Academia.edu no longer supports Internet Explorer.
To browse Academia.edu and the wider internet faster and more securely, please take a few seconds to upgrade your browser .
- We're Hiring!
- Help Center
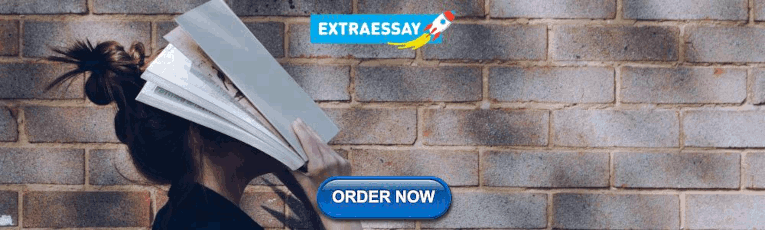
Language Acquisition
- Most Cited Papers
- Most Downloaded Papers
- Newest Papers
- Save to Library
- Last »
- Psycholinguistics Follow Following
- Linguistics Follow Following
- First Language Acquisition Follow Following
- Syntax Follow Following
- Bilingualism Follow Following
- Languages and Linguistics Follow Following
- Second Language Acquisition Follow Following
- Semantics Follow Following
- Cognitive Science Follow Following
- Morphology Follow Following
Enter the email address you signed up with and we'll email you a reset link.
- Academia.edu Publishing
- We're Hiring!
- Help Center
- Find new research papers in:
- Health Sciences
- Earth Sciences
- Cognitive Science
- Mathematics
- Computer Science
- Academia ©2024
CONCEPTUAL ANALYSIS article
This article is part of the research topic.
Evidence-informed practice for creating meaningful individual special education programs for diverse students with learning disabilities
Beyond English centrality: Integrating expansive conceptions of language for literacy programming into IEPs Provisionally Accepted
- 1 University of Colorado Denver, United States
The final, formatted version of the article will be published soon.
This paper addresses the English centrality in reading policy, assessment and instructional practices in the U.S. and its implications for the educational programing for emerging bilingual students (EBs) with disabilities. A recent review of the state of practice as it relates to EBs with disabilities reveals concerns that have endured for nearly six decades: biased assessment, disproportionality issues in special education, and teachers' lack of understanding of language acquisition and students' potential. These concerns demonstrate a need for the field to prioritize multilingual lenses for both the identification of and programming for EBs with disabilities. We propose attention to conceptions of language that expand beyond the structuralist standpoint that prevails in the current science of reading reform. We offer guiding principles for IEP development grounded in sociocultural perspectives when designing bilingual instructional practices, which can be applied to the educational programming for EBs with disabilities. Within a sociocultural view of bilingualism and biliteracy, language and literacy are understood by multiplicities in use, practice, form, and function, in which all communicators draw from expansive meaning-making repertoires, whether in listening, speaking, reading, writing, viewing, and multimodally representing. By expanding conceptions of a student's linguistic repertoire, we honor their use of language as one, holistic system in which their named languages plus a multitude of linguistic practices intersect and interact.
Keywords: bilingual special education1, emerging bilingual students with disabilitiess2, biliteracy3, IEPs4, science of reading
Received: 30 Nov 2023; Accepted: 29 Apr 2024.
Copyright: © 2024 Ferrell, Soltero-González and Kamioka. This is an open-access article distributed under the terms of the Creative Commons Attribution License (CC BY) . The use, distribution or reproduction in other forums is permitted, provided the original author(s) or licensor are credited and that the original publication in this journal is cited, in accordance with accepted academic practice. No use, distribution or reproduction is permitted which does not comply with these terms.
* Correspondence: Dr. Amy L. Ferrell, University of Colorado Denver, Denver, United States
People also looked at
Arabic Sign Language Alphabet Classification via Transfer Learning
- Conference paper
- First Online: 30 April 2024
- Cite this conference paper
- Muhammad Al-Barham 13 ,
- Osama Ahmad Alomari 13 &
- Ashraf Elnagar 14
Part of the book series: Lecture Notes in Networks and Systems ((LNNS,volume 960))
Included in the following conference series:
- International Conference on Emerging Trends and Applications in Artificial Intelligence
The integration of artificial intelligence (AI) has addressed the challenges associated with communication with the deaf community, which requires proficiency in various sign languages. This research paper presents the RGB Arabic Alphabet Sign Language (ArASL) dataset, the first publicly available high-quality RGB dataset. The dataset consists of 7,856 meticulously labeled RGB images representing the Arabic sign language alphabets. Its primary objective is to facilitate the development of practical Arabic sign language classification models. The dataset was carefully compiled with the participation of over 200 individuals, considering factors such as lighting conditions, backgrounds, image orientations, sizes, and resolutions. Domain experts ensured the dataset’s reliability through rigorous validation and filtering. Four models were trained using the ArASL dataset, with RESNET18 achieving the highest accuracy of 96.77%. The accessibility of ArASL on Kaggle encourages its use by researchers and practitioners, making it a valuable resource in the field ( https://www.kaggle.com/datasets/muhammadalbrham/rgb-arabic-alphabets-sign-language-dataset ).
This is a preview of subscription content, log in via an institution to check access.
Access this chapter
Institutional subscriptions
https://github.com/mohammad-albarham/Arabic-Sign-Language-Alphabet-Classification-via-Transfer-Learning .
AbdElghfar, H.A., et al.: A model for qur’anic sign language recognition based on deep learning algorithms. J. Sensors 2023 (2023)
Google Scholar
Abdulhussein, A.A., Raheem, F.A.: Hand gesture recognition of static letters American sign language (ASL) using deep learning. Eng. Technol. J. 38 (6), 926–937 (2020)
Article Google Scholar
Al-Barham, M., et al.: RGB Arabic alphabets sign language dataset. arXiv preprint arXiv:2301.11932 (2023)
ALtememe, M.S., El Abbadi, N.K.: Gesture interpreting of alphabet Arabic sign language based on machine learning algorithms. In: 2022 Iraqi International Conference on Communication and Information Technologies (IICCIT), pp. 177–183. IEEE (2022)
Duwairi, R.M., Halloush, Z.A.: Automatic recognition of Arabic alphabets sign language using deep learning. Int. J. Electr. Comput. Eng. (2088-8708) 12 (3) (2022)
Hdioud, B., Tirari, M.E.H.: A deep learning based approach for recognition of Arabic sign language letters. Int. J. Adv. Comput. Sci. Appl. 14 (4) (2023)
Ito, S.i., Ito, M., Fukumi, M.: Japanese sign language classification based on gathered images and neural networks. Int. J. Adv. Intell. Inform. 5 (3) (2019)
Kumar, K.: DEAF-BSL: deep learning framework for British sign language recognition. Trans. Asian Low-Resour. Lang. Inf. Process. 21 (5), 1–14 (2022)
Latif, G., Mohammad, N., Alghazo, J., AlKhalaf, R., AlKhalaf, R.: Arasl: Arabic alphabets sign language dataset. Data Brief 23 , 103777 (2019)
Pansare, J.R., Gawande, S.H., Ingle, M.: Real-time static hand gesture recognition for American sign language (ASL) in complex background (2012)
Saleh, Y., Issa, G.: Arabic sign language recognition through deep neural networks fine-tuning. Int. J. Online Biomed. Eng. (iJOE) 16 , 71 (2020). https://doi.org/10.3991/ijoe.v16i05.13087
Zakariah, M., Alotaibi, Y.A., Koundal, D., Guo, Y., Mamun Elahi, M., et al.: Sign language recognition for Arabic alphabets using transfer learning technique. Comput. Intell. Neurosci. 2022 (2022)
Download references
Acknowledgment
We extend our sincere appreciation to the Student Counseling Department at the University of Jordan for their valuable guidance and expertise, which greatly contributed to acquiring accurate and appropriate images based on their extensive experience. Furthermore, we express our gratitude to Jana M. AlNatour and Raneem F. Abdelraheem for their valuable assistance in the meticulous data collection process. Additionally, we would like to extend our special thanks to the Research Institute for Sciences and Engineering (RISE) at the University of Sharjah for their support and collaboration.
Author information
Authors and affiliations.
