren Thomas
Other students also liked, longitudinal study | definition, approaches & examples, correlational research | when & how to use, survey research | definition, examples & methods, "i thought ai proofreading was useless but..".
I've been using Scribbr for years now and I know it's a service that won't disappoint. It does a good job spotting mistakes”
Cross-Sectional Study: Definition, Designs & Examples
Julia Simkus
Editor at Simply Psychology
BA (Hons) Psychology, Princeton University
Julia Simkus is a graduate of Princeton University with a Bachelor of Arts in Psychology. She is currently studying for a Master's Degree in Counseling for Mental Health and Wellness in September 2023. Julia's research has been published in peer reviewed journals.
Learn about our Editorial Process
Saul Mcleod, PhD
Editor-in-Chief for Simply Psychology
BSc (Hons) Psychology, MRes, PhD, University of Manchester
Saul Mcleod, PhD., is a qualified psychology teacher with over 18 years of experience in further and higher education. He has been published in peer-reviewed journals, including the Journal of Clinical Psychology.
Olivia Guy-Evans, MSc
Associate Editor for Simply Psychology
BSc (Hons) Psychology, MSc Psychology of Education
Olivia Guy-Evans is a writer and associate editor for Simply Psychology. She has previously worked in healthcare and educational sectors.
On This Page:
A cross-sectional study design is a type of observational study, or descriptive research, that involves analyzing information about a population at a specific point in time.
This design measures the prevalence of an outcome of interest in a defined population. It provides a snapshot of the characteristics of the population at a single point in time.
It can be used to assess the prevalence of outcomes and exposures, determine relationships among variables, and generate hypotheses about causal connections between factors to be explored in experimental designs.
Typically, these studies are used to measure the prevalence of health outcomes and describe the characteristics of a population.
In this study, researchers examine a group of participants and depict what already exists in the population without manipulating any variables or interfering with the environment.
Cross-sectional studies aim to describe a variable , not measure it. They can be beneficial for describing a population or “taking a snapshot” of a group of individuals at a single moment in time.
In epidemiology and public health research, cross-sectional studies are used to assess exposure (cause) and disease (effect) and compare the rates of diseases and symptoms of an exposed group with an unexposed group.
Cross-sectional studies are also unique because researchers are able to look at numerous characteristics at once.
For example, a cross-sectional study could be used to investigate whether exposure to certain factors, such as overeating, might correlate to particular outcomes, such as obesity.
While this study cannot prove that overeating causes obesity, it can draw attention to a relationship that might be worth investigating.
Cross-sectional studies can be categorized based on the nature of the data collection and the type of data being sought.
Analytical Studies
In analytical cross-sectional studies, researchers investigate an association between two parameters. They collect data for exposures and outcomes at one specific time to measure an association between an exposure and a condition within a defined population.
The purpose of this type of study is to compare health outcome differences between exposed and unexposed individuals.
Descriptive Studies
- Descriptive cross-sectional studies are purely used to characterize and assess the prevalence and distribution of one or many health outcomes in a defined population.
- They can assess how frequently, widely, or severely a specific variable occurs throughout a specific demographic.
- This is the most common type of cross-sectional study.
- Evaluating the COVID-19 positivity rates among vaccinated and unvaccinated adolescents
- Investigating the prevalence of dysfunctional breathing in patients treated for asthma in primary care (Wang & Cheng, 2020)
- Analyzing whether individuals in a community have any history of mental illness and whether they have used therapy to help with their mental health
- Comparing grades of elementary school students whose parents come from different income levels
- Determining the association between gender and HIV status (Setia, 2016)
- Investigating suicide rates among individuals who have at least one parent with chronic depression
- Assessing the prevalence of HIV and risk behaviors in male sex workers (Shinde et al., 2009)
- Examining sleep quality and its demographic and psychological correlates among university students in Ethiopia (Lemma et al., 2012)
- Calculating what proportion of people served by a health clinic in a particular year have high cholesterol
- Analyzing college students’ distress levels with regard to their year level (Leahy et al., 2010)
Simple and Inexpensive
These studies are quick, cheap, and easy to conduct as they do not require any follow-up with subjects and can be done through self-report surveys.
Minimal room for error
Because all of the variables are analyzed at once, and data does not need to be collected multiple times, there will likely be fewer mistakes as a higher level of control is obtained.
Multiple variables and outcomes can be researched and compared at once
Researchers are able to look at numerous characteristics (ie, age, gender, ethnicity, and education level) in one study.
The data can be a starting point for future research
The information obtained from cross-sectional studies enables researchers to conduct further data analyses to explore any causal relationships in more depth.
Limitations
Does not help determine cause and effect.
Cross-sectional studies can be influenced by an antecedent consequent bias which occurs when it cannot be determined whether exposure preceded disease. (Alexander et al.)
Report bias is probable
Cross-sectional studies rely on surveys and questionnaires, which might not result in accurate reporting as there is no way to verify the information presented.
The timing of the snapshot is not always representative
Cross-sectional studies do not provide information from before or after the report was recorded and only offer a single snapshot of a point in time.
It cannot be used to analyze behavior over a period of time
Cross-sectional studies are designed to look at a variable at a particular moment, while longitudinal studies are more beneficial for analyzing relationships over extended periods.
Cross-Sectional vs. Longitudinal
Both cross-sectional and longitudinal studies are observational and do not require any interference or manipulation of the study environment.
However, cross-sectional studies differ from longitudinal studies in that cross-sectional studies look at a characteristic of a population at a specific point in time, while longitudinal studies involve studying a population over an extended period.
Longitudinal studies require more time and resources and can be less valid as participants might quit the study before the data has been fully collected.
Unlike cross-sectional studies, researchers can use longitudinal data to detect changes in a population and, over time, establish patterns among subjects.
Cross-sectional studies can be done much quicker than longitudinal studies and are a good starting point to establish any associations between variables, while longitudinal studies are more timely but are necessary for studying cause and effect.
Alexander, L. K., Lopez, B., Ricchetti-Masterson, K., & Yeatts, K. B. (n.d.). Cross-sectional Studies. Eric Notebook. Retrieved from https://sph.unc.edu/wp-content/uploads/sites/112/2015/07/nciph_ERIC8.pdf
Cherry, K. (2019, October 10). How Does the Cross-Sectional Research Method Work? Verywell Mind. Retrieved from https://www.verywellmind.com/what-is-a-cross-sectional-study-2794978
Cross-sectional vs. longitudinal studies. Institute for Work & Health. (2015, August). Retrieved from https://www.iwh.on.ca/what-researchers-mean-by/cross-sectional-vs-longitudinal-studies
Leahy, C. M., Peterson, R. F., Wilson, I. G., Newbury, J. W., Tonkin, A. L., & Turnbull, D. (2010). Distress levels and self-reported treatment rates for medicine, law, psychology and mechanical engineering tertiary students: cross-sectional study. The Australian and New Zealand journal of psychiatry, 44(7), 608–615.
Lemma, S., Gelaye, B., Berhane, Y. et al. Sleep quality and its psychological correlates among university students in Ethiopia: a cross-sectional study. BMC Psychiatry 12, 237 (2012).
Wang, X., & Cheng, Z. (2020). Cross-Sectional Studies: Strengths, Weaknesses, and Recommendations. Chest, 158(1S), S65–S71.
Setia M. S. (2016). Methodology Series Module 3: Cross-sectional Studies. Indian journal of dermatology, 61 (3), 261–264.
Shinde S, Setia MS, Row-Kavi A, Anand V, Jerajani H. Male sex workers: Are we ignoring a risk group in Mumbai, India? Indian J Dermatol Venereol Leprol. 2009;75:41–6.
Further Information
- Setia, M. S. (2016). Methodology series module 3: Cross-sectional studies. Indian journal of dermatology, 61(3), 261.
- Sedgwick, P. (2014). Cross sectional studies: advantages and disadvantages. Bmj, 348.
1. Are cross-sectional studies qualitative or quantitative?
Cross-sectional studies can be either qualitative or quantitative , depending on the type of data they collect and how they analyze it. Often, the two approaches are combined in mixed-methods research to get a more comprehensive understanding of the research problem.
2. What’s the difference between cross-sectional and cohort studies?
A cohort study is a type of longitudinal study that samples a group of people with a common characteristic. One key difference is that cross-sectional studies measure a specific moment in time, whereas cohort studies follow individuals over extended periods.
Another difference between these two types of studies is the subject pool. In cross-sectional studies, researchers select a sample population and gather data to determine the prevalence of a problem.
Cohort studies, on the other hand, begin by selecting a population of individuals who are already at risk for a specific disease.
3. What’s the difference between cross-sectional and case-control studies?
Case-control studies differ from cross-sectional studies in that case-control studies compare groups retrospectively and cannot be used to calculate relative risk.
In these studies, researchers study one group of people who have developed a particular condition and compare them to a sample without the disease.
Case-control studies are used to determine what factors might be associated with the condition and help researchers form hypotheses about a population.
4. Does a cross-sectional study have a control group?
A cross-sectional study does not need to have a control group , as the population studied is not selected based on exposure.
In a cross-sectional study, data are collected from a sample of the target population at a specific point in time, and everyone in the sample is assessed in the same way. There isn’t a manipulation of variables or a control group as there would be in an experimental study design.
5. Is a cross-sectional study prospective or retrospective?
A cross-sectional study is generally considered neither prospective nor retrospective because it provides a “snapshot” of a population at a single point in time.
Cross-sectional studies are not designed to follow individuals forward in time ( prospective ) or look back at historical data ( retrospective ), as they analyze data from a specific point in time.

Have a language expert improve your writing
Run a free plagiarism check in 10 minutes, automatically generate references for free.
- Knowledge Base
- Methodology
- Cross-Sectional Study | Definitions, Uses & Examples
Cross-Sectional Study | Definitions, Uses & Examples
Published on 5 May 2022 by Lauren Thomas .
A cross-sectional study is a type of research design in which you collect data from many different individuals at a single point in time. In cross-sectional research, you observe variables without influencing them.
Researchers in economics, psychology, medicine, epidemiology, and the other social sciences all make use of cross-sectional studies in their work. For example, epidemiologists who are interested in the current prevalence of a disease in a certain subset of the population might use a cross-sectional design to gather and analyse the relevant data.
Table of contents
Cross-sectional vs longitudinal studies, when to use a cross-sectional design, how to perform a cross-sectional study, advantages and disadvantages of cross-sectional studies, frequently asked questions about cross-sectional studies.
The opposite of a cross-sectional study is a longitudinal study . While cross-sectional studies collect data from many subjects at a single point in time, longitudinal studies collect data repeatedly from the same subjects over time, often focusing on a smaller group of individuals connected by a common trait.
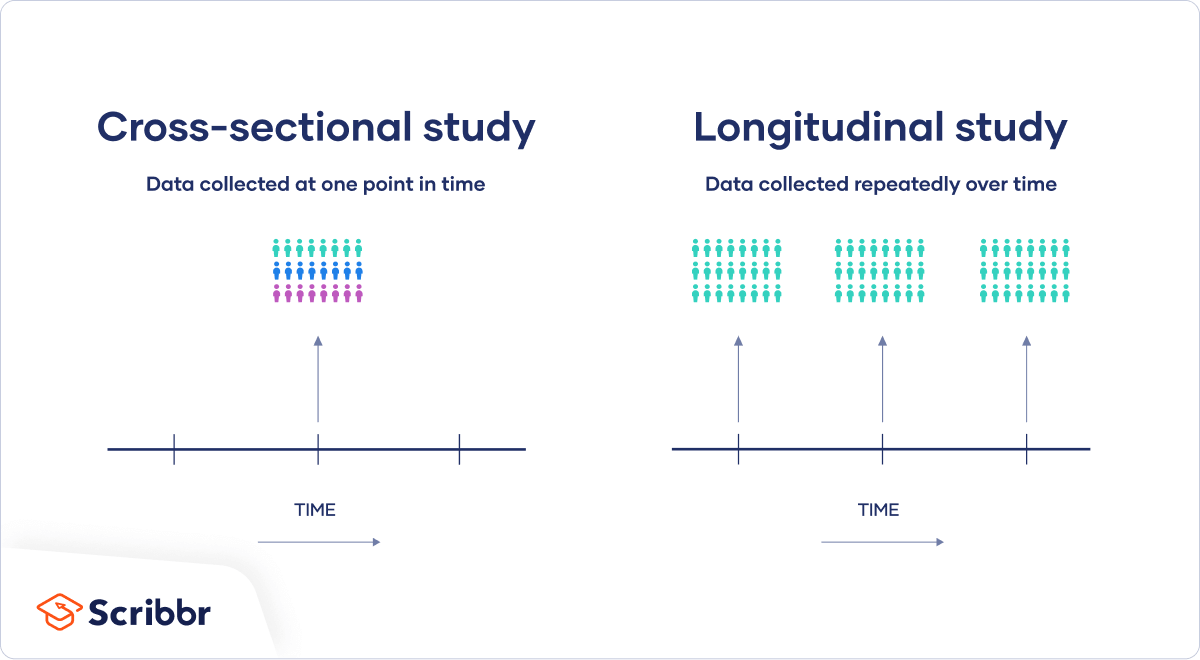
Both types are useful for answering different kinds of research questions . A cross-sectional study is a cheap and easy way to gather initial data and identify correlations that can then be investigated further in a longitudinal study.
Prevent plagiarism, run a free check.
When you want to examine the prevalence of some outcome at a certain moment in time, a cross-sectional study is the best choice.
Sometimes a cross-sectional study is the best choice for practical reasons – for instance, if you only have the time or money to collect cross-sectional data, or if the only data you can find to answer your research question were gathered at a single point in time.
As cross-sectional studies are cheaper and less time-consuming than many other types of study, they allow you to easily collect data that can be used as a basis for further research.
Descriptive vs analytical studies
Cross-sectional studies can be used for both analytical and descriptive purposes:
- An analytical study tries to answer how or why a certain outcome might occur.
- A descriptive study only summarises said outcome using descriptive statistics.
To implement a cross-sectional study, you can rely on data assembled by another source or collect your own. Governments often make cross-sectional datasets freely available online.
Prominent examples include the censuses of several countries like the US or France , which survey a cross-sectional snapshot of the country’s residents on important measures. International organisations like the World Health Organization or the World Bank also provide access to cross-sectional datasets on their websites.
However, these datasets are often aggregated to a regional level, which may prevent the investigation of certain research questions. You will also be restricted to whichever variables the original researchers decided to study.
If you want to choose the variables in your study and analyse your data on an individual level, you can collect your own data using research methods such as surveys . It’s important to carefully design your questions and choose your sample .
Like any research design , cross-sectional studies have various benefits and drawbacks.
- Because you only collect data at a single point in time, cross-sectional studies are relatively cheap and less time-consuming than other types of research.
- Cross-sectional studies allow you to collect data from a large pool of subjects and compare differences between groups.
- Cross-sectional studies capture a specific moment in time. National censuses, for instance, provide a snapshot of conditions in that country at that time.
Disadvantages
- It is difficult to establish cause-and-effect relationships using cross-sectional studies, since they only represent a one-time measurement of both the alleged cause and effect.
- Since cross-sectional studies only study a single moment in time, they cannot be used to analyse behavior over a period of time or establish long-term trends.
- The timing of the cross-sectional snapshot may be unrepresentative of behaviour of the group as a whole. For instance, imagine you are looking at the impact of psychotherapy on an illness like depression. If the depressed individuals in your sample began therapy shortly before the data collection, then it might appear that therapy causes depression even if it is effective in the long term.
Longitudinal studies and cross-sectional studies are two different types of research design . In a cross-sectional study you collect data from a population at a specific point in time; in a longitudinal study you repeatedly collect data from the same sample over an extended period of time.
Cross-sectional studies are less expensive and time-consuming than many other types of study. They can provide useful insights into a population’s characteristics and identify correlations for further research.
Sometimes only cross-sectional data are available for analysis; other times your research question may only require a cross-sectional study to answer it.
Cross-sectional studies cannot establish a cause-and-effect relationship or analyse behaviour over a period of time. To investigate cause and effect, you need to do a longitudinal study or an experimental study .
Cite this Scribbr article
If you want to cite this source, you can copy and paste the citation or click the ‘Cite this Scribbr article’ button to automatically add the citation to our free Reference Generator.
Thomas, L. (2022, May 05). Cross-Sectional Study | Definitions, Uses & Examples. Scribbr. Retrieved 15 April 2024, from https://www.scribbr.co.uk/research-methods/cross-sectional-design/
Is this article helpful?
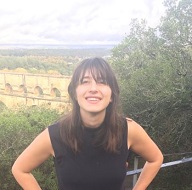
Lauren Thomas
Other students also liked, longitudinal study | definition, approaches & examples, descriptive research design | definition, methods & examples, correlational research | guide, design & examples.
- Bipolar Disorder
- Therapy Center
- When To See a Therapist
- Types of Therapy
- Best Online Therapy
- Best Couples Therapy
- Best Family Therapy
- Managing Stress
- Sleep and Dreaming
- Understanding Emotions
- Self-Improvement
- Healthy Relationships
- Student Resources
- Personality Types
- Guided Meditations
- Verywell Mind Insights
- 2023 Verywell Mind 25
- Mental Health in the Classroom
- Editorial Process
- Meet Our Review Board
- Crisis Support
How Do Cross-Sectional Studies Work?
Gathering Data From a Single Point in Time
Kendra Cherry, MS, is a psychosocial rehabilitation specialist, psychology educator, and author of the "Everything Psychology Book."
:max_bytes(150000):strip_icc():format(webp)/IMG_9791-89504ab694d54b66bbd72cb84ffb860e.jpg)
Steven Gans, MD is board-certified in psychiatry and is an active supervisor, teacher, and mentor at Massachusetts General Hospital.
:max_bytes(150000):strip_icc():format(webp)/steven-gans-1000-51582b7f23b6462f8713961deb74959f.jpg)
What Is a Cross-Sectional Study?
- Defining Characteristics
Advantages of Cross-Sectional Studies
Challenges of cross-sectional studies, cross-sectional vs. longitudinal studies.
Verywell / Jessica Olah
A cross-sectional study looks at data at a single point in time. The participants in this type of study are selected based on particular variables of interest. Cross-sectional studies are often used in developmental psychology , but this method is also used in many other areas, including social science and education.
Cross-sectional studies are observational in nature and are known as descriptive research, not causal or relational, meaning that you can't use them to determine the cause of something, such as a disease. Researchers record the information that is present in a population, but they do not manipulate variables .
This type of research can be used to describe characteristics that exist in a community, but not to determine cause-and-effect relationships between different variables. This method is often used to make inferences about possible relationships or to gather preliminary data to support further research and experimentation.
Example: Researchers studying developmental psychology might select groups of people who are different ages but investigate them at one point in time. By doing this, any differences among the age groups can be attributed to age differences rather than something that happened over time.
Defining Characteristics of Cross-Sectional Studies
Some of the key characteristics of a cross-sectional study include:
- The study takes place at a single point in time
- It does not involve manipulating variables
- It allows researchers to look at numerous characteristics at once (age, income, gender, etc.)
- It's often used to look at the prevailing characteristics in a given population
- It can provide information about what is happening in a current population
Think of a cross-sectional study as a snapshot of a particular group of people at a given point in time. Unlike longitudinal studies, which look at a group of people over an extended period, cross-sectional studies are used to describe what is happening at the present moment.This type of research is frequently used to determine the prevailing characteristics in a population at a certain point in time. For example, a cross-sectional study might be used to determine if exposure to specific risk factors might correlate with particular outcomes.
A researcher might collect cross-sectional data on past smoking habits and current diagnoses of lung cancer, for example. While this type of study cannot demonstrate cause and effect, it can provide a quick look at correlations that may exist at a particular point.
For example, researchers may find that people who reported engaging in certain health behaviors were also more likely to be diagnosed with specific ailments. While a cross-sectional study cannot prove for certain that these behaviors caused the condition, such studies can point to a relationship worth investigating further.
Cross-sectional studies are popular because they have several benefits that are useful to researchers.
Inexpensive and Fast
Cross-sectional studies typically allow researchers to collect a great deal of information quickly. Data is often obtained inexpensively using self-report surveys . Researchers are then able to amass large amounts of information from a large pool of participants.
For example, a university might post a short online survey about library usage habits among biology majors, and the responses would be recorded in a database automatically for later analysis. This is a simple, inexpensive way to encourage participation and gather data across a wide swath of individuals who fit certain criteria.
Can Assess Multiple Variables
Researchers can collect data on a few different variables to see how they affect a certain condition. For example, differences in sex, age, educational status, and income might correlate with voting tendencies or give market researchers clues about purchasing habits.
Might Prompt Further Study
Although researchers can't use cross-sectional studies to determine causal relationships, these studies can provide useful springboards to further research. For example, when looking at a public health issue, such as whether a particular behavior might be linked to a particular illness, researchers might utilize a cross-sectional study to look for clues that can spur further experimental studies.
For example, researchers might be interested in learning how exercise influences cognitive health as people age. They might collect data from different age groups on how much exercise they get and how well they perform on cognitive tests. Conducting such a study can give researchers clues about the types of exercise that might be most beneficial to the elderly and inspire further experimental research on the subject.
No method of research is perfect. Cross-sectional studies also have potential drawbacks.
Difficulties in Determining Causal Effects
Researchers can't always be sure that the conditions a cross-sectional study measures are the result of a particular factor's influence. In many cases, the differences among individuals could be attributed to variation among the study subjects. In this way, cause-and-effect relationships are more difficult to determine in a cross-sectional study than they are in a longitudinal study. This type of research simply doesn't allow for conclusions about causation.
For example, a study conducted some 20 years ago queried thousands of women about their consumption of diet soft drinks. The results of the study, published in the medical journal Stroke , associated diet soft drink intake with stroke risk that was greater than that of those who did not consume such beverages. In other words, those who drank lots of diet soda were more prone to strokes. However, correlation does not equal causation. The increased stroke risk might arise from any number of factors that tend to occur among those who drink diet beverages. For example, people who consume sugar-free drinks might be more likely to be overweight or diabetic than those who drink the regular versions. Therefore, they might be at greater risk of stroke—regardless of what they drink.
Cohort Differences
Groups can be affected by cohort differences that arise from the particular experiences of a group of people. For example, individuals born during the same period might witness the same important historical events, but their geographic regions, religious affiliations, political beliefs, and other factors might affect how they perceive such events.
Report Biases
Surveys and questionnaires about certain aspects of people's lives might not always result in accurate reporting. For example, respondents might not disclose certain behaviors or beliefs out of embarrassment, fear, or other limiting perception. Typically, no mechanism for verifying this information exists.
Cross-sectional research differs from longitudinal studies in several important ways. The key difference is that a cross-sectional study is designed to look at a variable at a particular point in time. A longitudinal study evaluates multiple measures over an extended period to detect trends and changes.
Evaluates variable at single point in time
Participants less likely to drop out
Uses new participant(s) with each study
Measures variable over time
Requires more resources
More expensive
Subject to selective attrition
Follows same participants over time
Longitudinal studies tend to require more resources; these are often more expensive than those used by cross-sectional studies. They are also more likely to be influenced by what is known as selective attrition , which means that some individuals are more likely to drop out of a study than others. Because a longitudinal study occurs over a span of time, researchers can lose track of subjects. Individuals might lose interest, move to another city, change their minds about participating, etc. This can influence the validity of the study.
One of the advantages of cross-sectional studies is that data is collected all at once, so participants are less likely to quit the study before data is fully collected.
A Word From Verywell
Cross-sectional studies can be useful research tools in many areas of health research. By learning about what is going on in a specific population, researchers can improve their understanding of relationships among certain variables and develop additional studies that explore these conditions in greater depth.
Levin KA. Study design III: Cross-sectional studies . Evid Based Dent . 2006;7(1):24-5. doi:10.1038/sj.ebd.6400375
Morin JF, Olsson C, Atikcan EO, eds. Research Methods in the Social Sciences: An A-Z of Key Concepts . Oxford University Press; 2021.
Abbasi J. Unpacking a recent study linking diet soda with stroke risks . JAMA . 2019;321(16):1554-1555. doi:10.1001/jama.2019.2123
Setia MS. Methodology series module 3: Cross-sectional studies . Indian J Dermatol . 2016;61(3):261-4. doi:10.4103/0019-5154.182410
By Kendra Cherry, MSEd Kendra Cherry, MS, is a psychosocial rehabilitation specialist, psychology educator, and author of the "Everything Psychology Book."

What (Exactly) Is A Cross-Sectional Study?
A plain-language explanation & definition (with examples).
By: Derek Jansen (MBA) | June 2020
If you’ve just started out on your dissertation, thesis or research project and it’s your first time carrying out formal research, you’ve probably encountered the terms “cross-sectional study” and “cross-sectional research” and are wondering what exactly they mean. In this post, we’ll explain exactly :
- What a cross-sectional study is (and what the alternative approach is)
- What the main advantages of a cross-sectional study are
- What the main disadvantages of a cross-sectional study are
- Whether you should use a cross-sectional or longitudinal study for your research
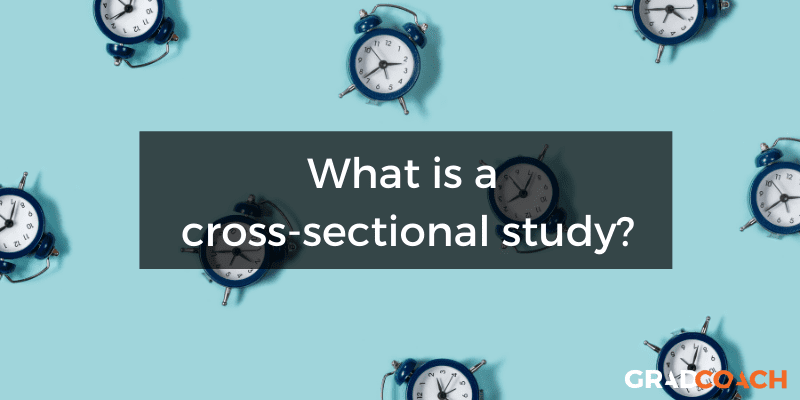
What (exactly) is a cross-sectional study?
A cross-sectional study (also referred to as cross-sectional research) is simply a study in which data are collected at one point in time . In other words, data are collected on a snapshot basis, as opposed to collecting data at multiple points in time (for example, once a week, once a month, etc) and assessing how it changes over time.
Example: Cross-Sectional vs Longitudinal
Here’s an example of what this looks like in practice:
Cross-sectional study: a study which assesses a group of people’s attitudes and feelings towards a newly elected president, directly after the election happened.
Longitudinal study: a study which assesses how people’s attitudes towards the president changed over a period of 3 years after the president is elected, assessing sentiment every 6 months.
As you can probably see, while both these studies are analysing the same topic (people’s sentiment towards the president), they each have a different focus. The cross-sectional study is interested in what people are feeling and thinking “ right now ”, whereas the longitudinal study is interested in not just what people are feeling and thinking, but how those thoughts and feelings change over time .
What are the advantages of a cross-sectional study?
There are many advantages to taking a cross-sectional approach, which makes it the more popular option for dissertations and theses. Some main advantages are:
- Speed – given the nature of a cross-sectional study, you can complete your research relatively quickly, as information only needs to be gathered once.
- Cost – because information only needs to be collected once, the cost is lower than a longitudinal approach.
- Control – because the data are only collected at one point in time, you have a lot more control over the measurement process (i.e. you don’t need to worry about measurement instruments changing over a period of years).
- Flexibility – using a cross-sectional approach, you can measure multiple factors at once. Your study can be descriptive (assessing the prevalence of something), analytical (assessing the relationship between two or more things) or both.
Need a helping hand?
What are the disadvantages of a cross-sectional study?
While the cross-sectional approach to research has many advantages, it (naturally) has its limitations and disadvantages too. Some of the main disadvantages are:
- Static – cross-sectional studies cannot establish any sequence of events, as they only assess data with a snapshot view.
- Causality – because cross-sectional studies look at data at a single point in time (no sequence of events), it’s sometimes difficult to understand which way causality flows – for example, does A cause B, or does B cause A? Without knowing whether A or B came first, it’s not always easy to tell which causes which.
- Sensitivity to timing – the exact time at which data are collected can have a large impact on the results, and therefore the findings of the study may not be representative.
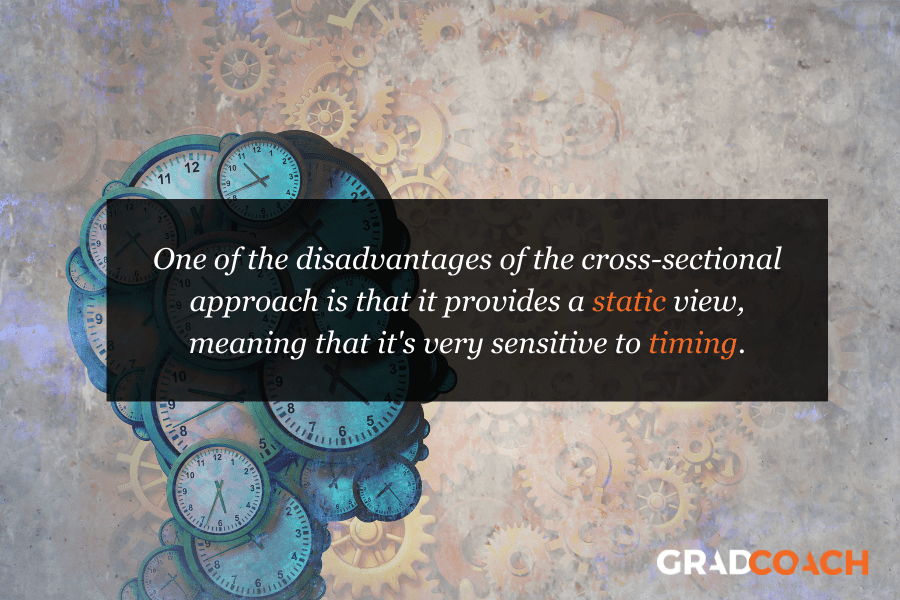
Should I use a cross-sectional study or longitudinal study design?
It depends… Your decision to use a cross-sectional or longitudinal approach needs to be informed by your overall research aims, objectives and research questions . As with most research design choices, the research aims will heavily influence your approach.
For example, if your research objective is to get a snapshot view of something, then a cross-sectional approach should work well for you. However, if your research aim is to understand how something has changed over time, a longitudinal approach might be more appropriate.
If you’re trying to make this decision for a dissertation or thesis, you also need to consider the practical limitations such as time and access to data. Chances are, you won’t have the luxury of conducting your research over a period of a few years, so you might be “forced” into a cross-sectional approach due to time restrictions.
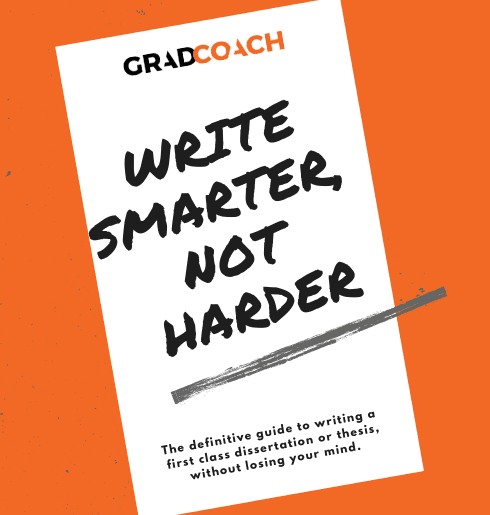
Psst… there’s more (for free)
This post is part of our dissertation mini-course, which covers everything you need to get started with your dissertation, thesis or research project.
You Might Also Like:
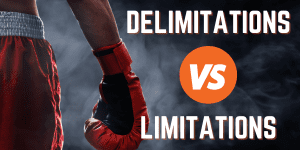
Submit a Comment Cancel reply
Your email address will not be published. Required fields are marked *
Save my name, email, and website in this browser for the next time I comment.
- Print Friendly
- What is a cross-sectional study?
Last updated
6 February 2023
Reviewed by
Miroslav Damyanov
Read on to learn about cross-sectional studies. We’ll explore examples, types, advantages, and limitations of cross-sectional studies, plus when you might use them.
Analyze cross-sectional studies
Dovetail streamlines cross-sectional studies to help you uncover and share actionable insights
A cross-sectional study is also known as a prevalence or transverse study. It’s a tool that allows researchers to collect data across a pre-defined subset or sample population at a single point in time. The information is typically about many individuals with multiple variables, such as gender and age. Although researchers get to analyze these variables, they do not manipulate them.
This study type is commonly used in clinical research, business-related studies, and population studies.
Once the researcher has selected the ideal study period and participant group, the study usually takes place as a survey or physical experiment.
- Characteristics of cross-sectional studies
Primary characteristics of cross-sectional studies include the following:
Consistent variables : Researchers carry out a cross-sectional study over a specific period with the same set of variables (income, gender, age, etc.).
Observational nature : Researchers record findings about a specific population but do not alter variables—they just observe.
Well-defined extremes : The analysis includes defined start and stop points which allow all variables to stay the same.
Singular instances : Only one topic or instance can be analyzed with a cross-sectional study. This allows for more accurate data collection .
- Examples of cross-sectional studies
Variables remain the same during a cross-sectional study. This makes it a useful research tool in various sectors and circumstances across multiple industries.
Here are some examples to give you better clarity:
Healthcare : Scientists might leverage cross-sectional research to assess how children aged 3–10 are prone to calcium deficiency.
Retail : Researchers use cross-sectional studies to identify similarities and differences in spending habits between men and women within a specific age group.
Education : These studies help reveal how students with a specific grade range perform when schools introduce a new curriculum.
Business: Researchers might leverage cross-sectional studies to understand how a geographic segment responds to offers and discounts.
- Types of cross-sectional studies
We can categorize cross-sectional studies into two distinct types: descriptive and analytical research. However, the researcher may use one or both types to gather and analyze data.
Here is a description of the two to help you understand how they may apply to your work.
Descriptive research
A descriptive cross-sectional survey or study assesses how commonly or frequently the primary variable occurs within a select demographic. This enables you to identify any problem areas within the group.
Descriptive research makes trend identification easy, facilitating the development of products and services that fit a particular population.
Analytical research
An analytical cross-sectional study investigates the relationship between two related or unrelated parameters. Outside variables may affect the study while the investigation is ongoing, however.
Note that the original results and data are studied together simultaneously in an analytical cross-sectional study.
- Cross-sectional versus longitudinal studies
Although longitudinal and cross-sectional studies are both observational, they are relatively different types of research design.
Below are the main differences between cross-sectional and longitudinal studies :
Sample group
A cross-sectional study will include several variables and sample groups, meaning it will collect data for all the different sample groups at once. However, in longitudinal studies, the same groups with similar variables can be observed repeatedly.
Cross-sectional studies are usually cheaper to conduct than longitudinal studies, so they are ideal if you have a limited budget.
Participants in longitudinal studies have to commit for an extended period, which significantly increases costs. Cross-sectional studies, on the other hand, are shorter and require less effort.
Data is collected only once in cross-sectional research. In contrast, longitudinal research takes considerable time because data is collected across numerous periods (potentially decades).
Researchers don’t necessarily seek causation in longitudinal research. This means the data will lack context regarding previous participant behavior.
Longitudinal research, on the other hand, clearly shows how data evolves. This means you can infer cause-and-effect relationships.
- How to perform a cross-sectional study
You will need to follow these steps to conduct a cross-sectional study:
Formulate research questions and hypotheses . You will also need to identify your target population at this stage.
Design the research . You will need to leverage observation rather than experiments when collecting data. However, you can always use non-experimental techniques such as questionnaires or surveys. As a result, this type of research will let you collect both quantitative and qualitative data .
Conduct the research . You can collect your data or assemble it from another source. In most instances, governments make cross-sectional datasets available to the public (through censuses) that can help with your research. The World Bank and World Health Organization also provide cross-sectional datasets on their websites.
Analyze the data . Data analysis will depend on the type of data collection method you use.
- Advantages and disadvantages of cross-sectional studies
Are you considering whether a cross-sectional study is an ideal approach for your next research? It’s an efficient and effective way to gather data. Check out some of the key advantages and disadvantages of cross-sectional studies.
Advantages of cross-sectional research
Quick to conduct
Multiple outcomes are researched at once
Relatively inexpensive
Used as a basis for further research
Researchers gather all variables at a single point in time
It’s possible to measure the prevalence of all factors
Ideal for descriptive analysis
Disadvantages of cross-sectional research
Preventing other variables from influencing the study is challenging
Researchers cannot infer cause-and-effect relationships
Requires large, heterogeneous samples, which increases the chances of sampling bias
The select population and period may not be representative
- When to use a cross-sectional design
Cross-sectional studies are useful when:
You need answers to questions regarding the prevalence and incidence of a situation, belief, or condition.
Establishing the norm in a particular demographic at a specified time. For instance, what is the average age for completing studies in Dallas?
Justifying the need to conduct further research on a specific topic. With cross-sectional research, you can infer a correlation without determining a direct cause. This makes it easier to justify conducting other investigations.
- The bottom line
A cross-sectional study is essential when researching the prevailing characteristics in a given population at a single point in time. Cross-sectional studies are often used to analyze demography, financial reports, and election polls. You could also use them in medical research or when building a marketing strategy, for instance.
Are cross-sectional studies quantitative or qualitative?
Cross-sectional research can be both qualitative and quantitative.
Do cross-sectional studies have control groups?
Cross-sectional studies don’t need a control group as the selected population is not based on exposure.
What are the limitations of cross-sectional studies?
Limitations of cross-sectional studies include the inability to make causal inferences, study rare illnesses, and access incidence. Researchers select a subject sample from a large and heterogeneous population.
Get started today
Go from raw data to valuable insights with a flexible research platform
Editor’s picks
Last updated: 21 December 2023
Last updated: 16 December 2023
Last updated: 6 October 2023
Last updated: 5 March 2024
Last updated: 25 November 2023
Last updated: 15 February 2024
Last updated: 11 March 2024
Last updated: 12 December 2023
Last updated: 6 March 2024
Last updated: 10 April 2023
Last updated: 20 December 2023
Latest articles
Related topics, log in or sign up.
Get started for free
Cross-Sectional Studies: Strengths, Weaknesses, and Recommendations
Affiliations.
- 1 Department of Quantitative Health Sciences, Lerner Research Institute, Cleveland Clinic, Cleveland, OH. Electronic address: [email protected].
- 2 Department of Respiratory Medicine, Zhongnan Hospital of Wuhan University, Wuhan, China.
- PMID: 32658654
- DOI: 10.1016/j.chest.2020.03.012
Cross-sectional studies are observational studies that analyze data from a population at a single point in time. They are often used to measure the prevalence of health outcomes, understand determinants of health, and describe features of a population. Unlike other types of observational studies, cross-sectional studies do not follow individuals up over time. They are usually inexpensive and easy to conduct. They are useful for establishing preliminary evidence in planning a future advanced study. This article reviews the essential characteristics, describes strengths and weaknesses, discusses methodological issues, and gives our recommendations on design and statistical analysis for cross-sectional studies in pulmonary and critical care medicine. A list of considerations for reviewers is also provided.
Keywords: bias; confounding; cross-sectional studies; prevalence; sampling.
Copyright © 2020 American College of Chest Physicians. Published by Elsevier Inc. All rights reserved.
Publication types
- Cross-Sectional Studies / standards
- Cross-Sectional Studies / statistics & numerical data*
- Guidelines as Topic

Cross-Sectional Studies
A cross-sectional study is useful for highlighting the extent of a problem in a community, identifying the need for services, or describing the burden of a condition within a population (Das-Munshi et al., 2020). For example, cross-sectional studies can be used to measure the prevalence of depression within a group of university students. They can also be used to compare experiences of an event or topic of interest among different populations. For instance, you can compare experiences with community engagement between groups of students with and without depression.
To put it in a nutshell, Keyes and Galea (2014) state: “any study that simply hits the pause button and samples a population at a single moment in time, counting cases of disease and potential causes at that point in time, is termed a cross-sectional study”. Such studies vary in their generalisability. According to Das-Munshi et al. (2020), findings from cross-sectional surveys “can be generalized to the base population for that survey and, to some extent, to other populations with similar characteristics” (p. 127).
Usually, cross-sectional studies are conducted using a questionnaire or survey , like the one below.

