- Utility Menu

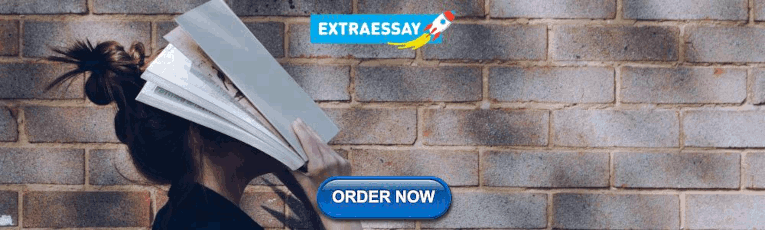
FAS Research Administration Services
Nsf data management plan.
NSF Guide and template for creating a data management plan
Filter by Policy Area
- Faculty Research Policies
- Effort Policies
- Research Compliance Policies
- Research Finances Policies
- Proposal Submission Policies
Filter by Policy Type
- FAS Policies
- University Policies
Create a research data management plan
All researchers at UWE Bristol must complete a research data management plan before collecting any data as part of research, or using any data for research.
A research data management plan is a document drawn up at the start of the research process. It outlines how all research data will be generated or collected, managed, stored and preserved, shared or disposed of. All researchers at UWE, including doctoral researchers, must complete a research data management plan before collecting any data as part of research, or using any data for research.
UWE Bristol research data management plan template
There is a specific research data management plan template for use by UWE Bristol researchers. Each question has accompanying guidance notes which can be accessed via hyperlinks from within the template itself, or can be viewed below.
An online training module on research data management to help you to complete your research data management plan is available on the My Learning platform for staff, and on the UWE Bristol Blackboard for students. Please note, the online module is mandatory for staff who conduct or supervise research. Please see the research governance pages on research data management for the most up-to-date information.
Access the UWE Bristol Research data management plan template (DOC) .
Note 1: What data will you collect, create or use?
Outline the types of research data that are expected to be created or collected.
Research data can be in any form, for example, electronic or hard copy, video, audio, artefacts, machine readouts. It can include survey/questionnaire data, consent forms, laboratory log books, videos of artistic performances, audio records of interviews and their transcripts, physical samples (including biological samples of animal or human origin), photographic images, environmental or habitat data, observational data (of humans or animals).
Please include:
- The source of the data - is the data coming from primary sources including data subjects, for example, survey participants, video recordings), being generated, or from a third party (such as secondary data)?
- The volume of data do you expect to generate.
- Who are the data subjects (for example survey participants or interviewees)? Are there, or might there be, any vulnerable subjects? These could include, but are not limited to, children, older people, patients, prisoners, asylum seekers, those who are physically or mentally unwell.
- How many data subjects will there be?
- What is the purpose of the data that is being collected?
- Are you creating or collecting personal, sensitive or special category data such as health, religion, race, sexual orientation, genetics and biometrics, relating to a living person or persons?
Note 2: How will you collect, create or access the data?
For primary data, briefly describe how the data will be collected or created.
- Will you be using recorded interviews, observation, physical measurement, taking samples, computer/instrument generated, survey, film etc.
- Outline any professional or community standards that will be adhered to.
- If you are generating digital survey data, what system will you use? Please note: Qualtrics is currently the only survey tool authorised by UWE Bristol.
If you are collaborating with any third party organisation or individual
- Outline any data handling agreements that are in place - such as data processing agreements, data sharing agreements or collaboration agreements which include data sharing/handling arrangements.
- If data is to be held by a third party describe when, where, and how the data will be held by the third party. Please note: personal data should not be moved outside of the European Economic area without safeguards being in place (please email The Data Protection Team at [email protected] for advice).
If you are using third party data (such as a secondary data source), say how this will be obtained
- Outline any restrictions on third party data use, or any access or use agreements that you/the University will need to sign. Please note: you should never sign anything without first consulting with Megan Wiggins in The Contracts Team at [email protected] , as you do not have authority to do so.
- Are you collecting or accessing data under restricted access conditions, for example within the NHS , ONS , or Criminal Justice System?
UWE Bristol Ethics process for human participants - Consent
Ethical approval MUST be granted by UWE Bristol's College or University Research Ethics Committee in respect of research and/or evaluations involving human participant data. Both legal and ethical dimensions of research data must be considered (please see note 7 in relation to Data Protection). In relation to personal data, the combined effect of ‘law’ and ‘ethics’ is generally that you may only do with data what you have told research participants you will do, and which you have gained consent for.
Please explain here briefly how you will:
- Please ensure that you consult the guidance on participant consent forms (DOC) . Will this consent enable future sharing and re-use? If this is not appropriate, and you do not propose to share and re-use, say so here.
- If you will not be gaining consent please set out your justification. Where professional standards may exist, for example in covert research, or in filming crowds, please set out any professional standards you will be adhering to.
- provide participant information Please see the guidance on participant information sheets (DOC) and the recommended Participant Privacy Notice (DOC) .
- handle personal, sensitive or other special category data.
- protect the interests and identity of any research participants - for example data anonymisation.
Note 3: Classify your data as public, restricted or confidential
See the Information Handling Policy (PDF) for definitions of data classifications.
Note 4: How will the data be stored and backed up at all stages of its lifecycle?
Please be clear how data will be stored at each stage of the research. You must comply with the Information Security Policy (PDF) and take account of the Information Security Toolkit .
You should take a "life course" approach to your data, being clear about the "data journey". For example, if you are interviewing human participants, taking samples, or collecting measurements or other data in the field, how will the data be stored at all stages of the research, how will it be securely transported, backed up, anonymised or pseudonymised, archived, securely disposed of?
Outline where data will be stored during the research
It is usually a requirement that research data is stored on a UWE Bristol networked drive (S or H), or on UWE Bristol OneDrive. If this is not possible, for example in the case of very large datasets, or situations where you will not have network access, you should consult the IT Service Desk for advice.
Please note: the only Cloud provision which is acceptable for use is UWE Bristol OneDrive, not Dropbox or any other provision.
How will data be backed up?
- Who is responsible for conducting data back up, and version control?
- How frequently will data be backed up?
- Is sufficient storage available via UWE Bristol systems, or do you need to consider additional storage needs and associated costs?
See UWE Bristol's data storage pages (intranet only) for information.
Note 5: How will the data be documented, described and maintained?
How will you manage file versioning during the course of the research.
Is there a standard that will be used?
Note what file format(s) you intend to use to store data
Where possible it is better to use non-proprietary file formats that are in widespread use to ensure on-going readability of data.
The UK Data Archive has a list of file formats which are in common use.
Outline how you will record information about your data
This is so that you, and others where appropriate, can access and use the data. This might be during the course of the research, or afterwards. Say where this information will be held, for example within the data itself, in a readme.txt file, in a database, or using another system.
As a minimum, you should record date, name and purpose of the data, the creator, details of how the data was created/analysed, explanations of any codes or abbreviations used.
For further information, see the Research Data Management guidance on documenting data .
Note 6: How will your data be processed?
Please describe here what you will actually do with the data, throughout its life course.
Set out the data security measures that you will take in relation to the way you will use the data. Data security measures should be appropriate to the data involved.
UK Data Protection legislation requires that personal data will be processed in a manner that ensures appropriate security including protection against unlawful processing, accidental loss, destruction or damage (also see Note 7 ). However, it is not just personal data; other kinds of data will also need careful handling, for example security sensitive research, commercially sensitive data, data subject to export controls, or environmental information (for example, in relation to rare or endangered populations of animals).
- Will any third party processing of data take place? If so, by whom and for what purpose? (for example, use of a UWE Bristol approved transcription service)
- How will you ensure only those who have a legitimate right to access the data can do so?
- By what mechanisms will data be shared between research partners?
- If you are generating data in the field, how will you ensure its safe transfer to UWE Bristol secure systems?
Note 7: Does the Data Protection Act (2018) apply to your research?
Collecting personal data and complying with data protection.
The General Data Protection Regulation (GDPR), implemented in the UK by the Data Protection Act (2018), states that there must be lawfulness, fairness and transparency in relation to any data subjects. Use this section to consider how you will ensure that this data principle is upheld. Please refer to the Data Protection Research Standard (intranet only) for information.
If you are working with human participants
- Outline how you will ensure you comply with the principles of accuracy and minimisation of data collected.
- Outline how you will ensure that data is kept in a form which permits identification for no longer than is necessary (storage limitation under GDPR ). Where data needs to be kept in this form for a longer period of time (for example, clinical trials data) please justify that here. Consult the Data Protection Research Standard (intranet only).
- Please set out here whether you have conducted a Data Protection Impact Assessment (DPIA) (staff intranet), and any necessary subsequent action. If you do not need to conduct a DPIA , please justify this here.
Note 8: Export controls and other legislation and regulation
- Please see Export Controls Regulations Guidance .
- If your research data may have a “dual use” or potentially be used in weapons of mass destruction (such as certain materials or algorithms) then you must set out here how you will ensure that you comply with the relevant regulations. Please note: the consequences of a breach of Export Controls can be extremely serious, so if you are uncertain, seek guidance from your College Management.
- In relation to other legislation and regulations, are there any specific implications for your research data? If so, please set them out here.
Note 9: What Intellectual Property will be collected or used in this research?
Who owns the ipr of any data collected or created.
- In the case of partnership working, is this covered by a collaboration agreement?
- In the case of derived products (including derived datasets), who owns, controls and has permission to use these?
- Are the measures you have set in place in relation to the research data appropriate to protect IP and not infringe third party IP?
Refer to The UWE Bristol Policy on Intellectual Property (PDF) for information.
Note 10: What are your plans for long-term preservation and data sharing, where appropriate, and data disposal?
Researchers are encouraged to think about preserving their data and, where appropriate, making it available for sharing and re-use. Some funders and publishers have specific requirements relating to data retention.
Please explain here:
- How you will decide which datasets to keep beyond the end of the research. Include the reasons for your decisions and be clear how this relates to participant consent if you are working with human participants.
- Are you using a schedule from a funder/publisher?
- Outline if there is any data which must be securely destroyed during, or at the end of, the research and how this will be achieved.
- Where any data to be preserved will be deposited. For example, in an established discipline specific, or other, national data repository, or in UWE Bristol's data repository.
- If you do not plan to use a data repository, explain how your data will curated and preserved beyond the end of the research, or why and how it will be securely and safely disposed of.
- If and how you intend to share your research data for re-use. If appropriate, note how participant consent allows for this.
- Note any access limits to the data. For example, does your data contain personal information about research participants that requires access controls, or are you working with an external collaborator?
- Will there be an embargo period?
- Do you have a funder that has a specific data release schedule?
If it is not appropriate to share your data, state why here.
For further information, see guidance on Preserving, Sharing and Disposing of Research Data .
Outline the proposed arrangements if any member of the research team leaves the University
Please note: no student or staff member is permitted to remove data from the University without the permission of the data owner. This is the Pro-Vice Chancellor Research and Business Engagement, delegated to College Deans. This permission will only be granted where it is consistent with the University’s interests and legal and ethical obligations.
Note 11: Who is responsible for enacting the different elements of the research data management plan?
The UWE Bristol project manager has overall responsibility for enacting the research data management plan, but others such as other UWE Bristol research team members, external collaborators, or other third parties will have a role to play. Please set this out here:
- What are the different roles within your research team - at UWE Bristol and/or external collaborators, in relation to the data?
- Do others have a role in relation to research data - for example, data gatekeepers, archivists, personnel in other organisations who are not part of the research team.
- Have formal agreements been set in place by the Contracts team - as appropriate, with research funders, collaborators or other partners?
- What are the different roles within your research team in relation to the data?
- How these roles, including the UWE project manager, will be fulfilled in the case of a significant period of unanticipated absence (such as sickness leave, or a member of staff leaving at short notice).
Note 12: What resources are needed to deliver the plan, and are these available?
Outline any resources you will need to fulfil your plan.
Consider, for example:
- equipment needs - such as an encrypted UWE Bristol laptop or recording device
- long-term storage provision - including resource to pay for it
- transcription costs
- staff time - to ensure data is properly handled
- encrypted additional hard drive or other additional storage
- training or additional staff expertise
- preservation costs - including time for preparation for archiving
- costs associated with any third party data.
See the UK Data Service guidance on costing data management for further guidance.
Funder Requirements
Many research funders also have requirements regarding the submission of a research data management plan. If you are externally funded, find out about your funder's requirements .
The University of Bristol has shared examples of data plans submitted to a range of funders. The University of Leeds has shared two examples of examples of ESRC data plans .
UK Data Archive's managing and sharing data guide provides information to assist researchers in sharing data.
Getting help with submitting a grant application
For help with submitting a data management plan as part of a research funding bid, please contact the Research and Knowledge Exchange Team .
MIT Libraries logo MIT Libraries
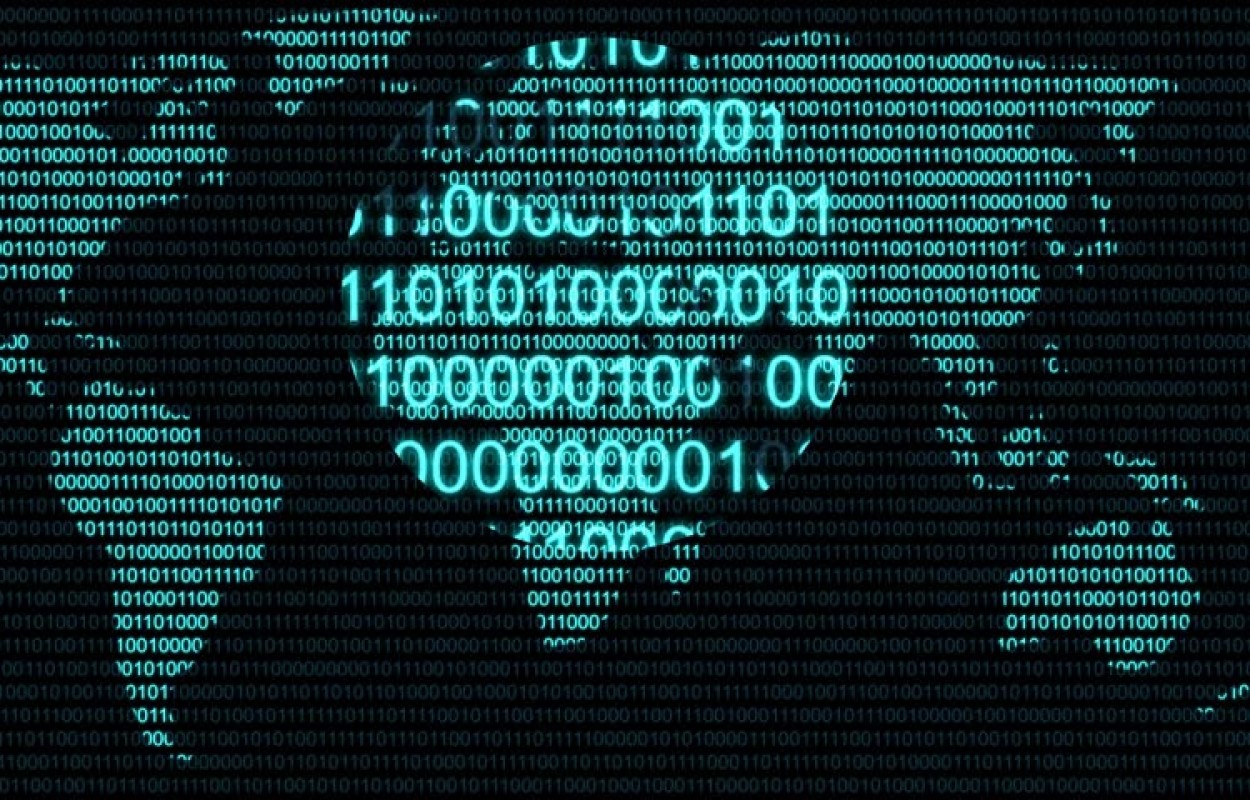
Write a data management plan
A data management plan (DMP) will help you manage your data, meet funder requirements, and help others use your data if shared.
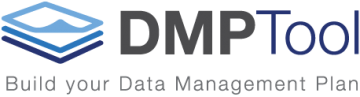
Alternatively, you can use the questions below and any specific data management requirements from your funding agency to write your data management plan. Additional resources for creating plans are also provided below.
- What’s the purpose of the research?
- What is the data? How and in what format will the data be collected? Is it numerical data, image data, text sequences, or modeling data?
- How much data will be generated for this research?
- How long will the data be collected and how often will it change?
- Are you using data that someone else produced? If so, where is it from?
- Who is responsible for managing the data? Who will ensure that the data management plan is carried out?
- What documentation will you be creating in order to make the data understandable by other researchers?
- Are you using metadata that is standard to your field? How will the metadata be managed and stored?
- What file formats will be used? Do these formats conform to an open standard and/or are they proprietary?
- Are you using a file format that is standard to your field? If not, how will you document the alternative you are using?
- What directory and file naming convention will be used?
- What are your local storage and backup procedures ? Will this data require secure storage?
- What tools or software are required to read or view the data?
- Who has the right to manage this data? Is it the responsibility of the PI, student, lab, MIT, or funding agency?
- What data will be shared , when, and how?
- Does sharing the data raise privacy, ethical, or confidentiality concerns ? Do you have a plan to protect or anonymize data, if needed?
- Who holds intellectual property rights for the data and other information created by the project? Will any copyrighted or licensed material be used? Do you have permission to use/disseminate this material?
- Are there any patent- or technology-licensing-related restrictions on data sharing associated with this grant? The Technology Licensing Office (TLO) can provide this information.
- Will this research be published in a journal that requires the underlying data to accompany articles?
- Will there be any embargoes on the data?
- Will you permit re-use , redistribution, or the creation of new tools, services, data sets, or products (derivatives)? Will commercial use be allowed?
- How will you be archiving the data? Will you be storing it in an archive or repository for long-term access? If not, how will you preserve access to the data?
- Is a discipline-specific repository available? If not, consider depositing your data into a generalist data repository . Email us at [email protected] if you’re interested in discussing repository options for your data.
- How will you prepare data for preservation or data sharing? Will the data need to be anonymized or converted to more stable file formats?
- Are software or tools needed to use the data? Will these be archived?
- How long should the data be retained? 3-5 years, 10 years, or forever?
Additional resources for creating plans
- Managing your data – Project Start & End Checklists (MIT Data Management Services) : Checklist (PDF) with detailed resources to help researchers set up and maintain robust data management practices for the full life of a project.
- ezDMP : a free web-based tool for creating DMPs specific to a subset of NSF funding requirements.
- Guidelines for Effective Data Management Plans and Data Management Plan Resources and Examples (ICPSR) : Framework for creating a plan and links to examples of data management plans in various scientific disciplines
- Example Plans (University of Minnesota)
- NSF (by the DART project) : assessment rubric and guidance
- NIH (by FASEB)
See other guides to data management for additional guidance on managing data and select information related to particular formats or disciplines.
What is DMPTool?
With DMPTool you can create and share data management plans. It provides step-by-step guidance for creating your own DMP, including templates and sample plans to help you address requirements specific to Harvard and your funding sources.
Using DMPTool at Harvard
Harvard is a DMPTool partner institution. Log in using your Harvard credentials.
- Go to DMPTool .
- Enter your Harvard email address in the "Sign in / Sign up" box and click "Continue"
- After you see the prompt "Your address is associated with: Harvard University (harvard.edu)," click "Sign in with Institution to Continue"
- Enter your Harvard Key credentials and click "Sign In"
Have questions about DMPTool or need a data management plan review?
WHAT IS A DATA MANAGEMENT PLAN?
A data management plan, or DMP (sometimes also called a data sharing plan), is a formal document that outlines what you will do with your data during and after a research project. Most researchers collect data with some form of plan in mind, but it's often inadequately documented and incomplete. Many data management issues can be handled easily or avoided entirely by planning ahead. With the right process and framework it doesn't take too long and can pay off enormously in the long run.
Many funding agencies, especially government funding sources, require a DMP as part of their application processes. Even if you are not seeking funding for your research, documenting a plan for your data is a best practice and will help your data comply with Harvard's policies for responsible data management. If your DMP provides for your data to be openly shared, the data necessary for external replication of your research findings will be available to the research community for the long term.
What do I include in a DMP?
Information contained in a data management plan describes your plan for addressing many aspects of working with data. A DMP doesn't need to be long. It should include things like:
- Types of data: What is the source of your data? In what formats are your data? Will your data be fixed or will it change over time? How much data will your project produce?
- Contextual details (metadata): How will you document and describe your data?
- Storage, backup and security: How and where will you store and secure your data?
- Provisions for protection/privacy: What privacy and confidentiality issues must you address?
- Policies for re-use: How may other researchers use your data?
- Access and sharing: How will you provide access to your data by other researchers? How will others discover your data?
- Archiving and providing access: What are your plans for preserving the data and providing long-term access?
View Sample Data Management Plans
Harvard DMPTool Templates
There are two templates available on the DMPTool that provide Harvard specific guidance, resources, and policies to help you create a relevant and useful data management plan for grants or other research projects.
Harvard University General DMP Template
This template provides guidance so you can create a data management plan. It uses the new NIH Data Sharing and Management structure but can be used for a variety of research types.
View the General template
Harvard Medical School Biomedical DMP Template
This DMPTool template offers organization-specific guidance for Harvard Medical School constituents. This is a funder agnostic template and can be used for a variety of grant types.
View the HMS template
Related Services & Tools
Research data services, harvard dataverse.
- University of Michigan Library
- Research Guides
Data Management Plans (ENG)
- Templates & Examples
- Funder Mandates
- Roles & Responsibilities
- Expected Data
- Dissemination
- Storage & Preservation
Tools for Writing DMPs
The following Microsoft Word documents are borrowed from DMPTool.org . DMPTool.org is created by a group of major research institutions to help researchers generate data management plans. These documents display the current (as of the date shown on the document) funding agency DMP guidance, followed by a listing of points from DMPTool on topics to consider when writing the relevant portion of your DMP.
- NSF-ENG Template (Word Document) DMP Template for the NSF Directorate for Engineering, from DMPTool.org
- NSF-BIO Template (Word Document) DMP Template for the NSF Directorate for Biological Sciences, from DMPTool.org
- NSF-CISE Template (Word Document) DMP Template for the NSF Directorate for Computer & Information Science & Engineering, from DMPTool.org
- NSF-AGS Template (Word Document) DMP Template for the NSF Division of Atmospheric & Geospace Sciences, from DMPTool.org
- NSF-EAR Template (Word Document) DMP Template for the NSF Division of Earth Sciences, from DMPTool.org
- NSF-AST Template (Word Document) DMP Template for the NSF Division of Astronomical Sciences, from DMPTool.org
- NSF-CHE Template DMP Template for the NSF Division of Chemistry, from DMPTool.org
- NSF-DMR Template (Word Document) DMP Template for the NSF Division of Materials Research, from DMPTool.org
- NSF-PHY Template (Word Document) DMP Template for the NSF Division of Physics, from DMPTool.org
- DoE Template (World Document) DMP template for the Department of Energy, from DMPTool.org
- DoE Template (Word Document) DMP Template for the Department of Energy, from DMPTool.org
- DoE Office of Science Template (Word Document) DMP Template for the Department of Energy, Office of Science, from DMPTool.org
- DoE-EERE Template (Word Document) DMP Template for the Department of Energy, Office of Energy Efficiency and Renewable Energy, from DMPTool.org
- DOD Template (Word Document) DMP template for the Department of Defense, from DMPTool.org
- NASA Template (Word Document) DMP Template for NASA, from DMPTool.org
- DOT Template (Word Document) DMP Template for the Department of Transportation, from DMPTool.org
Other DMP Templates
These are links to templates created for researchers at other colleges & universities.
- University of New Mexico Examples
- University of Illinois - Grainger Engineering Library Information Center
- ICPSR Natural Science Examples
- DataONE Examples
- Interlibrary Loan and Scan & Deliver
- Course Reserves
- Purchase Request
- Collection Development & Maintenance
- Current Negotiations
- Ask a Librarian
- Instructor Support
- Library How-To
- Research Guides
- Research Support
- Study Rooms
- Research Rooms
- Partner Spaces
- Loanable Equipment
- Print, Scan, Copy
- 3D Printers
- Poster Printing
- OSULP Leadership
- Strategic Plan
Data Management Plans
- Federal Access Mandates
General Data Management Plan Template
- NSF directorate-specific info
- NIH Data Sharing Plans
SEND US AN EMAIL
- L.K. Borland Data Management Support Coordinator Schedule an appointment with me!
- Diana Castillo College of Business/Social Sciences Data Librarian Assistant Professor 541-737-9494
- Clara Llebot Lorente Data Management Specialist Assistant Professor 541-737-1192 On sabbatical through June 2024
Templates in DMPTool
DMPTool maintains a collection of public templates based on the specific requirements listed in funder policy documents, and a list of links to funder requirements.

