To read this content please select one of the options below:
Please note you do not have access to teaching notes, a systematic literature review on image information needs and behaviors.
Journal of Documentation
ISSN : 0022-0418
Article publication date: 30 April 2021
Issue publication date: 22 February 2022
With ready access to search engines and social media platforms, the way people find image information has evolved and diversified in the past two decades. The purpose of this paper is to provide an overview of the literature on image information needs and behaviors.
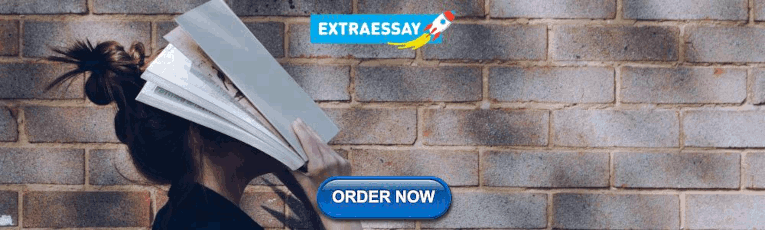
Design/methodology/approach
Following an eight-step procedure for conducting systematic literature reviews, the paper presents an analysis of peer-reviewed work on image information needs and behaviors, with publications ranging from the years 1997 to 2019.
Application of the inclusion criteria led to 69 peer-reviewed works. These works were synthesized according to the following categories: research methods, users targeted, image types, identified needs, search behaviors and search obstacles. The reviewed studies show that people seek and use images for multiple reasons, including entertainment, illustration, aesthetic appreciation, knowledge construction, engagement, inspiration and social interactions. The reviewed studies also report that common strategies for image searches include keyword searches with short queries, browsing, specialization and reformulation. Observed trends suggest common deployment of query analysis, survey questionnaires and undergraduate participant pools to research image information needs and behavior.
Originality/value
At this point, after more than two decades of image information needs research, a holistic systematic review of the literature was long overdue. The way users find image information has evolved and diversified due to technological developments in image retrieval. By synthesizing this burgeoning field into specific foci, this systematic literature review provides a foundation for future empirical investigation. With this foundation set, the paper then pinpoints key research gaps to investigate, particularly the influence of user expertise, a need for more diverse population samples, a dearth of qualitative data, new search features and information and visual literacies instruction.
- Systematic literature review (SLR)
- Image information needs
- Image information seeking behavior
- Visual information
Cho, H. , Pham, M.T.N. , Leonard, K.N. and Urban, A.C. (2022), "A systematic literature review on image information needs and behaviors", Journal of Documentation , Vol. 78 No. 2, pp. 207-227. https://doi.org/10.1108/JD-10-2020-0172
Emerald Publishing Limited
Copyright © 2021, Emerald Publishing Limited
Related articles
We’re listening — tell us what you think, something didn’t work….
Report bugs here
All feedback is valuable
Please share your general feedback
Join us on our journey
Platform update page.
Visit emeraldpublishing.com/platformupdate to discover the latest news and updates
Questions & More Information
Answers to the most commonly asked questions here
- advanced search
- submit works
- MOspace Home
- University of Missouri-Columbia
- Faculty Research, Scholarship, and Creative Works (MU)
A systematic literature review on image information needs and behaviors
![PDF file [PDF]](https://mospace.umsystem.edu/xmlui/themes/Mirage2//images/mimes_sm/pdf.png)
Collections

An official website of the United States government
The .gov means it’s official. Federal government websites often end in .gov or .mil. Before sharing sensitive information, make sure you’re on a federal government site.
The site is secure. The https:// ensures that you are connecting to the official website and that any information you provide is encrypted and transmitted securely.
- Publications
- Account settings
Preview improvements coming to the PMC website in October 2024. Learn More or Try it out now .
- Advanced Search
- Journal List
- Sensors (Basel)

Critical Analysis of the Current Medical Image-Based Processing Techniques for Automatic Disease Evaluation: Systematic Literature Review
Associated data.
The study does not report any data.
Medical image processing and analysis techniques play a significant role in diagnosing diseases. Thus, during the last decade, several noteworthy improvements in medical diagnostics have been made based on medical image processing techniques. In this article, we reviewed articles published in the most important journals and conferences that used or proposed medical image analysis techniques to diagnose diseases. Starting from four scientific databases, we applied the PRISMA technique to efficiently process and refine articles until we obtained forty research articles published in the last five years (2017–2021) aimed at answering our research questions. The medical image processing and analysis approaches were identified, examined, and discussed, including preprocessing, segmentation, feature extraction, classification, evaluation metrics, and diagnosis techniques. This article also sheds light on machine learning and deep learning approaches. We also focused on the most important medical image processing techniques used in these articles to establish the best methodologies for future approaches, discussing the most efficient ones and proposing in this way a comprehensive reference source of methods of medical image processing and analysis that can be very useful in future medical diagnosis systems.
1. Introduction
Classification methods have increased in importance and now play a significant role in image processing. Their importance stems from their applications in various fields, particularly in medicine. Given the importance of classification in medicine, new and sophisticated classification tools and methods are needed to diagnose and classify medical images efficiently [ 1 ]. Several classification algorithms encompass hundreds of different classification issues, and no single classification method can successfully and efficiently address all classification problems. As a result, answering the question concerning which classification approach is best for a particular study is challenging. The fast growth in medical data and imagery in recent years has necessitated the employment of new methodologies depending on big data technology, artificial intelligence, and machine learning in health care, making it an important research area [ 2 ]. Given the importance of classification in the medical field, new approaches for rapidly identifying and evaluating medical images are required. As a result, this research aims to compare existing and conventional methods for medical image classification and, based on these findings, suggest a novel algorithm for medical image classification [ 3 ].
The field of medical image processing and analysis has contributed to substantial medical achievements. A correct diagnosis necessitates the precise identification of each disease by integrating methods and techniques that support more effective clinical diagnosis depending on images obtained by various imaging modalities that have been used increasingly widely and successfully to detect illnesses [ 4 ]. This study aims to describe the process of medical image analysis, identify the techniques used in the analysis, and give a comprehensive literature review on illness identification based on medical imaging across various diseases and diverse fields and applications in medical imaging. This study searched for works related to the topic of the systematic literature review (SLR) and provided information about the process applied to article selection. In the final step, we kept only the forty most relevant articles that answered our research questions related to medical image processing and analysis techniques for diagnosis.
The study is organized as follows. Section 2 describes the methodology of research applied in the SLR. Section 3 provides a detailed explanation of medical imaging modalities and medical image analysis processes in the surveyed studies. Here, the image processing methods for disease diagnosis developed by the researcher are described. Various filtering and image improvement methods are discussed. Popular segmentation methods are presented, feature extraction methods are introduced, and the classification methods utilized for human disease diagnosis and evaluation metrics are discussed. Section 7 discusses the outcomes and the future work.
2. Research Methodology
This section describes the protocol utilized to locate, collect, and assess the state-of-the-art techniques under study. It is divided into four phases: research questions, research strategy, article selection criteria, and research results.
2.1. Research Questions
The SLR (Systematic Literature Review) aims to address the research questions by finding all relevant research outcomes from previous studies. The research questions are divided into five sub-questions:
Q1: What are the modalities of medical imaging?
Q2: What is the task of medical image processing and analysis?
Q3: Which medical image processing methods are most used in diagnostic systems?
Q4: What diagnostic techniques have been adopted and developed?
Q5: Is the system that has been adopted or designed capable of producing good results?
We searched various databases such as Elsevier, IEEE Explorer, Springer, and Google Scholar. We included the relevant studies that mainly focus on two or more questions based on our research questions.
2.2. Research Strategy
Our systematic literature review collected many studies related to our search topic over the last five years, between 2017 and 2021, from the following databases: Elsevier, IEEE Xplorer, Springer, and Google Scholar. We used combinations of keywords and terms, including “Medical Image Analysis”, “Medical Image Processing”, “Medical Image Processing Techniques”, “Medical Image Processing Techniques” AND “Disease Diagnosis”, and “Diagnostic Techniques” AND “Medical Image Processing”, and we obtained about 3204 articles meeting our searched keywords. After removing 206 duplicate articles, the remaining set consisted of 2998 articles. After examining these studies by their titles and abstracts, a new set of keywords were applied, including “Diseases Classification”, “Machine Learning”, “Deep Learning”, “Neural Networks”, and “Hybrid Diagnosis System”, and 2900 articles were excluded. Of the 98 articles remaining, 58 were excluded after deep reading and based on the exclusion criteria focused on articles that used machine learning and deep learning methods to create a hybrid diagnostic system based on the merging of two methods or the modification or improvement of a common method and its development for proposing possible areas for future research. These articles reached the fourth stage of our systematic exclusion technique and dealt with different topics and methods related to machine learning (ML) methods found in [ 5 , 6 , 7 , 8 , 9 , 10 , 11 , 12 , 13 , 14 , 15 , 16 , 17 , 18 , 19 , 20 , 21 , 22 , 23 , 24 , 25 , 26 , 27 , 28 , 29 , 30 , 31 , 32 ], deep learning (DL) strategies found in [ 33 , 34 , 35 , 36 , 37 , 38 , 39 , 40 , 41 , 42 , 43 , 44 , 45 , 46 , 47 , 48 , 49 , 50 , 51 , 52 , 53 , 54 ], and convolutional neural network (CNN) approaches found in [ 55 , 56 , 57 , 58 , 59 , 60 , 61 , 62 ]. Finally, 40 studies fulfilling our research criteria were obtained to be deeply analyzed. In this way, the most suitable articles were selected based on the PRISMA (Preferred Reporting Items for Systematic Reviews and Meta-Analyses) technique, as shown in Figure 1 . By applying the PRISMA technique, which is appropriate for any systematic literature review, we kept only the most relevant articles from large databases. In the final step, as shown in Figure 1 , the last article set was not only a result of the automatic selection based on keyword combinations but also represented the answers to our research questions that are discussed in Section 2.3 .

Flowchart of the process for article selection.
2.3. Criteria for Article Selection
The following criteria were determined to choose articles:
- Articles using the most up-to-date techniques for analyzing medical images.
- Articles that were written in the English language.
- Articles published in the last five years (2017–2021).
- Studies that were presented at a peer-reviewed conferences or journals.
- Following the definition of the inclusion criteria, the following exclusion criteria were determined:
- Duplicate references from the various electronic archives that were searched.
- Articles with a page count of less than four.
- Articles that fail to respond to any of the research questions.
- Articles that were written in a language other than English
- Articles that did not address the study’s goals.
2.4. Research Results
After searching the scientific databases and identifying the research results, the most relevant articles related to the research aims were found, which included articles that explored different medical image processing techniques and focused on the techniques of diagnosing diseases. The selected articles were carefully read, and the extracted results were analyzed and assessed to summarize the existing research, identify the most useful techniques, and propose possible areas for future research.
3. Results of Systematic Review
This section is divided into two parts: the first deals with medical imaging modalities, and the second deals with the analysis of medical images. Each revolves around the main objectives of the systematic review.
3.1. Medical Imaging Modalities
Medical images play a critical role in assisting health care workers in reaching patients for diagnosis and treatment. Medical image processing is a set of procedures for extracting clinically useful data from various imaging modalities for diagnosis [ 63 ]. Numerous medical imaging modalities include ionizing radiation, magnetic resonance, nuclear medicine, optical methods, and ultrasound as the media. Each modular media has unique characteristics and responses to the human body’s structure [ 64 ]. These modalities serve various purposes, such as obtaining images inside the human body or image samples of parts that cannot be seen with the naked eye [ 65 ]. The classification of medical imaging modalities and the main types of imaging methods addressed in the surveyed studies are illustrated in Figure 2 .

Classification of medical imaging modalities.
The distribution of the forty chosen studies that used different modalities is illustrated in Table 1 ; this table shows the detailed distribution of publication references, imaging modality, type of disease, and medical databases.
Distribution of studies for different medical imaging modalities.
3.2. Medical Image Analysis
This section is divided into subsections. These subsections introduce the major medical image analysis methods used in the studies reviewed, including image preprocessing, image segmentation, feature extraction to classification, and evaluation metrics.
3.2.1. Medical Image Preprocessing
Image processing is a method for enhancing the quality of an image after eliminating irrelevant image data. Medical images include many irrelevant and unwanted segments. To remove these segments in an image, some preprocessing methods are required. Image preprocessing aims to improve the quality of the images contained in the dataset, which improves the results of segmentation and feature extraction methods [ 106 ]. This section presents the preprocessing methods of the studies surveyed. One of the most important techniques to improve medical images and remove noise is filtering. One of the most important filters used is the median filter [ 69 , 73 , 87 , 91 , 92 , 95 , 99 ], with its major benefit being to preserve the edges and remove noise. In [ 71 ], the authors used the synthetic minority over-sampling technique to generate synthetic samples from minor classes rather than simply replicating them, and they used the changing perspective of images technique to expand the dataset. To generate new images, computer vision techniques such as gray scaling, blurring, enhancing contrast, changing the color channel, sharpening, minimizing noise, and smoothing were used. In [ 73 ], the authors described in their study how to utilize pixel-wise interpolation and the modified quadratic transform-based Radon transform algorithm to improve the contrast of the images. In [ 73 , 85 , 100 ], the authors applied the contrast limited adaptive histogram equalization (CLAHE) approach and morphological operations to remove noises and improve the images. In [ 76 , 81 ], the authors used a fast adaptive median filter to eliminate the noise from medical images and maintain accurate information. In [ 77 ], binarization and thinning techniques were applied to the selected images to produce better images. In [ 80 , 88 , 89 ], the authors improved the noise removal process for medical images to obtain clear images using a Weiner filter for contrast adjustment. In [ 84 ], the authors developed two methods to convert the input color images to grayscale, denoise, enhance contrast, and normalize the histogram. The first method utilized only a green channel, while the second method depended on calculating the grayscale by taking an average of red, green, and blue channels computed using certain weights.
In [ 86 , 96 , 102 ], the authors addressed the normalization method to enhance the visual quality of images. In [ 87 ] the authors used noise removal techniques such as max-min filter, midpoint filter, quantum noise filter, alpha-trimmed mean filter, impulse noise filter, and wavelet thresholding methods for noise removal from images to smoothened them to obtain better images. In [ 91 ], the authors employed linear and non-linear image processing filters such as mean, Gaussian, Log, and fuzzy filters to eliminate noise from images. In [ 92 ], the authors presented the BBHE approach (i.e., Brightness Preserving Bi- Histogram Equalization), a method that decomposes the original image into two sub-images to overcome the drawback of histogram equalization. This is accomplished using a gray-level and histogram equalization approach for each sub-image. The Gradwrap algorithm, addressed in [ 93 ], improves the image appearance by removing image distortions. The B1 non-uniformity algorithm corrects the image color and intensity information, and then the N3 bias field correlation is applied after the Gradwrap and B1 non-uniformity algorithms to correct intensity distortion. In [ 94 ], the authors used unsharp masking, a popular image sharpening method, to improve the contrast of histopathological images. In [ 97 ] the Wang–Mendel algorithm was employed to remove noise from the images; this algorithm is one of the most effective approaches for eliminating noise from medical images due to its high speed.
In [ 101 ], the authors analyzed fundus images for image scaling; R, G, and B channel selection; and the preprocessed green channel components were analyzed by the 2D-VMD technique, which has a non-stationary, non-recursive, and completely adaptive decomposition technique for signal image analysis. In [ 103 ], the authors used image normalization and data over-sampling techniques with data augmentation to enlarge the dataset so that it could be used for deep learning tasks. Data augmentation approaches use a variety of operations to images, including scaling, geometric deformation, noise addition, alterations to the lighting, and image flipping. The authors employed the straightforward and efficient semi-supervised learning technique of pseudo-label to improve the performance of deep neural network models.
3.2.2. Segmentation Techniques
Image segmentation is responsible for recognizing and outlining items of interest in input images. Automatic medical image segmentation aids in the diagnosis of diseases and the identification of pathogens. Image segmentation methods can be divided into machine learning methods such as supervised and unsupervised machine learning and classical segmentation methods such as threshold-based, edge-based, and region-based methods [ 107 ]. This section presents the segmentation methods used by the studies surveyed. In [ 69 , 73 , 74 , 76 , 77 , 81 ], the authors used thresholding. In this method, the image is partitioned into its foreground and background depending on the threshold value. In [ 69 , 96 ], the authors applied the contour technique; active contour is an active segmentation model that separates the pixels of interest from an image using energy forces and limits. In [ 79 , 91 ], the authors suggested using watershed segmentation techniques; this approach is used to separate different objects. The study [ 82 , 91 ] employed Otsu’s threshold approach to extract the object; this approach finds an optimum threshold automatically. Because of its simple calculation, Otsu’s is the most successful technique for image thresholding. The authors in [ 84 ] used the Gaussian matched filter approach with binarization realized with local entropy thresholding. This method allowed them to acquire more exact results than using only one binarization threshold. Additionally, the authors employed the crossing number algorithm or minutiae detection to extract minutiae and then automatically count their number.