MLALP Research Group, University of Sharjah, Sharjah, United Arab Emirates
Muhammad Al-Barham & Osama Ahmad Alomari
Department of Computer Science, University of Sharjah, Sharjah, United Arab Emirates
Ashraf Elnagar
You can also search for this author in PubMed Google Scholar
Corresponding author
Correspondence to Ashraf Elnagar .
Editor information
Editors and affiliations.
Ingenium Research Group, University of Castilla-La Mancha, Ciudad Real, Spain
Fausto Pedro García Márquez
National University of Computer and Emerging Sciences, Islamabad, Pakistan
Akhtar Jamil
Department of Computer Engineering, Istinye University, Istanbul, Türkiye
Alaa Ali Hameed
Ingenium Research Group, University of Castilla-La Mancha (UCLM), Ciudad Real, Spain
Isaac Segovia Ramírez
Rights and permissions
Reprints and permissions
Copyright information
© 2024 The Author(s), under exclusive license to Springer Nature Switzerland AG
About this paper
Cite this paper.
Al-Barham, M., Alomari, O.A., Elnagar, A. (2024). Arabic Sign Language Alphabet Classification via Transfer Learning. In: García Márquez, F.P., Jamil, A., Hameed, A.A., Segovia Ramírez, I. (eds) Emerging Trends and Applications in Artificial Intelligence. ICETAI 2023. Lecture Notes in Networks and Systems, vol 960. Springer, Cham. https://doi.org/10.1007/978-3-031-56728-5_20
Download citation
DOI : https://doi.org/10.1007/978-3-031-56728-5_20
Published : 30 April 2024
Publisher Name : Springer, Cham
Print ISBN : 978-3-031-56727-8
Online ISBN : 978-3-031-56728-5
eBook Packages : Engineering Engineering (R0)
Share this paper
Anyone you share the following link with will be able to read this content:
Sorry, a shareable link is not currently available for this article.
Provided by the Springer Nature SharedIt content-sharing initiative
- Publish with us
Policies and ethics
- Find a journal
- Track your research
- Category: AI
Tiny but mighty: The Phi-3 small language models with big potential
- Sally Beatty
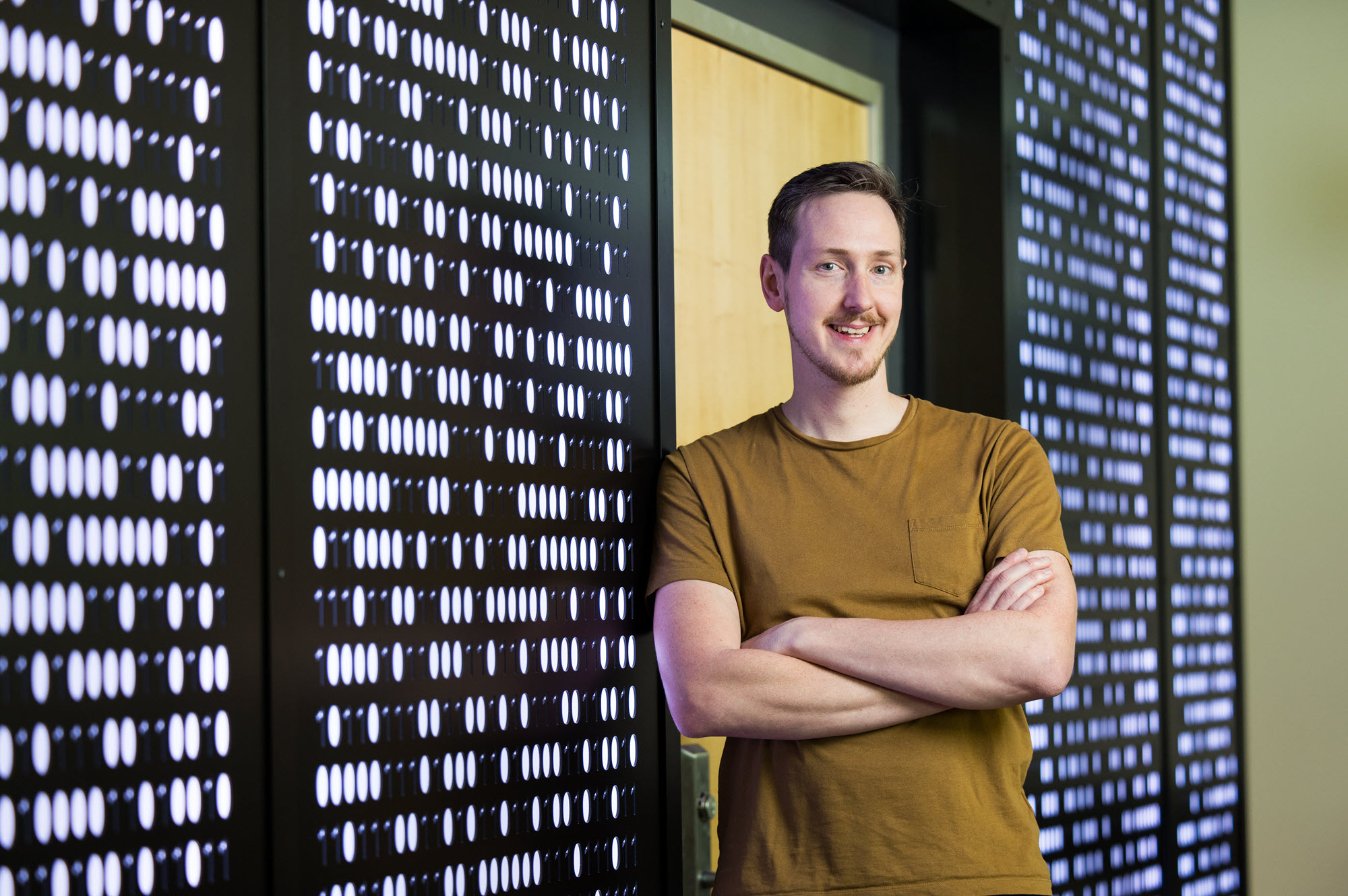
Sometimes the best way to solve a complex problem is to take a page from a children’s book. That’s the lesson Microsoft researchers learned by figuring out how to pack more punch into a much smaller package.
Last year, after spending his workday thinking through potential solutions to machine learning riddles, Microsoft’s Ronen Eldan was reading bedtime stories to his daughter when he thought to himself, “how did she learn this word? How does she know how to connect these words?”
That led the Microsoft Research machine learning expert to wonder how much an AI model could learn using only words a 4-year-old could understand – and ultimately to an innovative training approach that’s produced a new class of more capable small language models that promises to make AI more accessible to more people.
Large language models (LLMs) have created exciting new opportunities to be more productive and creative using AI. But their size means they can require significant computing resources to operate.
While those models will still be the gold standard for solving many types of complex tasks, Microsoft has been developing a series of small language models (SLMs) that offer many of the same capabilities found in LLMs but are smaller in size and are trained on smaller amounts of data.
The company announced today the Phi-3 family of open models , the most capable and cost-effective small language models available. Phi-3 models outperform models of the same size and next size up across a variety of benchmarks that evaluate language, coding and math capabilities, thanks to training innovations developed by Microsoft researchers.
Microsoft is now making the first in that family of more powerful small language models publicly available: Phi-3-mini , measuring 3.8 billion parameters, which performs better than models twice its size, the company said.
Starting today, it will be available in the Microsoft Azure AI Model Catalog and on Hugging Face , a platform for machine learning models, as well as Ollama , a lightweight framework for running models on a local machine. It will also be available as an NVIDIA NIM microservice with a standard API interface that can be deployed anywhere.
Microsoft also announced additional models to the Phi-3 family are coming soon to offer more choice across quality and cost. Phi-3-small (7 billion parameters) and Phi-3-medium (14 billion parameters) will be available in the Azure AI Model Catalog and other model gardens shortly.
