Home Blog Design Understanding Data Presentations (Guide + Examples)
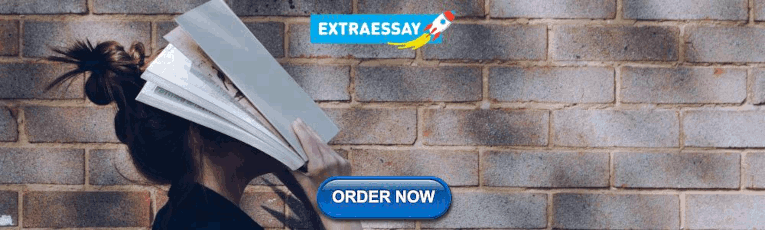
Understanding Data Presentations (Guide + Examples)
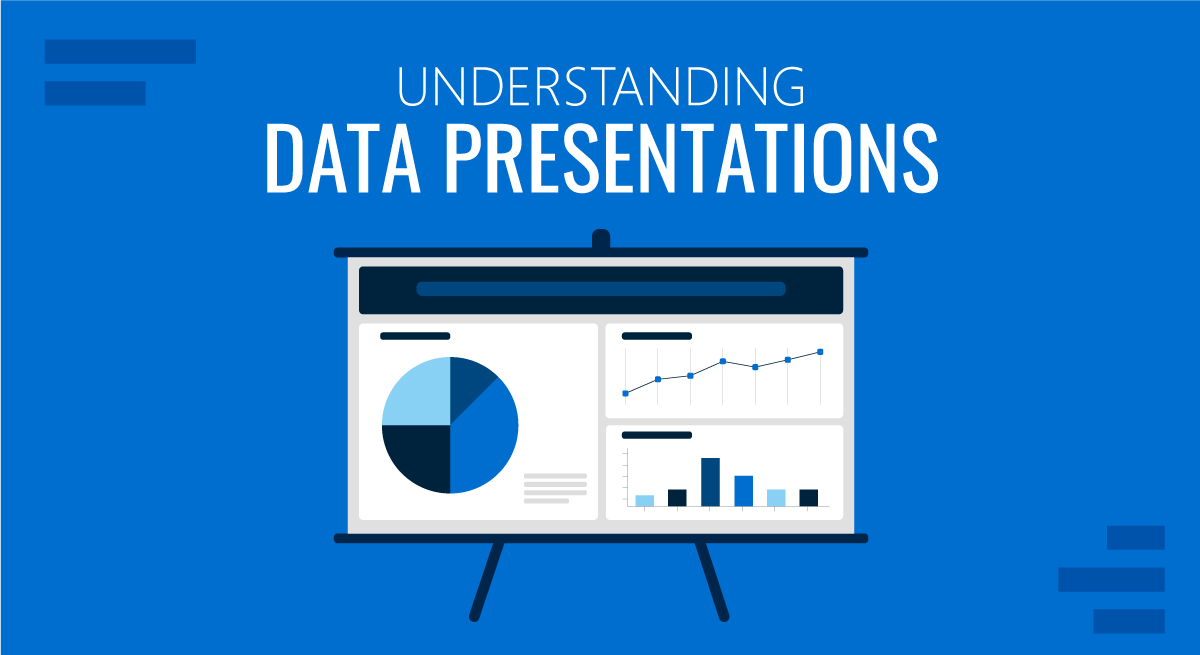
In this age of overwhelming information, the skill to effectively convey data has become extremely valuable. Initiating a discussion on data presentation types involves thoughtful consideration of the nature of your data and the message you aim to convey. Different types of visualizations serve distinct purposes. Whether you’re dealing with how to develop a report or simply trying to communicate complex information, how you present data influences how well your audience understands and engages with it. This extensive guide leads you through the different ways of data presentation.
Table of Contents
What is a Data Presentation?
What should a data presentation include, line graphs, treemap chart, scatter plot, how to choose a data presentation type, recommended data presentation templates, common mistakes done in data presentation.
A data presentation is a slide deck that aims to disclose quantitative information to an audience through the use of visual formats and narrative techniques derived from data analysis, making complex data understandable and actionable. This process requires a series of tools, such as charts, graphs, tables, infographics, dashboards, and so on, supported by concise textual explanations to improve understanding and boost retention rate.
Data presentations require us to cull data in a format that allows the presenter to highlight trends, patterns, and insights so that the audience can act upon the shared information. In a few words, the goal of data presentations is to enable viewers to grasp complicated concepts or trends quickly, facilitating informed decision-making or deeper analysis.
Data presentations go beyond the mere usage of graphical elements. Seasoned presenters encompass visuals with the art of data storytelling , so the speech skillfully connects the points through a narrative that resonates with the audience. Depending on the purpose – inspire, persuade, inform, support decision-making processes, etc. – is the data presentation format that is better suited to help us in this journey.
To nail your upcoming data presentation, ensure to count with the following elements:
- Clear Objectives: Understand the intent of your presentation before selecting the graphical layout and metaphors to make content easier to grasp.
- Engaging introduction: Use a powerful hook from the get-go. For instance, you can ask a big question or present a problem that your data will answer. Take a look at our guide on how to start a presentation for tips & insights.
- Structured Narrative: Your data presentation must tell a coherent story. This means a beginning where you present the context, a middle section in which you present the data, and an ending that uses a call-to-action. Check our guide on presentation structure for further information.
- Visual Elements: These are the charts, graphs, and other elements of visual communication we ought to use to present data. This article will cover one by one the different types of data representation methods we can use, and provide further guidance on choosing between them.
- Insights and Analysis: This is not just showcasing a graph and letting people get an idea about it. A proper data presentation includes the interpretation of that data, the reason why it’s included, and why it matters to your research.
- Conclusion & CTA: Ending your presentation with a call to action is necessary. Whether you intend to wow your audience into acquiring your services, inspire them to change the world, or whatever the purpose of your presentation, there must be a stage in which you convey all that you shared and show the path to staying in touch. Plan ahead whether you want to use a thank-you slide, a video presentation, or which method is apt and tailored to the kind of presentation you deliver.
- Q&A Session: After your speech is concluded, allocate 3-5 minutes for the audience to raise any questions about the information you disclosed. This is an extra chance to establish your authority on the topic. Check our guide on questions and answer sessions in presentations here.
Bar charts are a graphical representation of data using rectangular bars to show quantities or frequencies in an established category. They make it easy for readers to spot patterns or trends. Bar charts can be horizontal or vertical, although the vertical format is commonly known as a column chart. They display categorical, discrete, or continuous variables grouped in class intervals [1] . They include an axis and a set of labeled bars horizontally or vertically. These bars represent the frequencies of variable values or the values themselves. Numbers on the y-axis of a vertical bar chart or the x-axis of a horizontal bar chart are called the scale.
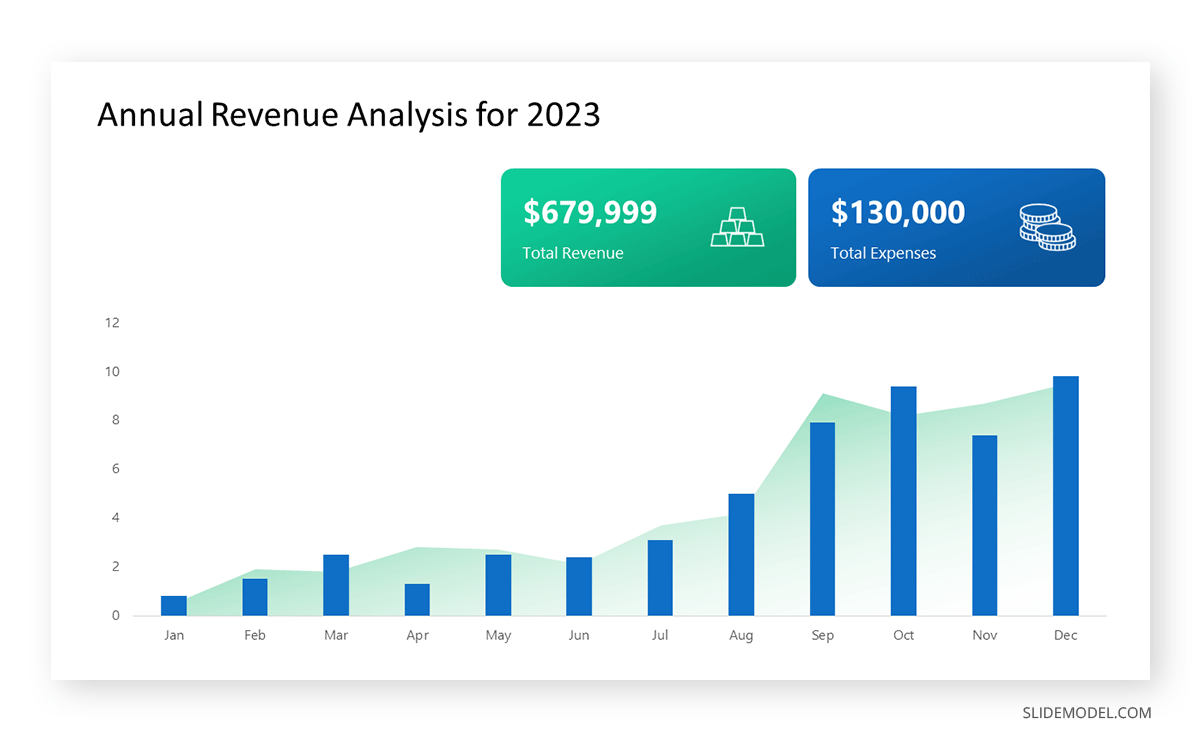
Real-Life Application of Bar Charts
Let’s say a sales manager is presenting sales to their audience. Using a bar chart, he follows these steps.
Step 1: Selecting Data
The first step is to identify the specific data you will present to your audience.
The sales manager has highlighted these products for the presentation.
- Product A: Men’s Shoes
- Product B: Women’s Apparel
- Product C: Electronics
- Product D: Home Decor
Step 2: Choosing Orientation
Opt for a vertical layout for simplicity. Vertical bar charts help compare different categories in case there are not too many categories [1] . They can also help show different trends. A vertical bar chart is used where each bar represents one of the four chosen products. After plotting the data, it is seen that the height of each bar directly represents the sales performance of the respective product.
It is visible that the tallest bar (Electronics – Product C) is showing the highest sales. However, the shorter bars (Women’s Apparel – Product B and Home Decor – Product D) need attention. It indicates areas that require further analysis or strategies for improvement.
Step 3: Colorful Insights
Different colors are used to differentiate each product. It is essential to show a color-coded chart where the audience can distinguish between products.
- Men’s Shoes (Product A): Yellow
- Women’s Apparel (Product B): Orange
- Electronics (Product C): Violet
- Home Decor (Product D): Blue
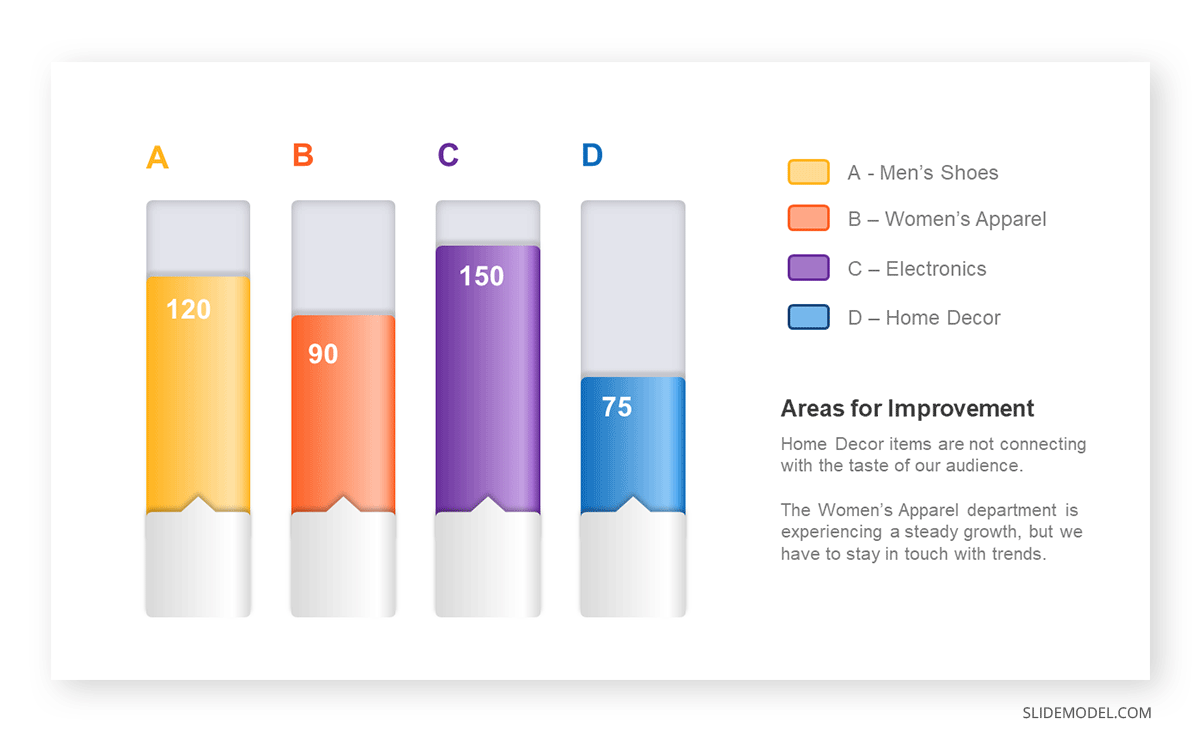
Bar charts are straightforward and easily understandable for presenting data. They are versatile when comparing products or any categorical data [2] . Bar charts adapt seamlessly to retail scenarios. Despite that, bar charts have a few shortcomings. They cannot illustrate data trends over time. Besides, overloading the chart with numerous products can lead to visual clutter, diminishing its effectiveness.
For more information, check our collection of bar chart templates for PowerPoint .
Line graphs help illustrate data trends, progressions, or fluctuations by connecting a series of data points called ‘markers’ with straight line segments. This provides a straightforward representation of how values change [5] . Their versatility makes them invaluable for scenarios requiring a visual understanding of continuous data. In addition, line graphs are also useful for comparing multiple datasets over the same timeline. Using multiple line graphs allows us to compare more than one data set. They simplify complex information so the audience can quickly grasp the ups and downs of values. From tracking stock prices to analyzing experimental results, you can use line graphs to show how data changes over a continuous timeline. They show trends with simplicity and clarity.
Real-life Application of Line Graphs
To understand line graphs thoroughly, we will use a real case. Imagine you’re a financial analyst presenting a tech company’s monthly sales for a licensed product over the past year. Investors want insights into sales behavior by month, how market trends may have influenced sales performance and reception to the new pricing strategy. To present data via a line graph, you will complete these steps.
First, you need to gather the data. In this case, your data will be the sales numbers. For example:
- January: $45,000
- February: $55,000
- March: $45,000
- April: $60,000
- May: $ 70,000
- June: $65,000
- July: $62,000
- August: $68,000
- September: $81,000
- October: $76,000
- November: $87,000
- December: $91,000
After choosing the data, the next step is to select the orientation. Like bar charts, you can use vertical or horizontal line graphs. However, we want to keep this simple, so we will keep the timeline (x-axis) horizontal while the sales numbers (y-axis) vertical.
Step 3: Connecting Trends
After adding the data to your preferred software, you will plot a line graph. In the graph, each month’s sales are represented by data points connected by a line.
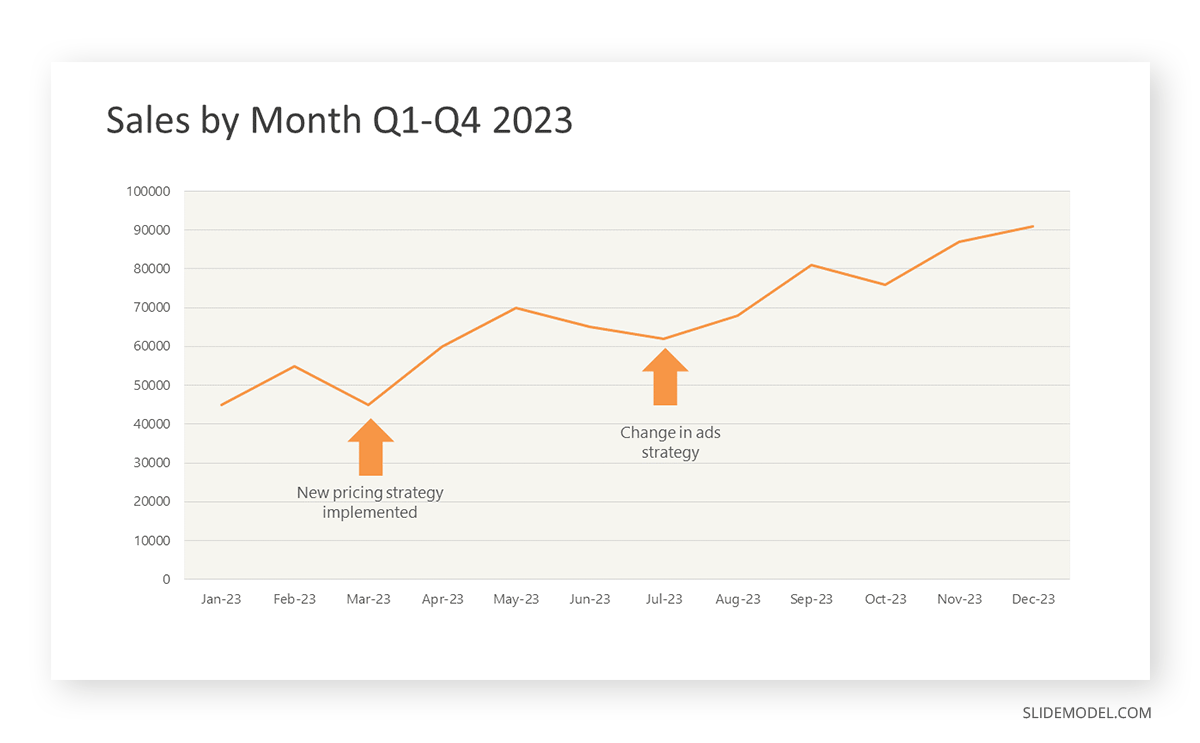
Step 4: Adding Clarity with Color
If there are multiple lines, you can also add colors to highlight each one, making it easier to follow.
Line graphs excel at visually presenting trends over time. These presentation aids identify patterns, like upward or downward trends. However, too many data points can clutter the graph, making it harder to interpret. Line graphs work best with continuous data but are not suitable for categories.
For more information, check our collection of line chart templates for PowerPoint and our article about how to make a presentation graph .
A data dashboard is a visual tool for analyzing information. Different graphs, charts, and tables are consolidated in a layout to showcase the information required to achieve one or more objectives. Dashboards help quickly see Key Performance Indicators (KPIs). You don’t make new visuals in the dashboard; instead, you use it to display visuals you’ve already made in worksheets [3] .
Keeping the number of visuals on a dashboard to three or four is recommended. Adding too many can make it hard to see the main points [4]. Dashboards can be used for business analytics to analyze sales, revenue, and marketing metrics at a time. They are also used in the manufacturing industry, as they allow users to grasp the entire production scenario at the moment while tracking the core KPIs for each line.
Real-Life Application of a Dashboard
Consider a project manager presenting a software development project’s progress to a tech company’s leadership team. He follows the following steps.
Step 1: Defining Key Metrics
To effectively communicate the project’s status, identify key metrics such as completion status, budget, and bug resolution rates. Then, choose measurable metrics aligned with project objectives.
Step 2: Choosing Visualization Widgets
After finalizing the data, presentation aids that align with each metric are selected. For this project, the project manager chooses a progress bar for the completion status and uses bar charts for budget allocation. Likewise, he implements line charts for bug resolution rates.
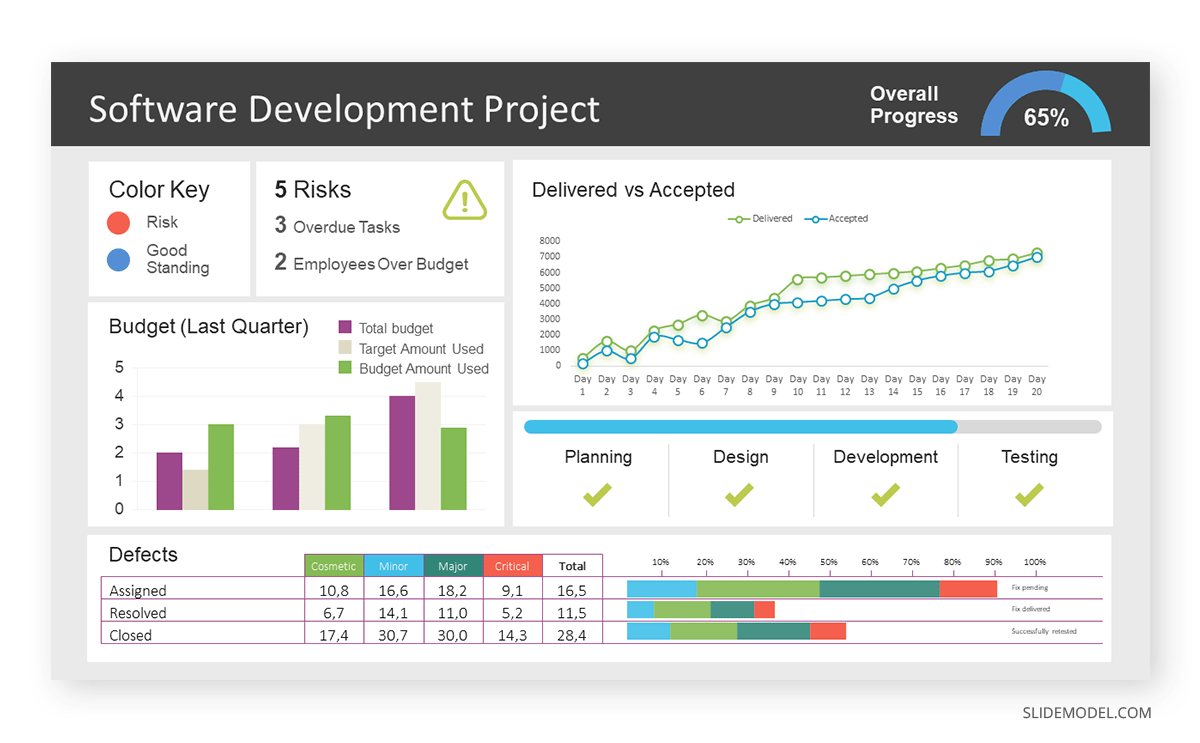
Step 3: Dashboard Layout
Key metrics are prominently placed in the dashboard for easy visibility, and the manager ensures that it appears clean and organized.
Dashboards provide a comprehensive view of key project metrics. Users can interact with data, customize views, and drill down for detailed analysis. However, creating an effective dashboard requires careful planning to avoid clutter. Besides, dashboards rely on the availability and accuracy of underlying data sources.
For more information, check our article on how to design a dashboard presentation , and discover our collection of dashboard PowerPoint templates .
Treemap charts represent hierarchical data structured in a series of nested rectangles [6] . As each branch of the ‘tree’ is given a rectangle, smaller tiles can be seen representing sub-branches, meaning elements on a lower hierarchical level than the parent rectangle. Each one of those rectangular nodes is built by representing an area proportional to the specified data dimension.
Treemaps are useful for visualizing large datasets in compact space. It is easy to identify patterns, such as which categories are dominant. Common applications of the treemap chart are seen in the IT industry, such as resource allocation, disk space management, website analytics, etc. Also, they can be used in multiple industries like healthcare data analysis, market share across different product categories, or even in finance to visualize portfolios.
Real-Life Application of a Treemap Chart
Let’s consider a financial scenario where a financial team wants to represent the budget allocation of a company. There is a hierarchy in the process, so it is helpful to use a treemap chart. In the chart, the top-level rectangle could represent the total budget, and it would be subdivided into smaller rectangles, each denoting a specific department. Further subdivisions within these smaller rectangles might represent individual projects or cost categories.
Step 1: Define Your Data Hierarchy
While presenting data on the budget allocation, start by outlining the hierarchical structure. The sequence will be like the overall budget at the top, followed by departments, projects within each department, and finally, individual cost categories for each project.
- Top-level rectangle: Total Budget
- Second-level rectangles: Departments (Engineering, Marketing, Sales)
- Third-level rectangles: Projects within each department
- Fourth-level rectangles: Cost categories for each project (Personnel, Marketing Expenses, Equipment)
Step 2: Choose a Suitable Tool
It’s time to select a data visualization tool supporting Treemaps. Popular choices include Tableau, Microsoft Power BI, PowerPoint, or even coding with libraries like D3.js. It is vital to ensure that the chosen tool provides customization options for colors, labels, and hierarchical structures.
Here, the team uses PowerPoint for this guide because of its user-friendly interface and robust Treemap capabilities.
Step 3: Make a Treemap Chart with PowerPoint
After opening the PowerPoint presentation, they chose “SmartArt” to form the chart. The SmartArt Graphic window has a “Hierarchy” category on the left. Here, you will see multiple options. You can choose any layout that resembles a Treemap. The “Table Hierarchy” or “Organization Chart” options can be adapted. The team selects the Table Hierarchy as it looks close to a Treemap.
Step 5: Input Your Data
After that, a new window will open with a basic structure. They add the data one by one by clicking on the text boxes. They start with the top-level rectangle, representing the total budget.
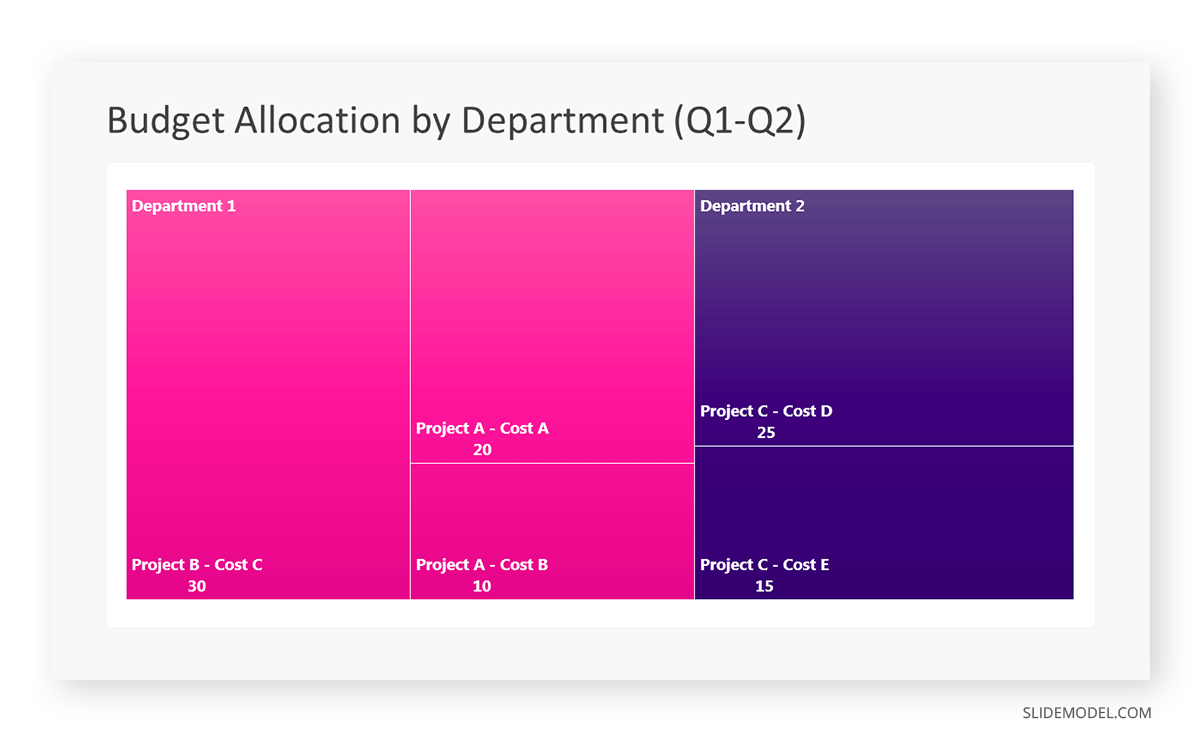
Step 6: Customize the Treemap
By clicking on each shape, they customize its color, size, and label. At the same time, they can adjust the font size, style, and color of labels by using the options in the “Format” tab in PowerPoint. Using different colors for each level enhances the visual difference.
Treemaps excel at illustrating hierarchical structures. These charts make it easy to understand relationships and dependencies. They efficiently use space, compactly displaying a large amount of data, reducing the need for excessive scrolling or navigation. Additionally, using colors enhances the understanding of data by representing different variables or categories.
In some cases, treemaps might become complex, especially with deep hierarchies. It becomes challenging for some users to interpret the chart. At the same time, displaying detailed information within each rectangle might be constrained by space. It potentially limits the amount of data that can be shown clearly. Without proper labeling and color coding, there’s a risk of misinterpretation.
A heatmap is a data visualization tool that uses color coding to represent values across a two-dimensional surface. In these, colors replace numbers to indicate the magnitude of each cell. This color-shaded matrix display is valuable for summarizing and understanding data sets with a glance [7] . The intensity of the color corresponds to the value it represents, making it easy to identify patterns, trends, and variations in the data.
As a tool, heatmaps help businesses analyze website interactions, revealing user behavior patterns and preferences to enhance overall user experience. In addition, companies use heatmaps to assess content engagement, identifying popular sections and areas of improvement for more effective communication. They excel at highlighting patterns and trends in large datasets, making it easy to identify areas of interest.
We can implement heatmaps to express multiple data types, such as numerical values, percentages, or even categorical data. Heatmaps help us easily spot areas with lots of activity, making them helpful in figuring out clusters [8] . When making these maps, it is important to pick colors carefully. The colors need to show the differences between groups or levels of something. And it is good to use colors that people with colorblindness can easily see.
Check our detailed guide on how to create a heatmap here. Also discover our collection of heatmap PowerPoint templates .
Pie charts are circular statistical graphics divided into slices to illustrate numerical proportions. Each slice represents a proportionate part of the whole, making it easy to visualize the contribution of each component to the total.