This general template for a data management plan is based on general NSF guidelines. BE SURE TO CHECK YOUR CALL FOR PROPOSALS AND WITH YOUR DIRECTORATE FOR MORE SPECIFIC DMP CONTENT REQUIREMENTS. In cases where the expected content of a DMP is not specified by your funding body, using this template should enable you to cover all of the appropriate material. This material is largely based on the DMPTool template and the Digital Curation Centre guidance ; we strongly encourage you to use DMPtool because it keeps all of the funder requirements up to date. The DMPTool allows you to create, maintain and archive your DMPs in one location.
1. Types of data & materials produced
Give a short description of the data and materials collected and created, and amounts (if known).
- Description of data to be produced (experimental, observational, raw or derived, physical collections, models, images, etc.)
- How data will be acquired (When? Where? Methods?)
- How data will be processed (software used, algorithms, workflows, protocols)
- File types and formats (CSV, tab-delimited, proprietary formats, naming conventions)
- How much data will be generated? (range is OK)
- Existing data: if existing data are used, what are their origins? Will your data be combined with existing data? What is the relationship between your and existing data?
- Information about data types and formats in the Research Data Services website .
2. Documentation and Metadata
Metadata includes all of the contextual information that would be necessary for someone to understand, use or recreate your dataset (even many years from now). It may include methodological information, definition of variables, units, assumptions made, etc... Metadata can take many forms, from readme files manually created, to automatically generated metadata by sensors, to metadata recorded in a metadata standard. Recording part of the metadata in normalized schemas and metadata standards will enhance discoverability and make your metadata computer friendly.
- Any details that make data understandable and useable
- Lab notebooks? GPS units? Auto-saved on instrument? Manually entered?
- Standards for community (EML, ISO 19115, Dublin Core, etc... .
- Justification for format chosen
TIPS :
- Information about data documentation and metadata in the Research Data Services website .
- When possible use data standards and/or metadata standards.
- A list of research metadata standards compiled by the Research Data Alliance can be found here .
- If you chose not to use a metadata standard, consider structuring metadata in the research project with your own template.
3. Ethics and Intellectual Property
If you are doing Human Subjects research or working with other types of sensitive data explain how you are going to manage the data to protect the identity of the participants if required.
- How will you store the data?
- Who will get access to the data?
- How will you gain consent for preserving and sharing the data?
- Explain how the research project will get an ethical review.
Are there any intellectual property issues that need to be addressed? If you are going to use a third party dataset, are there any restrictions that should be considered?
- Information about Intellectual Property and datasets in the Research Data Services website .
- OSU Data Management, Classification and Incident Response Policy defines three data categories: unrestricted, sensitive and confidential . The policy outlines baselines of care.
- Data Management Plans are written before IRB approval , so you may not know all the details about how your sensitive data will need to be managed. Having plans for data sharing before getting IRB approval may help you to write your consent forms taking your data sharing goals into consideration.
4. Storage and backup
Describe how the data will be stored.
Describe how the data will be backed up.
- Which locations
- Automatic or manual
- Who will be responsible
- How will the data be recovered in the event of an accident
- Information about storage and backups in the Research Data Services website .
- Oregon State University offers several cloud storage options .
- Data storage options for diferent clasifications of data at Oregon State University can be found at the OSU Data Management, Classification and Incident Response Policy .
5. Policies for Access and Sharing
- How will potential users find out about your data?
- When will you make the data available?
- How will you make the data available? (include resources needed to make the data available: equipment, systems, expertise, etc.)
- What is the process for gaining access to the data? (by request, open-access repository, etc.)
- Will access be chargeable?
- Will you be using persistent identifiers (e.g. DOI) for your datasets?
- Will a data sharing agreement or similar be required to share the dataset?
Explain how the policies & procedures you outlined above relate to the reuse and redistribution of your data. Identify who will be allowed to use your data, how they will be allowed to use it, and if they will be allowed to disseminate your data.
- Will any permission restrictions need to be placed on the data? If you will restrict access to certain users explain why.
- Are you going to attach any licenses to the dataset?
- Information about data sharing in the Research Data Services website .
- You are welcome to share your research data in OSU's institutional repository, ScholarsArchive@OSU .
ScholarsArchive@OSU is a digital repository that promises preservation, discoverability, and persistency of digital objects and is supported by the OSU Libraries & Press. All datasets deposited to ScholarsArchive@OSU will be assigned DOIs, and metadata for discoverability, usability, and administrative needs of the data.
A list of repositories can be found in re3data.org . Make sure that you evaluate the reliability of a repository first, if you choose it from this website without knowing much about it.
6. Plans for archiving data, samples, and other research products, and for preservation of access to them This section should cover your long-term strategy for preserving the data produced during your project. See section on data archiving .
- What data will be preserved for the long-term?
- What is the long-term strategy for maintaining, curating and archiving the data?
- Which archive/repository/database have you identified as a place to deposit data?
- What procedures does your intended long-term data storage facility have in place for preservation and backup?
- How long will/should data be kept beyond the life of the project?
- What transformations will be necessary to prepare data for preservation / data sharing?
- What metadata/ documentation will be submitted alongside the data or created on deposit/ transformation in order to make the data reusable?
- What related information will be deposited?
- Information about data archival and preservation in the Research Data Services website .
- You are welcome to preserve your research data in OSU's institutional repository, ScholarsArchive@OSU .
ScholarsArchive@OSU is a digital repository that promises preservation, discoverability, and persistency of digital objects and is supported by the OSU Libraries & Press. All datasets deposited to ScholarsArchive@OSU will be assigned DOIs, and metadata for discoverability, usability, and administrative needs of the data.
Sometimes the sharing and preservation section of a Data Management Plan overlap if you are using a repository as both a sharing and a preservation strategy.
7. Roles and responsibilities
Who will be responsible for making sure that the Data Management Plan will be implemented? Who will perform each of the data management tasks (data collection, data assurance and quality control, data documentation, data archiving, etc.). What are the procedures in place that ensure that if one member of the team leaves the project data management tasks will still be performed?
8. Budget You are encouraged to ask for funding support for the management and preservation of your data. Consider not only hardware costs, but also the personnel time needed to maintain them.
- Time for data preparation and documentation
- << Previous: Federal Access Mandates
- Next: NSF directorate-specific info >>
- Last Updated: Aug 30, 2023 9:25 AM
- URL: https://guides.library.oregonstate.edu/dmp
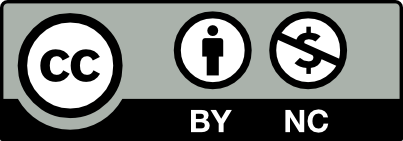
Contact Info
121 The Valley Library Corvallis OR 97331–4501
Phone: 541-737-3331
Services for Persons with Disabilities
In the Valley Library
- Oregon State University Press
- Special Collections and Archives Research Center
- Undergrad Research & Writing Studio
- Graduate Student Commons
- Tutoring Services
- Northwest Art Collection
Digital Projects
- Oregon Explorer
- Oregon Digital
- ScholarsArchive@OSU
- Digital Publishing Initiatives
- Atlas of the Pacific Northwest
- Marilyn Potts Guin Library  
- Cascades Campus Library
- McDowell Library of Vet Medicine

Ask Yale Library
My Library Accounts
Find, Request, and Use
Help and Research Support
Visit and Study
Explore Collections
Research Data Management: Plan for Data
- Plan for Data
- Organize & Document Data
- Store & Secure Data
- Validate Data
- Share & Re-use Data
- Data Use Agreements
- Research Data Policies
What is a Data Management Plan?
Data management plans (DMPs) are documents that outline how data will be collected , stored , secured , analyzed , disseminated , and preserved over the lifecycle of a research project. They are typically created in the early stages of a project, and they are typically short documents that may evolve over time. Increasingly, they are required by funders and institutions alike, and they are a recommended best practice in research data management.
Tab through this guide to consider each stage of the research data management process, and each correlated section of a data management plan.
Tools for Data Management Planning
DMPTool is a collaborative effort between several universities to streamline the data management planning process.
The DMPTool supports the majority of federal and many non-profit and private funding agencies that require data management plans as part of a grant proposal application. ( View the list of supported organizations and corresponding templates.) If the funder you're applying to isn't listed or you just want to create one as good practice, there is an option for a generic plan.
Key features:
Data management plan templates from most major funders
Guided creation of a data management plan with click-throughs and helpful questions and examples
Access to public plans , to review ahead of creating your own
Ability to share plans with collaborators as well as copy and reuse existing plans
How to get started:
Log in with your yale.edu email to be directed to a NetID sign-in, and review the quick start guide .
Research Data Lifecycle
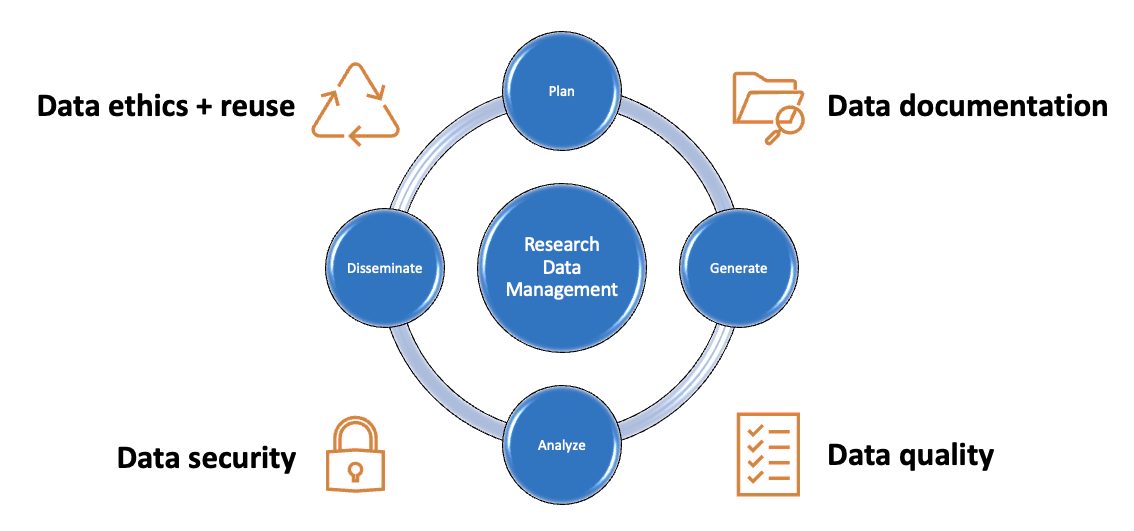
Additional Resources for Data Management Planning
- << Previous: Overview
- Next: Organize & Document Data >>
- Last Updated: Sep 27, 2023 1:15 PM
- URL: https://guides.library.yale.edu/datamanagement

Site Navigation
P.O. BOX 208240 New Haven, CT 06250-8240 (203) 432-1775
Yale's Libraries
Bass Library
Beinecke Rare Book and Manuscript Library
Classics Library
Cushing/Whitney Medical Library
Divinity Library
East Asia Library
Gilmore Music Library
Haas Family Arts Library
Lewis Walpole Library
Lillian Goldman Law Library
Marx Science and Social Science Library
Sterling Memorial Library
Yale Center for British Art
SUBSCRIBE TO OUR NEWSLETTER
@YALELIBRARY

Yale Library Instagram
Accessibility Diversity, Equity, and Inclusion Giving Privacy and Data Use Contact Our Web Team
© 2022 Yale University Library • All Rights Reserved
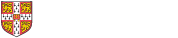
Study at Cambridge
About the university, research at cambridge.
- Undergraduate courses
- Events and open days
- Fees and finance
- Postgraduate courses
- How to apply
- Postgraduate events
- Fees and funding
- International students
- Continuing education
- Executive and professional education
- Courses in education
- How the University and Colleges work
- Term dates and calendars
- Visiting the University
- Annual reports
- Equality and diversity
- A global university
- Public engagement
- Give to Cambridge
- For Cambridge students
- For our researchers
- Business and enterprise
- Colleges & departments
- Email & phone search
- Museums & collections
- Data Management Guide
- Creating your data

- Data Management Guide overview
- Creating your data overview
- Organising your data
- Accessing your data overview
- Looking after your data
- Sharing your data
- Choosing a software licence
- Electronic Research Notebooks overview
- Support overview
- Resources and support at Cambridge overview
- Data Management Plan Support Service
- DMP Pilot for PhDs
- External support
- Data Repository overview
- Upload your data
- Depositor's checklist
- Guidance on the data submission process
- Data Policies overview
- University of Cambridge Research Data Management Policy Framework overview
- Cambridge data-related policies
- Funders Policies overview
- News overview
- Data Champions overview
- Data Champion list
- Data Champion Community
- Data Champions Cartoons
- Alumni Data Champions
- 2024 Call for Data Champions
- Events overview
- Love Data Week 2024
- Past Events
- Contact Us overview
- Our Governance
- Request a Meeting
- Data Management Plan
- Research Data Management
- Choosing Formats
- Intellectual Property Rights
- Data Protection and Ethics
- Accessing your data
- Electronic Research Notebooks