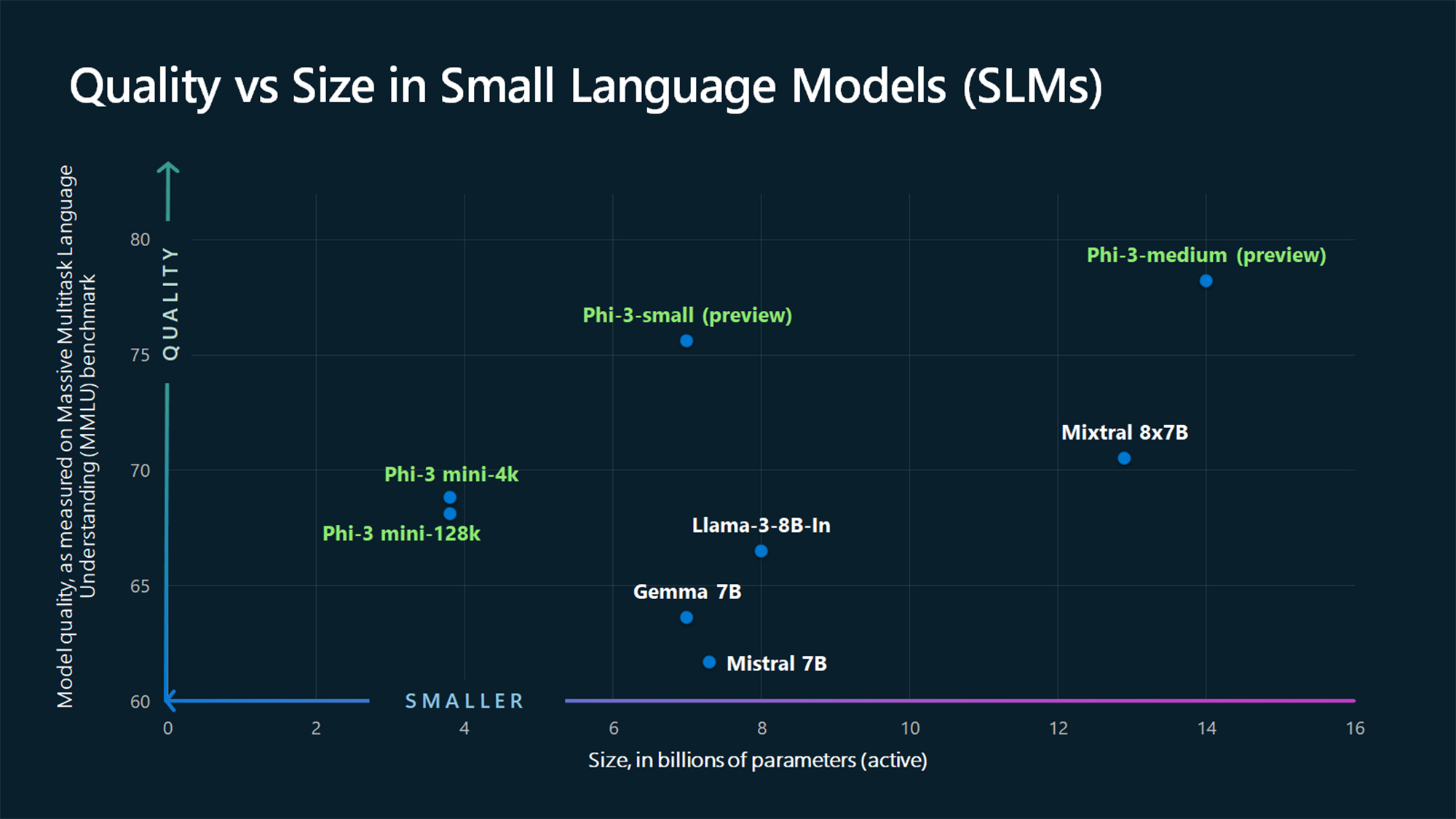
Small language models are designed to perform well for simpler tasks, are more accessible and easier to use for organizations with limited resources and they can be more easily fine-tuned to meet specific needs.
“What we’re going to start to see is not a shift from large to small, but a shift from a singular category of models to a portfolio of models where customers get the ability to make a decision on what is the best model for their scenario,” said Sonali Yadav, principal product manager for Generative AI at Microsoft.
“Some customers may only need small models, some will need big models and many are going to want to combine both in a variety of ways,” said Luis Vargas, vice president of AI at Microsoft.
Choosing the right language model depends on an organization’s specific needs, the complexity of the task and available resources. Small language models are well suited for organizations looking to build applications that can run locally on a device (as opposed to the cloud) and where a task doesn’t require extensive reasoning or a quick response is needed.
Large language models are more suited for applications that need orchestration of complex tasks involving advanced reasoning, data analysis and understanding of context.
Small language models also offer potential solutions for regulated industries and sectors that encounter situations where they need high quality results but want to keep data on their own premises, said Yadav.
Vargas and Yadav are particularly excited about the opportunities to place more capable SLMs on smartphones and other mobile devices that operate “at the edge,” not connected to the cloud. (Think of car computers, PCs without Wi-Fi, traffic systems, smart sensors on a factory floor, remote cameras or devices that monitor environmental compliance.) By keeping data within the device, users can “minimize latency and maximize privacy,” said Vargas.
Latency refers to the delay that can occur when LLMs communicate with the cloud to retrieve information used to generate answers to users prompts. In some instances, high-quality answers are worth waiting for while in other scenarios speed is more important to user satisfaction.
Because SLMs can work offline, more people will be able to put AI to work in ways that haven’t previously been possible, Vargas said.
For instance, SLMs could also be put to use in rural areas that lack cell service. Consider a farmer inspecting crops who finds signs of disease on a leaf or branch. Using a SLM with visual capability, the farmer could take a picture of the crop at issue and get immediate recommendations on how to treat pests or disease.
“If you are in a part of the world that doesn’t have a good network,” said Vargas, “you are still going to be able to have AI experiences on your device.”
The role of high-quality data
Just as the name implies, compared to LLMs, SLMs are tiny, at least by AI standards. Phi-3-mini has “only” 3.8 billion parameters – a unit of measure that refers to the algorithmic knobs on a model that help determine its output. By contrast, the biggest large language models are many orders of magnitude larger.
The huge advances in generative AI ushered in by large language models were largely thought to be enabled by their sheer size. But the Microsoft team was able to develop small language models that can deliver outsized results in a tiny package. This breakthrough was enabled by a highly selective approach to training data – which is where children’s books come into play.
To date, the standard way to train large language models has been to use massive amounts of data from the internet. This was thought to be the only way to meet this type of model’s huge appetite for content, which it needs to “learn” to understand the nuances of language and generate intelligent answers to user prompts. But Microsoft researchers had a different idea.
“Instead of training on just raw web data, why don’t you look for data which is of extremely high quality?” asked Sebastien Bubeck, Microsoft vice president of generative AI research who has led the company’s efforts to develop more capable small language models. But where to focus?
Inspired by Eldan’s nightly reading ritual with his daughter, Microsoft researchers decided to create a discrete dataset starting with 3,000 words – including a roughly equal number of nouns, verbs and adjectives. Then they asked a large language model to create a children’s story using one noun, one verb and one adjective from the list – a prompt they repeated millions of times over several days, generating millions of tiny children’s stories.
They dubbed the resulting dataset “TinyStories” and used it to train very small language models of around 10 million parameters. To their surprise, when prompted to create its own stories, the small language model trained on TinyStories generated fluent narratives with perfect grammar.
Next, they took their experiment up a grade, so to speak. This time a bigger group of researchers used carefully selected publicly-available data that was filtered based on educational value and content quality to train Phi-1. After collecting publicly available information into an initial dataset, they used a prompting and seeding formula inspired by the one used for TinyStories, but took it one step further and made it more sophisticated, so that it would capture a wider scope of data. To ensure high quality, they repeatedly filtered the resulting content before feeding it back into a LLM for further synthesizing. In this way, over several weeks, they built up a corpus of data large enough to train a more capable SLM.
“A lot of care goes into producing these synthetic data,” Bubeck said, referring to data generated by AI, “looking over it, making sure it makes sense, filtering it out. We don’t take everything that we produce.” They dubbed this dataset “CodeTextbook.”
The researchers further enhanced the dataset by approaching data selection like a teacher breaking down difficult concepts for a student. “Because it’s reading from textbook-like material, from quality documents that explain things very, very well,” said Bubeck, “you make the task of the language model to read and understand this material much easier.”