The size of the pie charts is influenced by the value of data points within each pie. The total of all data points in a pie determines its size. The pie with the highest data points appears as the largest, whereas the others are proportionally smaller. However, you can present all pies of the same size if proportional representation is not required [9] . Sometimes, pie charts are difficult to read, or additional information is required. A variation of this tool can be used instead, known as the donut chart , which has the same structure but a blank center, creating a ring shape. Presenters can add extra information, and the ring shape helps to declutter the graph.
Pie charts are used in business to show percentage distribution, compare relative sizes of categories, or present straightforward data sets where visualizing ratios is essential.
Real-Life Application of Pie Charts
Consider a scenario where you want to represent the distribution of the data. Each slice of the pie chart would represent a different category, and the size of each slice would indicate the percentage of the total portion allocated to that category.
Step 1: Define Your Data Structure
Imagine you are presenting the distribution of a project budget among different expense categories.
- Column A: Expense Categories (Personnel, Equipment, Marketing, Miscellaneous)
- Column B: Budget Amounts ($40,000, $30,000, $20,000, $10,000) Column B represents the values of your categories in Column A.
Step 2: Insert a Pie Chart
Using any of the accessible tools, you can create a pie chart. The most convenient tools for forming a pie chart in a presentation are presentation tools such as PowerPoint or Google Slides. You will notice that the pie chart assigns each expense category a percentage of the total budget by dividing it by the total budget.
For instance:
- Personnel: $40,000 / ($40,000 + $30,000 + $20,000 + $10,000) = 40%
- Equipment: $30,000 / ($40,000 + $30,000 + $20,000 + $10,000) = 30%
- Marketing: $20,000 / ($40,000 + $30,000 + $20,000 + $10,000) = 20%
- Miscellaneous: $10,000 / ($40,000 + $30,000 + $20,000 + $10,000) = 10%
You can make a chart out of this or just pull out the pie chart from the data.
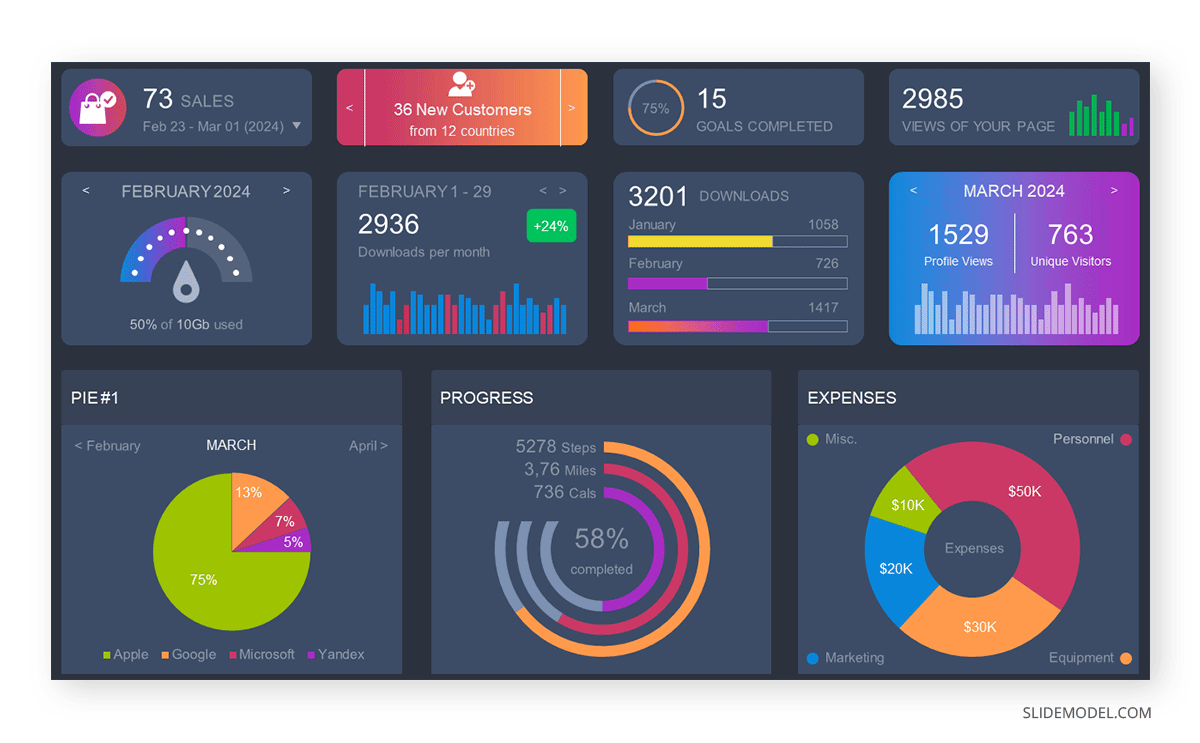
3D pie charts and 3D donut charts are quite popular among the audience. They stand out as visual elements in any presentation slide, so let’s take a look at how our pie chart example would look in 3D pie chart format.
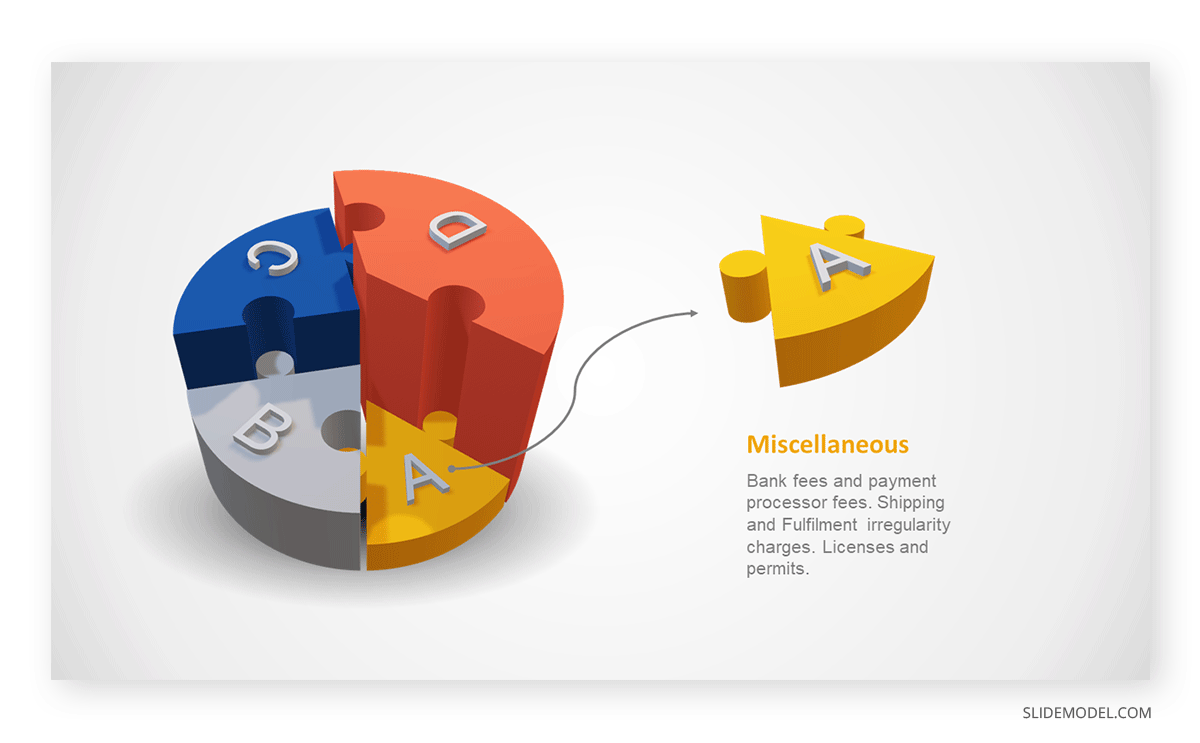
Step 03: Results Interpretation
The pie chart visually illustrates the distribution of the project budget among different expense categories. Personnel constitutes the largest portion at 40%, followed by equipment at 30%, marketing at 20%, and miscellaneous at 10%. This breakdown provides a clear overview of where the project funds are allocated, which helps in informed decision-making and resource management. It is evident that personnel are a significant investment, emphasizing their importance in the overall project budget.
Pie charts provide a straightforward way to represent proportions and percentages. They are easy to understand, even for individuals with limited data analysis experience. These charts work well for small datasets with a limited number of categories.
However, a pie chart can become cluttered and less effective in situations with many categories. Accurate interpretation may be challenging, especially when dealing with slight differences in slice sizes. In addition, these charts are static and do not effectively convey trends over time.
For more information, check our collection of pie chart templates for PowerPoint .
Histograms present the distribution of numerical variables. Unlike a bar chart that records each unique response separately, histograms organize numeric responses into bins and show the frequency of reactions within each bin [10] . The x-axis of a histogram shows the range of values for a numeric variable. At the same time, the y-axis indicates the relative frequencies (percentage of the total counts) for that range of values.
Whenever you want to understand the distribution of your data, check which values are more common, or identify outliers, histograms are your go-to. Think of them as a spotlight on the story your data is telling. A histogram can provide a quick and insightful overview if you’re curious about exam scores, sales figures, or any numerical data distribution.
Real-Life Application of a Histogram
In the histogram data analysis presentation example, imagine an instructor analyzing a class’s grades to identify the most common score range. A histogram could effectively display the distribution. It will show whether most students scored in the average range or if there are significant outliers.
Step 1: Gather Data
He begins by gathering the data. The scores of each student in class are gathered to analyze exam scores.
After arranging the scores in ascending order, bin ranges are set.
Step 2: Define Bins
Bins are like categories that group similar values. Think of them as buckets that organize your data. The presenter decides how wide each bin should be based on the range of the values. For instance, the instructor sets the bin ranges based on score intervals: 60-69, 70-79, 80-89, and 90-100.
Step 3: Count Frequency
Now, he counts how many data points fall into each bin. This step is crucial because it tells you how often specific ranges of values occur. The result is the frequency distribution, showing the occurrences of each group.
Here, the instructor counts the number of students in each category.
- 60-69: 1 student (Kate)
- 70-79: 4 students (David, Emma, Grace, Jack)
- 80-89: 7 students (Alice, Bob, Frank, Isabel, Liam, Mia, Noah)
- 90-100: 3 students (Clara, Henry, Olivia)
Step 4: Create the Histogram
It’s time to turn the data into a visual representation. Draw a bar for each bin on a graph. The width of the bar should correspond to the range of the bin, and the height should correspond to the frequency. To make your histogram understandable, label the X and Y axes.
In this case, the X-axis should represent the bins (e.g., test score ranges), and the Y-axis represents the frequency.
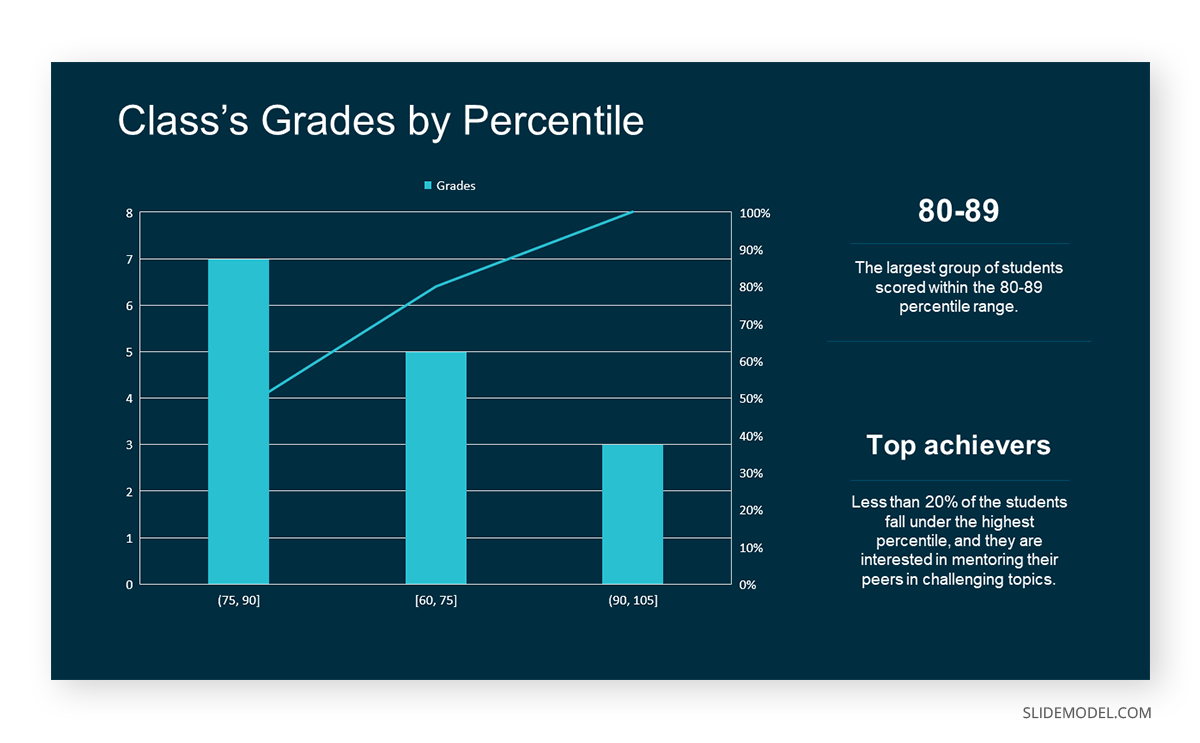
The histogram of the class grades reveals insightful patterns in the distribution. Most students, with seven students, fall within the 80-89 score range. The histogram provides a clear visualization of the class’s performance. It showcases a concentration of grades in the upper-middle range with few outliers at both ends. This analysis helps in understanding the overall academic standing of the class. It also identifies the areas for potential improvement or recognition.
Thus, histograms provide a clear visual representation of data distribution. They are easy to interpret, even for those without a statistical background. They apply to various types of data, including continuous and discrete variables. One weak point is that histograms do not capture detailed patterns in students’ data, with seven compared to other visualization methods.
A scatter plot is a graphical representation of the relationship between two variables. It consists of individual data points on a two-dimensional plane. This plane plots one variable on the x-axis and the other on the y-axis. Each point represents a unique observation. It visualizes patterns, trends, or correlations between the two variables.
Scatter plots are also effective in revealing the strength and direction of relationships. They identify outliers and assess the overall distribution of data points. The points’ dispersion and clustering reflect the relationship’s nature, whether it is positive, negative, or lacks a discernible pattern. In business, scatter plots assess relationships between variables such as marketing cost and sales revenue. They help present data correlations and decision-making.
Real-Life Application of Scatter Plot
A group of scientists is conducting a study on the relationship between daily hours of screen time and sleep quality. After reviewing the data, they managed to create this table to help them build a scatter plot graph:
In the provided example, the x-axis represents Daily Hours of Screen Time, and the y-axis represents the Sleep Quality Rating.
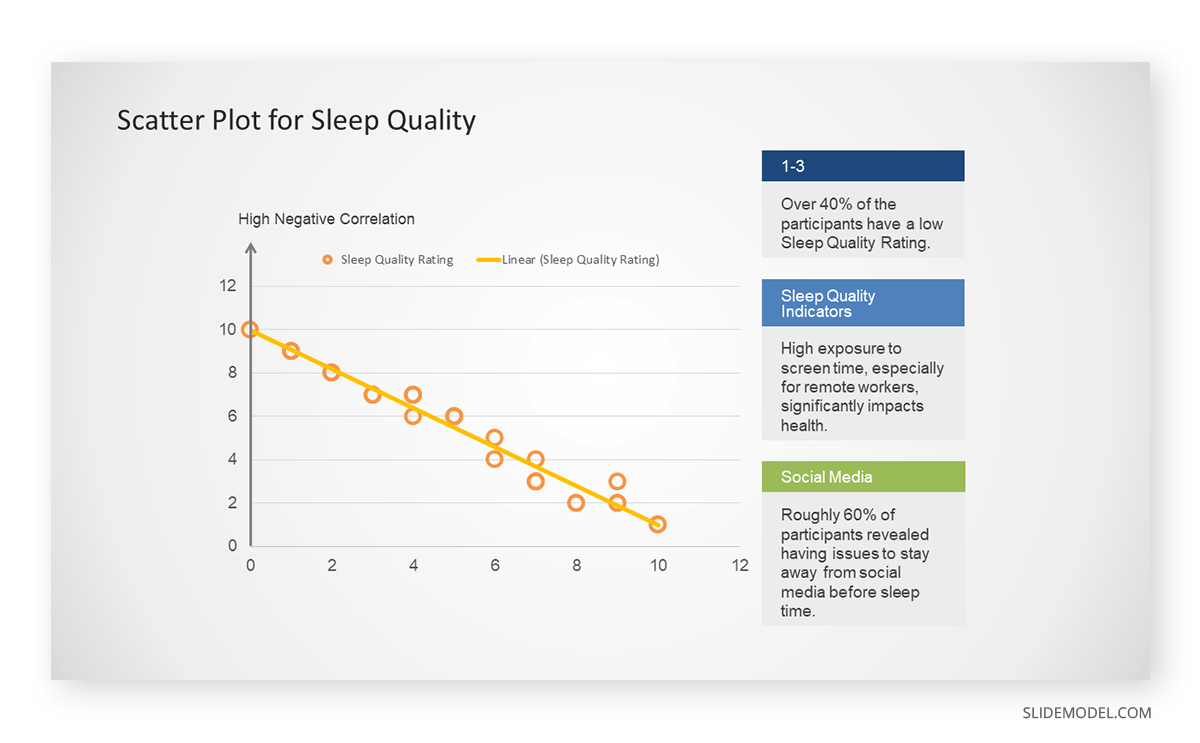
The scientists observe a negative correlation between the amount of screen time and the quality of sleep. This is consistent with their hypothesis that blue light, especially before bedtime, has a significant impact on sleep quality and metabolic processes.
There are a few things to remember when using a scatter plot. Even when a scatter diagram indicates a relationship, it doesn’t mean one variable affects the other. A third factor can influence both variables. The more the plot resembles a straight line, the stronger the relationship is perceived [11] . If it suggests no ties, the observed pattern might be due to random fluctuations in data. When the scatter diagram depicts no correlation, whether the data might be stratified is worth considering.
Choosing the appropriate data presentation type is crucial when making a presentation . Understanding the nature of your data and the message you intend to convey will guide this selection process. For instance, when showcasing quantitative relationships, scatter plots become instrumental in revealing correlations between variables. If the focus is on emphasizing parts of a whole, pie charts offer a concise display of proportions. Histograms, on the other hand, prove valuable for illustrating distributions and frequency patterns.
Bar charts provide a clear visual comparison of different categories. Likewise, line charts excel in showcasing trends over time, while tables are ideal for detailed data examination. Starting a presentation on data presentation types involves evaluating the specific information you want to communicate and selecting the format that aligns with your message. This ensures clarity and resonance with your audience from the beginning of your presentation.
1. Fact Sheet Dashboard for Data Presentation
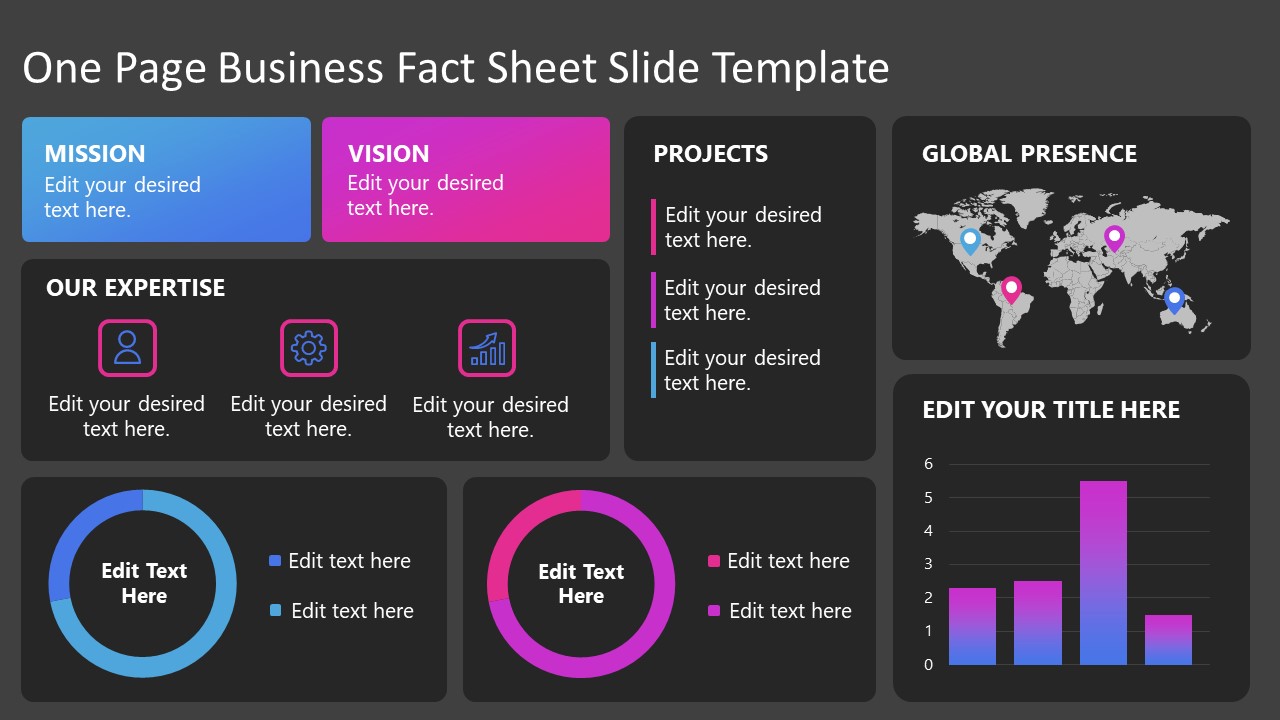
Convey all the data you need to present in this one-pager format, an ideal solution tailored for users looking for presentation aids. Global maps, donut chats, column graphs, and text neatly arranged in a clean layout presented in light and dark themes.
Use This Template
2. 3D Column Chart Infographic PPT Template
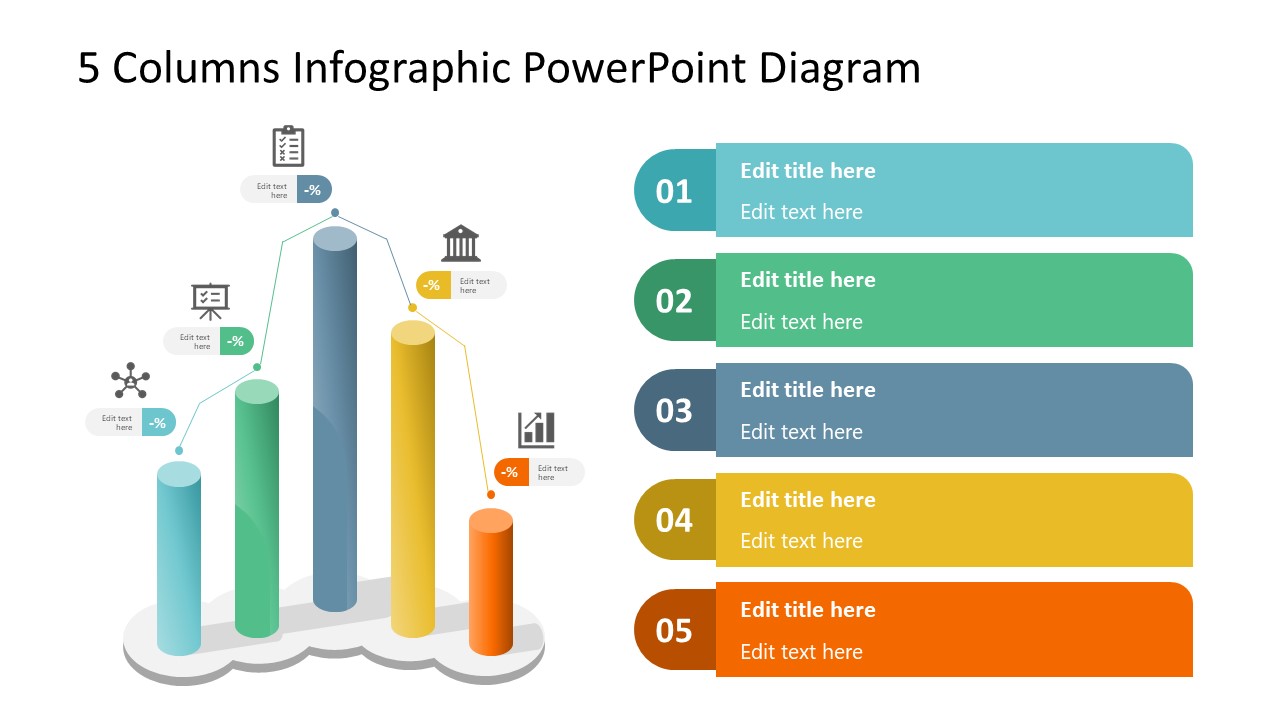
Represent column charts in a highly visual 3D format with this PPT template. A creative way to present data, this template is entirely editable, and we can craft either a one-page infographic or a series of slides explaining what we intend to disclose point by point.
3. Data Circles Infographic PowerPoint Template
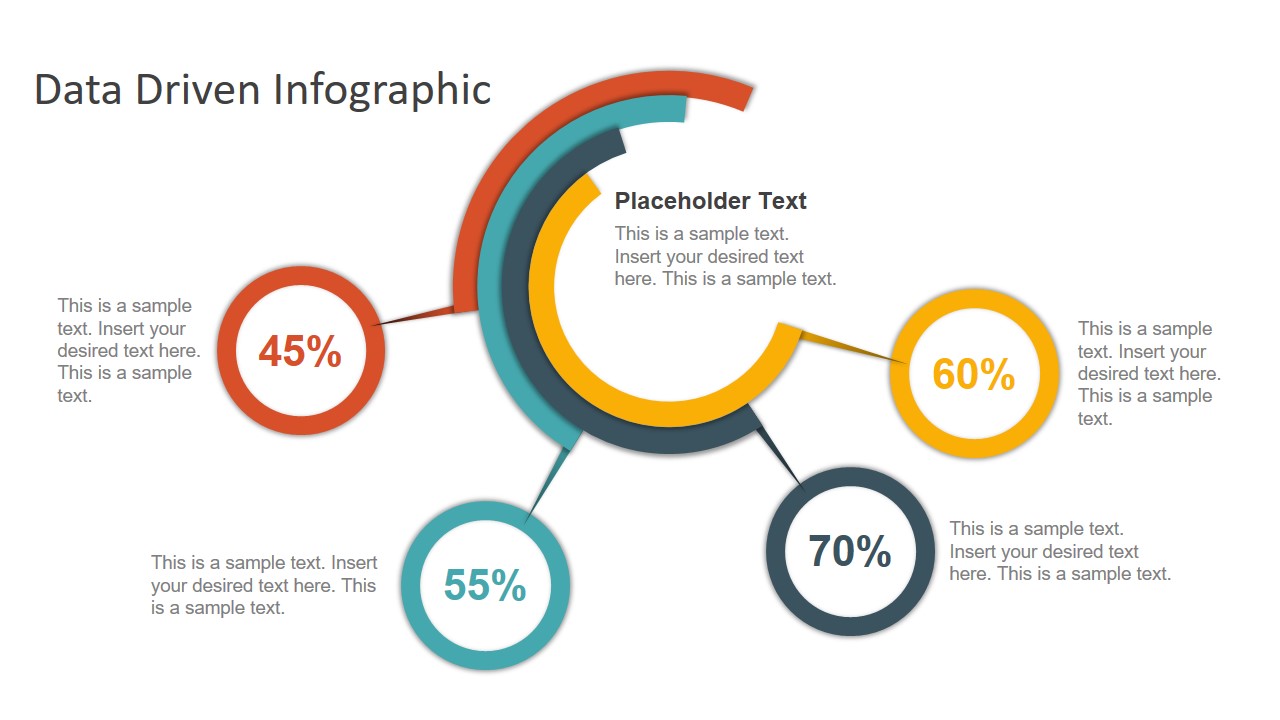
An alternative to the pie chart and donut chart diagrams, this template features a series of curved shapes with bubble callouts as ways of presenting data. Expand the information for each arch in the text placeholder areas.
4. Colorful Metrics Dashboard for Data Presentation
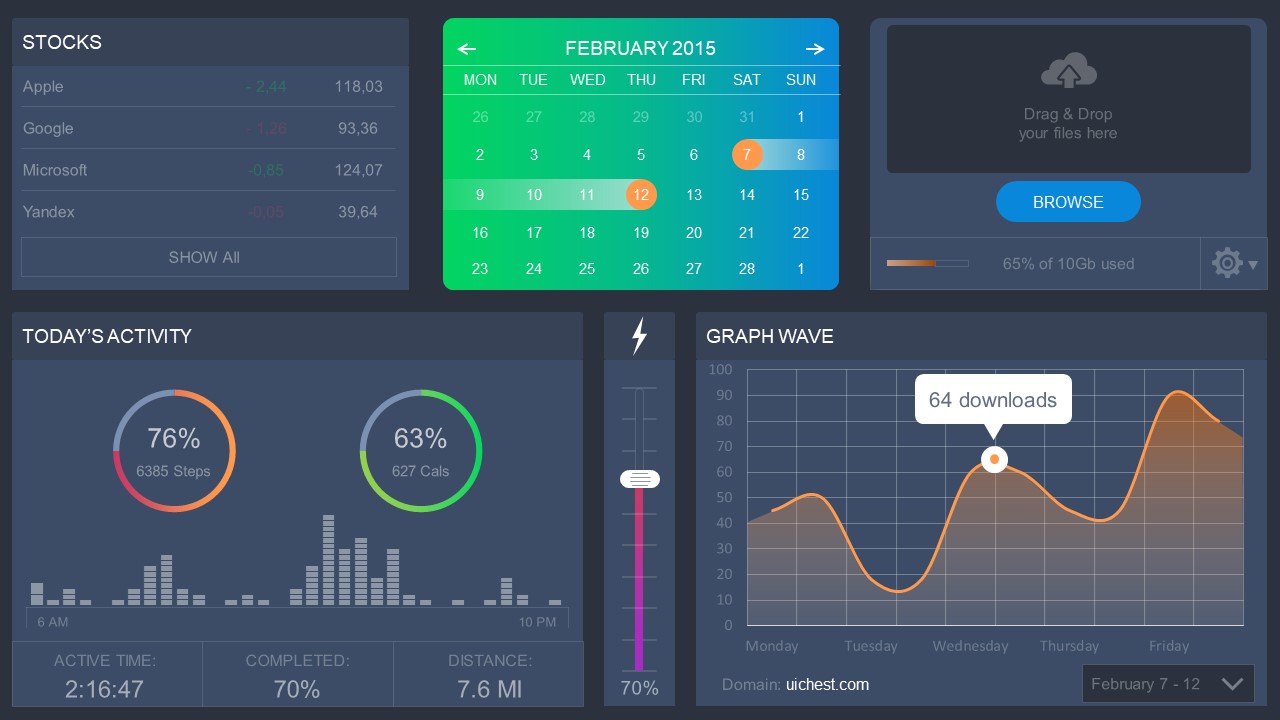
This versatile dashboard template helps us in the presentation of the data by offering several graphs and methods to convert numbers into graphics. Implement it for e-commerce projects, financial projections, project development, and more.
5. Animated Data Presentation Tools for PowerPoint & Google Slides
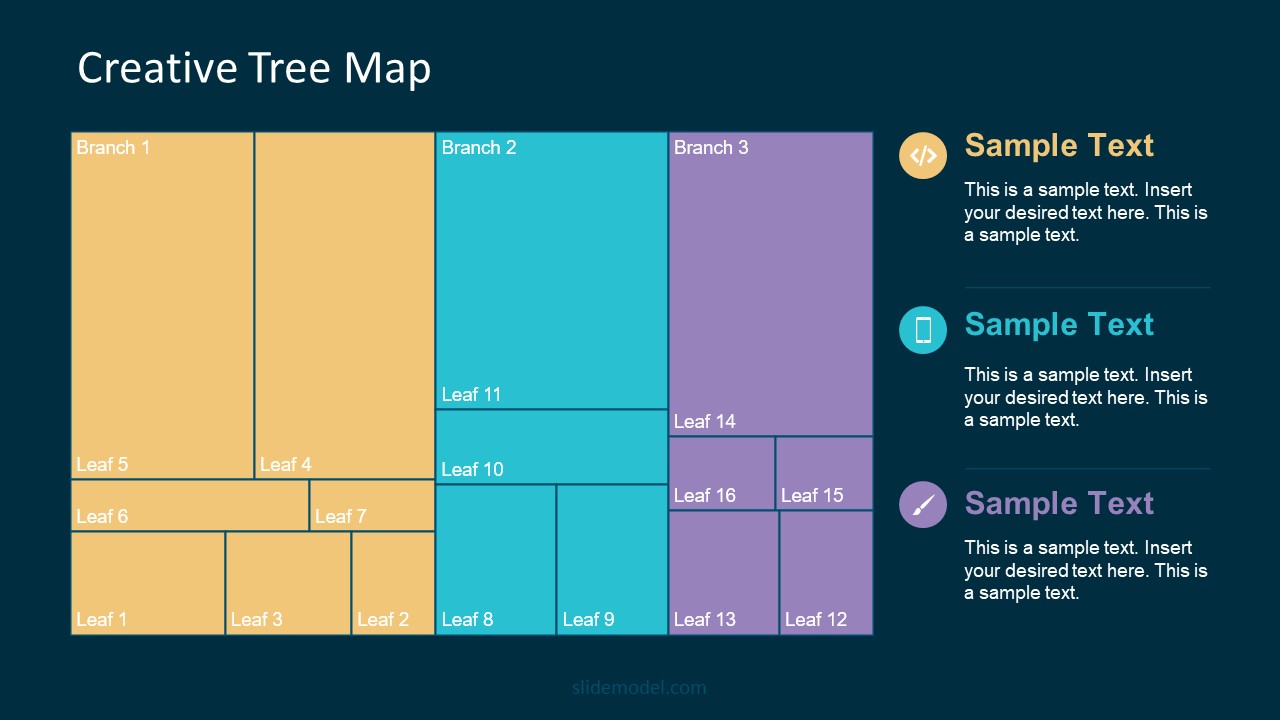
A slide deck filled with most of the tools mentioned in this article, from bar charts, column charts, treemap graphs, pie charts, histogram, etc. Animated effects make each slide look dynamic when sharing data with stakeholders.
6. Statistics Waffle Charts PPT Template for Data Presentations
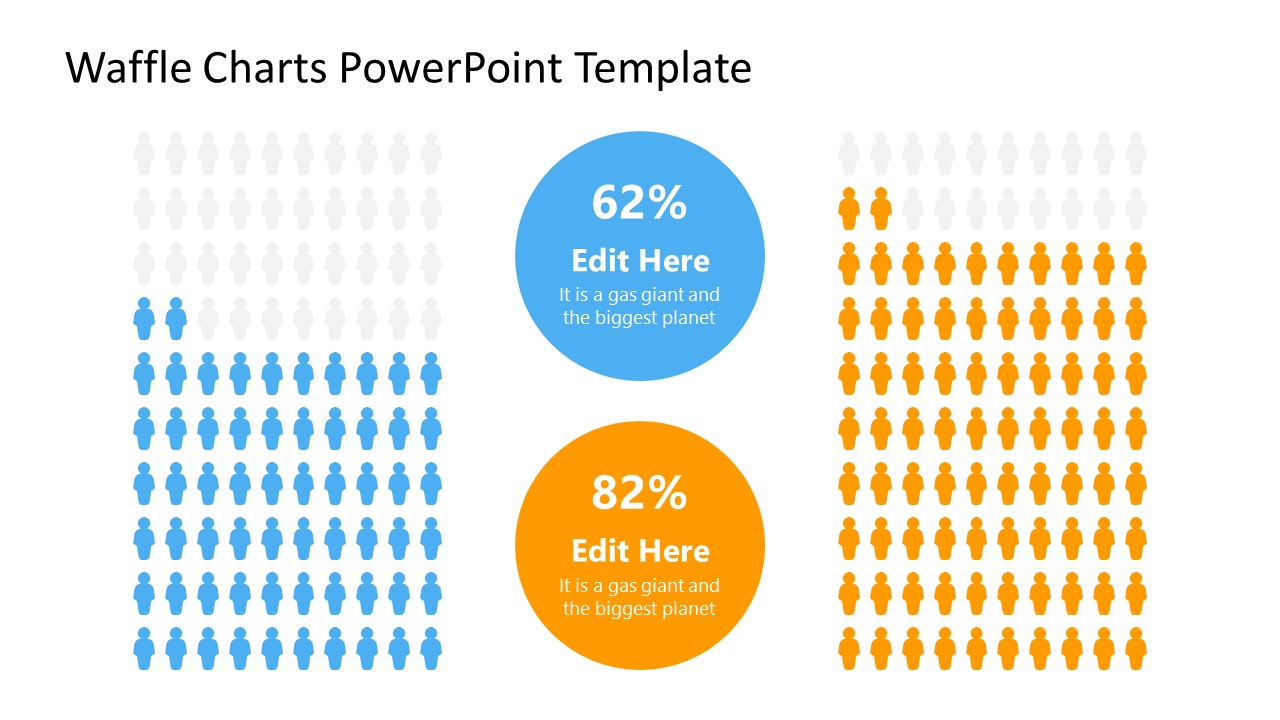
This PPT template helps us how to present data beyond the typical pie chart representation. It is widely used for demographics, so it’s a great fit for marketing teams, data science professionals, HR personnel, and more.
7. Data Presentation Dashboard Template for Google Slides
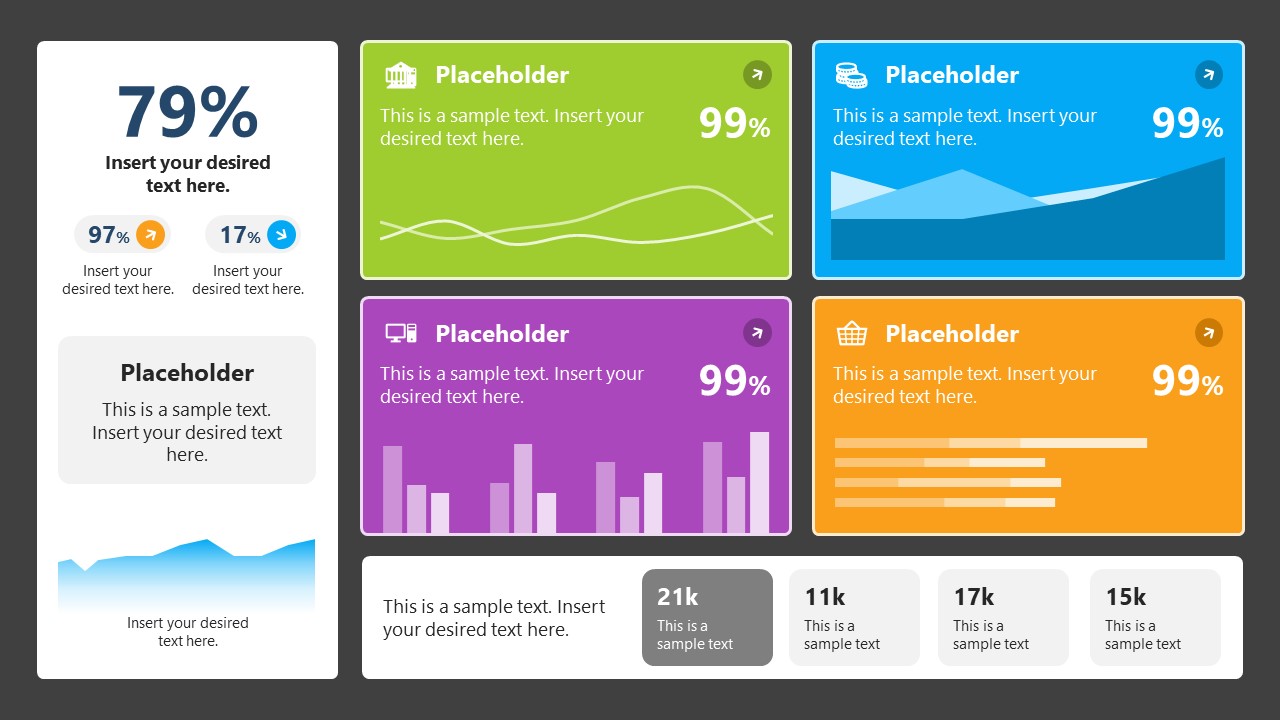
A compendium of tools in dashboard format featuring line graphs, bar charts, column charts, and neatly arranged placeholder text areas.
8. Weather Dashboard for Data Presentation
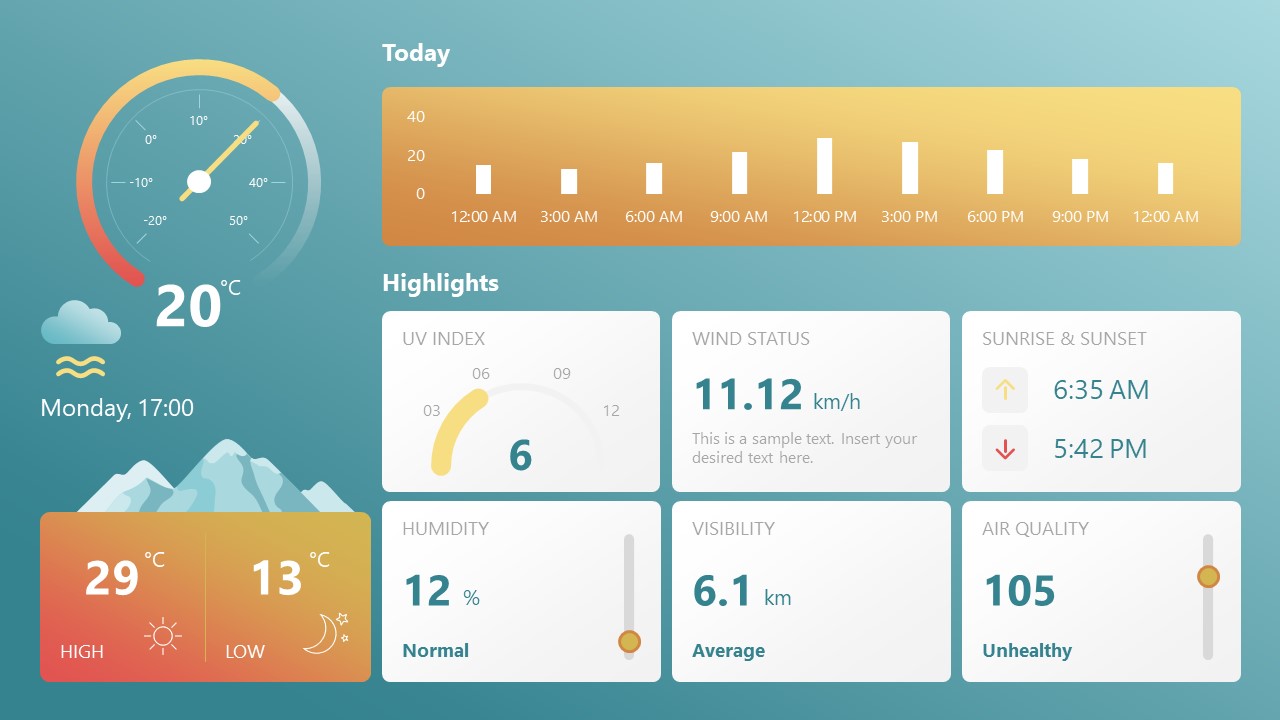
Share weather data for agricultural presentation topics, environmental studies, or any kind of presentation that requires a highly visual layout for weather forecasting on a single day. Two color themes are available.
9. Social Media Marketing Dashboard Data Presentation Template
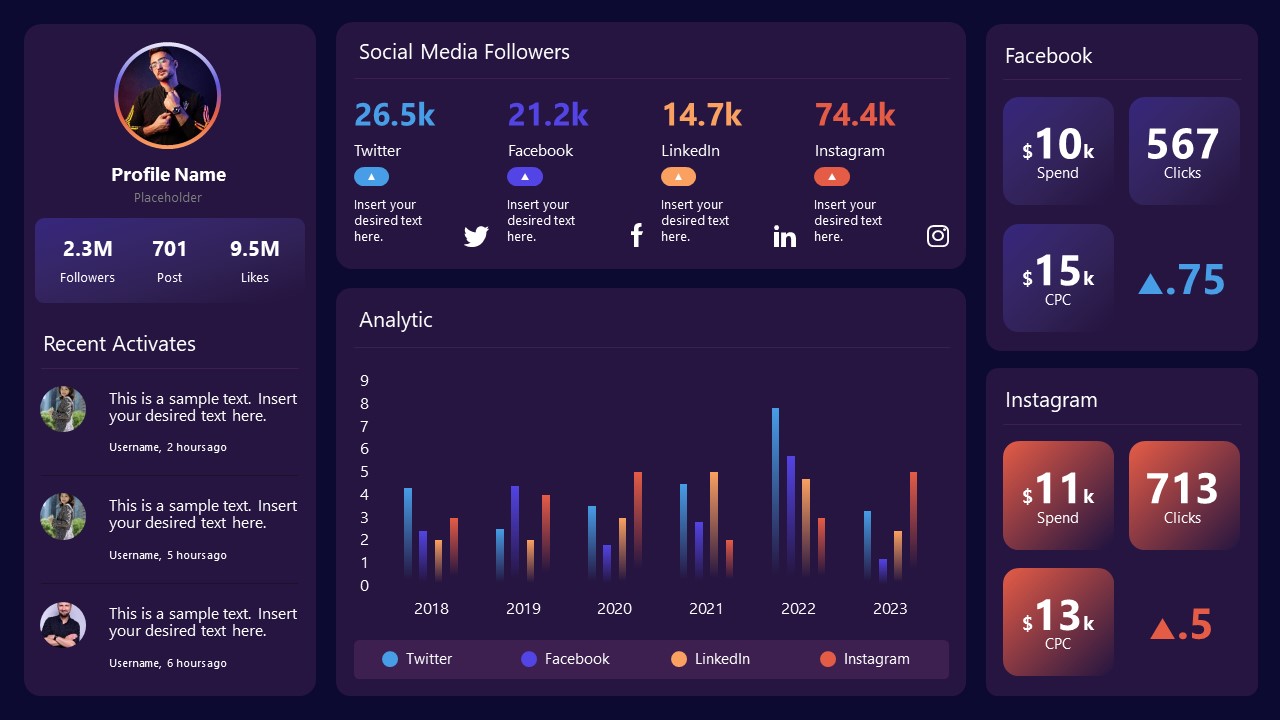
Intended for marketing professionals, this dashboard template for data presentation is a tool for presenting data analytics from social media channels. Two slide layouts featuring line graphs and column charts.
10. Project Management Summary Dashboard Template
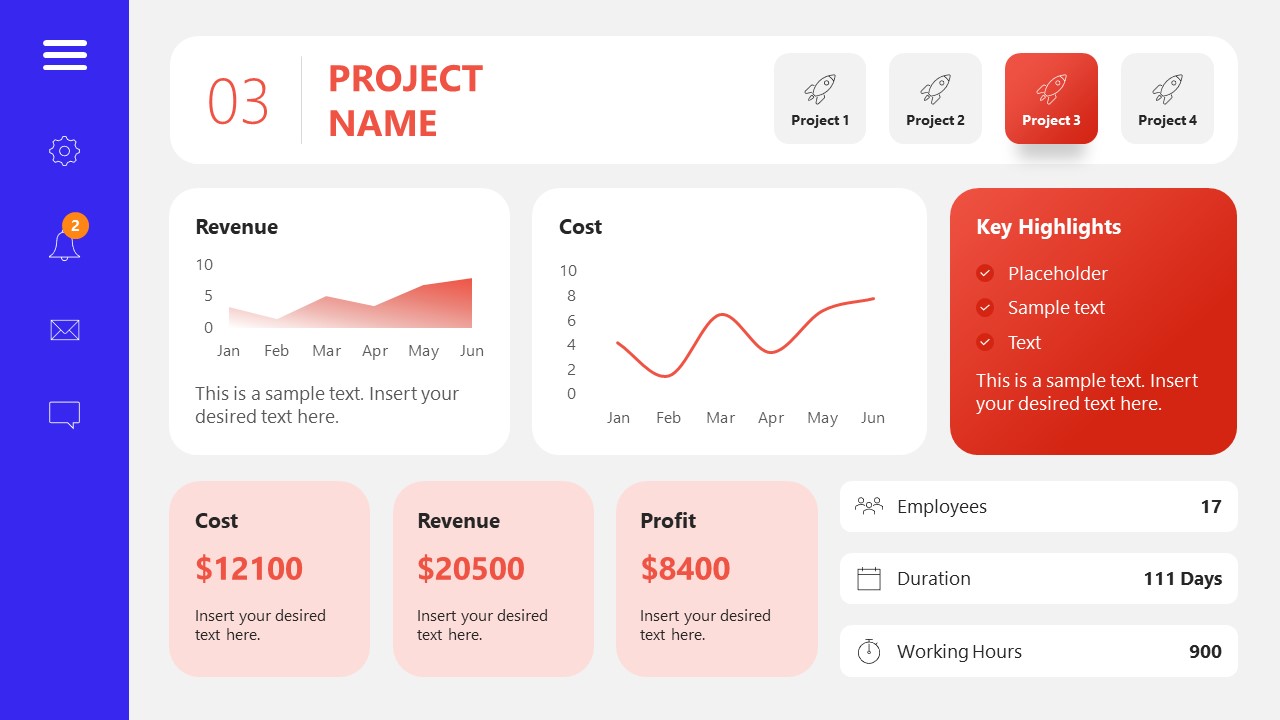
A tool crafted for project managers to deliver highly visual reports on a project’s completion, the profits it delivered for the company, and expenses/time required to execute it. 4 different color layouts are available.
11. Profit & Loss Dashboard for PowerPoint and Google Slides
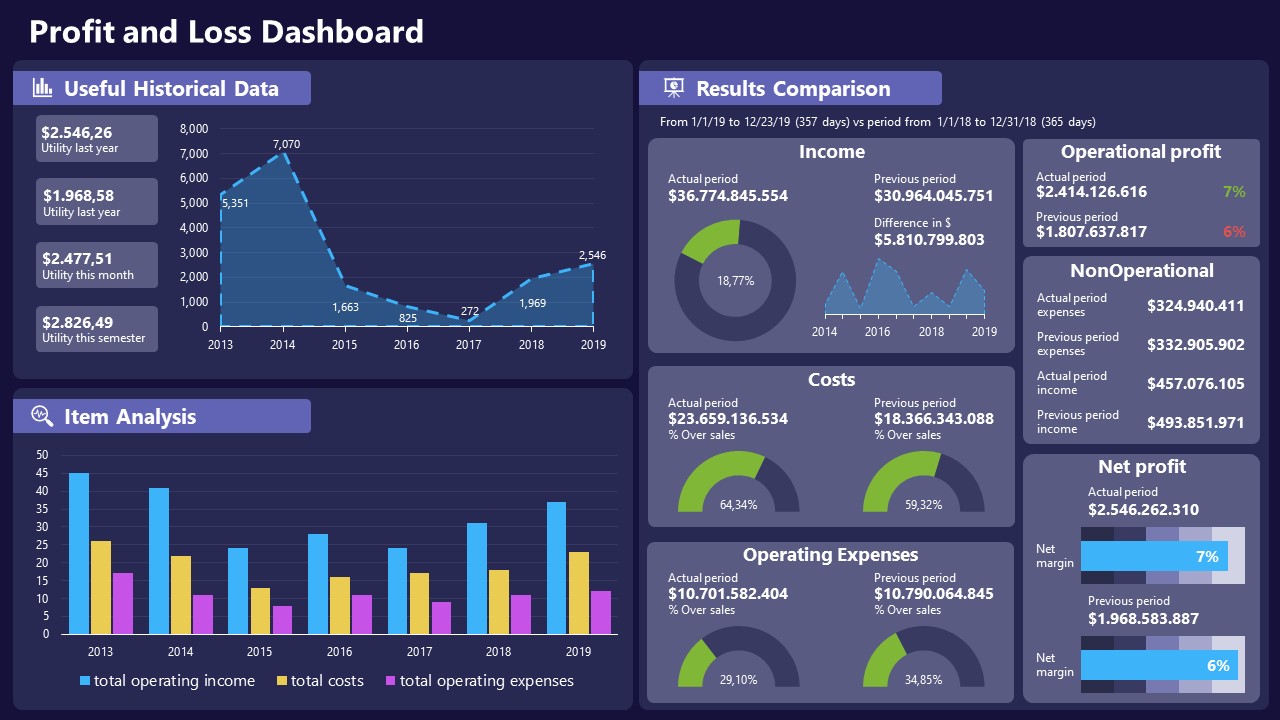
A must-have for finance professionals. This typical profit & loss dashboard includes progress bars, donut charts, column charts, line graphs, and everything that’s required to deliver a comprehensive report about a company’s financial situation.
Overwhelming visuals
One of the mistakes related to using data-presenting methods is including too much data or using overly complex visualizations. They can confuse the audience and dilute the key message.
Inappropriate chart types
Choosing the wrong type of chart for the data at hand can lead to misinterpretation. For example, using a pie chart for data that doesn’t represent parts of a whole is not right.
Lack of context
Failing to provide context or sufficient labeling can make it challenging for the audience to understand the significance of the presented data.
Inconsistency in design
Using inconsistent design elements and color schemes across different visualizations can create confusion and visual disarray.
Failure to provide details
Simply presenting raw data without offering clear insights or takeaways can leave the audience without a meaningful conclusion.
Lack of focus
Not having a clear focus on the key message or main takeaway can result in a presentation that lacks a central theme.
Visual accessibility issues
Overlooking the visual accessibility of charts and graphs can exclude certain audience members who may have difficulty interpreting visual information.
In order to avoid these mistakes in data presentation, presenters can benefit from using presentation templates . These templates provide a structured framework. They ensure consistency, clarity, and an aesthetically pleasing design, enhancing data communication’s overall impact.
Understanding and choosing data presentation types are pivotal in effective communication. Each method serves a unique purpose, so selecting the appropriate one depends on the nature of the data and the message to be conveyed. The diverse array of presentation types offers versatility in visually representing information, from bar charts showing values to pie charts illustrating proportions.
Using the proper method enhances clarity, engages the audience, and ensures that data sets are not just presented but comprehensively understood. By appreciating the strengths and limitations of different presentation types, communicators can tailor their approach to convey information accurately, developing a deeper connection between data and audience understanding.
[1] Government of Canada, S.C. (2021) 5 Data Visualization 5.2 Bar Chart , 5.2 Bar chart . https://www150.statcan.gc.ca/n1/edu/power-pouvoir/ch9/bargraph-diagrammeabarres/5214818-eng.htm
[2] Kosslyn, S.M., 1989. Understanding charts and graphs. Applied cognitive psychology, 3(3), pp.185-225. https://apps.dtic.mil/sti/pdfs/ADA183409.pdf
[3] Creating a Dashboard . https://it.tufts.edu/book/export/html/1870
[4] https://www.goldenwestcollege.edu/research/data-and-more/data-dashboards/index.html
[5] https://www.mit.edu/course/21/21.guide/grf-line.htm
[6] Jadeja, M. and Shah, K., 2015, January. Tree-Map: A Visualization Tool for Large Data. In GSB@ SIGIR (pp. 9-13). https://ceur-ws.org/Vol-1393/gsb15proceedings.pdf#page=15
[7] Heat Maps and Quilt Plots. https://www.publichealth.columbia.edu/research/population-health-methods/heat-maps-and-quilt-plots
[8] EIU QGIS WORKSHOP. https://www.eiu.edu/qgisworkshop/heatmaps.php
[9] About Pie Charts. https://www.mit.edu/~mbarker/formula1/f1help/11-ch-c8.htm
[10] Histograms. https://sites.utexas.edu/sos/guided/descriptive/numericaldd/descriptiven2/histogram/ [11] https://asq.org/quality-resources/scatter-diagram
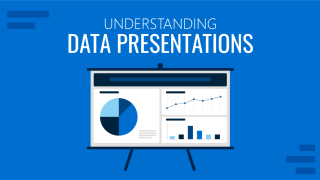
Like this article? Please share
Data Analysis, Data Science, Data Visualization Filed under Design
Related Articles
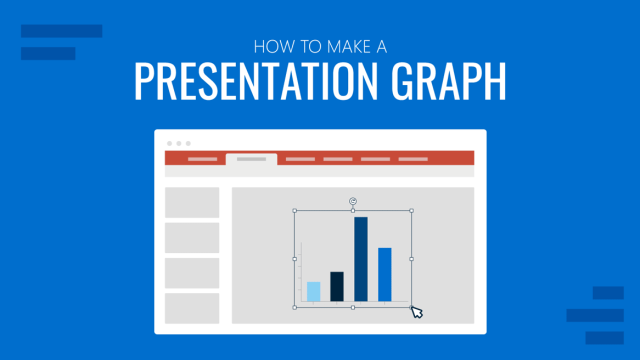
Filed under Design • March 27th, 2024
How to Make a Presentation Graph
Detailed step-by-step instructions to master the art of how to make a presentation graph in PowerPoint and Google Slides. Check it out!
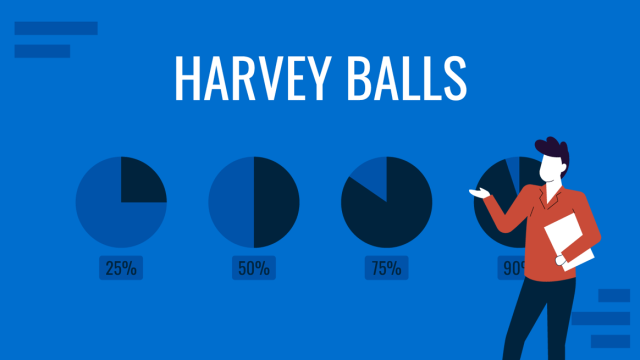
Filed under Presentation Ideas • January 6th, 2024
All About Using Harvey Balls
Among the many tools in the arsenal of the modern presenter, Harvey Balls have a special place. In this article we will tell you all about using Harvey Balls.
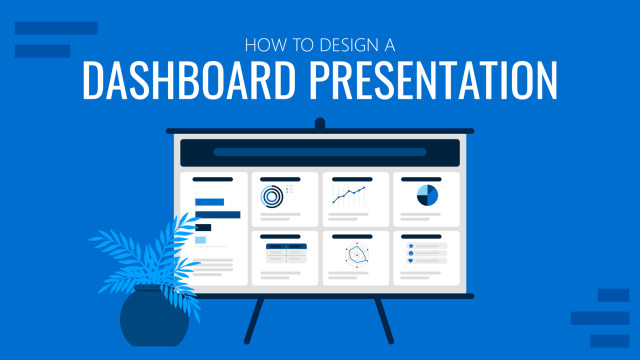
Filed under Business • December 8th, 2023
How to Design a Dashboard Presentation: A Step-by-Step Guide
Take a step further in your professional presentation skills by learning what a dashboard presentation is and how to properly design one in PowerPoint. A detailed step-by-step guide is here!
Leave a Reply

