- Privacy Policy
Buy Me a Coffee

Home » Mixed Methods Research – Types & Analysis
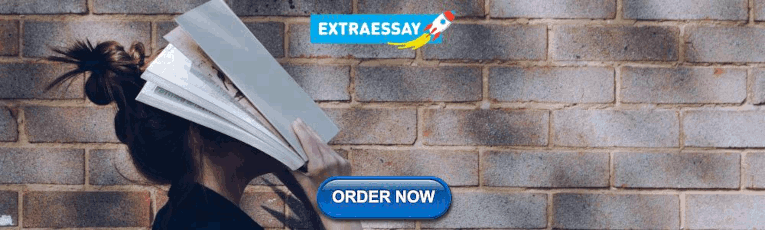
Mixed Methods Research – Types & Analysis
Table of Contents

Mixed Methods Research
Mixed methods research is an approach to research that combines both quantitative and qualitative research methods in a single study or research project. It is a methodological approach that involves collecting and analyzing both numerical (quantitative) and narrative (qualitative) data to gain a more comprehensive understanding of a research problem.
Types of Mixed Research

There are different types of mixed methods research designs that researchers can use, depending on the research question, the available data, and the resources available. Here are some common types:
Convergent Parallel Design
This design involves collecting both qualitative and quantitative data simultaneously, analyzing them separately, and then merging the findings to draw conclusions. The qualitative and quantitative data are given equal weight, and the findings are integrated during the interpretation phase.
Sequential Explanatory Design
In this design, the researcher collects and analyzes quantitative data first, and then uses qualitative data to explain or elaborate on the quantitative findings. The researcher may use the qualitative data to clarify unexpected or contradictory results from the quantitative analysis.
Sequential Exploratory Design
This design involves collecting qualitative data first, analyzing it, and then collecting and analyzing quantitative data to confirm or refute the qualitative findings. Qualitative data are used to generate hypotheses that are tested using quantitative data.
Concurrent Triangulation Design
This design involves collecting both qualitative and quantitative data concurrently and then comparing the results to find areas of agreement and disagreement. The findings are integrated during the interpretation phase to provide a more comprehensive understanding of the research question.
Concurrent Nested Design
This design involves collecting one type of data as the primary method and then using the other type of data to elaborate or clarify the primary data. For example, a researcher may use quantitative data as the primary method and qualitative data as a secondary method to provide more context and detail.
Transformative Design
This design involves using mixed methods research to not only understand the research question but also to bring about social change or transformation. The research is conducted in collaboration with stakeholders and aims to generate knowledge that can be used to improve policies, programs, and practices.
Concurrent Embedded Design
Concurrent embedded design is a type of mixed methods research design in which one type of data is embedded within another type of data. This design involves collecting both quantitative and qualitative data simultaneously, with one type of data being the primary method and the other type of data being the secondary method. The secondary method is embedded within the primary method, meaning that it is used to provide additional information or to clarify the primary data.
Data Collection Methods
Here are some common data collection methods used in mixed methods research:
Surveys are a common quantitative data collection method used in mixed methods research. Surveys involve collecting standardized responses to a set of questions from a sample of participants. Surveys can be conducted online, in person, or over the phone.
Interviews are a qualitative data collection method that involves asking open-ended questions to gather in-depth information about a participant’s experiences, perspectives, and opinions. Interviews can be conducted in person, over the phone, or online.
Focus groups
Focus groups are a qualitative data collection method that involves bringing together a small group of participants to discuss a topic or research question. The group is facilitated by a researcher, and the discussion is recorded and analyzed for themes and patterns.
Observations
Observations are a qualitative data collection method that involves systematically watching and recording behavior in a natural setting. Observations can be structured or unstructured and can be used to gather information about behavior, interactions, and context.
Document Analysis
Document analysis is a qualitative data collection method that involves analyzing existing documents, such as reports, policy documents, or media articles. Document analysis can be used to gather information about trends, policy changes, or public attitudes.
Experimentation
Experimentation is a quantitative data collection method that involves manipulating one or more variables and measuring their effects on an outcome. Experiments can be conducted in a laboratory or in a natural setting.
Data Analysis Methods
Mixed methods research involves using both quantitative and qualitative data analysis methods to analyze data collected through different methods. Here are some common data analysis methods used in mixed methods research:
Quantitative Data Analysis
Quantitative data collected through surveys or experiments can be analyzed using statistical methods. Statistical analysis can be used to identify relationships between variables, test hypotheses, and make predictions. Common statistical methods used in quantitative data analysis include regression analysis, t-tests, ANOVA, and correlation analysis.
Qualitative Data Analysis
Qualitative data collected through interviews, focus groups, or observations can be analyzed using a variety of qualitative data analysis methods. These methods include content analysis, thematic analysis, narrative analysis, and grounded theory. Qualitative data analysis involves identifying themes and patterns in the data, interpreting the meaning of the data, and drawing conclusions based on the findings.
Integration of Data
The integration of quantitative and qualitative data involves combining the results from both types of data analysis to gain a more comprehensive understanding of the research question. Integration can involve either a concurrent or sequential approach. Concurrent integration involves analyzing quantitative and qualitative data at the same time, while sequential integration involves analyzing one type of data first and then using the results to inform the analysis of the other type of data.
Triangulation
Triangulation involves using multiple sources or types of data to validate or corroborate findings. This can involve using both quantitative and qualitative data or multiple qualitative methods. Triangulation can enhance the credibility and validity of the research findings.
Mixed Methods Meta-analysis
Mixed methods meta-analysis involves the systematic review and synthesis of findings from multiple studies that use mixed methods designs. This involves combining quantitative and qualitative data from multiple studies to gain a broader understanding of a research question.
How to conduct Mixed Methods Research
Here are some general steps for conducting mixed methods research:
- Identify the research problem: The first step is to clearly define the research problem and determine if mixed methods research is appropriate for addressing it.
- Design the study: The research design should include both qualitative and quantitative data collection and analysis methods. The specific design will depend on the research question and the purpose of the study.
- Collect data : Data collection involves collecting both qualitative and quantitative data through various methods such as surveys, interviews, observations, and document analysis.
- Analyze data: Both qualitative and quantitative data need to be analyzed separately and then integrated. Analysis methods may include coding, statistical analysis, and thematic analysis.
- Interpret results: The results of the analysis should be interpreted, taking into account both the quantitative and qualitative findings. This involves integrating the results and identifying any patterns, themes, or discrepancies.
- Draw conclusions : Based on the interpretation of the results, conclusions should be drawn that address the research question and objectives.
- Report findings: Finally, the findings should be reported in a clear and concise manner, using both quantitative and qualitative data to support the conclusions.
Applications of Mixed Methods Research
Mixed methods research can be applied to a wide range of research fields and topics, including:
- Education : Mixed methods research can be used to evaluate educational programs, assess the effectiveness of teaching methods, and investigate student learning experiences.
- Health and social sciences: Mixed methods research can be used to study health interventions, understand the experiences of patients and their families, and assess the effectiveness of social programs.
- Business and management: Mixed methods research can be used to investigate customer satisfaction, assess the impact of marketing campaigns, and analyze the effectiveness of management strategies.
- Psychology : Mixed methods research can be used to explore the experiences and perspectives of individuals with mental health issues, investigate the impact of psychological interventions, and assess the effectiveness of therapy.
- Sociology : Mixed methods research can be used to study social phenomena, investigate the experiences and perspectives of marginalized groups, and assess the impact of social policies.
- Environmental studies: Mixed methods research can be used to assess the impact of environmental policies, investigate public perceptions of environmental issues, and analyze the effectiveness of conservation strategies.
Examples of Mixed Methods Research
Here are some examples of Mixed-Methods research:
- Evaluating a school-based mental health program: A researcher might use a concurrent embedded design to evaluate a school-based mental health program. The researcher might collect quantitative data through surveys and qualitative data through interviews with students and teachers. The quantitative data might be analyzed using statistical methods, while the qualitative data might be analyzed using thematic analysis. The results of the two types of data analysis could be integrated to provide a comprehensive evaluation of the program’s effectiveness.
- Understanding patient experiences of chronic illness: A researcher might use a sequential explanatory design to investigate patient experiences of chronic illness. The researcher might collect quantitative data through surveys and then use the results of the survey to inform the selection of participants for qualitative interviews. The qualitative data might be analyzed using content analysis to identify common themes in the patients’ experiences.
- Assessing the impact of a new public transportation system : A researcher might use a concurrent triangulation design to assess the impact of a new public transportation system. The researcher might collect quantitative data through surveys and qualitative data through focus groups with community members. The results of the two types of data analysis could be triangulated to provide a more comprehensive understanding of the impact of the new transportation system on the community.
- Exploring teacher perceptions of technology integration in the classroom: A researcher might use a sequential exploratory design to investigate teacher perceptions of technology integration in the classroom. The researcher might collect qualitative data through in-depth interviews with teachers and then use the results of the interviews to develop a survey. The quantitative data might be analyzed using descriptive statistics to identify trends in teacher perceptions.
When to use Mixed Methods Research
Mixed methods research is typically used when a research question cannot be fully answered by using only quantitative or qualitative methods. Here are some common situations where mixed methods research is appropriate:
- When the research question requires a more comprehensive understanding than can be achieved by using only quantitative or qualitative methods.
- When the research question requires both an exploration of individuals’ experiences, perspectives, and attitudes, as well as the measurement of objective outcomes and variables.
- When the research question requires the examination of a phenomenon in its natural setting and context, which can be achieved by collecting rich qualitative data, as well as the generalization of findings to a larger population, which can be achieved through the use of quantitative methods.
- When the research question requires the integration of different types of data or perspectives, such as combining data collected from participants with data collected from stakeholders or experts.
- When the research question requires the validation of findings obtained through one method by using another method.
- When the research question involves studying a complex phenomenon that cannot be understood by using only one method, such as studying the impact of a policy on a community’s well-being.
- When the research question involves studying a topic that has not been well-researched, and using mixed methods can help provide a more comprehensive understanding of the topic.
Purpose of Mixed Methods Research
The purpose of mixed methods research is to provide a more comprehensive understanding of a research problem than can be obtained through either quantitative or qualitative methods alone.
Mixed methods research is particularly useful when the research problem is complex and requires a deep understanding of the context and subjective experiences of participants, as well as the ability to generalize findings to a larger population. By combining both qualitative and quantitative methods, researchers can obtain a more complete picture of the research problem and its underlying mechanisms, as well as test hypotheses and identify patterns that may not be apparent with only one method.
Overall, mixed methods research aims to provide a more holistic and nuanced understanding of the research problem, allowing researchers to draw more valid and reliable conclusions, make more informed decisions, and develop more effective interventions and policies.
Advantages of Mixed Methods Research
Mixed methods research offers several advantages over using only qualitative or quantitative research methods. Here are some of the main advantages of mixed methods research:
- Comprehensive understanding: Mixed methods research provides a more comprehensive understanding of the research problem by combining both qualitative and quantitative data, which allows for a more nuanced interpretation of the data.
- Triangulation : Mixed methods research allows for triangulation, which is the use of multiple sources of data to verify findings. This improves the validity and reliability of the research.
- Addressing limitations: Mixed methods research can address the limitations of qualitative or quantitative research by compensating for the weaknesses of each method.
- Flexibility : Mixed methods research is flexible, allowing researchers to adapt the research design and methods as needed to best address the research question.
- Validity : Mixed methods research can increase the validity of the research by using multiple methods to measure the same concept.
- Generalizability : Mixed methods research can improve the generalizability of the findings by using quantitative data to test the applicability of qualitative findings to a larger population.
- Practical applications: Mixed methods research is useful for developing practical applications, such as interventions or policies, as it provides a more comprehensive understanding of the research problem.
Limitations of Mixed Methods Research
Here are some of the main limitations of mixed methods research:
- Time-consuming: Mixed methods research can be time-consuming and may require more resources than using only one research method.
- Complex data analysis: Integrating qualitative and quantitative data can be challenging and requires specialized skills for data analysis.
- Sampling bias: Mixed methods research can be subject to sampling bias, particularly if the sampling strategies for the qualitative and quantitative components are not aligned.
- Validity and reliability: Mixed methods research requires careful attention to the validity and reliability of both the qualitative and quantitative data, as well as the integration of the two data types.
- Difficulty in balancing the two methods: Mixed methods research can be difficult to balance the qualitative and quantitative methods effectively, particularly if one method dominates the other.
- Theoretical and philosophical issues: Mixed methods research raises theoretical and philosophical questions about the compatibility of qualitative and quantitative research methods and the underlying assumptions about the nature of reality and knowledge.
About the author
Muhammad Hassan
Researcher, Academic Writer, Web developer
You may also like

Questionnaire – Definition, Types, and Examples

Case Study – Methods, Examples and Guide

Observational Research – Methods and Guide

Quantitative Research – Methods, Types and...

