Have a language expert improve your writing
Run a free plagiarism check in 10 minutes, generate accurate citations for free.
- Knowledge Base
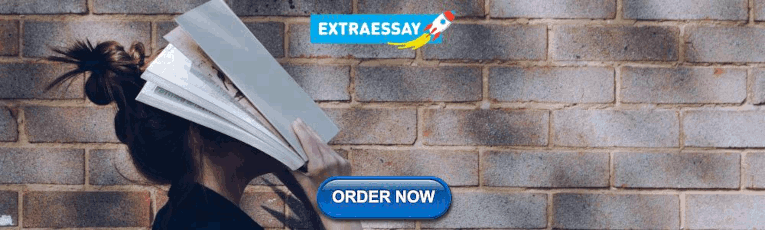
Hypothesis Testing | A Step-by-Step Guide with Easy Examples
Published on November 8, 2019 by Rebecca Bevans . Revised on June 22, 2023.
Hypothesis testing is a formal procedure for investigating our ideas about the world using statistics . It is most often used by scientists to test specific predictions, called hypotheses, that arise from theories.
There are 5 main steps in hypothesis testing:
- State your research hypothesis as a null hypothesis and alternate hypothesis (H o ) and (H a or H 1 ).
- Collect data in a way designed to test the hypothesis.
- Perform an appropriate statistical test .
- Decide whether to reject or fail to reject your null hypothesis.
- Present the findings in your results and discussion section.
Though the specific details might vary, the procedure you will use when testing a hypothesis will always follow some version of these steps.
Table of contents
Step 1: state your null and alternate hypothesis, step 2: collect data, step 3: perform a statistical test, step 4: decide whether to reject or fail to reject your null hypothesis, step 5: present your findings, other interesting articles, frequently asked questions about hypothesis testing.
After developing your initial research hypothesis (the prediction that you want to investigate), it is important to restate it as a null (H o ) and alternate (H a ) hypothesis so that you can test it mathematically.
The alternate hypothesis is usually your initial hypothesis that predicts a relationship between variables. The null hypothesis is a prediction of no relationship between the variables you are interested in.
- H 0 : Men are, on average, not taller than women. H a : Men are, on average, taller than women.
Receive feedback on language, structure, and formatting
Professional editors proofread and edit your paper by focusing on:
- Academic style
- Vague sentences
- Style consistency
See an example
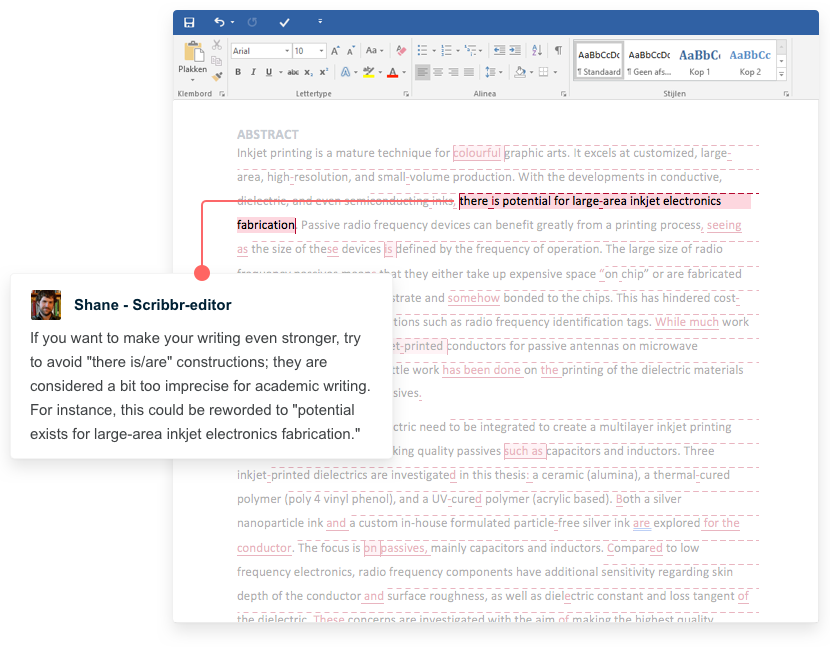
For a statistical test to be valid , it is important to perform sampling and collect data in a way that is designed to test your hypothesis. If your data are not representative, then you cannot make statistical inferences about the population you are interested in.
There are a variety of statistical tests available, but they are all based on the comparison of within-group variance (how spread out the data is within a category) versus between-group variance (how different the categories are from one another).
If the between-group variance is large enough that there is little or no overlap between groups, then your statistical test will reflect that by showing a low p -value . This means it is unlikely that the differences between these groups came about by chance.
Alternatively, if there is high within-group variance and low between-group variance, then your statistical test will reflect that with a high p -value. This means it is likely that any difference you measure between groups is due to chance.
Your choice of statistical test will be based on the type of variables and the level of measurement of your collected data .
- an estimate of the difference in average height between the two groups.
- a p -value showing how likely you are to see this difference if the null hypothesis of no difference is true.
Based on the outcome of your statistical test, you will have to decide whether to reject or fail to reject your null hypothesis.
In most cases you will use the p -value generated by your statistical test to guide your decision. And in most cases, your predetermined level of significance for rejecting the null hypothesis will be 0.05 – that is, when there is a less than 5% chance that you would see these results if the null hypothesis were true.
In some cases, researchers choose a more conservative level of significance, such as 0.01 (1%). This minimizes the risk of incorrectly rejecting the null hypothesis ( Type I error ).
Prevent plagiarism. Run a free check.
The results of hypothesis testing will be presented in the results and discussion sections of your research paper , dissertation or thesis .
In the results section you should give a brief summary of the data and a summary of the results of your statistical test (for example, the estimated difference between group means and associated p -value). In the discussion , you can discuss whether your initial hypothesis was supported by your results or not.
In the formal language of hypothesis testing, we talk about rejecting or failing to reject the null hypothesis. You will probably be asked to do this in your statistics assignments.
However, when presenting research results in academic papers we rarely talk this way. Instead, we go back to our alternate hypothesis (in this case, the hypothesis that men are on average taller than women) and state whether the result of our test did or did not support the alternate hypothesis.
If your null hypothesis was rejected, this result is interpreted as “supported the alternate hypothesis.”
These are superficial differences; you can see that they mean the same thing.
You might notice that we don’t say that we reject or fail to reject the alternate hypothesis . This is because hypothesis testing is not designed to prove or disprove anything. It is only designed to test whether a pattern we measure could have arisen spuriously, or by chance.
If we reject the null hypothesis based on our research (i.e., we find that it is unlikely that the pattern arose by chance), then we can say our test lends support to our hypothesis . But if the pattern does not pass our decision rule, meaning that it could have arisen by chance, then we say the test is inconsistent with our hypothesis .
If you want to know more about statistics , methodology , or research bias , make sure to check out some of our other articles with explanations and examples.
- Normal distribution
- Descriptive statistics
- Measures of central tendency
- Correlation coefficient
Methodology
- Cluster sampling
- Stratified sampling
- Types of interviews
- Cohort study
- Thematic analysis
Research bias
- Implicit bias
- Cognitive bias
- Survivorship bias
- Availability heuristic
- Nonresponse bias
- Regression to the mean
Hypothesis testing is a formal procedure for investigating our ideas about the world using statistics. It is used by scientists to test specific predictions, called hypotheses , by calculating how likely it is that a pattern or relationship between variables could have arisen by chance.
A hypothesis states your predictions about what your research will find. It is a tentative answer to your research question that has not yet been tested. For some research projects, you might have to write several hypotheses that address different aspects of your research question.
A hypothesis is not just a guess — it should be based on existing theories and knowledge. It also has to be testable, which means you can support or refute it through scientific research methods (such as experiments, observations and statistical analysis of data).
Null and alternative hypotheses are used in statistical hypothesis testing . The null hypothesis of a test always predicts no effect or no relationship between variables, while the alternative hypothesis states your research prediction of an effect or relationship.
Cite this Scribbr article
If you want to cite this source, you can copy and paste the citation or click the “Cite this Scribbr article” button to automatically add the citation to our free Citation Generator.
Bevans, R. (2023, June 22). Hypothesis Testing | A Step-by-Step Guide with Easy Examples. Scribbr. Retrieved April 9, 2024, from https://www.scribbr.com/statistics/hypothesis-testing/
Is this article helpful?
Rebecca Bevans
Other students also liked, choosing the right statistical test | types & examples, understanding p values | definition and examples, what is your plagiarism score.
Research Hypothesis In Psychology: Types, & Examples
Saul Mcleod, PhD
Editor-in-Chief for Simply Psychology
BSc (Hons) Psychology, MRes, PhD, University of Manchester
Saul Mcleod, PhD., is a qualified psychology teacher with over 18 years of experience in further and higher education. He has been published in peer-reviewed journals, including the Journal of Clinical Psychology.
Learn about our Editorial Process
Olivia Guy-Evans, MSc
Associate Editor for Simply Psychology
BSc (Hons) Psychology, MSc Psychology of Education
Olivia Guy-Evans is a writer and associate editor for Simply Psychology. She has previously worked in healthcare and educational sectors.
On This Page:
A research hypothesis, in its plural form “hypotheses,” is a specific, testable prediction about the anticipated results of a study, established at its outset. It is a key component of the scientific method .
Hypotheses connect theory to data and guide the research process towards expanding scientific understanding
Some key points about hypotheses:
- A hypothesis expresses an expected pattern or relationship. It connects the variables under investigation.
- It is stated in clear, precise terms before any data collection or analysis occurs. This makes the hypothesis testable.
- A hypothesis must be falsifiable. It should be possible, even if unlikely in practice, to collect data that disconfirms rather than supports the hypothesis.
- Hypotheses guide research. Scientists design studies to explicitly evaluate hypotheses about how nature works.
- For a hypothesis to be valid, it must be testable against empirical evidence. The evidence can then confirm or disprove the testable predictions.
- Hypotheses are informed by background knowledge and observation, but go beyond what is already known to propose an explanation of how or why something occurs.
Predictions typically arise from a thorough knowledge of the research literature, curiosity about real-world problems or implications, and integrating this to advance theory. They build on existing literature while providing new insight.
Types of Research Hypotheses
Alternative hypothesis.
The research hypothesis is often called the alternative or experimental hypothesis in experimental research.
It typically suggests a potential relationship between two key variables: the independent variable, which the researcher manipulates, and the dependent variable, which is measured based on those changes.
The alternative hypothesis states a relationship exists between the two variables being studied (one variable affects the other).
A hypothesis is a testable statement or prediction about the relationship between two or more variables. It is a key component of the scientific method. Some key points about hypotheses:
- Important hypotheses lead to predictions that can be tested empirically. The evidence can then confirm or disprove the testable predictions.
In summary, a hypothesis is a precise, testable statement of what researchers expect to happen in a study and why. Hypotheses connect theory to data and guide the research process towards expanding scientific understanding.
An experimental hypothesis predicts what change(s) will occur in the dependent variable when the independent variable is manipulated.
It states that the results are not due to chance and are significant in supporting the theory being investigated.
The alternative hypothesis can be directional, indicating a specific direction of the effect, or non-directional, suggesting a difference without specifying its nature. It’s what researchers aim to support or demonstrate through their study.
Null Hypothesis
The null hypothesis states no relationship exists between the two variables being studied (one variable does not affect the other). There will be no changes in the dependent variable due to manipulating the independent variable.
It states results are due to chance and are not significant in supporting the idea being investigated.
The null hypothesis, positing no effect or relationship, is a foundational contrast to the research hypothesis in scientific inquiry. It establishes a baseline for statistical testing, promoting objectivity by initiating research from a neutral stance.
Many statistical methods are tailored to test the null hypothesis, determining the likelihood of observed results if no true effect exists.
This dual-hypothesis approach provides clarity, ensuring that research intentions are explicit, and fosters consistency across scientific studies, enhancing the standardization and interpretability of research outcomes.
Nondirectional Hypothesis
A non-directional hypothesis, also known as a two-tailed hypothesis, predicts that there is a difference or relationship between two variables but does not specify the direction of this relationship.
It merely indicates that a change or effect will occur without predicting which group will have higher or lower values.
For example, “There is a difference in performance between Group A and Group B” is a non-directional hypothesis.
Directional Hypothesis
A directional (one-tailed) hypothesis predicts the nature of the effect of the independent variable on the dependent variable. It predicts in which direction the change will take place. (i.e., greater, smaller, less, more)
It specifies whether one variable is greater, lesser, or different from another, rather than just indicating that there’s a difference without specifying its nature.
For example, “Exercise increases weight loss” is a directional hypothesis.
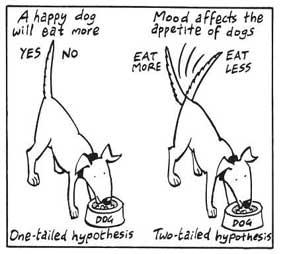
Falsifiability
The Falsification Principle, proposed by Karl Popper , is a way of demarcating science from non-science. It suggests that for a theory or hypothesis to be considered scientific, it must be testable and irrefutable.
Falsifiability emphasizes that scientific claims shouldn’t just be confirmable but should also have the potential to be proven wrong.
It means that there should exist some potential evidence or experiment that could prove the proposition false.
However many confirming instances exist for a theory, it only takes one counter observation to falsify it. For example, the hypothesis that “all swans are white,” can be falsified by observing a black swan.
For Popper, science should attempt to disprove a theory rather than attempt to continually provide evidence to support a research hypothesis.
Can a Hypothesis be Proven?
Hypotheses make probabilistic predictions. They state the expected outcome if a particular relationship exists. However, a study result supporting a hypothesis does not definitively prove it is true.
All studies have limitations. There may be unknown confounding factors or issues that limit the certainty of conclusions. Additional studies may yield different results.
In science, hypotheses can realistically only be supported with some degree of confidence, not proven. The process of science is to incrementally accumulate evidence for and against hypothesized relationships in an ongoing pursuit of better models and explanations that best fit the empirical data. But hypotheses remain open to revision and rejection if that is where the evidence leads.
- Disproving a hypothesis is definitive. Solid disconfirmatory evidence will falsify a hypothesis and require altering or discarding it based on the evidence.
- However, confirming evidence is always open to revision. Other explanations may account for the same results, and additional or contradictory evidence may emerge over time.
We can never 100% prove the alternative hypothesis. Instead, we see if we can disprove, or reject the null hypothesis.
If we reject the null hypothesis, this doesn’t mean that our alternative hypothesis is correct but does support the alternative/experimental hypothesis.
Upon analysis of the results, an alternative hypothesis can be rejected or supported, but it can never be proven to be correct. We must avoid any reference to results proving a theory as this implies 100% certainty, and there is always a chance that evidence may exist which could refute a theory.
How to Write a Hypothesis
- Identify variables . The researcher manipulates the independent variable and the dependent variable is the measured outcome.
- Operationalized the variables being investigated . Operationalization of a hypothesis refers to the process of making the variables physically measurable or testable, e.g. if you are about to study aggression, you might count the number of punches given by participants.
- Decide on a direction for your prediction . If there is evidence in the literature to support a specific effect of the independent variable on the dependent variable, write a directional (one-tailed) hypothesis. If there are limited or ambiguous findings in the literature regarding the effect of the independent variable on the dependent variable, write a non-directional (two-tailed) hypothesis.
- Make it Testable : Ensure your hypothesis can be tested through experimentation or observation. It should be possible to prove it false (principle of falsifiability).
- Clear & concise language . A strong hypothesis is concise (typically one to two sentences long), and formulated using clear and straightforward language, ensuring it’s easily understood and testable.
Consider a hypothesis many teachers might subscribe to: students work better on Monday morning than on Friday afternoon (IV=Day, DV= Standard of work).
Now, if we decide to study this by giving the same group of students a lesson on a Monday morning and a Friday afternoon and then measuring their immediate recall of the material covered in each session, we would end up with the following:
- The alternative hypothesis states that students will recall significantly more information on a Monday morning than on a Friday afternoon.
- The null hypothesis states that there will be no significant difference in the amount recalled on a Monday morning compared to a Friday afternoon. Any difference will be due to chance or confounding factors.
More Examples
- Memory : Participants exposed to classical music during study sessions will recall more items from a list than those who studied in silence.
- Social Psychology : Individuals who frequently engage in social media use will report higher levels of perceived social isolation compared to those who use it infrequently.
- Developmental Psychology : Children who engage in regular imaginative play have better problem-solving skills than those who don’t.
- Clinical Psychology : Cognitive-behavioral therapy will be more effective in reducing symptoms of anxiety over a 6-month period compared to traditional talk therapy.
- Cognitive Psychology : Individuals who multitask between various electronic devices will have shorter attention spans on focused tasks than those who single-task.
- Health Psychology : Patients who practice mindfulness meditation will experience lower levels of chronic pain compared to those who don’t meditate.
- Organizational Psychology : Employees in open-plan offices will report higher levels of stress than those in private offices.
- Behavioral Psychology : Rats rewarded with food after pressing a lever will press it more frequently than rats who receive no reward.

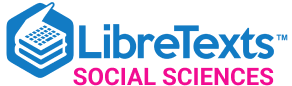
- school Campus Bookshelves
- menu_book Bookshelves
- perm_media Learning Objects
- login Login
- how_to_reg Request Instructor Account
- hub Instructor Commons
- Download Page (PDF)
- Download Full Book (PDF)
- Periodic Table
- Physics Constants
- Scientific Calculator
- Reference & Cite
- Tools expand_more
- Readability
selected template will load here
This action is not available.
