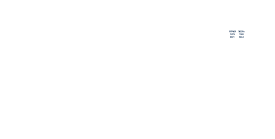
Popular pages
- Mental Health
- Artificial Intelligence
- Plastic Pollution
- COVID-19 Data Explorer
- CO₂ & Greenhouse Gas Emissions
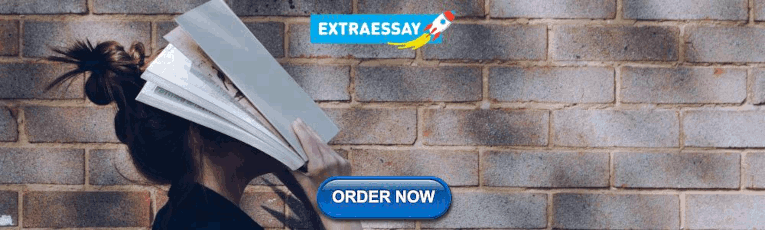
Research and data to make progress against the world’s largest problems.
12,872 charts across 115 topics All free: open access and open source
Our Mission
What do we need to know to make the world a better place?
To make progress against the pressing problems the world faces, we need to be informed by the best research and data.
Our World in Data makes this knowledge accessible and understandable, to empower those working to build a better world.
We are a non-profit — all our work is free to use and open source. Consider supporting us if you find our work valuable.
Featured work
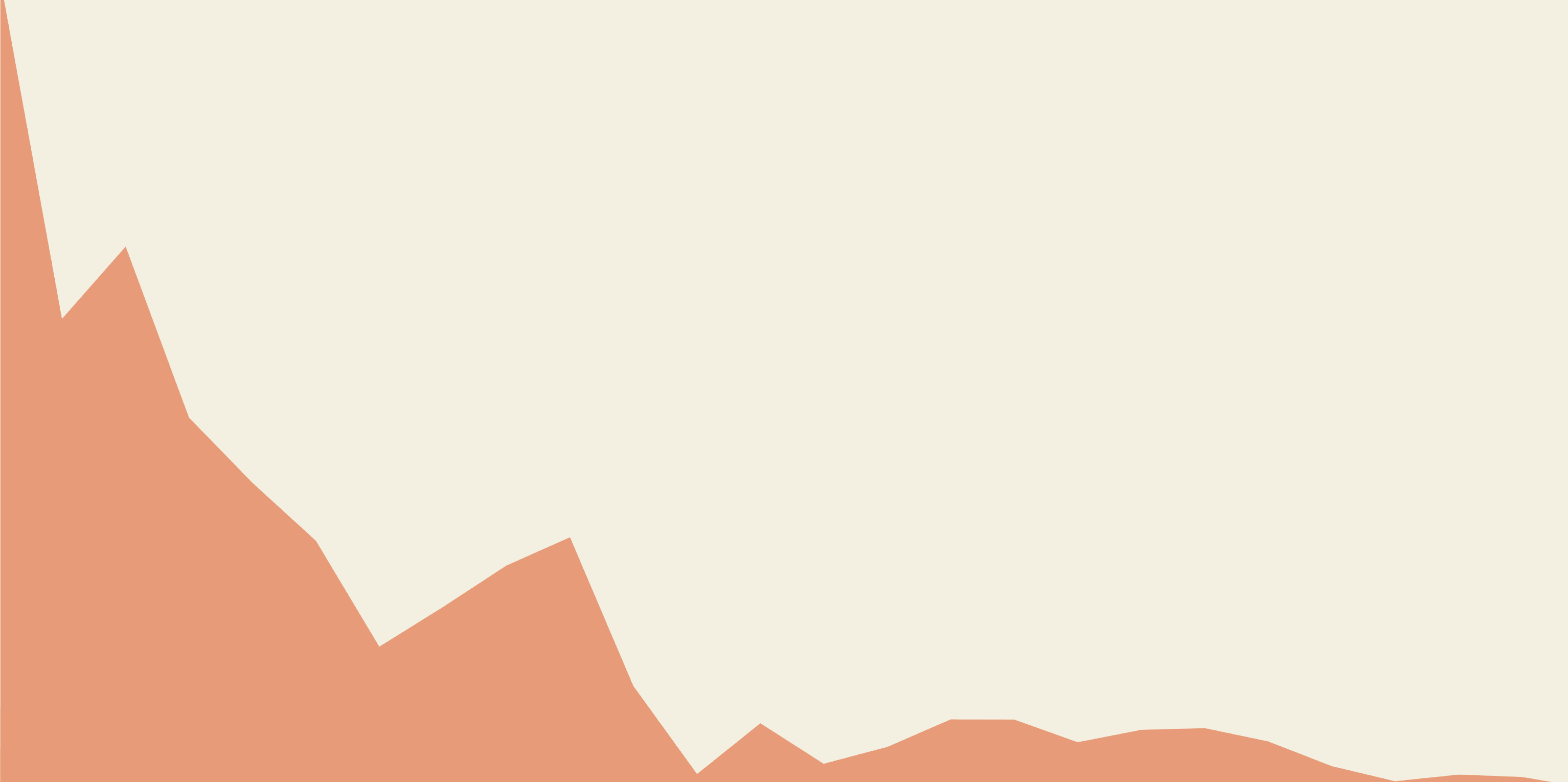
New polio vaccines are key to preventing outbreaks and achieving eradication
To reach the goal of polio eradication, we can use new vaccines to contain outbreaks and improve testing, outbreak responses, and sanitation.
Saloni Dattani
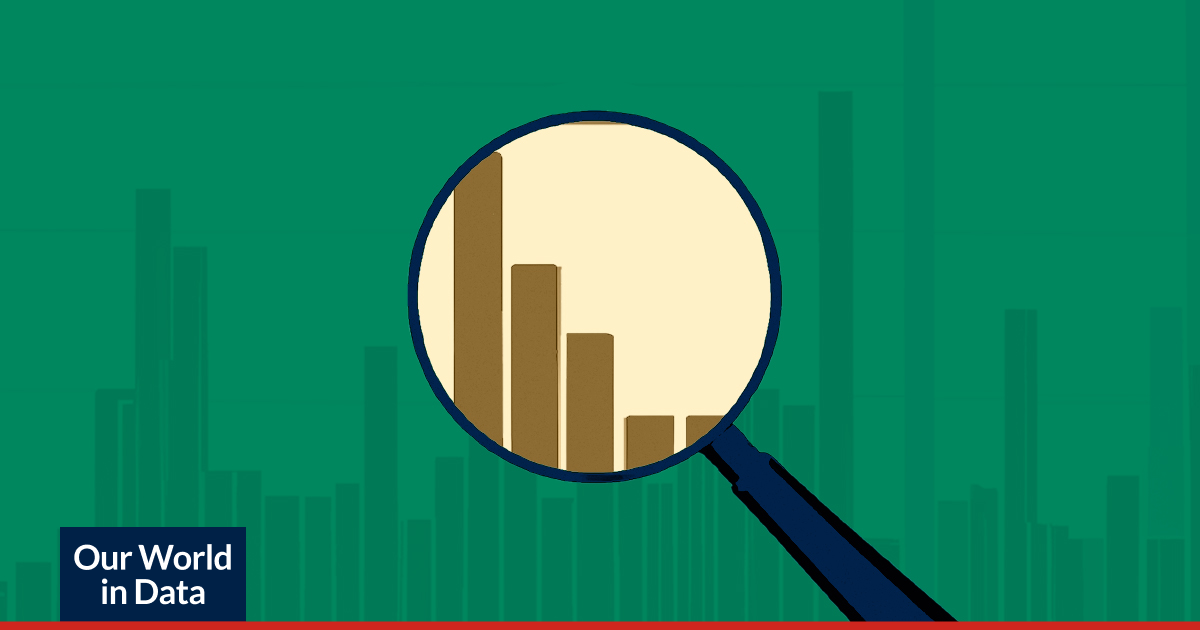
Browse our Data Insights
Bite-sized insights on how the world is changing, written by our team.
Our World in Data team
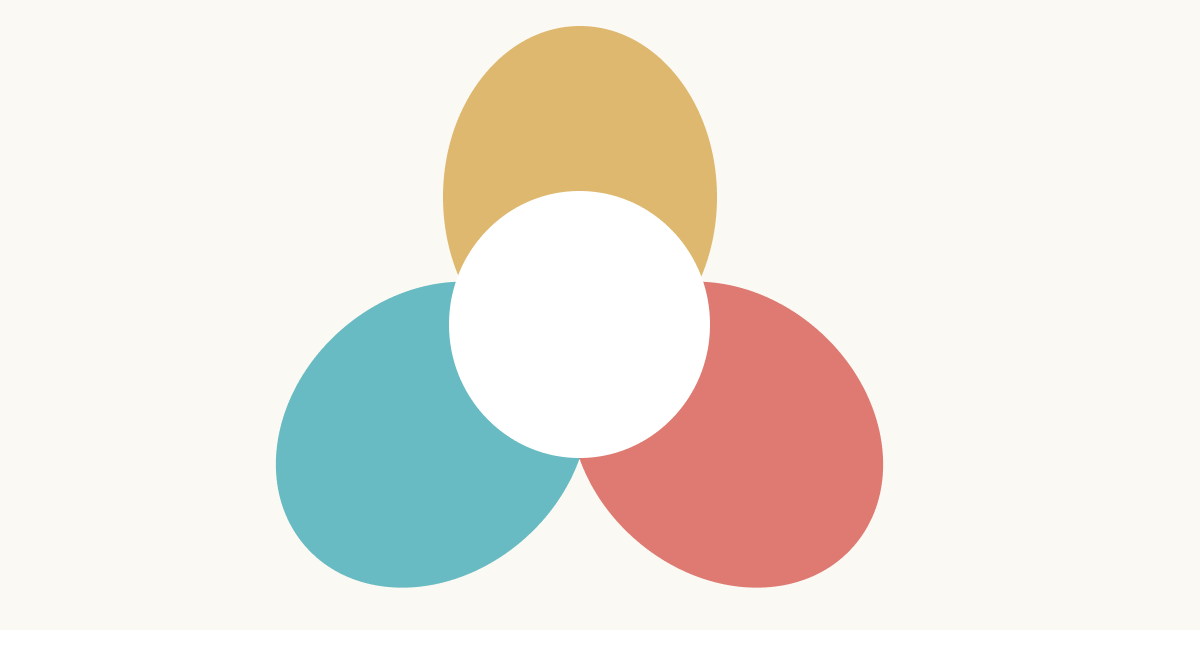
The world has become more resilient to disasters, but investment is needed to save more lives
Deaths from disasters have fallen, but we need to build even more resilience to ensure this progress doesn’t reverse.
Hannah Ritchie
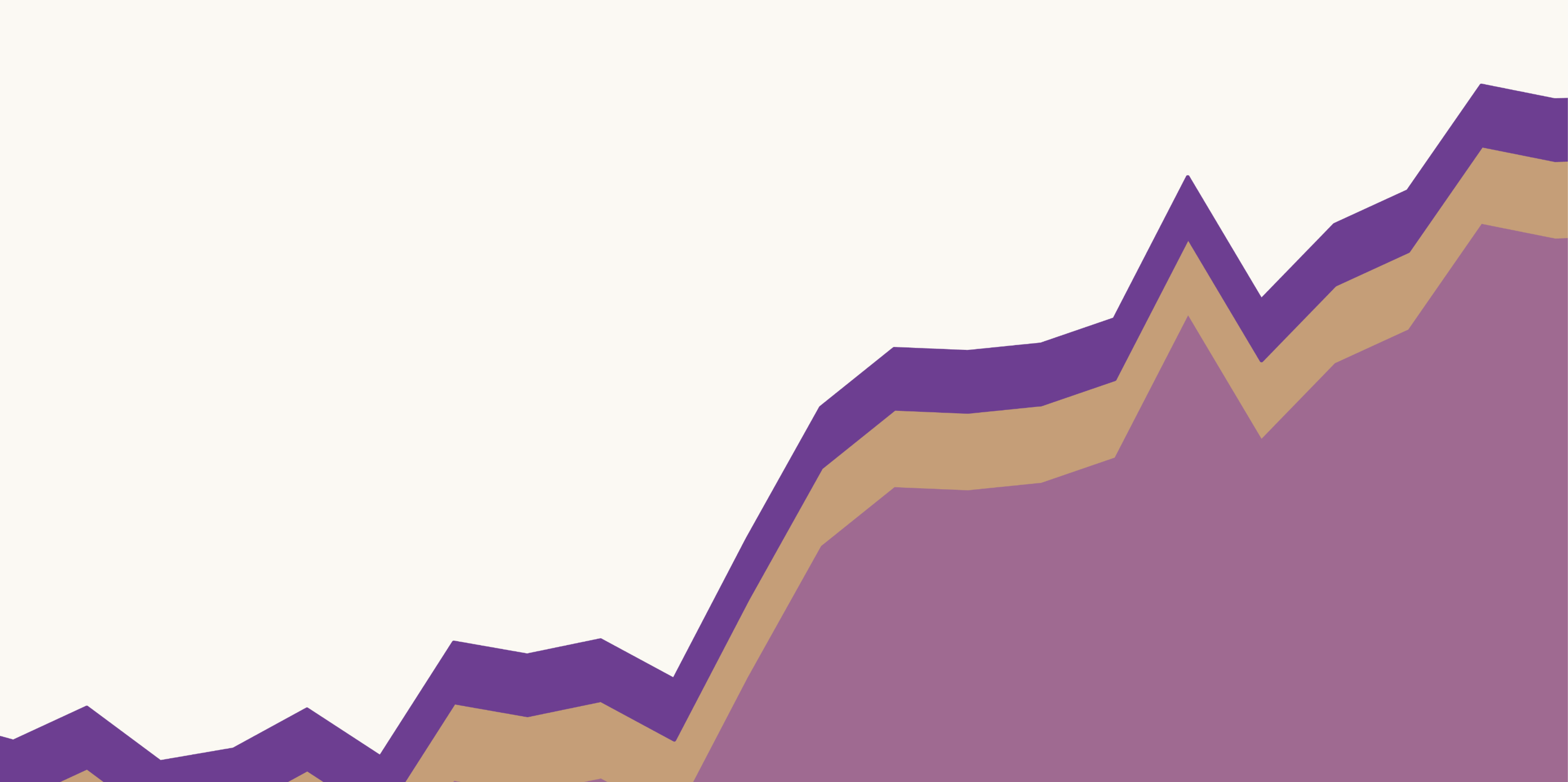
The rise in reported maternal mortality rates in the US is largely due to a change in measurement
Maternal mortality rates appear to have risen in the last 20 years in the US. But this reflects a change in measurement rather than an actual rise in mortality.
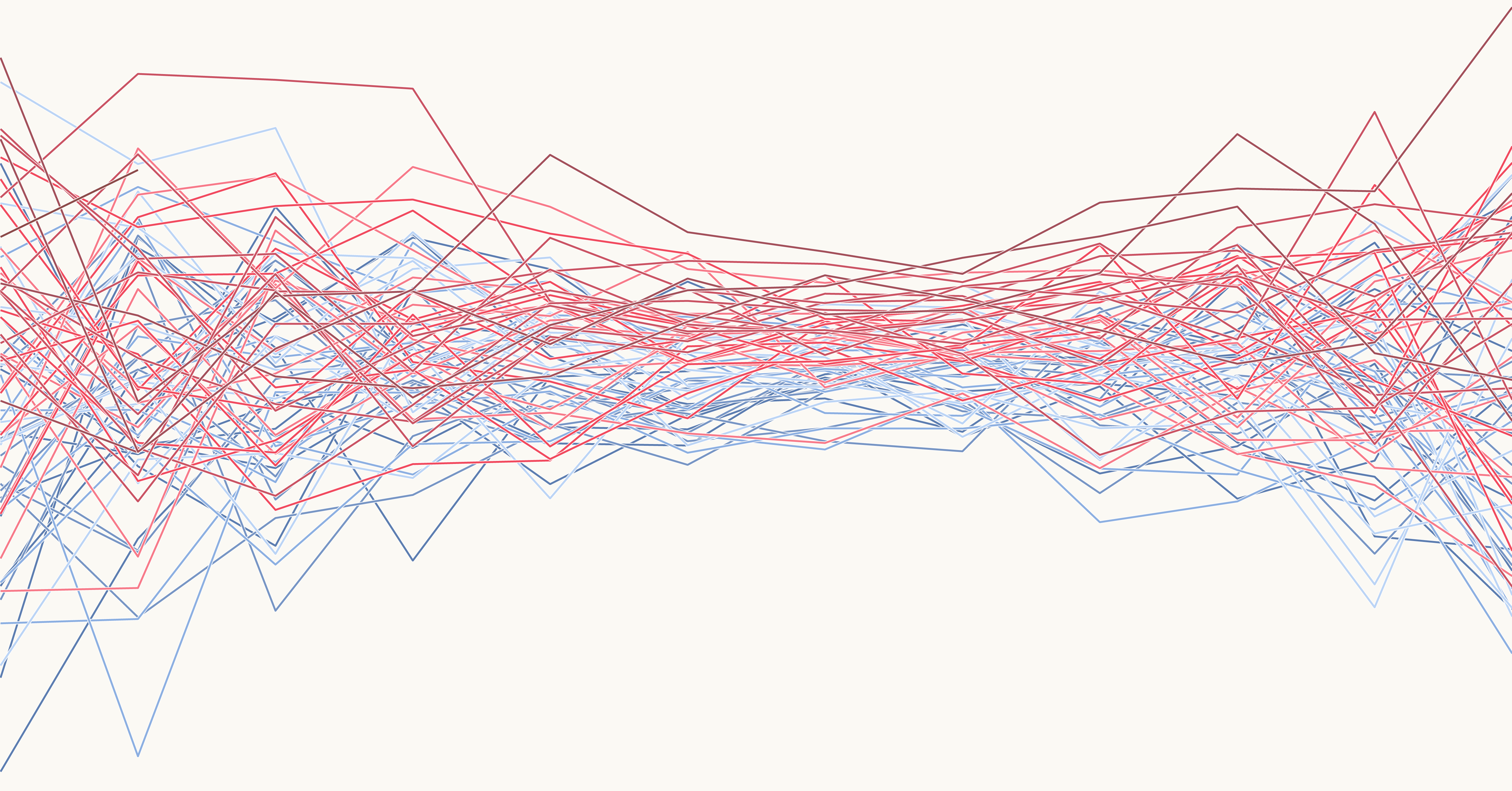
How much have temperatures risen in countries across the world?
Explore country-by-country data on monthly temperature anomalies.
Veronika Samborska
Latest Data Insights
Bite-sized insights on how the world is changing.
May 27, 2024
One in five democracies is eroding
May 24, 2024
There are huge inequalities in global CO2 emissions
May 23, 2024
In less than a decade, Peru has become the world's second-largest blueberry producer
May 22, 2024
Much more progress can be made against child mortality
Explore our data
Featured data from our collection of more than 12,800 interactive charts.
Under-five mortality rate Long-run estimates combining data from UN & Gapminder
What share of children die before their fifth birthday.
What could be more tragic than the death of a young child? Child mortality, the death of children under the age of five, is still extremely common in our world today.
The historical data makes clear that it doesn’t have to be this way: it is possible for societies to protect their children and reduce child mortality to very low rates. For child mortality to reach low levels, many things have to go right at the same time: good healthcare , good nutrition , clean water and sanitation , maternal health , and high living standards . We can, therefore, think of child mortality as a proxy indicator of a country’s living conditions.
The chart shows our long-run data on child mortality, which allows you to see how child mortality has changed in countries around the world.
Share of population living in extreme poverty World Bank
What share of the population is living in extreme poverty.
The UN sets the “International Poverty Line” as a worldwide comparable definition for extreme poverty. Living in extreme poverty is currently defined as living on less than $2.15 per day. This indicator, published by the World Bank, has successfully drawn attention to the terrible depths of poverty of the poorest people in the world.
Two centuries ago, the majority of the world’s population was extremely poor. Back then, it was widely believed that widespread poverty was inevitable. This turned out to be wrong. Economic growth is possible and makes it possible for entire societies to leave the deep poverty of the past behind. Whether or not countries are leaving the worst poverty behind can be monitored by relying on this indicator.
Life expectancy at birth Long-run estimates collated from multiple sources by Our World in Data
How has people’s life expectancy changed over time.
Across the world, people are living longer. In 1900, the global average life expectancy of a newborn was 32 years. By 2021, this had more than doubled to 71 years.
Big improvements were achieved by countries around the world . The chart shows that life expectancy has more than doubled in every region of the world. This improvement is not only due to declining child mortality; life expectancy increased at all ages .
This visualization shows long-run estimates of life expectancy brought together by our team from several different data sources. It also shows that the COVID-19 pandemic led to reduced life expectancy worldwide.
Per capita CO₂ emissions Long-run estimates from the Global Carbon Budget
How have co₂ emissions per capita changed.
The main source of carbon dioxide (CO 2 ) emissions is the burning of fossil fuels. It is the primary greenhouse gas causing climate change .
Globally, CO 2 emissions have remained at just below 5 tonnes per person for over a decade. Between countries, however, there are large differences, and while emissions are rapidly increasing in some countries, they are rapidly falling in others.
The source for this CO 2 data is the Global Carbon Budget, a dataset we update yearly as soon as it is published. In addition to these production-based emissions, they publish consumption-based emissions for the last three decades, which can be viewed in our Greenhouse Gas Emissions Data Explorer .
GDP per capita Long-run estimates from the Maddison Project Database
How do average incomes compare between countries around the world.
GDP per capita is a very comprehensive measure of people’s average income . This indicator reveals how large the inequality between people in different countries is. In the poorest countries, people live on less than $1,000 per year, while in rich countries, the average income is more than 50 times higher.
The data shown is sourced from the Maddison Project Database. Drawing together the careful work of hundreds of economic historians, the particular value of this data lies in the historical coverage it provides. This data makes clear that the vast majority of people in all countries were poor in the past. It allows us to understand when and how the economic growth that made it possible to leave the deep poverty of the past behind was achieved.
Share of people that are undernourished FAO
What share of the population is suffering from hunger.
Hunger has been a severe problem for most of humanity throughout history. Growing enough food to feed one’s family was a constant struggle in daily life. Food shortages, malnutrition, and famines were common around the world.
The UN’s Food and Agriculture Organization publishes global data on undernourishment, defined as not consuming enough calories to maintain a normal, active, healthy life. These minimum requirements vary by a person’s sex, weight, height, and activity levels. This is considered in these national and global estimates.
The world has made much progress in reducing global hunger in recent decades. But we are still far away from an end to hunger, as this indicator shows. Tragically, nearly one in ten people still do not get enough food to eat and in recent years — especially during the pandemic — hunger levels have increased.
Literacy rate Long-run estimates collated from multiple sources by Our World in Data
When has literacy become a widespread skill.
Literacy is a foundational skill. Children need to learn to read so that they can read to learn. When we fail to teach this foundational skill, people have fewer opportunities to lead the rich and interesting lives that a good education offers.
The historical data shows that only a very small share of the population, a tiny elite, was able to read and write. Over the course of the last few generations, literacy levels increased, but it remains an important challenge for our time to provide this foundational skill to all.
At Our World in Data, we investigated the strengths and shortcomings of the available data on literacy. Based on this work, our team brought together the long-run data shown in the chart by combining several different sources, including the World Bank, the CIA Factbook, and a range of research publications.
Share of the population with access to electricity World Bank
Where do people lack access to even the most basic electricity supply.
Light at night makes it possible to get together after sunset; mobile phones allow us to stay in touch with those far away; the refrigeration of food reduces food waste; and household appliances free up time from household chores. Access to electricity improves people’s living conditions in many ways.
The World Bank data on the world map captures whether people have access to the most basic electricity supply — just enough to provide basic lighting and charge a phone or power a radio for 4 hours per day.
It shows that, especially in several African countries, a large share of the population lacks the benefits that basic electricity offers. No radio and no light at night.
Data explorers
Interactive visualization tools to explore a wide range of related indicators.
Data Explorer
Population & Demography
- Global Health
Subscribe to our newsletter
- Research & Writing RSS Feed
- Data Insights RSS Feed
All our topics
All our data, research, and writing — topic by topic.
Population and Demographic Change
- Population Change:
- Population Growth
- Age Structure
- Gender Ratio
- Births and Deaths:
- Life Expectancy
- Child and Infant Mortality
- Fertility Rate
- Geography of the World Population:
- Urbanization
- Health Risks:
- Lead Pollution
- Alcohol Consumption
- Opioids, Cocaine, Cannabis, and Other Illicit Drugs
- Air Pollution
- Outdoor Air Pollution
- Indoor Air Pollution
- Infectious Diseases:
- Coronavirus Pandemic (COVID-19)
- Mpox (monkeypox)
- Diarrheal Diseases
- Tuberculosis
- Health Institutions and Interventions:
- Vaccination
- Healthcare Spending
- Eradication of Diseases
- Life and Death:
- Causes of Death
- Cardiovascular Diseases
- Burden of Disease
- Maternal Mortality
Energy and Environment
- Energy Systems:
- Access to Energy
- Fossil Fuels
- Renewable Energy
- Nuclear Energy
- Waste and Pollution:
- Climate and Air:
- CO₂ and Greenhouse Gas Emissions
- Climate Change
- Ozone Layer
- Clean Water and Sanitation
- Clean Water
- Water Use and Stress
- Environment and Ecosystems:
- Natural Disasters
- Biodiversity
- Environmental Impacts of Food Production
- Animal Welfare
- Forests and Deforestation
Food and Agriculture
- Hunger and Undernourishment
- Food Supply
- Food Prices
- Diet Compositions
- Human Height
- Micronutrient Deficiency
- Food Production:
- Agricultural Production
- Crop Yields
- Meat and Dairy Production
- Farm Size and Productivity
- Agricultural Inputs:
- Fertilizers
- Employment in Agriculture
Poverty and Economic Development
- Public Sector:
- State Capacity
- Government Spending
- Education Spending
- Military Personnel and Spending
- Poverty and Prosperity:
- Economic Inequality
- Economic Growth
- Economic Inequality by Gender
- Child Labor
- Working Hours
- Women’s Employment
- Global Connections:
- Trade and Globalization
Education and Knowledge
- Global Education
- Research and Development
Innovation and Technological Change
- Space Exploration and Satellites
- Technological Change
Living Conditions, Community, and Wellbeing
- Housing and Infrastructure:
- Light at Night
- Homelessness
- Relationships:
- Marriages and Divorces
- Loneliness and Social Connections
- Happiness and Wellbeing:
- Human Development Index (HDI)
- Happiness and Life Satisfaction
Human Rights and Democracy
- Human Rights
- Women’s Rights
- LGBT+ Rights
- Violence Against Children and Children’s Rights
Violence and War
- War and Peace
- Nuclear Weapons
- Biological and Chemical Weapons
Our World in Data is free and accessible for everyone.
Help us do this work by making a donation.
- Data, AI, & Machine Learning
- Managing Technology
- Social Responsibility
- Workplace, Teams, & Culture
- AI & Machine Learning
- Diversity & Inclusion
- Big ideas Research Projects
- Artificial Intelligence and Business Strategy
- Responsible AI
- Future of the Workforce
- Future of Leadership
- All Research Projects
AI in Action
- Most Popular
- The Truth Behind the Nursing Crisis
- Work/23: The Big Shift
- Coaching for the Future-Forward Leader
- Measuring Culture
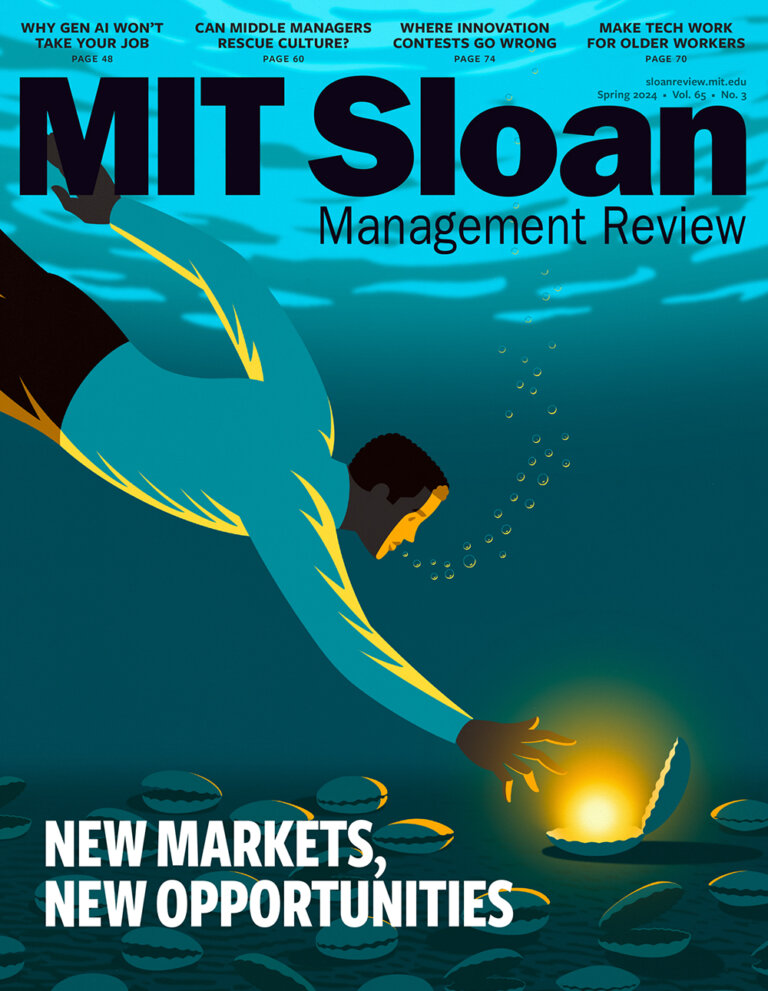
The spring 2024 issue’s special report looks at how to take advantage of market opportunities in the digital space, and provides advice on building culture and friendships at work; maximizing the benefits of LLMs, corporate venture capital initiatives, and innovation contests; and scaling automation and digital health platform.
- Past Issues
- Upcoming Events
- Video Archive
- Me, Myself, and AI
- Three Big Points
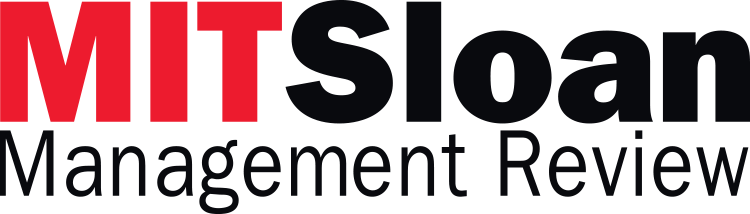
Five Key Trends in AI and Data Science for 2024
These developing issues should be on every leader’s radar screen, data executives say.
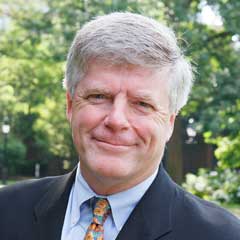
- Data, AI, & Machine Learning
- AI & Machine Learning
- Data & Data Culture
- Technology Implementation
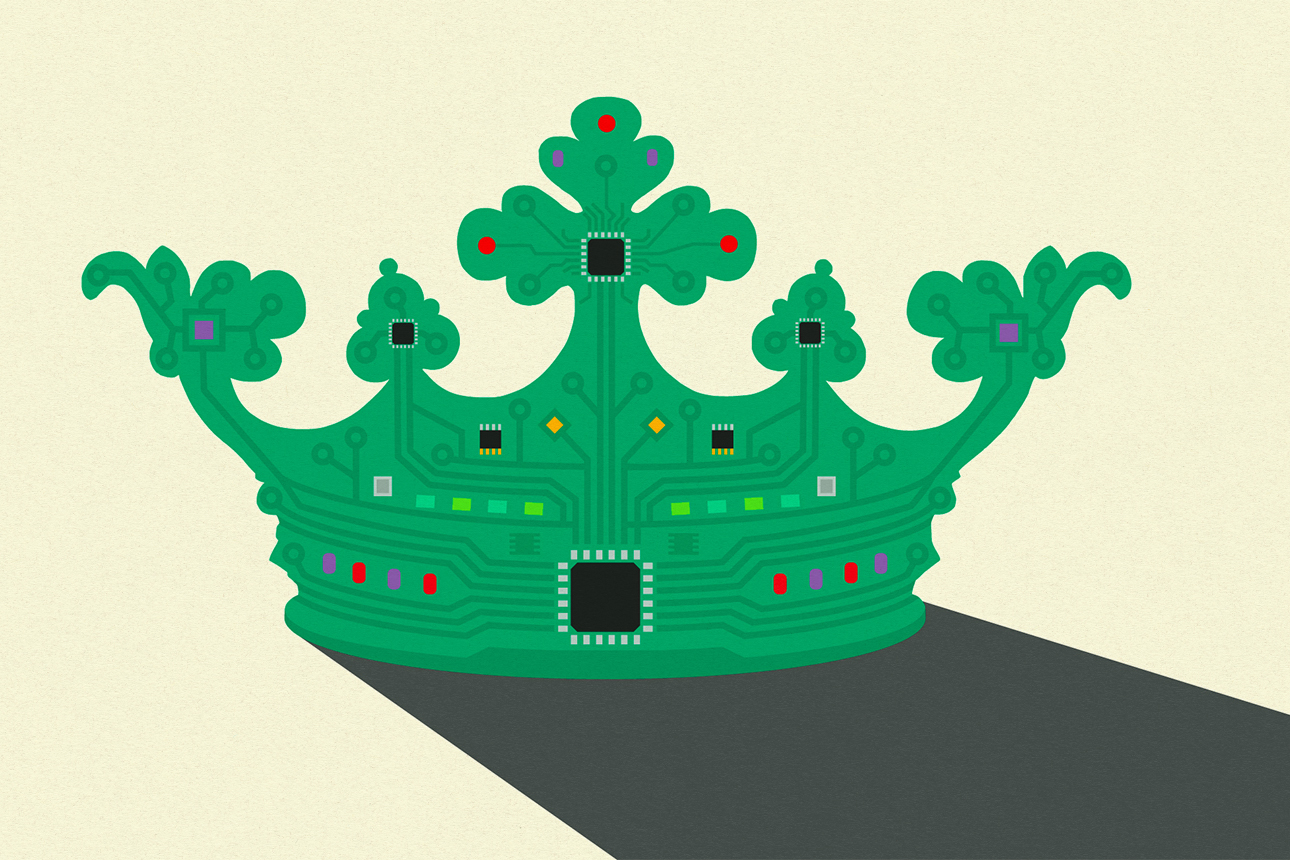
Carolyn Geason-Beissel/MIT SMR | Getty Images
Artificial intelligence and data science became front-page news in 2023. The rise of generative AI, of course, drove this dramatic surge in visibility. So, what might happen in the field in 2024 that will keep it on the front page? And how will these trends really affect businesses?
During the past several months, we’ve conducted three surveys of data and technology executives. Two involved MIT’s Chief Data Officer and Information Quality Symposium attendees — one sponsored by Amazon Web Services (AWS) and another by Thoughtworks . The third survey was conducted by Wavestone , formerly NewVantage Partners, whose annual surveys we’ve written about in the past . In total, the new surveys involved more than 500 senior executives, perhaps with some overlap in participation.
Get Updates on Leading With AI and Data
Get monthly insights on how artificial intelligence impacts your organization and what it means for your company and customers.
Please enter a valid email address
Thank you for signing up
Privacy Policy
Surveys don’t predict the future, but they do suggest what those people closest to companies’ data science and AI strategies and projects are thinking and doing. According to those data executives, here are the top five developing issues that deserve your close attention:
1. Generative AI sparkles but needs to deliver value.
As we noted, generative AI has captured a massive amount of business and consumer attention. But is it really delivering economic value to the organizations that adopt it? The survey results suggest that although excitement about the technology is very high , value has largely not yet been delivered. Large percentages of respondents believe that generative AI has the potential to be transformational; 80% of respondents to the AWS survey said they believe it will transform their organizations, and 64% in the Wavestone survey said it is the most transformational technology in a generation. A large majority of survey takers are also increasing investment in the technology. However, most companies are still just experimenting, either at the individual or departmental level. Only 6% of companies in the AWS survey had any production application of generative AI, and only 5% in the Wavestone survey had any production deployment at scale.
Surveys suggest that though excitement about generative AI is very high, value has largely not yet been delivered.
Production deployments of generative AI will, of course, require more investment and organizational change, not just experiments. Business processes will need to be redesigned, and employees will need to be reskilled (or, probably in only a few cases, replaced by generative AI systems). The new AI capabilities will need to be integrated into the existing technology infrastructure.
Perhaps the most important change will involve data — curating unstructured content, improving data quality, and integrating diverse sources. In the AWS survey, 93% of respondents agreed that data strategy is critical to getting value from generative AI, but 57% had made no changes to their data thus far.
2. Data science is shifting from artisanal to industrial.
Companies feel the need to accelerate the production of data science models . What was once an artisanal activity is becoming more industrialized. Companies are investing in platforms, processes and methodologies, feature stores, machine learning operations (MLOps) systems, and other tools to increase productivity and deployment rates. MLOps systems monitor the status of machine learning models and detect whether they are still predicting accurately. If they’re not, the models might need to be retrained with new data.
Producing data models — once an artisanal activity — is becoming more industrialized.
Most of these capabilities come from external vendors, but some organizations are now developing their own platforms. Although automation (including automated machine learning tools, which we discuss below) is helping to increase productivity and enable broader data science participation, the greatest boon to data science productivity is probably the reuse of existing data sets, features or variables, and even entire models.
3. Two versions of data products will dominate.
In the Thoughtworks survey, 80% of data and technology leaders said that their organizations were using or considering the use of data products and data product management. By data product , we mean packaging data, analytics, and AI in a software product offering, for internal or external customers. It’s managed from conception to deployment (and ongoing improvement) by data product managers. Examples of data products include recommendation systems that guide customers on what products to buy next and pricing optimization systems for sales teams.
But organizations view data products in two different ways. Just under half (48%) of respondents said that they include analytics and AI capabilities in the concept of data products. Some 30% view analytics and AI as separate from data products and presumably reserve that term for reusable data assets alone. Just 16% say they don’t think of analytics and AI in a product context at all.
We have a slight preference for a definition of data products that includes analytics and AI, since that is the way data is made useful. But all that really matters is that an organization is consistent in how it defines and discusses data products. If an organization prefers a combination of “data products” and “analytics and AI products,” that can work well too, and that definition preserves many of the positive aspects of product management. But without clarity on the definition, organizations could become confused about just what product developers are supposed to deliver.
4. Data scientists will become less sexy.
Data scientists, who have been called “ unicorns ” and the holders of the “ sexiest job of the 21st century ” because of their ability to make all aspects of data science projects successful, have seen their star power recede. A number of changes in data science are producing alternative approaches to managing important pieces of the work. One such change is the proliferation of related roles that can address pieces of the data science problem. This expanding set of professionals includes data engineers to wrangle data, machine learning engineers to scale and integrate the models, translators and connectors to work with business stakeholders, and data product managers to oversee the entire initiative.
Another factor reducing the demand for professional data scientists is the rise of citizen data science , wherein quantitatively savvy businesspeople create models or algorithms themselves. These individuals can use AutoML, or automated machine learning tools, to do much of the heavy lifting. Even more helpful to citizens is the modeling capability available in ChatGPT called Advanced Data Analysis . With a very short prompt and an uploaded data set, it can handle virtually every stage of the model creation process and explain its actions.
Of course, there are still many aspects of data science that do require professional data scientists. Developing entirely new algorithms or interpreting how complex models work, for example, are tasks that haven’t gone away. The role will still be necessary but perhaps not as much as it was previously — and without the same degree of power and shimmer.
5. Data, analytics, and AI leaders are becoming less independent.
This past year, we began to notice that increasing numbers of organizations were cutting back on the proliferation of technology and data “chiefs,” including chief data and analytics officers (and sometimes chief AI officers). That CDO/CDAO role, while becoming more common in companies, has long been characterized by short tenures and confusion about the responsibilities. We’re not seeing the functions performed by data and analytics executives go away; rather, they’re increasingly being subsumed within a broader set of technology, data, and digital transformation functions managed by a “supertech leader” who usually reports to the CEO. Titles for this role include chief information officer, chief information and technology officer, and chief digital and technology officer; real-world examples include Sastry Durvasula at TIAA, Sean McCormack at First Group, and Mojgan Lefebvre at Travelers.
Related Articles
This evolution in C-suite roles was a primary focus of the Thoughtworks survey, and 87% of respondents (primarily data leaders but some technology executives as well) agreed that people in their organizations are either completely, to a large degree, or somewhat confused about where to turn for data- and technology-oriented services and issues. Many C-level executives said that collaboration with other tech-oriented leaders within their own organizations is relatively low, and 79% agreed that their organization had been hindered in the past by a lack of collaboration.
We believe that in 2024, we’ll see more of these overarching tech leaders who have all the capabilities to create value from the data and technology professionals reporting to them. They’ll still have to emphasize analytics and AI because that’s how organizations make sense of data and create value with it for employees and customers. Most importantly, these leaders will need to be highly business-oriented, able to debate strategy with their senior management colleagues, and able to translate it into systems and insights that make that strategy a reality.
About the Authors
Thomas H. Davenport ( @tdav ) is the President’s Distinguished Professor of Information Technology and Management at Babson College, a fellow of the MIT Initiative on the Digital Economy, and senior adviser to the Deloitte Chief Data and Analytics Officer Program. He is coauthor of All in on AI: How Smart Companies Win Big With Artificial Intelligence (HBR Press, 2023) and Working With AI: Real Stories of Human-Machine Collaboration (MIT Press, 2022). Randy Bean ( @randybeannvp ) is an industry thought leader, author, founder, and CEO and currently serves as innovation fellow, data strategy, for global consultancy Wavestone. He is the author of Fail Fast, Learn Faster: Lessons in Data-Driven Leadership in an Age of Disruption, Big Data, and AI (Wiley, 2021).
More Like This
Add a comment cancel reply.
You must sign in to post a comment. First time here? Sign up for a free account : Comment on articles and get access to many more articles.
Comment (1)
Nicolas corzo.
Thank you for visiting nature.com. You are using a browser version with limited support for CSS. To obtain the best experience, we recommend you use a more up to date browser (or turn off compatibility mode in Internet Explorer). In the meantime, to ensure continued support, we are displaying the site without styles and JavaScript.
- View all journals
Research data articles from across Nature Portfolio
Research data comprises research observations or findings, such as facts, images, measurements, records and files in various formats, and can be stored in databases. Data publication and archiving is important for the reuse of research data and the reproducibility of scientific research.
Related Subjects
Latest research and reviews.
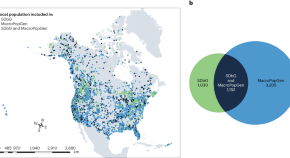
Best practices for genetic and genomic data archiving
This Review discusses challenges and best practices for archiving genetics and genomics data to make them more accessible and FAIR compliant.
- Deborah M. Leigh
- Amy G. Vandergast
- Ivan Paz-Vinas
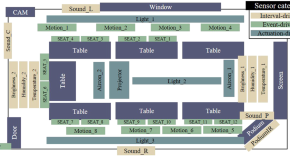
A dataset of ambient sensors in a meeting room for activity recognition
- Dongman Lee
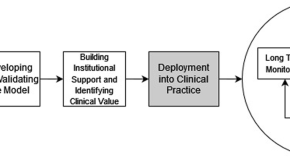
An operational guide to translational clinical machine learning in academic medical centers
- Mukund Poddar
- Jayson S. Marwaha
- Gabriel A. Brat
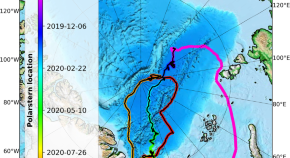
Cloud micro- and macrophysical properties from ground-based remote sensing during the MOSAiC drift experiment
- Hannes J. Griesche
- Patric Seifert
- Andreas Macke
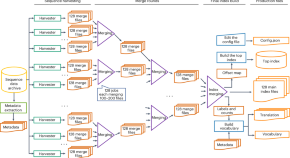
Indexing and searching petabase-scale nucleotide resources
The Pebblescout tool achieves an efficient search for subjects in a large nucleotide database such as runs in Sequence Read Archive data.
- Sergey A. Shiryev
- Richa Agarwala
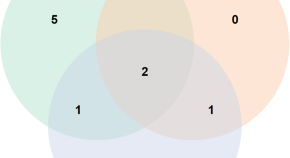
FAIR assessment of nanosafety data reusability with community standards
- Ammar Ammar
- Chris Evelo
- Egon Willighagen
News and Comment
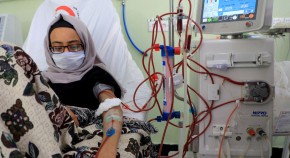
Ozempic keeps wowing: trial data show benefits for kidney disease
Semaglutide, the same compound in obesity drug Wegovy, slashes risk of kidney failure and death for people with diabetes.
- Rachel Fairbank
Standardized metadata for biological samples could unlock the potential of collections
- Vojtěch Brlík
Big data for everyone
- Henrietta Howells
Japan can embrace open science — but flexible approaches are key
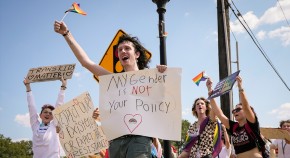
Why it’s essential to study sex and gender, even as tensions rise
Some scholars are reluctant to research sex and gender out of fear that their studies will be misused. In a series of specially commissioned articles, Nature encourages scientists to engage.
Response to “The perpetual motion machine of AI-generated data and the distraction of ChatGPT as a ‘scientist’”
- William Stafford Noble
Quick links
- Explore articles by subject
- Guide to authors
- Editorial policies