- SUGGESTED TOPICS
- The Magazine
- Newsletters
- Managing Yourself
- Managing Teams
- Work-life Balance
- The Big Idea
- Data & Visuals
- Reading Lists
- Case Selections
- HBR Learning
- Topic Feeds
- Account Settings
- Email Preferences
Present Your Data Like a Pro
- Joel Schwartzberg

Demystify the numbers. Your audience will thank you.
While a good presentation has data, data alone doesn’t guarantee a good presentation. It’s all about how that data is presented. The quickest way to confuse your audience is by sharing too many details at once. The only data points you should share are those that significantly support your point — and ideally, one point per chart. To avoid the debacle of sheepishly translating hard-to-see numbers and labels, rehearse your presentation with colleagues sitting as far away as the actual audience would. While you’ve been working with the same chart for weeks or months, your audience will be exposed to it for mere seconds. Give them the best chance of comprehending your data by using simple, clear, and complete language to identify X and Y axes, pie pieces, bars, and other diagrammatic elements. Try to avoid abbreviations that aren’t obvious, and don’t assume labeled components on one slide will be remembered on subsequent slides. Every valuable chart or pie graph has an “Aha!” zone — a number or range of data that reveals something crucial to your point. Make sure you visually highlight the “Aha!” zone, reinforcing the moment by explaining it to your audience.
With so many ways to spin and distort information these days, a presentation needs to do more than simply share great ideas — it needs to support those ideas with credible data. That’s true whether you’re an executive pitching new business clients, a vendor selling her services, or a CEO making a case for change.

- JS Joel Schwartzberg oversees executive communications for a major national nonprofit, is a professional presentation coach, and is the author of Get to the Point! Sharpen Your Message and Make Your Words Matter and The Language of Leadership: How to Engage and Inspire Your Team . You can find him on LinkedIn and X. TheJoelTruth
Partner Center