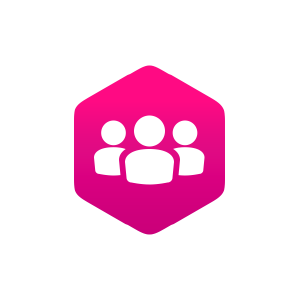
Developing Theories & Hypotheses
- Last updated
- Save as PDF
- Page ID 40843
2.5: Developing a Hypothesis
Learning objectives.
- Distinguish between a theory and a hypothesis.
- Discover how theories are used to generate hypotheses and how the results of studies can be used to further inform theories.
- Understand the characteristics of a good hypothesis.
Theories and Hypotheses
Before describing how to develop a hypothesis, it is important to distinguish between a theory and a hypothesis. A theory is a coherent explanation or interpretation of one or more phenomena. Although theories can take a variety of forms, one thing they have in common is that they go beyond the phenomena they explain by including variables, structures, processes, functions, or organizing principles that have not been observed directly. Consider, for example, Zajonc’s theory of social facilitation and social inhibition (1965) [1] . He proposed that being watched by others while performing a task creates a general state of physiological arousal, which increases the likelihood of the dominant (most likely) response. So for highly practiced tasks, being watched increases the tendency to make correct responses, but for relatively unpracticed tasks, being watched increases the tendency to make incorrect responses. Notice that this theory—which has come to be called drive theory—provides an explanation of both social facilitation and social inhibition that goes beyond the phenomena themselves by including concepts such as “arousal” and “dominant response,” along with processes such as the effect of arousal on the dominant response.
Outside of science, referring to an idea as a theory often implies that it is untested—perhaps no more than a wild guess. In science, however, the term theory has no such implication. A theory is simply an explanation or interpretation of a set of phenomena. It can be untested, but it can also be extensively tested, well supported, and accepted as an accurate description of the world by the scientific community. The theory of evolution by natural selection, for example, is a theory because it is an explanation of the diversity of life on earth—not because it is untested or unsupported by scientific research. On the contrary, the evidence for this theory is overwhelmingly positive and nearly all scientists accept its basic assumptions as accurate. Similarly, the “germ theory” of disease is a theory because it is an explanation of the origin of various diseases, not because there is any doubt that many diseases are caused by microorganisms that infect the body.
A hypothesis , on the other hand, is a specific prediction about a new phenomenon that should be observed if a particular theory is accurate. It is an explanation that relies on just a few key concepts. Hypotheses are often specific predictions about what will happen in a particular study. They are developed by considering existing evidence and using reasoning to infer what will happen in the specific context of interest. Hypotheses are often but not always derived from theories. So a hypothesis is often a prediction based on a theory but some hypotheses are a-theoretical and only after a set of observations have been made, is a theory developed. This is because theories are broad in nature and they explain larger bodies of data. So if our research question is really original then we may need to collect some data and make some observations before we can develop a broader theory.
Theories and hypotheses always have this if-then relationship. “ If drive theory is correct, then cockroaches should run through a straight runway faster, and a branching runway more slowly, when other cockroaches are present.” Although hypotheses are usually expressed as statements, they can always be rephrased as questions. “Do cockroaches run through a straight runway faster when other cockroaches are present?” Thus deriving hypotheses from theories is an excellent way of generating interesting research questions.
But how do researchers derive hypotheses from theories? One way is to generate a research question using the techniques discussed in this chapter and then ask whether any theory implies an answer to that question. For example, you might wonder whether expressive writing about positive experiences improves health as much as expressive writing about traumatic experiences. Although this question is an interesting one on its own, you might then ask whether the habituation theory—the idea that expressive writing causes people to habituate to negative thoughts and feelings—implies an answer. In this case, it seems clear that if the habituation theory is correct, then expressive writing about positive experiences should not be effective because it would not cause people to habituate to negative thoughts and feelings. A second way to derive hypotheses from theories is to focus on some component of the theory that has not yet been directly observed. For example, a researcher could focus on the process of habituation—perhaps hypothesizing that people should show fewer signs of emotional distress with each new writing session.
Among the very best hypotheses are those that distinguish between competing theories. For example, Norbert Schwarz and his colleagues considered two theories of how people make judgments about themselves, such as how assertive they are (Schwarz et al., 1991) [2] . Both theories held that such judgments are based on relevant examples that people bring to mind. However, one theory was that people base their judgments on the number of examples they bring to mind and the other was that people base their judgments on how easily they bring those examples to mind. To test these theories, the researchers asked people to recall either six times when they were assertive (which is easy for most people) or 12 times (which is difficult for most people). Then they asked them to judge their own assertiveness. Note that the number-of-examples theory implies that people who recalled 12 examples should judge themselves to be more assertive because they recalled more examples, but the ease-of-examples theory implies that participants who recalled six examples should judge themselves as more assertive because recalling the examples was easier. Thus the two theories made opposite predictions so that only one of the predictions could be confirmed. The surprising result was that participants who recalled fewer examples judged themselves to be more assertive—providing particularly convincing evidence in favor of the ease-of-retrieval theory over the number-of-examples theory.
Theory Testing
The primary way that scientific researchers use theories is sometimes called the hypothetico-deductive method (although this term is much more likely to be used by philosophers of science than by scientists themselves). Researchers begin with a set of phenomena and either construct a theory to explain or interpret them or choose an existing theory to work with. They then make a prediction about some new phenomenon that should be observed if the theory is correct. Again, this prediction is called a hypothesis. The researchers then conduct an empirical study to test the hypothesis. Finally, they reevaluate the theory in light of the new results and revise it if necessary. This process is usually conceptualized as a cycle because the researchers can then derive a new hypothesis from the revised theory, conduct a new empirical study to test the hypothesis, and so on. As Figure \(\PageIndex{1}\) shows, this approach meshes nicely with the model of scientific research in psychology presented earlier in the textbook—creating a more detailed model of “theoretically motivated” or “theory-driven” research.
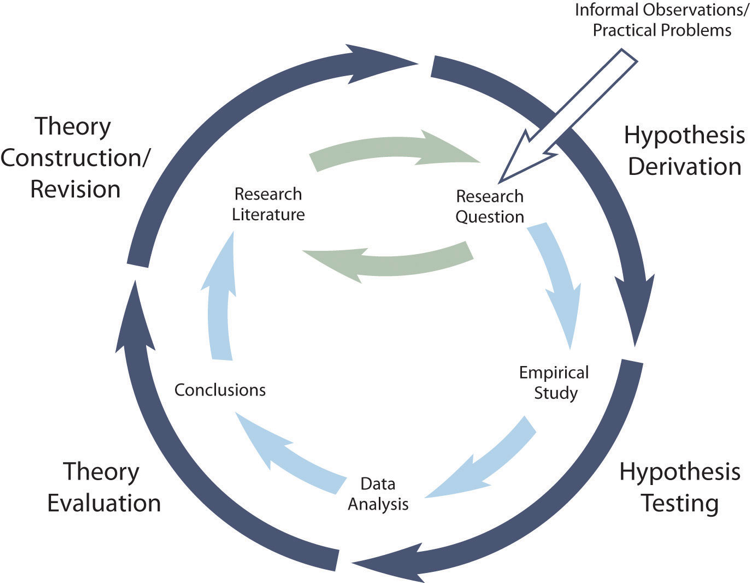
As an example, let us consider Zajonc’s research on social facilitation and inhibition. He started with a somewhat contradictory pattern of results from the research literature. He then constructed his drive theory, according to which being watched by others while performing a task causes physiological arousal, which increases an organism’s tendency to make the dominant response. This theory predicts social facilitation for well-learned tasks and social inhibition for poorly learned tasks. He now had a theory that organized previous results in a meaningful way—but he still needed to test it. He hypothesized that if his theory was correct, he should observe that the presence of others improves performance in a simple laboratory task but inhibits performance in a difficult version of the very same laboratory task. To test this hypothesis, one of the studies he conducted used cockroaches as subjects (Zajonc, Heingartner, & Herman, 1969) [3] . The cockroaches ran either down a straight runway (an easy task for a cockroach) or through a cross-shaped maze (a difficult task for a cockroach) to escape into a dark chamber when a light was shined on them. They did this either while alone or in the presence of other cockroaches in clear plastic “audience boxes.” Zajonc found that cockroaches in the straight runway reached their goal more quickly in the presence of other cockroaches, but cockroaches in the cross-shaped maze reached their goal more slowly when they were in the presence of other cockroaches. Thus he confirmed his hypothesis and provided support for his drive theory. (Zajonc also showed that drive theory existed in humans [Zajonc & Sales, 1966] [4] in many other studies afterward).
Incorporating Theory into Your Research
When you write your research report or plan your presentation, be aware that there are two basic ways that researchers usually include theory. The first is to raise a research question, answer that question by conducting a new study, and then offer one or more theories (usually more) to explain or interpret the results. This format works well for applied research questions and for research questions that existing theories do not address. The second way is to describe one or more existing theories, derive a hypothesis from one of those theories, test the hypothesis in a new study, and finally reevaluate the theory. This format works well when there is an existing theory that addresses the research question—especially if the resulting hypothesis is surprising or conflicts with a hypothesis derived from a different theory.
To use theories in your research will not only give you guidance in coming up with experiment ideas and possible projects, but it lends legitimacy to your work. Psychologists have been interested in a variety of human behaviors and have developed many theories along the way. Using established theories will help you break new ground as a researcher, not limit you from developing your own ideas.
There are three general characteristics of a good hypothesis. First, a good hypothesis must be testable and falsifiable . We must be able to test the hypothesis using the methods of science and if you’ll recall Popper’s falsifiability criterion, it must be possible to gather evidence that will disconfirm the hypothesis if it is indeed false. Second, a good hypothesis must be logical. As described above, hypotheses are more than just a random guess. Hypotheses should be informed by previous theories or observations and logical reasoning. Typically, we begin with a broad and general theory and use deductive reasoning to generate a more specific hypothesis to test based on that theory. Occasionally, however, when there is no theory to inform our hypothesis, we use inductive reasoning which involves using specific observations or research findings to form a more general hypothesis. Finally, the hypothesis should be positive. That is, the hypothesis should make a positive statement about the existence of a relationship or effect, rather than a statement that a relationship or effect does not exist. As scientists, we don’t set out to show that relationships do not exist or that effects do not occur so our hypotheses should not be worded in a way to suggest that an effect or relationship does not exist. The nature of science is to assume that something does not exist and then seek to find evidence to prove this wrong, to show that it really does exist. That may seem backward to you but that is the nature of the scientific method. The underlying reason for this is beyond the scope of this chapter but it has to do with statistical theory.
- Zajonc, R. B. (1965). Social facilitation. Science, 149 , 269–274 ↵
- Schwarz, N., Bless, H., Strack, F., Klumpp, G., Rittenauer-Schatka, H., & Simons, A. (1991). Ease of retrieval as information: Another look at the availability heuristic. Journal of Personality and Social Psychology, 61 , 195–202. ↵
- Zajonc, R. B., Heingartner, A., & Herman, E. M. (1969). Social enhancement and impairment of performance in the cockroach. Journal of Personality and Social Psychology, 13 , 83–92. ↵
- Zajonc, R.B. & Sales, S.M. (1966). Social facilitation of dominant and subordinate responses. Journal of Experimental Social Psychology, 2 , 160-168. ↵
- First Online: 01 January 2024
Cite this chapter
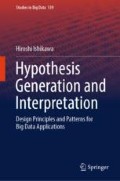
- Hiroshi Ishikawa 3
Part of the book series: Studies in Big Data ((SBD,volume 139))
122 Accesses
This chapter will explain the definition and properties of a hypothesis, the related concepts, and basic methods of hypothesis generation as follows.
Describe the definition, properties, and life cycle of a hypothesis.
Describe relationships between a hypothesis and a theory, a model, and data.
Categorize and explain research questions that provide hints for hypothesis generation.
Explain how to visualize data and analysis results.
Explain the philosophy of science and scientific methods in relation to hypothesis generation in science.
Explain deduction, induction, plausible reasoning, and analogy concretely as reasoning methods useful for hypothesis generation.
Explain problem solving as hypothesis generation methods by using familiar examples.
This is a preview of subscription content, log in via an institution to check access.
Access this chapter
- Available as PDF
- Read on any device
- Instant download
- Own it forever
- Available as EPUB and PDF
- Durable hardcover edition
- Dispatched in 3 to 5 business days
- Free shipping worldwide - see info
Tax calculation will be finalised at checkout
Purchases are for personal use only
Institutional subscriptions
Aufmann RN, Lockwood JS et al (2018) Mathematical excursions. CENGAGE
Google Scholar
Bortolotti L (2008) An introduction to the philosophy of science. Polity
Cairo A (2016) The truthful art: data, charts, and maps for communication. New Riders
Cellucci C (2017) Rethinking knowledge: the heuristic view. Springer
Chang M (2014) Principles of scientific methods. CRC Press
Crease RP (2010) The great equations: breakthroughs in science from Pythagoras to Heisenberg. W. W. Norton & Company
Danks D, Ippoliti E (eds) Building theories: Heuristics and hypotheses in sciences. Springer
Diggle PJ, Chetwynd AG (2011) Statistics and scientific method: an introduction for students and researchers. Oxford University Press
DOAJ (2022) Directory of open access journal. https://doaj.org/ Accessed 2022
Gilchrist P, Wheaton B (2011) Lifestyle sport, public policy and youth engagement: examining the emergence of Parkour. Int J Sport Policy Polit 3(1):109–131. https://doi.org/10.1080/19406940.2010.547866
Article Google Scholar
Google Maps. https://www.google.com/maps Accessed 2022.
Ishikawa H (2015) Social big data mining. CRC Press
Järvinen P (2008) Mapping research questions to research methods. In: Avison D, Kasper GM, Pernici B, Ramos I, Roode D (eds) Advances in information systems research, education and practice. Proceedings of IFIP 20th world computer congress, TC 8, information systems, vol 274. Springer. https://doi.org/10.1007/978-0-387-09682-7-9_3
JAXA (2022) Martian moons eXploration. http://www.mmx.jaxa.jp/en/ . Accessed 2022
Lewton T (2020) How the bits of quantum gravity can buzz. Quanta Magazine. 2020. https://www.quantamagazine.org/gravitons-revealed-in-the-noise-of-gravitational-waves-20200723/ . Accessed 2022
Mahajan S (2014) The art of insight in science and engineering: Mastering complexity. The MIT Press
Méndez A, Rivera–Valentín EG (2017) The equilibrium temperature of planets in elliptical orbits. Astrophys J Lett 837(1)
NASA (2022) Mars sample return. https://www.jpl.nasa.gov/missions/mars-sample-return-msr Accessed 2022
OpenStreetMap (2022). https://www.openstreetmap.org . Accessed 2022
Pólya G (2009) Mathematics and plausible reasoning: vol I: induction and analogy in mathematics. Ishi Press
Pólya G, Conway JH (2014) How to solve it. Princeton University Press
Rehm J (2019) The four fundamental forces of nature. Live science https://www.livescience.com/the-fundamental-forces-of-nature.html
Sadler-Smith E (2015) Wallas’ four-stage model of the creative process: more than meets the eye? Creat Res J 27(4):342–352. https://doi.org/10.1080/10400419.2015.1087277
Siegel E, This is why physicists think string theory might be our ‘theory of everything.’ Forbes, 2018. https://www.forbes.com/sites/startswithabang/2018/05/31/this-is-why-physicists-think-string-theory-might-be-our-theory-of-everything/?sh=b01d79758c25
Zeitz P (2006) The art and craft of problem solving. Wiley
Download references
Author information
Authors and affiliations.
Department of Systems Design, Tokyo Metropolitan University, Hino, Tokyo, Japan
Hiroshi Ishikawa
You can also search for this author in PubMed Google Scholar
Corresponding author
Correspondence to Hiroshi Ishikawa .
Rights and permissions
Reprints and permissions
Copyright information
© 2024 The Author(s), under exclusive license to Springer Nature Switzerland AG
About this chapter
Ishikawa, H. (2024). Hypothesis. In: Hypothesis Generation and Interpretation. Studies in Big Data, vol 139. Springer, Cham. https://doi.org/10.1007/978-3-031-43540-9_2
Download citation
DOI : https://doi.org/10.1007/978-3-031-43540-9_2
Published : 01 January 2024
Publisher Name : Springer, Cham
Print ISBN : 978-3-031-43539-3
Online ISBN : 978-3-031-43540-9
eBook Packages : Computer Science Computer Science (R0)
Share this chapter
Anyone you share the following link with will be able to read this content:
Sorry, a shareable link is not currently available for this article.
Provided by the Springer Nature SharedIt content-sharing initiative
- Publish with us
Policies and ethics
- Find a journal
- Track your research

Want to create or adapt books like this? Learn more about how Pressbooks supports open publishing practices.
Developing a Hypothesis
Rajiv S. Jhangiani; I-Chant A. Chiang; Carrie Cuttler; and Dana C. Leighton
Learning Objectives
- Distinguish between a theory and a hypothesis.
- Discover how theories are used to generate hypotheses and how the results of studies can be used to further inform theories.
- Understand the characteristics of a good hypothesis.