The study [ 95 ] adopted a color space-based method for mammography image segmentation, followed by mathematical morphology. Post-processing mathematical morphology improved performance, including filling, closure, and other operations.
3.2.3. Feature Extraction Techniques
Feature extraction is the process of extracting meaningful data from raw data. It is crucial in image processing as it enhances the image’s quality by reducing the dimensionality of the image, extracting the unique features, and transforming the input data into a set of features that are used for classification purposes. There are various features such as color, texture, and shape, and each type has several methods to extract features from medical images [ 108 ]. The distribution of the chosen studies that used different feature extraction and reduction methods is illustrated in Table 2 ; this table comprises the detailed distribution of publication references, the type of features, and the method used.
Distribution of studies for different feature extraction methods.
3.2.4. Classification Techniques
Image classification is a difficult task in image analysis. The primary purpose of medical image classification is to accurately establish which parts of the human body are infected with the disease [ 109 ]. This study reviews the chosen studies that used the newest classification techniques for medical image classification. The study [ 67 ] proposed a new classifier depending on KPCA and SVM. The support vector machine with kernel principal component analysis (SVM-KPCA) approach was designed to classify the images, and the proposed method achieved effective results, which gave 100% accuracy, 100% sensitivity, and 100% specificity. In the study [ 68 ], the authors suggested a hybrid approach consisting of a radial basis function neural network (RBFNN) to classify brain MRI images, the accuracy of this model varied from 80% to 90%.
The study [ 70 ] developed a neural-based detection system by employing skin imaging for two different skin disorders. The ANN was trained using the non-dominated sorting genetic algorithm-II, a well-known multi-objective optimization technique (NN-NSGA-II). The proposed model was compared to two well-known metaheuristic-based classifiers, NN-PSO (ANN trained with PSO) and NN-CS (ANN trained with Cuckoo Search). The proposed bag-of-features enabled the N-NSGA-II model and obtained 90.56% accuracy, 88.26% precision, 93.64% recall, and 90.87% F-measure with the experimental data, indicating its superiority over other models. The study [ 71 ] applied multiple artificial intelligence (AI) techniques, such as the convolutional neural network and support vector machine, which were combined with image processing tools to construct a superior structure; the accuracy attained after training with CNN alone was approximately 91%, which was raised to approximately 95.3% when combined with the SVM. In [ 79 ], the authors developed a new SVM-FA (support vector machine optimized with firefly technique) classifier for diagnosing lung cancer in CT images where the SVM classifier, optimized with the firefly technique, was applied to the preprocessed data. A comparative analysis was conducted between the proposed work, traditional work, and the SVM classifier to assess the competence level of the proposed SVM-FA technique. The suggested work was successful and efficient, achieving an accuracy of 96 % and specificity of 83.3%.
The study [ 86 ] applied a deep neural network (DNN) with the rectified Adam optimizer to detect Alzheimer’s disease in MRI images. The experimental outcomes showed that DNN with the rectified Adam optimizer outperformed the existing work, landmark-based features with the SVM classifier, with a 16% classification accuracy. In a study [ 92 ], the authors addressed a combination of MLC (maximum likelihood classifier) and SVM (support vector machine) classifiers for the classification and diagnosis of DR (diabetic retinopathy) disease in the fundus images. The researchers utilized these classifiers to raise the performance level, and the suggested approach demonstrated high accuracy (98.60%), sensitivity (99%), and specificity (99%). In [ 95 ], the authors introduced an individual classifier called: feed-forward ANN (FF-ANN) and two hybrid classifiers, namely: random subspace with random forest (RSwithRF) and random subspace with Bayesian network (RSwithBN), for the classification of MRI brain images. The proposed system showed that ANN and hybrid classification approaches are the most appropriate for classification because of their high accuracy rates and achieved an accurate classification of 95.83%, 97.14%, and 95.71%, respectively.
In [ 100 ], the authors used various techniques, such as machine learning and different deep learning models, to predict various ophthalmic diseases; the study showed that the efficiency of each strategy varied depending on the input dataset and based on the many symptoms of each disease.
3.2.5. Metric Evaluation
Accuracy, specificity, and sensitivity are the most prevalent metrics utilized in most disease diagnosis applications for humans. The number of true positive (TP), true negative (TN), false positive (FP), and false-negative (FN) samples are used to calculate these measures [ 110 ]. The sensitivity determines how many positive samples were identified (TP), whereas the specificity determines how many negative samples were identified (TN). The ratio of correctly recognized samples to the total number of samples is used to calculate classification accuracy [ 110 ]. Other metrics such as precision, recall, F1 score, and AUC must be used in conjunction with these metrics [ 111 ]. Table 3 illustrates the number of times each performance metric was utilized in each study.
Frequency count of performance metrics utilized in every chosen study.
4. Machine Learning Techniques
This study classified machine learning (ML) segmentation and classification techniques as supervised and unsupervised machine learning. Supervised learning algorithms generate mathematical models using a set of labeled data (images) which are utilized for training, examples of supervised machine learning algorithms are K-nearest neighbors (K-NN), support vector machines (SVM), decision trees (DT), linear regression, logistic regression, random forest (RF), artificial neural networks (ANN), gradient boosting, and naïve Bayes models. Unsupervised learning algorithms create mathematical models based on a set of data that only contains inputs and no required output labels. The algorithms identify patterns in the data and classify them. Examples of unsupervised machine learning algorithms are K-mean clustering, hierarchical clustering, Apriori algorithm, principal component analysis (PCA), and fuzzy C-means (FCM) [ 112 ].
In the surveyed studies, several techniques of machine learning (ML) in segmentation and classification were deployed. The study [ 85 ] compared the IPFCM (intuitionist possibilistic fuzzy C-mean) method with the hybridization of the negative function of the intuitionistic and the negative function of the possibilistic for mammogram image segmentation with conventional segmentation methods such as the Otsu algorithm, FCM (fuzzy C-mean) clustering, IFCM (intuitionist fuzzy C-mean) clustering, and PFCM (possibilistic fuzzy C-mean). Moreover, they found that this proposed method was the best approach. In studies [ 88 , 91 , 98 ], the authors used the fuzzy clustering method. Fuzzy clustering is the most extensively used for image segmentation because of its benefits compared with traditional clustering methods, which includes: making regions more homogeneous, decreasing the number of erroneous spots, reducing noise sensitivity, and removing noisy regions [ 88 ]. In [ 92 ], the authors introduced the MSW-FCM technique (modified spatial weighted fuzzy C-means) for accurately segmenting blood vessels in retinal fundus images.
The distribution of the chosen studies that used the most popular machine learning techniques to diagnose human body disease is illustrated in Table 4 . This table comprises the detailed distribution of publication references, techniques used, classified tasks, and classification accuracy results.
Distribution of studies for the most common ML classification methods.
5. Deep Learning
Deep learning (DL) is well-known for its performance in image segmentation and classification models [ 113 ]. Convolution neural networks (CNN) are the most extensively utilized deep learning approach in the articles reviewed. CNN is an important image processing approach that allows for accurately classifying aberrant and normal samples [ 114 ]. CNN employs a layered perceptron-driven architecture composed of fully connected networks in which every neuron in one layer is coupled to all neurons in the subsequent layers. The input images, an in-depth feature extractor, and a classifier are the three main components of a CNN [ 111 ]. There are three kinds of layers in CNN, each of which performs a different function: (1) convolutional, (2) pooling, and (3) fully connected. The convolutional layer extracts the characteristics of the structure. The fully connected layer then decides which class the current input belongs to, depending on the retrieved features. Then, the pooling layer is responsible for shrinking feature maps and network parameters. Transfer learning algorithms can increase CNN performance in the case of limited input data [ 115 ]. A CNN can be created from scratch using an existing pre-trained network without retraining or fine-tuning a pre-trained network on a target dataset [ 111 ].
According to this review, some research publications used distinct deep neural network architectures; Table 5 summarizes studies that used deep learning in disease diagnosis.
Summary of articles that included deep learning in detecting diseases.
The study [ 66 ] suggested a new classification structure that depends on a combination of 2D CNN and recurrent neural networks (RNN) that learn the properties of 3D PET images by decomposing them into a series of 2D slices. The intra-slice characteristics are captured using hierarchical 2D CNNs, while the inter-slice features are extracted using the gated recurrent unit (GRU) of an RNN for final classification. The experimental outcomes showed that the suggested method has promising performance for Alzheimer’s disease (AD) diagnosis. The authors in [ 80 ] applied CNN-based algorithm on a chest X-ray dataset to detect pneumonia. Three approaches were examined, a linear support vector machine classifier with local rotation and orientation-free features, transfer learning on two CNN models: Visual Geometry Group, i.e., VGG16 and InceptionV3, and a capsule network training from scratch. Data augmentation is a data preprocessing technique applied to all three approaches, the outcomes revealed that data augmentation is an effective technique for all three algorithms to improve performance and efficiency. In [ 86 ], the authors proposed a successful approach for predicting the probability of brain cancers in MRI images using CNNs and the (Adam) optimizer algorithm. An adaptive moment estimation (Adam) optimizer has been introduced to expedite training the network and evaluate the model to attain maximum accuracy. The study [ 93 ] introduced a new deep learning-based CNN model created by the Bayesian optimization algorithm for classifying Alzheimer’s disease, mild cognitive impairment (MCI), and cognitively normal (CN) in MRI images. The proposed method gives extraordinary outcomes compared to the existing techniques. The study [ 97 ] compared a new optimized version of CNN and a new, improved metaheuristic, named the advanced thermal exchange optimizer for the detection of breast cancers with three different techniques including multilayer perceptron (MLP), multiple instances (MI), and transfer learning (TL), which were applied in the MIAS mammography database to demonstrate the superiority of proposed method.
In a study [ 102 ], the authors suggested using the ML approach in the training process to create a prediction model by training the CNN algorithm in addition to using the “Adam” optimizer from the Python Keras optimization library that has an initial learning rate of 0.001. The evaluation was carried out after partitioning the data into 80% and 20% for training and evaluation, respectively, to compute the accuracy of classification and loss of model over a set number of 10 epochs, the proposed algorithm gave good results. In the study [ 103 ], the authors developed a transfer learning-based technique to determine the severity of diabetic retinopathy; the proposed model was a deep learning model that combined multiple pre-trained image classification CNN models with the global average pooling (GAP) technique. The accuracy of the model attained 82.4% quadratic weighted transfer learning kappa (QWK). In [ 104 ], the authors proposed a diagnosis system based on deep neural networks and image retrieval method. Transfer learning and hashing functions increased the CNN performance and image retrieval algorithms. The proposed system attained an accuracy of 97% for CNN and a content-based medical image retrieval (CBMIR) method. The study [ 105 ] compared the effectiveness of modern CNN models for the task of modality classification and reported the superiority of a deep learning-based method over classic feature engineering approaches based on multi-label learning algorithms. The experimental results demonstrated that deep learning is more efficient than traditional methods and produced better and more robust feature representations when compared to handcrafted feature extraction approaches. The findings showed that deep transfer learning techniques work well in the medical field, where data is scarce. The Google Inception-v3 model performed the best when it came to classifying medical picture modalities. Except for VGG-16 and Res-Net-50, the other models behaved similarly to Inception-v3.
Generally, the studies utilized accuracy, specificity, sensitivity, precision, recall, f1 score, and AUC as evaluation metrics. According to the conclusions of the studies, deep learning algorithms achieved good results in most of the evaluation metrics (as in Table 5 ).
6. Diseases Diagnosis System
Image processing has been extensively employed in various illness diagnosis procedures (human, animal, and plant), helping professionals select the appropriate treatment. In diagnosing human diseases, image processing techniques play an essential role. They can be used to identify disease signs (on the skin, for example) or in molecular research using microscope images that show the anatomy of the tissues [ 116 ]. The disease diagnosis systems comprise stages and methods for diagnosis. It starts from the first stage, which is the stage of collecting images from different sources, either through a database available online or collecting the images from different sources, i.e., images of patients available on the internet or from a specific hospital. Then the preprocessing image stage as different filtering methods are applied to improve the image, and then the segmentation stage follows by isolating the regions of interest and extracting the important features in the feature extraction stage. At the end of the process, the input image is classified and metrics for evaluating the effectiveness of disease diagnosis techniques are applied. The general system stages for the diagnosis of any disease in image processing are illustrated in Figure 3 . This figure shows a conceptual map that explains concepts linked to disease diagnosis steps and a descriptive brief of the major evaluation metrics.

Conceptual map of disease diagnosis system.
In this study, several disease diagnosis methods were studied that used different image treatment and classification strategies, which dealt with diagnosing human diseases, to examine the new and important methods that addressed in the studies.
7. Discussion and Future Directions
The growing interest in employing medical image processing approaches and AI techniques, such as ML and DL, may reduce the doctor’s workload and repetitive and monotonous procedures to diagnose and analyze patient data and images [ 117 ]. In this section, we discuss the state-of-the-art approaches and datasets used to diagnose human diseases through an answer to research questions and future directions.
7.1. Answer to Research Questions
The data were extracted from the studied articles as they were explained and clarified to answer the research questions.
“Q1: What are the modalities of medical imaging?” The methods of medical imaging were identified in the articles, where many modern imaging methods for diseases have been used, which help in diagnosing diseases in the early stages. The MRI imaging modality was used in fourteen articles in the diagnosis of brain diseases, lung diseases, cardiovascular diseases, and cardiac attacks; the CT imaging modality was used in eight articles in the diagnosis of lung diseases, liver tumors, and bone diseases. The X-Ray modality was utilized in seven articles to diagnose osteoarthritis disease, pneumonia disease, and breast cancer. Five articles used retina fundus images to diagnose eye illnesses for retina disease diagnosis. PET imaging was utilized in four articles to diagnose Alzheimer’s disease, and dermoscopy skin imaging was used to diagnose skin problems.
“Q2: What is the task of medical image processing and analysis?“ In the analysis of the articles, it was identified that the task of the analysis of medical images and processing it to diagnose several diseases in different parts of the human body to assist the patient in detecting the disease and treating it in the early stage.
“Q3: Which medical image processing methods are most used in diagnostic systems?” Through the analysis of the articles, we discovered that by using filtering techniques, we may improve the initial image and get a more exact detection of the ROI borders. Noise can be reduced by smoothing with a low pass or median filtering, while edge sharpening and increased contrast can help to keep the ROIs’ borders. Color normalization, such as histogram equalization or specification, can boost contrast. A variety of transforms might be used to extract important features. Fourier and wavelet transform can be utilized to locate conditions in a domain other than the spatial one. Because the borders of the object or regions containing the crucial information for the diagnosis must be identified correctly, image segmentation is one of the most essential phases in a disease diagnosis procedure. The simplest but effective method is gray-level segmentation utilizing a single, multiple, or more advanced (Otsu) criteria. Several human illness diagnosis approaches have used active contour detection and modifications, such as a snake. Several clustering approaches were applied in the segmentation process, such as K-mean and fuzzy C-means, to separate the ROIs. The articles used many methods to extract the important features from objects. We found that the texture features were the most used in analyzing the articles. It was obvious from the analysis of the articles that the most popular classification method used in articles was a neural network with all types and SVM, where it was used alone or with another technique as a hybrid system for the classification of diseases. The rest of the classification techniques examined in this review such as K-means, K-NN, decision trees, naïve Bayes, random forest, logistic regression, and gradient boosting. All the classification or clustering methods can be exploited in the last stage of the diagnosis application to create a new diagnosis system to identify disease.
“Q4: What diagnostic techniques have been adopted and developed?” Analyzing the articles, we found that some of the articles adopted new hybrid methods for classification and diagnosis of diseases, such as using the SVM method with KPSA, SVM with FA, SVM with MLC, as well as using it with fuzzy. Moreover, the combination of random forest (RF) with random subspace (RS) and the networks CNN with RNN and with optimizers were used to create systems for disease diagnosis.