Qualitative Research Methods

Explanatory Research – Types, Methods, Guide
The Use of Mixed Methods in Research
- Reference work entry
- First Online: 13 January 2019
- Cite this reference work entry
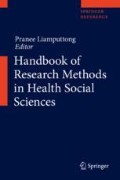
- Kate A. McBride 2 ,
- Freya MacMillan 3 ,
- Emma S. George 4 &
- Genevieve Z. Steiner 5
2470 Accesses
9 Citations
Mixed methods research is becoming increasingly popular and is widely acknowledged as a means of achieving a more complex understanding of research problems. Combining both the in-depth, contextual views of qualitative research with the broader generalizations of larger population quantitative approaches, mixed methods research can be used to produce a rigorous and credible source of data. Using this methodology, the same core issue is investigated through the collection, analysis, and interpretation of both types of data within one study or a series of studies. Multiple designs are possible and can be guided by philosophical assumptions. Both qualitative and quantitative data can be collected simultaneously or sequentially (in any order) through a multiphase project. Integration of the two data sources then occurs with consideration is given to the weighting of both sources; these can either be equal or one can be prioritized over the other. Designed as a guide for novice mixed methods researchers, this chapter gives an overview of the historical and philosophical roots of mixed methods research. We also provide a practical overview of its application in health research as well as pragmatic considerations for those wishing to undertake mixed methods research.
This is a preview of subscription content, log in via an institution to check access.
Access this chapter
- Available as PDF
- Read on any device
- Instant download
- Own it forever
- Available as EPUB and PDF
- Durable hardcover edition
- Dispatched in 3 to 5 business days
- Free shipping worldwide - see info
Tax calculation will be finalised at checkout
Purchases are for personal use only
Institutional subscriptions
Appleton JV, King L. Journeying from the philosophical contemplation of constructivism to the methodological pragmatics of health services research. J Adv Nurs. 2002;40(6):641–8.
Article Google Scholar
Baba CT, Oliveira IM, Silva AEF, Vieira LM, Cerri NC, Florindo AA, de Oliveira Gomes GA. Evaluating the impact of a walking program in a disadvantaged area: using the RE-AIM framework by mixed methods. BMC Public Health. 2017;17(1):709. https://doi.org/10.1186/s12889-017-4698-5 .
Bryman A. The research question in social research: what is its role? Int J Soc Res Methodol. 2007;10(1):5–20. https://doi.org/10.1080/13645570600655282 .
Bryman A. The end of the paradigm wars. In: Alasuutari P, Bickman L, Brannen J, editors. The Sage handbook of social research methods. Thousand Oaks: Sage; 2008. p. 13–25.
Google Scholar
Caracelli VJ, Greene JC. Data-analysis strategies for mixed-method evaluation designs. Educ Eval Policy Anal. 1993;15(2):195–207. https://doi.org/10.3102/01623737015002195 .
Castro FG, Kellison JG, Boyd SJ, Kopak A. A methodology for conducting integrative mixed methods research and data analyses. J Mixed Methods Res. 2010;4(4):342–60. https://doi.org/10.1177/1558689810382916 .
Center for Innovation in Teaching in Research. Choosing a mixed methods design. 2017. Retrieved 15 Nov 2017 from https://cirt.gcu.edu/research/developmentresources/research_ready/mixed_methods/choosing_design .
Creswell JW, Plano Clark VL. Designing and conducting mixed methods research. 3rd ed. Thousand Oaks: Sage; 2018.
Curry LA, Krumholz HM, O’Cathain A, Clark VLP, Cherlin E, Bradley EH. Mixed methods in biomedical and health services research. Circ-Cardiovasc Qual Outcomes. 2013;6(1):119–23. https://doi.org/10.1161/circoutcomes.112.967885 .
Denscombe M. Communities of practice: a research paradigm for the mixed methods approach. J Mixed Methods Res. 2008;2(3):270–83.
Doyle L, Brady A-M, Byrne G. An overview of mixed methods research. J Res Nurs. 2009;14(2):175–85.
Foster NE, Bishop A, Bartlam B, Ogollah R, Barlas P, Holden M, … Young J. Evaluating acupuncture and standard carE for pregnant women with back pain (EASE back): a feasibility study and pilot randomised trial. Health Technol Assess. 2016;20(33):1–236. https://doi.org/10.3310/hta20330 .
Haider AH, Schneider EB, Kodadek LM, Adler RR, Ranjit A, Torain M, … Lau BD. Emergency department query for patient-centered approaches to sexual orientation and gender identity the EQUALITY study. JAMA Intern Med. 2017;177(6):819–828. https://doi.org/10.1001/jamainternmed.2017.0906 .
Harding KE, Taylor NF, Bowers B, Stafford M, Leggat SG. Clinician and patient perspectives of a new model of triage in a community rehabilitation program that reduced waiting time: a qualitative analysis. Aust Health Rev. 2013;37(3):324–30. https://doi.org/10.1071/ah13033 .
Hoddinott P, Britten J, Prescott GJ, Tappin D, Ludbrook A, Godden DJ. Effectiveness of policy to provide breastfeeding groups ( BIG) for pregnant and breastfeeding mothers in primary care: cluster randomised controlled trial. Br Med J. 2009;338:a3026, 10. https://doi.org/10.1136/bmj.a3026 .
Ivankova NV, Creswell JW, Stick SL. Using mixed-methods sequential explanatory design: from theory to practice. Field Methods. 2006;18(1):3–20. https://doi.org/10.1177/1525822x05282260 .
Johnson B, Gray R. A history of philosophical and theoretical issues for mixed methods research. In: Tashakkori A, Teddlie C, editors. Sage handbook of mixed methods in social and behavioral research. 2nd ed. Thousand Oaks: Sage; 2010. p. 69–94.
Chapter Google Scholar
Johnson BR, Onwuegbuzie AJ. Mixed methods research: a research paradigm whose time has come. Educ Res. 2004;33(7):14–26. https://doi.org/10.3102/0013189x033007014 .
Keeney S, McKenna H, Fleming P, McIlfatrick S. Attitudes to cancer and cancer prevention: what do people aged 35–54 years think? Eur J Cancer Care. 2010;19(6):769–77. https://doi.org/10.1111/j.1365-2354.2009.01137.x .
McBride KA, Ballinger ML, Schlub TE, Young MA, Tattersall MHN, Kirk J, et al. Psychosocial morbidity in TP53 mutation carriers: is whole-body cancer screening beneficial? Familial Cancer. 2017;16(3):423–32. https://doi.org/10.1007/s10689-016-9964-7 .
Mertens DM. Transformative mixed methods: addressing inequities. Am Behav Sci. 2012;56(6):802–13. https://doi.org/10.1177/0002764211433797 .
Moffatt S, White M, Mackintosh J, Howel D. Using quantitative and qualitative data in health services research – what happens when mixed method findings conflict? BMC Health Serv Res. 2006;6:28. https://doi.org/10.1186/1472-6963-6-28 .
Morse JM. Approaches to qualitative-quantitative methodological triangulation. Nurs Res. 1991a;40(2):120–3.
Morse JM. Principles of mixed methods and multimethod research design. In: Tashakkori A, Teddilie C, editors. SAGE handbook of mixed methods in social and behavioral research. Thousand Oaks: Sage; 1991b.
Mutrie N, Doolin O, Fitzsimons CF, Grant PM, Granat M, Grealy M, et al. Increasing older adults’ walking through primary care: results of a pilot randomized controlled trial. Fam Pract. 2012;29(6):633–42. https://doi.org/10.1093/fampra/cms038 .
Newman I, Ridenour C, Newman C, De Marco G. A typology of research purposes and its relationship to mixed methods in social and behavioral research. Thousand Oaks: Sage; 2003. p. 167–88.
O’Cathain A, Murphy E, Nicholl J. The quality of mixed methods studies in health services research. J Health Serv Res Policy. 2008;13(2):92–8. https://doi.org/10.1258/jhsrp.2007.007074 .
Onwuegbuzie AJ, Collins KMT. A typology of mixed methods sampling designs in social science research. Qual Rep. 2007;12(2):281–316.
Plano Clark VL, Badiee M. Research questions in mixed methods research. In: Tashakkori A, Teddlie C, editors. SAGE handbook of mixed methods in social and behavioral research. 2nd ed. Thousand Oaks: Sage; 2010. p. 275–304.
Prades, J., Algara, M., Espinas, J. A., Farrus, B., Arenas, M., Reyes, V., . . . Borras, J. M. (2017). Understanding variations in the use of hypofractionated radiotherapy and its specific indications for breast cancer: a mixed-methods study. Radiother Oncol, 123(1), 22–28. doi: https://doi.org/10.1016/j.radonc.2017.01.014 .
Tariq S, Woodman J. Using mixed methods in health research. JRSM Short Rep. 2013; 4 (6):1–8. https://doi.org/10.1177/2042533313479197 .
Tashakkori A, Creswell JW. Editorial: the new era of mixed methods. J Mixed Methods Res. 2007;1(1):3–7. https://doi.org/10.1177/2345678906293042 .
Tashakkori A, Teddlie C. The past and future of mixed methods research: from data triangulation to mixed model designs. In: Tashakkori A, Teddlie C, editors. Handbook of mixed methods in social & behavioral research. Thousand Oaks: Sage; 2003. p. 671–701.
Teddlie C, Tashakkori A. Foundations of mixed methods research: integrating quantitative and qualitative approaches in the social and behavioral sciences. Thousand Oaks: Sage; 2009.
Teddlie C, Yu F. Mixed methods sampling a typology with examples. J Mixed Methods Res. 2007;1(1):77–100. https://doi.org/10.1177/2345678906292430 .
Wellard SJ, Rasmussen B, Savage S, Dunning T. Exploring staff diabetes medication knowledge and practices in regional residential care: triangulation study. J Clin Nurs. 2013;22(13–14):1933–40. https://doi.org/10.1111/jocn.12043 .
Zhang W. Mixed methods application in health intervention research: a multiple case study. Int J Mult Res Approaches. 2014;8(1):24–35. https://doi.org/10.5172/mra.2014.8.1.24 .
Download references
Author information
Authors and affiliations.
School of Medicine and Translational Health Research Institute, Western Sydney University, Sydney, NSW, Australia
Kate A. McBride
School of Science and Health and Translational Health Research Institute (THRI), Western Sydney University, Penrith, NSW, Australia
Freya MacMillan
School of Science and Health, Western Sydney University, Sydney, NSW, Australia
Emma S. George
NICM and Translational Health Research Institute (THRI), Western Sydney University, Penrith, NSW, Australia
Genevieve Z. Steiner
You can also search for this author in PubMed Google Scholar
Corresponding author
Correspondence to Kate A. McBride .
Editor information
Editors and affiliations.
School of Science and Health, Western Sydney University, Penrith, NSW, Australia
Pranee Liamputtong
Rights and permissions
Reprints and permissions
Copyright information
© 2019 Springer Nature Singapore Pte Ltd.
About this entry
Cite this entry.
McBride, K.A., MacMillan, F., George, E.S., Steiner, G.Z. (2019). The Use of Mixed Methods in Research. In: Liamputtong, P. (eds) Handbook of Research Methods in Health Social Sciences. Springer, Singapore. https://doi.org/10.1007/978-981-10-5251-4_97
Download citation
DOI : https://doi.org/10.1007/978-981-10-5251-4_97
Published : 13 January 2019
Publisher Name : Springer, Singapore
Print ISBN : 978-981-10-5250-7
Online ISBN : 978-981-10-5251-4
eBook Packages : Social Sciences Reference Module Humanities and Social Sciences Reference Module Business, Economics and Social Sciences
Share this entry
Anyone you share the following link with will be able to read this content:
Sorry, a shareable link is not currently available for this article.
Provided by the Springer Nature SharedIt content-sharing initiative
- Publish with us
Policies and ethics
- Find a journal
- Track your research
- Subject List
- Take a Tour
- For Authors
- Subscriber Services
- Publications
- African American Studies
- African Studies
- American Literature
- Anthropology
- Architecture Planning and Preservation
- Art History
- Atlantic History
- Biblical Studies
- British and Irish Literature
- Childhood Studies
- Chinese Studies
- Cinema and Media Studies
- Communication
- Criminology
- Environmental Science
- Evolutionary Biology
- International Law
- International Relations
- Islamic Studies
- Jewish Studies
- Latin American Studies
- Latino Studies
- Linguistics
- Literary and Critical Theory
- Medieval Studies
- Military History
- Political Science
- Public Health
- Renaissance and Reformation
- Social Work
- Urban Studies
- Victorian Literature
- Browse All Subjects
How to Subscribe
- Free Trials
In This Article Expand or collapse the "in this article" section Mixed Methods Research
Introduction, general overviews.
- Reference Works
- Conferences
- Social Justice
- Early Works
- Recent Thinking
- Rationale for Mixing Methods
- Purpose for Mixing Methods
- Research Questions
- Research Designs
- Collecting Data
- Using Technology
- Data Legitimation
- Triangulation
- Writing Research Reports
- Writing Grants
Related Articles Expand or collapse the "related articles" section about
About related articles close popup.
Lorem Ipsum Sit Dolor Amet
Vestibulum ante ipsum primis in faucibus orci luctus et ultrices posuere cubilia Curae; Aliquam ligula odio, euismod ut aliquam et, vestibulum nec risus. Nulla viverra, arcu et iaculis consequat, justo diam ornare tellus, semper ultrices tellus nunc eu tellus.
- Action Research in Education
- Case Study in Education Research
- Data Collection in Educational Research
- Educational Statistics for Longitudinal Research
- Grounded Theory
- Literature Reviews
- Meta-Analysis and Research Synthesis in Education
- Methodologies for Conducting Education Research
- Qualitative Data Analysis Techniques
- Qualitative, Quantitative, and Mixed Methods Research Sampling Strategies
- Qualitative Research Design
- Quantitative Research Designs in Educational Research
- Statistical Assumptions
Other Subject Areas
Forthcoming articles expand or collapse the "forthcoming articles" section.
- Gender, Power, and Politics in the Academy
- Girls' Education in the Developing World
- Non-Formal & Informal Environmental Education
- Find more forthcoming articles...
- Export Citations
- Share This Facebook LinkedIn Twitter
Mixed Methods Research by Nancy Leech LAST REVIEWED: 29 July 2020 LAST MODIFIED: 22 April 2013 DOI: 10.1093/obo/9780199756810-0074
Mixed methods research refers to studies in which researchers utilize qualitative and quantitative techniques, integrate findings, and draw inferences from both the qualitative and quantitative components. Researchers have been conducting mixed methods research for decades, yet it has recently become more prominent as a form of inquiry. Researchers who conduct mixed methods studies are faced with many challenges such as definitions of mixed methods, mixed methods research designs, integration of qualitative and quantitative data, sampling techniques, and using mixed methods research to promote social justice, to name just a few. It is important to note that mixed methods research is a field of its own with unique techniques and methods. Quantitative and qualitative research fields are more mature and researchers have agreed upon designs, sampling techniques, and so on. Yet, there is not much agreement in the mixed methods field on many of these topics, and there are multiple ideas available regarding the different steps of the research process (e.g., there are many types of mixed methods research designs available to researchers). Indeed, there is consensus that this field is changing and growing. This situation presents researchers with various challenges. One such challenge is that conducting mixed methods research can be difficult because a researcher needs to have knowledge of both qualitative and quantitative techniques. One remedy for this is to work in teams. When conducting mixed methods research it is imperative for researchers to learn how to integrate the qualitative and quantitative strands so that the results from mixed methods research studies provide deep understanding of the phenomena under investigation.
These articles are good for a general understanding of mixed methods research. Johnson and Onwuegbuzie 2004 presents data from leaders in the field answering the question “What is mixed methods research?” and includes their views of how mixed methods is its own research paradigm. The follow-up article Johnson, et al. 2007 also includes interviews with leaders in the field as well as a definition of mixed methods research. Greene 2008 and Greene 2012 outline how mixed methods research is its own methodology and that mixed methods research leads to more meaningful results than studies conducted using only one methodology. Finally, Teddlie and Tashakkori 2012 outlines what researchers should consider before engaging in a mixed methods study.
Greene, J. C. 2008. Is mixed methods social inquiry a distinctive methodology? Journal of Mixed Methods Research 2:7–22.
DOI: 10.1177/1558689807309969
Reviews the practical guidelines, philosophy, methodology, and sociopolitical commitments of mixed methods research to promote the field as its own methodology. Explores paradigms and presents the idea of mixing paradigms. This article is helpful for understanding that mixed methods research is a stand-alone methodology.
Greene, J. C. 2012. Engaging critical issues in social inquiry by mixing methods. American Behavioral Scientist 56:755–773.
DOI: 10.1177/0002764211433794
Argues that mixed methods research provides a deeper understanding of phenomena than either qualitative or quantitative methods. Two research articles, one qualitative and one quantitative, are examined and critiqued to show how conducting these studies using mixed methods research would have enriched the results.
Johnson, R. B., and A. J. Onwuegbuzie. 2004. Mixed methods research: A research paradigm whose time has come. Educational Researcher 33:13–36.
DOI: 10.3102/0013189X033007014
Includes responses from leaders in the field to the question “What is mixed methods research?” The authors use this information to develop an overarching definition of mixed methods research. Suggests that mixed methods is its own research paradigm. Good introduction to mixed methods research.
Johnson, R. B., A. J. Onwuegbuzie, and L. A. Turner. 2007. Toward a definition of mixed methods research. Journal of Mixed Methods Research 1:112–133.
DOI: 10.1177/1558689806298224
Argues that mixed methods research is one of the three major research paradigms. Based on interviews with leaders in the field of mixed methods, this article defines mixed methods research. Describes the history of mixed methods research and delineates future related issues.
Teddlie, C., and A. Tashakkori. 2012. Common “core” characteristics of mixed methods research: A review of critical issues and call for greater convergence. American Behavioral Scientist 56:774–788.
DOI: 10.1177/0002764211433795
Addressing 21st-century controversies in the field, these authors present four issues: training mixed methods researchers to have methodological eclecticism (i.e., having abilities in using both qualitative and quantitative techniques), using paradigm pluralism (i.e., the possibility of having more than one paradigm underlying one study), developing research and analytic techniques specific to mixed methods, and utilizing an iterative approach to research.
back to top
Users without a subscription are not able to see the full content on this page. Please subscribe or login .
Oxford Bibliographies Online is available by subscription and perpetual access to institutions. For more information or to contact an Oxford Sales Representative click here .
- About Education »
- Meet the Editorial Board »
- Academic Achievement
- Academic Audit for Universities
- Academic Freedom and Tenure in the United States
- Adjuncts in Higher Education in the United States
- Administrator Preparation
- Adolescence
- Advanced Placement and International Baccalaureate Courses
- Advocacy and Activism in Early Childhood
- African American Racial Identity and Learning
- Alaska Native Education
- Alternative Certification Programs for Educators
- Alternative Schools
- American Indian Education
- Animals in Environmental Education
- Art Education
- Artificial Intelligence and Learning
- Assessing School Leader Effectiveness
- Assessment, Behavioral
- Assessment, Educational
- Assessment in Early Childhood Education
- Assistive Technology
- Augmented Reality in Education
- Beginning-Teacher Induction
- Bilingual Education and Bilingualism
- Black Undergraduate Women: Critical Race and Gender Perspe...
- Blended Learning
- Changing Professional and Academic Identities
- Character Education
- Children’s and Young Adult Literature
- Children's Beliefs about Intelligence
- Children's Rights in Early Childhood Education
- Citizenship Education
- Civic and Social Engagement of Higher Education
- Classroom Learning Environments: Assessing and Investigati...
- Classroom Management
- Coherent Instructional Systems at the School and School Sy...
- College Admissions in the United States
- College Athletics in the United States
- Community Relations
- Comparative Education
- Computer-Assisted Language Learning
- Computer-Based Testing
- Conceptualizing, Measuring, and Evaluating Improvement Net...
- Continuous Improvement and "High Leverage" Educational Pro...
- Counseling in Schools
- Critical Approaches to Gender in Higher Education
- Critical Perspectives on Educational Innovation and Improv...
- Critical Race Theory
- Crossborder and Transnational Higher Education
- Cross-National Research on Continuous Improvement
- Cross-Sector Research on Continuous Learning and Improveme...
- Cultural Diversity in Early Childhood Education
- Culturally Responsive Leadership
- Culturally Responsive Pedagogies
- Culturally Responsive Teacher Education in the United Stat...
- Curriculum Design
- Data-driven Decision Making in the United States
- Deaf Education
- Desegregation and Integration
- Design Thinking and the Learning Sciences: Theoretical, Pr...
- Development, Moral
- Dialogic Pedagogy
- Digital Age Teacher, The
- Digital Citizenship
- Digital Divides
- Disabilities
- Distance Learning
- Distributed Leadership
- Doctoral Education and Training
- Early Childhood Education and Care (ECEC) in Denmark
- Early Childhood Education and Development in Mexico
- Early Childhood Education in Aotearoa New Zealand
- Early Childhood Education in Australia
- Early Childhood Education in China
- Early Childhood Education in Europe
- Early Childhood Education in Sub-Saharan Africa
- Early Childhood Education in Sweden
- Early Childhood Education Pedagogy
- Early Childhood Education Policy
- Early Childhood Education, The Arts in
- Early Childhood Mathematics
- Early Childhood Science
- Early Childhood Teacher Education
- Early Childhood Teachers in Aotearoa New Zealand
- Early Years Professionalism and Professionalization Polici...
- Economics of Education
- Education For Children with Autism
- Education for Sustainable Development
- Education Leadership, Empirical Perspectives in
- Education of Native Hawaiian Students
- Education Reform and School Change
- Educator Partnerships with Parents and Families with a Foc...
- Emotional and Affective Issues in Environmental and Sustai...
- Emotional and Behavioral Disorders
- Environmental and Science Education: Overlaps and Issues
- Environmental Education
- Environmental Education in Brazil
- Epistemic Beliefs
- Equity and Improvement: Engaging Communities in Educationa...
- Equity, Ethnicity, Diversity, and Excellence in Education
- Ethical Research with Young Children
- Ethics and Education
- Ethics of Teaching
- Ethnic Studies
- Evidence-Based Communication Assessment and Intervention
- Family and Community Partnerships in Education
- Family Day Care
- Federal Government Programs and Issues
- Feminization of Labor in Academia
- Finance, Education
- Financial Aid
- Formative Assessment
- Future-Focused Education
- Gender and Achievement
- Gender and Alternative Education
- Gender-Based Violence on University Campuses
- Gifted Education
- Global Mindedness and Global Citizenship Education
- Global University Rankings
- Governance, Education
- Growth of Effective Mental Health Services in Schools in t...
- Higher Education and Globalization
- Higher Education and the Developing World
- Higher Education Faculty Characteristics and Trends in the...
- Higher Education Finance
- Higher Education Governance
- Higher Education Graduate Outcomes and Destinations
- Higher Education in Africa
- Higher Education in China
- Higher Education in Latin America
- Higher Education in the United States, Historical Evolutio...
- Higher Education, International Issues in
- Higher Education Management
- Higher Education Policy
- Higher Education Research
- Higher Education Student Assessment
- High-stakes Testing
- History of Early Childhood Education in the United States
- History of Education in the United States
- History of Technology Integration in Education
- Homeschooling
- Inclusion in Early Childhood: Difference, Disability, and ...
- Inclusive Education
- Indigenous Education in a Global Context
- Indigenous Learning Environments
- Indigenous Students in Higher Education in the United Stat...
- Infant and Toddler Pedagogy
- Inservice Teacher Education
- Integrating Art across the Curriculum
- Intelligence
- Intensive Interventions for Children and Adolescents with ...
- International Perspectives on Academic Freedom
- Intersectionality and Education
- Knowledge Development in Early Childhood
- Leadership Development, Coaching and Feedback for
- Leadership in Early Childhood Education
- Leadership Training with an Emphasis on the United States
- Learning Analytics in Higher Education
- Learning Difficulties
- Learning, Lifelong
- Learning, Multimedia
- Learning Strategies
- Legal Matters and Education Law
- LGBT Youth in Schools
- Linguistic Diversity
- Linguistically Inclusive Pedagogy
- Literacy Development and Language Acquisition
- Mathematics Identity
- Mathematics Instruction and Interventions for Students wit...
- Mathematics Teacher Education
- Measurement for Improvement in Education
- Measurement in Education in the United States
- Methodological Approaches for Impact Evaluation in Educati...
- Mindfulness, Learning, and Education
- Mixed Methods Research
- Motherscholars
- Multiliteracies in Early Childhood Education
- Multiple Documents Literacy: Theory, Research, and Applica...
- Multivariate Research Methodology
- Museums, Education, and Curriculum
- Music Education
- Narrative Research in Education
- Native American Studies
- Note-Taking
- Numeracy Education
- One-to-One Technology in the K-12 Classroom
- Online Education
- Open Education
- Organizing for Continuous Improvement in Education
- Organizing Schools for the Inclusion of Students with Disa...
- Outdoor Play and Learning
- Outdoor Play and Learning in Early Childhood Education
- Pedagogical Leadership
- Pedagogy of Teacher Education, A
- Performance Objectives and Measurement
- Performance-based Research Assessment in Higher Education
- Performance-based Research Funding
- Phenomenology in Educational Research
- Philosophy of Education
- Physical Education
- Podcasts in Education
- Policy Context of United States Educational Innovation and...
- Politics of Education
- Portable Technology Use in Special Education Programs and ...
- Post-humanism and Environmental Education
- Pre-Service Teacher Education
- Problem Solving
- Productivity and Higher Education
- Professional Development
- Professional Learning Communities
- Program Evaluation
- Programs and Services for Students with Emotional or Behav...
- Psychology Learning and Teaching
- Psychometric Issues in the Assessment of English Language ...
- Qualitative, Quantitative, and Mixed Methods Research Samp...
- Queering the English Language Arts (ELA) Writing Classroom
- Race and Affirmative Action in Higher Education
- Reading Education
- Refugee and New Immigrant Learners
- Relational and Developmental Trauma and Schools
- Relational Pedagogies in Early Childhood Education
- Reliability in Educational Assessments
- Religion in Elementary and Secondary Education in the Unit...
- Researcher Development and Skills Training within the Cont...
- Research-Practice Partnerships in Education within the Uni...
- Response to Intervention
- Restorative Practices
- Risky Play in Early Childhood Education
- Scale and Sustainability of Education Innovation and Impro...
- Scaling Up Research-based Educational Practices
- School Accreditation
- School Choice
- School Culture
- School District Budgeting and Financial Management in the ...
- School Improvement through Inclusive Education
- School Reform
- Schools, Private and Independent
- School-Wide Positive Behavior Support
- Science Education
- Secondary to Postsecondary Transition Issues
- Self-Regulated Learning
- Self-Study of Teacher Education Practices
- Service-Learning
- Severe Disabilities
- Single Salary Schedule
- Single-sex Education
- Single-Subject Research Design
- Social Context of Education
- Social Network Analysis
- Social Pedagogy
- Social Science and Education Research
- Social Studies Education
- Sociology of Education
- Standards-Based Education
- Student Access, Equity, and Diversity in Higher Education
- Student Assignment Policy
- Student Engagement in Tertiary Education
- Student Learning, Development, Engagement, and Motivation ...
- Student Participation
- Student Voice in Teacher Development
- Sustainability Education in Early Childhood Education
- Sustainability in Early Childhood Education
- Sustainability in Higher Education
- Teacher Beliefs and Epistemologies
- Teacher Collaboration in School Improvement
- Teacher Evaluation and Teacher Effectiveness
- Teacher Preparation
- Teacher Training and Development
- Teacher Unions and Associations
- Teacher-Student Relationships
- Teaching Critical Thinking
- Technologies, Teaching, and Learning in Higher Education
- Technology Education in Early Childhood
- Technology, Educational
- Technology-based Assessment
- The Bologna Process
- The Regulation of Standards in Higher Education
- Theories of Educational Leadership
- Three Conceptions of Literacy: Media, Narrative, and Gamin...
- Tracking and Detracking
- Traditions of Quality Improvement in Education
- Transformative Learning
- Transitions in Early Childhood Education
- Tribally Controlled Colleges and Universities in the Unite...
- Understanding the Psycho-Social Dimensions of Schools and ...
- University Faculty Roles and Responsibilities in the Unite...
- Using Ethnography in Educational Research
- Value of Higher Education for Students and Other Stakehold...
- Virtual Learning Environments
- Vocational and Technical Education
- Wellness and Well-Being in Education
- Women's and Gender Studies
- Young Children and Spirituality
- Young Children's Learning Dispositions
- Young Children's Working Theories
- Privacy Policy
- Cookie Policy
- Legal Notice
- Accessibility
Powered by:
- [66.249.64.20|185.66.15.189]
- 185.66.15.189
- What is mixed methods research?
Last updated
20 February 2023
Reviewed by
Miroslav Damyanov
By blending both quantitative and qualitative data, mixed methods research allows for a more thorough exploration of a research question. It can answer complex research queries that cannot be solved with either qualitative or quantitative research .
Analyze your mixed methods research
Dovetail streamlines analysis to help you uncover and share actionable insights
Mixed methods research combines the elements of two types of research: quantitative and qualitative.
Quantitative data is collected through the use of surveys and experiments, for example, containing numerical measures such as ages, scores, and percentages.
Qualitative data involves non-numerical measures like beliefs, motivations, attitudes, and experiences, often derived through interviews and focus group research to gain a deeper understanding of a research question or phenomenon.
Mixed methods research is often used in the behavioral, health, and social sciences, as it allows for the collection of numerical and non-numerical data.
- When to use mixed methods research
Mixed methods research is a great choice when quantitative or qualitative data alone will not sufficiently answer a research question. By collecting and analyzing both quantitative and qualitative data in the same study, you can draw more meaningful conclusions.
There are several reasons why mixed methods research can be beneficial, including generalizability, contextualization, and credibility.
For example, let's say you are conducting a survey about consumer preferences for a certain product. You could collect only quantitative data, such as how many people prefer each product and their demographics. Or you could supplement your quantitative data with qualitative data, such as interviews and focus groups , to get a better sense of why people prefer one product over another.
It is important to note that mixed methods research does not only mean collecting both types of data. Rather, it also requires carefully considering the relationship between the two and method flexibility.
You may find differing or even conflicting results by combining quantitative and qualitative data . It is up to the researcher to then carefully analyze the results and consider them in the context of the research question to draw meaningful conclusions.
When designing a mixed methods study, it is important to consider your research approach, research questions, and available data. Think about how you can use different techniques to integrate the data to provide an answer to your research question.
- Mixed methods research design
A mixed methods research design is an approach to collecting and analyzing both qualitative and quantitative data in a single study.
Mixed methods designs allow for method flexibility and can provide differing and even conflicting results. Examples of mixed methods research designs include convergent parallel, explanatory sequential, and exploratory sequential.
By integrating data from both quantitative and qualitative sources, researchers can gain valuable insights into their research topic . For example, a study looking into the impact of technology on learning could use surveys to measure quantitative data on students' use of technology in the classroom. At the same time, interviews or focus groups can provide qualitative data on students' experiences and opinions.
- Types of mixed method research designs
Researchers often struggle to put mixed methods research into practice, as it is challenging and can lead to research bias. Although mixed methods research can reveal differences or conflicting results between studies, it can also offer method flexibility.
Designing a mixed methods study can be broken down into four types: convergent parallel, embedded, explanatory sequential, and exploratory sequential.
Convergent parallel
The convergent parallel design is when data collection and analysis of both quantitative and qualitative data occur simultaneously and are analyzed separately. This design aims to create mutually exclusive sets of data that inform each other.
For example, you might interview people who live in a certain neighborhood while also conducting a survey of the same people to determine their satisfaction with the area.
Embedded design
The embedded design is when the quantitative and qualitative data are collected simultaneously, but the qualitative data is embedded within the quantitative data. This design is best used when you want to focus on the quantitative data but still need to understand how the qualitative data further explains it.
For instance, you may survey students about their opinions of an online learning platform and conduct individual interviews to gain further insight into their responses.
Explanatory sequential design
In an explanatory sequential design, quantitative data is collected first, followed by qualitative data. This design is used when you want to further explain a set of quantitative data with additional qualitative information.
An example of this would be if you surveyed employees at a company about their satisfaction with their job and then conducted interviews to gain more information about why they responded the way they did.
Exploratory sequential design
The exploratory sequential design collects qualitative data first, followed by quantitative data. This type of mixed methods research is used when the goal is to explore a topic before collecting any quantitative data.
An example of this could be studying how parents interact with their children by conducting interviews and then using a survey to further explore and measure these interactions.
Integrating data in mixed methods studies can be challenging, but it can be done successfully with careful planning.
No matter which type of design you choose, understanding and applying these principles can help you draw meaningful conclusions from your research.
- Strengths of mixed methods research
Mixed methods research designs combine the strengths of qualitative and quantitative data, deepening and enriching qualitative results with quantitative data and validating quantitative findings with qualitative data. This method offers more flexibility in designing research, combining theory generation and hypothesis testing, and being less tied to disciplines and established research paradigms.
Take the example of a study examining the impact of exercise on mental health. Mixed methods research would allow for a comprehensive look at the issue from different angles.
Researchers could begin by collecting quantitative data through surveys to get an overall view of the participants' levels of physical activity and mental health. Qualitative interviews would follow this to explore the underlying dynamics of participants' experiences of exercise, physical activity, and mental health in greater detail.
Through a mixed methods approach, researchers could more easily compare and contrast their results to better understand the phenomenon as a whole.
Additionally, mixed methods research is useful when there are conflicting or differing results in different studies. By combining both quantitative and qualitative data, mixed methods research can offer insights into why those differences exist.
For example, if a quantitative survey yields one result while a qualitative interview yields another, mixed methods research can help identify what factors influence these differences by integrating data from both sources.
Overall, mixed methods research designs offer a range of advantages for studying complex phenomena. They can provide insight into different elements of a phenomenon in ways that are not possible with either qualitative or quantitative data alone. Additionally, they allow researchers to integrate data from multiple sources to gain a deeper understanding of the phenomenon in question.
- Challenges of mixed methods research
Mixed methods research is labor-intensive and often requires interdisciplinary teams of researchers to collaborate. It also has the potential to cost more than conducting a stand alone qualitative or quantitative study .
Interpreting the results of mixed methods research can be tricky, as it can involve conflicting or differing results. Researchers must find ways to systematically compare the results from different sources and methods to avoid bias.
For example, imagine a situation where a team of researchers has employed an explanatory sequential design for their mixed methods study. After collecting data from both the quantitative and qualitative stages, the team finds that the two sets of data provide differing results. This could be challenging for the team, as they must now decide how to effectively integrate the two types of data in order to reach meaningful conclusions. The team would need to identify method flexibility and be strategic when integrating data in order to draw meaningful conclusions from the conflicting results.
- Advanced frameworks in mixed methods research
Mixed methods research offers powerful tools for investigating complex processes and systems, such as in health and healthcare.
Besides the three basic mixed method designs—exploratory sequential, explanatory sequential, and convergent parallel—you can use one of the four advanced frameworks to extend mixed methods research designs. These include multistage, intervention, case study , and participatory.
This framework mixes qualitative and quantitative data collection methods in stages to gather a more nuanced view of the research question. An example of this is a study that first has an online survey to collect initial data and is followed by in-depth interviews to gain further insights.
Intervention
This design involves collecting quantitative data and then taking action, usually in the form of an intervention or intervention program. An example of this could be a research team who collects data from a group of participants, evaluates it, and then implements an intervention program based on their findings .
This utilizes both qualitative and quantitative research methods to analyze a single case. The researcher will examine the specific case in detail to understand the factors influencing it. An example of this could be a study of a specific business organization to understand the organizational dynamics and culture within the organization.
Participatory
This type of research focuses on the involvement of participants in the research process. It involves the active participation of participants in formulating and developing research questions, data collection, and analysis.
An example of this could be a study that involves forming focus groups with participants who actively develop the research questions and then provide feedback during the data collection and analysis stages.
The flexibility of mixed methods research designs means that researchers can choose any combination of the four frameworks outlined above and other methodologies , such as convergent parallel, explanatory sequential, and exploratory sequential, to suit their particular needs.
Through this method's flexibility, researchers can gain multiple perspectives and uncover differing or even conflicting results when integrating data.
When it comes to integration at the methods level, there are four approaches.
Connecting involves collecting both qualitative and quantitative data during different phases of the research.
Building involves the collection of both quantitative and qualitative data within a single phase.
Merging involves the concurrent collection of both qualitative and quantitative data.
Embedding involves including qualitative data within a quantitative study or vice versa.
- Techniques for integrating data in mixed method studies
Integrating data is an important step in mixed methods research designs. It allows researchers to gain further understanding from their research and gives credibility to the integration process. There are three main techniques for integrating data in mixed methods studies: triangulation protocol, following a thread, and the mixed methods matrix.
Triangulation protocol
This integration method combines different methods with differing or conflicting results to generate one unified answer.
For example, if a researcher wanted to know what type of music teenagers enjoy listening to, they might employ a survey of 1,000 teenagers as well as five focus group interviews to investigate this. The results might differ; the survey may find that rap is the most popular genre, whereas the focus groups may suggest rock music is more widely listened to.
The researcher can then use the triangulation protocol to come up with a unified answer—such as that both rap and rock music are popular genres for teenage listeners.
Following a thread
This is another method of integration where the researcher follows the same theme or idea from one method of data collection to the next.
A research design that follows a thread starts by collecting quantitative data on a specific issue, followed by collecting qualitative data to explain the results. This allows whoever is conducting the research to detect any conflicting information and further look into the conflicting information to understand what is really going on.
For example, a researcher who used this research method might collect quantitative data about how satisfied employees are with their jobs at a certain company, followed by qualitative interviews to investigate why job satisfaction levels are low. They could then use the results to explore any conflicting or differing results, allowing them to gain a deeper understanding of job satisfaction at the company.
By following a thread, the researcher can explore various research topics related to the original issue and gain a more comprehensive view of the issue.
Mixed methods matrix
This technique is a visual representation of the different types of mixed methods research designs and the order in which they should be implemented. It enables researchers to quickly assess their research design and adjust it as needed.
The matrix consists of four boxes with four different types of mixed methods research designs: convergent parallel, explanatory sequential, exploratory sequential, and method flexibility.
For example, imagine a researcher who wanted to understand why people don't exercise regularly. To answer this question, they could use a convergent parallel design, collecting both quantitative (e.g., survey responses) and qualitative (e.g., interviews) data simultaneously.
If the researcher found conflicting results, they could switch to an explanatory sequential design and collect quantitative data first, then follow up with qualitative data if needed. This way, the researcher can make adjustments based on their findings and integrate their data more effectively.
Mixed methods research is a powerful tool for understanding complex research topics. Using qualitative and quantitative data in one study allows researchers to understand their subject more deeply.
Mixed methods research designs such as convergent parallel, explanatory sequential, and exploratory sequential provide method flexibility, enabling researchers to collect both types of data while avoiding the limitations of either approach alone.
However, it's important to remember that mixed methods research can produce differing or even conflicting results, so it's important to be aware of the potential pitfalls and take steps to ensure that data is being correctly integrated. If used effectively, mixed methods research can offer valuable insight into topics that would otherwise remain largely unexplored.
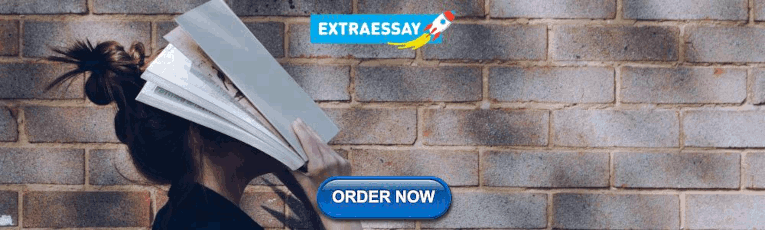
What is an example of mixed methods research?
An example of mixed methods research is a study that combines quantitative and qualitative data. This type of research uses surveys, interviews, and observations to collect data from multiple sources.
Which sampling method is best for mixed methods?
It depends on the research objectives, but a few methods are often used in mixed methods research designs. These include snowball sampling, convenience sampling, and purposive sampling. Each method has its own advantages and disadvantages.
What is the difference between mixed methods and multiple methods?
Mixed methods research combines quantitative and qualitative data in a single study. Multiple methods involve collecting data from different sources, such as surveys and interviews, but not necessarily combining them into one analysis. Mixed methods offer greater flexibility but can lead to differing or conflicting results when integrating data.
Get started today
Go from raw data to valuable insights with a flexible research platform
Editor’s picks
Last updated: 21 December 2023
Last updated: 16 December 2023
Last updated: 6 October 2023
Last updated: 5 March 2024
Last updated: 25 November 2023
Last updated: 15 February 2024
Last updated: 11 March 2024
Last updated: 12 December 2023
Last updated: 6 March 2024
Last updated: 10 April 2023
Last updated: 20 December 2023
Latest articles
Related topics, log in or sign up.
Get started for free
Log in using your username and password
- Search More Search for this keyword Advanced search
- Latest content
- Current issue
- Write for Us
- BMJ Journals More You are viewing from: Google Indexer
You are here
- Volume 20, Issue 3
- Mixed methods research: expanding the evidence base
- Article Text
- Article info
- Citation Tools
- Rapid Responses
- Article metrics