( University of Guelph, 2016 )
To illustrate the use of cross-sectional surveys further, let’s use a fun example: say you want to understand the relationship between eating pizza regularly and mental health. To do so, you could use a questionnaire to ask people how often they eat pizza and if they have good mental health. This means you will use the same survey to measure the exposure (pizza) and the outcome (having good mental health) at the same point in time. Using data from this cross-sectional survey you will provide the researcher with an understanding of:
- How many people eat pizza regularly, usually measured as a proportion or percentage
- How many people have good mental health, usually measured as a proportion of percentage
- The ‘association’, or relationship, between eating pizza and having good mental health
Cross-sectional studies have a limitation, however, which you may have already noticed above: because all data is collected at the same time, it is not possible to determine the ‘directionality’ of the relationship. Using the above study, for example, you will not be able to determine whether eating pizza regularly makes people more likely to have good mental health or if the relationship is the other way around. It may be that having good mental health means people have more energy to go pick up a pizza, and so they eat it more regularly!
Other advantages and disadvantages of cross-sectional studies include:
( Thomas, 2022 )
(Authors: Madison Wempe and Sam Davis)
What is it?
10.3 Cross sectional studies by Andrew Wills (2015)
This ten-minute video introduces cross-sectional studies, the information which they can be used to get, and their strengths and weaknesses. It uses several examples to aid understanding.
(Academic reference: Wills, A. (2015, September 16). 10.3 Cross sectional studies [Video]. YouTube. https://www.youtube.com/watch?v=ulRIqmJ9FiY&t=6s)
Methodology series module 3: cross-sectional studies by Maninder Singh Setia (2016)
This article describes the uses, strengths, and weaknesses of cross-sectional studies.
(Academic reference: Setia, M. S. (2016). Methodology series module 3: Cross-sectional studies. Indian Journal of Dermatology, 61 (3), 261—264. https://doi.org/10.4103/0019-5154.182410)
Cross-sectional studies: strengths, weaknesses, and recommendations by Xiaofeng Wang and Zhenshun Cheng (2020)
This resource outlines the strengths and weaknesses of cross-sectional studies. It also gives recommendations for their design and analysis.
(Academic reference: Wang, X. & Cheng, Z. (2020). Cross-sectional studies: Strengths, weaknesses, and recommendations. Chest , 158 (1), S65—S71. https://doi.org/10.1016/j.chest.2020.03.012)
Cross-sectional surveys by Martin Prince and Jayati Das-Munshi (2020)
This resource is the ninth chapter of an epidemiology methods book for psychiatric epidemiology. It begins by describing cross-sectional studies and their use and then applies specific examples to these concepts.
(Academic reference: Prince, M. & Das-Munshi, J. (2020). Cross-sectional surveys. In Das-Munshi, J., Ford, T., Hotopf, M., Prince, M., & Stewart, R. (Eds.), Practical psychiatric epidemiology (2nd ed., pp. 127-143). Oxford University Press)
Epidemiology matters: A New introduction to methodological foundations by Katherine Keyes and Sandro Galea (2014)
This textbook introduces the methodological foundations of epidemiology and population health research.
(Academic reference: Keyes, K. & Galea, S. (2014). Epidemiology matters: A new introduction to methodological foundations. Oxford University Press)
Online Course:
Epidemiology: the basic science of public health by the University of North Carolina Chapel Hill (2023)
This is a free online course which users can complete in their own time. Study designs, including cross-sectional studies, are discussed in week 3.
(Academic reference: The University of North Carolina Chapel Hill (2023).
Epidemiology: The basic science of public health . Retrieved March 10, 2023, from https://gb.coursera.org/learn/epidemiology)
How is it done?
Week 5: Cross-sectional studies by Data Learner (2013)
This is a 15-minute videoed lecture at the Harvard School of Public Health on cross-sectional studies. It provides an understanding of cross-sectional studies and their relation to measures like incidence and prevalence.
(Academic reference: Data Learner. (2013, August 24). Week 5: Cross-sectional studies [Video]. YouTube. https://www.youtube.com/watch?v=uuhZSfYcygY)
Cross-sectional study | definitions, uses & examples by Lauren Thomas (2022)
This website provides a definition of cross-sectional studies, outlines their strengths and weaknesses, and details how to do a cross-sectional study.
(Academic reference: Thomas, L. (2022, May 5). Cross-sectional study | definitions, uses & examples. Scribbr. https://www.scribbr.co.uk/research-methods/cross-sectional-design/)
Method in action
A cross-sectional study on mental health among health care workers during the outbreak of corona virus disease 2019 by Cai et al. (2020)
This article presents results from a cross-sectional study used to assess mental health during COVID-19. The methods section will be particularly useful for those looking to understand how cross-sectional studies are conducted.
(Academic reference: Cai, W., Lian, B., Song, X., Hou, T., Deng, G., & Li, H. (2020). A cross-sectional study on mental health among health care workers during the outbreak of corona virus disease 2019. Asian Journal of Psychiatry. https://doi.org/10.1016/j.ajp.2020.102111)
Social media use and mental health in young adults of Greece: a cross-sectional study by Epameinondas Leimonis and Katerina Koutra (2022)
This article presents results from a cross-sectional study exploring the association between social media use, depressive symptoms, and self-esteem among Greek young adults. The results suggest that social media use is closely related to self-esteem and depressive symptomatology in young adults.
(Academic reference: Leimonis, E. & Koutra, K. (2022). Social media use and mental health in young adults of Greece: a cross-sectional study. Clinical Psychology in Europe 4 (2) . https://doi.org/10.32872/cpe.4621)
Share This Post:
Related posts.

Quantitative Sampling

Records and Record Keeping

Before-and-After Study

Realist Evaluation
We’d love to hear from you.
Collaboration is at the heart of our Research Methods Toolkit which means we value your voice, and want to hear what you have to say. Questions, suggestions and comments help us understand what works and what needs improvement. Feel free to suggest new content and resources that we might be able to develop for the Toolkit.

Research Methods for Society and Mental Health
Privacy Policy
© Copyright 2024 | Website by Backhouse Creative
Quantitative study designs: Cross-Sectional Studies
Quantitative study designs.
- Introduction
- Cohort Studies
- Randomised Controlled Trial
- Case Control
- Cross-Sectional Studies
- Study Designs Home
Cross-Sectional Study
The Australian Census run by the Australian Bureau of Statistics, is an example of a whole of population cross-sectional study.
Data on a number of aspects of the Australian population is gathered through completion of a survey within every Australian household on the same night. This provides a snapshot of the Australian population at that instance.
Cross-sectional studies look at a population at a single point in time, like taking a slice or cross-section of a group, and variables are recorded for each participant.
This may be a single snapshot for one point in time or may look at a situation at one point in time and then follow it up with another or multiple snapshots at later points; this is then termed a repeated cross-sectional data analysis.
The stages of a Cross-Sectional study
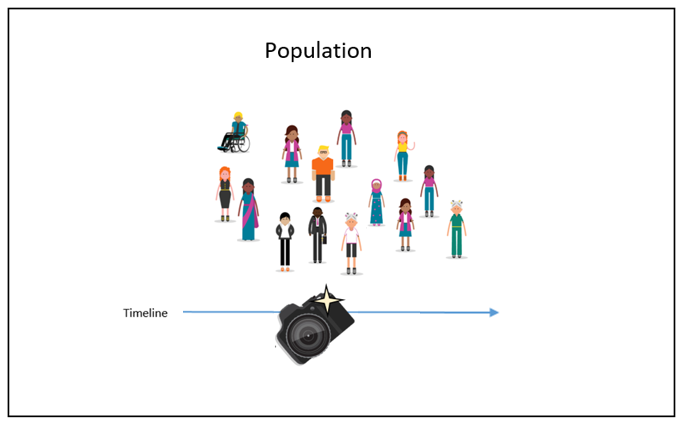
Repeated Cross-Sectional Data Analysis
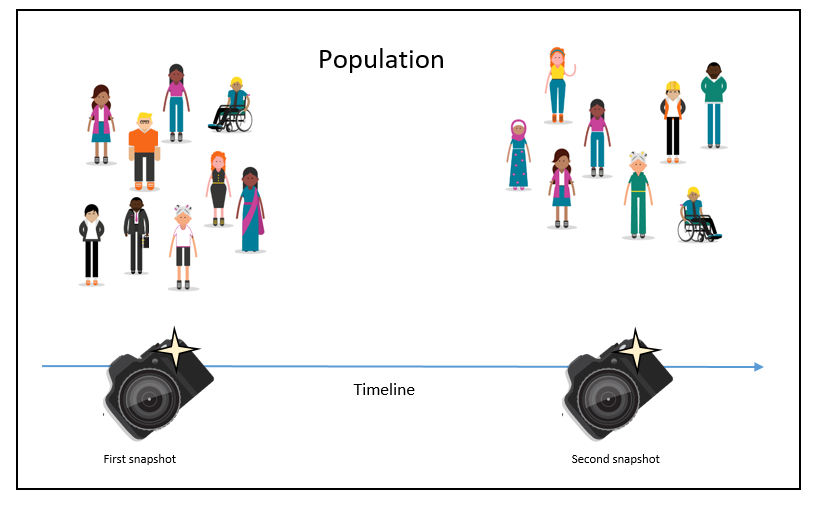
Which clinical questions does a Cross-Sectional study best answer?
Please note the Introduction , where there is a table under "Which study type will answer my clinical question?" . You may find that there are only one or two question types that your study answers – that’s ok.
Cross-sectional study designs are useful when:
- Answering questions about the incidence or prevalence of a condition, belief or situation.
- Establishing what the norm is for a specific demographic at a specific time. For example: what is the most common or normal age for students completing secondary education in Victoria?
- Justifying further research on a topic. Cross-sectional studies can infer a relationship or correlation but are not always sufficient to determine a direct cause. As a result, these studies often pave the way for other investigations.
What are the advantages and disadvantages to consider when using a Cross-Sectional study design?
What does a strong cross-sectional study look like.
- Appropriate recruitment of participants. The sample of participants must be an accurate representation of the population being measured.
- Sample size. As is the case for most study types a larger sample size gives greater power and is more ideal for a strong study design. Within a cross-sectional study a sample size of at least 60 participants is recommended, although this will depend on suitability to the research question and the variables being measured.
- A suitable number of variables. Cross-sectional studies ideally measure at least three variables in order to develop a well-rounded understanding of the potential relationships of the two key conditions being measured.
What are the pitfalls to look for?
Cross-sectional studies are at risk of participation bias, or low response rates from participants. If a large number of surveys are sent out and only a quarter are completed and returned then this becomes an issue as those who responded may not be a true representation of the overall population.
Critical appraisal tools
To assist with critically appraising cross-sectional studies there are some tools / checklists you can use.
- Axis Appraisal Tool for Cross Sectional Studies
- Critical Appraisal Tool for Cross- Sectional Studies (CAT-CSS)
- Critical Appraisal of a Cross-Sectional Study on Environmental Health
- Critical appraisal tool for cross-sectional studies using biomarker data (BIOCROSS)
- CEBM Critical Appraisal of a Cross-Sectional Study (Survey)
- JBI Critical Appraisal checklist for analytical cross-sectional studies
- Specialist Unit for Review Evidence (SURE) 2018. Questions to assist with the critical appraisal of cross sectional studies
- STROBE Checklist for cross-sectional studies
Real World Examples
The Australian National Survey of Mental Health and Wellbeing (NSMHWB)
https://www.abs.gov.au/statistics/health/mental-health/national-survey-mental-health-and-wellbeing-summary-results/2007
A widely known example of cross-sectional study design, the Australian National Survey of Mental Health and Wellbeing (NSMHWB). This study was a national epidemiological survey of mental disorders investigating the questions: How many people meet DSM-IV and ICD-10 diagnostic criteria for the major mental disorders? How disabled are they by their mental disorders? And, how many have seen a health professional for their mental disorder?
References and Further Reading
Australian Government Department of Health. (2003). The Australian National Survey of Mental Health and Wellbeing (NSMHWB). 2019, from https://www.abs.gov.au/statistics/health/mental-health/national-survey-mental-health-and-wellbeing-summary-results/2007
Bowers, D. a., Bewick, B., House, A., & Owens, D. (2013). Understanding clinical papers (Third edition. ed.): Wiley Blackwell.
Gravetter, F. J. a., & Forzano, L.-A. B. (2012). Research methods for the behavioral sciences (Fourth edition. ed.): Wadsworth Cengage Learning.
Greenhalgh, T. a. (2014). How to read a paper : the basics of evidence-based medicine (Fifth edition. ed.): John Wiley & Sons Inc.
Hoffmann, T. a., Bennett, S. P., & Mar, C. D. (2017). Evidence-Based Practice Across the Health Professions (Third edition. ed.): Elsevier.
Howitt, D., & Cramer, D. (2008). Introduction to research methods in psychology (Second edition. ed.): Prentice Hall.
Kelly, P. J., Kyngdon, F., Ingram, I., Deane, F. P., Baker, A. L., & Osborne, B. A. (2018). The Client Satisfaction Questionnaire‐8: Psychometric properties in a cross‐sectional survey of people attending residential substance abuse treatment. Drug and Alcohol Review, 37(1), 79-86. doi: 10.1111/dar.12522
Lawrence, D., Hancock, K. J., & Kisely, S. (2013). The gap in life expectancy from preventable physical illness in psychiatric patients in Western Australia: retrospective analysis of population based registers. BMJ: British Medical Journal, 346(7909), 13-13.
Nasir, B. F., Toombs, M. R., Kondalsamy-Chennakesavan, S., Kisely, S., Gill, N. S., Black, E., Ranmuthugala, G., Ostini, R., Nicholson, G. C., Hayman, N., & Beccaria, G.. (2018). Common mental disorders among Indigenous people living in regional, remote and metropolitan Australia: A cross-sectional study. BMJ Open , 8 (6). https://doi.org/10.1136/bmjopen-2017-020196
Robson, C., & McCartan, K. (2016). Real world research (Fourth Edition. ed.): Wiley.
Sedgwick, P. (2014). Cross sectional studies: advantages and disadvantages. BMJ : British Medical Journal, 348, g2276. doi: 10.1136/bmj.g2276
Setia, M. S. (2016). Methodology Series Module 3: Cross-sectional Studies. Indian journal of dermatology, 61(3), 261-264. doi: 10.4103/0019-5154.182410
Shafiei, T., Biggs, L. J., Small, R., McLachlan, H. L., & Forster, D. A. (2018). Characteristics of women calling the panda perinatal anxiety & depression australia national helpline: A cross-sectional study. Archives of Women's Mental Health. doi: 10.1007/s00737-018-0868-4
Van Heyningen, T., Honikman, S., Myer, L., Onah, M. N., Field, S., & Tomlinson, M. (2017). Prevalence and predictors of anxiety disorders amongst low-income pregnant women in urban South Africa: a cross-sectional study. Archives of Women's Mental Health(6), 765. doi: 10.1007/s00737-017-0768-z
Vogt, W. P. (2005). Dictionary of statistics & methodology : a nontechnical guide for the social sciences (Third edition. ed.): Sage Publications.
- << Previous: Case Control
- Next: Case Studies/ Case Report/ Case Series >>
- Last Updated: Feb 29, 2024 4:49 PM
- URL: https://deakin.libguides.com/quantitative-study-designs

An official website of the United States government
The .gov means it’s official. Federal government websites often end in .gov or .mil. Before sharing sensitive information, make sure you’re on a federal government site.
The site is secure. The https:// ensures that you are connecting to the official website and that any information you provide is encrypted and transmitted securely.
- Publications
- Account settings
Preview improvements coming to the PMC website in October 2024. Learn More or Try it out now .
- Advanced Search
- Journal List
- Emerg Med J
- v.20(1); 2003 Jan

Observational research methods. Research design II: cohort, cross sectional, and case-control studies
Cohort, cross sectional, and case-control studies are collectively referred to as observational studies. Often these studies are the only practicable method of studying various problems, for example, studies of aetiology, instances where a randomised controlled trial might be unethical, or if the condition to be studied is rare. Cohort studies are used to study incidence, causes, and prognosis. Because they measure events in chronological order they can be used to distinguish between cause and effect. Cross sectional studies are used to determine prevalence. They are relatively quick and easy but do not permit distinction between cause and effect. Case controlled studies compare groups retrospectively. They seek to identify possible predictors of outcome and are useful for studying rare diseases or outcomes. They are often used to generate hypotheses that can then be studied via prospective cohort or other studies.
The Full Text of this article is available as a PDF (149K).
Selected References
These references are in PubMed. This may not be the complete list of references from this article.
- Fowkes FG, Fulton PM. Critical appraisal of published research: introductory guidelines. BMJ. 1991 May 11; 302 (6785):1136–1140. [ PMC free article ] [ PubMed ] [ Google Scholar ]
- Lerner DJ, Kannel WB. Patterns of coronary heart disease morbidity and mortality in the sexes: a 26-year follow-up of the Framingham population. Am Heart J. 1986 Feb; 111 (2):383–390. [ PubMed ] [ Google Scholar ]
- Alberman ED, Butler NR, Sheridan MD. Visual acuity of a national sample (1958 Cohort) at 7 years. Dev Med Child Neurol. 1971 Feb; 13 (1):9–14. [ PubMed ] [ Google Scholar ]
- Smith GD, Hart C, Blane D, Hole D. Adverse socioeconomic conditions in childhood and cause specific adult mortality: prospective observational study. BMJ. 1998 May 30; 316 (7145):1631–1635. [ PMC free article ] [ PubMed ] [ Google Scholar ]
- Goyder EC, Goodacre SW, Botha JL, Bodiwala GG. How do individuals with diabetes use the accident and emergency department? J Accid Emerg Med. 1997 Nov; 14 (6):371–374. [ PMC free article ] [ PubMed ] [ Google Scholar ]
- Jaffe HW, Bregman DJ, Selik RM. Acquired immune deficiency syndrome in the United States: the first 1,000 cases. J Infect Dis. 1983 Aug; 148 (2):339–345. [ PubMed ] [ Google Scholar ]
- Reidy A, Minassian DC, Vafidis G, Joseph J, Farrow S, Wu J, Desai P, Connolly A. Prevalence of serious eye disease and visual impairment in a north London population: population based, cross sectional study. BMJ. 1998 May 30; 316 (7145):1643–1646. [ PMC free article ] [ PubMed ] [ Google Scholar ]
- Karjalainen J, Kujala UM, Kaprio J, Sarna S, Viitasalo M. Lone atrial fibrillation in vigorously exercising middle aged men: case-control study. BMJ. 1998 Jun 13; 316 (7147):1784–1785. [ PMC free article ] [ PubMed ] [ Google Scholar ]
- Kunst AE, Groenhof F, Mackenbach JP, Health EW. Occupational class and cause specific mortality in middle aged men in 11 European countries: comparison of population based studies. EU Working Group on Socioeconomic Inequalities in Health. BMJ. 1998 May 30; 316 (7145):1636–1642. [ PMC free article ] [ PubMed ] [ Google Scholar ]

- Basics of Research Process
- Methodology
Cross Sectional Study: Definition and Examples
- Speech Topics
- Basics of Essay Writing
- Essay Topics
- Other Essays
- Main Academic Essays
- Research Paper Topics
- Basics of Research Paper Writing
- Miscellaneous
- Chicago/ Turabian
- Data & Statistics
- Admission Writing Tips
- Admission Advice
- Other Guides
- Student Life
- Studying Tips
- Understanding Plagiarism
- Academic Writing Tips
- Basics of Dissertation & Thesis Writing

- Essay Guides
- Research Paper Guides
- Formatting Guides
- Admission Guides
- Dissertation & Thesis Guides

Table of contents

Use our free Readability checker
A cross-sectional study is a research design used to gather data from a population or sample at a specific point in time. It aims to provide a snapshot of a particular phenomenon or explore the relationship between variables at a given moment. Unlike longitudinal studies that track individuals over time, cross-sectional studies focus on a single interval.
Whether you are investigating an unstudied topic or just can’t afford to spend too much time on research, cross sectional designs can work wonders. All you need is a single time and many different participants. Sounds easy, right? And it should be if you follow this guide from our writing service . Get ready for lots of insights and useful examples as you read our blog post. But first things first – let’s begin with the basics.
What Is a Cross Sectional Study: Definition
A cross-sectional study is a type of observational research that allows assembling data from many different subjects at one point. Scientists usually rely on specific variables to pick the participants. As descriptive research, a cross-sectional study is used to observe something that already exists in a cohort. Thus, you won’t need to adjust or change variables. Here are the main attributes that set cross-sectional studies apart from other types of research:
- The population members are observed only once.
- Various traits can be examined simultaneously.
- Researchers don’t control the variables.
- Method allows investigating predominant qualities within a group.
As a rule, cross sectional studies are carried out in developmental psychology. However, research paper writers also widely use this type of study in economics, education, medicine and social sciences.
Cross-Sectional Study: When to Use
A cross-sectional study is used to explore the characteristics that are dominant in a specific group of people at a particular time. Researchers opt for this method when having to choose between time or expenses. It’s a time-wise option, especially if the data you have was gathered only once. Cross-sectional studies don’t require repeated experiments, and, thus, are budget-friendly.
Descriptive Cross Sectional Study vs Analytical Cross Sectional Study
Depending on their main purpose, cross sectional studies can be either descriptive or analytical. A descriptive cross sectional study is aimed at the prevalence of some characteristics in a population. It only describes the outcome. An analytical cross sectional study requires that you look for a relationship between the cause and outcome.
Cross-Sectional Study: How to Implement
There are two ways to conduct a cross sectional study design:
- Use the data collected by another researcher/ organization.
- Run your own research.
In the first case, you can use national or local government’s registers, surveys or reports by international organizations. Such data is easy to retrieve from official websites. On the flip side, your research question may differ, so do variables. If you decide to do your own cross-sectional research, make sure you follow these steps:
- Select participants using inclusion and exclusion criteria. Include only those subjects that have necessary attributes that will help to answer your research question. Consider such factors as age, gender , social status to include individuals in your research.
- Examine the influence and results at the same time. Try to find an association between variables of interest. Sometimes, researchers may observe only the outcomes in subjects.
- Measure the prevalence of traits within your population. Collect and analyze data about traits that prevail in your chosen group. You can also estimate odd ratios to explore the relation between variables.
Conducting a cross-sectional research study undeniably requires much effort. Sometimes, it’s better to buy research paper online or ask professionals to ‘ write my research paper .’
Cross Sectional Study vs Longitudinal Study
Now let’s look at the difference between a cross sectional study and a longitudinal study . Cross-sectional studies are executed to gather information from a population only once. Meanwhile, longitudinal studies are used to examine a small group of participants a number of times.
Longitudinal research is more time-consuming and requires more resources. For this reason, you should be 100% sure that there is some kind of correlation between variables. And that’s exactly what cross-sectional research can help you with. As a cheap and easy option, it allows you to collect initial information about the subjects. This should be enough to decide whether it’s worth continuing further research.
Advantages and Disadvantages of Cross Sectional Study
Cross sectional research is the best choice when it comes to gathering some basic information about some population. Advantages of cross sectional studies include:
- Cheap data collection methods
- Timesaving research option
- Measurements of several variables at a time
- Guidance to further experimental studies.
Limited time is one of the disadvantages of cross sectional studies. As an experiment that takes place only once, it also has some other limitations:
- Difficulty to identify causal relationship
- No opportunity for long-lasting observation
- Cohort effect among participants who share experience.
Cross Sectional Study Example
Now that you know all ins and outs, let’s review an example of cross sectional study. It should give you an idea of what this type of research should focus on.
Cross Sectional Research: A Word From StudyCrumb
Like any other research method, a cross-sectional study takes practice. Not that much as you would need to complete a longitudinal research, though… And yet, you should remember that this method won’t work if you want to identify a cause-and-effect relationship. Opt for this type of research if you want to run an initial experiment or just lack time.

Delegate your assignment to StudyCrumb – a term paper writing service assisting students with challenging academic tasks. Our writers have hands-on experience in research writing and will be happy to help you at any time.

Joe Eckel is an expert on Dissertations writing. He makes sure that each student gets precious insights on composing A-grade academic writing.
You may also like

Example of cross sectional study use case You want to find out how many people currently work remotely in your district. You only should learn the current number of individuals who work from home. For this reason, a cross-sectional study is preferred.
Example You are examining the occurrence of cardiovascular disease. In a descriptive research design, you will look for the prevalence of cardiovascular disease in older individuals. Meanwhile, in analytical research you will focus on recent radiation exposure as the main reason for heart diseases.
Cross-sectional research example Researchers examine the influence of vitamin C consumption on blood vessels. They first conduct cross-sectional research to identify if there is any change in blood vessels in those individuals who take in vitamin C. If there is some impact, researchers will want to explore this further.
FAQ About Cross Sectional Research Design
1. what is cross sectional correlational study.
As a correlational study, cross-sectional research is used to examine the association between two or more variables. However, as with any other correlational research, you won’t have any chance to manipulate the cause (an independent variable).
2. Is cross-sectional study qualitative?
In most instances, a cross-sectional study involves working with numbers and specific measurements, and, thus, is quantitative. However, sometimes researchers can also use this method to collect qualitative data or analyze both types of data.
3. How is cross sectional data collected?
Cross sectional data is usually gathered with the help of surveys, polls or self-administered questionnaires. These tools allow researchers to quickly collect information from a large population. However, surveys aren't always accurate and can lead to invalid results.
4. What evidence level is a cross-sectional study?
Based on the validity and overall quality, the evidence level of a cross-sectional study is rather low. The design takes the VI place in the hierarchy since it offers evidence from a single study.
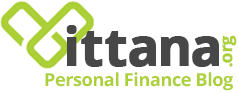
19 Advantages and Disadvantages of Cross Sectional Studies
A cross-sectional study involves the review of information from a population demographic at a specific point in time. The participants who get involved with this research are selected based on particular variables that researchers want to study. It is often used in developmental psychology, but this method is also useful in several other areas. Social sciences and educational processes benefit from this work.
Researchers who are following cross-sectional study techniques would study select groups of people in different age demographics. Their work would look at one investigatory point at a time. By taking this approach, any differences that exist between the demographics would be attributed to characteristics instead of something that happens.
These studies are observational in nature. They are sometimes described as descriptive research, but not causal or relational. That means researchers are unable to determine the cause of something, such as an illness, when using this method.
Several advantages and disadvantages are worth considering when looking at cross-sectional studies.
List of the Advantages of Cross-Sectional Studies
1. This study takes place during a specific moment in time. A cross-sectional study has defined characteristics that limit the size and scope of the work. Researchers look at specific relationships that happened during a particular moment in time. That means there are fewer risks to manage if tangents begin to develop in the data. The goal is to look for a meaningful result within an expected boundary.
2. No variable manipulation occurs with a cross-sectional study. Researchers directly observe the variables under study when using the cross-sectional technique. There is no reason to manipulate the environment because this is not an experimental technique. The data gathering process goes quickly because everything occurs within the scope of the research method. This advantage reduces the risk of having bias creep into the information being gathered.
3. It is an affordable way to conduct research. A cross-sectional study is much more affordable to complete when compared to the other options that are available to researchers today. No follow up work is necessary when taking this approach because once the information gets collected from the entire participant group, it can be analyzed immediately. This advantage as possible because only a single time reference is under consideration.
This approach allows for usable data to become available without the risk of a significant initial investment. Most of the data points collected using this method come from self-report surveys. Researchers can then collect a significant amount of information from a large pool of participants without a major time investment.
4. This study method provides excellent controls over the measurement process. Cross-sectional studies are only as good as the measurement processes that researchers used to collect data. Because there aren’t any long-term considerations involved with this specific approach, researchers have more control over the information acquisition process. Everything obtained during this work is quickly and easily measured and applied to the targeted demographics because the controls involved are straightforward to implement.
5. Researchers can look at several useful characteristics at once. Many researchers prefer the cross-sectional studies method because it allows them to look at numerous characteristics simultaneously. Instead of focusing on income, gender, age, or other separating factors, this method looks at each participant as an entire individual. That makes it possible for the work to include several useful characteristics that can each benefit from changing variables instead of using only one to determine an outcome.
This advantage is the reason why researchers often use cross-sectional studies to look at the prevailing characteristics in a given population. It is a process that lets different variables become the foundation of new correlations.
6. It provides relevant information in real-time updates. Cross-sectional studies provide us with a snapshot of a specific group of people at a particular point in time. Unlike other methods of research that look at demographics over an extended period, we use this information to look at what is happening in the present. That means the data researchers collect from this process is immediately relevant, giving us an opportunity to create real-time updates within specific population groups.
This process is how we can determine if there are specific risk factors that correlate to particular outcomes wit in that group. A cross-sectional study might look at a person’s past smoking and chewing habits to determine if there is a correlation with a recent lung cancer diagnosis. Although it won’t provide a cause-and-effect explanation, it does offer a fast look at potential correlations.
7. Cross-sectional studies miss fewer data points. The processes involved with cross-sectional studies reduce the risk of missing critical data points. Researchers have the ability to maximize their examination of the available information at any time because there are no time variables included in this work. That means a lower error rate typically occurs when using this method compared to the other approaches that are available to the scientific community.
8. It allows anyone to look at the data to determine a possible conclusion. The information that cross-sectional studies obtain is always suitable for secondary analysis. This advantage means that researchers can collect information for one set of purposes, and then use it to explore different variables that might exist in that specific demographic at the same time. That means an investment in this work can provide ongoing usefulness because it always applies to the people involved during that specific time. It is one of the easiest ways to maximize your research investment value.
9. Cross-sectional studies offer information that’s well-suited for descriptive analysis. If researchers want to develop a general hypothesis, then cross-sectional studies are the best way to generate specific situations that face a particular demographic. Each description of the critical data points creates the possibility of forwarding movement toward a future solution that may not have been considered previously.
Although this benefit doesn’t apply to causal relationships with this research method, the information collected from cross-sectional studies is a useful forward push toward additional research.
10. The focus of a cross-sectional study is to prove or disprove an assumption. A cross-sectional study is a research tool that is useful across various industries. The reason why it is such a generalized process that anyone can initiate is that the purpose of the work is to prove or disapprove and assumption or theory. Although health-related work tends to be the most popular industry that takes advantage of this approach, retail, education, social science, religion, and government industries can also benefit from this process.
The research that occurs allows each industry to learn more about the various demographics for the purpose of analyzing a target market. It creates data that’s useful when trying to determine what products or services to sell, or when it is necessary to look for specific patient outcomes.
List of the Disadvantages of Cross-Sectional Studies
1. It requires the entire population to be studied to create useful data. A correctly structured cross-sectional study must be representative of an entire demographic for it to provide useful information. If this representation is not possible, then the data collected from the participating individuals will have a built-in error rate that must come under consideration.
That’s why a complete generalization is not possible when using this approach. Environmental conditions, a person’s education, and several other factors can all change an individual’s perspective.
2. A researcher’s personal bias can influence the data from cross-sectional studies. Everyone has particular biases that influence their personality and general perspective on life. Many of these circumstances come from the conditioning that happens over the course of time. Even people who work hard to avoid showing bias in any situation can come under the influence of this disadvantage of cross-sectional studies.
Some demographics might include prison populations, the homeless, or people who are unable to leave their homes. If a researcher feels uncomfortable contacting individuals in these groups, then the final data points will not have as much relevance as they could.
3. The questions asked during cross-sectional studies may lead to specific results. If researchers want to achieve a specific result when performing a cross-sectional study, then they can ask questions in such a way that it leads participants to the desired answer. When there are surveys or questionnaires about specific aspects of a person’s life, then the answers received may not always result in an accurate report. Shared experiences can result in different perspectives.
We have seen this disadvantage play out numerous times throughout several generations. The people who were alive during the Vietnam war, the attack on Pearl Harbor, or the terrorist event in New York City on 9/11 have shared experiences that make them different from other age groups. The people who survived these events are another subgroup that can impact the quality of information gathered.
4. Large sample sizes are often necessary to generate usable information. A significant sample size is often necessary for a cross-sectional study to provide useful information. This disadvantage occurs because the entire population demographic must go through the research at once to prevent errors in the data. When a smaller sample size is the focus of the work, then the risk of errors entering into the information increases dramatically. There are more opportunities for coincidence or a chance to influence the results with a smaller research sample.
Although cross-sectional studies are often very affordable, the inclusion of an entire demographic pushes the cost of this work higher than it would be for other approaches.
5. Cross-sectional studies don’t offer any control over purpose or choice. When the data from a cross-sectional study is found useful for secondary data analysis, the bias of the researchers can influence the information without any future realization. The secondary approach has no control over how this work gets completed initially. That’s why an overview of the methods used and the purpose for collecting information in the first place are often included as part of the results of this work.
If these additional facts are not part of the final experience, and the usefulness of the information for future needs becomes questionable.
6. No information about causal relationships is possible with this approach. This research method does not provide information about causal relationships. The goal of this approach is to offer correlated data that is useful when drawing conclusions about a specific demographic. It can only let researchers see that a causal relationship exists without letting them know the reason behind its existence.
That’s why individualization is a disadvantage to this type of study. Researchers are wanting to see a generalized overview of a specific population sample instead of understanding why some people make particular choices.
7. Demographic definitions must be available to create a. successful result. The information collected during a cross-sectional study is not reliable unless there are specific definitions in place for a population sample that is large enough for generalization. If researchers want to look at a rare outcome or a unique event, then inappropriate conclusions could get gleaned from the collected data. Trying to force a specific question or result could encourage responses that are unnecessary within the study population.
The only way to avoid this disadvantage of a cross-sectional study is to create definitions that work specifically with the intended results.
8. Cross-sectional studies have no way to measure incidence. The goal of a cross-sectional study is to review the data that researchers collect as they study-specific variables. It does not take a look at the reason why the specific information points occur within the population demographics. This disadvantage limits the availability of an outcome for researchers in many situations because there is no determination available as to why the variables are present initially.
It only measures the existence and relationships that are present in that environment, not what triggers the variables.
9. It can be challenging to duplicate the results. Even though a large population sample is necessary to create an accurate dataset from a cross-sectional study, it is challenging to duplicate results from multiple efforts. This disadvantage occurs because work happens in real-time situations. What happens right now can create a very different result then what could happen in the future.
That’s why many institutions face some challenges when they attempt to put together a sampling pool. The variables that should be studied are available in complex ways that may be difficult to manage. This issue is so detrimental that the timing of a specific snapshot is never a 100% guarantee that it’s representative of the entire population group.
A cross-sectional study is a useful research tool in most areas of health and wellness. When we can learn more about what is happening within a specific population demographic, then researchers can better understand the relationships that could exist between particular variables. The information that comes out of this process allows us to develop further studies that can explore the results in greater depth.
Several other research study options are available when there is a need to collect information from a specific demographic. It is essential to compare the critical points from each approach to determine what the best possible solution will be for each situation.
These cross-sectional advantages and disadvantages show us that a massive undertaking of simultaneous data collection can provide unique results that can benefit an entire population group. Although there are some challenges to manage when taking this approach, it is one that most researchers find to be beneficial.
Click through the PLOS taxonomy to find articles in your field.
For more information about PLOS Subject Areas, click here .
Loading metrics
Open Access
Peer-reviewed
Research Article
Empowering adult patients with diabetes for health educators’ role within their family members: A cross-sectional study
Roles Conceptualization, Data curation, Formal analysis, Investigation, Methodology, Supervision, Validation, Visualization, Writing – original draft, Writing – review & editing
* E-mail: [email protected]
Affiliations College of Nursing, King Saud bin Abdulaziz University for Health Sciences, Riyadh, Saudi Arabia, King Abdullah International Medical Research Center, Riyadh, Saudi Arabia, Ministry of the National Guard Health Affairs Riyadh, Riyadh, Saudi Arabia