Distinguishing between high- and low-quality information isn’t difficult for a human, but sorting through more than a terabyte of data that Microsoft researchers determined they would need to train their SLM would be impossible without help from a LLM.
“The power of the current generation of large language models is really an enabler that we didn’t have before in terms of synthetic data generation,” said Ece Kamar, a Microsoft vice president who leads the Microsoft Research AI Frontiers Lab, where the new training approach was developed.
Starting with carefully selected data helps reduce the likelihood of models returning unwanted or inappropriate responses, but it’s not sufficient to guard against all potential safety challenges. As with all generative AI model releases, Microsoft’s product and responsible AI teams used a multi-layered approach to manage and mitigate risks in developing Phi-3 models.
For instance, after initial training they provided additional examples and feedback on how the models should ideally respond, which builds in an additional safety layer and helps the model generate high-quality results. Each model also undergoes assessment, testing and manual red-teaming, in which experts identify and address potential vulnerabilities.
Finally, developers using the Phi-3 model family can also take advantage of a suite of tools available in Azure AI to help them build safer and more trustworthy applications.
Choosing the right-size language model for the right task
But even small language models trained on high quality data have limitations. They are not designed for in-depth knowledge retrieval, where large language models excel due to their greater capacity and training using much larger data sets.
LLMs are better than SLMs at complex reasoning over large amounts of information due to their size and processing power. That’s a function that could be relevant for drug discovery, for example, by helping to pore through vast stores of scientific papers, analyze complex patterns and understand interactions between genes, proteins or chemicals.
“Anything that involves things like planning where you have a task, and the task is complicated enough that you need to figure out how to partition that task into a set of sub tasks, and sometimes sub-sub tasks, and then execute through all of those to come with a final answer … are really going to be in the domain of large models for a while,” said Vargas.
Based on ongoing conversations with customers, Vargas and Yadav expect to see some companies “offloading” some tasks to small models if the task is not too complex.
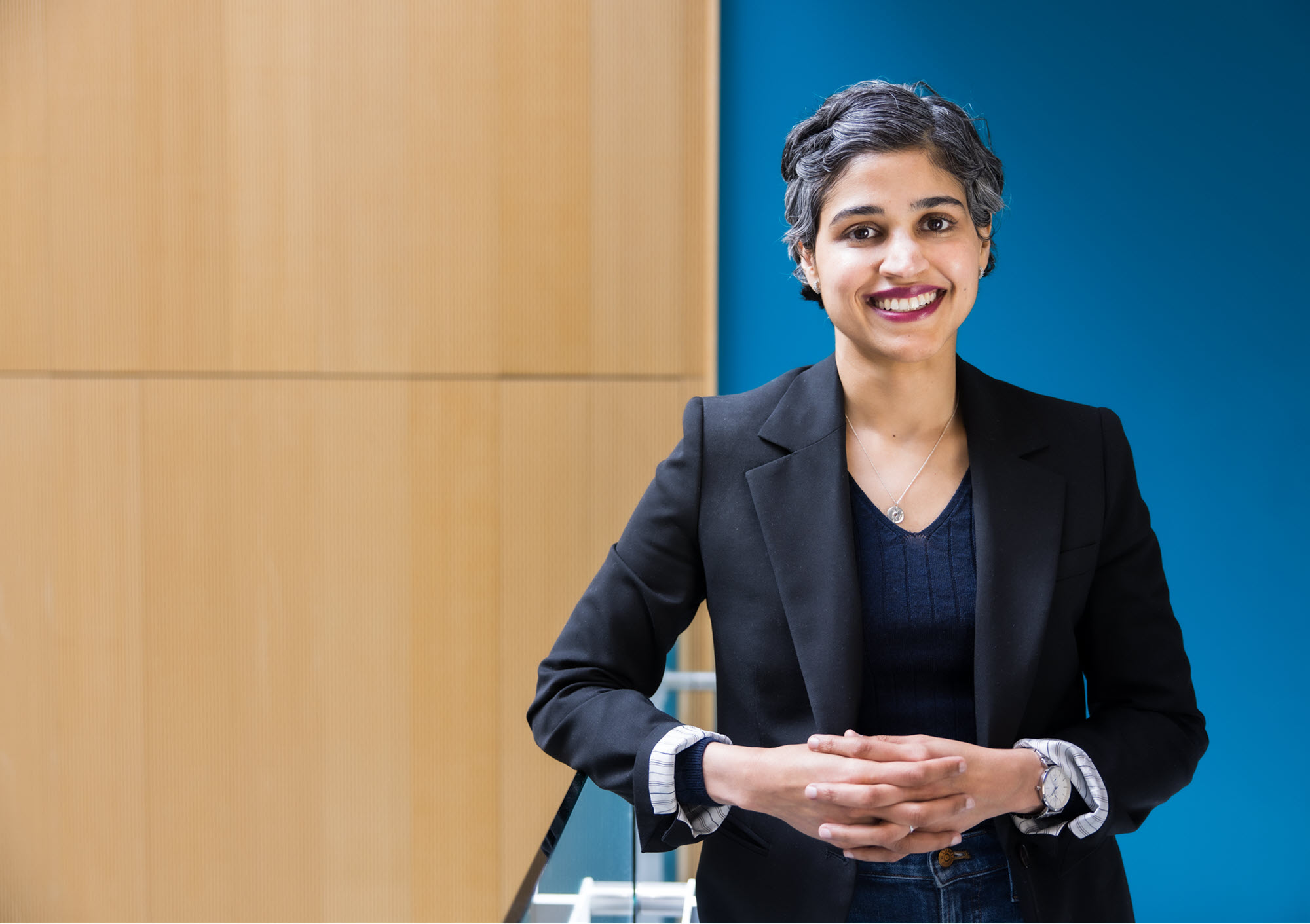
For instance, a business could use Phi-3 to summarize the main points of a long document or extract relevant insights and industry trends from market research reports. Another organization might use Phi-3 to generate copy, helping create content for marketing or sales teams such as product descriptions or social media posts. Or, a company might use Phi-3 to power a support chatbot to answer customers’ basic questions about their plan, or service upgrades.
Internally, Microsoft is already using suites of models, where large language models play the role of router, to direct certain queries that require less computing power to small language models, while tackling other more complex requests itself.
“The claim here is not that SLMs are going to substitute or replace large language models,” said Kamar. Instead, SLMs “are uniquely positioned for computation on the edge, computation on the device, computations where you don’t need to go to the cloud to get things done. That’s why it is important for us to understand the strengths and weaknesses of this model portfolio.”
And size carries important advantages. There’s still a gap between small language models and the level of intelligence that you can get from the big models on the cloud, said Bubeck. “And maybe there will always be a gap because you know – the big models are going to keep making progress.”
Related links:
- Read more: Introducing Phi-3, redefining what’s possible with SLMs
- Learn more: Azure AI
- Read more: Phi-3 Technical Report: A Highly Capable Language Model Locally on Your Phone
Top image: Sebastien Bubeck, Microsoft vice president of Generative AI research who has led the company’s efforts to develop more capable small language models. (Photo by Dan DeLong for Microsoft)
Main Navigation
- Contact NeurIPS
- Code of Ethics
- Code of Conduct
- Create Profile
- Journal To Conference Track
- Diversity & Inclusion
- Proceedings
- Future Meetings
- Exhibitor Information
- Privacy Policy
NeurIPS 2024
Conference Dates: (In person) 9 December - 15 December, 2024
Homepage: https://neurips.cc/Conferences/2024/
Call For Papers
Author notification: Sep 25, 2024
Camera-ready, poster, and video submission: Oct 30, 2024 AOE
Submit at: https://openreview.net/group?id=NeurIPS.cc/2024/Conference
The site will start accepting submissions on Apr 22, 2024
Subscribe to these and other dates on the 2024 dates page .