Theories and Hypotheses
Before describing how to develop a hypothesis, it is important to distinguish between a theory and a hypothesis. A theory is a coherent explanation or interpretation of one or more phenomena. Although theories can take a variety of forms, one thing they have in common is that they go beyond the phenomena they explain by including variables, structures, processes, functions, or organizing principles that have not been observed directly. Consider, for example, Zajonc’s theory of social facilitation and social inhibition (1965) [1] . He proposed that being watched by others while performing a task creates a general state of physiological arousal, which increases the likelihood of the dominant (most likely) response. So for highly practiced tasks, being watched increases the tendency to make correct responses, but for relatively unpracticed tasks, being watched increases the tendency to make incorrect responses. Notice that this theory—which has come to be called drive theory—provides an explanation of both social facilitation and social inhibition that goes beyond the phenomena themselves by including concepts such as “arousal” and “dominant response,” along with processes such as the effect of arousal on the dominant response.
Outside of science, referring to an idea as a theory often implies that it is untested—perhaps no more than a wild guess. In science, however, the term theory has no such implication. A theory is simply an explanation or interpretation of a set of phenomena. It can be untested, but it can also be extensively tested, well supported, and accepted as an accurate description of the world by the scientific community. The theory of evolution by natural selection, for example, is a theory because it is an explanation of the diversity of life on earth—not because it is untested or unsupported by scientific research. On the contrary, the evidence for this theory is overwhelmingly positive and nearly all scientists accept its basic assumptions as accurate. Similarly, the “germ theory” of disease is a theory because it is an explanation of the origin of various diseases, not because there is any doubt that many diseases are caused by microorganisms that infect the body.
A hypothesis , on the other hand, is a specific prediction about a new phenomenon that should be observed if a particular theory is accurate. It is an explanation that relies on just a few key concepts. Hypotheses are often specific predictions about what will happen in a particular study. They are developed by considering existing evidence and using reasoning to infer what will happen in the specific context of interest. Hypotheses are often but not always derived from theories. So a hypothesis is often a prediction based on a theory but some hypotheses are a-theoretical and only after a set of observations have been made, is a theory developed. This is because theories are broad in nature and they explain larger bodies of data. So if our research question is really original then we may need to collect some data and make some observations before we can develop a broader theory.
Theories and hypotheses always have this if-then relationship. “ If drive theory is correct, then cockroaches should run through a straight runway faster, and a branching runway more slowly, when other cockroaches are present.” Although hypotheses are usually expressed as statements, they can always be rephrased as questions. “Do cockroaches run through a straight runway faster when other cockroaches are present?” Thus deriving hypotheses from theories is an excellent way of generating interesting research questions.
But how do researchers derive hypotheses from theories? One way is to generate a research question using the techniques discussed in this chapter and then ask whether any theory implies an answer to that question. For example, you might wonder whether expressive writing about positive experiences improves health as much as expressive writing about traumatic experiences. Although this question is an interesting one on its own, you might then ask whether the habituation theory—the idea that expressive writing causes people to habituate to negative thoughts and feelings—implies an answer. In this case, it seems clear that if the habituation theory is correct, then expressive writing about positive experiences should not be effective because it would not cause people to habituate to negative thoughts and feelings. A second way to derive hypotheses from theories is to focus on some component of the theory that has not yet been directly observed. For example, a researcher could focus on the process of habituation—perhaps hypothesizing that people should show fewer signs of emotional distress with each new writing session.
Among the very best hypotheses are those that distinguish between competing theories. For example, Norbert Schwarz and his colleagues considered two theories of how people make judgments about themselves, such as how assertive they are (Schwarz et al., 1991) [2] . Both theories held that such judgments are based on relevant examples that people bring to mind. However, one theory was that people base their judgments on the number of examples they bring to mind and the other was that people base their judgments on how easily they bring those examples to mind. To test these theories, the researchers asked people to recall either six times when they were assertive (which is easy for most people) or 12 times (which is difficult for most people). Then they asked them to judge their own assertiveness. Note that the number-of-examples theory implies that people who recalled 12 examples should judge themselves to be more assertive because they recalled more examples, but the ease-of-examples theory implies that participants who recalled six examples should judge themselves as more assertive because recalling the examples was easier. Thus the two theories made opposite predictions so that only one of the predictions could be confirmed. The surprising result was that participants who recalled fewer examples judged themselves to be more assertive—providing particularly convincing evidence in favor of the ease-of-retrieval theory over the number-of-examples theory.
Theory Testing
The primary way that scientific researchers use theories is sometimes called the hypothetico-deductive method (although this term is much more likely to be used by philosophers of science than by scientists themselves). Researchers begin with a set of phenomena and either construct a theory to explain or interpret them or choose an existing theory to work with. They then make a prediction about some new phenomenon that should be observed if the theory is correct. Again, this prediction is called a hypothesis. The researchers then conduct an empirical study to test the hypothesis. Finally, they reevaluate the theory in light of the new results and revise it if necessary. This process is usually conceptualized as a cycle because the researchers can then derive a new hypothesis from the revised theory, conduct a new empirical study to test the hypothesis, and so on. As Figure 2.3 shows, this approach meshes nicely with the model of scientific research in psychology presented earlier in the textbook—creating a more detailed model of “theoretically motivated” or “theory-driven” research.
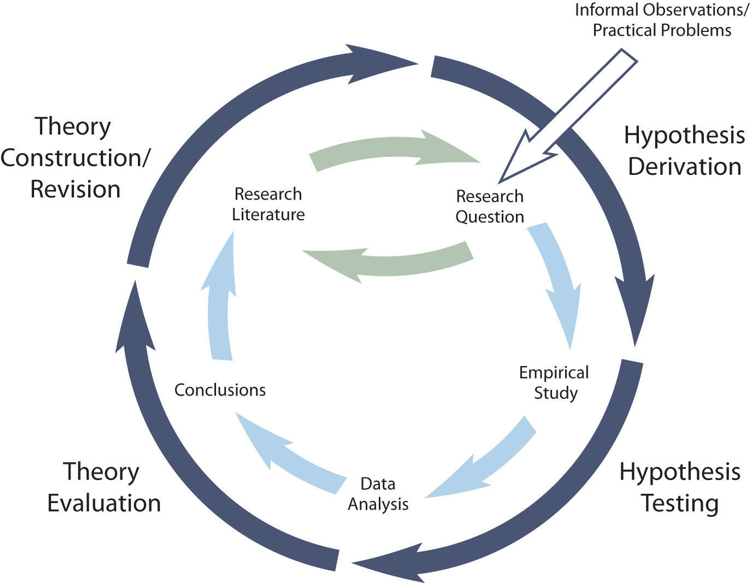
As an example, let us consider Zajonc’s research on social facilitation and inhibition. He started with a somewhat contradictory pattern of results from the research literature. He then constructed his drive theory, according to which being watched by others while performing a task causes physiological arousal, which increases an organism’s tendency to make the dominant response. This theory predicts social facilitation for well-learned tasks and social inhibition for poorly learned tasks. He now had a theory that organized previous results in a meaningful way—but he still needed to test it. He hypothesized that if his theory was correct, he should observe that the presence of others improves performance in a simple laboratory task but inhibits performance in a difficult version of the very same laboratory task. To test this hypothesis, one of the studies he conducted used cockroaches as subjects (Zajonc, Heingartner, & Herman, 1969) [3] . The cockroaches ran either down a straight runway (an easy task for a cockroach) or through a cross-shaped maze (a difficult task for a cockroach) to escape into a dark chamber when a light was shined on them. They did this either while alone or in the presence of other cockroaches in clear plastic “audience boxes.” Zajonc found that cockroaches in the straight runway reached their goal more quickly in the presence of other cockroaches, but cockroaches in the cross-shaped maze reached their goal more slowly when they were in the presence of other cockroaches. Thus he confirmed his hypothesis and provided support for his drive theory. (Zajonc also showed that drive theory existed in humans [Zajonc & Sales, 1966] [4] in many other studies afterward).
Incorporating Theory into Your Research
When you write your research report or plan your presentation, be aware that there are two basic ways that researchers usually include theory. The first is to raise a research question, answer that question by conducting a new study, and then offer one or more theories (usually more) to explain or interpret the results. This format works well for applied research questions and for research questions that existing theories do not address. The second way is to describe one or more existing theories, derive a hypothesis from one of those theories, test the hypothesis in a new study, and finally reevaluate the theory. This format works well when there is an existing theory that addresses the research question—especially if the resulting hypothesis is surprising or conflicts with a hypothesis derived from a different theory.
To use theories in your research will not only give you guidance in coming up with experiment ideas and possible projects, but it lends legitimacy to your work. Psychologists have been interested in a variety of human behaviors and have developed many theories along the way. Using established theories will help you break new ground as a researcher, not limit you from developing your own ideas.
Characteristics of a Good Hypothesis
There are three general characteristics of a good hypothesis. First, a good hypothesis must be testable and falsifiable . We must be able to test the hypothesis using the methods of science and if you’ll recall Popper’s falsifiability criterion, it must be possible to gather evidence that will disconfirm the hypothesis if it is indeed false. Second, a good hypothesis must be logical. As described above, hypotheses are more than just a random guess. Hypotheses should be informed by previous theories or observations and logical reasoning. Typically, we begin with a broad and general theory and use deductive reasoning to generate a more specific hypothesis to test based on that theory. Occasionally, however, when there is no theory to inform our hypothesis, we use inductive reasoning which involves using specific observations or research findings to form a more general hypothesis. Finally, the hypothesis should be positive. That is, the hypothesis should make a positive statement about the existence of a relationship or effect, rather than a statement that a relationship or effect does not exist. As scientists, we don’t set out to show that relationships do not exist or that effects do not occur so our hypotheses should not be worded in a way to suggest that an effect or relationship does not exist. The nature of science is to assume that something does not exist and then seek to find evidence to prove this wrong, to show that it really does exist. That may seem backward to you but that is the nature of the scientific method. The underlying reason for this is beyond the scope of this chapter but it has to do with statistical theory.
- Zajonc, R. B. (1965). Social facilitation. Science, 149 , 269–274 ↵
- Schwarz, N., Bless, H., Strack, F., Klumpp, G., Rittenauer-Schatka, H., & Simons, A. (1991). Ease of retrieval as information: Another look at the availability heuristic. Journal of Personality and Social Psychology, 61 , 195–202. ↵
- Zajonc, R. B., Heingartner, A., & Herman, E. M. (1969). Social enhancement and impairment of performance in the cockroach. Journal of Personality and Social Psychology, 13 , 83–92. ↵
- Zajonc, R.B. & Sales, S.M. (1966). Social facilitation of dominant and subordinate responses. Journal of Experimental Social Psychology, 2 , 160-168. ↵
A coherent explanation or interpretation of one or more phenomena.
A specific prediction about a new phenomenon that should be observed if a particular theory is accurate.
A cyclical process of theory development, starting with an observed phenomenon, then developing or using a theory to make a specific prediction of what should happen if that theory is correct, testing that prediction, refining the theory in light of the findings, and using that refined theory to develop new hypotheses, and so on.
The ability to test the hypothesis using the methods of science and the possibility to gather evidence that will disconfirm the hypothesis if it is indeed false.
Developing a Hypothesis Copyright © 2022 by Rajiv S. Jhangiani; I-Chant A. Chiang; Carrie Cuttler; and Dana C. Leighton is licensed under a Creative Commons Attribution-NonCommercial-ShareAlike 4.0 International License , except where otherwise noted.
Share This Book
Theories, Hypotheses, and Laws: Definitions, examples, and their roles in science
by Anthony Carpi, Ph.D., Anne E. Egger, Ph.D.
Listen to this reading
Did you know that the idea of evolution had been part of Western thought for more than 2,000 years before Charles Darwin was born? Like many theories, the theory of evolution was the result of the work of many different scientists working in different disciplines over a period of time.
A scientific theory is an explanation inferred from multiple lines of evidence for some broad aspect of the natural world and is logical, testable, and predictive.
As new evidence comes to light, or new interpretations of existing data are proposed, theories may be revised and even change; however, they are not tenuous or speculative.
A scientific hypothesis is an inferred explanation of an observation or research finding; while more exploratory in nature than a theory, it is based on existing scientific knowledge.
A scientific law is an expression of a mathematical or descriptive relationship observed in nature.
Imagine yourself shopping in a grocery store with a good friend who happens to be a chemist. Struggling to choose between the many different types of tomatoes in front of you, you pick one up, turn to your friend, and ask her if she thinks the tomato is organic . Your friend simply chuckles and replies, "Of course it's organic!" without even looking at how the fruit was grown. Why the amused reaction? Your friend is highlighting a simple difference in vocabulary. To a chemist, the term organic refers to any compound in which hydrogen is bonded to carbon. Tomatoes (like all plants) are abundant in organic compounds – thus your friend's laughter. In modern agriculture, however, organic has come to mean food items grown or raised without the use of chemical fertilizers, pesticides, or other additives.
So who is correct? You both are. Both uses of the word are correct, though they mean different things in different contexts. There are, of course, lots of words that have more than one meaning (like bat , for example), but multiple meanings can be especially confusing when two meanings convey very different ideas and are specific to one field of study.
- Scientific theories
The term theory also has two meanings, and this double meaning often leads to confusion. In common language, the term theory generally refers to speculation or a hunch or guess. You might have a theory about why your favorite sports team isn't playing well, or who ate the last cookie from the cookie jar. But these theories do not fit the scientific use of the term. In science, a theory is a well-substantiated and comprehensive set of ideas that explains a phenomenon in nature. A scientific theory is based on large amounts of data and observations that have been collected over time. Scientific theories can be tested and refined by additional research , and they allow scientists to make predictions. Though you may be correct in your hunch, your cookie jar conjecture doesn't fit this more rigorous definition.
All scientific disciplines have well-established, fundamental theories . For example, atomic theory describes the nature of matter and is supported by multiple lines of evidence from the way substances behave and react in the world around us (see our series on Atomic Theory ). Plate tectonic theory describes the large scale movement of the outer layer of the Earth and is supported by evidence from studies about earthquakes , magnetic properties of the rocks that make up the seafloor , and the distribution of volcanoes on Earth (see our series on Plate Tectonic Theory ). The theory of evolution by natural selection , which describes the mechanism by which inherited traits that affect survivability or reproductive success can cause changes in living organisms over generations , is supported by extensive studies of DNA , fossils , and other types of scientific evidence (see our Charles Darwin series for more information). Each of these major theories guides and informs modern research in those fields, integrating a broad, comprehensive set of ideas.
So how are these fundamental theories developed, and why are they considered so well supported? Let's take a closer look at some of the data and research supporting the theory of natural selection to better see how a theory develops.
Comprehension Checkpoint
- The development of a scientific theory: Evolution and natural selection
The theory of evolution by natural selection is sometimes maligned as Charles Darwin 's speculation on the origin of modern life forms. However, evolutionary theory is not speculation. While Darwin is rightly credited with first articulating the theory of natural selection, his ideas built on more than a century of scientific research that came before him, and are supported by over a century and a half of research since.
- The Fixity Notion: Linnaeus
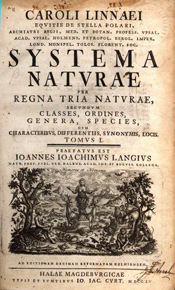
Figure 1: Cover of the 1760 edition of Systema Naturae .
Research about the origins and diversity of life proliferated in the 18th and 19th centuries. Carolus Linnaeus , a Swedish botanist and the father of modern taxonomy (see our module Taxonomy I for more information), was a devout Christian who believed in the concept of Fixity of Species , an idea based on the biblical story of creation. The Fixity of Species concept said that each species is based on an ideal form that has not changed over time. In the early stages of his career, Linnaeus traveled extensively and collected data on the structural similarities and differences between different species of plants. Noting that some very different plants had similar structures, he began to piece together his landmark work, Systema Naturae, in 1735 (Figure 1). In Systema , Linnaeus classified organisms into related groups based on similarities in their physical features. He developed a hierarchical classification system , even drawing relationships between seemingly disparate species (for example, humans, orangutans, and chimpanzees) based on the physical similarities that he observed between these organisms. Linnaeus did not explicitly discuss change in organisms or propose a reason for his hierarchy, but by grouping organisms based on physical characteristics, he suggested that species are related, unintentionally challenging the Fixity notion that each species is created in a unique, ideal form.
- The age of Earth: Leclerc and Hutton
Also in the early 1700s, Georges-Louis Leclerc, a French naturalist, and James Hutton , a Scottish geologist, began to develop new ideas about the age of the Earth. At the time, many people thought of the Earth as 6,000 years old, based on a strict interpretation of the events detailed in the Christian Old Testament by the influential Scottish Archbishop Ussher. By observing other planets and comets in the solar system , Leclerc hypothesized that Earth began as a hot, fiery ball of molten rock, mostly consisting of iron. Using the cooling rate of iron, Leclerc calculated that Earth must therefore be at least 70,000 years old in order to have reached its present temperature.
Hutton approached the same topic from a different perspective, gathering observations of the relationships between different rock formations and the rates of modern geological processes near his home in Scotland. He recognized that the relatively slow processes of erosion and sedimentation could not create all of the exposed rock layers in only a few thousand years (see our module The Rock Cycle ). Based on his extensive collection of data (just one of his many publications ran to 2,138 pages), Hutton suggested that the Earth was far older than human history – hundreds of millions of years old.