Roles Conceptualization, Data curation, Methodology, Writing – original draft, Writing – review & editing
Roles Conceptualization, Data curation, Writing – original draft, Writing – review & editing
Affiliation Nursing College-Muhyil Asir, King Khalid University, Abha, Saudi Arabia
Roles Conceptualization, Investigation, Writing – original draft, Writing – review & editing
Affiliation Nursing College, Umm Al-Qura University, Mecca, Saudi Arabia
- Mona Alanazi,
- Eman Bajmal,
- Abeer Aseeri,
- Ghaida Alsulami
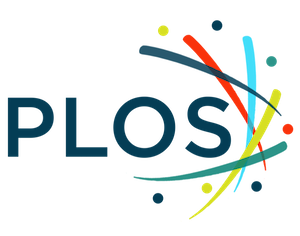
- Published: April 16, 2024
- https://doi.org/10.1371/journal.pone.0299790
- Peer Review
- Reader Comments
Patient engagement as partners in diabetes prevention for family members/close relatives is a novel and underexplored approach. This paper aims to assess patients’ willingness and confidence in their ability to succeed as health educators for their family members and investigate the influencing factors.
A cross-sectional descriptive study was conducted between January 2023 and April 2023. A newly developed and validated self-reported questionnaire, based on the Health Belief Model (HBM) and previous research, was administered to a convenient sample of 134 adult participants diagnosed with diabetes. These participants sought care at primary healthcare clinics at King Abdul-Aziz Medical City, Ministry of National Guard Health Affairs in Riyadh and Jeddah (MNGHA). The data was examined using statistical methods including descriptive analysis, ANOVA, Tukey’s HSD (Honestly Significant Difference) Post Hoc tests, and Pearson’s correlation coefficients.
The majority of participants expressed a willingness to assume the role of health educators for their family members (n = 117, 87.31%) and reported a high level of willingness and confidence, as indicated by self-efficacy scores ranging from 12.00 to 25.00, with a mean of 21.12 (SD = 2.76). Participants’ willingness to be health educators exhibited positive correlations with their perceptions of diabetes severity and susceptibility (r = .433, p < .01), perceived benefits and barriers (r = .451, p < .01), cues to action (r = .520, p < .01), self-efficacy (r = .789, p < .01), and the total score of the questionnaire (r = .640, p < .01).
Conclusions
The majority of participants expressed their willingness to assume the role of health educators for their family members, and a significant portion reported confidence in their capacity to accomplish this objective. Healthcare providers should emphasize the importance of equipping patients with the skills and knowledge necessary to effectively convey health messages and serve as health educators within their communities. This expansion of the approach holds the potential to have a significant impact on public health strategies for diabetes prevention.
Citation: Alanazi M, Bajmal E, Aseeri A, Alsulami G (2024) Empowering adult patients with diabetes for health educators’ role within their family members: A cross-sectional study. PLoS ONE 19(4): e0299790. https://doi.org/10.1371/journal.pone.0299790
Editor: Maher Abdelraheim Titi, King Saud University Medical City, SAUDI ARABIA
Received: October 17, 2023; Accepted: February 16, 2024; Published: April 16, 2024
Copyright: © 2024 Alanazi et al. This is an open access article distributed under the terms of the Creative Commons Attribution License , which permits unrestricted use, distribution, and reproduction in any medium, provided the original author and source are credited.
Data Availability: The datasets used and/or analyzed during the current study are available as attachment in the Supporting Information files.
Funding: The author(s) received no specific funding for this work.
Competing interests: The authors have declared that no competing interests exist.
Introduction
In the realm of healthcare, addressing the growing concerns surrounding diabetes has become increasingly important [ 1 ]. Among the innovative strategies emerging to tackle this global health issue, a particularly novel approach involves harnessing the power of those who are already familiar with the challenges of diabetes–patients themselves [ 2 , 3 ]. This innovative concept entails enlisting individuals living with diabetes to serve as health educators within their local communities [ 2 ]. By leveraging their experiences, these patients can offer unique insights, empathy, and practical advice to others who may be at risk of developing diabetes or struggling to manage the condition [ 4 ]. This approach not only enhances the understanding of diabetes within communities but also fosters a sense of empowerment, solidarity, and shared responsibility in the battle against this chronic illness [ 3 , 5 ].
However, the key question revolves around the feasibility of employing the "patients as health educators" approach to target high-risk individuals in Saudi Arabia. This approach becomes especially pertinent in light of the high prevalence of familial aggregation of diabetes within Saudi families, making it seemingly appropriate to leverage patients as health educators to educate their family members as a preventive strategy aimed at high-risk individuals [ 3 , 5 , 6 ]. In the context of Saudi Arabia, addressing diabetes takes on added significance due to its high prevalence within families. Engaging patients as educators could directly impact these familial clusters at higher risk. The need to explore the feasibility of this approach lies in its potential to empower and educate within these specific family units, potentially curbing the concerning rise of diabetes in our community. This aligns global strategies with targeted local interventions, emphasizing the potential impact within our specific familial context.
Nonetheless, several critical factors must be taken into account before implementing this approach. Patients’ beliefs, cultural backgrounds, and sociodemographic characteristics may exert a significant influence on various aspects related to diabetes prevention. These include their level of knowledge about diabetes, their perception of family risk, the seriousness attributed to diabetes, concerns regarding family health, and their willingness to engage in health education efforts [ 7 , 8 ]. These factors raise legitimate concerns about the effectiveness of patients serving as health educators to their family members and relatives in the context of diabetes prevention.
Theoretical framework
The Health Belief Model (HBM) provides a theoretical foundation for exploring the factors that may affect individuals’ decisions to act as health educators within their families. These factors include their beliefs about diabetes, perceived benefits and barriers to health education, self-efficacy in performing health education tasks, and concerns about their family members’ susceptibility to diabetes [ 8 ]. The presence of cues to action, as proposed by the HBM, can also play a role in motivating individuals to engage in health-promoting behaviors, including health education for their family members [ 9 , 10 ].
The proposed theoretical framework, based on the HBM and existing literature on diabetes prevention, aims to understand and explain why individuals with diabetes may choose to educate their family members about diabetes prevention. It suggests that individuals are more likely to take on this role if they perceive diabetes as a serious health problem, believe that health education can reduce the risk and severity of diabetes among their family members, have confidence in their ability to effectively educate, and are concerned about their family members’ health [ 8 , 10 ]. Fig 1 explains the substruction of the theoretical framework of HBM ( Fig 1 ).
- PPT PowerPoint slide
- PNG larger image
- TIFF original image
https://doi.org/10.1371/journal.pone.0299790.g001
Therefore, the aims of the study were to assess the patient’s willingness and confidence in their ability to succeed as health educators for their family members and investigate the influencing factors.
Materials and methods
Study design.
To achieve the study objectives, a cross-sectional descriptive study design was employed. The preparation of this report adhered to the Strengthening the Reporting of Observational Studies in Epidemiology (STROBE) checklist for cross-sectional studies [ 11 ].
Setting and participants
This research was conducted from January 2023 to April 2023. A convenience sample was recruited from the Primary Health Clinics (PHC) at King Abdul-Aziz Medical City, Ministry of National Guard Health Affairs in Riyadh and Jeddah cities (MNGHA). Inclusion criteria consisted of Saudi adult patients diagnosed with any type of diabetes who were Arabic speakers. Adults who were unable to read the questionnaire were not included. All eligible participants were invited to join the study voluntarily. The sample size was calculated using G*Power software, which is designed for precise sample size analysis and power calculations. The goal was to enroll n = 122 participants to achieve a statistical power of 95%. This calculation was based on a medium effect size of 0.3, an alpha error probability of 5%, and an estimated 10% rate of missing data. Consequently, the overall sample size was determined as N = (122 participants + 12 accounting for anticipated missing data), resulting in a total of 134 participants.
Recruitment
A recruitment flyer with the questionnaire barcode was distributed to all patients at the chosen site by the research team. Those who were interested in participating were guided either to access the questionnaire link online and complete the survey or to complete a paper-based questionnaire.
Data were collected for this study using the newly developed questionnaire based on the HBM and previous studies. The questionnaire included two parts.
Part one: Socio-demographics and diabetes-related characteristics.
Socio-demographics include age, self-reported gender, marital status, offspring, number of offspring, family type, role within the family, monthly income, and educational level. Diabetes-related characteristics include a family history of diabetes in first- and/or second-degree relatives, diabetes duration (less or more than ten years), treatment (diet, tablets, insulin), diabetes complications (diabetes causing problems with eyes, feet, and/or kidneys), comorbidities, including cardiovascular problems, previous diabetes-related education.
Part two: Patients’ willingness, confidence levels, and influencing factors.
Based on the HBM’s factors and previous studies, participants were asked about different dimensions to assess their willingness and confidence in their ability to succeed as health educators for their family members and to investigate the influencing factors [ 2 – 5 , 7 , 10 ]. Responses are based on their agreement on a 5-point Likert scale ranging from "Strongly agree" to "Strongly disagree". The total score varies from 14 to 70; a higher score indicates a smooth application of this approach, and a lower score indicates the need for interventions to prepare the participants to act as health educators. The cut of point is 45, which indicates the need for interventions.
Self-efficacy . Participants’ willingness and confidence in their ability to succeed as health educators for their family members (self-efficacy) were assessed by asking the participants to rate their agreement on the following items: (1) They already initiated diabetes prevention discussions with their family members and close relatives, (2) they have the ability to act as a health educator, (3) health education preparation will increase their confidence as health educators, (4) they must receive professional education before they act as health educators, and (5) they are willing to be a health educator for their family member to prevent diabetes. The willingness of patients to serve as health educators was also evaluated through a separate binary question. Participants were asked to respond with a "yes" or "no" to indicate whether they were open to acting as health educators for their families and close relatives.
Perceived severity and susceptibility . To assess the participants’ belief of the seriousness of diabetes and the perceived threat of diabetes, they were asked to rate their agreement on the severity of diabetes. Moreover, they were asked to rate the likelihood that their family members and close relatives will develop diabetes.
Perceived benefits and perceived barriers . To assess the possibility of preventing or delaying diabetes onset among family members (perceived benefits), participants were asked to rate their agreement on the following items if they were engaged in educating their family members: (1) make their family members more aware of the importance of lifestyle changes to prevent diabetes, (2) encourage family members to make lifestyle changes, and (3) help their family members in preventing diabetes. With regard to perceived barriers to action, participants were asked to rate their agreement on the following items: (1) they are knowledgeable regarding diabetes prevention education, (2) they know how to inform their family members about diabetes prevention, and (3) they know which family members to educate.
Cues to action . To measure the exposure to factors that prompt action (cues to action), participants were asked to rate their worries about their family members developing diabetes.
Reliability and validity
The first stage of this study involved piloting questionnaires to assess their validity, reliability, and feasibility. Pilot testing was conducted among 10% of the total required sample size and they were not included in the analysis of this paper. The aim of the pilot testing was to evaluate the questionnaire’s performance, identify potential issues, and gather initial data for assessing its reliability and validity.
The validity and reliability of the questionnaire were assessed using established statistical methods, including Cronbach’s alpha, internal consistency, and content validity.
Cronbach’s alpha is a commonly used measure of internal consistency reliability [ 12 ]. It assesses how well the items in a questionnaire or scale are correlated with each other. As shown in the results section of this research, the questionnaire revealed a good Cronbach’s alpha value which indicates good internal consistency, implying that the items in the questionnaire measure the same construct, referring to Table 3 .
Content validity is indeed a crucial aspect of questionnaire development, ensuring that the items effectively measure the intended construct [ 13 , 14 ]. In the current study, content validity was assessed through a process involving four experts and researchers who were knowledgeable about the subject matter. Therefore, four experts and researchers who were familiar with the topic of the questionnaire were selected to evaluate the content of the questionnaire. These individuals are considered experts in the field, making them well-qualified to assess the relevance and comprehensiveness of the questionnaire items. The experts and researchers reviewed the questionnaire items to determine whether they were relevant to the construct being measured. They assessed whether the questions accurately captured the key aspects of the subject matter under investigation. In addition to assessing relevance, the experts also evaluated the questionnaire’s comprehensiveness. They ensured that the items covered a broad range of aspects related to the subject matter, leaving no critical components unaddressed. To facilitate the evaluation process, a 4-point ordinal rating relevance scale was utilized [ 15 ]. This scale likely allowed the experts to provide structured feedback on the relevance of each item, helping to quantify their judgments. Based on the feedback and ratings provided by the experts, any items that were deemed irrelevant or insufficiently comprehensive could be adjusted or revised to enhance content validity, refer to Table 3 .
Statistical analysis
Upon completion of data collection, researchers input the data into an Excel database for subsequent analysis. Statistical Package for the Social Sciences computer software (SPSS for Mac, Version 21.0) was utilized for data analysis.
Regarding patients’ willingness to become health educators, responses were reassessed and categorized into ’Yes’ or ’No’. ’Strongly agree’ and ’Agree’ responses were grouped as ’Yes’, while ’Neutral’, ’Disagree’, or ’Strongly disagree’ were classified as ’No’.
In this study, different analyses were employed considering patients’ willingness to become health educators as the outcome variable. Descriptive statistics—means, standard deviations, frequencies, and percentages—were computed for all variables of interest to characterize the study variables. One-way analysis of variance (ANOVA) and Tukey’s HSD (Honestly Significant Difference) Post Hoc tests were applied to examine mean differences between demographic characteristics (marital status, family type, participant’s role in the family, employment status, education level, and family income) and study variables (perceived severity and susceptibility, benefits, barriers, self-efficacy, cues to action and scale total mean score). Additionally, Pearson’s correlation coefficients were utilized to investigate relationships among continuous variables. The threshold for statistical significance was set at the standard alpha level of .05.
Ethics statement
Institutional Review Board (IRB) approval was obtained from King Abdullah International Medical Research Center (KAIMRC) with IRB No NRC22R/336/08. Various measures were undertaken to protect study participants’ rights and assure confidentiality. Participants’ written informed consent to participate in the study was presumed, and no personally identifiable information was collected.
Demographic characteristics of the participants
A total of 134 participants were recruited from various primary healthcare centers affiliated with the Ministry of National Guard Health Affairs (NGHA). The participants’ ages ranged from 25 to 80 years, with a mean of 52.32 ( SD = 13.23) years. The majority of participants were female (n = 78, 57.8%), while males constituted a smaller proportion (n = 56, 41.5%). A significant percentage of participants, 88.9%, reported having children, whereas only 10.4% did not. Regarding family structure, the majority of participants (n = 69, 51.1%) resided in nuclear families, whereas the fewest participants lived in blended families (n = 5, 3.70%). Approximately 82.2% of participants received health education about diabetes mellitus (DM) from healthcare professionals, including physicians, nurses, and dieticians. For more detailed information about the sample characteristics, please refer to Table 1 .
https://doi.org/10.1371/journal.pone.0299790.t001
Patients’ willingness and confidence level of health educators
The majority of participants expressed their willingness to serve as health educators for diabetes prevention among their family members and close relatives (n = 117, 87.31%), as shown in Table 1 . In terms of self-efficacy scores, they reported from 12.00 to 25.00, with a mean of 21.12 (SD = 2.76), signifying a high level of willingness and confidence among participants regarding their ability to succeed as health educators for their family members, as detailed in Table 3 .
The influencing factors of willingness of health educators
The influencing factors on the willingness of health educators were investigated in this study. Significant differences in education levels were observed concerning both the total scale score and self-efficacy, with F (5, 128) = 3.865, p = .003, and F (5, 128) = 5.269, p < .001, respectively. Participants holding high school diploma certificates exhibited notably lower total mean scores (M = 54.54, SD = 8.33) and lower self-efficacy mean scores (M = 19.08, SD = 3.33) compared to those with lower or other levels of education. Additionally, significant disparities in family income were identified in relation to the total scale score, with F (4, 129) = 4.128, p = .004. Participants with family incomes between SR5,000 and SR10,000 had significantly lower mean scores (M = 55.75, SD = 8.18) than participants with family incomes below SR5,000 or above SR10,000.
With regard to the perceived susceptibility/severity domain, there was a significant difference based on the participant’s role within the family, employment status, education level, and family income. As shown in Table 1 , there were significant differences for the participant’s role within the family and perceived severity and susceptibility, F (5, 128) = 3.46, p = .007. Grandmother participants had significantly lower mean scores (M = 7.33, SD = .51) than being son (M = 8.00, SD = .92), daughter (M = 9.25, SD = .50), mother (M = 8.06, SD = 1.50), father (M = 8.33, SD = 1.30), and grandfather participants (M = 10.00, SD = .000). Moreover, there were significant employment status differences with perceived severity and susceptibility, F (4, 129) = 4.869, p = .001. Unemployed participants had significantly lower mean scores ( M = 7.34, SD = 1.49) than full-time employee ( M = 8.50, SD = 1.26), part-time employee ( M = 7.92, SD = 1.49), retired ( M = 8.43, SD = 1.09), and student participants ( M = 9.50, SD = .70). In addition, there were significant education level differences with perceived severity and susceptibility, F (5, 128) = 4.068, p = .002. Participants with high school certificate had significantly lower mean scores ( M = 7.44, SD = 1.62) than uneducated/other level of education participants. Lastly, there were significant family income differences with perceived severity and susceptibility, F (4, 129) = 5.155, p < .001. Participants with family income between SR5,000-SR10,000 had significantly lower mean scores (M = 7.70, SD = 1.51) than participants whose family income less than SR5,000 and more than SR10,000.
With regard to the perceived benefits/barriers domain, there was a significant difference based on marital status, education level, and family income. As shown in Table 1 , there were significant marital status differences with perceived benefits and barriers, F (3, 130) = 4.194, p = .007. Widowed participants had significantly lower mean scores (M = 21.62, SD = 3.73) than single (M = 26.90, SD = 2.80), married (M = 24.65, SD = 3.46) and divorced participants (M = 25.00, SD = 2.07). In addition, there were significant education level differences with perceived benefits and barriers, F (5, 128) = 3.947, p = .002. Participants with high diploma certificates had significantly lower mean scores (M = 22.80, SD = 3.02) than uneducated/other level of education participants. Also, there were significant family income differences with perceived benefits and barriers, F (4, 129) = 5.669, p < .001. Participants with family income between SR5,000-SR10,000 had significantly lower mean scores (M = 23.06, SD = 3.75) than participants whose family income less than SR5,000 and more than SR10,000.
With regard to cues to action domain, there was a significant difference based on the participants age and education level.
As shown in Table 1 , there were significant participants age differences with cues to action, F (5, 128) = 3.899, p = .003. Participants older than 70 years had significantly lower mean scores (M = 3.81, SD = .75) than those participants younger than 70 years. In addition, there were significant educational level differences with cues to action, F (5, 128) = 3.886, p = .003. Participants with high diploma certificate had significantly lower mean scores (M = 4.04, SD = .69) than uneducated/other level of education participants.
In the correlation analysis presented in Table 2 , participants’ willingness to serve as health educators for their family members and close relatives demonstrated positive and moderate correlations with perceived severity and susceptibility (r = .433, p < .01), perceived benefits and barriers (r = .451, p < .01), and cues to action (r = .520, p < .01). Conversely, participants’ willingness to take on this role exhibited a strong positive correlation with self-efficacy (r = .789, p < .01). Furthermore, self-efficacy was positively and moderately correlated with perceived severity and susceptibility (r = .678, p < .01) and cues to action (r = .633, p < .01), as well as positively and strongly correlated with perceived benefits and barriers (r = .720, p < .01).
https://doi.org/10.1371/journal.pone.0299790.t002
The questionnaire scores, as outlined in Table 3 , showcased the following statistics: total scores ranged from 38 to 70, with a mean of 58.44 (SD = 7.35); perceived severity and susceptibility scores ranged from 4.00 to 10.00, with a mean of 8.19 (SD = 1.36); perceived benefits and barriers scores ranged from 16.00 to 30.00, with a mean of 24.66 (SD = 3.47); self-efficacy scores ranged from 12.00 to 25.00, with a mean of 21.12 (SD = 2.76); and cues to action scores ranged from 2.00 to 5.00, with a mean of 4.45 (SD = 0.70).
https://doi.org/10.1371/journal.pone.0299790.t003
In Table 3 , the assessment of internal consistency using Cronbach’s alpha coefficient revealed satisfactory reliability measures for the scales employed in this study. The 2-item perceived severity and susceptibility scale achieved a Cronbach’s alpha coefficient of 0.79, with item total correlation coefficients spanning from 0.14 to 0.85. Similarly, the 6-item perceived benefits and barriers scale demonstrated a Cronbach’s alpha coefficient of 0.79, accompanied by item-total correlation coefficients ranging from 0.076 to 0.81. The 5-item self-efficacy scale achieved a Cronbach’s alpha coefficient of 0.77, with item total correlation coefficients ranging from 0.10 to 0.78, which meets acceptable reliability standards. Additionally, the 1-item cues to the action scale exhibited a Cronbach’s alpha coefficient of 1.00.
For content validity assessment, a 4-point ordinal rating relevance scale was employed to evaluate the appropriateness of the scale’s items (Lenz, 2020). As detailed in Table 3 , the interrater reliability for both the total scale score and the four individual domains exceeded 75%. Furthermore, both the item content validity index (I-CVI) and the scale content validity index (S-CVI) for the total scale score and its four domains surpassed the threshold of 0.80. This signifies that all scales utilized in the study demonstrated acceptable levels of content validity.
This study provides valuable insights into diabetes prevention research by introducing a novel approach that empowers patients to serve as health educators for their family members. To our knowledge, this study represents the initial endeavor to investigate the empowerment of patients as health educators. The study’s aims were to assess patients’ willingness to take on this role, measure their confidence in their ability to succeed as health educators and examine the relationship between various factors in the Health Belief Model (HBM) and the success of this approach.
The study’s findings reveal significant insights into the willingness and confidence of participants to take on the role of health educators for their family members and close relatives with regard to diabetes. A striking 87% of the participants expressed their willingness to serve as health educators for their family members and close relatives. This high percentage underscores a notable openness among patients to actively engage in health education within their immediate social circles. This willingness suggests a potential for leveraging patients as valuable resources in disseminating crucial information about diabetes prevention.
Another noteworthy finding is that the participants reported having a high self-efficacy level related to educating their family members and close relatives about diabetes. This level of self-efficacy among patients suggests that many of them perceive themselves as effective communicators and educators regarding diabetes-related information. This is particularly promising because confidence in one’s ability to convey health-related messages is a critical factor in the success of health education efforts [ 4 , 16 ]. A notable correlation emerged between higher education levels and self-efficacy. This result aligns with existing literature, supporting the idea that education significantly contributes to enhancing self-efficacy [ 17 ].
Moreover, the study’s findings shed light on the factors influencing participants’ willingness to take on the role of health educators for their family members and close relatives regarding diabetes. These correlations provide valuable insights into the motivations and determinants that influence their readiness to engage in this novel approach.
Looking at the total score, the results highlight that significant differences were found in the total score of the tool based on the patient’s education and income level. This aligns with existing literature suggesting a strong correlation between higher education levels and enhanced cognitive abilities or self-efficacy in various domains [ 18 ]. The disparity in scores emphasizes the potential influence of educational attainment on individuals’ abilities to engage effectively with health-related information or tasks. Furthermore, these findings highlight the need for tailored interventions or educational approaches targeted at individuals with lower educational backgrounds. With regards to income status, this finding aligns with existing socio-economic research indicating that lower income levels often correlate with reduced access to resources, which can influence health-related knowledge or practices [ 19 , 20 ]. Individuals with limited financial means might face barriers to accessing quality healthcare or health-related information, potentially impacting their overall understanding or engagement with health-related measures. Moreover, this finding emphasizes the necessity for targeted interventions or support programs that consider the socio-economic context of individuals. Efforts to bridge this gap might involve initiatives offering accessible health-related information or resources tailored to individuals from lower-income brackets. Additionally, advocating for policies that address socio-economic inequalities could contribute to more equitable health outcomes across diverse income groups.
The analysis revealed significant associations between the perceived susceptibility and severity domain and several participant characteristics, namely their role within the family, employment status, education level, and family income. These disparities shed light on the diverse perceptions of susceptibility and severity regarding health concerns among different demographic groups.
The findings indicated a notable connection between participants’ familial roles and their perceptions of susceptibility and severity. Grandmothers exhibited significantly lower mean scores in perceived susceptibility and severity compared to other family roles, such as sons, daughters, mothers, fathers, and grandfathers. This variation might stem from differing responsibilities or perspectives within the family structure, influencing their awareness or understanding of health-related risks. Moreover, employment status emerged as another influential factor. Unemployed participants displayed significantly lower mean scores in perceived susceptibility and severity compared to full-time employees, part-time employees, retirees, and students. This finding echoes the literature that employment status as a significant role in the perceived susceptibility and severity [ 21 – 23 ]. This suggests that the nature of one’s occupation or lack thereof might influence their perceptions of health risks and the severity of potential health outcomes. Similarly, education level played a crucial role, with participants holding a high school certificate reporting significantly lower mean scores in perceived susceptibility/severity compared to those with other education levels. This aligns with existing literature indicating a correlation between higher education and greater health literacy, potentially impacting individuals’ perceptions of health risks [ 24 ]. Furthermore, family income disparities were associated with differences in perceived susceptibility/severity. This suggests that varying financial situations might influence individuals’ perceptions of the severity of health-related issues, possibly due to differential access to healthcare or resources. These findings underscore the multifaceted nature of perceptions regarding health risks within diverse demographic groups. Tailored health interventions and educational programs considering these demographic variations might effectively address and mitigate disparities in health perceptions and enhance health-related understanding across different populations.
The analysis regarding the perceived benefits/barriers domain revealed significant associations with three key demographic factors: marital status, education level, and family income. These findings highlight the influence of these factors on individuals’ perceptions regarding the advantages and obstacles associated with health behaviors or practices.
Marital status emerged as a significant factor influencing perceived benefits and barriers, illustrating notable differences among widowed participants in comparison to other individuals. Widowed individuals reported significantly lower mean scores in perceived benefits and barriers, indicating potentially unique perspectives on health-related advantages and challenges compared to other marital statuses. These findings align with existing literature. For instance, research suggests higher mortality rates among individuals who are non-married or previously married compared to those who are married [ 25 ]. This discrepancy might reflect a lower engagement in protective or healthy behaviors among non-married individuals, contributing to adverse health outcomes [ 25 ]. Also this was supported in the literature that marital status is an important predictor of healthcare utilization [ 26 ].
Education level exhibited notable disparities in perceived benefits and barriers. Participants with high diploma certificates reported significantly lower mean scores compared to those with uneducated/other levels of education. This suggests that varying educational backgrounds may significantly influence individuals’ perceptions of the advantages and hindrances associated with health behaviors, potentially due to differences in health literacy or exposure to health-related information. Additionally, family income disparities were linked to variations in perceived benefits and barriers. Participants with mid-level family incomes reported significantly lower mean scores compared to those with lower or higher incomes. This findings is supported in the literature and underscores how differing financial circumstances might shape individuals’ perceptions of the benefits and barriers related to health practices, likely influenced by disparities in access to healthcare resources or information [ 27 – 29 ].
These findings emphasize the intricate relationship between demographic factors and perceptions of the benefits and barriers associated with health behaviors. Understanding these variations is vital for tailoring targeted health interventions and educational programs that account for diverse perspectives stemming from different demographic backgrounds. Addressing these discrepancies can aid in the development of more effective strategies to promote health behaviors and overcome barriers across various population groups.
One significant finding is a positive and moderate correlation between participants’ willingness to act as health educators and their perceptions of both the severity and susceptibility of diabetes in their family members. This suggests that individuals who view diabetes as a significant health threat and consider their family members susceptible to it are more likely to volunteer as health educators. This underscores the pivotal role of individuals’ perceptions of the severity of the health issue in motivating proactive health education, consistent with previous research [ 30 – 32 ].
A similar positive and moderate correlation emerged between individuals’ willingness to serve as health educators and their perceptions of benefits and barriers. Those who perceive greater advantages in health education and encounter fewer obstacles tend to be more inclined to express their readiness to educate their family members and close relatives. Consequently, interventions that emphasize the advantages of health education and mitigate barriers have the potential to boost individuals’ willingness to participate in such activities, as previous studies have demonstrated [ 33 ].
The study also revealed a strong and positive correlation between the willingness of individuals to become health educators and the presence of cues to action. Participants who reported receiving cues or prompts to engage in health education were more likely to express their readiness to educate their family members and close relatives. This underscores the significance of external triggers, reminders, or prompts in motivating individuals to embrace the role of health educators. These findings align with prior research that highlights the importance of triggers in maintaining self-management and promoting other favorable healthcare activities [ 34 ].
Finally, perhaps one of the most significant findings is the strong positive correlation between participants’ willingness to be health educators and their self-efficacy. This suggests that individuals who have higher levels of self-efficacy and confidence in their ability to educate others are more inclined to embrace the role of health educators within their families. As participants’ self-efficacy and confidence increased, their willingness to educate their family members and close relatives also increased.
These findings underscore the multifaceted nature of individuals’ willingness to serve as health educators for their family members and close relatives. They highlight the importance of addressing perceptions, motivations, and self-belief when designing interventions aimed at promoting health education within communities. Moreover, they emphasize the need for healthcare providers and public health initiatives to foster self-efficacy and provide cues to action as strategies to engage patients effectively in health education efforts, particularly in the context of diabetes prevention. These findings collectively highlight the potential for a patient-centric approach in diabetes prevention efforts. Patients’ willingness and confidence to take on the role of health educators for their family members and close relatives suggest an opportunity to empower individuals with diabetes to actively contribute to the health and well-being of their loved ones [ 16 ]. This approach not only capitalizes on the unique position of patients but also fosters a sense of shared responsibility within families and communities, which can be instrumental in combating the rising prevalence of diabetes.
Finally, these findings highlight the need for healthcare providers and public health initiatives to recognize and support patients in their roles as health educators. By providing the necessary resources, training, and guidance, healthcare systems can harness the potential of patients to extend the reach of diabetes prevention efforts and ultimately reduce the burden of this chronic condition within families and communities [ 16 ].
Limitations
This study has several notable limitations that should be acknowledged. Firstly, it relies on a convenience sample rather than employing a random sampling technique, which makes it challenging to generalize the results to broader populations. Additionally, there is a potential for a social desirability bias, as participants may have responded in ways they deemed favorable to the research team or societal expectations. Furthermore, the study tools used were not validated in a large sample, which may impact the reliability and validity of the measurements. Lastly, it is possible that highly motivated patients were more likely to respond to the questionnaire, while those with little interest in the topic may have been less inclined to participate, potentially introducing bias into the study. Nonetheless, it’s important to note that the data collected successfully addresses all the study’s objectives.
This study has illuminated significant insights into empowering patients as health educators for their family members in the context of diabetes prevention. Our investigation aimed to assess patients’ willingness, confidence, and the influencing factors related to this role, uncovering compelling findings. Notably, an overwhelming 87% of participants expressed willingness to act as health educators for their family members, indicating a high openness among patients to actively engage in health education. Moreover, participants exhibited high self-efficacy, feeling confident in their ability to effectively educate their relatives about diabetes. The study identified various factors influencing patients’ willingness to serve as health educators, such as perceptions of severity, susceptibility, benefits, and barriers related to diabetes within their families. Additionally, cues to action and increased self-efficacy emerged as strong motivators for patients to take on this role. These findings underscore the multifaceted nature of individuals’ readiness to act as health educators and stress the importance of addressing perceptions, motivations, and self-belief in designing effective health education interventions. Leveraging patients as educators not only capitalizes on their unique position but also fosters shared responsibility within families and communities, potentially instrumental in combating diabetes.
To optimize the impact of patients with diabetes as health educators, specific strategies are crucial. Develop comprehensive training modules to equip patients with effective communication skills for diabetes prevention and management. Practical examples and interactive sessions can enhance their teaching abilities. Moreover, create interactive online forums and support networks where patient educators can exchange experiences, successful approaches, and valuable insights. Platforms like virtual seminars or dedicated websites can amplify their collective impact. In addition, foster partnerships between healthcare institutions, community organizations, and patient advocacy groups is also important. These collaborations can broaden the reach of patient educators, leveraging varied platforms and resources for wider dissemination. Lastly, acknowledgment and incentives can play a crucial role, for example, recognize and celebrate the contributions of patient educators through public acknowledgments, awards, or incentives. Acknowledgment ceremonies or rewards for outstanding contributions can motivate others to embrace this crucial role. By incorporating these tailored strategies, the potential of patients with diabetes as health educators can be unlocked, significantly amplifying their positive influence on diabetes awareness, prevention, and care within communities.
Supporting information
S1 data set..
https://doi.org/10.1371/journal.pone.0299790.s001
https://doi.org/10.1371/journal.pone.0299790.s002
https://doi.org/10.1371/journal.pone.0299790.s003
https://doi.org/10.1371/journal.pone.0299790.s004
- View Article
- Google Scholar
- PubMed/NCBI
This paper is in the following e-collection/theme issue:
Published on 17.4.2024 in Vol 26 (2024)
Service Quality and Residents’ Preferences for Facilitated Self-Service Fundus Disease Screening: Cross-Sectional Study
Authors of this article:

Original Paper
- Senlin Lin 1, 2, 3 * , MSc ;
- Yingyan Ma 1, 2, 3, 4 * , PhD ;
- Yanwei Jiang 5 * , MPH ;
- Wenwen Li 6 , PhD ;
- Yajun Peng 1, 2, 3 , BA ;
- Tao Yu 1, 2, 3 , BA ;
- Yi Xu 1, 2, 3 , MD ;
- Jianfeng Zhu 1, 2, 3 , MD ;
- Lina Lu 1, 2, 3 , MPH ;
- Haidong Zou 1, 2, 3, 4 , MD
1 Shanghai Eye Diseases Prevention &Treatment Center/ Shanghai Eye Hospital, School of Medicine, Tongji University, Shanghai, China
2 National Clinical Research Center for Eye Diseases, Shanghai, China
3 Shanghai Engineering Research Center of Precise Diagnosis and Treatment of Eye Diseases, Shanghai, China
4 Shanghai General Hospital, School of Medicine, Shanghai Jiao Tong University, Shanghai, China
5 Shanghai Hongkou Center for Disease Control and Prevention, Shanghai, China
6 School of Management, Fudan University, Shanghai, China
*these authors contributed equally
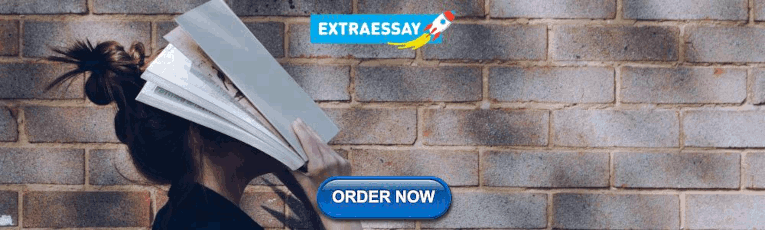
Corresponding Author:
Haidong Zou, MD
Shanghai Eye Diseases Prevention &Treatment Center/ Shanghai Eye Hospital
School of Medicine
Tongji University
No 1440, Hongqqiao Road
Shanghai, 200336
Phone: 86 02162539696
Email: [email protected]
Background: Fundus photography is the most important examination in eye disease screening. A facilitated self-service eye screening pattern based on the fully automatic fundus camera was developed in 2022 in Shanghai, China; it may help solve the problem of insufficient human resources in primary health care institutions. However, the service quality and residents’ preference for this new pattern are unclear.
Objective: This study aimed to compare the service quality and residents’ preferences between facilitated self-service eye screening and traditional manual screening and to explore the relationships between the screening service’s quality and residents’ preferences.
Methods: We conducted a cross-sectional study in Shanghai, China. Residents who underwent facilitated self-service fundus disease screening at one of the screening sites were assigned to the exposure group; those who were screened with a traditional fundus camera operated by an optometrist at an adjacent site comprised the control group. The primary outcome was the screening service quality, including effectiveness (image quality and screening efficiency), physiological discomfort, safety, convenience, and trustworthiness. The secondary outcome was the participants’ preferences. Differences in service quality and the participants’ preferences between the 2 groups were compared using chi-square tests separately. Subgroup analyses for exploring the relationships between the screening service’s quality and residents’ preference were conducted using generalized logit models.
Results: A total of 358 residents enrolled; among them, 176 (49.16%) were included in the exposure group and the remaining 182 (50.84%) in the control group. Residents’ basic characteristics were balanced between the 2 groups. There was no significant difference in service quality between the 2 groups (image quality pass rate: P =.79; average screening time: P =.57; no physiological discomfort rate: P =.92; safety rate: P =.78; convenience rate: P =.95; trustworthiness rate: P =.20). However, the proportion of participants who were willing to use the same technology for their next screening was significantly lower in the exposure group than in the control group ( P <.001). Subgroup analyses suggest that distrust in the facilitated self-service eye screening might increase the probability of refusal to undergo screening ( P =.02).
Conclusions: This study confirms that the facilitated self-service fundus disease screening pattern could achieve good service quality. However, it was difficult to reverse residents’ preferences for manual screening in a short period, especially when the original manual service was already excellent. Therefore, the digital transformation of health care must be cautious. We suggest that attention be paid to the residents’ individual needs. More efficient man-machine collaboration and personalized health management solutions based on large language models are both needed.
Introduction
Vision impairment and blindness are caused by a variety of eye diseases, including cataracts, glaucoma, uncorrected refractive error, age-related macular degeneration, diabetic retinopathy, and other eye diseases [ 1 ]. They not only reduce economic productivity but also harm the quality of life and increase mortality [ 2 - 6 ]. In 2020, an estimated 43.3 million individuals were blind, and 1.06 billion individuals aged 50 years and older had distance or near vision impairment [ 7 ]. With an increase in the aging population, the number of individuals affected by vision loss has increased substantially [ 1 ].
High-quality public health care for eye disease prevention, such as effective screening, can assist in eliminating approximately 57% of all blindness cases [ 8 ]. Digital technologies, such as telemedicine, 5G telecommunications, the Internet of Things, and artificial intelligence (AI), have provided the potential to improve the accessibility, availability, and productivity of existing resources and the overall efficiency of eye care services [ 9 , 10 ]. The use of digital technology not only reduces the cost of eye disease screening and improves its efficiency, but also assists residents living in remote areas to gain access to eye disease screening [ 11 - 13 ]. Therefore, an increasing number of countries (or regions) are attempting to establish eye screening systems based on digital technology [ 9 ].
Fundus photography is the most important examination in eye disease screening because the vast majority of diagnoses of blinding retinal diseases are based on fundus photographs. Diagnoses can be made by human experts or AI software. However, traditional fundus cameras must be operated by optometrists, who are usually in short supply in primary health care institutions when faced with the large demand for screening services.
Fortunately, the fully automatic fundus camera has been developed on the basis of digital technologies including AI, industrial automation, sensors, and voice navigation. It can automatically identify the person’s left and right eyes, search for pupils, adjust the lens position and shooting focus, and provide real-time voice feedback during the process, helping the residents to understand the current inspection steps clearly and cooperatively complete the inspection. Therefore, a facilitated self-service eye screening pattern has been newly established in 2022 in Shanghai, China.
However, evidence is inadequate about whether this new screening pattern performs well and whether the residents prefer it. Therefore, this cross-sectional study aims to compare the service quality and residents’ preferences of this new screening pattern with that of the traditional screening pattern. We aimed to (1) investigate whether the facilitated self-service eye screening can achieve service quality similar to that of traditional manual screening, (2) compare residents’ preferences between the facilitated self-service eye screening and traditional manual screening, and (3) explore the relationship between the screening service quality and residents’ preferences.
Study Setting
This study was conducted in Shanghai, China, in 2022. Since 2010, Shanghai has conducted an active community-based fundus disease telemedicine screening program. After 2018, an AI model was adopted ( Figure 1 ). At the end of 2021, the fully automatic fundus camera was adopted, and the facilitated self-service fundus disease screening pattern was established ( Figure 1 ). Within this new pattern, residents could perform fundus photography by themselves without professionals’ assistance ( Multimedia Appendix 1 ). The fundus images were sent to the cloud server center of the AI model, and the screening results were fed back immediately.