Want to create or adapt books like this? Learn more about how Pressbooks supports open publishing practices.
5.2 Three Types of Audience Analysis
Learning objectives.
- Understand how to gather and use demographic information.
- Understand how to gather and use psychographic information.
- Understand how to gather and use situational information.
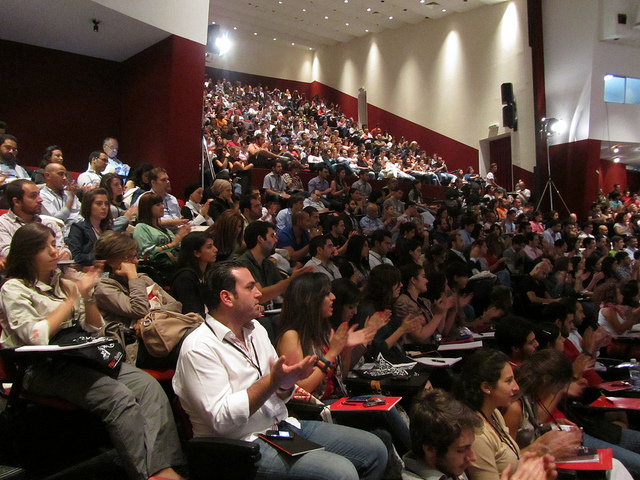
Thinkmedialabs – Audience – CC BY-NC 2.0.
While audience analysis does not guarantee against errors in judgment, it will help you make good choices in topic, language, style of presentation, and other aspects of your speech. The more you know about your audience, the better you can serve their interests and needs. There are certainly limits to what we can learn through information collection, and we need to acknowledge that before making assumptions, but knowing how to gather and use information through audience analysis is an essential skill for successful speakers.
Demographic Analysis
As indicated earlier, demographic information includes factors such as gender, age range, marital status, race and ethnicity, and socioeconomic status. In your public speaking class, you probably already know how many students are male and female, their approximate ages, and so forth. But how can you assess the demographics of an audience ahead of time if you have had no previous contact with them? In many cases, you can ask the person or organization that has invited you to speak; it’s likely that they can tell you a lot about the demographics of the people who are expected to come to hear you.
Whatever method you use to gather demographics, exercise respect from the outset. For instance, if you are collecting information about whether audience members have ever been divorced, be aware that not everyone will want to answer your questions. You can’t require them to do so, and you may not make assumptions about their reluctance to discuss the topic. You must allow them their privacy.
There are certain things you can learn about an audience based on age. For instance, if your audience members are first-year college students, you can assume that they have grown up in the post-9/11 era and have limited memory of what life was like before the “war on terror.” If your audience includes people in their forties and fifties, it is likely they remember a time when people feared they would contract the AIDS virus from shaking hands or using a public restroom. People who are in their sixties today came of age during the 1960s, the era of the Vietnam War and a time of social confrontation and experimentation. They also have frames of reference that contribute to the way they think, but it may not be easy to predict which side of the issues they support.
Gender can define human experience. Clearly, most women have had a different cultural experience from that of men within the same culture. Some women have found themselves excluded from certain careers. Some men have found themselves blamed for the limitations imposed on women. In books such as You Just Don’t Understand and Talking from 9 to 5 , linguist Deborah Tannen has written extensively on differences between men’s and women’s communication styles. Tannen explains, “This is not to say that all women and all men, or all boys and girls, behave any one way. Many factors influence our styles, including regional and ethnic backgrounds, family experience and individual personality. But gender is a key factor, and understanding its influence can help clarify what happens when we talk” (Tannen, 1994).
Marriage tends to impose additional roles on both men and women and divorce even more so, especially if there are children. Even if your audience consists of young adults who have not yet made occupational or marital commitments, they are still aware that gender and the choices they make about issues such as careers and relationships will influence their experience as adults.
In past generations, Americans often used the metaphor of a “melting pot” to symbolize the assimilation of immigrants from various countries and cultures into a unified, harmonious “American people.” Today, we are aware of the limitations in that metaphor, and have largely replaced it with a multiculturalist view that describes the American fabric as a “patchwork” or a “mosaic.” We know that people who immigrate do not abandon their cultures of origin in order to conform to a standard American identity. In fact, cultural continuity is now viewed as a healthy source of identity.
We also know that subcultures and cocultures exist within and alongside larger cultural groups. For example, while we are aware that Native American people do not all embrace the same values, beliefs, and customs as mainstream white Americans, we also know that members of the Navajo nation have different values, beliefs, and customs from those of members of the Sioux or the Seneca. We know that African American people in urban centers like Detroit and Boston do not share the same cultural experiences as those living in rural Mississippi. Similarly, white Americans in San Francisco may be culturally rooted in the narrative of distant ancestors from Scotland, Italy, or Sweden or in the experience of having emigrated much more recently from Australia, Croatia, or Poland.
Not all cultural membership is visibly obvious. For example, people in German American and Italian American families have widely different sets of values and practices, yet others may not be able to differentiate members of these groups. Differences are what make each group interesting and are important sources of knowledge, perspectives, and creativity.
There is wide variability in religion as well. The Pew Forum on Religion and Public Life found in a nationwide survey that 84 percent of Americans identify with at least one of a dozen major religions, including Christianity, Judaism, Buddhism, Islam, Hinduism, and others. Within Christianity alone, there are half a dozen categories including Roman Catholic, Mormon, Jehovah’s Witness, Orthodox (Greek and Russian), and a variety of Protestant denominations. Another 6 percent said they were unaffiliated but religious, meaning that only one American in ten is atheist, agnostic, or “nothing in particular” (Pew Forum on Religion & Public Life, 2008).
Even within a given denomination, a great deal of diversity can be found. For instance, among Roman Catholics alone, there are people who are devoutly religious, people who self-identify as Catholic but do not attend mass or engage in other religious practices, and others who faithfully make confession and attend mass but who openly question Papal doctrine on various issues. Catholicism among immigrants from the Caribbean and Brazil is often blended with indigenous religion or with religion imported from the west coast of Africa. It is very different from Catholicism in the Vatican.
The dimensions of diversity in the religion demographic are almost endless, and they are not limited by denomination. Imagine conducting an audience analysis of people belonging to an individual congregation rather than a denomination: even there, you will most likely find a multitude of variations that involve how one was brought up, adoption of a faith system as an adult, how strictly one observes religious practices, and so on.
Yet, even with these multiple facets, religion is still a meaningful demographic lens. It can be an indicator of probable patterns in family relationships, family size, and moral attitudes.
Group Membership
In your classroom audience alone, there will be students from a variety of academic majors. Every major has its own set of values, goals, principles, and codes of ethics. A political science student preparing for law school might seem to have little in common with a student of music therapy, for instance. In addition, there are other group memberships that influence how audience members understand the world. Fraternities and sororities, sports teams, campus organizations, political parties, volunteerism, and cultural communities all provide people with ways of understanding the world as it is and as we think it should be.
Because public speaking audiences are very often members of one group or another, group membership is a useful and often easy to access facet of audience analysis. The more you know about the associations of your audience members, the better prepared you will be to tailor your speech to their interests, expectations, and needs.
Education is expensive, and people pursue education for many reasons. Some people seek to become educated, while others seek to earn professional credentials. Both are important motivations. If you know the education levels attained by members of your audience, you might not know their motivations, but you will know to what extent they could somehow afford the money for an education, afford the time to get an education, and survive educational demands successfully.
The kind of education is also important. For instance, an airplane mechanic undergoes a very different kind of education and training from that of an accountant or a software engineer. This means that not only the attained level of education but also the particular field is important in your understanding of your audience.
People choose occupations for reasons of motivation and interest, but their occupations also influence their perceptions and their interests. There are many misconceptions about most occupations. For instance, many people believe that teachers work an eight-hour day and have summers off. When you ask teachers, however, you might be surprised to find out that they take work home with them for evenings and weekends, and during the summer, they may teach summer school as well as taking courses in order to keep up with new developments in their fields. But even if you don’t know those things, you would still know that teachers have had rigorous generalized and specialized qualifying education, that they have a complex set of responsibilities in the classroom and the institution, and that, to some extent, they have chosen a relatively low-paying occupation over such fields as law, advertising, media, fine and performing arts, or medicine. If your audience includes doctors and nurses, you know that you are speaking to people with differing but important philosophies of health and illness. Learning about those occupational realities is important in avoiding wrong assumptions and stereotypes. We insist that you not assume that nurses are merely doctors “lite.” Their skills, concerns, and responsibilities are almost entirely different, and both are crucially necessary to effective health care.
Psychographic Analysis
Earlier, we mentioned psychographic information, which includes such things as values, opinions, attitudes, and beliefs. Authors Grice and Skinner present a model in which values are the basis for beliefs, attitudes, and behaviors (Grice & Skinner, 2009). Values are the foundation of their pyramid model. They say, “A value expresses a judgment of what is desirable and undesirable, right and wrong, or good and evil. Values are usually stated in the form of a word or phrase. For example, most of us probably share the values of equality, freedom, honesty, fairness, justice, good health, and family. These values compose the principles or standards we use to judge and develop our beliefs, attitudes, and behaviors.”
It is important to recognize that, while demographic information as discussed in Section 5.2.1 “Demographic Analysis” is fairly straightforward and verifiable, psychographic information is much less clear-cut. Two different people who both say they believe in equal educational opportunity may have very different interpretations of what “equal opportunity” means. People who say they don’t buy junk food may have very different standards for what specific kinds of foods are considered “junk food.”
We also acknowledge that people inherit some values from their family upbringing, cultural influences, and life experiences. The extent to which someone values family loyalty and obedience to parents, thrift, humility, and work may be determined by these influences more than by individual choice.
Psychographic analysis can reveal preexisting notions that limit your audience’s frame of reference. By knowing about such notions ahead of time, you can address them in your speech. Audiences are likely to have two basic kinds of preexisting notions: those about the topic and those about the speaker.
Preexisting Notions about Your Topic
Many things are a great deal more complex than we realize. Media stereotypes often contribute to our oversimplifications. For instance, one of your authors, teaching public speaking in the past decade, was surprised to hear a student claim that “the hippies meant well, but they did it wrong.” Aside from the question of the “it” that was done wrong, there was a question about how little the student actually knew about the diverse hippy cultures and their aspirations. The student seemed unaware that some of “the hippies” were the forebears of such things as organic bakeries, natural food co-ops, urban gardens, recycling, alternative energy, wellness, and other arguably positive developments.
It’s important to know your audience in order to make a rational judgment about how their views of your topic might be shaped. In speaking to an audience that might have differing definitions, you should take care to define your terms in a clear, honest way.
At the opposite end from oversimplification is the level of sophistication your audience might embody. Your audience analysis should include factors that reveal it. Suppose you are speaking about trends in civil rights in the United States. You cannot pretend that advancement of civil rights is virtually complete nor can you claim that no progress has been made. It is likely that in a college classroom, the audience will know that although much progress has been made, there are still pockets of prejudice, discrimination, and violence. When you speak to an audience that is cognitively complex, your strategy must be different from one you would use for an audience that is less educated in the topic. With a cognitively complex audience, you must acknowledge the overall complexity while stating that your focus will be on only one dimension. With an audience that’s uninformed about your topic, that strategy in a persuasive speech could confuse them; they might well prefer a black-and-white message with no gray areas. You must decide whether it is ethical to represent your topic this way.
When you prepare to do your audience analysis, include questions that reveal how much your audience already knows about your topic. Try to ascertain the existence of stereotyped, oversimplified, or prejudiced attitudes about it. This could make a difference in your choice of topic or in your approach to the audience and topic.
Preexisting Notions about You
People form opinions readily. For instance, we know that students form impressions of teachers the moment they walk into our classrooms on the first day. You get an immediate impression of our age, competence, and attitude simply from our appearance and nonverbal behavior. In addition, many have heard other students say what they think of us.
The same is almost certainly true of you. But it’s not always easy to get others to be honest about their impressions of you. They’re likely to tell you what they think you want to hear. Sometimes, however, you do know what others think. They might think of you as a jock, a suit-wearing conservative, a nature lover, and so on. Based on these impressions, your audience might expect a boring speech, a shallow speech, a sermon, and so on. However, your concern should still be serving your audience’s needs and interests, not debunking their opinions of you or managing your image. In order to help them be receptive, you address their interests directly, and make sure they get an interesting, ethical speech.
Situational Analysis
The next type of analysis is called the situational audience analysis because it focuses on characteristics related to the specific speaking situation. The situational audience analysis can be divided into two main questions:
- How many people came to hear my speech and why are they here? What events, concerns, and needs motivated them to come? What is their interest level, and what else might be competing for their attention?
- What is the physical environment of the speaking situation? What is the size of the audience, layout of the room, existence of a podium or a microphone, and availability of digital media for visual aids? Are there any distractions, such as traffic noise?
Audience Size
In a typical class, your audience is likely to consist of twenty to thirty listeners. This audience size gives you the latitude to be relatively informal within the bounds of good judgment. It isn’t too difficult to let each audience member feel as though you’re speaking to him or her. However, you would not become so informal that you allow your carefully prepared speech to lapse into shallow entertainment. With larger audiences, it’s more difficult to reach out to each listener, and your speech will tend to be more formal, staying more strictly within its careful outline. You will have to work harder to prepare visual and audio material that reaches the people sitting at the back of the room, including possibly using amplification.
There are many occasions for speeches. Awards ceremonies, conventions and conferences, holidays, and other celebrations are some examples. However, there are also less joyful reasons for a speech, such as funerals, disasters, and the delivery of bad news. As always, there are likely to be mixed reactions. For instance, award ceremonies are good for community and institutional morale, but we wouldn’t be surprised to find at least a little resentment from listeners who feel deserving but were overlooked. Likewise, for a speech announcing bad news, it is likely that at least a few listeners will be glad the bad news wasn’t even worse. If your speech is to deliver bad news, it’s important to be honest but also to avoid traumatizing your audience. For instance, if you are a condominium board member speaking to a residents’ meeting after the building was damaged by a hurricane, you will need to provide accurate data about the extent of the damage and the anticipated cost and time required for repairs. At the same time, it would be needlessly upsetting to launch into a graphic description of injuries suffered by people, animals, and property in neighboring areas not connected to your condomium complex.
Some of the most successful speeches benefit from situational analysis to identify audience concerns related to the occasion. For example, when the president of the United States gives the annual State of the Union address, the occasion calls for commenting on the condition of the nation and outlining the legislative agenda for the coming year. The speech could be a formality that would interest only “policy wonks,” or with the use of good situational audience analysis, it could be a popular event reinforcing the connection between the president and the American people. In January 2011, knowing that the United States’ economy was slowly recovering and that jobless rates were still very high, President Barack Obama and his staff knew that the focus of the speech had to be on jobs. Similarly, in January 2003, President George W. Bush’s State of the Union speech focused on the “war on terror” and his reasons for justifying the invasion of Iraq. If you look at the history of State of the Union Addresses, you’ll often find that the speeches are tailored to the political, social, and economic situations facing the United States at those times.
Voluntariness of Audience
A voluntary audience gathers because they want to hear the speech, attend the event, or participate in an event. A classroom audience, in contrast, is likely to be a captive audience. Captive audiences are required to be present or feel obligated to do so. Given the limited choices perceived, a captive audience might give only grudging attention. Even when there’s an element of choice, the likely consequences of nonattendance will keep audience members from leaving. The audience’s relative perception of choice increases the importance of holding their interest.
Whether or not the audience members chose to be present, you want them to be interested in what you have to say. Almost any audience will be interested in a topic that pertains directly to them. However, your audience might also be receptive to topics that are indirectly or potentially pertinent to their lives. This means that if you choose a topic such as advances in the treatment of spinal cord injury or advances in green technology, you should do your best to show how these topics are potentially relevant to their lives or careers.
However, there are some topics that appeal to audience curiosity even when it seems there’s little chance of direct pertinence. For instance, topics such as Blackbeard the pirate or ceremonial tattoos among the Maori might pique the interests of various audiences. Depending on the instructions you get from your instructor, you can consider building an interesting message about something outside the daily foci of our attention.
Physical Setting
The physical setting can make or break even the best speeches, so it is important to exercise as much control as you can over it. In your classroom, conditions might not be ideal, but at least the setting is familiar. Still, you know your classroom from the perspective of an audience member, not a speaker standing in the front—which is why you should seek out any opporutunity to rehearse your speech during a minute when the room is empty. If you will be giving your presentation somewhere else, it is a good idea to visit the venue ahead of time if at all possible and make note of any factors that will affect how you present your speech. In any case, be sure to arrive well in advance of your speaking time so that you will have time to check that the microphone works, to test out any visual aids, and to request any needed adjustments in lighting, room ventilation, or other factors to eliminate distractions and make your audience more comfortable.
Key Takeaways
- Demographic audience analysis focuses on group memberships of audience members.
- Another element of audience is psychographic information, which focuses on audience attitudes, beliefs, and values.
- Situational analysis of the occasion, physical setting, and other factors are also critical to effective audience analysis.
- List the voluntary (political party, campus organization, etc.) and involuntary (age, race, sex, etc.) groups to which you belong. After each group, write a sentence or phrase about how that group influences your experience as a student.
- Visit http://www.claritas.com/MyBestSegments/Default.jsp and http://homes.point2.com and report on the demographic information found for several different towns or zip codes. How would this information be useful in preparing an audience analysis?
- In a short paragraph, define the term “fairness.” Compare your definition with someone else’s definition. What factors do you think contributed to differences in definition?
- With a partner, identify an instance when you observed a speaker give a poor speech due to failing to analyze the situation. What steps could the speaker have taken to more effectively analyze the situation?
Grice, G. L., & Skinner, J. F. (2009). Mastering public speaking: The handbook (7th ed.). Boston, MA: Pearson.
Pew Forum on Religion & Public Life. (2008, February). Summary of key findings. In U.S. religious landscape survey . Retrieved from http://religions.pewforum.org/reports#
Tannen, D. (1994, December 11). The talk of the sandbox: How Johnny and Suzy’s playground chatter prepares them for life at the office. The Washington Post . Retrieved from http://www9.georgetown.edu/faculty/tannend/sandbox.htm
Stand up, Speak out Copyright © 2016 by University of Minnesota is licensed under a Creative Commons Attribution-NonCommercial-ShareAlike 4.0 International License , except where otherwise noted.
Share This Book
- Business & tech
- Audience analysis: What it is and why it's important for presentations
Audience analysis: What it is and why it's important for presentations
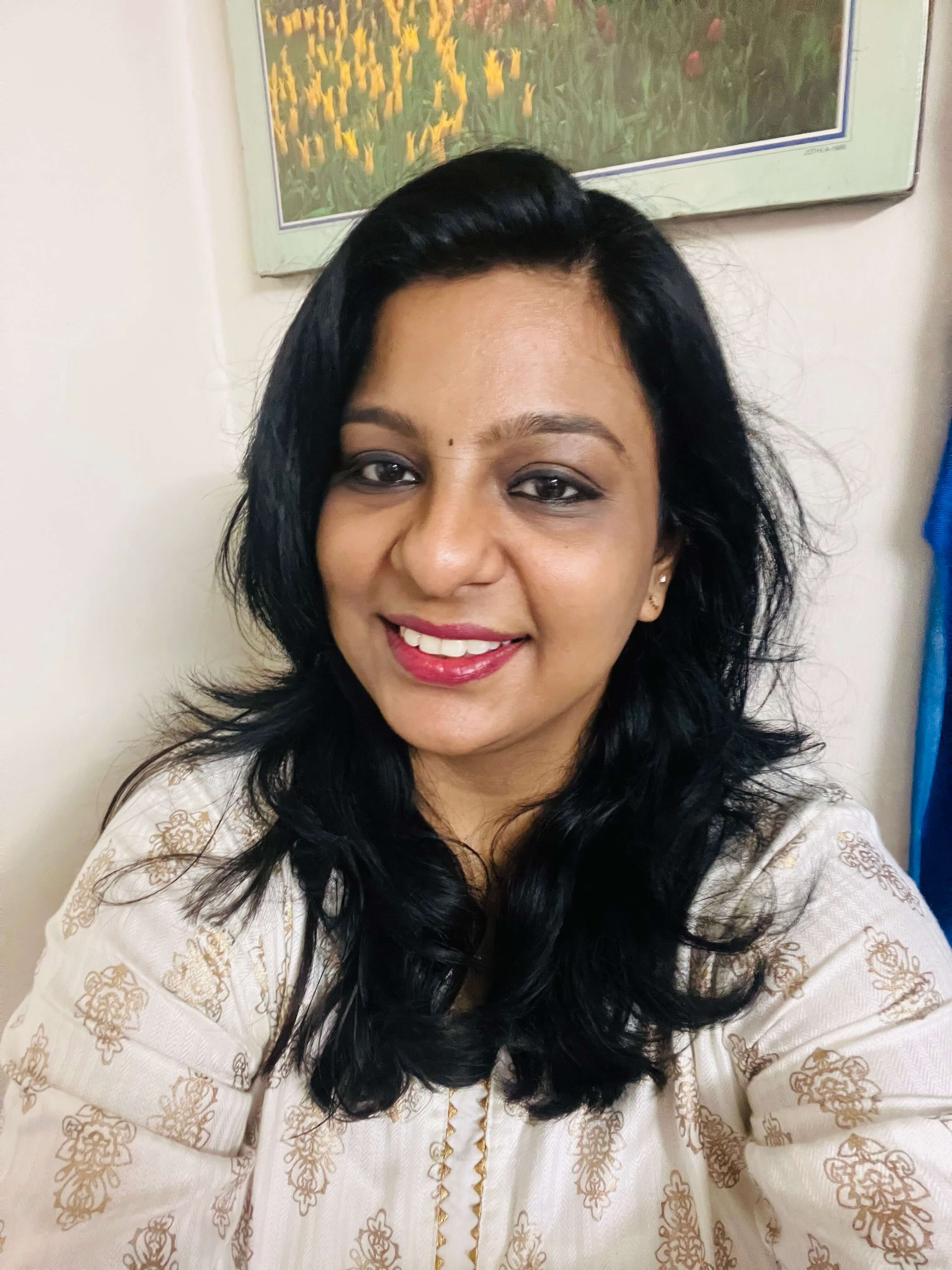
- Last Updated : December 19, 2023
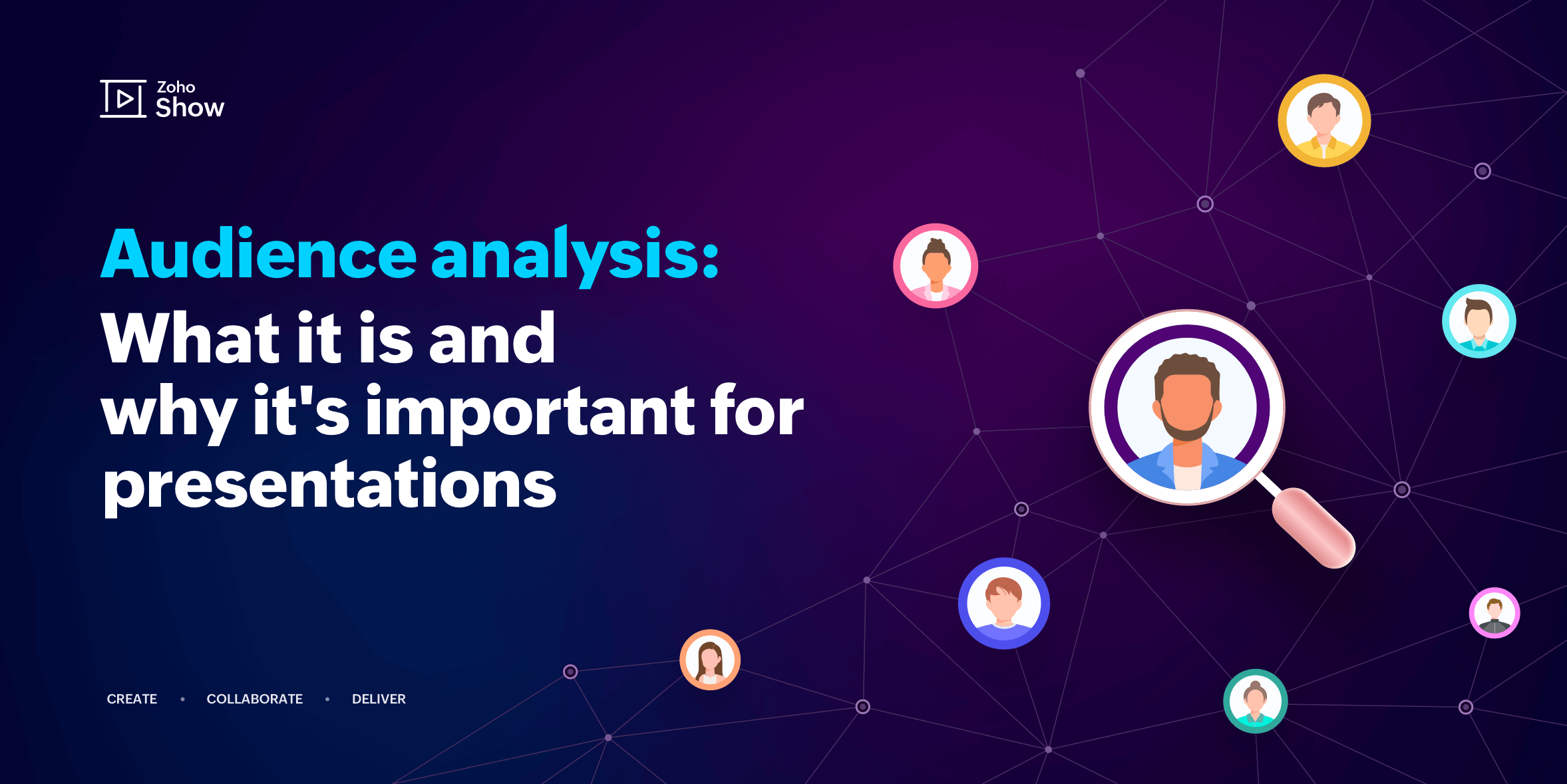
"Who are we going to present this to?"
This is the first question you should ask before preparing a speech. Whether it's a project proposal or a product launch, you should aim to customize your speech to address your audience's interests and needs. To do this, you may consider modifying the content, your tone, your body language, or other aspects of your speech.
Identifying and analyzing your target audience is crucial for any presentation. This allows you to find the right audience for your product or service and to figure what they care about. To build trust and establish strong relationships, it's important to understand who you are speaking to and why they are listening to you.
What is audience analysis?
Audience analysis is the collection and interpretation of data that helps you identify and classify your audience. For an effective analysis, you need to collect sufficient information, so you can understand who is in your audience and what they want to know about. Businesses build strategies for product branding, public relations, and marketing based on audience analyses.
Your audience can be analyzed using three methods: demographic, psychographic, and situational.
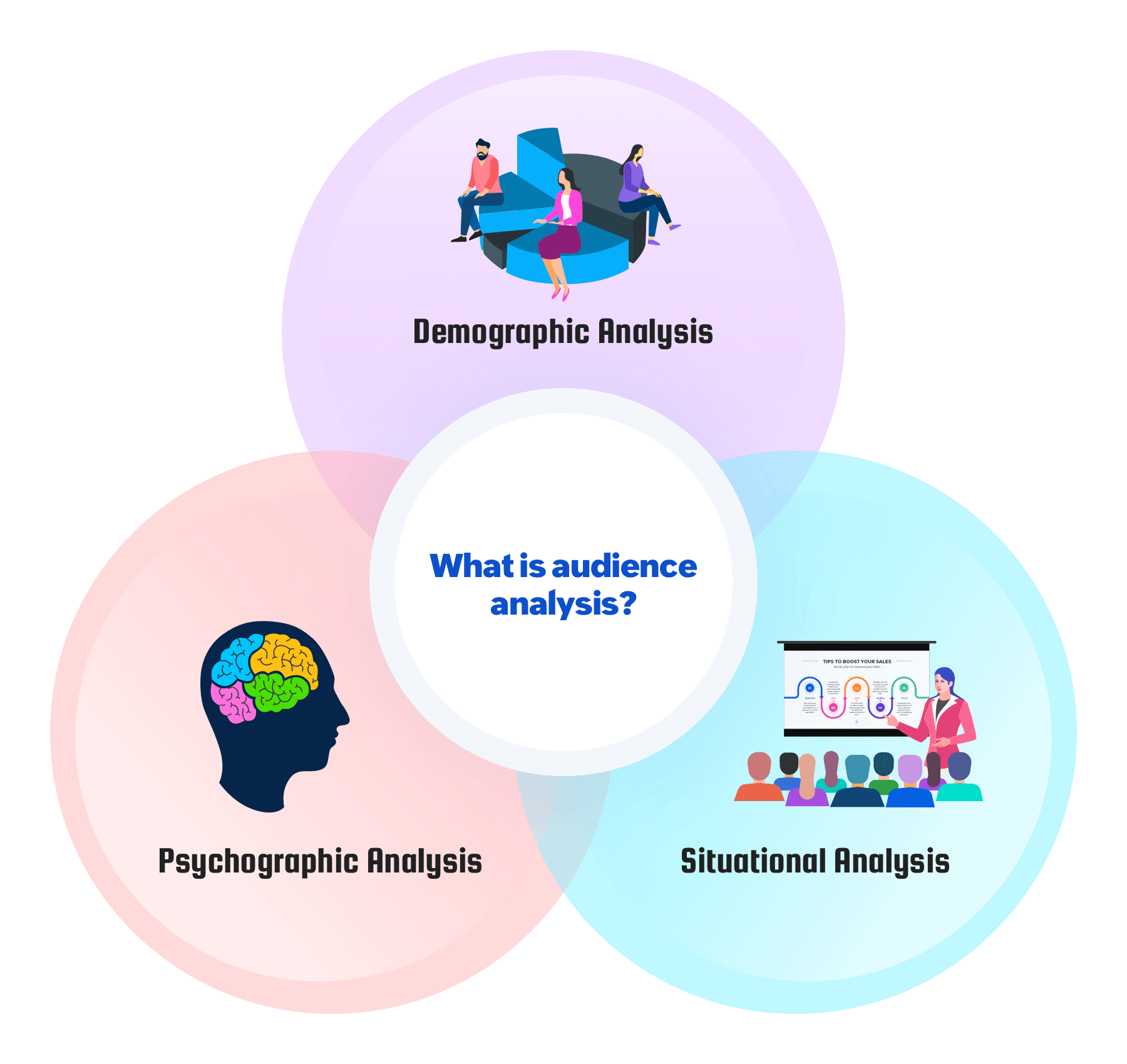
Demographic analysis
Classify your audience based on demographics, like age, sex, and location, and various socioeconomic factors, like employment, education, income, and marital status. This data can serve as a baseline for your analysis and can help with market segmentation, a process in which you categorize your audience into more defined groups.
Psychographic analysis
As the name suggests, psychographic analysis calls for classifying your audience based on their interests, opinions, values, desires, goals, and lifestyle choices. This will help you market your product more accurately. The retail, healthcare, and travel industries mostly use this type of analysis to market their products or services.
Situational analysis
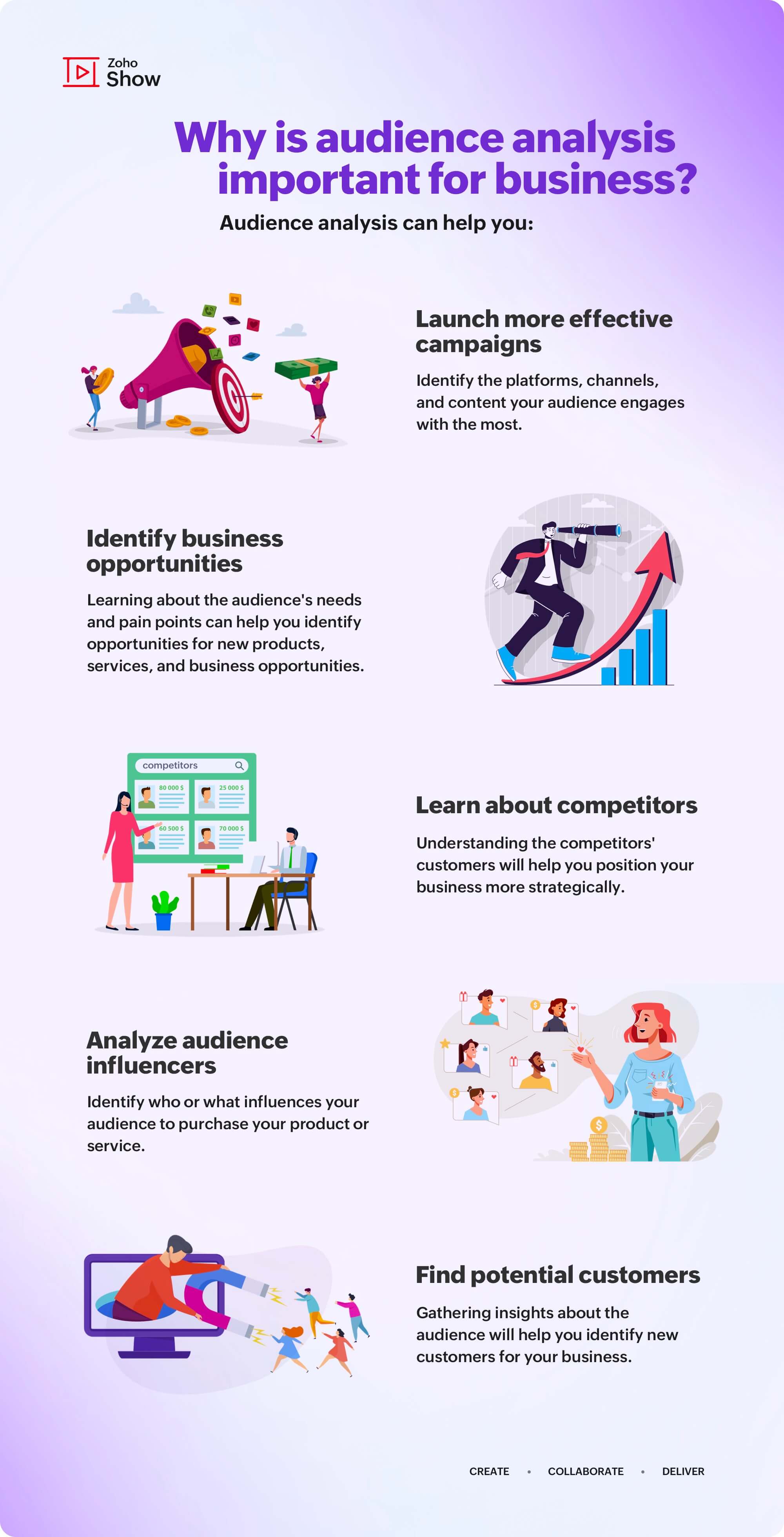
Importance of audience analysis for presentations
All of us prefer sitting through a speech that resonates with us. Whether you aim to inform, persuade, or entertain your listeners, you need to first know what your audience is interested in.
When you analyze your audience before a presentation, you can:
Tailor your content After analyzing the audience, you can curate content to ensure they gain enough knowledge on the topic and remain interested throughout the speech. Communicate effectively When you know what the audience expects from you, you can create an audience-centric speech. You can speak using language that resonates with your listeners to ensure a meaningful and impactful presentation.
Build a relationship When your speech is centered around the audience's interests, it's easier to build a connection with them. An audience is likely to feel a stronger connection to you and your material when they see how your presentation is relevant to their needs.
Achieve your goal What determines the success of a presentation? Tailoring your speech, body language, tone, and language to your audience can help you persuade them to buy your product or service.
Own the stage There are different ways of starting a speech—you can start with a story, anecdote, or even a joke. We all want to capture the audience's attention from the start. Your analysis can help you engage with your audience because you know what resonates with them.
How to collect data for audience analysis
Now that we have discussed why audience analysis is important for business presentations, it is important to know how to collect relevant data. Data collection is the most crucial step, though the best process can vary depending on the business and audience. Most organizations prefer to collect first-party data, which means collecting data from their audience directly.
Here are some different channels and ways to collect data:
- Customer surveys
- Social media
- Websites and chat bots
- Analytics tools
Closing thoughts:
"Everyone has a story to tell or a product to sell. Know your audience before you open your mouth." - Unknown.
No matter who you are presenting to, it's important to know the audience. Analyzing your audience can also give you the edge you need to convert customers who stay loyal to your brand.
Remember to get feedback from your audience after the speech is over. This will help you gauge the accuracy of your analysis.
Enjoy presenting!
Related Topics
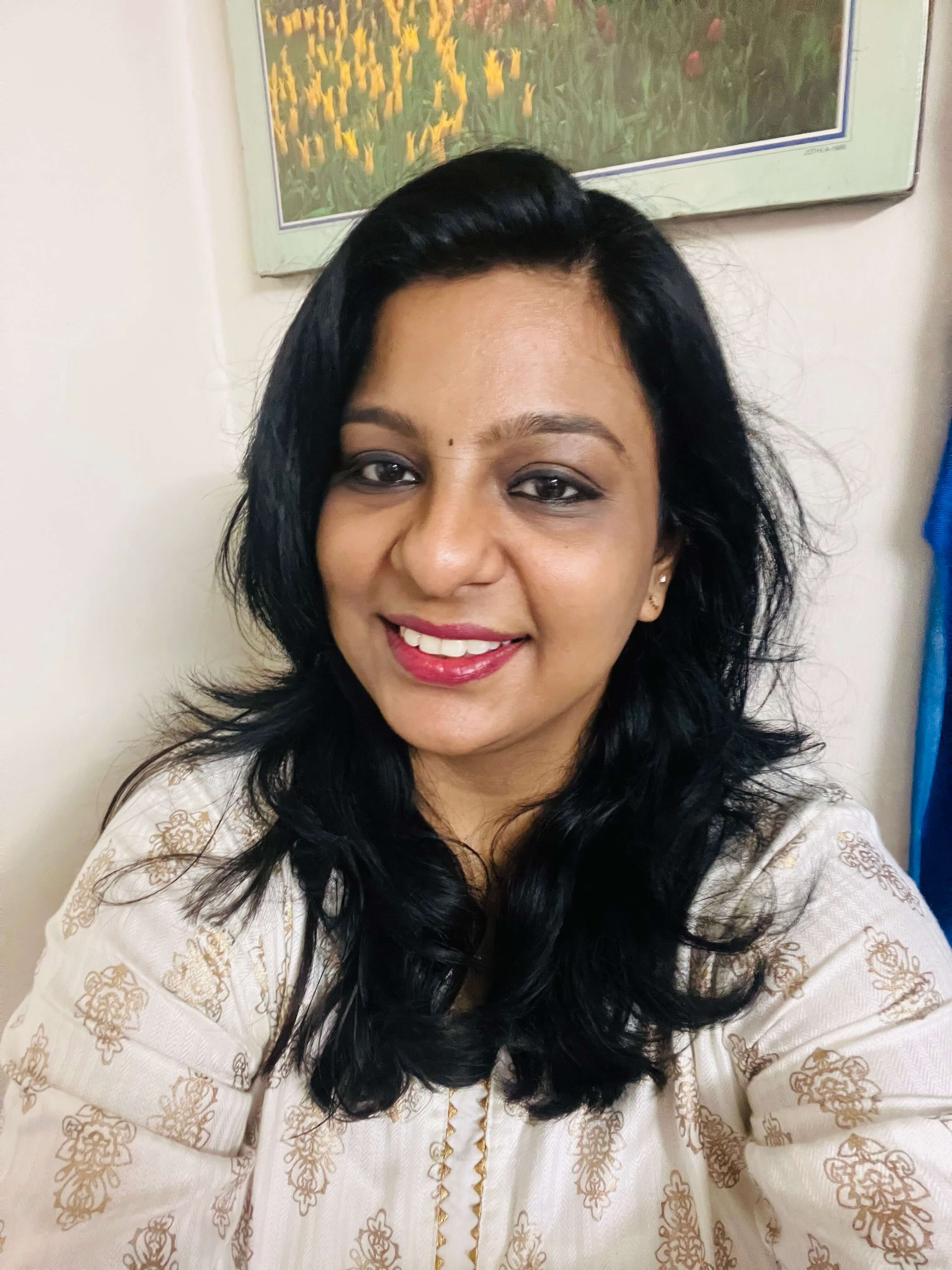
Leave a Reply
Your email address will not be published. Required fields are marked
You may also like
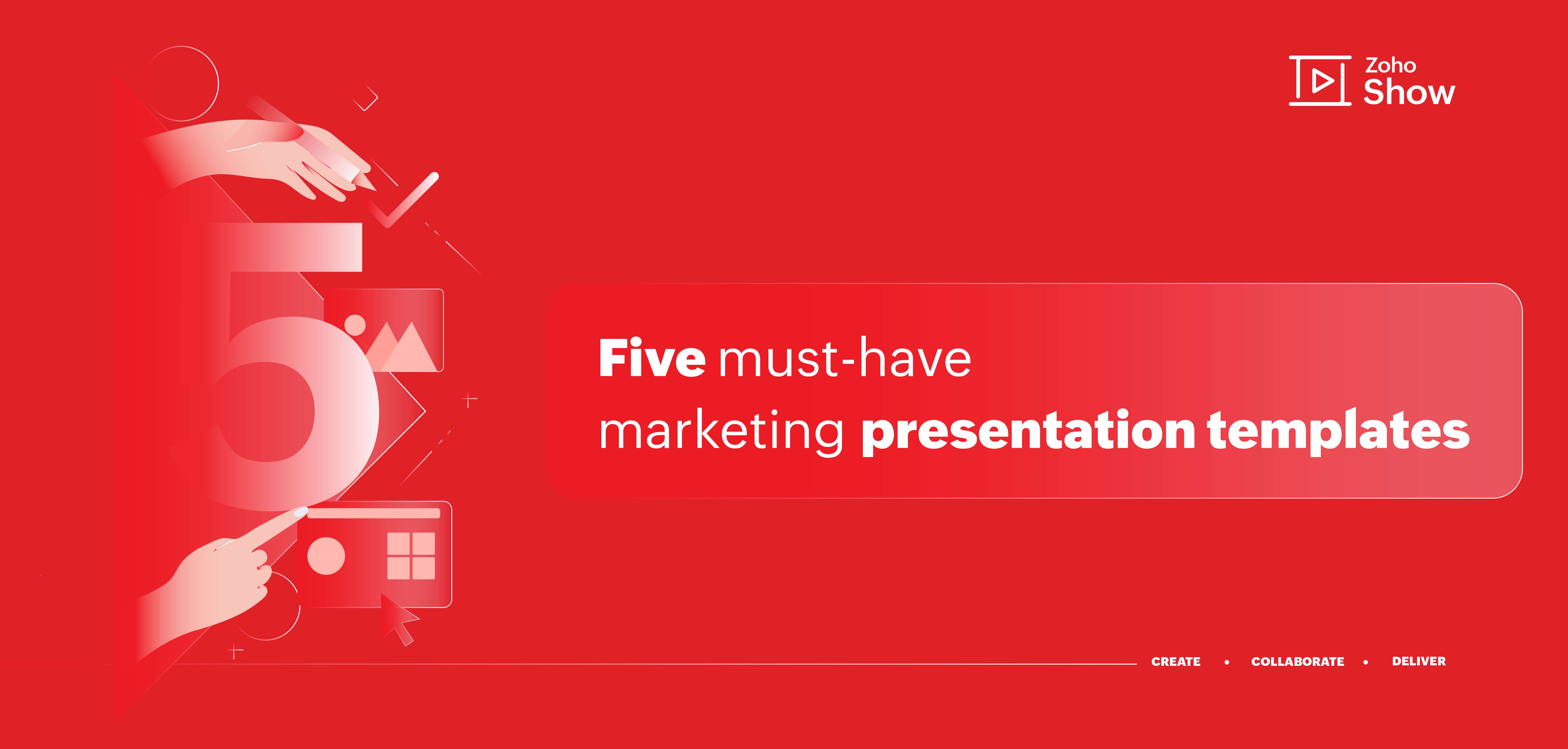
Five must-have marketing presentation templates
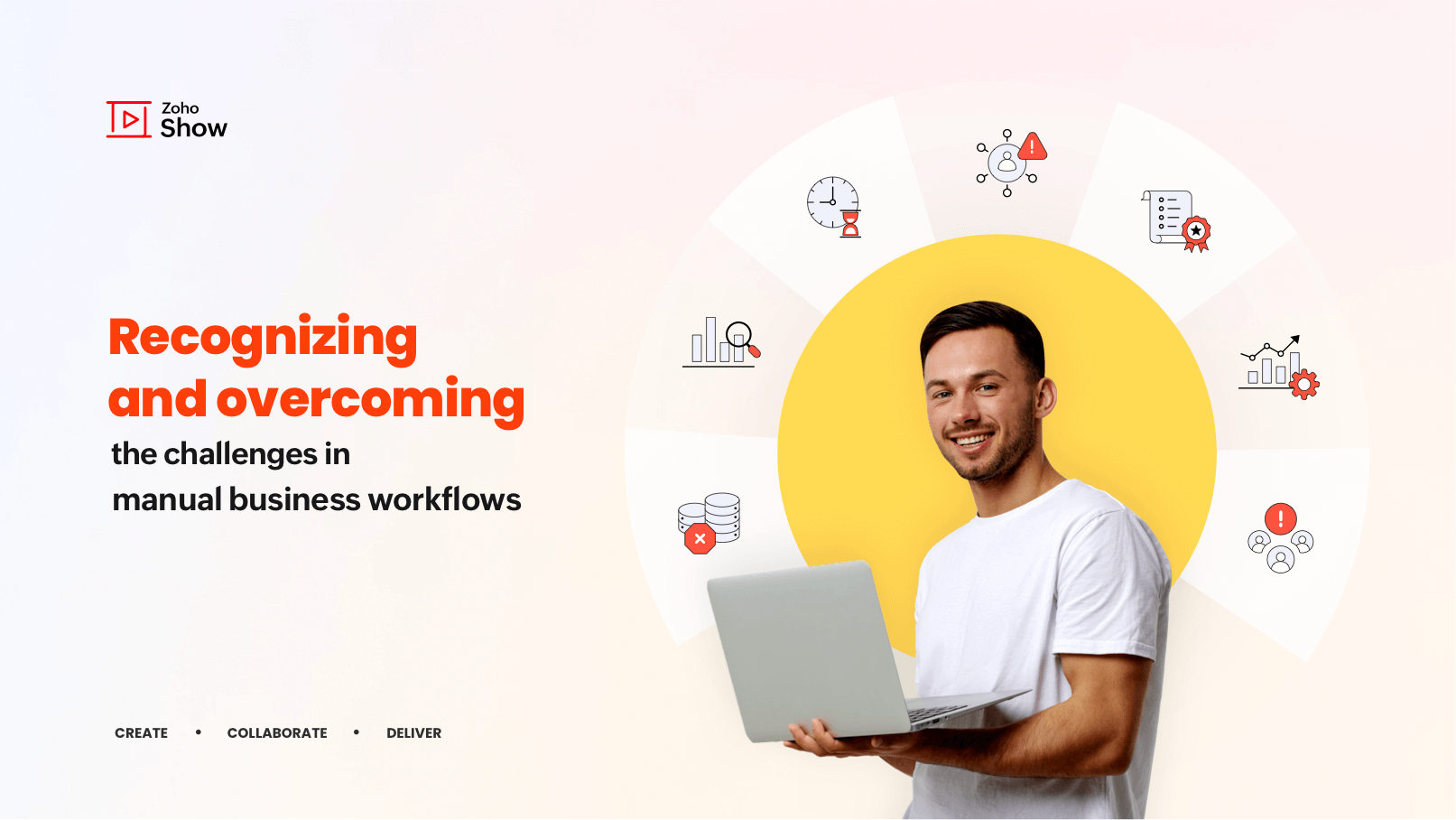
Recognizing and overcoming the challenges in manual business workflows
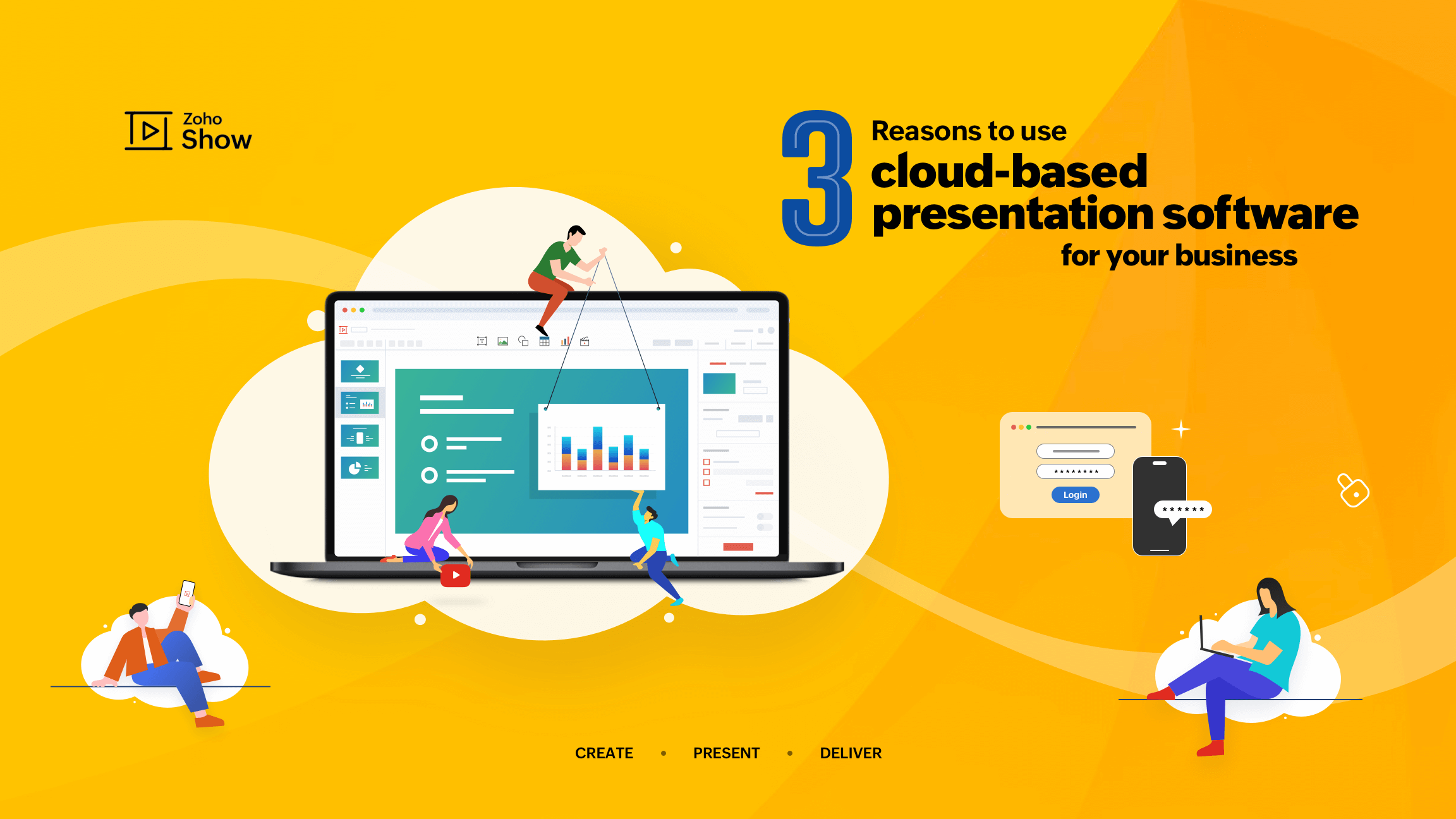
3 reasons to use cloud-based presentation software for your business
Data Collection, Presentation and Analysis
- First Online: 25 May 2023
Cite this chapter
- Uche M. Mbanaso 4 ,
- Lucienne Abrahams 5 &
- Kennedy Chinedu Okafor 6
514 Accesses
This chapter covers the topics of data collection, data presentation and data analysis. It gives attention to data collection for studies based on experiments, on data derived from existing published or unpublished data sets, on observation, on simulation and digital twins, on surveys, on interviews and on focus group discussions. One of the interesting features of this chapter is the section dealing with using measurement scales in quantitative research, including nominal scales, ordinal scales, interval scales and ratio scales. It explains key facets of qualitative research including ethical clearance requirements. The chapter discusses the importance of data visualization as key to effective presentation of data, including tabular forms, graphical forms and visual charts such as those generated by Atlas.ti analytical software.
This is a preview of subscription content, log in via an institution to check access.
Access this chapter
- Available as PDF
- Read on any device
- Instant download
- Own it forever
- Available as EPUB and PDF
- Durable hardcover edition
- Dispatched in 3 to 5 business days
- Free shipping worldwide - see info
Tax calculation will be finalised at checkout
Purchases are for personal use only
Institutional subscriptions
Bibliography
Abdullah, M. F., & Ahmad, K. (2013). The mapping process of unstructured data to structured data. Proceedings of the 2013 International Conference on Research and Innovation in Information Systems (ICRIIS) , Malaysia , 151–155. https://doi.org/10.1109/ICRIIS.2013.6716700
Adnan, K., & Akbar, R. (2019). An analytical study of information extraction from unstructured and multidimensional big data. Journal of Big Data, 6 , 91. https://doi.org/10.1186/s40537-019-0254-8
Article Google Scholar
Alsheref, F. K., & Fattoh, I. E. (2020). Medical text annotation tool based on IBM Watson Platform. Proceedings of the 2020 6th international conference on advanced computing and communication systems (ICACCS) , India , 1312–1316. https://doi.org/10.1109/ICACCS48705.2020.9074309
Cinque, M., Cotroneo, D., Della Corte, R., & Pecchia, A. (2014). What logs should you look at when an application fails? Insights from an industrial case study. Proceedings of the 2014 44th Annual IEEE/IFIP International Conference on Dependable Systems and Networks , USA , 690–695. https://doi.org/10.1109/DSN.2014.69
Gideon, L. (Ed.). (2012). Handbook of survey methodology for the social sciences . Springer.
Google Scholar
Leedy, P., & Ormrod, J. (2015). Practical research planning and design (12th ed.). Pearson Education.
Madaan, A., Wang, X., Hall, W., & Tiropanis, T. (2018). Observing data in IoT worlds: What and how to observe? In Living in the Internet of Things: Cybersecurity of the IoT – 2018 (pp. 1–7). https://doi.org/10.1049/cp.2018.0032
Chapter Google Scholar
Mahajan, P., & Naik, C. (2019). Development of integrated IoT and machine learning based data collection and analysis system for the effective prediction of agricultural residue/biomass availability to regenerate clean energy. Proceedings of the 2019 9th International Conference on Emerging Trends in Engineering and Technology – Signal and Information Processing (ICETET-SIP-19) , India , 1–5. https://doi.org/10.1109/ICETET-SIP-1946815.2019.9092156 .
Mahmud, M. S., Huang, J. Z., Salloum, S., Emara, T. Z., & Sadatdiynov, K. (2020). A survey of data partitioning and sampling methods to support big data analysis. Big Data Mining and Analytics, 3 (2), 85–101. https://doi.org/10.26599/BDMA.2019.9020015
Miswar, S., & Kurniawan, N. B. (2018). A systematic literature review on survey data collection system. Proceedings of the 2018 International Conference on Information Technology Systems and Innovation (ICITSI) , Indonesia , 177–181. https://doi.org/10.1109/ICITSI.2018.8696036
Mosina, C. (2020). Understanding the diffusion of the internet: Redesigning the global diffusion of the internet framework (Research report, Master of Arts in ICT Policy and Regulation). LINK Centre, University of the Witwatersrand. https://hdl.handle.net/10539/30723
Nkamisa, S. (2021). Investigating the integration of drone management systems to create an enabling remote piloted aircraft regulatory environment in South Africa (Research report, Master of Arts in ICT Policy and Regulation). LINK Centre, University of the Witwatersrand. https://hdl.handle.net/10539/33883
QuestionPro. (2020). Survey research: Definition, examples and methods . https://www.questionpro.com/article/survey-research.html
Rajanikanth, J. & Kanth, T. V. R. (2017). An explorative data analysis on Bangalore City Weather with hybrid data mining techniques using R. Proceedings of the 2017 International Conference on Current Trends in Computer, Electrical, Electronics and Communication (CTCEEC) , India , 1121-1125. https://doi/10.1109/CTCEEC.2017.8455008
Rao, R. (2003). From unstructured data to actionable intelligence. IT Professional, 5 , 29–35. https://www.researchgate.net/publication/3426648_From_Unstructured_Data_to_Actionable_Intelligence
Schulze, P. (2009). Design of the research instrument. In P. Schulze (Ed.), Balancing exploitation and exploration: Organizational antecedents and performance effects of innovation strategies (pp. 116–141). Gabler. https://doi.org/10.1007/978-3-8349-8397-8_6
Usanov, A. (2015). Assessing cybersecurity: A meta-analysis of threats, trends and responses to cyber attacks . The Hague Centre for Strategic Studies. https://www.researchgate.net/publication/319677972_Assessing_Cyber_Security_A_Meta-analysis_of_Threats_Trends_and_Responses_to_Cyber_Attacks
Van de Kaa, G., De Vries, H. J., van Heck, E., & van den Ende, J. (2007). The emergence of standards: A meta-analysis. Proceedings of the 2007 40th Annual Hawaii International Conference on Systems Science (HICSS’07) , USA , 173a–173a. https://doi.org/10.1109/HICSS.2007.529
Download references
Author information
Authors and affiliations.
Centre for Cybersecurity Studies, Nasarawa State University, Keffi, Nigeria
Uche M. Mbanaso
LINK Centre, University of the Witwatersrand, Johannesburg, South Africa
Lucienne Abrahams
Department of Mechatronics Engineering, Federal University of Technology, Owerri, Nigeria
Kennedy Chinedu Okafor
You can also search for this author in PubMed Google Scholar
Rights and permissions
Reprints and permissions
Copyright information
© 2023 The Author(s), under exclusive license to Springer Nature Switzerland AG
About this chapter
Mbanaso, U.M., Abrahams, L., Okafor, K.C. (2023). Data Collection, Presentation and Analysis. In: Research Techniques for Computer Science, Information Systems and Cybersecurity. Springer, Cham. https://doi.org/10.1007/978-3-031-30031-8_7
Download citation
DOI : https://doi.org/10.1007/978-3-031-30031-8_7
Published : 25 May 2023
Publisher Name : Springer, Cham
Print ISBN : 978-3-031-30030-1
Online ISBN : 978-3-031-30031-8
eBook Packages : Engineering Engineering (R0)
Share this chapter
Anyone you share the following link with will be able to read this content:
Sorry, a shareable link is not currently available for this article.
Provided by the Springer Nature SharedIt content-sharing initiative
- Publish with us
Policies and ethics
- Find a journal
- Track your research
- Speech Writing
- Delivery Techniques
- PowerPoint & Visuals
- Speaker Habits
- Speaker Resources
Speech Critiques
- Book Reviews
- Browse Articles
- ALL Articles
- Learn About Us
- About Six Minutes
- Meet Our Authors
- Write for Us
- Advertise With Us
Audience Analysis: A Guide for Speakers
Audience-centric speakers deliver the speech which the audience wants to hear, using words, concepts, stories, and visuals which will resonate with audience members and lead them to action.
But how do you know what the audience wants to hear? How do you know what will resonate with them? How do you know what they are thinking?
In this article, we define what audience analysis is , and look at the types of questions you should be asking about your audience.
- What is Audience Analysis?
“ Audience analysis is the process of learning who your audience is, what they are thinking, and how you can best reach them. ”
Audience analysis is the process of learning who your audience is, what they are thinking, and how you can best reach them.
Thoughtful audience analysis is one of the best habits you can develop as a speaker. It will help you understand your audience’s perspective and provide maximum value for them. If done well, your audience analysis will provide insights that will help you focus your message, select the most effective content and visuals, and tailor your delivery to suit this particular target audience.
- How to Conduct Audience Analysis
- How to Use Audience Analysis
- Audience Analysis Worksheet [PDF Download]
Audience analysis studies your audience along three primary dimensions:
- Demographic Analysis Who is in your audience? What are their individual and group characteristics?
- Psychological Analysis What does your audience know? What do they believe? What do they think about your topic?
- Contextual Analysis When and where are you presenting? Why is this audience listening to you?
Each of these three dimensions is examined in more detail in the following sections.
1. Demographic Audience Analysis
The aim of demographic audience analysis is to discover who you are speaking to.
Depending on your topic and message, some of the following questions will be relevant and some will not:
- How old are they? A talk about investment options would be very different if you are speaking with teenagers versus a group nearing retirement.
- Men? Women? Mixed? A talk about nutrition, fitness, or fashion may depend on the gender of your audience.
- Are audience members predominantly a certain race, culture, or ethnicity? This might impact your message, choice of language, gestures, and other aspects of your speech.
- Do they share a common primary language with you? If not, you may have to be careful with slang, idioms, and other language shortcuts.
- What is their profession ? Imagine talking about a scientific discovery with an audience of engineers versus accountants.
- What is their religion ? A talk about moral issues may depend heavily on the religion of your audience members.
- What is their educational level ? Imagine the difference speaking to high school students versus lawyers.
- Are they members of a relevant organization ? Imagine talking about volunteerism with members of the Lions club versus non-members.
- What is their personality type ? Introverts and extroverts have different preferences that may impact your seminar approach.
- Other qualities relevant to your speech ? e.g. business leaders, marital status, cell-phone users, avid readers, marathon runners
All of the above analysis may be impacted by qualities of the audience as a whole:
- Is the audience homogeneous or heterogeneous ? It usually makes your life easier if your audience is fairly homogeneous in certain ways. For example, you can be far more technical if you are talking to a room full of engineers. Just be careful not to assume your audience members are identical — they are not.
- What is the size of the audience ? Larger audiences dictate many presentation differences compared to smaller audiences. In addition, larger audiences will tend to be more heterogeneous, and so you can draw fewer conclusions about them.
Finally, consider how you relate to the audience with respect to several of the characteristics above:
- Are you similar to your audience, or are you different ? (In gender, age, profession, education level, etc.) Sometimes, it doesn’t matter. Other times, they will view you as an outsider, and that may influence your preparation.
- Are they your peers ? e.g. co-workers or classmates
- Or are they your superiors or subordinates ? Either could be good or bad…
2. Psychological Audience Analysis
“ Thoughtful audience analysis is one of the best habits you can develop as a speaker. ”
The aim of psychological audience analysis is to discover what your audience may be thinking before and during your presentation.
Psychological analysis covers both the knowledge (or lack of knowledge) and the beliefs of your audience.
Audience Knowledge
- What do they know about your topic? What don’t they know? These are critical questions to determine the “level” at which you target your presentation.
- What do they want to know? What do they need to know? It’s critical that you satisfy their expectations. Otherwise, your presentation will be seen as a failure for them.
- What specialized terminology are they familiar with? Using acronyms and technical terms is okay in a presentation, but only if your audience recognizes them (or you take the time to define them).
- What concepts, processes, or tools are they familiar with? Like terminology, you can draw upon these concepts, processes, and tools as you build your presentation. Consider them building blocks that are already in place before you begin.
It is also sometimes useful to gauge their knowledge compared to yours:
- Do some members of your audience know more about the topic than you ? This is a tricky situation to navigate.
Audience Beliefs
- Are they neutral , or are they predisposed to agree with or oppose your message ? Audiences of the three different types require three totally different presentations.
- What are the most important values to the audience? (Or, what are the values of their organization ?) It’s important to know what they value as these are often the best starting points upon which you can build your arguments.
- What problems do they have ? If your presentation aims to offer them a solution, it surely helps if they agree with you that the problem exists in the first place!
3. Contextual Audience Analysis
“ If done well, your audience analysis will provide insights that will help you focus your message, select the most effective content and visuals, and tailor your delivery to suit this particular target audience. ”
The aim of contextual audience analysis is to discover how the speaking event itself may influence your audience’s state of mind.
In some ways, this analysis takes who they are (demographic) and what they believe (psychological), and pins it to a certain time and place where you’ll be speaking.
Consider the following questions:
- Is their attendance voluntary or mandatory ? In most cases, audience members who are attending voluntarily are much more open-minded, more enthusiastic, and more motivated to hear what you have to say. On the other hand, mandatory attendees may require extra effort on your part to motivate.
- What has the audience been going through in the days or weeks prior to your speech? Have their been layoffs at the company? Has everyone been dealing with the impact of a storm?
- What style of presentation does the audience expect ? If your speech is part of a larger event, your audience may have certain expectations that your presentation will be similar to the others. Sometimes it is best to conform; other times it is best to stand out. Your audience analysis lets you make a deliberate decision either way.
- What are people wearing ? Not only will this dictate how you may want to dress, but it may also guide you in the level of formality you should maintain.
- What time of day are you speaking? Before/during/after a meal? Early/late in the day? Timing will influence your audience’s state of mind.
- What obstacles or distractions exist in the room that may impact your audience?
Remember that you are speaking to individuals
In rare circumstances, you are able to meet privately with each audience member before your presentation and learn about them and their expectations. However, this is rarely practical. So, you are usually required to infer a great deal from your audience analysis instead.
However, you don’t talk to amorphous blobs known as audiences. You talk to individual people, and no two people in your audience are identical. While the individuals in your audience may be similar in many ways, there will always be a range of characteristics: a range of knowledge levels, a range of beliefs, a range of expectations, etc. Even the best audience analysis will have a degree of uncertainty.
Next in this Series…
In subsequent articles in this series, we look at how to conduct audience analysis and how to incorporate what you learn to improve your presentations .
Please share this...
This is one of many public speaking articles featured on Six Minutes . Subscribe to Six Minutes for free to receive future articles.
Image credit: untitled by Incase ( CC BY 2.0 )
Add a Comment Cancel reply
E-Mail (hidden)
Subscribe - It's Free!
Similar articles you may like....
- How to Improve Your Speeches Through Audience Analysis
- 8 Faulty Speaker Assumptions and How to Fix Them
- How to get Useful Feedback: A Speaker’s Guide
- 7 Habits for Highly Effective Speakers
- 10 Presentation Bad Habits My College Students – And You – Must UN-Learn (Part 2)
Find More Articles Tagged:
Excellent article, Andrew, and particularly timely for me. I’m writing an article about the elevator pitch. One of my points was researching the audience, but as I began to cover the points in your article, giving examples, my article was getting too long. Now, all I have to do is to write one paragraph on this point and then refer them to your article, if you don’t mind.
Thanks for your comprehensive treatment of the topic.
Thanks for the feedback, Eugenia. I’m glad to hear that this article provides value for you.
And yes, please feel free to link to this article or any other on Six Minutes.
Please, I would like to be part of your team, am looking forward to becoming a great speaker of our time. Will be grateful if you add me and send me more of this guide in terms of public speaking. Thanks
Great communication resource!
Is their attendance voluntary or mandatory? In most cases, audience members who are attending voluntarily are much more open-minded, more enthusiastic, and more motivated to hear what you have to say. On the other hand, mandatory attendees may require extra effort on your part to motivate. What has the audience been going through in the days or weeks prior to your speech? Have their been layoffs at the company? Has everyone been dealing with the impact of a storm? What style of presentation does the audience expect? If your speech is part of a larger event, your audience may have certain expectations that your presentation will be similar to the others. Sometimes it is best to conform; other times it is best to stand out. Your audience analysis lets you make a deliberate decision either way. What are people wearing? Not only will this dictate how you may want to dress, but it may also guide you in the level of formality you should maintain. What time of day are you speaking? Before/during/after a meal? Early/late in the day? Timing will influence your audience’s state of mind. What obstacles or distractions exist in the room that may impact your audience?
This is a great resource! And not just for thinking about the delivery of your speech, but how to structure your ideas. What kinds of examples and stories will best illustrate your points with this audience? What experience does your audience have in the topic? How can you leverage their experience to craft your speech? Even if a speaker knows a topic inside & out, s/he may lose some valuable opportunities to connect if these audience analysis questions go unanswered. Thanks again!
Well done…so clear to understand
Recent Tweets
Audience Analysis: A Guide for Speakers https://t.co/yZ2WXwv0MH by @6minutes — Sinapsis-Lab (@SinapsisLab) Dec 11th, 2015
Audience Analysis: A Guide for Speakers https://t.co/osXx53790y #publicspeaking #toastmasters — @MySpeakingJ Dec 14th, 2015
#TuesdayTips Audience analysis is vital to crafting a presentation that resonates. Excellent article by @6minutes https://t.co/KZf8pFMNTN — PitchVantage (@pitchvantage) Jan 5th, 2016
4. I ask 6 questions of every audience. Andrew Dlugan asks 30! https://t.co/T1aHD2Svpi via @6minutes #100SpeakingTips — Rob Biesenbach (@RobBiesenbach) Jan 11th, 2016
One of my favorite blogs, “Six Minutes,” provides a guide to helping speakers read the audience, a critical skill. https://t.co/lfXilyNhmq — @JeffreySaviano Mar 29th, 2016
Super useful guide on audience analysis #speaking @Speakers_Corner @1publicspeaking https://t.co/jWt3PUw9At https://t.co/CGpQxQOIME — @MeggieLip May 9th, 2016
Audience Analysis: A Guide for Speakers https://t.co/ybJGcQgYX5 by @6minutes — @Afcamp25 Feb 14th, 2017
“Audience-centric speakers deliver the speech which the audience wants to hear, using words, concepts, stories, and… https://t.co/wLJpGb0xpZ — @ToppComm Jul 24th, 2018
Our training courses teach you to “flip the focus” to the audience, in @6minutes’ words, “deliver the speech which… https://t.co/a0bfMGn8Ck — @ChainsawComs Jul 25th, 2018
Shared by @MySpeakingJ: Audience Analysis: A Guide for Speakers https://t.co/39ODSFMGwF #publicspeaking #toastmasters — Expressions Training (@SpeakerRepeater) Dec 31st, 2018
5 Blog Links
Hobbits and the habits of highly effective communicators « Speak for Yourself — Nov 21st, 2012
A VERY unexpected journey « Speak for Yourself — Dec 25th, 2012
Segmenting the Audience | Tweak Your Slides — Jan 10th, 2013
Web resources for audience analysis « teachingpublicspeaking — Jan 18th, 2013
Fresh Links Sundae from David Lowe of Actionable ITSM | Actionable ITSM — Jan 27th, 2013
Featured Articles
- Majora Carter (TED, 2006) Energy, Passion, Speaking Rate
- Hans Rosling (TED, 2006) 6 Techniques to Present Data
- J.A. Gamache (Toastmasters, 2007) Gestures, Prop, Writing
- Steve Jobs (Stanford, 2005) Figures of speech, rule of three
- Al Gore (TED, 2006) Humor, audience interaction
- Dick Hardt (OSCON, 2005) Lessig Method of Presentation
Books We Recommend
Six Minutes Copyright © 2007-2019 All Rights Reserved.
Read our permissions policy , privacy policy , or disclosure policy .
Comments? Questions? Contact us .
Module 4: Considering the Audience
Factors to consider in audience analysis, learning objectives.
Describe factors to consider when performing audience analysis.
What does it mean to be audience-centered when you speak or present? To be audience-centered is to shift your focus from yourself (what do I want to say about this topic?) to your audience (what does my audience want or need to hear about this topic?) But how do we go about making this shift? In part, by asking questions. On this page, we’ll look at four questions you can ask yourself to guide your analysis of the audience: Why are they here? What do they know already? Where do they stand? Where are they coming from?
Expectations of the event: Why are they here?
The first question you could ask of your imaginary audience is, why are they here? What does this audience expect to hear and what do they hope to learn? Are they here because they have to be (e.g., a mandatory meeting or a class session) or because they want to be? Is your presentation the “main event,” or is something else bringing this audience together? All these factors can be extremely important in planning your speech.
Prior experience with the topic: What do they know already?
Another extremely important factor to consider is the audience’s prior experience with the topic you’re discussing. This experience can take many forms. One form would be technical , theoretical , or academic knowledge of the subject. Another would be first-hand , observational , or practical experience. For instance, if you were presenting about the criminal justice system to a group of sociologists, you could assume a high level of theoretical knowledge about various methodologies of modeling social systems, but not necessarily an understanding of how the system is experienced by an individual trying to negotiate the court system. If you were presenting about the same topic to a group of people who had all been in prison, you could assume a high level of experience with the criminal justice system, but not necessarily a familiarity with sociological models of this system.
Attitude toward the topic: Where do they stand?
Generally speaking, the audience’s attitude toward the topic you are presenting can be grouped into a few categories. Audiences are said to be friendly if you can assume they would be inclined to agree with your assumptions and argument. A hostile audience, on the other hand, would not agree with your conclusions unless the listeners were convinced by your argument. For instance, if you were arguing in favor of a tax on carbon emissions, an environmentalist organization might be considered a friendly audience, whereas a group of oil and gas executives could be a hostile audience. Note: hostile audiences usually won’t heckle you or throw tomatoes. This term isn’t describing rude audiences, but rather audiences who are likely to disagree with your argument or have negative feelings toward your subject. Finally, if an audience is neither friendly nor hostile, it may be neutral (not having strong inclinations one way or another) or mixed (containing both friendly and hostile listeners—and probably neutral listeners as well).
Frame of reference: Where are they coming from?
A frame of reference describes the assumptions, values, and standards by which a person evaluates a particular idea or position. As you think about audience analysis in this section, ask yourself which frames of reference are shared by most members of your audience, and which are different for various audience members. If you are speaking in class, for instance, you can assume that one shared frame of reference is the affiliation with your school, whether as students or as instructors. Within this academic frame of reference, then, we can start from a premise that the whole class shares a desire to gain skills, knowledge, and opportunity. If you’re speaking to your local Chamber of Commerce, one shared frame of reference might be an investment in the health of the local business community. A group of teachers might share certain values and standards related to the educational system. Members of the International Students Association might have a common experience of negotiating different national and cultural frames of reference.
In large part, audience analysis involves trying to learn about your audience’s frames of reference—cultural, philosophical, religious, demographic, economic, and so on. It is extremely important, however, to avoid jumping to conclusions about your listeners’ values, standards, and experiences based on your analysis of the audience.
As Daphne A. Jameson has pointed out, the most important starting point for audience analysis is self-understanding: “The metacognitive problem is to know what one knows—and does not know—about others.” [1]
- Jameson, D. A. (2007). Reconceptualizing Cultural Identity and Its Role in Intercultural Business Communication. Journal of Business Communication, 44 (3), 199–235. doi:10.1177/0021943607301346 ↵
- Factors to Consider in Audience Analysis. Authored by : Sandra K. Winn with Lumen Learning. License : CC BY: Attribution
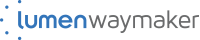