- Allison Shorten 1 ,
- Joanna Smith 2
- 1 School of Nursing , University of Alabama at Birmingham , USA
- 2 Children's Nursing, School of Healthcare , University of Leeds , UK
- Correspondence to Dr Allison Shorten, School of Nursing, University of Alabama at Birmingham, 1720 2nd Ave South, Birmingham, AL, 35294, USA; [email protected]; ashorten{at}uab.edu
https://doi.org/10.1136/eb-2017-102699
Statistics from Altmetric.com
Request permissions.
If you wish to reuse any or all of this article please use the link below which will take you to the Copyright Clearance Center’s RightsLink service. You will be able to get a quick price and instant permission to reuse the content in many different ways.
Introduction
‘Mixed methods’ is a research approach whereby researchers collect and analyse both quantitative and qualitative data within the same study. 1 2 Growth of mixed methods research in nursing and healthcare has occurred at a time of internationally increasing complexity in healthcare delivery. Mixed methods research draws on potential strengths of both qualitative and quantitative methods, 3 allowing researchers to explore diverse perspectives and uncover relationships that exist between the intricate layers of our multifaceted research questions. As providers and policy makers strive to ensure quality and safety for patients and families, researchers can use mixed methods to explore contemporary healthcare trends and practices across increasingly diverse practice settings.
What is mixed methods research?
Mixed methods research requires a purposeful mixing of methods in data collection, data analysis and interpretation of the evidence. The key word is ‘mixed’, as an essential step in the mixed methods approach is data linkage, or integration at an appropriate stage in the research process. 4 Purposeful data integration enables researchers to seek a more panoramic view of their research landscape, viewing phenomena from different viewpoints and through diverse research lenses. For example, in a randomised controlled trial (RCT) evaluating a decision aid for women making choices about birth after caesarean, quantitative data were collected to assess knowledge change, levels of decisional conflict, birth choices and outcomes. 5 Qualitative narrative data were collected to gain insight into women’s decision-making experiences and factors that influenced their choices for mode of birth. 5
In contrast, multimethod research uses a single research paradigm, either quantitative or qualitative. Data are collected and analysed using different methods within the same paradigm. 6 7 For example, in a multimethods qualitative study investigating parent–professional shared decision-making regarding diagnosis of suspected shunt malfunction in children, data collection included audio recordings of admission consultations and interviews 1 week post consultation, with interactions analysed using conversational analysis and the framework approach for the interview data. 8
What are the strengths and challenges in using mixed methods?
Selecting the right research method starts with identifying the research question and study aims. A mixed methods design is appropriate for answering research questions that neither quantitative nor qualitative methods could answer alone. 4 9–11 Mixed methods can be used to gain a better understanding of connections or contradictions between qualitative and quantitative data; they can provide opportunities for participants to have a strong voice and share their experiences across the research process, and they can facilitate different avenues of exploration that enrich the evidence and enable questions to be answered more deeply. 11 Mixed methods can facilitate greater scholarly interaction and enrich the experiences of researchers as different perspectives illuminate the issues being studied. 11
The process of mixing methods within one study, however, can add to the complexity of conducting research. It often requires more resources (time and personnel) and additional research training, as multidisciplinary research teams need to become conversant with alternative research paradigms and different approaches to sample selection, data collection, data analysis and data synthesis or integration. 11
What are the different types of mixed methods designs?
Mixed methods research comprises different types of design categories, including explanatory, exploratory, parallel and nested (embedded) designs. 2 Table 1 summarises the characteristics of each design, the process used and models of connecting or integrating data. For each type of research, an example was created to illustrate how each study design might be applied to address similar but different nursing research aims within the same general nursing research area.
- View inline
Types of mixed methods designs*
What should be considered when evaluating mixed methods research?
When reading mixed methods research or writing a proposal using mixed methods to answer a research question, the six questions below are a useful guide 12 :
Does the research question justify the use of mixed methods?
Is the method sequence clearly described, logical in flow and well aligned with study aims?
Is data collection and analysis clearly described and well aligned with study aims?
Does one method dominate the other or are they equally important?
Did the use of one method limit or confound the other method?
When, how and by whom is data integration (mixing) achieved?
For more detail of the evaluation guide, refer to the McMaster University Mixed Methods Appraisal Tool. 12 The quality checklist for appraising published mixed methods research could also be used as a design checklist when planning mixed methods studies.
- Elliot AE , et al
- Creswell JW ,
- Plano ClarkV L
- Greene JC ,
- Caracelli VJ ,
- Ivankova NV
- Shorten A ,
- Shorten B ,
- Halcomb E ,
- Cheater F ,
- Bekker H , et al
- Tashakkori A ,
- Creswell JW
- 12. ↵ National Collaborating Centre for Methods and Tools . Appraising qualitative, quantitative, and mixed methods studies included in mixed studies reviews: the MMAT . Hamilton, ON : BMJ Publishing Group , 2015 . http://www.nccmt.ca/resources/search/232 (accessed May 2017) .
Competing interests None declared.
Provenance and peer review Commissioned; internally peer reviewed.
Read the full text or download the PDF:
- News & Highlights

