
An official website of the United States government
The .gov means it’s official. Federal government websites often end in .gov or .mil. Before sharing sensitive information, make sure you’re on a federal government site.
The site is secure. The https:// ensures that you are connecting to the official website and that any information you provide is encrypted and transmitted securely.
- Publications
- Account settings
Preview improvements coming to the PMC website in October 2024. Learn More or Try it out now .
- Advanced Search
- Journal List
- HHS Author Manuscripts

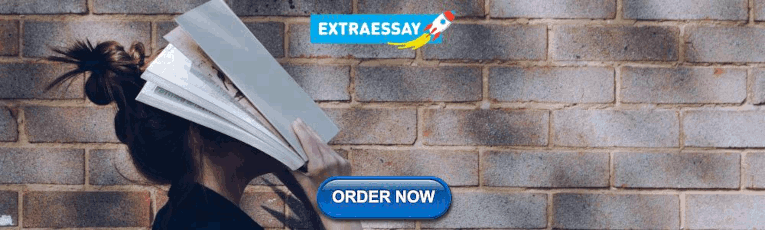
A Review of the Literature on Remote Monitoring Technology in Incentive-Based Interventions for Health-Related Behavior Change
Use of technology (e.g., Internet, cell phones) to allow remote implementation of incentives interventions for health-related behavior change is growing. To our knowledge, there has yet to be a systematic review of this literature reported. The present report provides a systematic review of the controlled studies where technology was used to remotely implement financial incentive interventions targeting substance use and other health behaviors published between 2004 and 2015. For inclusion in the review, studies had to use technology to remotely accomplish one of the following two aims alone or in combination: (a) monitor the target behavior, or (b) deliver incentives for achieving the target goal. Studies also had to examine financial incentives (e.g., cash, vouchers) for health-related behavior change, be published in peer-reviewed journals, and include a research design that allowed evaluation of the efficacy of the incentive intervention relative to another condition (e.g., non-contingent incentives, treatment as usual). Of the 39 reports that met inclusion criteria, 18 targeted substance use, 10 targeted medication adherence or home-based health monitoring, and 11 targeted diet, exercise, or weight loss. All 39 (100%) studies used technology to facilitate remote monitoring of the target behavior, and 26 (66.7%) studies also incorporated technology in the remote delivery of incentives. Statistically significant intervention effects were reported in 71% of studies reviewed. Overall, the results offer substantial support for the efficacy of remotely implemented incentive interventions for health-related behavior change, which have the potential to increase the cost-effectiveness and reach of this treatment approach.
The leading causes of morbidity and mortality in developed countries (e.g., cardiovascular disease, diabetes, hypertension) are increasingly attributable to behavior or lifestyle (e.g., Higgins, 2014 ). Consequently, new and more effective interventions to promote health-related behavior change are sorely needed. Behavioral economic interventions in which financial incentives (e.g., cash, vouchers exchangeable for goods or services) are provided contingent on objective evidence of behavior change (e.g., biochemically verified smoking abstinence) are gaining considerable attention in efforts to promote health-related behavior change (e.g., Higgins, Silverman, Sigmon, & Naito, 2012 ). These interventions leverage the same preference for short-term gains that often underpin substance abuse, unhealthy food choices, and other unhealthy behavior patterns by providing monetary rewards for shorter-term healthy choices ( Higgins et al., 2012 ; Lowenstein, Brennan, & Volpp, 2007 ). Such interventions are commonly referred to under the heading of Contingency Management (CM) in the substance abuse area (e.g., Higgins, Silverman, & Heil, 2008 ). These incentive-based interventions have been shown to be highly effective at producing healthy behavior change, including, for example, abstinence from substance abuse ( Dallery, Glenn, & Raiff, 2007 ; Higgins et al., 1994 ), increases in physical activity ( Kurti & Dallery, 2013 ; Pope & Harvey-Berino, 2013 ), greater medication adherence ( Rigsby et al., 2000 ; Sorensen et al., 2007 ) and improvements in attendance at therapy sessions ( Ledgerwood, Alessi, Hanson, Godley, & Petry, 2008 ).
Frequent and objective monitoring of attainment of behavior change goals and differential delivery of the incentives depending on goal attainment is a critically important element of an effective incentive program. When targeting changes in substance use, for example, drug metabolites or other byproducts of drug use are typically monitored in breath or body fluids and incentives provided when testing indicates no recent use. Such frequent monitoring can be burdensome on clinic staff and clients/patients alike if frequent clinic or home visits are involved. Moreover, those factors can also limit the geographical range over which clinical services can be delivered thereby denying treatment for individuals residing in remote or otherwise difficult to reach settings. An important strategy that is emerging to reduce or eliminate these burdens and barriers is the use of the Internet, Smartphones, or related technologies for remote monitoring of behavior change and delivery of incentives. These strategies are being used in incentive-based interventions for substance abuse (e.g., Alessi & Petry, 2013 ; Meredith, Grabinski, & Dallery, 2011 ), weight loss (e.g., Unick, Leahey, Kent, & Wing, 2015 ), as well as other health-related behavior problems. To our knowledge, there has not yet been a systematic review of this emerging literature, which is the purpose of the present report.
We focused on studies using technology to objectively monitor the target of the intervention or as a delivery system to provide reinforcement when the targeted goal is attained ( Dallery & Raiff, 2011 ). Another way that technology has been integrated into incentives interventions is to supplement the incentives with feedback to participants on progress or in combining the incentives intervention with another formal treatment (e.g., computer-based cognitive behavior therapy). Although some studies included in the present review used technology for these purposes in addition to monitoring behavior and/or delivering incentives, all included reports were required to use technology to accomplish one or both of the main elements of incentives interventions remotely and we focus specifically on how technology was used for these purposes. In addition to reviewing the efficacy of these interventions, we also characterized the particular health-related behaviors and populations targeted, reinforcement schedules employed, study research designs and sample sizes, and discernable trends in the literature over the years covered by the review.
Search Strategy
The review covered an approximately 11-year time period (April 2004–May 2015) that corresponds to the time-period covered in other reviews by our group on use of financial incentives for treating substance use disorders where we noted the growth in use of remotely-implemented CM interventions ( Davis et al., 2015 ; Higgins, Sigmon, & Heil, 2011 ). All studies identified for potential inclusion in this review were retrieved using a detailed search strategy. First, we used PubMed, the search engine of the U.S. National Library of Medicine, as well as PsycINFO, an electronic database offered through the American Psychological Association that focuses primarily on behavioral and social sciences. The search strategy was tailored appropriately for each database (see Appendices ), but in both cases involved searching for subject headings that matched the four concepts of (a) remote behavior monitoring technology (e.g., “accelerometers”, “Internet”, “email”, “Smartphone”, “remote monitoring application”); (b) contingent financial incentives (e.g., “financial incentives”, “contingency management”, “vouchers”, “deposit contract”, “gift cards”); (c) a disorder or condition related to the target behavior (e.g., “substance abuse”, “obesity”, “hypertension”); and (d) the target behavior of the treatment (e.g., “cigarette”, “tobacco use cessation”, “body weight”, “walking”, “antihypertensive medication”). The years searched included April 2004–May 2015.
Reports generated by the above search strategy were reviewed by at least two of the authors. For inclusion, reports had to meet the following criteria: (a) used technology to accomplish one or both of the main elements of incentives interventions (i.e., monitoring behavior, delivering incentives) remotely; (b) used financial incentives; (c) was published in a peer-reviewed journal; (d) included an experimental comparison condition; and (e) was an original, prospective experimental study that reported previously unpublished data. Retrospective data analyses and secondary analyses of previously published data were excluded. With respect to the requirement that the study include an experimental comparison condition, this condition could be a non-contingent incentives condition, a different treatment intervention (e.g., cognitive-behavioral therapy, treatment as usual), or varying intensities of the incentives intervention. For those studies that employed a within-subjects design, the comparison could be a no-intervention baseline phase that preceded and followed the intervention, or a multiple-baseline design wherein the timing of the incentives intervention was staggered in time across different targets or different participants. Because the incentives interventions we reviewed often included components (e.g., feedback about progress) whose independent influence on treatment efficacy has never been assessed, we did not require that studies have isolated the effects of incentives apart from those common elements for inclusion.
Second, we reviewed bibliographies of those articles that met the above criteria and were therefore included in the present review. Disagreements about whether a report met the above criteria were resolved through discussion until consensus was reached. Given heterogeneity across studies in the experimental designs used, participant populations targeted, and intervention durations and outcomes, a meta-analysis was not conducted. Instead, we characterized the literature in terms of efficacy, the health-related behaviors and populations targeted, purposes for using technology and specific technologies used, reinforcement schedules employed, as well as trends in the literature over the time period covered by this review. We also characterized the research designs and sample sizes used in included studies, the proportion of studies reporting significant treatment effects, and, if evaluated, whether treatment effects were maintained at a follow-up assessment occurring after incentives were discontinued.
All summaries below regarding the proportion of studies that produced a statistically significant treatment effect are limited to studies that used inferential statistics to detect differences between one or more treatment groups relative to a control group. Studies in which treatment effects were determined using other methods (e.g., visual analysis of the data) were excluded from these summaries as were studies in which two levels of the incentives intervention were compared to one another in the absence of a control condition. Maintenance of treatment effects refers to: (a) statistically significant differences favoring the incentives intervention over the comparison condition during treatment that remained significant at follow-up (between-subjects designs); (b) significant improvements in the target behavior produced by the intervention that remained significant at follow-up (i.e., pre-intervention versus post-intervention [within-subjects designs with a return to baseline]); and (c) significant improvements in the target behavior remained significant at follow-up, with no differences between the end of treatment versus follow-up (within-subjects multiple baseline designs).
The literature search identified 534 articles, from which 39 were selected for inclusion in the review. The main reasons for exclusion were: (a) incorporating technology in financial incentives interventions that were not delivered remotely (e.g., delivering computer-based therapy plus incentives in an outpatient research clinic; Budney et al., 2015 ; (b) using technology to monitor behavior remotely but not in the context of incentive-based interventions (e.g., Internet-based weight loss programs without financial incentives; e.g., Unick, Leahey, Kent, & Wing, 2015 ); or (c) delivering a remote incentives-based intervention without a comparison condition in between-subjects designs, or in the absence of a return to baseline or staggered introduction of the intervention in within-subjects designs [e.g., Leahey & Rosen, 2014 ]).
The target behavior was substance use in 18 of the 39 studies included (46.2%). Ten (25.6%) studies targeted medication adherence or adherence to home-based health monitoring (e.g., blood pressure, blood glucose), and 11 (28.2%) targeted diet, exercise, or weight loss. All 39 studies incorporated mobile technology into incentive-based health interventions to monitor the target behavior remotely. In addition, twenty-six of these studies (66.7%) also incorporated technology in the delivery of incentives. Of the 31 studies included in our calculation of the proportion of studies indicating treatment effects, 22 (71%) reported statistically significant treatment effects.
Substance Abuse
Study targets and populations.
A total of 18 studies focused on substance use ( Table 1 ), with 14 (77.8%) targeting cigarette smoking and four (22.2%) targeting alcohol use. The populations targeted by these interventions were primarily adult substance abusers. However, populations with co-morbid conditions or vulnerabilities were also targeted, including smokers with PTSD ( Hertzberg et al., 2013 ), pregnant smokers ( Harris & Reynolds, 2015 ; Ondersma et al., 2012 ), homeless Veterans who were smokers ( Carpenter et al., 2015 ), and rural smokers ( Stoops et al., 2009 ).
Interventions Targeting Substance Use Disorders
Note: Design refers to between-subjects (BS) or within-subjects (WS); n refers to sample size (across all groups); Schedule refers to the reinforcement schedule; Duration of Treatment refers to the number of weeks over which incentives contingent on the target behavior could be earned; Maximum earnings refers to the maximum amount that could be earned contingent on engaging in the target behavior (CNBD= maximum earnings could not be determined). Average or expected amounts are reported for studies using probabilistic schedules (e.g., prize-based); Use of technology refers to the purpose for which technology was used (“Other” may refer to additional components of the incentives intervention, or additional treatments in combined interventions); Statistically significant treatment effects and effects at follow-up= defined in all studies as differences in ≥ one primary outcome (p < .05) for comparisons between a control condition versus ≥ one incentives condition.
Technologies used in monitoring substance use
The most common method of remote monitoring involved wireless submission of data indicating participant’s substance use status. For example, in a majority of the cigarette smoking interventions, participants submitted videos of themselves taking a breath carbon monoxide (CO) test with results discernible via a web camera (e.g., Dallery & Glenn, 2005 ; Dallery, Meredith, and Glenn, 2008 ; Meredith et al., 2011 ; Stoops et al., 2009 ). These videos were submitted via email or uploaded to a secure website for later review by the researchers. Those studies targeting alcohol use monitored intake via the Secure Remote Alcohol Monitoring (SCRAM) bracelet, which detects metabolites of alcohol that are excreted through sweat (e.g., Barnett et al., 2011 ; Dougherty et al., 2014 , 2015 ). These data were collected continuously while participants wore the bracelet, and later downloaded and reviewed by the researchers at periodic in-person assessments.
Technologies used in delivering reinforcement
In 15 (83.3%) studies, technology was also used for remote delivery of financial incentives contingent on biochemically verified abstinence, and the means of doing so was similar across all 15 studies. More specifically, participants were provided with statements of their recent and/or accumulated earnings via either email (e.g., Dallery & Glenn, 2005 ), text message (e.g., Alessi & Petry, 2013 ), or by accessing a study website (e.g., Barnett et al., 2011 ; Dallery et al., 2007 , 2008 ; Dallery, Raiff, & Grabinski, 2013 ).
Reinforcement schedules
Consistent with in-person CM interventions, the technology-based substance use interventions in this review relied almost exclusively on differential reinforcement of other behavior (DRO) schedules to reinforce drug abstinence, in which the amount of reinforcement escalated contingent on biochemically confirmed, consecutive abstinent samples ( Higgins et al., 1991 ; Roll, Higgins, & Badger, 1996 ; Roll & Higgins, 2000 ). All of the studies that used escalating schedules also employed a reset contingency, where submitting a positive sample reset the participant’s voucher earnings to their starting value, however submitting a pre-determined number of negative samples following a slip restored participant’s voucher values to the magnitude they were at before the slip. A majority of the studies using escalating schedules with resets also provided a bonus after participants submitted a certain number of consecutive negative samples (e.g., a $5.00 bonus following every three consecutive negative samples; Dallery & Glenn, 2005 ). One exception to the above was Ondersma et al.’s (2012) study targeting pregnant cigarette smokers, in which “incentivization attempts” were self-initiated by participants on a maximum of five prenatal care visits, with a fixed $50.00 provided for abstinent samples. Another exception was Dougherty et al. (2014 ; 2015) where participants received fixed, non-escalating payments on a weekly basis contingent on abstinence from alcohol use.
The frequency of monitoring the target behavior ranged from a minimum of five times during the course of a 10-week intervention ( Ondersma et al., 2012 ), to continuous monitoring of alcohol use in real time using the SCRAM device (e.g., Barnett et al., 2011 ), with a majority of studies requiring participants to submit discrete samples at least twice daily (e.g., Dallery & Glenn, 2005 ; Dallery et al., 2008 ; Glenn & Dallery, 2007 [ Table 1 ]). In addition to frequent monitoring of the target behavior, most studies also minimized the delay between verification of participant’s goal attainment and reinforcement delivery. More specifically, participants in a majority of studies received feedback about their earnings directly after providing evidence of the target behavior, and were able to redeem their vouchers immediately upon learning of their recent earnings. Exceptions to this, however, included six studies in which the delay to receiving vouchers ranged from one week (e.g., Dougherty et al., 2014 ; Reynolds, Dallery, Shroff, Patak, & Leraas, 2008 ) up to the end of a four-week treatment ( Hertzberg et al., 2013 ; Carpenter et al., 2015 ).
Research designs and sample sizes
Five of the 18 (27.8%) reports targeting substance abuse employed between-subjects designs with random assignment to an intervention versus either a control or other experimental treatment condition, 10 reports used within-subjects designs, and the remaining three reports used mixed designs in which incentives were evaluated in a within subjects design but another aspect of the treatment differed between groups (e.g., access to a social forum, Dallery et al., 2015 , Meredith & Dallery, 2013 ; sequence in which study conditions were experienced, Dougherty et al., 2014 ).
Sample sizes varied considerably across reports, from four smokers in Dallery and Glenn’s (2005) initial assessment of the feasibility of delivering an Internet-based financial incentives intervention to reduce cigarette smoking, to 110 pregnant cigarette smokers in Ondersma et al. (2012) .
During- and post-treatment efficacy
Of the 13 studies that used inferential statistics to evaluate intervention effects, 10 of 13 (76.9%) reported significant effects of the intervention on substance use outcomes (e.g., proportion of drug-negative biological specimens, longest duration of abstinence). In the three with negative results, incentives did not produce greater abstinence than a control treatment ( Ondersma et al., 2012 ) or a baseline condition in a within-subjects design ( Carpenter et al., 2015 ; Hertzberg et al., 2013 ). These studies were smoking-cessation interventions conducted among smokers with co-morbid conditions (i.e., pregnant women, homeless Veterans, and individuals with PTSD, respectively), which may suggest that more tailored or intensive treatments are needed to promote abstinence among these relatively difficult-to-treat populations. For example, there is ample evidence supporting the efficacy of incentives for promoting smoking cessation among pregnant women in trials involving more intensive and larger magnitude incentives than were used in the Ondersma et al. report (see Higgins et al., 2012a ).
Seven of the 13 (53.8%) studies above conducted follow-up assessments. Treatment effects remained significant at follow-up in only two (28.6%) reports ( Dougherty et al., 2014 , 2015 ), both of which targeted alcohol intake among heavy drinkers with no other comorbidities present. In the initial study, follow up drinking status was objectively verified while in the latter study it was based on participant self-report. In the other six studies, statistically significant treatment effects were not observed at follow-up assessments. The lack of support for the maintenance of treatment gains in these studies is presumably due to the fact that, across all studies, no components of the treatment were available and no alternative treatments were provided during the period of time between the termination of the intervention and the follow-up assessment.