Skills for Learning : Research Skills
Data analysis is an ongoing process that should occur throughout your research project. Suitable data-analysis methods must be selected when you write your research proposal. The nature of your data (i.e. quantitative or qualitative) will be influenced by your research design and purpose. The data will also influence the analysis methods selected.
We run interactive workshops to help you develop skills related to doing research, such as data analysis, writing literature reviews and preparing for dissertations. Find out more on the Skills for Learning Workshops page.
We have online academic skills modules within MyBeckett for all levels of university study. These modules will help your academic development and support your success at LBU. You can work through the modules at your own pace, revisiting them as required. Find out more from our FAQ What academic skills modules are available?
Quantitative data analysis
Broadly speaking, 'statistics' refers to methods, tools and techniques used to collect, organise and interpret data. The goal of statistics is to gain understanding from data. Therefore, you need to know how to:
- Produce data – for example, by handing out a questionnaire or doing an experiment.
- Organise, summarise, present and analyse data.
- Draw valid conclusions from findings.
There are a number of statistical methods you can use to analyse data. Choosing an appropriate statistical method should follow naturally, however, from your research design. Therefore, you should think about data analysis at the early stages of your study design. You may need to consult a statistician for help with this.
Tips for working with statistical data
- Plan so that the data you get has a good chance of successfully tackling the research problem. This will involve reading literature on your subject, as well as on what makes a good study.
- To reach useful conclusions, you need to reduce uncertainties or 'noise'. Thus, you will need a sufficiently large data sample. A large sample will improve precision. However, this must be balanced against the 'costs' (time and money) of collection.
- Consider the logistics. Will there be problems in obtaining sufficient high-quality data? Think about accuracy, trustworthiness and completeness.
- Statistics are based on random samples. Consider whether your sample will be suited to this sort of analysis. Might there be biases to think about?
- How will you deal with missing values (any data that is not recorded for some reason)? These can result from gaps in a record or whole records being missed out.
- When analysing data, start by looking at each variable separately. Conduct initial/exploratory data analysis using graphical displays. Do this before looking at variables in conjunction or anything more complicated. This process can help locate errors in the data and also gives you a 'feel' for the data.
- Look out for patterns of 'missingness'. They are likely to alert you if there’s a problem. If the 'missingness' is not random, then it will have an impact on the results.
- Be vigilant and think through what you are doing at all times. Think critically. Statistics are not just mathematical tricks that a computer sorts out. Rather, analysing statistical data is a process that the human mind must interpret!
Top tips! Try inventing or generating the sort of data you might get and see if you can analyse it. Make sure that your process works before gathering actual data. Think what the output of an analytic procedure will look like before doing it for real.
(Note: it is actually difficult to generate realistic data. There are fraud-detection methods in place to identify data that has been fabricated. So, remember to get rid of your practice data before analysing the real stuff!)
Statistical software packages
Software packages can be used to analyse and present data. The most widely used ones are SPSS and NVivo.
SPSS is a statistical-analysis and data-management package for quantitative data analysis. Click on ‘ How do I install SPSS? ’ to learn how to download SPSS to your personal device. SPSS can perform a wide variety of statistical procedures. Some examples are:
- Data management (i.e. creating subsets of data or transforming data).
- Summarising, describing or presenting data (i.e. mean, median and frequency).
- Looking at the distribution of data (i.e. standard deviation).
- Comparing groups for significant differences using parametric (i.e. t-test) and non-parametric (i.e. Chi-square) tests.
- Identifying significant relationships between variables (i.e. correlation).
NVivo can be used for qualitative data analysis. It is suitable for use with a wide range of methodologies. Click on ‘ How do I access NVivo ’ to learn how to download NVivo to your personal device. NVivo supports grounded theory, survey data, case studies, focus groups, phenomenology, field research and action research.
- Process data such as interview transcripts, literature or media extracts, and historical documents.
- Code data on screen and explore all coding and documents interactively.
- Rearrange, restructure, extend and edit text, coding and coding relationships.
- Search imported text for words, phrases or patterns, and automatically code the results.
Qualitative data analysis
Miles and Huberman (1994) point out that there are diverse approaches to qualitative research and analysis. They suggest, however, that it is possible to identify 'a fairly classic set of analytic moves arranged in sequence'. This involves:
- Affixing codes to a set of field notes drawn from observation or interviews.
- Noting reflections or other remarks in the margins.
- Sorting/sifting through these materials to identify: a) similar phrases, relationships between variables, patterns and themes and b) distinct differences between subgroups and common sequences.
- Isolating these patterns/processes and commonalties/differences. Then, taking them out to the field in the next wave of data collection.
- Highlighting generalisations and relating them to your original research themes.
- Taking the generalisations and analysing them in relation to theoretical perspectives.
(Miles and Huberman, 1994.)
Patterns and generalisations are usually arrived at through a process of analytic induction (see above points 5 and 6). Qualitative analysis rarely involves statistical analysis of relationships between variables. Qualitative analysis aims to gain in-depth understanding of concepts, opinions or experiences.
Presenting information
There are a number of different ways of presenting and communicating information. The particular format you use is dependent upon the type of data generated from the methods you have employed.
Here are some appropriate ways of presenting information for different types of data:
Bar charts: These may be useful for comparing relative sizes. However, they tend to use a large amount of ink to display a relatively small amount of information. Consider a simple line chart as an alternative.
Pie charts: These have the benefit of indicating that the data must add up to 100%. However, they make it difficult for viewers to distinguish relative sizes, especially if two slices have a difference of less than 10%.
Other examples of presenting data in graphical form include line charts and scatter plots .
Qualitative data is more likely to be presented in text form. For example, using quotations from interviews or field diaries.
- Plan ahead, thinking carefully about how you will analyse and present your data.
- Think through possible restrictions to resources you may encounter and plan accordingly.
- Find out about the different IT packages available for analysing your data and select the most appropriate.
- If necessary, allow time to attend an introductory course on a particular computer package. You can book SPSS and NVivo workshops via MyHub .
- Code your data appropriately, assigning conceptual or numerical codes as suitable.
- Organise your data so it can be analysed and presented easily.
- Choose the most suitable way of presenting your information, according to the type of data collected. This will allow your information to be understood and interpreted better.
Primary, secondary and tertiary sources
Information sources are sometimes categorised as primary, secondary or tertiary sources depending on whether or not they are ‘original’ materials or data. For some research projects, you may need to use primary sources as well as secondary or tertiary sources. However the distinction between primary and secondary sources is not always clear and depends on the context. For example, a newspaper article might usually be categorised as a secondary source. But it could also be regarded as a primary source if it were an article giving a first-hand account of a historical event written close to the time it occurred.
- Primary sources
- Secondary sources
- Tertiary sources
- Grey literature
Primary sources are original sources of information that provide first-hand accounts of what is being experienced or researched. They enable you to get as close to the actual event or research as possible. They are useful for getting the most contemporary information about a topic.
Examples include diary entries, newspaper articles, census data, journal articles with original reports of research, letters, email or other correspondence, original manuscripts and archives, interviews, research data and reports, statistics, autobiographies, exhibitions, films, and artists' writings.
Some information will be available on an Open Access basis, freely accessible online. However, many academic sources are paywalled, and you may need to login as a Leeds Beckett student to access them. Where Leeds Beckett does not have access to a source, you can use our Request It! Service .
Secondary sources interpret, evaluate or analyse primary sources. They're useful for providing background information on a topic, or for looking back at an event from a current perspective. The majority of your literature searching will probably be done to find secondary sources on your topic.
Examples include journal articles which review or interpret original findings, popular magazine articles commenting on more serious research, textbooks and biographies.
The term tertiary sources isn't used a great deal. There's overlap between what might be considered a secondary source and a tertiary source. One definition is that a tertiary source brings together secondary sources.
Examples include almanacs, fact books, bibliographies, dictionaries and encyclopaedias, directories, indexes and abstracts. They can be useful for introductory information or an overview of a topic in the early stages of research.
Depending on your subject of study, grey literature may be another source you need to use. Grey literature includes technical or research reports, theses and dissertations, conference papers, government documents, white papers, and so on.
Artificial intelligence tools
Before using any generative artificial intelligence or paraphrasing tools in your assessments, you should check if this is permitted on your course.
If their use is permitted on your course, you must acknowledge any use of generative artificial intelligence tools such as ChatGPT or paraphrasing tools (e.g., Grammarly, Quillbot, etc.), even if you have only used them to generate ideas for your assessments or for proofreading.
- Academic Integrity Module in MyBeckett
- Assignment Calculator
- Building on Feedback
- Disability Advice
- Essay X-ray tool
- International Students' Academic Introduction
- Manchester Academic Phrasebank
- Quote, Unquote
- Skills and Subject Suppor t
- Turnitin Grammar Checker
{{You can add more boxes below for links specific to this page [this note will not appear on user pages] }}
- Research Methods Checklist
- Sampling Checklist
Skills for Learning FAQs

0113 812 1000
- University Disclaimer
- Accessibility
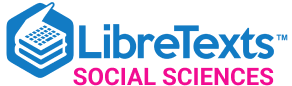
- school Campus Bookshelves
- menu_book Bookshelves
- perm_media Learning Objects
- login Login
- how_to_reg Request Instructor Account
- hub Instructor Commons
- Download Page (PDF)
- Download Full Book (PDF)
- Periodic Table
- Physics Constants
- Scientific Calculator
- Reference & Cite
- Tools expand_more
- Readability
selected template will load here
This action is not available.
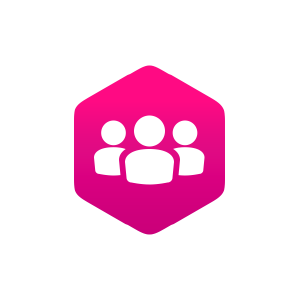
5.1: The Importance of Audience Analysis
- Last updated
- Save as PDF
- Page ID 106002
The Benefits of Understanding Your Audience
The more you know and understand about the background and needs of your audience, the better you can prepare your speech.
LEARNING OBJECTIVE
Explain why it is important to understand your audience prior to delivering a speech
KEY TAKEAWAYS
Knowing your audience —their general age, gender, education level, religion, language, culture, and group membership—is the single most important aspect of developing your speech.
- Analyzing your audience will help you discover information that you can use to build common ground between you and the members of your audience.
- A key characteristic in public speaking situations is the unequal distribution of speaking time between the speaker and the audience. This means that the speaker talks more and the audience listens, often without asking questions or responding with any feedback.
- audience : One or more people within hearing range of some message; for example, a group of people listening to a performance or speech; the crowd attending a stage performance.
- audience analysis : A study of the pertinent elements defining the makeup and characteristics of an audience.
- Audience-centered : Tailored to an audience. When preparing a message, the speaker analyzes the audience in order to adapt the content and language usage to the level of the listeners.
Benefits of Understanding Audiences
When you are speaking, you want listeners to understand and respond favorably to what you are saying. An audience is one or more people who come together to listen to the speaker. Audience members may be face to face with the speaker or they may be connected by communication technology such as computers or other media. The audience may be small and private or it may be large and public. A key characteristic of public speaking situations is the unequal distribution of speaking time between speaker and audience. As an example, the speaker usually talks more while the audience listens, often without asking questions or responding with any feedback. In some situations, the audience may ask questions or respond overtly by clapping or making comments.
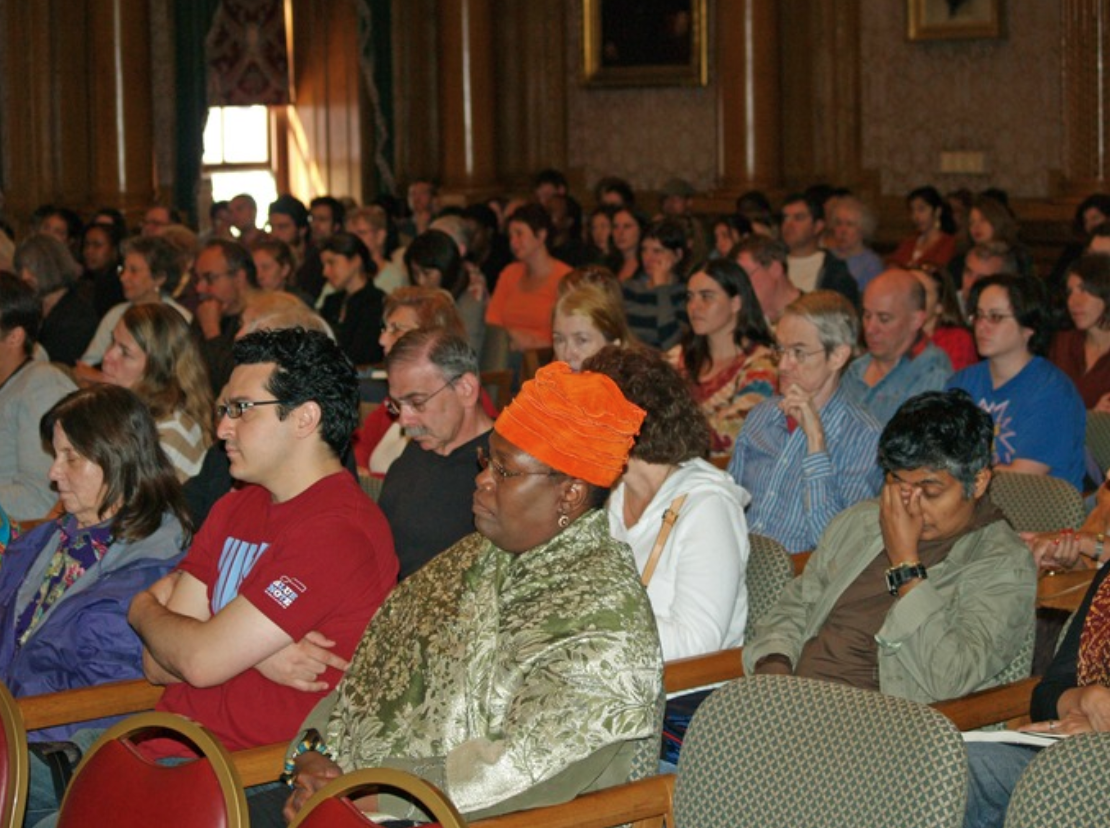
Audience-Centered Approach to Speaking
Since there is usually limited communication between the speaker and the audience, there is limited opportunity to go back to explain your meaning either during the speech or afterward. When planning a speech, it is important to know about the audience and to adapt the message to the audience. You want to prepare an audience-centered speech, a speech with a focus on the audience.
In public speaking, you are speaking to and for your audience; thus, understanding the audience is a major part of the speech-making process. In audience-centered speaking, getting to know your target audience is one of the most important tasks that you face. You want to learn about the major demographics of the audience, such as general age, gender, education, religion, and culture, as well as to what groups the audience members belong. Additionally, learning about the values, attitudes, and beliefs of the members of your audience will allow you to anticipate and plan your message.
Finding Common Ground by Taking Perspective
You want to analyze your audience prior to your speech so that during the speech you can create a link between you, the speaker, and the audience. You want to be able to figuratively step inside the minds of audience members to understand the world from their perspectives. Through this process, you can find common ground with your audience, which allows you to align your message with what the audience already knows or believes.
Gathering and Interpreting Information
Audience analysis involves gathering and interpreting information about the recipients of oral, written, or visual communication. There are very simple methods for conducting an audience analysis, such as interviewing a small group about its knowledge or attitudes or using more involved methods of analyzing demographic studies of relevant segments of the population. You may also find it useful to look at sociological studies of different age groups or cultural groups. You might also use a questionnaire or rating scale to collect data about the basic demographic information and opinions of your target audience. These examples do not form an all-inclusive list of methods to analyze your audience, but they can help you obtain a general understanding of how you can learn about your audience. After considering all the known factors, a profile of the intended audience can be created, allowing you to speak in a manner that is understood by the intended audience.
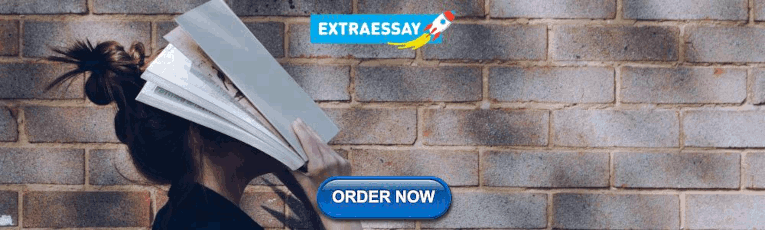
Practical Benefits for the Speaker
Understanding who makes up your target audience will allow you to carefully plan your message and adapt what you say to the level of understanding and background of the listeners. Two practical benefits of conducting an audience analysis are (1) to prevent you from saying the wrong thing, such as telling a joke which offends, and (2) to help you speak to your audience in a language they understand about things that interest them. Your speech will be more successful if you can create a message that informs and engages your audience.
What to Look For
Analyze the audience to find the mix of ages, genders, sexual orientations, educational levels, religions, cultures, ethnicities, and races.
Examine your audience based on demographics
- A speaker should look at his or her own values, beliefs, attitudes, and biases that may influence his or her perception of others.
- Guard against egocentrism. A speaker must not regard his or her own opinions or interests as being the most important or valid.
- Look at others to understand their background, attitudes, and beliefs.
- Focus on audience demographics such as age, gender, sexual orientation, education, religion, and other relevant population characteristics to analyze the audience.
- The depth of the audience analysis depends of the size of the intended audience and the method of delivery.
- egocentrism : Preoccupation with one’s own internal world; the belief that one’s own opinions or interests are the most important or valid.
- demographics : The characteristics of population such as age, gender, sexual orientation, occupation, education; classification of the characteristics of the people.
Look Inward to Uncover Blinders
A public speaker should turn her mental magnifying glass inward to examine the values, beliefs, attitudes, and biases that may influence her perception of others. The speaker should use this mental picture to look at the audience and view the world from the audience’s perspective. By looking at the audience, the speaker understands their reality.
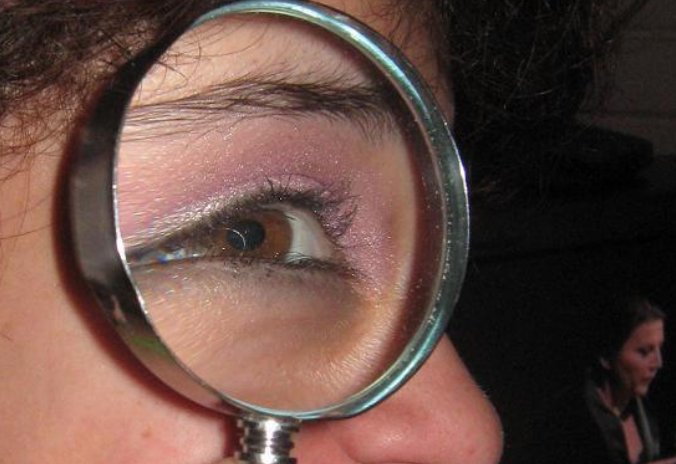
When the speaker views the audience only through her mental perception, she is likely to engage in egocentrism. Egocentrism is characterized by the preoccupation with one’s own internal world. Egocentrics regard themselves and their own opinions or interests as being the most important or valid. Egocentric people are unable to fully understand or cope with other people’s opinions and a reality that is different from what they are ready to accept.
Understanding Audience Background, Attitudes, and Beliefs
Public speakers must look at who their audience is, their background, attitudes, and beliefs. The speaker should attempt to reach the most accurate and effective analysis of her audience within a reasonable amount of time. For example, speakers can assess the demographics of her audience. Demographics are detailed accounts of human population characteristics and usually rendered as statistical population segments.
For an analysis of audience demographics for a speech, focus on the same characteristics studied in sociology. Audiences and populations comprise groups of people represented by different age groups that:
- Are of the same or mixed genders
- Have experienced the same events
- Have the same or different sexual orientation
- Have different educational attainment
- Participate in different religions
- Represent different cultures, ethnicities, or races
Speakers assess the audience’s attitude – a positive or negative evaluation of people, objects, event, activities, or ideas – toward a specific topic or purpose. The attitudes of the audience may vary from extremely negative to extremely positive, or completely ambivalent. By examining the preexisting beliefs of the audience regarding the speech’s general topic or particular purpose, speakers have the ability to persuade the audience members to buy into the speaker’s argument. This can also help with speech preparation.
Tips for the Speaker
The depth of the audience analysis depends of the size of the intended audience and method of delivery. Speakers use different methods to become familiar with the background, attitudes, and beliefs of audiences in different environments and using various mediums (e.g., videoconferencing, phone, etc). For a small audience, the speaker can simply speak with them in a physical environment. However, the speaker is addressing a larger audience or speaking via teleconferencing or webcasting tools, it may be useful to collect data via surveys or questionnaires.
What to Do with Your Knowledge
Use knowledge about your audience to step into their minds, create an imaginary scenario, and test your ideas.
Identify with your audience by adopting their perspective
- A successful speaker is able to step outside her own perceptual framework to understand the world as it is perceived by members of her audience.
- The speaker engages in a process of first encoding his or her ideas from thoughts into words, then forming a message to be delivered to a group of listeners, or audience. The audience members attempt to decode what the speaker is saying so that they can understand it.
- The better the speaker knows the members of the audience beforehand, the better the speaker can encode a message in a way that the audience can decode successfully.
- One of the most useful strategies for adapting your topic and message to your audience is to use the process of identification to find common ground with them.
- You can use your analysis to create a theoretical, imagined audience of individuals from the diverse backgrounds you have discovered in your audience analysis. Then you can decide whether or not the content will appeal to individuals within that audience.
- encode : to turn one’s ideas into spoken language in order to transmit them to listeners
- message : the verbal and nonverbal components of language, sent to the receiver by the sender, that convey an idea
- Decode : to translate the sender’s spoken idea/message into something the receiver understands by using his or her knowledge of language based on personal experience
Identifying with the listeners
Step in to the minds of your listeners and see if you can identify with them. A successful speaker engages in perspective-taking. While preparing her speech, the speaker steps outside her own perceptual framework to understand the world as it is perceived by members of the audience. When the speaker takes an audience-centered approach to speech preparation, she focuses on the audience and how it will respond to what is being said. In essence, the speaker wants to mentally adopt the perspective of members of the audience in order to see the world as the audience members see it.
Encoding and Decoding
The speaker engages a process of encoding his or her ideas from thoughts into words, and of forming a message which is then delivered to an audience. The audience members then attempt to decode what the speaker is saying so that they can understand it. To better imagine this process, consider the example of encoding and decoding as it applies to the idea of a tree. I know that my audience is in New England and that they are familiar with oak trees. I use the word tree to encode my idea, and because my audience has experienced similar trees, they decode the word tree in the way that I intended. However, I may be thinking about a tree (a palm tree) that is in Hawaii, where I used to live, when I use the word tree to encode my idea. Unfortunately, when my audience decodes my word now, they are still thinking about the oak tree and will not see my palm tree. The audience no longer shares my perspective of the world or my experience with trees.
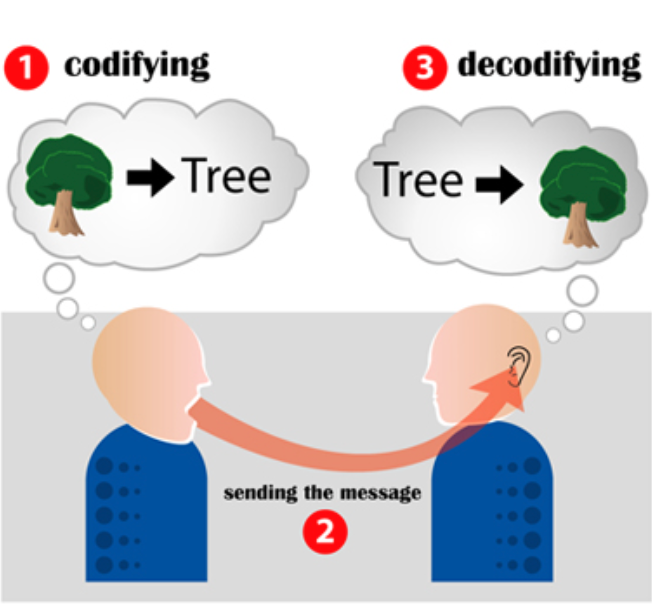
Finding Common Ground
The more you find out about your audience, the more you can adapt your message to the interests, values, beliefs, and language level of the audience. Once you collect data about your audience, you are ready to summarize your findings and select the language and structure that is best suited to your particular audience. You are on a journey to find common ground in order to identify with your audience. One of the most useful strategies for adapting your topic and message to your audience is to use the process of identification. What do you and your audience have in common? And, conversely, how are you different? What ideas or examples in your speech can your audience identify with?
Creating a Theoretical, Imagined Audience
Create a theoretical, imagined situation to test your view of an audience for practice. You can use your analysis to create what is called a “theoretical, universal audience. ” The universal audience is an imagined audience that serves as a test for the speaker. Imagine in your mind a composite audience that contains individuals from the diverse backgrounds you have discovered in your audience analysis. Next, decide whether or not the content of your speech would appeal to individuals within that audience. What words or examples will the audience understand and what will they not understand? What terms about your subject will you need to define or explain for this audience? How different are the values and opinions you want your audience to accept from the present attitudes and beliefs they may hold?
In summary, use your knowledge of the audience to adapt your speech accordingly. Adopt the perspective of the audience in order to identify with them, and test out your ideas with an imagined audience composed of people with the background you have discovered through your research.
Your Modern Business Guide To Data Analysis Methods And Techniques