- Publications and Documents
- Postgraduate Education
- Browse Our Courses
- C/T Research Academy
- K12 Investigator Training
- Translational Innovator
- SMART IRB Reliance Request
- Biostatistics Consulting
- Regulatory Support
- Pilot Funding
- Informatics Program
- Community Engagement
- Diversity Inclusion
- Research Enrollment and Diversity
- Harvard Catalyst Profiles
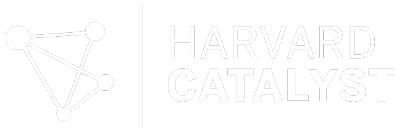
Community Engagement Program
Supporting bi-directional community engagement to improve the relevance, quality, and impact of research.
- Getting Started
- Resources for Equity in Research
- Community-Engaged Student Practice Placement
- Maternal Health Equity
- Youth Mental Health
- Leadership and Membership
- Past Members
- Study Review Rubric
- Community Ambassador Initiative
- Implementation Science Working Group
- Past Webinars & Podcasts
- Policy Atlas
- Community Advisory Board
For more information:
Mixed methods research.
According to the National Institutes of Health , mixed methods strategically integrates or combines rigorous quantitative and qualitative research methods to draw on the strengths of each. Mixed method approaches allow researchers to use a diversity of methods, combining inductive and deductive thinking, and offsetting limitations of exclusively quantitative and qualitative research through a complementary approach that maximizes strengths of each data type and facilitates a more comprehensive understanding of health issues and potential resolutions.¹ Mixed methods may be employed to produce a robust description and interpretation of the data, make quantitative results more understandable, or understand broader applicability of small-sample qualitative findings.
Integration
This refers to the ways in which qualitative and quantitative research activities are brought together to achieve greater insight. Mixed methods is not simply having quantitative and qualitative data available or analyzing and presenting data findings separately. The integration process can occur during data collection, analysis, or in the presentation of results.
¹ NIH Office of Behavioral and Social Sciences Research: Best Practices for Mixed Methods Research in the Health Sciences
Basic Mixed Methods Research Designs