Trends in research targeting substance use
One trend observed across the 18 reports targeting substance use was an increase in the number of interventions targeting hard to reach or difficult to treat populations in the later years encompassed by the review (e.g., rural populations, pregnant women). All of the reports targeting vulnerable populations were published between 2008–2015. As availability of Internet and particularly Smartphone services increases ( Smith, 2013 ), technology-based incentives interventions may become an increasingly feasible and efficacious method for surmounting barriers to reducing substance use in these sometimes hard-to-reach vulnerable populations.
A second trend observed in this literature was the emergence of interventions targeting alcohol. No studies published between 2004–2009 targeted drinking, primarily because of two deficiencies in technology: (a) a reliable method for detecting intermittent alcohol use other than breath alcohol sensors, and (b) a device that would allow such monitoring to be done remotely. The emergence of the SCRAM device surmounted both obstacles.
A third trend observed across the present reports involved capitalizing on newer technologies (e.g., study websites, systems for transmitting data) to support incentives interventions in ways that permitted researchers to improve upon some of the important determinants of intervention effectiveness. For example, in Dallery and Glenn’s (2005) initial Internet-based incentives study, participants submitted videos of themselves blowing into a breath CO monitor by email, which had to be reviewed by the researchers before participants received feedback about their progress and study earnings. By contrast, participants in later studies by this same group submitted breath CO samples over a website (Motiv8) which provided immediate, automatic feedback about their progress in the form of a cumulative progress graph and an account activity box showing their recent and overall earnings ( Dallery et al., 2008 ). This development decreased the delay between monitoring of the target behavior and the delivery of financial incentives, which has been shown to be a significant moderator of treatment effect size ( Lussier et al., 2006 ). Further developments of this website included the addition of a communication forum in which participants in group-based incentives interventions could write messages to one another and view each other’s cumulative progress graphs ( Dallery et al., 2015 ). Future studies conducted by this research group may incorporate a Smartphone-enabled CO monitor, which demonstrated good reliability and validity in pilot tests ( Meredith et al., 2014 ) and may reduce the response effort among participants in incentives interventions by permitting them to submit evidence of behavior change using a device that is becoming ubiquitous and is carried by many individuals during all waking hours.
Medication Adherence and Home-Based Health Monitoring
Of the 10 studies included in this topic area ( Table 2 ) four targeted adherence to antiretroviral medications among HIV-positive individuals ( Barnett, Sorensen, Wong, Haug, & Wong, 2009 ; Moore et al., 2015 ; Rosen et al., 2007 ; Sorensen et al., 2007 ), two targeted blood glucose monitoring among adolescents with Type I diabetes ( Raiff & Dallery, 2010 ; Stanger et al., 2013 ), two targeted Warfarin adherence among individuals with poor anticoagulation control ( Kimmel et al., 2012 ; Volpp et al., 2008 ), one targeted adherence to antihypertensive medications ( Petry, Alessi, Byrne, & White, 2015 ), and one targeted self-monitoring of blood glucose, blood pressure, and weight ( Sen et al., 2014 ). Other than the two studies targeting adolescents with diabetes, all eight of the other studies targeted adults, with four of eight (50%) targeting especially vulnerable populations. More specifically, two of the four samples of HIV-positive individuals were also receiving methadone maintenance therapy, and the other two samples of HIV-positive individuals were current illicit drug users.
Interventions Targeting Medication Adherence or Home-Based Health Monitoring
Technologies used in monitoring medication adherence or health monitoring
All 10 studies (100%) used technology to monitor the target behavior remotely. Among the medication adherence reports, four of seven studies monitored pill taking by measuring openings of electronic or medication event monitoring system (MEMS) caps ( Barnett et al., 2009 ; Moore et al., 2015 ; Rosen et al., 2007 ; Sorensen et al., 2007 ). MEMS are pill bottles or containers fitted with microcircuitry that provide time stamps when the container is opened or closed. In these four studies, this information was stored on the device for later review by the researchers, who tracked and delivered incentives for medication adherence. Similar technology was also employed in the Stanger et al. (2013) study with diabetic adolescents, in which a blood glucose monitor detected and stored data about glucose monitoring events, which were reviewed at periodic assessments at which teens received incentives contingent on that stored information.
Technology like MEMS but slightly more advanced was employed in the Volpp et al. (2008) and Kimmel et al. (2012) studies (i.e., the Informedix Med-eMonitor System ™ ) on Warfarin adherence. Rather than storing the data on the device until it could be reviewed in person by the researcher and participant, the Med-eMonitor ™ in these studies communicated with a central database to denote each instance of pillbox opening, and participants received an automatically generated message requesting that they confirm whether they had ingested their medication. Similarly, the three wireless biometric devices with which participants in Sen et al. (2014) monitored glucose, blood pressure, and weight automatically transferred data to a website that was accessible by both participants and researchers.
A different approach than the above was employed in the last two reports, in which researchers remotely observed participants while they engaged in the target behavior. In Raiff and Dallery (2010) , diabetic adolescents submitted videos of themselves monitoring their blood glucose via a web camera four times per day. Similarly, Petry et al. (2015) measured medication adherence by requiring participants to submit videos of themselves ingesting the antihypertensive medications using the video capabilities of a cell phone.
In addition to using technology to monitor the target behavior, five of the 10 (50%) studies in this area also incorporated technology in the remote delivery of reinforcement ( Kimmel et al., 2012 ; Moore et al., 2015 ; Raiff & Dallery, 2010 ; Sen et al., 2014 ; Volpp et al., 2008 ). Participants in all five studies received messages about their earnings, which were automatically generated shortly after participants engaged in the target behavior in all but one case ( Sen et al., 2014 ). Worth noting is that participants in the Sen et al. (2014) study received personalized texts or emails from the researchers about their earnings. In addition to receiving statements about their earnings, participants in Moore et al. (2015) also received electronically loaded increments on debit cards contingent on antiretroviral adherence.
Six of 10 (60%) studies used an escalating schedule of reinforcement contingent on pillbox opening or health monitoring. Of the four studies that used other schedules, three of the four studies ( Kimmel et al., 2012 ; Sen et al., 2014 ; Volpp et al., 2008 ) employed lottery systems in which participants had a larger probability of winning a small sum of money for engaging in the target behavior, or a smaller probability of winning a large sum of money (e.g., a one in five chance of winning $10.00 and a one in 100 chance of winning $100.00 for taking Warfarin, Volpp et al., 2008 ). In the fourth study that used a non-escalating schedule ( Raiff & Dallery, 2010 ), participants received incentives worth a fixed amount (i.e., $1.00) for every glucose testing video submitted, along with a $3.00 bonus upon submitting their fourth video.
In all but two of the medication adherence and home-based health monitoring studies, engagement in the target behavior was monitored continuously and detected passively. In the two studies that required active input by the user, one required glucose testing videos to be submitted four times daily ( Raiff & Dallery, 2010 ), and the other required participants to submit videos of themselves taking antihypertensive medications once per day ( Petry et al., 2015 ). In contrast to the incentives interventions targeting substance use disorders, there was greater variability across studies in this topic area in the frequency with which feedback about participant’s earnings was provided, and in the delays to receiving incentives. In six studies, for example, participants received feedback about their earnings either immediately ( Kimmel et al., 2012 ; Raiff & Dallery, 2010 ; Moore et al., 2015 ; Sen et al., 2014 ; Volpp et al., 2008 ) or within the same day ( Petry et al., 2015 ), but the delay before participants could redeem their vouchers ranged from the next day ( Sen et al., 2014 ) to the end of the study ( Raiff & Dallery, 2010 ). In the other four studies, participants met with the researchers periodically throughout the treatment to transfer the data stored on their devices onto a computer where the researcher could review them. Vouchers in these studies were typically delivered at these meetings, and thus were redeemable at delays that varied from twice weekly ( Moore et al., 2015 ) to every four weeks ( Rosen et al., 2007 ).
Six reports (60%) randomized participants to a financial incentives versus either a control or other treatment comparison condition (e.g., supportive counseling) using between-subjects designs ( Barnett et al., 2009 ; Kimmel et al., 2012 ; Petry et al., 2015 ; Rosen et al., 2007 ; Sen et al., 2014 ; Sorensen et al., 2007 ). The other four studies employed within-subjects designs. Sample sizes ranged from four teens diagnosed with Type I diabetes in Raiff and Dallery (2010) to 101 adults with poor anticoagulation control in Kimmel et al. (2012) .
Eight of 10 (80%) studies evaluated the effectiveness of the intervention via statistical analyses of appropriate comparison conditions, with six of eight (75%) reporting significant increases in adherence or self-monitoring. Those studies that reported null effects included Moore et al.’s (2015) intervention to promote antiretroviral adherence among HIV-positive, illicit drug abusers, and Kimmel et al.’s (2012) intervention to increase warfarin adherence. The former attributed the lack of effect to low power given a small sample size ( N = 10) while the latter attributed it to a ceiling effect (i.e., higher than anticipated pre-intervention adherence levels reduced the opportunity to observe significant increases in adherence).
Six of the eight studies above included follow-up assessments, of which three (50%) supported the maintenance of treatment gains ( Petry et al., 2015 ; Sen et al. 2014 ; Stanger et al., 2013 ). Significant increases in glucose monitoring, along with associated decreases in blood glucose levels, were maintained three months after the incentives intervention was discontinued in the Stanger et al. study. Similarly, adherence to antihypertensive medications in the Petry et al. study differed significantly between the end of treatment versus at the three and six month follow-ups. In Sen et al., which included both low and high-magnitude incentive conditions, participants in both incentives conditions maintained treatment-produced increases in glucose-, blood pressure- and weight-monitoring at an assessment conducted three months following termination of incentives. Interestingly, treatment-produced increases in self-monitoring at the four and five-month follow-ups persisted in the low incentives condition, but not among participants in the high incentives condition where they decreased to control levels at the later follow-ups.
Treatment effects were not maintained at follow-up in the remaining three studies ( Barnett et al., 2009 ; Rosen et al., 2007 ; Sorensen et al., 2007 ). Although voucher or cash incentives were no longer provided for medication adherence during the time between treatment completion and follow-up in any of these studies, participants in Sorensen et al. (2007) received lottery-based incentives for completing medication coaching sessions during the month leading up to the follow-up assessment.
Trends in research targeting medication adherence and home-based health monitoring
In contrast to the literature on technology-based incentives interventions targeting substance use, which grew substantially between the earlier and later half of the period surveyed, the number of medication adherence or home-based health monitoring studies grew minimally between 2004–2009 (four studies) and 2010–2015 (six studies). While this literature did not change substantially in quantity, several notable changes in qualitative aspects of these studies were discernible and are outlined below.
One trend in the present literature involved capitalizing on newer technologies to improve upon some of the limitations in earlier incentive-based interventions targeting medication adherence or other health monitoring behavior. Three of four studies published between 2004–2009 used the MEMS system to monitor medication adherence, and the Med-eMonitor ™ system used in the fourth study differed only slightly from the MEMS system. Because MEMS only detects pillbox opening, one cannot ascertain whether the medication was actually taken, or whether the intended individual opened the container. Given the emphasis on objectively verifying the target behavior in incentives interventions, methods of directly observing participants take their medications would circumvent these limitations of MEMS. Studies that used technology to facilitate direct observation of the target behavior in medication adherence or health monitoring studies (e.g., video-based methods) emerged in the later half of the period covered by this review (2010–2015) ( Raiff & Dallery, 2010 ; Petry et al., 2015 ). As Smartphones grow increasingly commonplace, monitoring medication taking via cell phone, as was done in Petry et al., may become the predominant method for verifying this study target in future research.
In addition to capitalizing on newly available technologies to circumvent limitations in monitoring medication adherence remotely, Moore et al.’s (2015) method of remotely loading participant debit cards represents an especially effective method for minimizing the delay between engaging in the target behavior and earning reinforcement.
Diet, Exercise, and Weight Loss
The specific behaviors targeted across the 11 diet, exercise, and weight loss interventions (see Table 3 ) differed substantially, with one study targeting both diet and exercise ( Spring et al., 2012 ), three targeting the duration of engagement in physical activity ( Finkelstein, Brown, Brown, & Buchner, 2008 ; Hunter, Tully, Davis, Stevenson, & Kee, 2013 ; Weinstock, Capizzi, Weber, Pescatello, & Petry, 2014 ), four (36%) targeting steps per day ( Donlin-Washington et al., 2014 ; Kullgren et al., 2014 ; Kurti & Dallery, 2013 ; Petry, Andrade, Barry, & Byrne, 2013 ), and three (27%) targeting weight loss ( Almeida et al., 2015 ; Kullgren et al., 2013 ; Leahey et al., 2015 ). Seven (64%) studies targeted sedentary adults with no additional vulnerabilities or medical conditions, three (27%) studies specifically targeted overweight and obese adults ( Almeida et al., 2015 ; Kullgren et al., 2013 ; Leahey et al., 2015 ), and one (9%) study targeted sedentary, hazardous drinking college students ( Weinstock et al., 2014 ).
Interventions Targeting Diet, Exercise or Weight Loss
Technologies used in monitoring the target behavior
All eleven (100%) studies used technology to monitor the target behavior remotely. Coinciding with the diversity in target behaviors was a diverse array of technologies used to monitor these targets. One (9%) study required participants to self-report their daily diet and exercise practices on a website ( Spring et al., 2012 ), four (36%) used accelerometers or other wireless sensor technology to capture bodily movements which were converted using algorithms into minutes of physical activity ( Finkelstein et al., 2008 ; Hunter et al., 2013 ; Petry et al., 2013 ; Weinstock et al., 2014 ), three (27%) used the Fitbit® advanced pedometer to measure participant’s steps per day ( Kullgren et al., 2014 ; Kurti & Dallery, 2013 ; Donlin-Washington et al., 2014 ), and three (27%) used wireless scales to measure participant’s weight ( Almeida et al., 2015 ; Kullgren et al., 2013 ; Leahey et al., 2015 ).
Four (36%) of the 11 studies incorporated technology in the remote delivery of reinforcement ( Kullgren et al., 2013 ; 2014 ; Kurti & Dallery, 2013 ; Leahey et al., 2015 ). In all four cases, participants received statements about their earnings either by email contingent on engaging in the target behavior (e.g., meeting step goals on the requisite five of seven days per week [ Kullgren et al. 2014 ; Kurti & Dallery, 2013 ]), or via a website that generated the messages automatically contingent on meeting a monthly weight-loss goal ( Kullgren et al., 2013 ; Leahey et al., 2015 ).
There was substantial variability in the reinforcement schedules used in the reports on diet, exercise, or weight loss. Two (19%) studies provided fixed, non-escalating payments for meeting diet, exercise, or weight loss goals ( Kullgren et al., 2013 ; Spring et al., 2012 ); three (27%) used escalating schedules with reset contingencies for consecutive time periods over which exercise goals were met ( Kurti & Dallery, 2013 ; Petry et al., 2013 ; Weinstock et al., 2014 ); one (9%) used percentile schedules to gradually increase steps per day ( Donlin-Washington et al., 2014 ); two (18%) involved variable payments depending on participant’s average number of aerobic minutes per week in one case ( Finkelstein et al., 2008 ) and percent weight loss in the other ( Almeida et al., 2015 ); one (9%) equated minutes of physical activity with points which could be redeemed at a later date ( Hunter et al., 2013 ); and the final two (18%) studies employed lottery systems in which participants could earn different amounts of money contingent on meeting exercise or weight loss goals ( Kullgren et al., 2014 , Leahey et al., 2015 , respectively).
Consistent with the variability in reinforcement schedules employed across the 11 diet, exercise, and weight loss studies, these studies also varied in terms of the frequency of behavior monitoring and delays to reinforcement. For example, behavior was monitored continuously in some of the studies targeting duration of engagement in physical activity (e.g., Finkelstein et al., 2008 ) and steps per day (e.g., Kurti & Dallery, 2013 ), but monthly or quarterly in the studies targeting weight loss ( Kullgren et al., 2013 , Almeida et al., 2015 , respectively). Of course, it should be noted that this variability may be related to differences in the nature of these two study targets, where weight would be expected to change more gradually over time relative to the other targets. With respect to the delay between engaging in the target and receiving reinforcement, participants in four (37%) studies could not redeem their incentives until the end of treatment ( Finkelstein et al., 2008 ; Kurti & Dallery, 2013 ; Leahey et al., 2015 ; Spring et al., 2012 ). Similarly, participants in Hunter et al. (2013) had only two opportunities during the 12-week intervention to exchange the points that they accumulated for engaging in physical activity for rewards. Those studies that involved relatively short delays between researcher verification of participant’s engagement in the target and delivery of incentives included studies where participants earned incentives at delays ranging from once weekly (e.g., Petry et al., 2013 ; Weinstock et al., 2014 ) up to three times per week ( Donlin-Washington et al., 2014 ).
All reports used between-subjects designs with the exceptions of Kurti and Dallery (2013) and Donlin-Washington et al. (2014) . Of the nine studies that used between-subjects designs, five randomized participants to an incentives condition versus either a control condition ( Finkelstein et al., 2008 ; Hunter et al., 2013 ; Leahey et al., 2015 ; Petry et al., 2013 ; Spring et al., 2012 ) or another treatment comparison condition ( Almeida et al., 2015 ; Weinstock et al., 2014 ). Participants in the last two between-subjects studies ( Kullgren et al., 2013 ; 2014 ) were assigned to either a non-contingent incentives condition versus one of two levels of the incentives condition. In both studies, the incentives arm included an individual- and a group-based condition, where the group condition involved access an online forum in one study ( Kullgren et al., 2013 ), and exposure to a contingency in which a $500.00 incentive was split among members who achieved their weight loss goals in the other study ( Kullgren et al., 2014 ). The sample sizes in these studies ranged from six sedentary older adults in Kurti and Dallery (2013) to 1,790 overweight/obese adults across 28 worksites in Almeida et al. (2015) .