While we now know that both Leclerc and Hutton significantly underestimated the age of the Earth (by about 4 billion years), their work shattered long-held beliefs and opened a window into research on how life can change over these very long timescales.
- Fossil studies lead to the development of a theory of evolution: Cuvier
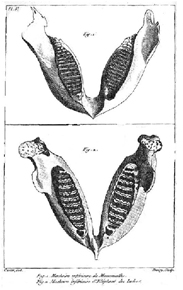
Figure 2: Illustration of an Indian elephant jaw and a mammoth jaw from Cuvier's 1796 paper.
With the age of Earth now extended by Leclerc and Hutton, more researchers began to turn their attention to studying past life. Fossils are the main way to study past life forms, and several key studies on fossils helped in the development of a theory of evolution . In 1795, Georges Cuvier began to work at the National Museum in Paris as a naturalist and anatomist. Through his work, Cuvier became interested in fossils found near Paris, which some claimed were the remains of the elephants that Hannibal rode over the Alps when he invaded Rome in 218 BCE . In studying both the fossils and living species , Cuvier documented different patterns in the dental structure and number of teeth between the fossils and modern elephants (Figure 2) (Horner, 1843). Based on these data , Cuvier hypothesized that the fossil remains were not left by Hannibal, but were from a distinct species of animal that once roamed through Europe and had gone extinct thousands of years earlier: the mammoth. The concept of species extinction had been discussed by a few individuals before Cuvier, but it was in direct opposition to the Fixity of Species concept – if every organism were based on a perfectly adapted, ideal form, how could any cease to exist? That would suggest it was no longer ideal.
While his work provided critical evidence of extinction , a key component of evolution , Cuvier was highly critical of the idea that species could change over time. As a result of his extensive studies of animal anatomy, Cuvier had developed a holistic view of organisms , stating that the
number, direction, and shape of the bones that compose each part of an animal's body are always in a necessary relation to all the other parts, in such a way that ... one can infer the whole from any one of them ...
In other words, Cuvier viewed each part of an organism as a unique, essential component of the whole organism. If one part were to change, he believed, the organism could not survive. His skepticism about the ability of organisms to change led him to criticize the whole idea of evolution , and his prominence in France as a scientist played a large role in discouraging the acceptance of the idea in the scientific community.
- Studies of invertebrates support a theory of change in species: Lamarck
Jean Baptiste Lamarck, a contemporary of Cuvier's at the National Museum in Paris, studied invertebrates like insects and worms. As Lamarck worked through the museum's large collection of invertebrates, he was impressed by the number and variety of organisms . He became convinced that organisms could, in fact, change through time, stating that
... time and favorable conditions are the two principal means which nature has employed in giving existence to all her productions. We know that for her time has no limit, and that consequently she always has it at her disposal.
This was a radical departure from both the fixity concept and Cuvier's ideas, and it built on the long timescale that geologists had recently established. Lamarck proposed that changes that occurred during an organism 's lifetime could be passed on to their offspring, suggesting, for example, that a body builder's muscles would be inherited by their children.
As it turned out, the mechanism by which Lamarck proposed that organisms change over time was wrong, and he is now often referred to disparagingly for his "inheritance of acquired characteristics" idea. Yet despite the fact that some of his ideas were discredited, Lamarck established a support for evolutionary theory that others would build on and improve.
- Rock layers as evidence for evolution: Smith
In the early 1800s, a British geologist and canal surveyor named William Smith added another component to the accumulating evidence for evolution . Smith observed that rock layers exposed in different parts of England bore similarities to one another: These layers (or strata) were arranged in a predictable order, and each layer contained distinct groups of fossils . From this series of observations , he developed a hypothesis that specific groups of animals followed one another in a definite sequence through Earth's history, and this sequence could be seen in the rock layers. Smith's hypothesis was based on his knowledge of geological principles , including the Law of Superposition.
The Law of Superposition states that sediments are deposited in a time sequence, with the oldest sediments deposited first, or at the bottom, and newer layers deposited on top. The concept was first expressed by the Persian scientist Avicenna in the 11th century, but was popularized by the Danish scientist Nicolas Steno in the 17th century. Note that the law does not state how sediments are deposited; it simply describes the relationship between the ages of deposited sediments.
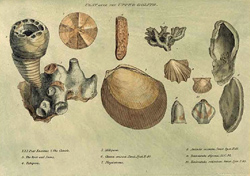
Figure 3: Engraving from William Smith's 1815 monograph on identifying strata by fossils.
Smith backed up his hypothesis with extensive drawings of fossils uncovered during his research (Figure 3), thus allowing other scientists to confirm or dispute his findings. His hypothesis has, in fact, been confirmed by many other scientists and has come to be referred to as the Law of Faunal Succession. His work was critical to the formation of evolutionary theory as it not only confirmed Cuvier's work that organisms have gone extinct , but it also showed that the appearance of life does not date to the birth of the planet. Instead, the fossil record preserves a timeline of the appearance and disappearance of different organisms in the past, and in doing so offers evidence for change in organisms over time.
- The theory of evolution by natural selection: Darwin and Wallace
It was into this world that Charles Darwin entered: Linnaeus had developed a taxonomy of organisms based on their physical relationships, Leclerc and Hutton demonstrated that there was sufficient time in Earth's history for organisms to change, Cuvier showed that species of organisms have gone extinct , Lamarck proposed that organisms change over time, and Smith established a timeline of the appearance and disappearance of different organisms in the geological record .
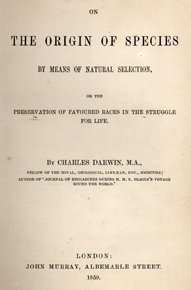
Figure 4: Title page of the 1859 Murray edition of the Origin of Species by Charles Darwin.
Charles Darwin collected data during his work as a naturalist on the HMS Beagle starting in 1831. He took extensive notes on the geology of the places he visited; he made a major find of fossils of extinct animals in Patagonia and identified an extinct giant ground sloth named Megatherium . He experienced an earthquake in Chile that stranded beds of living mussels above water, where they would be preserved for years to come.
Perhaps most famously, he conducted extensive studies of animals on the Galápagos Islands, noting subtle differences in species of mockingbird, tortoise, and finch that were isolated on different islands with different environmental conditions. These subtle differences made the animals highly adapted to their environments .
This broad spectrum of data led Darwin to propose an idea about how organisms change "by means of natural selection" (Figure 4). But this idea was not based only on his work, it was also based on the accumulation of evidence and ideas of many others before him. Because his proposal encompassed and explained many different lines of evidence and previous work, they formed the basis of a new and robust scientific theory regarding change in organisms – the theory of evolution by natural selection .
Darwin's ideas were grounded in evidence and data so compelling that if he had not conceived them, someone else would have. In fact, someone else did. Between 1858 and 1859, Alfred Russel Wallace , a British naturalist, wrote a series of letters to Darwin that independently proposed natural selection as the means for evolutionary change. The letters were presented to the Linnean Society of London, a prominent scientific society at the time (see our module on Scientific Institutions and Societies ). This long chain of research highlights that theories are not just the work of one individual. At the same time, however, it often takes the insight and creativity of individuals to put together all of the pieces and propose a new theory . Both Darwin and Wallace were experienced naturalists who were familiar with the work of others. While all of the work leading up to 1830 contributed to the theory of evolution , Darwin's and Wallace's theory changed the way that future research was focused by presenting a comprehensive, well-substantiated set of ideas, thus becoming a fundamental theory of biological research.
- Expanding, testing, and refining scientific theories
- Genetics and evolution: Mendel and Dobzhansky
Since Darwin and Wallace first published their ideas, extensive research has tested and expanded the theory of evolution by natural selection . Darwin had no concept of genes or DNA or the mechanism by which characteristics were inherited within a species . A contemporary of Darwin's, the Austrian monk Gregor Mendel , first presented his own landmark study, Experiments in Plant Hybridization, in 1865 in which he provided the basic patterns of genetic inheritance , describing which characteristics (and evolutionary changes) can be passed on in organisms (see our Genetics I module for more information). Still, it wasn't until much later that a "gene" was defined as the heritable unit.
In 1937, the Ukrainian born geneticist Theodosius Dobzhansky published Genetics and the Origin of Species , a seminal work in which he described genes themselves and demonstrated that it is through mutations in genes that change occurs. The work defined evolution as "a change in the frequency of an allele within a gene pool" ( Dobzhansky, 1982 ). These studies and others in the field of genetics have added to Darwin's work, expanding the scope of the theory .
- Evolution under a microscope: Lenski
More recently, Dr. Richard Lenski, a scientist at Michigan State University, isolated a single Escherichia coli bacterium in 1989 as the first step of the longest running experimental test of evolutionary theory to date – a true test meant to replicate evolution and natural selection in the lab.
After the single microbe had multiplied, Lenski isolated the offspring into 12 different strains , each in their own glucose-supplied culture, predicting that the genetic make-up of each strain would change over time to become more adapted to their specific culture as predicted by evolutionary theory . These 12 lines have been nurtured for over 40,000 bacterial generations (luckily bacterial generations are much shorter than human generations) and exposed to different selective pressures such as heat , cold, antibiotics, and infection with other microorganisms. Lenski and colleagues have studied dozens of aspects of evolutionary theory with these genetically isolated populations . In 1999, they published a paper that demonstrated that random genetic mutations were common within the populations and highly diverse across different individual bacteria . However, "pivotal" mutations that are associated with beneficial changes in the group are shared by all descendants in a population and are much rarer than random mutations, as predicted by the theory of evolution by natural selection (Papadopoulos et al., 1999).
- Punctuated equilibrium: Gould and Eldredge
While established scientific theories like evolution have a wealth of research and evidence supporting them, this does not mean that they cannot be refined as new information or new perspectives on existing data become available. For example, in 1972, biologist Stephen Jay Gould and paleontologist Niles Eldredge took a fresh look at the existing data regarding the timing by which evolutionary change takes place. Gould and Eldredge did not set out to challenge the theory of evolution; rather they used it as a guiding principle and asked more specific questions to add detail and nuance to the theory. This is true of all theories in science: they provide a framework for additional research. At the time, many biologists viewed evolution as occurring gradually, causing small incremental changes in organisms at a relatively steady rate. The idea is referred to as phyletic gradualism , and is rooted in the geological concept of uniformitarianism . After reexamining the available data, Gould and Eldredge came to a different explanation, suggesting that evolution consists of long periods of stability that are punctuated by occasional instances of dramatic change – a process they called punctuated equilibrium .
Like Darwin before them, their proposal is rooted in evidence and research on evolutionary change, and has been supported by multiple lines of evidence. In fact, punctuated equilibrium is now considered its own theory in evolutionary biology. Punctuated equilibrium is not as broad of a theory as natural selection . In science, some theories are broad and overarching of many concepts, such as the theory of evolution by natural selection; others focus on concepts at a smaller, or more targeted, scale such as punctuated equilibrium. And punctuated equilibrium does not challenge or weaken the concept of natural selection; rather, it represents a change in our understanding of the timing by which change occurs in organisms , and a theory within a theory. The theory of evolution by natural selection now includes both gradualism and punctuated equilibrium to describe the rate at which change proceeds.
- Hypotheses and laws: Other scientific concepts
One of the challenges in understanding scientific terms like theory is that there is not a precise definition even within the scientific community. Some scientists debate over whether certain proposals merit designation as a hypothesis or theory , and others mistakenly use the terms interchangeably. But there are differences in these terms. A hypothesis is a proposed explanation for an observable phenomenon. Hypotheses , just like theories , are based on observations from research . For example, LeClerc did not hypothesize that Earth had cooled from a molten ball of iron as a random guess; rather, he developed this hypothesis based on his observations of information from meteorites.
A scientist often proposes a hypothesis before research confirms it as a way of predicting the outcome of study to help better define the parameters of the research. LeClerc's hypothesis allowed him to use known parameters (the cooling rate of iron) to do additional work. A key component of a formal scientific hypothesis is that it is testable and falsifiable. For example, when Richard Lenski first isolated his 12 strains of bacteria , he likely hypothesized that random mutations would cause differences to appear within a period of time in the different strains of bacteria. But when a hypothesis is generated in science, a scientist will also make an alternative hypothesis , an explanation that explains a study if the data do not support the original hypothesis. If the different strains of bacteria in Lenski's work did not diverge over the indicated period of time, perhaps the rate of mutation was slower than first thought.
So you might ask, if theories are so well supported, do they eventually become laws? The answer is no – not because they aren't well-supported, but because theories and laws are two very different things. Laws describe phenomena, often mathematically. Theories, however, explain phenomena. For example, in 1687 Isaac Newton proposed a Theory of Gravitation, describing gravity as a force of attraction between two objects. As part of this theory, Newton developed a Law of Universal Gravitation that explains how this force operates. This law states that the force of gravity between two objects is inversely proportional to the square of the distance between those objects. Newton 's Law does not explain why this is true, but it describes how gravity functions (see our Gravity: Newtonian Relationships module for more detail). In 1916, Albert Einstein developed his theory of general relativity to explain the mechanism by which gravity has its effect. Einstein's work challenges Newton's theory, and has been found after extensive testing and research to more accurately describe the phenomenon of gravity. While Einstein's work has replaced Newton's as the dominant explanation of gravity in modern science, Newton's Law of Universal Gravitation is still used as it reasonably (and more simply) describes the force of gravity under many conditions. Similarly, the Law of Faunal Succession developed by William Smith does not explain why organisms follow each other in distinct, predictable ways in the rock layers, but it accurately describes the phenomenon.
Theories, hypotheses , and laws drive scientific progress
Theories, hypotheses , and laws are not simply important components of science, they drive scientific progress. For example, evolutionary biology now stands as a distinct field of science that focuses on the origins and descent of species . Geologists now rely on plate tectonics as a conceptual model and guiding theory when they are studying processes at work in Earth's crust . And physicists refer to atomic theory when they are predicting the existence of subatomic particles yet to be discovered. This does not mean that science is "finished," or that all of the important theories have been discovered already. Like evolution , progress in science happens both gradually and in short, dramatic bursts. Both types of progress are critical for creating a robust knowledge base with data as the foundation and scientific theories giving structure to that knowledge.
Table of Contents
- Theories, hypotheses, and laws drive scientific progress
Activate glossary term highlighting to easily identify key terms within the module. Once highlighted, you can click on these terms to view their definitions.
Activate NGSS annotations to easily identify NGSS standards within the module. Once highlighted, you can click on them to view these standards.
Psychological Research
The scientific process, learning objectives.
- Explain the steps of the scientific method
- Differentiate between theories and hypotheses
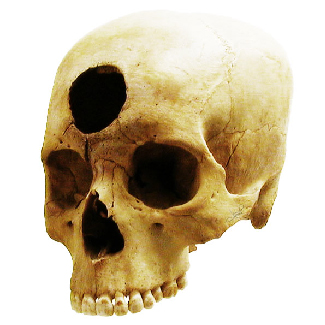
Figure 1 . Some of our ancestors, across the world and over the centuries, believed that trephination—the practice of making a hole in the skull, as shown here—allowed evil spirits to leave the body, thus curing mental illness and other disorders. (credit: “taiproject”/Flickr)
The goal of all scientists is to better understand the world around them. Psychologists focus their attention on understanding behavior, as well as the cognitive (mental) and physiological (body) processes that underlie behavior. In contrast to other methods that people use to understand the behavior of others, such as intuition and personal experience, the hallmark of scientific research is that there is evidence to support a claim. Scientific knowledge is empirical : It is grounded in objective, tangible evidence that can be observed time and time again, regardless of who is observing.
While behavior is observable, the mind is not. If someone is crying, we can see the behavior. However, the reason for the behavior is more difficult to determine. Is the person crying due to being sad, in pain, or happy? Sometimes we can learn the reason for someone’s behavior by simply asking a question, like “Why are you crying?” However, there are situations in which an individual is either uncomfortable or unwilling to answer the question honestly, or is incapable of answering. For example, infants would not be able to explain why they are crying. In such circumstances, the psychologist must be creative in finding ways to better understand behavior. This module explores how scientific knowledge is generated, and how important that knowledge is in forming decisions in our personal lives and in the public domain.
Process of Scientific Research
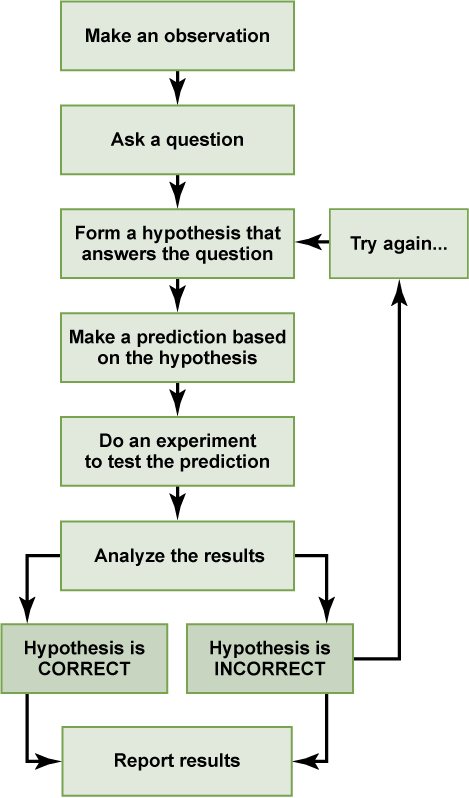
Figure 2 . The scientific method is a process for gathering data and processing information. It provides well-defined steps to standardize how scientific knowledge is gathered through a logical, rational problem-solving method.