- DE (Deutsch)
- Executive Team
- Supervisory Board
- Corporate Governance
- Our Research Division
- Our Education Division
- Our Health Division
- Our Professional Division
- Locations & Contact
- Press Office
- Overview About Us
- Our Communities
- Employee Networks
- Diversity, Equity & Inclusion
- Policies, Reports and Modern Slavery Act
- Overview Taking Responsibility
- Stay Curious
- Why join us
- Develop your curiosity
- Stretch your horizons
- Be yourself
- Life at Springer Nature
- Ways of Working
- Current opportunities
- Editorial & Publishing
- Other teams ↗
- Overview Careers
All Press Releases
The state of open data report 2022: researchers need more support to assist with open data mandates.
New findings provide update on researchers’ attitudes towards open data
London, 13 October 2022
Researchers worldwide will need further assistance to help comply with an increasing number of open data mandates, according to the authors of a new report. The State of Open Data Report 2022 – the latest in an annual collaborative series from Digital Science , Figshare and Springer Nature – is released today. Based on a global survey, the report is now in its seventh year and provides insights into researchers’ attitudes towards and experiences of open data. With more than 5,400 respondents, the 2022 survey is the largest since the COVID-19 pandemic began. This year’s report also includes guest articles from open data experts at the National Institutes of Health (NIH), the White House Office of Science and Technology Policy (OSTP), the Computer Network Information Center of the Chinese Academy of Sciences (CNIC, CAS), publishers and universities. Founder and CEO of Figshare Mark Hahnel says: “This year’s State of Open Data Report comes at a unique point in time when we’re seeing a growing number of open data mandates from funding organizations and policymakers, most notably the NIH and OSTP in the United States, but also recently from the National Health and Medical Research Council (NHMRC) in Australia, and in Europe and the UK.
“What is clear from the findings of our report is that while most researchers embrace the concepts of open data and open science, they also have some reasonable misgivings about how open data policies and practices impact on them. In an environment where open data mandates are increasing, funding organizations would benefit from working even more closely with researchers and providing them with additional support to help smooth the transition to a fully open data future.
“We all have a role to play in driving a better future for open data and accessible research, and one way we can do that through this report is by listening to the voices of researchers, funders, institutions, and publishers." Primary findings from this year’s report indicated that:
- There is a growing trend in researchers being in favour of data being made openly available as common practice (4 out of every five researchers were in agreement with this), supported somewhat by now over 70% of respondents being required to follow a policy on data sharing.
- However, researchers still cite a key need in helping them to share their data as being more training or information on policies for access, sharing and reuse (55%) as well as long-term storage and data management strategies (52%). Credit and recognition were once again a key theme for researchers in sharing their data . Of those who had previously shared data, 66% had received some form of recognition for their efforts – most commonly via full citation in another article (41%) followed by co-authorship on a paper that had used the data.
- Researchers are more inclined to share their research data where it can have an impact on citations (67%) and the visibility of their research (61%) , rather than being motivated by public benefit or journal/publisher mandate (both 56%)
Graham Smith , Open Data Program Manager, Springer Nature, says: “For the past seven years these surveys have helped paint a picture of researcher perspectives on open data. The report shows us not only the progress made but the steps that still need to be taken on the journey towards an open data future in support of the research community. Whether it’s the broad support of researchers for making research data openly available as common practice or the changing attitudes to open data mandates, we must learn from and deliver concrete steps forward to address what the community is telling us.
“Springer Nature is firmly committed to this and we continue to work closely with our partners, such as Figshare and Digital Science, to create better understanding around data sharing.”
Daniel Hook , CEO of Digital Science, says: “Digital Science is committed to making open, collaborative and inclusive research possible, as we believe this environment will lead to the greatest benefit for society. Now in its seventh year, while the articles in The State of Open Data Report represent a unique set of snapshots marking the evolution of attitudes about Open Data in our community, the data behind the survey constitutes a valuable resource to track researcher sentiment regarding open data and their experiences of data sharing. I believe that these data represent an amazing opportunity to understand the challenges and needs of our community so that we can collectively build better infrastructure to support research.” The full report can be accessed on Figshare here .
Notes to Editors
Key findings via theme of the report:
Support for open data
- Four out of every five respondents are in favour of research data being made openly available as common practice.
- 74% of respondents reported sharing their data during publication.
- Approximately one fifth of respondents reported having no concerns about sharing data openly – this proportion has been steadily growing since 2018.
- 88% of researchers surveyed are supportive of making research articles open access (OA) as a common scholarly practice.
Motivations and benefits
- When it comes to researchers sharing their data, citations of research papers (67%) and increased impact and visibility of papers (61%) outweigh public benefit or journal/publisher mandate (both 56%) as motivation.
- Of those who had previously shared data, 66% had received some form of recognition for their efforts – most commonly via full citation in another article (41%) followed by co-authorship on a paper that had used the data.
- A third of respondents indicated they had been involved in a research collaboration as a result of data they had previously shared.
Open data mandates
- 70% of respondents were required to follow a policy on data sharing for their most recent piece of research.
- More than two-thirds of respondents are supportive “to some extent” of a national mandate for making research data openly available. This number has been declining since 2019.
- Just over half (52%) of respondents in the 2022 survey felt that sharing data should be a part of the requirement for awarding research grants. Again, this number has been declining since 2019.
- Only 19% of respondents believe that researchers get sufficient credit for sharing their data, while 75% say they receive too little credit.
- Just under a quarter of respondents indicated that they had previously received support with planning, managing or sharing their research data.
- The greatest concern among respondents is misuse of their data (35%).
- The key needs of researchers which were felt more training or information would improve were better understanding and definitions for policies for access, sharing and reuse (55%) as well as long-term storage and data management strategies (52%) – things that impact both ends of the research cycle.
Key demographics of respondents
- Researchers from China now comprise 11% of all respondents, equal with that of the United States. China and the US are the two countries with the biggest response to the survey, followed by India, Japan, Germany, Italy, UK, Canada, Brazil, France and Spain.
- 31% of respondents were early career researchers (ECRs), while a further 31% classed themselves as senior researchers.
- Most respondents (42%) were from medicine & life sciences; 38% from mathematics, physics and applied sciences; and 17% from humanities and social sciences (an increase of 3%).
- Respondents were broadly categorised as: Open science advocates (32%), Open publishing advocates (26%), Cautiously pro open science (25%), Open science agnostics (11%), and Non-believers of open science (6%).
About Springer Nature
For over 180 years Springer Nature has been advancing discovery by providing the best possible service to the whole research community. We help researchers uncover new ideas, make sure all the research we publish is significant, robust and stands up to objective scrutiny, that it reaches all relevant audiences in the best possible format, and can be discovered, accessed, used, re-used and shared. We support librarians and institutions with innovations in technology and data; and provide quality publishing support to societies.
As a research publisher, Springer Nature is home to trusted brands including Springer, Nature Portfolio, BMC, Palgrave Macmillan and Scientific American. For more information, please visit springernature.com and @SpringerNature
About Figshare
Figshare is a leading provider of out-of-the-box, cloud repository software for research data, papers, theses, teaching materials, conference outputs, and more. Research outputs become more discoverable and impactful with search engine indexing and usage metrics including citations and altmetrics. Figshare provides a proficient platform for all types of research data to be shared and showcased in a FAIR way whilst enabling researchers to receive credit. Visit knowledge.figshare.com and follow @figshare on Twitter.
About Digital Science
Digital Science is a technology company working to make research more efficient. We invest in, nurture and support innovative businesses and technologies that make all parts of the research process more open and effective. Our portfolio includes admired brands including Altmetric, Dimensions, Figshare, ReadCube, Symplectic, IFI CLAIMS, Overleaf, Ripeta and Writefull. We believe that together, we can help researchers make a difference. Visit www.digital-science.com and follow @digitalsci on Twitter.
Sam Sule | Communications | Springer Nature
David Ellis | Digital Science
Simon Linacre | Digital Science
- Communiqués de presse
Stay up to date
Here to foster information exchange with the library community
Connect with us on LinkedIn and stay up to date with news and development.
- Executive team
- Our Research Business
- Our Education Business
- Our Professional Business
- Taking Responsibility
- Policies, Reports & Modern Slavery Act
- Why Work Here?
- Search our vacancies ↗
- Springer Nature
- Nature Portfolio
- Palgrave Macmillan
- Scientific American
- Macmillan Education
- Springer Healthcare
- © 2024 Springer Nature
- General terms and conditions
- Your US State Privacy Rights
- Your Privacy Choices / Manage Cookies
- Accessibility
- Legal notice
- Help us to improve this site, send feedback.
- Contact Tracing
- Pandemic Data Initiative
- Events & News
JHU has stopped collecting data as of
After three years of around-the-clock tracking of COVID-19 data from...
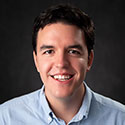
What is the JHU CRC Now?
The Johns Hopkins Coronavirus Resource Center established a new standard for infectious disease tracking by publicly providing pandemic data in near real time. It began Jan. 22, 2020 as the COVID-19 Dashboard , operated by the Center for Systems Science and Engineering and the Applied Physics Laboratory . But the map of red dots quickly evolved into the global go-to hub for monitoring a public health catastrophe. By March 3, 2020, Johns Hopkins expanded the site into a comprehensive collection of raw data and independent expert analysis known as the Coronavirus Resource Center (CRC) – an enterprise that harnessed the world-renowned expertise from across Johns Hopkins University & Medicine.
Why did we shut down?
After three years of 24-7 operations, the CRC is ceasing its data collection efforts due to an increasing number of U.S. states slowing their reporting cadences. In addition, the federal government has improved its pandemic data tracking enough to warrant the CRC’s exit. From the start, this effort should have been provided by the U.S. government. This does not mean Johns Hopkins believes the pandemic is over. It is not. The institution remains committed to maintaining a leadership role in providing the public and policymakers with cutting edge insights into COVID-19. See details below.
Ongoing Johns Hopkins COVID-19 Resources
The Hub — the news and information website for Johns Hopkins — publishes the latest updates on COVID-19 research about vaccines, treatments, and public health measures.
The Johns Hopkins Bloomberg School of Public Health maintains the COVID-19 Projects and Initiatives page to share the latest research and practice efforts by Bloomberg faculty.
The Johns Hopkins Center for Health Security has been at the forefront of providing policymakers and the public with vital information on how to mitigate disease spread.
The Johns Hopkins International Vaccine Access Center offers an online, interactive map-based platform for easy navigation of hundreds of research reports into vaccine use and impact.
Johns Hopkins Medicine provides various online portals that provide information about COVID-19 patient care, vaccinations, testing and more.
Accessing past data
Johns Hopkins maintains two data repositories for the information collected by the Coronavirus Resource Center between Jan. 22, 2020 and March 10, 2023. The first features global cases and deaths data as plotted by the Center for Systems Science and Engineering. The second features U.S. and global vaccination data , testing information and demographics that were maintained by Johns Hopkins University’s Bloomberg Center for Government Excellence .
How to use the CSSE GitHub
- Click on csse_covid_19_data to access chronological 'time series' data.
- Click on csse_covid_19_time_series to access daily data for cases and deaths.
- Click on 'confirmed_US' for U.S. cases and “deaths_US” for U.S. fatalities; and “confirmed_global” for international cases and 'deaths_global' for international fatalities.
- Click “View Raw.”
- Left click to “Save As” as spreadsheet.
How to use the GovEx GitHub
- Visit the U.S. 'data dictionary' and the global 'data dictionary' to understand the vaccine, testing and demographic data available for your use.
- Example: Click on either 'us_data' or 'global_data' for vaccine information.
- Click on 'time_series' to access daily reports for either the U.S. or the world.
- Select either 'vaccines' or “doses administered” for the U.S. or the world.
- For either database, click 'view raw' to view the data or to save it to a spreadsheet.
Government Resources
Us cases & deaths.
The U.S. Centers for Disease Control and Prevention maintains a national data tracker.
GLOBAL TRENDS
The World Health Organization provides information about global spread.
The CDC also provides a vaccine data tracker for the U.S., while Our World In Data from Oxford University provides global vaccine information.
HOSPITAL ADMISSIONS
The CDC and the U.S. Department of Health and Human Services have provided hospital admission data in the United States.
THANK YOU TO ALL CONTRIBUTORS TO THE JHU CRC TEAM
Thank you to our partners, thank you to our funders, special thanks to.
Aaron Katz, Adam Lee, Alan Ravitz, Alex Roberts, Alexander Evelo, Amanda Galante, Amina Mahmood, Angel Aliseda Alonso, Anna Yaroslaski, Arman Kalikian, Beatrice Garcia, Breanna Johnson, Cathy Hurley, Christina Pikas, Christopher Watenpool, Cody Meiners, Cory McCarty, Dane Galloway, Daniel Raimi Zlatic, David Zhang, Doug Donovan, Elaine Gehr, Emily Camacho, Emily Pond, Ensheng Dong, Eric Forte, Ethel Wong, Evan Bolt, Fardin Ganjkhanloo, Farzin Ahmadi, Fernando Ortiz-Sacarello, George Cancro, Grant Zhao, Greta Kinsley, Gus Sentementes, Heather Bree, Hongru Du, Ian Price, Jan LaBarge, Jason Williams, Jeff Gara, Jennifer Nuzzo, Jeremy Ratcliff, Jill Rosen, Jim Maguire, John Olson, John Piorkowski, Jordan Wesley, Joseph Duva, Joseph Peterson, Josh Porterfield, Joshua Poplawski, Kailande Cassamajor, Kevin Medina Santiago, Khalil Hijazi, Krushi Shah, Lana Milman, Laura Asher, Laura Murphy, Lauren Kennell, Louis Pang, Mara Blake, Marianne von Nordeck, Marissa Collins, Marlene Caceres, Mary Conway Vaughan, Meg Burke, Melissa Leeds, Michael Moore, Miles Stewart, Miriam McKinney Gray, Mitch Smallwood, Molly Mantus, Nick Brunner, Nishant Gupta, Oren Tirschwell, Paul Nicholas, Phil Graff, Phillip Hilliard, Promise Maswanganye, Raghav Ramachandran, Reina Chano Murray, Roman Wang, Ryan Lau, Samantha Cooley, Sana Talwar, Sara Bertran de Lis, Sarah Prata, Sarthak Bhatnagar, Sayeed Choudury, Shelby Wilson, Sheri Lewis, Steven Borisko, Tamara Goyea, Taylor Martin, Teresa Colella, Tim Gion, Tim Ng, William La Cholter, Xiaoxue Zhou, Yael Weiss
CRC in the Media
Time names crc go-to data source.
TIME Magazine named the Johns Hopkins Coronavirus Resource Center one of its Top 100 Inventions for 2020.
Research!America Praises CRC Work
Research!America awarded the CRC a public health honor for providing reliable real time data about COVID-19.
CRC Earns Award From Fast Company
The Johns Hopkins Coronavirus Resource Center wins Fast Company’s 2021 Innovative Team of the Year award.
Public Service
Lauren Gardner Wins Lasker Award for Service
Lauren Gardner, co-creator of the COVID-19 Dashboard, won the 2022 Lasker-Bloomberg Public Service Award.