Ten of 11 studies (90.9%) conducted statistical analyses to evaluate treatment effects, of which six (60%) reported significant effects ( Finkelstein et al., 2008 ; Hunter et al., 2013 ; Donlin-Washington et al., 2014 ; Leahey et al, 2015 ; Petry et al., 2013 ; Weinstock et al., 2014 ). In two instances these differences were based on self-reported attainment of the target behavior while the four others were based on objective measures ( Hunter et al., 2013 ; Weinstock et al. 2014 ). The remaining four studies reported that incentives had no influence on participant’s percent weight loss ( Almeida et al., 2015 ; Kullgren et al., 2013 ), step counts ( Kullgren et al., 2014 ), or diet and exercise behaviors ( Spring et al., 2012 ).
Seven of the 10 studies above (70%) also conducted follow-up assessments, of which three (42.9%) reported that statistically significant treatment effects were present at follow-up ( Hunter et al., 2013 ; Leahey et al., 2015 ; Petry et al., 2013 ). In contrast, intervention-produced increases in activity were not maintained in Finkelstein et al. (2008) or Weinstock et al. (2014) , and the non-significant effect of treatment in the two Kullgren et al. (2013 , 2014) studies remained non-significant at follow-up. In all of these studies, no treatment components remained in place following discontinuation of incentives, although participants in Kullgren et al. (2014) were permitted to keep their pedometers for the two-month period between treatment completion and follow-up.
Trends in research targeting diet, exercise, and weight loss
With the exception of Finkelstein et al. (2008) , the other ten studies in this section were published in a relatively short three-year span between 2012–2014. Therefore, the development of technology-based financial incentives interventions targeting these behaviors appears to be a very recent trend in the literature.
Related to the recent emergence of these reports, a second trend observed was variability in the definitions of exercise used in these studies, and consequently, differences in the technologies used to monitor exercise remotely. Achieving greater consistency with respect to how exercise is operationally defined is necessary for comparing interventions targeting this behavior. Such consistency might be achieved by taking into account the current national guidelines that specify the level of physical activity required to attain health benefits (e.g., decreasing risk for cardiovascular disease). For example, the Centers for Disease Control ( CDC, 2008 ) recommends that adults achieve at least 150 minutes of moderate intensity activity per week (i.e., 30 minutes per day on at least five days), which has been shown to be approximately equal to walking 10,000 steps per day ( Le-Masurier, Sidman, & Corbin, 2003 ). However, only three studies delivered incentives for targets that were broadly consistent with this goal ( Finkelstein et al., 2008 ; Kullgren et al., 2014 ; Kurti & Dallery, 2013 ), and all three of those studies were primarily designed to promote the acquisition of increased activity levels as opposed to maintaining them.
Given the shorter period over which the interventions in this topic area have evolved relative to those targeting substance use or medication adherence/home-based health monitoring, there has been little opportunity to observe whether technologies used in monitoring the target behavior have undergone substantial change over time. However, the fact that one of the first two studies targeting diet and exercise ( Spring et al., 2012 ) relied on self-reported behavior whereas later studies capitalized on technological advancements such as Fitbits ™ ( Donlin-Washington et al., 2014 ; Kullgren et al., 2014 ; Kurti & Dallery, 2013 ) may suggest that exercise-based incentives interventions that use newly developing and conveniently available technology are on the horizon. In addition, two of the seven reports targeting exercise or weight loss involved a group-based intervention arm ( Kullgren et al., 2013 , 2014 ). Perhaps such group approaches will comprise a reasonable portion of interventions targeting diet, exercise, and weight loss in future research. Doing so may appeal to a preference reported in the literature for home-based exercise interventions that incorporate social components ( Brawley, Rejeski, & King, 2003 ; Wilcox, Castro, King, Housemann, & Brownson, 2000 ).
The use of technology to facilitate the remote implementation of incentive-based interventions to improve health is a burgeoning area of research, especially interventions targeting substance use, home-based health monitoring, and diet, exercise, and weight loss. Considered collectively, the number of technology-based financial incentives interventions grew from 11 studies published between 2004–2009, to 28 studies published between 2010–2015. Of the 31 reports statistically assessing treatment effects, 22 (71%) reported significant changes in the target behavior, providing strong support for the promise of remotely implemented financial incentives interventions.
A majority of the reports included in the present review targeted substance abuse, of which 83.3% produced statistically significant increases in abstinence rates. The three studies that reported no difference between an incentives condition versus either a control or other treatment comparison condition targeted special populations ( Carpenter et al., 2015 ; Hertzberg et al., 2013 ; Ondersma et al., 2012 ). Whether these interventions failed to reduce substance use because of the methodology employed, small sample sizes, or because the populations targeted were particularly difficult to treat, was unclear. Nonetheless, the growth over time in technology-based incentives studies targeting vulnerable populations suggests that using technology to surmount geographical and socioeconomic barriers to treatment delivery may be a growing trend in the literature. This approach may be particularly promising among vulnerable populations in which the prevalence of substance use is disproportionately high (e.g., cigarette smoking among disadvantaged individuals), but who nonetheless have increasing access to technology (e.g., Internet, Smartphones [ Smith, 2013 ]).
Of all study targets examined in this review, technology-based incentives studies targeting medication adherence have arguably demonstrated the least change over time in terms of the diversity of technologies used to facilitate remote monitoring of the target behavior. Specifically, the predominant method of monitoring medication adherence across studies was the MEMS system, which indicates whether a pill container was opened but not whether the medication was actually taken. Studies such as Petry et al. (2015) , in which participants submitted videos of themselves ingesting their medications, help circumvent the limitations of MEMS but place a greater burden on the participant, as the target behavior is not verified automatically using this method. Interestingly, these were the only two methods of remote behavior monitoring represented across the studies targeting medication adherence. Developments in technology to detect medication taking passively, such as digital pills which produce a voltage during digestion and communicate this information to external sensors (e.g., Bosworth, 2012 ), should permit more accurate, remote monitoring in future incentive-based interventions targeting medication adherence.
Those reports targeting exercise demonstrated the greatest variability in how the target behavior was defined, and in the technologies used to monitor the target remotely. These differences may be attributable to the fact that all but one of the interventions targeting this class of behavior were published between 2012–2014, thereby reducing the opportunity to observe changes over time. As mentioned previously, future incentive-based interventions targeting exercise could achieve greater uniformity in how exercise is operationalized by referring to current exercise guidelines (e.g., CDC, 2008 ). Given the growing obesity crisis in the United States, it is critical to develop interventions that produce clinically significant improvements in this well-established predictor of negative health outcomes.
With respect to technology-based incentives interventions targeting substance use, the relatively poor maintenance of treatment effects at follow-up underscores the need for greater research attention to this topic. In particular, it will be important for future research to develop methods of programming for the maintenance of treatment gains. Examples of how this might be accomplished are evident in studies that integrated the community reinforcement approach (CRA) with contingent incentives for cocaine abstinence (e.g., Higgins, Wong, Badger, Odgen, & Dantona, 2000 ; Higgins et al., 2007 ; Garcia-Fernandez et al., 2011 ; Secades-Villa et al., 2011 ). In these studies, participants received in-person counseling that focused on developing naturalistic sources of reinforcement that would sustain behavior change once the contrived financial incentives were withdrawn, with the results indicating improvements is both sustained abstinence and psychological functioning.
Another model to sustain treatment effects among those with substance use disorders is one wherein incentives remain in place longer-term as developed by Silverman and colleagues ( Silverman et al., 2005 ). Serious consideration should be given to the possibility that keeping incentive-based interventions in place for extended durations may be cost-effective. Just as chronic medication regimens for conditions such as diabetes and hypertension cost substantially less than the medical crises that may result from discontinuing these therapies, it is certainly conceivable that chronic incentives interventions to sustain drug abstinence, medication adherence, or other health-related behavior change in high-risk populations may be a cost-effective but to date unexplored treatment option. Importantly, careful consideration must be given in designing programs that are intended to remain in place long term. For example, incentive-based wellness programs offered by employers (e.g., programs that provide rewards for using the gym) are not cost-effective when employee engagement rates are low, as a majority of the money spent on the program ends up being earned by people who would have engaged in the rewarded behavior even without the incentive, rather than by those who are non-adherent or not engaged (Loewenstein, Asch, & Volpp, 2013). However, such programs can be made more cost-effective by modifying particular design features of the intervention (e.g., separating incentive payments from paychecks to make the incentives more salient to participating employees).
Given the growing contribution of modifiable lifestyle factors to risk for chronic disease and premature death, the need for improved health-related behavior change interventions is clear. Remotely managed financial incentive programs hold substantial promise for reducing practical barriers and increasing the reach of efficacious health-promoting treatments. Of course, additional research will be needed to refine how we can better capitalize on technological advancements to accurately monitor and reinforce targeted changes in health behaviors. Moreover, cost-effectiveness studies will be critically important in evaluating the general strategy of using financial incentives to promote health-related behavior change as well as the relative merits of remote versus in-person incentives interventions. Accomplishing these research tasks has the potential to contribute substantially to reducing the advent impact of unhealthy lifestyles on individual and population health.
Acknowledgments
This project was supported in part by Research Grants R01HD075669 and R01HD078332 from the National Institute of Child Health and Human Development, Center of Biomedical Research Excellence award P20GM103644 from the National Institute of General Medical Sciences, and Institutional Training Award T32DA07242 from the National Institute on Drug Abuse. The funding sources had no other role in this project other than financial support.
We would like to acknowledge Alyssa Noble for her review of an earlier draft of this manuscript and her assistance ensuring that we adhered to APA style guidelines.
Appendix 1. PubMed Search Strategy
Appendix 2. psycinfo search strategy.
Academia.edu no longer supports Internet Explorer.
To browse Academia.edu and the wider internet faster and more securely, please take a few seconds to upgrade your browser .
Enter the email address you signed up with and we'll email you a reset link.
- We're Hiring!
- Help Center
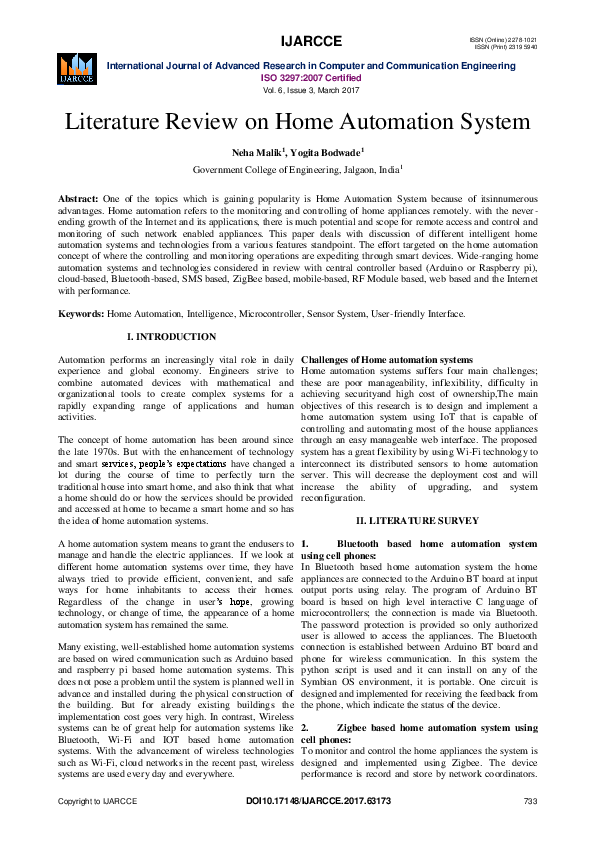
Literature Review on Home Automation System

One of the topics which is gaining popularity is Home Automation System because of itsinnumerous advantages. Home automation refers to the monitoring and controlling of home appliances remotely. with the never-ending growth of the Internet and its applications, there is much potential and scope for remote access and control and monitoring of such network enabled appliances. This paper deals with discussion of different intelligent home automation systems and technologies from a various features standpoint. The effort targeted on the home automation concept of where the controlling and monitoring operations are expediting through smart devices. Wide-ranging home automation systems and technologies considered in review with central controller based (Arduino or Raspberry pi), cloud-based, Bluetooth-based, SMS based, ZigBee based, mobile-based, RF Module based, web based and the Internet with performance.
Related Papers
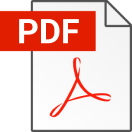
IRJET Journal
International Journal of Advance Research and Innovative Ideas in Education
Due to the proliferation of modern technology, these days, the world is increasingly experienced the use of wireless devices. The devices such as remote control and GSM phone could provide means for monitoring and controlling home appliances in a more convenient way. This project has explored the concept of home automation and ZigBee technology. A home automated system based on Arduino and ZigBee are developed which is tried to be integrated with Android application through Home Gateway for network interoperability. To show the effectiveness and feasibility of this proposed system, a remote control, indoor control and outdoor control systems have been developed and evaluated. However, integrating the system with android application remains unsuccessful
Home Automation using GSM and Arduino
Home appliances can be remotely be controlled using home automation system and makes it easily to accessed and effectively controlled. With the use of telecommunication device like mobile phone, computer and electronic technology electrical appliance can be controlled from any part of the world. The main reasons for home automation system are to make peoples’ lives easy, greater amount of energy will be saved and driven more by technology. Among others, home automation systems available are either based on Bluetooth technology, ZigBee technology, Infrared Remote (IR) controller, Radio Frequency (RF) technology. Though these systems are good, unfortunately, they can work effectively from a distance of (100 meters maximum) automation within a particular locality. This pose a big challenge/risk of electrical disaster to people outside their vicinities that mistakenly left their appliances ON/OFF, as the case may be, and could also lead to energy to wastage for appliances left connected to the mains for a period longer than required. Hence the need for effective means of remotely controlling home appliances beyond one's vicinity. This brought about the prose project for home automation using Arduino and GSM module (SIM900). The system uses SMS to send commands in order to remotely turn ON and OFF electrical devices. The system development involves the implementation of different hardware modules such as power supply, GSM, microcontroller, relays, and loads which make up the system as well as programming the system controller.
Revathi nunna95
With the increase in consumption of energy and population, there is a grave need to conserve energy in every way possible. The inability to access and control the appliances from remote locations is one of the major reasons for energy loss. A web or an android application is used by the users to give instructions to these systems. This system can make use of a host of communication methods such as Wi-Fi, GSM, Bluetooth, ZigBee. Different controlling devices and configurations can be found in existing systems. Such systems have been found already in many places for a wide variety of applications. This paper presents a survey of all such systems.
Prashant Chaudhary
Home automation trade has drawn goodish attention of researchers for quite a decade. The main attraction of any automated system is reducing human labor, effort, time and errors due to human negligence. With the development of modern technology, smart phones have become a necessity for every person on this planet. With the increase in consumption of energy and population, there is a grave need to conserve energy in every way possible. The inability to access and control the appliances from remote locations is one of the major reasons for energy loss. This paper presents a survey of all such systems.
Shanjedul Hassan
The home automation is one of the most emerging trends in modernization of home appliance control. Presently, conventional wall switches are located in different parts of the house and one has to physically go near them and press them to turn the loads on/off. It becomes very difficult for the elderly or physically handicapped people to do so. This system is designed to provide control of home appliances remote and via Bluetooth Android based Smart phone app. The proposed system does not require a dedicated server PC with respect to similar systems and offers a novel communication protocol to monitor and control the home environment with more than just the these devices can be controlled through a web application or via Bluetooth Android based Smart phone app. The proposed system does not require a dedicated server PC with respect to similar systems and offers a novel communication protocol to monitor and control the home environment with more than just the switching functionality. To demonstrate the feasibility and effectiveness of this system, devices such as light switches, temperature sensor, gas sensor and motion sensors have been integrated with the proposed home control system. This paper presents a low cost and flexible home control and environmental monitoring system.
Jatin Bajaj
The development of smart home automation system has great potential in today’s age of technology. According to the Smart Home Energy, a smart home, or smart house, is a home that incorporates advanced automation systems to provide the inhabitants with sophisticated monitoring and control over the building's functions. Such systems make use of different types of sensors to examine the environment and maintain a control over home appliances using latest communication and networking methods. The purpose of this paper is to provide information about the implementation and design of existing smart home technologies. This paper also discusses about our wireless, voice control Smart Home System that allow people control their home devices by voice command at home.
abhishek kumar
— Home automation which is playing a very vital role in modern times due to its flexibility, reliable in using at different places with high precision and in which will save money and thus time by decreasing human hardwork. General idea in automation of home shows the various quality of human being at the house. Main focus of technology is in controlling the household equipment " s like light, TV, fan, door, AC etc. automatically. In case of hazardous condition, it is useful for many old aged and handicapped persons. In Home Automation, remote, wireless controlled switches, PC, Laptop or smartphone are used for operating the appliance. In this paper we detailed a survey on home control automation using GSM and Bluetooth by considering the parameters like efficiency of working, controllers used, type of communication, the apps developed etc. A comparative analysis of home automation system is done at last.
RELATED PAPERS
Pathompong Pangamol
Corporate Ownership and Control
Muawya Hussein
Astronomy & Astrophysics - ASTRON ASTROPHYS
P. Andreani
enwa thomas
Revista Española de Educación Comparada
cecilia bocchio
World and Word
Andrzej Franaszek
International Organization of Citrus Virologists Conference Proceedings (1957-2010)
MOHAMAD NOR HAFIZZI BIN MOHD ROSLI Moe
Physical Review E
Antonio Palacios
Ted Kochanski
Global Journal of Public Health Medicine
Aisyah Abdul Jabar
Journal of Blood & Lymph
Rabindra Nath Das
Nugroho Utomo
Francesca Cavalieri
Journal of Experimental Biology
Klaus Beyenbach
Arquivos brasileiros de cardiologia
Felipe Ferreira
Siberian Scientific Medical Journal
Sergei Jargin
Boletin Cultural Y Bibliografico
Juan Manuel Palomino Borda
Cancer Epidemiology, Biomarkers & Prevention
Louise Izatt
Monica Gribben
Aji Saputra
JNPM (Jurnal Nasional Pendidikan Matematika)
International Journal of Heat and Mass Transfer
Kaspar Jansen
Baillière's clinical gastroenterology
Aiden McCormick
- We're Hiring!