Crafting your data management plan
Most research funders encourage researchers to think about their research data management activities from the beginning of the project. This will often mean a formal plan for managing data (a 'data management plan').
However, even informally setting out your plans and project guidelines can make your life much easier. If you want to be able to reuse your data or manage collaboration with colleagues, it helps to plan for that from the beginning. Decisions you make about which software to use, how to organise, store and manage your data, and the consent agreements you would have to negotiate, will all affect what is possible to do, and what data is shareable in the future.
Planning ahead for your data management needs and activities will help ensure that:
- you have adequate technological resources (e.g. storage space, support staff time)
- your data will be robust and free from versioning errors and gaps in documentation
- your data is backed up and safe from sudden loss or corruption
- you can meet legal and ethical requirements
- you are able to share your finalised data publicly, if you and/or your funder desires
- your data will remain accessible and comprehensible in the near, middle, and distant future.
What do research funders expect?
Most funders expect you to prepare a data management plan when applying for a research grant. Additionally, some funders, for example the Medical Research Council ( MRC ), will require you to regularly review your data management plan and make all necessary amendments while managing your grant. The Economic and Social Research Council (ESRC) provides comprehensive guidelines on how treating personal and sensitive data, as well as on obtaining consent for data collection from participants. The information on funder requirements is available here .
Where do I start?
Much of research data management is simply good research practice so you will already be some way down the line. Data plans are just a way of ensuring (and/or showing) that you have thought about how to create, store, backup, share and preserve your data.
The Digital Curation Centre ( DCC ) has produced an interactive online tool to help researchers create data management plans: DMPOnline . The website records all major UK/European funder requirements, and it automatically tailors the data management plan template to the needs of your funder. You can log in to DMPonline using your Raven account (to do this, simply select the University of Cambridge as your institution, and you will be re-directed to the Raven log-in interface). Data plans that you create are easily exportable to a desired file type (Word, Excel, pdf), so you can simply add them to your grant applications.
What should I include in my data plan?
The best way to start is to look for what your funder expects you to cover in your Data Management Plan. You can either check this on your funder's website or by using the DMPonline tool, which is populated with funder's template and will guide you through your funder's requirements.
Who can help with data planning at the University of Cambridge?
The University has a range of support staff who can help you create a data management plan, including:
- your departmental or college IT staff
- subject and departmental librarians
- your funder - some funders, for example, the Economic and Social Research Council (ESRC), offer support in preparation of data management plans
No matter who you ask for support, please get in touch early, so there is enough time for support staff to help.
Simple data management plan template
Have a look at our simple data management plan template here - if your funder does not provide guidance on data plans, this might be a good starting point.
Related links
- DMPonline - tool to create data management plans
- ESRC - support for data management plans
About this site :
This site is managed by the Research Data Team.
If you have any questions about this site, please e-mail us directly
The project is a joint initiative of Cambridge University Library and the Research Operations Office .
Privacy policy
© 2024 University of Cambridge
- Contact the University
- Accessibility
- Freedom of information
- Privacy policy and cookies
- Statement on Modern Slavery
- Terms and conditions
- University A-Z
- Undergraduate
- Postgraduate
- Research news
- About research at Cambridge
- Spotlight on...
- Current Students
- Bookable Spaces
- Click & Collect
- Book a Group Study Room
- Research Reserve
- Can't find your Library resources?
- Recommend a book not held by the Library
- Inter-Library Loans
- Academic staff - Recommend a journal or resource
- Databases, Journals and Reading Lists
- Faculty of Medical Sciences
- Faculty of Humanities and Social Sciences
- Faculty of Science, Agriculture and Engineering
- Resource Guides
- New Library Resources
- Academic Skills Kit
- Enhance Your Skills
- Self-enrol courses
- FMS Peer Mentors
- Science, Agriculture and Engineering Peer Mentors
- HASS Peer Mentors
- Working in Partnership
- Be Well@NCL
- Study Well@NCL
- Funder DMP Requirements
- Guide to DMPOnline
- Handling of Personal and Sensitive Data
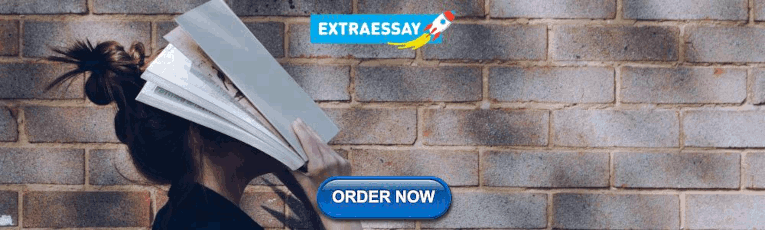
Guide to data.ncl
- Data Access Statement
- Data Selection
- Preserving and Publishing Data
- data.ncl Reviewing Guidelines
- REF OA Status
- UKRI Policy: Research Articles
- UKRI Policy: Long-form Publications
- Wellcome Trust Policy
- CRUK Policy
- Breast Cancer Now Policy
- Horizon 2020 Policy
- NIHR Policy
- Versus Arthritis
- Parkinson's UK Policy
- Blood Cancer UK Policy
- Apply for long-form publications
- Apply for funds
- Publisher Deals
- Research Publications & Copyright Policy
- Research metrics
- Google Scholar
- Social Media
- Copyright in Publications
- Copyright in Research Data
- Copyright in Theses
- Systematic Reviews
- Case Studies
- UKRN Open Research Programme
- UKRN Newcastle
- ReproducibiliTea
- Open Research Awards
- Scanning and CLA requests
- Digital and Information Skills
- Academic and Study Skills
- Special Collections and Archives
- Request Embedded Teaching
- Resources for teaching
- How we support students
- Research Data Management Training
- Open Access Training
- Information Skills & Using Research Materials
- Academic Skills Development
- Managing Information Training
- Systematic Reviews Development & Support
- Explore our Collections Guide
- Search our Collections
- Plan Your Visit
- Virtual Reading Room Service
- Digitisation Service
- Copyright, Acknowledgement and Re-use
- Teaching using Special Collections
- Cabinet of Curiosities
- Engagement using Special Collections
- Discover our collections
- Past Exhibitions
- Peter Bennet
- Sean O'Brien Archive
- Michael Chaplin on the Chaplin (Michael) Archive
- Peter Lewis & Margaret Lewis on Flambard Archive
- Peter Mortimer on the IRON Press Archive
- Michael Chaplin on the Chaplin (Sid) Archive
- Selima Hill
- Universities at War
- Petre’s Gradual
- Pybus Operating Theatre Logbook
- Trevelyan Family Albums
- The Bloodaxe Archive
- Gertrude Bell Online
- The Courier Archive
- Brian Alderson Online
- Special Collections Blog
- Virtual Walking Tour of Newcastle University
- Joining the Library
- Sixth Form Study Skills
- Sixth Form Study Skills workshops
- Archives Alive workshops
- Impact report
- Our History
- News & Events
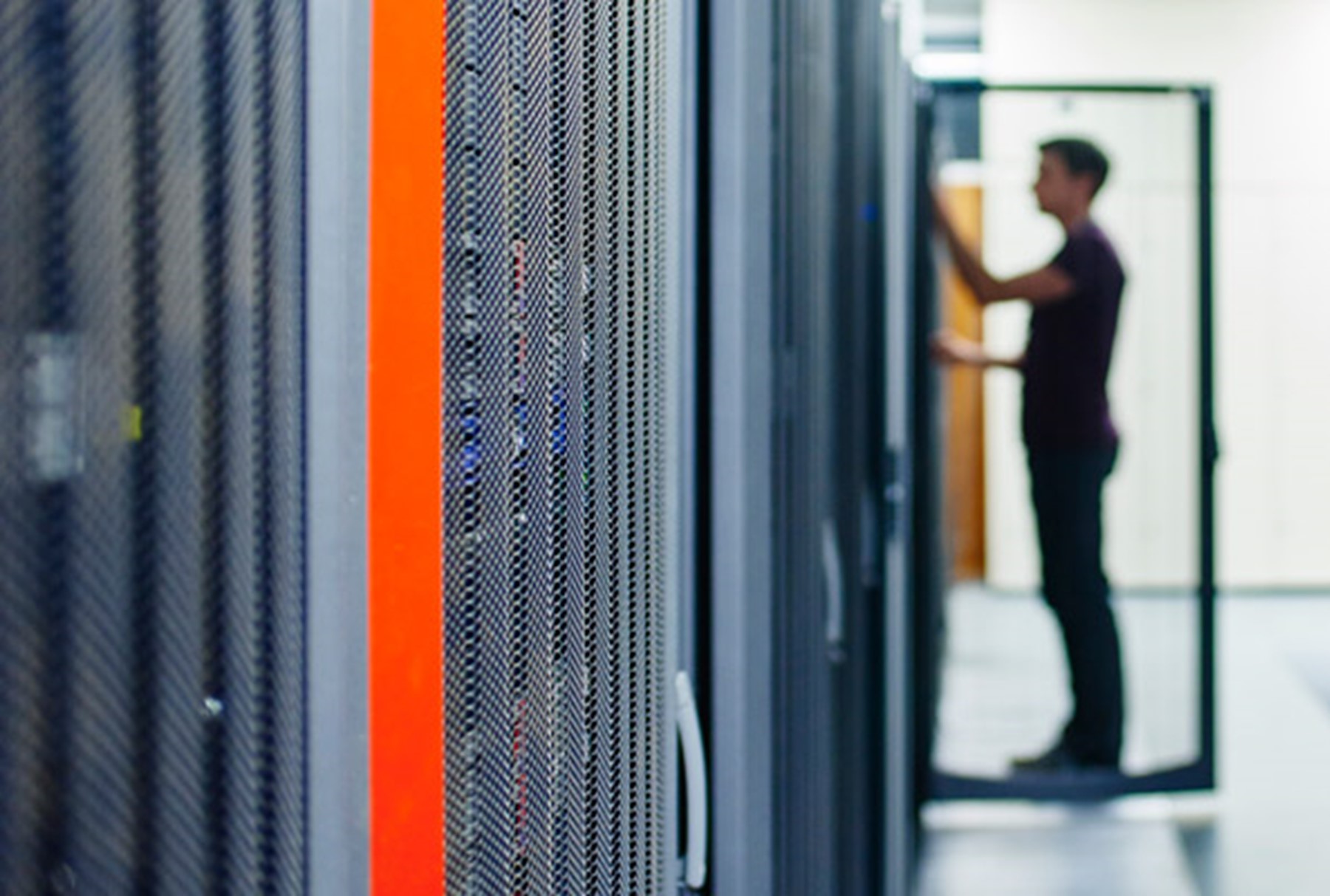
- Writing a Data Management Plan
Plan how you will organise, store, use and share your data throughout the research cycle.
- Newcastle University
- University Library
- Academics & Researchers
- Research Services
- Research Data Management
Data Management Plans (DMPs) generally require a researcher to describe the research data they will create, how the data will be stored and managed during the project, and how valuable data will be shared. The resulting plan will have short and long-term benefits:
- find and understand your data when needed
- avoid unnecessary duplication of data
- document your data to ensure access and continuity within your project’s team
- if required, allows for the validation of your published results
- ensure your research is visible and has impact
This website is designed to help you draft your Data Management Plan and we would advise that you look at these sections in particular.
Finding and reusing data
Explore data repositories and consider the ethical reuse of data.
Active data storage
Discover the options available to store active data.
Collaboration
Tools available to enable data sharing and collaboration in research teams.
Organise your data
Guidance for naming your files, file formats and documenting your data so that it is more retrievable.
Data sharing
How will you share your data?
Instructions for storing, sharing and managing your data with data.ncl.
Writing your plan
DMPonline is a flexible web-based tool to assist the creation of DMPs. It provides templates and guidelines for the majority of research funders.
The Research Data Service (RDS) can review draft DMPs making sure it covers all the key areas. Many funders make stipulations as to where and for how long data must be made available following the completion of a project. Knowledge of funder codes of practice and expectations upfront will benefit this process.
Email your draft DMP to the Research Data Service Team . To help provide the context to your research, please also send a draft case of support.
We aim to review DMPs as soon as possible, please let us know if you have a tight deadline.
Data Management Plan training
Explore training offered by the Research Data Service on creating data management plans for specific funders.
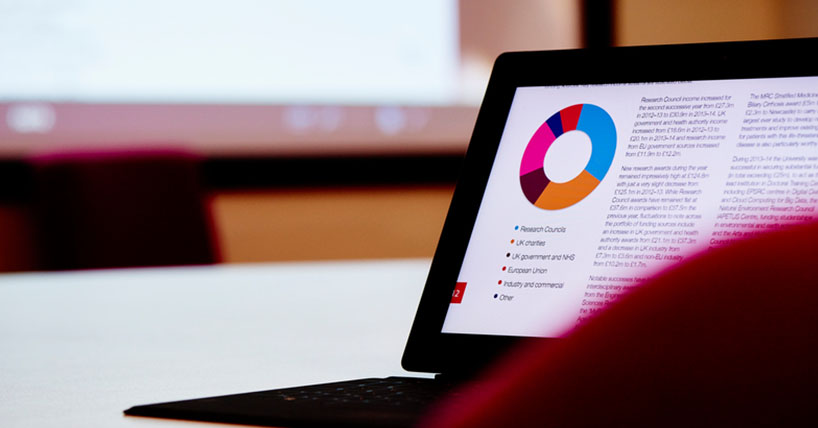
Research Data Management Planning

Research Data Management: Data Plan for your PhD
- Sensitive Data & Data Protection (GDPR)
- PhD DMP supervisors guide
- Support and Training
DMP for your PhD research
All first year post graduate researchers should complete a data management plan for their research and include it as part of their first three month review. There is also a Blackboard course Data Management Plans for Doctoral Students - mandatory for all new doctoral students - to introduce you to research data management and help you complete the plan. Log into Blackboard using your university username and password.
A data management plan or DMP is a living document that helps you consider how you will organise your data, files, research notes and other supporting documentation throughout the length of the project. The aim is to help you find these easily, keep them safe and have sufficient documentation to be able to re-use throughout your research and beyond.
You will need to complete a preliminary data management plan in your first three months, along with your Academic Needs Analysis. Your DMP will continue to develop as your research progresses and you will need to update and review your DMP at every progression review. ( Code of Practice for Research Degree Candidature and Supervision, )