Top Science News
Latest top headlines.
- Brain-Computer Interfaces
- Learning Disorders
- Brain Injury
- HIV and AIDS
- New Species
- Bird Flu Research
- Breast Cancer
- Colon Cancer
- Energy Technology
- Energy and Resources
- Electronics
- Wearable Technology
- Nature of Water
- Thermodynamics
- Materials Science
- Engineering and Construction
- Dolphins and Whales
- Global Warming
- Wild Animals
- Insects (including Butterflies)
- Endangered Plants
- Human Evolution
- Anthropology
- Human Brain: New Gene Transcripts
- Epstein-Barr Virus and Resulting Diseases
- Birdsong and Human Voice: Same Genetic Blueprint
- Predicting Individual Cancer Risk
Top Physical/Tech
- Charge Your Laptop in a Minute?
- 'Electronic Spider Silk' Printed On Human Skin
- Engineered Surfaces Made to Shed Heat
- Innovative Material for Sustainable Building
Top Environment
- Future Climate Impacts Put Whale Diet at Risk
- Caterpillars Detect Predators by Electricity
- Symbiotic Bacteria Communicate With Plants
- Early Arrival of Palaeolithic People On Cyprus
Health News
Latest health headlines.
- Immune System
- Medical Topics
- Diseases and Conditions
- Robotics Research
- Today's Healthcare
- Medical Devices
- Alzheimer's Research
- Alzheimer's
- Healthy Aging
- Staying Healthy
- Child Psychology
- K-12 Education
- Child Development
- Intelligence
- Language Acquisition
- Racial Issues
- Industrial Relations
- Breastfeeding
- Infant's Health
Health & Medicine
- Urban Gardening May Improve Human Health
- Enhancing Stereotactic Neurosurgery Precision
- New Molecular Drivers of Alzheimer's
- I'll Have What She's Having!
Mind & Brain
- More School Entry Disadvantages at Age 16-17
- End to Driving for Older Adults
- AI-Powered Headphones Filter Only Unwanted Noise
- US Hockey Players Use Canadian Accent
Living Well
- Does It Matter If Your Teens Listen to You?
- Stress and Busy Bragging at Work
- Health and Economic Benefits of Breastfeeding
- When Older Adults Stop Driving
Physical/Tech News
Latest physical/tech headlines.
- Medical Technology
- Energy and the Environment
- Environmental Science
- Wounds and Healing
- Extrasolar Planets
- Ancient Civilizations
- Black Holes
- Behavioral Science
- Quantum Computers
- Spintronics
- Spintronics Research
- Telecommunications
- Retail and Services
- Computers and Internet
- Privacy Issues
Matter & Energy
- Sulfur Trioxide in the Atmosphere
- Hidden Threats With Advanced X-Ray Imaging
- Recovering Electricity from Heat Storage: 44%
- New Tool to Move Tiny Bioparticles
Space & Time
- Intriguing World Sized Between Earth, Venus
- Cosmic Rays Illuminate the Past
- Star Suddenly Vanish from the Night Sky
- Triple-Star System
Computers & Math
- Finding the Beat of Collective Animal Motion
- Uncharted Territory in Quantum Devices
- 6G and Beyond: Next Gen Wireless
- Hospitality Sector: AI as Concierge
Environment News
Latest environment headlines.
- Molecular Biology
- Cell Biology
- Biotechnology
- Invasive Species
- Sustainability
- Environmental Policies
- Urbanization
- Drought Research
- Recycling and Waste
- World Development
- Evolutionary Biology
- Pests and Parasites
- Charles Darwin
- Animal Learning and Intelligence
- Origin of Life
Plants & Animals
- Tissue-Specific Protein-Protein Interactions
- Biodiversity in Crabs
- World Abuzz With New Bumble Bee Sightings
- Observing Cells With Superfast Soft X-Rays
Earth & Climate
- GBGI Infrastructure Mitigating Urban Heat
- Reduce Streamflow in Colorado River Basin
- Sewage Overflows and Gastrointestinal Illnesses
- Recurring Evolutionary Changes in Insects
Fossils & Ruins
- Elephant Hunting in Chile 12,000 Years Ago
- 250-Year-Old Mystery of the German Cockroach
- Cooperative Hunting Not Brain-Intensive
- Legacy of Indigenous Stewardship of Camas
Society/Education News
Latest society/education headlines.
- Environmental Awareness
- Gender Difference
- Mental Health
- Severe Weather
- Resource Shortage
- ADD and ADHD
- Neuroscience
- Information Technology
- Engineering
- Mathematical Modeling
- Energy Issues
- STEM Education
- Educational Policy
- Educational Technology
- Mathematics
- Computer Modeling
Science & Society
- Influencing Climate-Change Risk Perception
- Transitioning Gender Not Linked to Depression
- Climbing the Social Ladder Slows Dementia
- Drought-Monitoring Outpaced by Climate Changes
Education & Learning
- ADHD and Emotional Problems
- How Practice Forms New Memory Pathways
- No Inner Voice Linked to Poorer Verbal Memory
- AI Knowledge Gets Your Foot in the Door
Business & Industry
- Robot-Phobia and Labor Shortages
- Pulling Power of Renewables
- Can AI Simulate Multidisciplinary Workshops?
- New Sensing Checks Overhaul Manufacturing
- Origins of the Proton's Spin
Trending Topics
Strange & offbeat, about this site.
ScienceDaily features breaking news about the latest discoveries in science, health, the environment, technology, and more -- from leading universities, scientific journals, and research organizations.
Visitors can browse more than 500 individual topics, grouped into 12 main sections (listed under the top navigational menu), covering: the medical sciences and health; physical sciences and technology; biological sciences and the environment; and social sciences, business and education. Headlines and summaries of relevant news stories are provided on each topic page.
Stories are posted daily, selected from press materials provided by hundreds of sources from around the world. Links to sources and relevant journal citations (where available) are included at the end of each post.
For more information about ScienceDaily, please consult the links listed at the bottom of each page.
- - Google Chrome
Intended for healthcare professionals
- Access provided by Google Indexer
- My email alerts
- BMA member login
- Username * Password * Forgot your log in details? Need to activate BMA Member Log In Log in via OpenAthens Log in via your institution