“Q5: Is the system that has been adopted or designed capable of producing good results?” The main classification methods discussed in the articles achieved efficient and good results in diagnosing diseases. The accuracy of SVM ranged between 88% and 100% in the several human disease diagnosis applications examined, naïve Bayes achieved accuracy between 78% and 94%, decision trees ranged between 82% and 99%, and random forest ranged between 80% and 99%. The different kinds of neural networks achieved accuracy between 73% and 97%, and the accuracy of CNN ranged between 86% and 99.3%. Finally, the accuracy of the K-nearest neighbor ranged between 73% and 95.5% in the referenced approaches.
7.2. Future Directions
It takes great effort to improve the performance of classification or diagnosis of diseases using multiple methods of medical images. In future work, we will try to present new research directions that can be further exploited in disease diagnosis through medical image processing techniques. We will compare the most common methods that are used in the diagnosis systems and select the most effective methods that introduce higher accuracy to the diagnosis for the medical image database that we use to build a new diagnostic system for diseases. The future research directions are discussed briefly as follows:
A. Study and analysis of the best and most common classification and diagnosis methods.
B. Experimenting with a set of medical data to compute the classification accuracy of the methods used.
C. Comparison of classification accuracy of these methods to identify the methods that are highly accurate.
D. Building a new diagnostic method by deriving a hybrid method, modifying a previous method, or combining previous methods.
The current study improved our knowledge of finding the best techniques that can be beneficial to our future research. We hope that this systematic literature review will also be useful to other researchers in their endeavor to improve disease diagnosis through medical image processing techniques. Due to our comparisons, the most common methods used in diagnosis systems have been discussed, making it easier to select effective methods that introduce higher accuracy to diagnosis methods using medical databases.
8. Conclusions
Medical imaging plays a critical role in inspecting and diagnosing human diseases. For diagnosis, many algorithms based on diverse methodologies have been created. As a result, disease detection has become an important topic in medical image processing and medical imaging research.
This review studied the articles on disease diagnosis published between 2017 and 2021. Overall, forty articles were analyzed from specialized academic repositories. The review focused on six factors: datasets used, various medical imaging modalities, image preprocessing techniques, image segmentation techniques, feature extraction techniques, classification approaches, and performance metrics, which were used to build and evaluate the disease diagnostic models. In addition, this systematic review highlighted a variety of AI techniques and presented a comprehensive study by exploring new diagnosis techniques, disease diagnosis issues, provided a variety of insightful information (such as the use of ML and DL), and an evaluation for each study. We aimed to address our study questions about effective diagnosis methodologies and discover the solutions proposed by many researchers in the diagnosis of diseases based on this. It was discovered that developing a new disease diagnosis method is quite important.
According to the findings of this SLR, researchers have adopted various methods to classify medical images associated with multiple disease diagnoses. These methods have shown promising results in terms of accuracy, cost, and detection speed. We found after analyzing the forty articles that the best preprocessing technique was the median filter, which was used in many studies, as has proven its ability to reduce noise and preserve the boundaries of the object. Regarding segmentation approaches, threshold techniques were the most used to extract the lesion from an image. Threshold-based approaches are the most often utilized among all the traditional methods, according to classic review publications, due to their applicability for numerous segmentation issues in medical images [ 118 ]. The methods for extracting features from medical images depend on the images used by analyzing the articles, we found that extracting texture features gave the best results. As for the applied classification methods, we found that the support vector machine method gave the best result in classification, as its accuracy in [ 67 ] reached 100% when it was used with KPC (support vector machine with kernel principal component analysis (SVM- KPCA)) approach. Thus, this method achieved the best accuracy and the best performance in diagnosis. Among the machine learning-based approaches whose associated works and analyses are presented, the supervised learning methods, notably the neural network-based methods, were the most widely employed, with different kinds of neural networks used to identify various diseases.
We also discovered that deep learning utilizing the CNN network has unique skills and advances in recognizing and classifying medical images, particularly those connected to breast, lung, and brain cancer. Other common classification approaches comprise fuzzy clustering, K-NN, K-means, decision trees, random forests, and other prominent classification algorithms. The most utilized measures were accuracy, sensitivity, and specificity.
This study aimed to propose future research directions by focusing on imaging modalities, techniques, and procedures used in the reviewed articles. Furthermore, this publication will aid in the development of new research that assesses and compares various medical image processing and analysis techniques. The findings provided in this SRL reveal that tremendous progress has been made in medical image processing over the last five years. In addition, the goal of this SRL was to undertake a detailed analysis of research achievements linked to the usage of medical image processing techniques applied to medical databases to know the current state-of-the-art techniques.
Abbreviations
CT—Computed Tomography; MRI—Magnetic Resonance Imaging; US—Ultrasound; PET–Positron Emission Tomography; GI—Gastrointestinal Diseases; FCM—Fuzzy C-Mean; IFCM—Intuitionist Fuzzy C-Mean; PFCM—Possibilistic Fuzzy C-Mean; IPFCM—Intuitionist Possibilistic Fuzzy C-Mean; MSW-FCM—Modified Spatial Weighted Fuzzy C-mean; DWT—Discrete Wavelet Transform; PCA—Principal Component Analysis; KPCA—Kernel Principal Component Analysis; SFTA—Segmentation-based Fractal Textural Analysis; SIFT—Scale Invariant Feature Transform; GLCM—Gray Level Co-occurrence Matrix MI- Moment Invariant; WHT—Walsh Hadamard Transform; HOG—Histogram of Oriented Gradients; SVD—Single Value Decomposition; CCSA—Chaotic Crow Search Algorithm; 2D-FFT—Two Dimension Fourier Features Transform; 2D-DWT—Two Dimension Wavelet Features Transform CM—Color Moment; SVM—Support Vector Machine; RBFNN—Radial Basis Function Neural Network; ANN—Artificial Neural Network; SVM-FA—Support Vector Machine with Firefly Technique; DNN—Deep Neural Network; MLC—Maximum Likelihood Classifier; FF-ANN—Feed-Forward Artificial Neural Network; K-NN—K Nearest Neighbor; LDA—Linear Discriminate Analysis; PNN—Probabilistic Neural Network; RSDA—Rough Set DATA Analysis; FSVM—Fuzzy Support Vector Machine; CNN—Convolutional Neural Network; RF—Random Forest; DT—Decision Trees; ROI—Region Of Interest; RNN—Recurrent Neural Network.
Funding Statement
This research received no external funding.
Author Contributions
The concept of the article was proposed by N.P., the data resources and validation were contributed by B.M.R., the formal analysis, investigation, and draft preparation were performed by B.M.R. The supervision and review of the study were headed by N.P. The final writing was critically revised by N.P. and finally approved by the authors. All authors have read and agreed to the published version of the manuscript.
Institutional Review Board Statement
Not applicable.
Informed Consent Statement
Data availability statement, conflicts of interest.
The authors declare no conflict of interest.
Publisher’s Note: MDPI stays neutral with regard to jurisdictional claims in published maps and institutional affiliations.
Deepfake Detection: A Systematic Literature Review
Ieee account.
- Change Username/Password
- Update Address
Purchase Details
- Payment Options
- Order History
- View Purchased Documents
Profile Information
- Communications Preferences
- Profession and Education
- Technical Interests
- US & Canada: +1 800 678 4333
- Worldwide: +1 732 981 0060
- Contact & Support
- About IEEE Xplore
- Accessibility
- Terms of Use
- Nondiscrimination Policy
- Privacy & Opting Out of Cookies
A not-for-profit organization, IEEE is the world's largest technical professional organization dedicated to advancing technology for the benefit of humanity. © Copyright 2024 IEEE - All rights reserved. Use of this web site signifies your agreement to the terms and conditions.

- Ask a Librarian
Artificial Intelligence (AI)
Ai for systematic review.
- How to Cite AI Generated Content
- Prompt Design
- Resources for Educators
- Purdue AI Resources
- AI and Ethics
- Publisher Policies
- Selected Journals in AI
Various AI tools are invaluable throughout the systematic review or evidence synthesis process. While the consensus acknowledges the significant utility of AI tools across different review stages, it's imperative to grasp their inherent biases and weaknesses. Moreover, ethical considerations such as copyright and intellectual property must be at the forefront.
- Application ChatGPT in conducting systematic reviews and meta-analyses
- Are ChatGPT and large language models “the answer” to bringing us closer to systematic review automation?
- Artificial intelligence in systematic reviews: promising when appropriately used
- Harnessing the power of ChatGPT for automating systematic review process: methodology, case study, limitations, and future directions
- In-depth evaluation of machine learning methods for semi-automating article screening in a systematic review of mechanistic
- Tools to support the automation of systematic reviews: a scoping review
- The use of a large language model to create plain language summaries of evidence reviews in healthcare: A feasibility study
- Using artificial intelligence methods for systematic review in health sciences: A systematic review
AI Tools for Systematic Review
- DistillerSR Securely automate every stage of your literature review to produce evidence-based research faster, more accurately, and more transparently at scale.
- Rayyan A web-tool designed to help researchers working on systematic reviews, scoping reviews and other knowledge synthesis projects, by dramatically speeding up the process of screening and selecting studies.
- RobotReviewer A machine learning system aiming which aims to automate evidence synthesis.
- << Previous: AI Tools
- Next: How to Cite AI Generated Content >>
- Last Edited: Mar 25, 2024 4:20 PM
- URL: https://guides.lib.purdue.edu/ai
- Reference Manager
- Simple TEXT file
People also looked at
Systematic review article, nutrition education and its relationship to body image and food intake in asian young and adolescents: a systematic review.
- 1 PAPRSB Institute of Health Sciences, Universiti Brunei Darussalam, Gadong, Brunei
- 2 Sultan Hassanal Bolkiah Institute of Education, Universiti Brunei Darussalam, Bandar Seri Begawan, Brunei
Background: The literature brings to light the unhealthy nutritional habits prevalent among Asian adolescents and their high level of body image dissatisfaction. This study aims to conduct a systematic review of the literature on the effect of nutritional education interventions on their nutritional knowledge and food intake behavior, attitude, practice, and body image.
Methods: We searched relevant published studies in PubMed, Web of Science, Scopus, Science Direct, and Springer using the PICO framework and performed a quality assessment using the 10-point checklist adapted from the National Institutes for Health tool.
Results: The majority of the nutritional education interventions improve unhealthy food intake and body image misperception, particularly on nutritional knowledge/self-efficacy, healthy dietary habits, physical activities, and fruit and vegetable intake. We also found a negative association with excess weight gain, obesity, and unethical weight reduction practices, leading to dissatisfaction with body image.
Conclusion: These interventions can help address dietary problems and body image perception and support the development of future interventions.
1 Introduction
Obesity and excess weight gain are increasingly common issues among adolescents. Unhealthy diet choices and reduced physical activity are also prevalent and lead to a persistent positive energy balance ( 1 ). This, in turn, leads to excess weight gain among children and adolescents ( 2 ). Adolescents are particularly affected because they have higher nutritional needs as they undergo rapid growth and development. Significant physical, psychological, and social changes occur throughout this formative period of life. However, insufficient intake of essential nutrients such as carbohydrates, proteins, fats, vitamins, and minerals may harm their immediate growth and long-term development. Yet, a troubling pattern emerges where many young individuals, instead of prioritizing nutritious foods, opt for calorie-dense, nutrient-poor foods to meet their food cravings and stave their hunger. According to multiple reports, adolescents are more likely to consume unhealthy foods such as refined carbohydrates (snacks and beverages with added sugar), caffeine, and junk food than they are to consume fruit, milk, and vegetables ( 3 – 5 ). The following evidence highlights this: Most adolescents skip meals frequently, only up to 50% consume fruits and vegetables and use dietary supplements, 13.9% of the total energy intake of Korean adolescents comes from free sugar, significantly higher than the 5% recommended by the World Health Organization (WHO) ( 6 ), 53.9% of the beverages consumed contain added sugar, accounting for 20.5% of the daily sugar intake and 12.5% of high school pupils use more caffeine than is advised ( 7 ). This preference is what leads to an imbalance in their diet, a situation that can only be rectified through decisive efforts guiding the formulation of effective strategies promoting healthier eating habits.
Moreover, body image misconceptions distort the perception of one’s body and set unrealistic expectations of how one should look. Social media and advertising significantly perpetuate these misconceptions by showing idealized and often unattainable body types. It was reported that 24.2% of adolescents in middle and high school had negative body image issues despite having a normal body mass index (BMI) ( 8 ). This negative self-impression of body image may result in severe physical and mental effects ( 9 ) and may lead to melancholy, low self-esteem, binge eating, eating disorders, consuming unhealthy foods, and excessive weight loss practices, and this is a growing concern around the world ( 10 , 11 ). It has been identified as a global problem that transcends geographical boundaries and affects individuals of all income levels and linguistic backgrounds ( 12 , 13 ). Since proper nutrition is vital in promoting adolescents’ physical and mental health, a multifaceted approach that includes nutritional education and support for individuals struggling with body image is required. It is crucial to encourage a healthy relationship with food, promote self-acceptance, and focus on overall well-being rather than external appearance. Negative attitudes toward food and a distorted body image not only contribute to obesity but also exert a profound influence on an individual’s mental and physical well-being ( 14 ). Developing healthy eating habits during adolescence could prevent obesity, type 2 diabetes, cardiovascular disease, and other disorders. They also promote mental alertness and increased cognitive function and catalyze positive thoughts about one’s appearance and self-worth. Nutritional interventions aimed at fighting obesity can also be used to decrease the incidence of excessive food intake and wrong body image assessment.
Nutritional education interventions aim to improve nutrition-related knowledge, attitudes and practices. This provides individuals with skills and information to help them improve their diet, lifestyle, behavior and emotional well-being. Some of the common methods to deliver nutrition education interventions include lectures and counseling. Increasingly, web resources, social media, video-based learning, mobile apps and game-based learning are also being used. In an educational setting, nutritional interventions are integrated into the classroom through nutrition programs, peer education and healthy school meal initiatives. The nutrition curriculum often includes concepts of food production and preparation, healthy eating by making the right food choices, lifestyle modification, and cultural aspects of nutrition. Hands-on assignments include cooking classes, kitchen safety and field trips. Notably, school meal programs typically include the promotion of locally and regionally produced foods or food products, which contributes to a comprehensive approach. The success of these interventional measures depends on the active involvement of parents, the community and teachers, with a focus on a holistic approach.
The literature highlights the unhealthy nutritional habits prevalent among Asian adolescents and multiple cross-sectional studies have identified this subpopulation as exhibiting the highest level of dissatisfaction with their body image. Improving nutritional education interventions would lower the incidence of obesity among them ( 5 ). We can further note that many educational interventions have succeeded in addressing unhealthy eating behavior and body image dissatisfaction in a large number of countries. However, to date, there are no studies that systematically review the efficacy of these interventions. This study fills this gap and thoroughly analyzes the impact of various nutritional education interventions on nutritional knowledge and related food intake behavior, attitude and practice, and body image in the Asian young and adolescent population.
2 Materials and methods
We aligned the systematic review methodology with our research goal of exploring the effect of nutritional educational intervention on nutritional knowledge, attitude, practice, and body image in the Asian young and adolescent population. This procedure followed the six-stage technique of a systematic literature review, beginning with the creation of protocols and continuing into eligibility criteria, search strategy, study evaluation, data extraction, and result synthesis.
2.1 Development
The review protocol explains the review’s historical context, the publications chosen for review, and how it tackles publication quality evaluation and data retrieval. We employed the Preferred Reporting Items for Systematic Reviews and Meta-Analyses (PRISMA) statements as recommended for conducting a systematic literature review ( 15 ).
2.2 Research question
We used the problem/population, intervention, comparison, and outcome (PICO) framework to define the review questions. This framework informs the search string and the search strategy. Table 1 shows the list of terms comprising each of the four components of the PICO framework we have used to frame the two research questions. The review aims to answer two research questions: Research question 1 (RQ1): How does nutritional education intervention affect food intake behavior and its related outcomes? Research question 1 (RQ2): How does nutritional education intervention affect body image perception?

Table 1 . PICO framework for outlining the research questions.