View image description .
Five Key Questions for Getting Started
- What do you want to know?
- What will be the detailed quantitative, qualitative, and mixed methods research questions that you hope to address?
- What quantitative and qualitative data will you collect and analyze?
- Which rigorous methods will you use to collect data and/or engage stakeholders?
- How will you integrate the data in a way that allows you to address the first question?
Rationale for Using Mixed Methods
- Obtain different, multiple perspectives: validation
- Build comprehensive understanding
- Explain statistical results in more depth
- Have better contextualized measures
- Track the process of program or intervention
- Study patient-centered outcomes and stakeholder engagement
Sample Mixed Methods Research Study
The EQUALITY study used an exploratory sequential design to identify the optimal patient-centered approach to collect sexual orientation data in the emergency department.
Qualitative Data Collection and Analysis : Semi-structured interviews with patients of different sexual orientation, age, race/ethnicity, as well as healthcare professionals of different roles, age, and race/ethnicity.
Builds Into : Themes identified in the interviews were used to develop questions for the national survey.
Quantitative Data Collection and Analysis : Representative national survey of patients and healthcare professionals on the topic of reporting gender identity and sexual orientation in healthcare.
Other Resources:
Introduction to Mixed Methods Research : Harvard Catalyst’s eight-week online course offers an opportunity for investigators who want to understand and apply a mixed methods approach to their research.
Best Practices for Mixed Methods Research in the Health Sciences [PDF] : This guide provides a detailed overview of mixed methods designs, best practices, and application to various types of grants and projects.
Mixed Methods Research Training Program for the Health Sciences (MMRTP ): Selected scholars for this summer training program, hosted by Johns Hopkins’ Bloomberg School of Public Health, have access to webinars, resources, a retreat to discuss their research project with expert faculty, and are matched with mixed methods consultants for ongoing support.
Michigan Mixed Methods : University of Michigan Mixed Methods program offers a variety of resources, including short web videos and recommended reading.
To use a mixed methods approach, you may want to first brush up on your qualitative skills. Below are a few helpful resources specific to qualitative research:
- Qualitative Research Guidelines Project : A comprehensive guide for designing, writing, reviewing and reporting qualitative research.
- Fundamentals of Qualitative Research Methods – What is Qualitative Research : A six-module web video series covering essential topics in qualitative research, including what is qualitative research and how to use the most common methods, in-depth interviews, and focus groups.
View PDF of the above information.
Frequently asked questions
What is mixed methods research.
In mixed methods research , you use both qualitative and quantitative data collection and analysis methods to answer your research question .
Frequently asked questions: Methodology
Attrition refers to participants leaving a study. It always happens to some extent—for example, in randomized controlled trials for medical research.
Differential attrition occurs when attrition or dropout rates differ systematically between the intervention and the control group . As a result, the characteristics of the participants who drop out differ from the characteristics of those who stay in the study. Because of this, study results may be biased .
Action research is conducted in order to solve a particular issue immediately, while case studies are often conducted over a longer period of time and focus more on observing and analyzing a particular ongoing phenomenon.
Action research is focused on solving a problem or informing individual and community-based knowledge in a way that impacts teaching, learning, and other related processes. It is less focused on contributing theoretical input, instead producing actionable input.
Action research is particularly popular with educators as a form of systematic inquiry because it prioritizes reflection and bridges the gap between theory and practice. Educators are able to simultaneously investigate an issue as they solve it, and the method is very iterative and flexible.
A cycle of inquiry is another name for action research . It is usually visualized in a spiral shape following a series of steps, such as “planning → acting → observing → reflecting.”
To make quantitative observations , you need to use instruments that are capable of measuring the quantity you want to observe. For example, you might use a ruler to measure the length of an object or a thermometer to measure its temperature.
Criterion validity and construct validity are both types of measurement validity . In other words, they both show you how accurately a method measures something.
While construct validity is the degree to which a test or other measurement method measures what it claims to measure, criterion validity is the degree to which a test can predictively (in the future) or concurrently (in the present) measure something.
Construct validity is often considered the overarching type of measurement validity . You need to have face validity , content validity , and criterion validity in order to achieve construct validity.
Convergent validity and discriminant validity are both subtypes of construct validity . Together, they help you evaluate whether a test measures the concept it was designed to measure.
- Convergent validity indicates whether a test that is designed to measure a particular construct correlates with other tests that assess the same or similar construct.
- Discriminant validity indicates whether two tests that should not be highly related to each other are indeed not related. This type of validity is also called divergent validity .
You need to assess both in order to demonstrate construct validity. Neither one alone is sufficient for establishing construct validity.
- Discriminant validity indicates whether two tests that should not be highly related to each other are indeed not related
Content validity shows you how accurately a test or other measurement method taps into the various aspects of the specific construct you are researching.
In other words, it helps you answer the question: “does the test measure all aspects of the construct I want to measure?” If it does, then the test has high content validity.
The higher the content validity, the more accurate the measurement of the construct.
If the test fails to include parts of the construct, or irrelevant parts are included, the validity of the instrument is threatened, which brings your results into question.
Face validity and content validity are similar in that they both evaluate how suitable the content of a test is. The difference is that face validity is subjective, and assesses content at surface level.
When a test has strong face validity, anyone would agree that the test’s questions appear to measure what they are intended to measure.
For example, looking at a 4th grade math test consisting of problems in which students have to add and multiply, most people would agree that it has strong face validity (i.e., it looks like a math test).
On the other hand, content validity evaluates how well a test represents all the aspects of a topic. Assessing content validity is more systematic and relies on expert evaluation. of each question, analyzing whether each one covers the aspects that the test was designed to cover.
A 4th grade math test would have high content validity if it covered all the skills taught in that grade. Experts(in this case, math teachers), would have to evaluate the content validity by comparing the test to the learning objectives.
Snowball sampling is a non-probability sampling method . Unlike probability sampling (which involves some form of random selection ), the initial individuals selected to be studied are the ones who recruit new participants.
Because not every member of the target population has an equal chance of being recruited into the sample, selection in snowball sampling is non-random.
Snowball sampling is a non-probability sampling method , where there is not an equal chance for every member of the population to be included in the sample .
This means that you cannot use inferential statistics and make generalizations —often the goal of quantitative research . As such, a snowball sample is not representative of the target population and is usually a better fit for qualitative research .
Snowball sampling relies on the use of referrals. Here, the researcher recruits one or more initial participants, who then recruit the next ones.
Participants share similar characteristics and/or know each other. Because of this, not every member of the population has an equal chance of being included in the sample, giving rise to sampling bias .
Snowball sampling is best used in the following cases:
- If there is no sampling frame available (e.g., people with a rare disease)
- If the population of interest is hard to access or locate (e.g., people experiencing homelessness)
- If the research focuses on a sensitive topic (e.g., extramarital affairs)
The reproducibility and replicability of a study can be ensured by writing a transparent, detailed method section and using clear, unambiguous language.
Reproducibility and replicability are related terms.
- Reproducing research entails reanalyzing the existing data in the same manner.
- Replicating (or repeating ) the research entails reconducting the entire analysis, including the collection of new data .
- A successful reproduction shows that the data analyses were conducted in a fair and honest manner.
- A successful replication shows that the reliability of the results is high.
Stratified sampling and quota sampling both involve dividing the population into subgroups and selecting units from each subgroup. The purpose in both cases is to select a representative sample and/or to allow comparisons between subgroups.
The main difference is that in stratified sampling, you draw a random sample from each subgroup ( probability sampling ). In quota sampling you select a predetermined number or proportion of units, in a non-random manner ( non-probability sampling ).
Purposive and convenience sampling are both sampling methods that are typically used in qualitative data collection.
A convenience sample is drawn from a source that is conveniently accessible to the researcher. Convenience sampling does not distinguish characteristics among the participants. On the other hand, purposive sampling focuses on selecting participants possessing characteristics associated with the research study.
The findings of studies based on either convenience or purposive sampling can only be generalized to the (sub)population from which the sample is drawn, and not to the entire population.
Random sampling or probability sampling is based on random selection. This means that each unit has an equal chance (i.e., equal probability) of being included in the sample.
On the other hand, convenience sampling involves stopping people at random, which means that not everyone has an equal chance of being selected depending on the place, time, or day you are collecting your data.
Convenience sampling and quota sampling are both non-probability sampling methods. They both use non-random criteria like availability, geographical proximity, or expert knowledge to recruit study participants.
However, in convenience sampling, you continue to sample units or cases until you reach the required sample size.
In quota sampling, you first need to divide your population of interest into subgroups (strata) and estimate their proportions (quota) in the population. Then you can start your data collection, using convenience sampling to recruit participants, until the proportions in each subgroup coincide with the estimated proportions in the population.
A sampling frame is a list of every member in the entire population . It is important that the sampling frame is as complete as possible, so that your sample accurately reflects your population.
Stratified and cluster sampling may look similar, but bear in mind that groups created in cluster sampling are heterogeneous , so the individual characteristics in the cluster vary. In contrast, groups created in stratified sampling are homogeneous , as units share characteristics.
Relatedly, in cluster sampling you randomly select entire groups and include all units of each group in your sample. However, in stratified sampling, you select some units of all groups and include them in your sample. In this way, both methods can ensure that your sample is representative of the target population .
A systematic review is secondary research because it uses existing research. You don’t collect new data yourself.
The key difference between observational studies and experimental designs is that a well-done observational study does not influence the responses of participants, while experiments do have some sort of treatment condition applied to at least some participants by random assignment .
An observational study is a great choice for you if your research question is based purely on observations. If there are ethical, logistical, or practical concerns that prevent you from conducting a traditional experiment , an observational study may be a good choice. In an observational study, there is no interference or manipulation of the research subjects, as well as no control or treatment groups .
It’s often best to ask a variety of people to review your measurements. You can ask experts, such as other researchers, or laypeople, such as potential participants, to judge the face validity of tests.
While experts have a deep understanding of research methods , the people you’re studying can provide you with valuable insights you may have missed otherwise.
Face validity is important because it’s a simple first step to measuring the overall validity of a test or technique. It’s a relatively intuitive, quick, and easy way to start checking whether a new measure seems useful at first glance.
Good face validity means that anyone who reviews your measure says that it seems to be measuring what it’s supposed to. With poor face validity, someone reviewing your measure may be left confused about what you’re measuring and why you’re using this method.
Face validity is about whether a test appears to measure what it’s supposed to measure. This type of validity is concerned with whether a measure seems relevant and appropriate for what it’s assessing only on the surface.
Statistical analyses are often applied to test validity with data from your measures. You test convergent validity and discriminant validity with correlations to see if results from your test are positively or negatively related to those of other established tests.
You can also use regression analyses to assess whether your measure is actually predictive of outcomes that you expect it to predict theoretically. A regression analysis that supports your expectations strengthens your claim of construct validity .
When designing or evaluating a measure, construct validity helps you ensure you’re actually measuring the construct you’re interested in. If you don’t have construct validity, you may inadvertently measure unrelated or distinct constructs and lose precision in your research.
Construct validity is often considered the overarching type of measurement validity , because it covers all of the other types. You need to have face validity , content validity , and criterion validity to achieve construct validity.
Construct validity is about how well a test measures the concept it was designed to evaluate. It’s one of four types of measurement validity , which includes construct validity, face validity , and criterion validity.
There are two subtypes of construct validity.
- Convergent validity : The extent to which your measure corresponds to measures of related constructs
- Discriminant validity : The extent to which your measure is unrelated or negatively related to measures of distinct constructs
Naturalistic observation is a valuable tool because of its flexibility, external validity , and suitability for topics that can’t be studied in a lab setting.
The downsides of naturalistic observation include its lack of scientific control , ethical considerations , and potential for bias from observers and subjects.
Naturalistic observation is a qualitative research method where you record the behaviors of your research subjects in real world settings. You avoid interfering or influencing anything in a naturalistic observation.
You can think of naturalistic observation as “people watching” with a purpose.
A dependent variable is what changes as a result of the independent variable manipulation in experiments . It’s what you’re interested in measuring, and it “depends” on your independent variable.
In statistics, dependent variables are also called:
- Response variables (they respond to a change in another variable)
- Outcome variables (they represent the outcome you want to measure)
- Left-hand-side variables (they appear on the left-hand side of a regression equation)
An independent variable is the variable you manipulate, control, or vary in an experimental study to explore its effects. It’s called “independent” because it’s not influenced by any other variables in the study.
Independent variables are also called:
- Explanatory variables (they explain an event or outcome)
- Predictor variables (they can be used to predict the value of a dependent variable)
- Right-hand-side variables (they appear on the right-hand side of a regression equation).
As a rule of thumb, questions related to thoughts, beliefs, and feelings work well in focus groups. Take your time formulating strong questions, paying special attention to phrasing. Be careful to avoid leading questions , which can bias your responses.
Overall, your focus group questions should be:
- Open-ended and flexible
- Impossible to answer with “yes” or “no” (questions that start with “why” or “how” are often best)
- Unambiguous, getting straight to the point while still stimulating discussion
- Unbiased and neutral
A structured interview is a data collection method that relies on asking questions in a set order to collect data on a topic. They are often quantitative in nature. Structured interviews are best used when:
- You already have a very clear understanding of your topic. Perhaps significant research has already been conducted, or you have done some prior research yourself, but you already possess a baseline for designing strong structured questions.
- You are constrained in terms of time or resources and need to analyze your data quickly and efficiently.
- Your research question depends on strong parity between participants, with environmental conditions held constant.
More flexible interview options include semi-structured interviews , unstructured interviews , and focus groups .
Social desirability bias is the tendency for interview participants to give responses that will be viewed favorably by the interviewer or other participants. It occurs in all types of interviews and surveys , but is most common in semi-structured interviews , unstructured interviews , and focus groups .
Social desirability bias can be mitigated by ensuring participants feel at ease and comfortable sharing their views. Make sure to pay attention to your own body language and any physical or verbal cues, such as nodding or widening your eyes.
This type of bias can also occur in observations if the participants know they’re being observed. They might alter their behavior accordingly.
The interviewer effect is a type of bias that emerges when a characteristic of an interviewer (race, age, gender identity, etc.) influences the responses given by the interviewee.
There is a risk of an interviewer effect in all types of interviews , but it can be mitigated by writing really high-quality interview questions.
A semi-structured interview is a blend of structured and unstructured types of interviews. Semi-structured interviews are best used when:
- You have prior interview experience. Spontaneous questions are deceptively challenging, and it’s easy to accidentally ask a leading question or make a participant uncomfortable.
- Your research question is exploratory in nature. Participant answers can guide future research questions and help you develop a more robust knowledge base for future research.
An unstructured interview is the most flexible type of interview, but it is not always the best fit for your research topic.
Unstructured interviews are best used when:
- You are an experienced interviewer and have a very strong background in your research topic, since it is challenging to ask spontaneous, colloquial questions.
- Your research question is exploratory in nature. While you may have developed hypotheses, you are open to discovering new or shifting viewpoints through the interview process.
- You are seeking descriptive data, and are ready to ask questions that will deepen and contextualize your initial thoughts and hypotheses.
- Your research depends on forming connections with your participants and making them feel comfortable revealing deeper emotions, lived experiences, or thoughts.
The four most common types of interviews are:
- Structured interviews : The questions are predetermined in both topic and order.
- Semi-structured interviews : A few questions are predetermined, but other questions aren’t planned.
- Unstructured interviews : None of the questions are predetermined.
- Focus group interviews : The questions are presented to a group instead of one individual.
Deductive reasoning is commonly used in scientific research, and it’s especially associated with quantitative research .
In research, you might have come across something called the hypothetico-deductive method . It’s the scientific method of testing hypotheses to check whether your predictions are substantiated by real-world data.
Deductive reasoning is a logical approach where you progress from general ideas to specific conclusions. It’s often contrasted with inductive reasoning , where you start with specific observations and form general conclusions.
Deductive reasoning is also called deductive logic.
There are many different types of inductive reasoning that people use formally or informally.
Here are a few common types:
- Inductive generalization : You use observations about a sample to come to a conclusion about the population it came from.
- Statistical generalization: You use specific numbers about samples to make statements about populations.
- Causal reasoning: You make cause-and-effect links between different things.
- Sign reasoning: You make a conclusion about a correlational relationship between different things.
- Analogical reasoning: You make a conclusion about something based on its similarities to something else.
Inductive reasoning is a bottom-up approach, while deductive reasoning is top-down.
Inductive reasoning takes you from the specific to the general, while in deductive reasoning, you make inferences by going from general premises to specific conclusions.
In inductive research , you start by making observations or gathering data. Then, you take a broad scan of your data and search for patterns. Finally, you make general conclusions that you might incorporate into theories.
Inductive reasoning is a method of drawing conclusions by going from the specific to the general. It’s usually contrasted with deductive reasoning, where you proceed from general information to specific conclusions.
Inductive reasoning is also called inductive logic or bottom-up reasoning.
A hypothesis states your predictions about what your research will find. It is a tentative answer to your research question that has not yet been tested. For some research projects, you might have to write several hypotheses that address different aspects of your research question.
A hypothesis is not just a guess — it should be based on existing theories and knowledge. It also has to be testable, which means you can support or refute it through scientific research methods (such as experiments, observations and statistical analysis of data).
Triangulation can help:
- Reduce research bias that comes from using a single method, theory, or investigator
- Enhance validity by approaching the same topic with different tools
- Establish credibility by giving you a complete picture of the research problem
But triangulation can also pose problems:
- It’s time-consuming and labor-intensive, often involving an interdisciplinary team.
- Your results may be inconsistent or even contradictory.
There are four main types of triangulation :
- Data triangulation : Using data from different times, spaces, and people
- Investigator triangulation : Involving multiple researchers in collecting or analyzing data
- Theory triangulation : Using varying theoretical perspectives in your research
- Methodological triangulation : Using different methodologies to approach the same topic
Many academic fields use peer review , largely to determine whether a manuscript is suitable for publication. Peer review enhances the credibility of the published manuscript.
However, peer review is also common in non-academic settings. The United Nations, the European Union, and many individual nations use peer review to evaluate grant applications. It is also widely used in medical and health-related fields as a teaching or quality-of-care measure.
Peer assessment is often used in the classroom as a pedagogical tool. Both receiving feedback and providing it are thought to enhance the learning process, helping students think critically and collaboratively.
Peer review can stop obviously problematic, falsified, or otherwise untrustworthy research from being published. It also represents an excellent opportunity to get feedback from renowned experts in your field. It acts as a first defense, helping you ensure your argument is clear and that there are no gaps, vague terms, or unanswered questions for readers who weren’t involved in the research process.
Peer-reviewed articles are considered a highly credible source due to this stringent process they go through before publication.
In general, the peer review process follows the following steps:
- First, the author submits the manuscript to the editor.
- Reject the manuscript and send it back to author, or
- Send it onward to the selected peer reviewer(s)
- Next, the peer review process occurs. The reviewer provides feedback, addressing any major or minor issues with the manuscript, and gives their advice regarding what edits should be made.
- Lastly, the edited manuscript is sent back to the author. They input the edits, and resubmit it to the editor for publication.
Exploratory research is often used when the issue you’re studying is new or when the data collection process is challenging for some reason.
You can use exploratory research if you have a general idea or a specific question that you want to study but there is no preexisting knowledge or paradigm with which to study it.
Exploratory research is a methodology approach that explores research questions that have not previously been studied in depth. It is often used when the issue you’re studying is new, or the data collection process is challenging in some way.
Explanatory research is used to investigate how or why a phenomenon occurs. Therefore, this type of research is often one of the first stages in the research process , serving as a jumping-off point for future research.
Exploratory research aims to explore the main aspects of an under-researched problem, while explanatory research aims to explain the causes and consequences of a well-defined problem.
Explanatory research is a research method used to investigate how or why something occurs when only a small amount of information is available pertaining to that topic. It can help you increase your understanding of a given topic.
Clean data are valid, accurate, complete, consistent, unique, and uniform. Dirty data include inconsistencies and errors.
Dirty data can come from any part of the research process, including poor research design , inappropriate measurement materials, or flawed data entry.
Data cleaning takes place between data collection and data analyses. But you can use some methods even before collecting data.
For clean data, you should start by designing measures that collect valid data. Data validation at the time of data entry or collection helps you minimize the amount of data cleaning you’ll need to do.
After data collection, you can use data standardization and data transformation to clean your data. You’ll also deal with any missing values, outliers, and duplicate values.
Every dataset requires different techniques to clean dirty data , but you need to address these issues in a systematic way. You focus on finding and resolving data points that don’t agree or fit with the rest of your dataset.
These data might be missing values, outliers, duplicate values, incorrectly formatted, or irrelevant. You’ll start with screening and diagnosing your data. Then, you’ll often standardize and accept or remove data to make your dataset consistent and valid.
Data cleaning is necessary for valid and appropriate analyses. Dirty data contain inconsistencies or errors , but cleaning your data helps you minimize or resolve these.
Without data cleaning, you could end up with a Type I or II error in your conclusion. These types of erroneous conclusions can be practically significant with important consequences, because they lead to misplaced investments or missed opportunities.
Data cleaning involves spotting and resolving potential data inconsistencies or errors to improve your data quality. An error is any value (e.g., recorded weight) that doesn’t reflect the true value (e.g., actual weight) of something that’s being measured.
In this process, you review, analyze, detect, modify, or remove “dirty” data to make your dataset “clean.” Data cleaning is also called data cleansing or data scrubbing.
Research misconduct means making up or falsifying data, manipulating data analyses, or misrepresenting results in research reports. It’s a form of academic fraud.
These actions are committed intentionally and can have serious consequences; research misconduct is not a simple mistake or a point of disagreement but a serious ethical failure.
Anonymity means you don’t know who the participants are, while confidentiality means you know who they are but remove identifying information from your research report. Both are important ethical considerations .
You can only guarantee anonymity by not collecting any personally identifying information—for example, names, phone numbers, email addresses, IP addresses, physical characteristics, photos, or videos.
You can keep data confidential by using aggregate information in your research report, so that you only refer to groups of participants rather than individuals.
Research ethics matter for scientific integrity, human rights and dignity, and collaboration between science and society. These principles make sure that participation in studies is voluntary, informed, and safe.
Ethical considerations in research are a set of principles that guide your research designs and practices. These principles include voluntary participation, informed consent, anonymity, confidentiality, potential for harm, and results communication.
Scientists and researchers must always adhere to a certain code of conduct when collecting data from others .
These considerations protect the rights of research participants, enhance research validity , and maintain scientific integrity.
In multistage sampling , you can use probability or non-probability sampling methods .
For a probability sample, you have to conduct probability sampling at every stage.
You can mix it up by using simple random sampling , systematic sampling , or stratified sampling to select units at different stages, depending on what is applicable and relevant to your study.
Multistage sampling can simplify data collection when you have large, geographically spread samples, and you can obtain a probability sample without a complete sampling frame.
But multistage sampling may not lead to a representative sample, and larger samples are needed for multistage samples to achieve the statistical properties of simple random samples .
These are four of the most common mixed methods designs :
- Convergent parallel: Quantitative and qualitative data are collected at the same time and analyzed separately. After both analyses are complete, compare your results to draw overall conclusions.
- Embedded: Quantitative and qualitative data are collected at the same time, but within a larger quantitative or qualitative design. One type of data is secondary to the other.
- Explanatory sequential: Quantitative data is collected and analyzed first, followed by qualitative data. You can use this design if you think your qualitative data will explain and contextualize your quantitative findings.
- Exploratory sequential: Qualitative data is collected and analyzed first, followed by quantitative data. You can use this design if you think the quantitative data will confirm or validate your qualitative findings.
Triangulation in research means using multiple datasets, methods, theories and/or investigators to address a research question. It’s a research strategy that can help you enhance the validity and credibility of your findings.
Triangulation is mainly used in qualitative research , but it’s also commonly applied in quantitative research . Mixed methods research always uses triangulation.
In multistage sampling , or multistage cluster sampling, you draw a sample from a population using smaller and smaller groups at each stage.
This method is often used to collect data from a large, geographically spread group of people in national surveys, for example. You take advantage of hierarchical groupings (e.g., from state to city to neighborhood) to create a sample that’s less expensive and time-consuming to collect data from.
No, the steepness or slope of the line isn’t related to the correlation coefficient value. The correlation coefficient only tells you how closely your data fit on a line, so two datasets with the same correlation coefficient can have very different slopes.
To find the slope of the line, you’ll need to perform a regression analysis .
Correlation coefficients always range between -1 and 1.
The sign of the coefficient tells you the direction of the relationship: a positive value means the variables change together in the same direction, while a negative value means they change together in opposite directions.
The absolute value of a number is equal to the number without its sign. The absolute value of a correlation coefficient tells you the magnitude of the correlation: the greater the absolute value, the stronger the correlation.
These are the assumptions your data must meet if you want to use Pearson’s r :
- Both variables are on an interval or ratio level of measurement
- Data from both variables follow normal distributions
- Your data have no outliers
- Your data is from a random or representative sample
- You expect a linear relationship between the two variables
Quantitative research designs can be divided into two main categories:
- Correlational and descriptive designs are used to investigate characteristics, averages, trends, and associations between variables.
- Experimental and quasi-experimental designs are used to test causal relationships .
Qualitative research designs tend to be more flexible. Common types of qualitative design include case study , ethnography , and grounded theory designs.
A well-planned research design helps ensure that your methods match your research aims, that you collect high-quality data, and that you use the right kind of analysis to answer your questions, utilizing credible sources . This allows you to draw valid , trustworthy conclusions.
The priorities of a research design can vary depending on the field, but you usually have to specify:
- Your research questions and/or hypotheses
- Your overall approach (e.g., qualitative or quantitative )
- The type of design you’re using (e.g., a survey , experiment , or case study )
- Your sampling methods or criteria for selecting subjects
- Your data collection methods (e.g., questionnaires , observations)
- Your data collection procedures (e.g., operationalization , timing and data management)
- Your data analysis methods (e.g., statistical tests or thematic analysis )
A research design is a strategy for answering your research question . It defines your overall approach and determines how you will collect and analyze data.
Questionnaires can be self-administered or researcher-administered.
Self-administered questionnaires can be delivered online or in paper-and-pen formats, in person or through mail. All questions are standardized so that all respondents receive the same questions with identical wording.
Researcher-administered questionnaires are interviews that take place by phone, in-person, or online between researchers and respondents. You can gain deeper insights by clarifying questions for respondents or asking follow-up questions.
You can organize the questions logically, with a clear progression from simple to complex, or randomly between respondents. A logical flow helps respondents process the questionnaire easier and quicker, but it may lead to bias. Randomization can minimize the bias from order effects.
Closed-ended, or restricted-choice, questions offer respondents a fixed set of choices to select from. These questions are easier to answer quickly.
Open-ended or long-form questions allow respondents to answer in their own words. Because there are no restrictions on their choices, respondents can answer in ways that researchers may not have otherwise considered.
A questionnaire is a data collection tool or instrument, while a survey is an overarching research method that involves collecting and analyzing data from people using questionnaires.
The third variable and directionality problems are two main reasons why correlation isn’t causation .
The third variable problem means that a confounding variable affects both variables to make them seem causally related when they are not.
The directionality problem is when two variables correlate and might actually have a causal relationship, but it’s impossible to conclude which variable causes changes in the other.
Correlation describes an association between variables : when one variable changes, so does the other. A correlation is a statistical indicator of the relationship between variables.
Causation means that changes in one variable brings about changes in the other (i.e., there is a cause-and-effect relationship between variables). The two variables are correlated with each other, and there’s also a causal link between them.
While causation and correlation can exist simultaneously, correlation does not imply causation. In other words, correlation is simply a relationship where A relates to B—but A doesn’t necessarily cause B to happen (or vice versa). Mistaking correlation for causation is a common error and can lead to false cause fallacy .
Controlled experiments establish causality, whereas correlational studies only show associations between variables.
- In an experimental design , you manipulate an independent variable and measure its effect on a dependent variable. Other variables are controlled so they can’t impact the results.
- In a correlational design , you measure variables without manipulating any of them. You can test whether your variables change together, but you can’t be sure that one variable caused a change in another.
In general, correlational research is high in external validity while experimental research is high in internal validity .
A correlation is usually tested for two variables at a time, but you can test correlations between three or more variables.
A correlation coefficient is a single number that describes the strength and direction of the relationship between your variables.
Different types of correlation coefficients might be appropriate for your data based on their levels of measurement and distributions . The Pearson product-moment correlation coefficient (Pearson’s r ) is commonly used to assess a linear relationship between two quantitative variables.
A correlational research design investigates relationships between two variables (or more) without the researcher controlling or manipulating any of them. It’s a non-experimental type of quantitative research .
A correlation reflects the strength and/or direction of the association between two or more variables.
- A positive correlation means that both variables change in the same direction.
- A negative correlation means that the variables change in opposite directions.
- A zero correlation means there’s no relationship between the variables.
Random error is almost always present in scientific studies, even in highly controlled settings. While you can’t eradicate it completely, you can reduce random error by taking repeated measurements, using a large sample, and controlling extraneous variables .
You can avoid systematic error through careful design of your sampling , data collection , and analysis procedures. For example, use triangulation to measure your variables using multiple methods; regularly calibrate instruments or procedures; use random sampling and random assignment ; and apply masking (blinding) where possible.
Systematic error is generally a bigger problem in research.
With random error, multiple measurements will tend to cluster around the true value. When you’re collecting data from a large sample , the errors in different directions will cancel each other out.
Systematic errors are much more problematic because they can skew your data away from the true value. This can lead you to false conclusions ( Type I and II errors ) about the relationship between the variables you’re studying.
Random and systematic error are two types of measurement error.
Random error is a chance difference between the observed and true values of something (e.g., a researcher misreading a weighing scale records an incorrect measurement).
Systematic error is a consistent or proportional difference between the observed and true values of something (e.g., a miscalibrated scale consistently records weights as higher than they actually are).
On graphs, the explanatory variable is conventionally placed on the x-axis, while the response variable is placed on the y-axis.
- If you have quantitative variables , use a scatterplot or a line graph.
- If your response variable is categorical, use a scatterplot or a line graph.
- If your explanatory variable is categorical, use a bar graph.
The term “ explanatory variable ” is sometimes preferred over “ independent variable ” because, in real world contexts, independent variables are often influenced by other variables. This means they aren’t totally independent.
Multiple independent variables may also be correlated with each other, so “explanatory variables” is a more appropriate term.
The difference between explanatory and response variables is simple:
- An explanatory variable is the expected cause, and it explains the results.
- A response variable is the expected effect, and it responds to other variables.
In a controlled experiment , all extraneous variables are held constant so that they can’t influence the results. Controlled experiments require:
- A control group that receives a standard treatment, a fake treatment, or no treatment.
- Random assignment of participants to ensure the groups are equivalent.
Depending on your study topic, there are various other methods of controlling variables .
There are 4 main types of extraneous variables :
- Demand characteristics : environmental cues that encourage participants to conform to researchers’ expectations.
- Experimenter effects : unintentional actions by researchers that influence study outcomes.
- Situational variables : environmental variables that alter participants’ behaviors.
- Participant variables : any characteristic or aspect of a participant’s background that could affect study results.
An extraneous variable is any variable that you’re not investigating that can potentially affect the dependent variable of your research study.
A confounding variable is a type of extraneous variable that not only affects the dependent variable, but is also related to the independent variable.
In a factorial design, multiple independent variables are tested.
If you test two variables, each level of one independent variable is combined with each level of the other independent variable to create different conditions.
Within-subjects designs have many potential threats to internal validity , but they are also very statistically powerful .
Advantages:
- Only requires small samples
- Statistically powerful
- Removes the effects of individual differences on the outcomes
Disadvantages:
- Internal validity threats reduce the likelihood of establishing a direct relationship between variables
- Time-related effects, such as growth, can influence the outcomes
- Carryover effects mean that the specific order of different treatments affect the outcomes
While a between-subjects design has fewer threats to internal validity , it also requires more participants for high statistical power than a within-subjects design .
- Prevents carryover effects of learning and fatigue.
- Shorter study duration.
- Needs larger samples for high power.
- Uses more resources to recruit participants, administer sessions, cover costs, etc.
- Individual differences may be an alternative explanation for results.
Yes. Between-subjects and within-subjects designs can be combined in a single study when you have two or more independent variables (a factorial design). In a mixed factorial design, one variable is altered between subjects and another is altered within subjects.
In a between-subjects design , every participant experiences only one condition, and researchers assess group differences between participants in various conditions.
In a within-subjects design , each participant experiences all conditions, and researchers test the same participants repeatedly for differences between conditions.
The word “between” means that you’re comparing different conditions between groups, while the word “within” means you’re comparing different conditions within the same group.
Random assignment is used in experiments with a between-groups or independent measures design. In this research design, there’s usually a control group and one or more experimental groups. Random assignment helps ensure that the groups are comparable.
In general, you should always use random assignment in this type of experimental design when it is ethically possible and makes sense for your study topic.
To implement random assignment , assign a unique number to every member of your study’s sample .
Then, you can use a random number generator or a lottery method to randomly assign each number to a control or experimental group. You can also do so manually, by flipping a coin or rolling a dice to randomly assign participants to groups.
Random selection, or random sampling , is a way of selecting members of a population for your study’s sample.
In contrast, random assignment is a way of sorting the sample into control and experimental groups.
Random sampling enhances the external validity or generalizability of your results, while random assignment improves the internal validity of your study.
In experimental research, random assignment is a way of placing participants from your sample into different groups using randomization. With this method, every member of the sample has a known or equal chance of being placed in a control group or an experimental group.
“Controlling for a variable” means measuring extraneous variables and accounting for them statistically to remove their effects on other variables.
Researchers often model control variable data along with independent and dependent variable data in regression analyses and ANCOVAs . That way, you can isolate the control variable’s effects from the relationship between the variables of interest.
Control variables help you establish a correlational or causal relationship between variables by enhancing internal validity .
If you don’t control relevant extraneous variables , they may influence the outcomes of your study, and you may not be able to demonstrate that your results are really an effect of your independent variable .
A control variable is any variable that’s held constant in a research study. It’s not a variable of interest in the study, but it’s controlled because it could influence the outcomes.
Including mediators and moderators in your research helps you go beyond studying a simple relationship between two variables for a fuller picture of the real world. They are important to consider when studying complex correlational or causal relationships.
Mediators are part of the causal pathway of an effect, and they tell you how or why an effect takes place. Moderators usually help you judge the external validity of your study by identifying the limitations of when the relationship between variables holds.
If something is a mediating variable :
- It’s caused by the independent variable .
- It influences the dependent variable
- When it’s taken into account, the statistical correlation between the independent and dependent variables is higher than when it isn’t considered.
A confounder is a third variable that affects variables of interest and makes them seem related when they are not. In contrast, a mediator is the mechanism of a relationship between two variables: it explains the process by which they are related.
A mediator variable explains the process through which two variables are related, while a moderator variable affects the strength and direction of that relationship.
There are three key steps in systematic sampling :
- Define and list your population , ensuring that it is not ordered in a cyclical or periodic order.
- Decide on your sample size and calculate your interval, k , by dividing your population by your target sample size.
- Choose every k th member of the population as your sample.
Systematic sampling is a probability sampling method where researchers select members of the population at a regular interval – for example, by selecting every 15th person on a list of the population. If the population is in a random order, this can imitate the benefits of simple random sampling .
Yes, you can create a stratified sample using multiple characteristics, but you must ensure that every participant in your study belongs to one and only one subgroup. In this case, you multiply the numbers of subgroups for each characteristic to get the total number of groups.
For example, if you were stratifying by location with three subgroups (urban, rural, or suburban) and marital status with five subgroups (single, divorced, widowed, married, or partnered), you would have 3 x 5 = 15 subgroups.
You should use stratified sampling when your sample can be divided into mutually exclusive and exhaustive subgroups that you believe will take on different mean values for the variable that you’re studying.
Using stratified sampling will allow you to obtain more precise (with lower variance ) statistical estimates of whatever you are trying to measure.
For example, say you want to investigate how income differs based on educational attainment, but you know that this relationship can vary based on race. Using stratified sampling, you can ensure you obtain a large enough sample from each racial group, allowing you to draw more precise conclusions.
In stratified sampling , researchers divide subjects into subgroups called strata based on characteristics that they share (e.g., race, gender, educational attainment).
Once divided, each subgroup is randomly sampled using another probability sampling method.
Cluster sampling is more time- and cost-efficient than other probability sampling methods , particularly when it comes to large samples spread across a wide geographical area.
However, it provides less statistical certainty than other methods, such as simple random sampling , because it is difficult to ensure that your clusters properly represent the population as a whole.
There are three types of cluster sampling : single-stage, double-stage and multi-stage clustering. In all three types, you first divide the population into clusters, then randomly select clusters for use in your sample.
- In single-stage sampling , you collect data from every unit within the selected clusters.
- In double-stage sampling , you select a random sample of units from within the clusters.
- In multi-stage sampling , you repeat the procedure of randomly sampling elements from within the clusters until you have reached a manageable sample.
Cluster sampling is a probability sampling method in which you divide a population into clusters, such as districts or schools, and then randomly select some of these clusters as your sample.
The clusters should ideally each be mini-representations of the population as a whole.
If properly implemented, simple random sampling is usually the best sampling method for ensuring both internal and external validity . However, it can sometimes be impractical and expensive to implement, depending on the size of the population to be studied,
If you have a list of every member of the population and the ability to reach whichever members are selected, you can use simple random sampling.
The American Community Survey is an example of simple random sampling . In order to collect detailed data on the population of the US, the Census Bureau officials randomly select 3.5 million households per year and use a variety of methods to convince them to fill out the survey.
Simple random sampling is a type of probability sampling in which the researcher randomly selects a subset of participants from a population . Each member of the population has an equal chance of being selected. Data is then collected from as large a percentage as possible of this random subset.
Quasi-experimental design is most useful in situations where it would be unethical or impractical to run a true experiment .
Quasi-experiments have lower internal validity than true experiments, but they often have higher external validity as they can use real-world interventions instead of artificial laboratory settings.
A quasi-experiment is a type of research design that attempts to establish a cause-and-effect relationship. The main difference with a true experiment is that the groups are not randomly assigned.
Blinding is important to reduce research bias (e.g., observer bias , demand characteristics ) and ensure a study’s internal validity .
If participants know whether they are in a control or treatment group , they may adjust their behavior in ways that affect the outcome that researchers are trying to measure. If the people administering the treatment are aware of group assignment, they may treat participants differently and thus directly or indirectly influence the final results.
- In a single-blind study , only the participants are blinded.
- In a double-blind study , both participants and experimenters are blinded.
- In a triple-blind study , the assignment is hidden not only from participants and experimenters, but also from the researchers analyzing the data.
Blinding means hiding who is assigned to the treatment group and who is assigned to the control group in an experiment .
A true experiment (a.k.a. a controlled experiment) always includes at least one control group that doesn’t receive the experimental treatment.
However, some experiments use a within-subjects design to test treatments without a control group. In these designs, you usually compare one group’s outcomes before and after a treatment (instead of comparing outcomes between different groups).
For strong internal validity , it’s usually best to include a control group if possible. Without a control group, it’s harder to be certain that the outcome was caused by the experimental treatment and not by other variables.
An experimental group, also known as a treatment group, receives the treatment whose effect researchers wish to study, whereas a control group does not. They should be identical in all other ways.
Individual Likert-type questions are generally considered ordinal data , because the items have clear rank order, but don’t have an even distribution.
Overall Likert scale scores are sometimes treated as interval data. These scores are considered to have directionality and even spacing between them.
The type of data determines what statistical tests you should use to analyze your data.
A Likert scale is a rating scale that quantitatively assesses opinions, attitudes, or behaviors. It is made up of 4 or more questions that measure a single attitude or trait when response scores are combined.
To use a Likert scale in a survey , you present participants with Likert-type questions or statements, and a continuum of items, usually with 5 or 7 possible responses, to capture their degree of agreement.
In scientific research, concepts are the abstract ideas or phenomena that are being studied (e.g., educational achievement). Variables are properties or characteristics of the concept (e.g., performance at school), while indicators are ways of measuring or quantifying variables (e.g., yearly grade reports).
The process of turning abstract concepts into measurable variables and indicators is called operationalization .
There are various approaches to qualitative data analysis , but they all share five steps in common:
- Prepare and organize your data.
- Review and explore your data.
- Develop a data coding system.
- Assign codes to the data.
- Identify recurring themes.
The specifics of each step depend on the focus of the analysis. Some common approaches include textual analysis , thematic analysis , and discourse analysis .
There are five common approaches to qualitative research :
- Grounded theory involves collecting data in order to develop new theories.
- Ethnography involves immersing yourself in a group or organization to understand its culture.
- Narrative research involves interpreting stories to understand how people make sense of their experiences and perceptions.
- Phenomenological research involves investigating phenomena through people’s lived experiences.
- Action research links theory and practice in several cycles to drive innovative changes.
Hypothesis testing is a formal procedure for investigating our ideas about the world using statistics. It is used by scientists to test specific predictions, called hypotheses , by calculating how likely it is that a pattern or relationship between variables could have arisen by chance.
Operationalization means turning abstract conceptual ideas into measurable observations.
For example, the concept of social anxiety isn’t directly observable, but it can be operationally defined in terms of self-rating scores, behavioral avoidance of crowded places, or physical anxiety symptoms in social situations.
Before collecting data , it’s important to consider how you will operationalize the variables that you want to measure.
When conducting research, collecting original data has significant advantages:
- You can tailor data collection to your specific research aims (e.g. understanding the needs of your consumers or user testing your website)
- You can control and standardize the process for high reliability and validity (e.g. choosing appropriate measurements and sampling methods )
However, there are also some drawbacks: data collection can be time-consuming, labor-intensive and expensive. In some cases, it’s more efficient to use secondary data that has already been collected by someone else, but the data might be less reliable.
Data collection is the systematic process by which observations or measurements are gathered in research. It is used in many different contexts by academics, governments, businesses, and other organizations.
There are several methods you can use to decrease the impact of confounding variables on your research: restriction, matching, statistical control and randomization.
In restriction , you restrict your sample by only including certain subjects that have the same values of potential confounding variables.
In matching , you match each of the subjects in your treatment group with a counterpart in the comparison group. The matched subjects have the same values on any potential confounding variables, and only differ in the independent variable .
In statistical control , you include potential confounders as variables in your regression .
In randomization , you randomly assign the treatment (or independent variable) in your study to a sufficiently large number of subjects, which allows you to control for all potential confounding variables.
A confounding variable is closely related to both the independent and dependent variables in a study. An independent variable represents the supposed cause , while the dependent variable is the supposed effect . A confounding variable is a third variable that influences both the independent and dependent variables.
Failing to account for confounding variables can cause you to wrongly estimate the relationship between your independent and dependent variables.
To ensure the internal validity of your research, you must consider the impact of confounding variables. If you fail to account for them, you might over- or underestimate the causal relationship between your independent and dependent variables , or even find a causal relationship where none exists.
Yes, but including more than one of either type requires multiple research questions .
For example, if you are interested in the effect of a diet on health, you can use multiple measures of health: blood sugar, blood pressure, weight, pulse, and many more. Each of these is its own dependent variable with its own research question.
You could also choose to look at the effect of exercise levels as well as diet, or even the additional effect of the two combined. Each of these is a separate independent variable .
To ensure the internal validity of an experiment , you should only change one independent variable at a time.
No. The value of a dependent variable depends on an independent variable, so a variable cannot be both independent and dependent at the same time. It must be either the cause or the effect, not both!
You want to find out how blood sugar levels are affected by drinking diet soda and regular soda, so you conduct an experiment .
- The type of soda – diet or regular – is the independent variable .
- The level of blood sugar that you measure is the dependent variable – it changes depending on the type of soda.
Determining cause and effect is one of the most important parts of scientific research. It’s essential to know which is the cause – the independent variable – and which is the effect – the dependent variable.
In non-probability sampling , the sample is selected based on non-random criteria, and not every member of the population has a chance of being included.
Common non-probability sampling methods include convenience sampling , voluntary response sampling, purposive sampling , snowball sampling, and quota sampling .
Probability sampling means that every member of the target population has a known chance of being included in the sample.
Probability sampling methods include simple random sampling , systematic sampling , stratified sampling , and cluster sampling .
Using careful research design and sampling procedures can help you avoid sampling bias . Oversampling can be used to correct undercoverage bias .
Some common types of sampling bias include self-selection bias , nonresponse bias , undercoverage bias , survivorship bias , pre-screening or advertising bias, and healthy user bias.
Sampling bias is a threat to external validity – it limits the generalizability of your findings to a broader group of people.
A sampling error is the difference between a population parameter and a sample statistic .
A statistic refers to measures about the sample , while a parameter refers to measures about the population .
Populations are used when a research question requires data from every member of the population. This is usually only feasible when the population is small and easily accessible.
Samples are used to make inferences about populations . Samples are easier to collect data from because they are practical, cost-effective, convenient, and manageable.
There are seven threats to external validity : selection bias , history, experimenter effect, Hawthorne effect , testing effect, aptitude-treatment and situation effect.
The two types of external validity are population validity (whether you can generalize to other groups of people) and ecological validity (whether you can generalize to other situations and settings).
The external validity of a study is the extent to which you can generalize your findings to different groups of people, situations, and measures.
Cross-sectional studies cannot establish a cause-and-effect relationship or analyze behavior over a period of time. To investigate cause and effect, you need to do a longitudinal study or an experimental study .
Cross-sectional studies are less expensive and time-consuming than many other types of study. They can provide useful insights into a population’s characteristics and identify correlations for further research.
Sometimes only cross-sectional data is available for analysis; other times your research question may only require a cross-sectional study to answer it.
Longitudinal studies can last anywhere from weeks to decades, although they tend to be at least a year long.
The 1970 British Cohort Study , which has collected data on the lives of 17,000 Brits since their births in 1970, is one well-known example of a longitudinal study .
Longitudinal studies are better to establish the correct sequence of events, identify changes over time, and provide insight into cause-and-effect relationships, but they also tend to be more expensive and time-consuming than other types of studies.
Longitudinal studies and cross-sectional studies are two different types of research design . In a cross-sectional study you collect data from a population at a specific point in time; in a longitudinal study you repeatedly collect data from the same sample over an extended period of time.
There are eight threats to internal validity : history, maturation, instrumentation, testing, selection bias , regression to the mean, social interaction and attrition .
Internal validity is the extent to which you can be confident that a cause-and-effect relationship established in a study cannot be explained by other factors.
The research methods you use depend on the type of data you need to answer your research question .
- If you want to measure something or test a hypothesis , use quantitative methods . If you want to explore ideas, thoughts and meanings, use qualitative methods .
- If you want to analyze a large amount of readily-available data, use secondary data. If you want data specific to your purposes with control over how it is generated, collect primary data.
- If you want to establish cause-and-effect relationships between variables , use experimental methods. If you want to understand the characteristics of a research subject, use descriptive methods.
A confounding variable , also called a confounder or confounding factor, is a third variable in a study examining a potential cause-and-effect relationship.
A confounding variable is related to both the supposed cause and the supposed effect of the study. It can be difficult to separate the true effect of the independent variable from the effect of the confounding variable.
In your research design , it’s important to identify potential confounding variables and plan how you will reduce their impact.
Discrete and continuous variables are two types of quantitative variables :
- Discrete variables represent counts (e.g. the number of objects in a collection).
- Continuous variables represent measurable amounts (e.g. water volume or weight).
Quantitative variables are any variables where the data represent amounts (e.g. height, weight, or age).
Categorical variables are any variables where the data represent groups. This includes rankings (e.g. finishing places in a race), classifications (e.g. brands of cereal), and binary outcomes (e.g. coin flips).
You need to know what type of variables you are working with to choose the right statistical test for your data and interpret your results .
You can think of independent and dependent variables in terms of cause and effect: an independent variable is the variable you think is the cause , while a dependent variable is the effect .
In an experiment, you manipulate the independent variable and measure the outcome in the dependent variable. For example, in an experiment about the effect of nutrients on crop growth:
- The independent variable is the amount of nutrients added to the crop field.
- The dependent variable is the biomass of the crops at harvest time.
Defining your variables, and deciding how you will manipulate and measure them, is an important part of experimental design .
Experimental design means planning a set of procedures to investigate a relationship between variables . To design a controlled experiment, you need:
- A testable hypothesis
- At least one independent variable that can be precisely manipulated
- At least one dependent variable that can be precisely measured
When designing the experiment, you decide:
- How you will manipulate the variable(s)
- How you will control for any potential confounding variables
- How many subjects or samples will be included in the study
- How subjects will be assigned to treatment levels
Experimental design is essential to the internal and external validity of your experiment.
I nternal validity is the degree of confidence that the causal relationship you are testing is not influenced by other factors or variables .
External validity is the extent to which your results can be generalized to other contexts.
The validity of your experiment depends on your experimental design .
Reliability and validity are both about how well a method measures something:
- Reliability refers to the consistency of a measure (whether the results can be reproduced under the same conditions).
- Validity refers to the accuracy of a measure (whether the results really do represent what they are supposed to measure).
If you are doing experimental research, you also have to consider the internal and external validity of your experiment.
A sample is a subset of individuals from a larger population . Sampling means selecting the group that you will actually collect data from in your research. For example, if you are researching the opinions of students in your university, you could survey a sample of 100 students.
In statistics, sampling allows you to test a hypothesis about the characteristics of a population.
Quantitative research deals with numbers and statistics, while qualitative research deals with words and meanings.
Quantitative methods allow you to systematically measure variables and test hypotheses . Qualitative methods allow you to explore concepts and experiences in more detail.
Methodology refers to the overarching strategy and rationale of your research project . It involves studying the methods used in your field and the theories or principles behind them, in order to develop an approach that matches your objectives.
Methods are the specific tools and procedures you use to collect and analyze data (for example, experiments, surveys , and statistical tests ).
In shorter scientific papers, where the aim is to report the findings of a specific study, you might simply describe what you did in a methods section .
In a longer or more complex research project, such as a thesis or dissertation , you will probably include a methodology section , where you explain your approach to answering the research questions and cite relevant sources to support your choice of methods.
Ask our team
Want to contact us directly? No problem. We are always here for you.
- Email [email protected]
- Start live chat
- Call +1 (510) 822-8066
- WhatsApp +31 20 261 6040
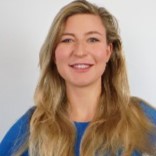
Our team helps students graduate by offering:
- A world-class citation generator
- Plagiarism Checker software powered by Turnitin
- Innovative Citation Checker software
- Professional proofreading services
- Over 300 helpful articles about academic writing, citing sources, plagiarism, and more
Scribbr specializes in editing study-related documents . We proofread:
- PhD dissertations
- Research proposals
- Personal statements
- Admission essays
- Motivation letters
- Reflection papers
- Journal articles
- Capstone projects
Scribbr’s Plagiarism Checker is powered by elements of Turnitin’s Similarity Checker , namely the plagiarism detection software and the Internet Archive and Premium Scholarly Publications content databases .
The add-on AI detector is powered by Scribbr’s proprietary software.
The Scribbr Citation Generator is developed using the open-source Citation Style Language (CSL) project and Frank Bennett’s citeproc-js . It’s the same technology used by dozens of other popular citation tools, including Mendeley and Zotero.
You can find all the citation styles and locales used in the Scribbr Citation Generator in our publicly accessible repository on Github .
Have a language expert improve your writing
Run a free plagiarism check in 10 minutes, automatically generate references for free.
- Knowledge Base
- Methodology
- Mixed Methods Research | Definition, Guide, & Examples
Mixed Methods Research | Definition, Guide, & Examples
Published on 4 April 2022 by Tegan George . Revised on 25 October 2022.
Mixed methods research combines elements of quantitative research and qualitative research in order to answer your research question . Mixed methods can help you gain a more complete picture than a standalone quantitative or qualitative study, as it integrates benefits of both methods.
Mixed methods research is often used in the behavioral, health, and social sciences, especially in multidisciplinary settings and complex situational or societal research.
- To what extent does the frequency of traffic accidents ( quantitative ) reflect cyclist perceptions of road safety ( qualitative ) in Amsterdam?
- How do student perceptions of their school environment ( qualitative ) relate to differences in test scores ( quantitative ) ?
- How do interviews about job satisfaction at Company X ( qualitative ) help explain year-over-year sales performance and other KPIs ( quantitative ) ?
- How can voter and non-voter beliefs about democracy ( qualitative ) help explain election turnout patterns ( quantitative ) in Town X?
- How do average hospital salary measurements over time (quantitative) help to explain nurse testimonials about job satisfaction (qualitative) ?
Table of contents
When to use mixed methods research, mixed methods research designs, benefits of mixed methods research, disadvantages of mixed methods research, frequently asked questions about mixed methods research.
Mixed methods research may be the right choice if your research process suggests that quantitative or qualitative data alone will not sufficiently answer your research question. There are several common reasons for using mixed methods research:
- Generalisability : Qualitative research usually has a smaller sample size , and thus is not generalisable . In mixed methods research, this comparative weakness is mitigated by the comparative strength of ‘large N’, externally valid quantitative research.
- Contextualisation: Mixing methods allows you to put findings in context and add richer detail to your conclusions. Using qualitative data to illustrate quantitative findings can help ‘put meat on the bones’ of your analysis.
- Credibility: Using different methods to collect data on the same subject can make your results more credible. If the qualitative and quantitative data converge, this strengthens the validity of your conclusions. This process is called triangulation .
As you formulate your research question , try to directly address how qualitative and quantitative methods will be combined in your study. If your research question can be sufficiently answered via standalone quantitative or qualitative analysis, a mixed methods approach may not be the right fit.
Keep in mind that mixed methods research doesn’t just mean collecting both types of data; you need to carefully consider the relationship between the two and how you’ll integrate them into coherent conclusions. Mixed methods can be very challenging to put into practice, so it’s a less common choice than standalone qualitative or qualitative research.
Prevent plagiarism, run a free check.
There are different types of mixed methods research designs . The differences between them relate to the aim of the research, the timing of the data collection , and the importance given to each data type.
As you design your mixed methods study, also keep in mind:
- Your research approach ( inductive vs deductive )
- Your research questions
- What kind of data is already available for you to use
- What kind of data you’re able to collect yourself.
Here are a few of the most common mixed methods designs.
Convergent parallel
In a convergent parallel design, you collect quantitative and qualitative data at the same time and analyse them separately. After both analyses are complete, compare your results to draw overall conclusions.
- On the qualitative side, you analyse cyclist complaints via the city’s database and on social media to find out which areas are perceived as dangerous and why.
- On the quantitative side, you analyse accident reports in the city’s database to find out how frequently accidents occur in different areas of the city.
In an embedded design, you collect and analyse both types of data at the same time, but within a larger quantitative or qualitative design. One type of data is secondary to the other.
This is a good approach to take if you have limited time or resources. You can use an embedded design to strengthen or supplement your conclusions from the primary type of research design.
Explanatory sequential
In an explanatory sequential design, your quantitative data collection and analysis occurs first, followed by qualitative data collection and analysis.
You should use this design if you think your qualitative data will explain and contextualise your quantitative findings.
Exploratory sequential
In an exploratory sequential design, qualitative data collection and analysis occurs first, followed by quantitative data collection and analysis.
You can use this design to first explore initial questions and develop hypotheses. Then you can use the quantitative data to test or confirm your qualitative findings.
‘Best of both worlds’ analysis
Combining the two types of data means you benefit from both the detailed, contextualised insights of qualitative data and the generalisable, externally valid insights of quantitative data. The strengths of one type of data often mitigate the weaknesses of the other.
For example, solely quantitative studies often struggle to incorporate the lived experiences of your participants, so adding qualitative data deepens and enriches your quantitative results.
Solely qualitative studies are often not very generalisable, only reflecting the experiences of your participants, so adding quantitative data can validate your qualitative findings.
Method flexibility
Mixed methods are less tied to disciplines and established research paradigms. They offer more flexibility in designing your research, allowing you to combine aspects of different types of studies to distill the most informative results.
Mixed methods research can also combine theory generation and hypothesis testing within a single study, which is unusual for standalone qualitative or quantitative studies.
Mixed methods research is very labour-intensive. Collecting, analysing, and synthesising two types of data into one research product takes a lot of time and effort, and often involves interdisciplinary teams of researchers rather than individuals. For this reason, mixed methods research has the potential to cost much more than standalone studies.
Differing or conflicting results
If your analysis yields conflicting results, it can be very challenging to know how to interpret them in a mixed methods study. If the quantitative and qualitative results do not agree or you are concerned you may have confounding variables , it can be unclear how to proceed.
Quantitative research deals with numbers and statistics, while qualitative research deals with words and meanings.
Quantitative methods allow you to test a hypothesis by systematically collecting and analysing data, while qualitative methods allow you to explore ideas and experiences in depth.
In mixed methods research , you use both qualitative and quantitative data collection and analysis methods to answer your research question .
Data collection is the systematic process by which observations or measurements are gathered in research. It is used in many different contexts by academics, governments, businesses, and other organisations.
Triangulation in research means using multiple datasets, methods, theories and/or investigators to address a research question. It’s a research strategy that can help you enhance the validity and credibility of your findings.
Triangulation is mainly used in qualitative research , but it’s also commonly applied in quantitative research . Mixed methods research always uses triangulation.
These are four of the most common mixed methods designs :
- Convergent parallel: Quantitative and qualitative data are collected at the same time and analysed separately. After both analyses are complete, compare your results to draw overall conclusions.
- Embedded: Quantitative and qualitative data are collected at the same time, but within a larger quantitative or qualitative design. One type of data is secondary to the other.
- Explanatory sequential: Quantitative data is collected and analysed first, followed by qualitative data. You can use this design if you think your qualitative data will explain and contextualise your quantitative findings.
- Exploratory sequential: Qualitative data is collected and analysed first, followed by quantitative data. You can use this design if you think the quantitative data will confirm or validate your qualitative findings.
Cite this Scribbr article
If you want to cite this source, you can copy and paste the citation or click the ‘Cite this Scribbr article’ button to automatically add the citation to our free Reference Generator.
George, T. (2022, October 25). Mixed Methods Research | Definition, Guide, & Examples. Scribbr. Retrieved 15 April 2024, from https://www.scribbr.co.uk/research-methods/mixed-methods/
Is this article helpful?
Tegan George
Read our research on: Gun Policy | International Conflict | Election 2024
Regions & Countries
1. the partisanship and ideology of american voters.
The partisan identification of registered voters is now evenly split between the two major parties: 49% of registered voters are Democrats or lean to the Democratic Party, and a nearly identical share – 48% – are Republicans or lean to the Republican Party.
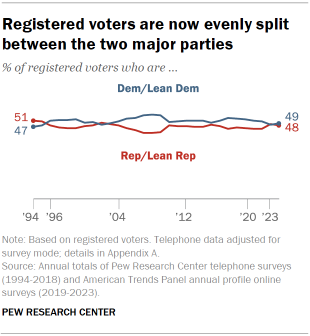
The partisan balance has tightened in recent years following a clear edge in Democratic Party affiliation during the last administration.
- Four years ago, in the run-up to the 2020 election, Democrats had a 5 percentage point advantage over the GOP (51% vs. 46%).
The share of voters who are in the Democratic coalition reached 55% in 2008. For much of the last three decades of Pew Research Center surveys, the partisan composition of registered voters has been more closely divided.
Partisans and partisan leaners in the U.S. electorate
About two-thirds of registered voters identify as a partisan, and they are roughly evenly split between those who say they are Republicans (32% of voters) and those who say they are Democrats (33%). Roughly a third instead say they are independents or something else (35%), with most of these voters leaning toward one of the parties. Partisan leaners often share the same political views and behaviors as those who directly identify with the party they favor.
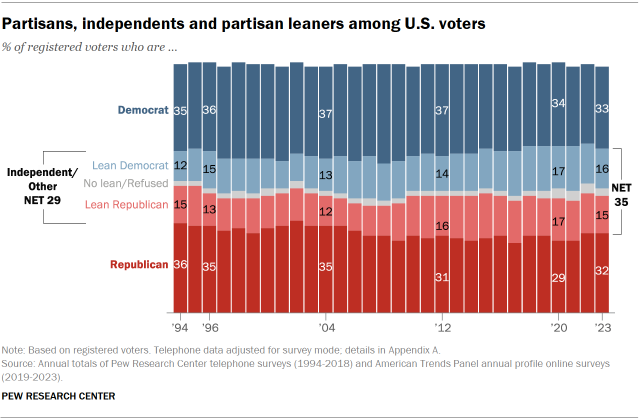
The share of voters who identify as independent or something else is somewhat higher than in the late 1990s and early 2000s. As a result, there are more “leaners” today than in the past. Currently, 15% of voters lean toward the Republican Party and 16% lean toward the Democratic Party. By comparison, in 1994, 27% of voters leaned toward either the GOP (15%) or the Democratic Party (12%).
Party identification and ideology
While the electorate overall is nearly equally divided between those who align with the Republican and Democratic parties, a greater share of registered voters say they are both ideologically conservative and associate with the Republican Party (33%) than say they are liberal and align with the Democratic Party (23%).
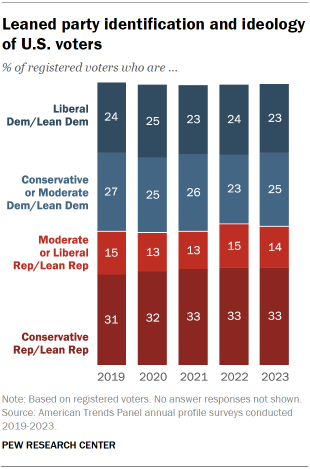
A quarter of voters associate with the Democratic Party and describe their views as either conservative or moderate, and 14% identify as moderates or liberals and are Republicans or Republican leaners.
The partisan and ideological composition of voters is relatively unchanged over the last five years.
(As a result of significant mode differences in measures of ideology between telephone and online surveys, there is not directly comparable data on ideology prior to 2019.)
Add Pew Research Center to your Alexa
Say “Alexa, enable the Pew Research Center flash briefing”
Report Materials
Table of contents, behind biden’s 2020 victory, a voter data resource: detailed demographic tables about verified voters in 2016, 2018, what the 2020 electorate looks like by party, race and ethnicity, age, education and religion, interactive map: the changing racial and ethnic makeup of the u.s. electorate, in changing u.s. electorate, race and education remain stark dividing lines, most popular.
About Pew Research Center Pew Research Center is a nonpartisan fact tank that informs the public about the issues, attitudes and trends shaping the world. It conducts public opinion polling, demographic research, media content analysis and other empirical social science research. Pew Research Center does not take policy positions. It is a subsidiary of The Pew Charitable Trusts .