Scientific knowledge is advanced through a process known as the scientific method. Basically, ideas (in the form of theories and hypotheses) are tested against the real world (in the form of empirical observations), and those empirical observations lead to more ideas that are tested against the real world, and so on.
The basic steps in the scientific method are:
- Observe a natural phenomenon and define a question about it
- Make a hypothesis, or potential solution to the question
- Test the hypothesis
- If the hypothesis is true, find more evidence or find counter-evidence
- If the hypothesis is false, create a new hypothesis or try again
- Draw conclusions and repeat–the scientific method is never-ending, and no result is ever considered perfect
In order to ask an important question that may improve our understanding of the world, a researcher must first observe natural phenomena. By making observations, a researcher can define a useful question. After finding a question to answer, the researcher can then make a prediction (a hypothesis) about what they think the answer will be. This prediction is usually a statement about the relationship between two or more variables. After making a hypothesis, the researcher will then design an experiment to test their hypothesis and evaluate the data gathered. These data will either support or refute the hypothesis. Based on the conclusions drawn from the data, the researcher will then find more evidence to support the hypothesis, look for counter-evidence to further strengthen the hypothesis, revise the hypothesis and create a new experiment, or continue to incorporate the information gathered to answer the research question.
Basic Principles of the Scientific Method
Two key concepts in the scientific approach are theory and hypothesis. A theory is a well-developed set of ideas that propose an explanation for observed phenomena that can be used to make predictions about future observations. A hypothesis is a testable prediction that is arrived at logically from a theory. It is often worded as an if-then statement (e.g., if I study all night, I will get a passing grade on the test). The hypothesis is extremely important because it bridges the gap between the realm of ideas and the real world. As specific hypotheses are tested, theories are modified and refined to reflect and incorporate the result of these tests.
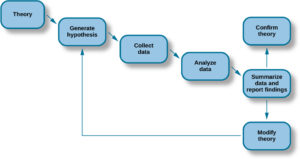
Figure 3 . The scientific method involves deriving hypotheses from theories and then testing those hypotheses. If the results are consistent with the theory, then the theory is supported. If the results are not consistent, then the theory should be modified and new hypotheses will be generated.
Other key components in following the scientific method include verifiability, predictability, falsifiability, and fairness. Verifiability means that an experiment must be replicable by another researcher. To achieve verifiability, researchers must make sure to document their methods and clearly explain how their experiment is structured and why it produces certain results.
Predictability in a scientific theory implies that the theory should enable us to make predictions about future events. The precision of these predictions is a measure of the strength of the theory.
Falsifiability refers to whether a hypothesis can be disproved. For a hypothesis to be falsifiable, it must be logically possible to make an observation or do a physical experiment that would show that there is no support for the hypothesis. Even when a hypothesis cannot be shown to be false, that does not necessarily mean it is not valid. Future testing may disprove the hypothesis. This does not mean that a hypothesis has to be shown to be false, just that it can be tested.
To determine whether a hypothesis is supported or not supported, psychological researchers must conduct hypothesis testing using statistics. Hypothesis testing is a type of statistics that determines the probability of a hypothesis being true or false. If hypothesis testing reveals that results were “statistically significant,” this means that there was support for the hypothesis and that the researchers can be reasonably confident that their result was not due to random chance. If the results are not statistically significant, this means that the researchers’ hypothesis was not supported.
Fairness implies that all data must be considered when evaluating a hypothesis. A researcher cannot pick and choose what data to keep and what to discard or focus specifically on data that support or do not support a particular hypothesis. All data must be accounted for, even if they invalidate the hypothesis.
Applying the Scientific Method
To see how this process works, let’s consider a specific theory and a hypothesis that might be generated from that theory. As you’ll learn in a later module, the James-Lange theory of emotion asserts that emotional experience relies on the physiological arousal associated with the emotional state. If you walked out of your home and discovered a very aggressive snake waiting on your doorstep, your heart would begin to race and your stomach churn. According to the James-Lange theory, these physiological changes would result in your feeling of fear. A hypothesis that could be derived from this theory might be that a person who is unaware of the physiological arousal that the sight of the snake elicits will not feel fear.
Remember that a good scientific hypothesis is falsifiable, or capable of being shown to be incorrect. Recall from the introductory module that Sigmund Freud had lots of interesting ideas to explain various human behaviors. However, a major criticism of Freud’s theories is that many of his ideas are not falsifiable; for example, it is impossible to imagine empirical observations that would disprove the existence of the id, the ego, and the superego—the three elements of personality described in Freud’s theories. Despite this, Freud’s theories are widely taught in introductory psychology texts because of their historical significance for personality psychology and psychotherapy, and these remain the root of all modern forms of therapy.
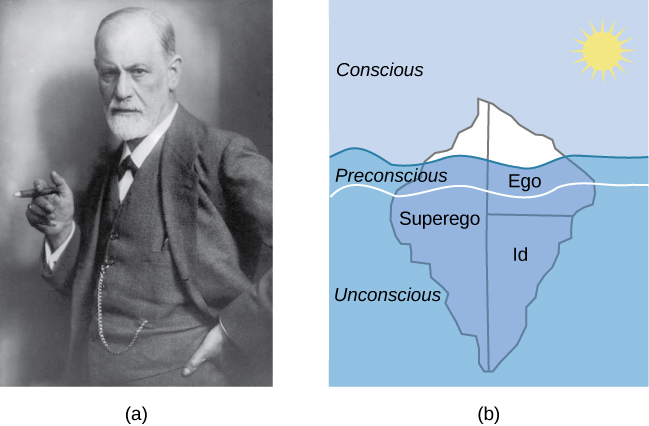
Figure 4 . Many of the specifics of (a) Freud’s theories, such as (b) his division of the mind into id, ego, and superego, have fallen out of favor in recent decades because they are not falsifiable. In broader strokes, his views set the stage for much of psychological thinking today, such as the unconscious nature of the majority of psychological processes.
In contrast, the James-Lange theory does generate falsifiable hypotheses, such as the one described above. Some individuals who suffer significant injuries to their spinal columns are unable to feel the bodily changes that often accompany emotional experiences. Therefore, we could test the hypothesis by determining how emotional experiences differ between individuals who have the ability to detect these changes in their physiological arousal and those who do not. In fact, this research has been conducted and while the emotional experiences of people deprived of an awareness of their physiological arousal may be less intense, they still experience emotion (Chwalisz, Diener, & Gallagher, 1988).
Link to Learning
Want to participate in a study? Visit this Psychological Research on the Net website and click on a link that sounds interesting to you in order to participate in online research.
Why the Scientific Method Is Important for Psychology
The use of the scientific method is one of the main features that separates modern psychology from earlier philosophical inquiries about the mind. Compared to chemistry, physics, and other “natural sciences,” psychology has long been considered one of the “social sciences” because of the subjective nature of the things it seeks to study. Many of the concepts that psychologists are interested in—such as aspects of the human mind, behavior, and emotions—are subjective and cannot be directly measured. Psychologists often rely instead on behavioral observations and self-reported data, which are considered by some to be illegitimate or lacking in methodological rigor. Applying the scientific method to psychology, therefore, helps to standardize the approach to understanding its very different types of information.
The scientific method allows psychological data to be replicated and confirmed in many instances, under different circumstances, and by a variety of researchers. Through replication of experiments, new generations of psychologists can reduce errors and broaden the applicability of theories. It also allows theories to be tested and validated instead of simply being conjectures that could never be verified or falsified. All of this allows psychologists to gain a stronger understanding of how the human mind works.
Scientific articles published in journals and psychology papers written in the style of the American Psychological Association (i.e., in “APA style”) are structured around the scientific method. These papers include an introduction, which introduces the background information and outlines the hypotheses; a methods section, which outlines the specifics of how the experiment was conducted to test the hypothesis; a results section, which includes the statistics that tested the hypothesis and state whether it was supported or not supported, and a discussion and conclusion, which state the implications of finding support for, or no support for, the hypothesis. Writing articles and papers that adhere to the scientific method makes it easy for future researchers to repeat the study and attempt to replicate the results.
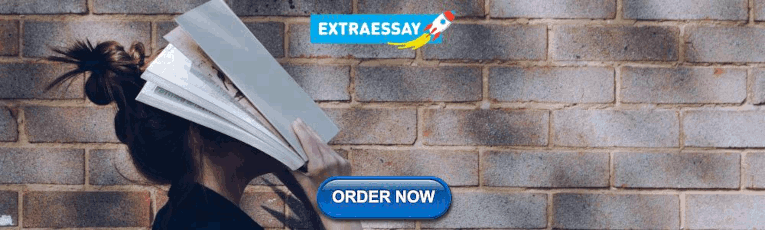
Notable Researchers
Psychological research has a long history involving important figures from diverse backgrounds. While the introductory module discussed several researchers who made significant contributions to the discipline, there are many more individuals who deserve attention in considering how psychology has advanced as a science through their work. For instance, Margaret Floy Washburn (1871–1939) was the first woman to earn a PhD in psychology. Her research focused on animal behavior and cognition (Margaret Floy Washburn, PhD, n.d.). Mary Whiton Calkins (1863–1930) was a preeminent first-generation American psychologist who opposed the behaviorist movement, conducted significant research into memory, and established one of the earliest experimental psychology labs in the United States (Mary Whiton Calkins, n.d.).
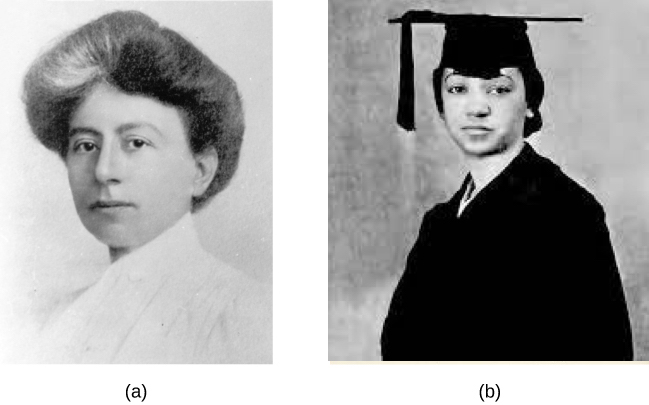
Figure 5 . (a) Margaret Floy Washburn was the first woman to earn a doctorate degree in psychology. (b) Psychologist Inez Beverly Prosser, who was the first African American woman to earn a PhD in psychology.
Francis Sumner (1895–1954) was the first African American to receive a PhD in psychology in 1920. His dissertation focused on issues related to psychoanalysis. Sumner also had research interests in racial bias and educational justice. Sumner was one of the founders of Howard University’s department of psychology, and because of his accomplishments, he is sometimes referred to as the “Father of Black Psychology.” Thirteen years later, Inez Beverly Prosser (1895–1934) became the first African American woman to receive a PhD in psychology. Prosser’s research highlighted issues related to education in segregated versus integrated schools, and ultimately, her work was very influential in the hallmark Brown v. Board of Education Supreme Court ruling that segregation of public schools was unconstitutional (Ethnicity and Health in America Series: Featured Psychologists, n.d.).
Although the establishment of psychology’s scientific roots occurred first in Europe and the United States, it did not take much time until researchers from around the world began to establish their own laboratories and research programs. For example, some of the first experimental psychology laboratories in South America were founded by Horatio Piñero (1869–1919) at two institutions in Buenos Aires, Argentina (Godoy & Brussino, 2010). In India, Gunamudian David Boaz (1908–1965) and Narendra Nath Sen Gupta (1889–1944) established the first independent departments of psychology at the University of Madras and the University of Calcutta, respectively. These developments provided an opportunity for Indian researchers to make important contributions to the field (Gunamudian David Boaz, n.d.; Narendra Nath Sen Gupta, n.d.).
When the American Psychological Association (APA) was first founded in 1892, all of the members were white males. However, by 1905, Mary Whiton Calkins was elected as the first female president of the APA, and by 1946, nearly one-quarter of American psychologists were female. Psychology became a popular degree option for students enrolled in the nation’s historically black higher education institutions, increasing the number of black Americans who went on to become psychologists. Given demographic shifts occurring in the United States and increased access to higher educational opportunities among historically underrepresented populations, there is reason to hope that the diversity of the field will increasingly match the larger population, and that the research contributions made by the psychologists of the future will better serve people of all backgrounds (Women and Minorities in Psychology, n.d.).
Contribute!
Improve this page Learn More
- Modification and adaptation. Provided by : Lumen Learning. License : CC BY-SA: Attribution-ShareAlike
- Why is Research Important?. Authored by : OpenStax College. Located at : https://openstax.org/books/psychology-2e/pages/2-1-why-is-research-important . License : CC BY: Attribution . License Terms : Download for free at https://openstax.org/books/psychology-2e/pages/1-introduction
- Psychology and the Scientific Method: From Theory to Conclusion, content on the scientific method principles. Provided by : Boundless. Located at : https://www.boundless.com/psychology/textbooks/boundless-psychology-textbook/researching-psychology-2/the-scientific-method-26/psychology-and-the-scientific-method-from-theory-to-conclusion-123-12658/images/the-scientific-method/ . License : CC BY-SA: Attribution-ShareAlike
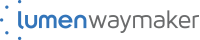
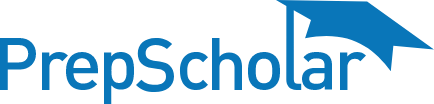
Choose Your Test
Sat / act prep online guides and tips, what is a hypothesis and how do i write one.
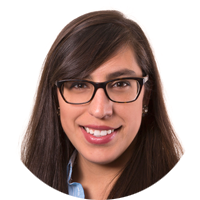
General Education
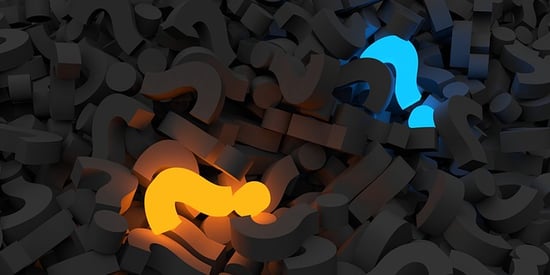
Think about something strange and unexplainable in your life. Maybe you get a headache right before it rains, or maybe you think your favorite sports team wins when you wear a certain color. If you wanted to see whether these are just coincidences or scientific fact, you would form a hypothesis, then create an experiment to see whether that hypothesis is true or not.
But what is a hypothesis, anyway? If you’re not sure about what a hypothesis is--or how to test for one!--you’re in the right place. This article will teach you everything you need to know about hypotheses, including:
- Defining the term “hypothesis”
- Providing hypothesis examples
- Giving you tips for how to write your own hypothesis
So let’s get started!
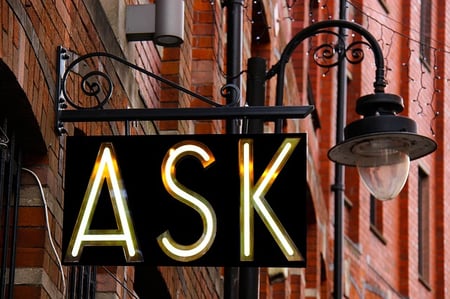
What Is a Hypothesis?
Merriam Webster defines a hypothesis as “an assumption or concession made for the sake of argument.” In other words, a hypothesis is an educated guess . Scientists make a reasonable assumption--or a hypothesis--then design an experiment to test whether it’s true or not. Keep in mind that in science, a hypothesis should be testable. You have to be able to design an experiment that tests your hypothesis in order for it to be valid.
As you could assume from that statement, it’s easy to make a bad hypothesis. But when you’re holding an experiment, it’s even more important that your guesses be good...after all, you’re spending time (and maybe money!) to figure out more about your observation. That’s why we refer to a hypothesis as an educated guess--good hypotheses are based on existing data and research to make them as sound as possible.
Hypotheses are one part of what’s called the scientific method . Every (good) experiment or study is based in the scientific method. The scientific method gives order and structure to experiments and ensures that interference from scientists or outside influences does not skew the results. It’s important that you understand the concepts of the scientific method before holding your own experiment. Though it may vary among scientists, the scientific method is generally made up of six steps (in order):
- Observation
- Asking questions
- Forming a hypothesis
- Analyze the data
- Communicate your results
You’ll notice that the hypothesis comes pretty early on when conducting an experiment. That’s because experiments work best when they’re trying to answer one specific question. And you can’t conduct an experiment until you know what you’re trying to prove!
Independent and Dependent Variables
After doing your research, you’re ready for another important step in forming your hypothesis: identifying variables. Variables are basically any factor that could influence the outcome of your experiment . Variables have to be measurable and related to the topic being studied.
There are two types of variables: independent variables and dependent variables. I ndependent variables remain constant . For example, age is an independent variable; it will stay the same, and researchers can look at different ages to see if it has an effect on the dependent variable.
Speaking of dependent variables... dependent variables are subject to the influence of the independent variable , meaning that they are not constant. Let’s say you want to test whether a person’s age affects how much sleep they need. In that case, the independent variable is age (like we mentioned above), and the dependent variable is how much sleep a person gets.