All researchers will have data. Data can be broadly defined as 'Material intended for analysis'. This covers many forms and formats, and is not just about digital data.
For example,
Art History - high resolution reproductions of photographs, notebook describing context
English literature - research notes on text, textual analysis
Engineering - experimental measurements on the physical properties of liquid metals
The University also has a definition for “Research Data” in its Research Data Management Policy that you should consider.
A PhD DMP template and guidance on how to complete your Data Management Plan is available ( see below ). All new doctoral students should complete the Data Management Plans for Doctoral Students module on Blackboard. Contact us if you need further information or have feedback via [email protected]
Guidance on depositing your research data at the end of your doctorate can be found on the Thesis Data Deposit guide. Please also see our depositing research data videos at https://library.soton.ac.uk/researchdata/datasetvideos
Creating your DMP
- Introduction
- DMP and Project Overview
- About your Project Data
- Making Data Findable
- Making Data Accessible
- Making Data Reusable
- Making Data Secure
- Implementing the Plan
- Example Plans
What are data management plans? A data management plan is a document that describes:
- What data will be created
- What policies will apply to the data
- Who will own and have access to the data
- What data management practices will be used
- What facilities and equipment will be required
- Who will be responsible for each of these activities
Your data management plan should be written specifically for the research that you will be doing. Our template is a guide to help you identify the key areas that you need to consider, but not all sections will apply to everyone. You may need to seek further guidance from your supervisor, colleagues in your department or other sources on best practice in your discipline. We provide some details of guidance available in our training section and on our general research data management pages.
Each of the tabs looks at the different topics that can be included in a data management plan. You can move through the tabs in any order.
Describing your Project
At the start of your data management plan (DMP) it is useful to include some basic information about the research you are planning to do. This may already exist in other documents in more detail, but for the purposes of the DMP try to summarise in as few sentences as possible.
What policies will apply?
It is important that you think about who is funding your research and whether there are any requirements that you need to meet. Are you funded by a UK Research Council? What policies do they have on research data - see Funder Guidance . What does our University Research Data Management policy and Code for Conduct for Research state is required?
Does the type of data you will be creating, using, collecting mean that you have to meet certain legal conditions? Will you be collecting any form of personal data, (see ICO Personal Data Definition ), special category data (see ICS Special Category definition ) or is it commercially sensitive? For example, if you are involved in population health and clinical studies research data and records minimum retention could be 20-25 years for certain types of data - see the MRC Retention framework for research data and records for further details.
Do you need Ethics Approval?
Anyone who is dealing with human subjects or cultural heritage (see University policies ) will require to obtain ethics approval and this must be done prior to collecting any data. Your DMP should inform what you say in your ethics application about how you will collect, store and re-use your data. It is important that your DMP and your ethics application are in agreement and you provide your participants with the correct information. Once you receive your ethics approval, review your data management plan and update as necessary.
Reviewing your Data Management Plan
A DMP should be a living document and should be updated as your research develops. It should be reviewed on a regular basis and good practice would encourage that the dates of review are included in the plan itself. Use of a version table in any document can be helpful.
What data will be created?
In your data management plan you need to provide some detail about the material you will be collecting to support your research. This should cover how you will collect notes, supporting documentation and bibliographic management as well as your primary data. Will all your data be held electronically or will you require to maintain a print notebook to collect your observations?
Are you using Secondary Data?
Not everyone has to collect their own data, it may already have been collected and made available. This data is known as secondary data. Some secondary data are freely available, but other data are released with terms and conditions that you need to meet. In some cases this may influence where you can store and analyse the data. You need to be aware of this as you plan the work you intend to do.
How are you collecting or creating your data?
How you collect or gather the material for your research will influence what you need to do to manage them. The way you do this may alter as your research progresses and you should update your plan as required. Will you be collecting data by observing, note-taking in an archive, carrying out experiments or a mixture of these?
How much data are you likely to have?
Knowing how much data you might create is important as it will dictate where you can store your data and whether you need to ask for additional storage from iSolutions. It is unlikely that you can say exactly what volume of data you might create, but you will have an idea of individual file sizes. If you will be working with word, excel documents and a reference management software library then you are likely to be dealing with megabytes or gigabytes of data. If you will be collecting high resolution images then you may end up needing to store terabytes. Estimate as early as possible and if you think you may need additional space you should discuss this with your supervisor.
What formats will you be using?
A crucial factor in being able to share data is that it is in an open format or collected using disciplinary standard software that allow export to open formats. Consider how open the format of your data will be when selecting the software, instruments, word processing packages that you use. See the Data formats section in Introducing Research Data Part III for points to consider.
Who will own the data?
If you have been sponsored by a research council, government, industry or commercial body the agreement you signed may cover ownership of the data that you create. Being aware of this early is useful as it will influence what you are able to do when you come to writing papers, sharing and depositing your data when your finish. It may also impact on where you can store your data.
How will you make your data findable?
Using standards to capture the essential metadata is a good way to help create data that will be easy to find. It will also make preparing for deposit in the future more straightforward. The Research Data Alliance has a helpful list of disciplinary metadata and use case examples. You can make reference to these in your plan once you know what will be most appropriate to use.
Where will you store the data during your PhD?
Where you store your data will depend on things such as the type and size of data you are collecting. Certain types of data, such as personal , special category data (formerly referred to as sensitive data) or commercially confidential data, will require to be stored more securely than others. This type of data generally requires to be stored on University network drives that have additional protection and not on personal computers or cloud storage (for example, Office 365, One Drive). Where you are collecting less sensitive data your choice of storage is wider. For all storage it should in a location with good back-up procedures in place. Consult iSolutions knowledge base for further information.
How will you name your files and folders?
It can be helpful to think about creating a procedure on how you will name your files. This is a basic step where it is useful to consider how easy it will be to interpret the name in the future. Abbreviations can be good, but ask yourself how someone else might understand the file name should you need to share it with them. What would make it easy to know what each file contains? While it is possible to have quite longer file names this can cause problems when you zip files.
How will you tell one version of a file from another?
How will you be able to tell whether you are dealing with the latest version of a file? How will you manage major versus minor changes? What if you want to return to an earlier version? Use the data management plan to investigate what would be the optimum method for you and establish a good procedure from the beginning. Generally the use of 'draft', 'latest' or 'final' should be avoided. Instead consider using the data (YYYY-MM-DD) or a version number, for example, v.1.0 where the nominal value increases with major changes and decimal for minor ones. Adding a version table at the end of a document can also be helpful.
How can you share your data?
To make data accessible is not about doing something at the end of the project, but needs to be planned for from the beginning. During your research you are likely to have colleagues or collaborators who will need to be able to access the data - how will you do this? Will you need a collaborative space and if so what can you use? Does it need to be is a protected location with restricted access due to the type of data you are using? By establishing good procedures on documentation, metadata collection, file-naming and using disciplinary standards this will assist you throughout your research, as well as helping at the end.
How do you handle personal, sensitive or commercially confidential data?
If the data you are collecting contains personal , special category data (formerly referred to as sensitive data) or commercially confidential data then sharing or transferring the files needs to be carried out in a way that does not make the data vulnerable. Data should be anonymised or pseudo-anonymised as early as possible after collection, seek disciplinary guidance prior to collection.
The medium of transfer must be secure and where necessary encryption should be used. You may want to consider one of the following:
There may be other software available and you should check if there is a standard in your discipline.
Transferring data via USB or external drives is not recommended, but where required these should be encrypted. Avoid using email to send files and instead use our University SafeSend service. This offers transfer of files up to 50GB and your files can be encrypted by ticking "Encrypt every file" when creating a new drop-off - see ' How secure is SaveSend'
What data do you need to keep and what do you need to destroy?
Not all the data from a project needs to be kept and the data you collect should be reviewed regularly. The Digital Curation Centre (2014) guide ' Five steps to decide what data to keep: a checklist for appraising research data v.1 ' may help you to decide what to retain. It is important that you retain or discard data in line with your ethics approval.
You also need to consider what data needs to be destroyed, how you will mark the data for destruction and when this needs to happen. Destroying paper based records is relatively easy through our confidential waste system. Destroying digital data is less so as it may need to be done so that it cannot be forensically recovered. Guidance on destroying your data is available or contact iSolutions for advice.
Why do you need to consider the long-term storage now?
At the end of your PhD you will be encouraged to share your data as openly as possible, and as closed as necessary. To do this safely consider what you need to do to enable your data to be accessible in the future. Knowing where the best place to store your data may inform what you need to plan for in its creation or collection. Are you aware of any disciplinary data repositories that hold similar data? Examples are:
- Archaeology - Archaeology Data Service
- ESRC - UK Data Archive
- STFC - eData
- NERC - data centres
- Biology - GenBank
- General repository - Zenodo
Investigate what requirements these repositories have on formats, documentation etc and incorporate these into your plan. Otherwise you should plan to deposit in the University Institutional Repository .
There are currently no costs for depositing most dataset in our Institutional Repository unless the data requires specialist archive storage or is in excess of 1TB. External repositories may have charges for depositing data.
Who will be creating the archive?
Generally as a PhD the job of drawing together your data into a dataset ready for deposit will fall to you as the researcher. It is not the responsibility of your supervisor, although they may be able to advise on what needs to be done. If you are part of a larger project there may be someone designated to curate the project data. For further assistance contact [email protected] .
How long should the data be kept?
This will depend on a number of factors. Your funder may have a policy that requires the data to be held for a minimum of 10 years from last use. If you are working in certain medical areas the data may need to be held for 25 years. There may be some restrictions on how long you can retain personal data relating to Data Protection Act 2018 (GDPR). Significant data that has been given a persistent identifier (DOI) will be kept permanently.
What documentation or additional information needs to accompany the data?
Keeping a record of what changes you have made, when data was collected, where data was collected from, observations, definitions of what has been collected are all crucial to allowing data to be used safely and with integrity. How do you plan to do this? How will you make sure that you can match up your notes with the files they refer to? Some programming languages such as Python and R allow you to make notes in the files about what you are doing which is really helpful. Where this is not an option then you will need to develop your own method to make sure that processes applied to the data are recorded and available to you to refer back to later. Creating a register of your files by type using an excel spreadsheet may be worth considering, but it should be manageable and importantly kept up-to-date.
In order for data to be reusable it requires data provenance. Data provenance is used to document where a piece of data comes from and the process and methodology by which it is produced. It is important to confirm the authenticity of data enabling trust, credibility and reproducibility. This is becoming increasingly important, especially in the eScience community where research is data intensive and often involves complex data transformations and procedures.
- Research Data Management and Sharing - Documentation The importance of systematically documenting your research data. more... less... From the Coursera Research Data Management and Sharing course https://www.coursera.org/learn/data-management
What restrictions will need to apply?
Not all data can be made openly available. Some data may only be shared once a data sharing agreement has been signed, while other data may not be suitable for sharing. Funding councils encourage all data to be as open as possible and as closed as necessary. Where will your data fit with this? What agreements do you need to be able to share your data?
When can data be made available?
Data can be deposited in our Institutional Repository and kept as an 'entry in progress' until it is ready for publication.
Not all data needs to be made immediately available at the end of your PhD. It is possible to add an embargo to give yourself some additional time to find funding to continue your work and re-use your own data. See Regulations on embargoes.
However, it is not always necessary for you to wait until the end of your PhD before depositing data. If you write a conference or journal paper it is likely that you will be asked to make the underpinning data available.
How will you keep your data safe?
What would happen if your files became corrupted or your laptop was stolen, would you be able to restore them? What would happen if someone was able to access your data without your knowledge or approval? If you are holding personal or special category data (formerly referred to as sensitive data) and these became public this would be a data breach with potentially serious consequences.
Dr Fitzgerald Loss of seven years of Ebola research
Consider carefully the impact to you and your research if these were to happen and what procedures you may need to put into place to reduce the risk of these happening.
- Research Data Management and Sharing - Data Security Ensuring your research data are kept safe from corruption, and that access is suitably controlled more... less... From the Coursera Research Data Management and Sharing course https://www.coursera.org/learn/data-management
How will you back up your data?
Good housing keeping of your data is important and this includes doing regular back ups of your data. University storage is backed up regularly but it is important to have your own 'back up' folders, kept separately from your working files. Back up should be done on as regular a basis as required. This can be defined by the length of time you are prepared to repeat work lost. You may need to back up daily, weekly or monthly depending on the nature of your research.
- Research Data Management and Sharing - Backup Effective backup strategies for your research data. more... less... From the Coursera Research Data Management and Sharing course https://www.coursera.org/learn/data-management
As well as establishing a process for backing up your files, you should check the process of restoring your files. You will need to check that the files restore correctly. Having good documentation on what your files contain, what transformations or analysis has been carried out will be invaluable for this process.
How can you safely destroy data?
Destroying data, especially personal , special category data (formerly referred to as sensitive data) or commercially confidential data , is not as straightforward as just deleting the file. Further action is required otherwise the data could be recovered. Please read our guidance on destruction of data and GDPR regulations .
- Data Disposal Essential guidance from the UK Data Archive on data disposal
An important part of research data management is that your plan is implemented and part of your everyday good research practice. The plan should be a living document and reflect your practice. You may find that some parts become redundant or that there is a better way to carry out a process so your plan should be updated. As a PhD researcher it is likely that you will be the person responsible for implementing the plan. If your research is part of a wider research project there may be someone in the team who has been given the role and you should discuss your data management plan with them.
Having written your plan consider what actions do you need to take in order to carry it out? What further information do you need to find? Investigate what training or briefing sessions are available via PGR Manager. If you want to enhance your data analysis skills check out material on Linked in Learning
Over time we will add plans to this section as we get permission to share them.
- PhD DMP Example (Web Science) This is an example PhD Data Management Plan for a research project looking at learner engagement and peer support in digital environments.
- Arts and Humanities
- Science, Medicine and Engineering
- Social Sciences
- Further Reading
Courses offered by the University:
Data Management Plans for Doctoral Students - mandatory course on for all new doctoral students. Log into Blackboard using your university username and password.
Data Management Plan: Q&A Clinic - as a follow up to the compulsary online course, the Library is running twice weekly clinics to answer your DMP queries. Book PGR Development Hub .
Data Management Plan: Why Plan? 45 minute briefing. A Panopto recording of this course is available
Research Data Management: What you need to know from the start . 45 minute briefing. Book via Gradbook
Research Data Management Workshop .180 minute workshop Book via Gradbook

- Introduction to research data (visual arts) Introduction to research data in the visual arts, wirtten by Marie-Therese Gramstadt as part of the Kultur project
- Manage, improve and open up your research and data PARTHENOS training module on various aspect on data management
- VADS4R Data Management Planning A toolkit developed by the Visual Arts Data Skills for Researchers (vads4R)
- Cross-Linguistic Data Formats, advancing data sharing and re-use in comparative linguistics The Cross-Linguistic Data Formats initiative proposes new standards for two basic types of data in historical and typological language comparison (word lists, structural datasets) and a framework to incorporate more data types (e.g. parallel texts, and dictionaries). The new specification for cross-linguistic data formats comes along with a software package for validation and manipulation, a basic ontology which links to more general frameworks, and usage examples of best practices. [article]
- EMBL-EBI Training EMBL-EBI train scientists at all levels to get the most out of publicly available biological data.
- Datatree - Data Training A free online course, aimed at PhD and early career researchers, with all you need to know for research data management, along with ways to engage and share data with business, policymakers, media and the wider public. more... less... The course is for any scientist, whether you look after your own data or are guided by an organisation.
- Expert Tour Guide on Data Management A guide for social science researchers who are in an early stage of practising research data management.
- CESSDA ERIC RDM User Guides Brief guides on important topics in data management and a helpful checklist
- Guide to Social Science Data Preparation and Archiving An important guide covering the different stages of data management to enable the sharing and preserving of data in the Social Sciences
- Managing your dissertation data : Thinking ahead Maureen Haaker and Scott Summers from the UK Data Service gave this presentation. The session sought to help the students ensure transparency in the collection and writing up of their dissertation, whilst also ensuring that good practices in data management were followed. more... less... Although aimed at undergraduate dissertation it provides useful information for everyone.
- UK Data Service Prepare and Manage Data Good data management practices are essential in research, to make sure that research data are of high quality, are well organised, documented, preserved and accessible and their validity controlled at all times. This results in efficient and excelling research.
- FAIR Principlies Guidelines to improve the findability, accessibility, interoperability, and reuse of digital assets
- How to Develop a Data Management and Sharing Plan Jones, S. (2011). ‘How to Develop a Data Management and Sharing Plan’. DCC How-to Guides. Edinburgh: Digital Curation Centre. Available online: http://www.dcc.ac.uk/resources/how-guides
- MRC Retention framework for research data and records guidance on retention of research data an records resulting from population health and clinical studies
- Open Data Handbook Handbook that discusses the why, what and how of open data – why to go open, what open is, and the how to ‘open’ data.
- Open Research Data and Materials Open Science Training Handbook section on research data
Key Documents
- DMP Templates
- Deposit Guide
The template below has been provided to assist you in writing your data management plan. Not all sections will be relevant, but you should consider carefully each section.
- Template for PhD DMP (pdf)
- Template for PhD DMP (Word)
- Code of Conduct for Research University of Southampton policy - October 2017
- Data Protection Policy University of Southampton policy May 2018
- Data Sharing Protocol University of Southampton protocol - May 2018. [Login required]
- Ethics - Human participant policy University of Southampton policy - March 2012
- Ethics - Policy on Cultural Heritage University of Southampton policy - October 2018
- Research Data Management Policy University of Southampton policy - 2015
When the time comes to deposit your data, follow the advice in our Thesis Data Deposit guide .

Email us on: [email protected]
Who's Who in the Research Engagement Team
Research Support Guide
research support.
- << Previous: Sensitive Data & Data Protection (GDPR)
- Next: PhD DMP supervisors guide >>
- Last Updated: Apr 23, 2024 2:22 PM
- URL: https://library.soton.ac.uk/researchdata
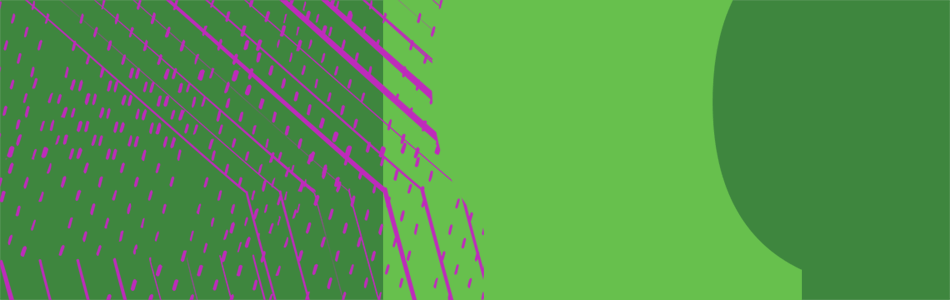
MRC data management plan template
This template should be used to develop a data management plan, part of a research proposal, for the Medical Research Council (MRC).
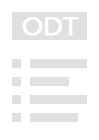
MRC data management plan template (ODT)
ODT , 26 KB
A data management plan should be submitted as part of a research proposal.
You should use this template to make sure that the right information is included.
- 1 April 2024 The data management plan has been updated in line with the revised MRC data sharing policy. It must also include considerations in relation to the UKRI principles and guidance on trusted research and innovation.
This is the website for UKRI: our seven research councils, Research England and Innovate UK. Let us know if you have feedback or would like to help improve our online products and services .
DMP template
Dmp template for postgraduate research projects.
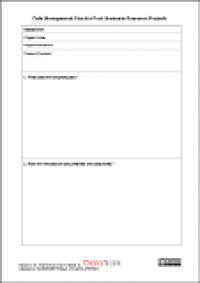
A template for a simple data management plan, suitable for postgraduate research projects. This covers five key areas: what data will be produced, how it will be documented, how it will be stored and backed up, legal and ethical issues, and plans for long-term archiving and sharing.
- Template for Data Management Plan for Postgraduate Research (PDF)
- Template for Data Management Plan for Postgraduate Research (Word document)
Other templates
For other data management plan templates, see the DCC’s DMPonline tool. Templates for major UK funding bodies are available, plus a generic template, which can be used if you are creating the plan for your own benefit, rather than as part of a funding application.
View other items in these categories:
- Data management planning
- Tools and services
Data Management Plans
A data management plan (DMP) is a formal document that outlines how a researcher intends to manage their research data during and after a project.
Creating a DMP can help you:
- Make decisions about managing your data and understand the implications of those decisions
- Identify resources and tools needed for your research
- Estimate costs for resources and tools and budget accordingly
- Anticipate and mitigate problems (e.g. data loss, duplication of effort, security breaches)
- Ensure everyone in your research group understands what is happening with the data
Creating a DMP
A DMP should follow the format specified by a funder or any other relevant requirements. If no format is specified you can create a DMP in whatever way works for you (e.g., a text document or Excel file). You can use the DMP Planning Checklist and Question Guide to help develop your DMP.
DMPs should be created in consultation with all research group members and partners. If you are conducting research with Indigenous communities, your DMP should be co-created with those communities.
Online DMP tools
There are also a number of free, online tools that can help you draft a DMP. These tools provide templates based on discipline, research method, institutional context, and funding requirements.
- DMP Assistant (Canada, provides templates based on discipline and research method)
- DMPOnline (United Kingdom, provides templates from various UK funding agencies)
- DMPTool (United States, provides templates from various US funding agencies)
Examples of DMPs
- Canadian examples from the Digital Research Alliance of Canada
- Canadian examples from DMP Assistant (public plans, not vetted)
- NIH sample data management and sharing plans
- US examples from DMPTool (public plans, not vetted)
- UK examples from the Digital Curation Centre
- UK examples from DMPonline (public plans, not vetted)
Library resources
- Data Management Plan (DMP) - Planning Checklist - this checklist can be used to ensure you’ve included all relevant information in a DMP or to help you gather information you will need to complete your DMP.
- Data Management Plan (DMP) - Question Guide - this guide includes prompting questions and guidance to help you develop a DMP.
Library services The library provides support for:
- Understanding what information and level of detail to include in a DMP
- Navigating funder and journal requirements
- Reviewing DMPs for completeness
- DMP Assistant
Please note that our services are intended to provide support and guidance. The library cannot validate methodological approaches.
External resources
- Brief Guide - Create an Effective Data Management Plan (Digital Research Alliance of Canada)
- Brief Guide - Data Management Plan (Digital Research Alliance of Canada)
- DMP Assistant Video Tutorial Series (Digital Research Alliance of Canada)
- Primer - Data Management Plans (Digital Research Alliance of Canada)
- Guide to Preparing a Data Management Plan (SSHRC - Social Sciences and Humanities Research Council)
- Data Management Plans for NEH Office of Digital Humanities Proposals and Awards (NEH - National Endowment for the Humanities)
- Writing a Data Management & Sharing Plan (NIH - National Institutes of Health)
- Preparing Your Data Management Plan (NSF - National Science Foundation)
- Costs of Data Management (Utrecht University)
- Data management costing tool and checklist (UK Data Service)
- How to Develop a Data Management and Sharing Plan (Digital Curation Centre)
- University home
- For business
- Alumni and supporters
- Our departments
- Visiting us
Data management plans
- Costing data management
- Planning for sensitive data
A data management plan (DMP) describes how the research data will be managed throughout the research lifecycle. This includes what research data will be created and/or collected, how it will be managed during the project, and how it will be shared and preserved at the end of the project. It should typically also describe any potential legal or ethical issues that need to be addressed. A DMP is a dynamic document, and should be updated as a project progresses and it should be reviewed regularly as your data needs change.
Data management plans in funding bids
The University’s Open Access Research and Research Data Management Policy states that all research proposals must include a DMP.
Additionally, most research funders require you to complete a DMP as part of the application process, although the format and content of these plans can differ between funders. A number of bids have been rejected on the basis of weak DMPs, so make sure you give yourself enough time to put together a good quality document.
The table below highlights the funder requirements for DMPs at the grant application stage, but some funders, including the Natural Environment Research Council, require more detailed data management plans to be submitted after a project has started.
Funder DMP Requirements
Writing a data management plan
DMPonline , developed by the Digital Curation Centre (DCC), is a helpful tool for writing DMPs. DMPonline contains data management plan templates for all of the major research funders, and provides guidance and advice on what to include. DMPonline also has the DCC DMP checklist and example DMPs, illustrating the content and level of detail required. Guidance on using DMPonline can be found in the DMPonline User Guide .
Although each DMP is individual, they should all include the same basic information:
- What data will be created?
- How will the data be documented and described?
- How will you manage ethics and intellectual property rights?
- What are the plans for data sharing?
- What is the strategy for long-term preservation and sustainability?
The Research Data Management team are happy to discuss your DMP with you and to provide feedback and comments on your draft DMP. To help you get started, the table below covers the elements that typically make up a DMP. It contains a list of points that you should think about, along with providing suggestions on possible wording.
Typical elements of a DMP