Table of Contents
1) What Is Data Analysis?
2) Why Is Data Analysis Important?
3) What Is The Data Analysis Process?
4) Types Of Data Analysis Methods
5) Top Data Analysis Techniques To Apply
6) Quality Criteria For Data Analysis
7) Data Analysis Limitations & Barriers
8) Data Analysis Skills
9) Data Analysis In The Big Data Environment
In our data-rich age, understanding how to analyze and extract true meaning from our business’s digital insights is one of the primary drivers of success.
Despite the colossal volume of data we create every day, a mere 0.5% is actually analyzed and used for data discovery , improvement, and intelligence. While that may not seem like much, considering the amount of digital information we have at our fingertips, half a percent still accounts for a vast amount of data.
With so much data and so little time, knowing how to collect, curate, organize, and make sense of all of this potentially business-boosting information can be a minefield – but online data analysis is the solution.
In science, data analysis uses a more complex approach with advanced techniques to explore and experiment with data. On the other hand, in a business context, data is used to make data-driven decisions that will enable the company to improve its overall performance. In this post, we will cover the analysis of data from an organizational point of view while still going through the scientific and statistical foundations that are fundamental to understanding the basics of data analysis.
To put all of that into perspective, we will answer a host of important analytical questions, explore analytical methods and techniques, while demonstrating how to perform analysis in the real world with a 17-step blueprint for success.
What Is Data Analysis?
Data analysis is the process of collecting, modeling, and analyzing data using various statistical and logical methods and techniques. Businesses rely on analytics processes and tools to extract insights that support strategic and operational decision-making.
All these various methods are largely based on two core areas: quantitative and qualitative research.
To explain the key differences between qualitative and quantitative research, here’s a video for your viewing pleasure:
Gaining a better understanding of different techniques and methods in quantitative research as well as qualitative insights will give your analyzing efforts a more clearly defined direction, so it’s worth taking the time to allow this particular knowledge to sink in. Additionally, you will be able to create a comprehensive analytical report that will skyrocket your analysis.
Apart from qualitative and quantitative categories, there are also other types of data that you should be aware of before dividing into complex data analysis processes. These categories include:
- Big data: Refers to massive data sets that need to be analyzed using advanced software to reveal patterns and trends. It is considered to be one of the best analytical assets as it provides larger volumes of data at a faster rate.
- Metadata: Putting it simply, metadata is data that provides insights about other data. It summarizes key information about specific data that makes it easier to find and reuse for later purposes.
- Real time data: As its name suggests, real time data is presented as soon as it is acquired. From an organizational perspective, this is the most valuable data as it can help you make important decisions based on the latest developments. Our guide on real time analytics will tell you more about the topic.
- Machine data: This is more complex data that is generated solely by a machine such as phones, computers, or even websites and embedded systems, without previous human interaction.
Why Is Data Analysis Important?
Before we go into detail about the categories of analysis along with its methods and techniques, you must understand the potential that analyzing data can bring to your organization.
- Informed decision-making : From a management perspective, you can benefit from analyzing your data as it helps you make decisions based on facts and not simple intuition. For instance, you can understand where to invest your capital, detect growth opportunities, predict your income, or tackle uncommon situations before they become problems. Through this, you can extract relevant insights from all areas in your organization, and with the help of dashboard software , present the data in a professional and interactive way to different stakeholders.
- Reduce costs : Another great benefit is to reduce costs. With the help of advanced technologies such as predictive analytics, businesses can spot improvement opportunities, trends, and patterns in their data and plan their strategies accordingly. In time, this will help you save money and resources on implementing the wrong strategies. And not just that, by predicting different scenarios such as sales and demand you can also anticipate production and supply.
- Target customers better : Customers are arguably the most crucial element in any business. By using analytics to get a 360° vision of all aspects related to your customers, you can understand which channels they use to communicate with you, their demographics, interests, habits, purchasing behaviors, and more. In the long run, it will drive success to your marketing strategies, allow you to identify new potential customers, and avoid wasting resources on targeting the wrong people or sending the wrong message. You can also track customer satisfaction by analyzing your client’s reviews or your customer service department’s performance.
What Is The Data Analysis Process?

When we talk about analyzing data there is an order to follow in order to extract the needed conclusions. The analysis process consists of 5 key stages. We will cover each of them more in detail later in the post, but to start providing the needed context to understand what is coming next, here is a rundown of the 5 essential steps of data analysis.
- Identify: Before you get your hands dirty with data, you first need to identify why you need it in the first place. The identification is the stage in which you establish the questions you will need to answer. For example, what is the customer's perception of our brand? Or what type of packaging is more engaging to our potential customers? Once the questions are outlined you are ready for the next step.
- Collect: As its name suggests, this is the stage where you start collecting the needed data. Here, you define which sources of data you will use and how you will use them. The collection of data can come in different forms such as internal or external sources, surveys, interviews, questionnaires, and focus groups, among others. An important note here is that the way you collect the data will be different in a quantitative and qualitative scenario.
- Clean: Once you have the necessary data it is time to clean it and leave it ready for analysis. Not all the data you collect will be useful, when collecting big amounts of data in different formats it is very likely that you will find yourself with duplicate or badly formatted data. To avoid this, before you start working with your data you need to make sure to erase any white spaces, duplicate records, or formatting errors. This way you avoid hurting your analysis with bad-quality data.
- Analyze : With the help of various techniques such as statistical analysis, regressions, neural networks, text analysis, and more, you can start analyzing and manipulating your data to extract relevant conclusions. At this stage, you find trends, correlations, variations, and patterns that can help you answer the questions you first thought of in the identify stage. Various technologies in the market assist researchers and average users with the management of their data. Some of them include business intelligence and visualization software, predictive analytics, and data mining, among others.
- Interpret: Last but not least you have one of the most important steps: it is time to interpret your results. This stage is where the researcher comes up with courses of action based on the findings. For example, here you would understand if your clients prefer packaging that is red or green, plastic or paper, etc. Additionally, at this stage, you can also find some limitations and work on them.
Now that you have a basic understanding of the key data analysis steps, let’s look at the top 17 essential methods.
17 Essential Types Of Data Analysis Methods
Before diving into the 17 essential types of methods, it is important that we go over really fast through the main analysis categories. Starting with the category of descriptive up to prescriptive analysis, the complexity and effort of data evaluation increases, but also the added value for the company.
a) Descriptive analysis - What happened.
The descriptive analysis method is the starting point for any analytic reflection, and it aims to answer the question of what happened? It does this by ordering, manipulating, and interpreting raw data from various sources to turn it into valuable insights for your organization.
Performing descriptive analysis is essential, as it enables us to present our insights in a meaningful way. Although it is relevant to mention that this analysis on its own will not allow you to predict future outcomes or tell you the answer to questions like why something happened, it will leave your data organized and ready to conduct further investigations.
b) Exploratory analysis - How to explore data relationships.
As its name suggests, the main aim of the exploratory analysis is to explore. Prior to it, there is still no notion of the relationship between the data and the variables. Once the data is investigated, exploratory analysis helps you to find connections and generate hypotheses and solutions for specific problems. A typical area of application for it is data mining.
c) Diagnostic analysis - Why it happened.
Diagnostic data analytics empowers analysts and executives by helping them gain a firm contextual understanding of why something happened. If you know why something happened as well as how it happened, you will be able to pinpoint the exact ways of tackling the issue or challenge.
Designed to provide direct and actionable answers to specific questions, this is one of the world’s most important methods in research, among its other key organizational functions such as retail analytics , e.g.
c) Predictive analysis - What will happen.
The predictive method allows you to look into the future to answer the question: what will happen? In order to do this, it uses the results of the previously mentioned descriptive, exploratory, and diagnostic analysis, in addition to machine learning (ML) and artificial intelligence (AI). Through this, you can uncover future trends, potential problems or inefficiencies, connections, and casualties in your data.
With predictive analysis, you can unfold and develop initiatives that will not only enhance your various operational processes but also help you gain an all-important edge over the competition. If you understand why a trend, pattern, or event happened through data, you will be able to develop an informed projection of how things may unfold in particular areas of the business.
e) Prescriptive analysis - How will it happen.
Another of the most effective types of analysis methods in research. Prescriptive data techniques cross over from predictive analysis in the way that it revolves around using patterns or trends to develop responsive, practical business strategies.
By drilling down into prescriptive analysis, you will play an active role in the data consumption process by taking well-arranged sets of visual data and using it as a powerful fix to emerging issues in a number of key areas, including marketing, sales, customer experience, HR, fulfillment, finance, logistics analytics , and others.

As mentioned at the beginning of the post, data analysis methods can be divided into two big categories: quantitative and qualitative. Each of these categories holds a powerful analytical value that changes depending on the scenario and type of data you are working with. Below, we will discuss 17 methods that are divided into qualitative and quantitative approaches.
Without further ado, here are the 17 essential types of data analysis methods with some use cases in the business world:
A. Quantitative Methods
To put it simply, quantitative analysis refers to all methods that use numerical data or data that can be turned into numbers (e.g. category variables like gender, age, etc.) to extract valuable insights. It is used to extract valuable conclusions about relationships, differences, and test hypotheses. Below we discuss some of the key quantitative methods.
1. Cluster analysis
The action of grouping a set of data elements in a way that said elements are more similar (in a particular sense) to each other than to those in other groups – hence the term ‘cluster.’ Since there is no target variable when clustering, the method is often used to find hidden patterns in the data. The approach is also used to provide additional context to a trend or dataset.
Let's look at it from an organizational perspective. In a perfect world, marketers would be able to analyze each customer separately and give them the best-personalized service, but let's face it, with a large customer base, it is timely impossible to do that. That's where clustering comes in. By grouping customers into clusters based on demographics, purchasing behaviors, monetary value, or any other factor that might be relevant for your company, you will be able to immediately optimize your efforts and give your customers the best experience based on their needs.
2. Cohort analysis
This type of data analysis approach uses historical data to examine and compare a determined segment of users' behavior, which can then be grouped with others with similar characteristics. By using this methodology, it's possible to gain a wealth of insight into consumer needs or a firm understanding of a broader target group.
Cohort analysis can be really useful for performing analysis in marketing as it will allow you to understand the impact of your campaigns on specific groups of customers. To exemplify, imagine you send an email campaign encouraging customers to sign up for your site. For this, you create two versions of the campaign with different designs, CTAs, and ad content. Later on, you can use cohort analysis to track the performance of the campaign for a longer period of time and understand which type of content is driving your customers to sign up, repurchase, or engage in other ways.
A useful tool to start performing cohort analysis method is Google Analytics. You can learn more about the benefits and limitations of using cohorts in GA in this useful guide . In the bottom image, you see an example of how you visualize a cohort in this tool. The segments (devices traffic) are divided into date cohorts (usage of devices) and then analyzed week by week to extract insights into performance.
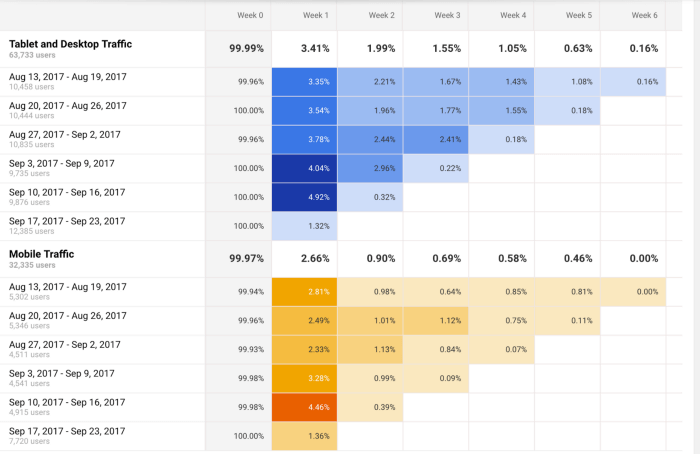
3. Regression analysis
Regression uses historical data to understand how a dependent variable's value is affected when one (linear regression) or more independent variables (multiple regression) change or stay the same. By understanding each variable's relationship and how it developed in the past, you can anticipate possible outcomes and make better decisions in the future.
Let's bring it down with an example. Imagine you did a regression analysis of your sales in 2019 and discovered that variables like product quality, store design, customer service, marketing campaigns, and sales channels affected the overall result. Now you want to use regression to analyze which of these variables changed or if any new ones appeared during 2020. For example, you couldn’t sell as much in your physical store due to COVID lockdowns. Therefore, your sales could’ve either dropped in general or increased in your online channels. Through this, you can understand which independent variables affected the overall performance of your dependent variable, annual sales.
If you want to go deeper into this type of analysis, check out this article and learn more about how you can benefit from regression.
4. Neural networks
The neural network forms the basis for the intelligent algorithms of machine learning. It is a form of analytics that attempts, with minimal intervention, to understand how the human brain would generate insights and predict values. Neural networks learn from each and every data transaction, meaning that they evolve and advance over time.
A typical area of application for neural networks is predictive analytics. There are BI reporting tools that have this feature implemented within them, such as the Predictive Analytics Tool from datapine. This tool enables users to quickly and easily generate all kinds of predictions. All you have to do is select the data to be processed based on your KPIs, and the software automatically calculates forecasts based on historical and current data. Thanks to its user-friendly interface, anyone in your organization can manage it; there’s no need to be an advanced scientist.
Here is an example of how you can use the predictive analysis tool from datapine:

**click to enlarge**
5. Factor analysis
The factor analysis also called “dimension reduction” is a type of data analysis used to describe variability among observed, correlated variables in terms of a potentially lower number of unobserved variables called factors. The aim here is to uncover independent latent variables, an ideal method for streamlining specific segments.
A good way to understand this data analysis method is a customer evaluation of a product. The initial assessment is based on different variables like color, shape, wearability, current trends, materials, comfort, the place where they bought the product, and frequency of usage. Like this, the list can be endless, depending on what you want to track. In this case, factor analysis comes into the picture by summarizing all of these variables into homogenous groups, for example, by grouping the variables color, materials, quality, and trends into a brother latent variable of design.
If you want to start analyzing data using factor analysis we recommend you take a look at this practical guide from UCLA.
6. Data mining
A method of data analysis that is the umbrella term for engineering metrics and insights for additional value, direction, and context. By using exploratory statistical evaluation, data mining aims to identify dependencies, relations, patterns, and trends to generate advanced knowledge. When considering how to analyze data, adopting a data mining mindset is essential to success - as such, it’s an area that is worth exploring in greater detail.
An excellent use case of data mining is datapine intelligent data alerts . With the help of artificial intelligence and machine learning, they provide automated signals based on particular commands or occurrences within a dataset. For example, if you’re monitoring supply chain KPIs , you could set an intelligent alarm to trigger when invalid or low-quality data appears. By doing so, you will be able to drill down deep into the issue and fix it swiftly and effectively.
In the following picture, you can see how the intelligent alarms from datapine work. By setting up ranges on daily orders, sessions, and revenues, the alarms will notify you if the goal was not completed or if it exceeded expectations.
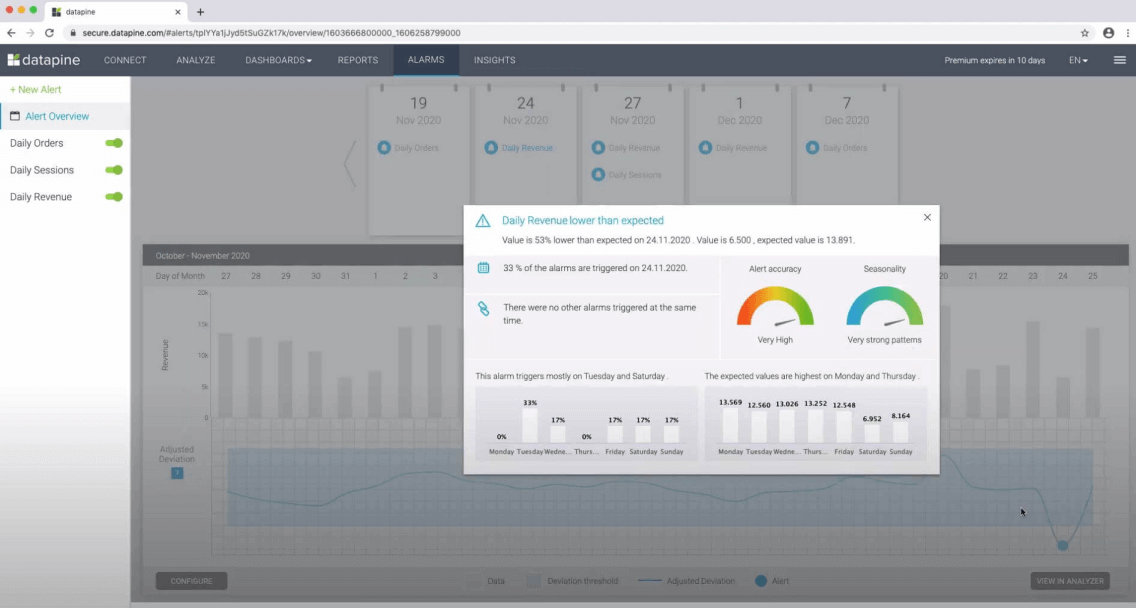
7. Time series analysis
As its name suggests, time series analysis is used to analyze a set of data points collected over a specified period of time. Although analysts use this method to monitor the data points in a specific interval of time rather than just monitoring them intermittently, the time series analysis is not uniquely used for the purpose of collecting data over time. Instead, it allows researchers to understand if variables changed during the duration of the study, how the different variables are dependent, and how did it reach the end result.
In a business context, this method is used to understand the causes of different trends and patterns to extract valuable insights. Another way of using this method is with the help of time series forecasting. Powered by predictive technologies, businesses can analyze various data sets over a period of time and forecast different future events.
A great use case to put time series analysis into perspective is seasonality effects on sales. By using time series forecasting to analyze sales data of a specific product over time, you can understand if sales rise over a specific period of time (e.g. swimwear during summertime, or candy during Halloween). These insights allow you to predict demand and prepare production accordingly.
8. Decision Trees
The decision tree analysis aims to act as a support tool to make smart and strategic decisions. By visually displaying potential outcomes, consequences, and costs in a tree-like model, researchers and company users can easily evaluate all factors involved and choose the best course of action. Decision trees are helpful to analyze quantitative data and they allow for an improved decision-making process by helping you spot improvement opportunities, reduce costs, and enhance operational efficiency and production.
But how does a decision tree actually works? This method works like a flowchart that starts with the main decision that you need to make and branches out based on the different outcomes and consequences of each decision. Each outcome will outline its own consequences, costs, and gains and, at the end of the analysis, you can compare each of them and make the smartest decision.
Businesses can use them to understand which project is more cost-effective and will bring more earnings in the long run. For example, imagine you need to decide if you want to update your software app or build a new app entirely. Here you would compare the total costs, the time needed to be invested, potential revenue, and any other factor that might affect your decision. In the end, you would be able to see which of these two options is more realistic and attainable for your company or research.
9. Conjoint analysis
Last but not least, we have the conjoint analysis. This approach is usually used in surveys to understand how individuals value different attributes of a product or service and it is one of the most effective methods to extract consumer preferences. When it comes to purchasing, some clients might be more price-focused, others more features-focused, and others might have a sustainable focus. Whatever your customer's preferences are, you can find them with conjoint analysis. Through this, companies can define pricing strategies, packaging options, subscription packages, and more.
A great example of conjoint analysis is in marketing and sales. For instance, a cupcake brand might use conjoint analysis and find that its clients prefer gluten-free options and cupcakes with healthier toppings over super sugary ones. Thus, the cupcake brand can turn these insights into advertisements and promotions to increase sales of this particular type of product. And not just that, conjoint analysis can also help businesses segment their customers based on their interests. This allows them to send different messaging that will bring value to each of the segments.
10. Correspondence Analysis
Also known as reciprocal averaging, correspondence analysis is a method used to analyze the relationship between categorical variables presented within a contingency table. A contingency table is a table that displays two (simple correspondence analysis) or more (multiple correspondence analysis) categorical variables across rows and columns that show the distribution of the data, which is usually answers to a survey or questionnaire on a specific topic.
This method starts by calculating an “expected value” which is done by multiplying row and column averages and dividing it by the overall original value of the specific table cell. The “expected value” is then subtracted from the original value resulting in a “residual number” which is what allows you to extract conclusions about relationships and distribution. The results of this analysis are later displayed using a map that represents the relationship between the different values. The closest two values are in the map, the bigger the relationship. Let’s put it into perspective with an example.
Imagine you are carrying out a market research analysis about outdoor clothing brands and how they are perceived by the public. For this analysis, you ask a group of people to match each brand with a certain attribute which can be durability, innovation, quality materials, etc. When calculating the residual numbers, you can see that brand A has a positive residual for innovation but a negative one for durability. This means that brand A is not positioned as a durable brand in the market, something that competitors could take advantage of.
11. Multidimensional Scaling (MDS)
MDS is a method used to observe the similarities or disparities between objects which can be colors, brands, people, geographical coordinates, and more. The objects are plotted using an “MDS map” that positions similar objects together and disparate ones far apart. The (dis) similarities between objects are represented using one or more dimensions that can be observed using a numerical scale. For example, if you want to know how people feel about the COVID-19 vaccine, you can use 1 for “don’t believe in the vaccine at all” and 10 for “firmly believe in the vaccine” and a scale of 2 to 9 for in between responses. When analyzing an MDS map the only thing that matters is the distance between the objects, the orientation of the dimensions is arbitrary and has no meaning at all.
Multidimensional scaling is a valuable technique for market research, especially when it comes to evaluating product or brand positioning. For instance, if a cupcake brand wants to know how they are positioned compared to competitors, it can define 2-3 dimensions such as taste, ingredients, shopping experience, or more, and do a multidimensional scaling analysis to find improvement opportunities as well as areas in which competitors are currently leading.
Another business example is in procurement when deciding on different suppliers. Decision makers can generate an MDS map to see how the different prices, delivery times, technical services, and more of the different suppliers differ and pick the one that suits their needs the best.
A final example proposed by a research paper on "An Improved Study of Multilevel Semantic Network Visualization for Analyzing Sentiment Word of Movie Review Data". Researchers picked a two-dimensional MDS map to display the distances and relationships between different sentiments in movie reviews. They used 36 sentiment words and distributed them based on their emotional distance as we can see in the image below where the words "outraged" and "sweet" are on opposite sides of the map, marking the distance between the two emotions very clearly.

Aside from being a valuable technique to analyze dissimilarities, MDS also serves as a dimension-reduction technique for large dimensional data.
B. Qualitative Methods
Qualitative data analysis methods are defined as the observation of non-numerical data that is gathered and produced using methods of observation such as interviews, focus groups, questionnaires, and more. As opposed to quantitative methods, qualitative data is more subjective and highly valuable in analyzing customer retention and product development.
12. Text analysis
Text analysis, also known in the industry as text mining, works by taking large sets of textual data and arranging them in a way that makes it easier to manage. By working through this cleansing process in stringent detail, you will be able to extract the data that is truly relevant to your organization and use it to develop actionable insights that will propel you forward.
Modern software accelerate the application of text analytics. Thanks to the combination of machine learning and intelligent algorithms, you can perform advanced analytical processes such as sentiment analysis. This technique allows you to understand the intentions and emotions of a text, for example, if it's positive, negative, or neutral, and then give it a score depending on certain factors and categories that are relevant to your brand. Sentiment analysis is often used to monitor brand and product reputation and to understand how successful your customer experience is. To learn more about the topic check out this insightful article .
By analyzing data from various word-based sources, including product reviews, articles, social media communications, and survey responses, you will gain invaluable insights into your audience, as well as their needs, preferences, and pain points. This will allow you to create campaigns, services, and communications that meet your prospects’ needs on a personal level, growing your audience while boosting customer retention. There are various other “sub-methods” that are an extension of text analysis. Each of them serves a more specific purpose and we will look at them in detail next.
13. Content Analysis
This is a straightforward and very popular method that examines the presence and frequency of certain words, concepts, and subjects in different content formats such as text, image, audio, or video. For example, the number of times the name of a celebrity is mentioned on social media or online tabloids. It does this by coding text data that is later categorized and tabulated in a way that can provide valuable insights, making it the perfect mix of quantitative and qualitative analysis.
There are two types of content analysis. The first one is the conceptual analysis which focuses on explicit data, for instance, the number of times a concept or word is mentioned in a piece of content. The second one is relational analysis, which focuses on the relationship between different concepts or words and how they are connected within a specific context.
Content analysis is often used by marketers to measure brand reputation and customer behavior. For example, by analyzing customer reviews. It can also be used to analyze customer interviews and find directions for new product development. It is also important to note, that in order to extract the maximum potential out of this analysis method, it is necessary to have a clearly defined research question.
14. Thematic Analysis
Very similar to content analysis, thematic analysis also helps in identifying and interpreting patterns in qualitative data with the main difference being that the first one can also be applied to quantitative analysis. The thematic method analyzes large pieces of text data such as focus group transcripts or interviews and groups them into themes or categories that come up frequently within the text. It is a great method when trying to figure out peoples view’s and opinions about a certain topic. For example, if you are a brand that cares about sustainability, you can do a survey of your customers to analyze their views and opinions about sustainability and how they apply it to their lives. You can also analyze customer service calls transcripts to find common issues and improve your service.
Thematic analysis is a very subjective technique that relies on the researcher’s judgment. Therefore, to avoid biases, it has 6 steps that include familiarization, coding, generating themes, reviewing themes, defining and naming themes, and writing up. It is also important to note that, because it is a flexible approach, the data can be interpreted in multiple ways and it can be hard to select what data is more important to emphasize.
15. Narrative Analysis
A bit more complex in nature than the two previous ones, narrative analysis is used to explore the meaning behind the stories that people tell and most importantly, how they tell them. By looking into the words that people use to describe a situation you can extract valuable conclusions about their perspective on a specific topic. Common sources for narrative data include autobiographies, family stories, opinion pieces, and testimonials, among others.
From a business perspective, narrative analysis can be useful to analyze customer behaviors and feelings towards a specific product, service, feature, or others. It provides unique and deep insights that can be extremely valuable. However, it has some drawbacks.
The biggest weakness of this method is that the sample sizes are usually very small due to the complexity and time-consuming nature of the collection of narrative data. Plus, the way a subject tells a story will be significantly influenced by his or her specific experiences, making it very hard to replicate in a subsequent study.
16. Discourse Analysis
Discourse analysis is used to understand the meaning behind any type of written, verbal, or symbolic discourse based on its political, social, or cultural context. It mixes the analysis of languages and situations together. This means that the way the content is constructed and the meaning behind it is significantly influenced by the culture and society it takes place in. For example, if you are analyzing political speeches you need to consider different context elements such as the politician's background, the current political context of the country, the audience to which the speech is directed, and so on.
From a business point of view, discourse analysis is a great market research tool. It allows marketers to understand how the norms and ideas of the specific market work and how their customers relate to those ideas. It can be very useful to build a brand mission or develop a unique tone of voice.
17. Grounded Theory Analysis
Traditionally, researchers decide on a method and hypothesis and start to collect the data to prove that hypothesis. The grounded theory is the only method that doesn’t require an initial research question or hypothesis as its value lies in the generation of new theories. With the grounded theory method, you can go into the analysis process with an open mind and explore the data to generate new theories through tests and revisions. In fact, it is not necessary to collect the data and then start to analyze it. Researchers usually start to find valuable insights as they are gathering the data.
All of these elements make grounded theory a very valuable method as theories are fully backed by data instead of initial assumptions. It is a great technique to analyze poorly researched topics or find the causes behind specific company outcomes. For example, product managers and marketers might use the grounded theory to find the causes of high levels of customer churn and look into customer surveys and reviews to develop new theories about the causes.
How To Analyze Data? Top 17 Data Analysis Techniques To Apply

Now that we’ve answered the questions “what is data analysis’”, why is it important, and covered the different data analysis types, it’s time to dig deeper into how to perform your analysis by working through these 17 essential techniques.
1. Collaborate your needs
Before you begin analyzing or drilling down into any techniques, it’s crucial to sit down collaboratively with all key stakeholders within your organization, decide on your primary campaign or strategic goals, and gain a fundamental understanding of the types of insights that will best benefit your progress or provide you with the level of vision you need to evolve your organization.
2. Establish your questions
Once you’ve outlined your core objectives, you should consider which questions will need answering to help you achieve your mission. This is one of the most important techniques as it will shape the very foundations of your success.
To help you ask the right things and ensure your data works for you, you have to ask the right data analysis questions .
3. Data democratization
After giving your data analytics methodology some real direction, and knowing which questions need answering to extract optimum value from the information available to your organization, you should continue with democratization.
Data democratization is an action that aims to connect data from various sources efficiently and quickly so that anyone in your organization can access it at any given moment. You can extract data in text, images, videos, numbers, or any other format. And then perform cross-database analysis to achieve more advanced insights to share with the rest of the company interactively.
Once you have decided on your most valuable sources, you need to take all of this into a structured format to start collecting your insights. For this purpose, datapine offers an easy all-in-one data connectors feature to integrate all your internal and external sources and manage them at your will. Additionally, datapine’s end-to-end solution automatically updates your data, allowing you to save time and focus on performing the right analysis to grow your company.
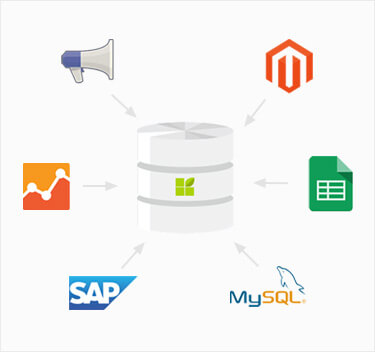
4. Think of governance
When collecting data in a business or research context you always need to think about security and privacy. With data breaches becoming a topic of concern for businesses, the need to protect your client's or subject’s sensitive information becomes critical.
To ensure that all this is taken care of, you need to think of a data governance strategy. According to Gartner , this concept refers to “ the specification of decision rights and an accountability framework to ensure the appropriate behavior in the valuation, creation, consumption, and control of data and analytics .” In simpler words, data governance is a collection of processes, roles, and policies, that ensure the efficient use of data while still achieving the main company goals. It ensures that clear roles are in place for who can access the information and how they can access it. In time, this not only ensures that sensitive information is protected but also allows for an efficient analysis as a whole.
5. Clean your data
After harvesting from so many sources you will be left with a vast amount of information that can be overwhelming to deal with. At the same time, you can be faced with incorrect data that can be misleading to your analysis. The smartest thing you can do to avoid dealing with this in the future is to clean the data. This is fundamental before visualizing it, as it will ensure that the insights you extract from it are correct.
There are many things that you need to look for in the cleaning process. The most important one is to eliminate any duplicate observations; this usually appears when using multiple internal and external sources of information. You can also add any missing codes, fix empty fields, and eliminate incorrectly formatted data.
Another usual form of cleaning is done with text data. As we mentioned earlier, most companies today analyze customer reviews, social media comments, questionnaires, and several other text inputs. In order for algorithms to detect patterns, text data needs to be revised to avoid invalid characters or any syntax or spelling errors.
Most importantly, the aim of cleaning is to prevent you from arriving at false conclusions that can damage your company in the long run. By using clean data, you will also help BI solutions to interact better with your information and create better reports for your organization.
6. Set your KPIs
Once you’ve set your sources, cleaned your data, and established clear-cut questions you want your insights to answer, you need to set a host of key performance indicators (KPIs) that will help you track, measure, and shape your progress in a number of key areas.
KPIs are critical to both qualitative and quantitative analysis research. This is one of the primary methods of data analysis you certainly shouldn’t overlook.
To help you set the best possible KPIs for your initiatives and activities, here is an example of a relevant logistics KPI : transportation-related costs. If you want to see more go explore our collection of key performance indicator examples .

7. Omit useless data
Having bestowed your data analysis tools and techniques with true purpose and defined your mission, you should explore the raw data you’ve collected from all sources and use your KPIs as a reference for chopping out any information you deem to be useless.
Trimming the informational fat is one of the most crucial methods of analysis as it will allow you to focus your analytical efforts and squeeze every drop of value from the remaining ‘lean’ information.
Any stats, facts, figures, or metrics that don’t align with your business goals or fit with your KPI management strategies should be eliminated from the equation.
8. Build a data management roadmap
While, at this point, this particular step is optional (you will have already gained a wealth of insight and formed a fairly sound strategy by now), creating a data governance roadmap will help your data analysis methods and techniques become successful on a more sustainable basis. These roadmaps, if developed properly, are also built so they can be tweaked and scaled over time.
Invest ample time in developing a roadmap that will help you store, manage, and handle your data internally, and you will make your analysis techniques all the more fluid and functional – one of the most powerful types of data analysis methods available today.
9. Integrate technology
There are many ways to analyze data, but one of the most vital aspects of analytical success in a business context is integrating the right decision support software and technology.
Robust analysis platforms will not only allow you to pull critical data from your most valuable sources while working with dynamic KPIs that will offer you actionable insights; it will also present them in a digestible, visual, interactive format from one central, live dashboard . A data methodology you can count on.
By integrating the right technology within your data analysis methodology, you’ll avoid fragmenting your insights, saving you time and effort while allowing you to enjoy the maximum value from your business’s most valuable insights.
For a look at the power of software for the purpose of analysis and to enhance your methods of analyzing, glance over our selection of dashboard examples .
10. Answer your questions
By considering each of the above efforts, working with the right technology, and fostering a cohesive internal culture where everyone buys into the different ways to analyze data as well as the power of digital intelligence, you will swiftly start to answer your most burning business questions. Arguably, the best way to make your data concepts accessible across the organization is through data visualization.
11. Visualize your data
Online data visualization is a powerful tool as it lets you tell a story with your metrics, allowing users across the organization to extract meaningful insights that aid business evolution – and it covers all the different ways to analyze data.
The purpose of analyzing is to make your entire organization more informed and intelligent, and with the right platform or dashboard, this is simpler than you think, as demonstrated by our marketing dashboard .