The Thirty-Eighth Annual Conference on Neural Information Processing Systems (NeurIPS 2024) is an interdisciplinary conference that brings together researchers in machine learning, neuroscience, statistics, optimization, computer vision, natural language processing, life sciences, natural sciences, social sciences, and other adjacent fields. We invite submissions presenting new and original research on topics including but not limited to the following:
- Applications (e.g., vision, language, speech and audio, Creative AI)
- Deep learning (e.g., architectures, generative models, optimization for deep networks, foundation models, LLMs)
- Evaluation (e.g., methodology, meta studies, replicability and validity, human-in-the-loop)
- General machine learning (supervised, unsupervised, online, active, etc.)
- Infrastructure (e.g., libraries, improved implementation and scalability, distributed solutions)
- Machine learning for sciences (e.g. climate, health, life sciences, physics, social sciences)
- Neuroscience and cognitive science (e.g., neural coding, brain-computer interfaces)
- Optimization (e.g., convex and non-convex, stochastic, robust)
- Probabilistic methods (e.g., variational inference, causal inference, Gaussian processes)
- Reinforcement learning (e.g., decision and control, planning, hierarchical RL, robotics)
- Social and economic aspects of machine learning (e.g., fairness, interpretability, human-AI interaction, privacy, safety, strategic behavior)
- Theory (e.g., control theory, learning theory, algorithmic game theory)
Machine learning is a rapidly evolving field, and so we welcome interdisciplinary submissions that do not fit neatly into existing categories.
Authors are asked to confirm that their submissions accord with the NeurIPS code of conduct .
Formatting instructions: All submissions must be in PDF format, and in a single PDF file include, in this order:
- The submitted paper
- Technical appendices that support the paper with additional proofs, derivations, or results
- The NeurIPS paper checklist
Other supplementary materials such as data and code can be uploaded as a ZIP file
The main text of a submitted paper is limited to nine content pages , including all figures and tables. Additional pages containing references don’t count as content pages. If your submission is accepted, you will be allowed an additional content page for the camera-ready version.
The main text and references may be followed by technical appendices, for which there is no page limit.
The maximum file size for a full submission, which includes technical appendices, is 50MB.
Authors are encouraged to submit a separate ZIP file that contains further supplementary material like data or source code, when applicable.
You must format your submission using the NeurIPS 2024 LaTeX style file which includes a “preprint” option for non-anonymous preprints posted online. Submissions that violate the NeurIPS style (e.g., by decreasing margins or font sizes) or page limits may be rejected without further review. Papers may be rejected without consideration of their merits if they fail to meet the submission requiremhttps://www.overleaf.com/read/kcffhyrygkqc#85f742ents, as described in this document.
Paper checklist: In order to improve the rigor and transparency of research submitted to and published at NeurIPS, authors are required to complete a paper checklist . The paper checklist is intended to help authors reflect on a wide variety of issues relating to responsible machine learning research, including reproducibility, transparency, research ethics, and societal impact. The checklist forms part of the paper submission, but does not count towards the page limit.
Supplementary material: While all technical appendices should be included as part of the main paper submission PDF, authors may submit up to 100MB of supplementary material, such as data, or source code in a ZIP format. Supplementary material should be material created by the authors that directly supports the submission content. Like submissions, supplementary material must be anonymized. Looking at supplementary material is at the discretion of the reviewers.
We encourage authors to upload their code and data as part of their supplementary material in order to help reviewers assess the quality of the work. Check the policy as well as code submission guidelines and templates for further details.
Use of Large Language Models (LLMs): We welcome authors to use any tool that is suitable for preparing high-quality papers and research. However, we ask authors to keep in mind two important criteria. First, we expect papers to fully describe their methodology, and any tool that is important to that methodology, including the use of LLMs, should be described also. For example, authors should mention tools (including LLMs) that were used for data processing or filtering, visualization, facilitating or running experiments, and proving theorems. It may also be advisable to describe the use of LLMs in implementing the method (if this corresponds to an important, original, or non-standard component of the approach). Second, authors are responsible for the entire content of the paper, including all text and figures, so while authors are welcome to use any tool they wish for writing the paper, they must ensure that all text is correct and original.
Double-blind reviewing: All submissions must be anonymized and may not contain any identifying information that may violate the double-blind reviewing policy. This policy applies to any supplementary or linked material as well, including code. If you are including links to any external material, it is your responsibility to guarantee anonymous browsing. Please do not include acknowledgements at submission time. If you need to cite one of your own papers, you should do so with adequate anonymization to preserve double-blind reviewing. For instance, write “In the previous work of Smith et al. [1]…” rather than “In our previous work [1]...”). If you need to cite one of your own papers that is in submission to NeurIPS and not available as a non-anonymous preprint, then include a copy of the cited anonymized submission in the supplementary material and write “Anonymous et al. [1] concurrently show...”). Any papers found to be violating this policy will be rejected.
OpenReview: We are using OpenReview to manage submissions. The reviews and author responses will not be public initially (but may be made public later, see below). As in previous years, submissions under review will be visible only to their assigned program committee. We will not be soliciting comments from the general public during the reviewing process. Anyone who plans to submit a paper as an author or a co-author will need to create (or update) their OpenReview profile by the full paper submission deadline. Your OpenReview profile can be edited by logging in and clicking on your name in https://openreview.net/ . This takes you to a URL "https://openreview.net/profile?id=~[Firstname]_[Lastname][n]" where the last part is your profile name, e.g., ~Wei_Zhang1. The OpenReview profiles must be up to date, with all publications by the authors, and their current affiliations. The easiest way to import publications is through DBLP but it is not required, see FAQ . Submissions without updated OpenReview profiles will be desk rejected. The information entered in the profile is critical for ensuring that conflicts of interest and reviewer matching are handled properly. Because of the rapid growth of NeurIPS, we request that all authors help with reviewing papers, if asked to do so. We need everyone’s help in maintaining the high scientific quality of NeurIPS.
Please be aware that OpenReview has a moderation policy for newly created profiles: New profiles created without an institutional email will go through a moderation process that can take up to two weeks. New profiles created with an institutional email will be activated automatically.
Venue home page: https://openreview.net/group?id=NeurIPS.cc/2024/Conference
If you have any questions, please refer to the FAQ: https://openreview.net/faq
Ethics review: Reviewers and ACs may flag submissions for ethics review . Flagged submissions will be sent to an ethics review committee for comments. Comments from ethics reviewers will be considered by the primary reviewers and AC as part of their deliberation. They will also be visible to authors, who will have an opportunity to respond. Ethics reviewers do not have the authority to reject papers, but in extreme cases papers may be rejected by the program chairs on ethical grounds, regardless of scientific quality or contribution.
Preprints: The existence of non-anonymous preprints (on arXiv or other online repositories, personal websites, social media) will not result in rejection. If you choose to use the NeurIPS style for the preprint version, you must use the “preprint” option rather than the “final” option. Reviewers will be instructed not to actively look for such preprints, but encountering them will not constitute a conflict of interest. Authors may submit anonymized work to NeurIPS that is already available as a preprint (e.g., on arXiv) without citing it. Note that public versions of the submission should not say "Under review at NeurIPS" or similar.
Dual submissions: Submissions that are substantially similar to papers that the authors have previously published or submitted in parallel to other peer-reviewed venues with proceedings or journals may not be submitted to NeurIPS. Papers previously presented at workshops are permitted, so long as they did not appear in a conference proceedings (e.g., CVPRW proceedings), a journal or a book. NeurIPS coordinates with other conferences to identify dual submissions. The NeurIPS policy on dual submissions applies for the entire duration of the reviewing process. Slicing contributions too thinly is discouraged. The reviewing process will treat any other submission by an overlapping set of authors as prior work. If publishing one would render the other too incremental, both may be rejected.
Anti-collusion: NeurIPS does not tolerate any collusion whereby authors secretly cooperate with reviewers, ACs or SACs to obtain favorable reviews.
Author responses: Authors will have one week to view and respond to initial reviews. Author responses may not contain any identifying information that may violate the double-blind reviewing policy. Authors may not submit revisions of their paper or supplemental material, but may post their responses as a discussion in OpenReview. This is to reduce the burden on authors to have to revise their paper in a rush during the short rebuttal period.
After the initial response period, authors will be able to respond to any further reviewer/AC questions and comments by posting on the submission’s forum page. The program chairs reserve the right to solicit additional reviews after the initial author response period. These reviews will become visible to the authors as they are added to OpenReview, and authors will have a chance to respond to them.