Redirect Notice

Writing a Data Management & Sharing Plan
Learn what NIH expects Data Management & Sharing Plans to address, as well as how to submit your Plan.
- Applications for Receipt Dates BEFORE Jan 25 2023
- Applications for Receipt Dates ON/AFTER Jan 25 2023
Writing a Data Sharing Plan
Under its 2003 data sharing policy , NIH expects investigators to submit a data sharing plan with requests for funding or grants, cooperative agreements, intramural research, contracts, or other funding agreements of $500,000 or more per year.
Data sharing plans should describe how an applicant will share their final research data. The specifics of the plan will vary on a case-by-case basis, depending on the type of data to be shared and how the investigator plans to share the data.
Examples of information to cover in a data sharing plan include:
- The expected schedule for data sharing
- The format of the dataset
- The documentation to be provided with the dataset
- Whether any analytic tools also will be provided
- A brief description of such an agreement
- Criteria for deciding who can receive the data
- Whether or not any conditions will be placed on their use
- Investigators choosing to handle their own data sharing may wish to enter into a data-sharing agreement.
Generating large-scale genomic data? NIH’s Genomic Data Sharing (GDS) policy may also apply to your research. See our GDS Policy Overview to learn more.
Examples of Data Sharing Plans
The exact content and level of detail to be included in a data sharing plan depends on the specifics of the project, such as how the investigator is planning to share data, or the size and complexity of the dataset. The examples below give a sense of what a data sharing plan can look like.
Example 1 This application requests support to collect public-use data from a survey of more than 22,000 Americans over the age of 50 every 2 years. Data products from this study will be made available without cost to researchers and analysts. User registration is required in order to access or download files. As part of the registration process, users must agree to the conditions of use governing access to the public release data, including restrictions against attempting to identify study participants, destruction of the data after analyses are completed, reporting responsibilities, restrictions on redistribution of the data to third parties, and proper acknowledgment of the data resource. Registered users will receive user support, as well as information related to errors in the data, future releases, workshops, and publication lists. The information provided to users will not be used for commercial purposes, and will not be redistributed to third parties.
Example 2 The proposed research will include data from approximately 500 subjects being screened for three bacterial sexually transmitted diseases (STDs) at an inner city STD clinic. The final dataset will include self-reported demographic and behavioral data from interviews with the subjects and laboratory data from urine specimens provided. Because the STDs being studied are reportable diseases, we will be collecting identifying information. Even though the final dataset will be stripped of identifiers prior to release for sharing, we believe that there remains the possibility of deductive disclosure of subjects with unusual characteristics. Thus, we will make the data and associated documentation available to users only under a data-sharing agreement that provides for: (1) a commitment to using the data only for research purposes and not to identify any individual participant; (2) a commitment to securing the data using appropriate computer technology; and (3) a commitment to destroying or returning the data after analyses are completed.
Example 3 The proposed research will involve a small sample (less than 20 participants) recruited from clinical facilities in the New York City area with Williams syndrome. This rare craniofacial disorder is associated with distinguishing facial features. Even with the removal of all identifiers, we believe that it would be difficult if not impossible to protect the identities of subjects given the physical characteristics of subjects, the type of clinical data (including imaging) that we will be collecting, and the relatively restricted area from which we are recruiting subjects. Therefore, we are not planning to share the data.
What data that will be shared:
I will share phenotypic data associated with the collected samples by depositing these data at ________________ which is an NIH-funded repository. Genotype data will be shared by depositing these data at ________________. Additional data documentation and de-identified data will be deposited for sharing along with phenotypic data, which includes demographics, family history of XXXXXX disease, and diagnosis, consistent with applicable laws and regulations. I will comply with the NIH GWAS Policy and the funding IC’s existing policies on sharing data on XXXXXX disease genetics to include secondary analysis of data resulting from a genome wide association study through the repository. Meta-analysis data and associated phenotypic data, along with data content, format, and organization, will be available at ____________. Submitted data will confirm with relevant data and terminology standards.
Who will have access to the data:
I agree that data will be deposited and made available through ________________ which is an NIH-funded repository, and that these data will be shared with investigators working under an institution with a Federal Wide Assurance (FWA) and could be used for secondary study purposes such as finding genes that contribute to process of XXXXXX. I agree that the names and Institutions of persons either given or denied access to the data, and the bases for such decisions, will be summarized in the annual progress report. Meta-analysis data and associated phenotypic data, along with data content, format, and organization, will be made available to investigators through ____________.
Where will the data be available:
I agree to deposit and maintain the phenotypic data, and secondary analysis of data (if any) at ________________, which is an NIH-funded repository and that the repository has data access policies and procedures consistent with NIH data sharing policies.
When will the data be shared:
I agree to deposit genetic outcome data into ________________ repository as soon as possible but no later than within one year of the completion of the funded project period for the parent award or upon acceptance of the data for publication, or public disclosure of a submitted patent application, whichever is earlier.
How will researchers locate and access the data:
I agree that I will identify where the data will be available and how to access the data in any publications and presentations that I author or co-author about these data, as well as acknowledge the repository and funding source in any publications and presentations. As I will be using ________________, which is an NIH-funded repository, this repository has policies and procedures in place that will provide data access to qualified researchers, fully consistent with NIH data sharing policies and applicable laws and regulations.
How to Submit Data Sharing Plans
The plan should be included in the Resource Sharing section of the application. See the How to Apply – Application Guide for form instructions.
Writing a Data Management and Sharing Plan
Under the 2023 Data Management and Sharing (DMS) Policy , NIH expects researchers to maximize the appropriate sharing of scientific data, taking into account factors such as legal, ethical, or technical issues that may limit the extent of data sharing and preservation.
NIH requires all applicants planning to generate scientific data to prepare a DMS Plan that describes how the scientific data will be managed and shared. For more on what constitutes scientific data, see Research Covered Under the Data Management & Sharing Policy .
Applications subject to NIH’s Genomic Data Sharing (GDS) Policy should also address GDS-specific considerations within the elements of a DMS Plan (see NOT-OD-22-189 and details below).
Submitting Data Management and Sharing Plans
The DMS Plan should be submitted as follows:
- DMS Plans should be included within the “Other Plan(s)” field on the PHS 398 Research Plan or PHS 398 Career Development Award Supplemental Form as indicated in the Application Instructions . See below for details on developing and formatting Plans.
- A brief summary and associated costs should be submitted as part of the budget and budget justification (see Budgeting for Data Management and Sharing and the Application Instructions for details).
- Extramural (contracts) : as part of the technical evaluation
- Intramural : determined by the Intramural Research Program
- Other funding agreements : prior to the release of funds
Data Management and Sharing Plan Format
DMS Plans are recommended to be two pages or less in length.
NIH has developed an optional DMS Plan format page that aligns with the recommended elements of a DMS Plan.
Important: Do not include hypertext (e.g., hyperlinks and URLs) in the DMS Plan attachment.
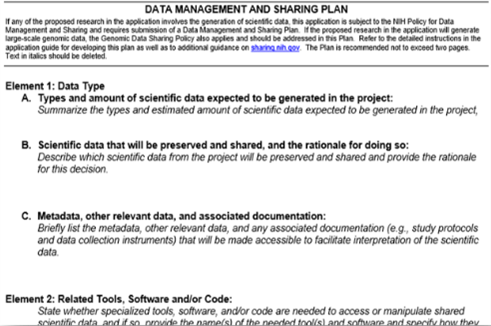
Elements to Include in a Data Management and Sharing Plan
As outlined in NIH Guide Notice Supplemental Policy Information: Elements of an NIH Data Management and Sharing Plan , DMS Plans should address the following recommended elements and are recommended to be two pages or less in length. As described in the Application Guide, the DMS Plan should be attached to the application as a PDF file. See NIH’s Format Attachments page.
1. Data Type
Briefly describe the scientific data to be managed and shared:
- Summarize the types (for example, 256-channel EEG data and fMRI images) and amount (for example, from 50 research participants) of scientific data to be generated and/or used in the research. Descriptions may include the data modality (e.g., imaging, genomic, mobile, survey), level of aggregation (e.g., individual, aggregated, summarized), and/or the degree of data processing.
- Describe which scientific data from the project will be preserved and shared. NIH does not anticipate that researchers will preserve and share all scientific data generated in a study. Researchers should decide which scientific data to preserve and share based on ethical, legal, and technical factors. The plan should provide the reasoning for these decisions.
A brief listing of the metadata, other relevant data, and any associated documentation (e.g., study protocols and data collection instruments) that will be made accessible to facilitate interpretation of the scientific data
For data subject to the GDS Policy: Data types expected to be shared under the GDS Policy should be described in this element. Note that the GDS Policy expects certain types of data to be shared that may not be covered by the DMS Policy’s definition of “scientific data”. For more information on the data types to be shared under the GDS Policy, consult Data Submission and Release Expectations .
2. Related Tools, Software and/or Code
Indicate whether specialized tools are needed to access or manipulate shared scientific data to support replication or reuse, and name(s) of the needed tool(s) and software. If applicable, specify how needed tools can be accessed.
3. Standards
Describe what standards, if any, will be applied to the scientific data and associated metadata (i.e., data formats, data dictionaries, data identifiers, definitions, unique identifiers, and other data documentation).
4. Data Preservation, Access, and Associated Timelines
Give plans and timelines for data preservation and access, including:
- The name of the repository(ies) where scientific data and metadata arising from the project will be archived. See Selecting a Data Repository for information on selecting an appropriate repository.
- How the scientific data will be findable and identifiable, i.e., via a persistent unique identifier or other standard indexing tools.
When the scientific data will be made available to other users and for how long. Identify any differences in timelines for different subsets of scientific data to be shared.
- Note that NIH encourages scientific data to be shared as soon as possible, and no later than the time of an associated publication or end of the performance period, whichever comes first. NIH also encourages researchers to make scientific data available for as long as they anticipate it being useful for the larger research community, institutions, and/or the broader public.
For data subject to the GDS Policy: For human genomic data: Investigators are expected to submit data to a repository acceptable under the Genomic Data Sharing Policy. See Where to Submit Genomic Data . Human genomic data is expected to be shared according to NIH’s Data Submission and Release Expectations , but no later than the end of the performance period, whichever comes first. For Non-human genomic data: Investigators may submit data to any widely used repository. Non-human genomic data is expected to be shared as soon as possible, but no later than the time of an associated publication, or end of the performance period, whichever is first.
5. Access, Distribution, or Reuse Considerations
Describe any applicable factors affecting subsequent access, distribution, or reuse of scientific data related to:
- Informed consent
- Privacy and confidentiality protections consistent with applicable federal, Tribal, state, and local laws, regulations, and policies
- Whether access to scientific data derived from humans will be controlled
- Any restrictions imposed by federal, Tribal, or state laws, regulations, or policies, or existing or anticipated agreements
Any other considerations that may limit the extent of data sharing. Any potential limitations on subsequent data use should be communicated to the individuals or entities (for example, data repository managers) that will preserve and share the scientific data. The NIH ICO will assess whether an applicant’s DMS plan appropriately considers and describes these factors. For more examples, see Frequently Asked Questions for examples of justifiable reasons for limiting sharing of data.
Expectations for human genomic data subject to the GDS Policy: Informed Consent Expectations: For research involving the generation of large-scale human genomic data from cell lines or clinical specimens that were created or collected AFTER the effective date of the GDS Policy (January 25, 2015): NIH expects that informed consent for future research use and broad data sharing will have been obtained. This expectation applies to de-identified cell lines or clinical specimens regardless of whether the data meet technical and/or legal definitions of de-identified (i.e. the research does not meet the definition of “human subjects research” under the Common Rule). For research involving the generation of large-scale human genomic data from cell lines or clinical specimens that were created or collected BEFORE the effective date of the GDS Policy: There may or may not have been consent for research use and broad data sharing. NIH will accept data derived from de-identified cell lines or clinical specimens lacking consent for research use that were created or collected before the effective date of this Policy. Institutional Certifications and Data Sharing Limitation Expectations: DMS Plans should address limitations on sharing by anticipating sharing according to the criteria of the Institutional Certification . In cases where it is anticipated that Institutional Certification criteria cannot be met (i.e., data cannot be shared as expected by the GDS Policy), investigators should state the institutional Certification criteria in their DMS Plan, explaining why the element cannot be met, and indicating what data, if any, can be shared and how to enable sharing to the maximal extent possible (for example, sharing data in a summary format). In some instances, the funding NIH ICO may need to determine whether to grant an exception to the data submission expectation under the GDS Policy. Genomic Summary Results: Investigators conducting research subject to the GDS Policy should indicate in their DMS Plan if a study should be designated as “sensitive” for the purposes of access to Genomic Summary Results (GSR), as described in NOT-OD-19-023 .
6. Oversight of Data Management and Sharing
Indicate how compliance with the DMS Plan will be monitored and managed, the frequency of oversight, and by whom (e.g., title, roles). This element refers to oversight by the funded institution, rather than by NIH. The DMS Policy does not create any expectations about who will be responsible for Plan oversight at the institution.
Sample Plans
NIH has provided sample DMS Plans as examples of how a DMS Plan could be completed in different contexts, conforming to the elements described above. These sample DMS Plans are provided for educational purposes to assist applicants with developing Plans but are not intended to be used as templates and their use does not guarantee approval by NIH.
Note that the sample DMS Plans provided below may reflect additional expectations established by NIH or specific NIH Institutes, Centers, or Offices that go beyond the DMS Policy. Applicants will need to ensure that their Plan reflects any additional, applicable expectations (including from NIH policies and any ICO- or program-specific expectations as stated in the FOA).
Assessment of Data Management and Sharing Plans
Program staff at the proposed NIH Institute or Center (IC) will assess DMS Plans to ensure the elements of a DMS Plan have been adequately addressed and to assess the reasonableness of those responses. Applications selected for funding will only be funded if the DMS Plan is complete and acceptable.
During peer review, reviewers will not be asked to comment on the DMS Plan nor will they factor the DMS Plan into the Overall Impact score, unless sharing data is integral to the project design and specified in the funding opportunity (see NOT-OD-22-189 ).
If data sharing is integral to the project and tied to a scored review criterion in the funding opportunity, program staff will assess the adequacy of the DMS Plan per standard procedure, but peer reviewers will also be able to view the DMS Plan attachment and may factor that information into scores as outlined in the evaluation criteria.
For information about budget assessment by peer reviewers, see Budgeting for Data Management and Sharing .
Revising Data Management and Sharing Plans
Pre-Award Plan Revisions: If the DMS Plan provided in the application cannot be approved based on the information provided, applicants will be notified that additional information is needed. This will occur through the Just-in-Time (JIT) process. Applicants will be expected to communicate with their Program Officer and/or Grants Management Specialist to resolve any issues that prevent the funding IC from approving the DMS Plan. If needed, applicants should submit a revised DMS Plan. Refer to NIH Grants Policy Statement Section 2.5.1 Just-in-Time Procedures for additional guidance.
Post-Award Plan Revisions: Although investigators submit plans before research begins, plans may need to be updated or revised over the course of a project for a variety of reasons for example, if the type(s) of data generated change(s), a more appropriate data repository becomes available, or if the sharing timeline shifts. If any changes occur during the award or support period that affects how data is managed or shared, investigators should update the Plan to reflect the changes. It may be helpful to discuss potential changes with the Program Officer. In addition, the funding NIH ICO will need to approve the updated Plan. NIH staff will monitor compliance with approved DMS Plans during the annual RPPR process as well. For more details, please refer to NOT-OD-23-185: Prior Approval Requests for Revisions to an Approved Data Management and Sharing (DMS) Plan Must be Submitted Using the Prior Approval Module .
Additional Considerations
Note that funding opportunities or ICs may have specific expectations (for example: scientific data to share, relevant standards, repository selection). View a list of NIH Institute or Center data sharing policies . Investigators are encouraged to reach out to program officers with questions about specific ICO requirements.
Please note that a Plan is part of an application, and, as such, an institution takes responsibility for the Plan and the rest of the application's contents when submitting an application. Although part of the official submission, when not considered during peer review the attachment is maintained as a separate “Data Management and Sharing (DMS) Plan” document in the grant folder viewable via the Status Information screen in eRA Commons. This document is viewable by authorized users and is not part of the assembled e-Application.
New Data Management & Sharing Policy Effective January 25, 2023!
Related resources.
Selecting a Data Repository
Budgeting for Data Management & Sharing
Data Management
NIH Institute or Center Data Sharing Policies
Digital Library Services
- UCT Libraries
- Research Data Management
- Digital Scholarship
- Data Curation
- GIS Support
- Digitisation
- Ibali | Digital Collections UCT
- Izolo | Digital Preservation UCT
- ZivaHub | Open Data UCT
- OSF | Research Project Dashboard
- UCT DMP | Planning for Data
- Vision and Mission
- RDS at UCTL
- Data Stewards
- News Archive
- What are Data?
- Good Practice: Linking ZivaHub to ORCiD
- Good Practice: Managing Files and Folders
- Good Practice: Data Storage and Publishing
- Good Practice: DMP Checklist
- ZivaHub Terms of Data Deposit
- Funder Guidelines
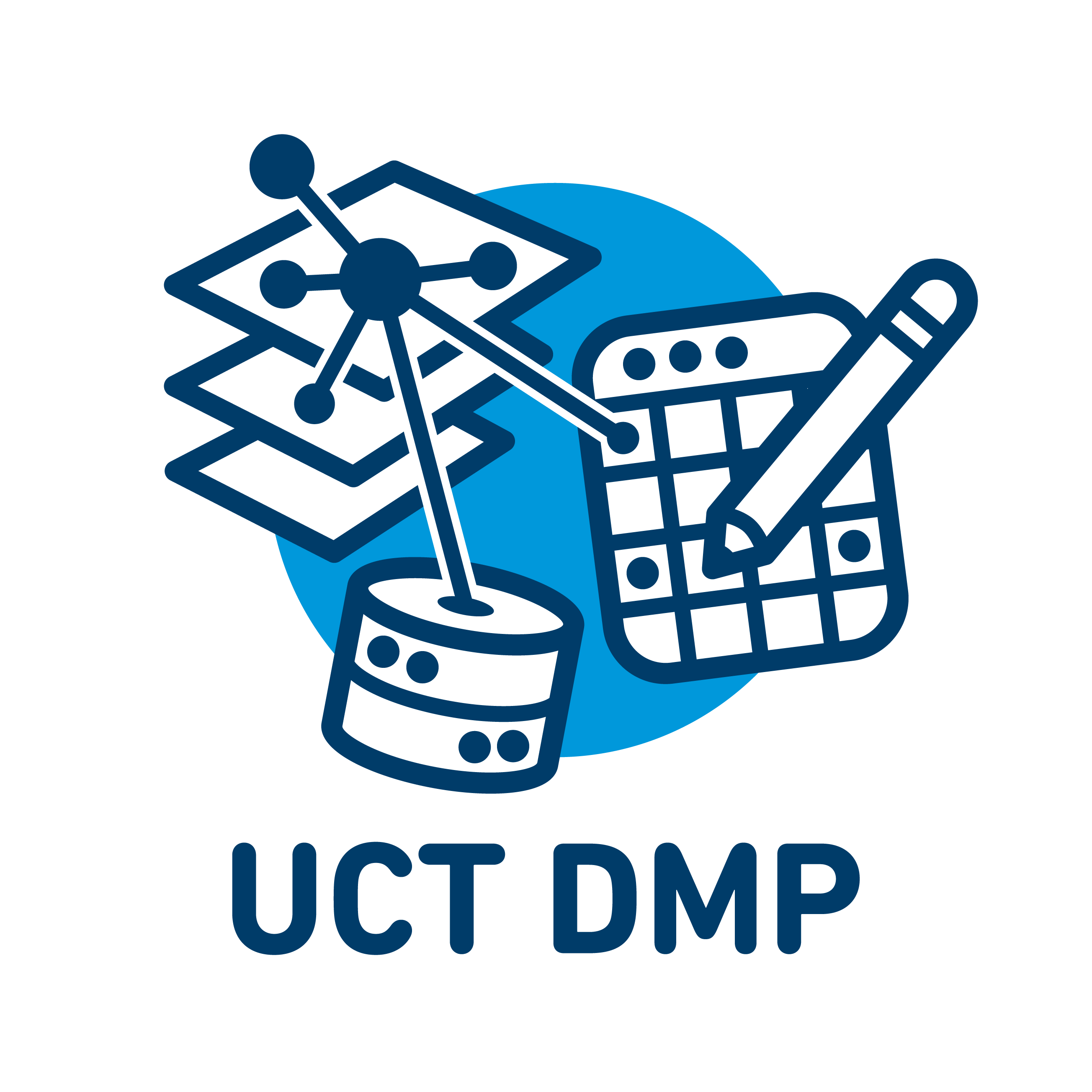
Data Management Planning
Writing a Data Management Plan (DMP) is a way to articulate and plan for your research data needs, and will help you address issues and focus on areas that need further thought.
Submitting a DMP is a requirement of many funding agencies, and since 2019 it is a requirement for UCT researchers and postgraduate students (specifically, Masters and Ph.D. students who produce dissertations, mini-dissertations, and theses). To determine whether your funder has a specific template they require you to use, you may want to view the DLS funder guidelines , or the DCC's overview of funder's data policies .
For further assistance with writing your DMP contact us .
UCT DMP is an online tool provided specifically to help you develop a data management plan.
It provides funder and departmental guidance tailored to specific Data Management Planning (DMP) requirements and allows you to share your plan easily with collaborators, as well as export your document in a variety of formats. UCT's DMP templates contain detailed information on formulating your own research data management plan.
It is important to establish whether your funder has their own DMP requirements so that customised template can be created based on those requirements. Some of these templates are available on UCT DMP .
UCT DMP Data Management Checklist
Before you start your plan, go through our checklist .
Using UCT DMP
The platform allows you to use your UCT credentials to sign in. This can be done by clicking on the ‘Sign In with your UCT credentials’ button on the UCT DMP home page .
If you require further help with your own DMP, please contact your Subject Librarian , departmental data manager, or email us .
Plans currently available on UCT DMP
Funder specific:.
- European Research Council (ERC) - Full DMP
- European Union (Horizon 2020) - Full DMP
- Medical Research Council (MRC) - Full DMP
- National Environmental Research Council (NERC) - Outline and Full DMP
- National Science Foundation- Biological Sciences (NSF-Bio) - Full DMP
- National Institutes of Health (NIH) - Full DMP
- Wellcome Trust - Full DMP
- Clinical Research Centre (CRC-UCT)
To add a funder-specific data management plan that is not currently available on UCT DMP, please contact DLS .
Institution/Department Specific:
Certain UCT departments and research units have customised DMP templates for their researchers and students. Currently, the following units have customised templates:
- University of Cape Town (UCT) - Full DMP (available as default option)
- Centre for Bioprocess Engineering Research (CeBER)
- Centre for MINERALS Research
- Centre for Theatre, Dance and Performance Studies (CTDPS)
- Construction, Economics and management
- Centre for Higher Education Development (CHED) - Full DMP
- School of Economics (Full DMP)
- UCT Clinical Research Centre (CRC) - Full DMP
- Gender, Health and Justice Research Unit (GHJRU) - Full DMP
- Department of Knowledge and Information Stewardship (DKIS) Full DMP
- Data Curation Profile
These templates contain specific guidance for their units, drawn from existing departmental and faculty documentation and curatorial practices. If you would are interested in creating a DMP template for your department or unit, please contact DLS .
Sample Data Management Plans
To help guide you in developing your own DMP, various institutions have made example DMPs available on their websites. For example, the Digital Curation Centre has provided a list of sample DMPs , drawing primarily from UK-based funding agencies.
Currently, the majority of DMP examples originate in the UK and involve UK funding agencies. As time passes, more South African sample DMPs will be added for illustrative purposes.
What you need to do?
Pre-Award or Pre-Project Stage
- If required, write a funder-specific Data Management Plan (DMP) using one of the funder templates on UCT DMP
- Complete the UCT outline DMP (or UCT student outline DMP) on UCT DMP
The outline DMP should demonstrate initial thinking around your research data management practices. As with research proposals, there is an understanding that your data management practices may change over the course of your research.
Consider all relevant costs (article processing charges, data storage, digitisation of materials, etc.) in your application. It is good practice to know the requirements for deposit of data and metadata in your designated repository/repositories before you commence your research.
Award or Project Stage
- Update your funder DMP
- If you are using the UCT DMP template, start working on your full DMP.
Allocate sufficient time for describing your data and deciding which data will need to be preserved for long-term and short-term access. Periodically cross-check your DMP with any faculty- or department-specific checklists that apply to you. Certain funders will require access to your DMP and research data as part of the conditions of the grant, to monitor your progress and compliance. Having a rigorous DMP in place will help you meet their requirements.
Post-Project Stage
- Deposit (and if appropriate share) your data through mandatory funder repositories or through UCT .
- Ensure that the deposit of your published outputs and supporting data meets the requirements of your funder and/or UCT guidelines. For example, have you met the requirements for citation (i.e. obtaining DOIs or other types of persistent identifiers), and user access? Do the required repositories have a URL/handle for your published outputs?
The inclusion of a data statement is a requirement stipulated in a number of leading academic journal publishers such as PLOS One and Nature . Ensure that your statement style complies with the requirements of your funder and publisher.