Variables will be crucial in writing your hypothesis. You need to be able to identify which variable is which, as both the independent and dependent variables will be written into your hypothesis. For instance, in a study about exercise, the independent variable might be the speed at which the respondents walk for thirty minutes, and the dependent variable would be their heart rate. In your study and in your hypothesis, you’re trying to understand the relationship between the two variables.
Elements of a Good Hypothesis
The best hypotheses start by asking the right questions . For instance, if you’ve observed that the grass is greener when it rains twice a week, you could ask what kind of grass it is, what elevation it’s at, and if the grass across the street responds to rain in the same way. Any of these questions could become the backbone of experiments to test why the grass gets greener when it rains fairly frequently.
As you’re asking more questions about your first observation, make sure you’re also making more observations . If it doesn’t rain for two weeks and the grass still looks green, that’s an important observation that could influence your hypothesis. You'll continue observing all throughout your experiment, but until the hypothesis is finalized, every observation should be noted.
Finally, you should consult secondary research before writing your hypothesis . Secondary research is comprised of results found and published by other people. You can usually find this information online or at your library. Additionally, m ake sure the research you find is credible and related to your topic. If you’re studying the correlation between rain and grass growth, it would help you to research rain patterns over the past twenty years for your county, published by a local agricultural association. You should also research the types of grass common in your area, the type of grass in your lawn, and whether anyone else has conducted experiments about your hypothesis. Also be sure you’re checking the quality of your research . Research done by a middle school student about what minerals can be found in rainwater would be less useful than an article published by a local university.
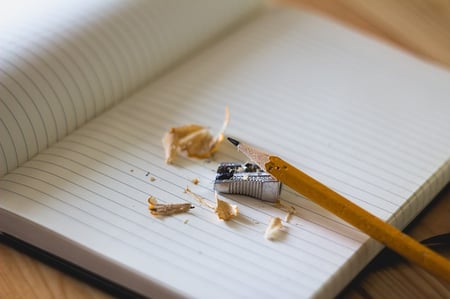
Writing Your Hypothesis
Once you’ve considered all of the factors above, you’re ready to start writing your hypothesis. Hypotheses usually take a certain form when they’re written out in a research report.
When you boil down your hypothesis statement, you are writing down your best guess and not the question at hand . This means that your statement should be written as if it is fact already, even though you are simply testing it.
The reason for this is that, after you have completed your study, you'll either accept or reject your if-then or your null hypothesis. All hypothesis testing examples should be measurable and able to be confirmed or denied. You cannot confirm a question, only a statement!
In fact, you come up with hypothesis examples all the time! For instance, when you guess on the outcome of a basketball game, you don’t say, “Will the Miami Heat beat the Boston Celtics?” but instead, “I think the Miami Heat will beat the Boston Celtics.” You state it as if it is already true, even if it turns out you’re wrong. You do the same thing when writing your hypothesis.
Additionally, keep in mind that hypotheses can range from very specific to very broad. These hypotheses can be specific, but if your hypothesis testing examples involve a broad range of causes and effects, your hypothesis can also be broad.
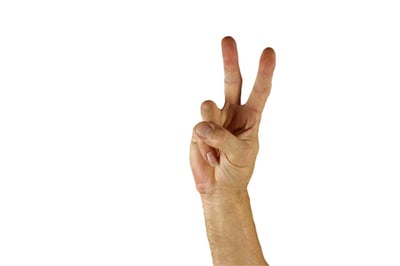
The Two Types of Hypotheses
Now that you understand what goes into a hypothesis, it’s time to look more closely at the two most common types of hypothesis: the if-then hypothesis and the null hypothesis.
#1: If-Then Hypotheses
First of all, if-then hypotheses typically follow this formula:
If ____ happens, then ____ will happen.
The goal of this type of hypothesis is to test the causal relationship between the independent and dependent variable. It’s fairly simple, and each hypothesis can vary in how detailed it can be. We create if-then hypotheses all the time with our daily predictions. Here are some examples of hypotheses that use an if-then structure from daily life:
- If I get enough sleep, I’ll be able to get more work done tomorrow.
- If the bus is on time, I can make it to my friend’s birthday party.
- If I study every night this week, I’ll get a better grade on my exam.
In each of these situations, you’re making a guess on how an independent variable (sleep, time, or studying) will affect a dependent variable (the amount of work you can do, making it to a party on time, or getting better grades).
You may still be asking, “What is an example of a hypothesis used in scientific research?” Take one of the hypothesis examples from a real-world study on whether using technology before bed affects children’s sleep patterns. The hypothesis read s:
“We hypothesized that increased hours of tablet- and phone-based screen time at bedtime would be inversely correlated with sleep quality and child attention.”
It might not look like it, but this is an if-then statement. The researchers basically said, “If children have more screen usage at bedtime, then their quality of sleep and attention will be worse.” The sleep quality and attention are the dependent variables and the screen usage is the independent variable. (Usually, the independent variable comes after the “if” and the dependent variable comes after the “then,” as it is the independent variable that affects the dependent variable.) This is an excellent example of how flexible hypothesis statements can be, as long as the general idea of “if-then” and the independent and dependent variables are present.
#2: Null Hypotheses
Your if-then hypothesis is not the only one needed to complete a successful experiment, however. You also need a null hypothesis to test it against. In its most basic form, the null hypothesis is the opposite of your if-then hypothesis . When you write your null hypothesis, you are writing a hypothesis that suggests that your guess is not true, and that the independent and dependent variables have no relationship .
One null hypothesis for the cell phone and sleep study from the last section might say:
“If children have more screen usage at bedtime, their quality of sleep and attention will not be worse.”
In this case, this is a null hypothesis because it’s asking the opposite of the original thesis!
Conversely, if your if-then hypothesis suggests that your two variables have no relationship, then your null hypothesis would suggest that there is one. So, pretend that there is a study that is asking the question, “Does the amount of followers on Instagram influence how long people spend on the app?” The independent variable is the amount of followers, and the dependent variable is the time spent. But if you, as the researcher, don’t think there is a relationship between the number of followers and time spent, you might write an if-then hypothesis that reads:
“If people have many followers on Instagram, they will not spend more time on the app than people who have less.”
In this case, the if-then suggests there isn’t a relationship between the variables. In that case, one of the null hypothesis examples might say:
“If people have many followers on Instagram, they will spend more time on the app than people who have less.”
You then test both the if-then and the null hypothesis to gauge if there is a relationship between the variables, and if so, how much of a relationship.
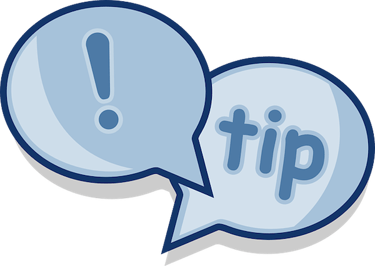
4 Tips to Write the Best Hypothesis
If you’re going to take the time to hold an experiment, whether in school or by yourself, you’re also going to want to take the time to make sure your hypothesis is a good one. The best hypotheses have four major elements in common: plausibility, defined concepts, observability, and general explanation.
#1: Plausibility
At first glance, this quality of a hypothesis might seem obvious. When your hypothesis is plausible, that means it’s possible given what we know about science and general common sense. However, improbable hypotheses are more common than you might think.
Imagine you’re studying weight gain and television watching habits. If you hypothesize that people who watch more than twenty hours of television a week will gain two hundred pounds or more over the course of a year, this might be improbable (though it’s potentially possible). Consequently, c ommon sense can tell us the results of the study before the study even begins.
Improbable hypotheses generally go against science, as well. Take this hypothesis example:
“If a person smokes one cigarette a day, then they will have lungs just as healthy as the average person’s.”
This hypothesis is obviously untrue, as studies have shown again and again that cigarettes negatively affect lung health. You must be careful that your hypotheses do not reflect your own personal opinion more than they do scientifically-supported findings. This plausibility points to the necessity of research before the hypothesis is written to make sure that your hypothesis has not already been disproven.
#2: Defined Concepts
The more advanced you are in your studies, the more likely that the terms you’re using in your hypothesis are specific to a limited set of knowledge. One of the hypothesis testing examples might include the readability of printed text in newspapers, where you might use words like “kerning” and “x-height.” Unless your readers have a background in graphic design, it’s likely that they won’t know what you mean by these terms. Thus, it’s important to either write what they mean in the hypothesis itself or in the report before the hypothesis.
Here’s what we mean. Which of the following sentences makes more sense to the common person?
If the kerning is greater than average, more words will be read per minute.
If the space between letters is greater than average, more words will be read per minute.
For people reading your report that are not experts in typography, simply adding a few more words will be helpful in clarifying exactly what the experiment is all about. It’s always a good idea to make your research and findings as accessible as possible.
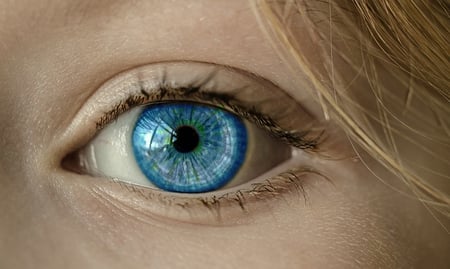
Good hypotheses ensure that you can observe the results.
#3: Observability
In order to measure the truth or falsity of your hypothesis, you must be able to see your variables and the way they interact. For instance, if your hypothesis is that the flight patterns of satellites affect the strength of certain television signals, yet you don’t have a telescope to view the satellites or a television to monitor the signal strength, you cannot properly observe your hypothesis and thus cannot continue your study.
Some variables may seem easy to observe, but if you do not have a system of measurement in place, you cannot observe your hypothesis properly. Here’s an example: if you’re experimenting on the effect of healthy food on overall happiness, but you don’t have a way to monitor and measure what “overall happiness” means, your results will not reflect the truth. Monitoring how often someone smiles for a whole day is not reasonably observable, but having the participants state how happy they feel on a scale of one to ten is more observable.
In writing your hypothesis, always keep in mind how you'll execute the experiment.
#4: Generalizability
Perhaps you’d like to study what color your best friend wears the most often by observing and documenting the colors she wears each day of the week. This might be fun information for her and you to know, but beyond you two, there aren’t many people who could benefit from this experiment. When you start an experiment, you should note how generalizable your findings may be if they are confirmed. Generalizability is basically how common a particular phenomenon is to other people’s everyday life.
Let’s say you’re asking a question about the health benefits of eating an apple for one day only, you need to realize that the experiment may be too specific to be helpful. It does not help to explain a phenomenon that many people experience. If you find yourself with too specific of a hypothesis, go back to asking the big question: what is it that you want to know, and what do you think will happen between your two variables?
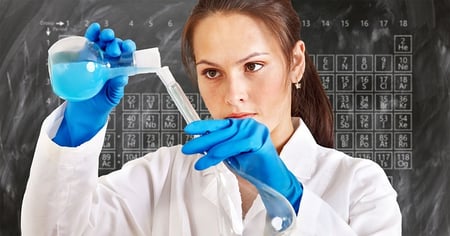
Hypothesis Testing Examples
We know it can be hard to write a good hypothesis unless you’ve seen some good hypothesis examples. We’ve included four hypothesis examples based on some made-up experiments. Use these as templates or launch pads for coming up with your own hypotheses.
Experiment #1: Students Studying Outside (Writing a Hypothesis)
You are a student at PrepScholar University. When you walk around campus, you notice that, when the temperature is above 60 degrees, more students study in the quad. You want to know when your fellow students are more likely to study outside. With this information, how do you make the best hypothesis possible?
You must remember to make additional observations and do secondary research before writing your hypothesis. In doing so, you notice that no one studies outside when it’s 75 degrees and raining, so this should be included in your experiment. Also, studies done on the topic beforehand suggested that students are more likely to study in temperatures less than 85 degrees. With this in mind, you feel confident that you can identify your variables and write your hypotheses:
If-then: “If the temperature in Fahrenheit is less than 60 degrees, significantly fewer students will study outside.”
Null: “If the temperature in Fahrenheit is less than 60 degrees, the same number of students will study outside as when it is more than 60 degrees.”
These hypotheses are plausible, as the temperatures are reasonably within the bounds of what is possible. The number of people in the quad is also easily observable. It is also not a phenomenon specific to only one person or at one time, but instead can explain a phenomenon for a broader group of people.
To complete this experiment, you pick the month of October to observe the quad. Every day (except on the days where it’s raining)from 3 to 4 PM, when most classes have released for the day, you observe how many people are on the quad. You measure how many people come and how many leave. You also write down the temperature on the hour.
After writing down all of your observations and putting them on a graph, you find that the most students study on the quad when it is 70 degrees outside, and that the number of students drops a lot once the temperature reaches 60 degrees or below. In this case, your research report would state that you accept or “failed to reject” your first hypothesis with your findings.
Experiment #2: The Cupcake Store (Forming a Simple Experiment)
Let’s say that you work at a bakery. You specialize in cupcakes, and you make only two colors of frosting: yellow and purple. You want to know what kind of customers are more likely to buy what kind of cupcake, so you set up an experiment. Your independent variable is the customer’s gender, and the dependent variable is the color of the frosting. What is an example of a hypothesis that might answer the question of this study?
Here’s what your hypotheses might look like:
If-then: “If customers’ gender is female, then they will buy more yellow cupcakes than purple cupcakes.”
Null: “If customers’ gender is female, then they will be just as likely to buy purple cupcakes as yellow cupcakes.”
This is a pretty simple experiment! It passes the test of plausibility (there could easily be a difference), defined concepts (there’s nothing complicated about cupcakes!), observability (both color and gender can be easily observed), and general explanation ( this would potentially help you make better business decisions ).
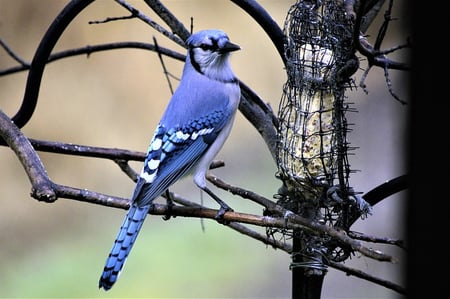
Experiment #3: Backyard Bird Feeders (Integrating Multiple Variables and Rejecting the If-Then Hypothesis)
While watching your backyard bird feeder, you realized that different birds come on the days when you change the types of seeds. You decide that you want to see more cardinals in your backyard, so you decide to see what type of food they like the best and set up an experiment.
However, one morning, you notice that, while some cardinals are present, blue jays are eating out of your backyard feeder filled with millet. You decide that, of all of the other birds, you would like to see the blue jays the least. This means you'll have more than one variable in your hypothesis. Your new hypotheses might look like this:
If-then: “If sunflower seeds are placed in the bird feeders, then more cardinals will come than blue jays. If millet is placed in the bird feeders, then more blue jays will come than cardinals.”
Null: “If either sunflower seeds or millet are placed in the bird, equal numbers of cardinals and blue jays will come.”
Through simple observation, you actually find that cardinals come as often as blue jays when sunflower seeds or millet is in the bird feeder. In this case, you would reject your “if-then” hypothesis and “fail to reject” your null hypothesis . You cannot accept your first hypothesis, because it’s clearly not true. Instead you found that there was actually no relation between your different variables. Consequently, you would need to run more experiments with different variables to see if the new variables impact the results.
Experiment #4: In-Class Survey (Including an Alternative Hypothesis)
You’re about to give a speech in one of your classes about the importance of paying attention. You want to take this opportunity to test a hypothesis you’ve had for a while:
If-then: If students sit in the first two rows of the classroom, then they will listen better than students who do not.
Null: If students sit in the first two rows of the classroom, then they will not listen better or worse than students who do not.
You give your speech and then ask your teacher if you can hand out a short survey to the class. On the survey, you’ve included questions about some of the topics you talked about. When you get back the results, you’re surprised to see that not only do the students in the first two rows not pay better attention, but they also scored worse than students in other parts of the classroom! Here, both your if-then and your null hypotheses are not representative of your findings. What do you do?
This is when you reject both your if-then and null hypotheses and instead create an alternative hypothesis . This type of hypothesis is used in the rare circumstance that neither of your hypotheses is able to capture your findings . Now you can use what you’ve learned to draft new hypotheses and test again!
Key Takeaways: Hypothesis Writing
The more comfortable you become with writing hypotheses, the better they will become. The structure of hypotheses is flexible and may need to be changed depending on what topic you are studying. The most important thing to remember is the purpose of your hypothesis and the difference between the if-then and the null . From there, in forming your hypothesis, you should constantly be asking questions, making observations, doing secondary research, and considering your variables. After you have written your hypothesis, be sure to edit it so that it is plausible, clearly defined, observable, and helpful in explaining a general phenomenon.
Writing a hypothesis is something that everyone, from elementary school children competing in a science fair to professional scientists in a lab, needs to know how to do. Hypotheses are vital in experiments and in properly executing the scientific method . When done correctly, hypotheses will set up your studies for success and help you to understand the world a little better, one experiment at a time.
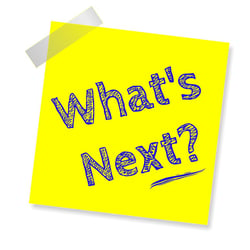
What’s Next?
If you’re studying for the science portion of the ACT, there’s definitely a lot you need to know. We’ve got the tools to help, though! Start by checking out our ultimate study guide for the ACT Science subject test. Once you read through that, be sure to download our recommended ACT Science practice tests , since they’re one of the most foolproof ways to improve your score. (And don’t forget to check out our expert guide book , too.)