After the notification deadline, accepted and opted-in rejected papers will be made public and open for non-anonymous public commenting. Their anonymous reviews, meta-reviews, author responses and reviewer responses will also be made public. Authors of rejected papers will have two weeks after the notification deadline to opt in to make their deanonymized rejected papers public in OpenReview. These papers are not counted as NeurIPS publications and will be shown as rejected in OpenReview.
Publication of accepted submissions: Reviews, meta-reviews, and any discussion with the authors will be made public for accepted papers (but reviewer, area chair, and senior area chair identities will remain anonymous). Camera-ready papers will be due in advance of the conference. All camera-ready papers must include a funding disclosure . We strongly encourage accompanying code and data to be submitted with accepted papers when appropriate, as per the code submission policy . Authors will be allowed to make minor changes for a short period of time after the conference.
Contemporaneous Work: For the purpose of the reviewing process, papers that appeared online within two months of a submission will generally be considered "contemporaneous" in the sense that the submission will not be rejected on the basis of the comparison to contemporaneous work. Authors are still expected to cite and discuss contemporaneous work and perform empirical comparisons to the degree feasible. Any paper that influenced the submission is considered prior work and must be cited and discussed as such. Submissions that are very similar to contemporaneous work will undergo additional scrutiny to prevent cases of plagiarism and missing credit to prior work.
Plagiarism is prohibited by the NeurIPS Code of Conduct .
Other Tracks: Similarly to earlier years, we will host multiple tracks, such as datasets, competitions, tutorials as well as workshops, in addition to the main track for which this call for papers is intended. See the conference homepage for updates and calls for participation in these tracks.
Experiments: As in past years, the program chairs will be measuring the quality and effectiveness of the review process via randomized controlled experiments. All experiments are independently reviewed and approved by an Institutional Review Board (IRB).
Financial Aid: Each paper may designate up to one (1) NeurIPS.cc account email address of a corresponding student author who confirms that they would need the support to attend the conference, and agrees to volunteer if they get selected. To be considered for Financial the student will also need to fill out the Financial Aid application when it becomes available.
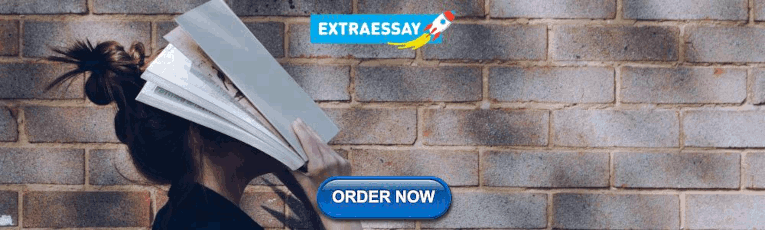
The Evolution of Black-White Differences in Occupational Mobility Across Post-Civil War America

This paper studies long-run differences in intergenerational occupational mobility between Black and White Americans. Combining data from linked historical censuses and contemporary large-scale surveys, we provide a comprehensive set of mobility measures based on Markov chains that trace the short-and long-run dynamics of occupational differences. Our findings highlight the unique importance of changes in mobility experienced by the 1940–1950 birth cohort in shaping the current occupational distribution and reducing the racial occupational gap. We further explore the properties of continuing occupational inequalities and argue that these disparities are better understood by a lack of exchange mobility rather than structural mobility. Thus, contemporary occupational disparities cannot be expected to disappear based on the occupational dynamics seen historically.
More Research From These Scholars
A trajectories-based approach to measuring intergenerational mobility, the great gatsby curve, everybody’s talkin’ at me: levels of majority language acquisition by minority language speakers.
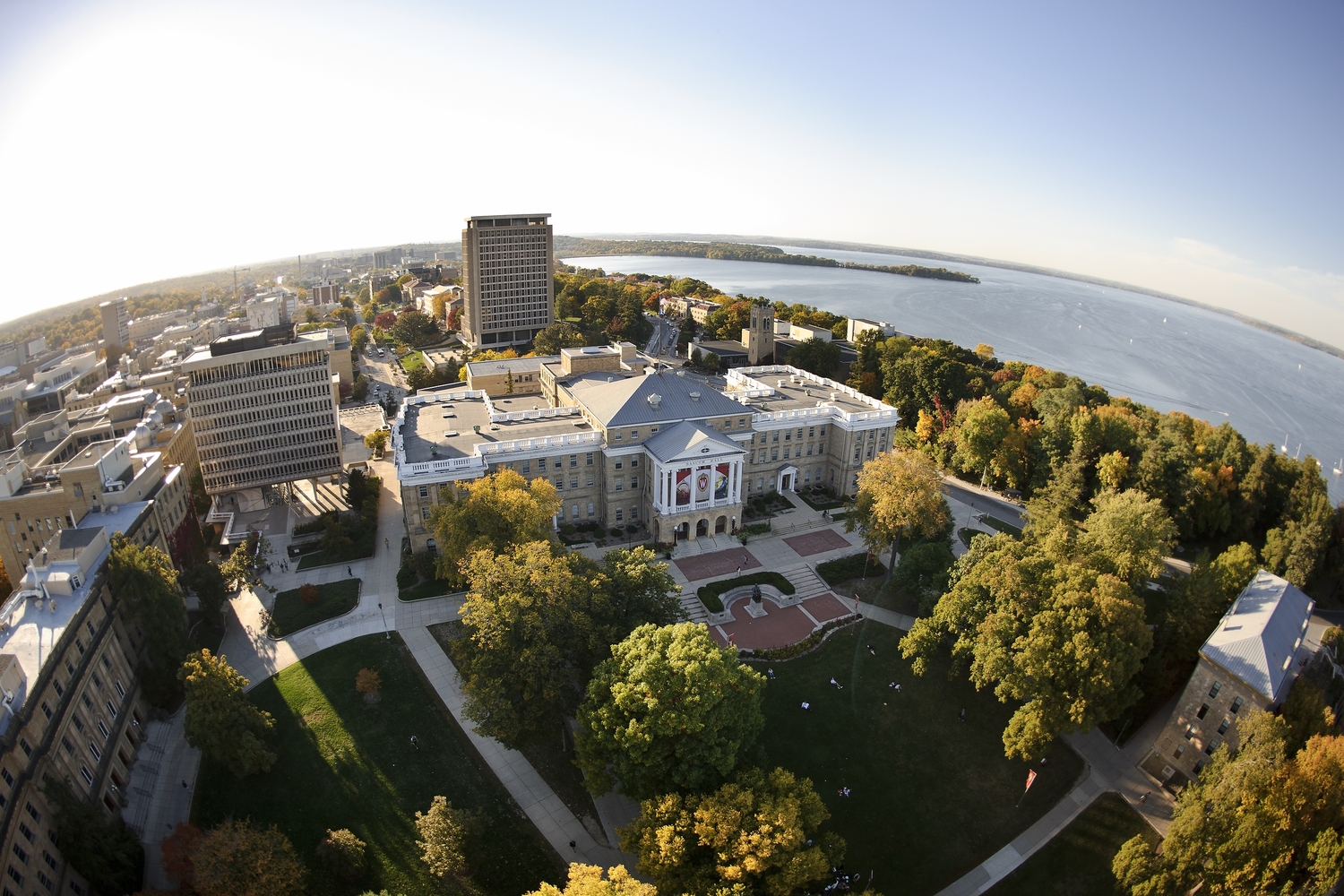
WIDA Assistant Director of Standards
- Madison, Wisconsin
- SCHOOL OF EDUCATION/WIS CENTER FOR EDUCATION RESCH-GEN
- Staff-Full Time
- Opening at: Apr 30 2024 at 14:20 CDT
- Closing at: May 22 2024 at 23:55 CDT
Job Summary:
The WIDA Assistant Director of Standards position is located within the Educator Learning, Research, and Practice (ELRP) team and will report to the Director of Professional Learning Curriculum. This position will be responsible for managing the roadmap for WIDA's English Language Development (ELD) and Spanish Language Development (SLD) standards frameworks, including professional learning content and implementation resources to support educators. The Assistant Director of Standards will manage a team of content developers and work cross-functionally to support research-based updates to the standards frameworks and their associated resources. For more detailed job expectations, please visit our website: https://wida.wisc.edu/about/careers . Working fully remote is an option for this position within the United States (working remotely internationally is not an option). Requests to work remotely would need to be reviewed based on the UW and School of Education (SoE) remote work policies and go through the SoE implementation process. To learn more about these policies, visit https://businessoffice.education.wisc.edu/human-resources/remote-work/ . WIDA values linguistic and cultural diversity both as an end and a means for success in the field of education. As such, we strongly encourage applications from a diverse pool of candidates. For more information on WIDA's Mission and Values, please visit https://wida.wisc.edu/about/mission-history
Responsibilities:
- 30% Plans and directs the day-to-day operational activities of one or multiple research programs or units according to established research objectives in alignment with strategic plans and initiatives
- 20% Assists in the development, coordination, and facilitation of trainings and workshops for internal and external audiences to disseminate research program developments and information
- 10% Plans, develops, and implements processes and protocols to support research aims
- 10% Exercises supervisory authority, including hiring, transferring, suspending, promoting, managing conduct and performance, discharging, assigning, rewarding, disciplining, and/or approving hours worked of at least 2.0 full-time equivalent (FTE) employees
- 20% Serves as a unit liaison and subject matter expert among internal and external stakeholder groups, collaborates across disciplines and functional areas, provides program information, and promotes the accomplishments and developments of scholars and research initiatives
- 5% Monitors program budget(s) and approves unit expenditures
- 5% Develops policies, procedures, and institutional agreements on behalf of the program
Institutional Statement on Diversity:
Diversity is a source of strength, creativity, and innovation for UW-Madison. We value the contributions of each person and respect the profound ways their identity, culture, background, experience, status, abilities, and opinion enrich the university community. We commit ourselves to the pursuit of excellence in teaching, research, outreach, and diversity as inextricably linked goals. The University of Wisconsin-Madison fulfills its public mission by creating a welcoming and inclusive community for people from every background - people who as students, faculty, and staff serve Wisconsin and the world. For more information on diversity and inclusion on campus, please visit: Diversity and Inclusion
Required Master's Degree in education or a related field.