Search form
- Advanced search
- Search responses
- Search blogs
- When and how to update...
When and how to update systematic reviews: consensus and checklist
- Related content
Peer review
This article has a correction. please see:.
- Errata - September 06, 2016
- Paul Garner , professor 1 ,
- Sally Hopewell , associate professor 2 ,
- Jackie Chandler , methods coordinator 3 ,
- Harriet MacLehose , senior editor 3 ,
- Elie A Akl , professor 5 6 ,
- Joseph Beyene , associate professor 7 ,
- Stephanie Chang , director 8 ,
- Rachel Churchill , professor 9 ,
- Karin Dearness , managing editor 10 ,
- Gordon Guyatt , professor 4 ,
- Carol Lefebvre , information consultant 11 ,
- Beth Liles , methodologist 12 ,
- Rachel Marshall , editor 3 ,
- Laura Martínez García , researcher 13 ,
- Chris Mavergames , head 14 ,
- Mona Nasser , clinical lecturer in evidence based dentistry 15 ,
- Amir Qaseem , vice president and chair 16 17 ,
- Margaret Sampson , librarian 18 ,
- Karla Soares-Weiser , deputy editor in chief 3 ,
- Yemisi Takwoingi , senior research fellow in medical statistics 19 ,
- Lehana Thabane , director and professor 4 20 ,
- Marialena Trivella , statistician 21 ,
- Peter Tugwell , professor of medicine, epidemiology, and community medicine 22 ,
- Emma Welsh , managing editor 23 ,
- Ed C Wilson , senior research associate in health economics 24 ,
- Holger J Schünemann , professor 4 5
- 1 Cochrane Infectious Diseases Group, Department of Clinical Sciences, Liverpool School of Tropical Medicine, Liverpool L3 5QA, UK
- 2 Oxford Clinical Trials Research Unit, University of Oxford, Oxford, UK
- 3 Cochrane Editorial Unit, Cochrane Central Executive, London, UK
- 4 Department of Clinical Epidemiology and Biostatistics and Department of Medicine, McMaster University, Hamilton, ON, Canada
- 5 Cochrane GRADEing Methods Group, Ottawa, ON, Canada
- 6 Department of Internal Medicine, American University of Beirut, Beirut, Lebanon
- 7 Department of Mathematics and Statistics, McMaster University
- 8 Evidence-based Practice Center Program, Agency for Healthcare and Research Quality, Rockville, MD, USA
- 9 Centre for Reviews and Dissemination, University of York, York, UK
- 10 Cochrane Upper Gastrointestinal and Pancreatic Diseases Group, Hamilton, ON, Canada
- 11 Lefebvre Associates, Oxford, UK
- 12 Kaiser Permanente National Guideline Program, Portland, OR, USA
- 13 Iberoamerican Cochrane Centre, Barcelona, Spain
- 14 Cochrane Informatics and Knowledge Management, Cochrane Central Executive, Freiburg, Germany
- 15 Plymouth University Peninsula School of Dentistry, Plymouth, UK
- 16 Department of Clinical Policy, American College of Physicians, Philadelphia, PA, USA
- 17 Guidelines International Network, Pitlochry, UK
- 18 Children’s Hospital of Eastern Ontario, Ottawa, ON, Canada
- 19 Institute of Applied Health Research, University of Birmingham, Birmingham, UK
- 20 Biostatistics Unit, Centre for Evaluation, McMaster University, Hamilton, ON, Canada
- 21 Centre for Statistics in Medicine, University of Oxford, Oxford, UK
- 22 University of Ottawa, Ottawa, ON, Canada
- 23 Cochrane Airways Group, Population Health Research Institute, St George’s, University of London, London, UK
- 24 Cambridge Centre for Health Services Research, University of Cambridge, Cambridge, UK
- Correspondence to: P Garner Paul.Garner{at}lstmed.ac.uk
- Accepted 26 May 2016
Updating of systematic reviews is generally more efficient than starting all over again when new evidence emerges, but to date there has been no clear guidance on how to do this. This guidance helps authors of systematic reviews, commissioners, and editors decide when to update a systematic review, and then how to go about updating the review.
Systematic reviews synthesise relevant research around a particular question. Preparing a systematic review is time and resource consuming, and provides a snapshot of knowledge at the time of incorporation of data from studies identified during the latest search. Newly identified studies can change the conclusion of a review. If they have not been included, this threatens the validity of the review, and, at worst, means the review could mislead. For patients and other healthcare consumers, this means that care and policy development might not be fully informed by the latest research; furthermore, researchers could be misled and carry out research in areas where no further research is actually needed. 1 Thus, there are clear benefits to updating reviews, rather than duplicating the entire process as new evidence emerges or new methods develop. Indeed, there is probably added value to updating a review, because this will include taking into account comments and criticisms, and adoption of new methods in an iterative process. 2 3 4 5 6
Cochrane has over 20 years of experience with preparing and updating systematic reviews, with the publication of over 6000 systematic reviews. However, Cochrane’s principle of keeping all reviews up to date has not been possible, and the organisation has had to adapt: from updating when new evidence becomes available, 7 to updating every two years, 8 to updating based on need and priority. 9 This experience has shown that it is not possible, sensible, or feasible to continually update all reviews all the time. Other groups, including guideline developers and journal editors, adopt updating principles (as applied, for example, by the Systematic Reviews journal; https://systematicreviewsjournal.biomedcentral.com/ ).
The panel for updating guidance for systematic reviews (PUGs) group met to draw together experiences and identify a common approach. The PUGs guidance can help individuals or academic teams working outside of a commissioning agency or Cochrane, who are considering writing a systematic review for a journal or to prepare for a research project. The guidance could also help these groups decide whether their effort is worthwhile.
Summary points
Updating systematic reviews is, in general, more efficient than starting afresh when new evidence emerges. The panel for updating guidance for systematic reviews (PUGs; comprising review authors, editors, statisticians, information specialists, related methodologists, and guideline developers) met to develop guidance for people considering updating systematic reviews. The panel proposed the following:
Decisions about whether and when to update a systematic review are judgments made for individual reviews at a particular time. These decisions can be made by agencies responsible for systematic review portfolios, journal editors with systematic review update services, or author teams considering embarking on an update of a review.
The decision needs to take into account whether the review addresses a current question, uses valid methods, and is well conducted; and whether there are new relevant methods, new studies, or new information on existing included studies. Given this information, the agency, editors, or authors need to judge whether the update will influence the review findings or credibility sufficiently to justify the effort in updating it.
Review authors and commissioners can use a decision framework and checklist to navigate and report these decisions with “update status” and rationale for this status. The panel noted that the incorporation of new synthesis methods (such as Grading of Recommendations Assessment, Development and Evaluation (GRADE)) is also often likely to improve the quality of the analysis and the clarity of the findings.
Given a decision to update, the process needs to start with an appraisal and revision of the background, question, inclusion criteria, and methods of the existing review.
Search strategies should be refined, taking into account changes in the question or inclusion criteria. An analysis of yield from the previous edition, in relation to databases searched, terms, and languages can make searches more specific and efficient.
In many instances, an update represents a new edition of the review, and authorship of the new version needs to follow criteria of the International Committee of Medical Journal Editors (ICMJE). New approaches to publishing licences could help new authors build on and re-use the previous edition while giving appropriate credit to the previous authors.
The panel also reflected on this guidance in the context of emerging technological advances in software, information retrieval, and electronic linkage and mining. With good synthesis and technology partnerships, these advances could revolutionise the efficiency of updating in the coming years.
Panel selection and procedures
An international panel of authors, editors, clinicians, statisticians, information specialists, other methodologists, and guideline developers was invited to a two day workshop at McMaster University, Hamilton, Canada, on 26-27 June 2014, organised by Cochrane. The organising committee selected the panel (web appendix 1). The organising committee invited participants, put forward the agenda, collected background materials and literature, and drafted the structure of the report.
The purpose of the workshop was to develop a common approach to updating systematic reviews, drawing on existing strategies, research, and experience of people working in this area. The selection of participants aimed on broad representation of different groups involved in producing systematic reviews (including authors, editors, statisticians, information specialists, and other methodologists), and those using the reviews (guideline developers and clinicians). Participants within these groups were selected on their expertise and experience in updating, in previous work developing methods to assess reviews, and because some were recognised for developing approaches within organisations to manage updating strategically. We sought to identify general approaches in this area, and not be specific to Cochrane; although inevitably most of the panel were somehow engaged in Cochrane.
The workshop structure followed a series of short presentations addressing key questions on whether, when, and how to update systematic reviews. The proceedings included the management of authorship and editorial decisions, and innovative and technological approaches. A series of small group discussions followed each question, deliberating content, and forming recommendations, as well as recognising uncertainties. Large group, round table discussions deliberated further these small group developments. Recommendations were presented to an invited forum of individuals with varying levels of expertise in systematic reviews from McMaster University (of over 40 people), widely known for its contributions to the field of research evidence synthesis. Their comments helped inform the emerging guidance.
The organising committee became the writing committee after the meeting. They developed the guidance arising from the meeting, developed the checklist and diagrams, added examples, and finalised the manuscript. The guidance was circulated to the larger group three times, with the PUGs panel providing extensive feedback. This feedback was all considered and carefully addressed by the writing committee. The writing committee provided the panel with the option of expressing any additional comments from the general or specific guidance in the report, and the option for registering their own view that might differ to the guidance formed and their view would be recorded in an annex. In the event, consensus was reached, and the annex was not required.
Definition of update
The PUGs panel defined an update of a systematic review as a new edition of a published systematic review with changes that can include new data, new methods, or new analyses to the previous edition. This expands on a previous definition of a systematic review update. 10 An update asks a similar question with regard to the participants, intervention, comparisons, and outcomes (PICO) and has similar objectives; thus it has similar inclusion criteria. These inclusion criteria can be modified in the light of developments within the topic area with new interventions, new standards, and new approaches. Updates will include a new search for potentially relevant studies and incorporate any eligible studies or data; and adjust the findings and conclusions as appropriate. Box 1 provides some examples.
Box 1: Examples of what factors might change in an updated systematic review
A systematic review of steroid treatment in tuberculosis meningitis used GRADE methods and split the composite outcome in the original review of death plus disability into its two components. This improved the clarity of the reviews findings in relation to the effects and the importance of the effects of steroids on death and on disability. 11
A systematic review of dihydroartemisinin-piperaquine (DHAP) for treating malaria was updated with much more detailed analysis of the adverse effect data from the existing trials as a result of questions raised by the European Medicines Agency. Because the original review included other comparisons, the update required extracting only the DHAP comparisons from the original review, and a modification of the title and the PICO. 12
A systematic review of atorvastatin was updated with simple uncontrolled studies. 13 This update allowed comparisons with trials and strengthened the review findings. 14
Which systematic reviews should be updated and when?
Any group maintaining a portfolio of systematic reviews as part of their normative work, such as guidelines panels or Cochrane review groups, will need to prioritise which reviews to update. Box 2 presents the approaches used by the Agency for HealthCare Research and Quality (AHRQ) and Cochrane to prioritise which systematic reviews to update and when. Clearly, the responsibility for deciding which systematic reviews should be updated and when they will be updated will vary: it may be centrally organised and resourced, as with the AHRQ scientific resource centre (box 2). In Cochrane, the decision making process is decentralised to the Cochrane Review Group editorial team, with different approaches applied, often informally.
Box 2: Examples of how different organisations decide on updating systematic reviews
Agency for healthcare research and quality (us).
The AHRQ uses a needs based approach; updating systematic reviews depends on an assessment of several criteria:
Stakeholder impact
Interest from stakeholder partners (such as consumers, funders, guideline developers, clinical societies, James Lind Alliance)
Use and uptake (for example, frequency of citations and downloads)
Citation in scientific literature including clinical practice guidelines
Currency and need for update
New research is available
Review conclusions are probably dated
Update decision
Based on the above criteria, the decision is made to either update, archive, or continue surveillance.
Of over 50 Cochrane editorial teams, most but not all have some systems for updating, although this process can be informal and loosely applied. Most editorial teams draw on some or all of the following criteria:
Strategic importance
Is the topic a priority area (for example, in current debates or considered by guidelines groups)?
Is there important new information available?
Practicalities in organising the update that many groups take into account
Size of the task (size and quality of the review, and how many new studies or analyses are needed)
Availability and willingness of the author team
Impact of update
New research impact on findings and credibility
Consider whether new methods will improve review quality
Priority to update, postpone update, class review as no longer requiring an update
The PUGs panel recommended an individualised approach to updating, which used the procedures summarised in figure 1 ⇓ . The figure provides a status category, and some options for classifying reviews into each of these categories, and builds on a previous decision tool and earlier work developing an updating classification system. 15 16 We provide a narrative for each step.
Fig 1 Decision framework to assess systematic reviews for updating, with standard terms to report such decisions
- Download figure
- Open in new tab
- Download powerpoint
Step 1: assess currency
Does the published review still address a current question.
An update is only worthwhile if the question is topical for decision making for practice, policy, or research priorities (fig 1 ⇑ ). For agencies, people responsible for managing a portfolio of systematic reviews, there is a need to use both formal and informal horizon scanning. This type of scanning helps identify questions with currency, and can help identify those reviews that should be updated. The process could include monitoring policy debates around the review, media outlets, scientific (and professional) publications, and linking with guideline developers.
Has the review had good access or use?
Metrics for citations, article access and downloads, and sharing via social or traditional media can be used as proxy or indicators for currency and relevance of the review. Reviews that are widely cited and used could be important to update should the need arise. Comparable reviews that are never cited or rarely downloaded, for example, could indicate that they are not addressing a question that is valued, and might not be worth updating.
In most cases, updated reviews are most useful to stakeholders when there is new information or methods that result in a change in findings. However, there are some circumstances in which an up to date search for information is important for retaining the credibility of the review, regardless of whether the main findings would change or not. For example, key stakeholders would dismiss a review if a study is carried out in a relevant geographical setting but is not included; if a large, high profile study that might not change the findings is not included; or if an up to date search is required for a guideline to achieve credibility. Box 3 provides such examples. If the review does not answer a current question, the intervention has been superseded, then a decision can be made not to update and no further intelligence gathering is required (fig 1 ⇑ ).
Box 3: Examples of a systematic review’s currency
The public is interested in vitamin C for preventing the common cold: the Cochrane review includes over 29 trials with either no or small effects, concluding good evidence of no important effects. 17 Assessment: still a current question for the public.
Low osmolarity oral rehydration salt (ORS) solution versus standard solution for acute diarrhoea in children: the 2001 Cochrane review 18 led the World Health Organization to recommend ORS solution formula worldwide to follow the new ORS solution formula 19 and this has now been accepted globally. Assessment: no longer a current question.
Routine prophylactic antibiotics with caesarean section: the Cochrane review reports clear evidence of maternal benefit from placebo controlled trials but no information on the effects on the baby. 20 Assessment: this is a current question.
A systematic review published in the Lancet examined the effects of artemisinin based combination treatments compared with monotherapy for treating malaria and showed clear benefit. 21 Assessment: this established the treatment globally and is no longer a current question and no update is required.
A Cochrane review of amalgam restorations for dental caries 22 is unlikely to be updated because the use of dental amalgam is declining, and the question is not seen as being important by many dental specialists. Assessment: no longer a current question.
Did the review use valid methods and was it well conducted?
If the question is current and clearly defined, the systematic review needs to have used valid methods and be well conducted. If the review has vague inclusion criteria, poorly articulated outcomes, or inappropriate methods, then updating should not proceed. If the question is current, and the review has been cited or used, then it might be appropriate to simply start with a new protocol. The appraisal should take into account the methods in use when the review was done.
Step 2: identify relevant new methods, studies, and other information
Are there any new relevant methods.
If the question is current, but the review was done some years ago, the quality of the review might not meet current day standards. Methods have advanced quickly, and data extraction and understanding of the review process have become more sophisticated. For example:
Methods for assessing risk of bias of randomised trials, 23 diagnostic test accuracy (QUADAS-2), 24 and observational studies (ROBINS-1). 25
Application of summary of findings, evidence profiles, and related GRADE methods has meant the characteristics of the intervention, characteristics of the participants, and risk of bias are more thoroughly and systematically documented. 26 27
Integration of other study designs containing evidence, such economic evaluation and qualitative research. 28
There are other incremental improvements in a wide range of statistical and methodological areas, for example, in describing and taking into account cluster randomised trials. 29 AMSTAR can assess the overall quality of a systematic review, 30 and the ROBIS tool can provide a more detailed assessment of the potential for bias. 31
Are there any new studies or other information?
If an authoring or commissioning team wants to ensure that a particular review is up to date, there is a need for routine surveillance for new studies that are potentially relevant to the review, by searching and trial register inspection at regular intervals. This process has several approaches, including:
Formal surveillance searching 32
Updating the full search strategies in the original review and running the searches
Tracking studies in clinical trial and other registers
Using literature appraisal services 33
Using a defined abbreviated search strategy for the update 34
Checking studies included in related systematic reviews. 35
How often this surveillance is done, and which approaches to use, depend on the circumstances and the topic. Some topics move quickly, and the definition of “regular intervals” will vary according to the field and according to the state of evidence in the field. For example, early in the life of a new intervention, there might be a plethora of studies, and surveillance would be needed more frequently.
Step 3: assess the effect of updating the review
Will the adoption of new methods change the findings or credibility.
Editors, referees, or experts in the topic area or methodologists can provide an informed view of whether a review can be substantially improved by application of current methodological expectations and new methods (fig 1 ⇑ ). For example, a Cochrane review of iron supplementation in malaria concluded that there was “no significant difference between iron and placebo detected.” 36 An update of the review included a GRADE assessment of the certainty of the evidence, and was able to conclude with a high degree of certainty that iron does not cause an excess of clinical malaria because the upper relative risk confidence intervals of harm was 1.0 with high certainty of evidence. 37
Will the new studies, information, or data change the findings or credibility?
The assessment of new data contained in new studies and how these data might change the review is often used to determine whether an update should go ahead, and the speed with which the update should be conducted. The appraisal of these new data can be carried out in different ways. Initially, methods focused on statistical approaches to predict an overturning of the current review findings in terms of the primary or desired outcome (table 1 ⇓ ). Although this aspect is important, additional studies can add important information to a review, which is more than just changing the primary outcome to a more accurate and reliable estimate. Box 4 gives examples.
Formal prediction tools: how potentially relevant new studies can affect review conclusions
- View inline
Box 4: Examples of new information other than new trials being important
The iconic Cochrane review of steroids in preterm labour was thought to provide evidence of benefit in infants, and this question no longer required new trials. However, a new large trial published in the Lancet in 2015 showed that in low and middle income countries, strategies to promote the uptake of neonatal steroids increased neonatal mortality and suspected maternal infection. 49 This information needs to somehow be incorporated into the review to maintain its credibility.
A Cochrane review of community deworming in developing countries indicates that in recent studies, there is little or no effect. 50 The inclusion of a large trial of two million children confirmed that there was no effect on mortality. Although the incorporation of the trial in the review did not change the review’s conclusions, the trial’s absence would have affected the credibility of the review, so it was therefore updated.
A new paper reporting long term follow-up data on anthracycline chemotherapy as part of cancer treatment was published. Although the effects from the outcomes remained essentially unchanged, apart from this longer follow-up, the paper also included information about the performance bias in the original trial, shifting the risk of bias for several outcomes from “unknown” to “high” in the Cochrane review. 51
Reviews with a high level of certainty in the results (that is, when the GRADE assessment for the body of evidence is high) are less likely to change even with the addition of new studies, information, or data, by definition. GRADE can help guide priorities in whether to update, but it is still important to assess new studies that might meet the inclusion criteria. New studies can show unexpected effects (eg, attenuation of efficacy) or provide new information about the effects seen in different circumstances (eg, groups of patients or locations).
Other tools are specifically designed to help decision making in updating. For example, the Ottawa 39 and RAND 45 methods focus on identification of new evidence, the statistical predication tool 15 calculates the probability of new evidence changing the review conclusion, and the value of information analysis approach 52 calculates the expected health gain (table 1 ⇑ ). As yet, there has been limited external validation of these tools to determine which approach would be most effective and when.
If potentially relevant studies are identified that have not previously been assessed for inclusion, authors or those managing the updating process need to assess whether including them might affect the conclusions of the review. They need to examine the weight and certainty of the new evidence to help determine whether an update is needed and how urgent that update is. The updating team can assess this informally by judging whether new studies or data are likely to substantively affect the review, for example, by altering the certainty in an existing comparison, or by generating new comparisons and analyses in the existing review.
New information can also include fresh follow-up data on existing included studies, or information on how the studies were carried out. These should be assessed in terms of whether they might change the review findings or improve its credibility (fig 1 ⇑ ). Indeed, if any study has been retracted, it is important the authors assess the reasons for its retraction. In the case of data fabrication, the study needs to be removed from the analysis and this recorded. A decision needs to be made as to whether other studies by the same author should be removed from the review and other related reviews. An investigation should also be initiated following guidelines from the Committee on Publication Ethics (COPE). Additional published and unpublished data can become available from a wide range of sources—including study investigators, regulatory agencies and industry—and are important to consider.
Preparing for an update
Refresh background, objectives, inclusion criteria, and methods
Before including new studies in the review, authors need to revisit the background, objectives, inclusion criteria, and methods of the current review. In Cochrane, this is referred to as the protocol, and editors are part of this process. The update could range from simply endorsing the current question and inclusion criteria, through to full rewriting of the question, inclusion criteria and methods, and republishing the protocol. As a field progresses with larger and better quality trials rigorously testing the questions posed, it may be appropriate to exclude weaker study designs (such as quasi-randomised comparisons or very small trials) from the update (table 2 ⇓ ). The PUGs panel recommended that a protocol refresh will require the authors to use the latest accepted methods of synthesis, even if this means repeating data extraction for all studies.
New authors and authorship
Updated systematic reviews are new publications with new citations. An authorship team publishing an update in a scientific or medical journal is likely to manage the new edition of a review in the same way as with any other publication, and follow the ICMJE authorship criteria. 56 If the previous author or author team steps down, then they should be acknowledged in the new version. However, some might perceive that their efforts in the first version warrant continued authorship, which may be valid. The management of authorship between versions can sometimes be complicated. At worst, it delays new authors completing an update and leads to long authorship lists of people from previous versions who probably do not meet ICMJE authorship criteria. One approach with updates including new authors is to have an opt-in policy for the existing authors: they can opt in to the new edition, provided that they make clear their contribution, and this is then agreed with the entire author team.
Although they are new publications, updates will generally include content from the published version. Changing licensing rights around systematic reviews to allow new authors of future updates to remix, tweak, or build on the contributions of the original authors of the published version (similar to the rights available via a Creative Commons licence; https://creativecommons.org ) could be a more sustainable and simpler approach. This approach would allow systematic reviews to continue to evolve and build on the work of a range of authors over time, and for contributors to be given credit for contributions to this previous work.
Efficient searching
In performing an update, a search based on the search conducted for the original review is required. The updated search strategy will need to take into account changes in the review question or inclusion criteria, for example, and might be further adjusted based on knowledge of running the original search strategy. The search strategy for an update need not replicate the original search strategy, but could be refined, for example, based on an analysis of the yield of the original search. These new search approaches are currently undergoing formal empirical evaluation, but they may well provide much more efficient search strategies in the future. Some examples of these possible new methods for review updates are described in web appendix 2.
In reporting the search process for the update, investigators must ensure transparency for any previous versions and the current update, and use an adapted flow diagram based on PRISMA reporting (preferred reporting items for systematic reviews and meta-analyses). 57 The search processes and strategies for the update must be adequately reported such that they could be replicated.
Systematic reviews published for the first time in peer reviewed journals are by definition peer reviewed, but practice for updates remains variable, because an update might have few changes (such as an updated search but no new studies found and therefore included) or many changes (such as revise methods and inclusion of several new studies leading to revised conclusions). Therefore, and to use peer reviewers’ time most effectively, editors need to consider when to peer review an update and the type of peer reviewer most useful for a particular update (for example, topic specialist, methodologist). The decision to use peer review, and the number and expertise of the peer reviewers could depend on the nature of the update and the extent of any changes to the systematic review as part of an editor assessment. A change in the date of the search only (where no new studies were identified) would not require peer review (except, arguably, peer review of the search), but the addition of studies that lead to a change in conclusions or significant changes to the methods would require peer review. The nature of the peer review could be described within the published article.
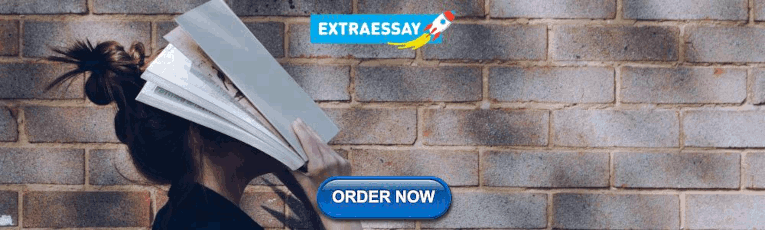
Reporting changes
Authors should provide a clear description of the changes in approach or methods between different editions of a review. Also, authors need to report the differences in findings between the original and updated edition to help users decide how to use the new edition. The approach or format used to present the differences in findings might vary with the target user group. 58 Publishers need to ensure that all previous versions of the review remain publically accessible.
Updates can range from small adjustments to reviews being completely rewritten, and the PUGs panel spent some time debating whether the term “new edition” would be a better description than “update.” However, the word “update” is now in common parlance and changing the term, the panel judged, could cause confusion. However, the debate does illustrate that an update could represent a review that asks a similar question but has been completely revised.
Technology and innovation
The updating of systematic review is generally done manually and is time consuming. There are opportunities to make better use of technology to streamline the updating process and improve efficiency (table 3 ⇓ ). Some of these tools already exist and are in development or in early use, and some are commercially available or freely available. The AHRQ’s evidence based practice centre team has recently published tools for searching and screening, and will provide an assessment of the use, reliability, and availability of these tools. 63
Technological innovations to improve the efficiency of updating systematic reviews
Other developments, such as targeted updates that are performed rapidly and focus on updating only key components of a review, could provide different approaches to updating in the future and are being piloted and evaluated. 64 With implementation of these various innovations, the longer term goal is for “living” systematic reviews, which identify and incorporate information rapidly as it evolves over time. 60
Concluding remarks
Updating systematic reviews, rather than addressing the same question with a fresh protocol, is generally more efficient and allows incremental improvement over time. Mechanical rules appear unworkable, but there is no clear unified approach on when to update, and how implement this. This PUGs panel of authors, editors, statisticians, information specialists, other methodologists, and guideline developers brought together current thinking and experience in this area to provide guidance.
Decisions about whether and when to update a systematic review are judgments made at a point in time. They depend on the currency of the question asked, the need for updating to maintain credibility, the availability of new evidence, and whether new research or new methods will affect the findings.
Whether the review uses current methodological standards is important in deciding if the update will influence the review findings, quality, reliability, or credibility sufficiently to justify the effort in updating it. Those updating systematic reviews to author clinical practice guidelines might consider the influence of new study results in potentially overturning the conclusions of an existing review. Yet, even in cases where new study findings do not change the primary outcome measure, new studies can carry important information about subgroup effects, duration of treatment effects, and other relevant clinical information, enhancing the currency and breadth of review results.
An update requires appraisal and revision of the background, question, inclusion criteria, and methods of the existing review and the existing certainty in the evidence. In particular, methods might need to be updated, and search strategies reconsidered. Authors of updates need to consider inputs to the current edition, and follow ICMJE criteria regarding authorship. 56
The PUGs panel proposed a decision framework (fig 1 ⇑ ), with terms and categories for reporting the decisions made for updating procedures for adoption by Cochrane and other stakeholders. This framework includes journals publishing systematic review updates and independent authors considering updates of existing published reviews. The panel developed a checklist to help judgements about when and how to update.
The current emphasis of authors, guideline developers, Cochrane, and consequently this guidance has been on effects reviews. The checklists and guidance here still applies to other types of systematic reviews, such as those on diagnostic test accuracy, and this guidance will need adapting. Accumulative experience and methods development in reviews other than those of effects are likely to help refine guidance in the future.
This guidance could help groups identify and prioritise reviews for updating and hence use their finite resources to greatest effect. Software innovation and new management systems are being developed and in early use to help streamline review updates in the coming years.
Contributors: HJS initiated the workshop. JC, SH, PG, HM, and HJS organised the materials and the agenda. SH wrote up the proceedings. PG wrote the paper from the proceedings and coordinated the development of the final guidance; JC, SH, HM, and HJS were active in the finalising of the guidance. All PUGs authors contributed to three rounds of manuscript revision.
Funding: Attendance at this meeting, for those attendees not directly employed by Cochrane, was not funded by Cochrane beyond the reimbursement of out of pocket expenses for those attendees for whom this was appropriate. Expenses were not reimbursed for US federal government attendees, in line with US government policy. Statements in the manuscript should not be construed as endorsement by the US Agency for Healthcare Research and Quality or the US Department of Health and Human Services.
Competing interests: All participants have a direct or indirect interest in systematic reviews and updating as part of their job or academic career. Most participants contribute to Cochrane, whose mission includes a commitment to the updating of its systematic review portfolio. JC, HM, RM, CM, KS-W, and MT are, or were at that time, employed by the Cochrane Central Executive.
Provenance and peer review: Not commissioned; externally peer reviewed.
This is an Open Access article distributed in accordance with the terms of the Creative Commons Attribution (CC BY 3.0) license, which permits others to distribute, remix, adapt and build upon this work, for commercial use, provided the original work is properly cited. See: http://creativecommons.org/licenses/by/3.0/ .
- ↵ Shekelle PG, Ortiz E, Rhodes S, et al. Validity of the Agency for Healthcare Research and Quality clinical practice guidelines: how quickly do guidelines become outdated? JAMA 2001 ; 286 : 1461 - 7 . doi:10.1001/jama.286.12.1461 pmid:11572738 . OpenUrl CrossRef PubMed Web of Science
- ↵ Claxton K, Cohen JT, Neumann PJ. When is evidence sufficient? Health Aff (Millwood) 2005 ; 24 : 93 - 101 . doi:10.1377/hlthaff.24.1.93 pmid:15647219 . OpenUrl Abstract / FREE Full Text
- ↵ Fenwick E, Claxton K, Sculpher M, et al. Improving the efficiency and relevance of health technology assessment: the role of decision analytic modelling. Paper 179. Centre for Health Economics, University of York, 2000 .
- ↵ Sculpher M, Claxton K. Establishing the cost-effectiveness of new pharmaceuticals under conditions of uncertainty—when is there sufficient evidence? Value Health 2005 ; 8 : 433 - 46 . doi:10.1111/j.1524-4733.2005.00033.x pmid:16091019 . OpenUrl CrossRef PubMed Web of Science
- ↵ Sculpher M, Drummond M, Buxton M. The iterative use of economic evaluation as part of the process of health technology assessment. J Health Serv Res Policy 1997 ; 2 : 26 - 30 . pmid:10180650 . OpenUrl Abstract / FREE Full Text
- ↵ Wilson E, Abrams K. From evidence based economics to economics based evidence: using systematic review to inform the design of future research. In: Shemilt I, Mugford M, Vale L, et al, eds. Evidence based economics. Blackwell Publishing, 2010 doi:10.1002/9781444320398.ch12 .
- ↵ Chalmers I, Enkin M, Keirse MJ. Preparing and updating systematic reviews of randomized controlled trials of health care. Milbank Q 1993 ; 71 : 411 - 37 . doi:10.2307/3350409 pmid:8413069 . OpenUrl CrossRef PubMed Web of Science
- ↵ Higgins J, Green S, Scholten R. Chapter 3. Maintaining reviews: updates, amendments and feedback: Version 5.1.0 (updated March 2011). Cochrane Collaboration, 2011 .
- ↵ Cochrane. Editorial and publishing policy resource. http://community.cochrane.org/editorial-and-publishing-policy-resource . 2016.
- ↵ Moher D, Tsertsvadze A. Systematic reviews: when is an update an update? Lancet 2006 ; 367 : 881 - 3 . doi:10.1016/S0140-6736(06)68358-X pmid:16546523 . OpenUrl CrossRef PubMed Web of Science
- ↵ Prasad K, Singh MB, Ryan H. Corticosteroids for managing tuberculous meningitis. Cochrane Database Syst Rev 2016 ; 4 : CD002244 . pmid:27121755 . OpenUrl PubMed
- ↵ Zani B, Gathu M, Donegan S, Olliaro PL, Sinclair D. Dihydroartemisinin-piperaquine for treating uncomplicated Plasmodium falciparum malaria. Cochrane Database Syst Rev 2014 ; 1 : CD010927 . pmid:24443033 . OpenUrl CrossRef PubMed
- ↵ Adams SP, Tsang M, Wright JM. Lipid lowering efficacy of atorvastatin. Cochrane Database Syst Rev 2012 ; 12 : CD008226 . pmid:23235655 . OpenUrl PubMed
- ↵ Higgins J. Convincing evidence from controlled and uncontrolled studies on the lipid-lowering effect of a statin. Cochrane Database Syst Rev 2012 ; 12 : ED000049 . pmid:23361645 . OpenUrl PubMed
- ↵ Takwoingi Y, Hopewell S, Tovey D, Sutton AJ. A multicomponent decision tool for prioritising the updating of systematic reviews. BMJ 2013 ; 347 : f7191 . doi:10.1136/bmj.f7191 pmid:24336453 . OpenUrl FREE Full Text
- ↵ MacLehose H, Hilton J, Tovey D, et al. The Cochrane Library: revolution or evolution? Shaping the future of Cochrane content. Background paper for The Cochrane Collaboration’s Strategic Session Paris, France, 18 April 2012. http://editorial-unit.cochrane.org/sites/editorial-unit.cochrane.org/files/uploads/2012-CC-strategic-session_full-report.pdf .
- ↵ Hemilä H, Chalker E. Vitamin C for preventing and treating the common cold. Cochrane Database Syst Rev 2013 ;( 1 ): CD000980 . pmid:23440782 .
- ↵ Hahn S, Kim S, Garner P. Reduced osmolarity oral rehydration solution for treating dehydration caused by acute diarrhoea in children. Cochrane Database Syst Rev 2002 ;( 1 ): CD002847 . pmid:11869639 .
- ↵ World Health Organization (WHO). Reduced osmolarity oral rehydration salts (ORS) formulation. A report from a meeting of Experts jointly organized by UNICEF and WHO. New York: Child and Adolescent Health and Development, 18 July 2001 http://apps.who.int/iris/bitstream/10665/67322/1/WHO_FCH_CAH_01.22.pdf .
- ↵ Smaill FM, Grivell RM. Antibiotic prophylaxis versus no prophylaxis for preventing infection after cesarean section. Cochrane Database Syst Rev 2014 ;( 10 ): CD007482 . pmid:25350672 .
- ↵ Adjuik M, Babiker A, Garner P, Olliaro P, Taylor W, White N. International Artemisinin Study Group. Artesunate combinations for treatment of malaria: meta-analysis. Lancet 2004 ; 363 : 9 - 17 . doi:10.1016/S0140-6736(03)15162-8 pmid:14723987 . OpenUrl CrossRef PubMed Web of Science
- ↵ Agnihotry A, Fedorowicz Z, Nasser M. Adhesively bonded versus non-bonded amalgam restorations for dental caries. Cochrane Database Syst Rev 2016 ; 3 : CD007517 . pmid:26954446 . OpenUrl PubMed
- ↵ Higgins JP, Altman DG, Gøtzsche PC, et al. Cochrane Bias Methods Group Cochrane Statistical Methods Group. The Cochrane Collaboration’s tool for assessing risk of bias in randomised trials. BMJ 2011 ; 343 : d5928 . doi:10.1136/bmj.d5928 pmid:22008217 . OpenUrl FREE Full Text
- ↵ Whiting PF, Rutjes AW, Westwood ME, et al. QUADAS-2 Group. QUADAS-2: a revised tool for the quality assessment of diagnostic accuracy studies. Ann Intern Med 2011 ; 155 : 529 - 36 . doi:10.7326/0003-4819-155-8-201110180-00009 pmid:22007046 . OpenUrl CrossRef PubMed Web of Science
- ↵ Sterne JAC, Higgins JPT, Reeves BC; on behalf of the development group for ROBINS-I. A tool for assessing risk of bias in non-randomized studies of interventions, version 7. March 2016. www.riskofbias.info .
- ↵ Guyatt GH, Oxman AD, Vist GE, et al. GRADE Working Group. GRADE: an emerging consensus on rating quality of evidence and strength of recommendations. BMJ 2008 ; 336 : 924 - 6 . doi:10.1136/bmj.39489.470347.AD pmid:18436948 . OpenUrl FREE Full Text
- ↵ Schünemann HJ. Interpreting GRADE’s levels of certainty or quality of the evidence: GRADE for statisticians, considering review information size or less emphasis on imprecision? J Clin Epidemiol 2016 ; 75 : 6 - 15 . doi:10.1016/j.jclinepi.2016.03.018 pmid:27063205 . OpenUrl CrossRef PubMed
- ↵ Gough D. Qualitative and mixed methods in systematic reviews. Syst Rev 2015 ; 4 : 181 . doi:10.1186/s13643-015-0151-y pmid:26670769 . OpenUrl CrossRef PubMed
- ↵ Richardson M, Garner P, Donegan S. Cluster randomised trials in Cochrane reviews: evaluation of methodological and reporting practice. PLoS One 2016 ; 11 : e0151818 . doi:10.1371/journal.pone.0151818 pmid:26982697 . OpenUrl CrossRef PubMed
- ↵ Shea BJ, Grimshaw JM, Wells GA, et al. Development of AMSTAR: a measurement tool to assess the methodological quality of systematic reviews. BMC Med Res Methodol 2007 ; 7 : 10 . doi:10.1186/1471-2288-7-10 pmid:17302989 . OpenUrl CrossRef PubMed
- ↵ Whiting P, Savović J, Higgins JP, et al. ROBIS group. ROBIS: A new tool to assess risk of bias in systematic reviews was developed. J Clin Epidemiol 2016 ; 69 : 225 - 34 . doi:10.1016/j.jclinepi.2015.06.005 pmid:26092286 . OpenUrl CrossRef PubMed
- ↵ Sampson M, Shojania KG, McGowan J, et al. Surveillance search techniques identified the need to update systematic reviews. J Clin Epidemiol 2008 ; 61 : 755 - 62 . doi:10.1016/j.jclinepi.2007.10.003 pmid:18586179 . OpenUrl CrossRef PubMed Web of Science
- ↵ Hemens BJ, Haynes RB. McMaster Premium LiteratUre Service (PLUS) performed well for identifying new studies for updated Cochrane reviews. J Clin Epidemiol 2012 ; 65 : 62 - 72.e1 . doi:10.1016/j.jclinepi.2011.02.010 pmid:21856121 . OpenUrl CrossRef PubMed
- ↵ Sagliocca L, De Masi S, Ferrigno L, Mele A, Traversa G. A pragmatic strategy for the review of clinical evidence. J Eval Clin Pract 2013 ; 19 : 689 - 96 . doi:10.1111/jep.12020 pmid:23317014 . OpenUrl CrossRef PubMed
- ↵ Rada G, Peña J, Capurro D, et al. How to create a matrix of evidence in Epistemonikos. Abstracts of the 22nd Cochrane Colloquium; Evidence-informed public health: opportunities and challenges; Hyderabad, India. Cochrane Database Syst Rev 2014 ; suppl 1 : 132 .
- ↵ Okebe JU, Yahav D, Shbita R, Paul M. Oral iron supplements for children in malaria-endemic areas. Cochrane Database Syst Rev 2011 ;( 10 ): CD006589 . pmid:21975754 .
- ↵ Neuberger A, Okebe J, Yahav D, Paul M. Oral iron supplements for children in malaria-endemic areas. Cochrane Database Syst Rev 2016 ; 2 : CD006589 . pmid:26921618 . OpenUrl PubMed
- Balshem H, Helfand M, Schünemann HJ, et al. GRADE guidelines: 3. Rating the quality of evidence. J Clin Epidemiol 2011 ; 64 : 401 - 6 . doi:10.1016/j.jclinepi.2010.07.015 pmid:21208779 . OpenUrl CrossRef PubMed Web of Science
- ↵ Chung M, Newberry SJ, Ansari MT, et al. Two methods provide similar signals for the need to update systematic reviews. J Clin Epidemiol 2012 ; 65 : 660 - 8 . doi:10.1016/j.jclinepi.2011.12.004 pmid:22464414 . OpenUrl CrossRef PubMed
- Shojania KG, Sampson M, Ansari MT, Ji J, Doucette S, Moher D. How quickly do systematic reviews go out of date? A survival analysis. Ann Intern Med 2007 ; 147 : 224 - 33 . doi:10.7326/0003-4819-147-4-200708210-00179 pmid:17638714 . OpenUrl CrossRef PubMed Web of Science
- Shojania K, Sampson M, Ansari M, et al. Updating systematic reviews; AHRQ technical reviews; report no 07-0087. Agency for Healthcare Research and Quality, 2007 .
- Pattanittum P, Laopaiboon M, Moher D, Lumbiganon P, Ngamjarus C. A comparison of statistical methods for identifying out-of-date systematic reviews. PLoS One 2012 ; 7 : e48894 . doi:10.1371/journal.pone.0048894 pmid:23185281 . OpenUrl CrossRef PubMed
- Shekelle PG, Motala A, Johnsen B, Newberry SJ. Assessment of a method to detect signals for updating systematic reviews. Syst Rev 2014 ; 3 : 13 . doi:10.1186/2046-4053-3-13 pmid:24529068 . OpenUrl CrossRef PubMed
- Shekelle PG, Newberry SJ, Wu H, et al. Identifying signals for updating systematic reviews: a comparison of two methods; report no 11-EHC042-EF. Agency for Healthcare Research and Quality, 2011 .
- ↵ Shekelle P, Newberry S, Maglione M, et al. Assessment of the need to update comparative effectiveness reviews: report of an initial rapid program assessment (2005-2009). Agency for Healthcare Research and Quality, 2009 .
- Tovey D, Marshall R, Bazian, Hopewell S, Rader T. Fit for purpose: centralised updating support for high-priority Cochrane Reviews; National Institute for Health Research Cochrane-National Health Service Engagement Award Scheme, July 2011. https://editorial-unit.cochrane.org/sites/editorial-unit.cochrane.org/files/uploads/10_4000_01%20Fit%20for%20purpose%20-%20centralised%20updating%20support%20for%20high%20priority%20Cochrane%20Reviews%20FINAL%20REPORT.pdf .
- Claxton K. The irrelevance of inference: a decision-making approach to the stochastic evaluation of health care technologies. J Health Econ 1999 ; 18 : 341 - 64 . doi:10.1016/S0167-6296(98)00039-3 pmid:10537899 . OpenUrl CrossRef PubMed Web of Science
- Wilson EC. A practical guide to value of information analysis. Pharmacoeconomics 2015 ; 33 : 105 - 21 . doi:10.1007/s40273-014-0219-x pmid:25336432 . OpenUrl CrossRef PubMed
- ↵ Althabe F, Belizán JM, McClure EM, et al. A population-based, multifaceted strategy to implement antenatal corticosteroid treatment versus standard care for the reduction of neonatal mortality due to preterm birth in low-income and middle-income countries: the ACT cluster-randomised trial. Lancet 2015 ; 385 : 629 - 39 . doi:10.1016/S0140-6736(14)61651-2 pmid:25458726 . OpenUrl CrossRef PubMed
- ↵ Taylor-Robinson D, Maayan N, Soares-Weiser K, et al. Deworming drugs for soil-transmitted intestinal worms in children: effects on nutritional indicators, haemoglobin and school performance. Cochrane Database Syst Rev 2012 ;( 11 ): CD000371 .
- ↵ van Dalen EC, van der Pal HJ, Kremer LC. Different dosage schedules for reducing cardiotoxicity in people with cancer receiving anthracycline chemotherapy. Cochrane Database Syst Rev 2016 ; 3 : CD005008 . pmid:26938118 . OpenUrl PubMed
- ↵ Wilson E. on behalf of the Cochrane Priority Setting and Campbell & Cochrane Economics Methods Groups. Which study when? Proof of concept of a proposed automated tool to help decision which reviews to update first. Cochrane Database Syst Rev 2014 ; suppl 2 : 29 - 31 .
- Rosenbaum SE, Glenton C, Nylund HK, Oxman AD. User testing and stakeholder feedback contributed to the development of understandable and useful Summary of Findings tables for Cochrane reviews. J Clin Epidemiol 2010 ; 63 : 607 - 19 . doi:10.1016/j.jclinepi.2009.12.013 pmid:20434023 . OpenUrl CrossRef PubMed
- Rosenbaum SE, Glenton C, Oxman AD. Summary-of-findings tables in Cochrane reviews improved understanding and rapid retrieval of key information. J Clin Epidemiol 2010 ; 63 : 620 - 6 . doi:10.1016/j.jclinepi.2009.12.014 pmid:20434024 . OpenUrl CrossRef PubMed Web of Science
- Vandvik PO, Santesso N, Akl EA, et al. Formatting modifications in GRADE evidence profiles improved guideline panelists comprehension and accessibility to information. A randomized trial. J Clin Epidemiol 2012 ; 65 : 748 - 55 . doi:10.1016/j.jclinepi.2011.11.013 pmid:22564503 . OpenUrl CrossRef PubMed
- ↵ International Committee of Medical Journal Editors (ICMJE). Defining the role of authors and contributors. 2016. www.icmje.org/recommendations/browse/roles-and-responsibilities/defining-the-role-of-authors-and-contributors.html .
- ↵ Stovold E, Beecher D, Foxlee R, Noel-Storr A. Study flow diagrams in Cochrane systematic review updates: an adapted PRISMA flow diagram. Syst Rev 2014 ; 3 : 54 . doi:10.1186/2046-4053-3-54 pmid:24886533 . OpenUrl CrossRef PubMed
- ↵ Newberry SJ, Shekelle PG, Vaiana M, et al. Reporting the findings of updated systematic reviews of comparative effectiveness: how do users want to view new information? report no 13-EHC093-EF. Agency for Healthcare Research and Quality, 2013 .
- Marshall IJ, Kuiper J, Wallace BC. Automating risk of bias assessment for clinical trials BCB’14. Proceedings of the 5th ACM conference on Bioinformatics, computational biology, and health informatics. 2014:88-95. http://thirdworld.nl/automating-risk-of-bias-assessment-for-clinical-trials .
- ↵ Elliott JH, Turner T, Clavisi O, et al. Living systematic reviews: an emerging opportunity to narrow the evidence-practice gap. PLoS Med 2014 ; 11 : e1001603 . doi:10.1371/journal.pmed.1001603 pmid:24558353 . OpenUrl CrossRef PubMed
- Elliott J, Sim I, Thomas J, et al. #CochraneTech: technology and the future of systematic reviews. Cochrane Database Syst Rev 2014 ;( 9 ): ED000091 . pmid:25288182 .
- Cochrane. Project transform: the Cochrane Collaboration. 2016. http://community.cochrane.org/tools/project-coordination-and-support/transform .
- ↵ Paynter R, Bañez L, Berlinerm E, et al. EPC methods: an exploration of the use of text-mining software in systematic reviews. Research white paper. AHRQ publication 16-EHC023-EF. Agency for Healthcare Research and Quality, April 2016. https://www.effectivehealthcare.ahrq.gov/search-for-guides-reviews-and-reports/?pageaction=displayproduct&productID=2214 .
- ↵ Soares-Weiser K, Marshall R, Bergman H, et al. Updating Cochrane Reviews: results of the first pilot of a focused update. Cochrane Database Syst Rev 2014 ; suppl 1 : 31 - 3 .
You are using an outdated browser. Please upgrade your browser to improve your experience.
Downtime Scheduled for May 29, 2024
The WRDS technical infrastructure will be undergoing a major upgrade from 8:00 - 11:00 AM Eastern on Wednesday, May 29, 2024 . During this time, most WRDS services will be unavailable.
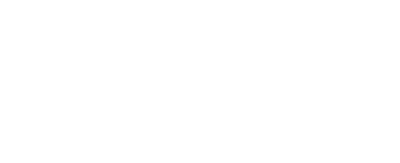
The Global Standard for Business Research

WRDS Enables Impactful Research
Global data, research analytics, academic support, for 25+ years , wharton research data services (wrds) has supported users with targeted solutions that underpin research , reinforce learning , and enable discovery . wrds advances comprehensive thought leadership by giving users the power to analyze complex information., data access.
WRDS democratizes data access so that all disciplines can easily search for concepts across the data repository.
Research Tools
WRDS dedicated PhD-level specialists developed Research Applications, Linking Tools, Guides, and Sample Programs to improve productivity and research speed.
WRDS Classroom
Classroom Teaching toolkit and video Learning Guides support all areas of research and customized for Researchers, Instructors, and Librarians.
Advanced Analytics
WRDS-developed, powerful analytics tools support original research.
Data Validation
Eliminate data infrastructure costs; ensure timely updates with ready-to-use standardized data.
Supports Accreditation
WRDS resources map to Accreditation Standards, impacting your institution’s trajectory from learning and discovery to research and publication.
Data Vendors
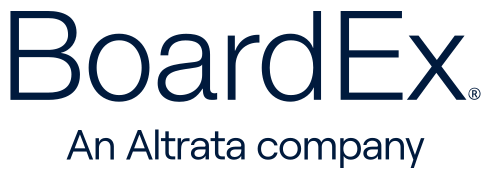
Testimonials
The Haslam College of Business has been on an outstanding research trajectory in recent years. Much of the research that we're doing are based on insights that have come through WRDS. It has allowed our faculty and students to explore a wide array of rich resources and data they never could before. Charles Noble Dean, Haslam College of Business, University of Tennessee Knoxville
WRDS offers a “complete package” for academic and professional business research: Financial and markets data from the most reliable third-party sources; a convenient data access interface tailored to research applications; a PC-SAS link with sample programs that allows you to access and manipulate data directly from your personal computer; and a responsive technical support staff. Put simply, WRDS is the only single source solution for your business research needs. Paul Chaney, Professor Owen Graduate School of Management at Vanderbilt University
Once again, you guys are on top of it. I really, really, really appreciate your quick response and I am grateful for the wonderful and prompt responses that I get to any question I ask. It is truly a pleasure to work with your team. Thanks again. Eduardo Tinoco Librarian, University of Southern California
The beauty of the system is that wherever you go, whatever system you use, the data are accessible and appear in identical form. It reduces the time researchers spend extracting data and allows them to concentrate on analysis. Richard J. Herring Jacob Safra Professor of International Banking, The Wharton School of Business
I wanted to express my pleasure with the WRDS system and support. It took me just 5 minutes to download data. I have requested assistance from the WRDS staff on several occasions, and have always received prompt, courteous, and knowledgeable assistance. Furthermore, I have found the WRDS sample programs to be extremely helpful. If only WRDS had been available sooner, my cv would be much longer. Judith A. Chevalier Professor of Economics and Finance, Yale School of Management
WRDS is the gold standard for a research and data platform and has been indispensable for my research. WRDS has been an important contributor to the development of empirical research in academic finance over the last decade. The professional and technical staff at WRDS are always a few steps ahead of the needs of finance academics. Robin Greenwood Associate Professor, Harvard Business School, Harvard University
It raises the performance bar - and expectations - for students and faculty alike. It takes the chore out of analysis and places added emphasis directly on critical thinking, which is what students should be doing. Robert W. Holthausen Nomura Securities Co. Professor of Accounting and Finance, The Wharton School Publication: Wired@Wharton
The seamless access to Wharton's financial data sets has been a key component of my curriculum development. It has influenced my lecture style, which now combines a formal lecture with a directed recitation. This teaching technique allows the students to see the data unfold in real time. Dr. Michael Phelan Associate Professor of Statistics, The Wharton School Publication: The Wharton Research Data System, Information at your fingertips
The comprehensive data and the user-friendly learning materials at WRDS have raised the productivity of academia tremendously. The earlier you learn how to take advantage of WRDS, the more productive and innovative you will be. Jianfeng Yu Jianshu Chair Professor of Finance, PBC School of Finance, Tsinghua University
Latest News & Updates
James madison university awarded wrds-ssrn innovation prize, s&p award: call for proposals, cuhk shenzhen named wrds-ssrn innovation award winner for research excellence, happy new year from wrds, university of hamburg awarded the 2023 wrds-ssrn innovation prize for commitment to academic research.
See where in the world WRDS is being used!
Learn More About WRDS!
Contact WRDS
Thank you for contacting wrds.