If you love science and want to major in a scientific field, you should start preparing in high school . Here are the science classes you should take to set yourself up for success.
If you’re trying to think of science experiments you can do for class (or for a science fair!), here’s a list of 37 awesome science experiments you can do at home
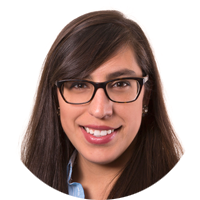
Ashley Sufflé Robinson has a Ph.D. in 19th Century English Literature. As a content writer for PrepScholar, Ashley is passionate about giving college-bound students the in-depth information they need to get into the school of their dreams.
Student and Parent Forum
Our new student and parent forum, at ExpertHub.PrepScholar.com , allow you to interact with your peers and the PrepScholar staff. See how other students and parents are navigating high school, college, and the college admissions process. Ask questions; get answers.

Ask a Question Below
Have any questions about this article or other topics? Ask below and we'll reply!
Improve With Our Famous Guides
- For All Students
The 5 Strategies You Must Be Using to Improve 160+ SAT Points
How to Get a Perfect 1600, by a Perfect Scorer
Series: How to Get 800 on Each SAT Section:
Score 800 on SAT Math
Score 800 on SAT Reading
Score 800 on SAT Writing
Series: How to Get to 600 on Each SAT Section:
Score 600 on SAT Math
Score 600 on SAT Reading
Score 600 on SAT Writing
Free Complete Official SAT Practice Tests
What SAT Target Score Should You Be Aiming For?
15 Strategies to Improve Your SAT Essay
The 5 Strategies You Must Be Using to Improve 4+ ACT Points
How to Get a Perfect 36 ACT, by a Perfect Scorer
Series: How to Get 36 on Each ACT Section:
36 on ACT English
36 on ACT Math
36 on ACT Reading
36 on ACT Science
Series: How to Get to 24 on Each ACT Section:
24 on ACT English
24 on ACT Math
24 on ACT Reading
24 on ACT Science
What ACT target score should you be aiming for?
ACT Vocabulary You Must Know
ACT Writing: 15 Tips to Raise Your Essay Score
How to Get Into Harvard and the Ivy League
How to Get a Perfect 4.0 GPA
How to Write an Amazing College Essay
What Exactly Are Colleges Looking For?
Is the ACT easier than the SAT? A Comprehensive Guide
Should you retake your SAT or ACT?
When should you take the SAT or ACT?
Stay Informed

Get the latest articles and test prep tips!
Looking for Graduate School Test Prep?
Check out our top-rated graduate blogs here:
GRE Online Prep Blog
GMAT Online Prep Blog
TOEFL Online Prep Blog
Holly R. "I am absolutely overjoyed and cannot thank you enough for helping me!”

AI-Driven Hypotheses: Real world examples exploring the potential and challenges of AI-generated hypotheses in science
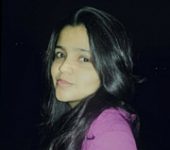
Artificial intelligence (AI) is no longer confined to mere automation; it is now an active participant in the pursuit of knowledge and understanding. However, in the realm of scientific research, the integration of AI marks a significant paradigm shift, ushering in an era where machines and human actively collaborate to formulate research hypotheses and questions. While AI systems have traditionally served as powerful tools for data analysis, their evolution now allows them to go beyond analysis and generate hypotheses, prompting researchers to explore uncharted domains of research.
Let’s delve deeper in understanding this transformative capability of AI and the challenges established in research hypothesis formation, emphasizing the crucial role of human intervention throughout the AI integration process.
Table of Contents
Potential of AI-Generated Research Hypothesis: Is it enough?
The discerning ability of AI, particularly through machine learning algorithms, has demonstrated a unique capacity to identify patterns across vast datasets. This has given rise to AI systems not only proficient in analyzing existing data but also in formulating hypotheses based on patterns that may elude human observation alone. The synergy between machine-driven hypothesis generation and human expertise represents a promising frontier for scientific discovery, underscoring the importance of human oversight and interpretation.
The capability of AI to generate hypotheses raises thought-provoking questions about the nature of creativity in the research process. However, although AI can identify patterns within data, the question remains: can they exhibit true creativity in proposing hypotheses, or are they limited to recognizing patterns within existing data?
Furthermore, the intersection of AI and research transcends the generation of hypotheses to include the formulation of research questions. By actively engaging with data and recognizing gaps in knowledge, AI systems can propose insightful questions that guide researchers toward unexplored avenues. This collaborative approach between machines and researchers enhances the scope and depth of scientific inquiry, emphasizing the indispensable role of human insight in shaping the research agenda.
Challenges in AI-Driven Hypothesis Formation
Despite the immense potential, the integration of AI in hypothesis formation is not without its challenges. One significant concern is the “black box” nature of many advanced AI algorithms. As these systems become more complex, understanding the reasoning behind their generated hypotheses becomes increasingly challenging for human researchers. This lack of interpretability can hinder the acceptance of AI-driven hypotheses in the scientific community.
Moreover, biases inherent in the training data of AI models can influence the hypotheses generated. If not carefully addressed, this bias could lead to skewed perspectives and reinforce existing stereotypes. It is crucial to recognize that while AI can process vast amounts of information, it lacks the nuanced understanding and contextual awareness that human researchers bring to the table.
Real Concerns of the Scholarly Community Clouding the Integration of AI in Research Hypothesis Generation
Instance 1 In a paper published in JAMA Ophthalmology, researchers utilized GPT-4, the latest version of the language model powering ChatGPT, in conjunction with Advanced Data Analysis (ADA), a model incorporating Python for statistical analysis. The AI-generated data incorrectly suggested the superiority of one surgical procedure over another in treating keratoconus. The study aimed to demonstrate the ease with which AI could create seemingly authentic but fabricated datasets. Despite flaws detectable under scrutiny, the authors expressed concern over the potential misuse of AI in generating convincing yet false data, raising issues of research integrity. Experts emphasize the need for updated quality checks and automated tools to identify AI-generated synthetic data in scientific publishing.
Instance 2 In early October, a gathering of researchers, including a past Nobel laureate, convened in Stockholm to explore the evolving role of AI in scientific processes. Led by Hiroaki Kitano, a biologist and CEO of Sony AI, the workshop considered introducing awards for AIs and AI-human collaborations producing outstanding scientific contributions. The discussion revolved around the potential of AI in various scientific tasks, including hypothesis generation, a process traditionally requiring human creativity. While AI has long been involved in literature-based discovery and knowledge graph analysis, recent advancements, particularly in large language models, are enabling the generation of hypotheses and the exploration of unconventional ideas.
The potential for AI to make ‘alien’ hypotheses—those unlikely to be conceived by humans—has been demonstrated, raising questions about the interpretability and clarity of AI-generated hypotheses.
Ethical Considerations in AI-Driven Research Hypothesis
As AI takes on a more active role in hypothesis formation, ethical considerations become paramount. The responsible use of AI requires continuous vigilance to prevent unintended consequences. Researchers must be vigilant in identifying and mitigating biases in training data, ensuring that AI systems are not perpetuating or exacerbating existing inequalities.
Additionally, the ethical implications of AI-generated hypotheses, particularly in sensitive areas such as genetics or social sciences, demand careful scrutiny. Transparency in the decision-making process of AI algorithms is essential to build trust within the scientific community and society at large. Striking the right balance between innovation and ethical responsibility is a challenge that requires constant attention as the collaboration between humans and AI evolves.
The Human Touch: A crucial element in AI-driven research
Nuanced thinking, creativity, and contextual understanding that humans possess play a vital role in refining and validating the hypotheses generated by AI. Researchers must act as critical evaluators, questioning the assumptions made by AI algorithms and ensuring that the proposed hypotheses align with existing knowledge. Furthermore, the interpretability challenge can be addressed through interdisciplinary collaboration. Scientists working closely with experts in AI ethics, philosophy, and computer science can develop frameworks to enhance the transparency of AI-generated hypotheses. This not only fosters a better understanding of the underlying processes but also ensures that the generated hypotheses align with ethical and scientific standards.
What the Future Holds
The integration of AI in hypothesis formation is an ongoing journey with vast potential. The collaborative efforts of humans and machines hold the promise of accelerating scientific discovery, unlocking new insights, and addressing complex challenges facing humanity. However, this journey requires a balanced approach, acknowledging the strengths of AI while respecting the unique capabilities and ethical considerations that humans bring to the table.
To Conclude…
The transformative capability of AI in hypothesis formation is reshaping the landscape of scientific research. But this is not possible without the collaborative partnership between humans and machines, having the potential to drive unprecedented progress. Thus, it is imperative to navigate the challenges associated with AI integration and embrace a symbiotic relationship between human intellect and AI; with which we can unlock the full potential of this dynamic collaboration and usher in a new era of scientific exploration and understanding.

Interesting article
Rate this article Cancel Reply
Your email address will not be published.
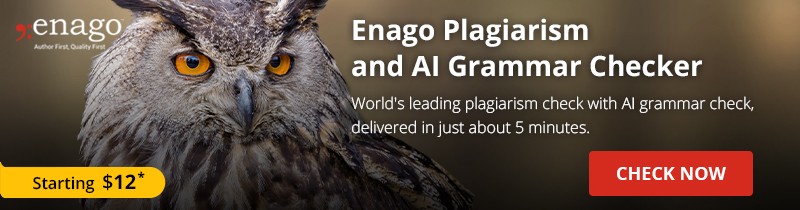
Enago Academy's Most Popular Articles
- Old Webinars
- Trending Now
- Webinar Mobile App
Using AI for Plagiarism Prevention and AI-Content Detection in Academic Writing
Watch Now In today’s digital age, where information is readily accessible and content creation…
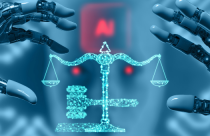
- AI in Academia
- Thought Leadership
Authenticating AI-Generated Scholarly Outputs: Practical approaches to take
The rapid advancements in artificial intelligence (AI) have given rise to a new era of…
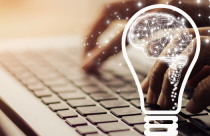
Simplifying the Literature Review Journey — A comparative analysis of 6 AI summarization tools
Imagine having to skim through and read mountains of research papers and books, only to…
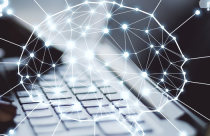
6 Leading AI Detection Tools for Academic Writing — A comparative analysis
The advent of AI content generators, exemplified by advanced models like ChatGPT, Claude AI, and…
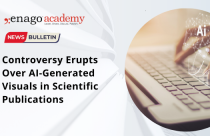
- Industry News
Controversy Erupts Over AI-Generated Visuals in Scientific Publications
In recent months, the scientific community has been grappling with a growing trend: the integration…
Simplifying the Literature Review Journey — A comparative analysis of 6 AI…

Sign-up to read more
Subscribe for free to get unrestricted access to all our resources on research writing and academic publishing including:
- 2000+ blog articles
- 50+ Webinars
- 10+ Expert podcasts
- 50+ Infographics
- 10+ Checklists
- Research Guides
We hate spam too. We promise to protect your privacy and never spam you.
I am looking for Editing/ Proofreading services for my manuscript Tentative date of next journal submission:
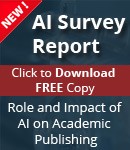
What should universities' stance be on AI tools in research and academic writing?

An official website of the United States government
The .gov means it’s official. Federal government websites often end in .gov or .mil. Before sharing sensitive information, make sure you’re on a federal government site.
The site is secure. The https:// ensures that you are connecting to the official website and that any information you provide is encrypted and transmitted securely.
- Publications
- Account settings
Preview improvements coming to the PMC website in October 2024. Learn More or Try it out now .
- Advanced Search
- Journal List
- v.23(7); 2013 Jul
Hypothesis-generating research and predictive medicine
Leslie g. biesecker.
National Human Genome Research Institute, National Institutes of Health, Bethesda, Maryland 20892, USA
Genomics has profoundly changed biology by scaling data acquisition, which has provided researchers with the opportunity to interrogate biology in novel and creative ways. No longer constrained by low-throughput assays, researchers have developed hypothesis-generating approaches to understand the molecular basis of nature—both normal and pathological. The paradigm of hypothesis-generating research does not replace or undermine hypothesis-testing modes of research; instead, it complements them and has facilitated discoveries that may not have been possible with hypothesis-testing research. The hypothesis-generating mode of research has been primarily practiced in basic science but has recently been extended to clinical-translational work as well. Just as in basic science, this approach to research can facilitate insights into human health and disease mechanisms and provide the crucially needed data set of the full spectrum of genotype–phenotype correlations. Finally, the paradigm of hypothesis-generating research is conceptually similar to the underpinning of predictive genomic medicine, which has the potential to shift medicine from a primarily population- or cohort-based activity to one that instead uses individual susceptibility, prognostic, and pharmacogenetic profiles to maximize the efficacy and minimize the iatrogenic effects of medical interventions.
The goal of this article is to describe how recent technological changes provide opportunities to undertake novel approaches to biomedical research and to practice genomic preventive medicine. Massively parallel sequencing is the primary technology that will be addressed here ( Mardis 2008 ), but the principles apply to many other technologies, such as proteomics, metabolomics, transcriptomics, etc. Readers of this journal are well aware of the precipitous fall of sequencing costs over the last several decades. The consequence of this fall is that we are no longer in a scientific and medical world where the throughput (and the costs) of testing is the key limiting factor around which these enterprises are organized. Once one is released from this limiting factor, one may ask whether these enterprises should be reorganized. Here I outline the principles of how these enterprises are organized, show how high-throughput biology can allow alternative organizations of these enterprises to be considered, and show how biology and medicine are in many ways similar. The discussion includes three categories of enterprises: basic research, clinical research, and medical practice.
The basic science hypothesis-testing paradigm
The classical paradigm for basic biological research has been to develop a specific hypothesis that can be tested by the application of a prospectively defined experiment (see Box 1 ). I suggest that one of the major (although not the only) factors that led to the development of this paradigm is that experimental design was limited by the throughput of available assays. This low throughput mandated that the scientific question had to be focused narrowly to make the question tractable. However, the paradigm can be questioned if the scientist has the ability to assay every potential attribute of a given type (e.g., all genes). If the hypothesis is only needed to select the assay, one does not need a hypothesis to apply a technology that assays all attributes. In the case of sequencing, the radical increase in throughput can release scientists from the constraint of the specific hypothesis because it has allowed them to interrogate essentially all genotypes in a genome in a single assay. This capability facilitates fundamental biological discoveries that were impossible or impractical with a hypothesis-testing mode of scientific inquiry. Examples of this approach are well demonstrated by several discoveries that followed the sequencing of a number of genomes. An example was the discovery that the human gene count was just over 20,000 ( International Human Genome Sequencing Consortium 2004 ), much lower than prior estimates. This result, although it was much debated and anticipated, was not a hypothesis that drove the human genome project, but nonetheless was surprising and led to insights into the nuances of gene regulation and transcriptional isoforms to explain the complexity of the human organism. The availability of whole genome sequence data from multiple species facilitated analyses of conservation. While it was expected that protein-coding regions, and to a lesser extent promoters and 5′- and 3′-untranslated regions of genes, would exhibit recognizable sequence conservation, it was unexpected that an even larger fraction of the genomes outside of genes are highly conserved ( Mouse Genome Sequencing Consortium 2002 ). This surprising and unanticipated discovery has spawned a novel field of scientific inquiry to determine the functional roles of these elements, which are undoubtedly important in physiology and pathophysiology. These discoveries demonstrate the power of hypothesis-generating basic research to illuminate important biological principles.
Basic science hypothesis-testing and hypothesis-generating paradigms

Clinical and translational research
The approach to clinical research grew out of the basic science paradigm as described above. The first few steps of selecting a scientific problem and developing a hypothesis are similar, with the additional step ( Box 2 ) of rigorously defining a phenotype and then carefully selecting research participants with and without that trait. As in the basic science paradigm, the hypothesis is tested by the application of a specific assay to the cases and controls. Again, this paradigm has been incredibly fruitful and should not be abandoned, but the hypothesis-generating approach can be used here as well. In this approach, a cohort of participants is consented, basic information is gathered on their health, and then a high-throughput assay, such as genome or exome sequencing, is applied to all of the participants. Again, because the assay tests all such attributes, the research design does not necessitate a priori selections of phenotypes and genes to be interrogated. Then, the researcher can examine the sequence data set for patterns and perturbations, form hypotheses about how such perturbations might affect the phenotype of the participants, and test that hypothesis with a clinical research evaluation. This approach has been used with data from genome-wide copy number assessments (array CGH and SNP arrays), but sequencing takes it to a higher level of interrogation and provides innumerable variants with which to work.