This visual, dynamic, and interactive online dashboard is a data analysis example designed to give Chief Marketing Officers (CMO) an overview of relevant metrics to help them understand if they achieved their monthly goals.
In detail, this example generated with a modern dashboard creator displays interactive charts for monthly revenues, costs, net income, and net income per customer; all of them are compared with the previous month so that you can understand how the data fluctuated. In addition, it shows a detailed summary of the number of users, customers, SQLs, and MQLs per month to visualize the whole picture and extract relevant insights or trends for your marketing reports .
The CMO dashboard is perfect for c-level management as it can help them monitor the strategic outcome of their marketing efforts and make data-driven decisions that can benefit the company exponentially.
12. Be careful with the interpretation
We already dedicated an entire post to data interpretation as it is a fundamental part of the process of data analysis. It gives meaning to the analytical information and aims to drive a concise conclusion from the analysis results. Since most of the time companies are dealing with data from many different sources, the interpretation stage needs to be done carefully and properly in order to avoid misinterpretations.
To help you through the process, here we list three common practices that you need to avoid at all costs when looking at your data:
- Correlation vs. causation: The human brain is formatted to find patterns. This behavior leads to one of the most common mistakes when performing interpretation: confusing correlation with causation. Although these two aspects can exist simultaneously, it is not correct to assume that because two things happened together, one provoked the other. A piece of advice to avoid falling into this mistake is never to trust just intuition, trust the data. If there is no objective evidence of causation, then always stick to correlation.
- Confirmation bias: This phenomenon describes the tendency to select and interpret only the data necessary to prove one hypothesis, often ignoring the elements that might disprove it. Even if it's not done on purpose, confirmation bias can represent a real problem, as excluding relevant information can lead to false conclusions and, therefore, bad business decisions. To avoid it, always try to disprove your hypothesis instead of proving it, share your analysis with other team members, and avoid drawing any conclusions before the entire analytical project is finalized.
- Statistical significance: To put it in short words, statistical significance helps analysts understand if a result is actually accurate or if it happened because of a sampling error or pure chance. The level of statistical significance needed might depend on the sample size and the industry being analyzed. In any case, ignoring the significance of a result when it might influence decision-making can be a huge mistake.
13. Build a narrative
Now, we’re going to look at how you can bring all of these elements together in a way that will benefit your business - starting with a little something called data storytelling.
The human brain responds incredibly well to strong stories or narratives. Once you’ve cleansed, shaped, and visualized your most invaluable data using various BI dashboard tools , you should strive to tell a story - one with a clear-cut beginning, middle, and end.
By doing so, you will make your analytical efforts more accessible, digestible, and universal, empowering more people within your organization to use your discoveries to their actionable advantage.
14. Consider autonomous technology
Autonomous technologies, such as artificial intelligence (AI) and machine learning (ML), play a significant role in the advancement of understanding how to analyze data more effectively.
Gartner predicts that by the end of this year, 80% of emerging technologies will be developed with AI foundations. This is a testament to the ever-growing power and value of autonomous technologies.
At the moment, these technologies are revolutionizing the analysis industry. Some examples that we mentioned earlier are neural networks, intelligent alarms, and sentiment analysis.
15. Share the load
If you work with the right tools and dashboards, you will be able to present your metrics in a digestible, value-driven format, allowing almost everyone in the organization to connect with and use relevant data to their advantage.
Modern dashboards consolidate data from various sources, providing access to a wealth of insights in one centralized location, no matter if you need to monitor recruitment metrics or generate reports that need to be sent across numerous departments. Moreover, these cutting-edge tools offer access to dashboards from a multitude of devices, meaning that everyone within the business can connect with practical insights remotely - and share the load.
Once everyone is able to work with a data-driven mindset, you will catalyze the success of your business in ways you never thought possible. And when it comes to knowing how to analyze data, this kind of collaborative approach is essential.
16. Data analysis tools
In order to perform high-quality analysis of data, it is fundamental to use tools and software that will ensure the best results. Here we leave you a small summary of four fundamental categories of data analysis tools for your organization.
- Business Intelligence: BI tools allow you to process significant amounts of data from several sources in any format. Through this, you can not only analyze and monitor your data to extract relevant insights but also create interactive reports and dashboards to visualize your KPIs and use them for your company's good. datapine is an amazing online BI software that is focused on delivering powerful online analysis features that are accessible to beginner and advanced users. Like this, it offers a full-service solution that includes cutting-edge analysis of data, KPIs visualization, live dashboards, reporting, and artificial intelligence technologies to predict trends and minimize risk.
- Statistical analysis: These tools are usually designed for scientists, statisticians, market researchers, and mathematicians, as they allow them to perform complex statistical analyses with methods like regression analysis, predictive analysis, and statistical modeling. A good tool to perform this type of analysis is R-Studio as it offers a powerful data modeling and hypothesis testing feature that can cover both academic and general data analysis. This tool is one of the favorite ones in the industry, due to its capability for data cleaning, data reduction, and performing advanced analysis with several statistical methods. Another relevant tool to mention is SPSS from IBM. The software offers advanced statistical analysis for users of all skill levels. Thanks to a vast library of machine learning algorithms, text analysis, and a hypothesis testing approach it can help your company find relevant insights to drive better decisions. SPSS also works as a cloud service that enables you to run it anywhere.
- SQL Consoles: SQL is a programming language often used to handle structured data in relational databases. Tools like these are popular among data scientists as they are extremely effective in unlocking these databases' value. Undoubtedly, one of the most used SQL software in the market is MySQL Workbench . This tool offers several features such as a visual tool for database modeling and monitoring, complete SQL optimization, administration tools, and visual performance dashboards to keep track of KPIs.
- Data Visualization: These tools are used to represent your data through charts, graphs, and maps that allow you to find patterns and trends in the data. datapine's already mentioned BI platform also offers a wealth of powerful online data visualization tools with several benefits. Some of them include: delivering compelling data-driven presentations to share with your entire company, the ability to see your data online with any device wherever you are, an interactive dashboard design feature that enables you to showcase your results in an interactive and understandable way, and to perform online self-service reports that can be used simultaneously with several other people to enhance team productivity.
17. Refine your process constantly
Last is a step that might seem obvious to some people, but it can be easily ignored if you think you are done. Once you have extracted the needed results, you should always take a retrospective look at your project and think about what you can improve. As you saw throughout this long list of techniques, data analysis is a complex process that requires constant refinement. For this reason, you should always go one step further and keep improving.
Quality Criteria For Data Analysis
So far we’ve covered a list of methods and techniques that should help you perform efficient data analysis. But how do you measure the quality and validity of your results? This is done with the help of some science quality criteria. Here we will go into a more theoretical area that is critical to understanding the fundamentals of statistical analysis in science. However, you should also be aware of these steps in a business context, as they will allow you to assess the quality of your results in the correct way. Let’s dig in.
- Internal validity: The results of a survey are internally valid if they measure what they are supposed to measure and thus provide credible results. In other words , internal validity measures the trustworthiness of the results and how they can be affected by factors such as the research design, operational definitions, how the variables are measured, and more. For instance, imagine you are doing an interview to ask people if they brush their teeth two times a day. While most of them will answer yes, you can still notice that their answers correspond to what is socially acceptable, which is to brush your teeth at least twice a day. In this case, you can’t be 100% sure if respondents actually brush their teeth twice a day or if they just say that they do, therefore, the internal validity of this interview is very low.
- External validity: Essentially, external validity refers to the extent to which the results of your research can be applied to a broader context. It basically aims to prove that the findings of a study can be applied in the real world. If the research can be applied to other settings, individuals, and times, then the external validity is high.
- Reliability : If your research is reliable, it means that it can be reproduced. If your measurement were repeated under the same conditions, it would produce similar results. This means that your measuring instrument consistently produces reliable results. For example, imagine a doctor building a symptoms questionnaire to detect a specific disease in a patient. Then, various other doctors use this questionnaire but end up diagnosing the same patient with a different condition. This means the questionnaire is not reliable in detecting the initial disease. Another important note here is that in order for your research to be reliable, it also needs to be objective. If the results of a study are the same, independent of who assesses them or interprets them, the study can be considered reliable. Let’s see the objectivity criteria in more detail now.
- Objectivity: In data science, objectivity means that the researcher needs to stay fully objective when it comes to its analysis. The results of a study need to be affected by objective criteria and not by the beliefs, personality, or values of the researcher. Objectivity needs to be ensured when you are gathering the data, for example, when interviewing individuals, the questions need to be asked in a way that doesn't influence the results. Paired with this, objectivity also needs to be thought of when interpreting the data. If different researchers reach the same conclusions, then the study is objective. For this last point, you can set predefined criteria to interpret the results to ensure all researchers follow the same steps.
The discussed quality criteria cover mostly potential influences in a quantitative context. Analysis in qualitative research has by default additional subjective influences that must be controlled in a different way. Therefore, there are other quality criteria for this kind of research such as credibility, transferability, dependability, and confirmability. You can see each of them more in detail on this resource .
Data Analysis Limitations & Barriers
Analyzing data is not an easy task. As you’ve seen throughout this post, there are many steps and techniques that you need to apply in order to extract useful information from your research. While a well-performed analysis can bring various benefits to your organization it doesn't come without limitations. In this section, we will discuss some of the main barriers you might encounter when conducting an analysis. Let’s see them more in detail.
- Lack of clear goals: No matter how good your data or analysis might be if you don’t have clear goals or a hypothesis the process might be worthless. While we mentioned some methods that don’t require a predefined hypothesis, it is always better to enter the analytical process with some clear guidelines of what you are expecting to get out of it, especially in a business context in which data is utilized to support important strategic decisions.
- Objectivity: Arguably one of the biggest barriers when it comes to data analysis in research is to stay objective. When trying to prove a hypothesis, researchers might find themselves, intentionally or unintentionally, directing the results toward an outcome that they want. To avoid this, always question your assumptions and avoid confusing facts with opinions. You can also show your findings to a research partner or external person to confirm that your results are objective.
- Data representation: A fundamental part of the analytical procedure is the way you represent your data. You can use various graphs and charts to represent your findings, but not all of them will work for all purposes. Choosing the wrong visual can not only damage your analysis but can mislead your audience, therefore, it is important to understand when to use each type of data depending on your analytical goals. Our complete guide on the types of graphs and charts lists 20 different visuals with examples of when to use them.
- Flawed correlation : Misleading statistics can significantly damage your research. We’ve already pointed out a few interpretation issues previously in the post, but it is an important barrier that we can't avoid addressing here as well. Flawed correlations occur when two variables appear related to each other but they are not. Confusing correlations with causation can lead to a wrong interpretation of results which can lead to building wrong strategies and loss of resources, therefore, it is very important to identify the different interpretation mistakes and avoid them.
- Sample size: A very common barrier to a reliable and efficient analysis process is the sample size. In order for the results to be trustworthy, the sample size should be representative of what you are analyzing. For example, imagine you have a company of 1000 employees and you ask the question “do you like working here?” to 50 employees of which 49 say yes, which means 95%. Now, imagine you ask the same question to the 1000 employees and 950 say yes, which also means 95%. Saying that 95% of employees like working in the company when the sample size was only 50 is not a representative or trustworthy conclusion. The significance of the results is way more accurate when surveying a bigger sample size.
- Privacy concerns: In some cases, data collection can be subjected to privacy regulations. Businesses gather all kinds of information from their customers from purchasing behaviors to addresses and phone numbers. If this falls into the wrong hands due to a breach, it can affect the security and confidentiality of your clients. To avoid this issue, you need to collect only the data that is needed for your research and, if you are using sensitive facts, make it anonymous so customers are protected. The misuse of customer data can severely damage a business's reputation, so it is important to keep an eye on privacy.
- Lack of communication between teams : When it comes to performing data analysis on a business level, it is very likely that each department and team will have different goals and strategies. However, they are all working for the same common goal of helping the business run smoothly and keep growing. When teams are not connected and communicating with each other, it can directly affect the way general strategies are built. To avoid these issues, tools such as data dashboards enable teams to stay connected through data in a visually appealing way.
- Innumeracy : Businesses are working with data more and more every day. While there are many BI tools available to perform effective analysis, data literacy is still a constant barrier. Not all employees know how to apply analysis techniques or extract insights from them. To prevent this from happening, you can implement different training opportunities that will prepare every relevant user to deal with data.
Key Data Analysis Skills
As you've learned throughout this lengthy guide, analyzing data is a complex task that requires a lot of knowledge and skills. That said, thanks to the rise of self-service tools the process is way more accessible and agile than it once was. Regardless, there are still some key skills that are valuable to have when working with data, we list the most important ones below.
- Critical and statistical thinking: To successfully analyze data you need to be creative and think out of the box. Yes, that might sound like a weird statement considering that data is often tight to facts. However, a great level of critical thinking is required to uncover connections, come up with a valuable hypothesis, and extract conclusions that go a step further from the surface. This, of course, needs to be complemented by statistical thinking and an understanding of numbers.
- Data cleaning: Anyone who has ever worked with data before will tell you that the cleaning and preparation process accounts for 80% of a data analyst's work, therefore, the skill is fundamental. But not just that, not cleaning the data adequately can also significantly damage the analysis which can lead to poor decision-making in a business scenario. While there are multiple tools that automate the cleaning process and eliminate the possibility of human error, it is still a valuable skill to dominate.
- Data visualization: Visuals make the information easier to understand and analyze, not only for professional users but especially for non-technical ones. Having the necessary skills to not only choose the right chart type but know when to apply it correctly is key. This also means being able to design visually compelling charts that make the data exploration process more efficient.
- SQL: The Structured Query Language or SQL is a programming language used to communicate with databases. It is fundamental knowledge as it enables you to update, manipulate, and organize data from relational databases which are the most common databases used by companies. It is fairly easy to learn and one of the most valuable skills when it comes to data analysis.
- Communication skills: This is a skill that is especially valuable in a business environment. Being able to clearly communicate analytical outcomes to colleagues is incredibly important, especially when the information you are trying to convey is complex for non-technical people. This applies to in-person communication as well as written format, for example, when generating a dashboard or report. While this might be considered a “soft” skill compared to the other ones we mentioned, it should not be ignored as you most likely will need to share analytical findings with others no matter the context.
Data Analysis In The Big Data Environment
Big data is invaluable to today’s businesses, and by using different methods for data analysis, it’s possible to view your data in a way that can help you turn insight into positive action.
To inspire your efforts and put the importance of big data into context, here are some insights that you should know:
- By 2026 the industry of big data is expected to be worth approximately $273.4 billion.
- 94% of enterprises say that analyzing data is important for their growth and digital transformation.
- Companies that exploit the full potential of their data can increase their operating margins by 60% .
- We already told you the benefits of Artificial Intelligence through this article. This industry's financial impact is expected to grow up to $40 billion by 2025.
Data analysis concepts may come in many forms, but fundamentally, any solid methodology will help to make your business more streamlined, cohesive, insightful, and successful than ever before.
Key Takeaways From Data Analysis
As we reach the end of our data analysis journey, we leave a small summary of the main methods and techniques to perform excellent analysis and grow your business.
17 Essential Types of Data Analysis Methods:
- Cluster analysis
- Cohort analysis
- Regression analysis
- Factor analysis
- Neural Networks
- Data Mining
- Text analysis
- Time series analysis
- Decision trees
- Conjoint analysis
- Correspondence Analysis
- Multidimensional Scaling
- Content analysis
- Thematic analysis
- Narrative analysis
- Grounded theory analysis
- Discourse analysis
Top 17 Data Analysis Techniques:
- Collaborate your needs
- Establish your questions
- Data democratization
- Think of data governance
- Clean your data
- Set your KPIs
- Omit useless data
- Build a data management roadmap
- Integrate technology
- Answer your questions
- Visualize your data
- Interpretation of data
- Consider autonomous technology
- Build a narrative
- Share the load
- Data Analysis tools
- Refine your process constantly
We’ve pondered the data analysis definition and drilled down into the practical applications of data-centric analytics, and one thing is clear: by taking measures to arrange your data and making your metrics work for you, it’s possible to transform raw information into action - the kind of that will push your business to the next level.
Yes, good data analytics techniques result in enhanced business intelligence (BI). To help you understand this notion in more detail, read our exploration of business intelligence reporting .
And, if you’re ready to perform your own analysis, drill down into your facts and figures while interacting with your data on astonishing visuals, you can try our software for a free, 14-day trial .
- Skip to main content
- Skip to primary sidebar
- Skip to footer
- QuestionPro

- Solutions Industries Gaming Automotive Sports and events Education Government Travel & Hospitality Financial Services Healthcare Cannabis Technology Use Case NPS+ Communities Audience Contactless surveys Mobile LivePolls Member Experience GDPR Positive People Science 360 Feedback Surveys
- Resources Blog eBooks Survey Templates Case Studies Training Help center

Home Market Research Research Tools and Apps
Audience Analysis: Definition with types and uses
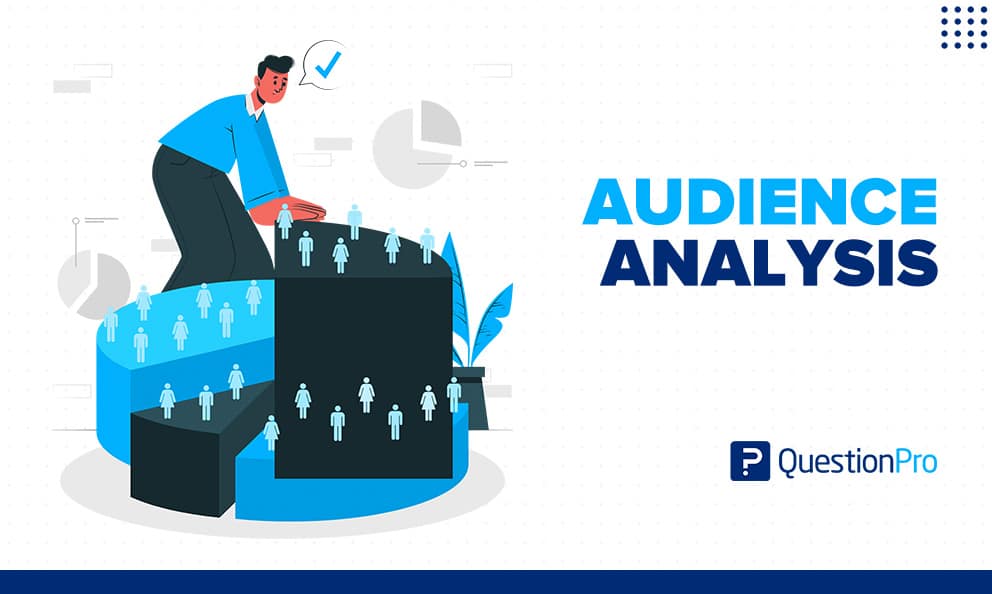
It can be hard to figure out who your audience is and what they want, both in business and beyond. You can use audience analysis to learn more about your audience and how to serve them better.
To reach your full potential, you must improve your analysis of the audience skills. In the age of online, it is easier than ever to capture audience data. If you perform this analysis, you can figure out your effective audience and reach your maximum potential while keeping them in mind. In this article, you will learn about the analysis of the audience, its types, and its uses.
What is audience analysis?
Audience analysis is the method of obtaining information about the people in your audience to better understand their wants, needs, values, and attitudes.
It would be best if you first defined your target audience by determining their demographics, such as their gender, age, and where they live. After you’ve defined the potential audiences, you’ll be able to narrow the list down to a single target group for in-depth analysis .
Intended analysis of the audience has always been key to a successful business. Because of advanced technology, this process is perhaps simpler than ever before. Companies now have easy access to a wide range of audience information via social media channels.
If you can turn all that data into clear insights about your audience and what they care about, you can design a better business strategy.
Types of audience analysis
There are three types of audience analysis:
Demographic analysis
Psychographic analysis.
- Situational analysis.
Let’s look at these types!
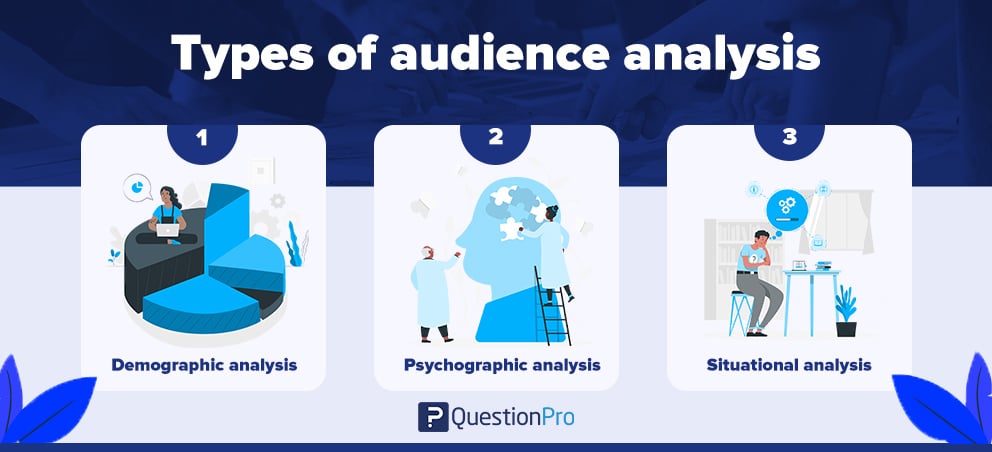
Demographic data include things like gender, age range, marital status, race and ethnicity, and socioeconomic status. You probably already know how many male and female students are in your public speaking class, how old they are roughly, and so on.
But how can you figure out who will be in an audience if you have never talked to them? Most of the time, you can ask the person or group that asked you to speak. They can probably tell you a lot about the types of people who are expected to come to hear you.
No matter what method you use to collect demographic information, be respectful from the start. For example, if you want to know if people in the audience have ever been divorced, you should know that not everyone will want to answer your questions. You can’t demand that they do it. And you can’t just guess why they don’t want to talk about it. You have to give them some space.
- Age is not just a number, as it can tell a lot about a person. For example, teens and people in their 20s have no memory of wars or living under a totalitarian regime.
- Even in the same culture, women and men have different experiences based on their gender. For instance, women are often kept out of jobs that are thought to be better suited for men.
- Religions are also very different: Christianity, Judaism, Buddhism, Islam, Hinduism, and many more. Even within Christianity, there are Roman Catholic, Mormon, Jehovah’s Witnesses, Orthodox (Greek and Russian), and many Protestant denominations.
- In the demographic analysis , the level and type of education are also essential factors. An airplane mechanic gets a very different education and training than an accountant, musician, or software engineer. Fraternities and sororities, sports teams, campus organizations, political parties, volunteer work, and cultural communities all shape how we see the world.
- Lastly, their jobs affect people’s ideas and interests because most appointments are misunderstood in many ways. For example, many think teachers work eight hours a day and get the summer off.
By doing demographic data analysis, you can find out a lot about your customers, as you can see. The best part is that many tools make it easy to do this analysis. For example, if you run ads on Facebook or Google, these platforms already have a way for you to study your audience that you can use.
Demographic information is pretty clear and easy to check, but psychographic information is not that easy to understand. A psychographic analysis looks at things like values, beliefs, opinions, and attitudes. Even though two people say they don’t buy junk food, they might have very different ideas about what foods are “junk food.”
Audiences will likely already know two main things: what you’re talking about and your brand. Psychographic segmentation can show how your audience is limited by the ideas they already have. If you know about these ideas ahead of time, you can write your message in a way that fits them.
- There may be misconceptions or stereotypes about your topic that people already know. When you do your audience analysis, include questions that show how much your audience already knows about your case. Try to find out if they have any stereotypes, oversimplified ideas, or biased beliefs about it. This could change the message you want to send and how you want to send it.
- On the other hand, pre-existing ideas about your brand are more about how customers see you at first. But it would be best if you focused on meeting the needs and interests of your audience, not on changing their minds about you or managing your image.
Google Trends is one way to do a psychographic analysis. Google Trends can be used to find the latest trends, but it can also be used to track marketing performance.
LEARN ABOUT: Level of Analysis
Situational analysis
In the realm of more traditional forms of speech, situational aspects of an audience include the audience’s size, attitude toward the speaker, prior knowledge of the topic, and occasion.
In the world of digital marketing and media, this refers to the size of your preferred audience, their attitude towards your product/company, their prior knowledge, and the websites or social media channels. They view your advertisements or interact with your products and services by this.
It is more challenging to collect this information when your audience is geographically dispersed because you are not addressing the audience directly at this time. But, similar to a world leader delivering a speech, it is essential to learn how to engage potential customers online, especially in a world of limitless options and audience information.
Uses of audience analysis in your business
Any successful marketing campaign must analyze audience data. Even creating your target audience teaches you about yourself and your smart goals . By understanding your target audiences, you can adapt your messaging to meet their needs and analyze a product’s flexibility in different contexts. Below are two uses of audience analysis:
Customer behavior
By doing audience analysis, you’ll learn how your customers act offline and online. With audience data from social media, you can see what other products and personalities people like. That will helps you figure out what they want.
For example, instead of just figuring out who is most likely to go to a spa, analysis tells you what kinds of treatments people are most likely to want based on the stressors in their lives. Once you have this information, you’ll be able to predict better what services to promote to which groups. This will help you be more successful in the long run by speaking to each person’s needs instead of painting with broad strokes.
Taking risks
There is always a time when a company or small business must decide to grow. Taking risks is a critical component of this growth. But you don’t want to take uninformed risks. With the right audience analysis questions, you can determine what risks are likely to pay off.
For example, if your audience data shows that most of your customers are younger, making changes to fit the needs of that group (like creating a product that is cheaper, more eco-friendly, etc.) helps ensure growth. On the other hand, if the people you want to reach are less tech-savvy, an interesting newsletter sent through email might work better than a social media campaign.
LEARN ABOUT: Social Communication Questionnaire
In our global, online society, your audience may be spread out around the world. This connected world has lots of audience data. It can be hard to determine customer beliefs and preferences without immediate crowd feedback.
Using social media sites, audience profiles, and understanding where your purchases come from can help with audience analysis. Knowing your audience enables you to make smart choices and save money.
In the end, we had a clear idea of what data analysis is, what types there are, and its uses. Now it’s time to make changes in your organization to match. For your good, we suggest you use QuestionPro, which is much more than survey software. We have a solution for every specific problem and industry.
We also offer data management platforms, like our Insights Hub research repository.
LEARN MORE FREE TRIAL
MORE LIKE THIS
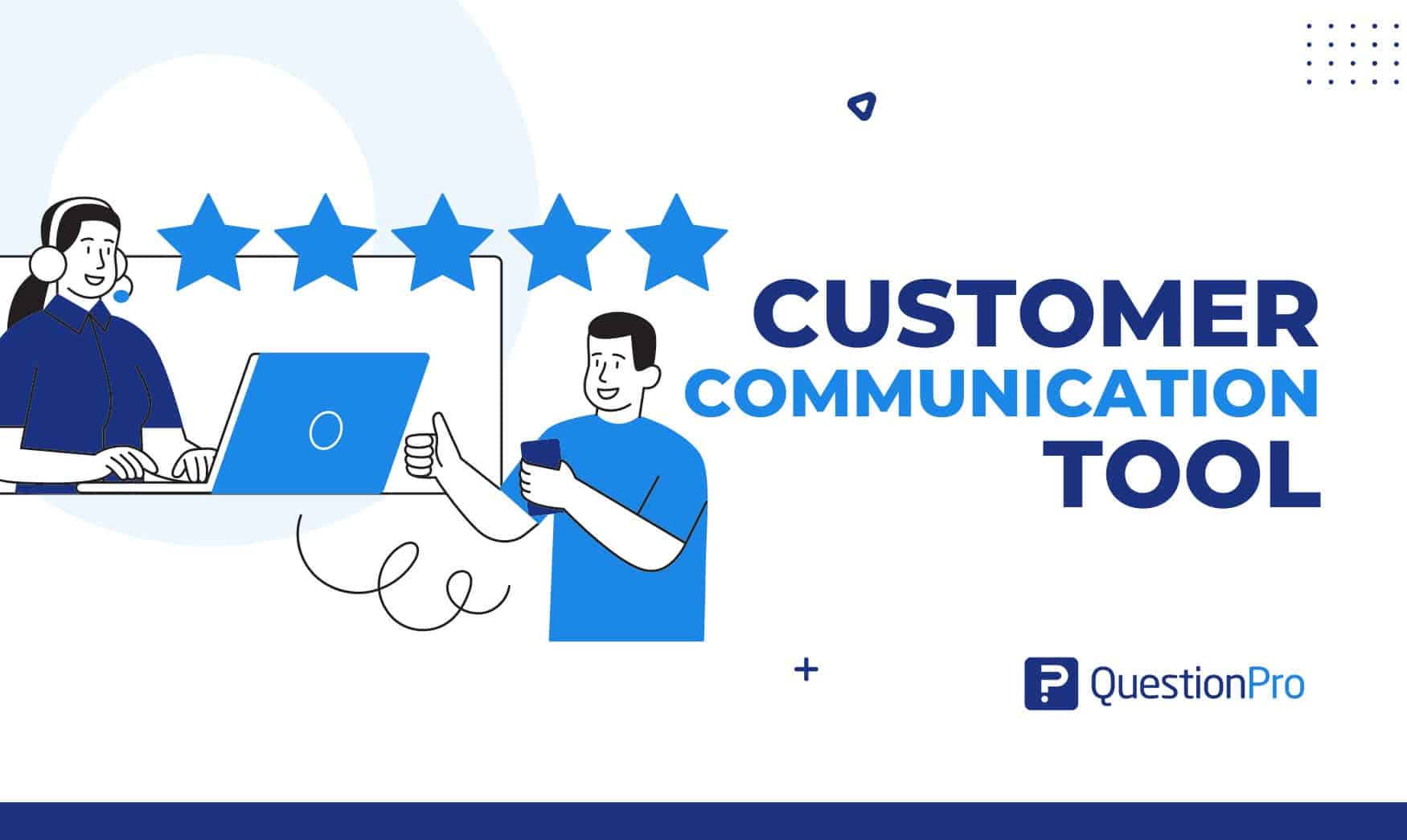
Customer Communication Tool: Types, Methods, Uses, & Tools
Apr 23, 2024
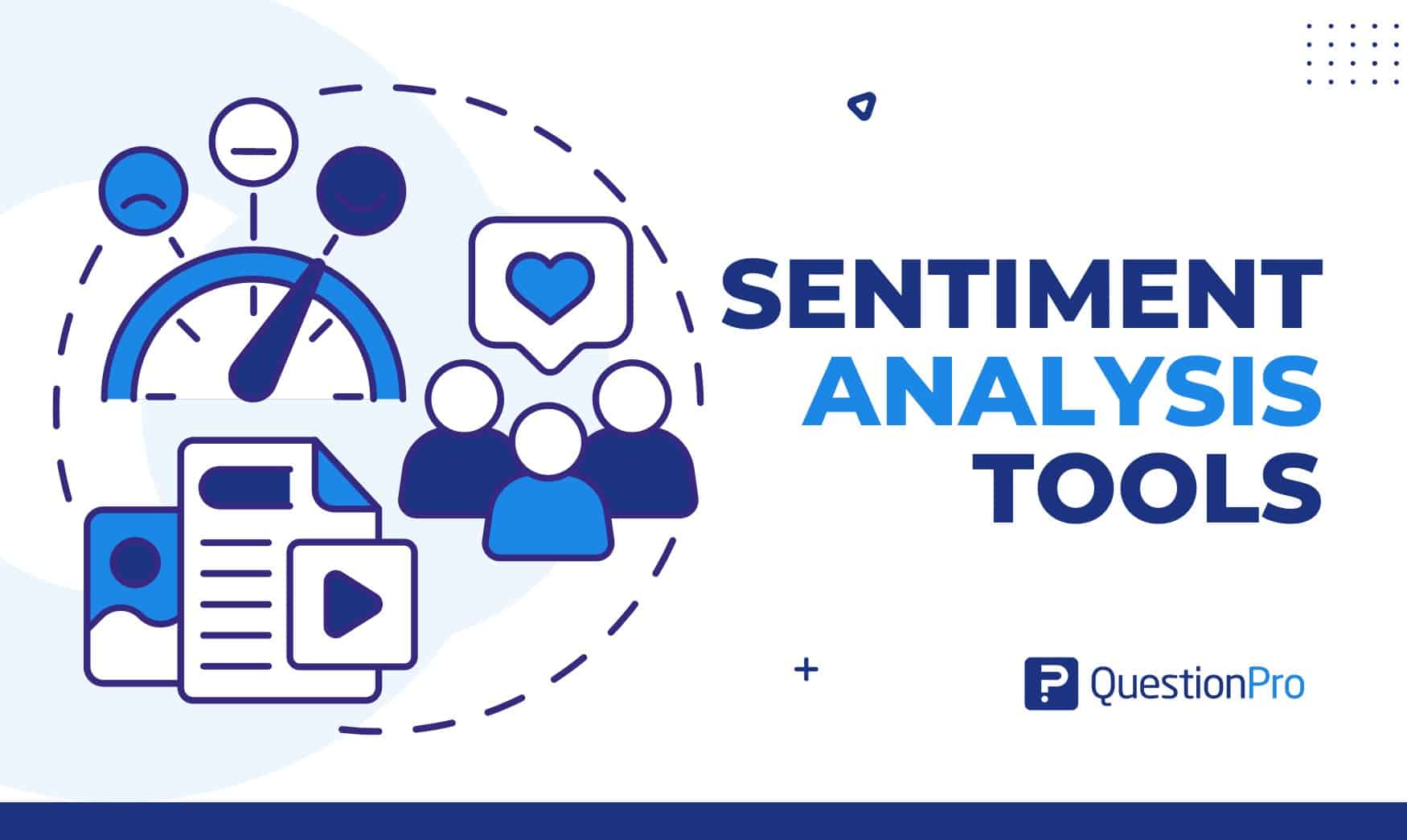
Top 12 Sentiment Analysis Tools for Understanding Emotions
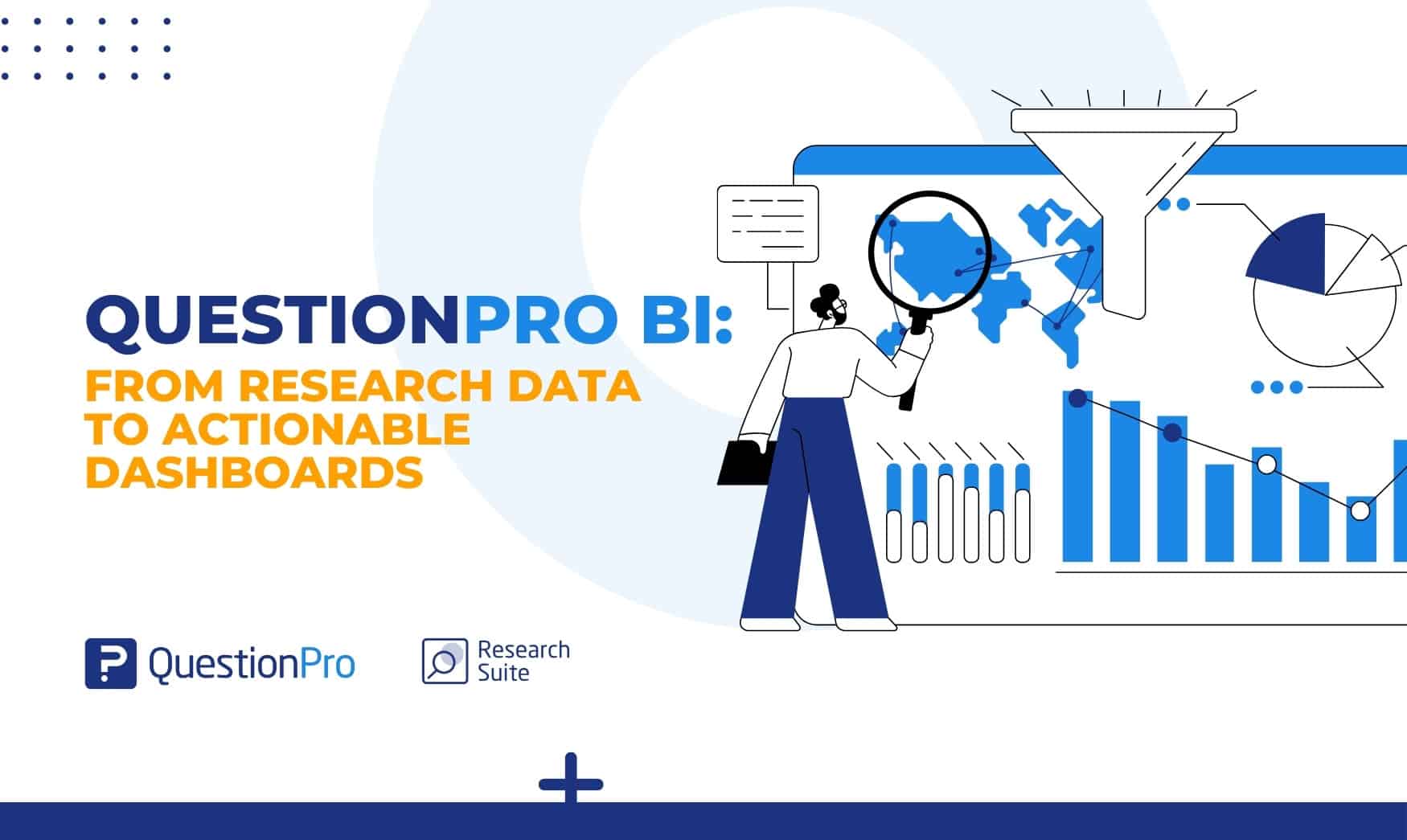
QuestionPro BI: From research data to actionable dashboards within minutes
Apr 22, 2024
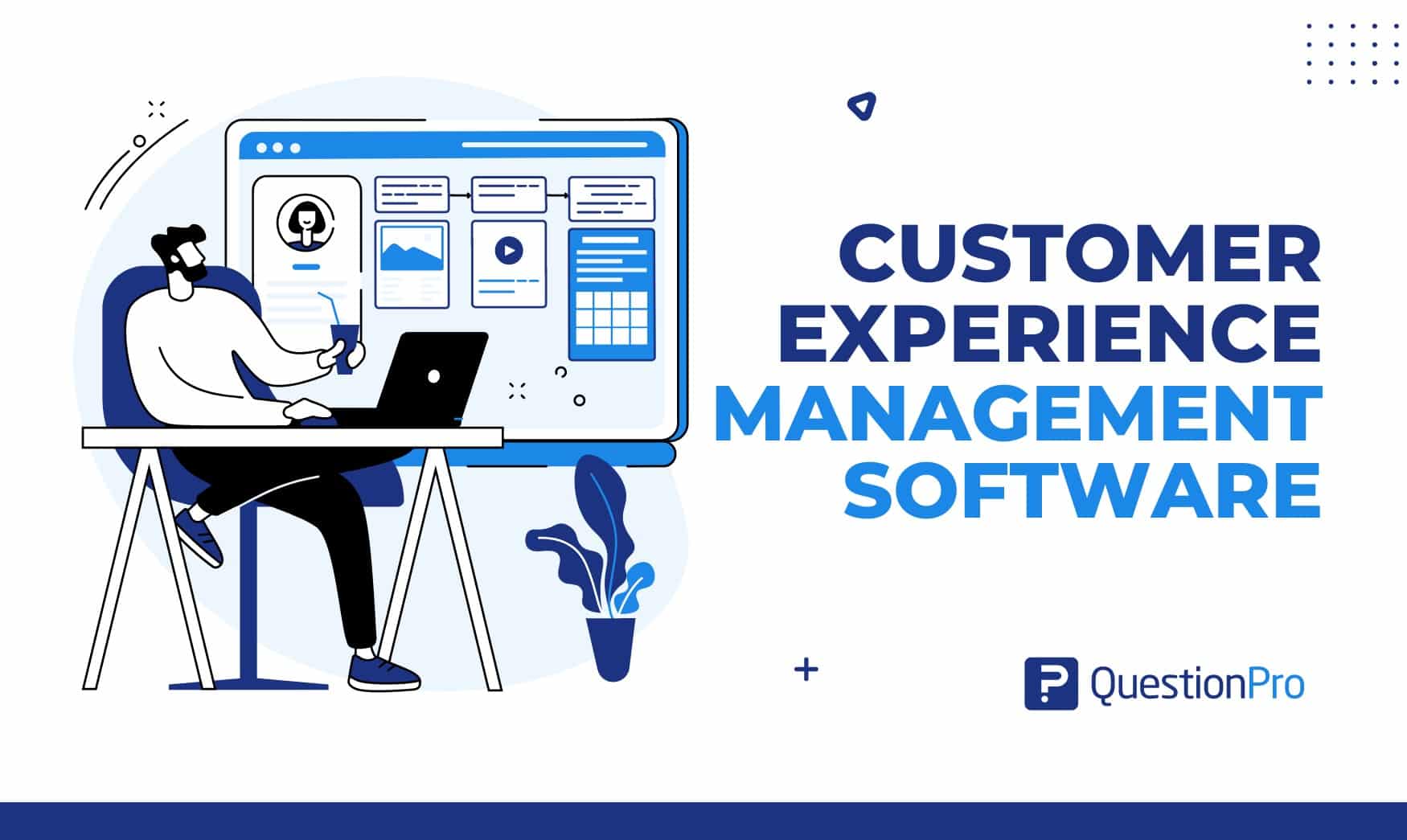
21 Best Customer Experience Management Software in 2024
Other categories.
- Academic Research
- Artificial Intelligence
- Assessments
- Brand Awareness
- Case Studies
- Communities
- Consumer Insights
- Customer effort score
- Customer Engagement
- Customer Experience
- Customer Loyalty
- Customer Research
- Customer Satisfaction
- Employee Benefits
- Employee Engagement
- Employee Retention
- Friday Five
- General Data Protection Regulation
- Insights Hub
- Life@QuestionPro
- Market Research
- Mobile diaries
- Mobile Surveys
- New Features
- Online Communities
- Question Types
- Questionnaire
- QuestionPro Products
- Release Notes
- Research Tools and Apps
- Revenue at Risk
- Survey Templates
- Training Tips
- Uncategorized
- Video Learning Series
- What’s Coming Up
- Workforce Intelligence
data analysis, interpretation, and presentation
Depends on data.
quantitative
qualitative
mixed methods
translating raw data
questionnaires