- Help Center
- Find new research papers in:
- Health Sciences
- Earth Sciences
- Cognitive Science
- Mathematics
- Computer Science
- Academia ©2024
A review on drones controlled in real-time
- Open access
- Published: 05 January 2021
- Volume 9 , pages 1832–1846, ( 2021 )
Cite this article
You have full access to this open access article
- Vemema Kangunde ORCID: orcid.org/0000-0001-7169-7632 1 ,
- Rodrigo S. Jamisola Jr. 1 &
- Emmanuel K. Theophilus 1
23k Accesses
46 Citations
Explore all metrics
This paper presents related literature review on drones or unmanned aerial vehicles that are controlled in real-time. Systems in real-time control create more deterministic response such that tasks are guaranteed to be completed within a specified time. This system characteristic is very much desirable for drones that are now required to perform more sophisticated tasks. The reviewed materials presented were chosen to highlight drones that are controlled in real time, and to include technologies used in different applications of drones. Progress has been made in the development of highly maneuverable drones for applications such as monitoring, aerial mapping, military combat, agriculture, etc. The control of such highly maneuverable vehicles presents challenges such as real-time response, workload management, and complex control. This paper endeavours to discuss real-time aspects of drones control as well as possible implementation of real-time flight control system to enhance drones performance.
Similar content being viewed by others
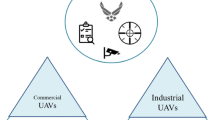
Unmanned aerial vehicles (UAVs): practical aspects, applications, open challenges, security issues, and future trends
Syed Agha Hassnain Mohsan, Nawaf Qasem Hamood Othman, … Muhammad Asghar Khan
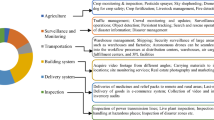
Recent Advances in Unmanned Aerial Vehicles: A Review
Faiyaz Ahmed, J. C. Mohanta, … Pankaj Singh Yadav
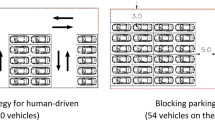
Exploring the implications of autonomous vehicles: a comprehensive review
Kareem Othman
Avoid common mistakes on your manuscript.
1 Introduction
A drone, also known as unmanned aerial vehicle (UAV), is an aircraft without a human pilot on board [ 1 , 2 ]. There has been a rapid development of drones for the past few decades due to the advancement of components such as micro electro-mechanical systems (MEMS) sensors, microprocessors, high energy lithium polymer (LiPo) batteries, as well as more efficient and compact actuators [ 3 , 4 , 5 ]. Drones are now present in many daily life activities [ 2 , 6 , 7 , 8 ]. They are used in many applications such as inspecting pipelines and power lines, surveillance and mapping, military combat, agriculture, delivery of medicines in remote areas, aerial mapping, and many others [ 2 , 9 , 10 , 11 , 12 ]. See Figs. 1 and 2 for some drones applications. Robotic manipulators, found in many applications [ 13 , 14 , 15 ], have in recent years been implemented on UAV platforms [ 16 , 17 , 18 ] for tasks such as aerial manipulation, grasping, and cooperative transportation. The unstable dynamics of the robotic arm, which increase control complexity of UAVs, have widely been studied in the literature [ 19 , 20 , 21 , 22 ].
UAVs technology is rapidly growing while UAV solutions are being proposed at faster rates as various needs arise. Drone features are determined by specific UAV applications as well as competition in the commercial market [ 23 , 23 , 24 , 25 ]. In [ 26 ], a review of the most recent applications of UAVs in the cryosphere was conducted. Compared to conventional spaceborne or airborne remote sensing platforms [ 27 , 28 , 29 ], UAVs offer more advantages in terms of data acquisition windows, revisits, sensor types, viewing angles, flying altitudes, and overlap dimensions [ 26 , 30 , 31 , 32 ]. The review shows that across the world, applications used various multirotor and fixed-wing UAV platforms. Red, green, blue (RGB) sensors were the most used, and applications utilised quality video transmission to the ground control station. The study in [ 33 ] demonstrates how versatile and fast-growing is the adoption of UAV solutions in daily life scenarios. They propose the design of a system capable of detecting coronavirus automatically from the thermal image quickly and with less human interactions using IoT-based drone technology. The UAV system is equipped with two cameras: an optical camera and a thermal camera. It conveys to the ground control station (GCS) the image of the person, the global positioning system (GPS) location as well as a thermal image of the hot body detected. The system combines IoT, virtual reality, and live video feedback to control the camera for monitoring people.
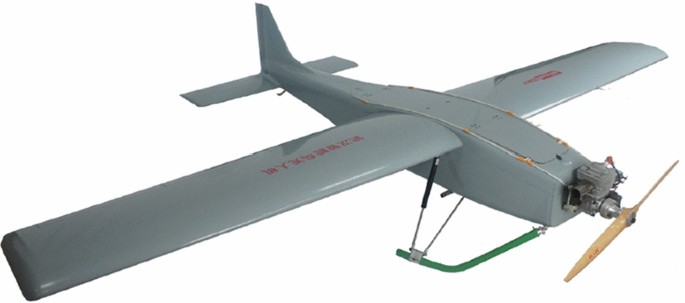
Picture reprinted from https://aibirduav.diytrade.com
The KC2800 is a fixed-wing drone used for surveillance and mapping
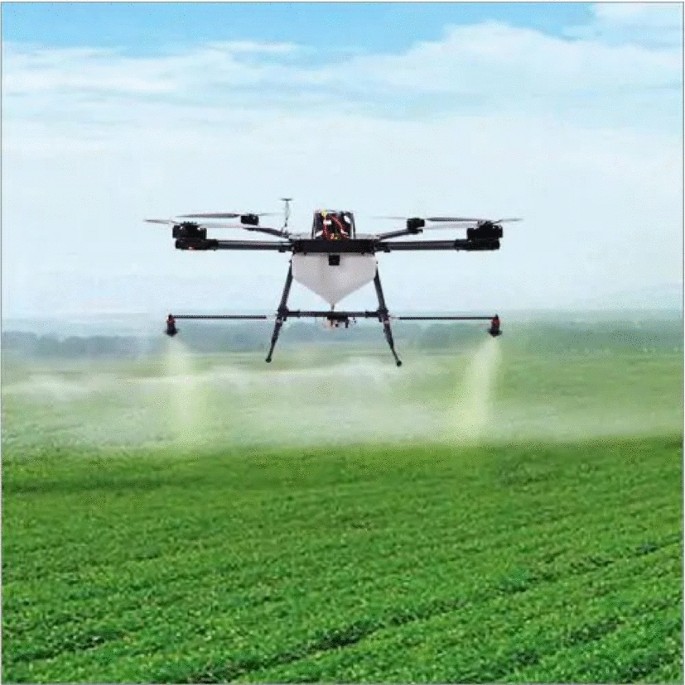
Picture reprinted from https://www.indiamart.com
Quadrotor drone spraying pesticide on crops
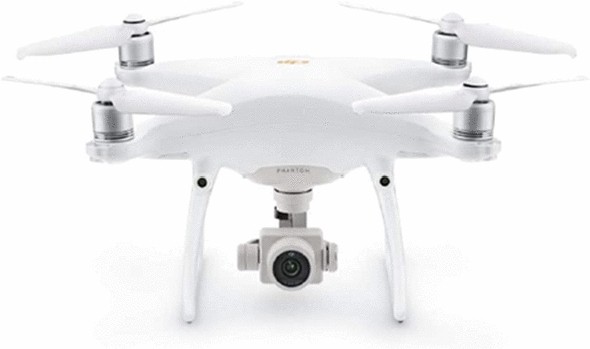
Picture reprinted from https://thewiredshopper.com
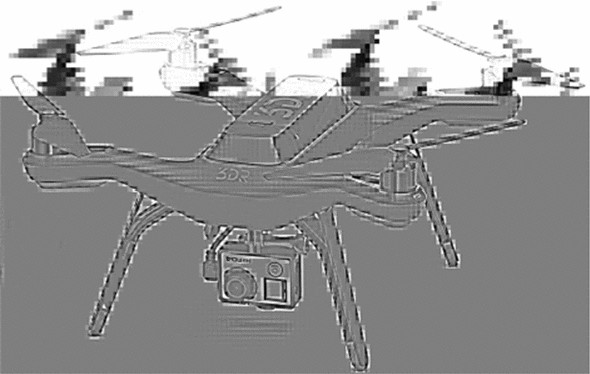
Picture reprinted from https://thewiredshopper.com
On the other hand, apart from advancements in custom-made drones, commercial drone manufacturers are actively improving their products. Latest, more advanced drones are presented at https://thewiredshopper.com , see Figs. 3 and 4 . DJI Phantom 4, for example, is equipped with an automatic collision avoidance system. It has a sport mode that disables collision detection and enables fast speeds. It also has an active tracking technology that enables the selection of another moving object, like a car or another drone, and the Phantom 4 will autonomously follow it without assistance from the human pilot. The drone is equipped with a 3-axis camera and can record 4K resolution video at 30 fps and 1080p resolution at 12 fps. It will take 12-megapixel images in Adobe DNG raw format. It has gimbal stabilization technology and a built-in video editor. Other latest drones in the market include the AirDog drone by AirDog, 3DR Solo Drone by 3DRobotics, and Yuneec Typhoon H by Yuneec. A UAV’s operational environment is highly dynamic due to unpredictable changes in weather conditions affecting the air space. For drones to be reliable, their flight controllers must adapt to these environmental changes in real-time. Control of highly maneuverable UAVs has been extensively studied for the past decades.
2 Drone hardware overview
A UAV is controlled by an embedded computer called the Flight Control System (FCS) or flight controller [ 34 , 35 , 36 ], basically consisting of a control software loaded into a microcontroller. The microcontroller reads information from on-board sensors, such as accelerometers, gyroscopes, magnetometers, pressure sensors, GPS, etc.,as well as input from the pilot, perform control calculations, and control the motors on the UAV [ 37 , 38 ]. The FCS as well as the set of sensors would be mounted on the drone air frame. Drone air frames, typically made of strong, light composite materials, are mostly relatively small with limited space for avionics [ 39 , 40 ]. A set of sensors, such as TV cameras, infrared cameras, thermal sensors, chemical, biological sensors, meteorological sensors etc., used to gather information during drone applications need to be lightweight to reduce UAV payload [ 41 , 42 , 43 , 44 ]. The information gathered from the sensors can be partially processed on-board or transmitted to the ground station for further processing [ 45 , 46 , 47 ]. An on-board controller, separate from the flight controller, can be used to operate the payload sensors [ 48 , 49 , 50 ]. Figure 5 shows the Cc3d open source flight controller used as a UAV flight controller.
The Pixhawk flight controller is an open-source hardware project equipped with sensors necessary for flight control [ 51 , 52 , 53 ]. It includes a CPU with RAM as well as gyroscope, compass, 3-axis accelerometer, barometric pressure, and magnetometer [ 54 , 55 ]. The Paparazzi flight controller, developed by Ecole Nationale de lAviation Civil (ENAC) UAV Lab since 2003 [ 34 ], is the first and oldest open-source drone hardware and software project. In March 2017 ENAC Lab released the Paparazzi Chimera autopilot. A detailed survey on open-source flight controllers was disclosed by Ebeid et.al in [ 34 ]. An autopilot software is used for drone automatic flight control [ 56 ]. On the other hand, drones can be operated remotely through a remote controller [ 57 , 58 , 59 ].
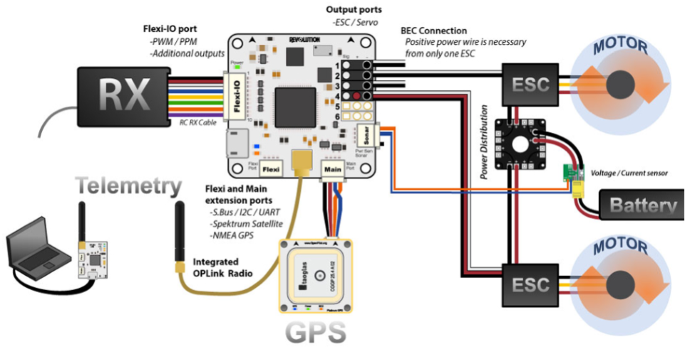
Picture reprinted from https://www.google.com/search?q=Cc3d++flight+controller
UAV hardware components
2.1 State observation
The FCS requires information on UAV states such as attitude, position, and velocity for control implementation [ 60 ]. The commonly used state observer is the inertial guidance system. Other attitude determination devices such as infrared or vision based sensors can be used [ 61 , 62 ]. The inertial guidance system (IGS), also referred to as inertial navigation system (INS) [ 63 ] consists of the inertial measurement unit (IMU) and the navigation computer. The IMU has three orthogonal rate-gyroscopes, three orthogonal accelerometers and sometimes 3-axis magnetometer to determine angular velocity, linear acceleration and orientation respectively [ 64 ]. Inertial guidance systems are entirely self reliant within a vehicle where they are used. They do not rely on transmission of signals from the vehicle or reception of signals from external sources. Inertial guidance systems can be used to estimate the location of the UAV relative to its initial position using a method known as dead reckoning [ 65 ]. Global navigation satellite system (GNSS) provides location estimates using at least four satellites [ 65 ].
2.2 State estimation
State estimation feedback is required for UAV control, such estimates are usually for attitude, position, and velocity [ 66 ]. On board sensor readings are fed to the UAV autopilot system to generate UAV state estimates [ 67 ]. The need for state estimation is due to the fact that data from measurement sensors is prone to uncertainties due to atmospheric disturbances, vibrations noise, inaccuracy of coordinate transformations, and missing measurements [ 68 ]. Sensors such as the GPS suffers from signal obstruction and reflections caused by nearby objects leading to missing or inadequate information [ 69 ].
To compensate for uncertainties and lack of information from individual sensors, multiple sensor data fusion can be employed to incorporate advantages of different types of sensors [ 70 ]. The altitude heading and reference system combines gyroscope, accelerometer, magnetometer, GPS and pressure sensors to measure UAV states. Sensor data for state estimates need to be updated at a relatively high frequency, normally above 20 Hz for small UAVs. Kalman filtering can be employed to make optimal estimations for sensors with lower update frequencies, such as the GPS, which typically has an update frequency of 4 Hz. Kalman filtering can also be used to process gyroscope readings which are susceptible to noise and drift. The other technique to improve gyroscopic readings is to model the gyroscope random noise and then offsetting it according to the model, this is referred to as model compensation [ 71 ].
2.3 Controller design for autopilots
Most current commercial and research autopilots focus on GPS-based waypoints navigation to follow a desires path [ 72 ]. Waypoint navigation is essential for autonomous control of UAVs for UAV tasks beyond the pilot’s sight. The pilot could control the UAV from the GCS using a graphical User Interface (GUI), the location as well as other needed information about the UAV would be displayed at the the GCS [ 45 ]. The path following control of a UAV involves the control of roll, pitch, altitude and air speed for trajectory tracking and waypoint navigation [ 73 ]. GPS waypoint navigation involves providing sequential GPS coordinates that contains locations and heights of the UAV flight [ 72 ]. The set of pr-programmed GPS waypoints then becomes the path for the UAV to follow [ 74 ]. In
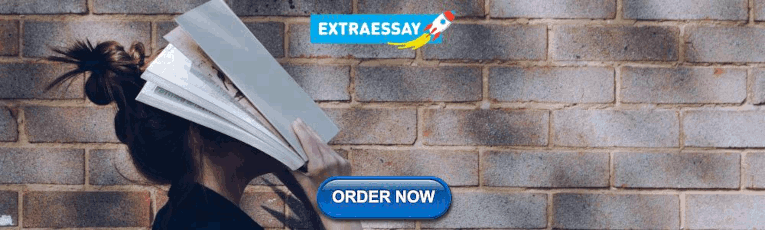
2.4 Microcontrollers used
An FCS has sensor packages for state determination, on-board processors for control and estimation uses, and peripherals for communication links and data transfer. For small UAV applications , small, light weight, and often low power consumption hardware components for the FCS are preferable. Successful UAV control requires sensors used for attitude estimation to have good performance especially in mobile and temperature-varying environments [ 75 ]. Arduino is an open-source electronics platform found in a wide variety of application projects. The board is capable of reading inputs from various sensors and generates required outputs. It comes comes with different processors and board sizes. Arduino Nano was used in [ 76 ] to develop an instrumentation system to collect flight data such as airspeed, orientation, and altitude, e.t.c. The system will then transmit the flight data over a radio frequency module.
2.5 Rotors configuration
There are different types of drones, they can generally be categorised as single rotor helicopter, fixed wing and multi-rotor drones [ 77 , 78 ]. Nowadays researchers endeavors to combine the advantages of fixed wing and multi-rotor drones [ 77 ]. Fixed wing drones are renowned for their endurance whereas helicopters and multirotors have the the advantage of VTOL as well as hovering. Quad-rotor drones are most common and belongs to the multi-copter family [ 77 ]. The quad-rotor unmanned aerial vehicle (UAV) are drones with four rotors typically designed in a cross configuration with two pairs of opposite rotors rotating clockwise and the other rotor pair rotating counter-clockwise to balance the torque. The roll, pitch, yaw and up-thrust actions are controlled by changing the thrusts of the rotors using pulse width modulation (PWM) to give the desired output [ 79 ]. Typically, the structure of a quad-rotor is simple enough, which comprises four rotors attached at the ends of arms under a symmetric frame. The dominating forces and moments acting on the quadrotor are given by rotors, driven with motors, mostly brushless DC motors. There are two basic types of quad-rotor configurations; plus and cross configurations [ 80 ]. The difference between these configurations is where the front of the quadcopter is located. To counteract reactional torque due to propeller rotation, two diagonal pair of motors (1 and 2) rotate anticlockwise while the other pair, motors (3 and 4), rotate clockwise [ 80 ]. In contrast to the plus configuration, for the same desired motion, the cross-style provides higher momentum which can increase the maneuverability performances, each move requires all four blades to vary their rotation speed [ 81 ]. However, the attitude control is basically analogous. Figure 6 shows the quadrotor cross and plus configurations respectively. The red cross depicts direction to the front of the quadrotor, in this case to the right of the pictures in the figure.