Clinical research paradigms

An example of this type of sequence-based hypothesis-generating clinical research started with a collaborative project in which we showed that mutations in the gene ACSF3 caused the biochemical phenotype of combined malonic and methylmalonic acidemia ( Sloan et al. 2011 ). At that time, the disorder was believed to be a classic pediatric, autosomal-recessive severe metabolic disorder with decompensation and sometimes death. We then queried the ClinSeq cohort ( Biesecker et al. 2009 ) to assess the carrier frequency, to estimate the population frequency of this rare disorder. Because ClinSeq is a cohort of adults with a range of atherosclerosis severity, we reasoned that this would serve as a control population for an unbiased estimate of ACSF3 heterozygote mutant alleles. Surprisingly, we identified a ClinSeq participant who was homozygous for one of the mutations identified in the children with the typical phenotype. Indeed, one potential interpretation of the data would be that the variant is, in fact, benign and was erroneously concluded to be pathogenic, based on finding it in a child with the typical phenotype. It has been shown that this error is common, with up to 20% of variants listed in databases as pathogenic actually being benign ( Bell et al. 2011 ). Further clinical research on this participant led to the surprising result that she had severely abnormal blood and urine levels of malonic and methylmalonic acid ( Sloan et al. 2011 ). This novel approach to translational research was a powerful confirmation that the mutation was indeed pathogenic, but there was another, even more important conclusion. We had conceptualized the disease completely incorrectly. Instead of being only a severe, pediatric metabolic disorder, it was instead a disorder with a wide phenotypic spectrum in which one component of the disease is a metabolic perturbation and another component is a susceptibility to severe decompensation and strokes. This research indeed raises many questions about the natural history of the disorder, whether the pediatric decompensation phenotype is attributable to modifiers, what the appropriate management of such an adult would be, etc.
Irrespective of these limitations, the understanding of the disease has markedly advanced, and the key to understanding the broader spectrum of this disease was the hypothesis-generating approach enabled by the massively parallel sequence data and the ability to phenotype patients iteratively from ClinSeq. The iterative phenotyping was essential because we could not have anticipated when the patients were originally ascertained that we would need to assay malonic and methylmalonic acid. Nor did we recognize prospectively that we should be evaluating apparently healthy patients in their seventh decade for this phenotype. Indeed, it is impossible to evaluate patients for all potential phenotypes prospectively, and it is essential to minimize ascertainment bias for patient recruitment in order to allow the discovery of the full spectrum of phenotypes associated with genomic variations. This latter issue has become a critical challenge for implementing predictive medicine, as described below.
Predictive genomic medicine in practice
The principles of scientific inquiry are parallel to the processes of clinical diagnosis ( Box 3 ). In the classic, hypothesis-testing paradigm, clinicians gather background information including chief complaint, 2 medical and family history, and physical examination, and use these data to formulate the differential diagnosis, which is a set of potential medical diagnoses that could explain the patient's signs and symptoms. Then, the clinician selects, among the myriad of tests (imaging, biochemical, genetic, physiologic, etc.), a few tests, the results of which should distinguish among (or possibly exclude entirely) the disorders on the differential diagnosis. Like the scientist, the physician must act as a test selector, because each of the tests is low throughput, time consuming, and expensive.
Clinical practice paradigms—hypothesis testing and hypothesis generating

As in the basic and translational research discussion above, the question could be raised as to whether the differential diagnostic paradigm is necessary for genetic disorders. Indeed, the availability of clinical genome and exome sequencing heralds an era when the test could be ordered relatively early in the diagnostic process, with the clinician serving in a more interpretative role, rather than as a test selector ( Hennekam and Biesecker 2012 ). This approach has already been adopted for copy number variation, because whole genome array CGH- or SNP-based approaches have mostly displaced more specific single-gene or single-locus assays and standard chromosome analyses ( Miller et al. 2010 ). But the paradigm can be taken beyond hypothesis-generating clinical diagnosis into predictive medicine. One can now begin to envision how whole genome approaches could be used to assess risks prospectively for susceptibility to late-onset disorders or occult or subclinical disorders. The heritable cancer susceptibility syndromes are a good example of this. The current clinical approach is to order a specific gene test if a patient presents with a personal history of an atypical or early-onset form of a specific cancer syndrome, or has a compelling family history of the disease. As in the prior examples, this is because individual cancer gene testing is expensive and low throughput. One can ask the question whether this is the ideal approach or if we could be screening for these disorders from genome or exome data. Again, we applied sequencing analysis for these genes to the ClinSeq cohort because they were not ascertained for that phenotype. In a published study of 572 exomes ( Johnston et al. 2012 ), updated here to include 850 exomes, we have identified 10 patients with seven distinct cancer susceptibility syndrome mutations. These were mostly familial breast and ovarian cancer ( BRCA1 and BRCA2 ), with one patient each with paraganglioma and pheochromocytoma ( SDHC ) and one with Lynch syndrome ( MSH6 ). What is remarkable about these diagnoses is that only about half of them had a convincing personal or family history of the disease, and thus most would have not been offered testing using the current, hypothesis-testing clinical paradigm. These data suggest that screening for these disorders using genome or exome sequencing could markedly improve our ability to identify such families before they develop or die from these diseases—the ideal of predictive genomic medicine.
Despite these optimistic scenarios and examples, it remains true that our ability to perform true predictive medicine is limited. These limitations include technical factors such as incomplete sequence coverage, imperfect sequence quality, inadequate knowledge regarding the penetrance and expressivity of most variants, uncertain medical approaches and utility of pursuing variants from genomic sequencing, and the poor preparation of most clinicians for addressing genomic concerns in the clinic ( Biesecker 2013 ). Recognizing all of these limitations, it is clear that we are not prepared to launch broad-scale implementation of predictive genomic medicine, nor should all research be structured using the hypothesis-generating approach.
Hypothesis-testing approaches to science and medicine have served us well and should continue. However, the advent of massively parallel sequencing and other high-throughput technologies provides opportunities to undertake hypothesis-generating approaches to science and medicine, which in turn provide unprecedented opportunities for discovery in the research realm. This can allow the discovery of results that were not anticipated or intended by the research design, yet provide critical insights into biology and pathophysiology. Similarly, hypothesis-generating clinical research has the potential to provide these same insights and, in addition, has the potential to provide us with data that will illuminate the full spectrum of genotype–phenotype correlations, eliminating the biases that have limited this understanding in the past. Finally, applying these principles to clinical medicine can provide new pathways to diagnosis and provide the theoretical basis for predictive medicine that can detect disease susceptibility and allow health to be maintained, instead of solely focusing on the treatment of evident disease.
Article is online at http://www.genome.org/cgi/doi/10.1101/gr.157826.113 .
2 The chief complaint is a brief description of the problem that led the patient to the clinician, such as “I have a cough and fever.”
- Bell CJ, Dinwiddie DL, Miller NA, Hateley SL, Ganusova EE, Mudge J, Langley RJ, Zhang L, Lee CC, Schilkey FD, et al. 2011. Carrier testing for severe childhood recessive diseases by next-generation sequencing . Sci Transl Med 3 : 65ra64 [ PMC free article ] [ PubMed ] [ Google Scholar ]
- Biesecker LG 2013. Incidental findings are critical for genomics . Am J Hum Genet 92 : 648–651 [ PMC free article ] [ PubMed ] [ Google Scholar ]
- Biesecker LG, Mullikin JC, Facio FM, Turner C, Cherukuri PF, Blakesley RW, Bouffard GG, Chines PS, Cruz P, Hansen NF, et al. 2009. The ClinSeq Project: Piloting large-scale genome sequencing for research in genomic medicine . Genome Res 19 : 1665–1674 [ PMC free article ] [ PubMed ] [ Google Scholar ]
- Hennekam RC, Biesecker LG 2012. Next-generation sequencing demands next-generation phenotyping . Hum Mutat 33 : 884–886 [ PMC free article ] [ PubMed ] [ Google Scholar ]
- International Human Genome Sequencing Consortium 2004. Finishing the euchromatic sequence of the human genome . Nature 431 : 931–945 [ PubMed ] [ Google Scholar ]
- Johnston JJ, Rubinstein WS, Facio FM, Ng D, Singh LN, Teer JK, Mullikin JC, Biesecker LG 2012. Secondary variants in individuals undergoing exome sequencing: Screening of 572 individuals identifies high-penetrance mutations in cancer-susceptibility genes . Am J Hum Genet 91 : 97–108 [ PMC free article ] [ PubMed ] [ Google Scholar ]
- Mardis ER 2008. The impact of next-generation sequencing technology on genetics . Trends Genet 24 : 133–141 [ PubMed ] [ Google Scholar ]
- Miller DT, Adam MP, Aradhya S, Biesecker LG, Brothman AR, Carter NP, Church DM, Crolla JA, Eichler EE, Epstein CJ, et al. 2010. Consensus statement: Chromosomal microarray is a first-tier clinical diagnostic test for individuals with developmental disabilities or congenital anomalies . Am J Hum Genet 86 : 749–764 [ PMC free article ] [ PubMed ] [ Google Scholar ]
- Mouse Genome Sequencing Consortium. 2002. Initial sequencing and comparative analysis of the mouse genome . Nature 420 : 520–562 [ PubMed ] [ Google Scholar ]
- Sloan JL, Johnston JJ, Manoli I, Chandler RJ, Krause C, Carrillo-Carrasco N, Chandrasekaran SD, Sysol JR, O'Brien K, Hauser NS, et al. 2011. Exome sequencing identifies ACSF3 as a cause of combined malonic and methylmalonic aciduria . Nat Genet 43 : 883–886 [ PMC free article ] [ PubMed ] [ Google Scholar ]
Hypothesis Generation:
Using Descriptive Epidemiology to Generate a Hypothesis Regarding the Source of Infection and Mode of Transmission
****************************
In reality one begins to form hypotheses as soon as information about the outbreak begins to emerge. If you know what the disease is, your hypotheses will take into account its biology, what the reservoirs of disease are, how it is usually transmitted, its known risk factors, and its incubation period.
In addition, descriptive epidemiology attempts to identify similarities in person, time, or place that may suggest hypotheses regarding the cause of the outbreak.
For example, an epidemic curve illustrates the number of cases that occurred over time ; it helps establish that there is an outbreak, and the shape of the curve may also suggest the nature of the source (e.g. isolated point source versus continuous common source).
Spot maps can be used to illustrate the residence or work place of each of the cases that may suggest the source, such as John Snow’s map of cholera cases clustered around the Broad St. pump.
Similarities in personal characteristics may also suggest the cause (e.g. common occupations, age, school attended, etc).
Descriptive epidemiology focuses entirely on similarities among the cases and does not establish the cause. Hypotheses that are generated need to be tested by analytic studies that compare two or more groups of people (e.g. cases and controls who did not get the disease).
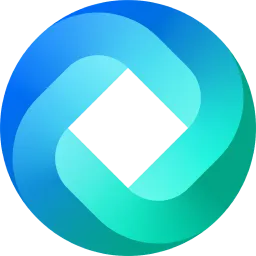
Hypothesis Generator
Generate hypotheses for your research.
- Academic Research: Generate hypotheses for your thesis, dissertation, or any academic paper.
- Data Analysis: Create hypotheses to guide your data exploration and analysis.
- Market Research: Develop hypotheses to understand market trends and consumer behavior.
- Product Development: Formulate hypotheses to guide your product testing and development process.
- Scientific Research: Generate hypotheses for your experiments or observational studies.
New & Trending Tools
Notes to blog post, cultural name generator, nonprofit grant proposal writer.
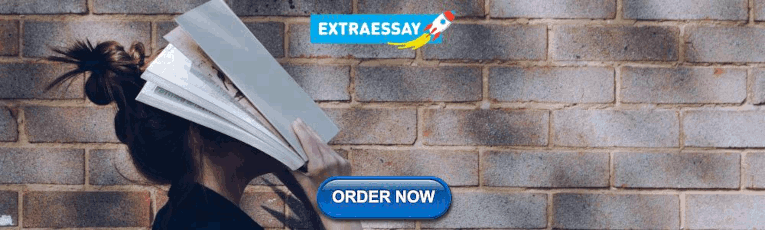
IMAGES
VIDEO
COMMENTS
The specific group being studied. The predicted outcome of the experiment or analysis. 5. Phrase your hypothesis in three ways. To identify the variables, you can write a simple prediction in if…then form. The first part of the sentence states the independent variable and the second part states the dependent variable.
It seeks to explore and understand a particular aspect of the research subject. In contrast, a research hypothesis is a specific statement or prediction that suggests an expected relationship between variables. It is formulated based on existing knowledge or theories and guides the research design and data analysis. 7.
Hypothesis testing is a formal procedure for investigating our ideas about the world using statistics. It is most often used by scientists to test specific predictions, called hypotheses, that arise from theories. ... In most cases you will use the p-value generated by your statistical test to guide your decision. And in most cases, ...
A research hypothesis, in its plural form "hypotheses," is a specific, testable prediction about the anticipated results of a study, established at its outset. It is a key component of the scientific method. Hypotheses connect theory to data and guide the research process towards expanding scientific understanding.
One of the main concerns relates to the ethical implications of the generated hypotheses. The authors may need to outline potential benefits and limitations of their suggestions and target widely visible publication outlets to ignite discussion by experts and start testing the hypotheses. ... Reflecting on general and specific issues around the ...
A hypothesis, on the other hand, is a specific prediction about a new phenomenon that should be observed if a particular theory is accurate. It is an explanation that relies on just a few key concepts. Hypotheses are often specific predictions about what will happen in a particular study. They are developed by considering existing evidence and ...
Generating a testable working hypothesis is the first step towards conducting original research. Such research may prove or disprove the proposed hypothesis. ... Review articles are generated by secondary research. These could be systematic reviews which follow methods akin to primary research but with the unit of study being published papers ...
Hypothesis-generating studies differ methodologically from confirmatory studies. A generated hypothesis must be confirmed in a new study. An experiment is usually required for confirmation as an observational study cannot provide unequivocal results. For example, selection and confounding bias can be prevented by randomization and blinding in a ...
Verify the generated hypothesis by appropriate means such as evaluation and statistical test (i.e., hypothesis verification). 7. Interpret and explain the hypothesis so that the user can understand how it is generated, what features it has, and how it works. 8.
However, exactly how a scientific hypothesis is generated, even as shown by secondary data analysis in clinical research, is unknown. Understanding the detailed process of scientific hypothesis generation could improve the efficiency of delineating clinical research questions and, consequently, clinical research.
A theory is describes as "an organized body of concepts and principles intended to explain a particular phenomenon" (Leedy and Ormrod, 2001). A theory is similar to a hypothesis in that it offers a tentative explanation for a phenomenon that new data will either support or not support. Both are supported or rejected based on testing by various ...
Theories and Hypotheses. Before describing how to develop a hypothesis, it is important to distinguish between a theory and a hypothesis. A theory is a coherent explanation or interpretation of one or more phenomena. Although theories can take a variety of forms, one thing they have in common is that they go beyond the phenomena they explain by including variables, structures, processes ...
A scientific hypothesis is an inferred explanation of an observation or research finding; while more exploratory in nature than a theory, it is based on existing scientific knowledge. ... But when a hypothesis is generated in science, a scientist will also make an alternative hypothesis, an explanation that explains a study if the data do not ...
Make a hypothesis, or potential solution to the question; Test the hypothesis; If the hypothesis is true, find more evidence or find counter-evidence; ... To see how this process works, let's consider a specific theory and a hypothesis that might be generated from that theory. As you'll learn in a later module, the James-Lange theory of ...
Merriam Webster defines a hypothesis as "an assumption or concession made for the sake of argument.". In other words, a hypothesis is an educated guess. Scientists make a reasonable assumption--or a hypothesis--then design an experiment to test whether it's true or not.
How to use Hypothesis Maker. Visit the tool's page. Enter your research question into the provided field. Click the 'Generate' button to let the AI generate a hypothesis based on your research question. Review the generated hypothesis and adjust it as necessary to fit your research context and objectives. Copy and paste the hypothesis into your ...
Despite the immense potential, the integration of AI in hypothesis formation is not without its challenges. One significant concern is the "black box" nature of many advanced AI algorithms. As these systems become more complex, understanding the reasoning behind their generated hypotheses becomes increasingly challenging for human researchers.
Hypothesis testing: survey research to test and measure hypotheses generated based on questionnaires, and rank and sample statistical analyses realized in the first step. Behavioral change scenario process: construction of long-term scenarios including behavioral change, in particular expert-built scenarios from the collected data.
The hypothesis-generating mode of research has been primarily practiced in basic science but has recently been extended to clinical-translational work as well. Just as in basic science, this approach to research can facilitate insights into human health and disease mechanisms and provide the crucially needed data set of the full spectrum of ...
Hypothesis generation is an educated "guess" of various factors that are impacting the business problem that needs to be solved using machine learning. In framing a hypothesis, the data scientist must not know the outcome of the hypothesis that has been generated based on any evidence. "A hypothesis may be simply defined as a guess.
Hypothesis Generation: Using Descriptive Epidemiology to Generate a Hypothesis Regarding the Source of Infection and Mode of Transmission. In reality one begins to form hypotheses as soon as information about the outbreak begins to emerge. If you know what the disease is, your hypotheses will take into account its biology, what the reservoirs ...
How to use Hypothesis Generator. Visit the tool's page. Input your research question and a description of your dataset. Click the 'Submit' button to let the AI generate your null and alternative hypotheses. Review the generated hypotheses, making any necessary edits or adjustments to ensure they align with your research objectives and dataset.
This ensures every hypothesis generated is data-driven, accurate, and aligns with your specified parameters. Swift, On-Point Outputs: Time is of the essence in research. EssayGPT's hypothesis generator pledges quick turnarounds, without compromising the quality and relevance of the generated hypotheses.
That supports our hypothesis of the crucial role of the characterization of physical and chemical materials in adequately understanding the mechanisms of their interaction with biota. Microscopical methods (confocal, fluorescent, scanning electron) and Raman and FT-IR spectroscopy were used to track the particles' passage in a food web and ...