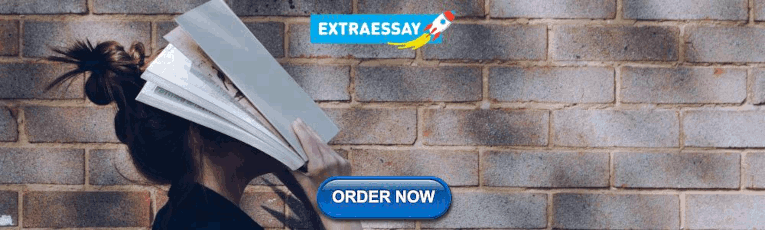
2.3 Search strategy
We selected and then searched five electronic databases PubMed, Web of Science, Scopus, Science Direct, and Springer. The search was conducted on the 17th of March, 2023. Search terms included terms such as ‘nutritional knowledge,’ ‘dietary intervention,’ ‘nutritional knowledge,’ ‘nutritional attitude,’ ‘eating practice,’ ‘body image perception,’ and ‘body image satisfaction’ and different Asian geographical locations. Search terms were combined using Boolean operators “AND” and “OR” in the title and abstract index. The search was limited to articles published between January 2000 and December 2022.
2.4 Eligibility criteria
We defined the criteria for including or excluding retrieved studies. Table 2 shows the inclusion and exclusion criteria. No studies were excluded based on the year of publication.
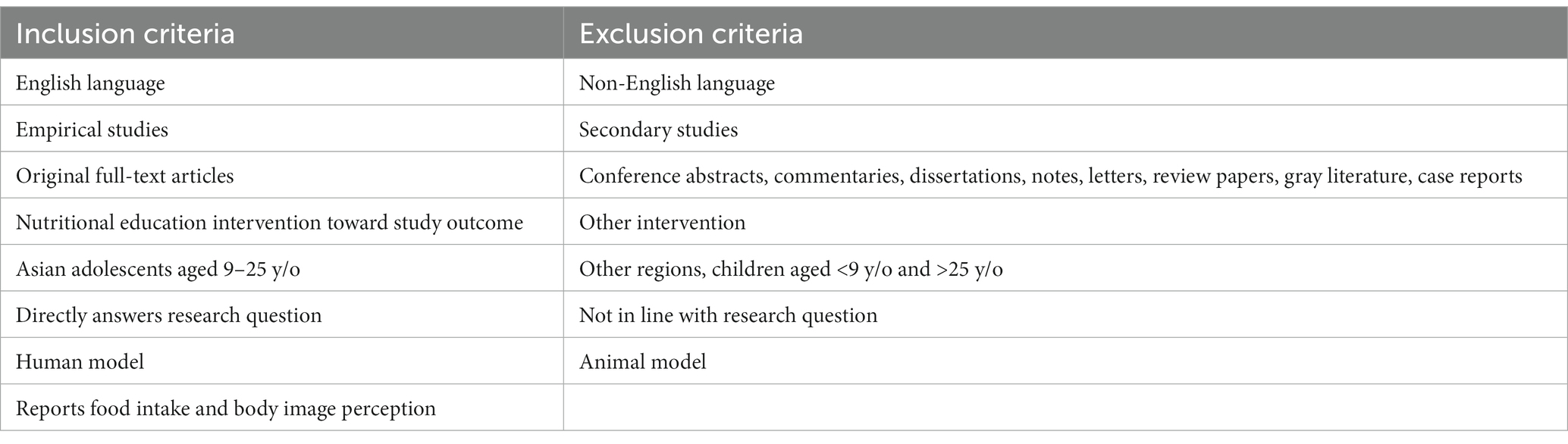
Table 2 . Criteria for inclusion and exclusion.
2.5 Article screening
We employed Covidence screening software to manage and screen the bibliographic data from the five electronic sources. The software automatically excluded duplicate articles. The references were upvoted on the software using the inclusion and exclusion criteria. We examined both article’s title and abstract to determine their alignment with the research objectives. Then we read the full text of the shortlisted papers. Two independent reviewers screened the article’s titles, abstracts, and full text to reduce bias. All eligible articles were thoroughly reviewed, and the discrepancy between the reviewers during the screening was resolved by discussion and consensus.
2.6 Study appraisal
The objective of this appraisal was to evaluate the quality of the articles in terms of their methodology and to address the possibility of bias in their planning, execution, and analysis. Following the inclusion criteria, two critical reviewers conducted a rigorous evaluation of all articles selected for this systematic review. The quality assessment was performed using the 10-point checklist adapted from the National Institutes for Health tool ( 16 ) for appraising observational, cohort, and cross-sectional studies. Table 3 shows the 10 items on the checklist considered in this study which help assess the quality of the articles. The 10 items were evaluated using the dichotomous Yes or No rating method. The quality of evaluated studies was subcategorized as high (above 70% of Yes responses), moderately high (50–69% of Yes responses), or low (below 50% of Yes responses), depending on their assessment score.
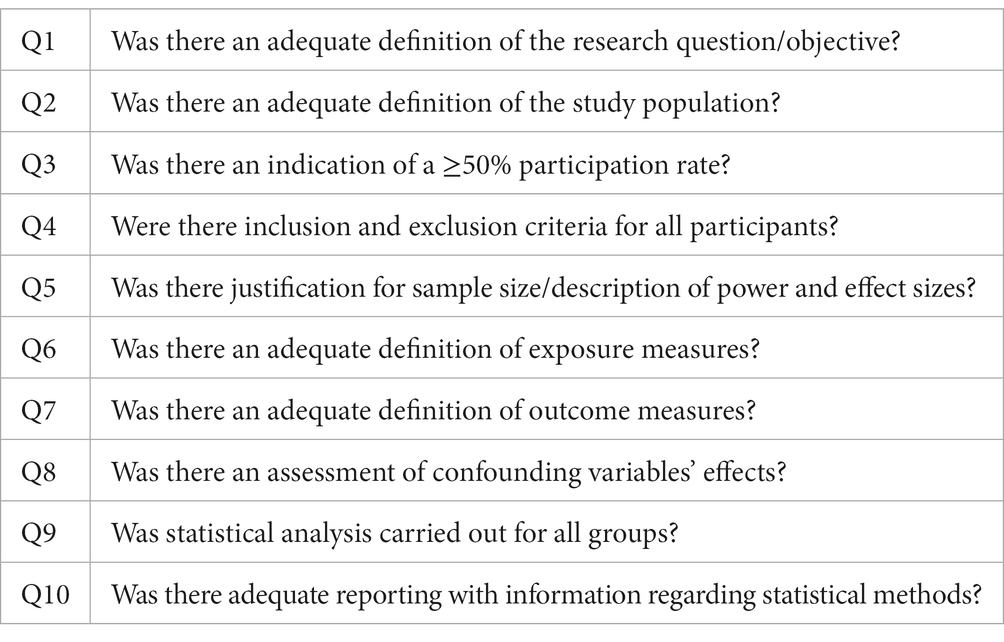
Table 3 . Assessment checklist items (adapted from the National Institutes for Health).
2.7 Data extraction
Data was extracted from the studies using an electronic data extraction form developed with Microsoft Excel. The characteristics of each study were summarized under various headings, allowing the most important findings to be synthesized. The information extracted includes the following: (i) primary author’s information and affiliations, (ii) publication year, (iii) country, (iv) aim of the article, (v) study design, (vi) sample characteristics including the educational level of the participants, sample size, age and gender, (vii) description of the nutritional interventions used, (viii) duration of the experiment to follow-up period, (ix) method of assessing the intervention outcome, (x) outcome, (xi) statistical analysis, (xii) results, and (xiii) the main findings of the articles. After data extraction, we organized and summarized the article characteristics to answer the systematic review questions.
This section presents the selection process of the papers in the systematic review and the results of both the quality assessment and data extraction.
3.1 Study selection
We retrieved 2,671 articles from the five electronic databases and exported them to the Covidence software for reference management. The software excluded 525 duplicate records. Screening the titles and abstracts of the remaining studies disqualified 2,119 articles as they did not align with the research question. Two independent reviewers evaluated the remaining 27 studies and based on the inclusion criteria excluded 17 studies. Finally, 10 articles met the inclusion criteria and were included in the review. Figure 1 displays the PRISMA chart that explains the screening procedure.
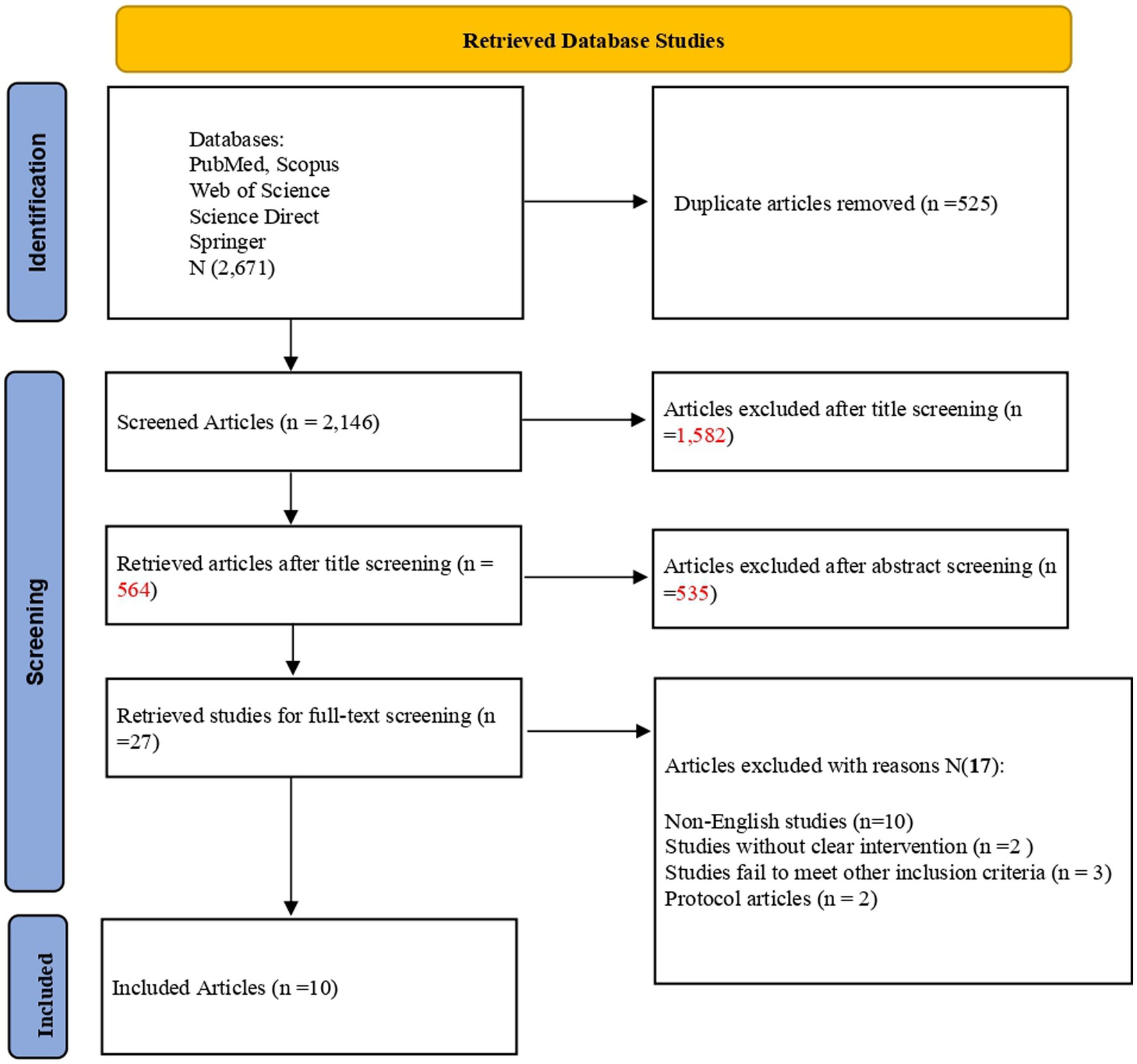
Figure 1 . PRISMA diagram showing screening process.
3.2 Quality assessment
We evaluated the 10 articles’ methodological and reporting quality. The detailed results are presented in Table 4 as outlined in Section 2.6. All of them obtained a score of more than 70% and thus were of good quality. Only 10% of the articles —one article— met all 10 items on the checklist and therefore scored 100%. Eight studies scored between 80 and 90%, while the remaining two studies scored 70%.
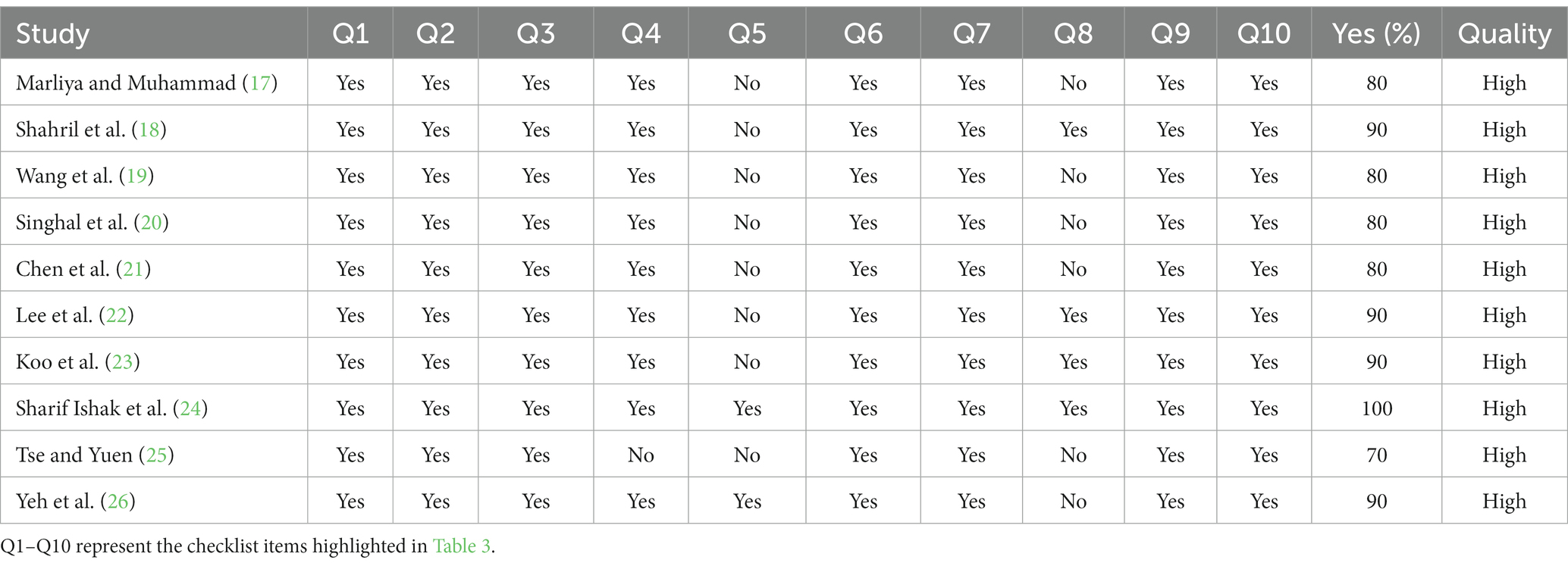
Table 4 . Quality assessment of the studies.
Specifically, we can note that all the studies had a defined research question and an adequate description of the study population, at least 50% of the study population had participated, both the exposure and outcome measures were adequately defined, statistical analysis had been conducted and they all had adequate reporting. As listed in Table 4 , all the studies outlined inclusion and exclusion criteria for all participants ( 17 – 24 , 26 ) except for Tse and Yuen ( 25 ). Conversely, only Sharifa Ishak et al. ( 24 ) and Yeh et al. ( 26 ) justified the sample size and described power and effect sizes. Finally, only four studies ( 18 , 22 – 24 ) included an assessment of confounding variable effects.
3.3 Result synthesis
The selected studies covered five Asian countries. Most of the articles examined Malaysian ( 18 , 23 , 24 ) and Chinese adolescents ( 19 , 25 , 26 ), whereas others focused on Korean adolescents ( 21 , 22 ), Indonesian adolescents ( 17 ), and Indian adolescents ( 20 ). The studies were published between 2009 and 2021. The study population of the reviewed articles included university undergraduates, middle and high school students, secondary school students, and 4th, 5th, 6th, 8th, and 11th-grade students. All the participants were aged 9–25 years old. Male students who participated in the experiments were slightly older than the female participants.
These studies adopted various study designs like randomized controlled trials (RCT) ( 17 , 21 ), non-randomized controlled trials ( 19 ), cross-sectional ( 20 , 22 ) quasi-experimental design ( 23 , 24 , 26 ), pre-post study design ( 25 ), and cluster RCT ( 18 ). Of the 61,893 Asian adolescents enrolled in the different studies, 29,670 participants received nutrition education intervention, whereas 32,223 did not.