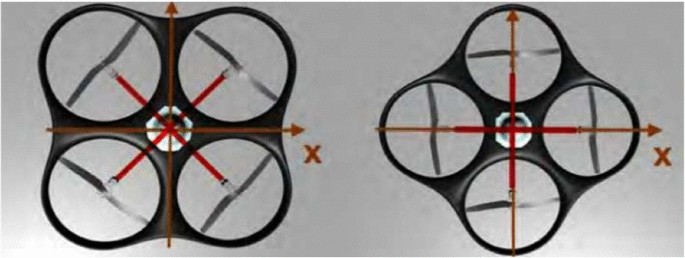
Picture reprinted from [ 82 ]
Quadrotor cross and plus Configuaration
The quad-rotors translational motion depends on the tilting of rotor craft platform towards the desired orientation. Hence, it should be noted that the translational and rotational motion are tightly coupled because the change of rotating speed of one rotor causes motion in three degrees of freedom. This is the reason that allows the quad-rotor with six degrees of freedom (DOF) to be controlled by four rotors; therefore the quad-rotor is an under actuated system [ 83 ]. In principle, a quad-rotor is dynamically unstable and therefore proper control is necessary to make it stable. Despite the unstable dynamics, it has good agility. The instability comes from the changing rotor craft parameters and the environmental disturbances such as wind. In addition, the lack of damping and the cross-coupling between degrees of freedom make it very sensitive to disturbances.
2.6 Sensors used
Essential to drone flight is the Inertial Guidance System, this is an electronic system that continuously monitors position, velocity and acceleration by means of incorporated sensor set. It consists of 3-axis rate gyro and 3-axis accelerometer as well as a magnetometer. The IGS readings are filtered to estimate the attitude of the UAV. Recent developments in computing and MEMs technology has seen the decrease in size of IGS sensors [ 84 ]. Thus for small UAVs, a micro IGS can be used to provide a complete set of sensor readings [ 75 ]. Attitude information can also be estimated using infrared (IR) thermopile sensors. They work on the fact that the earth emits more IR than the sky by measuring the heat difference between two sensors on one axis to determine the angle of the UAV. Other sensors such as Vision sensors, either by themselves or combined with inertial measurements sensors can also be used for attitude estimation [ 85 ].
3 Required software components for real-time implementation
Real-time control requires hardware and software systems to be implemented together. Several definitions for real-time systems can be found in the literature. A good definition that we found states that; “a real-time system is one in which the correctness of a result not only depends on the logical correctness of a calculation but also upon the time at which the result is made available” https://www.ibm.com . There is a time requirement, referred to as a deadline, under which the system tasks must be performed. The primary objective is to ensure a timely and deterministic response to events. In the context of drone control, such tasks are normally intended to react to external events in real-time. Thus such real-time tasks are required to keep up with external changes affecting drone performance. Tasks required to meet their deadlines to avoid catastrophic consequences are called hard real-time tasks. When meeting the deadline is desirable but not mandatory, the task is considered soft real-time task [ 86 ].
3.1 Real-time operating systems
A real-time operating system (RTOS) provides services such as multitasking, scheduling, inter-task communication, etc., to facilitate the implementation of real time-time systems [ 87 ]. An RTOS is the key component needed to build a real-time system. Other software pieces such as compilers, linker, debugger and drivers are necessary to interface with system hardware: https://www.ni.com . RTOSs are employed in the development of many applications such as Internet of Things (IoT), automotive , medical suystems, robotics, industrial automation, avionics, and flight control systems [ 88 , 89 ]. RTOSs mainly focus on task predictability and efficiency, therefore have features to support timing constraints for application tasks [ 90 ]. There are several categories of RTOS; small, proprietary kernels as well as real-time extensions to commercial time-sharing operating systems such as Unix and Linux. The kernel is the core, an essential center of the RTOS, or any computer operating system. It is responsible for memory management, processing, and task management, and to interface with hardware and application software. Small, proprietary kernels are often used in embedded applications when very fast and highly predictable execution must be guaranteed. Meeting time constraints requires kernels to be small in size, which reduces RTOS overhead. Kernels must also have a fast context switch, support for multi-tasking, priority-based preemption, provide a bounded execution time for most primitives, and maintain a high-resolution real-time clock [ 90 ].
3.2 Scheduling and prioritisation
Appropriate task scheduling in real-time applications is the basic mechanism adopted by an RTOS to meet time constraints of tasks [ 90 ]. It is the responsibility of the application developer to choose an RTOS that will schedule and execute these tasks to meet their constraints. For a given application, if a set of tasks can be scheduled such that they all meet their deadline, then the tasks are said to schedulable [ 91 ] In priority-driven (PD) scheduling, priorities are assigned to tasks. A task with the closest deadline than any other task is considered the highest priority task [ 92 ]. Embedded time critical applications employ the real-time scheduler to ensure low latency and meeting time constraints. Numeric priorities are assigned to threads constituting tasks, and only the highest priority task is selected to run by the scheduler. A higher priority task can preempt a lower priority task at any point of its execution [ 93 ].
However task priorities can also be dynamic such that a low priority task may temporary elevates its priority to prevent interruption during execution of its critical section. Preemption thresholds can also be set by considering task priority as well as task urgency. Both priority and Urgency are quantified such that it is possible for urgency to take precedence when scheduling tasks [ 86 , 93 ]. Multithreaded parallel programming systems (MPPS) has a characteristic that data is shared among threads. It is important that access to shared data is controlled to avoid associated concurrency errors. As an example, suppose a task alters or updates a global variable, it is necessary for the task to have exclusive access to that variable while it is executing, otherwise concurrent access to the same variable by other tasks will lead to data races, leading to miscompilations.Access of shared data by one task at a time can be achieved by use of Mutual exclusion locks (mutexes) [ 93 ].
3.3 Sensor inputs and feedback control
The common drone platform has a specialised software running on a computer at the ground control station. It allows users to monitor and send control messages to affect drone’s state and actions remotely. Aboard the drone, the autopilot software combines operator inputs and sensor feedback information to directly control UAV actuators [ 94 ]. Sensors onboard the UAV provide feedback data essential to determine the drone’s position and attitude. A stereo camera was proposed for obstacle avoidance as well as velocity estimation in [ 95 ]. In [ 96 ], vision and IMU sensors were employed for automatic navigation and landing of an AR drone quadrotor. A landing marker was positioned in the drone frontal camera’s sight of view, see Fig. 7 . The landing marker position is the desired position \(X_d\) = ( \(x_{d_G}\) , \(y_{d_G}\) , \(z_{d_G}\) ), which corresponds to a height above the landing marker. Position \(X = (x_G, y_G, z_G)\) denotes the drone current location. The position error is then denoted as \(E = X_d - X\) , where \(E = (e_x,e_y,e_z)\) . The symbols \(e_x,e_y,e_z\) are position errors in directions \(X_G\) , \(Y_G\) , and \(Z_G\) , respectively. The PID controller was applied to the position error in accordance with ( 1 ) and ( 2 ). The drone will land when above the marker, i.e., when the error \(E =0\) .
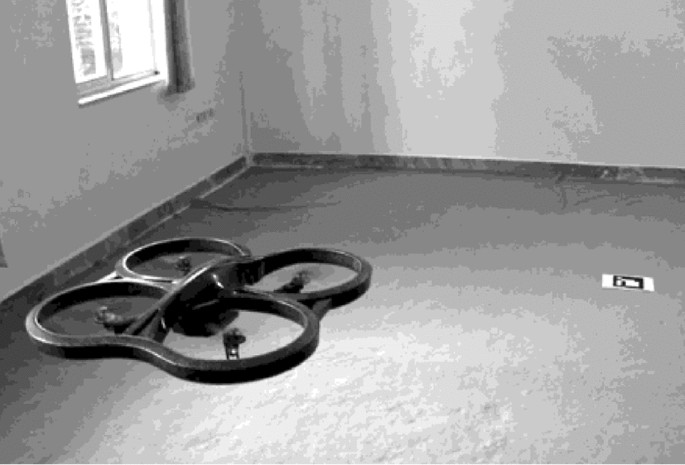
Picture reprinted from [ 96 ]
Automatic navigation and landing of an AR drone quadrotor
3.3.1 Localisation using differential global positioning system (DGPS)
Differential global positioning system (DGPS) is extensively used for accurate localisation of drones. The scope of localization and mapping for an agent is the method to locate itself locally, estimate its state, and build a 3D model of its surroundings, by employing among others vision sensors [ 97 ]. Towards this direction, a visual pose-estimation system from multiple cameras on-board a UAV, known as multi-camera parallel tracking and mapping (PTAM), has been presented in [ 98 ]. This solution was based on the monocular PTAM and was able to integrate concepts from the field of multi-camera ego-motion estimation. Additionally, in this work, a novel extrinsic parameter calibration method for the non-overlapping field of view cameras has been proposed.
3.3.2 Mobile phone technology in UAV applications
UAV applications encompass many areas, including, aerial surveillance ,reconnaissance, underground mine rescue operations, and so on [ 25 , 99 ]. Some of these application areas are GPS denied, thus GPS can not provide the location for a UAV. Currently, vision sensors, laser scanners, and the IMU are the most common position sensors used for UAV self-localisation. In some applications, small UAVs are preferred for their cost and high maneuverability. Considering the limited load capacity and the cost of small UAVs, it cannot be equipped with sensors of high precision and large volume [ 100 ].
Micro-electro-mechanical system (MEMS) sensors are therefore preferred alternatives because they are small and cheap. On the other hand, mobile phones contain multi-sensors, multi-core processors, have a small volume, and lightweight. In [ 101 ], Nexus 4 smartphone developed by Google, was used as a flight controller. The phone is equipped with inbuilt MEMS sensors such as accelerometer, gyroscope, magnetometer, global navigation satellite system (GNSS), and barometer. The implementation exclusively used sensors and processors from the smartphone, see Figs. 8 and 9 . Mobile phone usage possibilities in UAV platforms are further elaborated in [ 102 ], where a smart phone is proposed for implementation of drone control algorithms. The usage of smart phones can reduce development time as it it cuts down the need for integration of different drone hardware components, instead the proposed solution uses smart phone inbuilt sensors [ 102 ].
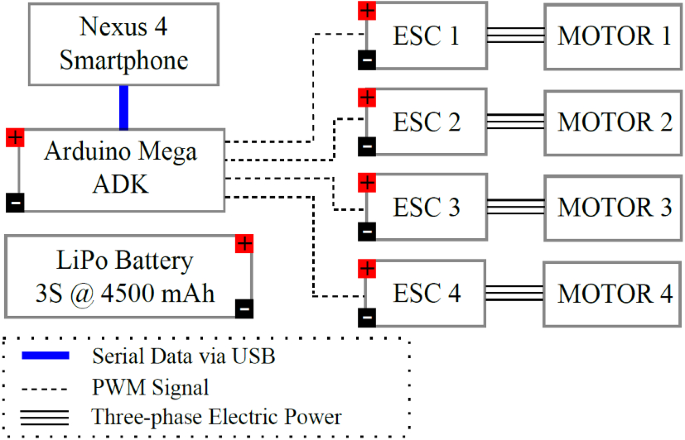
Picture reprinted from [ 101 ]
Schematic diagram for on-board smartphone flight controller using Arduino Mega to interface with the electronic speed controllers (ESCs)
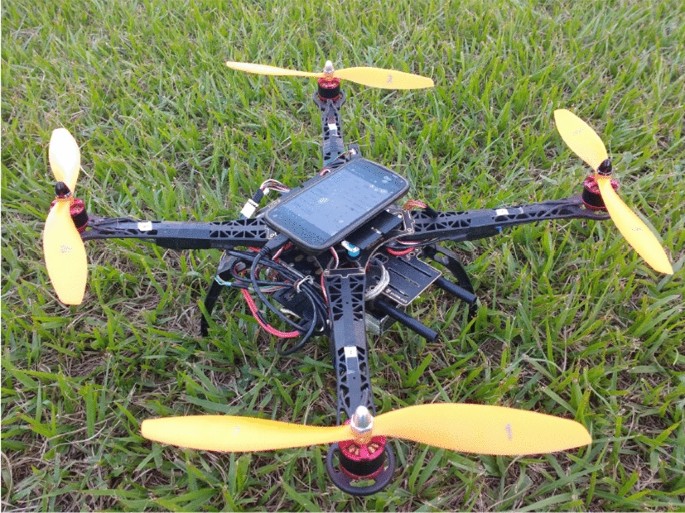
Quadcopter used in [ 101 ] with an on-board smartphone as flight controller
3.3.3 Communication to the ground control station
Communication to the ground control station allows drone pilots to remotely configure mission parameters, such as coordinates to cover during way-point navigation and the action to take at each way-point. Most existing drone platforms have the configuration shown in Fig. 10 . A specialized software runs at a ground-control station (GCS) to let users configure mission parameters. The Ground Control Station is a system made up of software and hardware necessary for UAV remote control. Hardware, such as the joystic, takes the pilot’s command which is transmitted to the drone via radio transmitter. The GCS software collects tellemetry data transmitted from the UAV and displays it the on the GCS user interface [ 103 ]. Communication networking is responsible for the information flow between GCS and UAV on a mission. It needs to be robust against uncertainties in the environment and quickly adapt to changes in the network topology. Communication is not only needed for disseminating observations, tasks, and control information but also needed to coordinate the vehicles more effectively toward a global goal. The goal could be tasks such as areal monitoring or detecting events within the shortest time, which are especially important in disaster situations. Some specific issues that need to be addressed [ 41 ] are connectivity, routing-and-scheduling, communication link models, and data transmission.
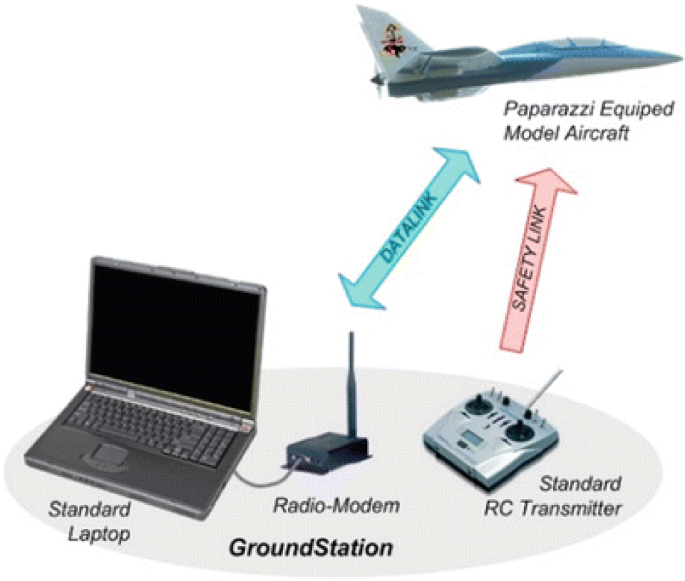
Picture reprinted from https://www.google.com/search?q=multirotor+UAV++ground+control+station+images
Platform for drone control from GCS
3.4 Real-time scheduling algorithms
Real-time scheduling aims to complete tasks within specific time constraints and avoiding simultaneous access to resources shared amongst application tasks. To guarantee real-time performance while meeting all timing, precedence and resource usage specifications requires employment of efficient scheduling algorithms supported by accurate schedulability analysis techniques [ 104 ]. Real-time scheduling algorithms can be implemented for uniprocessor or multiprocessor systems [ 105 , 106 , 107 ].
In the context of drone applications, an example could be implementing a flight control system using Arduino Uno or other single processor boards. The Arduino Uno uses the ATMEGA 328P processor (uni-processor), whereas embedded computers like the Rasberry-Pi uses a quad core ARM Cortex-A72 processor (multi-processor). Scheduling algorithms can be broadly divided into two major subsets: offline scheduling and online scheduling algorithms [ 104 ]. In offline scheduling algorithms, task scheduling is carried out before system execution, also known as pre-run time scheduling. The scheduling information is then employed during run-time. The YDS algorithm (named after the author) [ 108 ], which schedules tasks according to earliest deadline first (EDF) precedence [ 109 ] is an example of an offline scheduling algorithm. By contrast, online scheduling algorithms schedule tasks at run-time.An online scheduling algorithm that encoporates event-driven and periodic rolling strategies (EDPRS) is discussed in [ 110 ].
4 Types of controllers
UAV control requires an accurate and robust controller for altitude as well as velocity-and-heading [ 111 ].The altitude controller drives the UAV to fly at the desired altitude, including landing and take-off stages. The heading and velocity control enables UAV to fly through desired waypoints [ 112 ]. To achieve the above control requirements, different control strategies such as Fuzzy Logic,Linear Quadratic Regulator (LQG), Sliding Mode Control (SMC), Proportional Integral Derivative (PID), Neural Network (NN), e.t.c can be used. Robust control systems have been widely developed to address parametric uncertainties and external disturbance. In case of multirotor UAVs uncertainties arising from propeller rotation, blades flapping, change in propeller rotational speed and center of mass position dictates the need for a robust nonlinear controller [ 113 ]. In [ 113 ] robustness as well as compensation forsysten nonlinearities was adresses by combinig the nonlinear sliding mode control (SMC), robust backstepping controller and a nonlinear disturbance observer (NDO). The backstepping controller stabilised translational movement while the SMC controlled the rotational movement of the quadrotor.
The NDO provided all the estimates of disturbances ensuring robustness of the feedback controls. The PID controller was compared with a neural network controller, specifically the direct inverse control neural network (DIC-ANN) in [ 114 ]. The comparison was done in simulation, where both controllers were excited with the same reference altitude reference input and their performances plotted together.The simulation aimed to mimic a quadrotor flight in four phases comprising take-off and climb phase at \(0~<~t<~10~s\) , hovering phase at \(10~<~t~<20~s\) , climb in ramp phase at \(20~<~t<~22.5~s\) , and lastly the final altitude phase at \(22.5~<~t<~50~s\) . The comparison results showed that the DIC-ANN performed better than the PID controller in handling quadrotor altitude dynamics.Also at hovering conditions the DIC-ANN exhibited less steady state error as compared to the PID controller and the transient oscillations damped faster with the DIC-ANN showing that it handles nonlinearities better than the PID controller.