observations
begins with initial reactions or observations
identify patterns
calculating values
data cleansing: check for errors
use structured frameworks and theories
interpretation
parallel with analysis
results interpreted different ways
make sure data supports conclusion
avoid biases
avoid over claiming
presentation
different methods, depends on goals
affects interpretation

quantitative analysis
Statistical analysis.
percentages
individual differences
task times: 45, 50, 55, 55, 60, 65
task times: 45, 50, 55, 55, 60, 300
task times: 10, 10, 50, 55, 60, 300
median: 52.5
data format
table: rows and columns
how to represent responses and measures
participants as rows
responses: single or multiple
spreadsheet applications
analysis tools: R, SPSS, SAS

scatter plots
presenting percentages
almost always better ways
comparison difficult when percentages similar
include percentages as text
provide overview with other representations
qualitative analysis
Initial approach.
gain overall understanding
look for interesting features
highlight common, record surprises
inductive vs deductive
inductive: extract concepts from the data
deductive: use existing theory to categorize
depends on data and goals
inductive, then deductive
reliable analysis
replication
training towards consensus
inter-rater reliability or agreement
Cohen's kappa
transcription: oTranscribe , Otter.ai , Trint
coding and analysis: Dedoose, Atlas.ai, Nvivo
identifying themes
Categorizing data.
analyzing critical incidents
thematic analysis
identify, analyze, and report patterns
themes represent important, relevant, or unexpected patterns
open coding: initial pass
axial coding: themes across participants, connections, categories
selective coding: validate relationships, consistency, themes
find further themes
step back, look at big picture
affinity diagrams
organize ideas and insights into hierarchy
groupings emerge through data
analysis frame chosen beforehand
study goals
categorization schemes
evolve with analysis
- Verbalizations show evidence of dissatisfaction about an aspect of the interface.
- Verbalizations show evidence of confusion/uncertainty about an aspect of the interface.
- Verbalizations show evidence of dissatisfaction about aspects of the content of the electronic text.
critical incident analysis
help deal with lots of data
identify significant subsets
critical events
focus on important or unique events
apply in both data gathering and analysis
useful when cause of problem unknown
disadvantages
may miss routine incidents
not as good for general task analysis
interpreting qualitative data
Analytic frameworks, conversation analysis, discourse analysis, content analysis, interaction analysis, grounded theory.
semantics of conversation in detail
focus on how conversation conducted
compare conversations across different media
voice-assisted technologies
voice assistants
conversational interactions
how do these devices change social behaviors? in home? at work?
do these devices change the way we talk?
interaction interwoven with other activities Martin Porcheron et al. [2018]
interleaving conversations with people and devices
instructing rather than conversing
communication tools
zoom, discord
mediate much of our communication
do these tools change the way we talk to each other?
the ways we collaborate?
meaning of what is said and how words are used
context important
strongly interpretive
no objective scientific truth
how people use language to construct perspectives

identify subtle and implicit meaning
communication: e-mails, social media, interviews
scraping data
time consuming
analysis via statistics and visualizations
tag / word clouds

classifying data into themes
studying frequency of theme occurrence
used for a range of media
used in conjunction with other approaches
interactions of humans with each other and objects
verbal and non-verbal
data: video recordings
knowledge and action are social
goal: derive generalizations of activities based on actions
interaction analysis: first step
teams suggest general patterns from multiple observations
collaborative
first: create content logs summarizing happenings
categories emerge through repeated play and discussion
intentions, motivations, and understandings
cannibalizing videos
extracting interesting material
reclassifying in term of material
instances assembled in playlist
derive theory grounded in data
from sociology
theory: set of concepts that constitute a framework for explaining or predicting phenomena
grounded theory: method
alternating data collection and analysis
identify themes, then refine with more data
continues until saturation, no new emergent themes
balance objectivity and sensitivity
goal: define properties and dimensions of relevant themes (categories)
grounded theory: data gathering
focus on analysis
interviews and observations
written records and diagrammatic representations
physical code books
grounded theory: coding
open coding: categories, properties, and dimensions discovered
axial coding: flesh out categories and relate to subcategories
selecting coding: refining and integrating categories
grounded theory: analytic tools
question the data: different perspectives, escape ruts
analysis of a word, phrase, or sentence: different perspectives
comparison: between objects or categories → alternative interpretations
interpreting and presenting findings
Presenting findings.
tables of numbers and text
graphical devices, such as charts and diagrams
set of themes or categories
choice depends on the data and analytic method
details of data collection and analysis
set of complementary representations
structured notations
analyze, capture, and present information
clear syntax and semantics
structured notation: advantages
meaning of symbols well-defined
highlights what to look for
enforces precision in expression
structured notation: disadvantages
by highlighting, ignores other aspects
precise expression lost on an unfamiliar audience
combine with other more accessible formats
storytelling
easy and intuitive approach
stories told by participants during data gathering
stories based on observation
stories constructed from snippets or repeated episodes in data
scenarios derived from stories collected during data gathering

summarizing findings
combination of presentation formats
careful interpretation and presentation important
not specific to interaction design
common pitfall: over-generalizing results
reading for next class
Chapter 12: Design, Prototyping, and Construction Interaction Design: Beyond Human-Computer Interaction

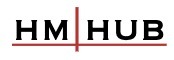
Data Analysis, Interpretation, and Presentation Techniques: A Guide to Making Sense of Your Research Data
by Prince Kumar
Last updated: 27 February 2023
Table of Contents
Data analysis, interpretation, and presentation are crucial aspects of conducting high-quality research. Data analysis involves processing and analyzing the data to derive meaningful insights, while data interpretation involves making sense of the insights and drawing conclusions. Data presentation involves presenting the data in a clear and concise way to communicate the research findings. In this article, we will discuss the techniques for data analysis, interpretation, and presentation.
1. Data Analysis Techniques
Data analysis techniques involve processing and analyzing the data to derive meaningful insights. The choice of data analysis technique depends on the research question and objectives. Some common data analysis techniques are:
a. Descriptive Statistics
Descriptive statistics involves summarizing and describing the data using measures such as mean, median, and standard deviation.
b. Inferential Statistics
Inferential statistics involves making inferences about the population based on the sample data. This technique involves hypothesis testing, confidence intervals, and regression analysis.
c. Content Analysis
Content analysis involves analyzing the text, images, or videos to identify patterns and themes.
d. Data Mining
Data mining involves using statistical and machine learning techniques to analyze large datasets and identify patterns.
2. Data Interpretation Techniques
Data interpretation involves making sense of the insights derived from the data analysis. The choice of data interpretation technique depends on the research question and objectives. Some common data interpretation techniques are:
a. Data Visualization
Data visualization involves presenting the data in a visual format, such as charts, graphs, or tables, to communicate the insights effectively.
b. Storytelling
Storytelling involves presenting the data in a narrative format, such as a story, to make the insights more relatable and memorable.
c. Comparative Analysis
Comparative analysis involves comparing the research findings with the existing literature or benchmarks to draw conclusions.
3. Data Presentation Techniques
Data presentation involves presenting the data in a clear and concise way to communicate the research findings. The choice of data presentation technique depends on the research question and objectives. Some common data presentation techniques are:
a. Tables and Graphs
Tables and graphs are effective data presentation techniques for presenting numerical data.
b. Infographics
Infographics are effective data presentation techniques for presenting complex data in a visual and easy-to-understand format.
c. Data Storytelling
Data storytelling involves presenting the data in a narrative format to communicate the research findings effectively.
In conclusion, data analysis, interpretation, and presentation are crucial aspects of conducting high-quality research. By using the appropriate data analysis, interpretation, and presentation techniques, researchers can derive meaningful insights, make sense of the insights, and communicate the research findings effectively. By conducting high-quality data analysis, interpretation, and presentation in research, researchers can provide valuable insights into the research question and objectives.
How useful was this post?
5 star mean very useful & 1 star means not useful at all.
Average rating / 5. Vote count:
No votes so far! Be the first to rate this post.
We are sorry that this post was not useful for you! 😔
Let us improve this post!
Tell us how we can improve this post?
Syllabus – Research Methodology
01 Introduction To Research Methodology
- Meaning and objectives of Research
- Types of Research
- Research Approaches
- Significance of Research
- Research methods vs Methodology
- Research Process
- Criteria of Good Research
- Problems faced by Researchers
- Techniques Involved in defining a problem
02 Research Design
- Meaning and Need for Research Design
- Features and important concepts relating to research design
- Different Research design
- Important Experimental Designs
03 Sample Design
- Introduction to Sample design
- Censure and sample survey
- Implications of Sample design
- Steps in sampling design
- Criteria for selecting a sampling procedure
- Characteristics of a good sample design
- Different types of Sample design
- Measurement Scales
- Important scaling Techniques
04 Methods of Data Collection
- Introduction
- Collection of Primary Data
- Collection through Questionnaire and schedule collection of secondary data
- Differences in Questionnaire and schedule
- Different methods to collect secondary data
05 Data Analysis Interpretation and Presentation Techniques
- Hypothesis Testing
- Basic concepts concerning Hypothesis Testing
- Procedure and flow diagram for Hypothesis Testing
- Test of Significance
- Chi-Square Analysis
- Report Presentation Techniques

An official website of the United States government
Here's how you know
The .gov means it's official. Federal government websites often end in .gov or .mil. Before sharing sensitive information, make sure you’re on a federal government site.
The site is secure. The https:// ensures that you are connecting to the official website and that any information you provide is encrypted and transmitted securely.
What the New Overtime Rule Means for Workers
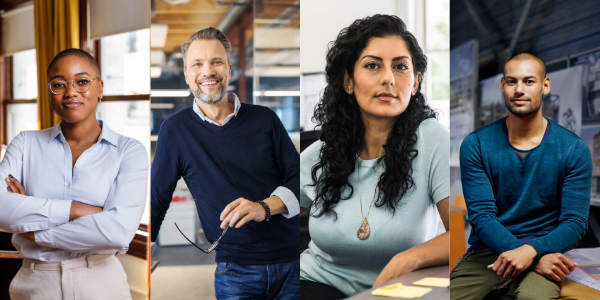
One of the basic principles of the American workplace is that a hard day’s work deserves a fair day’s pay. Simply put, every worker’s time has value. A cornerstone of that promise is the Fair Labor Standards Act ’s (FLSA) requirement that when most workers work more than 40 hours in a week, they get paid more. The Department of Labor ’s new overtime regulation is restoring and extending this promise for millions more lower-paid salaried workers in the U.S.
Overtime protections have been a critical part of the FLSA since 1938 and were established to protect workers from exploitation and to benefit workers, their families and our communities. Strong overtime protections help build America’s middle class and ensure that workers are not overworked and underpaid.
Some workers are specifically exempt from the FLSA’s minimum wage and overtime protections, including bona fide executive, administrative or professional employees. This exemption, typically referred to as the “EAP” exemption, applies when:
1. An employee is paid a salary,
2. The salary is not less than a minimum salary threshold amount, and
3. The employee primarily performs executive, administrative or professional duties.
While the department increased the minimum salary required for the EAP exemption from overtime pay every 5 to 9 years between 1938 and 1975, long periods between increases to the salary requirement after 1975 have caused an erosion of the real value of the salary threshold, lessening its effectiveness in helping to identify exempt EAP employees.
The department’s new overtime rule was developed based on almost 30 listening sessions across the country and the final rule was issued after reviewing over 33,000 written comments. We heard from a wide variety of members of the public who shared valuable insights to help us develop this Administration’s overtime rule, including from workers who told us: “I would love the opportunity to...be compensated for time worked beyond 40 hours, or alternately be given a raise,” and “I make around $40,000 a year and most week[s] work well over 40 hours (likely in the 45-50 range). This rule change would benefit me greatly and ensure that my time is paid for!” and “Please, I would love to be paid for the extra hours I work!”
The department’s final rule, which will go into effect on July 1, 2024, will increase the standard salary level that helps define and delimit which salaried workers are entitled to overtime pay protections under the FLSA.
Starting July 1, most salaried workers who earn less than $844 per week will become eligible for overtime pay under the final rule. And on Jan. 1, 2025, most salaried workers who make less than $1,128 per week will become eligible for overtime pay. As these changes occur, job duties will continue to determine overtime exemption status for most salaried employees.
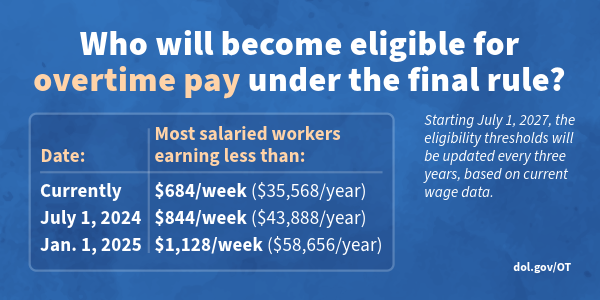
The rule will also increase the total annual compensation requirement for highly compensated employees (who are not entitled to overtime pay under the FLSA if certain requirements are met) from $107,432 per year to $132,964 per year on July 1, 2024, and then set it equal to $151,164 per year on Jan. 1, 2025.
Starting July 1, 2027, these earnings thresholds will be updated every three years so they keep pace with changes in worker salaries, ensuring that employers can adapt more easily because they’ll know when salary updates will happen and how they’ll be calculated.
The final rule will restore and extend the right to overtime pay to many salaried workers, including workers who historically were entitled to overtime pay under the FLSA because of their lower pay or the type of work they performed.
We urge workers and employers to visit our website to learn more about the final rule.
Jessica Looman is the administrator for the U.S. Department of Labor’s Wage and Hour Division. Follow the Wage and Hour Division on Twitter at @WHD_DOL and LinkedIn . Editor's note: This blog was edited to correct a typo (changing "administrator" to "administrative.")
- Wage and Hour Division (WHD)
- Fair Labor Standards Act
- overtime rule
SHARE THIS:
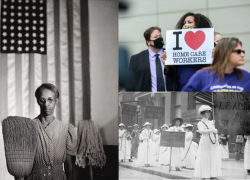
Numbers, Facts and Trends Shaping Your World
Read our research on:
Full Topic List
Regions & Countries
- Publications
- Our Methods
- Short Reads
- Tools & Resources
Read Our Research On:
A look at small businesses in the U.S.
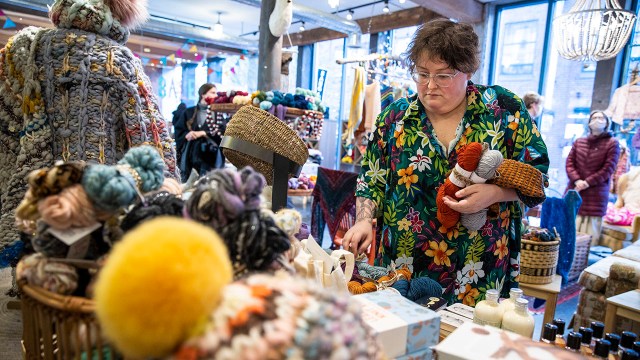
Most U.S. adults (86%) say small businesses have a positive effect on the way things are going in the country these days, according to a recent Pew Research Center survey . Small businesses, in fact, receive by far the most positive reviews of any of the nine U.S. institutions we asked about, outranking even the military and churches.
Despite their name, small businesses loom large in the United States. These businesses – defined here as those with 500 employees or fewer – account for 99.9% of U.S. firms, according to the Small Business Administration . While most of these 33 million firms don’t have paid employees, about 6 million of them do . They account for just under half of total private sector employment (46%).
As National Small Business Week approaches, here’s a look at small businesses in the U.S. and public attitudes about them, based on federal data and Center surveys.
Pew Research Center conducted this analysis to provide a glimpse into the state of American small businesses ahead of National Small Business Week .
In this analysis, “small businesses” are defined as employer firms with fewer than 500 workers. The analysis relies primarily on data from several Census Bureau sources: the Annual Business Survey (ABS), the Business Dynamics Statistics (BDS), and the Business Formation Statistics (BFS).
The ABS – conducted annually since 2017 – includes all non-farm U.S. firms with paid employees and receipts of $1,000 or more. Majority business ownership is characterized in the survey as having 51% or more of the stock or equity in the firm. The Census Bureau counts multiracial firm owners under all racial categories they identify with; Hispanic firm owners may be of any race. Read more about the ABS methodology .
Data on the age of small business comes from the BDS. Data on the annual number of high-propensity business applications in the United States is based on the number of Employer Identification Number applications used for tax purposes and is not seasonally adjusted. Read more about the BFS methodology . Per capita calculations use state-level resident population data from the Census Bureau; estimates are as of July 1, 2023.
This analysis also draws on findings from recent Center surveys. More information on the methodology for these surveys can be found by following the links in the text.
There’s no single way to define a “small business.” Economists sometimes use the size of the establishment or firm, or turn to industry-specific size standards based on average revenue. For this analysis, we’ve used the U.S. Small Business Administration’s broadest definition: employer firms with fewer than 500 workers .
An establishment is a business with one physical location. A firm is a business organization that may have multiple locations (i.e., multiple establishments).
Just how ‘small’ are small businesses ?
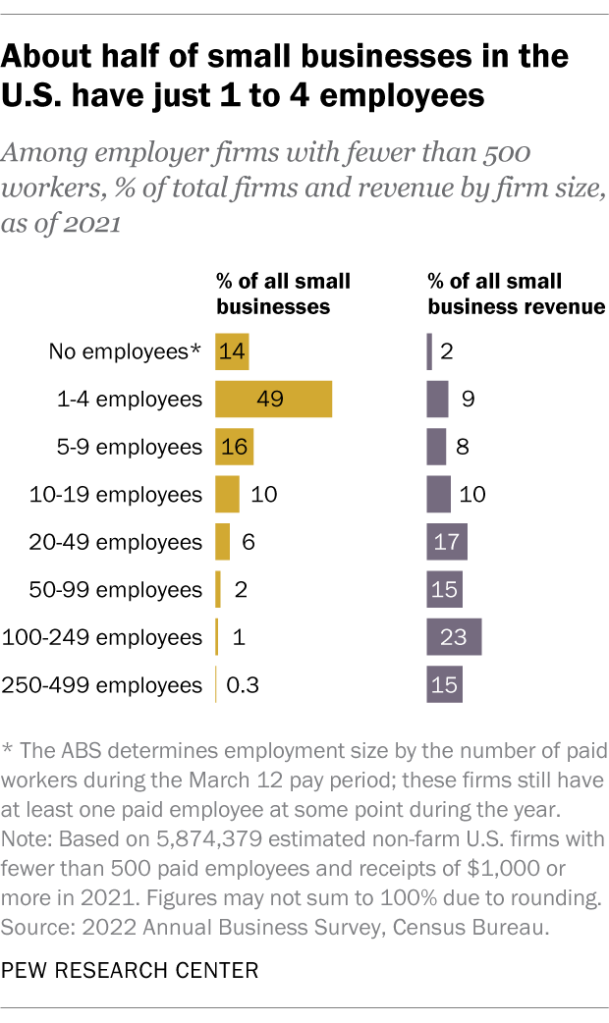
Among the roughly 6 million small businesses with employees, 49% have just one to four workers, according to the latest estimates for 2021 from the Census Bureau’s Annual Business Survey (ABS). About a quarter (27%) have between five and 19 employees; 8% have 20 to 99; and just 1% have 100 to 499 workers. The remaining 14% had paid employees at some point during the year, but not during the March 12 pay period, which the ABS uses to determine employment size.
Overall, small businesses employed an estimated 56.4 million workers in 2021 and brought in over $16.2 trillion in revenue, according to ABS data. Perhaps unsurprisingly, small businesses with more employees tend to account for larger shares of overall revenue than those with fewer workers.
Who owns and runs small businesses?
Some small businesses are family-owned, but the vast majority are not. Among small businesses that reported this type of information for 2021, 27% were family-owned and 73% were not.
So-called “mom and pop shops” account for a relatively modest share of small businesses for which information is available. Overall, 10% of small businesses in the U.S. were jointly owned and operated equally by spouses in 2021. Another 11% were jointly owned by spouses but separately operated, with men more likely than women to serve as primary operators.
Franchises aren’t very common among small businesses. Just 5% of small businesses that reported this information were fully or partially operated as franchises in 2021.
In terms of demographics, men own a greater share of small businesses overall. About six-in-ten small businesses (61%) were majority-owned by men in 2021, while 22% were majority-owned by women. Another 14% were owned equally by men and women. (The ABS defines majority ownership as having at least 51% equity in the firm.)
Looking at small businesses where estimates of majority owners’ race and ethnicity are available, most (85%) had majority-White ownership in 2021. Smaller shares were majority-owned by Asian Americans (11%), Hispanic adults (7%), and Black or African American adults (3%). About 1% were estimated to have either American Indian and Alaska Native, or Native Hawaiian and other Pacific Islander majority owners.
Related: A look at Black-owned businesses in the U.S.
Despite owning small shares of these firms overall, many Black and Asian Americans see entrepreneurship as a marker of success, according to Center surveys.
For example, 30% of Asian Americans say owning a business is important to their own view of the American dream, according to a Center survey conducted from July 2022 to January 2023 . And 36% of Black adults say owning a business is important to their personal definition of financial success, with another 22% saying it’s essential , according to a September 2023 survey .
Still, Black and Asian Americans are more likely to place emphasis on other measures asked about in these surveys, such as owning a home, having a good family life and being debt-free, among others.
How old are most small businesses?
Many small businesses have stood the test of time. In 2021, the majority of these firms (59%) had been operational for at least six years, according to the Census Bureau’s Business Dynamics Statistics . This includes 15% that had been in business for more than 25 years.
On the other end of the spectrum, about a third of small businesses (35%) had been running for five years or fewer in 2021, including 9% that had launched in the last year. (The bureau could not determine the age of the remaining 6% of firms.)
How often do new businesses open?
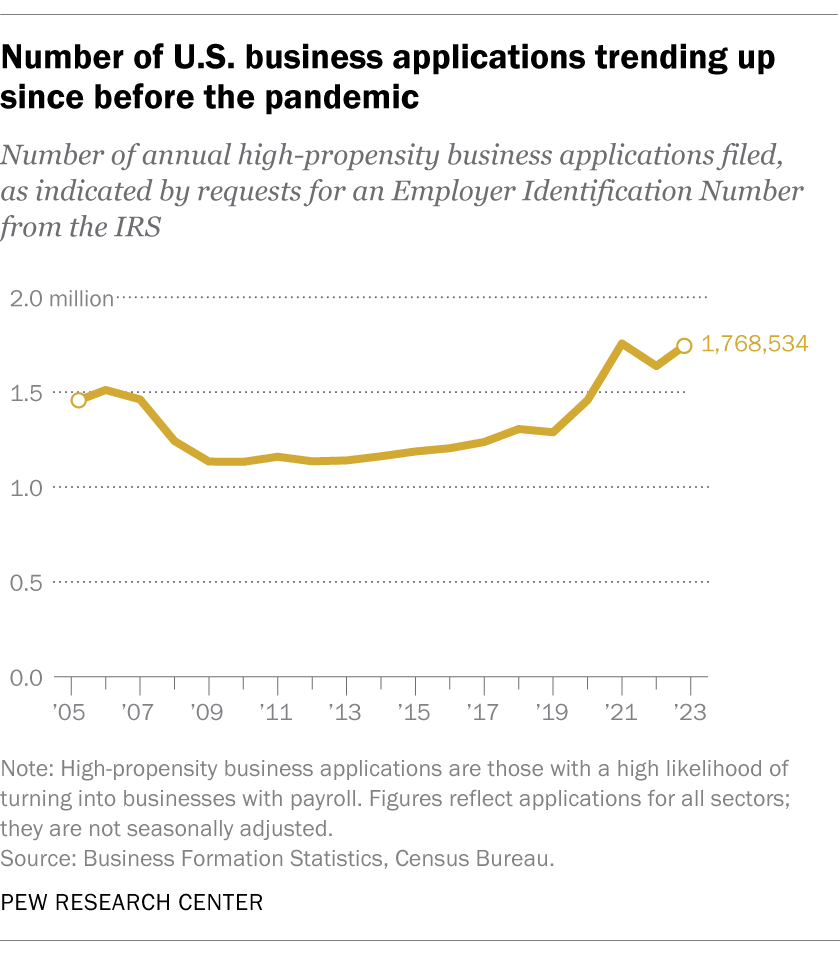
Small businesses have reported financial and staffing challenges in the years following the coronavirus pandemic . But federal data reveals the staying power of entrepreneurship in the U.S.
The number of high-propensity business applications – those that are highly likely to turn into businesses with payrolls – remained relatively stable between 2009 and 2019, according to Census Bureau data . But the number of applications has risen since before the pandemic: There were nearly 1.8 million high-propensity business applications in 2023, up from about 1.3 million in 2019.
On the state level, places with larger populations saw the most high-propensity business applications in 2023. Florida (225,809) topped the list, followed by California (221,571), Texas (151,888), New York (131,206) and Georgia (80,403). But Missouri, Wyoming, Delaware, Florida and Colorado had the most applications per capita that year.
- Business & Workplace
- Economic Conditions
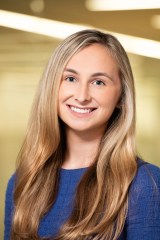
Rebecca Leppert is a copy editor at Pew Research Center
Majorities of adults see decline of union membership as bad for the U.S. and working people
A look at black-owned businesses in the u.s., from businesses and banks to colleges and churches: americans’ views of u.s. institutions, 2023 saw some of the biggest, hardest-fought labor disputes in recent decades, older workers are growing in number and earning higher wages, most popular.
1615 L St. NW, Suite 800 Washington, DC 20036 USA (+1) 202-419-4300 | Main (+1) 202-857-8562 | Fax (+1) 202-419-4372 | Media Inquiries
Research Topics
- Age & Generations
- Coronavirus (COVID-19)
- Economy & Work
- Family & Relationships
- Gender & LGBTQ
- Immigration & Migration
- International Affairs
- Internet & Technology
- Methodological Research
- News Habits & Media
- Non-U.S. Governments
- Other Topics
- Politics & Policy
- Race & Ethnicity
- Email Newsletters
ABOUT PEW RESEARCH CENTER Pew Research Center is a nonpartisan fact tank that informs the public about the issues, attitudes and trends shaping the world. It conducts public opinion polling, demographic research, media content analysis and other empirical social science research. Pew Research Center does not take policy positions. It is a subsidiary of The Pew Charitable Trusts .
Copyright 2024 Pew Research Center
Terms & Conditions
Privacy Policy
Cookie Settings
Reprints, Permissions & Use Policy
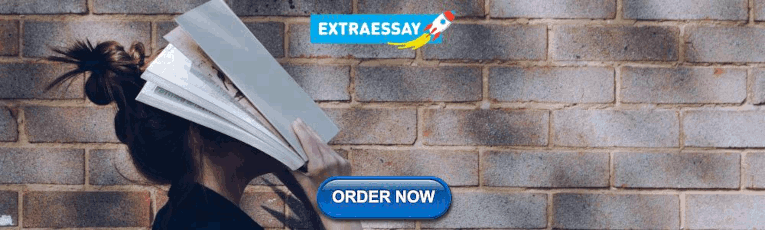
IMAGES
VIDEO
COMMENTS
Step 1: Define Your Data Hierarchy. While presenting data on the budget allocation, start by outlining the hierarchical structure. The sequence will be like the overall budget at the top, followed by departments, projects within each department, and finally, individual cost categories for each project. Example:
TheJoelTruth. While a good presentation has data, data alone doesn't guarantee a good presentation. It's all about how that data is presented. The quickest way to confuse your audience is by ...
1. Define your objectives. 2. Choose your methods. 3. Analyze your results. 4. Here's what else to consider. You have prepared and delivered a presentation on a topic related to your skill.
Values are usually stated in the form of a word or phrase. For example, most of us probably share the values of equality, freedom, honesty, fairness, justice, good health, and family. These values compose the principles or standards we use to judge and develop our beliefs, attitudes, and behaviors.".
Situational analysis. This type of analysis focuses on the situation. To conduct a situational analysis, collect information on factors like the size of the audience, their knowledge of the product or service, the occasion when you are conducting the analysis, and the physical setting of the presentation.
Data presentation and analysis are closely related activities, noting that the analysis that the researcher makes must be based on the evidence contained in the data. Data analysis may involve deductive reasoning, inductive reasoning and/or counter-inductive reasoning. To conduct data analysis, the following are crucial to achieve credible results:
Overview Audience analysis involves identifying the audience and adapting a speech to their interests, level of understanding, attitudes, and beliefs. Taking an audience-centered approach is important because a speaker's effectiveness will be improved if the presentation is created and delivered in an appropriate manner. Identifying the audience through extensive research is often difficult ...
Audience analysis is the process of learning who your audience is, what they are thinking, and how you can best reach them. Thoughtful audience analysis is one of the best habits you can develop as a speaker. It will help you understand your audience's perspective and provide maximum value for them. If done well, your audience analysis will ...
Generally, the framework for audience analysis consists of four steps: defining the purpose and scope of a presentation, researching the audience's background and needs, profiling the audience ...
Learn how to plan, design, and present your data analysis effectively and efficiently. Follow these tips to know your audience, define your purpose, structure your presentation, and more.
In this section the aim is to discuss quantitative and qualitative analysis and how to present research. You have already been advised to read widely on the method and techniques of your choice, and this is emphasized even more in this section. It is outwith the scope of this text to provide the detail and depth necessary for you to master any ...
Another extremely important factor to consider is the audience's prior experience with the topic you're discussing. This experience can take many forms. One form would be technical, theoretical, or academic knowledge of the subject. Another would be first-hand, observational , or practical experience. For instance, if you were presenting ...
Overview. Data analysis is an ongoing process that should occur throughout your research project. Suitable data-analysis methods must be selected when you write your research proposal. The nature of your data (i.e. quantitative or qualitative) will be influenced by your research design and purpose. The data will also influence the analysis ...
The influence of standardisation and task load on team coordination patterns during anaesthesia inductions. Quality and Safety in Health Care, 18 ( 2 ), 127 - 130. doi: 10.1136/qshc.2007.025973 CrossRef Google Scholar. The Cambridge Handbook of Group Interaction Analysis - August 2018.
1. Knowing your audience helps you speak their language and gain their trust. Audience analysis makes it easier to form a connection with the audience since you already know what they are looking for. Effective communication is about both speaker and audience being in sync in terms of their motives and actions.
Key Terms. audience: One or more people within hearing range of some message; for example, a group of people listening to a performance or speech; the crowd attending a stage performance. audience analysis: A study of the pertinent elements defining the makeup and characteristics of an audience. Audience-centered: Tailored to an audience.
A method of data analysis that is the umbrella term for engineering metrics and insights for additional value, direction, and context. By using exploratory statistical evaluation, data mining aims to identify dependencies, relations, patterns, and trends to generate advanced knowledge.
What Is Data Analysis? (With Examples) Data analysis is the practice of working with data to glean useful information, which can then be used to make informed decisions. "It is a capital mistake to theorize before one has data. Insensibly one begins to twist facts to suit theories, instead of theories to suit facts," Sherlock Holme's proclaims ...
Audience Analysis: Definition with types and uses. It can be hard to figure out who your audience is and what they want, both in business and beyond. You can use audience analysis to learn more about your audience and how to serve them better. To reach your full potential, you must improve your analysis of the audience skills.
Data Analysis, Interpretation, and Presentation Anna Loparev Intro HCI 02/26/2013 Qualitative vs. quantitative 2 Data analysis . 2/26/2013 2 ... Quantitative analysis -wars galaxies shuts down/121004_sonygame/ 2/26/2013 7 Example 13www.id Quantitative analysis -book.com Example 14www.id
DATA PRESENTATION, ANALYSIS AND INTERPRETATION. 4.0 Introduction. This chapter is concerned with data pres entation, of the findings obtained through the study. The. findings are presented in ...
analysis. begins with initial reactions or observations. identify patterns. calculating values. data cleansing: check for errors. interpretation. parallel with analysis. results interpreted different ways. make sure data supports conclusion. avoid biases. avoid over claiming. presentation. different methods, depends on goals. affects ...
Data analysis, interpretation, and presentation are crucial aspects of conducting high-quality research. Data analysis involves processing and analyzing the data to derive meaningful insights, while data interpretation involves making sense of the insights and drawing conclusions.
The department's final rule, which will go into effect on July 1, 2024, will increase the standard salary level that helps define and delimit which salaried workers are entitled to overtime pay protections under the FLSA.
Memorandum provides direction about how the EPA will exercise its enforcement discretion under the Comprehensive Environmental Response, Compensation, and Liability Act (CERCLA) in matters involving per- and polyfluoroalkyl substances (PFAS).
• The Regulatory Impact Analysis projects reductions of 1.38 billion metric tons of CO2 systemwide over the 2028 to 2047 timeframe along with tens of thousands of tons of PM2.5, SO2, and NOx - harmful air pollutants that are known to endanger public health. Snapshot of emissions changes • In 2035, the power sector systemwide would emit
There's no single way to define a "small business." Economists sometimes use the size of the establishment or firm, or turn to industry-specific size standards based on average revenue. For this analysis, we've used the U.S. Small Business Administration's broadest definition: employer firms with fewer than 500 workers.. An establishment is a business with one physical location.
The following slide deck was published by Altisource Portfolio Solutions S.A.
The following slide deck was published by American Airlines Group Inc.
The following slide deck was published by Carlisle Companies Incorporated in conjunction with their 2024 Q1 earnings call.