The nutritional education interventions covered in the articles were the following: animation movies and lectures ( 17 ); a multimodal nutrition education module ( 18 , 20 ); a WeChat app ( 19 ); iStartSmart for teens educational program using an online format consisting of a short video ( 21 ) and an e-book ( 22 ); GReat-Child Trial™ based on social cognitive theory improved knowledge, attitudes and practices ( 23 ); module delivery focused on healthy eating, positive body image, an active lifestyle ( 24 ); tailor-made nutritional education ( 25 ); and an educational program on body image ( 26 ). The duration of the experimental follow-up period varied across the studies, ranging from 3 to 36 weeks after the administration of the intervention.
The outcomes measured in the articles were the following: obesity and weight gain ( 17 ), dietary intake habits ( 18 , 25 ), physical fitness tests ( 19 ), anthropometry ( 19 – 21 , 24 ), nutritional change and blood pressure ( 21 ), sedentary activity ( 21 ), self-efficacy and quality of life ( 21 ), body image misperception ( 22 ), breakfast frequency ( 22 ), knowledge-attitude-practice towards whole grain consumption ( 23 ), level of physical activity ( 19 , 21 , 25 ) and perceptional, attitudinal, and behavioral aspects of body image ( 26 ).
The statistical analyses used by the authors to measure the significance of the outcomes included independent samples t -test ( 17 , 20 , 22 ), paired samples t -test ( 17 , 20 , 25 ), Wilcoxon signed rank test ( 17 , 19 ), spearman correlation ( 17 ), analysis of covariance (ANCOVA) and adjusted effect size ( 18 , 23 , 24 ), McNemar test ( 20 ), linear mixed effects models and regress models ( 21 ), weighted chi-square test ( 22 , 24 ), and weighted multivariate logistic regression analysis ( 22 ). One included study did not report statistical methods ( 26 ).
3.4 Intervention outcome
The interventions reviewed positively affected dietary intake behaviors and were especially effective in reducing the consumption of unhealthy food, thus leading to a reduction in body image misperception. Table 5 lists the 31 different outcomes covered by the 10 articles reviewed by source and a detailed synthesis of results is summarized in Table 6 .
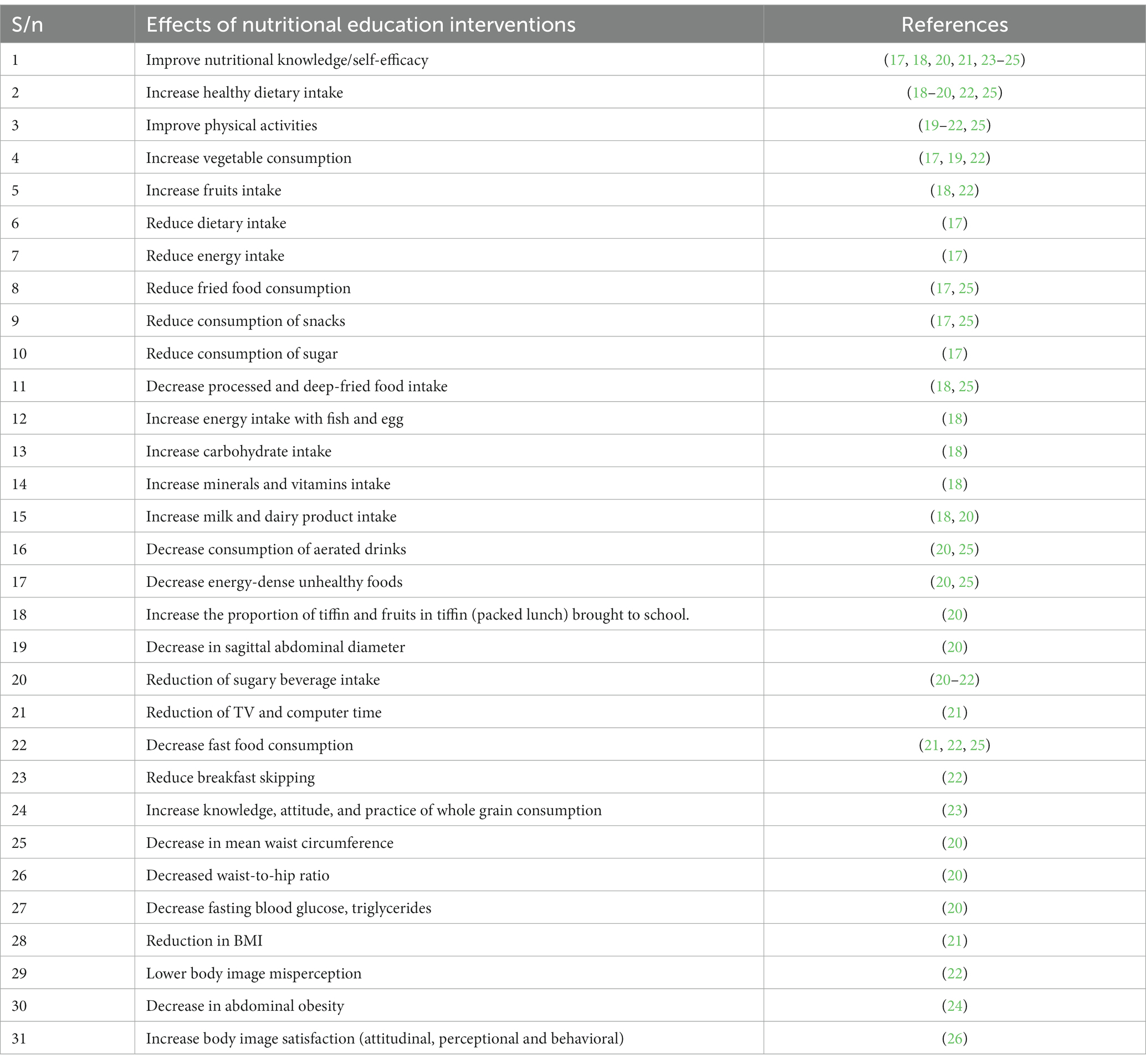
Table 5 . Impact of nutritional education intervention.
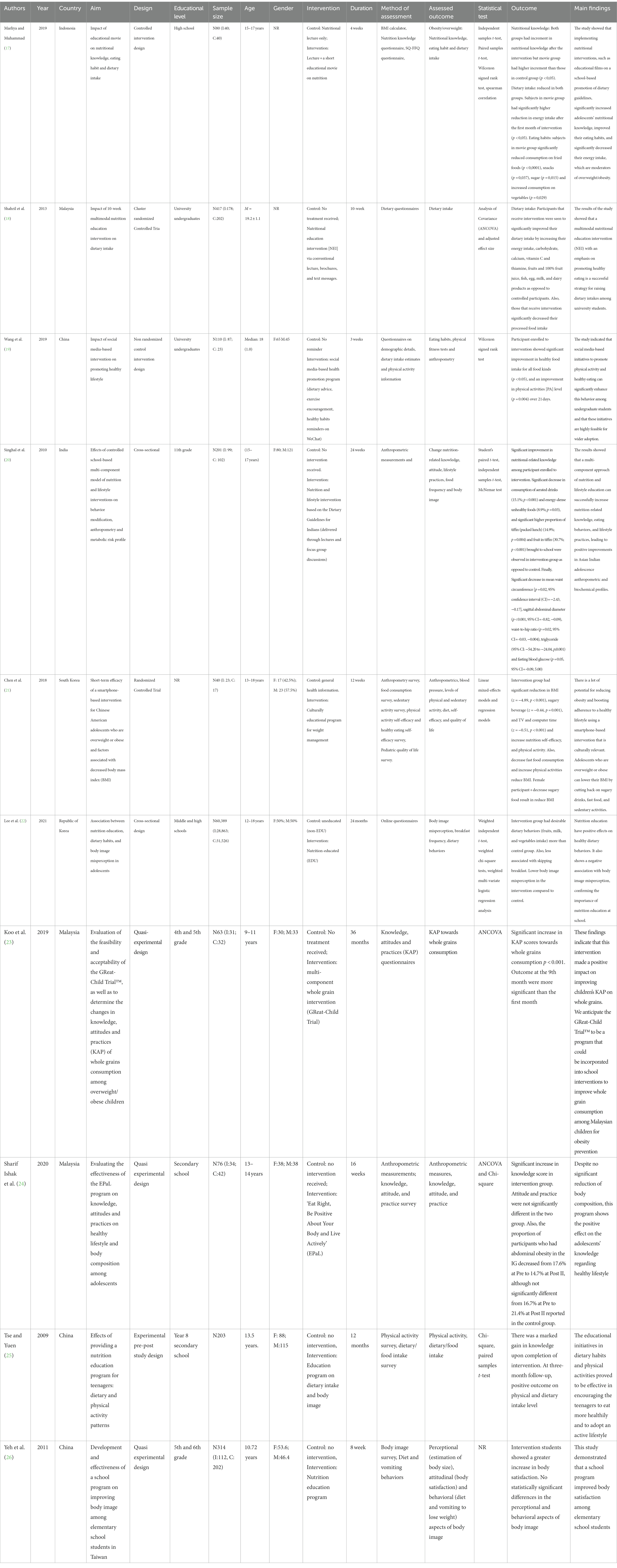
Table 6 . Synthesis table.
3.4.1 Nutritional education intervention and dietary knowledge
Five studies emphasized a significant improvement in dietary/nutritional knowledge among adolescents ( 17 , 20 , 21 , 24 , 25 ). These studies revealed high levels of knowledge across different areas of diet and nutrition after intervention. The participants demonstrated increased nutritional knowledge of dietary types, attitudes and behavior and physical activities, as well as a new awareness of body image following the intervention. In particular, their new knowledge included information about simple and complex carbohydrates, the concept of empty calories, sources, adverse effects of trans-fat, high-fat dairy products, dietary fiber, refined grains, whole grains, sugary contents, and glucose elevators.
3.4.2 Nutritional education intervention and dietary attitude and practice
All of the articles found interventions to have a significant effect on the nutritional attitudes and practices of Asian adolescents ( 17 – 26 ). These attitudes and practices revolve around healthy food intake behaviors such as increasing the consumption of fruits and vegetables ( 17 – 20 , 22 , 23 ) and the proportion of fruits in packed lunches brought to school ( 20 ), increasing the consumption of healthy carbohydrates, minerals and vitamins, whole grain, and dairy products ( 18 , 20 , 23 , 25 ), and reducing the consumption of unhealthy foods (energy-dense, fried, sugary, processed, junk foods, snacks, carbonated drinks and excessive food intake) ( 17 , 18 , 20 , 21 , 25 ).
There was also a lower exposure to TV and screens, a lower intake of food in canteens, a lower consumption of fast food and a reduction in the number of participants skipping breakfast ( 20 , 21 , 23 ). One study reported that 93% of participants felt happy with the lifestyle changes made after the intervention ( 21 ), while another study reported a significant change in knowledge, attitudes, and practice ( 23 ). Conversely, one study reported an increase in knowledge but no change in practices and attitudes ( 24 ). Another study highlighted that more participants recognized that eating a serving of unhealthy foods would harm their health and thus decrease their intake of food from restaurants, kiosks, and eateries ( 20 ).
3.4.3 Nutritional education intervention and physical activity
Five studies identified that nutritional intervention significantly improved adolescent physical activity levels ( 19 – 22 , 25 ). Reported physical activity included increasing the duration of quiet squats ( 19 ) and plank time ( 19 ), as well as incorporating 30–60 min of physical activity per day or participating in physical activity more than 4 days per week ( 20 ). These adolescents demonstrated less sedentary lifestyles, more hours of exercise ( 25 ), and more participation in household chores ( 25 ). There was also a significant increase in the number of adolescents who walked instead of taking transport as an activity ( 25 ).
3.4.4 Nutritional education intervention and body weight
Four studies highlighted the significant effect of a nutritional education intervention on adolescents’ body weight and its associated factors ( 20 , 21 , 23 , 24 ). According to these studies, nutritional education interventions significantly reduced sagittal abdominal diameter ( 20 ), abdominal obesity ( 24 ), body mass index ( 21 ), fasting blood glucose level ( 20 ), waist circumference, and waist-to-hip ratio, which significantly altered anthropometry, body composition, and metabolic parameters ( 20 ). In another study, more participants correctly identified their body size at pre-intervention, post-intervention, and 3 months after the intervention ( 26 ).
3.4.5 Nutritional education intervention and body image
Several studies demonstrated lower body image misperception ( 23 ) and a significant increase in body image satisfaction 3 months after intervention ( 26 ). Surprisingly, no significant difference in the scores for vomiting behavior post-food intake was reported ( 26 ). An article reported slightly higher overall satisfaction with physical appearance and fewer participants who avoided clothes that made them look overweight ( 20 ).
4 Discussion
The systematic literature review analyzed the findings of 10 articles retrieved from five electronic databases—PubMed, Web of Science, Scopus, Science Direct, and Springer—which focus on the impact of nutritional education interventions on nutritional knowledge and related food intake behavior, attitude, practice, and body image among the Asian young and adolescent population. The PICO framework was used to formulate the review questions, ensuring a focused and systematic research strategy. All the articles met the inclusion criteria and were checked for methodological quality using the National Institutes for Health tool. The guidelines of the PRISMA and Cochrane were thoroughly followed.
The studies cover very different interventions and outcomes, but because of this diversity and the high degree of overlap between them, we can infer meaningful conclusions. We explored multiple interventions of nutrition education, including lectures, movies, social media, text messages and notes ( 17 – 19 ). These interventions impacted key factors such as nutritional knowledge/self-efficacy, healthy dietary habits, physical activity, and fruit and vegetable intake. The reviewed studies demonstrate the potential of nutritional educational interventions to address dietary problems and body image challenges during childhood and adolescence. No distinction due to age was observed, despite taking into account a wide age span.
Such interventions affected food intake behaviors and their related outcomes (RQ1). They improved dietary and nutritional knowledge ( 17 , 20 , 21 , 24 , 25 ). Empowering adolescents with accurate and relevant nutritional knowledge enables them to make informed dietary choices, resulting in healthier eating patterns. They effectively improved dietary attitudes and practices, leading to increased consumption of nutritious foods and reduced intake of unhealthy options. Many of the nutritional educational interventions also positively influenced physical activity levels among participants. Several studies showed that post-intervention participants had increased physical activity duration and decreased sedentary behavior. This is consistent with previous research which shows that nutritional interventions significantly enhance both physical activity and literacy ( 27 – 29 ). Physical activity is critical in maintaining overall health and preventing obesity and related health issues, making this an important benefit of the interventions. Enhanced literacy skills are crucial in understanding and applying nutritional knowledge, fostering a comprehensive approach to health.
Furthermore, the review found that the interventions significantly reduced obesity and weight gain among Asian adolescents through better food choices, healthier eating habits, and more physical activity. The studies showed a correlation between nutritional education and improved body mass index, waist circumference, and other anthropometric measures ( 20 ). Beyond weight loss, the focus is on enhancing overall well-being, recognizing that comprehensive health extends beyond weight management. These anthropometric measures are vital indicators of overall health and well-being. With well-structured nutritional education interventions, adolescents are encouraged to make better dietary decisions, leading to a better long-term lifestyle with less obesity ( 1 , 2 ) and related disease risk.
The findings also suggested that nutritional education interventions affected body image perception (RQ2). They reduced misperception and increased satisfaction with body image among Asian adolescents ( 22 , 23 ). These interventions have the potential to address unrealistic ideals and focus on long-term approaches that can reinforce positive mindset changes. Thus, they could be crucial in improving the overall health and well-being of Asian adolescents.
Future policymaking could draw from the findings of this review. In particular, this research underscores the importance of incorporating nutritional education programs in schools, universities, and other educational settings. It demonstrates that interventions can impact adolescents’ eating habits and body image perceptions, setting the foundation for a healthier future if proper education is provided at an early growing phase of development.
Increased awareness, literacy and knowledge about diets, as well as groundwork that empowers and encourages families to get involved, can reinforce the effectiveness of educational interventions. The research showed that creating supportive environments and providing practical tools that enable behavioral changes is crucial, leading to improved overall health and reduced risk of diet-related diseases ( 4 ). Well-structured interventions must educate adolescents to understand and interpret nutrition information on food packaging, as well as provide them with a new understanding of the long-term consequences of unhealthy food intake. An effective intervention will also promote behavioral change techniques that help adolescents set goals, plan their meals, and track their progress. Group-based educational interventions can encourage adolescents to adopt healthier eating habits with the support of their peers.