PID controllers are widely used in autopilots due to their ease of implementation, how ever they have limitations when operating in unpredictable and harsh environments. In [ 115 ] the performance of and acuracy of an attitude controller was investigated. The attitude controller is a neural network (NN) based controller trained through reinforcement learning (RL) state of the art algorithms, the Deep Deterministic Policy Gradient (DDPG), Trust Region Pocy Optimisation (TRPO), and the Proximal Policy Optimisation (PPO). The NN controller performance was compared to the performance of a PID controller to determine the appropriacy of NN controller in high precision, time-critical flight control. The contoller performance was evaluated in simulation using GYMFC environment. The results showed that RL can trail accurate attitude attitude controllers, also the controller trained with PPO outperformed a fully tuned PID controller on almost every metric.
The linear quadratic regulation (LQR) optimal control algorithm operates a dynamic system by minimizing a suitable cost function [ 79 ]. When the LQR is used with linear quadratic estimator (LQE) and Kalman filter, it is then referred to as the linear quadratic Gaussian (LQG) The LQG was applied in [ 116 ] for altitude control of a quadrotor micro aerial vehicles (MAVs). Ignoring air resistance, the linearized model for altitude control problem was obtained as ( 3 ), the state space model is represented by ( 4 ) , while the cost function is given by ( 5 ), also refered to in [ 116 ] as the quadratic form creterion. The control objective is to determine the control input U ( t ) to minimise cost function [ 79 ].
The linear Quadratic regulator with and integral with an integral term (LQTI) and a model predictive controller were employed to develop an automatic carrier landing system for a UAV [ 117 ]. The LQTI was applied to the coupled multi-input multi-output (MIMO) UAV dynamic model to reduce steady stare error while the model predictive controller was applied to the final phase landing of the UAV. Automatic carrier landing was performed sequentially by the two controllers. The LQTI controller was applied up to a few seconds before touch down followed by the MPC controller during the final stage of landing. The controller was verified via simulations on HSS Hydro toolbox. Simulation results indicated that the proposed carrier landing system can improve landing accuracy. The performance of the controllers indicted that the LQTI is suitable for calm sea environments while the MPC performs better even in rough sea environments [ 117 ]. Some implementations for UAV control employ the sliding-mode control (SMC) strategy. Sliding-mode control is a nonlinear control method that that utilises a high-frequency switching control signal to the system to command it to slide along a prescribed sliding manifold [ 118 , 119 ]. It encompasses a broad range of varying fields, from pure mathematical problems to application aspects [ 120 ] (Fig. 11 ).
An SMC based fault tolerant control design for underactuated UAVs was implemented on a quadrotor in [ 121 ]. The design approach separated system dynamics into two sub-systems, a fully actuated and an under-actuated subsystem. A Nonsingular Fast Terminal Sliding Mode Controller (NFTSMC) was then designed for the fully actuated subsystem, the Under-actuated Sliding Mode Controller (USSMC) was then derived for the under-actuated subsystem. The controller performance, on a quadrotor platform, demonstrated excellent robustness to actuator faults, disturbances. It had fast convergence and high precision tracking. Herrera et al. designed a sliding-mode controller and applied it in simulation of a quadrotor. They considered a PD sliding surface for vertical take-off and landing. Broad coverage of control algorithms for quadrotors can be found in [ 79 , 122 , 123 , 124 ]. Figures 12 , 13 and 14 shows the PID, LQG, and SMC controllers applied to a quadrotor respectively.
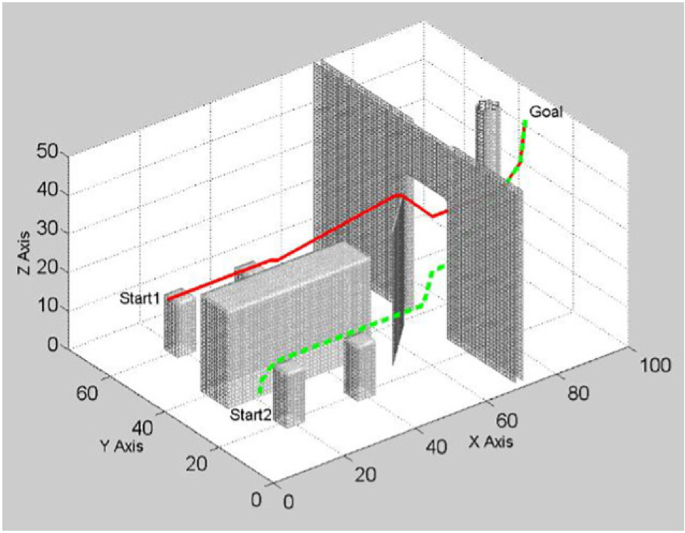
Picture reprinted [ 127 ]
Drone path planning from start 1 and Start 2 to Goal, shortest path taken from both starting points
5 Path planning
Missions of UAVs usually involve travelling from some initial point to a goal point [ 125 , 126 ]. A mission requires generating a path for the UAV to follow. Path planning is one of the main aspects of autonomous navigation [ 127 ]. The path planning problem is to produce a path or set of waypoints for the drone to follow while taking into account the environmental and physical constraints of the drone in order to achieve a collision free flight [ 128 , 129 ]. This is obstacle avoidance while executing the the UAV’s mission. Figure 11 depicts drone paths from start to goal position for two drones launched from different locations, each calculating its best path to reach the goal position.
In the literature pertinent to UAV path planning, several algorithms for measuring distances to obstacles and calculations of the drone’s path are suggested [ 130 , 131 , 132 ]. An optimal flight path planning mechanism to determine the best path of the UAV was developed in [ 133 ]. Consideration of environmental information such as geographical topology,location dependent wireless communication channel statistics and flight risk, sensor node deployment and worth of sensing information for different sensor types was made. The implementation aimed at determining the best path to maximise the value of gathered sensing information as well as to minimise flying time, energy consumption, and UAV operational risks. In [ 127 ], 3D propagation approximate Euclidean distance transformation algorithm was formulated to achieve safe path planning by calculating a 3D buffer around the obstacles. The algorithm prevents the drone from flying too close to obstacles by setting the minimal distance from obstacles according to the size of the drone. The algorithm is also used for drone path planning in [ 127 ]. It is worth noting that current techniques for UAVs path planning are application dependent. Different applications require different path-planning approaches.
A method to enhance massive unmanned aerial vehicles for mission critical applications (e.g., dispatching many UAVs from a source to a destination for firefighting) is investigated in [ 134 ]. The method aims to achieve UAV fast travel while avoiding inter-UAV collision while executing their mission. The path planning problem is tackled by exploiting a mean-field game (MFG) theoretic control method. The method requires UAV state exchange only once at launch, thereafter each UAV controls its acceleration by locally solving two partial differential equations, the Hamilton-Jacobi-Bellman (HJB) and Fokker-Planck-Kolmogorov (FPK) equations. Due to high computational burden posed by solving the partial differential equations, two machine learning models were used to approximate the solutions of the HJB and the FPK. The performance of the proposed method was validated on simulation, showing that the mean-field game method guarantees UAV collision avoidance. Also for the proposed approach, the effectiveness of the mean field game method is determined by the level of the HJB and FPK training.
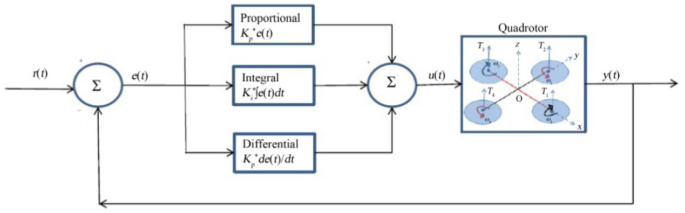
Block diagram of PID controller applied to a quadrotor [ 79 ]
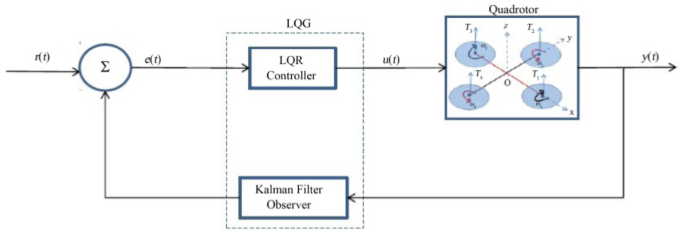
Block diagram of LQG controller applied to a quadrotor [ 79 ]
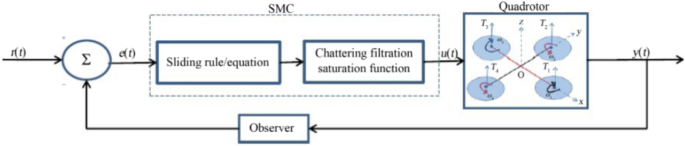
Block diagram of an SMC controller applied to a quadrotor [ 79 ]
6 UAV real-time control implementation
In order to implement real-time control for UAVs, tasks have to be defined. An RTOS is required for tasks scheduling, inter-task communication, and management of available resources such memory, and power consumption [ 135 , 136 , 137 ]. Each task is allocated a memory space, called a stack, in the microprocessor. This is enabled by the RTOS kernel’s support for multi-threading [ 138 , 139 ]. Scheduling and prioritisation of tasks, as well as the update frequency of the sensors providing essential data for task execution, ensure that application time constraints are met [ 140 ]. In [ 141 ], an embedded RTOS (RT-Thread) is applied to a quadcopter to address problems of real-time response, heavy workload and difficulty in control. Practical tests in this work indicated that quadcopter control system based on RT-Thread responded real-timely, and ensured smooth flight with a PID control algorithm.
The application tasks defined in this work are attitude information acquisition, attitude information fusion, and PID control. The latter is for quadcopter control. The application task is developed on top of RT-Thread RTOS running on STM32F407VGT6 microprocessor. The processor is equipped with high-performance ARM Cortex-M4 core with maximum system frequency of 168MHz, an FPU (floating-point unit), 1 Mbyte of flash, and 192 Kbytes of SRAM. It has peripherals such as ADC, SPI, USART, controller area network (CAN) bus, DMA, etc. High operating frequencies and high-speed memory provide high computational power to enable quadcopter complex calculations to be performed. Also additional peripherals reduce the need for external IC and reduce computational burden from the microprocessor. The implementation in [ 142 ] uses a dual processor configuration.
One processor is used for telemetry and another for control of a custom quadcopter used as a test-bed. The telemetry processor executes software tasks such as communicating reconfiguration and monitoring data with the GCS, data collection from sensors, and wirelessly transmitting data to the GCS. The tasks are managed by \(\mu \) C/OS-II™, an RTOS. The control processor runs the PID controller algorithm for the quadcopter stabilization and navigation. This task was achieved through several tasks allocated to the control processor. Tasks include reading GPS, compass, IMU, and altitude sensor data received from the telemetry processor. Other tasks include implementation of the roll, pitch, yaw, and altitude PID control loops, and communicating reconfiguration and monitoring data with the telemetry processor via CAN bus. Figure 15 shows the PID controllers used in the implementation.
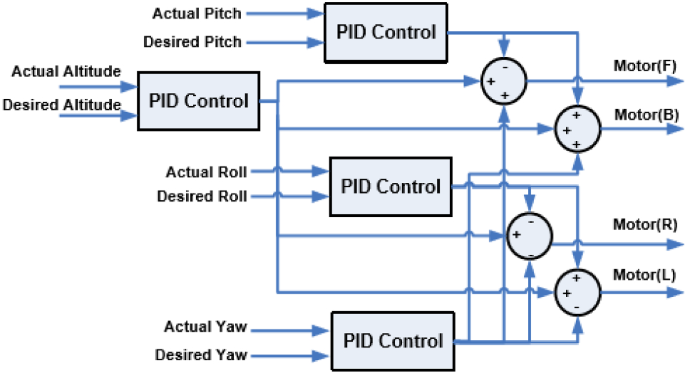
Picture reprinted from [ 142 ]
PID control loops implemented by the control processor
7 Essential components for UAV real-time applications
7.1 real-time operating system (rtos).
The literature pertaining to real-time implementation of drone control systems is relatively limited, and the number of reported studies on UAV scheduling has been minimal [ 143 ]. The main feature of real-time implementation in drones control is that an embedded RTOS, also referred to as UAV operation system in some literature, is required [ 67 , 144 ]. The RTOS provides a real-time kernel on which the control program running on a micro-controller is implemented. The real-time kernel guarantees application tasks meet their time constraints by employing the UAV scheduling system [ 143 ]. Consequently, a Real-Time Operating System (RTOS) that provides operating environments for various mission services on UAVs is crucial [ 145 ]. The commonly used RTOS for UAVs is FreeRTOS, and an empirical study of this RTOS was conducted in [ 145 ]. The study looked at aspects such as functionality changes during the evolution of FreeRTOS. A total of 85 releases of FreeRTOS, from V2.4.2 to V10.0.0 were considered.
7.2 Microcontroller
The microcontroller is the UAV onboard processing unit for UAV computations and UAV state monitoring [ 146 , 147 ]. It is selected such that it matches application task requirements. Considerations such as computational speeds and communication with onboard sensors have to be made. Palossi et al. [ 146 ] extended the hardware and software of a 27 grams nano-size, commercial off-the-shelf (COTS) quadrotor, the crazyflie 2.0, to achieve object tracking capability. The quadrotor platform consists the STM32F405 microcontroller as the main onboard processing unit, the Nordic nRF51 module for wireless communication. The STM32 is an ARM Cortex-M4F microcontroller, operating at 168MHz. The on-board sensing is performed by a 9-axis IMU, the MPU-9250 with a gyroscope, an accelerometer, a magnetometer, and an ST LPS25H pressure sensor with a typical accuracy of \(\pm 1\) meter. The vehicle is powered by a 240mAh Li-Po battery.
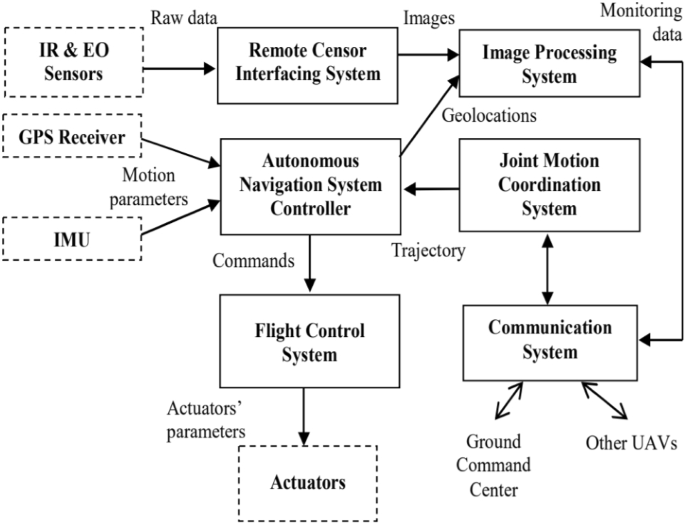
Reprinted from [ 150 ]
Sensors connected to microcontroller
7.3 Sensors and actuators
In UAV applications several sensors and actuators are connected to the microprocessor for UAV control. Table 1 highlights the vital components for real-time implementation of UAV control, the table also lists various sensors used. Figure 16 shows the UAV onboard sensors used in a fire fighting remote-sensing system in [ 150 ]. Various sensors as well as the overall connection network is depicted.
8 Conclusion
Real-time control of drones requires an embedded RTOS for implementation. The RTOS provides facilities such as multi-threading, scheduling and priority assignment. These support real-time response of the drone control system to feedback from GPS and IMU. The drone control system subsequently apply the corresponding motor speeds to achieve the desired drone’s movements. Multitasking enables tasks, such as position and orientation feedback, path-planning, and control implementation to run in parallel. This facilitates real-time response of the drone. Tasks may need results from other tasks for their computations. Scheduling and prioritisation of tasks ensures that at any point in time critical tasks are given computational resources by the microprocessor. For example obstacle avoidance is the highest priority task to ensure that the drone does not collide with other drones as well as other obstacles.