Research Data Management (RDM): Data Management Plan (DMP)
- What is Research Data
- What is Research Data Management
- FAIR Principles
- Open Datasets
- Data Management Policies
- External Funder Requirements
- Journals and Publishers Policies
- Research Data Lifecycle
- Data Classification
- Data Storage
- Data Security
- Sensitive data
- RDM and Primary Materials Checklist
Data Management Plan (DMP)
- Existing Data
- Data Retention
- Publishing Research Data
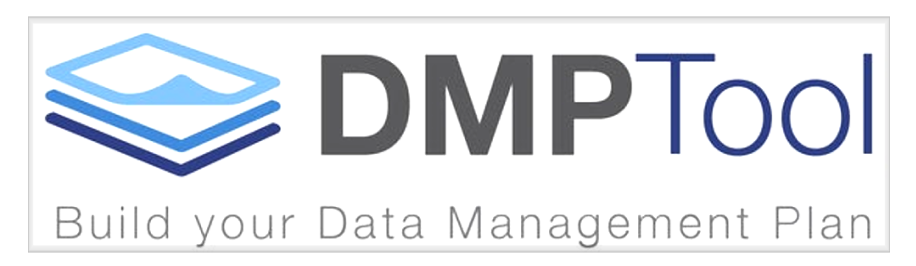
Click the image above to start building your Research Data Management Plan
A Data Management Plan is a document that specifies how research data will be handled both during and after a research project.
It identifies key actions and strategies to ensure that research data are of high quality, secure, sustainable, and – to the extent possible – accessible and reusable.
Many public research funders and ethics committees require a data management plan to be submitted as part of their application.
What to include in DMP
A good DMP takes into account the applicable regulations and data policies and considers the whole research data lifecycle. It typically addresses the following topics:
- What data will be collected, and how.
- How data will be documented.
- How any ethical and legal issues will be dealt with.
- How data will be stored and backed up during research.
- Any plans for preserving (some of the) data beyond the project's end.
- Any plans for sharing or providing access to (some of the) data.
- Who will be responsible for data management, and what additional resources may be required.
- What to cover in your DMP may also depend on your funder, who may provide their own DMP template.
Charles Darwin University recommends completing the RDM and Primary Materials Checklist as part of the DMP and provides a full DMP template
- << Previous: RDM and Primary Materials Checklist
- Next: Existing Data >>
- Last Updated: Apr 29, 2024 4:16 PM
- URL: https://libguides.cdu.edu.au/RDM
- Data management
- Data management planning
- Finding data
- Sharing data
What is research data?
Research data is described by the Australia National Data Service (ANDS) as
The data, records, files or other evidence, irrespective of their content or form (for example, in print, digital, physical or other forms), that comprise research observations, findings or outcomes, including primary materials and analysed data.
What is data management?
Data management includes all activities associated with data other than the direct use of the data, such as:
- data organisation
- archiving data for long-term preservation
- data sharing or publishing
- ensuring security of confidential data and
- data synchronisation.
Under the Australian Code for Responsible Conduct of Research , good data management aligns with a researchers responsibilities.
Benefits of data management
Data management is a forward planning exercise that assists a researcher to:
- explicitly state who owns the copyright and intellectual property of the research
- secure the protection of research data by making a plan of when, where, how and who will back up the project material
- organise data by establishing a version control and naming convention system
- raise their research profile and find new collaborators by using metadata to aid data sharing and gain data citations
- ensure long term access to research data by considering appropriate file formats for storage
- gain access to research data sets to improve research productivity.
Research data management
When outlining how you will deal with the data you will be gathering, it is recommended that a data management plan be completed. To access the Research Data Management Plan, log in to the UniSQ RISE research repository . Please view the Research Data Management Plan (RDMP) guide before filling in the online template.
If you need assistance with completing a data management plan, please contact the Research Support Team .
Research data storage
As a researcher at UniSQ you have access to secure storage for your research data.