Study Design
We conducted a cross-sectional study at 2 adjacent screening sites. These 2 sites were expected to be very similar in terms of their socioeconomic and educational aspects since they were located next to each other. One site provided facilitated self-service fundus disease screening, and the residents who participated therein comprised the exposure group; the other site provided screening with a traditional fundus camera operated by an optometrist, and the residents who participated therein comprised the control group. All the adult residents could participant in our screening program, but their data were used for analysis only if they signed the informed consent form. Residents could opt out of the study at any time during the screening.
In the exposure group, the residents were assessed using an updated version of the nonmydriatic fundus camera Kestrel 3100m (Shanghai Top View Industrial Co Ltd) with a self-service module. In the process of fundus photography, the residents pressed the “Start” button by themselves. All checking steps (including focusing, shooting, and image quality review) were undertaken automatically by the fundus camera ( Figure 2 ). Screening data were transmitted to the AI algorithm on a cloud-based server center through the telemedicine platform, and the screening results were fed back immediately. Residents were fully informed that the assessment was fully automated and not performed by the optometrist.

In the control group, the residents were assessed using the basic version of the same nonmydriatic fundus camera. The optical components were identical to those in the exposure group but without the self-service module. In the process of fundus photography, all steps were carried out by the optometrist (including focusing, shooting, and image quality review). Screening data were transmitted to the AI algorithm on a cloud-based server center through the telemedicine platform, and the screening results were fed back immediately. Residents were also fully informed.
Measures and Outcomes
The primary outcome was the screening service’s quality. Based on the World Health Organization’s recommendations for the evaluation of AI-based medical devices [ 14 ] and the European Union’s Assessment List for Trustworthy Artificial Intelligence [ 15 ], 5 dimensions were selected to reflect the service quality of eye disease screening: effectiveness, physiological discomfort, safety, convenience, and trustworthiness.
Furthermore, effectiveness was based on 2 indicators: image quality and screening efficiency. A staff member recorded the time required for each resident to take fundus photographs (excluding the time taken for diagnosis) at the screening site. Then, a professional ophthalmologist evaluated the quality of each fundus photograph after the on-site experiment. The ophthalmologist was blinded to the grouping of participants. Image quality was assessed on the basis of the image quality pass rate, expressed as the number of eyes with high-quality fundus images per 100 eyes. Screening efficiency was assessed on the basis of the average screening time, expressed as the mean of the time required for each resident to take fundus photographs.
To assess physiological discomfort, safety, convenience, and trustworthiness of screening services, residents were asked to finish a questionnaire just after they received the screening results. A 5-point Likert scale was adopted for each dimension, from the best to the worst, except for the physiological discomfort ( Multimedia Appendix 2 ). A no physiological discomfort rate was expressed as the number of residents who chose the “There is no physiological discomfort during the screening” per 100 individuals in each group. Safety rate is expressed as the number of residents who chose “The screening is very safe” or “The screening is safe” per 100 individuals in each group. Convenience rate is expressed as the number of residents who chose “The screening is very convenient” or “The screening is convenient” per 100 individuals in each group. The trustworthiness rate is expressed as the number of residents who chose “The screening result is very trustworthy” or “The screening result is trustworthy” per 100 individuals in each group.
The secondary outcome was the preference rate, expressed as the number of residents who were willing to use the same technology for their next screening per 100 individuals. In detail, in the exposure group, the preference rate was expressed as the number of the residents who preferred facilitated self-service eye screening per 100 individuals, while in the control group, it was expressed as the number of residents who preferred traditional manual screening per 100 individuals.
To understand the residents’ preference, a video displaying the processes of both facilitated self-service eye screening and traditional manual screening was shown to the residents. Then, the following question was asked: “At your next eye disease screening, you can choose either facilitated self-service eye screening or traditional manual screening. Which one do you prefer?” A total of 4 alternatives were set: “Prefer traditional manual screening,” “Prefer facilitated self-service eye screening,” “Both are acceptable,” and “Neither is acceptable (Refusal of screening).” Each resident could choose only 1 option, which best reflected their preference.
Sample Size
The rule of events per variable was used for sample size estimation. In this study, 2 logit models were established for the 2 groups separately, each containing 8 independent variables. We set 10 events per variable in general. According to a previous study [ 16 ], when the decision-making process had high uncertainty, the proportion of individuals who preferred the algorithms was about 50%. This led us to arrive at a sample size of 160 (8 variables multiplied by 10 events each, with 50% of individuals potentially preferring facilitated screening [ie, 50% of 8×10]) for each group.
Every dimension of the screening service quality and the preference rate were calculated separately. Chi-square and t tests were used to test whether the service quality or the residents’ preferences differed between the 2 groups. A total of 7 hypotheses were tested, as shown in Textbox 1 .
- H1: image quality pass rate exposure group ≠ image quality pass rate control group H0: image quality pass rate exposure group =image quality pass rate control group
- H1: screening time exposure group ≠screening time control group H0: screening time exposure group =screening time control group
- H1: no discomfort rate exposure group ≠no discomfort rate control group H0: no discomfort rate exposure group = no discomfort rate control group
- H1: safety rate exposure group ≠safety rate control group H0: safety rate exposure group = safety rate control group
- H1: convenience rate exposure group ≠convenience rate control group H0: convenience rate exposure group = convenience rate control group
- H1: trustworthiness rate exposure group ≠trustworthiness rate control group H0: trustworthiness rate exposure group = trustworthiness rate control group
- H1: preference rate exposure group ≠preference rate control group H0: preference rate exposure group = preference rate control group
If any of the hypotheses among hypotheses 1-6 ( Textbox 1 ) were significant, it indicated that the service quality was different between facilitated self-service eye screening and traditional manual screening. If hypothesis 7 was significant, it meant that the residents’ preference for facilitated self-service eye screening was different from that for traditional manual screening.
Additionally, subgroup analyses in the exposure and control groups were conducted to explore the relationships between the screening service quality and the residents’ preferences, using generalized logit models. The option “Prefer facilitated self-service eye screening” was used as the reference level for the dependent variable in the models. The independent variables included age, sex, image quality, screening efficiency, physiological discomfort, safety, convenience, and trustworthiness. All statistics were performed using SAS (version 9.4; SAS Institute).
Ethical Considerations
The study adhered to the ethical principles of the Declaration of Helsinki and was approved by the Shanghai General Hospital Ethics Committee (2022SQ272). All participants provided written informed consent before participating in this study. The study data were anonymous, and no identification of individual participants in any images of the manuscript or supplementary material is possible.
Participants’ Characteristics
A total of 358 residents enrolled; among them, 176 (49.16%) were in the exposure group and the remaining 182 (50.84%) were in the control group. Residents’ basic characteristics were balanced between the 2 groups. The mean age was 65.05 (SD 12.28) years for the exposure group and 63.96 (SD 13.06) years for the control group; however, this difference was nonsignificant ( P =.42). The proportion of women was 67.05% (n=118) for the exposure group and 62.09% (n=113) for the control group; this difference was also nonsignificant between the 2 groups ( P =.33).
Screening Service Quality
In the exposure group, high-quality fundus images were obtained for 268 out of 352 eyes (image quality pass rate=76.14%; Figure 3 ). The average screening time was 81.03 (SD 36.98) seconds ( Figure 3 ). In the control group, high-quality fundus images were obtained for 274 out of 364 eyes (image quality pass rate=75.27%; Figure 3 ). The average screening time was 78.22 (SD 54.01) seconds ( Figure 3 ). There was no significant difference in the image quality pass rate ( χ 2 1 =0.07, P =.79) and average screening time ( t 321.01 =–0.58 [Welch–Satterthwaite–adjusted df ], P =.56) between the 2 groups ( Figure 3 ).

For the other dimensions, detailed information is shown in Figure 3 . There were no significant differences between any of these rates between the 2 groups (no physiological discomfort rate: χ 2 1 =0.01, P =.92; safety rate: χ 2 1 =0.08, P =.78; convenience rate: χ 2 1 =0.004, P =.95; trustworthiness rate: χ 2 1 =1.63, P =.20).
Residents’ Preferences
In the exposure group, 120 (68.18%) residents preferred traditional manual screening, 19 (10.80%) preferred facilitated self-service eye screening, 19 (10.80%) preferred both, and the remaining 18 (10.23%) preferred neither. In the control group, 123 (67.58%) residents preferred traditional manual screening, 14 (7.69%) preferred facilitated self-service eye screening, 20 (10.99%) preferred both, and the remaining 25 (13.74%) preferred neither.
The proportion of residents who chose the category “Prefer facilitated self-service eye screening” in the exposure group was significantly lower than that of residents who chose the category “Prefer traditional manual screening” in the control group ( χ 2 1 =120.57, P <.001; Figure 3 ).
Subgroup Analyses
In the exposure group, 4 generalized logit models were generated ( Table 1 ). Regarding the effectiveness of facilitated self-service eye screening, neither the image quality nor the screening time had an impact on the residents’ preferences. Regarding the other dimensions for facilitated self-service eye screening service quality, models 3 and 4 demonstrated that distrust in the results of facilitated self-service eye screening might decrease the probability of preferring this screening service and increase the probability of preferring neither of the 2 screening services.
a Age and gender were adjusted in model 1. Age, gender, image quality, and screening efficiency were adjusted in model 2. Age, gender, physiological discomfort, safety, convenience, and trustworthiness were adjusted in model 3. Age, gender, image quality, screening efficiency, physiological discomfort, safety, convenience, and trustworthiness were adjusted in model 4.
b In the exposure group, distrust in the results of facilitated self-service eye screening might decrease the probability of preferring this screening service and increase the probability of preferring neither the traditional nor the facilitated self-service screening services.
c Not available.
In the control group, another 4 generalized logit models were generated ( Table 2 ). Men were more likely to choose a preference both screening services. The probability of preferring manual screening might increase with age, as long as the probability of preferring facilitated self-service eye screening decreased. Regarding the effectiveness of traditional manual screening, neither the image quality pass rate nor the screening time had an impact on the residents’ preferences. For the other dimensions of the quality of traditional manual screening, models 7 and 8 showed that if the residents feel unsafe about traditional manual screening, their preference for traditional manual screening might decrease, and they might turn to facilitated self-service eye screening.
a Age and gender were adjusted in model 5. Age, gender, image quality, and screening efficiency were adjusted in model 6. Age, gender, physiological discomfort, safety, convenience, and trustworthiness were adjusted in model 7. Age, gender, image quality, screening efficiency, physiological discomfort, safety, convenience, and trustworthiness were adjusted in model 8.
b In the control group, if the residents feel unsafe about traditional manual screening, their preference for traditional manual screening might decrease, and they might turn to facilitated self-service eye screening.
A new fundus disease screening pattern was established using the fully automatic fundus camera without any manual intervention. Our findings suggest that facilitated self-service eye screening can achieve a service quality similar to that of traditional manual screening. The study further evaluated the residents’ preferences and associated factors for the newly established self-service fundus disease screening. Our study found that the residents’ preference for facilitated self-service eye screening is significantly less than that for traditional manual screening. This implies that the association between the service quality of the screening technology and residents’ preferences was weak, suggesting that aversion to the algorithm might exist. In addition, the subgroup analyses suggest that even the high quality of facilitated self-service eye screening cannot increase the residents’ preference for this new screening pattern. Worse still, distrust in the results of this new pattern may lead to lower usage of eye disease screening services as a whole. To the best of our knowledge, this study is one of the first to evaluate service quality and residents’ preferences for facilitated self-service fundus disease screening.
Previous studies have suggested that people significantly prefer manual services to algorithms in the field of medicine [ 16 - 18 ]. Individuals have an aversion to algorithms underlying digital technology, especially when they see errors in the algorithm’s functioning [ 18 ]. The preference for algorithms does not increase even if the residents are told that the algorithm outperforms human doctors [ 19 , 20 ]. Our results confirm that fundus image quality in the exposure group is similar to that in the control group in our study, and both are similar to or even better than those reported in previous studies [ 21 , 22 ]. However, the preference for facilitated self-service fundus disease screening is significantly less than that for traditional manual screening. One possible explanation is that uniqueness neglect—a concern that algorithm providers are less able than human providers to account for residents’ (or patients’) unique characteristics and circumstances—drives consumer resistance to digital medical technology [ 23 ]. Therefore, personalized health management solutions based on large language models should be developed urgently [ 24 ] to meet the residents’ individual demands. In addition, a survey of population preferences for medical AI indicated that the most important factor for the public is that physicians are ultimately responsible for diagnosis and treatment planning [ 25 ]. As a result, man-machine collaboration, such as human supervision, is still necessary [ 26 ], especially in the early stages of digital transformation to help residents understand and accept the digital technologies.
Furthermore, our study suggests that distrust in the results of facilitated self-service fundus disease screening may cause residents to abandon eye disease screening, irrespective of whether it is provided using this new screening pattern or via the traditional manual screening pattern. This is critical to digital transformation in medicine. This implies that if the digital technology does not perform well, residents will not only be averse to the digital technology itself but also be more likely to abandon health care services as a whole. Digital transformation is a fundamental change to the health care delivery system. This implies that it can self-disrupt its ability to question the practices and production models of existing health care services. As a result, it may become incompatible with the existing models, processes, activities, and even cultures [ 27 ]. Therefore, it is important to assess whether the adoption of digital technologies contributes to health system objectives in an optimal manner, and this assessment should be carried out at the level of health services but not at the level of digital transformation [ 28 ].
The most prominent limitation of our study is that it was conducted only in Shanghai, China. Because of the sound health care system in Shanghai, residents have already received high-quality eye disease screening services before the adoption of the facilitated self-service eye screening pattern. Consequently, residents are bound to demand more from this new pattern. This situation is quite different from that in lower-income regions. Digital technology was adapted in poverty-stricken areas to build an eye care system, but it did not replace the original system that is based on manually delivered services [ 13 ]. Therefore, the framing effect may be weak [ 29 ], and there is little practical value in comparing digital technology and manual services in these regions. Second, our study is an observational study and blind grouping was not practical due to the special characteristics of fundus examination. However, we have attempted to use blind processing whenever possible. For instance, ophthalmologists’ evaluation of image quality was conducted in a blinded manner. Third, the manner in which we inquired about residents’ preferences might affect the results. For example, participants in the exposure group generally have experience with manual screening, but those in the control group may not have had enough experience with facilitated screening despite having been shown a video. This might make the participants in the control group more likely to choose manual screening because the new technology was unfamiliar. Finally, individual-level socioeconomic factors or educational level were not recorded, so we cannot rule out the influence of these factors on residents’ preferences.
In summary, this study confirms that the facilitated self-service fundus disease screening pattern could achieve high service quality. The preference of the residents for this new mode, however, was not ideal. It was difficult to reverse residents’ preference for manual screening in a short period, especially when the original manual service was already excellent. Therefore, the digital transformation of health care must proceed with caution. We suggest that attention be paid to the residents’ individual needs. Although more efficient man-machine collaboration is necessary to help the public understand and accept new technologies, personalized health management solutions based on large language models are required.
Acknowledgments
This study was funded by the Shanghai Public Health Three-Year Action Plan (GWVI-11.1-30, GWVI-11.1-22), Science and Technology Commission of Shanghai Municipality (20DZ1100200 and 23ZR1481000), Shanghai Municipal Health Commission (2022HP61, 2022YQ051, and 20234Y0062), Shanghai First People's Hospital featured research projects (CCTR-2022C08) and Medical Research Program of Hongkou District Health Commission (Hongwei2202-07).
Data Availability
Data are available from the corresponding author upon reasonable request.
Authors' Contributions
SL, YM, and YJ contributed to the conceptualization and design of the study. SL, YM, YJ, YP, TY, and YX collected the data. SL and YM analyzed the data. SL, YM, and YJ drafted the manuscript. WL, YX, JZ, LL, and HZ extensively revised the manuscript. All authors read and approved the final manuscript submitted.
Conflicts of Interest
None declared.
Video of the non-mydriatic fundus camera Kestrel-3100m with the self-service module.
Questions for screening service quality.
- GBD 2019 BlindnessVision Impairment Collaborators, Vision Loss Expert Group of the Global Burden of Disease Study. Causes of blindness and vision impairment in 2020 and trends over 30 years, and prevalence of avoidable blindness in relation to VISION 2020: the Right to Sight: an analysis for the Global Burden of Disease Study. Lancet Glob Health. Feb 2021;9(2):e144-e160. [ FREE Full text ] [ CrossRef ] [ Medline ]
- Marques AP, Ramke J, Cairns J, Butt T, Zhang JH, Muirhead D, et al. Global economic productivity losses from vision impairment and blindness. EClinicalMedicine. May 2021;35:100852. [ FREE Full text ] [ CrossRef ] [ Medline ]
- Jan C, Li S, Kang M, Liu L, Li H, Jin L, et al. Association of visual acuity with educational outcomes: a prospective cohort study. Br J Ophthalmol. Nov 18, 2019;103(11):1666-1671. [ CrossRef ] [ Medline ]
- Chai YX, Gan ATL, Fenwick EK, Sui AY, Tan BKJ, Quek DQY, et al. Relationship between vision impairment and employment. Br J Ophthalmol. Mar 16, 2023;107(3):361-366. [ CrossRef ] [ Medline ]
- Nayeni M, Dang A, Mao AJ, Malvankar-Mehta MS. Quality of life of low vision patients: a systematic review and meta-analysis. Can J Ophthalmol. Jun 2021;56(3):151-157. [ CrossRef ] [ Medline ]
- Wang L, Zhu Z, Scheetz J, He M. Visual impairment and ten-year mortality: the Liwan Eye Study. Eye (Lond). Aug 19, 2021;35(8):2173-2179. [ FREE Full text ] [ CrossRef ] [ Medline ]
- GBD 2019 BlindnessVision Impairment Collaborators, Vision Loss Expert Group of the Global Burden of Disease Study. Trends in prevalence of blindness and distance and near vision impairment over 30 years: an analysis for the Global Burden of Disease Study. Lancet Glob Health. Feb 2021;9(2):e130-e143. [ FREE Full text ] [ CrossRef ] [ Medline ]
- Cheng C, Wang N, Wong TY, Congdon N, He M, Wang YX, et al. Vision Loss Expert Group of the Global Burden of Disease Study. Prevalence and causes of vision loss in East Asia in 2015: magnitude, temporal trends and projections. Br J Ophthalmol. May 28, 2020;104(5):616-622. [ CrossRef ] [ Medline ]
- Li JO, Liu H, Ting DS, Jeon S, Chan RP, Kim JE, et al. Digital technology, tele-medicine and artificial intelligence in ophthalmology: a global perspective. Prog Retin Eye Res. May 2021;82:100900. [ FREE Full text ] [ CrossRef ] [ Medline ]
- Ting DSW, Pasquale LR, Peng L, Campbell JP, Lee AY, Raman R, et al. Artificial intelligence and deep learning in ophthalmology. Br J Ophthalmol. Feb 25, 2019;103(2):167-175. [ FREE Full text ] [ CrossRef ] [ Medline ]
- Xie Y, Nguyen QD, Hamzah H, Lim G, Bellemo V, Gunasekeran DV, et al. Artificial intelligence for teleophthalmology-based diabetic retinopathy screening in a national programme: an economic analysis modelling study. Lancet Digit Health. May 2020;2(5):e240-e249. [ CrossRef ]
- Tang J, Liang Y, O'Neill C, Kee F, Jiang J, Congdon N. Cost-effectiveness and cost-utility of population-based glaucoma screening in China: a decision-analytic Markov model. Lancet Glob Health. Jul 2019;7(7):e968-e978. [ CrossRef ]
- Xiao X, Xue L, Ye L, Li H, He Y. Health care cost and benefits of artificial intelligence-assisted population-based glaucoma screening for the elderly in remote areas of China: a cost-offset analysis. BMC Public Health. Jun 04, 2021;21(1):1065. [ FREE Full text ] [ CrossRef ] [ Medline ]
- Generating Evidence for Artificial Intelligence Based Medical Devices: A Framework for Training Validation and Evaluation. World Health Organization. URL: https://www.who.int/publications/i/item/9789240038462 [accessed 2024-03-27]
- The Assessment List for Trustworthy Artificial Intelligence. URL: https://altai.insight-centre.org/ [accessed 2024-03-27]
- Dietvorst BJ, Bharti S. People reject algorithms in uncertain decision domains because they have diminishing sensitivity to forecasting error. Psychol Sci. Oct 11, 2020;31(10):1302-1314. [ CrossRef ] [ Medline ]
- DeCamp M, Tilburt JC. Why we cannot trust artificial intelligence in medicine. Lancet Digit Health. Dec 2019;1(8):e390. [ CrossRef ]
- Frank D, Elbæk CT, Børsting CK, Mitkidis P, Otterbring T, Borau S. Drivers and social implications of artificial intelligence adoption in healthcare during the COVID-19 pandemic. PLoS One. Nov 22, 2021;16(11):e0259928. [ FREE Full text ] [ CrossRef ] [ Medline ]
- Juravle G, Boudouraki A, Terziyska M, Rezlescu C. Trust in artificial intelligence for medical diagnoses. Prog Brain Res. 2020;253:263-282. [ CrossRef ] [ Medline ]
- Liu X, Faes L, Kale AU, Wagner SK, Fu DJ, Bruynseels A, et al. A comparison of deep learning performance against health-care professionals in detecting diseases from medical imaging: a systematic review and meta-analysis. Lancet Digit Health. Oct 2019;1(6):e271-e297. [ CrossRef ]
- Scanlon PH, Foy C, Malhotra R, Aldington SJ. The influence of age, duration of diabetes, cataract, and pupil size on image quality in digital photographic retinal screening. Diabetes Care. Oct 2005;28(10):2448-2453. [ FREE Full text ] [ CrossRef ] [ Medline ]
- Cen L, Ji J, Lin J, Ju S, Lin H, Li T, et al. Automatic detection of 39 fundus diseases and conditions in retinal photographs using deep neural networks. Nat Commun. Aug 10, 2021;12(1):4828. [ FREE Full text ] [ CrossRef ] [ Medline ]
- Longoni C, Bonezzi A, Morewedge C. Resistance to medical artificial intelligence. J Consum Res. 2019;46:650. [ CrossRef ]
- Huang AS, Hirabayashi K, Barna L, Parikh D, Pasquale LR. Assessment of a Large Language Model's Responses to Questions and Cases About Glaucoma and Retina Management. JAMA Ophthalmol. Feb 22, 2024. [ FREE Full text ] [ CrossRef ] [ Medline ]
- Ploug T, Sundby A, Moeslund TB, Holm S. Population preferences for performance and explainability of artificial intelligence in health care: choice-based conjoint survey. J Med Internet Res. Dec 13, 2021;23(12):e26611. [ FREE Full text ] [ CrossRef ] [ Medline ]
- Young AT, Amara D, Bhattacharya A, Wei ML. Patient and general public attitudes towards clinical artificial intelligence: a mixed methods systematic review. Lancet Digit Health. Sep 2021;3(9):e599-e611. [ CrossRef ]
- Alami H, Gagnon M, Fortin J. Digital health and the challenge of health systems transformation. Mhealth. Aug 08, 2017;3:31-31. [ FREE Full text ] [ CrossRef ] [ Medline ]
- Ricciardi W, Pita Barros P, Bourek A, Brouwer W, Kelsey T, Lehtonen L, et al. Expert Panel on Effective Ways of Investing in Health (EXPH). How to govern the digital transformation of health services. Eur J Public Health. Oct 01, 2019;29(Supplement_3):7-12. [ FREE Full text ] [ CrossRef ] [ Medline ]
- Khan WU, Shachak A, Seto E. Understanding decision-making in the adoption of digital health technology: the role of behavioral economics' prospect theory. J Med Internet Res. Feb 07, 2022;24(2):e32714. [ FREE Full text ] [ CrossRef ] [ Medline ]
Abbreviations
Edited by A Mavragani; submitted 06.01.23; peer-reviewed by B Li, A Bate, CW Pan; comments to author 13.09.23; revised version received 15.10.23; accepted 12.03.24; published 17.04.24.
©Senlin Lin, Yingyan Ma, Yanwei Jiang, Wenwen Li, Yajun Peng, Tao Yu, Yi Xu, Jianfeng Zhu, Lina Lu, Haidong Zou. Originally published in the Journal of Medical Internet Research (https://www.jmir.org), 17.04.2024.
This is an open-access article distributed under the terms of the Creative Commons Attribution License (https://creativecommons.org/licenses/by/4.0/), which permits unrestricted use, distribution, and reproduction in any medium, provided the original work, first published in the Journal of Medical Internet Research, is properly cited. The complete bibliographic information, a link to the original publication on https://www.jmir.org/, as well as this copyright and license information must be included.
Log in using your username and password
- Search More Search for this keyword Advanced search
- Latest content
- Current issue
- For authors
- New editors
- BMJ Journals More You are viewing from: Google Indexer
You are here
- Online First
- Strength, power and aerobic capacity of transgender athletes: a cross-sectional study
- Article Text
- Article info
- Citation Tools
- Rapid Responses
- Article metrics