An official website of the United States government
The .gov means it’s official. Federal government websites often end in .gov or .mil. Before sharing sensitive information, make sure you’re on a federal government site.
The site is secure. The https:// ensures that you are connecting to the official website and that any information you provide is encrypted and transmitted securely.
- Publications
- Account settings
Preview improvements coming to the PMC website in October 2024. Learn More or Try it out now .
- Advanced Search
- Journal List

- PMC8361807.1 ; 2021 May 19
- ➤ PMC8361807.2; 2023 Oct 9
Data extraction methods for systematic review (semi)automation: Update of a living systematic review
Lena schmidt.
1 NIHR Innovation Observatory, Newcastle University, Newcastle upon Tyne, NE4 5TG, UK
2 Sciome LLC, Research Triangle Park, North Carolina, 27713, USA
3 Bristol Medical School, University of Bristol, Bristol, BS8 2PS, UK
Ailbhe N. Finnerty Mutlu
4 UCL Social Research Institute, University College London, London, WC1H 0AL, UK
Rebecca Elmore
Babatunde k. olorisade.
5 Evaluate Ltd, London, SE1 2RE, UK
6 Cardiff School of Technologies, Cardiff Metropolitan University, Cardiff, CF5 2YB, UK
James Thomas
Julian p. t. higgins, associated data, underlying data.
Harvard Dataverse: Appendix for base review. https://doi.org/10.7910/DVN/LNGCOQ . 127
This project contains the following underlying data:
- • Appendix_A.zip (full database with all data extraction and other fields for base review data)
- • Appendix B.docx (further information about excluded publications)
- • Appendix_C.zip (code, weights, data, scores of abstract classifiers for Web of Science content)
- • Appendix_D.zip (full database with all data extraction and other fields for LSR update)
- • Supplementary_key_items.docx (overview of items extracted for each included study)
- • table 1. csv and table 1_long.csv (Table A1 in csv format, the long version includes extra data)
- • table 1_long_updated.csv (LSR update for Table A1 in csv format, the long version includes extra data)
- • included.ris and background.ris (literature references from base review)
Harvard Dataverse: Available datasets for SR automation. https://doi.org/10.7910/DVN/0XTV25 . 128
- • Datasets shared by authors of the included publications
Data are available under the terms of the Creative Commons Zero “No rights reserved” data waiver (CC0 1.0 Public domain dedication).
Extended data
Open Science Framework: Data Extraction Methods for Systematic Review (semi)Automation: A Living Review Protocol. https://doi.org/10.17605/OSF.IO/ECB3T . 15
This project contains the following extended data:
- • Review protocol
- • Additional_Fields.docx (overview of data fields of interest for text mining in clinical trials)
- • Search.docx (additional information about the searches, including full search strategies)
- • PRISMA P checklist for ‘Data extraction methods for systematic review (semi)automation: A living review protocol.’
Data are available under the terms of the Creative Commons Attribution 4.0 International license (CC-BY 4.0).
Reporting guidelines
Harvard Dataverse: PRISMA checklist for ‘Data extraction methods for systematic review (semi)automation: A living systematic review’ https://doi.org/10.7910/DVN/LNGCOQ . 127
Software availability
The development version of the software for automated searching is available from Github: https://github.com/mcguinlu/COVID_suicide_living .
Archived source code at time of publication: http://doi.org/10.5281/zenodo.3871366 . 17
License: MIT
Version Changes
Updated. changes from version 1.
This version of the LSR includes 23 new papers, a change in the title indicates that the current version is an update. Ailbhe Finnerty and Rebecca Elmore joined the author team after contributing to screening and data extraction; Luke A. McGuinness contributed to the base-review but is not listed as an author in this update. The abstract and conclusions were updated to reflect changes and new research trends such as increased availability of datasets, source code, more papers describing relation extraction and summarisation. We updated existing figures and tables with the exception of Table 1(pre-processing techniques), because reliance on pre-processing has decreased in recent years. Table 1 in the appendix was renamed as ‘Table A1’ to avoid confusion with Table 1 in the main text. In the base-review we assessed the included publications based on a list of 17 items in the domains of reproducibility (3.4.1), transparency (3.4.2), description of testing (3.4.3), data availability (3.4.4), and internal and external validity (3.4.5). The list of items was reduced to six items for the update, more information about the removed items can be found in the methods section of this LSR. We still include the following items:
- 3.4.2.2 Is there a description of the dataset used and of its characteristics?
- 3.4.2.4 Is the source code available?
- 3.4.3.2 Are basic metrics reported (true/false positives and negatives)?
- 3.4.4.1 Can we obtain a runnable version of the software based on the information in the publication?
- 3.4.4.2 Persistence: Can data be retrieved based on the information given in the publication?
- 3.4.5.1 Does the dataset or assessment measure provide a possibility to compare to other tools in the same domain?
Additionally, spreadsheets with all extracted data and updated figures are available as Appendix D.
Background: The reliable and usable (semi)automation of data extraction can support the field of systematic review by reducing the workload required to gather information about the conduct and results of the included studies. This living systematic review examines published approaches for data extraction from reports of clinical studies.
Methods: We systematically and continually search PubMed, ACL Anthology, arXiv, OpenAlex via EPPI-Reviewer, and the dblp computer science bibliography . Full text screening and data extraction are conducted within an open-source living systematic review application created for the purpose of this review. This living review update includes publications up to December 2022 and OpenAlex content up to March 2023.
Results: 76 publications are included in this review. Of these, 64 (84%) of the publications addressed extraction of data from abstracts, while 19 (25%) used full texts. A total of 71 (93%) publications developed classifiers for randomised controlled trials. Over 30 entities were extracted, with PICOs (population, intervention, comparator, outcome) being the most frequently extracted. Data are available from 25 (33%), and code from 30 (39%) publications. Six (8%) implemented publicly available tools
Conclusions: This living systematic review presents an overview of (semi)automated data-extraction literature of interest to different types of literature review. We identified a broad evidence base of publications describing data extraction for interventional reviews and a small number of publications extracting epidemiological or diagnostic accuracy data. Between review updates, trends for sharing data and code increased strongly: in the base-review, data and code were available for 13 and 19% respectively, these numbers increased to 78 and 87% within the 23 new publications. Compared with the base-review, we observed another research trend, away from straightforward data extraction and towards additionally extracting relations between entities or automatic text summarisation. With this living review we aim to review the literature continually.
1. Introduction
In a systematic review, data extraction is the process of capturing key characteristics of studies in structured and standardised form based on information in journal articles and reports. It is a necessary precursor to assessing the risk of bias in individual studies and synthesising their findings. Interventional, diagnostic, or prognostic systematic reviews routinely extract information from a specific set of fields that can be predefined. 1 The most common fields for extraction in interventional reviews are defined in the PICO framework (population, intervention, comparison, outcome) and similar frameworks are available for other review types. The data extraction task can be time-consuming and repetitive when done by hand. This creates opportunities for support through intelligent software, which identify and extract information automatically. When applied to the field of health research, this (semi) automation sits at the interface between evidence-based medicine (EBM) and data science, and as described in the following section, interest in its development has grown in parallel with interest in AI in other areas of computer science.
1.1. Related systematic reviews and overviews
This review is, to the best of our knowledge, the only living systematic review (LSR) of data extraction methods. We identified four previous reviews of tools and methods in the first iteration of this living review (called base-review hereafter), 2 – 5 and two documents providing overviews and guidelines relevant to our topic. 3 , 6 , 7 Between base-review and this update, we identified six more related (systematic) literature reviews that will be summarised in the following paragraphs. 8 – 13
Related reviews up to 2014: The systematic reviews from 2014 to 2015 present an overview of classical machine learning and natural language processing (NLP) methods applied to tasks such as data mining in the field of evidence-based medicine. At the time of publication of these documents, methods such as topic modelling (Latent Dirichlet Allocation) and support vector machines (SVM) were considered state-of-the art for language models.
In 2014, Tsafnat et al. provided a broad overview on automation technologies for different stages of authoring a systematic review. 5 O’Mara-Eves et al . published a systematic review focusing on text-mining approaches in 2015. 4 It includes a summary of methods for the evaluation of systems, such as recall, accuracy, and F1 score (the harmonic mean of recall and precision, a metric frequently used in machine-learning). The reviewers focused on tasks related to PICO classification and supporting the screening process. In the same year, Jonnalagadda, Goyal and Huffman 3 described methods for data extraction, focusing on PICOs and related fields. The age of these publications means that the latest static or contextual embedding-based and neural methods are not included. These newer methods, 14 however, are used in contemporary systematic review automation software which will be reviewed in the scope of this living review.
Related reviews up to 2020: Reviews up to 2020 focus on discussions around tool development and integration in practice, and mark the starting date of the inclusion of automation methods based on neural networks. Beller et al. describe principles for development and integration of tools for systematic review automation. 6 Marshall and Wallace 7 present a guide to automation technology, with a focus on availability of tools and adoption into practice. They conclude that tools facilitating screening are widely accessible and usable, while data extraction tools are still at piloting stages or require a higher amount of human input.
A systematic review of machine-learning for systematic review automation, published in Portuguese in 2020, included 35 publications. The authors examined journals in which publications about systematic review automation are published, and conducted a term-frequency and citation analysis. They categorised papers by systematic review task, and provided a brief overview of data extraction methods. 2
Related reviews after 2020: These six reviews include and discuss end-user tools and cover different tasks across the SR workflow, including data extraction. Compared with this LSR, these reviews are broader in scope but have less included references on the automation of data extraction. Ruiz and Duffy 10 did a literature and trend analysis showing that the number of published references about SR automation is steadily increasing. Sundaram and Berleant 11 analyse 29 references applying text mining to different parts of the SR process and note that 24 references describe automation in study selection while research gaps are most prominent for data extraction, monitoring, quality assessment, and synthesis. 11 Khalil et al. 9 include 47 tools and descriptions of validation studies in a scoping review, of which 8 are available end-user tools that mostly focus on screening, but also cover data extraction and risk of bias assessments. They discuss limitations of tools such as lack of generalisability, integration, funding, and limited performance or access. 9 Cierco Jimenez et al. 8 included 63 references in a mapping review of machine-learning to assist SRs during different workflow steps, of which 41 were available end-user tools for use by researchers without informatics background. In accordance with other reviews they describe screening as the most frequently automated step, while automated data extraction tools are lacking due to the complexity of the task. Zhang et al. 12 included 49 references on automation of data extraction fields such as diseases, outcomes, or metadata. They focussed on extraction from traditional Chinese medicine texts such as published clinical trial texts, health records, or ancient literature. 12 Schmidt et al. 13 published a narrative review of tools with a focus on living systematic review automation. They discuss tools that automate or support the constant literature retrieval that is the hallmark of LSRs, while well-integrated (semi) automation of data extraction and automatic dissemination or visualisation of results between official review updates is supported by some, but less common.
We aim to review published methods and tools aimed at automating or (semi) automating the process of data extraction in the context of a systematic review of medical research studies. We will do this in the form of a living systematic review, keeping information up to date and relevant to the challenges faced by systematic reviewers at any time.
Our objectives in reviewing this literature are two-fold. First, we want to examine the methods and tools from the data science perspective, seeking to reduce duplicate efforts, summarise current knowledge, and encourage comparability of published methods. Second, we seek to highlight the added value of the methods and tools from the perspective of systematic reviewers who wish to use (semi) automation for data extraction, i.e., what is the extent of automation? Is it reliable? We address these issues by summarising important caveats discussed in the literature, as well as factors that facilitate the adoption of tools in practice.
2.1. Registration/protocol
This review was conducted following a preregistered and published protocol. 15 PROSPERO was initially considered as platform for registration, but it is limited to reviews with health-related outcomes. Any deviations from the protocol have been described below.
2.2. Living review methodology
We are conducting a living review because the field of systematic review (semi) automation is evolving rapidly along with advances in language processing, machine-learning and deep-learning.
The process of updating started as described in the protocol 15 and is shown in Figure 1 . In short, we will continuously update the literature search results, using the search strategies and methods described in the section ‘Search’ below. PubMed and arXiv search results are updated daily in a completely automated fashion via APIs. Articles from the dblp, ACL, and OpenAlex via EPPI-Reviewer are added every two months. All search results are automatically imported to our living review screening and data extraction web-application, which is described in the section ‘Data collection and analysis’ below.

This image is reproduced under the terms of a Creative Commons Attribution 4.0 International license (CC-BY 4.0) from Schmidt et al. 15
The decision for full review updates is made every six months based on the number of new publications added to the review. For more details about this, please refer to the protocol or to the Cochrane living systematic review guidance . In between updates, the screening process and current state of the data extraction is visible via the living review website .
2.3. Eligibility criteria
- • We included full text publications that describe an original NLP approach for extracting data related to systematic reviewing tasks. Data fields of interest (referred to here as entities or as sentences) were adapted from the Cochrane Handbook for Systematic Reviews of Interventions, 1 and are defined in the protocol. 15 We included the full range of NLP methods (e.g., regular expressions, rule-based systems, machine learning, and deep neural networks).
- • Publications must describe a full cycle of the implementation and evaluation of a method. For example, they must report training and at least one measure of evaluating the performance of a data extraction algorithm.
- • We included reports published from 2005 until the present day, similar to previous work. 3 We would have translated non-English reports, had we found any.
- • The data that the included publications use for mining must be texts from randomised controlled trials, comparative cohort studies, case control studies or comparative cross-sectional studies (e.g., for diagnostic test accuracy). The scope of data extraction methods can be applied to the full text or to abstracts within each eligible publication’s corpus. We included publications that extracted data from other study types, as long as at least one of our study types of interest was contained in the corpus.
We excluded publications reporting:
- • Methods and tools related solely to image processing and importing biomedical data from PDF files without any NLP approach, including data extraction from graphs.
- • Any research that focuses exclusively on protocol preparation, synthesis of already extracted data, write-up, solely the pre-processing of text or its dissemination.
- • Methods or tools that provided no natural language processing approach and offered only organisational interfaces, document management, databases, or version control
- • Any publications related to electronic health reports or mining genetic data.
2.4. Search
Base-review: We searched five electronic databases, using the search methods previously described in our protocol. 15 In short, we searched MEDLINE via Ovid, using a search strategy developed with the help of an information specialist, and searched Web of Science Core Collection and IEEE using adaptations of this strategy, which were made by the review authors. Searches on the arXiv (computer science) and dblp were conducted on full database dumps using the search functionality described by McGuinness and Schmidt. 16 The full search results and further information about document retrieval are available in Underlying data: Appendix A and B. 127
Originally, we planned to include a full literature search from the Web of Science Core Collection. Due to the large number of publications retrieved via this search (n = 7822) we decided to first screen publications from all other sources, to train a machine-learning ensemble classifier, and to only add publications that were predicted as relevant for our living review. This reduced the Web of Science Core Collection publications to 547 abstracts, which were added to the studies in the initial screening step. The dataset, code and weights of trained models are available in Underlying data: Appendix C. 127 This includes plots of each model’s evaluation in terms of area under the curve (AUC), accuracy, F1, recall, and variance of cross-validation results for every metric.
Update: As planned, we changed to the PubMed API for searching MEDLINE. This decision was made to facilitate continuous reference retrieval. We searched only for pre-print or published literature and therefore did not search sources such as GITHUB or other source code repositories.
Update: We searched PubMed via its API, arXiv (computer science), ACL-Anthology, dblp, and used EPPI-Reviewer to collect citations from MicrosoftAcademic and later OpenAlex using the ‘Bi-Citation AND Recommendations’ method.
2.5. Data collection and analysis
2.5.1 Selection of studies
Initial screening and data extraction were conducted as stated in the protocol. In short, for the base-review we screened all retrieved publications using the Abstrackr tool. All abstracts were screened by two independent reviewers. Conflicting judgements were resolved by the authors who made the initial screening decisions. Full texts screening was conducted in a similar manner to abstract screening but used our web application for LSRs described in the following section.
For the updated review we used our living review web application to retrieve all publications with the exception of the items retrieved by EPPI-Reviewer (these are added to the dataset separately). We further used our application to de-duplicate, screen, and data-extract all publications.
A methodological update to the screening process included a change to single-screening to assess eligibility on both abstract and full-text level, reducing dual-screening to 10% of the publications.
2.5.2 Data extraction, assessment, and management
We previously developed a web application to automate reference retrieval for living review updates (see Software availability 17 ), to support both abstract and full text screening for review updates, and to manage the data extraction process throughout. 17 For future updates of this living review we will use the web application, and not Abstrackr, for screening references. This web application is already in use by another living review. 18 It automates daily reference retrieval from the included sources and has a screening and data extraction interface. All extracted data are stored in a database. Figures and tables can be exported on a daily basis and the progress in between review updates is shared on our living review website. The full spreadsheet of items extracted from each included reference is available in the Underlying data. 127 As previously described in the protocol, quality of reporting and reproducibility was initially assessed based on a previously published checklist for reproducibility in text mining, but some of the items were removed from the scope of this review update. 19
As planned in the protocol, a single reviewer conducted data extraction, and a random 10% of the included publications were checked by a second reviewer.
2.5.3 Visualisation
The creation of all figures and interactive plots on the living review website and in this review’s ‘Results’ section was automated based on structured content from our living review database (see Appendix A and D, Underlying data 127 ). We automated the export of PDF reports for each included publication. Calculation of percentages, export of extracted text, and creation of figures was also automated.
2.5.4 Accessibility of data
All data and code are free to access. A detailed list of sources is given in the ‘Data availability’ and ‘Software availability’ sections.
2.6. Changes from protocol and between updates
In the protocol we stated that data would be available via an OSF repository. Instead, the full review data are available via the Harvard Dataverse, as this repository allows us to keep an assigned DOI after updating the repository with new content for each iteration of this living review. We also stated that we would screen all publications from the Web of Science search. Instead, we describe a changed approach in the Methods section, under ‘Search’. For review updates, Web of Science was dropped and replaced with OpenAlex searches via EPPI-Reviewer.
We added a data extraction item for the type of information which a publication mines (e.g. P, IC, O) into the section of primary items of interest, and we moved the type of input and output format from primary to secondary items of interest. We grouped the secondary item of interest ‘Other reported metrics, such as impacts on systematic review processes (e.g., time saved during data extraction)’ with the primary item of interest ‘Reported performance metrics used for evaluation’.
The item ‘Persistence: is the dataset likely to be available for future use?’ was changed to: ‘Can data be retrieved based on the information given in the publication?’. We decided not to speculate if a dataset is likely to be available in the future and chose instead to record if the dataset was available at the time when we tried to access it.
The item ‘Can we obtain a runnable version of the software based on the information in the publication?’ was changed to ‘Is an app available that does the data mining, e.g. a web-app or desktop version?’.
In this current version of the review we did not yet contact the authors of the included publications. This decision was made due to time constraints, however reaching out to authors is planned as part of the first update to this living review.
In the base-review we assessed the included publications based on a list of 17 items in the domains of reproducibility (3.4.1), transparency (3.4.2), description of testing (3.4.3), data availability (3.4.4), and internal and external validity (3.4.5). The list of items was reduced to six items for the update:
- • 3.4.2.2 Is there a description of the dataset used and of its characteristics?
- • 3.4.2.4 Is the source code available?
- • 3.4.3.2 Are basic metrics reported (true/false positives and negatives)?
- • 3.4.4.1 Can we obtain a runnable version of the software based on the information in the publication?
- • 3.4.4.2 Persistence: Can data be retrieved based on the information given in the publication?
- • 3.4.5.1 Does the dataset or assessment measure provide a possibility to compare to other tools in the same domain?
The following items were removed, although the results and discussion from the assessment of these items in the base-review remains within the review text:
- • 3.4.1.1 Are the sources for training/testing data reported?
- • 3.4.1.2 If pre-processing techniques were applied to the data, are they described?
- • 3.4.2.1 Is there a description of the algorithms used?
- • 3.4.2.3 Is there a description of the hardware used?
- • 3.4.3.1 Is there a justification/an explanation of the model assessment?
- • 3.4.3.3 Does the assessment include any information about trade-offs between recall or precision (also known as sensitivity and positive predictive value)?
- • 3.4.4.3 Is the use of third-party frameworks reported and are they accessible?
- • 3.4.5.2 Are explanations for the influence of both visible and hidden variables in the dataset given?
- • 3.4.5.3 Is the process of avoiding overfitting or underfitting described?
- • 3.4.5.4 Is the process of splitting training from validation data described?
- • 3.4.5.5 Is the model’s adaptability to different formats and/or environments beyond training and testing data described?
3.1. Results of the search
Our database searches identified 10,107 publications after duplicates were removed (see Figure 2 ). We identified one more publication manually.

This iteration of the living review includes 76 publications, summarised in Table A1 in Underlying data 127 ).
3.1.1 Excluded publications
Across the base-review and the update, 216 publications were excluded at the full text screening stage, with the most common reason for exclusion being that it did not fit target entities or target data. In most cases, this was due to the text-types mined in the publications. Electronic health records and non-trial data were common, and we created a list of datasets that would be excluded in this category (see more information in Underlying data: Appendix B 127 ). Some publications addressed the right kind of text but were excluded for not mining data of interest to this review. For example, Norman, Leeflang and Névéol 23 performed data extraction for diagnostic test accuracy reviews, but focused on extracting the results and data for statistical analyses. Millard, Flach and Higgins 24 and Marshall, Kuiper and Wallace 25 looked at risk of bias classification, which is beyond the scope of this review. Boudin, Nie and Dawes 26 developed a weighing scheme based on an analysis of PICO element locations, leaving the detection of single PICO elements for future work. Luo et al . 27 extracted data from clinical trial registrations but focused on parsing inclusion criteria into event or temporal entities to aid participant selection for randomised controlled trials (RCTs).
The second most common reason for study exclusion was that they had ‘no original data extraction approach’. Rathbone et al ., 28 for example, used hand-crafted Boolean searches specific to a systematic review’s PICO criteria to support the screening process of a review within Endnote. We classified this article as not having any original data extraction approach because it does not create any structured outputs specific to P, IC, or O. Malheiros et al. 29 performed visual text mining, supporting systematic review authors by document clustering and text highlighting. Similarly, Fabbri et al. 30 implemented a tool that supports the whole systematic review workflow, from protocol to data extraction, performing clustering and identification of similar publications. Other systematic reviewing tasks that can benefit from automation but were excluded from this review are listed in Underlying data: Appendix B. 127
3.2. Results from the data extraction: Primary items of interest
3.2.1 Automation approaches used
Figure 3 shows aspects of the system architectures implemented in the included publications. A short summary of these for each publication is provided in Table A1 in Underlying data. 127 Where possible, we tried to break down larger system architectures into smaller components. For example, an architecture combining a word embedding + long short-term memory (LSTM) network would have been broken down into the two respective sub-components. We grouped binary classifiers, such as naïve Bayes and logistic regression. Although SVM is also binary classifier, it was assigned as separate category due to its popularity. The final categories are a mixture of non-machine-leaning automation (application programming interface (API) and metadata retrieval, PDF extraction, rule-base), classic machine-learning (naïve Bayes, decision trees, SVM, or other binary classifiers) and neural or deep-learning approaches (convolutional neural network (CNN), LSTM, transformers, or word embeddings). This figure shows that there is no obvious choice of system architecture for this task. For the LSR update, the strongest trend was the increasing application of BERT (Bidirectional Encoder Representations from Transformers). BERT was published in 2018 and other architecturally-identical versions of it tailored to using scientific text, such as SciBERT, are summarised under the same category in this review. 14 , 31 In the base-review, BERT was used three times, whilst now appearing 21 times. Other transformer-based architectures such as the bio-pretrained version of ELECTRA, are also gaining attention, 32 , 33 as well as FLAIR-based models. 34 – 36

Results are divided into different categories of machine-learning and natural language processing approaches and coloured by the year of publication. More than one architecture component per publication is possible. Where API, application programming interface; BERT, bidirectional encoder representations from Transformers; CNN, convolutional neural network; CRF, conditional random fields; LSTM, long short-term memory; PICO, population, intervention, comparison, outcome; RNN, recurrent neural networks; SVM, support vector machines.
Rule-bases, including approaches using heuristics, wordlists, and regular expressions, were one of the earliest techniques used for data extraction in EBM literature. It remains the most frequently used approaches to automation. Nine publications (12%) use rule-bases alone, while the rest of the publications use them in combination with other classifiers (data shown in Underlying data: Appendix A and D 127 ). Although used more frequently in the past, the 11 publications published between 2017 and now that use this approach alongside other architectures such as BERT, 33 , 37 – 39 conditional random fields (CRF), 40 use it with SVM 41 or other binary classifiers. 42 In practice, these systems use rule-bases in the form of hand-crafted lists to identify candidate phrases for amount entities such as sample size 42 , 43 or to refine a result obtained by a machine-learning classifier on the entity level (e.g., instances where a specific intervention or outcome is extracted from a sentence). 40
Binary classifiers, most notably naïve Bayes and SVMs, are also frequently used system components in the data extraction literature. They are frequently used in studies published between 2005 and now but their usage started declining with the advent of neural models.
Embedding and neural architectures are increasingly being used in literature over the past seven years. Recurrent neural networks (RNN), CNN, and LSTM networks require larger amounts of training data; by using transformer-based embeddings with pre-training algorithms based on unlabelled data they have become increasingly more interesting in fields such as data extraction for EBM- where high-quality training data are difficult and expensive to obtain.
In the ‘Other’ category, tools mentioned were mostly other classifiers such as maximum entropy classifiers (n = 3), kLog, J48, and various position or document-length classification algorithms. We also added methods such as supervised distant supervision (n = 3, see Ref. 44 ) and novel training approaches to existing neural architectures in this category.
3.2.2 Reported performance metrics used for evaluation
Precision (i.e., positive predictive value), recall (i.e., sensitivity), and F1 score (harmonic mean of precision and recall) are the most widely used metrics for evaluating classifiers. This is reflected in Figure 4 , which shows that at least one of these metrics was used in the majority of the included publications. Accuracy and area under the curve - receiver operator characteristics (AUC-ROC) were less frequently used.

More than one metric per publication is possible, which means that the total number of included publications (n = 76) is lower than the sum of counts of the bars within this figure. AUC-ROC, area under the curve - receiver operator characteristics; F1, harmonic mean of precision and recall.
There were several approaches and justifications of using macro- or micro-averaged precision, recall, or F1 scores in the included publications. Micro or macro scores are computed in multi-class cases, and the final scores can differ whenever the classes in a dataset are imbalanced (as is the case in most datasets used for automating data extraction in SR automation).
Both micro and macro scores were reported by Singh et al. (2021), 45 Kilicoglu et al. (2021), 38 Kiritchenko et al. (2010), 46 Fiszman et al. (2007) 47 whereas Karystianis et al. (2014, 2017) 48 , 49 reported micro across documents, and macro across the classes.
Macro-scores were used in one publication. 37
Micro scores were used by Fiszman et al. 47 for class-level results. In one publication harmonic mean was used for precision and recall, while micro-scoring was used for F1. 50 Micro scores were most widely used, including Al-Hussaini et al. (2022), 32 Sanchez-Graillet et al. (2022), 51 Kim et al. (2011), 52 Verbeke et al. (2012), 53 and Jin and Szolovits (2020) 54 were used in the evaluation script of Nye et al. (2018). 55
In the category ‘Other’ we added several instances where a relaxation of a metric was introduced, e.g., precision using top-n classified sentences 44 , 46 , 56 or mean average precision and the metric ‘precision @rank 10’ for sentence ranking exercises. 57 , 58 Another type of relaxation for standard metrics is a distance relaxation when normalising entities into concepts in medical subject headings (MesH) or unified medical language system (UMLS), to allow N hops between predicted and target concepts. 59
The LSR update showed an increasing trend of text summarisation and relation extraction algorithms. ROGUE, ∆EI, or Jaccard similarity were metrics for summarisation. 60 , 61 For relation extraction F1, precision, and recall remained the most common metrics. 62 , 63
Other metrics were kappa, 58 random shuffling 64 or binomial proportion test 65 to test statistical significance, given with confidence intervals. 41 Further metrics included under ‘Other’ were odds ratios, 66 normalised discounted cumulative gain, 44 , 67 ‘sentences needed to screen per article’ in order to find one relevant sentence, 68 McNemar test, 65 C-statistic (with 95% CI) and Brier score (with 95% CI). 69 Barnett (2022) 70 extracted sample sizes and reported the mean difference between true and extracted numbers.
Real-life evaluations, such as the percentage of outputs needing human correction, or time saved per article, were reported by two publications, 32 , 46 and an evaluation as part of a wider screening system was done in another. 71
3.2.3 Type of data
3.2.3.1 Scope and data
Most data extraction is carried out on abstracts (See Table A1 in Underlying data , 127 and the supplementary table giving an overview of all included publications). Abstracts are the most practical choice, due to the possibility of exporting them along with literature search results from databases such as MEDLINE. In total, 84% (N=64) of the included publications directly reported using abstracts. Within the 19 references (25%) that reported usage of full texts, eight specifically mentioned that this also included abstracts but it is unclear if all full texts included abstract text. Descriptions of the benefits of using full texts for data extraction include having access to a more complete dataset, while the benefits of using titles (N=4, 5%) include lower complexity for the data extraction task. 43 Xu et al. (2010) 72 exclusively used titles, while the other three publications that specifically mentioned titles also used abstracts in their datasets. 43 , 73 , 74
Figure 5 shows that RCTs are the most common study design texts used for data extraction in the included publications (see also extended Table A1 in Underlying data 127 ). This is not surprising, because systematic reviews of interventions are the most common type of systematic review, and they are usually focusing on evidence from RCTs. Therefore, the literature for automation of data extraction focuses on RCTs, and their related PICO elements. Systematic reviews of diagnostic test accuracy are less frequent, and only one included publication specifically focused on text and entities related to these studies, 75 while two mentioned diagnostic procedures among other fields of interest. 35 , 76 Eight publications focused on extracting data specifically from epidemiology research, non-randomised interventional studies, or included text from cohort studies as well as RCT text. 48 , 49 , 61 , 72 – 74 , 76 , 77 More publications mining data from surveys, animal RCTs, or case series might have been found if our search and review had concentrated on these types of texts.