Kanellakis C, Nikolakopoulos G (2017) Survey on computer vision for UAVs: current developments and trends. J Intell Robot Syst 87(1):141–168
Google Scholar
Han D, Gwak DY, Lee S (2020) Noise prediction of multi-rotor UAV by RPM fluctuation correction method. J Mech Sci Technol 34:1429–1443
Fotouhi A, Qiang H, Ding M, Hassan M, Giordano LG, Garcia-Rodriguez A, Yuan J (2019) Survey on UAV cellular communications: practical aspects, standardization advancements, regulation, and security challenges. IEEE Commun Surv Tutor 21(4):3417–3442
Atyabi A, Mahmoud-Zadeh S, Nefti-Meziani S (2018) Current advancements on autonomous mission planning and management systems: an AUV and UAV perspective. Annu Rev Control 46:196–215
Jawhar I, Mohamed N, Al-Jaroodi J, Agrawal DP, Zhang S (2017) Communication and networking of UAV-based systems: classification and associated architectures. J Netw Comput Appl 84:93–108
Radoglou-Grammatikis P, Sarigiannidis P, Lagkas T, Moscholios I (2020) A compilation of UAV applications for precision agriculture. Comput Netw 172:107148
Balasingam M (2017) Drones in medicine—the rise of the machines. Int J Clin Pract 71(9):1–4
Parks L (2016) Drones, vertical mediation, and the targeted class. Fem Stud 42(1):227–235
Abbasi Y, Moosavian SAA, Novinzadeh AB (2017) Vision-based formation control of aerial robots in the presence of sensor failure. J Mech Sci Technol 31(3):1413–1426
Rao B, Gopi AG, Maione R (2016) The societal impact of commercial drones. Technol Soc 45:83–90
Nex F, Remondino F (2014) UAV for 3D mapping applications: a review. Appl Geomat 6(1):1–15
Menouar H, Guvenc I, Akkaya K, Uluagac AS, Kadri A, Tuncer A (2017) UAV-enabled intelligent transportation systems for the smart city: applications and challenges. IEEE Commun Mag 55(3):22–28
Williams HA, Jones MH, Nejati M, Seabright MJ, Bell J, Penhall ND, Barnett JJ, Duke MD, Scarfe AJ, Ahn HS et al (2019) Robotic kiwifruit harvesting using machine vision, convolutional neural networks, and robotic arms. Biosyst Eng 181:140–156
Park D, Shaear M, Chen YH, Russell JO, Kim HY, Tufano RP (2019) Transoral robotic thyroidectomy on two human cadavers using the intuitive da vinci single port robotic surgical system and CO2 insufflation: preclinical feasibility study. Head Neck 41(12):4229–4233
Jamisola RS, Maciejewski AA, Roberts RG (2006) Failure-tolerant path planning for kinematically redundant manipulators anticipating locked-joint failures. IEEE Trans Rob 22(4):603–612
Kim MJ, Kondak K, Ott C (2018) A stabilizing controller for regulation of UAV with manipulator. IEEE Robot Autom Lett 3(3):1719–1726
Muscio G, Pierri F, Trujillo MA, Cataldi E, Antonelli G, Caccavale F, Viguria A, Chiaverini S, Ollero A (2017) Coordinated control of aerial robotic manipulators: theory and experiments. IEEE Trans Control Syst Technol 26(4):1406–1413
Ramon Soria P, Arrue BC, Ollero A (2017) Detection, location and grasping objects using a stereo sensor on UAV in outdoor environments. Sensors 17(1):103
Xiao B, Yin S (2018) Exponential tracking control of robotic manipulators with uncertain dynamics and kinematics. IEEE Trans Ind Inf 15(2):689–698
Yang C, Jiang Y, Na J, Li Z, Cheng L, Su CY (2018) Finite-time convergence adaptive fuzzy control for dual-arm robot with unknown kinematics and dynamics. IEEE Trans Fuzzy Syst 27(3):574–588
Jamisola RS Jr, Mastalli C, Ibikunle F (2016) Modular relative jacobian for combined 3-arm parallel manipulators. Int J Mech Eng Robot Res 5(2):90–95
Jamisola RS, Kormushev PS, Roberts RG, Caldwell DG (2016) Task-space modular dynamics for dual-arms expressed through a relative jacobian. J Intell Robot Syst 83(2):205–218
Chen S, Laefer DF, Mangina E (2016) State of technology review of civilian UAVs. Recent Patents Eng 10(3):160–174
Dupont QF, Chua DK, Tashrif A, Abbott EL (2017) Potential applications of UAV along the construction’s value chain. Procedia Eng 182:165–173
Otto A, Agatz N, Campbell J, Golden B, Pesch E (2018) Optimization approaches for civil applications of unmanned aerial vehicles (UAVs) or aerial drones: a survey. Networks 72(4):411–458
MathSciNet Google Scholar
Gaffey C, Bhardwaj A (2020) Applications of unmanned aerial vehicles in cryosphere: latest advances and prospects. Remote Sens 12(6):1–40
Garaba SP, Dierssen HM (2018) An airborne remote sensing case study of synthetic hydrocarbon detection using short wave infrared absorption features identified from marine-harvested macro-and microplastics. Remote Sens Environ 205:224–235
Zhang J, Yang C, Song H, Hoffmann WC, Zhang D, Zhang G (2016) Evaluation of an airborne remote sensing platform consisting of two consumer-grade cameras for crop identification. Remote Sens 8(3):257
Koc CB, Osmond P, Peters A, Irger M (2018) Understanding land surface temperature differences of local climate zones based on airborne remote sensing data. IEEE J Sel Top Appl Earth Obs Remote Sens 11(8):2724–2730
Bhardwaj A, Sam L, Martín-Torres FJ, Kumar R et al (2016) UAVs as remote sensing platform in glaciology: present applications and future prospects. Remote Sens Environ 175:196–204
Yang G, Liu J, Zhao C, Li Z, Huang Y, Yu H, Xu B, Yang X, Zhu D, Zhang X et al (2017) Unmanned aerial vehicle remote sensing for field-based crop phenotyping: current status and perspectives. Front Plant Sci 8:1–26
Näsi R, Honkavaara E, Blomqvist M, Lyytikäinen-Saarenmaa P, Hakala T, Viljanen N, Kantola T, Holopainen M (2018) Remote sensing of bark beetle damage in urban forests at individual tree level using a novel hyperspectral camera from UAV and aircraft. Urban For Urban Green 30:72–83
Mohammed M, Hazairin NA, Al-Zubaidi S, Sairah AK, Mustapha S, Yusuf E (2020) Toward a novel design for coronavirus detection and diagnosis system using IoT based drone technology. Int J Psychosoc Rehabil 24(7):2287–2295
Ebeid E, Skriver M, Terkildsen KH, Jensen K, Schultz UP (2018) A survey of open-source UAV flight controllers and flight simulators. Microprocess Microsyst 61:11–20
Zhang G, Hsu LT (2018) Intelligent GNSS/INS integrated navigation system for a commercial UAV flight control system. Aerosp Sci Technol 80:368–380
Zhou L, Zhang J, She H, Jin H (2019) Quadrotor UAV flight control via a novel saturation integral backstepping controller. Automatika 60(2):193–206
Silvagni M, Tonoli A, Zenerino E, Chiaberge M (2017) Multipurpose UAV for search and rescue operations in mountain avalanche events. Geomat Nat Hazards Risk 8(1):18–33
Khalesi MH, Salarieh H, Foumani MS (2019) Dynamic modeling, control system design and MIL–HIL tests of an unmanned rotorcraft using novel low-cost flight control system. Iran J Sci Technol Trans Mech Eng 1–20
Corrigan F (2018) How do drones work and what is drone technology. DroneZon. 24 June 2018
Vasić Z, Maksimović S, Georgijević D (2018) Applied integrated design in composite UAV development. Appl Compos Mater 25(2):221–236
Yanmaz E, Yahyanejad S, Rinner B, Hellwagner H, Bettstetter C (2018) Drone networks: communications, coordination, and sensing. Ad Hoc Netw 68:1–15
Aasen H, Burkart A, Bolten A, Bareth G (2015) Generating 3D hyperspectral information with lightweight UAV snapshot cameras for vegetation monitoring: from camera calibration to quality assurance. ISPRS J Photogramm Remote Sens 108:245–259
Zhong Y, Wang X, Xu Y, Wang S, Jia T, Hu X, Zhao J, Wei L, Zhang L (2018) Mini-UAV-borne hyperspectral remote sensing: from observation and processing to applications. IEEE Geosci Remote Sens Mag 6(4):46–62
Natesan S, Armenakis C, Benari G, Lee R (2018) Use of UAV-borne spectrometer for land cover classification. Drones 2(2):16
Li B, Jiang Y, Sun J, Cai L, Wen CY (2016) Development and testing of a two-UAV communication relay system. Sensors 16(10):1696
She C, Liu C, Quek TQ, Yang C, Li Y (2019) Ultra-reliable and low-latency communications in unmanned aerial vehicle communication systems. IEEE Trans Commun 67(5):3768–3781
Rafifandi R, Asri DL, Ekawati E, Budi EM (2019) Leader-follower formation control of two quadrotor UAVs. SN Appl Sci 1(6):539
Pastor E, Lopez J, Royo P (2007) UAV payload and mission control hardware/software architecture. IEEE Aerosp Electron Syst Mag 22(6):3–8
González-deSantos LM, Martínez-Sánchez J, González-Jorge H, Navarro-Medina F, Arias P (2020) UAV payload with collision mitigation for contact inspection. Autom Constr 115:103200
González-de Santos L, Martínez-Sánchez J, González-Jorge H, Novo A, Arias P (2019) First approach to UAV-based contact inspection: a smart payload for navigation in the neighbourhood of structures. ISPAr 4213:323–328
Sanchez-Lopez JL, Pestana J, De La Puente P, Campoy P (2016) A reliable open-source system architecture for the fast designing and prototyping of autonomous multi-UAV systems: simulation and experimentation. J Intell Robot Syst 84(1–4):779–797
Sabikan S, Nawawi S (2016) Open-source project (OSPs) platform for outdoor quadcopter. J Adv Res Des 24:13–27
Yang K, Yang GY, Fu SIH (2020) Research of control system for plant protection UAV based on pixhawk. Procedia Comput Sci 166:371–375
Bingler A, Mohseni K (2017) Dual radio autopilot system for lightweight, swarming micro/miniature aerial vehicles. J Aerosp Inf Syst 14(5):293–306
Garberoglio L, Meraviglia M, Pose CD, Giribet JI, Mas I (2018) Choriboard III: a small and powerful flight controller for autonomous vehicles. In: 2018 Argentine Conference on Automatic Control (AADECA), Buenos Aires, Argentina. IEEE, pp 1–6
Zareb M, Nouibat W, Bestaoui Y, Ayad R, Bouzid Y (2020) Evolutionary autopilot design approach for UAV quadrotor by using GA. Iran J Sci Technol Trans Electr Eng 44(1):347–375
González JMF, Padilla P, Valenzuela-Valdes JF, Padilla JL, Sierra-Perez M (2017) An embedded lightweight folded printed quadrifilar helix antenna: UAV telemetry and remote control systems. IEEE Antennas Propag Mag 59(3):69–76
Wang H, Wang J, Chen J, Gong Y, Ding G (2018) Network-connected UAV communications: potentials and challenges. China Commun 15(12):111–121
Bhola R, Krishna NH, Ramesh K, Senthilnath J, Anand G (2018) Detection of the power lines in UAV remote sensed images using spectral–spatial methods. J Environ Manag 206:1233–1242
Cui JQ, Lai S, Dong X, Chen BM (2016) Autonomous navigation of UAV in foliage environment. J Intell Robot Syst 84(1–4):259–276
Rawashdeh NA, Rawashdeh OA, Sababha BH (2017) Vision-based sensing of UAV attitude and altitude from downward in-flight images. J Vib Control 23(5):827–841
Chen S, Duan H, Deng Y, Li C, Zhao G, Xu Y (2017) Drogue pose estimation for unmanned aerial vehicle autonomous aerial refueling system based on infrared vision sensor. Opt Eng 56(12):124105
Cai X, Hsu H, Chai H, Ding L, Wang Y (2019) Multi-antenna GNSS and INS integrated position and attitude determination without base station for land vehicles. J Navig 72(2):342–358
Kumar GA, Patil AK, Patil R, Park SS, Chai YH (2017) A LiDAR and IMU integrated indoor navigation system for UAVs and its application in real-time pipeline classification. Sensors 17(6):1268
Kuutti S, Fallah S, Katsaros K, Dianati M, Mccullough F, Mouzakitis A (2018) A survey of the state-of-the-art localization techniques and their potentials for autonomous vehicle applications. IEEE Internet Things J 5(2):829–846
Noor-A-Rahim M, Khyam MO, Ali GMN, Liu Z, Pesch D, Chong PH (2019) Reliable state estimation of an unmanned aerial vehicle over a distributed wireless IoT network. IEEE Trans Reliab 68(3):1061–1069
Hujja RM, Sumiharto R, Setyawan Bekti W (2018) Realtime operating system implementation on AVR XMEGA for unmanned aerial vehicle autopilot. Int J Adv Res Sci Eng Technol 5:5762–5768
Al-Mashhadani MA (2019) Optimal control and state estimation for unmanned aerial vehicle under random vibration and uncertainty. Meas Control 52(9–10):1264–1271
Wright WC, Wilkinson BE, Cropper WP Jr (2017) Estimating GPS signal loss in a natural deciduous forest using sky photography. Pap Appl Geogr 3(2):119–128
Lu Y, Xue Z, Xia GS, Zhang L (2018) A survey on vision-based UAV navigation. Geo-Spat Inf Sci 21(1):21–32
Guo H, Hong H (2019) Research on filtering algorithm of MEMS gyroscope based on information fusion. Sensors 19(16):3552
Kwak J, Sung Y (2018) Autonomous UAV flight control for GPS-based navigation. IEEE Access 6:37947–37955
Capello E, Guglieri G, Ristorto G (2017) Guidance and control algorithms for mini UAV autopilots. Aircr Eng Aerosp Technol 89(1):133–144
Vanegas F, Gonzalez F (2016) Enabling UAV navigation with sensor and environmental uncertainty in cluttered and GPS-denied environments. Sensors 16(5):1–17
Chao H, Cao Y, Chen Y (2010) Autopilots for small unmanned aerial vehicles: a survey. Int J Control Autom Syst 8(1):36–44
Dapper e Silva T, Cabreira V, De Freitas EP (2018) Development and testing of a low-cost instrumentation platform for fixed-wing UAV performance analysis. Drones 2(2):19
Hassanalian M, Abdelkefi A (2017) Classifications, applications, and design challenges of drones: a review. Prog Aerosp Sci 91:99–131
Yang H, Lee Y, Jeon SY, Lee D (2017) Multi-rotor drone tutorial: systems, mechanics, control and state estimation. Intell Serv Robot 10(2):79–93
Zulu A, John S (2014) A review of control algorithms for autonomous quadrotors. Open J Appl Sci 4:547–556
Noordin A, Basri MM, Mohamed Z, Abidin AZ (2017) Modelling and PSO fine-tuned PID control of quadrotor UAV. Int J Adv Sci Eng Inf Technol 7(4):1367–1373
Gupte S, Mohandas PIT, Conrad JM (2012) A survey of quadrotor unmanned aerial vehicles. In: 2012 Proceedings of IEEE Southeastcon. IEEE, pp 1–6
Orsag M, Bogdan S (2012) Influence of forward and descent flight on quadrotor dynamics. Recent Adv Aircr Technol 141–156
Emran BJ, Najjaran H (2018) A review of quadrotor: an underactuated mechanical system. Annu Rev Control 46:165–180
Roh MS, Kang BS (2018) Dynamic accuracy improvement of a MEMS AHRS for small UAVs. Int Jo Precis Eng Manuf 19(10):1457–1466
Tang Y, Hu Y, Cui J, Liao F, Lao M, Lin F, Teo RS (2018) Vision-aided multi-UAV autonomous flocking in GPS-denied environment. IEEE Trans Ind Electron 66(1):616–626
Wang W, Wang Y, Dai J, Cao Z (2019) Dynamic soft real-time scheduling with preemption threshold for streaming media. Int J Digit Multimed Broadcast 1–8
Turci LDO (2017) Real-time operating system freertos application for fire alarm project in reduced scale. Int J Comput Digit Syst 6(04):197–204
Nissimagoudar P, Mane V, Shamshuddin K, Gireesha H, Joshi R, Kiran M, Desai B (2016) Integrated course projects in automotive electronics and RTOS. J Eng Educ Transform 30(2):71–78
Macher G, Atas M, Armengaud E, Kreiner C (2015) Automotive real-time operating systems: a model-based configuration approach. ACM Sigbed Rev 11(4):67–72
Stankovic JA, Rajkumar R (2004) Real-time operating systems. Real-Time Syst 28(2–3):237–253
MATH Google Scholar
Andersson B, Kim H, Niz DD, Klein M, Rajkumar R, Lehoczky J (2018) Schedulability analysis of tasks with corunner-dependent execution times. ACM Trans Embed Comput Syst (TECS) 17(3):1–29
Kang Y, Joo W, Lee S, Shin D (2017) Priority-driven spatial resource sharing scheduling for embedded graphics processing units. J Syst Architect 76:17–27
Miné A (2017) Static analysis of embedded real-time concurrent software with dynamic priorities. Electron Notes Theor Comput Sci 331:3–39
Hellaoui H, Bekkouche O, Bagaa M, Taleb T (2018) Aerial control system for spectrum efficiency in UAV-to-cellular communications. IEEE Commun Mag 56(10):108–113
Mcguire K, Croon GD, Wagter CD, Tuyls K, Kappen H (2017) Efficient optical flow and stereo vision for velocity estimation and obstacle avoidance on an autonomous pocket drone. IEEE Robot Autom Lett 2(2):1070–1076. https://doi.org/10.1109/lra.2017.2658940
Article Google Scholar
Sani MF, Karimian G (2017) Automatic navigation and landing of an indoor AR. drone quadrotor using AruUo marker and inertial sensors. In: 2017 International Conference on Computer and Drone Applications (IConDA), Kuching, Sarawak, Malaysia. IEEE, pp 102–107
Artieda J, Sebastian JM, Campoy P, Correa JF, Mondragón IF, Martínez C, Olivares M (2009) Visual 3D slam from UAVs. J Intell Robot Syst 55(4–5):299
Harmat A, Trentini M, Sharf I (2015) Multi-camera tracking and mapping for unmanned aerial vehicles in unstructured environments. J Intell Robot Syst 78(2):291–317
Molaei F, Rahimi E, Siavoshi H, Afrouz SG, Tenorio V (2020) A comprehensive review on internet of things (IoT) and its implications in the mining industry. Am J Eng Appl Sci 13(3):499–515
Zhao B, Chen X, Zhao X, Jiang J, Wei J (2018) Real-time UAV autonomous localization based on smartphone sensors. Sensors 18(12):1–17
Astudillo A, Muñoz P, Álvarez F, Rosero E (2017) Altitude and attitude cascade controller for a smartphone-based quadcopter. In: 2017 International Conference on Unmanned Aircraft Systems (ICUAS), Miami, FL, USA. IEEE, pp 1447–1454
Hayajneh M, Melega M, Marconi L (2018) Design of autonomous smartphone based quadrotor and implementation of navigation and guidance systems. Mechatronics 49:119–133
Carlson DF, Rysgaard S (2018) Adapting open-source drone autopilots for real-time iceberg observations. MethodsX 5:1059–1072
Roy SK, Devaraj R, Sarkar A, Maji K, Sinha S (2020) Contention-aware optimal scheduling of real-time precedence-constrained task graphs on heterogeneous distributed systems. J Syst Architect 105:1–14
Tobita T, Kasahara H (2002) A standard task graph set for fair evaluation of multiprocessor scheduling algorithms. J Sched 5(5):379–394
MathSciNet MATH Google Scholar
Singh A, Ekberg P, Baruah S (2019) Uniprocessor scheduling of real-time synchronous dataflow tasks. Real-Time Syst 55(1):1–31
Panda SK, Jana PK (2015) Efficient task scheduling algorithms for heterogeneous multi-cloud environment. J Supercomput 71(4):1505–1533
Albers S (2009) Algorithms for energy saving. In: Albers S, Alt H, Näher S (eds) Efficient algorithms: essays dedicated to Kurt Mehlhorn on the occasion of his 60th birthday. Springer, Berlin, pp 173–186
Gerards MET, Hurink JL, Hölzenspies PKF (2016) A survey of offline algorithms for energy minimization under deadline constraints. J Sched 19(1):3–19. https://doi.org/10.1007/s10951-015-0463-8
Article MathSciNet MATH Google Scholar
Chen H, Zhu J, Zhang Z, Ma M, Shen X (2017) Real-time workflows oriented online scheduling in uncertain cloud environment. J Supercomput 73(11):4906–4922
Li H, Li C, Li H, Li Y, Xing Z (2017) An integrated altitude control design for a tail-sitter UAV equipped with turbine engines. IEEE Access 5:10941–10952
Zeng Y, Xu X, Zhang R (2018) Trajectory design for completion time minimization in UAV-enabled multicasting. IEEE Trans Wirel Commun 17(4):2233–2246
Labbadi M, Cherkaoui M (2019) Robust adaptive backstepping fast terminal sliding mode controller for uncertain quadrotor UAV. Aerosp Sci Technol 93:105306