- http://orcid.org/0000-0001-7412-1188 Blair Hamilton 1 , 2 ,
- http://orcid.org/0009-0005-9553-3081 Andrew Brown 2 ,
- http://orcid.org/0009-0007-0957-5002 Stephanie Montagner-Moraes 2 ,
- http://orcid.org/0000-0001-9483-3262 Cristina Comeras-Chueca 3 ,
- http://orcid.org/0000-0001-8609-2812 Peter G Bush 2 ,
- http://orcid.org/0000-0002-8526-9169 Fergus M Guppy 4 ,
- http://orcid.org/0000-0001-6210-2449 Yannis P Pitsiladis 5 , 6
- 1 School of Sport and Health Sciences , University of Brighton , Brighton , UK
- 2 School of Applied Sciences University , Brighton , UK
- 3 Health Sciences Faculty , Universidad San Jorge , Zaragoza , Spain
- 4 Heriot-Watt University , Edinburgh , UK
- 5 Department of Movement, Human and Health Sciences , University of Rome ‘Foro Italico’ , Rome , Italy
- 6 Department of Sport, Physical Education and Health , Hong Kong Baptist University , Hong Kong , Hong Kong SAR
- Correspondence to Professor Yannis P Pitsiladis, Department of Sport, Physical Education and Health, Hong Kong Baptist University, Hong Kong, Hong Kong SAR; ypitsiladis{at}hkbu.edu.hk
Objective The primary objective of this cross-sectional study was to compare standard laboratory performance metrics of transgender athletes to cisgender athletes.
Methods 19 cisgender men (CM) (mean±SD, age: 37±9 years), 12 transgender men (TM) (age: 34±7 years), 23 transgender women (TW) (age: 34±10 years) and 21 cisgender women (CW) (age: 30±9 years) underwent a series of standard laboratory performance tests, including body composition, lung function, cardiopulmonary exercise testing, strength and lower body power. Haemoglobin concentration in capillary blood and testosterone and oestradiol in serum were also measured.
Results In this cohort of athletes, TW had similar testosterone concentration (TW 0.7±0.5 nmol/L, CW 0.9±0.4 nmol/), higher oestrogen (TW 742.4±801.9 pmol/L, CW 336.0±266.3 pmol/L, p=0.045), higher absolute handgrip strength (TW 40.7±6.8 kg, CW 34.2±3.7 kg, p=0.01), lower forced expiratory volume in 1 s:forced vital capacity ratio (TW 0.83±0.07, CW 0.88±0.04, p=0.04), lower relative jump height (TW 0.7±0.2 cm/kg; CW 1.0±0.2 cm/kg, p<0.001) and lower relative V̇O 2 max (TW 45.1±13.3 mL/kg/min/, CW 54.1±6.0 mL/kg/min, p<0.001) compared with CW athletes. TM had similar testosterone concentration (TM 20.5±5.8 nmol/L, CM 24.8±12.3 nmol/L), lower absolute hand grip strength (TM 38.8±7.5 kg, CM 45.7±6.9 kg, p = 0.03) and lower absolute V̇O 2 max (TM 3635±644 mL/min, CM 4467±641 mL/min p = 0.002) than CM.
Conclusion While longitudinal transitioning studies of transgender athletes are urgently needed, these results should caution against precautionary bans and sport eligibility exclusions that are not based on sport-specific (or sport-relevant) research.
Data availability statement
Data are available on reasonable request.
This is an open access article distributed in accordance with the Creative Commons Attribution Non Commercial (CC BY-NC 4.0) license, which permits others to distribute, remix, adapt, build upon this work non-commercially, and license their derivative works on different terms, provided the original work is properly cited, appropriate credit is given, any changes made indicated, and the use is non-commercial. See: http://creativecommons.org/licenses/by-nc/4.0/ .
https://doi.org/10.1136/bjsports-2023-108029
Statistics from Altmetric.com
Request permissions.
If you wish to reuse any or all of this article please use the link below which will take you to the Copyright Clearance Center’s RightsLink service. You will be able to get a quick price and instant permission to reuse the content in many different ways.
WHAT IS ALREADY KNOWN ON THIS TOPIC
There is currently a lack of laboratory data on strength, power and V̇O 2 max from transgender athlete populations.
WHAT THIS STUDY ADDS
This research compares laboratory measures of strength, power and V̇O 2 max of transgender male and female athletes to their cisgender counterparts.
Transgender women athletes demonstrated lower performance than cisgender women in the metrics of forced expiratory volume in 1 s:forced vital capacity ratio, jump height and relative V̇O 2 max.
Transgender women athletes demonstrated higher absolute handgrip strength than cisgender women, with no difference found relative to fat-free mass or hand size.
HOW THIS STUDY MIGHT AFFECT RESEARCH, PRACTICE, OR POLICY
This study provides sport governing bodies with laboratory-based performance-related data from transgender athletes.
Longitudinal studies are needed to confirm if these results are a direct result of gender affirmation hormone therapy.
Sports-specific studies are necessary to inform policy-making.
Introduction
Transgender athletes can experience conflict between the gender that they were assigned and their experienced gender. 1 The question of integrating transgender athletes into their affirmed gender categories is becoming more prominent, with sports’ governing bodies using varied approaches, from bans on transgender women in the female category 2 requiring the reduction of testosterone in the female category for some time 3 to self-identification into the athletes chosen category. 4
As part of gender affirmation hormone therapy (GAHT), some transgender women undergo testosterone suppression (target ≤1.8 nmol/L 5 ) coupled with oestrogen supplementation (target 400–600 pmol/L 5 ), while some transgender men undergo testosterone supplementation (National Health Service (NHS, UK) target 15–20 nmol/L, 6 Endocrine Society Target 11–34.7 nmol/L 7 ). Testosterone is known to impact sporting performances, with differences in circulating testosterone concentration between cisgender men (CM) and women proposed to explain most of the laboratory-measured differences in sports performance. 8 9 GAHT of transgender men and women alters the body composition of transgender athletes via testosterone-mediated effects on fat-free mass 8 and oestrogens on subcutaneous fat distribution 9 and maintenance of muscle mass. 10 An often-held assumption against transgender women athletes competing in the female category of sport is that transgender women have benefited from a high testosterone concentration from assigned male-at-birth puberty until the administration of GAHT that cannot be mitigated 11 and that cisgender women competitors are unable to achieve similar benefits naturally. 12 To date, this assumption has yet to be tested and confirmed in transgender athlete cohorts. The low serum testosterone concentrations from an assigned female-at-birth puberty would hypothetically not give transgender men the competitive advantages of higher testosterone concentrations over CM, and this viewpoint is reflected in the current inclusion sports policies for transgender men. 2
Lab-derived data on a cohort of transgender athletes, as requested in article 6.1b of the International Olympic Committee Framework On Fairness, Inclusion And Non-Discrimination based on Gender Identity and Sex Variations, 4 must be generated to better inform a decision-making process. 13 Therefore, the primary aim of this study was to compare cardiorespiratory fitness, strength and body composition of transgender women and men athletes to that of matched cisgender cohorts.
Study design
This cross-sectional study involved a single visit to the laboratory at the School of Applied Sciences, University of Brighton, UK. Each participant arrived at ~9:00 hours. after an overnight fast and departed from testing at ~15:00 hours. The complete study design can be found in the study protocol, available as a preprint. 14
Recruitment
Following ethical approval (ref: 9496), 75 (19 CM, 12 transgender men, 23 transgender women and 21 cisgender women) participants were recruited through social media advertising on Meta Platforms (Facebook and Instagram, Meta Platforms, California, USA) and X (Twitter, California, USA). Following the initial response, all participants were provided with the participant information sheet by email at least 7 days before being invited to travel to the laboratory, with further oral information about the study procedures and written informed consent provided on their visit to the laboratory.
Participants and eligibility criteria
Participants were required to participate in competitive sports or undergo physical training at least three times per week. Following written consent, participants were asked to record their last four training sessions and self-rate their training intensity for each session on a scale of 1–10 (10=maximum intensity). The mean of the four sessions was recorded to represent the athletes’ training intensity. The transgender athletes must have completed ≥1 year of GAHT, voluntarily disclosed during consent and verified during blood test analysis. The full inclusion/exclusion criteria can be found in the study protocol, available as a preprint. 14 Two cisgender women and one transgender man could not provide blood samples and were consequently excluded from all analyses as their endocrine profiles could not be verified. Furthermore, two transgender women and one cisgender woman were excluded from all analyses due to testosterone concentrations exceeding recommended female testosterone concentrations (2.7 nmol/L 15 ).
Laboratory assessments
Blood sampling and analysis.
Prior to venous blood sampling, haemoglobin concentration ((Hb)) was sampled via the third drop of a Unistik 3 Comfort lancet (Owen Mumford, Woodstock, UK) finger prick capillary blood sample analysed immediately using a HemoCue 201+ (HemoCue AB, Ängelholm, Sweden). Capillary blood was used for (Hb) analysis for practical reasons such as ease of use. It is important to note that the HemoCue 201+used in the present study is expected to yield higher (Hb) values than venous blood. 16 After capillary sampling, one 10 mL whole venous blood sample was collected from an antecubital vein into a BD serum tube (Becton, Dickinson and Company, Wokingham, Berkshire, UK) for serum extraction. Once collected, the tubes were left at room temperature (18°C±5°C) for 1 hour and then stored in a fridge (3°C±2°C) for up to 4 hours before being centrifuged (PK 120 centrifuge, ALC, Winchester, Virginia, USA) using a T515 rotor at 1300G for 10 min at 4°C, before storage at −80°C until analysis. Before analysis, the samples were stored between −25°C and −15°C, thawed at room temp until liquid, vortexing to remix samples, centrifuged at 2876G for 8 min to remove any precipitant and then analysed for participant’s testosterone and oestradiol concentrations on an immunoassay analyser (Roche Cobas 8000 e801, Roche Diagnostics, Burgess Hill, UK).
Body composition and bone mass
Participants’ body mass was measured (OMRON Healthcare, Kyoto, Japan) while participants were lightly dressed, representing clothed body mass. Body composition and bone mass were measured by DXA (Horizon W, Hologic, Massachusetts, USA). Each participant underwent a whole-body, a proximal-femur and a lumbar spine scan. The participant was asked to lie on the scan bed, and the first author (BH) performed all participant placement and scanning for the three scans. Due to inbuilt assumptions of body fat percentage for the head and scanning bed area imitations, whole-body less head data are reported for the whole-body scan. Body mass index (BMI), Fat Mass Index (FMI) and Fat-Free Mass Index (FFMI) were calculated by taking the appropriate mass value and dividing it by height (m 2 ).
Lung function
Lung function was measured using a Vitalograph Alpha spirometer (Vitalograph, Kansas, USA) with an antibacterial filter and a nose clip on the bridge of the participant’s nose. Each participant was asked to perform the flow-volume-loop spirometry to test forced vital capacity (FVC), forced expiratory volume in 1 s (FEV 1 ) and peak expiratory flow. The test was repeated until a trend of declining performance occurred. The highest numeric value for each metric obtained during a test with the correct procedure was then recorded. The FEV1:FVC ratio was used to assess the presence of obstructed lung function.
Strength was measured using a handgrip dynamometer (TAKEI 5401, TAKEI Scientific Instruments, Japan). The participants’ hand sizes were also measured around the metacarpophalangeal joints of both hands prior to testing. Each hand was tested three times in sequential order of left-right to allow each hand to rest; the mean scores were taken from the three attempts for each hand.
Lower body power
Lower body power was measured with the countermovement jump on a JUM001 Jump Mat (Probotics, Alabama, USA). During the test, if the participant went beyond 45° of countermovement or the hands came off the hips, the test would be declared void for that attempt. After recording three legitimate attempts, the mean scores were recorded.
Cardiopulmonary exercise testing
Cardiopulmonary exercise testing was performed using a 95T Engage Treadmill ergometer (Life Fitness, Illinois, USA) and a COSMED QUARK (COSMED, Rome, Italy). All V̇O 2 max tests were conducted and analysed by the first author (BH) to avoid interinvestigator variability. 17 The ramp protocol of Badawy and Muaidi treadmill V̇O 2 max testing 18 was used for each V̇O 2 max test, involving gradual increases in speed every 3 min at a 1% incline. One cisgender man and two cisgender women were excluded from the analysis as they did not meet the required respiratory exchange ratio of ≥1.1 to classify the test as maximal (cisgender men (CM), n=18, transgender men (TM), n=11; cisgender women (CW) n=16; transgender women (TW), n=21).
Statistical analysis
Data meeting the assumptions of normality and homogeneity of variance were analysed using a one-way analysis of variance along with Bonferroni post hoc corrections for pairwise comparisons. Data not meeting the parametric assumptions were compared using a Kruskal-Wallis ANOVA with Dwass-Steel-Critchlow-Fligner post hoc test for multiple comparisons, with an alpha level of 0.05 for both types of analysis. Statistical analysis and presentation are consistent with the checklist for statistical assessment of medical papers statement 19 found in online supplemental files 1–3 at Hamilton et al , The Strength, Power and Aerobic Capacity of Transgender Athletes: A Cross-Sectional Study (Internet). OSF; 2023. Available from: osf.io/a684b.
Supplemental material
Equity, diversity and inclusion statement.
The author group consists of early (n=3) and senior researchers (n=3) from different disciplines and universities (n=3). Two authors are members of a marginalised community; the lead early-career author is a transgender woman, and one of the junior authors is a woman from the global south. Our study population included male and female transgender athletes from within the UK participating in competitive sports in comparison with cisgender male and female athletes participating in competitive sports; thus, findings may not be generalisable to global athlete populations.
Participant characteristics
Our investigation encompassed a diverse cohort of athletes, with endurance sports representing 36% of the athlete cohort, team sports representing 26% and power sports representing 38%. No cisgender or transgender athletes were competing at the national or international level. No significant differences were found in age (F (3–66) =1.9, p=0.14), training intensity score (χ 2 (3) =1.2, p=0.76) or length of GAHT between transgender men and transgender women (F (1–32) =0.5, p=0.48, table 1 ).
- View inline
Significant differences were found in height (F (3–66) =21.3, p<0.001), with CM being taller than transgender men (t (66) =3.8, p=0.002, table 1 ). Transgender women were also taller than transgender men (t (66) =3.3, p=0.01) and cisgender women (t (66) =6.5, p<0.001, table 1 ).
Significant differences were found in clothed mass (F (3–66) =10.6, p<0.001), with transgender women found to be heavier than cisgender women (t (66) =5.6, p<0.001, table 1 ).
BMI was also significantly different between the groups in this Study (F (3–66) =3.6, p=0.02). Transgender women athletes demonstrated higher BMI than cisgender women (t (66) =2.9, p=0.03, table 1 ), with no further differences observed.
Blood measures
There was a significant gender effect on testosterone concentration (F (3–66) =80.6, p<0.001). CM (20.5±5.8 nmol/L) exhibited significantly higher total testosterone concentration than transgender women (0.7±0.5 nmol/L, t (66) = 11.1, p<0.001, figure 1A ). Transgender men (24.8±12.3 nmol/L) had elevated total testosterone concentration compared with transgender women (t (66) =11.3) and cisgender women (0.9±0.4 nmol/L, t (66) =10.9, both p<0.001, figure 1A ). There was also a significant gender effect on oestradiol concentration (F (3−66) =7.6, p<0.001), with transgender women (742.4±801.9 pmol/L) showing higher oestradiol concentration than CM (104.3±24.8 pmol/L, t (66) =4.4 p<0.001), cisgender women (336.0±266.3 pmol/L, t (66) =2.7, p=0.045) and transgender men (150.2±59.4 pmol/L, t (66) =3.4, p=0.01, figure 1B ).
- Download figure
- Open in new tab
- Download powerpoint
Blood measures. (A) testosterone; (B) oestradiol; (C) haemoglobin. *p<0.05, **p<0.01, ***p<0.001, ****p<0.0001. CM, cisgender men; CW, cisgender women; TM, transgender men; TW, transgender women.
Transgender women’s total testosterone concentration (0.7±0.5 nmol/L) falls within the recommendations for GAHT of ≤1.8 nmol/L, 5 and oestradiol concentrations (742.4±801.9 pmol/L) exceed the target of 400–600 pmol/L 5 for GAHT. Transgender men’s testosterone concentration (24.8±12.3 nmol/L) exceeds the NHS target of 15–20 nmol/L 6 for GAHT, although not the Endocrine Society target of 11–34.7 nmol/L. 7
Differences were reported in (Hb) concentration (F (3–66) =3.3, p=0.03), although a post hoc Bonferroni analysis showed no differences between the various groups (CM 142.8±12.5 g/L; transgender men, 143.3±19.5 g/L; transgender women, 131.3±14.2 g/L; cisgender women, 133.3±12.7 g/L; figure 1C ).
DXA assessment
There was a significant gender effect on percentage fat mass (F (3–66) =6.6, p<0.001), with CM having a lower percentage fat mass than transgender women (t (66) =−4.4, p<0.001, table 2 ), with no other differences observed. A significant gender effect was also found on absolute fat mass (F (3–66) =6.6, p<0.001), with transgender women having more absolute fat mass than CM (t (66) =3.8, p=0.002, table 2 ) and cisgender women (t (66) =3.9, p=0.002, table 2 ). FMI measures revealed a gender effect (F (3–66) =5.2, p=0.003), with transgender women found to have a higher FMI than CM (t (66) =3.7, p=0.002, table 2 ) and cisgender women (t (66) =2.8, p=0.04, table 2 ). Android to gynoid ratio analysis (F (3–66) =10.7, p<0.001) revealed cisgender women had a lower ratio than transgender men (t (66) =−2.9, p=0.03, table 2 ), and transgender women (t (66) =−4.0, p=0.001, table 2 ).
Body composition, BMD data, handgrip strength, lower anaerobic power and cardiopulmonary exercise testing
Fat-free mass
There was a significant gender effect on absolute fat-free mass (F (3–66) =24.6, p<0.001), with CM having significantly more absolute fat-free mass than transgender men (t (66) =3.5, p=0.01, table 2 ). Cisgender women had less absolute fat-free mass than transgender men (t (66) =−3.5, p=0.01, table 2 ) and transgender women (t (66) =−6.6, p<0.001, table 2 ). No gender-based effects were found when comparing transgender women athletes to cisgender women athletes, or transgender men athletes to CM athletes in the measures of FFMI (F (3–66) =3.7, p=0.02, table 2 ), percentage of fat-free mass (F (3–66) =2.4, p=0.08, table 2 ) or appendicular FFMI (F (3–66) =5.1, p=0.003, table 2 ).
Bone mineral density
No differences in whole-body bone mineral density (BMD) (F (3–66) =4.6, p=0.01), femoral neck BMD (F (3–66) =1.0, p=0.39, table 2 ), total proximal femur BMD (F (3–66) =1.5, p=0.22, table 2 ) or total lumbar spine BMD (F (3–66) =0.4, p=0.78, table 2 ) were found between transgender athletes and cisgender athletes ( table 2 ).
Lung function data for all groups can be found in table 2 . FEV 1 had an effect of gender (F (3–66) =14.7, p<0.001), with CM having greater FEV 1 than transgender men (t (66) = 4.5, p<0.001, figure 2A ). Transgender women also had greater FEV 1 than cisgender women (t (66) =4.2, p<0.001, figure 2A ) and transgender men (t (66) =2.9, p=0.03, figure 2A ). There was a similar effect of gender on FVC (F (3–66) =21.6, p<0.001, figure 2B ), with CM having greater FVC than transgender men (t (66) =5.2, p<0.001, figure 2B ). Transgender women also had greater FVC than cisgender women (t (66) =5.6, p<0.001, figure 2B ) and transgender men (t (66) =4.0, p=0.001, figure 2B ). A significant effect of gender was also seen on the FEV 1 :FVC ratio (F (3–66) =3.3, p=0.03 figure 2C ), with transgender women showing a reduced FEV 1 :FVC ratio compared with cisgender women (t (66) =−2.8, p=0.04, figure 2C ) with no differences observed in transgender or CM. Peak expiratory flow (F (3–66) =5.5, p=0.002) had a minor gender-based effect, with cisgender women having lower peak expiratory flow than transgender women (t (66) −3.0, p=0.02, figure 2D ).
Lung function measures. (A) Forced rxpiratory volume in 1 s (FEV 1 ); (B) forced vital capacity (FVC) (C) modified Tiffeneau-Pinelli Index (FEV 1 :FVC); (D) peak expiratory flow (PEF). *p<0.05, **p<0.01, ***p<0.001, ****p<0.0001. CM, cisgender men; CW, cisgender women; TM, transgender men; TW, transgender women.
Handgrip strength
Handgrip strength data can be found in table 2 . Absolute right handgrip strength was significantly different between the groups (F (3–66) =10.5, p<0.001), with CM having greater absolute right handgrip strength than transgender men (t (66) =2.9, p=0.03, figure 3B ). Transgender women also had greater absolute right handgrip strength than cisgender women (t (66) =3.2, p=0.01, figure 3B ). Absolute left handgrip was significantly different between the groups (F (3–66) =8.6, p<0.001). However, no differences were found between transgender and cisgender athletes ( figure 3A ). There was no effect on the right (F (3–66) =0.8, p=0.53, figure 3F ) or left-hand grip strength (F (3–66) =1.0, p=0.39, figure 3E ) relative to fat-free mass, nor was there any gender effect on the right (F (3–66) =1.6, p=0.20, figure 3D ) or left-hand grip-strength (F (3–66) =2.1, p=0.11) relative to hand size.
Absolute and relative handgrip strength (GS) measures. (A) Absolute strength (right hand); B) Absolute strength (left hand) (C) relative strength to hand size (right hand); (D) relative strength to hand size (left hand); (E) relative strength to fat-free mass (FFM) (right hand); (F) relative strength to fat-free mass (left hand). *p<0.05, ***p<0.001, ****p<0.0001. CM, cisgender men; CW, cisgender women; TM, transgender men; TW, transgender women.
Lower body anaerobic power
Lower body anaerobic power data are shown in table 2 . Gender had a significant effect on absolute countermovement jump height (F (3–66) =7.2, p<0.001), with CM having greater absolute jump height than transgender women (t (66) =4.5, p<0.001, figure 4A ). A significant effect of gender was found in countermovement jump height relative to fat-free mass (F (3–66) =10.1, p<0.001, figure 4B ), with transgender women found to have lower countermovement jump height relative to fat-free mass than both cisgender women (t (66) =−5.3, p<0.001) and transgender men (t (66) =–3.2, p=0.01, figure 4B ).
Absolute and relative anaerobic power measures. (A) Absolute CMJ height; B) Relative CMJ height to fat-free mass (FFM); (C) absolute peak power; (D) relative peak power to FFM; (E) absolute average power; (F) relative average power to FFM. *p<0.05, **p<0.01, ***p<0.001, ****p<0.0001. CM, cisgender men; CMJ, Counter Movement Jump; CW, cisgender women; TM, transgender men; TW, transgender women.
There was a significant difference in absolute peak power (F (3–66) =8.7, p<0.001), with cisgender women having reduced peak power compared with transgender men (t (66) =−3.3, p=0.01) and transgender women (t (66) =−3.6, p=0.004, figure 4C ). Peak power relative to fat-free mass had a more negligible gender effect (F (3–66) =4.2, p=0.01), with no difference in peak power relative to fat-free mass found between transgender and cisgender athletes ( figure 4D ).
There was a significant gender effect of absolute average power (F (3–66) =5.9, p=0.001), with cisgender women having reduced absolute average power compared with transgender men (t (66) =–3.1, p=0.02, figure 4E ). There was no effect of gender on average power relative to fat-free mass (F (3–66) =2.6, p=0.06, figure 4F ).
Cardiopulmonary exercise testing data are shown in table 2 . A significant effect of gender was found on absolute V̇O 2 max (F (3–62) =14.1, p<0.001) with CM having greater absolute V̇O 2 max than transgender men (t (66) =3.8, p=0.002, figure 5A ) and transgender women (t (66) =4.3, p<0.001, figure 5A ). Relative V̇O 2 max to body mass also showed a significant gender effect (F (3–62) =9.8, p<0.001) with transgender women having lower relative V̇O 2 max than CM (t (66) =–5.3, p<0.001, figure 5B ) and cisgender women (t (66) =−3.3, p=0.01, figure 5B ). No significant gender effect was found on the measure of V̇O 2 max relative to fat-free mass (F (3–62) =2.0, p=0.12).
Absolute and relative cardiopulmonary exercise testing measures. (A) Absolute V̇O 2 max; (B) relative V̇O 2 max to body weight; (C) absolute anaerobic threshold (AT); (D) anaerobic threshold (%V̇O 2 max); (E) relative anaerobic threshold relative to body mass; (F) AT relative to at-free mass (FFM). *p<0.05, **p<0.01, ***p<0.001, ****p<0.0001. CM, cisgender men; CW, cisgender women; TM, transgender men; TW, transgender women.
Gender affected the absolute anaerobic threshold (F (3–62) =14.1, p<0.001), with cisgender (3924±628 mL/min) men having a higher absolute anaerobic threshold than transgender men (3089±546 mL/min, t (66) =4.2, p<0.001, figure 5C ), and transgender women (3122±438 mL/min, t (66) =4.8, p<0.001, figure 5C ). No significant gender effect was found on the measure of anaerobic threshold as a percentage of V̇O 2 max (F (3–62) =0.8, p=0.51, figure 5D ). A gender effect was also seen on the anaerobic threshold relative to body mass (F (3–62) =10.7, p<0.001), with transgender women (38.3±6.6 mL/kg/min) showing a lower relative anaerobic threshold than both cisgender women (47.2±6.1 mL/kg/min, t (66) =–3.3, p=0.01, figure 5E ) and CM (52.2±9.5 mL/kg/min, t (66) =−5.4, p<0.001, figure 5E ). CM also showed a higher relative anaerobic threshold than transgender men (42.1±9.9 mL/kg/min, t (66) =3.3, p=0.01, figure 5E ). Anaerobic threshold relative to fat-free mass also had a small gender effect (F (3–62) =3.2, p=0.03), with transgender women (60.8±12.2 mL/kg FFM /min) having a lower anaerobic threshold relative to fat-free mass than CM (71.2±13.3 mL/kg FFM /min, t (66) =−2.8, p=0.045, figure 5F ).
The results presented in this study provide valuable insights into laboratory-based performance-related metrics of gender-diverse athletes participating in competitive sports. Given the primary aim of GAHT, 20 it is noteworthy that although this study is cross-sectional in design, transgender women’s oestradiol was higher than that of cisgender women ( figure 1B ). The presence of outliers affecting transgender women’s oestrogen concentration ( figure 1B ) is evident. This underscores that transgender women in this cohort of athletes exhibit a distinct endocrine profile from CM and share a similar endocrine profile with cisgender women, whom many transgender women aim to integrate into a sporting category. One of the most noticeable disparities between gender groups was in height and mass ( table 1 ), with CM and transgender women being taller and heavier than their cisgender and transgender counterparts ( table 1 ). Body composition measures (fat mass % and fat-free mass %, table 2 ) between transgender women and cisgender women found no difference. However, transgender women are, on average as a cohort taller and heavier.
In this cohort, the average difference in haemoglobin (Hb) between cisgender women and CM athletes was 7% ( figure 1C ), lower than previously described (12% 8 ). Notably, the (Hb) profiles of all the athlete groups were not significantly different, concurring with earlier research 21 and contradicting research in sedentary populations. 22 (Hb) is crucial in O 2 transport 23 and vital for endurance sports performance, 24 with O 2 delivery to the tissues a limiting factor in V̇O 2 max attainment. 25 The lack of differences in (Hb) is consistent with the lack of observed difference in absolute V̇O 2 max between transgender women, transgender men and cisgender women in this cohort. However, as cardiac output, the most crucial variable influencing V̇O 2 max 25 was not assessed in the present study, a more comprehensive mechanistic explanation for the similar maximal aerobic capacity between groups cannot be provided.
No differences in BMD were observed between transgender and cisgender women athletes in this study ( table 2 ), despite prior research hypothesising that transgender women athletes have a significant BMD advantage over cisgender women. 11 The sample size for each gender was n<30 participants and may be insufficient to characterise BMD differences reliably. Exercise has been shown to have a protective effect on BMD in CM 26 and CW, 27 and our results suggest a protective effect of exercise in transgender women, given that there is evidence of low BMD in transgender women with low weekly sports activity. 28 Nevertheless, the results suggest the complexity of bone health in athlete populations and the need for a more comprehensive assessment to understand the long-term impact of GAHT on transgender athletes’ BMD.
The differences observed in body composition in this population ( table 2 ) indirectly show the potential role of androgens in body composition, owing to the role of oestradiol in fat accumulation 29 and transgender women’s oestradiol concentrations ( figure 1B ) and fat mass ( table 2 ) being greater than all other groups. Body composition differences may have implications for sports that prioritise exercise economy, 30 defined as the average V̇O 2 relative to body mass between submaximal intensities, 31 as athletes with a higher fat mass percentage will present with a lower exercise economy owing to the increased O 2 cost of exercise. 32 The android-to-gynoid ratio analysis ( table 2 ) suggests that hormone therapy ( figure 1A,B ) influences differences in fat distribution patterns. However, fat distribution patterns of the present transgender female athlete cohort ( table 2 ) do not reach ratios previously reported in cisgender female populations (0.8). 33 Understanding these variations is essential for evaluating performance in sports where body composition is a determining factor, for example, weightlifting or boxing.
Cisgender women had lower absolute fat-free mass than transgender men and transgender women ( table 2 ). When analysing absolute fat and fat-free mass data ( table 2 ), these results can be affected by sample size and/or athlete diversity limitations. A purposefully designed future study with height-matched and sport-matched cisgender and transgender female athletes is crucial to understanding differences in these parameters, as they are influenced by height disparities ( table 1 ) and variations in sampled sports.
FVC, FEV 1 and FEV 1 :FVC ratio are higher in athletes than in the normal sedentary control individuals, 34 and there is no difference in all three metrics between aerobic athletes and anaerobic athletes. 35 Therefore, the lung function differences observed in figure 2A,B may be attributed to factors such as skeletal size benefiting lung capacity and function, 36 with transgender women’s FVC results ( figure 2B ) suggesting gender-affirming hormone care did not impact changing lung volumes owing to the GAHTs lack of effect on skeletal stature. 11 Transgender women showed a significantly reduced FEV 1 :FVC ratio compared with cisgender women ( figure 2C ). The FEV 1 :FVC ratio has been used as a screening index for identifying obstructive lung conditions globally, 37 as a lower FEV 1 owing to obstruction of air escaping from the lungs will reduce the FEV 1 :FVC ratio. Transgender women’s results ( figure 2C ) suggest obstructed airflow in the lungs 38 when compared with cisgender women. However, this observation of transgender women is unlikely to be pathological (<0.70), 39 as seen in chronic obstructive pulmonary disease.
Nevertheless, this reduced airflow could potentially lead to exercise-induced dyspnoea, resulting in performance limitations 40 in comparison to cisgender women. When comparing both the CM and transgender women athletes’ groups with identical heights (1.8 m, table 1 ), while both groups exhibit similar FVC, transgender women demonstrate a lower FEV 1 , leading to a reduced FEV 1 :FVC ratio compared with CM, although not significant. If there were a significant difference between CM and transgender women, our preliminary hypothesis would have attributed this divergence to testosterone suppression in transgender women. However, comparing transgender women to cisgender women who do not share similar height and or exhibit a comparable FVC, the observed differences become more complex to interpret. The possibility arises that factors beyond hormonal influences, such as varying levels of aerobic training, may contribute to the significant difference found in the FEV 1 :FVC ratio between transgender women and cisgender women. Further longitudinal investigation is required to elucidate if the causation underlying these pulmonary function disparities is indeed testosterone suppression.
Strength results in figure 3 disagree with previous literature in a non-athlete transgender cohort using the same methodology that showed transgender women and CM had significantly different absolute and relative hand grip strength. 41 Our results showed no differences in absolute strength between transgender women and CM and no difference in relative handgrip between any of the groups in this study ( figure 3 ). These results highlight the differences between athlete and sedentary populations. However, the results relative to hand size also concur with the notion that greater handgrip strength is caused by greater hand size, 42 as there were no differences in results between the four groups when normalised for hand size ( figure 3C,D ). Therefore, investigations with more accurate measures of strength are warranted in transgender athletes.
Transgender women presented lower absolute jump height than CM and lower relative jump height, normalised for fat-free mass, than transgender men and cisgender women ( figure 4 ). These results in this study cohort suggest that transgender women lack lower body anaerobic power compared with the other groups. Transgender women’s higher absolute peak power than cisgender women ( figure 4C ), coupled with higher fat mass potentially driven by higher oestradiol concentrations ( figure 1B ), suggest that transgender women had more inertia to overcome during the explosive phase of the countermovement jump, which may lead to decreased performance. However, when normalised for fat-free mass ( figure 4D ), transgender women’s peak power was lower than that of cisgender women, showing that this cohort also lacks peak power relatively, indicating that the higher fat mass may not be the primary contributing factor. Further investigations are warranted to find the causation of this poor lower anaerobic power performance in transgender women.
The lack of differences in anaerobic threshold (%V̇O 2 max, figure 5D ) suggests that the athletes in this study had a similar fitness status, which is an essential underlying finding given that CM showed greater absolute V̇O 2 max than all groups ( figure 5A ), with no differences between transgender women and cisgender women found, and transgender women exhibited lower relative V̇O 2 max compared with both CM and women ( figure 5B ). In this cohort, the finding of no statistical difference in absolute V̇O 2 max between transgender women and cisgender women contrasts the idea that transgender women’s bigger lung size ( table 2 ) is an inherent respiratory function advantage over cisgender women. 11 Both the absolute and relative V̇O 2 max differences between groups contradict one previous study in non-athlete transgender populations that found transgender women had higher absolute V̇O 2 peak and no difference in relative V̇O 2 peak compared with cisgender women. 41 This contradictory finding further highlights population differences between non-athlete and athlete cohorts while also contradicting literature hypothesising that there would be a baseline gap in aerobic capacity between transgender women and cisgender women. 11 The results in this athlete cohort warrant further research to elucidate the mechanisms behind this deviation, as they may be metabolic, as transgender women also exhibited a lower relative anaerobic threshold ( figure 5E ). The findings in table 2 reveal notable disparities in fat mass, fat-free mass, laboratory sports performance measures and hand-grip strength measures between cisgender male and transgender female athletes. These differences underscore the inadequacy of using cisgender male athletes as proxies for transgender women athletes. Therefore, based on these limited findings, we recommend that transgender women athletes be evaluated as their own demographic group, in accordance with the principles outlined in Article 6.1b of the International Olympic Committee Framework on Fairness, Inclusion and Non-Discrimination based on Gender Identity and Sex Variations. 4
Study limitations
The limitations of this study primarily relate to its cross-sectional design, making it challenging to establish causation or examine if the performance of athletes changes as a result of undergoing GAHT. Longitudinal studies are needed to examine how GAHT, and other factors impact athletes’ physiology and performance over time. Additionally, the composition of the study cohort may not fully represent the diversity of athletes in elite sports from worldwide populations. Athletes from various sporting disciplines and performance levels were included, and the athlete training intensity was self-reported. Therefore, the results may suffer from selection and recall bias. 43 The results may not apply to all levels or ages of athletes, specifically as this research did not include any adolescent athletes competing at the national or international level. The athletes participating in the present study represented a variety of different sports, and this would have undoubtedly impacted the results of the study as different sports stress different training and sports modalities. Exercise type, intensity and duration all have an impact on physiological responses and overall laboratory performance metrics. 44 The subgroups of sports that emerged were also too dissimilar to allow meaningful subgroup analysis. The complexity and difficulty of this area of activity means that while this study provides a starting point for understanding the complex physiology in GAHT and athletic performance, this study does not provide evidence that is sufficient to influence policy for either inclusion or exclusion. However, this is the first study to assess laboratory-based measures of performance in transgender athletes, and this opens up interesting avenues for replication and extension into the longitudinal effects of GAHT on athletic performance.
Future research should include more extensive and diverse samples to enhance the generalisability of findings or smaller, more specific cohorts to hone in on a particular sports discipline. However, such studies may be complex due to the low numbers of transgender athletes. The recruitment method of this study also provided a limitation as social media advertising was used rather than recruitment from a clinical provider. Social media recruitment leaves this study open to sample bias as social media advertising, although great for recruiting hard-to-reach participants for observational studies, 44 45 does not represent a clinical population in 86% of comparisons. 44 As the participants were not recruited from a clinic, this also means that the gender-affirming treatment of the transgender athletes was not controlled. For example, different testosterone suppression methods have different efficacies, 46 and future studies should consider differences in the prescribed GAHT to participants. Lastly, the participants were not screened by a clinician before participation, and any medical conditions were self-reported in the physical activity readiness questionnaire (PAR-Q). This method of medical reporting leaves the data open to self-reporting bias, which can mislead descriptive statistics and causal inferences 47 as participants’ cognitive processes, such as social desirability, can alter participants’ responses. 48 Therefore, it is recommended to use a clinic to screen and recruit participants to avoid such bias in a longitudinal study of transgender athlete sports performance.
Conclusions
This research compares transgender male and transgender female athletes to their cisgender counterparts. Compared with cisgender women, transgender women have decreased lung function, increasing their work in breathing. Regardless of fat-free mass distribution, transgender women performed worse on the countermovement jump than cisgender women and CM. Although transgender women have comparable absolute V̇O 2 max values to cisgender women, when normalised for body weight, transgender women’s cardiovascular fitness is lower than CM and women. Therefore, this research shows the potential complexity of transgender athlete physiology and its effects on the laboratory measures of physical performance. A long-term longitudinal study is needed to confirm whether these findings are directly related to gender-affirming hormone therapy owing to the study’s shortcomings, particularly its cross-sectional design and limited sample size, which make confirming the causal effect of gender-affirmative care on sports performance problematic.
Ethics statements
Patient consent for publication.
Not applicable.
Ethics approval
This study involves human participants and ethical approval for this study has been granted by the School of Applied Sciences Research Ethics Committee of the University of Brighton, Brighton, UK (Ref: 9496). Participants gave informed consent to participate in the study before taking part.
Acknowledgments
We thank Associate Professor Ada Cheung of the Department of Medicine (Austin Health) at the University of Melbourne, Australia for her valuable review of this work prior to publication.
- Arcelus J ,
- Bouman WP , et al
- World Aquatics
- USA Gymnastics
- International Olympic Committee
- National Health Service
- Hembree WC ,
- Cohen-Kettenis PT ,
- Gooren L , et al
- Handelsman DJ ,
- Hirschberg AL ,
- Alvero-Cruz JR ,
- Chilibeck P , et al
- Gharahdaghi N ,
- Phillips BE ,
- Szewczyk NJ , et al
- Hilton EN ,
- Lundberg TR
- Hamilton BR ,
- Barrett J , et al
- Hamilton B ,
- Comeras-Chueca C ,
- Bush P , et al
- Leitman SF , et al
- Popović ZB ,
- Badawy MM ,
- Mansournia MA ,
- Collins GS ,
- Nielsen RO , et al
- Malczewska-Lenczowska J ,
- Sitkowski D ,
- Orysiak J , et al
- Roberts TK ,
- French D , et al
- Schmidt W ,
- Bassett DR ,
- Staines KA ,
- Kelley GA , et al
- Hofmeister M
- Rothman MS ,
- Mareschal J ,
- Karsegard VL , et al
- Losnegard T ,
- Schäfer D ,
- V Mendonca G ,
- Imboden MT ,
- Swartz AM , et al
- Rajaure YS ,
- Budhathoki L , et al
- van der Plaat D ,
- Dharmage S , et al
- Min HK , et al
- Schiöler L ,
- Lindberg A , et al
- Anzueto A , et al
- Alvares LAM ,
- Santos MR ,
- Souza FR , et al
- Alahmari KA ,
- Kakaraparthi VN ,
- Reddy RS , et al
- Tripepi G ,
- Dekker FW , et al
- Topolovec-Vranic J ,
- Natarajan K
- Whitaker C ,
- Stevelink S ,
- Leemaqz S ,
- Ooi O , et al
- Mathiowetz N
Supplementary materials
Supplementary data.
This web only file has been produced by the BMJ Publishing Group from an electronic file supplied by the author(s) and has not been edited for content.
- Data supplement 1
- Data supplement 2
- Data supplement 3
X @BlairH_PhD
Contributors BH, FMG and YPP designed the study. Material preparation, reporting and critical revision of the work were performed by BH, PGB, FMG and YPP. Data collection was performed by CC-C, AB, SM-M and BH. BH wrote the first draft of the manuscript, and all authors critically revised subsequent versions until all authors could approve the final manuscript. YPP is the guarantor.
Funding The study has been funded by a research grant awarded by the International Olympic Committee, Lausanne, Switzerland.
Competing interests YPP is a member of the IOC Medical and Scientific Commission, which recently published articles and framework documents on the topic. BH and FMG have recently published articles on the topic on behalf of the International Federation of Sports Medicine (FIMS). All authors declare no further conflict of interest or competing interests.
Patient and public involvement Patients and/or the public were not involved in the design, or conduct, or reporting, or dissemination plans of this research.
Provenance and peer review Not commissioned; externally peer reviewed.
Supplemental material This content has been supplied by the author(s). It has not been vetted by BMJ Publishing Group Limited (BMJ) and may not have been peer-reviewed. Any opinions or recommendations discussed are solely those of the author(s) and are not endorsed by BMJ. BMJ disclaims all liability and responsibility arising from any reliance placed on the content. Where the content includes any translated material, BMJ does not warrant the accuracy and reliability of the translations (including but not limited to local regulations, clinical guidelines, terminology, drug names and drug dosages), and is not responsible for any error and/or omissions arising from translation and adaptation or otherwise.
Read the full text or download the PDF:
Log in using your username and password
- Search More Search for this keyword Advanced search
- Latest content
- Current issue
- For authors
- BMJ Journals More You are viewing from: Google Indexer
You are here
- Online First
- Masculinising and feminising hormone interventions for adolescents experiencing gender dysphoria or incongruence: a systematic review
- Article Text
- Article info
- Citation Tools
- Rapid Responses
- Article metrics