Commonly, randomized controlled trials (RCT) text was at least one of the target text types used in the included publications.
3.2.3.2 Data extraction targets
Mining P, IC, and O elements is the most common task performed in the literature of systematic review (semi-) automation (see Table A1 in Underlying data , 127 and Figure 6 ). In the base-review, P was the most common entity. After the LSR update, O (n=52, 68%) has become the most popular, due to the emerging trend of relation-extraction models that focus on the relationship between O and I entities and therefore may omit the automatic extraction of P. Some of the less-frequent data extraction targets in the literature can be categorised as sub-classes of a PICO, 55 for example, by annotating hierarchically multiple entity types such as health condition, age, and gender under the P class. The entity type ‘P (Condition and disease)’, was the most common entity closely related to the P class, appearing in twelve included publications, of which four were published in 2021 or later. 35 , 36 , 51 , 55 , 63 , 71 , 75 , 76 , 78 – 81

More than one entity type per publication is common, which means that the total number of included publications (n = 76) is lower than the sum of counts within this figure. P, population; I, intervention; C, comparison; O, outcome.
Notably, eleven publications annotated or worked with datasets that differentiated between intervention and control arms, four of these published after 2020 with a trend towards relation extraction and summarisation tasks requiring this type of data. 46 , 47 , 51 , 56 , 62 , 63 , 66 , 82 – 84 Usually, I and C are merged (n=47). Most data extraction approaches focused on recognising instances of entity or sentence classes, and a small number of publications went one step further to normalise to actual concepts and including data sources such as UMLS (Unified Medical Language System). 35 , 39 , 59 , 73 , 85
The ‘Other’ category includes some more detailed drug annotations 65 or information such as confounders 49 and other entity types (see the full dataset in Underlying data: Appendix A and D for more information 127 ).
3.3. Results from the data extraction: Secondary items of interest
3.3.1 Granularity of data extraction
A total of 54 publications (71%) extracted at least one type of information at the entity level, while 46 publications (60%) used sentence level (see Table A1 extended version in Underlying data 127 ). We defined the entity level as any number of words that is shorter than a whole sentence, e.g., noun-phrases or other chunked text. Data types such as P, IC, or O commonly appeared to be extracted on both entity and sentence level, whereas ‘N’, the number of people participating in a study, was commonly extracted on entity level only.
3.3.2 Type of input
The majority of publications and benchmark corpora mentioned MEDLINE, via PubMed, as the data source for text. Text files (n = 64), next to XML (n = 8), or HTML (n = 3), are the most common format of the data downloaded from these sources. Therefore, most systems described using, or were assumed to use, text files as input data. Eight included publications described using PDF files as input. 44 , 46 , 59 , 68 , 75 , 81 , 86 , 87
3.3.3 Type of output
A limited number of publications described structured summaries as output of their extracted data (n = 14, increasing trend between LSR updates). Alternatives to exporting structured summaries were JSON (n = 4), XML, and HTML (n = 2 each). Two publications mentioned structured data outputs in the form of an ontology. 51 , 88 Most publications mentioned only classification scores without specifying an output type. In these cases, we assumed that the output would be saved as text files, for example as entity span annotations or lists of sentences (n = 55).
3.4. Assessment of the quality of reporting
In the base-review we used a list of 17 items to investigate reproducibility, transparency, description of testing, data availability, and internal and external validity of the approaches in each publication. The maximum and minimum number of items that were positively rated were 16 and 1, respectively, with a median of 10 (see Table A1 in Underlying data 127 ). Scores were added up and calculated based on the data provided in Appendix A and D (see Underlying data 127 ), using the sum and median functions integrated in Excel. Publications from recent years up to 2021 showed a trend towards more complete and clear reporting.
3.4.1 Reproducibility
3.4.1.1 Are the sources for training/testing data reported?
Of the included publications in the base-review, 50 out of 53 (94%) clearly stated the sources of their data used for training and evaluation. MEDLINE was the most popular source of data, with abstracts usually described as being retrieved via searches on PubMed, or full texts from PubMed Central. A small number of publications described using text from specific journals such as PLoS Clinical Trials, New England Journal of Medicine, The Lancet, or BMJ. 56 , 83 Texts and metadata from Cochrane, either provided in full or retrieved via PubMed, were used in five publications. 57 , 59 , 68 , 75 , 86 Corpora such as the ebm-nlp dataset, 55 or PubMed-PICO 54 are available for direct download. Publications published in recent years are increasingly reporting that they are using these benchmark datasets rather than creating and annotating their own corpora (see 4 for more details).
3.4.1.2 If pre-processing techniques were applied to the data, are they described?
Of the included publications in the base-review, 47 out of 53 (89%) reported processing the textual data before applying/training algorithms for data extraction. Different types of pre-processing, with representative examples for usage and implementation, are listed in Table 1 below.
After the publication of the base-review, transformer models such as BERT became dominant in the literature (see Figure 3 ). With their word-piece vocabulary, contextual embeddings, and self-supervised pre-training on large unlabelled corpora these models have essentially removed the need for most pre-processing beyond automatically-applied lower-casing. 14 , 31 We are therefore not going to update this table in this, or any future iterations of this LSR. We leave it for reference to publications that may still use these methods in the future.
3.4.2 Transparency of methods
3.4.2.1 Is there a description of the algorithms used?
Figure 7 shows that 43 out of 53 publications in the base-review (81%) provided descriptions of their data extraction algorithm. In the case of machine learning and neural networks, we looked for a description of hyperparameters and feature generation, and for the details of implementation (e.g. the machine-learning framework). Hyperparameters were rarely described in full, but if the framework (e.g., Scikit-learn, Mallet, or Weka) was given, in addition to a description of implementation and important parameters for each classifier, then we rated the algorithm as fully described. For rule-based methods we looked for a description of how rules were derived, and for a list of full or representative rules given as examples. Where multiple data extraction approaches were described, we gave a positive rating if the best-performing approach was described.

3.4.2.2 Is there a description of the dataset used and of its characteristics?
Of the included publications in the review update, 73 out of 76 (97%) provided descriptions of their dataset and its characteristics.
Most publications provided descriptions of the dataset(s) used for training and evaluation. The size of each dataset, as well as the frequencies of classes within the data, were transparent and described for most included publications. All dataset citations, along with a short description and availability of the data, are shown in Table 4 .
RCT, randomized controlled trials; IR, information retrieval; PICO, population, intervention, comparison, outcome; UMLS, unified medical language system.
3.4.2.3 Is there a description of the hardware used?
Most included publications in the base-review did not report their hardware specifications, though five publications (9%) did. One, for example, applied their system to new, unlabelled data and reported that classifying the whole of PubMed takes around 20 hours using a graphics processing unit (GPU). 69 In another example, the authors reported using Google Colab GPUs, along with estimates of computing time for different training settings. 95
3.4.2.4 Is the source code available?
Figure 8 shows that most of the included publications did not provide any source code, although there is a very strong trend towards better code-availabilty in the publications from the review update (n=19 published code, 83% of the new publications provided code). Publications that did provide the source code were exclusively published or last updated in the last seven years. GitHub is the most popular platform for making code accessible. Some publications also provided links to notebooks on Google Colab, which is a cloud-based platform to develop and execute code online. Two publications provided access to parts of the code, or access was restricted. A full list of code repositories from the included publications is available in Table 2 .

3.4.3 Testing
3.4.3.1 Is there a justification/an explanation of the model assessment?
Of the included publications in the base-review, 47 out of 53 (89%) gave a detailed assessment of their data extraction algorithms. We rated this item as negative if only the performance scores were given, i.e., if no error analysis was performed and no explanations or examples were given to illustrate model performance. In most publications a brief error analysis was common, for example discussions on representative examples for false negatives and false positives, 47 major error sources 90 or highlighting errors with respect to every entity class. 76 Both Refs. 52 , 53 used structured and unstructured abstracts, and therefore discussed the implications of unstructured text data for classification scores.
A small number of publications did a real-life assessment, where the data extraction algorithm was applied to different, unlabelled, and often much larger datasets or tested while conducting actual systematic reviews. 46 , 58 , 63 , 69 , 48 , 95 , 101 , 102
3.4.3.2 Are basic metrics reported (true/false positives and negatives)?
Figure 9 shows the extent to which all raw basic metrics, such as true-positives, were reported in the included publications in the LSR update. In most publications (n = 62) these basic metrics are not reported, and there is a trend between base-review and this update towards not reporting these. However, basic metrics could be obtained since the majority of new included publications made source code available and used publicly available datasets. When dealing with entity-level data extraction it can be challenging to define the quantity of true negative entities. This is true especially if entities are labelled and extracted based on text chunks, because there can be many combinations of phrases and tokens that constitute an entity. 47 This problem was solved in more recent publications by conducting a token-based evaluation that computes scores across every single token, hence gaining the ability to score partial matches for multi-word entities. 55

For each included paper. More than one selection is possible, which means that the total number of included publications (n=76) is lower than the sum of counts within this figure.
3.4.3.3 Does the assessment include any information about trade-offs between recall or precision (also known as sensitivity and positive predictive value)?
Of the included publications in the base-review, 17 out of 53 (32%) described trade-offs or provided plots or tables showing the development of evaluation scores if certain parameters were altered or relaxed. Recall (i.e., sensitivity) is often described as the most important metric for systematic review automation tasks, as it is a methodological demand that systematic reviews do not exclude any eligible data.
References 56 and 76 showed how the decision of extracting the top two or N predictions impacts the evaluation scores, for example precision or recall. Reference 102 shows precision-recall plots for different classification thresholds. Reference 72 shows four cut-offs, whereas Ref. 95 shows different probability thresholds for their classifier, and describe the impacts of this on precision, recall, and F1 curves.
Some machine-learning architectures need to convert text into features before performing classification. A feature can be, for example, the number of times that a certain word occurs, or the length of an abstract. The number of features used, e.g. for CRF algorithms, which was given in multiple publications, 92 together with a discussion of classifiers that should be used in high recall is needed. 42 , 103 show ROC curves quantifying the amount of training data and its impact on the scores.
3.4.4 Availability of the final model or tool
3.4.4.1 Can we obtain a runnable version of the software based on the information in the publication?
Compiling and testing code from every publication is outside the scope of this review. Instead, in Figure 10 and Table 3 we recorded the publications where a (web) interface or finished application was available. Counting RobotReviewer and Trialstreamer as separate projects, 12% of the included publications had an application associated with it, but only 5 (6%) are available and directly usable via web-apps. Applications were available as open-source, completely free, or free basic versions with optional features that can be purchased or subscribed to.

3.4.4.2 Persistence: Can data be retrieved based on the information given in the publication?
We observed an increasing trend of dataset availability and publications re-using benchmark corpora within the LSR update. Only seven of the included publications in the base-review (13%) made their datasets publicly available, out of the 36 unique corpora found then.
After the LSR update we accumulated 55 publications that describe unique new corpora. Of these, 23 corpora were available online and a total of 40 publication mentioned using one of these public benchmarking sets. Table 4 shows a summary of the corpora, their size, classes, links to the datasets, and cross-reference to known publications re-using each data set. For the base review, we collected the corpora, provide a central link to all datasets, and will add datasets as they become available during the life span of this living review (see Underlying data 127 , 128 below). Due to the increased number of available corpora we stopped downloading the data and provide links instead. When a dataset is made freely available without barriers (i.e., direct downloads of text and labels), then any researcher can re-use the data and publish results from different models, which become comparable to one another. Copyright issues surrounding data sharing were noted by Ref. 75 , therefore they shared the gold-standard annotations used as training or evaluation data and information on how to obtain the texts.
3.4.4.3 Is the use of third-party frameworks reported and are they accessible?
Of the included publications in the base-review, 47 out of 53 (88%) described using at least one third-party framework for their data extraction systems. The following list is likely to be incomplete, due to non-available code and incomplete reporting in the included publications. Most commonly, there was a description of machine-learning toolkits (Mallet, N = 12; Weka, N = 6; tensorflow, N = 5; scikit-learn, N = 3). Natural language processing toolkits such as Stanford parser/CoreNLP (N = 12) or NLTK (N = 3), were also commonly reported for the pre-processing and dependency parsing steps within publications. The MetaMap tool was used in nine publications, and the GENIA tagger in four. For the complete list of frameworks please see Appendix A and D in Underlying data. 127
3.4.5 Internal and external validity of the model
3.4.5.1 Does the dataset or assessment measure provide a possibility to compare to other tools in the same domain?
With this item we aimed to assess publications to see if the evaluation results from models are comparable with the results from other models. Ideally, a publication would have reported the results of another classification model on the same dataset, either by re-implementing the model themselves 96 or by describing results of other models when using benchmark datasets. 64 This was rarely the case for the publications in the base-review, as most datasets were curated and used in single publications only. However, the re-use of benchmark corpora increased with the publications in the LSR update, where we found 40 publications that report results on one of the previously published benchmark datasets (see Table 4 ).
Addtionally, in the base-review, in 40 publications (75%) data were well described, and they utilised commonly used entities and common assessment metrics, such as precision, recall, and F1-scores, leading to a limited comparability of results. In these cases, the comparability is limited because those publications used different data sets, which can influence the difficulty of the data extraction task and lead to better results within for example structured datasets or topic-specific datasets.
3.4.5.2 Are explanations for the influence of both visible and hidden variables in the dataset given?
This item relates only to publications using machine learning or neural networks. Rule-based classification systems (N = 8, 15% reporting rule-base as sole approach) are not applicable to this item, because the rules leading to decisions are intentionally chosen by the creators of the system and are therefore always visible.
Ten publications in the base-review (19%) discussed hidden variables. 83 discussed that the identification of the treatment group entity yielded the best results. However, when neither the words ‘group’ nor ‘arm’ were present in the text then the system had problems with identifying the entity. ‘Trigger tokens’ 104 and the influence of common phrases were also described by Ref. 68 , the latter showed that their system was able to yield some positive classifications in the absence of common phrases. 103 went a step further and provided a table with words that had the most impact on the prediction of each class. 57 describes removing sentence headings in structured abstracts in order to avoid creating a system biased towards common terms, while Ref. 90 discussed abbreviations and grammar as factors influencing the results. Length of input text 59 and position of a sentence within a paragraph or abstract, e.g. up to 10% lower classification scores for certain sentence combinations in unstructured abstracts, were shown in several publications. 46 , 66 , 102
3.4.5.3 Is the process of avoiding overfitting or underfitting described?
‘Overfitted’ is a term used to describe a system that shows particularly good evaluation results on a specific dataset because it has learned to classify noise and other intrinsic variations in the data as part of its model. 105
Of the included publications in the base-review, 33 out of 53 (62%) reported that they used methods to avoid overfitting. Eight (15%) of all publications reported rule-based classification as their only approach, allowing them to not be susceptible to overfitting by machine learning.
Furthermore, 28 publications reported cross-validation to avoid overfitting. Mostly these classifiers were in the domain of machine-learning, e.g. SVMs. Most commonly, 10 folds were used (N = 15), but depending on the size of evaluation corpora, 3, 6, 5 or 15 folds were also described. Two publications 55 , 85 cautioned that cross-validation with a high amount of folds (e.g. 10) causes high variance in evaluation results when using small datasets such as NICTA-PIBOSO. One publication 104 stratified folds by class in order to avoid this variance in evaluation results in a fold which is caused by a sparsity of positive instances.
Publications in the neural and deep-learning domain described approaches such as early stopping, dropout, L2-regularisation, or weight decay. 59 , 96 , 106 Some publications did not specifically discuss overfitting in the text, but their open-source code indicated that the latter techniques were used. 55 , 75
3.4.5.4 Is the process of splitting training from validation data described?
Random allocation to treatment groups is an important item when assessing bias in RCTs, because selective allocation can lead to baseline differences. 1 Similarly the process of splitting a dataset randomly, or in a stratified manner, into training (or rule-crafting) and test data is important when constructing classifiers and intelligent systems. 117
All included publications in the base-review gave indications of how different train and evaluation datasets were obtained. Most commonly there was one dataset and the splitting ratio which indicated that splits were random. This information was provided in 36 publications (68%).
For publications mentioning cross-validation (N = 28, 53%) we assumed that splits were random. The ratio of splitting (e.g. 80:20 for training and test data) was clear in the cross-validation cases and was described in the remainder of publications.
It was also common for publications to use completely different datasets, or multiple iterations of splitting, training and testing (N = 13, 24%). For example Ref. 56 used cross-validation to train and evaluate their model, and then used an additional corpus after the cross-validation process. Similarly Ref. 59 , used 60:40 train/test splits, but then created an additional corpus of 88 documents to further validate the model’s performance on previously unseen data.
3.4.5.5 Is the model’s adaptability to different formats and/or environments beyond training and testing data described?
For this item we aimed to find out how many of the included publications in the base-review tested their data extraction algorithms on different datasets. A limitation often noted in the literature was that gold-standard annotators have varying styles and preferences, and that datasets were small and limited to a specific literature search. Evaluating a model on multiple independent datasets provides the possibility of quantifying how well data can be extracted across domains and how flexible a model is in real-life application with completely new data sets. Of the included publications, 19 (36%) discussed how their model performed on datasets with characteristics that were different to those used for training and testing. In some instances, however, this evaluation was qualitative where the models were applied to large unlabelled, real-life datasets. 46 , 58 , 69 , 48 , 95 , 101 , 102
3.4.6 Other
3.4.6.1 Caveats
Caveats were extracted as free text. Included publications (N = 64, 86%) reported a variety of caveats. After extraction we structured them into six different domains:
- 1. Label-quality and inter-annotator disagreements
- 2. Variations in text
- 3. Domain adaptation and comparability
- 4. Computational or system architecture implications
- 5. Missing information in text or knowledge base
- 6. Practical implications
These are further discussed in the ‘Discussion’ section of this living review.
3.4.6.2 Sources of funding and conflict of interest
Figure 11 shows that most of the included publications in the base review did not declare any conflict of interest. This is true for most publications published before 2010, and about 50% of the literature published in more recent years. However, sources of funding were declared more commonly, with 69% of all publications including statements for this item. This reflects a trend of more complete reporting in more recent years.

4. Discussion
4.1. summary of key findings.
4.1.1 System architectures
Systems described within the included publications are changing over time. Non-machine-learning data extraction via rule-base and API is one of the earliest and most frequently used approaches. Various classical machine-learning classifiers such as naïve Bayes and SVMs are very common in the literature published between 2005-2018. Up until 2020 there was a trend towards word embeddings and neural networks such as LSTMs. Between 2020 and 2022 we observed a trend towards transformers, especially the BERT, RoBERTa and ELECTRA architectures pre-trained on biomedical or scientific text.
4.1.2 Evaluation
We found that precision, recall, and F1 were used as evaluation metrics in most publications, although sometimes these metrics were adapted or relaxed in order to account for partial or similar matches.
4.1.3 Scope
Most of the included publications focused on extracting data from abstracts. The reasons for this include the availability of data and ease of access, as well as the high coverage of information and the availability of structured abstracts that can automatically derive labelled training data. A much smaller number of the included publications (n=19, 25%) extracted data from full texts. Half of the systems that extract data from full text were published within the last seven years. In systematic review practice, manually extracting data from abstracts is quicker and easier than manually extracting data from full texts. Therefore, the potential time-saving and utility of full text data extraction is much higher because more time can be saved by automation and it provides automation that more closely reflects the work done by systematic reviewers in practice. However, the data extraction literature on full text is still sparse and extraction from abstracts may be of limited value to reviewers in practice because it carries the risk of missing information. Whenever a publication reported full-text extraction we tried to find out if this also included abstract text, in which case we would count the publication in both categories. However, this information was not always clearly reported.
4.1.4 Target texts
Reports of randomised controlled trials were the most common texts used for data extraction. Evidence concerning data extraction from other study types was rare and is discussed further in the following sections.
4.2. Assessment of the quality of reporting
We only assessed full quality of reporting in the base-review, and assessed selected items during the review update. The quality of reporting in the included studies in the base-review is improving over time We assessed the included publications based on a list of 17 items in the domains of reproducibility, transparency, description of testing, data availability, and internal and external validity.
Base-review: Reproducibility was high throughout, with information about sources of training and evaluation data reported in 94% of all publications and pre-processing described in 89%.
Base-review: In terms of transparency, 81% of the publications provided a clear description of their algorithm, 94% described the characteristics of their datasets, but only 9% mentioned hardware specifications or feasibility of using their algorithm on large real-world datasets such as PubMed.
Update: Availability of source code was high in the publications added in the LSR update (N=19, 83%). Before the update, 15% of all included publications had made their code available. Overall, 39% (N=30) now have their code available and all links to code repositories are shown in Table 2 .
Base-review: Testing of the systems was generally described, 89% gave a detailed assessment of their algorithms. Trade-offs between precision and recall were discussed in 32%.
Update: Basic metrics were reported in only 19% (N=14) of the included publications, which is a downward trend from 24% in the base-review. However, more complete reporting of source-code and public datasets still leads to increased transparency and comparability.
Update: Availability of the final models as end-user tools was very poor. Only 12% of the included publications had an application associated with it, but only 5 (6%) are available and directly usable via web-apps (see Table 3 for links). Furthermore, it is unclear how many of the other tools described in the literature are used in practice, even if only used internally within their authors research groups. There was a surprisingly strong trend towards sharing and re-using already published corpora in the LSR update. Earlier, labelled training and evaluation data were available from 13% of the publications, and only a further 32% of all publications reported using one of these available datasets. Within the LSR update, 22 corpora were available online and at least 40 other included publication mention using them. Table 4 provides the sources of all corpora and publications using them. For named-entity recognition, EBM-NLP 55 is the most popular dataset, used by at least 10 other publications and adapted and used by another four. For sentence classification the NICTA gold-standard 52 is used by eight others, and the automatically labelled corpus by Jin and Szolovits 96 is used by five others and was adapted once. For relation extraction the EvidenceInference 2.0 corpus is gaining attention, being used in at least three other publications.
Base-review: A total of 88% of the publications described using at least one accessible third-party framework for their data extraction system. Internal and external validity of each model was assessed based on its comparability to other tools (75%), assessment of visible and hidden variables in the data (19%), avoiding overfitting (62%, not applicable to non-machine learning systems), descriptions of splitting training from validation data (100%), and adaptability and external testing on datasets with different characteristics (36%). These items, together with caveats and limitations noted in the included publications are discussed in the following section.
4.3. Caveats and challenges for systematic review (semi)automation
In the following section we discuss caveats and challenges highlighted by the authors of the included publications. We found a variety of topics discussed in these publications and summarised them under seven different domains. Due to the increasing trend of relation-extraction and text summarisation models we now summarise any challenges or caveats related to these within the updated text at the end of each applicable domain.
4.3.1 Label-quality and inter-annotator disagreements
The quality of labels in annotated datasets was identified as a problem by several authors. The length of the entity being annotated, for example O or P entities, often caused disagreements between annotators. 46 , 48 , 58 , 69 , 95 , 101 , 102 We created an example in Figure 12 , which shows two potentially correct, but nevertheless different annotations on the same sentence.

P, population; I, intervention; C, comparison; O, outcome.
Similar disagreements, 65 , 85 , 104 along with missed annotations, 72 are time-intensive to reconciliate 97 and make the scores less reliable. 95 As examples of this, two publications observed that their system performed worse on classes with high disagreement. 75 , 104 There exist different explanations for worse performance in these cases. It is possibly harder for models to learn from labelled data with systematic differences within. Another reason is that the model learns predictions based on one annotation style and therefore artificial errors are produced when evaluated against differently labelled data, or that the annotation task itself is naturally harder in cases with high inter-annotator disagreement, and therefore lower performance from the models might be explainable. An overview of the included publications discussing this, together with their inter-annotator disagreement scores, is given in Table 5 .
Please see each included publication for further details on corpus quality.
To mitigate these problems, careful training and guides for expert annotators are needed. 58 , 77 For example, information should be provided on whether multiple basic entities or one longer entity annotation are preferred. 85 Crowd-sourced annotations can contain noisy or incorrect information and have low interrater reliability. However, they can be aggregated to improve quality. 55 In recent publications, partial entity matches (i.e., token-wise evaluation) downstream were generally favoured above complete detection, which helps to mitigate this problem’s impact on final evaluation scores. 55 , 83
For automatically labelled or distantly supervised data, label quality is generally lower. This is primarily caused by incomplete annotation due to missing headings, or by ambiguity in sentence data, which is discussed as part of the next domain. 44 , 57 , 103
4.3.2 Ambiguity
The most common source of ambiguity in labels described in the included publications is associated with automatically labelled sentence-level data. Examples of this are sentences that could belong to multiple categories, e.g., those that should have both ‘P’ and an ‘I’ label, or sentences that were assigned to the class ‘other’ while containing PICO information (Refs. 54 , 95 , 96 , among others). Ambiguity was also discussed with respect to intervention terms 76 or when distinguishing between ‘control’ and ‘intervention’ arms. 46 When using, or mapping to UMLS concepts, ambiguity was discussed in Refs. 41 , 52 , 72 .
At the text level, ambiguity around the meaning of specific wordings was discussed as a challenge, e.g., the word 'concentration' can be a quantitative measure or a mental concept. 41 Numbers were also described as challenging due to ambiguity, because they can refer to the total number of participants, number per arm of a trial, or can just refer to an outcome-related number. 84 , 113 When classifying participants, the P entity or sentence is often overloaded because it includes too much information on different, smaller, entities within it, such as age, gender, or diagnosis. 89
Ambiguity in relation-extraction can include cases where interventions and comparators are classified separately in a trial with more than two arms, thus leading to an increased complexity in correctly grouping and extracting data for each separate comparison.
4.3.3 Variations in text
Variations in natural language, wording, or grammar were identified as challenges in many references that looked closer at the texts within their corpora. Such variation may arise when describing entities or sentences (e.g., Refs. 48 , 79 , 97 ) or may reflect idiosyncrasies specific to one data source, e.g., the position of entities in a specific journal. 46 In particular, different styles or expressions were noted as caveats in rule-based systems. 42 , 48 , 80
There is considerable variation in how an entity is reported, for example between intervention types (drugs, therapies, routes of application) 56 or in outcome measures. 46 In particular, variations in style between structured and unstructured abstracts 65 , 78 and the description lengths and detail 59 , 79 can cause inconsistent results in the data extraction, for example by not detecting information correctly or extracting unexpected information. Complex sentence structure was mentioned as a caveat especially for rule-based systems. 80 An example of a complex structure is when more than one entity is described (e.g., Refs. 93 , 102 ) or when entities such as ‘I’ and ‘O’ are mentioned close to each other. 57 Finally, different names for the same entity within an abstract are a potential source of problems. 84 When using non-English texts, such as Spanish articles, it was noted that mandatory translation of titles can lead to spelling mistakes and translation errors. 35
Another common variation in text was implied information. For example, rather than stating dosage specifically, a trial text might report dosages of ‘10 or 20 mg’, where the ‘mg’ unit is implied for the number 10, making it a ‘dosage’ entity. 46 , 48 , 90
Implied information was also mentioned as problem in the field of relation-extraction, with Nye et al. (2021) 63 discussing importance of correctly matching and resolving intervention arm names that only imply which intervention was used. Examples are using ‘Group 1’ instead of referring to the actual intervention name, or implying effects across a group of outcomes, such as all adverse events. 63
4.3.4 Domain adaptation and comparability
Because of the wide variation across medical domains, there is no guarantee that a data extraction system developed on one dataset automatically adapts to produce reliable results across different datasets relating to other domains. The hyperparameter configuration or rule-base used to conceive a system may not retrieve comparable results in a different medical domain. 40 , 68 Therefore, scores might not be similar between different datasets, especially for rule-based classifiers, 80 when datasets are small, 35 , 49 when structure and distribution of class of interest varies, 40 or when the annotation guidelines vary. 85 A model for outcome detection, for example, might learn to be biased towards outcomes frequently appearing in a certain domain, such as chemotherapy-related outcomes for cancer literature or it might favour to detect outcomes more frequent in older trial texts if the underlying training data are older or outdated. 73 Another caveat mentioned by Refs. 59 , 85 is that the size of the label space must be considered when comparing scores, as models that normalise to specific concepts rather than detecting entities tend to have lower precision, recall, and F1 scores.
Comparability between models might be further decreased by comparing results between publications that use relaxed vs. strict evaluation approaches for token-based evaluation, 34 or publications that use the same dataset but with different random seeds to split training and testing data. 33 , 118
Therefore, several publications discuss that a larger amount of benchmarking datasets with standardised splits for train, development, and evaluation datasets and standardised evaluation scripts could increase the comparability between published systems. 46 , 92 , 114
4.3.5 Computational or system architecture implications
Computational cost and scalability were described in two publications. 53 , 114 Problems within the system, e.g., encoding 97 or PDF extraction errors 75 lead to problems downstream and ultimately result in bias, favouring articles from big publishers with better formatted data. 75 Similarly, grammar and parsing part-of-speech and/or chunking errors (Refs. 76 , 80 , 90 , among others) or faulty parse-trees 78 can reduce a system’s performance if it relies on access to correct grammatical structure. In terms of system evaluation, 10-fold cross-validation causes high variance in results when using small datasets such as NICTA-PIBOSO, 54 , 85 , 104 described that the same problem needs to be addressed through stratification of the positive instances of each class within folds.
4.3.6 Missing information in text or knowledge base
Information in text can be incomplete. 114 For example, the number of patients in a study might not be explicitly reported, 76 or abstracts lacking information about study design and methods can appear, especially in unstructured abstracts and older trial texts. 91 , 96 In some cases, abstracts can be missing entirely. These problems can sometimes be solved by considering the use of full texts as input. 71 , 87
Where a model relies on features, e.g., MetaMap, then missing UMLS coverage causes errors. 72 , 76 This also applies to models like CNNs that assign specific concepts, where unseen entities are not defined in the output label space. 59
In terms of automatic summarisation and relation extraction it was also cautioned that relying on abstracts will lead to a low sensitivity of retrieved information, as not all information of interest may be reported in sufficient detail to allow comprehensive summaries or statements about relationships between interventions and outcomes to be made. 60 , 63
4.3.7 Practical and other implications
In contrast to the problem of missing information, too much information can also have practical implications. For instance, often there are multiple sentences with each label, of which one is ‘key’, e.g., the descriptions of inclusion and exclusion criteria often span multiple sentences, and for a data extraction system it can be challenging to work out which sentence is the key sentence. The same problem applies to methods that select and rank the top-n sentences for each data extraction target, where a system risks including too much, or not enough results depending on the amount of sentences that are kept. 46
Low recall is an important practical implication, 53 especially in entities that appear infrequently in the training data, and are therefore not well represented in the training process of the classification system. 48 In other words, an entity such as ‘Race’ might not be labelled very often is a training corpus, and systematically missed or wrongly classified when the data extraction system is used on new texts. Therefore, human involvement is needed, 86 and scores need to be improved. 41 It is challenging to find the best set of hyperparameters 106 and to adjust precision and recall trade-offs to maximise the utility of a system while being transparent about the number of data points that might be missed when increasing system precision to save work for a human reviewer. 69 , 95 , 101
For relation extraction or normalisation tasks, error-propagation was noted as a practical issue in joint models. 63 , 67 To extract relations, first a model to identify entities is needed, and then another model to classify relationships is applied in a pipeline. Neither human nor machine can instantly perform perfect data extraction or labelling, 37 and thus errors done in earlier classification steps can be carried forwards and accumulate.
For relation extraction and summarisation, the importance of qualitative real-world evaluation was discussed. This was due to missing clarity of how well summarisation metrics relate to the actual usefulness or completeness of a summary and because challenges such as contradictions or negations within and between trial texts need to be evaluated within the context of a review and not just a trial itself. 61 , 63
A separate practical caveat with relation-extraction models are longer dependencies, i.e. bigger gaps between salient pieces of information in text that lead to a conclusion. This leads to increased complexity of the task and thus to reduced performance. 99
In their statement on ethical concerns, DeYoung et al. (2021) 61 mention that these complex relation and summarisation models can produce correct-looking but factually incorrect statements and are risky to be applied in practice without extra caution.
4.4. Explainability and interpretability of data extraction systems
The neural networks or machine-learning models from publications included in this review learn to classify and extract data by adjusting numerical weights and by applying mathematical functions to these sets of weights. The decision-making process behind the classification of a sentence or an entity is therefore comparable with a black box, because it is very hard to comprehend how, or why the model made its predictions. A recent comment published in Nature has called for a more in-depth analysis and explanation of the decision-making process within neural networks. 117 Ultimately, hidden tendencies in the training data can influence the decision-making processes of a data extraction model in a non-transparent way. Many of the examples discussed in the comment are related to healthcare, but in practice there is a very limited understanding of their inherent biases despite the broad application of machine learning and neural networks. 117
A deeper understanding of what occurs between data entry and the point of prediction can benefit the general performance of a system, because it uncovers shortcomings in the training process. These shortcomings can be related to the composition of training data (e.g. overrepresentation or underrepresentation of groups), the general system architecture, or to other unintended tendencies in a system’s prediction. 119 A small number of included publications in the base-review (N = 10) discussed issues related to hidden variables as part of an extensive error analysis (see section 3.5.2). The composition of training and testing data were described in most publications, but no publication that specifically addresses the issues of interpretability or explainability was found.
4.5. Availability of corpora, and copyright issues
There are several corpora described in the literature, many with manual gold-standard labels (see Table 4 ). There are still publications with custom, unshared datasets. Possible reasons for this are concerns over copyright, or malfunctioning download links from websites mentioned in older publications. Ideally, data extraction algorithms should be evaluated on different datasets in order to detect over-fitting, to test how the systems react to data from different domains and different annotators, and to enable the comparison of systems in a reliable way. As a supplement to this manuscript, we have collected links to datasets in Table 4 and encourage researchers to share their automatically or manually annotated labels and texts so that other researchers may use them for development and evaluation of new data extraction systems.
4.6. Latest developments and upcoming research
This is a timely LSR update, since it has a cut-off just before a the arrival of a new generation of tools: generative ‘Large Language Models’ (LLMs), such as ChatGPT from OpenAI, based on the GPT-3.5 model [ 1 ]. 120 As such, it may mark the current state of the field at the end of a challenging period of investigation, where the limitations of recent machine learning approaches have been apparent, and the automation of data extraction was quite limited.
The arrival of transformer-based methods in 2018 marked the last big change in the field, as documented by this LSR. Methods of our included papers only rarely progressed beyond the original BERT architecture, 14 varying mostly just in terms of datasets used in pre-training. Few used models only marginally different to BERT, such as RoBERTa with its altered pre-training strategy. 121 However, Figure 13 (reproduced from Yang et al. (2023) 122 ) shows that there has been a vast amount of NLP research and whole families of new methods that have not yet been tested to advance our target task of data extraction. For example within the new GPT-4 technical report, OpenAI describe increased performance, predictability, and closer adherence to the expected behaviour of their model, 123 and some other (open-source) LLMs shown in Figure 13 may have similar potential.