- SUGGESTED TOPICS
- The Magazine
- Newsletters
- Managing Yourself
- Managing Teams
- Work-life Balance
- The Big Idea
- Data & Visuals
- Reading Lists
- Case Selections
- HBR Learning
- Topic Feeds
- Account Settings
- Email Preferences
6 Common Leadership Styles — and How to Decide Which to Use When
- Rebecca Knight

Being a great leader means recognizing that different circumstances call for different approaches.
Research suggests that the most effective leaders adapt their style to different circumstances — be it a change in setting, a shift in organizational dynamics, or a turn in the business cycle. But what if you feel like you’re not equipped to take on a new and different leadership style — let alone more than one? In this article, the author outlines the six leadership styles Daniel Goleman first introduced in his 2000 HBR article, “Leadership That Gets Results,” and explains when to use each one. The good news is that personality is not destiny. Even if you’re naturally introverted or you tend to be driven by data and analysis rather than emotion, you can still learn how to adapt different leadership styles to organize, motivate, and direct your team.
Much has been written about common leadership styles and how to identify the right style for you, whether it’s transactional or transformational, bureaucratic or laissez-faire. But according to Daniel Goleman, a psychologist best known for his work on emotional intelligence, “Being a great leader means recognizing that different circumstances may call for different approaches.”

- RK Rebecca Knight is a journalist who writes about all things related to the changing nature of careers and the workplace. Her essays and reported stories have been featured in The Boston Globe, Business Insider, The New York Times, BBC, and The Christian Science Monitor. She was shortlisted as a Reuters Institute Fellow at Oxford University in 2023. Earlier in her career, she spent a decade as an editor and reporter at the Financial Times in New York, London, and Boston.
Partner Center

An official website of the United States government
The .gov means it’s official. Federal government websites often end in .gov or .mil. Before sharing sensitive information, make sure you’re on a federal government site.
The site is secure. The https:// ensures that you are connecting to the official website and that any information you provide is encrypted and transmitted securely.
- Publications
- Account settings
Preview improvements coming to the PMC website in October 2024. Learn More or Try it out now .
- Advanced Search
- Journal List
- Acta Inform Med
- v.30(1); 2022 Mar
Mixed Methodology of Scientific Research in Healthcare
Emina smajic.
1 Agram Polyclinic, Sarajevo, Bosnia and Herzegovina
Dijana Avdic
2 Faculty of Health Studies, Sarajevo, Bosnia and Herzegovina
Aleksandra Pasic
3 Clinical Biochemistry with Immunology, Sarajevo, Clinical Center University of Sarajevo, Bosnia and Herzegovina
Alden Prcic
4 General Hospital “Prim dr. Abdulah Nakas”, Sarajevo, Bosnia and Herzegovina
Maja Stancic
Background:.
Scientific research is usually classified as quantitative or qualitative. However, methodologists are increasingly emphasizing the integration of qualitative and quantitative data as the center of mixed methods (mix methodologies). Mixed research method implies the use of different research methods, ie. quantitative and qualitative methods in one study.
The aim of this review paper is to present the purpose of using a mixed methodology in health research.
The relevant articles were searched from online data sources including PubMed and Google Scholar.
This approach to the use of mixed methods creates opportunities for a deeper study of various problems. The purpose of using mixed research methods is to obtain valid answers to research questions, however the researcher may still have different reasons or purposes for which he wants to strengthen the research study and its conclusions by applying mixed methods. The use of mixed scientific methodology is widely used in the field of health outcomes and should not be limited to a closed list of possible methodological options.
Conclusion:
Recently, there has been an increase in the number of scientific studies in healthcare that use mixed research methods. The advantage of applying this scientific method is that through the triangulation of data obtained by different (quantitative / qualitative) approaches, we get a deeper and more complete picture of the phenomenon in health care that we observe.
1. BACKGROUND
In health science research, there is a priority to develop new methodologies to improve the quality and scientific strength of data leading to an extraordinary increase in methodological diversity. This diversity reflects the nature of public health problems, such as differences between populations, age groups, ethnic groups and cultures, poor adherence to treatments considered effective, behavioral factors contributing to disability and health, and translational needs for health research. Diversity also signals a growing acceptance of qualitative and social science research, the formation of interdisciplinary research teams, and the use of multilevel approaches to research complex health issues such as patient attitudes and cultural and social models of disease and health (1) .
2. OBJECTIVE
The retrieved articles were reviewed by the authors and the results are presented along with the relevant discussion
4.1. MIXED METHODOLOGY
Scientific research is usually classified as quantitative or qualitative. However, methodologists are increasingly emphasizing the integration of qualitative and quantitative data as the center of mixed methods (mix methodologies). Integration is a deliberate process by which the researcher combines quantitative and qualitative approaches in the study. Quantitative and qualitative data then become interdependent in solving questions and hypotheses. Mixed research method implies the use of different research methods, quantitative and qualitative methods in one study ( 2 , 3 ). Research on mixed methods should be distinguished from multi-method research (method-combination) in which either multiple qualitative approaches or only multiple quantitative approaches are combined (4) .

The most accepted definition of a mixed research method is that it is a research in which a researcher or team of researchers combines elements of a qualitative and quantitative approach to research (use of qualitative and quantitative perspectives, data collection, analysis, inference techniques) to understand and support research. As we see in the definition, the use of both quantitative and qualitative methods in a single study (or series of related studies) is crucial, unlike the use of combined methods that combine two or more quantitative or two or more qualitative research methods ( 2 , 4 ).
The basic premise of using mixed research methods is that some research issues can be addressed more comprehensively than using either quantitative or qualitative methods. The issues that benefit most from the design of mixed methods tend to be broad and complex, with multiple aspects that each can have. Mixed research methods can exploit the strengths and weaknesses of both approaches and can be particularly useful when addressing complex, multifaceted issues such as health service interventions and living with chronic diseases (2) . This approach to the use of mixed methods creates opportunities for a deeper study of various problems (5) .
4.2. PURPOSE OF USING MIXED RESEARCH
The purpose of using mixed research methods is to obtain valid answers to research questions, however the researcher may still have different reasons or purposes for which he wants to strengthen the research study and its conclusions by applying mixed methods. The purpose classification of mixed research methods was first introduced in 1989 by Greene, Caracelli, and Graham, based on an analysis of published studies of mixed methods. This classification is still used and we have a total of five “purposes” for why a mixed methodology is used in research (4) . Classification of the purpose of using mixed methodology:
- Complementarity. Using data obtained from one method to illustrate the results of another method.
- Development. Using the results of one method to develop or inform about the use of another method.
- Initiation. We can use the results of different methods to search for areas of non-compliance in certain areas in order to create new insights.
- Expansion. Examining different aspects of a research question, with each aspect justifying different methods.
- Triangulation. Use of data obtained by both methods to support the findings (2) .
In the last 28 years, this classification has been supplemented by several other authors. So in 2006 Bryman compiled a list of more specific rationales for the use or purpose of mixed research methods. Bryman’s classification decomposes the categorization of Greene et al. on several aspects and adds a number of additional aspects (3) .