- http://orcid.org/0000-0001-5898-0900 Jo Taylor ,
- Alex Mitchell ,
- Ruth Hall ,
- Trilby Langton ,
- Lorna Fraser ,
- http://orcid.org/0000-0002-0415-3536 Catherine Elizabeth Hewitt
- Department of Health Sciences , University of York , York, YO10 5DD , UK
- Correspondence to Dr Jo Taylor, Department of Health Sciences, University of York, York, YO10 5DD, UK; dohs-gender-research{at}york.ac.uk
Background Clinical guidelines outline the use of hormones for masculinisation/feminisation in adolescents experiencing gender dysphoria or incongruence. Robust evidence concerning risks and benefits is lacking. There is a need to aggregate evidence as research becomes available.
Aim Identify and synthesise studies assessing the outcomes of hormones for masculinisation/feminisation in adolescents experiencing gender dysphoria/incongruence.
Methods Systematic review and narrative synthesis. Database searches (MEDLINE, Embase, CINAHL, PsycINFO, Web of Science) were performed in April 2022, with results assessed independently by two reviewers. An adapted version of the Newcastle-Ottawa Scale for Cohort Studies was used to assess study quality. Moderate- and high-quality studies were synthesised.
Results 12 cohort, 9 cross-sectional and 32 pre–post studies were included (n=53). One cohort study was high-quality. Other studies were moderate (n=33) and low-quality (n=19). Synthesis of high and moderate-quality studies showed consistent evidence demonstrating induction of puberty, although with varying feminising/masculinising effects. There was limited evidence regarding gender dysphoria, body satisfaction, psychosocial and cognitive outcomes, and fertility. Evidence from mainly pre–post studies with 12-month follow-up showed improvements in psychological outcomes. Inconsistent results were observed for height/growth, bone health and cardiometabolic effects. Most studies included adolescents who received puberty suppression, making it difficult to determine the effects of hormones alone.
Conclusions There is a lack of high-quality research assessing the use of hormones in adolescents experiencing gender dysphoria/incongruence. Moderate-quality evidence suggests mental health may be improved during treatment, but robust study is still required. For other outcomes, no conclusions can be drawn. More recent studies published since April 2022 until January 2024 also support the conclusions of this review.
PROSPERO registration number: CRD42021289659.
Data availability statement
Data sharing is not applicable as no datasets were generated and/or analysed for this study.
This is an open access article distributed in accordance with the Creative Commons Attribution Non Commercial (CC BY-NC 4.0) license, which permits others to distribute, remix, adapt, build upon this work non-commercially, and license their derivative works on different terms, provided the original work is properly cited, appropriate credit is given, any changes made indicated, and the use is non-commercial. See: http://creativecommons.org/licenses/by-nc/4.0/ .
https://doi.org/10.1136/archdischild-2023-326670
Statistics from Altmetric.com
Request permissions.
If you wish to reuse any or all of this article please use the link below which will take you to the Copyright Clearance Center’s RightsLink service. You will be able to get a quick price and instant permission to reuse the content in many different ways.
WHAT IS ALREADY KNOWN ON THIS TOPIC
Increasing numbers of children and adolescents experiencing gender dysphoria/incongruence are being referred to specialist gender services.
National and international guidelines outline the use of hormones for masculinisation or feminisation in adolescents experiencing gender dysphoria/incongruence.
Several systematic reviews report a limited evidence base for initiating these treatments during adolescence, and uncertainty about benefits, risks and long-term effects.
WHAT THIS STUDY ADDS
There is a lack of high-quality research assessing the outcomes of hormones for masculinisation or feminisation in adolescents experiencing gender dysphoria/incongruence.
There is limited or inconsistent evidence regarding gender dysphoria, body satisfaction, psychosocial and cognitive outcomes, fertility, height/growth, bone health and cardiometabolic effects.
There is moderate-quality evidence from mainly pre–post studies that hormone treatment may in the short-term improve psychological health.
HOW THIS STUDY MIGHT AFFECT RESEARCH, POLICY OR PRACTICE
There is a lack of high-quality evidence to support the initiation of hormones for masculinisation or feminisation in adolescents experiencing gender dysphoria/incongruence. Agreement on core outcomes and high-quality research are needed.
Introduction
Over the last 10-15 years, there has been a rise in the number of children and adolescents being referred to specialist paediatric gender services. 1 2 Clinical guidelines for managing gender dysphoria/incongruence recommend assessment and psychosocial care to alleviate gender-related distress and any co-occurring difficulties. For pubertal adolescents, medications to suppress puberty in early to mid-adolescence followed by hormones that induce feminisation/masculinisation are outlined in what is described as a staged model of care. These interventions, often referred to as cross-sex hormones or gender-affirming hormones, comprise testosterone for birth-registered females and oestrogen for birth-registered males, which is sometimes given in combination with gonadotropin-releasing hormone analogues (GnRH-a), progestins, or other medications with anti-androgenic properties. 3 4
In early treatment protocols and clinical guidelines, treatments for feminisation/masculinisation were offered from age 16, and mainly in adulthood. 5 6 Over the last decade, guidelines have broadened these criteria, for example, removing minimum age 4 7 8 and making changes to the requirement of a diagnosis of gender dysphoria (Diagnostic and Statistical Manual of Mental Disorders 5th edition, DSM-5), being replaced by gender incongruence (International Classification of Diseases 11th revision, ICD-11). 3 In a study of 1766 children and adolescents receiving care at a national gender service in the Netherlands (1997–2018), 202 birth-registered males and 454 birth-registered females received hormones at a median age of 16.0 (IQR 15.5–17.1) and 16.7 (16.0–17.5), respectively. 9 In the UK, the mean age of consent for treatment was 17.3 (SD 0.1). 10
Robust evidence concerning the risks and benefits of initiating hormones during adolescence is lacking. Several systematic reviews have found mainly low-quality or limited evidence. 11–20 Due to the proliferation of research in this area, there is a need to update systematic reviews as evidence becomes available. This systematic review aims to synthesise evidence published up to April 2022 that reports outcomes of feminising/masculinising hormones in adolescents experiencing gender dysphoria/incongruence.
The review forms part of a linked series examining the epidemiology, care pathways, outcomes and experiences of children and adolescents experiencing gender dysphoria/incongruence and is reported according to Preferred Reporting Items for Systematic review and Meta-Analysis guidelines. 21 The protocol was registered on PROSPERO (CRD42021289659). 22
Search strategy
A single search strategy was used to identify studies comprising two combined concepts: ‘children’, which included all terms for children and adolescents; and ‘gender dysphoria’, which included associated terms such as gender-related distress and gender incongruence, and gender identity terms including transgender, gender diverse and non-binary.
MEDLINE ( online supplemental table S1 ), Embase and PsycINFO through OVID, CINAHL Complete through EBSCO and Web of Science (Social Science Citation Index) were searched (13–23 May 2021; updated 27 April 2022).
Supplemental material
Reference lists of included studies and relevant systematic reviews were assessed. 11–20
Inclusion criteria
The review included published research that reported outcomes of hormones used for masculinisation/feminisation in adolescents experiencing gender dysphoria/incongruence ( table 1 ).
- View inline
Inclusion and exclusion criteria
Selection process
Results of all searches were uploaded to Covidence 23 and screened independently by two reviewers. Full texts of potentially relevant articles were reviewed against inclusion criteria by two reviewers independently. Disagreements were resolved through discussion or by a third reviewer.
Data extraction
Data on study characteristics, methods and outcomes were extracted into pre-piloted data extraction templates by one reviewer and second-checked by another.
Study quality
Critical appraisal was undertaken by two reviewers independently, with consensus reached through discussion or involvement of a third reviewer.
Quality was assessed using a modified version ( online supplemental file 1 ) of the Newcastle-Ottawa Scale for cohort studies, a validated scale of eight items covering three domains: selection, comparability and outcome. 24 Scale modification included not scoring certain question(s) for cross-sectional or single-group designs, or particular outcomes; specification of key confounders to assess comparability of cohorts; guidance regarding sufficiency of follow-up; and use of numerical scores for items and overall (maximum score 9 for cohorts, 8 for pre–post and cross-sectional studies with a comparator). Total scores are presented as percentages to account for different total scores (≤50% low, >50–75% moderate, >75% high quality).
Narrative synthesis methods were used because of heterogeneity in study design, intervention, comparator, outcome and measurement. Low-quality studies were excluded from the synthesis due to the high risk of bias. For studies that reported outcomes for both puberty suppression and hormones, only the findings relating to hormones were synthesised.
Outcomes were grouped into distinct and clinically meaningful categories for synthesis, based on what had been measured across the studies in addition to the a priori categories informing the review. Care was taken to differentiate between different study designs, comparators and interventions. Where possible, potential differences by birth-registered sex, and treatment duration/initiation were examined.
Database searches yielded 28 147 records, 3181 of which were identified as potentially relevant for the linked systematic reviews, and full texts reviewed. From these, 53 studies met the inclusion criteria for this review ( figure 1 ).
- Download figure
- Open in new tab
- Download powerpoint
Study flow diagram.
Study characteristics
Studies were published from 2006 to 2022 (with 60% from 2020 to 2022 (n=32)), and conducted in the Netherlands (n=17), 25–41 US (n=24), 42–65 Israel (n=3), 66–68 Belgium (n=2), 69 70 Canada (n=2) 71 72 and one in Brazil, 73 Finland, 74 Germany, 75 Spain 76 and the UK 77 ( online supplemental table S2 ).
Of the 53 studies, 12 were cohorts comparing adolescents experiencing gender dysphoria/incongruence receiving hormones with a comparator, 34 37–39 42 43 46 49 54 64 75 76 9 cross-sectional with comparator 25 45 50 51 57 60 65 73 77 and 32 pre–post designs. 26–33 35 36 40 41 44 47 48 52 53 55 56 58 59 61–63 66–72 74 Over half of the studies (n=30) used retrospective chart review.
All but five studies recruited adolescents experiencing gender dysphoria/incongruence from specialist gender or endocrinology services: 46 from single clinics (in Belgium, Israel, Netherlands and the UK these were large regional/national services), and 2 from multiple US clinics. Of the remaining five, four were US studies (national survey recruiting via community settings, 65 clinical and community settings, 45 social media platforms, 50 US Military Healthcare Data Repository 47 ). The final study from Brazil recruited via Facebook. 73
Overall, the studies included 40 906 participants, of which 22 192 were adolescents experiencing gender dysphoria/incongruence (8164 received hormone treatments and 14 028 did not), and 18 714 comparators. Comparator groups included adolescents who had either not received hormones or received it in adulthood 34 39 42 43 45 46 49–51 65 73 75 78 ; adolescents not experiencing gender dysphoria/incongruence 37 38 57 60 64 76 ; both comparators 25 ; or studies comparing those receiving hormones alone with those receiving it in combination with GnRH-a/progestins/anti-androgens. 54
The most frequently reported outcomes were physical health outcomes (n=29) and puberty development (n=25) ( figure 2 , online supplemental table S3 ). Side effects, bone health and fertility were measured in six, five and one study, respectively. Psychological health was measured in 15 studies, psychosocial in 7 and cognitive/neurodevelopmental outcomes in 4. Gender-related outcomes and body image were each measured in three studies.
Outcome categories by study quality and design.
One cohort study measuring side effects only was rated as high-quality, 54 33 were moderate 25 30–41 45 47 48 50–53 55 56 59–63 66 67 69 70 74 76 and 19 low. 26–29 42–44 46 49 57 58 64 65 68 71–73 75 77 Of the 12 cohort studies, (the only studies to include a comparator group and assess outcomes over time), 6 were rated as high or moderate quality ( figure 2 , online supplemental table S4 ). 34 37–39 54 76
In most studies, there were concerns about the representativeness of the population due to single-site recruitment, selective inclusion and/or poor reporting of the eligible population. In the 21 studies including a comparator, most did not report or control for key differences between groups, and only 6 used matched controls. 25 37 38 60 64 76 Most studies presented results separately by birth-registered sex or controlled for this, but few controlled for age, Tanner stage or co-interventions.
Overall, studies used appropriate methods to ascertain exposure and assess outcomes. Follow-up was adequate in 22 studies, with others not reporting clear information, or follow-up varying between participants or not linked to treatment initiation. Missing data at follow-up/analysis or poor reporting of this affected many studies.
Three studies did not report separate outcomes for adolescents receiving puberty suppression or hormones (one was moderate quality and excluded from synthesis). 47 73 77 One moderate-quality study assessing the amplitude of click-evoked otoacoustic emissions was excluded from the synthesis due to not being clinically relevant. 25
Synthesis of outcomes
Gender dysphoria and body satisfaction.
One cohort study measured gender dysphoria pre–post, and reported a reduction in dysphoria with no participants in clinical range at follow-up. 76 One cross-sectional study measured body satisfaction in birth-registered females and reported lower dissatisfaction in those receiving hormone treatment compared with those who had not 51 ( online supplemental table S5 ).
Psychological health
Five studies (one cohort, 76 two pre–post 48 74 and two cross-sectional 50 51 ) measured psychological health. In four studies, participants had received hormones for ~12 months at follow-up. One cross-sectional study did not report treatment duration. 50 Reported outcomes were depression (n=4), anxiety (n=3), suicide and/or self-harm (n=4), need for specialist-level psychiatric treatment for different mental health difficulties (n=1) and internalising and externalising symptoms (n=1) ( online supplemental table S5 ).
Studies found a reduction in depression and anxiety at follow-up (cohort 76 ) and for birth-registered females receiving hormones compared with females not receiving hormones (cross-sectional 51 ), but levels were higher when compared with adolescents not experiencing gender dysphoria/incongruence (cohort 76 ). Lower treatment needs for depression and anxiety were reported after treatment in a pre–post study. 74 A cross-sectional study reported lower levels of depression in adolescents who had received hormones compared with those who had wanted hormones but had not received them. 50
A pre–post study found no changes in treatment need for conduct problems, psychotic symptoms/psychosis, substance abuse, autism spectrum condition, attention-deficit hyperactivity disorder or eating disorders, 74 but two pre–post studies found a reduction in treatment needs for (or lower levels of) suicidality/self-harm. 48 74 Two cross-sectional studies found conflicting results: those receiving hormones were less likely to have seriously considered/attempted suicide compared with adolescents not receiving hormones, 50 and in birth-registered females there was no difference between groups. 51
One cohort study reported a significant decrease in total psychological difficulties and scores for hyperactivity, emotional and conduct problems, with fewer participants in borderline and abnormal ranges at follow-up. 76 Compared with adolescents not experiencing gender dysphoria/incongruence, psychological difficulties were higher at baseline but similar at follow-up.
Psychosocial functioning
A cohort study reported no change in family functioning or peer problems but more peer problems when compared with adolescents not experiencing gender dysphoria/incongruence. 76 A small improvement was reported in prosocial skills. A pre–post study measured peer relations, living arrangements, school/work participation, romantic involvement and competence in managing everyday matters, with the only changes being a decrease in participants living with parents/guardians at follow-up and a small decrease in normative peer relationships. 74 A pre–post study reported an increase in well-being after receipt of hormones 48 ( online supplemental table S5 ).
Cognitive outcomes
Two cohort studies of birth-registered females assessed whether exogenous testosterone-induced changes reflect sex-based differences in brain activity. One study measured visuospatial working memory, and found no difference in performance between those treated and female and male controls not experiencing gender dysphoria/incongruence, but did observe stronger frontal and parietal activation at follow-up in male controls and those treated. 38 The second study measured amygdala activation, observing slightly more rightward lateralisation following treatment, and similar lateralisation in those treated compared with female and male controls who did not change. 37
Amygdala activation was also assessed in a cross-sectional study of birth-registered females, finding greater activation and increased connectivity between the amygdala and prefrontal cortex in those receiving hormones compared with those who had not. 51
A cross-sectional study found those receiving hormones had better executive functioning, cognitive flexibility and working memory compared with a group not receiving hormones 45 ( online supplemental table S5 ).
Physical health outcomes
Bone health.
Four pre–post studies 30 32 33 36 measured bone health. Two reported an increase at follow-up in absolute measures of bone density and SD scores 32 33 (one included birth-registered females only 33 ), and two reported no change in these and/or bone biomarkers, although these included small samples 30 36 ( online supplemental table S6 ).
Cardiometabolic health
Body mass index (BMI) and/or a standardised measure (BMI SD/z score or percentile) was reported in 16 studies (1 cohort, 34 14 pre–post 30 33 35 40 53 55 56 61–63 66 67 69 70 and 1 cross-sectional 60 ), showing no change overall but some inconsistencies ( online supplemental table S6 ).
For birth-registered males, one study reported an increase in SD score, 30 one a decrease 40 and two no change. 66 70 All reported no clinically significant change in BMI. 30 40 66 70 Four studies only reported BMI: three found no change 53 56 62 and one reported an increase for participants starting GnRH-a in early puberty prior to initiating hormones. 35
For birth-registered females, two studies reported an increase in SD score 30 69 and four no change. 33 55 63 67 All but one of these, which found an increase in BMI as well as SD score, 69 reported no change in BMI. 33 55 63 67 Five studies only reported BMI: four found no change, 53 56 61 62 and one an increase in the early puberty group. 35
A cross-sectional study of both sexes found no difference in BMI percentile between those receiving hormones compared with controls, 60 and a single cohort study found those who started hormones earlier had a lower BMI (although not clinically significant) than those who started treatment later. 34
Seven pre–post studies assessed cholesterol markers: three reported a decrease in high-density lipoprotein (HDL), 33 53 55 one an increase 62 70 and three no change. 61 69 70 A cross-sectional study found that birth-registered females receiving hormones had lower HDL than controls, whereas birth-registered males had higher HDL than controls. 60
Eight pre–post studies measured blood pressure 33 40 53 61 62 66 67 and one hypertension, 55 all reported no clinically significant change. One cross-sectional study found similar blood pressure in adolescents receiving hormones compared with adolescents not experiencing gender dysphoria/incongruence. 60
Six studies measured HbA1c (glycated haemoglobin), 33 40 53 60 69 70 four glucose levels, 60 62 69 70 three fasting insulin 60 69 70 and two homeostatic model assessment (HOMA) index. 60 69 One cross-sectional study reported differences between birth-registered males and controls in the inverse of fasting insulin (lower) and HOMA insulin resistance (higher) compared with controls. 60 No changes were reported by other studies.
Other parameters
Twelve studies assessed other physiological parameters obtained from blood tests (11 pre–post 31 33 40 41 53 55 56 61 62 69 70 and one cross-sectional 60 ; ( online supplemental table S6 )): dehydroepiandrosterone sulfate (n=3), androstenedione (n=2), creatinine (n=6), estimated glomerular filtration rate (n=1), prolactin (n=5), alanine transaminase (n=8), aspartate transaminase (n=8), g-glutamyl transferase (n=1), haematocrit (n=7), serum urea nitrogen (n=1), haemoglobin (n=7), potassium (n=1), vitamin D (n=1), thyroid stimulating hormone (n=3), free thyroxin (n=3), anti-Müllerian hormone (n=1), alkaline phosphatase (n=2), sex hormone binding globulin (n=4), free androgen index (n=1) and urea (n=1). For most outcomes there were no changes pre–post or differences between groups; where there were changes results were inconsistent.
One pre–post study found no occurrence of thrombosis after masculinising/feminising hormones. 59
Pubertal development
Hormone levels.
Fifteen studies measured hormone levels (2 cohort, 37 38 12 pre–post 33 35 40 53 55 59 61 62 66 67 69 70 and 1 cross-sectional 60 ), 7 studies in birth-registered females, 33 37 38 55 61 67 69 3 in birth-registered males 40 66 70 and 5 both ( online supplemental table 7 ). 35 53 59 60 62
All pre–post studies and a cohort reporting pre–post data found increased/heightened testosterone and oestradiol in birth-registered females and males, respectively. 33 35 40 53 55 59 61 62 66 67 69 70 Three studies (two cohort and one cross-sectional) found testosterone levels in birth-registered females receiving hormones were higher than female controls but lower than male controls. 37 38 60 The cross-sectional study reported similar oestradiol levels in birth-registered males receiving hormones and female controls, and higher levels compared with male controls. 60 Luteinising and follicle-stimulating hormones remained constant or decreased slightly. 33 40 60 66 67 69 70
In birth-registered females, three studies reported increases in oestradiol, 33 35 67 two no change 53 69 and three reported decreases 55 61 62 (with varying follow-up across studies). In birth-registered males, one study reported no change in testosterone, 66 four a decrease 35 53 62 70 and one observed higher testosterone levels compared with female but lower compared with male controls. 60
Induced pubertal progression
Four pre–post studies with at least 12 months follow-up measured pubertal development, 33 36 40 70 and three Tanner breast stage ( online supplemental table 7 ). 36 40 70 Two observed an increase in breast volume in birth-registered males after hormones, 40 70 although objectively breast volume was small, 70 and another reported no change in breast volume in birth-registered females. 36 One study of birth-registered females reported an increase in facial, abdominal, chest and extremities hair, and voice deepening in all participants at follow-up. 33 Another reported no change in Tanner genital stage in birth-registered males, 36 and no change in Tanner pubic hair stage for both. 36
Menstrual suppression
Three pre–post studies reported suppression in most participants ( online supplemental table 7 ): 85% cessation after 6 months, 61 80% no breakthrough bleeding at 12 months 52 and nearly all reported suppression on 200 mg of subcutaneous testosterone, although just over half reported suppression on 140 mg (median follow-up 1.9 years). 55
Height/growth
One cohort 34 and six pre–post studies 30 33 35 36 40 70 reported height and/or height SD score, showing mixed results (online supplementary material S7).
For birth-registered males, two studies reported an increase in height SD score 30 70 and one no change. 40 Three studies reported an increase in absolute height. 30 36 40 An increase was observed in height SD when using affirmed-gender references. 40
For birth-registered females, two studies reported no change in height SD score, 30 33 and three reported an increase in absolute height. 30 33 36
One study reported that birth-registered females who received hormones earlier and for longer, were taller than those who started treatment later. There was no difference for birth-registered males. 34 All participants in the study had first received puberty suppression. A second study found that for both sexes, the average height at follow-up was higher in those who started hormone treatment earlier. 35
Body composition/shape
Birth-registered females receiving testosterone had lower body fat percentage and fat mass, and higher lean tissue percentage and lean mass than female controls not experiencing gender dysphoria/incongruence, and the converse compared with males controls. 60 Higher body fat percentage and fat mass and lower lean tissue percentage were seen for birth-registered males compared with male controls, and the converse compared with females controls ( online supplemental table 7 ).
A pre–post study in birth-registered males reported no change in fat mass after 2 years of treatment, but at 3 years it was higher compared with baseline ( online supplemental table 7 ). Both fat percentage and lean body mass percentage remained the same. 40 The same study reported a decrease in absolute and SD score for waist–hip ratio (compared with reference data for males and females), a decrease in SD score but no absolute change in waist circumference, and no change in SD score but an absolute increase in hip circumference. 40
Bone age and geometry
Two pre–post studies measured bone age and reported an increase after treatment (online supplementary material S7). 36 40 In birth-registered males who started GnRH-a in mid-puberty or late-puberty prior to initiating hormones, there was an increase in subperiosteal width and endocortical diameter, but not in those who started this treatment in early puberty. 35 For birth-registered females, there was no evidence of change.
One cohort study measured fertility in birth-registered males by comparing orchiectomy specimens of those who started hormone treatment at Tanner stage 2/3, 4/5 or in adulthood, with all adolescents first receiving puberty suppression (online supplemantary table S6). 39 Mature spermatozoa were only encountered in those who started this treatment at Tanner stage 4 or higher. Immature germ cells were present in all those treated in early puberty. Duration of hormone treatment did not influence study outcomes.
Side effects
Four studies reported side effects (one cohort 54 and three pre–post 55 69 70 ). Three studies of birth-registered females reported an increase in acne at follow-up. 54 55 69 One study also reported mood changes, elevated red blood markers and increased appetite as common, with headaches, hot flashes, fatigue and hair loss less commonly reported. 54 One study reported a slight increase in metrorrhagia after adding testosterone following <6 months of lynestrenol. 69
Breast tenderness was commonly reported in two studies of birth-registered males, 54 70 with less common reports of increased liver enzymes and oestradiol levels above the normal limit, 54 70 and mood swings and increased appetite frequently reported. 70 The cohort study reported similar side effect profiles in those receiving GnRH-a concurrently with hormones and those receiving hormones alone. 54
This systematic review identified 53 studies reporting outcomes for feminising/masculinising hormones for adolescents experiencing gender dysphoria/incongruence. Only 6 of the 12 cohort studies were rated as high or moderate quality. 34 37–39 54 76
There was evidence from multiple studies that exogenous hormones increase hormone levels and to varying degrees induce pubertal development, with potential differences depending on birth-registered sex and timing of treatment. Inconsistent results were found for height/growth, bone health and cardiometabolic health. There was insufficient evidence regarding changes to gender dysphoria, body satisfaction, psychosocial and cognitive outcomes, or fertility (no study assessed fertility in birth-registered females). These findings add to other systematic reviews in concluding there is insufficient and/or inconsistent evidence about the risks and benefits of hormone interventions in this population. 11–20
Regarding psychological health, evidence from mainly pre–post studies suggests hormones are associated with improvements in depression, anxiety and other mental health difficulties after 12 months of treatment, although there were inconsistencies regarding suicidality and/or self-harm, with three of four studies reporting an improvement and one no change. Concerns about study representativeness and comparability of control groups (where used) mean these findings must be interpreted with caution. Well-designed robust studies that control for key confounding factors with longer-term follow-up are needed.
Over half of the studies reported the effects of both puberty suppression and hormones. In adolescents, GnRH-a often continues during hormone treatment. 9 For adolescents who do not first receive puberty suppression, GnRH-a or another anti-androgenic treatment may be offered at the initiation of hormones although the reasons for this are unclear. 79 Although recent studies suggest most adolescents who proceed with hormones will receive puberty suppression before this, 9 10 80 research that robustly compares outcomes for adolescents on this treatment pathway versus receiving hormones alone is needed, especially given recent studies suggesting the effect on secondary sex characteristics and fertility may be different. 34 39
Agreement about core aims and outcomes for hormone interventions for adolescents would facilitate future aggregation of evidence. Included studies assessed multiple different outcomes across various domains. The rationale for cognitive outcomes varied, with some studies primarily focusing on sex-based differences presumed from wider research. 81 Few studies examined whether hormones influence cognitive development in adolescence, which is identified as a key area of uncertainty.
Clinicians should ensure that adolescents considering hormone interventions are fully informed about the potential risks and benefits including side-effects, and the lack of high-quality evidence regarding these. In response to their own evidence review, the Swedish National Board of Health and Welfare now recommends that hormone treatments should only be provided under a research framework, a key aim for which is to develop a stronger evidence base. 82 As they point out, this approach is common practice in other clinical specialties, where to receive treatments for which the benefits and risks are uncertain, patients must take part in research.
Strengths and limitations
Strengths include a published protocol with robust search strategies, and comprehensive synthesis of high-quality and moderate-quality studies. Poor study reporting may have resulted in moderate-quality studies being rated as low-quality and excluded from the synthesis. As searches were conducted to April 2022 this review does not include more recently published studies. However, this review draws similar conclusions to other reviews despite including numerous additional studies. Of other studies published since April 2022 until January 2024, very few used a cohort design or an appropriate comparator and were of similar low-quality to moderate-quality. Of those likely to contribute new data to the synthesis, five focused on bone health and growth, 83–87 one on cardiometabolic risk 88 and two assessed psychological health, 89 90 one of which also assessed life satisfaction and congruence with gender identity and appearance, 89 and the other additionally assessed body image and sex-typed brain activity. 90
All three studies assessing bone health examined changes over time for participants treated with GnRH-a followed by hormones and found, overall, that after hormone treatment, bone mineral density scores were in line with expected maturation, despite a deceleration during GnRH-a treatment. 83 86 87 Two of these studies also found that height growth was not affected following hormone treatment, 84 87 and this was found in a third study as well. 85 The increasing number of studies assessing bone health and height growth potentially indicate that following hormone treatment, bone health is in line with expected maturation and height growth is not affected. However, there remains uncertainty about these outcomes due to the lack of high-quality studies that use a longitudinal design and appropriate comparator with longer-term follow-up.
A single new study assessed changes in body composition. 83 It found an increase in lean body mass z-scores and a decrease in fat mass z-scores during the first year of treatment in birth-registered females, which remained stable over 3 years of treatment. For birth-registered males, there was a slight decrease in lean mass z-scores in the first year which remained stable over time, but little change in fat mass z-scores. This study adds to the limited evidence base for body composition, but no conclusions can be drawn due to the inconsistency in results across studies.
One study examined whether receipt of hormone interventions was associated with cardiometabolic-related diagnoses and found that certain diagnoses were more likely in birth-registered females receiving testosterone, suggesting that cardiometabolic health may be compromised in this group. 88 However, it is the only study that has examined diagnoses rather than cardiometabolic markers, and it used a cross-sectional design, therefore no conclusions can be drawn about these outcomes.
Two studies measured psychological health. 89 90 They both found lower levels of anxiety and depression for birth-registered females, in one study during 2 years after hormone initiation, 89 and in the other when compared with those not receiving hormones, 90 which also found lower levels of suicidality in those receiving hormones but no difference for internalising symptoms. 90 Overall, no differences for birth-registered males were observed for the same outcomes in both studies, although one study found that for both sexes, taking hormones for longer durations was associated with fewer depression and suicidality symptoms, with a stronger association between longer duration and lower suicidality in birth-registered males. 90 These studies add to the moderate-quality evidence that hormone treatment may improve psychological health, although robust research with long-term follow-up is still needed.
A single study assessing outcomes during the 2 years after hormone initiation found that scores for gender congruence and life satisfaction increased, but there were differences by birth-registered sex and timing of hormone initiation. 89 Lastly, a single cross-sectional study explored body image and sex-typed brain activity. 90 It found body image satisfaction was higher in those receiving hormones compared with those not receiving hormones and those taking hormones for longer durations. In terms of sex-typed brain activity, analysis of amygdala-vmPFC (ventromedial prefrontal cortex) coupling found greater coupling in those receiving hormones. However, as there is still limited evidence about the effect of hormones on gender-related, psychosocial and cognitive outcomes, no further conclusions can be drawn.
Conclusions
There is a lack of high-quality research assessing the outcomes of hormone interventions in adolescents experiencing gender dysphoria/incongruence, and few studies that undertake long-term follow-up. No conclusions can be drawn about the effect on gender-related outcomes, body satisfaction, psychosocial health, cognitive development or fertility. Uncertainty remains about the outcomes for height/growth, cardiometabolic and bone health. There is suggestive evidence from mainly pre–post studies that hormone treatment may improve psychological health although robust research with long-term follow-up is needed.
Ethics statements
Patient consent for publication.
Not applicable.
Ethics approval
- Thompson L ,
- Sarovic D ,
- Wilson P , et al
- Kaltiala R ,
- Bergman H ,
- Carmichael P , et al
- Coleman E ,
- Bouman WP , et al
- Hembree WC ,
- Cohen-Kettenis PT ,
- Gooren L , et al
- de Vries ALC ,
- Cohen-Kettenis PT
- Telfer MM ,
- Tollit MA ,
- Pace CC , et al
- University of California San Francisco Gender Affirming Health Program
- van der Loos MATC ,
- Hannema SE , et al
- Carruthers P , et al
- Pasternack I ,
- Söderström I ,
- Saijonkari M , et al
- Ludvigsson JF ,
- Adolfsson J ,
- Höistad M , et al
- Wilson LM ,
- Sharma R , et al
- Anderson J ,
- Williams K , et al
- National Institute for Health and Care Excellence (NICE)
- Singh-Ospina N ,
- Rodriguez-Gutierrez R , et al
- Singh Ospina N ,
- Karalexi MA ,
- Georgakis MK ,
- Dimitriou NG , et al
- McKenzie JE ,
- Bossuyt PM , et al
- Taylor J , et al
- Veritas Health Innovation
- O’Connell D , et al
- van Heesewijk JO ,
- Menks WM , et al
- McGuire JK ,
- Steensma TD , et al
- Delemarre-van de Waal HA ,
- de Mutsert R ,
- Wiepjes CM , et al
- van der Loos MATC , et al
- Heijboer A , et al
- Schagen SEE ,
- Lustenhouwer P ,
- Cohen-Kettenis PT , et al
- Wouters FM ,
- Stoffers IE ,
- de Vries MC ,
- van de Grift TC ,
- van Gelder ZJ ,
- Mullender MG , et al
- van der Loos MA ,
- Hellinga I ,
- Vlot MC , et al
- den Heijer M , et al
- Geuze RH , et al
- Kreukels BPC ,
- Mulder CL ,
- Meißner A , et al
- Hannema SE ,
- Madsen MC ,
- van Dijk D ,
- Achille C ,
- Taggart T ,
- Eaton NR , et al
- Grimstad FW ,
- Jacobson JD
- Stewart S ,
- Preston S , et al
- Strang JF ,
- Nelson E , et al
- Tordoff DM ,
- Collin A , et al
- Hisle-Gorman E ,
- Schvey NA ,
- Adirim TA , et al
- Watson LB ,
- Egan AM , et al
- Connelly KJ , et al
- DeChants JP ,
- Price MN , et al
- Grannis C ,
- Leibowitz SF ,
- Gahn S , et al
- Grimstad F ,
- Shim J , et al
- Pine-Twaddell E ,
- Trotman G , et al
- Jensen RK ,
- Jensen JK ,
- Simons LK , et al
- Laurenzano SE ,
- Newfield RS ,
- Lee E , et al
- Millington K ,
- Barrera E ,
- Daga A , et al
- Mullins ES ,
- Metcalf M , et al
- Nokoff NJ ,
- Scarbro SL ,
- Moreau KL , et al
- Schrager SM ,
- Clark LF , et al
- Olson-Kennedy J ,
- Sequeira GM ,
- El Nokali NE , et al
- Valentine A ,
- Bonny A , et al
- Turban JL ,
- Kobe J , et al
- Elkon-Tamir E ,
- Segev-Becker A , et al
- Segev-Becker A ,
- Israeli G , et al
- Israeli G ,
- Elkon-Tamir E , et al
- Dhondt K , et al
- Craen M , et al
- Khatchadourian K ,
- Chiniara LN ,
- Bonifacio HJ ,
- Fontanari AMV ,
- Vilanova F ,
- Schneider MA , et al
- Työläjärvi M , et al
- Becker-Hebly I ,
- Fahrenkrug S ,
- Campion F , et al
- López de Lara D ,
- Pérez Rodríguez O ,
- Cuellar Flores I , et al
- Arcelus J ,
- Witcomb GL , et al
- Bouman WP ,
- Van Den Noortgate W , et al
- Lapauw B , et al
- van der Loos M ,
- Klink DT , et al
- Khan H , et al
- The Swedish National Board of Health and Welfare
- Boogers LS ,
- Reijtenbagh SJP ,
- Wiepjes CM ,
- Ciancia S ,
- Willemsen LA ,
- Furniss A , et al
- Chan Y-M , et al
- Mattson WI ,
- Leibowitz SF , et al
Supplementary materials
Supplementary data.
This web only file has been produced by the BMJ Publishing Group from an electronic file supplied by the author(s) and has not been edited for content.
- Data supplement 1
- Data supplement 2
- Data supplement 3
- Data supplement 4
- Data supplement 5
- Data supplement 6
- Data supplement 7
- Data supplement 8
Contributors LF, RH, TL and JT contributed to the conception of this review. RH, CEH, AM and JT contributed to screening and selection. AM and JT completed data extraction. CEH, RH, AM and JT contributed to critical appraisal. CEH, AM and JT completed the synthesis and drafted the manuscript. All authors contributed to the interpretation, and reviewed and approved the manuscript. CEH accepts full responsibility for the finished work and/or the conduct of the study, had access to the data, and controlled the decision to publish.
Funding This work was funded by NHS England to inform the Cass Review (Independent review of gender identity services for children and young people). The funder and Cass Review team had a role in commissioning the research programme but no role in the study conduct, interpretation or conclusion.
Competing interests None declared.
Provenance and peer review Commissioned; externally peer reviewed.
Supplemental material This content has been supplied by the author(s). It has not been vetted by BMJ Publishing Group Limited (BMJ) and may not have been peer-reviewed. Any opinions or recommendations discussed are solely those of the author(s) and are not endorsed by BMJ. BMJ disclaims all liability and responsibility arising from any reliance placed on the content. Where the content includes any translated material, BMJ does not warrant the accuracy and reliability of the translations (including but not limited to local regulations, clinical guidelines, terminology, drug names and drug dosages), and is not responsible for any error and/or omissions arising from translation and adaptation or otherwise.
Linked Articles
- Original research Clinical guidelines for children and adolescents experiencing gender dysphoria or incongruence: a systematic review of guideline quality (part 1) Jo Taylor Ruth Hall Claire Heathcote Catherine Elizabeth Hewitt Trilby Langton Lorna Fraser Archives of Disease in Childhood 2024; - Published Online First: 09 Apr 2024. doi: 10.1136/archdischild-2023-326499
- Original research Care pathways of children and adolescents referred to specialist gender services: a systematic review Jo Taylor Ruth Hall Trilby Langton Lorna Fraser Catherine Elizabeth Hewitt Archives of Disease in Childhood 2024; - Published Online First: 09 Apr 2024. doi: 10.1136/archdischild-2023-326760
- Original research Psychosocial support interventions for children and adolescents experiencing gender dysphoria or incongruence: a systematic review Claire Heathcote Jo Taylor Ruth Hall Stuart William Jarvis Trilby Langton Catherine Elizabeth Hewitt Lorna Fraser Archives of Disease in Childhood 2024; - Published Online First: 09 Apr 2024. doi: 10.1136/archdischild-2023-326347
- Original research Gender services for children and adolescents across the EU-15+ countries: an online survey Ruth Hall Jo Taylor Claire Heathcote Trilby Langton Catherine Elizabeth Hewitt Lorna Fraser Archives of Disease in Childhood 2024; - Published Online First: 09 Apr 2024. doi: 10.1136/archdischild-2023-326348