Early evaluations of LLMs suggest that these models may produce a step-change in both the accuracy and the efficiency of automated information extraction, while in parallel reducing the need for expensive labelled training data: a pre-print by Shaib et al. 124 describes a new dataset [ 2 ] and an evaluation of GPT-3-produced RCT summaries; 124 Wadhwa, DeYoung, et al. 125 use the Evidence Inference dataset and it’s annotations of RCT intervention-comparator-outcome triplets to train and evaluate BRAN, DyGIE++, ELI, BART, T5-base, and several FLAN models in a pre-print; 125 and in a separate pre-print Wadhwa, Amir, et al. 126 used the Flan-T5 and GPT-3 models to extract and predict relations between drugs and adverse events. 126 In the near future we expect the number of studies in this review to grow, as more evaluations of LLMs move into pre-print or published literature.
4.6.1 Limitations of this living review
This review focused on data extraction from reports of clinical trials and epidemiological research. This mostly includes data extraction from reports of randomised controlled trials where intervention and comparators are usually jointly extracted, and only a very small fraction of the evidence that addresses other important study types (e.g., diagnostic accuracy studies). During screening we excluded all publications related to clinical data (such as electronic health records) and publications extracting disease, population, or intervention data from genetic and biological research. There is a wealth of evidence and potential training and evaluation data in these publications, but it was not feasible to include them in the living review.
5. Conclusion
This LSR presents an overview of the data-extraction literature of interest to different types of systematic review. We included a broad evidence base of publications describing data extraction for interventional systematic reviews (focusing on P, IC, and O classes and RCT data), and a very small number of publications extracting epidemiological and diagnostic accuracy data. Within the LSR update we identified research trends such as the emergence of relation-extraction methods, the current dominance of transformer neural networks, or increased code and dataset availability between 2020-2022. However, the number of accessible tools that can help systematic reviewers with data extraction is still very low. Currently, only around one in ten publications is linked to a usable tool or describes an ongoing implementation.
The data extraction algorithms and the characteristics of the data they were trained and evaluated on were well reported. Around three in ten publications made their datasets available to the public, and more than half of all included publications reported training or evaluating on these datasets. Unfortunately, usage of different evaluation scripts, different methods for averaging of results, or custom adaptations to datasets still make it difficult to draw conclusions on which is the best performing system. Additionally, data extraction is a very hard task. It usually requires conflict resolution between expert systematic reviewers when done manually, and consequently creates problems when creating the gold standards used for training and evaluation of the algorithms in this review.
We listed many ongoing challenges in the field of data extraction for systematic review (semi) automation, including ambiguity in clinical trial texts, incomplete data, and previously unseen data. With this living review we aim to review the literature continuously as it becomes available. Therefore, the most current review version, along with the number of abstracts screened and included after the publication of this review iteration, is available on our website.
Data availability
Author contributions.
LS: Conceptualization, Investigation, Methodology, Software, Visualization, Writing – Original Draft Preparation
ANFM: Data Curation, Investigation, Writing – Review & Editing
RE: Data Curation, Investigation, Writing – Review & Editing
BKO: Conceptualization, Investigation, Methodology, Software, Writing – Review & Editing
JT: Conceptualization, Investigation, Methodology, Writing – Review & Editing
JPTH: Conceptualization, Funding Acquisition, Investigation, Methodology, Writing – Review & Editing
Acknowledgements
We thank Luke McGuinness for his contribution to the base-review, specifically the LSR web-app programming, screening, conflict-resolution, and his feedback to the base-review manuscript.
We thank Patrick O’Driscoll for his help with checking data, counts, and wording in the manuscript and the appendix.
We thank Sarah Dawson for developing and evaluating the search strategy, and for providing advice on databases to search for this review. Many thanks also to Alexandra McAleenan and Vincent Cheng for providing valuable feedback on this review and its protocol.
[version 2; peer review: 3 approved]
Funding Statement
We acknowledge funding from NIHR (LAM through NIHR Doctoral Research Fellowship (DRF-2018-11-ST2-048), and LS through NIHR Systematic Reviews Fellowship (RM-SR-2017-09-028)). LAM is a member of the MRC Integrative Epidemiology Unit at the University of Bristol. The views expressed in this article are those of the authors and do not necessarily represent those of the NHS, the NIHR, MRC, or the Department of Health and Social Care.
The funders had no role in study design, data collection and analysis, decision to publish, or preparation of the manuscript.
1 https://openai.com/blog/chatgpt (last accessed 22/05/2023).
2 https://github.com/cshaib/summarizing-medical-evidence (last accessed 22/05/2022).
- Version 2. F1000Res. 2021; 10: 401.
Reviewer response for version 1
Carmen amezcua-prieto.
1 Department of Preventive Medicine and Public Health, University of Granada, Granada, Spain
Data extraction in a systematic review is a hard and time-consuming task. The (semi) automation of data extraction in systematic reviews is an advantage for researchers and ultimately for evidence-based clinical practice. This living systematic review examines published approaches for data extraction from reports of clinical studies published up to a cut-off date of 22 April 2020. The authors included more than 50 publications in this version of their review that addressed extraction of data from abstracts, while less (26%) used full texts. They identified more publications describing data extraction for interventional reviews. Publications extracting epidemiological or diagnostic accuracy data were limited.
Main important issues have been addressed in the systematic review:
- This living systematic review has been justified. The field of systematic review (semi) automation is evolving rapidly along with advances in language processing, machine learning, and deep learning.
- Searching and update schedules have been clearly defined, shown in Figure 1.
- There are sufficient details of the methods and analysis provided to allow replication.
- Conclusions are drawn adequately supported by the results presented in the review.
A minor consideration is suggested:
- An incomplete sentence in Methods: ‘We included reports published from 2005 until the present day, similar to’.
Are the rationale for, and objectives of, the Systematic Review clearly stated?
Is the statistical analysis and its interpretation appropriate?
Not applicable
Have the search and update schedule been clearly defined and justified?
Is the living method justified?
Are sufficient details of the methods and analysis provided to allow replication by others?
Are the conclusions drawn adequately supported by the results presented in the review?
Reviewer Expertise:
I confirm that I have read this submission and believe that I have an appropriate level of expertise to confirm that it is of an acceptable scientific standard.
Kathryn A. Kaiser
1 Department of Health Behavior, School of Public Health, University of Alabama at Birmingham, Birmingham, AL, USA
The authors have undertaken and documented the steps taken to monitor an area of research methods that is important to many around the world by use of a “living systematic review”. The specific focus is on automated or semi-automated data extraction around the PICO structure often used in biomedicine, whether it be to summarize a body of literature narratively or using meta-analysis techniques. A significant irony about the body of papers included in this review is that there is a large amount of missingness related to the performance of such methods. Those who conduct systematic reviews know well the degree of missing information sought to summarize a group of studies.
Readers who will be most interested in this ongoing work can maintain an eye on the authors’ progress in identifying activities in this space. It is not clear, however, how long the funding will support this effort or how long the authors will remain engaged in advancing this project. The data represented in this paper does not give readers confidence that the community is approaching acceptable methods that are superior to other, less automated methods (the latter of which are not well-discussed).
Some aspects of the paper would benefit from additional detail (in no particular order of importance):
- The end game for the tracking of this area of literature is not explicitly described in the abstract, nor is it discussed to a great extent at the end of the paper. Much of the results presented do not paint a bright future for this area of research as conditions presently are. While the aim is laid out well in section 1.2, the large amount of missing performance data (reported to be 87%) is unable to address the “Is it reliable?” question. One might suspect that if particularly stellar performance were demonstrated by a project, those data would be prominently advertised. Thus, the yet-to-be-done contacting of authors step would be enlightening if either performance data can be obtained, or if authors remain silent on that request. This follow-up task will be a major point of interest for many who will follow updates to this paper. It is likely that the particular research context (e.g. see Pham et al ., 2021 1 ) will have a large degree of influence on the performance metrics to be had if they can be determined.
- The description of how the 17 “Key items of interest” were determined and if there is a plan to put these forth as methodological guidelines or a reporting checklist would be helpful. Either of these would help to advance the field further.
- On Page 5, the exclusions listed have the use of pre-processing of text, yet the results discuss the many papers that appear to have used that in their methods. Perhaps this is a deviation from the original protocol after the review began (an understandable decision)?
- In section 2.4 about searching Pubmed, can the authors clarify that the Pubmed 2.0 API or GUI will be used to access candidate literature?
- Also relevant to section 2.4 on searching, since GITHUB is so popular, might this also be a fruitful place to routinely search?
- Clarification of the ability to obtain cited software packages (whether for no cost or at some cost) would be helpful.
- Figure 3 explanation of PICO is a typo – “PCIO”.
- Table 5 is shown before Table 1. Please check and correct flow and references to table numbers (5,1,4,2,3 is the flow now).
- One of the major limitations to be noted is the unfortunate issue of the lack of specific data in abstracts about interventions and comparators.
Systematic reviews in biomedicine topics, issues with time and effort required to complete reviews with generally available tools.
Emma McFarlane
1 Centre for Guidelines, National Institute for Health and Care Excellence, London, UK
This is a living systematic review of published methods and tools aimed at automating or semi-automating the process of data extraction in the context of a systematic review. Automating data extraction is an area of interest among evidence-based medicine.
The methods are sufficiently described to be replicated, but further details of analysis to determine the items of interest would be helpful to link into the results. Additionally, the authors may want to consider commenting on the topic areas covered by the included studies and whether that has an impact on any of the metrics measured.
In the discussion section, it's interesting that fewer studies extracted data from the full text. Could the authors comment on the implications of this in terms of using tools in a live review as it's not common to manually only extract data from an abstract.
Evidence-based medicine, systematic reviews, automation techniques.

A .gov website belongs to an official government organization in the United States.
A lock ( ) or https:// means you've safely connected to the .gov website. Share sensitive information only on official, secure websites.
- About Heart Disease
- Risk Factors
- Heart Disease Facts
- Healthy People 2030
- Heart Disease Communications Toolkit
- Publication
- Community-Clinical Linkages Health Equity Guide
- Emergency Medical Services (EMS) Home Rule State Law Fact Sheet
- American Heart Month Toolkits
- Healthy Eating Communications Kit
- About Stroke
- About High Blood Pressure
- About Cholesterol
What to know
Learn facts about how race, ethnicity, age, and other risk factors can contribute to heart disease risk. It’s important for everyone to know the facts about heart disease.
Heart disease in the United States
In the United States:
- Heart disease is the leading cause of death for men, women, and people of most racial and ethnic groups. 1
- One person dies every 33 seconds from cardiovascular disease. 1
- About 695,000 people died from heart disease in 2021—that's 1 in every 5 deaths . 1 2
- Heart disease costs about $239.9 billion each year from 2018 to 2019. 3 This includes the cost of health care services, medicines, and lost productivity due to death.
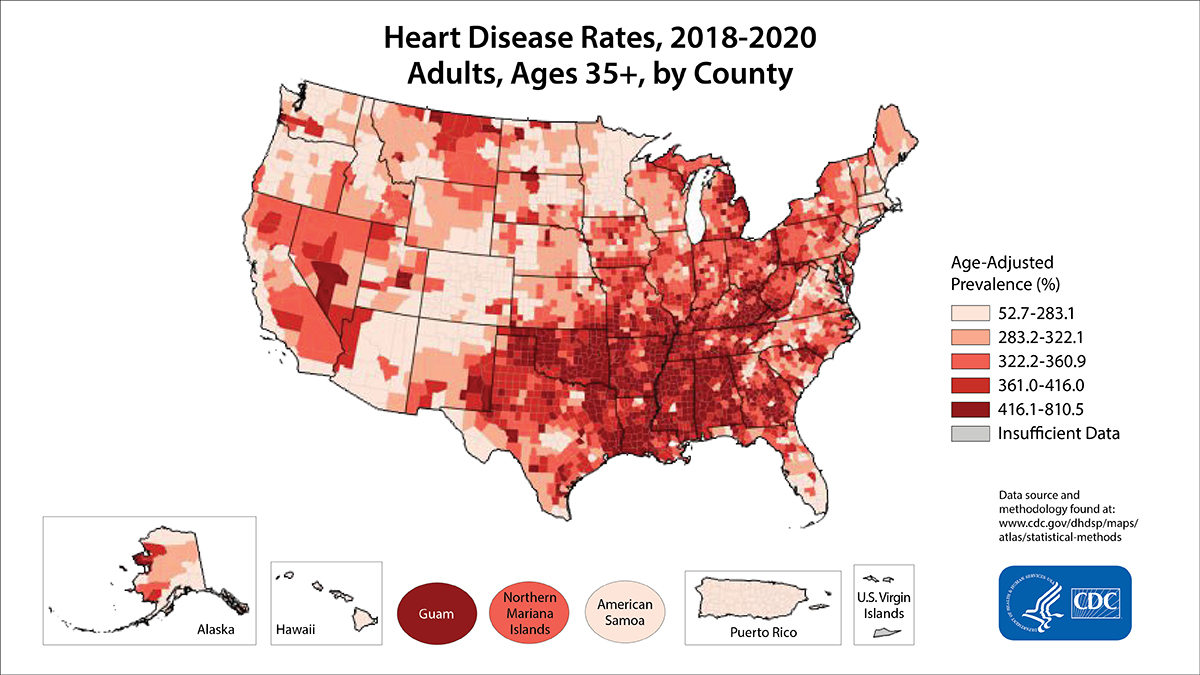
Coronary artery disease (CAD)
- Coronary heart disease is the most common type of heart disease, killing 375,476 people in 2021. 2
- About 1 in 20 adults age 20 and older have CAD (about 5%). 2
- In 2021, about 2 in 10 deaths from CAD happened in adults less than 65 years old. 1
Heart attack
- In the United States, someone has a heart attack every 40 seconds. 2
- 605,000 are a first heart attack. 2
- 200,000 happen to people who have already had a heart attack. 2
- About 1 in 5 heart attacks are silent—the damage is done, but the person is not aware of it. 2
Did you know?
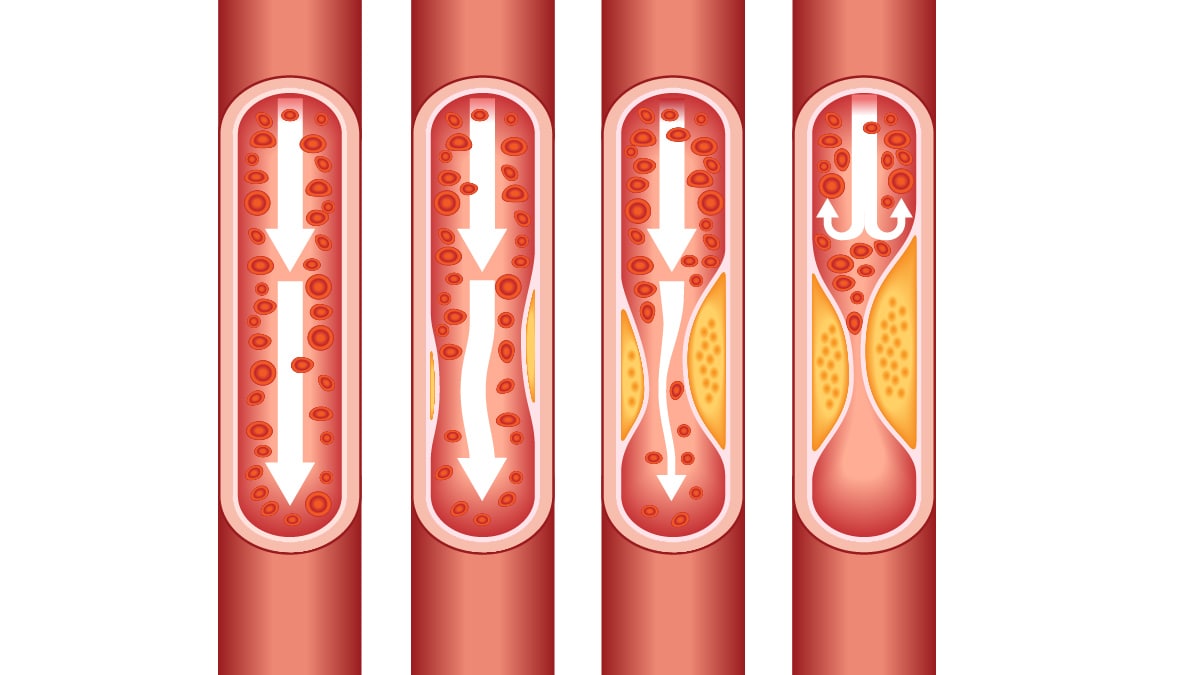
Who is affected
Heart disease deaths vary by sex, race, and ethnicity.
Heart disease is the leading cause of death for people of most racial and ethnic groups in the United States. These include African American, American Indian, Alaska Native, Hispanic, and White men. For women from the Pacific Islands and Asian American, American Indian, Alaska Native, and Hispanic women, heart disease is second only to cancer. 1
Below are the percentages of all deaths caused by heart disease in 2021, listed by ethnicity, race, and sex.
Race or Ethnic Group
% of Deaths
American Indian or Alaska Native
Black (Non-Hispanic)
Native Hawaiin or Other Pacific Islander
White (Non-Hispanic)
Americans at risk for heart disease
High blood pressure , high blood cholesterol , and smoking are key risk factors for heart disease.
Several other medical conditions and lifestyle choices can also put people at a higher risk for heart disease, including:
- Overweight and obesity
- Unhealthy diet
- Physical inactivity
- Excessive alcohol use
What CDC is doing
- Million Hearts ®
- CDC: Heart Disease Communications Kit
- National Heart, Lung, and Blood Institute
- National Center for Health Statistics. Multiple Cause of Death 2018–2021 on CDC WONDER Database. Accessed February 2, 2023. https://wonder.cdc.gov/mcd.html
- Tsao CW, Aday AW, Almarzooq ZI, et al. Heart Disease and Stroke Statistics—2023 Update: A Report From the American Heart Association. Circulation. 2023;147:e93–e621.
- National Center for Health Statistics. Percentage of coronary heart disease for adults aged 18 and over, United States, 2019—2021. National Health Interview Survey. Accessed February 17, 2023. https://wwwn.cdc.gov/NHISDataQueryTool/SHS_adult/index.html
Heart Disease
Heart disease is the leading cause of death in the United States.
For Everyone
Public health.
Reports and Insights
- Access and equity
- Become a member
- Common App impact
- Guiding principles
- Join our board
- Leadership and board
- Next Chapter
- Partner organizations
- Reports and insights
As a non-profit membership organization serving millions of students each year, Common App is uniquely positioned to share data and provide insight into the college admissions process.
Our reports and insights are driven by more than 40 years of experience supporting applicants, recommenders, and admissions professionals. With more than 1,000 member colleges and universities serving over 1 million applicants each year, we are able to devise insight into application trends across the applicant lifecycle and identify potential barriers and opportunities to improve college access.
Core to our mission of access, equity, and integrity is a responsibility to move our practice forward by collaborating with like-minded organizations, developing best practices, and sharing the results of our findings.
Common App application trends
March 1 deadline update Data Analytics and Research March 14, 2024
February 1 deadline update Data Analytics and Research February 14, 2024
January 1 deadline update Data Analytics and Research January 14, 2024
December 1 deadline update Data Analytics and Research December 14, 2023
November 1 deadline update - supplement Data Analytics and Research November 27, 2023
November 1 deadline update Data Analytics and Research November 1, 2023
Common App state reports Data Analytics and Research
Common App research briefs
Exploring the complexities of detailed parental education First-generation status in context • Part 3 Data Analytics and Research April 4, 2024
Differing definitions and their implications First-generation status in context • Part 2 Data Analytics and Research February 8, 2024
Trends in parental education & family structures over time First-generation status in context • Part 1 Data Analytics and Research November 8, 2023
Common App for transfer: a four-year retrospective Data Analytics and Research April 24, 2023
Research summary: trends and disparities in extracurricular activity reporting Data Analytics and Research April 18, 2023
Beyond ‘international:’ Understanding nuances of a diverse applicant pool Data Analytics and Research March 28, 2023
First-year applications per applicant Data Analytics and Research December 12, 2022
Unpacking applicant race and ethnicity, part 1 Data Analytics and Research October 17, 2022
Unpacking applicant race and ethnicity, part 2 Data Analytics and Research October 17, 2022
Long-term progress toward diversifying the Common App applicant pool Data Analytics and Research September 15, 2022
First-year admission pl ans: trends over time and applicant composition Data Analytics and Research November 21, 2021
Growth and change: long-term trends in Common App membership Data Analytics and Research October 19, 2021
Applying to college in a test-optional landscape Data Analytics and Research September 8, 2021
Pandemic patterns Data Analytics and Research August 30, 2021
Third-party research and collaboration
A brief guide to Common Apps name, sex, and gender questions for member institutions Source: Campus Pride
A majority of U.S. colleges admit most students who apply Source: Pew Research Center
Nudging at a National Scale: Experimental Evidence from a FAFSA Completion Campaign Source: EdPolicyWorks - University of Virginia
Mindsets and the Learning Environment: A Big Biodata Approach to Mindsets, Learning Environments, and College Success Source: Sidney D’Mello (PI), University of Colorado Boulder, Angela Duckworth (Co-PI), University of Pennsylvania
True Merit: Ensuring Our Brightest Students Have Access to Our Best Colleges and Universities Source: The Jack Kent Cooke Foundation
The Michelle Obama Effect: How Former First Lady Michelle Obama’s Community Events Impact FAFSA Completion Source: EdPolicyWorks - University of Virginia
Common App member case studies
Navigating the new normal: How the University of Georgia streamlined the application process and improved student and family communication during COVID-19 Source: Common App