Bryman’s addition to the classification of Greene et al.
Credibility. It refers to suggestions that the application of both methodological approaches improves the integrity of the results.
Context. Refers to cases where the combination is justified in terms of qualitative research that provides contextual understanding, along with generalized, externally valid results, or broad relationships between the variables identified by the survey.
Illustration. It refers to the use of qualitative data to illustrate quantitative findings, often referred to as putting “meat on the bone” “dry” quantitative findings.
Usefulness or improvement of usefulness of results. It refers to the suggestion, which is more likely to be highlighted among articles with an applied focus, that combining the two approaches will be more useful for practitioners.
Confirmation and discovery. It involves the use of qualitative data to generate hypotheses and the use of quantitative research to test them within a single project.
Diversity of views. This includes two slightly different explanations–namely, combining the perspective of researchers and participants through quantitative and qualitative research, and discovering the relationship between variables through quantitative research, while revealing meanings among research participants through qualitative research (3) .
4.3. RESEARCH DESIGN
Research designs are procedures for collecting, analyzing, interpreting, and reporting data in research studies. They represent different models for doing research, and these models have distinct names and procedures associated with them (6) .
The four major types of mixed methods designs are the Triangulation Design, the Embedded Design, the Explanatory Design, and the Exploratory Design (6) .
4.4. HEALTH APPLICATION
There is a wide range of methods used to collect both quantitative and qualitative data. And the research question and the necessary data are the main determinants of the methods used. To a lesser extent, the choice of methods may be influenced by feasibility. Method priority refers to the emphasis on each method in the study. For example, a study may be predominantly quantitative with a small qualitative component or vice versa. Alternatively, both quantitative and qualitative methods and data may be equally weighted. The emphasis on each methodological component of the study will be driven mainly by the research question, research team skills and feasibility. Finally, researchers must decide when each method will be used in research (2) . By analyzing the research methods and research designs used, Bryman suggests that on the quantitative side, structured interview and questionnaire research within cross-sectional design predominates, while on the qualitative side, semi-structured interviews within cross-sectional design predominate (7) . A key feature of mixed-method research is its methodological pluralism, which often results in research that provides broader perspectives than those offered by monomethodal designs (8) .
The use of mixed scientific methodology is widely used in the field of health outcomes and should not be limited to a closed list of possible methodological options, but should be seen as a framework for a specific research issue to be addressed using quantitative and qualitative components (data and / or methods) , when quantitative and qualitative components are articulated intentionally and prospectively in a well-defined, pre-specified research design and as a framework for meta-inference (9) .
The importance of applying mixed research methods can be reflected in the trend of “measuring / analysing what is important” for patients and the treatment outcomes reported by patients are increasingly used in clinical care and research. However, a recent review of studies documenting the development of outcome measures reported by patients highlights that only 11% of them were developed actually asking patients which outcomes are important to them. This emphasizes the importance of applying mixed qualitative and quantitative methods in health research to ensure a focus on the priorities identified by the patient, scientific rigor, and improved patient outcomes (9) . Mixed methods are also an increasingly accepted approach used to investigate organizational phenomena in health care (10) .
The application of the mix methodology is considered a significant contribution to health science. By combining quantitative and qualitative data in the same study, health researchers can reap the benefits of each approach while minimizing their disadvantages. In practice, this endeavor facilitates research by health science researchers on the complex and multifactorial nature of human health and disease. Researchers using the mixed method approach for the first time can easily feel overwhelmed by uncertainty about the philosophical foundations of the method, as well as the multitude of typologies of mixed method research. Although further research and debate are warranted, health researchers seeking solutions to real problems are increasingly embracing pragmatism as a paradigm of choice (11) .
Author’s contribution:
All authors were involved in preparation of this article. Final proofreading was made by first author.
Financial support and sponsorship:
Conflicts of interest:.
There are no conflicts of interest.

- Bioenergy Technologies Office
- Accomplishments
- National Biotechnology & Biomanufacturing Initiative
- Bold Goals for U.S. Biotechnology & Biomanufacturing
- 2023 Multi-Year Program Plan
- Bioproduct Production
- CO2 Utilization
- Deconstruction & Fractionation
- Synthesis & Upgrading
- Conversion Technologies Related Links
- Sustainability
- Data, Modeling, & Analysis Related Links
- Feedstock-Conversion Interface R&D
- Feedstock Technologies Related Links
- Algal Logistics
- Algal Production
- Algal Related Links
- Development
- Infrastructure
- Systems Development & Integration Related Links
- Sustainable Aviation Fuels
- Sustainable Marine Fuels
- Waste-to-Energy
- CO2 Reduction & Upgrading for e-Fuels Consortium
- Consortium for Computational Physics & Chemistry
- Co-Optimization of Fuels & Engines
- Feedstock-Conversion Interface Consortium
- Bioenergy FAQs
- Biofuel Basics
- Biopower Basics
- Bioproduct Basics
- Biomass Feedstocks
- Career Exploration Wheel
- Career Grid
- Workforce Training Opportunities Map
- Algae Technology Educational Consortium
- Workforce Development Strategy
- Bioenergy Jobs at BETO
- BRIDGES Events
- BRIDGES Webinar Recordings and Presentations
- Internships & Fellowships
- Teacher & Classroom Resources
- BioenergizeME Infographic Challenge
- Wood Heater Design Challenge
- Funding Opportunities
- Analytical Tools
- Bioenergy Publications
- Bioenergy Related Links
- State Biomass Contacts
- BioProse: Bioenergy R&D Blog
- Bioenergy News
- Bioeconomy 2020
- Bioeconomy 2018
- Bioeconomy 2017
- Bioenergy 2016
- Bioenergy 2015
- Biomass 2014
- Biomass 2013
- Biomass 2012
- Biomass 2011
- Inaccessible
- Biomass 2009
- Peer Review 2023
- Peer Review 2021
- Peer Review 2019
- Peer Review 2017
- Peer Review 2015
- Peer Review 2013
- Peer Review 2011
- Past Meetings & Workshops
- Bioenergy Newsletter
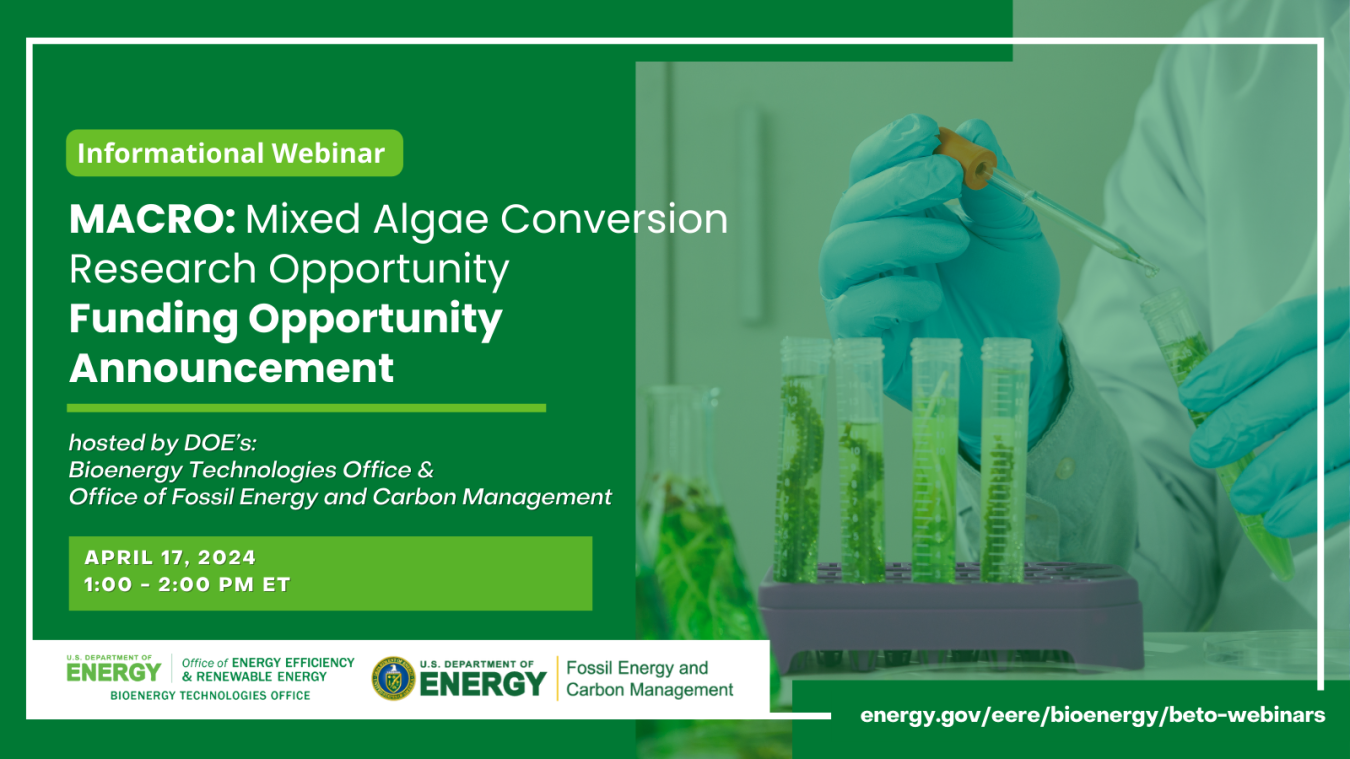
Register for this webinar today!
Join this informational webinar on April 17, 2024 , from 1:00 p.m. to 2:00 p.m. ET , to learn more about the MACRO: Mixed Algae Conversion Research Opportunity and the application process. The U.S. Department of Energy’s Bioenergy Technologies Office (BETO) and Office of Fossil Energy and Carbon Management (FECM) announced the MACRO funding opportunity announcement (FOA) . The FOA will award up to $18.8 million to address research and development (R&D) challenges in converting algae, such as seaweeds and other wet waste feedstocks, to biofuels and bioproducts that can decarbonize domestic transportation, industry, and communities.
Aligning with BETO and FECM’s strategic program goals, the FOA will fund selected projects in two focus areas:
- Topic Area 1 (funded by BETO) - Conversion of Seaweeds to Low Carbon Fuels and Bioproducts will focus on laboratory scale R&D on conversion of seaweeds and seaweed blends with other wet wastes to renewable fuels and bioproducts to enable these readily available feedstocks to access new markets.
- Topic Area 2 (funded by FECM) - Conversion of Algal Biomass for Low Carbon Agricultural Bioproducts will focus on near or completely integrated technologies that utilize CO 2 emissions streams from industrial sources or utilities to grow algae for source material and create value-added bioproducts. Of particular interest is the conversion and processing of bioproducts for use in agriculture and animal feed.
Register today for this informational session taking place on April 17, 2024 , from 1:00 p.m. to 2:00 p.m. ET , to learn more about the FOA and the application process.
- DocuSign-stock
- News for DocuSign
Analysts’ Opinions Are Mixed on These Technology Stocks: Salesforce (CRM) and DocuSign (DOCU)
Analysts have been eager to weigh in on the Technology sector with new ratings on Salesforce ( CRM – Research Report ) and DocuSign ( DOCU – Research Report ).
Salesforce (CRM)
In a report released today, Arjun Bhatia from William Blair maintained a Buy rating on Salesforce. The company’s shares closed last Friday at $294.32.
According to TipRanks.com , Bhatia is a 3-star analyst with an average return of 1.4% and a 46.1% success rate. Bhatia covers the Technology sector, focusing on stocks such as Zoom Video Communications, Zeta Global Holdings Corp, and DoubleVerify Holdings.
The word on The Street in general, suggests a Moderate Buy analyst consensus rating for Salesforce with a $338.06 average price target, implying a 13.9% upside from current levels. In a report released today, JMP Securities also maintained a Buy rating on the stock with a $342.00 price target.
See Insiders’ Hot Stocks on TipRanks >>
DocuSign (DOCU)
In a report released today, Jake Roberge from William Blair maintained a Hold rating on DocuSign. The company’s shares closed last Friday at $58.90.
According to TipRanks.com , Roberge is a 2-star analyst with an average return of 0.1% and a 47.2% success rate. Roberge covers the Technology sector, focusing on stocks such as Upland Software, Mitek Systems, and Jamf Holding.
Currently, the analyst consensus on DocuSign is a Hold with an average price target of $66.77, which is an 11.2% upside from current levels. In a report issued on April 12, UBS also upgraded the stock to Hold with a $62.00 price target.
TipRanks has tracked 36,000 company insiders and found that a few of them are better than others when it comes to timing their transactions. See which 3 stocks are most likely to make moves following their insider activities.
Read More on CRM:
- M&A News: Salesforce in Talks to Acquire Informatica
- Salesforce in advanced talks to acquire Informatica, WSJ reports
- Salesforce price target raised to $350 from $325 at RBC Capital
- Iqvia, Salesforce expand global partnership
- UiPath (NYSE:PATH) Rises amid Analyst Praise
DocuSign News MORE
Related stocks.
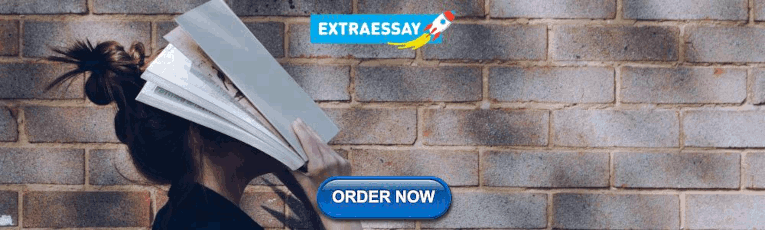
IMAGES
VIDEO
COMMENTS
Mixed methods research combines elements of quantitative research and qualitative research in order to answer your research question. Mixed methods can help you gain a more complete picture than a standalone quantitative or qualitative study, as it integrates benefits of both methods. Mixed methods research is often used in the behavioral ...
Quantitative dominant [or quantitatively driven] mixed methods research is the type of mixed research in which one relies on a quantitative, postpositivist view of the research process, while concurrently recognizing that the addition of qualitative data and approaches are likely to benefit most research projects. (p. 124)
The Nature and Design of Mixed Methods Research / 6. Best Practices for Mixed Methods Research in the Health Sciences • Embedding data. In this form of integration, a dataset of secondary priority is embedded within a larger, primary design. An example is the collection of supplemental qualitative data about how participants are
Mixed Methods Research. Mixed methods research is an approach to research that combines both quantitative and qualitative research methods in a single study or research project. It is a methodological approach that involves collecting and analyzing both numerical (quantitative) and narrative (qualitative) data to gain a more comprehensive understanding of a research problem.
Mixed‐methods research, or multi‐strategy designs, 1 can be defined as 'the collection, analysis and integration of both qualitative and quantitative data in a single study': 2 semi‐structured interviews and workplace measures (e.g. attendance data) might be undertaken concurrently to gain a multifaceted perspective on a particular ...
Mixed Methods. The interest in combining methods in social scientific research has a long history. Terms such as "triangulation," "combining methods," and "multiple methods" have been around for quite a while to designate using different methods of data analysis in empirical studies. However, this practice has gained new momentum ...
Mixed methods approaches allows researchers to use a diversity of methods, combining inductive and deductive thinking, and offsetting limitations of exclusively quantitative and qualitative research through a complementary approach that maximizes strengths of each data type and facilitates a more comprehensive understanding of health issues and ...
Abstract. Mixed methods research is becoming increasingly popular and is widely acknowledged as a means of achieving a more complex understanding of research problems. Combining both the in-depth, contextual views of qualitative research with the broader generalizations of larger population quantitative approaches, mixed methods research can be ...
DOI: 10.1177/1558689806298224. Argues that mixed methods research is one of the three major research paradigms. Based on interviews with leaders in the field of mixed methods, this article defines mixed methods research. Describes the history of mixed methods research and delineates future related issues. Teddlie, C., and A. Tashakkori. 2012.
A mixed methods research design is an approach to collecting and analyzing both qualitative and quantitative data in a single study. Mixed methods designs allow for method flexibility and can provide differing and even conflicting results. Examples of mixed methods research designs include convergent parallel, explanatory sequential, and ...
The relevance of mixed-methods in health research. The overall goal of the mixed-methods research design is to provide a better and deeper understanding, by providing a fuller picture that can enhance description and understanding of the phenomena [].Mixed-methods research has become popular because it uses quantitative and qualitative data in one single study which provides stronger inference ...
Mixed methods research is a research design with philosophical assump-tions as well as methods of inquiry. As a methodology, it involves philo-sophical assumptions that guide the direction of the collection and analysis of data and the mixture of qualitative and quantitative approaches
The scope includes delineating where mixed methods research may be used most effectively, illuminating design and procedure issues, and determining the logistics of conducting mixed methods research. This journal is a member of COPE. View full journal description.
Qualitative research is mainly applicable to exploratory, interpretative, and complex problems. One of the main reasons for using mixed methods research is the need for multiple data sources and data collection and analysis methods to address research problems that are too complex to be approached onedimensionally. Mixed methods research is ...
Mixed Methods Research is defined as a type of user research that combines qualitative and quantitative methods into a single study. Companies like Spotify, Airbnb and Lyft are using Mixed Methods ...
Mixed methods research (MMR) has been widely adopted in a plethora of disciplines. Integration is the pressing issue regarding the legitimation, the added value, and the quality of using MMR, though inadequate literature has discussed effective strategies used in the field of education, including school psychology, counseling, and teacher education.
'Mixed methods' is a research approach whereby researchers collect and analyse both quantitative and qualitative data within the same study.1 2 Growth of mixed methods research in nursing and healthcare has occurred at a time of internationally increasing complexity in healthcare delivery. Mixed methods research draws on potential strengths of both qualitative and quantitative methods,3 ...
Mixed Methods Research. According to the National Institutes of Health, mixed methods strategically integrates or combines rigorous quantitative and qualitative research methods to draw on the strengths of each.Mixed method approaches allow researchers to use a diversity of methods, combining inductive and deductive thinking, and offsetting limitations of exclusively quantitative and ...
What is mixed methods research? In mixed methods research, you use both qualitative and quantitative data collection and analysis methods to answer your research question. Frequently asked questions: Methodology What is differential attrition? Attrition refers to participants leaving a study. It always happens to some extent—for example, in ...
Mixed methods research can be viewed as an approach which draws upon the strengths and perspectives of each method, recognising the existence and importance of the physical, natural world as well as the importance of reality and influence of human experience (Johnson and Onquegbuzie, 2004). Rather than continue these debates in this paper, we ...
Mixed methods research examples. Here is a real world mixed methods research example to help you better understand this research approach. In Newman, Shell, Ming, Jianping, and Mass' paper on adolescent alcohol use, the researchers used the mixed methods research approach to understand the characteristics of adolescents who drink and don't drink alcohol.
1 MMR Basics. Mixed methods research (MMR) is a hybrid model that brings together the numerical precision of quantitative research and the rich, contextual insights of qualitative research. In ...
Mixed methods research combines elements of quantitative research and qualitative research in order to answer your research question. Mixed methods can help you gain a more complete picture than a standalone quantitative or qualitative study, as it integrates benefits of both methods. Mixed methods research is often used in the behavioral ...
The research utilizes a mixed-methods approach, incorporating observations, geospatial mapping, and surveys to gather data. The findings from this study contribute to the broader understanding of how urban design and management strategies can shape conscious attitudes and behaviors towards environmental sustainability, ultimately supporting the ...
The partisanship and ideology of American voters. The partisan identification of registered voters is now evenly split between the two major parties: 49% of registered voters are Democrats or lean to the Democratic Party, and a nearly identical share - 48% - are Republicans or lean to the Republican Party. The partisan balance has tightened ...
Summary. Research suggests that the most effective leaders adapt their style to different circumstances — be it a change in setting, a shift in organizational dynamics, or a turn in the business ...
Climate change is hampering mountain pine beetle reproduction but also appears to slightly benefit the invasive insect in other ways, new University of Alberta research shows. The mixed scenario provides "a deeper understanding of dynamics that are crucial to building effective forest management and conservation strategies in the face of ...
The purpose classification of mixed research methods was first introduced in 1989 by Greene, Caracelli, and Graham, based on an analysis of published studies of mixed methods. This classification is still used and we have a total of five "purposes" for why a mixed methodology is used in research (4). Classification of the purpose of using ...
Join this informational webinar on April 17, 2024, from 1:00 p.m. to 2:00 p.m. ET, to learn more about the MACRO: Mixed Algae Conversion Research Opportunity and the application process. The U.S. Department of Energy's Bioenergy Technologies Office (BETO) and Office of Fossil Energy and Carbon Management (FECM) announced the MACRO funding opportunity announcement (FOA).
Analysts have been eager to weigh in on the Technology sector with new ratings on Salesforce (CRM - Research Report) and DocuSign (DOCU - Research Report).Salesforce (CRM) In a report released ...