- Original research Impact of social transition in relation to gender for children and adolescents: a systematic review Ruth Hall Jo Taylor Catherine Elizabeth Hewitt Claire Heathcote Stuart William Jarvis Trilby Langton Lorna Fraser Archives of Disease in Childhood 2024; - Published Online First: 09 Apr 2024. doi: 10.1136/archdischild-2023-326112
- Original research Characteristics of children and adolescents referred to specialist gender services: a systematic review Jo Taylor Ruth Hall Trilby Langton Lorna Fraser Catherine Elizabeth Hewitt Archives of Disease in Childhood 2024; - Published Online First: 09 Apr 2024. doi: 10.1136/archdischild-2023-326681
- Original research Interventions to suppress puberty in adolescents experiencing gender dysphoria or incongruence: a systematic review Jo Taylor Alex Mitchell Ruth Hall Claire Heathcote Trilby Langton Lorna Fraser Catherine Elizabeth Hewitt Archives of Disease in Childhood 2024; - Published Online First: 09 Apr 2024. doi: 10.1136/archdischild-2023-326669
- Original research Clinical guidelines for children and adolescents experiencing gender dysphoria or incongruence: a systematic review of recommendations (part 2) Jo Taylor Ruth Hall Claire Heathcote Catherine Elizabeth Hewitt Trilby Langton Lorna Fraser Archives of Disease in Childhood 2024; - Published Online First: 09 Apr 2024. doi: 10.1136/archdischild-2023-326500
- Editorial Holistic approach to gender questioning children and young people Camilla C Kingdon Archives of Disease in Childhood 2024; - Published Online First: 09 Apr 2024. doi: 10.1136/archdischild-2024-327100
Read the full text or download the PDF:
- Open access
- Published: 14 October 2023
A scoping review of ‘Pacing’ for management of Myalgic Encephalomyelitis/Chronic Fatigue Syndrome (ME/CFS): lessons learned for the long COVID pandemic
- Nilihan E. M. Sanal-Hayes 1 , 7 ,
- Marie Mclaughlin 1 , 8 ,
- Lawrence D. Hayes 1 ,
- Jacqueline L. Mair ORCID: orcid.org/0000-0002-1466-8680 2 , 3 ,
- Jane Ormerod 4 ,
- David Carless 1 ,
- Natalie Hilliard 5 ,
- Rachel Meach 1 ,
- Joanne Ingram 6 &
- Nicholas F. Sculthorpe 1
Journal of Translational Medicine volume 21 , Article number: 720 ( 2023 ) Cite this article
3348 Accesses
5 Citations
21 Altmetric
Metrics details
Controversy over treatment for people with myalgic encephalomyelitis/chronic fatigue syndrome (ME/CFS) is a barrier to appropriate treatment. Energy management or pacing is a prominent coping strategy for people with ME/CFS. Whilst a definitive definition of pacing is not unanimous within the literature or healthcare providers, it typically comprises regulating activity to avoid post exertional malaise (PEM), the worsening of symptoms after an activity. Until now, characteristics of pacing, and the effects on patients’ symptoms had not been systematically reviewed. This is problematic as the most common approach to pacing, pacing prescription, and the pooled efficacy of pacing was unknown. Collating evidence may help advise those suffering with similar symptoms, including long COVID, as practitioners would be better informed on methodological approaches to adopt, pacing implementation, and expected outcomes.
In this scoping review of the literature, we aggregated type of, and outcomes of, pacing in people with ME/CFS.
Eligibility criteria
Original investigations concerning pacing were considered in participants with ME/CFS.
Sources of evidence
Six electronic databases (PubMed, Scholar, ScienceDirect, Scopus, Web of Science and the Cochrane Central Register of Controlled Trials [CENTRAL]) were searched; and websites MEPedia, Action for ME, and ME Action were also searched for grey literature, to fully capture patient surveys not published in academic journals.
A scoping review was conducted. Review selection and characterisation was performed by two independent reviewers using pretested forms.
Authors reviewed 177 titles and abstracts, resulting in 17 included studies: three randomised control trials (RCTs); one uncontrolled trial; one interventional case series; one retrospective observational study; two prospective observational studies; four cross-sectional observational studies; and five cross-sectional analytical studies. Studies included variable designs, durations, and outcome measures. In terms of pacing administration, studies used educational sessions and diaries for activity monitoring. Eleven studies reported benefits of pacing, four studies reported no effect, and two studies reported a detrimental effect in comparison to the control group.
Conclusions
Highly variable study designs and outcome measures, allied to poor to fair methodological quality resulted in heterogenous findings and highlights the requirement for more research examining pacing. Looking to the long COVID pandemic, our results suggest future studies should be RCTs utilising objectively quantified digitised pacing, over a longer duration of examination (i.e. longitudinal studies), using the core outcome set for patient reported outcome measures. Until these are completed, the literature base is insufficient to inform treatment practises for people with ME/CFS and long COVID.
Introduction
Post-viral illness occurs when individuals experience an extended period of feeling unwell after a viral infection [ 1 , 2 , 3 , 4 , 5 , 6 ]. While post-viral illness is generally a non-specific condition with a constellation of symptoms that may be experienced, fatigue is amongst the most commonly reported [ 7 , 8 , 9 ]. For example, our recent systematic review found there was up to 94% prevalence of fatigue in people following acute COVID-19 infection [ 3 ]. The increasing prevalence of long COVID has generated renewed interest in symptomology and time-course of post-viral fatigue, with PubMed reporting 72 articles related to “post-viral fatigue” between 2020 and 2022, but less than five for every year since 1990.
As the coronavirus pandemic developed, it became clear that a significant proportion of the population experienced symptoms which persisted beyond the initial viral infection, meeting the definition of a post-viral illness. Current estimates suggest one in eight people develop long COVID [ 10 ] and its symptomatology has repeatedly been suggested to overlap with clinical demonstrations of myalgic encephalomyelitis/chronic fatigue syndrome (ME/CFS). In a study by Wong and Weitzer [ 11 ], long COVID symptoms from 21 studies were compared to a list of ME/CFS symptoms. Of the 29 known ME/CFS symptoms the authors reported that 25 (86%) were reported in at least one long COVID study suggesting significant similarities. Sukocheva et al. [ 12 ] reported that long COVID included changes in immune, cardiovascular, metabolic, gastrointestinal, nervous and autonomic systems. When observed from a pathological stance, this list of symptoms is shared with, or is similar to, the symptoms patients with ME/CFS describe [ 13 ]. In fact, a recent article reported 43% of people with long COVID are diagnosed with ME/CFS [ 13 ], evidencing the analogous symptom loads.
A striking commonality between long COVID and similar conditions such as ME/CFS is the worsening of symptoms including fatigue, pain, cognitive difficulties, sore throat, and/or swollen lymph nodes following exertion. Termed post exertional malaise (PEM) [ 14 , 15 , 16 , 17 ], lasting from hours to several days, it is arguably one of the most debilitating side effects experienced by those with ME/CFS [ 16 , 17 , 18 ]. PEM is associated with considerably reduced quality of life amongst those with ME/CFS, with reduced ability to perform activities of daily living, leading to restraints on social and family life, mental health comorbidities such as depression and anxiety, and devastating employment and financial consequences [ 19 , 20 , 21 , 22 ]. At present, there is no cure or pharmacological treatments for PEM, and therefore, effective symptom management strategies are required. This may be in part because the triggers of PEM are poorly understood, and there is little evidence for what causes PEM, beyond anecdotal evidence. The most common approach to manage PEM is to incorporate activity pacing into the day-to-day lives of those with ME/CFS with the intention of reducing the frequency of severity of bouts of PEM [ 23 ]. Pacing is defined as an approach where patients are encouraged to be as active as possible within the limits imposed by the illness [ 23 , 24 , 25 ]. In practice, pacing requires individuals to determine a level at which they can function, but which does not lead to a marked increase in fatigue and other symptoms [ 26 , 27 ].
Although long COVID is a new condition [ 3 , 14 ], the available evidence suggests substantial overlap with the symptoms of conditions such as ME/CFS and it is therefore pragmatic to consider the utility of management strategies (such as pacing) used in ME/CFS for people with long COVID. In fact, a recent Delphi study recommended that management of long COVID should incorporate careful pacing to avoid PEM relapse [ 28 ]. This position was enforced by a multidisciplinary consensus statement considering treatment of fatigue in long COVID, recommending energy conservation strategies (including pacing) for people with long COVID [ 29 ]. Given the estimated > 2 million individuals who have experienced long COVID in the UK alone [ 30 , 31 , 32 ], there is an urgent need for evidence-based public health strategies. In this context, it seems pragmatic to borrow from the ME/CFS literature.
From a historical perspective, the 2007 NICE guidelines for people with ME/CFS advised both cognitive behavioural therapy (CBT) and graded exercise therapy (GET) should be offered to people with ME/CFS [ 33 ]. As of the 2021 update, NICE guidelines for people with ME/CFS do not advise CBT or GET, and the only recommended management strategy is pacing [ 34 ]. In the years between changes to these guidelines, the landmark PACE trial [ 35 ] was published in 2011. This large, randomised control trial (RCT; n = 639) compared pacing with CBT and reported GET and CBT were more effective than pacing for improving symptoms. Yet, this study has come under considerable criticism from patient groups and clinicians alike [ 36 , 37 , 38 , 39 ]. This may partly explain why NICE do not advise CBT or GET as of 2021, and only recommend pacing for symptom management people with ME/CFS [ 34 ]. There has been some controversy over best treatment for people with ME/CFS in the literature and support groups, potentially amplified by the ambiguity of evidence for pacing efficacy and how pacing should be implemented. As such, before pacing can be advised for people with long COVID, it is imperative previous literature concerning pacing is systematically reviewed. This is because a consensus is needed within the literature for implementing pacing so practitioners treating people with ME/CFS or long COVID can do so effectively. A lack of agreement in pacing implementation is a barrier to adoption for both practitioners and patients. Despite several systematic reviews concerning pharmacological interventions or cognitive behavioural therapy in people with ME/CFS [ 36 , 40 , 41 ], to date, there are no systematic reviews concerning pacing.
Despite the widespread use of pacing, the literature base is limited and includes clinical commentaries, case studies, case series, and few randomised control trials. Consequently, while a comprehensive review of the effects of pacing in ME/CFS is an essential tool to guide symptom management advice, the available literature means that effective pooling of data is not feasible [ 42 ] and therefore, a traditional systematic review and meta-analysis, with a tightly focussed research question would be premature [ 43 ]. Consequently, we elected to undertake a scoping review. This approach retains the systematic approach to literature searching but aims to map out the current state of the research [ 43 ]. Using the framework of Arksey and O'Malley [ 44 ], a scoping review aims to use a broad set of search terms and include a wide range of study designs and methods (in contrast to a systematic review [ 44 ]). This approach, has the benefit of clarifying key concepts, surveying current data collection approaches, and identifying critical knowledge gaps.
We aimed to provide an overview of existing literature concerning pacing in ME/CFS. Our three specific objectives of this scoping review were to (1) conduct a systematic search of the published literature concerning ME/CFS and pacing, (2) map characteristics and methodologies used, and (3) provide recommendations for the advancement of the research area.
Protocol and registration
The review was conducted and reported according to the Preferred Reporting Items for Systematic Reviews and Meta-Analyses extension for scoping reviews (PRISMA-ScR) guidelines [ 45 ] and the five-stage framework outlined in Arksey and O’Malley [ 44 ]. Registration is not recommended for scoping reviews.
Studies that met the following criteria were included in this review: (1) published as a full-text manuscript; (2) not a review; (3) participants with ME/CFS; (4) studies employed a pacing intervention or retrospective analysis of pacing or a case study of pacing. Studies utilising sub-analysis of the pacing, graded activity, and cognitive behaviour therapy: a randomised evaluation (PACE) trial were included as these have different outcome measures and, as this is not a meta-analysis, this will not influence effect size estimates. Additionally, due to the paucity of evidence, grey literature has also been included in this review.
Search strategy
The search strategy consisted of a combination of free-text and MeSH terms relating to ME/CFS and pacing, which were developed through an examination of published original literature and review articles. Example search terms for PubMed included: ‘ME/CFS’ OR ‘ME’ OR ‘CFS’ OR ‘chronic fatigue syndrome’ OR ‘PEM’ OR ‘post exertional malaise’ OR ‘pene’ OR ‘post-exertion neurogenic exhaust’ AND ‘pacing’ OR ‘adaptive pacing’. The search was performed within title/abstract. Full search terms can be found in Additional file 1 .
Information sources
Six electronic databases [PubMed, Scholar, ScienceDirect, Scopus, Web of Science, and the Cochrane Central Register of Controlled Trials (CENTRAL)] were searched to identify original research articles published from the earliest available date up until 02/02/2022. Additional records were identified through reference lists of included studies. ‘Grey literature’ repositories including MEPedia, Action for ME, and ME Action were also searched with the same terms.
Study selection and data items
Once each database search was completed and manuscripts were sourced, all studies were downloaded into a single reference list (Zotero, version 6.0.23) and duplicates were removed. Titles and abstracts were screened for eligibility by two reviewers independently and discrepancies were resolved through discussion between reviewers. Subsequently, full text papers of potentially relevant studies were retrieved and assessed for eligibility by the same two reviewers independently. Any uncertainty by reviewers was discussed in consensus meetings and resolved by agreement. Data extracted from each study included sample size, participant characteristics, study design, trial registration details, study location, pacing description (type), intervention duration, intervention adherence, outcome variables, and main outcome data. Descriptions were extracted with as much detail as was provided by the authors. Study quality was assessed using the Physiotherapy Evidence Database (PEDro) scale [ 46 , 47 ].
Role of the funding source
The study sponsors had no role in study design, data collection, analysis, or interpretation, nor writing the report, nor submitting the paper for publication.
Study selection
After the initial database search, 281 records were identified (see Fig. 1 ). Once duplicates were removed, 177 titles and abstracts were screened for inclusion resulting in 22 studies being retrieved as full text and assessed for eligibility. Of those, five were excluded, and 17 articles remained and were used in the final qualitative synthesis.
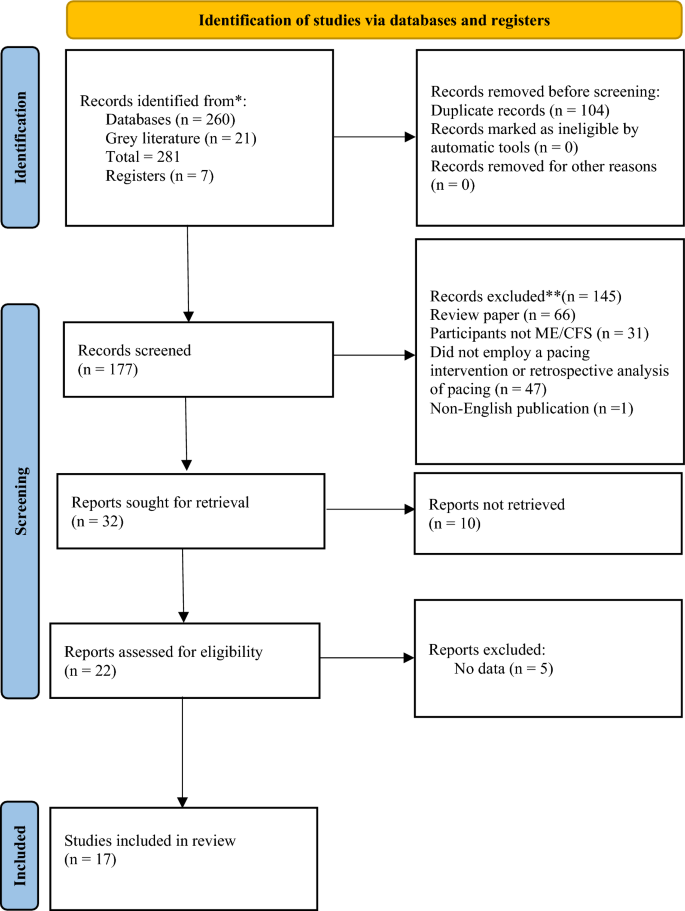
Schematic flow diagram describing exclusions of potential studies and final number of studies. RCT = randomized control trial. CT = controlled trial. UCT = uncontrolled trial
Study characteristics
Study characteristics are summarised in Table 1 . Of the 17 studies included, three were randomised control trials (RCTs [ 35 , 48 , 49 ]); one was an uncontrolled trial [ 50 ]; one was a case series [ 51 ]; one was a retrospective observational study [ 52 ], two were prospective observational studies [ 53 , 54 ]; four were cross-sectional observational studies [ 25 , 55 , 56 ]; and five were cross-sectional analytical studies [ 57 , 58 , 59 , 60 , 61 ] including sub-analysis of the PACE trial [ 35 , 56 , 59 , 61 ]. Seven of the studies were registered trials [ 35 , 48 , 49 , 50 , 56 , 57 , 58 ]. Diagnostic criteria for ME/CFS are summarised in Table 2 .
Types of pacing
Pacing interventions.
Of the 17 studies included, five implemented their own pacing interventions and will be discussed in this section. Sample sizes ranged from n = 7 in an interventional case series [ 51 ] to n = 641 participants in the largest RCT [ 35 ]. The first of these five studies considered an education session on pacing and self-management as the ‘pacing’ group, and a ‘pain physiology education’ group as the control group [ 49 ]. Two studies included educational sessions provided by a therapist plus activity monitoring via ActiGraph accelerometers [ 51 ] and diaries [ 48 ] at baseline and follow-up. In the first of these two studies, Nijs and colleagues [ 51 ] implemented a ‘self-management program’ which asked patients to estimate their current physical capabilities prior to commencing an activity and then complete 25–50% less than their perceived energy envelope. They[ 51 ] did not include a control group and had a sample size of only n = 7. Six years later, the same research group [ 48 ] conducted another pacing study which utilised relaxation as a comparator group (n = 12 and n = 14 in the pacing and relaxation groups, respectively). The pacing group underwent a pacing phase whereby participants again aimed to complete 25–50% less than their perceived energy envelope, followed by a gradual increase in exercise after the pacing phase (the total intervention spanned three weeks, and it is unclear how much was allocated to pacing, and how much to activity increase). Therefore, it could be argued that Kos et al. [ 48 ] really assessed pacing followed by a gradual exercise increase as outcome measures were assessed following the graded activity phase. Another pacing intervention delivered weekly educational sessions for six weeks and utilised a standardised rehabilitation programme using the ‘activity pacing framework’ [ 50 ] in a single-arm, no comparator group feasibility study. Finally, the PACE trial adopted an adaptive pacing therapy intervention consisting of occupational therapists helping patients to plan and pace activities utilising activity diaries to identify activities associated with fatigue and staying within their energy envelope [ 35 ]. This study incorporated standard medical care, cognitive behavioural therapy (CBT) and graded exercise therapy (GET) as comparator groups [ 35 ]. It is worth noting that the pacing group and the CBT group were both ‘encouraged’ to increase physical activity levels as long as participants did not exceed their energy envelope. Although not all five intervention studies explicitly mentioned the “Energy Envelope Theory”, which dictates that people with ME/CFS should not necessarily increase or decrease their activity levels, but moderate activity and practice energy conservation [ 62 ], all intervention studies used language analogous to this theory, such as participants staying within limits, within capacity, or similar.
The interventions included in this review were of varying durations, from a single 30-min education session [ 49 ], a 3-week (one session a week) educational programme [ 51 ], a 3-week (3 × 60–90 min sessions/week) educational programme [ 48 ], a 6-week rehabilitation programme [ 50 ], to a 24-week programme [ 35 ]. Intervention follow-up durations also varied across studies from immediately after [ 49 ], 1-week [ 51 ], 3-weeks [ 48 ], 3-months [ 50 ], and 1-year post-intervention [ 35 ].
Observational studies of pacing
Eight studies were observational and, therefore, included no intervention. Observational study sample sizes ranged from 16 in a cross-sectional interview study [ 25 ] to 1428 in a cross-sectional survey [ 52 ]. One study involved a retrospective analysis of participants’ own pacing strategies varying from self-guided pacing or pacing administered by a therapist compared with implementation of CBT and GET [ 52 ]. Five involved a cross-sectional analysis of participants own pacing strategies which varied from activity adjustment, planning and acceptance [ 50 , 55 ], and the Energy Envelope method [ 58 , 60 ]. Two studies were prospective observational studies investigating the Energy Envelope theory [ 53 , 54 ]. Four studies [ 56 , 57 , 59 , 61 ] included in this review involved sub-analysis of results of the PACE trial [ 35 ].
Outcome measures
Quantitative health outcomes.
ME/CFS severity and general health status were the most common outcome measures across studies (16/17) [ 35 , 48 , 49 , 50 , 51 , 52 , 53 , 54 , 55 , 56 , 57 , 58 , 59 , 60 , 61 , 63 ]. Studies utilised different instruments, including the Short-Form 36 (SF-36; 8/16) [ 35 , 51 , 53 , 54 , 56 , 57 , 58 , 60 ], SF-12 (2/16) [ 50 , 63 ], ME symptom and illness severity (2/16) [ 52 , 55 ], Patient health (PHQ-15; 1/16) [ 59 ], DePaul symptom questionnaire (DSQ; 1/16) [ 58 ], and the Patient health questionnaire-9 (1/16) [ 50 ]. Additionally, some studies used diagnostic criteria for ME/CFS as an outcome measure to determine recovery [ 57 , 59 , 61 ].
Pain was assessed by most included studies (11/17) [ 35 , 49 , 50 , 51 , 53 , 54 , 55 , 57 , 59 , 60 , 61 , 63 ]. Two studies [ 59 , 61 ] included the international CDC criteria for CFS which contain five painful symptoms central to a diagnosis of CFS: muscle pain and joint pain. Other methods of assessment included Brief Pain Inventory (1/11) [ 53 ], Chronic Pain Coping Inventory (CPCI; 1/11) [ 49 ], Pain Self Efficacy Questionnaire (PSEQ; 1/11) [ 50 ], Tampa Scale for Kinesiophobia–version CFS (1/11) [ 49 ], algometry (1/11) [ 49 ], Knowledge of Neurophysiology of Pain Test (1/12) [ 49 ], Pain Catastrophizing Scale (1/11) [ 49 ], Pain Anxiety Symptoms Scale short version (PASS-20; 1/11) [ 50 ], Pain Numerical Rating Scale (NRS; 1/11) [ 63 ].
Fatigue or post-exertional malaise was assessed by 11 of the 17 studies [ 35 , 48 , 50 , 51 , 53 , 54 , 56 , 57 , 60 , 61 , 63 ]. Again, measurement instruments were divergent between studies and included the Chalder Fatigue Questionnaire (CFQ; 4/11) [ 35 , 50 , 57 , 63 ], Fatigue Severity Scale (2/11) [ 53 , 60 ], the Chronic Fatigue Syndrome Medical Questionnaire (1/11) [ 60 ], and Checklist Individual Strength (CIS; 2/11) [ 48 , 51 ].
Anxiety and depression were also common outcome measures, utilised by four studies (4/17) [ 50 , 53 , 59 , 63 ]. These were also assessed using different instruments including Hospital Anxiety and Depression Scale (HADS; 2/4) [ 59 , 63 ], Generalised Anxiety Disorder Assessment (1/4 [ 50 ]), Beck Depression Inventory (BDI-II; 1/4) [ 53 ], Beck Anxiety Inventory (BAI; 1/4) [ 53 ], and Perceived Stress Scale (PSS; 1/4) [ 53 ].
Outcome measures also included sleep (2/17) [ 53 , 59 ], assessed by The Pittsburgh Sleep Quality Index (1/2) [ 53 ] and Jenkins sleep scale (1/2) [ 59 ]; and quality of life (2/17) [ 50 , 53 ] as assessed by the EuroQol five-dimensions, five-levels (EQ-5D-5L; 1/2) [ 50 ] and The Quality-of-Life Scale (1/2) [ 53 ]. Self-Efficacy was measured in four studies [ 50 , 53 , 59 , 60 ], assessed by the Brief Coping Orientation to Problems Experienced Scale (bCOPE; 1/4) [ 60 ] and the Chronic Disease Self-Efficacy measure (3/4) [ 50 , 53 , 59 ].
Quantitative evaluation of pacing
Some studies (4/17) [ 25 , 50 , 52 , 63 ] included assessments of the participants’ experiences of pacing, using the Activity Pacing Questionnaire (APQ-28; 1/4 [ 50 ], APQ-38 (2/4) [ 25 , 63 ]), a re-analysis of the 228 question survey regarding treatment (1/4) [ 52 ] originally produced by the ME Association [ 55 ], and qualitative semi-structured telephone interviews regarding appropriateness of courses in relation to individual patient needs (1/4) [ 25 ]. The APQ-28 and -38 have been previously validated, but the 228-question survey has not. When outcome measures included physical activity levels (4/17), the Canadian Occupational Performance Measure (COPM) was used in two studies [ 48 , 51 ], and two studies used accelerometers to record physical activity [ 51 , 54 ]. Of these two studies, Nijs [ 51 ] examined accelerometery after a 3-week intervention based on the Energy Envelope Theory and Brown et al. [ 54 ] evaluated the Energy Envelope Theory of pacing over 12 months.
Other outcomes
Two [ 53 , 59 ] of the 17 studies included structured clinical interviews for the Diagnostic and Statistical Manual of Mental Disorders, 4th edition (DSM-IV) to assess psychiatric comorbidity and psychiatric exclusions. One study included a disability benefits questionnaire [ 55 ], and one study included employment and education questionnaire [ 55 ]. Additionally, satisfaction of primary care was also used as an outcome measure (2/17) [ 25 , 55 ] assessed using the Chronic Pain Coping Inventory (CPCI).
Efficacy of pacing interventions
The majority of studies (12/17) [ 25 , 48 , 50 , 51 , 52 , 53 , 54 , 55 , 56 , 58 , 60 , 63 ] highlighted improvements in at least one outcome following pacing (Fig. 2 ). When the effect of pacing was assessed by ME symptomology and general health outcomes, studies reported pacing to be beneficial [ 25 , 50 , 51 , 53 , 54 , 55 , 56 , 58 ]. It is worth noting however that pacing reportedly worsened ME symptoms in 14% of survey respondents, whilst improving symptoms in 44% of respondents [ 52 ]. Most studies using fatigue as an outcome measure reported pacing to be efficacious (7/10) [ 50 , 51 , 53 , 54 , 56 , 60 , 63 ]. However, one study reported no change in fatigue with a pacing intervention (1/10) [ 35 ], and 2/10 studies [ 53 , 63 ] reported a worsening of fatigue with pacing. Physical function was used to determine the efficacy of pacing in 11 studies [ 35 , 48 , 50 , 51 , 53 , 54 , 56 , 58 , 59 , 60 , 63 ]. Of these, the majority found pacing improved physical functioning (8/10) [ 48 , 50 , 51 , 53 , 54 , 56 , 58 , 60 ], with 1/10 [ 35 ] studies reporting no change in physical functioning, and 1/10 [ 59 ] reporting a worsening of physical functioning from pre- to post-pacing. Of the seven studies [ 35 , 49 , 50 , 51 , 53 , 54 , 60 ] which used pain to assess pacing efficacy, 4/7 [ 50 , 51 , 53 , 60 ] reported improvements in pain and 3/7 [ 35 , 51 , 53 ] reported no change in pain scores with pacing. All studies reporting quality of life (1/1) [ 53 ], self-efficacy (3/3) [ 50 , 53 , 59 ], sleep (2/2) [ 53 , 59 ], and depression and anxiety (4/4) [ 50 , 53 , 59 , 63 ], found pacing to be efficacious for ME/CFS participants.
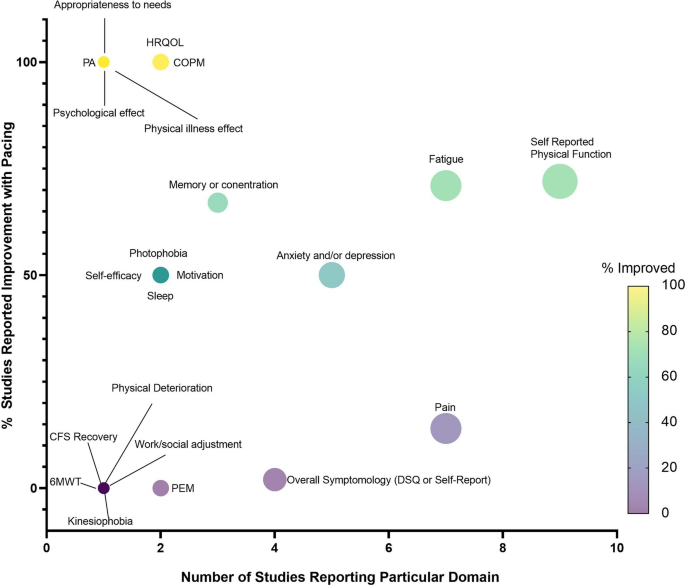
Bubble plot displaying number of studies reporting each domain (x-axis) and the percentage of studies reporting improvement with pacing (y-axis), including a coloured scale of improvement from 0–100%. PEM = post-exertional malaise, 6MWT = 6-min walk time, CFS = chronic fatigue syndrome, DSQ = DePaul Symptom Questionnaire, PA = Physical Activity, HRQOL = Health-related quality of life, COPM = The Canadian Occupational Performance Measure
Participant characteristics
The majority of studies (10/17) [ 25 , 50 , 52 , 53 , 54 , 58 , 59 , 60 , 61 , 63 ] did not report age of the participants. For those which did report age, this ranged from 32 ± 14 to 43 ± 13 years. Where studies reported sex (11/17) [ 35 , 48 , 49 , 50 , 51 , 54 , 55 , 56 , 57 , 58 , 60 ], this was predominantly female, ranging from 75 to 100% female. Only six studies [ 35 , 54 , 56 , 57 , 58 , 60 ] reported ethnicity, with cohorts predominantly Caucasian (94–98%). Time since diagnosis was mostly unreported (12/17) [ 25 , 48 , 49 , 50 , 52 , 53 , 54 , 58 , 59 , 60 , 61 , 63 ] but ranged from 32 to 96 months, with a cross-sectional survey reporting 2% of the participants were diagnosed 1–2 years previously; 6% 3–4 years since diagnosis; 13% 3–4 years since diagnosis; 12% 5–6 years since diagnosis; 20% 7–10 years since diagnosis; 29% 11–21 years since diagnosis; 13% 21–30 years since diagnosis; and 5% > 30 years since diagnosis. Of the studies which reported comorbidities of the participants (6/17) [ 25 , 35 , 50 , 56 , 57 , 63 ], the comorbidities were chronic pain, depressive disorder, psychiatric disorder.
Study location
Of the 17 studies, 14 were from Europe [ 25 , 35 , 48 , 49 , 50 , 51 , 52 , 55 , 56 , 57 , 58 , 59 , 61 , 63 ], and three from North America [ 53 , 54 , 60 ]. Of the 14 studies[ 25 , 35 , 48 , 49 , 50 , 51 , 52 , 55 , 56 , 57 , 58 , 59 , 61 , 63 ] from Europe, ten [ 25 , 35 , 50 , 52 , 55 , 56 , 57 , 58 , 59 , 61 , 63 ] were conducted in the United Kingdom, three in Belgium [ 48 , 49 , 51 ], and one was a multicentred study between the United Kingdom and Norway [ 58 ].
Recruitment strategy
Of the 17 studies, three [ 53 , 54 , 60 ] used announcements in a newspaper and physician referrals to recruit participants, two [ 50 , 63 ] recruited patients referred by a consultant from a National Health Service (NHS) Trust following a pain diagnosis, two [ 52 , 55 ] concerned online platforms on the web, two [ 59 , 61 ] recruited from secondary care clinics, and two used the PACE trial databases [ 56 , 57 ]. Moreover, one study recruited from the hospital [ 58 ], one from physiotherapist referrals [ 25 ], two from specialist clinic centres [ 35 , 64 ], one from waiting list of rehabilitation centre [ 48 ], and one from medical files [ 49 ].
Study settings
Ten studies were carried out in hospital and clinic setting [ 25 , 35 , 48 , 49 , 50 , 51 , 58 , 59 , 61 , 63 ]. Two studies were performed on online platforms [ 52 , 55 ]. Three studies did not report study setting [ 53 , 54 , 60 ]. Two studies generated output from PACE trial databases [ 56 , 57 ]
Adherence and feasibility
All five intervention studies reported adherence rates (which they defined as number of sessions attended), which ranged from 4–44% (4% [ 49 ], 8% [ 35 ], 25% [ 48 ], 29% [ 51 ], and 44% [ 50 ]). One study reported the median number of rehabilitation programme sessions attended was five out of six possible sessions, with 58.9% [ 50 ] participants attending ≥ 5 sessions; 83.2% participants attending at least one educational session on activity pacing and 56.1% attending both activity pacing sessions.
This scoping review summarises the existing literature, with a view to aid physicians and healthcare practitioners better summarise evidence for pacing in ME/CFS and use this knowledge for other post-viral fatiguing conditions. Overall, studies generally reported pacing to be beneficial for people with ME/CFS. The exception to this trend is the controversial PACE trial [ 36 , 37 , 38 , 39 ], which we will expand on in subsequent sections. We believe information generated within this review can facilitate discussion of research opportunities and issues that need to be addressed in future studies concerning pacing, particularly given the immediate public health issue of the long COVID pandemic. As mentioned, we found some preliminary evidence for improved symptoms following pacing interventions or strategies. However, we wish to caution the reader that the current evidence base is extremely limited and hampered by several limitations which preclude clear conclusions on the efficacy of pacing. Firstly, studies were of poor to fair methodological quality (indicated by the PEDro scores), often with small sample sizes, and therefore unknown power to detect change. Moreover, very few studies implemented pacing, with most studies merely consulting on people’s views on pacing. This may of course lead to multiple biases such as reporting, recruitment, survivorship, confirmation, availability heuristic, to name but a few. Thus, there is a pressing need for more high-quality intervention studies. Secondly, the reporting of pacing strategies used was inconsistent and lacked detail, making it difficult to describe current approaches, or implement them in future research or symptom management strategies. Furthermore, outcome evaluations varied greatly between studies. This prevents any appropriate synthesis of research findings.
The lack of evidence concerning pacing is concerning given pacing is the only NICE recommended management strategy for ME/CFS following the 2021 update [ 34 ]. Given the analogous nature of long COVID with ME/CFS, patients and practitioners will be looking to the ME/CFS literature for guidance for symptom management. There is an urgent need for high quality studies (such as RCTs) investigating the effectiveness of pacing and better reporting of pacing intervention strategies so that clear recommendations can be made to patients. If this does not happen soon, there will be serious healthcare and economic implications for years to come [ 65 , 66 ].
Efficacy of pacing
Most studies (12/17) highlighted improvements in at least one outcome measure following pacing. Pacing was self-reported to be the most efficacious, safe, acceptable, and preferred form of activity management for people with ME/CFS [ 55 ]. Pacing was reported to improve symptoms and improve general health outcomes [ 25 , 50 , 52 , 58 , 63 ], fatigue and PEM [ 48 , 50 , 51 , 53 , 54 , 55 , 56 , 60 , 63 ], physical functioning [ 48 , 50 , 51 , 53 , 56 , 58 , 60 , 63 ], pain [ 25 , 50 , 55 , 63 ], quality of life [ 50 ], self-efficacy [ 50 , 53 ], sleep [ 53 , 55 ], and depression and anxiety [ 50 , 53 , 63 ]. These positive findings provide hope for those with ME/CFS, and other chronic fatiguing conditions such as long COVID, to improve quality of life through symptom management.
Conversely, some studies reported no effects of pacing on ME/CFS symptoms [ 52 ], fatigue, physical functioning [ 35 ], or pain scores [ 49 , 61 ]. Some studies even found pacing to have detrimental effects in those with ME/CFS, including a worsening of symptoms in 14% of survey participants recalling previous pacing experiences [ 52 ]. Furthermore, a worsening of fatigue [ 35 , 59 ], and physical functioning from pre- to post-pacing [ 35 , 57 , 59 , 61 ] was reported by the PACE trial and sub-analysis of the PACE trial [ 56 , 57 , 61 ]. The PACE trial [ 35 ], a large RCT (n = 639) comparing pacing with CBT and GET, reported GET and CBT were more effective for reducing ME/CFS-related fatigue and improving physical functioning than pacing. However, the methodology and conclusions from the PACE trial have been heavily criticised, mainly due to the authors lowering the thresholds they used to determine improvement [ 36 , 37 , 38 , 67 ]. With this in mind, Sharpe et al. [ 56 ] surveyed 75% of the participants from the PACE trial 1-year post-intervention and reported pacing improved fatigue and physical functioning, with effects similar to CBT and GET.
Lessons for pacing implementation
All pacing intervention studies (5/5) implemented educational or coaching sessions. These educational components were poorly reported in terms of the specific content and how and where they had been developed, with unclear pedagogical approaches. Consequently, even where interventions reported reduction in PEM or improved symptoms, it would be impossible to transfer that research into practice, future studies, or clinical guidance, given the ambiguity of reporting. Sessions typically contained themes of pacing such as activity adjustment (decrease, break-up, and reschedule activities based on energy levels), activity consistency (maintaining a consistently low level of activity to prevent PEM), activity planning (planning activities and rest around available energy levels), and activity progression (slowly progressing activity once maintaining a steady baseline) [ 35 , 48 , 49 , 50 , 51 ]. We feel it is pertinent to note here that although activity progression has been incorporated as a pacing strategy in these included studies, some view activity progression as a form of GET. The NICE definition of GET is “first establishing an individual's baseline of achievable exercise or physical activity, then making fixed incremental increases in the time spent being physically active” [ 34 ]. Thus, this form of pacing can also be considered a type of ‘long-term GET’ in which physical activity progression is performed over weeks or months with fixed incremental increases in time spent being physically.
Intervention studies attempted to create behaviour change, through educational programmes to modify physical activity, and plan behaviours. However, none of these studies detailed integrating any evidence-based theories of behaviour change [ 68 ] or reported using any frameworks to support behaviour change objectives. This is unfortunate since there is good evidence that theory-driven behaviour change interventions result in greater intervention effects [ 69 ]. Indeed, there is a large body of work regarding methods of behaviour change covering public health messaging, education, and intervention design, which has largely been ignored by the pacing literature. Interventions relied on subjective pacing (5/5 studies), with strategies including keeping an activity diary (3/5 studies) to identify links between activity and fatigue [ 35 , 48 , 50 ]. Given the high prevalence of ‘brain fog’ within ME/CFS [ 70 , 71 , 72 , 73 ], recall may be extremely difficult and there is significant potential for under-reporting. Other strategies included simply asking participants to estimate energy levels available for daily activities (2/5 studies [ 48 , 51 ]). Again, this is subjective and relies on participants’ ability to recall previous consequences of the activity. Other methods of activity tracking and measuring energy availability, such as wearable technology [ 74 , 75 , 76 , 77 , 78 ] could provide a more objective measure of adherence and pacing strategy fidelity in future studies. Despite technology such as accelerometers being widely accessible since well-before the earliest interventional study included in this review (which was published in 2009), none of the interventional studies utilised objective activity tracking to track pacing and provide feedback to participants. One study considered accelerometery alongside an activity diary [ 51 ]. However, accelerometery was considered the outcome variable, to assess change in activity levels from pre- to post-intervention and was not part of the intervention itself (which was one pacing coaching sessions per week for 3 weeks). Moreover, most research-grade accelerometers cannot be used as part of the intervention since they have no ability to provide continuous feedback and must be retrieved by the research team in order to access any data. Consequently, their use is mostly limited to outcome assessments only. As pacing comprises a limit to physical activity to prevent push-crash cycles, it is an astonishing observation from this scoping review that only two studies objectively measured physical activity to quantify changes to activity as a result of pacing [ 51 , 54 ]. If the aim of pacing is to reduce physical activity, or reduce variations in physical activity (i.e., push-crash cycles), only two studies have objectively quantified the effect pacing had on physical activity, so it is unclear whether pacing was successfully implemented in any of the other studies.
By exploring the pacing strategies previously used, in both intervention studies and more exploratory studies, we can identify and recommend approaches to improve symptoms of ME/CFS. These approaches can be categorised as follows: activity planning, activity consistency, activity progression, activity adjustment and staying within the Energy Envelope [ 50 , 53 , 60 , 63 ]. Activity planning was identified as a particularly effective therapeutic strategy, resulting in improvement of mean scores of all symptoms included in the APQ-28, reducing current pain, improvement of physical fatigue, mental fatigue, self-efficacy, quality of life, and mental and physical functioning [ 50 ]. Activity planning aligns with the self-regulatory behaviour change technique ‘Action Planning’ [ 79 ] which is commonly used to increase physical activity behaviour. In the case of ME/CFS, activity planning is successfully used to minimise rather than increase physical activity bouts to prevent expending too much energy and avoid PEM. Activity consistency, meaning undertaking similar amounts of activity each day, was also associated with reduced levels of depression, exercise avoidance, and higher levels of physical function [ 63 ]. Activity progression was associated with higher levels of current pain. Activity adjustment associated with depression and avoidance, and lower levels of physical function [ 63 ]. Staying within the Energy Envelope was reported to reduce PEM severity [ 53 , 60 ], improve physical functioning [ 53 , 60 ] and ME/CFS symptom scores [ 53 ], and more hours engaged in activity than individuals with lower available energy [ 53 ]. These results suggest that effective pacing strategies would include activity planning, consistency, and energy management techniques while avoiding progression. This data is, of course, limited by the small number of mostly low-quality studies and should be interpreted with some caution. Nevertheless, these are considerations that repeatedly appear in the literature and, as such, warrant deeper investigation. In addition, and as outlined earlier, most studies are relatively old, and we urgently need better insight into how modern technologies, particularly longitudinal activity tracking and contemporaneous heart-rate feedback, might improve (or otherwise) adaptive pacing. Such longitudinal tracking would also enable activities and other behaviours (sleep, diet, stress) to be linked to bouts of PEM. Linking would enable a deeper insight into potential PEM triggers and mitigations that might be possible.
The PACE trial
We feel it would be remiss of us to not specifically address the PACE trial within this manuscript, as five of the 17 included studies resulted from the PACE trial [ 35 , 56 , 57 , 59 , 61 ]. There has been considerable discussion around the PACE trial, which has been particularly divisive and controversial [ 37 , 38 , 39 , 59 , 67 , 80 , 81 ]. In the PACE trial, GET and CBT were deemed superior to pacing by the authors. Despite its size and funding, the PACE trial has received several published criticisms and rebuttals. Notably, NICE's most recent ME/CFS guideline update removed GET and CBT as suggested treatment options, which hitherto had been underpinned by the PACE findings. While we will not restate the criticisms and rebuttals here, what is not in doubt, is that the PACE trial has dominated discussions of pacing, representing almost a third of all the studies in this review. However, the trial results were published over a decade ago, with the study protocol devised almost two decades ago [ 82 ]. The intervening time has seen a revolution in the development of mobile and wearable technology and an ability to remotely track activity and provide real-time feedback in a way which was not available at that time. Furthermore, there has been no substantive research since the PACE trial that has attempted such work. Indeed, possibly driven by the reported lack of effect of pacing in the PACE trial, this review has demonstrated the dearth of progress and innovation in pacing research since its publication. Therefore, regardless of its findings or criticisms, the pacing implementation in the PACE trial is dated, and there is an urgent need for more technologically informed approaches to pacing research.