Nutritional knowledge does not always translate into behavioral change, as significant shifts in behavior often demand a multidisciplinary approach. This is consistent with the findings of Yun and colleagues, who demonstrated that although all students from the University Brunei Darussalam knew the importance of a balanced diet, more than 50% of the students skipped breakfast and consumed fried food at least three times a week ( 30 ). Hence, the university was encouraged to form a multi-disciplinary team to be responsible for designing and conducting various programs to promote physical activity and reduce unhealthy eating habits.
It is important to note that program design and mode of administration will dictate the effectiveness of any nutritional education intervention. In this sense, continued evaluation of these programs is essential. Interventions must be well targeted in terms of the recipient population and time frame. A regular and repetitive intervention ( 20 ) of adequate duration ( 24 ) seems to achieve better results. Some studies even suggest that customized nutrition education programs are needed in the future ( 18 ). The use of innovative technology specifically adapted to young people such as movies ( 17 ), text messaging ( 18 ), or mobile apps ( 19 ) can be useful from the point of view of participant satisfaction and to increase adherence rates. As such, peer education might be helpful to boost both ( 24 ). These innovative solutions are also generally cost-effective, scalable and easy to implement ( 19 ). If the intervention is to be successful, it is essential to target feasible objectives and to use clear, simple, and culturally appropriate messages ( 18 , 21 ). It is also worth mentioning that nutritional interventions aimed at addressing obesity should never conflict with any negative effects on body image ( 26 ).
Apart from the large number of interventions and outcomes considered in the present review which demonstrate the potential of nutritional education interventions to address dietary problems and body image, other strengths of the reviewed studies include the fact that some of these innovative solutions have been explored and, overall, have shown positive compliance and a generally high rate of satisfaction, novel data have been collected, and a wide range of different methodological designs supported by robust statistical analysis have been implemented. As a matter of fact, our quality assessment yielded very good results, and all the reviewed articles were rated above 80%. Altogether, this provides a solid basis for future developments.
Notwithstanding the many merits of the methodologies used in the reviewed articles, some limitations emerge. These limitations may affect the generalization of the results, such as the use of relatively small sample sizes ( 17 , 21 ), homogeneous samples ( 21 ), or unbalanced samples across genders ( 18 ), or focusing on a specific population ( 17 , 24 ). They may affect the precision of measurement. For example, self-reported measures may have introduced potential biases ( 18 , 22 , 23 ), it was not possible to assess the quality of education or to ensure that it was provided equally in all cases ( 22 , 24 ) and one study notes that it may be necessary to keep working on the design of a reliable measure of body image ( 26 ). These limitations may also threaten the study’s validity as some experimental designs did not involve random allocation in the intervention and control groups ( 19 , 24 , 26 ). Moreover, potential contamination bias may have occurred ( 26 ) and the use of non-blinded methods may have overestimated intervention effects ( 23 ). The cross-sectional nature of the study is also acknowledged ( 19 ) and some articles agree on the need to conduct future longitudinal designs to assess long-term effects ( 18 ).
Further research is needed to validate the benefits of current educational material and to further explore other innovative technologies such as game-based interventions. Furthermore, it would be highly desirable to have more evidence of the suitability and effectiveness of the programs in terms of target population, duration, participation, adherence rates or mode of administration. More empirical analyses of longitudinal data are needed to test the long-run effects of nutrition education interventions in schools, universities and other educational settings and whether they improve eating habits and good health later in life. Finally, further research is needed to investigate the factors that lead to behavior change. While have found that there is consistent evidence of correlations between nutrition knowledge and food intake and body image, we are aware it is also important to consider the different ways in which correlations between nutritional education and food intake or body image may arise.
5 Conclusion
This systematic review highlights the impact of nutritional education interventions on improving the overall health and well-being of Asian adolescents by promoting healthier food choices and fostering positive body image perceptions. We strongly recommend continuing efforts to integrate nutritional education programs into educational settings to combat adolescent obesity and related health issues effectively, with a multidisciplinary approach in mind. Further research is needed to investigate the factors that lead to behavior change. While we found that there is consistent evidence of correlations between nutrition knowledge and food intake and body image, we are aware it is also important to consider the different ways in which correlations between nutritional education and food intake or body image may arise. The results of this study emphasize the importance of nutrition education for Asian adolescents to promote healthier choices and maintain a positive body image. Integrating nutrition education into an educational setting is therefore a proactive step to address these issues comprehensively. The far-reaching implications of this research are recommendations for policymakers, educators and healthcare providers. If implemented effectively, nutrition education could significantly improve the health and well-being of Asian adolescents.
Data availability statement
The original contributions presented in the study are included in the article/supplementary material, further inquiries can be directed to the corresponding author.
Author contributions
BP: Conceptualization, Data curation, Formal analysis, Investigation, Methodology, Resources, Software, Writing – original draft. SNA: Conceptualization, Formal analysis, Investigation, Methodology, Supervision, Validation, Writing – review & editing. SS: Conceptualization, Methodology, Supervision, Validation, Writing – review & editing. ZM: Supervision, Validation, Writing – review & editing. SRA: Conceptualization, Formal analysis, Investigation, Methodology, Supervision, Validation, Writing – review & editing.
The author(s) declare financial support was received for the research, authorship, and/or publication of this article. The article processing fee was sponsored by Univerisiti Brunei Darussalam.
Acknowledgments
The authors would like to thank the Universiti Brunei Darussalam (UBD).
Conflict of interest
The authors declare that the research was conducted in the absence of any commercial or financial relationships that could be construed as a potential conflict of interest.
Publisher’s note
All claims expressed in this article are solely those of the authors and do not necessarily represent those of their affiliated organizations, or those of the publisher, the editors and the reviewers. Any product that may be evaluated in this article, or claim that may be made by its manufacturer, is not guaranteed or endorsed by the publisher.
10 References
1. D’Addesa, D, D’Addezio, L, Martone, D, Censi, L, Scanu, A, Cairella, G, et al. Dietary intake and physical activity of normal weight and overweight/obese adolescents. Int J Pediatr . (2010) 2010:785649. doi: 10.1155/2010/785649
PubMed Abstract | Crossref Full Text | Google Scholar
2. Pereira, HRC, Bobbio, TG, Antonio, MRGM, and Barros Filho, ADA. Childhood and adolescent obesity: how many extra calories are responsible for excess of weight? Rev Paul Pediatr . (2013) 31:252–7. doi: 10.1590/s0103-05822013000200018
3. National Center for Chronic Disease Prevention and Health Promotion (U.S.). Comprehensive framework for addressing the school nutrition environment and services . Atlanta: Centers for Disease Control and Prevention (2019).
Google Scholar
4. Cunha, CM, Costa, PRF, de Oliveira, LPM, Queiroz, VAO, Pitangueira, JCD, and Oliveira, AM. Dietary patterns and cardiometabolic risk factors among adolescents: systematic review and meta-analysis. Br J Nutr . (2018) 119:859–79. doi: 10.1017/s0007114518000533
5. Vanhelst, J, Beghin, L, Duhamel, A, De Henauw, S, Ruiz, JR, Kafatos, A, et al. Do adolescents accurately evaluate their diet quality? The HELENA study. Clin Nutr . (2017) 36:1669–73. doi: 10.1016/j.clnu.2016.10.019
6. World Health Organization. Guideline: sugars intake for adults and children . Geneva: World Health Organization (2015).
7. Park, JH, Hahm, M-I, Kim, SJ, and Min, IS. Association between high-caffeine energy drink intake and suicidal ideation in Korean adolescents. J. Korean Soc. Sch. Health . (2016) 29:71–80. doi: 10.15434/kssh.2016.29.2.71
Crossref Full Text | Google Scholar
8. So, ES. Perceptual body image and the relationship with weight control across the adult lifespan by sex in Koreans. J Public Health . (2017) 39:777–86. doi: 10.1093/pubmed/fdx021
9. Griffiths, S, Murray, SB, Bentley, C, Gratwick-Sarll, K, Harrison, C, and Mond, JM. Sex differences in quality-of-life impairment associated with body dissatisfaction in adolescents. J Adolesc Health . (2017) 61:77–82. doi: 10.1016/j.jadohealth.2017.01.016
10. Patton, GC, Sawyer, SM, Santelli, JS, Ross, DA, Afifi, R, Allen, NB, et al. Our future: a lancet commission on adolescent health and wellbeing. Lancet . (2016) 387:2423–78. doi: 10.1016/S0140-6736(16)00579-1
11. Baker, JH, Higgins Neyland, MK, Thornton, LM, Runfola, CD, Larsson, H, Lichtenstein, P, et al. Body dissatisfaction in adolescent boys. Dev Psychol . (2019) 55:1566–78. doi: 10.1037/dev0000724
12. Van den Berg, P, Paxton, SJ, Keery, H, Wall, M, Guo, J, and Neumark-Sztainer, D. Body dissatisfaction and body comparison with media images in males and females. Body Image . (2007) 4:257–68. doi: 10.1016/j.bodyim.2007.04.003
13. Bornioli, A, Lewis-Smith, H, Smith, A, Slater, A, and Bray, I. Adolescent body dissatisfaction and disordered eating: predictors of later risky health behaviors. Soc Sci Med . (2019) 238:112458. doi: 10.1016/j.socscimed.2019.112458
14. Haines, J, and Neumark-Sztainer, D. Prevention of obesity and eating disorders: a consideration of shared risk factors. Health Educ Res . (2006) 21:770–82. doi: 10.1093/her/cyl094
15. Page, MJ, McKenzie, JE, Bossuyt, PM, Boutron, I, Hoffmann, TC, Mulrow, CD, et al. The PRISMA 2020 statement: an updated guideline for reporting systematic reviews. BMJ . (2021) 372:n71. doi: 10.1136/bmj.n71
16. Study Quality Assessment Tools (2013). Available at: https://www.nhlbi.nih.gov/health-topics/study-quality-assessment-tools
17. Marliya, M, and Muhammad, HFL. Introducing the new nutrition guideline to Indonesian overweight/obese adolescents using a short movie: the impact on nutritional knowledge, eating habit and dietary intake. Prog Nutr . (2019) 21:227–33. doi: 10.23751/pn.v21i1-S.5944
18. Shahril, MR, Wan Dali, WPE, and Lua, PL. A 10-week multimodal nutrition education intervention improves dietary intake among university students: cluster-randomised controlled trial. J Nutr Metab . (2013) 2013:658642. doi: 10.1155/2013/658642
19. Wang, M, Guo, Y, Zhang, Y, Xie, S, Yu, Z, Luo, J, et al. Promoting healthy lifestyle in Chinese college students: evaluation of a social media-based intervention applying the RE-AIM framework. Eur J Clin Nutr . (2021) 75:335–44. doi: 10.1038/s41430-020-0643-2
20. Singhal, N, Misra, A, Shah, P, and Gulati, S. Effects of controlled school-based multi-component model of nutrition and lifestyle interventions on behavior modification, anthropometry and metabolic risk profile of urban Asian Indian adolescents in North India. Eur J Clin Nutr . (2010) 64:364–73. doi: 10.1038/ejcn.2009.150
21. Chen, JL, Guedes, CM, and Lung, AE. Smartphone-based healthy weight management intervention for Chinese American adolescents: short-term efficacy and factors associated with decreased weight. J Adolesc Health . (2019) 64:443–9. doi: 10.1016/j.jadohealth.2018.08.022
22. Lee, JH, Lee, HS, Kim, H, Kwon, YJ, Shin, J, and Lee, JW. Association between nutrition education, dietary habits, and body image misperception in adolescents. Asia Pac J Clin Nutr . (2021) 30:512–21. doi: 10.6133/apjcn.202109_30(3).0018
23. Koo, HC, Poh, BK, and Ruzita, AT. GReat-child trial™ based on social cognitive theory improved knowledge, attitudes and practices toward whole grains among Malaysian overweight and obese children. BMC Public Health . (2019) 19:1574. doi: 10.1186/s12889-019-7888-5
24. Sharif Ishak, SIZ, Chin, YS, Taib, M, Nasir, M, Chan, YM, and Shariff, ZM. Effectiveness of a school-based intervention on knowledge, attitude and practice on healthy lifestyle and body composition in Malaysian adolescents. BMC Paediatr . (2020) 20:122. doi: 10.1186/s12887-020-02023-x
25. Tse, MMY, and Yuen, DTW. Effects of providing a nutrition education program for teenagers: dietary and physical activity patterns. Nurs Health Sci . (2009) 11:160–5. doi: 10.1111/j.1442-2018.2009.00443.x
26. Yeh, MC, Liou, YM, and Chien, LY. Development and effectiveness of a school programme on improving body image among elementary school students in Taiwan. J Adv Nurs . (2012) 68:434–43. doi: 10.1111/j.1365-2648.2011.05735.x
27. Beets, MW, Beighle, A, Erwin, HE, and Huberty, JL. After-school program impact on physical activity and fitness: a meta-analysis. Am J Prev Med . (2009) 36:527–37. doi: 10.1016/j.amepre.2009.01.033
28. Pate, RR, and O'Neill, JR. After-school interventions to increase physical activity among youth. Br J Sports Med . (2009) 43:14–8. doi: 10.1136/bjsm.2008.055517
29. Egan, CA, Webster, CA, Stewart, GL, Weaver, RG, Russ, LB, Brian, A, et al. Case study of a health optimizing physical education-based comprehensive school physical activity program. Eval Program Plann . (2019) 72:106–17. doi: 10.1016/j.evalprogplan.2018.10.006
30. Yun, TC, Ahmad, SR, and Quee, DSQ. Dietary habits and lifestyle practices among university students in Universiti Brunei Darussalam. Malays J Med Sci . (2018) 25:56–66. doi: 10.21315/mjms2018.25.3.6
Keywords: nutritional education, body image, Asian adolescents, perception, diet, food intake
Citation: Pushpa BS, Abdul Latif SN, Sharbini S, Murang ZR and Ahmad SR (2024) Nutrition education and its relationship to body image and food intake in Asian young and adolescents: a systematic review. Front. Nutr . 11:1287237. doi: 10.3389/fnut.2024.1287237
Received: 04 September 2023; Accepted: 21 February 2024; Published: 22 March 2024.
Reviewed by:
Copyright © 2024 Pushpa, Abdul Latif, Sharbini, Murang and Ahmad. This is an open-access article distributed under the terms of the Creative Commons Attribution License (CC BY) . The use, distribution or reproduction in other forums is permitted, provided the original author(s) and the copyright owner(s) are credited and that the original publication in this journal is cited, in accordance with accepted academic practice. No use, distribution or reproduction is permitted which does not comply with these terms.
*Correspondence: Baladandapla Shivappa Pushpa, [email protected]
This article is part of the Research Topic
Intuitive Eating, Health, and Body: Research, Prevention, and Treatment

- News Releases
Illinois study: Systematic review of agricultural injuries can help inform safety measures
University of Illinois College of Agricultural, Consumer and Environmental Sciences
University of Illinois researchers reviewed U.S. and global literature on agricultural injuries, helping to inform safety education and policies.
Credit: College of ACES
URBANA, Ill. – Agricultural occupations are hazardous with one of the highest rates of workplace injuries and fatalities in the U.S. The manual and often strenuous nature of the work, combined with the use of machinery and exposure to environmental hazards create a challenging work environment. Understanding the nature and causes of injuries can help improve safety guidelines and policy measures. However, obtaining a comprehensive overview of injuries is hindered by the absence of a central reporting system. Two new papers from the University of Illinois Urbana-Champaign provide a systematic review of academic literature on agricultural injuries in the U.S. and globally.
“When it comes to agriculture, there's no single source for injury data. In other occupations, work injuries in the U.S. must be reported to the Occupational Safety and Health Administration (OSHA), but farm work is often exempt from these requirements because many farms are small and have less than 10 full-time employees,” said Salah Issa , an assistant professor in the Department of Agricultural and Biological Engineering (ABE) and an Illinois Extension specialist; both units are part of the College of Agricultural, Consumer and Environmental Sciences (ACES) at Illinois. ABE is also part of The Grainger College of Engineering at Illinois.
“There have been a lot of grassroots efforts to track surveillance data, but they are based on different methods so it’s hard to get a complete look at agricultural injuries. Our work combines results into one large dataset, providing a comprehensive overview of previous research,” Issa explained.
In the first study, the researchers conducted a systematic literature review of 48 academic papers published in the U.S. and Canada from 1985 to 2022.
“We identified five different surveillance methods: newspaper clippings, surveys, death certificates, hospital records and emergency medical services (EMS) data, and multiple sources,” said Sihan Li , a doctoral student in ABE and lead author on the first paper.
The researchers also analyzed and categorized information such as the type and source of injury, the event leading up to it, and the gender of the victim.