Qualifications:
Required * Minimum 3 years of experience in K-12 curriculum development and/or the development and alignment of K-12 standards. * Demonstrated knowledge of the field of second language acquisition, including the use of English language development (ELD) and Spanish language development (SLD) standards, the role of academic language in content learning, and research-based instructional practices. * Experience in the development of organizational systems, ongoing strategic planning, administration, and management of projects, deadlines, and budgets. * Experience with the development of professional learning content for educators across various mediums. * Superior writing, communication, and organizational skills. * Proficiency in using digital tools for coordinating workflow and documenting plans and outcomes. * Experience supervising and coaching a team and contributing to strategic planning. * Multilingual or bilingual/biliterate in Spanish. Preferred * Familiarity with the WIDA English Language Development (ELD) and Spanish Language Development (SLD) standards. * Familiarity with Universal Design for Learning and andragogy/adult learning. * Direct K-12 classroom experience supporting multilingual learners.
Full Time: 100% It is anticipated this position will be remote and requires work be performed at an offsite, non-campus work location.
Appointment Type, Duration:
Ongoing/Renewable
Minimum $90,000 ANNUAL (12 months) Depending on Qualifications This position offers a comprehensive benefits package, including generous paid time off, competitively priced health/dental/vision/life insurance, tax-advantaged savings accounts, and participation in the nationally recognized Wisconsin Retirement System (WRS) pension fund. For a summary of benefits, please see: https://hr.wisc.edu/benefits/ https://www.wisconsin.edu/ohrwd/benefits/download/fasl.pdf .
Additional Information:
What is WIDA? For nearly 20 years, WIDA has provided a trusted, comprehensive approach to supporting, teaching, and assessing multilingual learners. We are an educational services organization that advances language development and academic achievement for multilingual children in early childhood and grades K-12. In short, we provide educators around the world with high-quality language standards, assessments, professional learning, and research. We are proud to be a part of the Wisconsin Center for Education Research (WCER) within UW-Madison's nationally ranked School of Education. Visit https://wida.wisc.edu/about/careers and watch our Introduction to WIDA video to see why WIDA is an incredible place to work! The Wisconsin Center for Education Research (WCER), established in 1964, is one of the first, most productive, and largest university-based education research and development centers in the world. WCER's researchers and staff work to make teaching and learning as effective as possible for all ages and all people. WCER's mission is to improve educational outcomes for diverse student populations, impact education practice positively and foster collaborations among academic disciplines and practitioners. To this end, our center helps scholars and practitioners develop, submit, conduct, and share grant-funded education research. WCER's Commitment to Diversity, Equity & Inclusion: Diversity is a source of strength, creativity, and innovation for UW-Madison and The Wisconsin Center for Education Research (WCER). Individual differences and group diversity inspire creative and equitable outcomes. WCER actively affirms values and seeks to increase diversity in our everyday interactions, practices, and policies. For more information and news about our center, please go to https://wcer.wisc.edu/ .
How to Apply:
Please click on the "Apply Now" button to start the application process. As part of the application process, you will be required to submit: - A cover letter describing how your experience and qualifications meet the requirements of this position. - A current resume or CV. - A list of at least three professional references, including contact information. A successful applicant will be responsible for ensuring eligibility for employment in the United States on or before the effective date of the appointment.
Kelly Krahenbuhl [email protected] 608-263-4776 Relay Access (WTRS): 7-1-1. See RELAY_SERVICE for further information.
Official Title:
Research Program Manager(RE046)
Department(s):
A17-SCHOOL OF EDUCATION/WCER
Employment Class:
Academic Staff-Renewable
Job Number:
The university of wisconsin-madison is an equal opportunity and affirmative action employer..
You will be redirected to the application to launch your career momentarily. Thank you!
Frequently Asked Questions
Applicant Tutorial
Disability Accommodations
Pay Transparency Policy Statement
Refer a Friend
You've sent this job to a friend!
Website feedback, questions or accessibility issues: [email protected] .
Learn more about accessibility at UW–Madison .
© 2016–2024 Board of Regents of the University of Wisconsin System • Privacy Statement
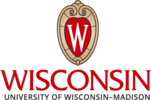
We use cookies on this site. By continuing to browse without changing your browser settings to block or delete cookies, you agree to the UW–Madison Privacy Notice .
Help | Advanced Search
Computer Science > Machine Learning
Title: mer 2024: semi-supervised learning, noise robustness, and open-vocabulary multimodal emotion recognition.
Abstract: Multimodal emotion recognition is an important research topic in artificial intelligence. Over the past few decades, researchers have made remarkable progress by increasing dataset size and building more effective architectures. However, due to various reasons (such as complex environments and inaccurate labels), current systems still cannot meet the demands of practical applications. Therefore, we plan to organize a series of challenges around emotion recognition to further promote the development of this field. Last year, we launched MER2023, focusing on three topics: multi-label learning, noise robustness, and semi-supervised learning. This year, we continue to organize MER2024. In addition to expanding the dataset size, we introduce a new track around open-vocabulary emotion recognition. The main consideration for this track is that existing datasets often fix the label space and use majority voting to enhance annotator consistency, but this process may limit the model's ability to describe subtle emotions. In this track, we encourage participants to generate any number of labels in any category, aiming to describe the emotional state as accurately as possible. Our baseline is based on MERTools and the code is available at: this https URL .
Submission history
Access paper:.
- HTML (experimental)
- Other Formats
References & Citations
- Google Scholar
- Semantic Scholar
BibTeX formatted citation

Bibliographic and Citation Tools
Code, data and media associated with this article, recommenders and search tools.
- Institution
arXivLabs: experimental projects with community collaborators
arXivLabs is a framework that allows collaborators to develop and share new arXiv features directly on our website.
Both individuals and organizations that work with arXivLabs have embraced and accepted our values of openness, community, excellence, and user data privacy. arXiv is committed to these values and only works with partners that adhere to them.
Have an idea for a project that will add value for arXiv's community? Learn more about arXivLabs .