Limitations of the current evidence
The first limitation to the literature included in this scoping review is that not all studies followed the minimum data set (MDS) of patient-reported outcome measures (PROMs) agreed upon by the British Association of CFS/ME Professionals (BACME) (fatigue, sleep quality, self-efficacy, pain/discomfort, anxiety/depression, mobility, activities of daily living, self-care, and illness severity) [ 83 , 84 ]. All but one study included in this review measured illness severity, most studies included fatigue and pain/discomfort, and some studies included assessments of anxiety/depression. There was a lack of quantitative assessment of sleep quality, self-efficacy, mobility, activities of daily living, and self-care. Therefore, studies did not consistently capture the diverse nature of the symptoms experienced, with crucial domains missing from the analyses. The MDS of PROMs were established in 2012 [ 83 , 84 ] and therefore, for studies published out prior to 2012, these are not applicable [ 35 , 49 , 51 , 53 , 54 ]. However, for the 12 studies carried out after this time, the MDS should have been considered elucidate the effects of pacing on ME/CFS. Importantly, despite PEM being a central characteristic of ME/CFS, only two studies included PEM as an outcome measure [ 55 , 60 ]. This may be because of the difficulty of accurately measuring fluctuating symptoms, as PEM occurs multiple times over a period of months, and therefore pre- to post- studies and cross-sectional designs cannot adequately capture PEM incidence. Therefore, it is likely studies opted for measuring general fatigue instead. More appropriate longitudinal study designs are required to track PEM over time to capture a more representative picture of PEM patterns. Secondly, reporting of participant characteristics was inadequate, but in the studies that did describe participants, characteristics were congruent with the epidemiological literature and reporting of ME/CFS populations (i.e., 60–65% female) [ 85 ]. Therefore, in this respect, studies included herein were representative samples. However, the lack of reporting of participant characteristics limits inferences we can draw concerning any population-related effects (i.e. whether older, or male, or European, or people referred by a national health service would be more or less likely to respond positively to pacing). Thirdly, comparison groups (where included) were not ideal, with CBT or GET sometimes used as comparators to pacing [ 35 ], and often no true control group included. Penultimately, there is a distinct lack of high-quality RCTs (as mentioned throughout this manuscript). Finally, in reference to the previous section, inferences from the literature are dated and do not reflect the technological capabilities of 2023.
Recommendations for advancement of the investigative area
It is clear from the studies included in this scoping review for the last decade or more, progress and innovation in pacing research have been limited. This is unfortunate for several reasons. People with ME/CFS or long COVID are, of course, invested in their recovery. From our patient and public involvement (PPI) group engagement, it is clear many are ahead of the research and are using wearable technology to track steps, heart rate, and, in some cases, heart rate variability to improve their own pacing practice. While the lack of progress in the research means this is an understandable response by patients, it is also problematic. Without underpinning research, patients may make decisions based on an individual report of trial-and-error approaches given the lack of evidence-based guidance.
A more technologically-informed pacing approach could be implemented by integrating wearable trackers [ 77 , 78 , 86 , 87 ] to provide participants with live updates on their activity and could be integrated with research-informed messaging aimed at supporting behaviour change, as has been trialled in other research areas [ 88 , 89 , 90 , 91 ]. However, more work is needed to evaluate how to incorporate wearable activity trackers and which metrics are most helpful.
A more technologically-informed approach could also be beneficial for longitudinal symptom tracking, particularly useful given the highly variable symptom loads of ME/CFS and episodic nature of PEM. This would overcome reliance on assessments at a single point in time (as the studies within this review conducted). Similarly, mobile health (mHealth) approaches also allow questionnaires to be digitised to make it easier for participants to complete if they find holding a pen or reading small font problematic [ 92 ]. Reminders and notifications can also be helpful for patients completing tasks [ 77 , 93 , 94 , 95 ]. This approach has the added advantage of allowing contemporaneous data collection rather than relying on pre- to post-intervention designs limited by recall bias. Future work must try to leverage these approaches, as unless we collect large data sets on symptoms and behaviours (i.e. activity, diet, sleep, and pharmacology) in people with conditions like ME/CFS we will not be able to leverage emerging technologies such as AI and machine learning to improve the support and care for people with these debilitating conditions. The key areas for research outline in the NICE guidelines (2021 update) speaks to this, with specific mention of improved self-monitoring strategies, sleep strategies, and dietary strategies, all of which can be measured using mHealth approaches, in a scalable and labour-inexpensive way.
The potential for existing pacing research to address the long COVID pandemic
There is now an urgent public health need to address long COVID, with over 200 million sufferers worldwide [ 30 ]. Given the analogous symptomology between ME/CFS and long COVID, and the lack of promising treatment and management strategies in ME/CFS, pacing remains the only strategy for managing long COVID symptoms. This is concerning as the quality of evidence to support pacing is lacking. Given long COVID has reached pandemic proportions, scalable solutions will be required. In this context, we propose that technology should be harnessed to a) deliver, but also b) evaluate, pacing. We recently reported on a just-in-time adaptive intervention to increase physical activity during the pandemic [ 78 ]. However, this method could be adapted to decrease or maintain physical activity levels (i.e., pacing) in long COVID. This method has the advantage of scalability and remote data collection, reducing resource commitments and participant burden, essential for addressing a condition with so many sufferers.
This review highlights the need for more studies concerning pacing in chronic fatiguing conditions. Future studies would benefit from examining pacing’s effect on symptomology and PEM with objectively quantified pacing, over a longer duration of examination, using the MDS. It is essential this is conducted as an RCT, given that in the case of long COVID, participants may improve their health over time, and it is necessary to determine whether pacing exerts an additional effect over time elapsing. Future studies would benefit from digitising pacing to support individuals with varying symptom severity and personalise support. This would improve accessibility and reduce selection bias, in addition to improving scalability of interventions. Finally, clinicians and practitioners should be cognisant of the strength of evidence reported in this review and should exert caution when promoting pacing in their patients, given the varying methods utilised herein.
Availability of data and materials
The datasets used and/or analysed during the current study are available from the corresponding author on reasonable request.
Abbreviations
Activity Pacing Questionnaire
Beck Anxiety Inventory
Beck Depression Inventory
Brief Coping Orientation to Problems Experienced Scale
Canadian Occupational Performance Measure
Centers for disease control and prevention
Chalder Fatigue Questionnaire
Checklist Individual Strength
Chronic Pain Coping Inventory
Cognitive behavioural therapy
Cochrane Central Register of Controlled Trials
DePaul symptom questionnaire
EuroQol five-dimensions, five-levels questionnaire
Graded exercise therapy
Hospital Anxiety and Depression Scale
Myalgic encephalomyelitis/chronic fatigue syndrome
Pain Self Efficacy Questionnaire
Pain Anxiety Symptoms Scale short version
Pain Numerical Rating Scale
Patient health questionnaire
Patient reported outcome measures
Physiotherapy Evidence Database
Perceived Stress Scale
Post exertional malaise
Preferred Reporting Items for Systematic Reviews and Meta-Analyses extension for scoping reviews
Randomised control trial
McMurray JC, May JW, Cunningham MW, Jones OY. Multisystem Inflammatory Syndrome in Children (MIS-C), a post-viral myocarditis and systemic vasculitis-a critical review of its pathogenesis and treatment. Front Pediatr. 2020;8: 626182.
Article PubMed PubMed Central Google Scholar
Perrin R, Riste L, Hann M, Walther A, Mukherjee A, Heald A. Into the looking glass: post-viral syndrome post COVID-19. Med Hypotheses. 2020;144: 110055.
Article PubMed PubMed Central CAS Google Scholar
Hayes LD, Ingram J, Sculthorpe NF. More than 100 persistent symptoms of SARS-CoV-2 (Long COVID): A scoping review. Front Med. 2021. https://doi.org/10.3389/fmed.2021.750378 .
Article Google Scholar
McLaughlin M, Cerexhe C, Macdonald E, Ingram J, Sanal-Hayes NEM, Hayes LD, et al. A Cross-sectional study of symptom prevalence, frequency, severity, and impact of long-COVID in Scotland: part I. Am J Med. 2023. https://doi.org/10.1016/j.amjmed.2023.07.009 .
Article PubMed Google Scholar
McLaughlin M, Cerexhe C, Macdonald E, Ingram J, Sanal-Hayes NEM, Hayes LD, et al. A cross-sectional study of symptom prevalence, frequency, severity, and impact of long-COVID in Scotland: part II. Am J Med. 2023. https://doi.org/10.1016/j.amjmed.2023.07.009 .
Hayes LD, Sanal-Hayes NEM, Mclaughlin M, Berry ECJ, Sculthorpe NF. People with long covid and ME/CFS exhibit similarly impaired balance and physical capacity: a case-case-control study. Am J Med. 2023;S0002–9343(23):00465–75.
Google Scholar
Jenkins R. Post-viral fatigue syndrome. Epidemiology: lessons from the past. Br Med Bull. 1991;47:952–65.
Article PubMed CAS Google Scholar
Sandler CX, Wyller VBB, Moss-Morris R, Buchwald D, Crawley E, Hautvast J, et al. Long COVID and post-infective fatigue syndrome: a review. Open Forum Infect Dis. 2021;8:440.
Carod-Artal FJ. Post-COVID-19 syndrome: epidemiology, diagnostic criteria and pathogenic mechanisms involved. Rev Neurol. 2021;72:384–96.
PubMed CAS Google Scholar
Ballering AV, van Zon SKR, Olde Hartman TC, Rosmalen JGM. Lifelines corona research initiative. Persistence of somatic symptoms after COVID-19 in the Netherlands: an observational cohort study. Lancet. 2022;400:452–61.
Wong TL, Weitzer DJ. Long COVID and Myalgic Encephalomyelitis/Chronic Fatigue Syndrome (ME/CFS)-a systemic review and comparison of clinical presentation and symptomatology. Medicina (Kaunas). 2021;57:418.
Sukocheva OA, Maksoud R, Beeraka NM, Madhunapantula SV, Sinelnikov M, Nikolenko VN, et al. Analysis of post COVID-19 condition and its overlap with myalgic encephalomyelitis/chronic fatigue syndrome. J Adv Res. 2021. https://doi.org/10.1016/j.jare.2021.11.013 .
Bonilla H, Quach TC, Tiwari A, Bonilla AE, Miglis M, Yang P, et al. Myalgic Encephalomyelitis/Chronic Fatigue Syndrome (ME/CFS) is common in post-acute sequelae of SARS-CoV-2 infection (PASC): results from a post-COVID-19 multidisciplinary clinic. medrxiv. 2022. https://doi.org/10.1101/2022.08.03.22278363v1 .
Twomey R, DeMars J, Franklin K, Culos-Reed SN, Weatherald J, Wrightson JG. Chronic fatigue and postexertional malaise in people living with long COVID: an observational study. Phys Ther. 2022;102:005.
Barhorst EE, Boruch AE, Cook DB, Lindheimer JB. Pain-related post-exertional malaise in myalgic encephalomyelitis/chronic fatigue syndrome (ME/CFS) and fibromyalgia: a systematic review and three-level meta-analysis. Pain Med. 2022;23:1144–57.
Goudsmit EM. The psychological aspects and management of chronic fatigue syndrome [Internet] [Thesis]. Brunel University, School of Social Sciences; 1996 [cited 2022 Jan 20]. https://scholar.google.co.uk/scholar_url?url=https://bura.brunel.ac.uk/bitstream/2438/4283/1/FulltextThesis.pdf&hl=en&sa=X&ei=kNYjZdeuA4-8ywTAmKmADQ&scisig=AFWwaeZvdxcuHmzGL08L3jp-QwNn&oi=scholarr . Accessed 2 Aug 2022
Stussman B, Williams A, Snow J, Gavin A, Scott R, Nath A, et al. Characterization of post-exertional malaise in patients with myalgic encephalomyelitis/chronic fatigue syndrome. Front Neurol. 2020;11:1025.
Holtzman CS, Bhatia KP, Cotler J, La J. Assessment of Post-Exertional Malaise (PEM) in Patients with Myalgic Encephalomyelitis (ME) and Chronic Fatigue Syndrome (CFS): a patient-driven survey. Diagnostics. 2019. https://doi.org/10.3390/diagnostics9010026 .
Fukuda K, Straus SE, Hickie I, Sharpe MC, Dobbins JG, Komaroff A. The chronic fatigue syndrome: a comprehensive approach to its definition and study. International Chronic Fatigue Syndrome Study Group. Ann Intern Med. 1994;121:953–9.
Carruthers BM, van de Sande MI, De Meirleir KL, Klimas NG, Broderick G, Mitchell T, et al. Myalgic encephalomyelitis: international consensus criteria. J Intern Med. 2011;270:327–38.
Carruthers JD, Lowe NJ, Menter MA, Gibson J, Eadie N, Botox Glabellar Lines II Study Group. Double-blind, placebo-controlled study of the safety and efficacy of botulinum toxin type A for patients with glabellar lines. Plast Reconstr Surg. 2003;112:1089–98.
Jason LA, Jordan K, Miike T, Bell DS, Lapp C, Torres-Harding S, et al. A pediatric case definition for myalgic encephalomyelitis and chronic fatigue syndrome. J Chronic Fatigue Syndrome. 2006;13:1–44.
Goudsmit EM, Nijs J, Jason LA, Wallman KE. Pacing as a strategy to improve energy management in myalgic encephalomyelitis/chronic fatigue syndrome: a consensus document. Disabil Rehabil. 2012;34:1140–7.
Antcliff D, Keenan A-M, Keeley P, Woby S, McGowan L. Engaging stakeholders to refine an activity pacing framework for chronic pain/fatigue: a nominal group technique. Musculoskeletal Care. 2019;17:354–62.
Antcliff D, Keeley P, Campbell M, Woby S, McGowan L. Exploring patients’ opinions of activity pacing and a new activity pacing questionnaire for chronic pain and/or fatigue: a qualitative study. Physiotherapy. 2016;102:300–7.
Yoshiuchi K, Cook DB, Ohashi K, Kumano H, Kuboki T, Yamamoto Y, et al. A real-time assessment of the effect of exercise in chronic fatigue syndrome. Physiol Behav. 2007;92:963–8.
Davenport TE, Stevens SR, Baroni K, Van Ness M, Snell CR. Diagnostic accuracy of symptoms characterising chronic fatigue syndrome. Disabil Rehabil. 2011;33:1768–75.
Nurek M, Rayner C, Freyer A, Taylor S, Järte L, MacDermott N, Delaney BC, Panellists D, et al. Recommendations for the recognition, diagnosis, and management of long COVID: a Delphi study. Br J Gen Pract. 2021. https://doi.org/10.3399/BJGP.2021.0265 .
Herrera JE, Niehaus WN, Whiteson J, Azola A, Baratta JM, Fleming TK, Kim SY, Naqvi H, Sampsel S, Silver JK, Gutierrez MV, Maley J, Herman E, Abramoff Benjamin, et al. Multidisciplinary collaborative consensus guidance statement on the assessment and treatment of fatigue in postacute sequelae of SARS-CoV-2 infection (PASC) patients. PM & R. 2021. https://doi.org/10.1002/pmrj.12684 .
Chen C, Haupert SR, Zimmermann L, Shi X, Fritsche LG, Mukherjee B. Global prevalence of post COVID-19 condition or long COVID: a meta-analysis and systematic review. J Infect Dis. 2022. https://doi.org/10.1093/infdis/jiac136 .
Office for National Statistics. Prevalence of ongoing symptoms following coronavirus (COVID-19) infection in the UK [Internet]. https://www.ons.gov.uk/peoplepopulationandcommunity/healthandsocialcare/conditionsanddiseases/bulletins/prevalenceofongoingsymptomsfollowingcoronaviruscovid19infectionintheuk/7july2022 . Accessed 2 Aug 2022
Office for National Statistics. Prevalence of ongoing symptoms following coronavirus (COVID-19) infection in the UK [Internet]. [cited 2022 Apr 1]. https://www.ons.gov.uk/peoplepopulationandcommunity/healthandsocialcare/conditionsanddiseases/bulletins/prevalenceofongoingsymptomsfollowingcoronaviruscovid19infectionintheuk/3march2022
Baker R, Shaw EJ. Diagnosis and management of chronic fatigue syndrome or myalgic encephalomyelitis (or encephalopathy): summary of NICE guidance. BMJ. 2007;335:446–8.
NICE. Overview | Myalgic encephalomyelitis (or encephalopathy)/chronic fatigue syndrome: diagnosis and management | Guidance | NICE [Internet]. NICE; [cited 2022 Aug 22]. https://www.nice.org.uk/guidance/ng206 . Accessed 2 Aug 2022
White P, Goldsmith K, Johnson A, Potts L, Walwyn R, DeCesare J, et al. Comparison of adaptive pacing therapy, cognitive behaviour therapy, graded exercise therapy, and specialist medical care for chronic fatigue syndrome (PACE): a randomised trial. The Lancet. 2011;377:823–36.
Article CAS Google Scholar
Vink M. PACE trial authors continue to ignore their own null effect. J Health Psychol. 2017;22:1134–40.
Petrie K, Weinman J. The PACE trial: it’s time to broaden perceptions and move on. J Health Psychol. 2017;22:1198–200.
Stouten B. PACE-GATE: an alternative view on a study with a poor trial protocol. J Health Psychol. 2017;22:1192–7.
Agardy S. Chronic fatigue syndrome patients have no reason to accept the PACE trial results: response to Keith J Petrie and John Weinman. J Health Psychol. 2017;22:1206–8.
Kim D-Y, Lee J-S, Park S-Y, Kim S-J, Son C-G. Systematic review of randomized controlled trials for chronic fatigue syndrome/myalgic encephalomyelitis (CFS/ME). J Transl Med. 2020;18:7.
Twisk FNM, Maes M. A review on cognitive behavorial therapy (CBT) and graded exercise therapy (GET) in myalgic encephalomyelitis (ME) / chronic fatigue syndrome (CFS): CBT/GET is not only ineffective and not evidence-based, but also potentially harmful for many patients with ME/CFS. Neuro Endocrinol Lett. 2009;30:284–99.
PubMed Google Scholar
Mays N, Roberts E, Popay J. Synthesising research evidence. In: Fulop N, Allen P, Clarke A, Black N, editors. Studying the organisation and delivery of health services: research methods. London: Routledge; 2001. p. 188–220.
Munn Z, Peters MDJ, Stern C, Tufanaru C, McArthur A, Aromataris E. Systematic review or scoping review? Guidance for authors when choosing between a systematic or scoping review approach. BMC Med Res Methodol. 2018;18:143.
Arksey H, O’Malley L. Scoping studies: towards a methodological framework. Int J Soc Res Methodol. 2005;8:19–32.
Tricco AC, Lillie E, Zarin W, O’Brien KK, Colquhoun H, Levac D, et al. PRISMA extension for scoping reviews (PRISMA-ScR): checklist and explanation. Ann Intern Med. 2018;169:467–73.
de Morton NA. The PEDro scale is a valid measure of the methodological quality of clinical trials: a demographic study. Aust J Physiother. 2009;55:129–33.
Maher CG, Sherrington C, Herbert RD, Moseley AM, Elkins M. Reliability of the PEDro scale for rating quality of randomized controlled trials. Phys Ther. 2003;83:713–21.
Kos D, van Eupen I, Meirte J, Van Cauwenbergh D, Moorkens G, Meeus M, et al. Activity pacing self-management in chronic fatigue syndrome: a randomized controlled trial. Am J Occup Ther. 2015;69:6905290020.
Meeus M, Nijs J, Van Oosterwijck J, Van Alsenoy V, Truijen S. Pain physiology education improves pain beliefs in patients with chronic fatigue syndrome compared with pacing and self-management education: a double-blind randomized controlled trial. Arch Phys Med Rehabil. 2010;91:1153–9.
Antcliff D, Keenan A-M, Keeley P, Woby S, McGowan L. Testing a newly developed activity pacing framework for chronic pain/fatigue: a feasibility study. BMJ Open. 2021;11: e045398.
Nijs J, van Eupen I, Vandecauter J, Augustinus E, Bleyen G, Moorkens G, et al. Can pacing self-management alter physical behavior and symptom severity in chronic fatigue syndrome? A case series. J Rehabil Res Dev. 2009;46:985–96.
Geraghty K, Hann M, Kurtev S. Myalgic encephalomyelitis/chronic fatigue syndrome patients’ reports of symptom changes following cognitive behavioural therapy, graded exercise therapy and pacing treatments: Analysis of a primary survey compared with secondary surveys. J Health Psychol. 2019;24:1318–33.
Jason L, Muldowney K, Torres-Harding S. The energy envelope theory and myalgic encephalomyelitis/chronic fatigue syndrome. AAOHN J. 2008;56:189–95.
Brown M, Khorana N, Jason LA. The role of changes in activity as a function of perceived available and expended energy in non-pharmacological treatment outcomes for ME/CFS. J Clin Psychol. 2011;67:253.
Association ME. ME/CFS illness management survey results:‘“No decisions about me without me.” Part 1: Results and in-depth analysis of the 2012 ME association patient survey examining the acceptability, efficacy and safety of cognitive behavioural therapy, graded exercise therapy and pacing, as interventions used as management strategies for ME/CFS. 2015. https://www.meassociation.org.uk/wp-content/uploads/NO-DECISIONS-WITHOUT-ME-report.docx . Accessed 2 Feb 2022
Sharpe M, Goldsmith KA, Johnson AL, Chalder T, Walker J, White PD. Rehabilitative treatments for chronic fatigue syndrome: long-term follow-up from the PACE trial. The Lancet Psychiatry. 2015;2:1067–74.
White PD, Goldsmith K, Johnson AL, Chalder T, Sharpe M. Recovery from chronic fatigue syndrome after treatments given in the PACE trial. Psychol Med. 2013;43:2227–35.
O’connor K, Sunnquist M, Nicholson L, Jason LA, Newton JL, Strand EB. Energy envelope maintenance among patients with myalgic encephalomyelitis and chronic fatigue syndrome: Implications of limited energy reserves. Chronic Illn. 2019;15:51–60.
Dougall D, Johnson A, Goldsmith K, Sharpe M, Angus B, Chalder T, et al. Adverse events and deterioration reported by participants in the PACE trial of therapies for chronic fatigue syndrome. J Psychosom Res. 2014;77:20–6.
Brown AA, Evans MA, Jason LA. Examining the energy envelope and associated symptom patterns in chronic fatigue syndrome: does coping matter? Chronic Illn. 2013;9:302–11.
Bourke JH, Johnson AL, Sharpe M, Chalder T, White PD. Pain in chronic fatigue syndrome: response to rehabilitative treatments in the PACE trial. Psychol Med. 2014;44:1545–52.
Jason LA, Brown M, Brown A, Evans M, Flores S, Grant-Holler E, et al. Energy conservation/envelope theory interventions to help patients with myalgic encephalomyelitis/chronic fatigue syndrome. Fatigue. 2013;1:27–42.
Antcliff D, Campbell M, Woby S, Keeley P. Activity pacing is associated with better and worse symptoms for patients with long-term conditions. Clin J Pain. 2017;33:205–14.
Nijs T, Klein Y, Mousavi S, Ahsan A, Nowakowska S, Constable E, et al. The different faces of 4’-Pyrimidinyl-Functionalized 4,2’:6’,4"-Terpyridin es: metal-organic assemblies from solution and on Au(111) and Cu(111) surface platforms. J Am Chem Soc. 2018;140:2933–9.
Cutler DM, Summers LH. The COVID-19 pandemic and the $16 Trillion Virus. JAMA. 2020;324:1495–6.
Cutler DM. The costs of long COVID. JAMA Health Forum. 2022;3:e221809–e221809.
Geraghty K. ‘PACE-Gate’: when clinical trial evidence meets open data access. J Health Psychol. 2017;22:1106–12.
Davis R, Campbell R, Hildon Z, Hobbs L, Michie S. Theories of behaviour and behaviour change across the social and behavioural sciences: a scoping review. Health Psychol Rev. 2015;9:323–44.
Prestwich A, Sniehotta FF, Whittington C, Dombrowski SU, Rogers L, Michie S. Does theory influence the effectiveness of health behavior interventions? Meta-analysis Health Psychol. 2014;33:465–74.
Balinas C, Eaton-Fitch N, Maksoud R, Staines D, Marshall-Gradisnik S. Impact of life stressors on Myalgic encephalomyelitis/chronic fatigue syndrome symptoms: an Australian longitudinal study. Int J Environ Res Public Health. 2021;18:10614.
McGregor NR, Armstrong CW, Lewis DP, Gooley PR. Post-exertional malaise is associated with hypermetabolism, hypoacetylation and purine metabolism deregulation in ME/CFS cases. Diagnostics. 2019;9:70.
Nacul LC, Lacerda EM, Campion P, Pheby D, de Drachler M, Leite JC, et al. The functional status and well being of people with myalgic encephalomyelitis/chronic fatigue syndrome and their carers. BMC Public Health. 2011;11:402.
Deumer U-S, Varesi A, Floris V, Savioli G, Mantovani E, López-Carrasco P, et al. Myalgic Encephalomyelitis/Chronic Fatigue Syndrome (ME/CFS): an overview. J Clin Med. 2021. https://doi.org/10.3390/jcm10204786 .
Düking P, Giessing L, Frenkel MO, Koehler K, Holmberg H-C, Sperlich B. Wrist-worn wearables for monitoring heart rate and energy expenditure while sitting or performing light-to-vigorous physical activity: validation study. JMIR Mhealth Uhealth. 2020;8: e16716.
Falter M, Budts W, Goetschalckx K, Cornelissen V, Buys R. Accuracy of apple watch measurements for heart rate and energy expenditure in patients with cardiovascular disease: cross-sectional study. JMIR Mhealth Uhealth. 2019;7: e11889.
Fuller D, Colwell E, Low J, Orychock K, Tobin MA, Simango B, et al. Reliability and validity of commercially available wearable devices for measuring steps, energy expenditure, and heart rate: systematic review. JMIR Mhealth Uhealth. 2020;8: e18694.
Mair JL, Hayes LD, Campbell AK, Sculthorpe N. Should we use activity tracker data from smartphones and wearables to understand population physical activity patterns? J Measur Phys Behav. 2022;1:1–5.
Mair JL, Hayes LD, Campbell AK, Buchan DS, Easton C, Sculthorpe N. A personalized smartphone-delivered just-in-time adaptive intervention (JitaBug) to increase physical activity in older adults: mixed methods feasibility study. JMIR Formative Res. 2022;6: e34662.
Michie S, Richardson M, Johnston M, Abraham C, Francis J, Hardeman W, et al. The behavior change technique taxonomy (v1) of 93 hierarchically clustered techniques: building an international consensus for the reporting of behavior change interventions. Ann Behav Med. 2013;46:81–95.
Feehan SM. The PACE trial in chronic fatigue syndrome. The Lancet. 2011;377:1831–2.
Giakoumakis J. The PACE trial in chronic fatigue syndrome. The Lancet. 2011;377:1831.
White PD, Sharpe MC, Chalder T, DeCesare JC, Walwyn R, PACE trial group. Protocol for the PACE trial: a randomised controlled trial of adaptive pacing, cognitive behaviour therapy, and graded exercise, as supplements to standardised specialist medical care versus standardised specialist medical care alone for patients with the chronic fatigue syndrome/myalgic encephalomyelitis or encephalopathy. BMC Neurol. 2007;7:6.
Reuben DB, Tinetti ME. Goal-oriented patient care–an alternative health outcomes paradigm. N Engl J Med. 2012;366:777–9.
Roberts D. Chronic fatigue syndrome and quality of life. PROM. 2018;9:253–62.
Valdez AR, Hancock EE, Adebayo S, Kiernicki DJ, Proskauer D, Attewell JR, et al. Estimating prevalence, demographics, and costs of ME/CFS using large scale medical claims data and machine learning. Front Pediatr. 2019. https://doi.org/10.3389/fped.2018.00412 .
Greiwe J, Nyenhuis SM. Wearable technology and how this can be implemented into clinical practice. Curr Allergy Asthma Rep. 2020;20:36.
Sun S, Folarin AA, Ranjan Y, Rashid Z, Conde P, Stewart C, et al. Using smartphones and wearable devices to monitor behavioral changes during COVID-19. J Med Internet Res. 2020;22: e19992.
Hardeman W, Houghton J, Lane K, Jones A, Naughton F. A systematic review of just-in-time adaptive interventions (JITAIs) to promote physical activity. Int J Behav Nutr Phys Act. 2019;16:31.
Perski O, Hébert ET, Naughton F, Hekler EB, Brown J, Businelle MS. Technology-mediated just-in-time adaptive interventions (JITAIs) to reduce harmful substance use: a systematic review. Addiction. 2022;117:1220–41.
AhmedS A, van Luenen S, Aslam S, van Bodegom D, Chavannes NH. A systematic review on the use of mHealth to increase physical activity in older people. Clinical eHealth. 2020;3:31–9.
Valenzuela T, Okubo Y, Woodbury A, Lord SR, Delbaere K. Adherence to technology-based exercise programs in older adults: a systematic review. J Geriatric Phys Ther. 2018;41:49–61.
Bowling A. Mode of questionnaire administration can have serious effects on data quality. J Public Health. 2005;27:281–91.
Burns SP, Terblanche M, Perea J, Lillard H, DeLaPena C, Grinage N, et al. mHealth intervention applications for adults living with the effects of stroke: a scoping review. Arch Rehabil Res Clin Transl. 2021;3: 100095.
Vandelanotte C, Müller AM, Short CE, Hingle M, Nathan N, Williams SL, et al. Past, present, and future of eHealth and mHealth research to improve physical activity and dietary behaviors. J Nutr Educ Behav. 2016;48:219-228.e1.
Ludwig K, Arthur R, Sculthorpe N, Fountain H, Buchan DS. Text messaging interventions for improvement in physical activity and sedentary behavior in youth: systematic review. JMIR Mhealth Uhealth. 2018;6:e10799.
Download references
Acknowledgements
We have no acknowledgements to make.
Open access funding provided by Swiss Federal Institute of Technology Zurich. This work was supported by grants from the National Institute for Health and Care Research (COV-LT2-0010) and the funder had no role in the conceptualisation, design, data collection, analysis, decision to publish, or preparation of the manuscript.
Author information
Authors and affiliations.
Sport and Physical Activity Research Institute, School of Health and Life Sciences, University of the West of Scotland, Glasgow, UK
Nilihan E. M. Sanal-Hayes, Marie Mclaughlin, Lawrence D. Hayes, David Carless, Rachel Meach & Nicholas F. Sculthorpe
Future Health Technologies, Singapore-ETH Centre, Campus for Research Excellence and Technological Enterprise (CREATE), Singapore, Singapore
Jacqueline L. Mair
Saw Swee Hock School of Public Health, National University of Singapore, Singapore, Singapore
Long COVID Scotland, 12 Kemnay Place, Aberdeen, UK
Jane Ormerod
Physios for ME, London, UK
Natalie Hilliard
School of Education and Social Sciences, University of the West of Scotland, Glasgow, UK
Joanne Ingram
School of Health and Society, University of Salford, Salford, UK
Nilihan E. M. Sanal-Hayes
School of Sport, Exercise & Rehabilitation Sciences, University of Hull, Hull, UK
Marie Mclaughlin
You can also search for this author in PubMed Google Scholar
Contributions
Authors’ contributions are given according to the CRediT taxonomy as follows: Conceptualization, N.E.M.S–H., M.M., L.D.H, and N.F.S.; methodology, N.E.M.S–H., M.M., L.D.H., and N.F.S.; software, N.E.M.S–H., M.M., L.D.H., and N.F.S.B.; validation, N.E.M.S–H., M.M., L.D.H, and N.F.S.; formal analysis, N.E.M.S–H., M.M., L.D.H., and N.F.S.; investigation, N.E.M.S–H., M.M., L.D.H., and N.F.S.; resources, L.D.H., J.O., D.C., N.H., J.L.M., and N.F.S.; data curation, N.E.M.S.-H., M.M., L.D.H., and N.F.S.; writing—original draft preparation, N.E.M.S.-H., M.M., L.D.H., and N.F.S.; writing—review and editing, N.E.M.S–H., M.M., L.D.H., J.O., D.C., N.H., R.M., J.L.M., J.I., and N.F.S.; visualisation, N.E.M.S–H. and M.M., supervision, N.F.S; project administration, N.E.M.S–H., M.M., L.D.H., and N.F.S.; funding acquisition, L.D.H., J.O., D.C., N.H., J.L.M., J.I., and N.F.S. All authors have read and agreed to the published version of the manuscript.
Corresponding author
Correspondence to Jacqueline L. Mair .
Ethics declarations
Ethical approval and content to participate.
This manuscript did not involve human participants, data, or tissues, so did not require ethical approval.
Consent for publication
This paper does not contain any individual person’s data in any form.
Competing interests
We report no financial and non-financial competing interests.
Additional information
Publisher's note.
Springer Nature remains neutral with regard to jurisdictional claims in published maps and institutional affiliations.
Supplementary Information
Additional file 1..
Supplementary file 1. Full search string for databse searching.
Rights and permissions
Open Access This article is licensed under a Creative Commons Attribution 4.0 International License, which permits use, sharing, adaptation, distribution and reproduction in any medium or format, as long as you give appropriate credit to the original author(s) and the source, provide a link to the Creative Commons licence, and indicate if changes were made. The images or other third party material in this article are included in the article's Creative Commons licence, unless indicated otherwise in a credit line to the material. If material is not included in the article's Creative Commons licence and your intended use is not permitted by statutory regulation or exceeds the permitted use, you will need to obtain permission directly from the copyright holder. To view a copy of this licence, visit http://creativecommons.org/licenses/by/4.0/ . The Creative Commons Public Domain Dedication waiver ( http://creativecommons.org/publicdomain/zero/1.0/ ) applies to the data made available in this article, unless otherwise stated in a credit line to the data.
Reprints and permissions
About this article
Cite this article.
Sanal-Hayes, N.E.M., Mclaughlin, M., Hayes, L.D. et al. A scoping review of ‘Pacing’ for management of Myalgic Encephalomyelitis/Chronic Fatigue Syndrome (ME/CFS): lessons learned for the long COVID pandemic. J Transl Med 21 , 720 (2023). https://doi.org/10.1186/s12967-023-04587-5
Download citation
Received : 30 June 2023
Accepted : 03 October 2023
Published : 14 October 2023
DOI : https://doi.org/10.1186/s12967-023-04587-5
Share this article
Anyone you share the following link with will be able to read this content:
Sorry, a shareable link is not currently available for this article.
Provided by the Springer Nature SharedIt content-sharing initiative
- Myalgic encephalomyelitis
- Chronic fatigue syndrome
- Post-exertional malaise
Journal of Translational Medicine
ISSN: 1479-5876
- Submission enquiries: Access here and click Contact Us
- General enquiries: [email protected]
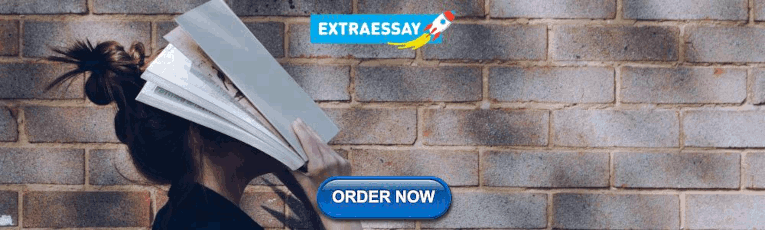
IMAGES
VIDEO
COMMENTS
Revised on June 22, 2023. A cross-sectional study is a type of research design in which you collect data from many different individuals at a single point in time. In cross-sectional research, you observe variables without influencing them. Researchers in economics, psychology, medicine, epidemiology, and the other social sciences all make use ...
Introduction. Cross-sectional study design is a type of observational study design. As discussed in the earlier articles, we have highlighted that in an observational study, the investigator does not alter the exposure status. The investigator measures the outcome and the exposure (s) in the population, and may study their association.
A cross-sectional study design is a type of observational study, or descriptive research, that involves analyzing information about a population at a specific point in time. This design measures the prevalence of an outcome of interest in a defined population. It provides a snapshot of the characteristics of the population at a single point in ...
A cross-sectional study is a type of research design in which you collect data from many different individuals at a single point in time. In cross-sectional research, you observe variables without influencing them. Researchers in economics, psychology, medicine, epidemiology, and the other social sciences all make use of cross-sectional studies ...
Observational studies monitor study participants without providing study interventions. This paper describes the cross-sectional design, examines the strengths and weaknesses, and discusses some methods to report the results. Future articles will focus on other observational methods, the cohort, and case-control designs.
A cross-sectional study looks at data at a single point in time. The participants in this type of study are selected based on particular variables of interest. Cross-sectional studies are often used in developmental psychology, but this method is also used in many other areas, including social science and education.
3.1. Strengths: when to use cross-sectional data. The strengths of cross-sectional data help to explain their overuse in IS research. First, such studies can be conducted efficiently and inexpensively by distributing a survey to a convenient sample (e.g., the researcher's social network or students) (Compeau et al., 2012) or by using a crowdsourcing website (Lowry et al., 2016, Steelman et ...
In medical research, a cross-sectional study is a type of observational study design that involves looking at data from a population at one specific point in time. In a cross-sectional study, investigators measure outcomes and exposures of the study subjects at the same time. It is described as taking a "snapshot" of a group of individuals.
In medical research, social science, and biology, a cross-sectional study (also known as a cross-sectional analysis, transverse study, prevalence study) is a type of observational study that analyzes data from a population, or a representative subset, at a specific point in time—that is, cross-sectional data. [definition needed]In economics, cross-sectional studies typically involve the use ...
A cross-sectional study (also referred to as cross-sectional research) is simply a study in which data are collected at one point in time. In other words, data are collected on a snapshot basis, as opposed to collecting data at multiple points in time (for example, once a week, once a month, etc) and assessing how it changes over time. ...
Cross-sectional studies are a type of observational studies in which the researcher commonly assesses the exposure, outcome, and other variables (such as confounding variables) at the same time. ... Book: The Cambridge Handbook of Research Methods and Statistics for the Social and Behavioral Sciences; Online publication: 25 May 2023; Chapter ...
The bottom line. A cross-sectional study is essential when researching the prevailing characteristics in a given population at a single point in time. Cross-sectional studies are often used to analyze demography, financial reports, and election polls. You could also use them in medical research or when building a marketing strategy, for instance.
Section snippets A brief overview of cross-sectional studies. A cross-sectional study, also known as a prevalence or transverse study, uses a snapshot of participants' beliefs, behaviors, or other variables of interest of a study population (e.g., a group of individuals or organizations) at a specified point in time (Grimes and Schulz, 2002, Hua and David, 2008) to examine research questions ...
In medical research, a cross-sectional study is a type of observational study design that involves looking at data from a population at one specific point in time. ... Martínez-Mesa et al 9 provided a useful discussion on the basic elements of selection of participants for a clinical study. Commonly used sampling methods are summarized in ...
Abstract. Cross-sectional study design is a type of observ ational study design. In a cross-sectional study, the investigator measur es the outcome and th e exposures in the study participan ts at ...
Abstract. Cross-sectional studies are observational studies that analyze data from a population at a single point in time. They are often used to measure the prevalence of health outcomes, understand determinants of health, and describe features of a population. Unlike other types of observational studies, cross-sectional studies do not follow ...
A cross-sectional study is useful for highlighting the extent of a problem in a community, identifying the need for services, or describing the burden of a condition within a population (Das-Munshi et al., 2020). For example, cross-sectional studies can be used to measure the prevalence of depression within a group of university students. They can
As is the case for most study types a larger sample size gives greater power and is more ideal for a strong study design. Within a cross-sectional study a sample size of at least 60 participants is recommended, although this will depend on suitability to the research question and the variables being measured. A suitable number of variables.
Cross-sectional research studies are a type of descriptive research that provides information from groups. Because it is a snapshot of a moment in time, this type of research cannot be used to ...
Cohort, cross sectional, and case-control studies are collectively referred to as observational studies. Often these studies are the only practicable method of studying various problems, for example, studies of aetiology, instances where a randomised controlled trial might be unethical, or if the condition to be studied is rare.
A cross-sectional study is a type of observational research that allows assembling data from many different subjects at one point. Scientists usually rely on specific variables to pick the participants. As descriptive research, a cross-sectional study is used to observe something that already exists in a cohort.
7. Cross-sectional studies miss fewer data points. The processes involved with cross-sectional studies reduce the risk of missing critical data points. Researchers have the ability to maximize their examination of the available information at any time because there are no time variables included in this work.
Method Cross-sectional, analytical study, carried out between May and June 2020, with a convenience sample (n=398) of health workers from 10 professional categories. ... A cross-sectional research ...
The research was conducted in two separate studies, one cross-sectional and the other longitudinal. Study I examined students' hopelessness level in relation to gender, job or income loss, social media use, academic unit, and grade. Study II compared students' hopelessness level during the distance and hybrid education periods.
Methods. A cross-sectional descriptive study was conducted between January 2023 and April 2023. A newly developed and validated self-reported questionnaire, based on the Health Belief Model (HBM) and previous research, was administered to a convenient sample of 134 adult participants diagnosed with diabetes. These participants sought care at primary healthcare clinics at King Abdul-Aziz ...
Methods: We conducted a cross-sectional study in Shanghai, China. Residents who underwent facilitated self-service fundus disease screening at one of the screening sites were assigned to the exposure group; those who were screened with a traditional fundus camera operated by an optometrist at an adjacent site comprised the control group ...
The present study aimed to compare scapular-related kinematic and electromyographic outcomes during different shoulder movements (with and without load) and the drinking task, between symptomatic and asymptomatic subjects. Forty subjects (divided into two groups) participated in this cross-sectional observational study.
Objective The primary objective of this cross-sectional study was to compare standard laboratory performance metrics of transgender athletes to cisgender athletes. Methods 19 cisgender men (CM) (mean±SD, age: 37±9 years), 12 transgender men (TM) (age: 34±7 years), 23 transgender women (TW) (age: 34±10 years) and 21 cisgender women (CW) (age: 30±9 years) underwent a series of standard ...
An adapted version of the Newcastle-Ottawa Scale for Cohort Studies was used to assess study quality. Moderate- and high-quality studies were synthesised. Results 12 cohort, 9 cross-sectional and 32 pre-post studies were included (n=53). One cohort study was high-quality. Other studies were moderate (n=33) and low-quality (n=19).
Observational study sample sizes ranged from 16 in a cross-sectional interview study to 1428 in a cross-sectional survey . One study involved a retrospective analysis of participants' own pacing strategies varying from self-guided pacing or pacing administered by a therapist compared with implementation of CBT and GET [ 52 ].