Overall, vehicles (including tractors and ATVs) were the most common source of injury, with over 55,000 incidents reported, as well as the leading source of fatalities. Other significant causes of injury included machinery, slips and trips, animals, chemicals, and tools. Men were more than twice as likely as women to be victims of injury. Age varied by surveillance method, with newspaper clippings skewed to younger victims (22% of incidents) and death certificates skewed to older victims (30% over 65).
In the second study , the researchers reviewed 69 articles from 17 countries in North America, Europe, and Asia, including the U.S., Canada, Turkey, India, Pakistan, Austria, Italy, and others.
The main data sources identified in these studies were hospital records, followed by surveys, government records (including death certificates), insurance claims, and multiple sources.
“For the global perspective, we narrowed our scope to focus primarily on machine-related injuries, which involves tractors and farm equipment,” said Mian Muhammad Sajid Raza , a doctoral student in ABE and lead author on the second paper.
The researchers found that tractors stand out as the leading cause of fatal incidents, with tractor overturns accounting for 45% of all machinery-related incidents in North America. Furthermore, injuries linked overall to agricultural machinery significantly contribute to both fatal and non-fatal incidents.
“It is also interesting to look at other sources of injury. In North America and Europe, animals are the cause of less than 3% of all injuries. But in Asia, animals represent 7% of the total injuries and 35% of the fatalities. This is likely because farming is less automated and animals are still used extensively in some Asian countries,” Raza said.
The research shows agriculture is a dangerous occupation globally, with injuries reported in at least three continents. Overall trends are as expected, with vehicles and machinery playing a large role in injuries and fatalities, Issa noted.
“One of our most important findings is that the way you conduct injury surveillance will have an impact on your results,” he said. “For example, if you use newspaper clippings, your findings will skew towards a younger age group. The discrepancies are so large it’s clearly worth evaluating the type of surveillance methods employed, and it’s important to use multiple sources to get a good picture of what’s going on.”
Understanding the nature and source of injuries is important for developing educational programs and interventions, Issa concluded.
Both papers, “Agricultural Injury Surveillance in the United States and Canada: A Systematic Literature Review’ [DOI: 10.1080/1059924X.2024.2304699 ] and “Global Patterns of Agricultural Machine and Equipment Injuries- A Systematic Literature Review” [DOI: 10.1080/1059924X.2024.2304704 ] are published in the Journal of Agromedicine.
Journal of Agromedicine
10.1080/1059924X.2024.2304699
Method of Research
Literature review
Article Title
Agricultural Injury Surveillance in the United States and Canada: A Systematic Literature Review
Article Publication Date
22-Jan-2024
Disclaimer: AAAS and EurekAlert! are not responsible for the accuracy of news releases posted to EurekAlert! by contributing institutions or for the use of any information through the EurekAlert system.
Original Source
Image-based artificial intelligence for the prediction of pathological complete response to neoadjuvant chemoradiotherapy in patients with rectal cancer: a systematic review and meta-analysis
- Diagnostic Imaging in Oncology
- Published: 21 March 2024
Cite this article
- Hui Shen 1 na1 ,
- Zhe Jin 1 na1 ,
- Qiuying Chen 1 ,
- Lu Zhang 1 ,
- Jingjing You 1 ,
- Shuixing Zhang 1 &
- Bin Zhang ORCID: orcid.org/0000-0002-6286-6227 1
48 Accesses
Explore all metrics
Artificial intelligence (AI) holds enormous potential for noninvasively identifying patients with rectal cancer who could achieve pathological complete response (pCR) following neoadjuvant chemoradiotherapy (nCRT). We aimed to conduct a meta-analysis to summarize the diagnostic performance of image-based AI models for predicting pCR to nCRT in patients with rectal cancer.
This study followed the Preferred Reporting Items for Systematic Reviews and Meta-Analyses guidelines. A literature search of PubMed, Embase, Cochrane Library, and Web of Science was performed from inception to July 29, 2023. Studies that developed or utilized AI models for predicting pCR to nCRT in rectal cancer from medical images were included. The Quality Assessment of Diagnostic Accuracy Studies-AI was used to appraise the methodological quality of the studies. The bivariate random-effects model was used to summarize the individual sensitivities, specificities, and areas-under-the-curve (AUCs). Subgroup and meta-regression analyses were conducted to identify potential sources of heterogeneity. Protocol for this study was registered with PROSPERO (CRD42022382374).
Thirty-four studies (9933 patients) were identified. Pooled estimates of sensitivity, specificity, and AUC of AI models for pCR prediction were 82% (95% CI: 76–87%), 84% (95% CI: 79–88%), and 90% (95% CI: 87–92%), respectively. Higher specificity was seen for the Asian population, low risk of bias, and deep-learning, compared with the non-Asian population, high risk of bias, and radiomics (all P < 0.05). Single-center had a higher sensitivity than multi-center ( P = 0.001). The retrospective design had lower sensitivity ( P = 0.012) but higher specificity ( P < 0.001) than the prospective design. MRI showed higher sensitivity ( P = 0.001) but lower specificity ( P = 0.044) than non-MRI. The sensitivity and specificity of internal validation were higher than those of external validation (both P = 0.005).
Conclusions
Image-based AI models exhibited favorable performance for predicting pCR to nCRT in rectal cancer. However, further clinical trials are warranted to verify the findings.
This is a preview of subscription content, log in via an institution to check access.
Access this article
Price includes VAT (Russian Federation)
Instant access to the full article PDF.
Rent this article via DeepDyve
Institutional subscriptions
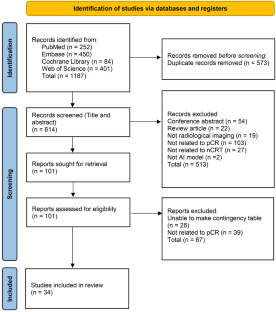
Data availability
The original data generated in the current study are available from the corresponding author on reasonable request.
Siegel RL, Wagle NS, Cercek A, Smith RA, Jemal A (2023) Colorectal cancer statistics. A Cancer J Clin 73(3):233–254
Article Google Scholar
Watanabe T, Muro K, Ajioka Y, Hashiguchi Y, Ito Y, Saito Y et al (2018) Japanese society for cancer of the colon and rectum (JSCCR) guidelines 2016 for the treatment of colorectal cancer. Int J Clin Oncol 23(1):1–34
Article PubMed Google Scholar
Wan T, Zhang XF, Liang C, Liao CW, Li JY, Zhou YM (2019) The prognostic value of a pathologic complete response after neoadjuvant therapy for digestive cancer: systematic review and meta-analysis of 21 studies. Ann Surg Oncol 26(5):1412–1420
Maas M, Nelemans PJ, Valentini V, Das P, Rödel C, Kuo LJ et al (2010) Long-term outcome in patients with a pathological complete response after chemoradiation for rectal cancer: a pooled analysis of individual patient data. Lancet Oncol 11(9):835–844
Marijnen CA (2015) Organ preservation in rectal cancer: have all questions been answered? Lancet Oncol 16(1):e13-22
Smith JJ, Paty PB, Garcia-Aguilar J (2020) Watch and wait in rectal cancer or more wait and see? JAMA Surg 155(7):657–658
Article PubMed PubMed Central Google Scholar
LeBlanc JK (2007) Imaging and management of rectal cancer. Nat Clin Pract Gastroenterol Hepatol 4(12):665–676
Yan J, Zhang B, Zhang S, Cheng J, Liu X, Wang W et al (2021) Quantitative MRI-based radiomics for noninvasively predicting molecular subtypes and survival in glioma patients. NPJ Precision Oncol 5(1):72
Article CAS Google Scholar
Gollub MJ, Blazic I, Felder S, Knezevic A, Gonen M, Garcia-Aguilar J et al (2019) Value of adding dynamic contrast-enhanced MRI visual assessment to conventional MRI and clinical assessment in the diagnosis of complete tumour response to chemoradiotherapy for rectal cancer. Eur Radiol 29(3):1104–1113
Park SH, Cho SH, Choi SH, Jang JK, Kim MJ, Kim SH et al (2020) MRI assessment of complete response to preoperative chemoradiation therapy for rectal cancer: 2020 guide for practice from the korean society of abdominal radiology. Korean J Radiol 21(7):812–828
Schurink NW, van Kranen SR, Roberti S, van Griethuysen JJM, Bogveradze N, Castagnoli F et al (2022) Sources of variation in multicenter rectal MRI data and their effect on radiomics feature reproducibility. Eur Radiol 32(3):1506–1516
Gillies RJ, Kinahan PE, Hricak H (2016) Radiomics: images are more than pictures. They Are Data Radiol 278(2):563–577
Google Scholar
Tomaszewski MR, Gillies RJ (2021) The biological meaning of radiomic features. Radiology 299(2):E256
Jia LL, Zheng QY, Tian JH, He DL, Zhao JX, Zhao LP et al (2022) Artificial intelligence with magnetic resonance imaging for prediction of pathological complete response to neoadjuvant chemoradiotherapy in rectal cancer: a systematic review and meta-analysis. Front Oncol 12:1026216
Article CAS PubMed PubMed Central Google Scholar
Park SH, Han K (2018) Methodologic guide for evaluating clinical performance and effect of artificial intelligence technology for medical diagnosis and prediction. Radiology 286(3):800–809
Park SH, Kressel HY (2018) Connecting technological innovation in artificial intelligence to real-world medical practice through rigorous clinical validation: what peer-reviewed medical journals could do. J Korean Med Sci 33(22):e152
Kirienko M, Sollini M, Ninatti G, Loiacono D, Giacomello E, Gozzi N et al (2021) Distributed learning: a reliable privacy-preserving strategy to change multicenter collaborations using AI. Eur J Nucl Med Mol Imaging 48(12):3791–3804
Jin C, Chen W, Cao Y, Xu Z, Tan Z, Zhang X et al (2020) Development and evaluation of an artificial intelligence system for COVID-19 diagnosis. Nat Commun 11(1):5088
Reichstein M, Camps-Valls G, Stevens B, Jung M, Denzler J, Carvalhais N et al (2019) Deep learning and process understanding for data-driven Earth system science. Nature 566(7743):195–204
Article CAS PubMed Google Scholar
Page MJ, McKenzie JE, Bossuyt PM, Boutron I, Hoffmann TC, Mulrow CD et al (2021) The PRISMA 2020 statement: an updated guideline for reporting systematic reviews. BMJ (Clin Res ed) 372:n71
Sounderajah V, Ashrafian H, Rose S, Shah NH, Ghassemi M, Golub R et al (2021) A quality assessment tool for artificial intelligence-centered diagnostic test accuracy studies: QUADAS-AI. Nat Med 27(10):1663–1665
Whiting PF, Rutjes AW, Westwood ME, Mallett S, Deeks JJ, Reitsma JB et al (2011) QUADAS-2: a revised tool for the quality assessment of diagnostic accuracy studies. Ann Intern Med 155(8):529–536
Yang B, Mallett S, Takwoingi Y, Davenport CF, Hyde CJ, Whiting PF et al (2021) QUADAS-C: a tool for assessing risk of bias in comparative diagnostic accuracy studies. Ann Intern Med 174(11):1592–1599
Altman DG, Bland JM (2003) Interaction revisited: the difference between two estimates. BMJ (Clin Res ed) 326(7382):219
Abbaspour S, Abdollahi H, Arabalibeik H, Barahman M, Arefpour AM, Fadavi P, Ay M, Mahdavi SR (2022) Endorectal ultrasound radiomics in locally advanced rectal cancer patients: despeckling and radiotherapy response prediction using machine learning. Abdom Radiol 47(11):3645–3659
Antunes JT, Ofshteyn A, Bera K, Wang EY, Brady JT, Willis JE et al (2020) Radiomic features of primary rectal cancers on baseline T-2-weighted MRI are associated with pathologic complete response to neoadjuvant chemoradiation: a multisite study. J Magn Reson Imaging 52(5):1531–1541
Bibault JE, Giraud P, Housset M, Durdux C, Taieb J, Berger A et al (2018) Deep learning and radiomics predict complete response after neo-adjuvant chemoradiation for locally advanced rectal cancer. Sci Rep 8(1):12611
Boldrini L, Lenkowicz J, Orlandini LC, Yin G, Cusumano D, Chiloiro G et al (2022) Applicability of a pathological complete response magnetic resonance-based radiomics model for locally advanced rectal cancer in intercontinental cohort. Radiat Oncol (London, England) 17(1):78
Bordron A, Rio E, Badic B, Miranda O, Pradier O, Hatt M, Visvikis D, Lucia F, Schick U, Bourbonne V (2022) External validation of a radiomics model for the prediction of complete response to neoadjuvant chemoradiotherapy in rectal cancer. Cancers 14(4):1079
Bulens P, Couwenberg A, Intven M, Debucquoy A, Vandecaveye V, Van Cutsem E et al (2020) Predicting the tumor response to chemoradiotherapy for rectal cancer: model development and external validation using MRI radiomics. Radiother Oncol 142:246–252
Cheng Y, Luo Y, Hu Y, Zhang Z, Wang X, Yu Q et al (2021) Multiparametric MRI-based radiomics approaches on predicting response to neoadjuvant chemoradiotherapy (nCRT) in patients with rectal cancer. Abdom Radiol (New York) 46(11):5072–5085
Chiloiro G, Cusumano D, Romano A, Boldrini L, Nicolì G, Votta C, Tran HE, Barbaro B, Carano D, Valentini V, Gambacorta MA (2023) Delta radiomic analysis of mesorectum to predict treatment response and prognosis in locally advanced rectal cancer. Cancers 15(12):3082
Cui Y, Yang X, Shi Z, Yang Z, Du X, Zhao Z et al (2019) Radiomics analysis of multiparametric MRI for prediction of pathological complete response to neoadjuvant chemoradiotherapy in locally advanced rectal cancer. Eur Radiol 29(3):1211–1220
Feng L, Liu Z, Li C, Li Z, Lou X, Shao L et al (2022) Development and validation of a radiopathomics model to predict pathological complete response to neoadjuvant chemoradiotherapy in locally advanced rectal cancer: a multicentre observational study. Lancet Digital health 4(1):e8–e17
Ferrari R, Mancini-Terracciano C, Voena C, Rengo M, Zerunian M, Ciardiello A et al (2019) MR-based artificial intelligence model to assess response to therapy in locally advanced rectal cancer. Eur J Radiol 118:1–9
Horvat N, Veeraraghavan H, Khan M, Blazic I, Zheng J, Capanu M et al (2018) MR imaging of rectal cancer: radiomics analysis to assess treatment response after neoadjuvant therapy. Radiology 287(3):833–843
Horvat N, Veeraraghavan H, Nahas CSR, Bates DDB, Ferreira FR, Zheng J et al (2022) Combined artificial intelligence and radiologist model for predicting rectal cancer treatment response from magnetic resonance imaging: an external validation study. Abdom Radiol (New York) 47(8):2770–2782
Jang BS, Lim YJ, Song C, Jeon SH, Lee KW, Kang SB et al (2021) Image-based deep learning model for predicting pathological response in rectal cancer using post-chemoradiotherapy magnetic resonance imaging. Radiother Oncol: J Eur Soc Ther Radiol Oncol 161:183–190
Jayaprakasam VS, Paroder V, Gibbs P, Bajwa R, Gangai N, Sosa RE et al (2022) MRI radiomics features of mesorectal fat can predict response to neoadjuvant chemoradiation therapy and tumor recurrence in patients with locally advanced rectal cancer. Eur Radiol 32(2):971–980
Jin C, Yu H, Ke J, Ding P, Yi Y, Jiang X et al (2021) Predicting treatment response from longitudinal images using multi-task deep learning. Nat Commun 12(1):1851
Lee S, Lim J, Shin J, Kim S, Hwang H (2021) Pathologic complete response prediction after neoadjuvant chemoradiation therapy for rectal cancer using radiomics and deep embedding network of MRI. Appl Sci 11(20):9494
Li Z, Ma X, Shen F, Lu H, Xia Y, Lu J (2021) Evaluating treatment response to neoadjuvant chemoradiotherapy in rectal cancer using various MRI-based radiomics models. BMC Med Imaging 21(1):30
Liu Z, Zhang XY, Shi YJ, Wang L, Zhu HT, Tang Z et al (2017) Radiomics analysis for evaluation of pathological complete response to neoadjuvant chemoradiotherapy in locally advanced rectal cancer. Clin Cancer Res: An Official J Am Associat Cancer Res 23(23):7253–7262
Mao Y, Pei Q, Fu Y, Liu H, Chen C, Li H et al (2022) Pre-Treatment computed tomography radiomics for predicting the response to neoadjuvant chemoradiation in locally advanced rectal cancer: a retrospective study. Front Oncol 12:850774
Nardone V, Reginelli A, Grassi R, Vacca G, Giacobbe G, Angrisani A, Clemente A, Danti G, Correale P, Carbone SF, Pirtoli L (2022) Ability of delta radiomics to predict a complete pathological response in patients with loco-regional rectal cancer addressed to neoadjuvant chemo-radiation and surgery. Cancers 14(12):3004
Pang X, Wang F, Zhang Q, Li Y, Huang R, Yin X et al (2021) A Pipeline for predicting the treatment response of neoadjuvant chemoradiotherapy for locally advanced rectal cancer using single mri modality: combining deep segmentation network and radiomics analysis based on “suspicious region.” Front Oncol 11:711747