Data Science Journal
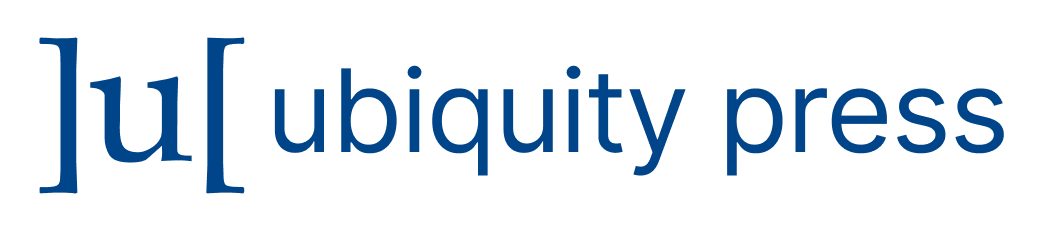
- Download PDF (English) XML (English)
- Alt. Display
- Collection: Data Management Planning across Disciplines and Infrastructures
Research Papers
Road to a chemistry-specific data management plan.
- Ann-Christin Andres
- Daniela Adele Hausen
- Jochen Ortmeyer
- Sonja Herres-Pawlis
In order to develop a discipline-specific data management plan (DMP) template, it is important to obtain information from researchers. For a chemistry-specific template, NFDI4Chem conducted a series of interviews with 27 participants and used data from the RDA WG Discipline-specific Guidance for DMP online survey.
The interviews showed that the implementation of research data management in everyday work is a big challenge. Key findings from the interview series highlight challenges in implementing FAIR principles, with a focus on “Findability” and “Reusability.” The importance of linking physical samples and data in chemistry is emphasised, with discussions on storage, archiving, and the use of tools like electronic lab notebooks and repositories. However, documentation methods, software tools, and naming conventions commonly used in chemical research are also addressed. Overall, the study underscores the need for improved resources and strategies to enhance data management practices in the field of chemistry.
All the gathered information and examples will be used to develop a DMP template in line with chemistry-specific requirements. The results provide a comprehensive outlook on the future developments of research data management (RDM) in chemistry.
- Research Data Management
- Data Management Plan
- discipline-specific
Introduction
In the past, it has been shown that data management plans (DMP) were an important building block for research data management (RDM). In recent years, DMPs have been used much more as generic tools by research funders, who are increasingly moving away from the original idea of a project-specific practical plan ( Smale 2018 ).
This became clear in 2021, when the German Research Foundation (DFG) published a checklist for the appropriate handling of research data ( DFG 2021 ). Similar to a DMP, this checklist is intended to support researchers in Germany in thinking about RDM as early as the project application stage. In contrast to a classic DMP, the DFG connects the individual questions and corresponding answers directly to the specific topics in the proposal text. This emphasises the proximity of data management to research. Similarly, to other research funders, this checklist must also be submitted at the end of the project (DFG, recommendations). Due to that, it is useful to maintain the topics over the project’s funding period. Easy maintenance of the checklist is possible, for example, with the Research Data Management Organiser (RDMO) tool ( RDMO Community ). The DFG website also contains subject-specific statements on the handling of research data in the regarded disciplines ( Deutsche Forschungsgemeinschaft 2023 ). As a more specific example, there is one from the Chemistry Review Board, which briefly touches on the most important areas such as physical samples, analytical measurement methods, use of lab notebooks, etc. ( Fachkollegien Chemie 2021 ).
Chemistry has a strong affinity for documentation. Laboratory notebooks have existed for a long time, and in recent years Electronic Laboratory Notebooks (ELN) have become another important tool for documenting research and, in particular, research data. Ideally, an ELN covers the entire life cycle of the data and enables structured documentation of the individual steps ( NFDI4Chem, Knowledge Base ). Similarly, a DMP ideally covers the entire life cycle, but this is more at the project management level. A DMP is not used for the research data itself, but to describe how the research data is handled. It contains a detailed description of the data that go into the research project and the data that are generated in the research process. A strategy for handling the data as well as rights and role management are usually included ( OpenAire ). These are two different types of documentation that complement each other.
Nevertheless, the checklist on most DMP templates is not self-explanatory, and the meaning of some questions is not clear to researchers, or researchers are confused about which answer is the correct one. However, since this checklist is important for researchers in Germany and especially within the National Research Data Infrastructure ( NFDI ), the consortium NFDI4Chem is also dealing with the topic of DMP for chemists ( NFDI4Chem Consortium ).
The NFDI4Chem aims to support researchers by providing direct guidance on and in the checklist. For this purpose, the consortium has supported the working group of the Research Data Alliance ( RDA WG ) on Discipline-specific Guidance for DMP (RDA). This working group was established in 2019 and conducted a survey at international level. The objective was to reach as many disciplines as possible and to cover the various domains of the Science Europe guidance.
Methodology and Materials
To take forward the issue of a chemistry-specific template, a working group of six professors and research associates was formed within NFDI4Chem. This group first analysed the RDA WG Discipline-specific Guidance for DMP online survey ( Wham et al. 2022 ) (further described as RDA survey) dataset which, after cleaning, yielded 20 results for chemistry. The working group decided to take this dataset as a base and enrich it. Additionally, to fill information gaps in the RDA survey dataset by means of a pre-questionnaire (further described as pre-questionnaire) and interview series (further described as interviews). This data collection was carried out in a structured two-step process. First, the 18-question pre-questionnaire was distributed to potential interviewees with a request to complete it before the interview. The pre-questionnaire was almost identical to the RDA survey. The only difference is the way of handling – the RDA survey was carried out with the tool SoSciSurvey and the pre-questionnaire was a text file.
This was followed by an in-depth interview of 30–40 minutes covering the topics policy, FAIR principles, data, workflows/samples, software, publication, collaboration, and data retention. The interview was minimally adapted based on the interviewee’s responses of the pre-questionnaire, with specific follow-up questions tailored to each participant’s situation, such as asking for examples if the FAIR principles were being implemented, or asking about challenges and barriers if they were not.
For the pre-questionnaire and the interviews, the target group was defined as academic chemists. As far as possible, the different areas of chemistry should be covered. They did not necessarily have to be associated with NFDI4Chem, but should have relevant experience in research data management (RDM), see Figure 1 . In this way, 27 interviews and 27 pre-questionnaires were conducted and important information was obtained. This results in a data set of 47 for the RDA survey and pre-questionnaire (further described as survey) and 27 additional interviews.
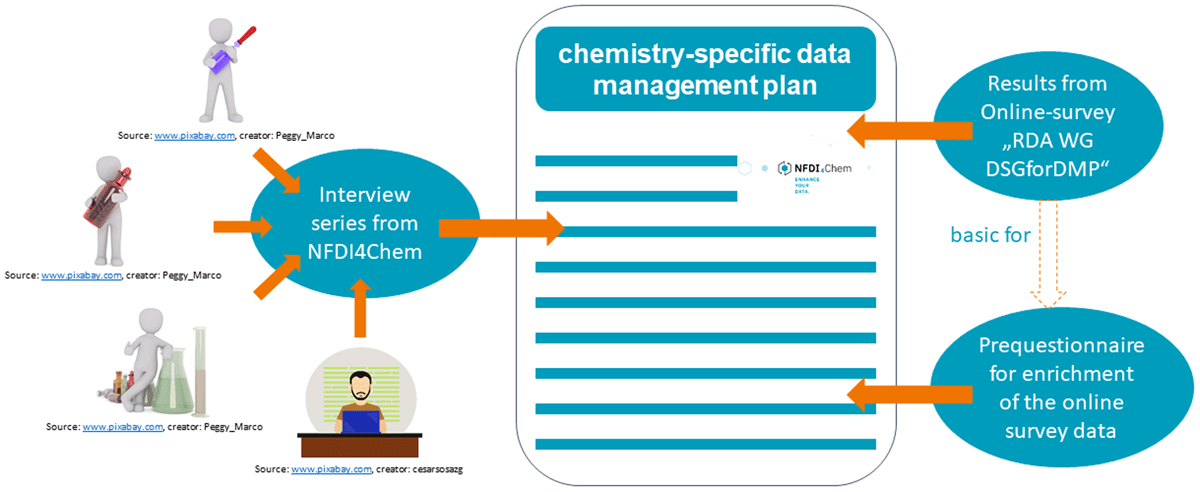
Data generation and reuse that will be included in a chemistry-specific DMP template.
At the current time, in the pre-questionnaire and interviews participated PhD candidates, postdocs, junior professors/ group leaders, and professors. The participants came from the various sub-disciplines of chemistry. The DFG classification was used for the online survey. This results in the distribution shown in Figure 2 . This is a very rough distribution for the chemical subdisciplines. For the pre-questionnaire, a finer breakdown could be made.
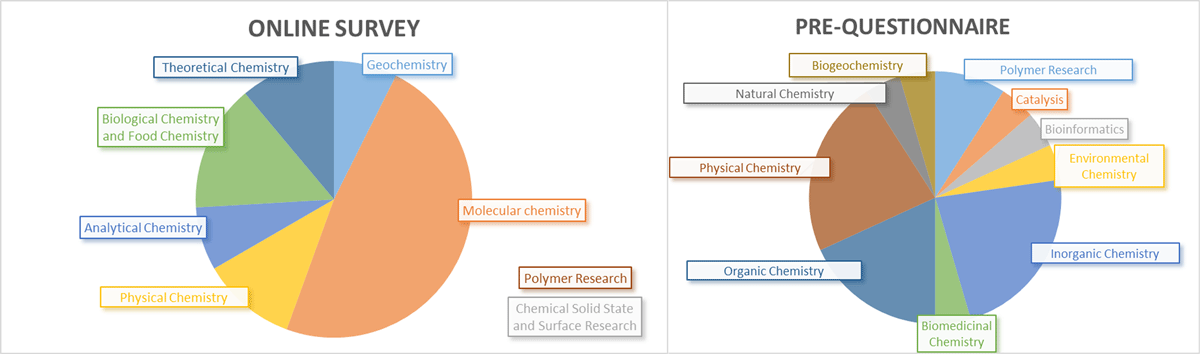
Chemical subdisciplines in the online survey and pre-questionnaire.
The survey, including data processing, data analysis, and data visualisation, was analysed by using Excel (2016). In the analysis of the survey data, responses are analysed by question to account for differences in response rates. The reference point is the total number of respondents. The data can be retrieved from the following link: https://doi.org/10.5281/zenodo.7443839 .
The survey contains more questions than are shown here. Some of them have already been reproduced in other surveys ( Herres-Pawlis, Liermann & Koepler 2020 ; Hausen 2019 ), e.g. on the kind of research data or on data collection. In this section the new and additional results are presented. However, the results will be included in the DMP template and the guidance.
Policies and FAIR
Policy can play an important role for DMPs as the two elements are often complementary. Policies often provide a framework which DMPs may wish to reflect in part. Therefore, the introduction to the DMP refers to a RDM policy and best practice examples.
When asked about their policies, 11 of the interviewees reported the implementation of written policies within their respective working groups. These policies include a range of information like experiments including standard operating procedures (SOPs), documentation wikis, and best practice examples. They also specify details such as file naming conventions and data organisation and storage. Four of the respondents shared information with their colleagues partially in writing and partially verbally, while a quarter passed on information exclusively through verbal communication. Training for new staff is typically facilitated by experienced team members, and RDM is covered in group seminars. Of the interviewees, five are actively engaged in the process of developing guidelines, utilising existing guidelines, or seeking recommendations.
An exemplary case of policy implementation can be found in the Daumann group, which has publicly shared a comprehensive policy on its working group’s website ( Daumann Group 2022 ). This policy outlines data generated during projects, provides detailed instructions for ensuring data’s FAIR (Findable, Accessible, Interoperable, Reusable) characteristics, recommends tools, and prescribes documentation in electronic lab notebooks (ELNs). The rules span the entire data lifecycle, covering everything from experiment planning and execution to data storage, archiving, and publication. In addition to the example from the Daumann group, guidelines from journals such as Angewandte Chemie were also mentioned. Six interviewees stated that they find these guidelines very helpful and have incorporated aspects of them into their research group.
Furthermore, both guidelines and ELNs are regarded as crucial elements for fully implementing FAIR principles. In the survey, 12 interviewees indicated partial implementation of FAIR principles, while four interviewees did not implement them, and 11 participants abstained from responding to the question. Interviews further revealed that implementing FAIR principles is challenging under current framework conditions. Interviewees primarily focused on the ‘Findability’ and ‘Reusability’ aspects of FAIR. Findability, especially within the organisation, was seen as time-dependent, with ELNs and repositories highlighted as key tools for ensuring reusability. ‘Accessibility’ and ‘Interoperability’ aspects were viewed more critically overall.
In summary, it is evident that resources for fully implementing FAIR principles are lacking, and weaknesses in ‘Accessibility’ and ‘Interoperability’ should be addressed in the DMP template. It is imperative to gather more information and engage in discussions within NFDI4Chem and the chemical community to tackle these challenges effectively.
Storage and decommission strategy of physical samples and data
Another important part of the data management plan is storage and archiving. As mentioned previously, various sub-disciplines within chemistry produce physical samples. Currently, these physical samples are not addressed in any DMP template. However, this gap holds significant importance in the field of chemistry, given the link between data and physical samples. Almost 50% of the interviewees maintain a sample database, documenting details like the substances, their storage locations, and analysis data. In addition to its availability, the continuous maintenance of this database as well as a permanent person in charge are really important. Normally, the physical samples are stored on site in a chemical storage facility or in the laboratory itself under the necessary conditions such as darkness, low temperatures, etc. Three researchers have opted for the Compound Platform - a molecular archive - as an alternative. The Compound Platform serves as a repository for samples which makes the accessibility of substances and the collaborative work easy.
While chemical substances are stored under appropriate conditions, they often have a shorter lifespan than data. Nearly all, 25 from 27 interviewees, indicated that they store both physical samples and data indefinitely ( Figure 3 ).
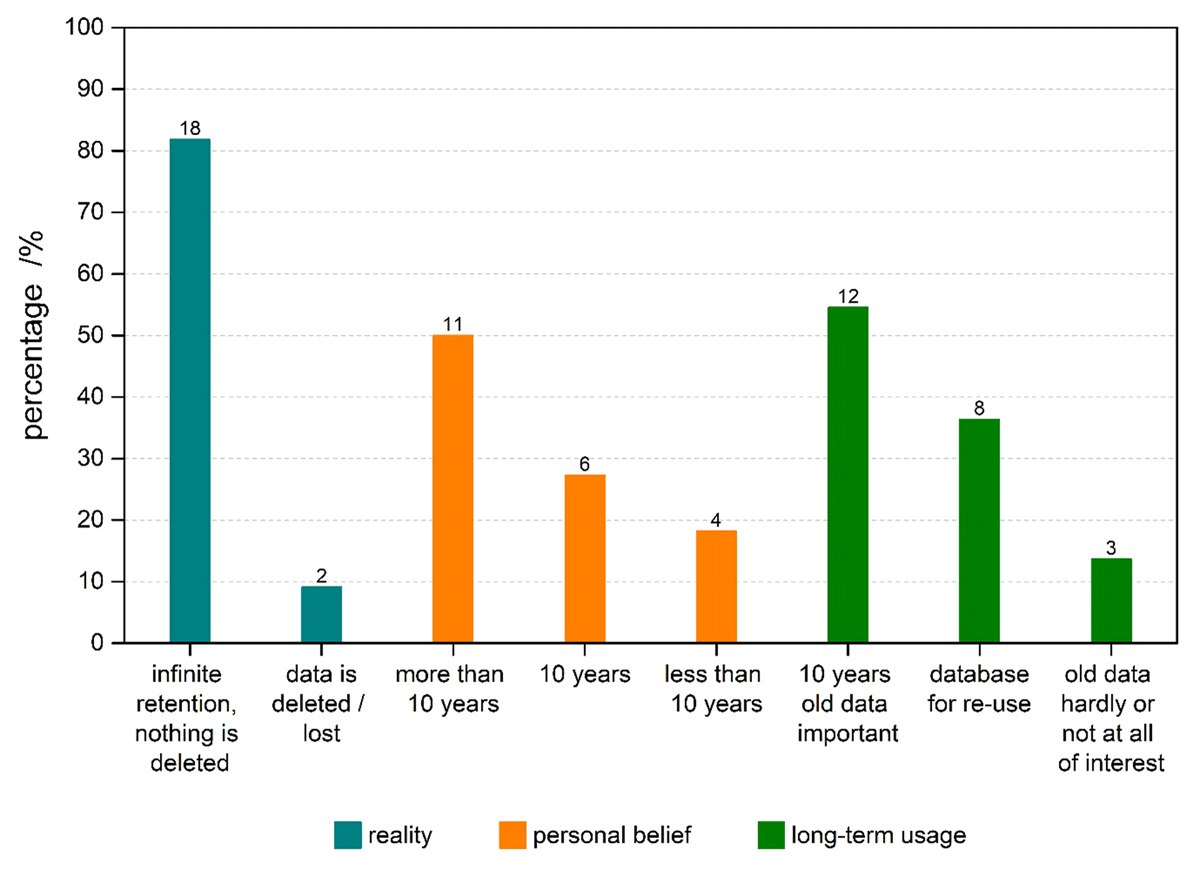
The behaviour of chemists regarding research data deletion and storage. The numbers above the bars are the answers in absolute values.
This perspective contrasts with their personal beliefs about how data storage should be handled. Roughly 50% retain the data for more than 10 years, while seven interviewees consider 10 years of storage appropriate. Five interviewees suggest that storing data for less than 10 years should suffice. Moreover, 10 interviewees mentioned that data is required to compile databases, either for long-term use within their own research groups or for the broader scientific community, aiding in experiment replication and machine learning applications. The majority of the data is stored on institutional servers.
Nevertheless, in general, data at all stages of the examination process are stored, from raw data to processed spectra. This is highlighted by the responses from the survey to the question about saving different types and multiple versions of data ( Figure 4 ).
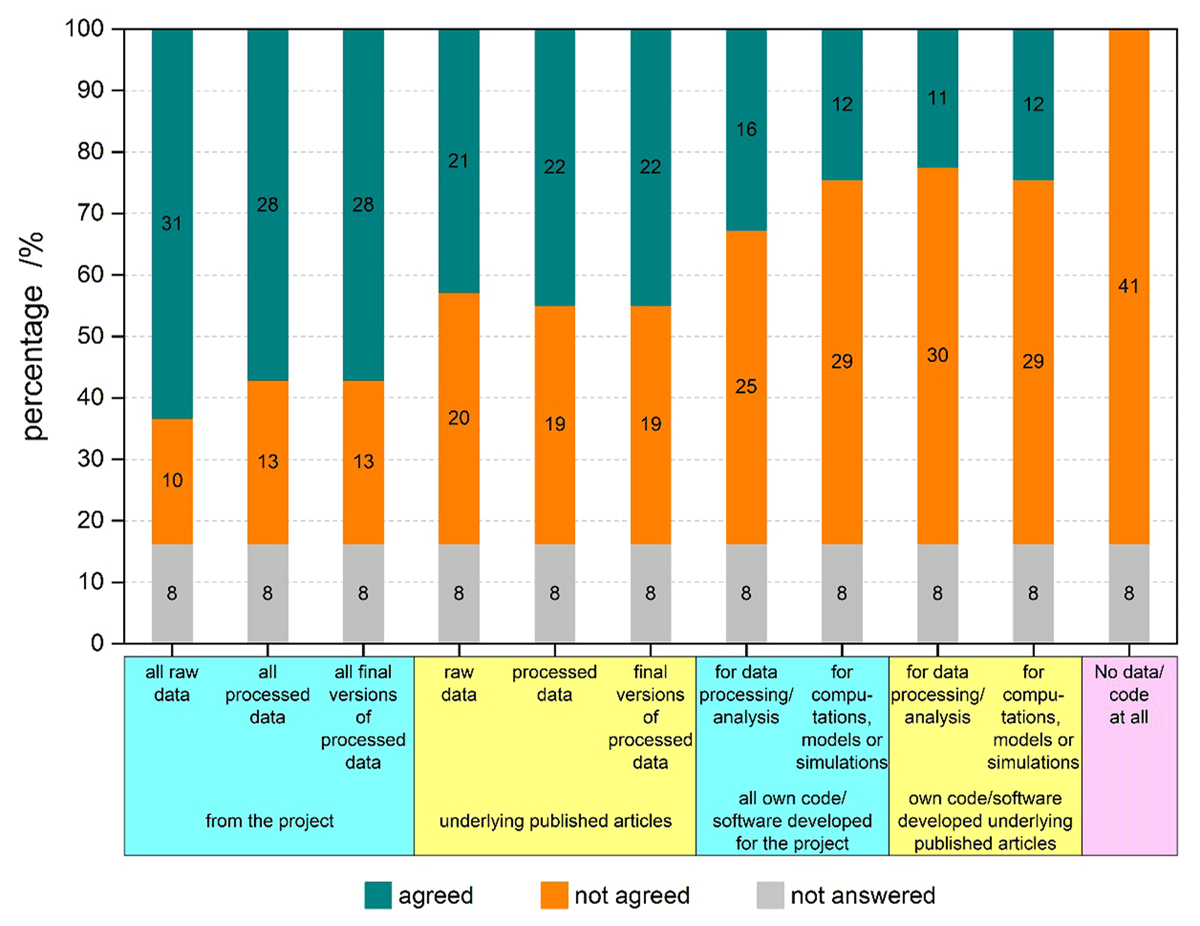
Survey question: Which data/code do you or the researchers you support keep for the long-term (at least 10 years) after the project? The numbers within the bars are the answers in absolute values.
The response indicates an average of four different types of data being stored. The most common types are raw data (17 interviewees), all processed data (16 interviewees) and all final versions of processed data (14 interviewees) from the project as well as processed data underlying published articles and their final versions (12 interviewees each).
Typically, there is no specific strategy for data deletion or the disposal of physical samples. While some participants indicated that the data would not be deleted, some reasons such as the energy crisis or the actuality of the data argued for deletion or a deletion strategy. Therefore, careful consideration should be given to which data and samples warrant long-term preservation. A chemistry-specific DMP should therefore cover the management of physical samples and include strategies for data deletion and sample disposal.
Software in chemistry
In addition to storing data and samples, it is important to know which software was used to create, process, and analyse the data. As expected, chemists are using a wide range of software solutions for data collection and analysis. All of the interviewees stated they rely on method-specific proprietary software ( Figure 5 ). In terms of data analysis, almost no one sticks exclusively to software specific to their instruments. While 10 interviewees switch directly after data collection to other programs, e.g. electronic lab notebooks (ELNs), Excel, or OriginLab, 15 participants employ both device-specific and other software during their data evaluation. Due to the lack of suitable software solutions, 12 interview partners have to use self-developed software, scripts, or code. For example, one interviewee mentioned that ~50 % of their software is in fact developed by themselves. Interestingly, already 10 interviewees apply open-source solutions, such as open-source ELNs or JupyterLab. However, only five interviewees store their data centralised on institute-provided servers or in clouds. To make it easier for researchers to complete the DMP template, those software solutions already in use should be mentioned. There should also be an indication that there is still a lot of proprietary software in use and that the NFDI4Chem consortium is working on converters for different proprietary formats.
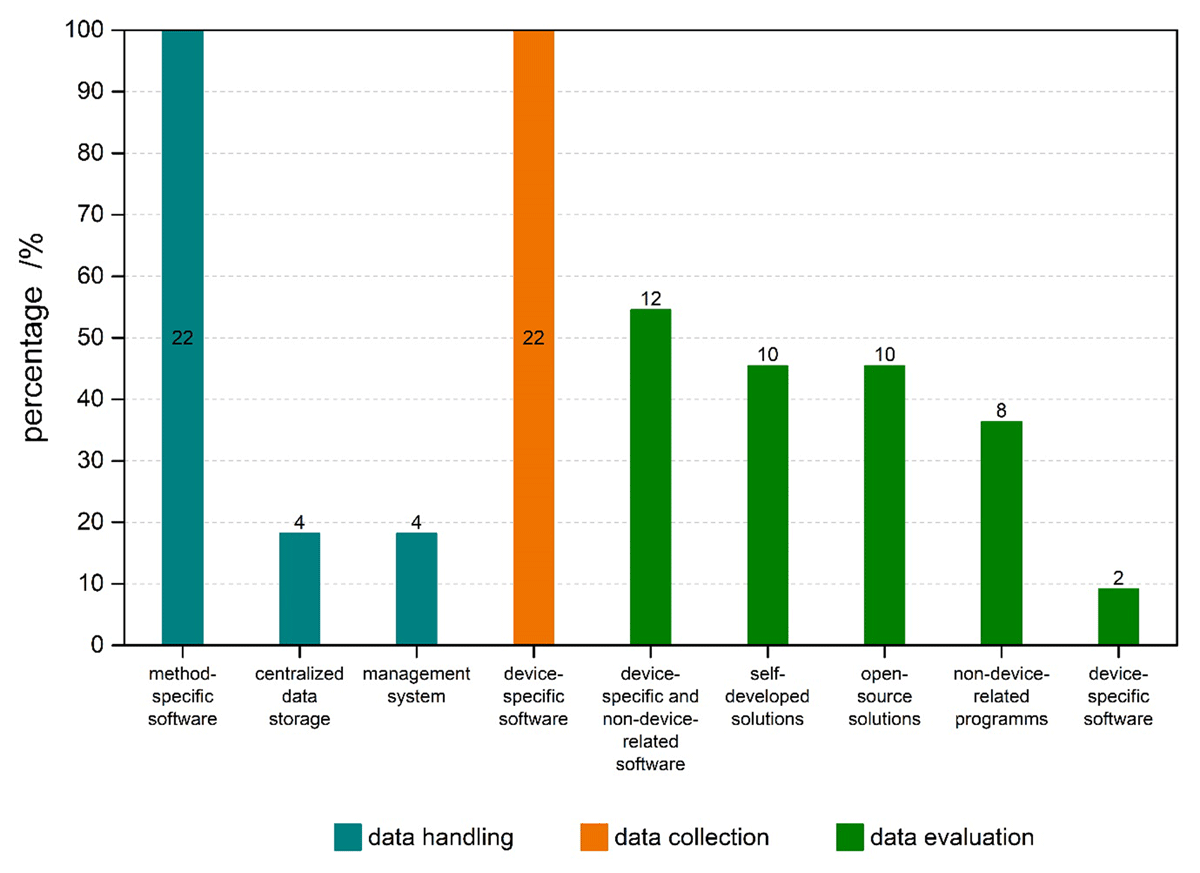
Distribution of categorised software solutions during management of research data. The numbers above the bars are the answers in absolute values.
Data publication, documentation and electronic lab notebooks
As mentioned earlier, chemists have physical samples as well as data that they need to document and manage. Chemists are very used to documentation because chemistry has been using laboratory books for a long time.
In the interview series two electronic lab notebooks (ELN) were explicitly mentioned - chemotion and eLabFTW , which seems to be a good open source solution for physical chemists and material scientists ( Cautaerts 2021 ). The two interviewees emphasised its flexibility, although adapting it to existing frameworks and laboratory conditions took some time. ELNs or currently still widely used paper lab books serve as an important documentation option in chemistry. It obtains for example how molecules are synthesised, how an analysis of certain samples is performed, etc. as well as observation comments, which normally are not passed with a text or data publication. This information represents an essential resource for researchers looking to reuse the information. Therefore, it is crucial in future work to aim for a combination of these two documentation types. For now, the lab notebook topic will be included in the DMP template to provide context regarding where data documentation occurred and where data can be readily accessed and used by following collaborators.
The question regarding documentation methods received different responses in the survey. In the online survey (OS), participants were asked to rate the use of various documentation types on a scale of 1-5, while in the pre-questionnaire (PQ) they indicated if they use them or not. Nevertheless, the results tend to be very similar. Notebooks (OS 7; PQ 16) 1 and naming conventions (OS 6, PQ 16) were common across both parts. The main difference was in the most frequently named item - hardware/equipment (OS 7) and ELNs (PQ 19).
Another question in the survey targeted naming conventions. Standardised conventions (19 interviewees) were followed by the part using no conventions (11 interviewees) or ad hoc conventions (4 interviewees). The naming conventions often follow a structured format, including a 2–3-digit personal code, a running number for the experiment, and details about the analysis being performed. Some experimental metadata is given in the naming, this facilitates the findability of the data and the sample equally.
Making the data findable is the first step towards data publication. Some ELNs, such as chemotion ( Tremouilhac et al. 2020 ), offer the functionality to publish data directly from the ELN to a linked repository.