Muliadi J, Kusumoputro B (2018) Neural network control system of UAV altitude dynamics and its comparison with the PID control system. J Adv Transp 3823201
Koch W, Mancuso R, West R, Bestavros A (2019) Reinforcement learning for UAV attitude control. ACM Trans Cyber-Phys Syst 3(2):1–21
Wang W, Ma H, Xia M, Weng L, Ye X (2013) Attitude and altitude controller design for quad-rotor type MAVs. Math Probl Eng 1–10
Koo S, Kim S, Suk J, Kim Y, Shin J (2018) Improvement of shipboard landing performance of fixed-wing UAV using model predictive control. Int J Control Autom Syst 16(6):2697–2708
Jing C, Xu H, Niu X, Song X (2019) Adaptive nonsingular terminal sliding mode control for attitude tracking of spacecraft with actuator faults. IEEE Access 7:31485–31493
Chen F, Jiang R, Zhang K, Jiang B, Tao G (2016) Robust backstepping sliding-mode control and observer-based fault estimation for a quadrotor UAV. IEEE Trans Ind Electron 63(8):5044–5056
Alsmadi YM, Utkin V, Haj-ahmed MA, Xu L (2018) Sliding mode control of power converters: DC/DC converters. Int J Control 91(11):2472–2493
Mallavalli S, Fekih A (2018) An SMC-based fault tolerant control design for a class of underactuated unmanned aerial vehicles. In: 2018 4th International Conference on Control, Automation and Robotics (ICCAR), Auckland, New Zealand. IEEE, pp 152–155
Mo H, Farid G (2019) Nonlinear and adaptive intelligent control techniques for quadrotor UAV—a survey. Asian J Control 21(2):989–1008
Cajo R, Mac TT, Plaza D, Copot C, De Keyser R, Ionescu C (2019) A survey on fractional order control techniques for unmanned aerial and ground vehicles. IEEE Access 7:66864–66878
Amin R, Aijun L, Shamshirband S (2016) A review of quadrotor UAV: control methodologies and performance evaluation. Int J Autom Control 10(2):87–103
Atencia CR, Del Ser J, Camacho D (2019) Weighted strategies to guide a multi-objective evolutionary algorithm for multi-UAV mission planning. Swarm Evolut Comput 44:480–495
Ramirez-Atencia C, Bello-Orgaz G, R-Moreno MD, Camacho D (2017) Solving complex multi-UAV mission planning problems using multi-objective genetic algorithms. Soft Comput 21(17):4883–4900
Li F, Zlatanova S, Koopman M, Bai X, Diakité A (2018) A universal path planning for an indoor drone. Autom Constr 95:275–283. https://doi.org/10.1016/j.autcon.2018.07.025
Chen Y, Luo G, Mei Y, Yu J, Su X (2016) UAV path planning using artificial potential field method updated by optimal control theory. Int J Syst Sci 47(6):1407–1420
Lin Y, Saripalli S (2017) Sampling-based path planning for UAV collision avoidance. IEEE Trans Intell Transp Syst 18(11):3179–3192
Li J, Deng G, Luo C, Lin Q, Yan Q, Ming Z (2016) A hybrid path planning method in unmanned air/ground vehicle (UAV/UGV) cooperative systems. IEEE Trans Veh Technol 65(12):9585–9596
Chen Y, Yu J, Mei Y, Wang Y, Su X (2016) Modified central force optimization (MCFO) algorithm for 3D UAV path planning. Neurocomputing 171:878–888
Silva Arantes JD, Silva Arantes MD, Motta-Toledo CF, Júnior OT, Williams BC (2017) Heuristic and genetic algorithm approaches for UAV path planning under critical situation. Int J Artif Intell Tools 26(1):1760008
Yang Q, Yoo SJ (2018) Optimal UAV path planning: sensing data acquisition over IoT sensor networks using multi-objective bio-inspired algorithms. IEEE Access 6:13671–13684
Shiri, H, Park J, Bennis M (2019) Massive autonomous UAV path planning: a neural network based mean-field game theoretic approach. In: 2019 IEEE Global Communications Conference (GLOBECOM), Waikoloa, Hawaii. IEEE, pp 1–6
Musaddiq A, Zikria YB, Hahm O, Yu H, Bashir AK, Kim SW (2018) A survey on resource management in IoT operating systems. IEEE Access 6:8459–8482
Zagan I, Găitan VG (2019) Hardware RTOS: custom scheduler implementation based on multiple pipeline registers and MIPS32 architecture. Electronics 8(2):211
Chandane MP (2016) Real time operating systems: a complete overview. Int J Electr Electron Eng (IJEEE) [e-journal] 8(1):361–365
Li Y, Matsubara Y, Takada H (2018) A comparative analysis of RTOS and linux scalability on an embedded many-core processor. J Inf Process 26:225–236
Zaykov PG, Kuzmanov G, Molnos A, Goossens K (2016) RTOS acceleration in an MPSoC with reconfigurable hardware. Comput Electr Eng 53:89–105
Dietrich C, Lohmann D (2017) OSEK-V: application-specific RTOS instantiation in hardware. ACM SIGPLAN Notices 52(5):111–120
Bonarini A, Matteucci M, Migliavacca M, Rizzi D (2014) R2p: an open source hardware and software modular approach to robot prototyping. Robot Auton Syst 62(7):1073–1084
Sababha B, Yang HC, Rawashdeh O (2010) An RTOS-based run-time reconfigurable avionics system for UAVs, Atlanta, Georgia. In: AIAA Infotech@ Aerospace 2010, p 3414
Khosiawan Y, Park Y, Moon I, Nilakantan JM, Nielsen I (2019) Task scheduling system for UAV operations in indoor environment. Neural Comput Appl 31(9):5431–5459
Alvear O, Zema NR, Natalizio E, Calafate CT (2017) Using UAV-based systems to monitor air pollution in areas with poor accessibility. J Adv Transp 2017:1–14
Zheng Z, Guanping X (2019) Evolution analysis of a UAV real-time operating system from a network perspective. Chin J Aeronaut 32(1):176–185
Palossi D, Singh J, Magno M, Benini L (2017) Target following on nano-scale unmanned aerial vehicles. In: 2017 7th IEEE International Workshop on Advances in Sensors and Interfaces (IWASI), Vieste, Italy. IEEE, pp 170–175
Bulka E, Nahon M (2019) Automatic control for aerobatic maneuvering of agile fixed-wing UAVs. J Intell Robot Syst 93(1–2):85–100
Artale V, Collotta M, Milazzo C, Pau G, Ricciardello A (2016) An integrated system for UAV control using a neural network implemented in a prototyping board. J Intell Robot Syst 84(1–4):5–19
Dong M, Chen BM, Cai G, Peng K (2007) Development of a real-time onboard and ground station software system for a UAV helicopter. J Aerosp Comput Inf Commun 4(8):933–955
Sherstjuk V, Zharikova M, Sokol I (2018) Forest fire-fighting monitoring system based on UAV team and remote sensing. In: 2018 IEEE 38th International Conference on Electronics and Nanotechnology (ELNANO), National Technical University of Ukraine, Kyiv. IEEE, pp 663–668
Download references
Acknowledgements
The authors would like to acknowledge the funding support on this work from the Botswana International University of Science and Technology (BIUST) Drones Project with project number P00015. The authors would also like to thank Boyce Segweni for his help in the preparation of this manuscript.
Author information
Authors and affiliations.
BIUST, Palapye, Botswana
Vemema Kangunde, Rodrigo S. Jamisola Jr. & Emmanuel K. Theophilus
You can also search for this author in PubMed Google Scholar
Corresponding author
Correspondence to Vemema Kangunde .
Ethics declarations
Conflict of interest.
All authors declares that they have no conflict of interest.
Rights and permissions
Open Access This article is licensed under a Creative Commons Attribution 4.0 International License, which permits use, sharing, adaptation, distribution and reproduction in any medium or format, as long as you give appropriate credit to the original author(s) and the source, provide a link to the Creative Commons licence, and indicate if changes were made. The images or other third party material in this article are included in the article’s Creative Commons licence, unless indicated otherwise in a credit line to the material. If material is not included in the article’s Creative Commons licence and your intended use is not permitted by statutory regulation or exceeds the permitted use, you will need to obtain permission directly from the copyright holder. To view a copy of this licence, visit http://creativecommons.org/licenses/by/4.0/ .
Reprints and permissions
About this article
Kangunde, V., Jamisola, R.S. & Theophilus, E.K. A review on drones controlled in real-time. Int. J. Dynam. Control 9 , 1832–1846 (2021). https://doi.org/10.1007/s40435-020-00737-5
Download citation
Received : 16 October 2020
Revised : 11 November 2020
Accepted : 19 November 2020
Published : 05 January 2021
Issue Date : December 2021
DOI : https://doi.org/10.1007/s40435-020-00737-5
Share this article
Anyone you share the following link with will be able to read this content:
Sorry, a shareable link is not currently available for this article.
Provided by the Springer Nature SharedIt content-sharing initiative
- Unmanned areal vehicles
- Real-time control
- Real-time operating system
- Global positioning system
- Inertial measurement unit
Advertisement
- Find a journal
- Publish with us
- Track your research
Essays service custom writing company - The key to success
Quality is the most important aspect in our work! 96% Return clients; 4,8 out of 5 average quality score; strong quality assurance - double order checking and plagiarism checking.
Finished Papers
Customer Reviews
Our writers always follow the customers' requirements very carefully
Frequently Asked Questions
Why choose us.

Earl M. Kinkade
Can I pay someone to write my essay?
Time does not stand still and the service is being modernized at an incredible speed. Now the customer can delegate any service and it will be carried out in the best possible way.
Writing essays, abstracts and scientific papers also falls into this category and can be done by another person. In order to use this service, the client needs to ask the professor about the topic of the text, special design preferences, fonts and keywords. Then the person contacts the essay writing site, where the managers tell him about the details of cooperation. You agree on a certain amount that you are ready to give for the work of a professional writer.
A big bonus of such companies is that you don't have to pay money when ordering. You first receive a ready-made version of the essay, check it for errors, plagiarism and the accuracy of the information, and only then transfer funds to a bank card. This allows users not to worry about the site not fulfilling the agreements.
Go to the website and choose the option you need to get the ideal job, and in the future, the best mark and teacher's admiration.
Experts to Provide You Writing Essays Service.
You can assign your order to:
- Basic writer. In this case, your paper will be completed by a standard author. It does not mean that your paper will be of poor quality. Before hiring each writer, we assess their writing skills, knowledge of the subjects, and referencing styles. Furthermore, no extra cost is required for hiring a basic writer.
- Advanced writer. If you choose this option, your order will be assigned to a proficient writer with a high satisfaction rate.
- TOP writer. If you want your order to be completed by one of the best writers from our essay writing service with superb feedback, choose this option.
- Your preferred writer. You can indicate a specific writer's ID if you have already received a paper from him/her and are satisfied with it. Also, our clients choose this option when they have a series of assignments and want every copy to be completed in one style.
Customer Reviews
Finished Papers
Megan Sharp
Finished Papers
Essay Writing Service
Have a native essay writer do your task from scratch for a student-friendly price of just per page. Free edits and originality reports.

Team of Essay Writers
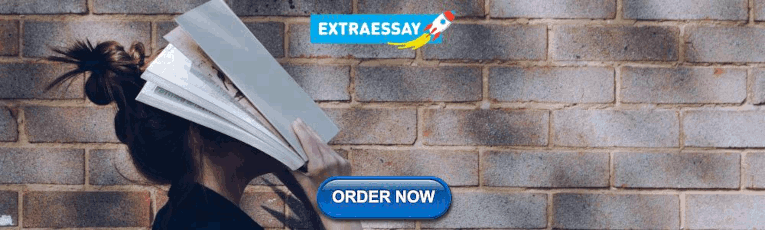
IMAGES
VIDEO
COMMENTS
Abstract. The remote control is a device normally used in conjunction with the TV-set, being this the preferred via of interaction. However, users like older adults frequently have difficulties using this device, due to the decrease in fine motor skills such as coordination of movements, grabbing objects, and also to memory and visual impairments.
The remote control is a device normally used in conjunction with the. TV -set, being this the preferred via of interaction. However, users like older adults. frequently have difficulties using ...
This extended search was carried on to ensure that the literature review did not leave out relevant works dealing with control-related issues in RWAs. ... Figure 2 provides a graphical representation of key findings related to the control of remote workers according to the proposed conceptualization of control reported in Figure 1. FIGURE 2 ...
We review the literature on remote work to explain how these apparently contradictory effects interact. We show that the joint effect of CMI and CMS goes beyond changing the amount of control over employees. Instead, this joint effect requires managers to ensure the accessibility necessary for control of remote work: that employees make their ...
In this paper, a systematic literature review of various scientific research papers have been conducted regarding the mentioned phenomenon by using the keywords; "Remote Work", "Work from Home", "Stress", and "Mental Health" in three different databases; Scopus, Web of Science, and Google Scholar. The analysis shown in the ...
The results of a quick literature review were summarised below to help better understand remote appliance technology. To run home appliances, Narendra Reddy and Sukumar [] use an ARM board and a universal remote controller to develop a circuit for the Internet of Things (IoT), Wi-Fi and bluetooth (BLE).By utilising the concept of multiple data fusion, the circuit is built with decreased ...
In conducting the literature review, since we assumed a broad perspective on organizational control we did not limit our investigation to traditional control mechanisms (e.g. behavior) but also ...
We review the literature on remote work to explain how these apparently contradictory effects interact. We show that the joint effect of CMI and CMS goes beyond changing the amount of control over ...
This systematic literature review (SLR) informed this task force. Herein, we summarise available data on efficacy, safety, cost-effectiveness, satisfaction, adherence and the potential barriers and drivers of remote care for patients with RMDs. ... Remote care was superior to the control group in seven studies with respect to pain, 29 31 33 36 ...
We present a systematic literature review on the security and privacy harms of smart homes. PRISMA methodology is used to systematically review 63 studies published between January 2011 and October 2021; and a review of known cases is undertaken to illustrate the literature review findings with real-world scenarios. ... remote baby or pet ...
The conducting stage of the review involved the systematic search, based on relevant search terms. The electronic database Scopus was selected as it represents the largest database of citations and abstracts of the research literature and provided a wide coverage of the review topic (Bar-Ilan, 2008).The key word selection revolved around the term "smart home".
Search Strategy. The review covered an approximately 11-year time period (April 2004-May 2015) that corresponds to the time-period covered in other reviews by our group on use of financial incentives for treating substance use disorders where we noted the growth in use of remotely-implemented CM interventions (Davis et al., 2015; Higgins, Sigmon, & Heil, 2011).
The rest of the article is organized as follows: section "Literature review" reviews the present literature on the underwater vehicles. Section "State of the art" offers the state of the art research on ROUV. ... Simetti E, De Palma D, et al. Advanced ROV autonomy for efficient remote control in the DexROV project. Mar Technol Soc J ...
comprehensive understanding of how organizational control is implied in remote work arrangements (RWAs) is still lacking. To fill this gap, a research synthesis— that is, a systematic review of 131 studies that empirically investigated this issue— is carried on. The analysis is not limited to direct forms of control (e.g., output
Microcontrollers can be found in implantable medical devices, process control systems, automobile engine control systems, remote control systems, industrial instrumentation devices, voltmeter, office equipment, electronic appliances, power tools, and toys [4]. Most of these devices and products are automatically controlled, have dedicated input ...
Moreover, a lot of home automation protocols, communication and interface standards. 3. LITERATURE REVIEW. In this section, discussed different Home Automation System with their technology with features, benefit and limitations they have. "The Figure 2" shows Basic Architecture of Remote Home Automation. Device, Things or Machine.
"Zenith Space Command" then the remote was produced and it was the first practical wireless remote control system. Ahmed et al. and team designed the first remote control of the fan regulator using analogy and digital component but it proved to be a controlling technique for aparticular function. Nhivekar et al. [2] carry forwarded the work ...
The devices such as remote control and GSM phone could provide means for monitoring and controlling home appliances in a more convenient way. This project has explored the concept of home automation and ZigBee technology. ... March 2017 Literature Review on Home Automation System Neha Malik1, Yogita Bodwade1 Government College of Engineering ...
This paper presents related literature review on drones or unmanned aerial vehicles that are controlled in real-time. Systems in real-time control create more deterministic response such that tasks are guaranteed to be completed within a specified time. This system characteristic is very much desirable for drones that are now required to perform more sophisticated tasks. The reviewed materials ...
Literature Review - Free download as Word Doc (.doc / .docx), PDF File (.pdf), Text File (.txt) or read online for free. this is literature reviww of my final year project which was based on facial recoginition
Literature Review On Remote Control. The shortest time frame in which our writers can complete your order is 6 hours. Length and the complexity of your "write my essay" order are determining factors. If you have a lengthy task, place your order in advance + you get a discount!
Literature Review On Remote Control. You are going to request writer Estevan Chikelu to work on your order. We will notify the writer and ask them to check your order details at their earliest convenience. The writer might be currently busy with other orders, but if they are available, they will offer their bid for your job.
Literature Review On Remote Control - Gustavo Almeida Correia #27 in Global Rating Nursing Business and Economics Psychology Management +86. BA/MA/MBA/PhD writers. A writer who is an expert in the respective field of study will be assigned. REVIEWS HIRE. Literature Review On Remote Control: Essay, Discussion Board Post, Coursework, Research ...