Petkovska I, Tixier F, Ortiz EJ, Golia Pernicka JS, Paroder V, Bates DD et al (2020) Clinical utility of radiomics at baseline rectal MRI to predict complete response of rectal cancer after chemoradiation therapy. Abdom Radiol (New York) 45(11):3608–3617
Shen WC, Chen SW, Wu KC, Lee PY, Feng CL, Hsieh TC et al (2020) Predicting pathological complete response in rectal cancer after chemoradiotherapy with a random forest using (18)F-fluorodeoxyglucose positron emission tomography and computed tomography radiomics. Ann Translat Med 8(5):207
Shin J, Seo N, Baek SE, Son NH, Lim JS, Kim NK et al (2022) MRI radiomics model predicts pathologic complete response of rectal cancer following chemoradiotherapy. Radiology 303(2):351–358
Tochigi T, Kamran SC, Parakh A, Noda Y, Ganeshan B, Blaszkowsky LS et al (2022) Response prediction of neoadjuvant chemoradiation therapy in locally advanced rectal cancer using CT-based fractal dimension analysis. Eur Radiol 32(4):2426–2436
Wan L, Peng W, Zou S, Ye F, Geng Y, Ouyang H et al (2021) MRI-based delta-radiomics are predictive of pathological complete response after neoadjuvant chemoradiotherapy in locally advanced rectal cancer. Acad Radiol 28(Suppl 1):S95-s104
Wang D, Lee SH, Geng H, Zhong H, Plastaras J, Wojcieszynski A et al (2022) Interpretable machine learning for predicting pathologic complete response in patients treated with chemoradiation therapy for rectal adenocarcinoma. Front Artif Intelligence 5:1059033
Wei Q, Chen Z, Tang Y, Chen W, Zhong L, Mao L et al (2023) External validation and comparison of MR-based radiomics models for predicting pathological complete response in locally advanced rectal cancer: a two-centre, multi-vendor study. Eur Radiol 33(3):1906–1917
Wen L, Liu J, Hu P, Bi F, Liu S, Jian L, Zhu S, Nie S, Cao F, Lu Q, Yu X (2023) MRI-based radiomic models outperform radiologists in predicting pathological complete response to neoadjuvant chemoradiotherapy in locally advanced rectal cancer. Acad Radiol 30:S176–S184
Yardimci AH, Kocak B, Sel I, Bulut H, Bektas CT, Cin M et al (2023) Radiomics of locally advanced rectal cancer: machine learning-based prediction of response to neoadjuvant chemoradiotherapy using pre-treatment sagittal T2-weighted MRI. Jpn J Radiol 41(1):71–82
Zhang XY, Wang L, Zhu HT, Li ZW, Ye M, Li XT et al (2020) Predicting rectal cancer response to neoadjuvant chemoradiotherapy using deep learning of diffusion kurtosis MRI. Radiology 296(1):56–64
Zhu HT, Zhang XY, Shi YJ, Li XT, Sun YS (2022) The conversion of MRI data with multiple b-values into signature-like pictures to predict treatment response for rectal cancer. J Magn Resonance Imaging: JMRI 56(2):562–569
Zhuang Z, Liu Z, Li J, Wang X, Xie P, Xiong F et al (2021) Radiomic signature of the FOWARC trial predicts pathological response to neoadjuvant treatment in rectal cancer. J Transl Med 19(1):256
Lu L, Phua QS, Bacchi S, Goh R, Gupta AK, Kovoor JG et al (2022) Small study effects in diagnostic imaging accuracy: a meta-analysis. JAMA Netw Open 5(8):e2228776
Lian S, Liu H, Meng T, Ma L, Zeng W, Xie C (2023) Quantitative synthetic MRI for predicting locally advanced rectal cancer response to neoadjuvant chemoradiotherapy. Eur Radiol 33(3):1737–1745
Lambin P, Rios-Velazquez E, Leijenaar R, Carvalho S, van Stiphout RG, Granton P et al (2012) Radiomics: extracting more information from medical images using advanced feature analysis. Eur J Cancer 48(4):441–446
Traverso A, Wee L, Dekker A, Gillies R (2018) Repeatability and reproducibility of radiomic features: a systematic review. Int J Radiat Oncol Biol Phys 102(4):1143–1158
Gidwani M, Chang K, Patel JB, Hoebel KV, Ahmed SR, Singh P et al (2023) Inconsistent partitioning and unproductive feature associations yield idealized radiomic models. Radiology 307(1):e220715
Jiao Y, Li J, Qian C, Fei S (2021) Deep learning-based tumor microenvironment analysis in colon adenocarcinoma histopathological whole-slide images. Comput Methods Programs Biomed 204:106047
Chen W, Liu Y, Wang W, Bakker EM, Georgiou T, Fieguth P et al (2023) Deep learning for instance retrieval: a survey. IEEE Trans Pattern Anal Mach Intell 45(6):7270–7292
Guyatt GH, Oxman AD, Vist G, Kunz R, Brozek J, Alonso-Coello P et al (2011) GRADE guidelines: 4. Rating the quality of evidence–study limitations (risk of bias). J Clin Epidemiol 64(4):407–415
Orlhac F, Eertink JJ, Cottereau AS, Zijlstra JM, Thieblemont C, Meignan M et al (2022) A guide to ComBat Harmonization of Imaging biomarkers in multicenter studies. J Nucl Med 63(2):172–179
Download references
Acknowledgements
We acknowledge financial support from National Key Research and Development Program of China (2023YFF1204600); National Natural Science Foundation of China (82227802, 82302306); Clinical Frontier Technology Program of the First Affiliated Hospital of Jinan University, China (JNU1AF-CFTP-2022-a01201); Science and Technology Projects in Guangzhou (202201020022, 2023A03J1036, 2023A03J1038); Science and Technology Youth Talent Nurturing Program of Jinan University (21623209); and Postdoctoral Science Foundation of China (2022M721349).
The authors have not disclosed any funding.
Author information
Hui Shen and Zhe Jin contributed equally to this study.
Authors and Affiliations
Department of Radiology, The First Affiliated Hospital of Jinan University, No. 613 Huangpu West Road, Tianhe District, Guangzhou, 510627, Guangdong, China
Hui Shen, Zhe Jin, Qiuying Chen, Lu Zhang, Jingjing You, Shuixing Zhang & Bin Zhang
You can also search for this author in PubMed Google Scholar
Contributions
Conception and design of the research were involved by HS and BZ; acquisition and interpretation of the data were performed by HS, BZ, ZJ, and QYC; statistical analysis and writing of the manuscript were done by HS and BZ; critical revision of the manuscript was contributed by all authors. All authors reviewed the manuscript, approved the submitted version, had full access to all the raw data in the study, and had final responsibility for the decision to submit for publication.
Corresponding author
Correspondence to Bin Zhang .
Ethics declarations
Conflict of interest.
All authors declare that there is no conflict of interest.
Ethical approval
This article does not contain any studies with human participants or animals performed by any of the authors.
Additional information
Publisher's note.
Springer Nature remains neutral with regard to jurisdictional claims in published maps and institutional affiliations.
Supplementary Information
Below is the link to the electronic supplementary material.
Supplementary file1 (DOCX 31323 kb)
Rights and permissions.
Springer Nature or its licensor (e.g. a society or other partner) holds exclusive rights to this article under a publishing agreement with the author(s) or other rightsholder(s); author self-archiving of the accepted manuscript version of this article is solely governed by the terms of such publishing agreement and applicable law.
Reprints and permissions
About this article
Shen, H., Jin, Z., Chen, Q. et al. Image-based artificial intelligence for the prediction of pathological complete response to neoadjuvant chemoradiotherapy in patients with rectal cancer: a systematic review and meta-analysis. Radiol med (2024). https://doi.org/10.1007/s11547-024-01796-w
Download citation
Received : 21 November 2023
Accepted : 24 January 2024
Published : 21 March 2024
DOI : https://doi.org/10.1007/s11547-024-01796-w
Share this article
Anyone you share the following link with will be able to read this content:
Sorry, a shareable link is not currently available for this article.
Provided by the Springer Nature SharedIt content-sharing initiative
- Artificial intelligence
- Diagnostic imaging
- Rectal neoplasms
- Pathologic complete response
- Deep-learning
- Meta-analysis
- Find a journal
- Publish with us
- Track your research
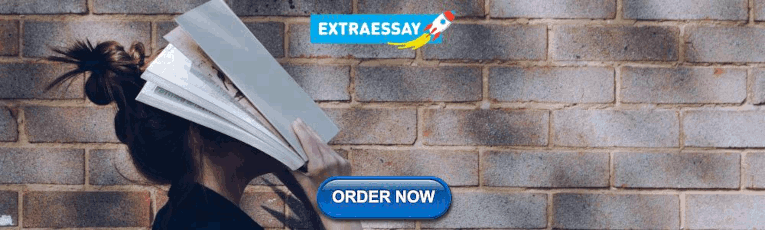
IMAGES
VIDEO
COMMENTS
In this study a comprehensive Systematic Literature Review (SLR) provides a brief overview of improvements in image captioning over the last four years. The main focus of the paper is to explain the most common techniques and the biggest challenges in image captioning and to summarize the results from the newest papers.
Data augmentation is a strategy to increase the diversity and amount of data available for training DNNs, without actually collecting new samples [4]. This is achieved by applying several image manipulation techniques on the original data or by creating new samples by means of generative models.
The purpose of this paper is to provide an overview of the literature on image information needs and behaviors.,Following an eight-step procedure for conducting systematic literature reviews, the paper presents an analysis of peer-reviewed work on image information needs and behaviors, with publications ranging from the years 1997 to 2019 ...
In this systematic literature review, we follow a systematic approach to investigate state-of-the-art image captioning research. We use a PRISMA-based[] approach to guide the review process, as Fig. 1 shows.2.1 Research Questions. Firstly, we formulate three research questions (RQs) to construct the review:
In this study a comprehensive Systematic Literature Review (SLR) provides a brief overview of improvements in image captioning over the last four years. The main focus of the paper is to explain ...
holistic systematic review of the literature was long overdue. The way users find image information has evolved and diversified due to technological developments in image retrieval. By synthesizing this burgeoning field into specific foci, this systematic literature review provides a foundation for future empirical investigation.
In this study a comprehensive Systematic Literature Review (SLR) provides a brief overview of improvements in image captioning over the last four years and to summarize the results from the newest papers. Natural language problems have already been investigated for around five years. Recent progress in artificial intelligence (AI) has greatly improved the performance of models. However, the ...
In this study a comprehensive Systematic Literature Review (SLR) provides a brief overview of improvements in image captioning over the last four years. The main focus of the paper is to explain ...
The purpose of this paper is to provide an overview of the literature on image information needs and behaviors. Design/methodology/approach: Following an eight-step procedure for conducting systematic literature reviews, the paper presents an analysis of peer-reviewed work on image information needs and behaviors, with publications ranging from ...
An analysis of peer-reviewed work on image information needs and behaviors, with publications ranging from the years 1997 to 2019, provides an overview of the literature and pinpoints key research gaps to investigate, particularly the influence of user expertise. PurposeWith ready access to search engines and social media platforms, the way people find image information has evolved and ...
Image captioning is a technology that generates textual descriptions of images by integrating computer vision and natural language processing. This review aims to provide a comprehensive overview ...
Systematic literature review on approaches of extracting image merits. Texture analysis is gaining popularity among the scientific community. A wide variety of applications use texture analysis method. Texture analysis methods can be used for image segmentation, pattern analysis and pattern classification tasks.
Method details Overview. A Systematic Literature Review (SLR) is a research methodology to collect, identify, and critically analyze the available research studies (e.g., articles, conference proceedings, books, dissertations) through a systematic procedure [12].An SLR updates the reader with current literature about a subject [6].The goal is to review critical points of current knowledge on a ...
The aims of this paper are threefold: first, via a systematic literature review, we identify high-quality scholarly articles published between 1991 and 2016 that measure brand image. To provide an overview of the brand image measurement techniques used most frequently in marketing research, 224 articles were identified and analyzed.
Our systematic literature review collected many studies related to our search topic over the last five years, between 2017 and 2021, from the following databases: Elsevier, IEEE Xplorer, Springer, and Google Scholar. ... A Review of Image Processing Techniques Common in Human and Plant Disease Diagnosis. Symmetry. 2018; 10:270. doi: 10.3390 ...
1.1 Prior research. There have been multiple review papers and comprehensive surveys published between the period 2015-2021 on medical image fusion. Tawfik et al. [] conducted a survey of 65 papers and categorized the methods into six pixel-level, feature-level, and decision-level fusion techniques with clearly defined advantages and disadvantages.. Huang et al. [] reviewed 11 multi-modal ...
A systematic review of the literature on EIC and AIC approaches published in the past nine years (2015-2023) from well-known databases (Google Scholar, ScienceDirect, IEEE Xplore) is undertaken. We have identified 52 primary English and Arabic studies relevant to our objectives (The number of articles on Arabic captioning is 11, and the rest ...
A Systematic Literature Review of Medical Image Analysis Using Deep Learning @article{Buettner2020ASL, title={A Systematic Literature Review of Medical Image Analysis Using Deep Learning}, author={Ricardo Buettner and Marcus Bilo and Nico Bay and Toni Zubac}, journal={2020 IEEE Symposium on Industrial Electronics \& Applications (ISIEA)}, year ...
Abstract: We review literature in top journals and conferences on the usage of deep learning for medical image analysis in modern healthcare. As a result it is shown that deep learning offers unique capabilities and breakthroughs in identifying, classifying and segmenting different kinds of medical images, especially related to cancer in the breast, lung, and brain.
To provide an updated overview of the research works in Deepfake detection, we conduct a systematic literature review (SLR) in this paper, summarizing 112 relevant articles from 2018 to 2020 that presented a variety of methodologies. We analyze them by grouping them into four different categories: deep learning-based techniques, classical ...
Abstract. Objective This review article explores the connection between self-esteem and body image. It has been demonstrated that body image, particularly in respect to physical appearance, has a ...
Recent improvements in Machine Learning (ML), specifically in Deep Learning (DL), help in identifying, classifying and measuring patterns in medical images. This paper is focused on the Systematic Literature Review (SLR) of various microservice events like image localization, segmentation, detection, and classification tasks.
Securely automate every stage of your literature review to produce evidence-based research faster, more accurately, and more transparently at scale. Rayyan A web-tool designed to help researchers working on systematic reviews, scoping reviews and other knowledge synthesis projects, by dramatically speeding up the process of screening and ...
BackgroundThe literature brings to light the unhealthy nutritional habits prevalent among Asian adolescents and their high level of body image dissatisfaction. This study aims to conduct a systematic review of the literature on the effect of nutritional education interventions on their nutritional knowledge and food intake behavior, attitude, practice, and body image.MethodsWe searched ...
This paper presents an SLR on multi-modal medical image registration. A systematic review is a means of identifying, evaluating and interpreting all available research relevant to a particular research question (RQ), topic area, or phenomenon of interest in an unbiased and repeatable manner [ 10 ].
PDF | On Jan 25, 2022, Muhammad Adnan Aslam and others published Image Steganography using Least Significant Bit (LSB) - A Systematic Literature Review | Find, read and cite all the research you ...
In the first study, the researchers conducted a systematic literature review of 48 academic papers published in the U.S. and Canada from 1985 to 2022. "We identified five different surveillance ...
This study followed the Preferred Reporting Items for Systematic Reviews and Meta-Analyses guidelines. A literature search of PubMed, Embase, Cochrane Library, and Web of Science was performed from inception to July 29, 2023. Studies that developed or utilized AI models for predicting pCR to nCRT in rectal cancer from medical images were included.