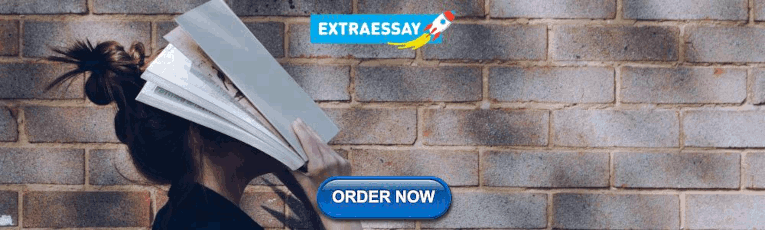
IMAGES
VIDEO
COMMENTS
In the present paper, we will use the terms interchangeably.) ... On the issue of completeness in second language acquisition. Second Language Research. 1990; 6 (2):93-124. doi: 10.1177/026765839000600201. [Google Scholar] Sebastián-Gallés N, Echeverría S, Bosch L. The influence of initial exposure on lexical representation: Comparing ...
2.1 Second Language Acquisition Research dur ing the 1950s and 1960s. As stated above, the area of SLA did not initiate as a sepa rate area of study with its distinctiveness but as an. addition to ...
The past 20 years have seen research on language acquisition in the cognitive sciences grow immensely. The current paper offers a fairly extensive review of this literature, arguing that new cognitive theories and empirical data are perfectly consistent with core predictions a behavior analytic approach makes about language development. The review focuses on important examples of productive ...
Tools for language acquisition, such as movies, games and social networks, are explained clearly in this research paper followed by the definitions of language acquisition and its characteristics.
The research published in Language Acquisition makes a clear contribution to linguistic theory by increasing our understanding of how language is acquired. The journal focuses on the acquisition of syntax, semantics, phonology, and morphology, and considers theoretical, experimental, and computational perspectives.
Language Acquisition is an interdisciplinary journal serving the fields of first and second language acquisition. Research published in the journal addresses theoretical questions about language acquisition and language development from a variety of perspectives and a variety of methodological approaches. Studies may have implications for our ...
Second Language Research is an international peer-reviewed, quarterly journal, publishing original theory-driven research concerned with second language acquisition and second language performance. This includes both experimental studies and contributions aimed at exploring conceptual issues. In addition to providing a forum for investigators in the field of non-native language learning...
Studies in Second Language Acquisition is a refereed journal of international scope devoted to the scientific discussion of acquisition or use of non-native and heritage languages. Each volume (five issues) contains research articles of either a quantitative, qualitative, or mixed-methods nature in addition to essays on current theoretical matters.
The past 20 years have seen research on language acquisition in the cognitive sciences grow immensely. The current paper offers a fairly extensive review of this literature, arguing that new cognitive theories and empirical ... The current paper reviews recent research in developmental psycholinguistics relevant to
Language acquisition research is challenging — the intricate behavioral and cognitive foundations of speech are difficult to measure objectively. The audible components of speech, however, are quantifiable and thus provide crucial data. This practical guide synthesizes the authors' decades of experience into a comprehensive set of tools that will allow students and early career researchers ...
Abstract. This paper reviews three main theoretical perspectives on language learning and acquisition in an attempt to elucidate how people acquire their first language (L1) and learn their second language (L2). Behaviorist, Innatist and Interactionist offer different perspectives on language learning and acquisition which influence the ...
SUBMIT PAPER. Review of Educational Research. Impact Factor: 11.2 / 5-Year Impact Factor: 16.6 . JOURNAL HOMEPAGE. SUBMIT PAPER. Close ... The age-length-onset problem in research on second language acquisition among immigrants. Language Learning, 56, 671-692. Crossref. ISI. Google Scholar. Swain M., Deters P. (2007). "New" mainstream SLA ...
Abstract. Language acquisition provides a new window into the rich structure of the human language faculty and explains how it develops in interaction with its environment and the rest of human ...
Usage-based approaches to language acquisition. This paper leans towards the functionalist usage-based approaches, such as Goldberg (1995, 2006) and Tomasello (2000, M. 2003, 2008). 1 Within this strand of approaches, linguistic input is assumed to be the main factor in language acquisition, powered by pattern finding and intention-reading as ...
The field of second language acquisition (SLA) is by nature of its subject a highly interdisciplinary area of research. Learning a (foreign) language, for example, involves encoding new words, consolidating and committing them to long-term memory, and later retrieving them. All of these processes have direct parallels in the domain of human memory and have been thoroughly studied by ...
Language acquisition is based on the neuro-psychological processes (Maslo, 2007: 41). Language acquistion is opposed to learning and is a subconscious process similar to that by which children acquire their first language (Kramina, 2000: 27). Hence, language acquisition is an integral part of the unity of all language (Robbins, 2007: 49).
ABSI'RACT. After discussing the ties between language teaching and second language acquisition research, the present paper reviews the role that second language acquisition research has played on two recent pedagogical proposals. First, communicative language teaching, advocated in the early eighties, in which focus on the code was excluded ...
The purpose of this manual is to introduce the concepts, principles, and procedures of a unique field of linguistic study, that of language acquisition. Our objective is to provide an overview of scientific methods for the study of language acquisition and to present a systematic, scientifically sound approach to this study.
The paper focuses on the use of social media in the process of acquisition of the second language (L2) or foreign language learning (FLL), namely, English in university students. Recently, we have seen a dramatic change in favour of distance learning due to the COVID-19 pandemics, therefore, it could be useful to analyse the impact of social ...
Second language acquisition studies can contribute to the body of research on the influence of language on thought by examining cognitive change as a result of second language learning. We conducted a longitudinal study that examined how the acquisition of Spanish grammatical gender influences categorization in native English-speaking adults.
This paper addresses the English centrality in reading policy, assessment and instructional practices in the U.S. and its implications for the educational programing for emerging bilingual students (EBs) with disabilities. A recent review of the state of practice as it relates to EBs with disabilities reveals concerns that have endured for nearly six decades: biased assessment ...
learning a language (Rahimi, Riazi & Saif, 2008). As a result, language learning strategies "emerged not only as integral components of various theoretical models of language proficiency but also as a means of achieving learners' autonomy in the process of language learning" (p. 32). Thus, this paper focuses on the language learning ...
The recent success of large language models (LLMs) trained on static, pre-collected, general datasets has sparked numerous research directions and applications. One such direction addresses the non-trivial challenge of integrating pre-trained LLMs into dynamic data distributions, task structures, and user preferences. Pre-trained LLMs, when tailored for specific needs, often experience ...
The research paper discussed in introduces an innovative system designed for recognizing Arabic sign language through the utilization of transfer learning techniques. The proposed system comprises several crucial steps, including image acquisition, preprocessing, and feature extraction, leveraging pretrained models.
The acquisition of the German case system in a formal language learning setting has hardly been studied from a developmental point of view at all (in contrast to L1 research).
That led the Microsoft Research machine learning expert to wonder how much an AI model could learn using only words a 4-year-old could understand - and ultimately to an innovative training approach that's produced a new class of more capable small language models that promises to make AI more accessible to more people.
The paper checklist is intended to help authors reflect on a wide variety of issues relating to responsible machine learning research, including reproducibility, transparency, research ethics, and societal impact. ... Use of Large Language Models (LLMs): We welcome authors to use any tool that is suitable for preparing high-quality papers and ...
This paper studies long-run differences in intergenerational occupational mobility between Black and White Americans. Combining data from linked historical censuses and contemporary large-scale surveys, we provide a comprehensive set of mobility measures based on Markov chains that trace the short-and long-run dynamics of occupational differences.
Job Summary: The WIDA Assistant Director of Standards position is located within the Educator Learning, Research, and Practice (ELRP) team and will report to the Director of Professional Learning Curriculum. This position will be responsible for managing the roadmap for WIDA's English Language Development (ELD) and Spanish Language Development (SLD) standards frameworks, including professional ...
Multimodal emotion recognition is an important research topic in artificial intelligence. Over the past few decades, researchers have made remarkable progress by increasing dataset size and building more effective architectures. However, due to various reasons (such as complex environments and inaccurate labels), current systems still cannot meet the demands of practical applications ...