In the interview series, 24 interviewees mentioned that they publish the data in parallel with the text publication or at the end of the project. Only three interviewees have not published any data. Of interest for the DMP template is not only the list of chemistry-specific repositories like Chemotion, RADAR4Chem, etc; 2 it’s also about clarifying how data should be published. In fact, responses from the interviews vary from data publication via a repository to supporting information (SI). Within the SI, the processed data are provided as images, tables, etc. in a pdf format. The practice of providing additional information in an SI is common practice in chemistry and has grown historically. Therefore, it is not surprising that chemists consider it as a data publication. The survey reflects similar trends ( Figure 6 ), with over 50% of respondents indicating that they publish their data as SI, six interviewees each indicated data publication via a chemistry-specific repository, and using GitHub for sharing code.
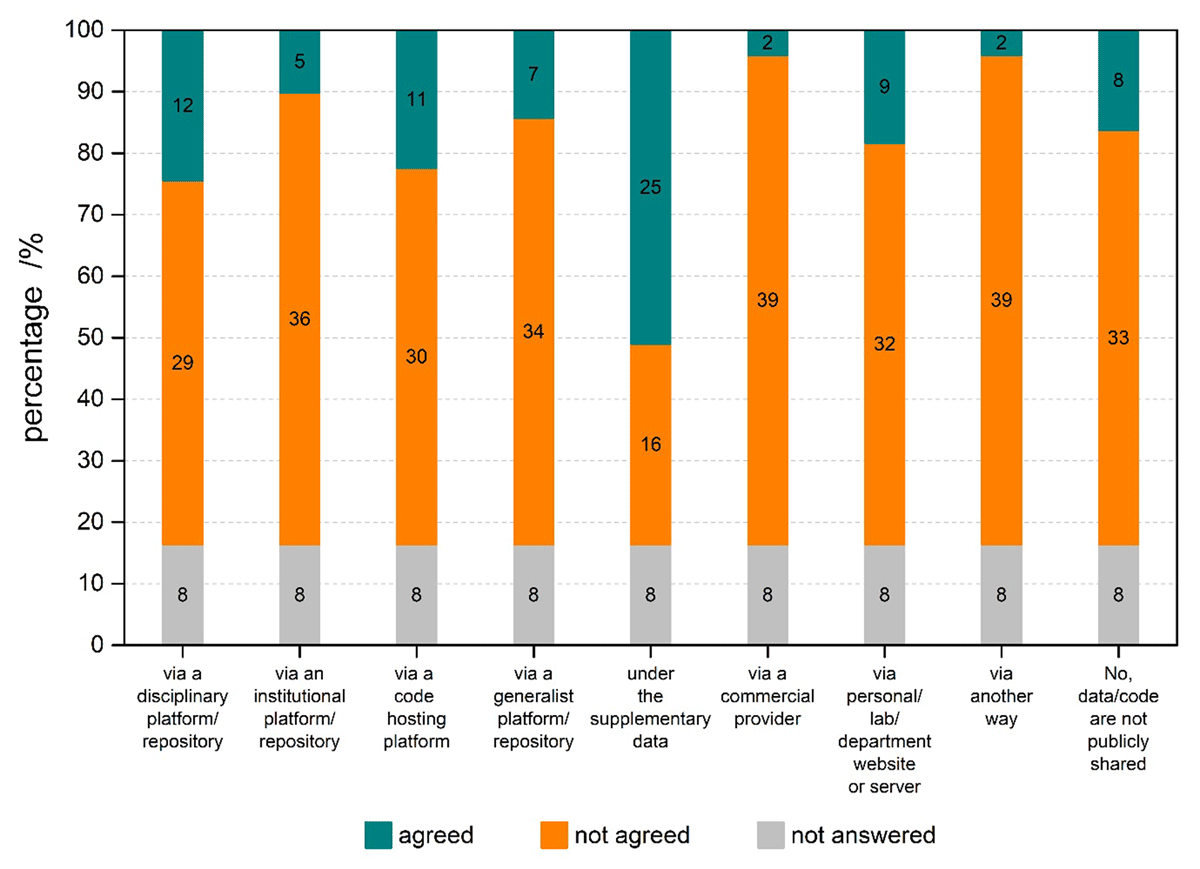
Survey question: Do you or the researchers you support publicly/openly share data/code for the long-term (at least 10 years) after the project? The numbers within the bars are the answers in absolute values.
As already mentioned, the DMP template will ask for additional information beyond the repository to be used. The DMP should be clear about the process for publishing data and linking to related texts.
Exemplary datasets demonstrating high-quality data publication practices, such as those recognised as the FAIRest datasets ( Krausch & Giessmann 2021 ; Kessler et al. 2021 ) in chemistry by NFDI4Chem the last years will be linked from the template. These and more can also be found e.g. in the NFDI4Chem Knowledge Base under Lead-by-Example section. As mentioned earlier in the paragraph, another best practice for data publications, which should be linked in the DMP, is the ability to publish data from the chemotion ELN to the chemotion repository. Data publications with embargo periods are possible in Chemotion through a simple internal workflow. Digital Object Identifiers (DOI) are assigned to the data, so that data and text publication can be linked to each other.
Collaborations and obligations
One of the issues that makes data publication difficult is collaboration with other groups or with industry. A total of 25 researchers have past or ongoing collaborations with industry or research institutes, ranging from occasional partnerships to day-to-day collaboration. Typically, research data management, data rights and roles are contractually defined before the project starts to avoid conflicts. There are individual conflicts with regard to the publication of data. Internal discussions delay publication, even where contractual arrangements exist. The EU, which insists on publishing openly, was seen as a negative example.
Another problematic case occurs in the field of machine learning: The affiliation of data and models is not easy to define. If the training data comes from proprietary sources, the data belongs to the industry partner, even if the model used is publicly available. Further complications occur:
- The confidentiality of the data varies.
- In some collaborations, the data from the joint research project is handled openly.
- In other collaborations, access to the data is severely restricted and only the collaborators involved have access rights. The data is stored on a separate server and a partner’s laptop is used to send the data in encrypted form.
Within industry collaborations, the industry partner typically presents a specific question, and the outcomes are not predetermined. However, challenges arise when the outcome is predefined prior to the project, preventing the possibility of conducting an independent analysis of the question.
Another important aspect mentioned by an interviewee should be considered from the very beginning and should already be taken into account when creating a DMP: all cooperation partners should work to follow the FAIR principles from the beginning, so that the data could be FAIR in the end. Therefore, recommendations and guidance on cooperation should be included in the DMP template. Additionally, addressing issues such as contractual regulations, data confidentiality, rights, and role management is equally critical.
Summary and Conclusion
In order to develop a subject-specific DMP template, a lot of information is needed to properly support the target subject. In the case of a chemistry-focused DMP template, this includes documentation practices centered around laboratory notebooks, or the linking of physical samples and data. In addition, the development is simplified by starting from an already existing or predefined template and developing it further.
In this paper and for our DMP template, we have taken the DFG checklist as a starting point, as it is relevant for our project and many projects in Germany. To gather information, we used data from the RDA online survey, interviews, and a prequestionnaire.
In summary, the data from the RDA online survey provides a basis that needs to be enriched quantitatively and qualitatively through further data collection. Through the interview series, many examples were obtained that will be used to support the chemistry-specific DMP template. In particular, these examples can be built directly into the template as a hint and also allow for the creation of response options to choose from when writing the DMP.
Moreover, the survey and interviews revealed that linking physical samples and data is particularly important in chemistry. The DMP template needs to be expanded to include this point. In addition, the DMP template should refer to a deletion strategy as well as a disposal strategy for physical samples. In today’s times, it is important to use the available resources wisely. The aspects explained in the interviews as to why data and samples are kept indefinitely make it possible to provide concrete guidance for the deletion and disposal strategy: what should be kept, for how long and under what conditions. What are the reasons for deleting data or disposing of samples? The template will ask the researcher these questions while offering concrete solutions.
During the interviews, it was possible to gather a whole range of software used in chemistry, covering different areas such as measurements, analytics, analysis, etc. It became apparent that researchers are bound to proprietary software when using a measurement technique, but as soon as it goes a step further, many turn to open source solutions. The different software solutions should be listed as answer options in the DMP template. A hint to open source solutions should also be created.
One field of application in which open source solutions are widely used is ELNs. In this context, the respondents mainly use the two open-source ELNs Chemotion and eLabFTW. ELNs are seen as an important building block for the implementation of FAIR principles in workflows.
Likewise, awareness for data publication needs to be created in the DMP template. A significant portion of chemists still ‘publish data’ as Supplement Information.
Restrictions on data publication arise in chemistry due to collaborations e.g. with industry and patents. Many respondents stated that they have collaborations and patents, but that this is only a small part of the work. However, it affects almost everyone, so in the DMP template attention should be drawn to the fact that the handling of data on patents or on publications must be a different one, which should also be implemented at an early stage.
The results of the interviews were analysed and presented. The information collected from these interviews will be integrated into the DMP template for chemistry. In the next step, a first draft of the chemistry-specific DMP template is published under 10.5281/zenodo.10948510 . The information will be included either as help, answer options or as a modified question.
Interviewees related to the single categories - online survey 20 and pre-questionnaire 27 participants.
A detailed list can be found in the NFDI4Chem knowledge base https://knowledgebase.nfdi4chem.de/knowledge_base/ .
Acknowledgements
We thank all participants of the online survey, the pre-questionnaire and interviews providing valuable insights in their data handling, research and daily work.
Funding Information
The presented work was conducted as part of the NFDI4Chem project (DFG project) (no. 441958208). The authors would like to thank the Deutsche Forschungsgemeinschaft (DFG, German Research Foundation) for funding and support.
Competing Interests
The authors have no competing interests to declare.
Cautaerts, N. 2021. Using ElabFTW for materials science. FOSDEM , 2021. DOI: https://doi.org/10.5446/53345
Daumann Group. 2022. Research data management plan https://www.cup.lmu.de/ac/daumann/erc-starting-grant-lanthanophor/research-data-management-plan/ .
Deutsche Forschungsgemeinschaft. 2021. Handling of research data: Checklist for planning and description of handling of research data in research projects https://www.dfg.de/download/pdf/foerderung/grundlagen_dfg_foerderung/forschungsdaten/forschungsdaten_checkliste_en.pdf .
Deutsche Forschungsgemeinschaft. 2023. Handling of research data https://www.dfg.de/en/research_funding/principles_dfg_funding/research_data/index.html .
eLabFTW. Homepage . https://www.elabftw.net/ .
Fachkollegien, C. 2021. Handlungsempfehlung zum Umgang mit Forschungsdaten https://www.dfg.de/download/pdf/foerderung/grundlagen_dfg_foerderung/forschungsdaten/handreichung_fachkollegien_chemie_forschungsdaten.pdf .
Hausen, D. 2019. Forschungsdaten in der Chemie, publiscologne , urn:nbn:de:hbz:79pbc-opus-15024 .
Herres-Pawlis, S, Liermann, J and Koepler, O. 2020. Research data in chemistry – Results of the first NFDI4Chem Community Survey. Z. Anorg. Allg. Chem ., 646: 1748–1757. DOI: https://doi.org/10.1002/zaac.202000339
Kessler, C, Eller, J, Gross, J and Hansen, N. 2021. Supplementary material for ‘Adsorption of Light Gases in Covalent Organic Frameworks: Comparison of Classical Density Functional Theory and Grand Canonical Monte Carlo Simulations’. DaRUS . DOI: https://doi.org/10.1016/j.micromeso.2021.111263
KIT. ComPlat – the Compound Platform . https://www.ioc.kit.edu/braese/english/1020.php .
Krausch, N and Giessmann, RT. 2021. Collection of UV/Vis spectra acquired while monitoring reaction progress of thymidine phosphorolysis with varying reactant concentrations. Zenodo . DOI: https://doi.org/10.5281/zenodo.3243352
Nationale Forschungsdateninfrastruktur. https://www.nfdi.de/ .
NFDI4Chem Consortium. https://www.nfdi4chem.de .
NFDI4Chem. FAIR4Chem Award https://nfd4chem.t3cm.com/index.php/2022/02/03/fair4chem-award-winners/ .
NFDI4Chem. Knowledge Base https://knowledgebase.nfdi4chem.de/knowledge_base/ .
OpenAire. 2018. Why is this a good Data Management Plan? https://www.openaire.eu/blogs/why-is-this-a-good-data-management-plan .
RDMO Community. https://rdmorganiser.github.io/ .
Research Data Alliance. Working Group DSGforDMP https://www.rd-alliance.org/groups/discipline-specific-guidance-data-management-plans-wg .
Smale, N, Unsworth, K, Denyer, G and Barr, D. 2018. The history, advocacy and efficacy of data management plans. bioRxiv , 443499. DOI: https://doi.org/10.1101/443499
SoSciSurvey. https://www.soscisurvey.de/ .
Tremouilhac, P, Lin, C-L, Huang, P-C, Huang, Y-C, Nguyen, A, Jung, N, Bach, F, Ulrich, R, Neumair, B, Streit, A and Bräse, S. 2020. The Repository Chemotion: Infrastructure for Sustainable Research in Chemistry. Angew. Chem. Int. Ed ., 59. DOI: https://doi.org/10.26434/chemrxiv.12195318
Wham, B, Hausen, DA, Anders, I, Sheridan, S, Ilamparuthi, S and Turkyilmaz-vander Vel den, Y. 2022. Dataset and R code from RDA WG Discipline-Specific Guidance on DMP – Online Survey [Data set]. Zenodo . DOI: https://doi.org/10.5281/zenodo.8367217
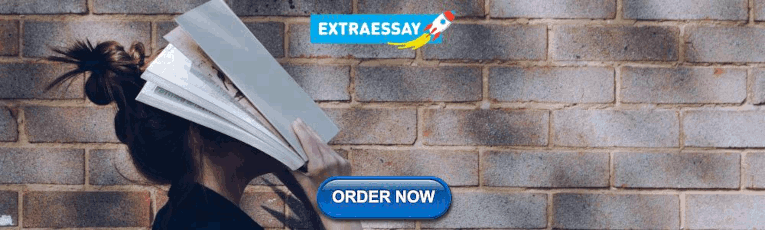
IMAGES
VIDEO
COMMENTS
DMPTool. The DMPTool is web-based and provides basic templates to help you construct a Data Management Plan. Using DMPTool, researchers can access a template, example answers, and guiding resources to successfully write a data management plan for any research project or grant.
NSF Guide and template for creating a data management plan. NSF Guide and template for creating a data management plan. Skip to main content. Main Menu; Utility Menu; Search; FACULTY OF ARTS AND SCIENCES | HARVARD.EDU. FAS Research Administration Services ... [email protected]. Admin Login.
A research data management plan is a document drawn up at the start of the research process. It outlines how all research data will be generated or collected, managed, stored and preserved, shared or disposed of. All researchers at UWE, including doctoral researchers, must complete a research data management plan before collecting any data as ...
A data management plan (DMP) will help you manage your data, meet funder requirements, and help others use your data if shared. The DMPTool is a web-based tool that helps you construct data management plans using templates that address specific funder requirements. From within this tool, you can save your plans, access MIT-specific information ...
A data management plan, or DMP (sometimes also called a data sharing plan), is a formal document that outlines what you will do with your data during and after a research project. ... It uses the new NIH Data Sharing and Management structure but can be used for a variety of research types. View the General template. Harvard Medical School ...
DMPTool.org is created by a group of major research institutions to help researchers generate data management plans. These documents display the current (as of the date shown on the document) funding agency DMP guidance, followed by a listing of points from DMPTool on topics to consider when writing the relevant portion of your DMP.
Introduction. This guide outlines a writing strategy for creating a data management plan based on requirements common to many funding agencies. Some of the advice in this guide also applies to data sharing plans or data availability statements required by journals and certain funding organizations.
The DMPTool allows you to create, maintain and archive your DMPs in one location. 1. Types of data & materials produced. Give a short description of the data and materials collected and created, and amounts (if known). Description of data to be produced (experimental, observational, raw or derived, physical collections, models, images, etc.)
Tab through this guide to consider each stage of the research data management process, and each correlated section of a data management plan. Tools for Data Management Planning The DMPTool allows you to create data management plans from templates based on funder requirements using a quick-and-easy click-through wizard.
Crafting your data management plan. Most research funders encourage researchers to think about their research data management activities from the beginning of the project. This will often mean a formal plan for managing data (a 'data management plan'). However, even informally setting out your plans and project guidelines can make your life much easier.
A DMP (or DMSP, Data Management and Sharing Plan) describes what data will be acquired or generated as part of a research project, how the data will be managed, described, analyzed, and stored, and what mechanisms will be used to at the end of your project to share and preserve the data. One of the key advantages to writing a DMP is that it ...
Planning. Writing a Data Management Plan. Data Management Plans (DMPs) generally require a researcher to describe the research data they will create, how the data will be stored and managed during the project, and how valuable data will be shared. The resulting plan will have short and long-term benefits: find and understand your data when needed.
The University also has a definition for "Research Data" in its Research Data Management Policy that you should consider. A PhD DMP template and guidance on how to complete your Data Management Plan is available . All new doctoral students should complete the Data Management Plans for Doctoral Students module on Blackboard.
A data management plan should be submitted as part of a research proposal. You should use this template to make sure that the right information is included. Updates. 1 April 2024 The data management plan has been updated in line with the revised MRC data sharing policy.
A template for a simple data management plan, suitable for postgraduate research projects. This covers five key areas: what data will be produced, how it will be documented, how it will be stored and backed up, legal and ethical issues, and plans for long-term archiving and sharing. Template for Data Management Plan for Postgraduate Research (PDF)
Data Management Plans. A data management plan (DMP) is a formal document that outlines how a researcher intends to manage their research data during and after a project. Creating a DMP can help you: Make decisions about managing your data and understand the implications of those decisions. Identify resources and tools needed for your research.
A data management plan (DMP) describes how the research data will be managed throughout the research lifecycle. This includes what research data will be created and/or collected, how it will be managed during the project, and how it will be shared and preserved at the end of the project. It should typically also describe any potential legal or ...
H2020 templates: Data management plan v2.0 - 15.02.2018 2 Project1 Number: [insert project reference number] Project Acronym: [insert acronym] Project title: [insert project title] DATA MANAGEMENT PLAN 1 The term 'project' used in this template equates to an 'action' in certain other Horizon 2020 documentation
Writing a Data Sharing Plan. Under its 2003 data sharing policy, NIH expects investigators to submit a data sharing plan with requests for funding or grants, cooperative agreements, intramural research, contracts, or other funding agreements of $500,000 or more per year.. Data sharing plans should describe how an applicant will share their final research data.
Writing a Data Management Plan (DMP) is a way to articulate and plan for your research data needs, and will help you address issues and focus on areas that need further thought. Submitting a DMP is a requirement of many funding agencies, and since 2019 it is a requirement for UCT researchers and postgraduate students (specifically, Masters and ...
A Data Management Plan is a document that specifies how research data will be handled both during and after a research project. It identifies key actions and strategies to ensure that research data are of high quality, secure, sustainable, and - to the extent possible - accessible and reusable.
Costs related to open access to research data in Horizon 2020 are eligible for reimbursement during the duration of the project under the conditions defined in the H2020 Grant Agreement, in particular Article 6 and Article 6.2.D.3, but also other articles relevant for the cost category chosen. Data Management Plan. A DMP template is provided in ...
Data management is a forward planning exercise that assists a researcher to: explicitly state who owns the copyright and intellectual property of the research. secure the protection of research data by making a plan of when, where, how and who will back up the project material. organise data by establishing a version control and naming ...
The CODATA Data Science Journal is a peer-reviewed, open access, electronic journal, publishing papers on the management, dissemination, use and reuse of research data and databases across all research domains, including science, technology, the humanities and the arts. The scope of the journal includes descriptions of data systems, their implementations and their publication, applications ...
Data Management Plan The Data Management Plan (DMP) is a methodological document that describes the lifecycle of data collected, generated, and processed during the doctoral thesis. This plan must include at least the types of data to be processed, their format, origin, storage, and measures to ensure that they will be treated