- NIH Grants & Funding
- Blog Policies
NIH Extramural Nexus

Reminders, Updates, and Some Data for Participant Inclusion
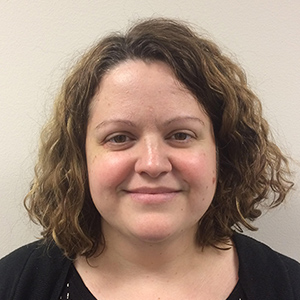
Last November, the White House announced its Initiative on Women’s Health Research to “fundamentally change how we approach and fund women’s health research, and pioneer the next generation of discoveries in women’s health.” Relatedly, the NIH Director has emphasized the importance of ensuring proper inclusion of participants in NIH-supported clinical research (see her opening remarks at December’s Advisory Committee to the NIH Director meeting and a National Academies meeting in May). We, as part of this wider effort, want to remind the research community about relevant NIH inclusion policies and resources, as well as where inclusion data can be found.
As we have said before , appropriate inclusion of research participants ensures that NIH supports science that will inform clinical practice to benefit all who are affected by the disease or condition under study. We have had policies in place for over three decades to ensure appropriate inclusion of women and members of racial and ethnic minority groups in NIH-supported clinical research. And, our Inclusion Across the Lifespan policy (effective January 2019) requires individuals of all ages (including children and older adults) be included in clinical research studies unless there are scientific or ethical reasons to exclude them. Since these policies have been in effect, they have required, among other things, that recipients report to NIH on the sex or gender, race, and ethnicity, and more recently age of enrolled participants ( in .csv format ). These NIH All About Grants podcasts share helpful advice related to inclusion policies when planning and reporting on your study.
These inclusion data allow us to report the breakdown of participants in NIH-funded clinical research, so that the public has a better understanding of who is enrolled in our supported studies. For instance, in fiscal year 2023:
- Women represented 57 percent of participants in all NIH-supported clinical research (55 percent for U.S. participants).
- 44 percent of participants identified as members of racial or ethnic minority groups (31 percent for U.S. participants).
- 9 percent of participants were children under 18 years of age and 13 percent were adults older than 65 years (7 percent children and 15 percent older adults for U.S. participants).
Inclusion data are publicly available for many different research areas that NIH supports. Data broken down by NIH Research, Condition, and Disease Classification (RCDC) categories for women and members of racial and ethnic minority populations were first announced in 2019 and by age in 2022 . Up until now, these data were only publicly updated once every three years. Going forward, we will produce inclusion by RCDC data annually to allow better insight into the demographics of participants in clinical research more often.
Finally, we sought public input on how common data elements may be used in NIH-supported clinical research. One area in particular focused on what demographic characteristics common data elements should collect. Although the comment period is now closed , we still encourage you to stay tuned for more information to come.
We appreciate the research community’s continued efforts to ensure proper inclusion of participants in NIH-funded research and in publications . We will continue providing resources and other educational materials (e.g. allowable costs for participant enrollment , reports on women’s health research , and NIH Institute and Center’s participant inclusion ), so that we support science that will ultimately inform clinical practice to benefit all who are affected by the disease or condition under study.
RELATED NEWS
Before submitting your comment, please review our blog comment policies.
Your email address will not be published. Required fields are marked *
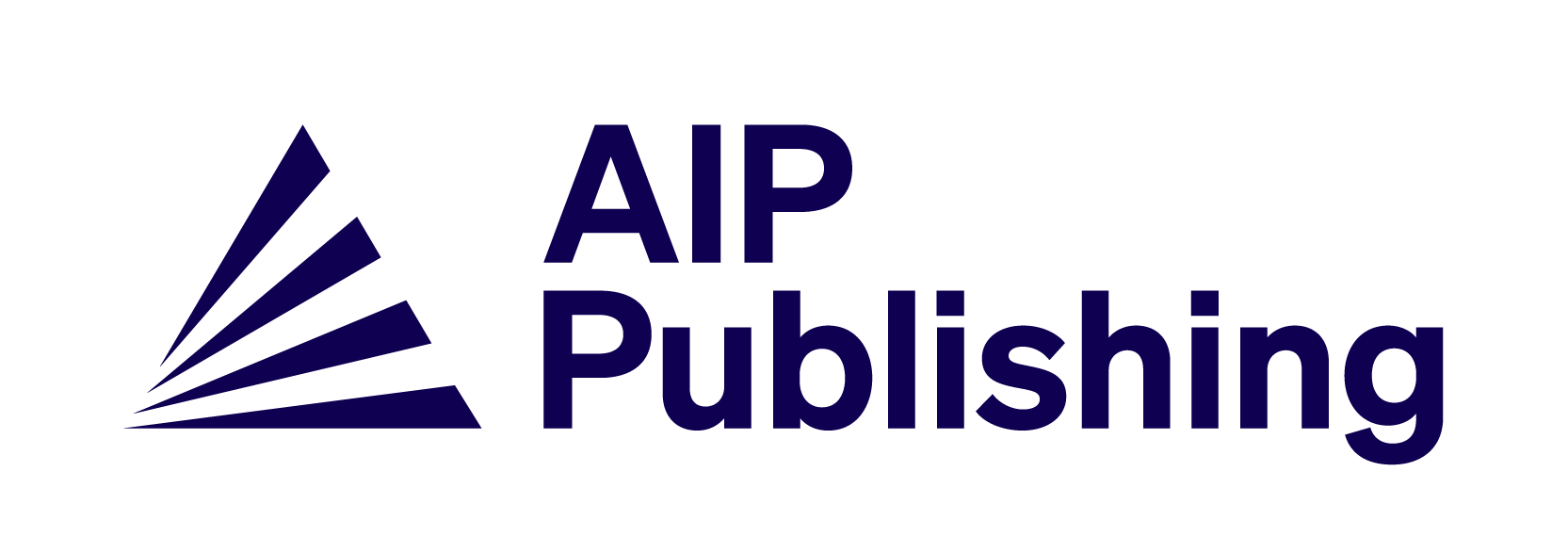
- Previous Article
- Next Article
I. INTRODUCTION
Ii. examples of data reuse, a. applications of computational databases to theoretical studies, b. applications of computational databases to experimental studies, iii. technical aspects of data sharing, a. data formats, b. data dissemination strategies, c. data centralization, iv. challenges and outlook, acknowledgments, research update: the materials genome initiative: data sharing and the impact of collaborative ab initio databases.
Author to whom correspondence should be addressed. Electronic mail: [email protected]
- Split-Screen
- Article contents
- Figures & tables
- Supplementary Data
- Peer Review
- Open the PDF for in another window
- Reprints and Permissions
- Cite Icon Cite
- Search Site
Anubhav Jain , Kristin A. Persson , Gerbrand Ceder; Research Update: The materials genome initiative: Data sharing and the impact of collaborative ab initio databases. APL Mater. 1 May 2016; 4 (5): 053102. https://doi.org/10.1063/1.4944683
Download citation file:
- Ris (Zotero)
- Reference Manager
Materials innovations enable new technological capabilities and drive major societal advancements but have historically required long and costly development cycles. The Materials Genome Initiative (MGI) aims to greatly reduce this time and cost. In this paper, we focus on data reuse in the MGI and, in particular, discuss the impact of three different computational databases based on density functional theory methods to the research community. We also discuss and provide recommendations on technical aspects of data reuse, outline remaining fundamental challenges, and present an outlook on the future of MGI’s vision of data sharing.
Materials innovations are critical to technological progress. Many authors have noted that advanced materials are so vital to society that they serve as eponyms for historical periods such as the Stone Age, the Bronze Age, the Steel Age, the Age of Plastic, and the Silicon Age. 1–3 A recent study by Magee suggests that materials innovation has driven two-thirds of today’s advancements in computation (in terms of calculations per second per dollar) and has similarly transformed other technologies such as information transport and energy storage. 4 However, while the benefits of new materials and processes are well established, the difficulties in achieving these breakthroughs and translating them to the commercial market 5 are not widely appreciated. It takes approximately 20 years to commercialize new materials technologies 6 and often even longer to develop those technologies in the first place. The long time scale of materials innovation stifles investment in early stage research because payback is unlikely. 6 Thus, it is natural to wonder whether it is possible to catalyze materials design such that decades of research and development can occur in the span of years.
One contributing factor that stunts materials development is lack of information. For newly hypothesized compounds, the answer to several important questions must typically be guided only by intuition. Can the material be synthesized? What are its electronic properties? What defects are likely to form? The dearth of materials property information can cause researchers to focus on the wrong compounds or persist needlessly in optimizing of materials that, even under ideal conditions, would not be able to meet performance specifications. LiFePO 4 —a successful lithium ion battery cathode material—serves as a good example of how overlooked opportunities stem from a lack of information. Its crystal structure was first reported 7 in 1938, but LiFePO 4 ’s application to lithium ion batteries was discovered only in 1997 by Padhi et al. 8 If the compound’s lithium insertion voltage and its exceptional lithium mobility were known earlier, it is likely that LiFePO 4 ’s application to batteries would have arrived one or two decades earlier. If its phase diagram had been previously charted, the synthesis conditions under which this material is made could have been more rapidly optimized.
Today, it is possible to compute many such properties from first-principles using a technique called density functional theory (DFT), which solves the electronic structure of a material by approximating solutions to Schrödinger’s equation. 9 New capabilities developed in the last one or two decades make it possible to reimagine the path to materials discovery. As one example of a disruptive change, a single computational materials science research group today might have access to a level of computing power equivalent to personal ownership of the world’s top supercomputer from 15 years ago or the sum of the top 500 supercomputers from 20 years ago. 10 New theoretical methods that more accurately predict fundamental materials properties 11,12 have made it possible, in many well-documented cases, to design materials properties in a computer before demonstrating their functionality in the laboratory. 13,14 Similar advancements in experimental capabilities, including combinatorial materials synthesis, 15–17 have made it possible to collect data at unprecedented rates and with much greater fidelity. 18–21 In almost all instances, the situation remains far from ideal. For example, material properties computable with DFT are typically not directly equivalent to the final engineering property of interest. However, several case studies have already shown that some forms of computational materials engineering can save tens of millions of dollars and result in returns on investment of up to 7:1 and with shorter design cycles. 5,22,23
The Materials Genome Initiative (MGI) recognizes that these advancements, if nurtured, can lead to a discontinuous shift in the time needed to discover and optimize new materials. Its intention is to enable “discovery, development, manufacturing, and deployment of advanced materials at least twice as fast as possible today, at a fraction of the cost.” 24 The MGI, which receives major funding from the Department of Energy, Department of Defense, the National Science Foundation, and the National Institute of Standards and Technology, encompasses various individual projects and larger research centers to advance this vision. 25 The four pillars upon which the MGI aims to achieve its goals are (i) to lead a culture shift in materials research that encourages multi-institute collaboration, (ii) to integrate experiment, computation, and theory across length scales and development cycle, (iii) to make digital data accessible and reusable, and (iv) to train a world-class materials workforce. 24
In this manuscript, we focus on the topic of data reuse, noting that many of the other MGI pillars have been discussed elsewhere. 2,5,26–29 As illustrated in Figure 1 , data sharing can drastically shorten the materials research cycle by (i) reducing the burden of data collection for individual research groups and (ii) enabling more efficient development of scientific hypotheses and property prediction models. In this manuscript, we focus on a form of data sharing that is still in its early stages: the use of DFT databases by both theory and experimental groups towards a variety of materials design applications.
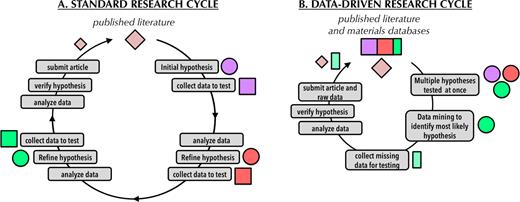
Schematic to illustrate differences between standard, single-group research (left) and new opportunities afforded by large materials databases (right). The standard research cycle is considerably longer because testing and refining hypotheses typically involves several time-consuming data collection steps. In contrast, the availability of large data sets in the data-driven approach enables multiple hypotheses to be tested simultaneously through novel informatics-based approaches, reducing the burden of data collection.
Material scientists have been sharing data for several decades. For example, several longstanding crystal structure databases have recently been reviewed by Glasser 30 and their impact on materials science has been reported by Le Page. 31 Experimental researchers routinely rely on diffraction pattern databases such as the Powder Diffraction File from the International Center for Diffraction Data 32 for phase identification of their samples. Thermodynamic databases 33–35 and handbooks 36,37 have been used to build and refine highly successful models such as the Calphad method 27,38,39 for generating phase diagrams. Thus, data sharing in materials science certainly predates the MGI.
What is novel under the MGI and related programs is (i) the scale at which data sharing is emphasized and (ii) the rapid expansion of theory-driven databases. Data sharing is heavily encouraged and enforced, 40–42 and new experimental databases such as the Structural Materials Data Demonstration Project, Materials Data Facility, and Materials Atlas are being funded. Large Department of Energy research hubs are incorporating combinatorial screening, 43 high-throughput computational screening, 44,45 and data mining 46 into the discovery process. New resources are rapidly coming online: the authors knew of no database of ab initio calculations that existed a decade ago, yet a recent review by Lin 47 lists 13 such databases that exist today (many of which contain hundreds of thousands of data points).
Three databases that have received MGI support are the Materials Project (MP), 48 AFLOWlib, 49 and Open Quantum Materials Database (OQMD). 50 The MP is centered at Lawrence Berkeley National Laboratory. Distinguishing features include its large and growing user base (currently over 17 000 registered users), its emphasis on “apps” for exploring the data, and database of elastic 51 and piezoelectric 52 tensor properties. The AFLOWLib consortium is centered at Duke University. A major feature of this resource is its large number of total compounds and its applications towards alloys, spintronics, and scintillation. The OQMD is centered at Northwestern University. Distinguishing features include comprehensive calculations for popular structure types (e.g., Heusler and perovskite compounds) and a focus on new compound discovery.
Unsurprisingly, all three of these databases have been used extensively by their respective development teams. However, although these resources are each only a few years old, there is already an emerging body of literature demonstrating their usage. Next, we present some early examples of research using DFT database resources conducted without the involvement of the development team.
A first application of computational databases is to aid and inspire further computational studies. Indeed, database projects such as ESTEST 53 and NoMaD 54 are geared especially to aid in reproducibility, verification, and validation of computational results. Parameters documented and tested by one project 55–57 can, in some cases, develop into a semi-standard methodology for the community. For example, the Hubbard interaction term, pseudopotentials, and reference state energies employed by the MP for DFT calculations are often re-used by the community. 58–64
The atomistic modeling community has similarly established databases of interatomic potentials such as OpenKIM 1,65 and NIST Interatomic Potentials Repository Project 66 that can be recycled for new applications. Such resources can be complementary, and information from DFT databases can help parameterize interatomic potentials. For example, Pun et al. 67 used energies computed by the AFLOWlib and OQMD databases to develop a force field for the Cu-Ta system. Data from the ab initio scale can inform materials behavior at higher length scales in other ways as well. For example, Gibson and Schuh 68 employed formation energies from the MP to help establish energy scales for grain boundary cohesion. These kinds of approaches that bridge length scales encompass a key component of the MGI called integrated computational materials engineering (ICME). 22,23,69
A second use case of DFT databases is as an established reference against which to compare results. For example, a study by Miletic et al. used the AFLOWlib database to test their computations of lattice parameters and magnetic moment of YNi 5 . 70 Romero et al. developed a new structure search algorithm and used all three database resources (MP, AFLOWlib, and OQMD) to verify that none of the new predicted compounds were previously recorded. 71 Sarmiento-Pérez et al. , 72 and Jauho et al. , 73 used extensive sets of the ground state structures from the MP to test new exchange-correlation functionals, and many other examples of such usage can be found. 74,75
One computational method that has particularly benefited from DFT materials databases is the estimation of the thermodynamic stability of new and hypothetical materials. Estimating this quantity requires calculating all known phases within the chemical space of the target phase and then applying a convex hull analysis to determine the lowest energy combination of phases at the target composition. This process, which was in the past tedious and time-consuming, can today be performed within minimal effort using the data and software tools 77 already provided by larger DFT data providers (Fig. 2 ). Examples include stability analysis of new photocatalysts 78,79 and other applications 80–84 (assisted by MP data), high entropy alloys 76 (assisted by AFLOWlib data), and dichalcogenides for hydrogen evolution 85 (assisted by OQMD data).
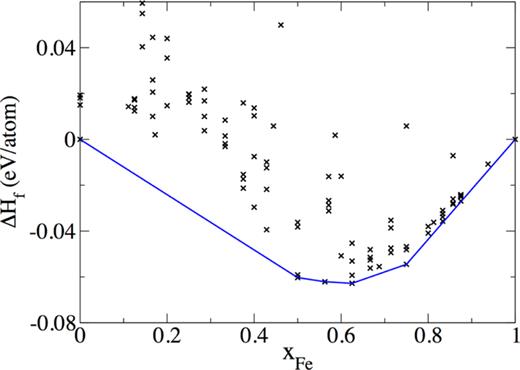
Example of convex hull stability analysis for Fe-Co alloys, with data points from the AFLOWlib database, used by Troparevsky et al. 76 for the purposes of designing high-entropy alloys. Reproduced with permission from Troparevsky et al. , JOM 67 , 2350–2363 (2015). Copyright 2015 Springer.
Finally, computational material databases can be used for property prediction and materials screening. For example, Sun et al. used the OQMD to help predict lithiation energies of MXene compounds, 86 and Gschwind et al. similarly used the MP to predict fluoride ion battery voltages. 87 Hong et al. employed MP data to screen new materials as oxygen evolution reaction catalysts. 88 Impressively, some groups have been able to download and use very large data sets in their study. For example, Seko et al. 89 extracted 54 779 relaxed compounds as starting points from the MP to virtually screen for low thermal conductivity compounds using anharmonic lattice dynamics calculations. Tada et al. used 34 000 compounds from the MP as a basis for screening new materials as two-dimensional electrides. 90 Thus, the usage of computational databases can involve confirming a few data points or screening tens of thousands of compounds.
Perhaps an even greater impact to materials science will come from the experimental community’s usage of computational databases. A first example application is comparing theoretical and experimental data in order to verify both or fill gaps in information. For example, the MP is often used to look up materials properties such as lattice parameters, XRD peak positions, or even battery conversion voltages. 91–93 The comprehensive nature of these databases can make them very powerful tools when used in concert with experiment. For example, both the AFLOWlib and OQMD contain data on a large number of Heusler-type phases. This helped the research group of Nash perform multiple studies that map out the thermodynamics and phase equilibria of Heusler 94–97 and half-Heusler 98 phases.
Similar to the situation for theorists, one of the most popular uses of computational databases by experimental researchers has been to generate phase diagrams. Multiple studies 80,100–102 have employed the MP Phase Diagram App to establish whether their materials of interest are likely to form under certain conditions. For example Martinolich and Neilson 100 report that NaFeS 2 is reported (by MP) to be unstable with respect to decomposition into FeS 2 , FeS, and Na 2 S, which is consistent with their unsuccessful attempts to prepare the ternary phase.
Computed phase diagrams from DFT are also useful for other purposes. For example, He et al. investigated the heterogeneous sodiation mechanism of iron fluoride (FeF 2 ) nanoparticle electrodes by combining in situ and ex situ microscopy and spectroscopy techniques. 99 The MP Li-Fe-F phase diagram was used to interpret the observed reaction pathways and illustrate the difference between equilibrium reactions and the metastable phases that may form because of kinetic limitations (Figure 3 ). Another example is from the work of MacEachern et al. , 103 who superimposed the results of sputtering experiments onto the MP phase diagram for Fe-Si-Zn. Similarly, Nagase et al. 104 employed the computed MP Co-Cu-Zr-B quarternary phase diagram from MP to guide experimental exploration of amorphous phase formation. In another work, the MP phase diagram of Li-Ni-Si was used to highlight the existence of a ternary lithiated phase that could impact the performance of nickel silicides as Li-ion anode materials. 105
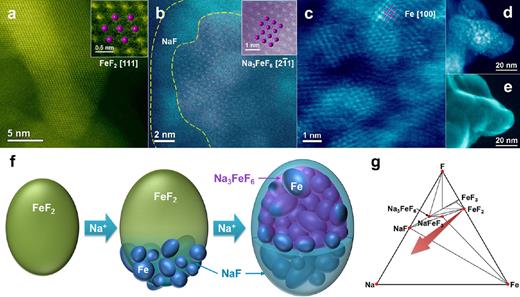
Information from high resolution STEM images ((a)-(d)) and the computational phase diagram from the Materials Project (g) were employed by He et al. 99 to propose a mechanism for sodium incorporation into FeF 2 particles for battery applications. Reprinted with permission from He et al. , ACS Nano 8 , 7251–7259 (2014). Copyright 2014 American Chemical Society.
The calculated electronic structure (e.g., the band structure of compounds) is also frequently used in experimental studies despite the known underestimation of band gaps in standard DFT. 107 For example, Fondell et al. used the MP-calculated band structure of hematite to explain its limited charge transport in the bulk phase and motivate the need for low-dimensional particles or thin films for solar hydrogen production (Fig. 4 ). 106
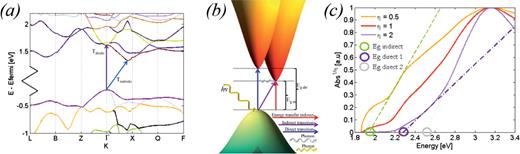
(a) Calculated band structure of Fe 2 O 3 from the Materials Project, (b) a schematic of the indirect nature of the transition (c) and its effect on optical absorption measurements from the work of Fondell et al. 106 Reproduced with permission from Fondell et al. , J. Mater. Chem. A 2 , 3352 (2014). Copyright 2014 Royal Society of Chemistry.
When considering that the role of density functional theory calculations was limited to the dominion of the theoretical physicist only two to three decades ago, these examples indicate an encouraging trend of DFT calculations becoming practical materials science design tools that are accessible to the broader research community. In alignment with the MGI vision, the examples above highlight a growing trend in which research data (whether computational or experimental) are routinely compared against past results and in which new studies are motivated or explained at least in part by data compiled by computational databases.
With the benefits of data sharing established, we now concentrate on three technical issues in data sharing: data formats, data dissemination strategies, and data centralization. Our discussion is rooted in our experience in collaboratively building two tools: the Materials Project API (application programming interface) (MAPI), 108 which has been used by over 300 distinct users to download approximately 15 × 10 6 data points, and the MPContribs framework, 109,110 which allows researchers to contribute external data into the MP.
Simulation software and experimental instruments typically output their own proprietary data file formats. Professional societies often develop custom data formats, such as the CIF (Crystallographic Information File) standard, 111 which define their own semantics and require building custom parsers to support that standard. Currently, the situation for data formats in materials science resembles a “wild west” scenario that makes it difficult for end users to work with data from multiple sources.
One strategy that can help promote standardization to build materials-centric specifications off of standard data formats that are fully open and cross-platform. For example, tabular data can conform to a comma-separated-values (CSV) format that is easily readable by almost all analysis packages and programming languages. Large, array-based data can be formatted as HDF5 112 or netCDF. 113 For more complex data, e.g., the representation of crystal structures or input parameters and output quantities such as band structures, options include Extensible Markup Language (XML) 114 and Javascript Object Notation (JSON) 115 formats. Successful examples include ChemML 116 and MatML, 117 which build scientific specifications on top of the XML standard. Our preference is for the JSON format, which is smaller in size and simpler to construct. JSON documents can be directly stored in next-generation database technologies such as MongoDB 118 which allow rich search capabilities over these documents. JSON can also be interconverted to the Yet Another Markup Language (YAML) format, which can be easily read and edited in text editors.
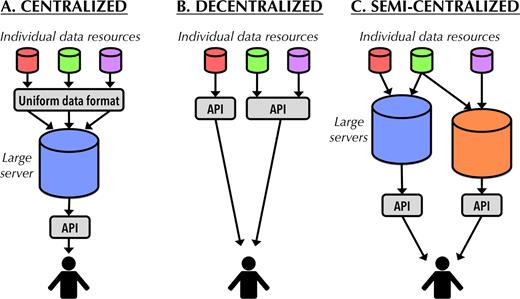
Potential models for data unification. In the (a) centralized model, data are homogenized and submitted to a single entity that manages data storage and data access through an application programming interface (API). In the (b) decentralized model, each data resource maintains its own data and exposes its own API (either coordinated or uncoordinated with other providers) for the end user. The most likely scenario is the (c) semi-centralized model in which several large providers each handle data contributions for different sub-domains of materials science.
Another technical aspect of data reuse concerns the most appropriate way to expose large data sets to the research community. Individual data providers can select from several options for exposing their data, including file download (e.g., as CSV or ZIP archive), a database dump (e.g., a MySQL dump file), or exposing an API over the web. The first two of these methods are relatively straightforward; the final method merits further clarification. An API is a protocol for interacting with a piece of software or data resource. For sharing data over the web, a common pattern is to use a REpresentaitional State Transfer (REST) API. 119 REST APIs are employed by several modern software companies, including Google, Microsoft, Facebook, Twitter, Dropbox, and others. In the most straightforward use case, one can imagine a REST API as mapping web URLs to data in the same way that a folder structure is used to organize files. For example, an API can be set up such that requesting the URL “ https://www.materialsproject.org/rest/v2/materials/Fe2O3/vasp ” returns a JSON representation of the data on all Fe 2 O 3 compounds as computed by the VASP 120 software. However, RESTful APIs are more powerful than file folder trees. For example, the HTTP request used to fetch the URL can additionally incorporate parameters. Such parameters might include an API key that manages user access control over different parts of the data or a constraint that helps in filtering the search. REST URLs can additionally point not only to data but also to backend functions. For example, the URL “ https://www.materialsproject.org/rest/v1/materials/snl/submit ” might trigger a backend function that registers a request to compute the desired structure embedded in an Hypertext Transfer Protocol (HTTP) POST parameter. RESTful APIs can be intimidating to novices but can be made more user-friendly by making the URL scheme explorable and through intermediate software layers. We present a comparison between data dissemination methods in Table I .
A comparison of different methods for exposing data using three different technologies, including positive aspects (pros) and negative aspects (cons) for each selection.
A final technical aspect we discuss is the strategy for combining information from distinct data resources. In particular, data can be stored and managed by a small, large, or intermediate number of entities, which we broadly classify as “centralized,” “decentralized,” and “semi-centralized,” respectively (Figure 5 ).
One organization that has made significant progress in establishing a centralized data resource for materials scientists is Citrine Informatics, a company that specializes in applying data mining to materials discovery and optimization. Citrine’s data sharing service benefits its core business because its proprietary data mining algorithms become more powerful with access to larger datasets. Data providers and research groups that contribute data to the Citrine database benefit from greater visibility of their work and straightforward compliance with data management requirements. Major benefits of such data centralization by industry include no cost to government funding agencies and the ability to leverage a professional software engineering team to build and support the infrastructure. A potential concern is longevity of the data, which is mitigated through an open API that allows making public copies of the data.
As depicted more generally in Figure 5(a) , data contributed to Citrine’s platform (“Citrination”) 121 are reshaped into their custom Materials Information File (MIF) format, 122 a JSON-based materials data standard. The data are hosted on Citrine’s servers and can be accessed by the public either through Citrination’s web interface or through a RESTful API. The Citrination search engine today includes almost 250 data sets from various experimental and computational sources. Examples include data on thermoelectric materials, bulk metallic glasses, and combinatorial photocatalysis experiments.
A different organizational structure, depicted in Figure 5(b) , is to decentralize data providers and to combine data as needed from multiple data APIs. Advantages of this technique include ease of supporting diverse data, a greater likelihood of maintaining up-to-date search results, and reducing the burden (e.g., storage, bandwidth) on any single data provider. A disadvantage is that each data provider must host and maintain its public API. The decentralized system, which is in some ways analogous to the way that Google indexes web pages hosted by many different servers, requires the development of search engines that are capable of working with multiple data resources. For example, in the chemistry community, the ChemSpider 123 search engine has had considerable success with this strategy.
The most likely scenario is that the community will reach an equilibrium that balances both strategies, as in Figure 5(c) . Larger data providers that are continuously generating new data and new features will perhaps maintain their own data service, whereas smaller data outfits that want to submit “one-time” data sets will likely contribute to a larger service.
Many of the issues specific to sharing data in materials have been outlined previously. 124,125 Here, we focus on three key challenges that require innovative solutions: parsing unstructured data, combining multiple data sources, and communicating the nuances of different data sets.
Most materials measurements are not available in a structured format. Instead, they are isolated in laboratory notebooks or embedded within a chart, table, or text of a written document. For example, routes for synthesizing materials are almost exclusively reported as text without a formal data structure. Extracting such data into structured knowledge, e.g., using natural language processing, represents a major challenge for materials research. 126
A second challenge is in combining information from multiple data sets. To illustrate this problem, consider the case of the experimental thermoelectrics data set from the work of Gaultois et al. 127 and a second computational data set from the work of Gorai et al. , 128 both of which can be downloaded from each project’s respective web site or from the Citrination platform. Ideally, one would like to make a comparison, e.g., to assess the accuracy of the computational data with respect to the experimental measurements. Such a study requires matching common entries between databases. As a first step, one can match the entries in each database which pertain to the same composition. However, ensuring that the crystal structures also match is usually much more challenging. In the example of the two thermoelectrics data sets, once can make a match based on Inorganic Crystal Structure Database (ICSD) identification number. In other situations, little to no crystal structure information may be available in one or both data sets. Next, one must align the physical conditions of each entry; in this instance, the computational data are reported at 0 K, whereas the experimental measurements are reported at multiple temperatures between 300 K and 1000 K. Thus, a researcher must select the 300 K experimental data as the most relevant, keeping in mind that the remaining difference in temperature represents a potential source of error. Finally, other material parameters must be considered: for example, the microstructure and defect concentrations of the experimental data can be very different than the pure, single crystal model of many computations. Much of the time, such detailed information on the material is not reported or even known. The situation becomes more troublesome as data sets grow larger because it becomes increasingly time-consuming to employ manual analysis to aid in the process. Better strategies and additional metadata on each measurement are needed to confidently perform analyses across data sets.
A third major challenge is in understanding and communicating the level of accuracy that can be expected from different characterization methods. Interpreting the nuances and evaluating the potential errors of a measurement or simulation technique is typically a subject reserved for domain experts. For example, we have observed that the error bar of “standard” DFT methods in predicting the reaction enthalpies between oxides is approximately 24 meV/atom. 129 However, the error bar is much higher (172 meV/atom) for reactions that involve both metals and oxides. More complicated still, by applying a correction strategy, once can reduce the latter error bar to approximately 45 meV/atom, 130 although different correction strategies have been proposed by different research groups. 131–133 Issues of this kind remain very difficult to communicate to non-specialists but are very important when advocating data reuse.
Looking forward, we expect that learning to work with data, and particularly data that are not self-generated, to become an important skill for material scientists. The integration of computer scientists and statisticians into the domain of materials science will also be vital. The path paved by the biological sciences serves as a good model: bioinformatics and biostatistics are today well-recognized fields in their own right, and biology and health-related fields are recognized as being more multidisciplinary than materials science today. 134
One can also ask whether materials science will be impacted by the revolution in “big data.” 135 While big data can be a nebulous term, reports often point to the “three V’s”—i.e., the volume, velocity, and variety of the data (and sometimes extended to include veracity and value). 136 Other definitions of big data are that it occurs when there are significant problems in loading, transferring, and processing the data set using conventional hardware and software, or when the data size reaches a scale that enables qualitatively new types of analysis approaches. With respect to volume and velocity (rate at which data is generated), materials science has not hit the “big data” mark as compared with other fields. For example, the European Bioinformatics Institute stores approximately 20 petabytes of data, and the European Organization for Nuclear Research (CERN) generates approximately 15 petabytes of data and has stored approximately 200 petabytes of results. 137 For comparison, all the raw data stored in the MP amount to approximately 100 terabytes (a factor of 2000 less than CERN) and the final data sets exposed to users amount to approximately 1 terabyte. Data from large experimental facilities such as light sources may change this assessment in the future, 135 as might software that allows running several orders of magnitude more computations on ever-larger computing resources. 138–140 Although materials science data are not particularly large, it is challenging to work with in terms of the variety of data types and the complexity of objects such as periodically repeating crystals or mass spectroscopy data. As for whether data sets will open new qualitative research avenues, the size of materials data sets is likely still too small to apply some machine learning techniques such as “deep learning.” 141,142 However, more efficient machine learning algorithms for such kinds of learning are being developed 143 which hint that the coming wave in materials data will indeed open up new types of analysis methods.
Transitioning from data to insight will remain a major research topic. Much recent research has begun applying data mining techniques to materials data sets, but “materials informatics” 144 remains a nascent field of study. To propel this field forward, Materials Hackathons 145 organized by Citrine Informatics and a Materials Data Challenge hosted by NIST 146 have been announced to encourage advancement through competition. Further progress might be stimulated by incorporating data visualization and analysis tools directly into common materials database portals. For example, we envision that in the future, one will be able to log on to the MP, identify a target property, visualize the distribution of that property over known materials, extract relevant descriptors, and build and test a predictive model.
Many challenges lie ahead for uncovering the materials genome, i.e., to understand what factors are responsible for the complex behavior of advanced materials through the use of data-driven methods. However, the recent examples demonstrating that theorists and experimental researchers alike can apply online ab initio computation databases towards cutting-edge research problems is an encouraging harbinger of a new and exciting era in collaborative materials discovery.
This work was funded and intellectually led by the Materials Project (DOE Basic Energy Sciences Grant No. EDCBEE). Work at the Lawrence Berkeley National Laboratory was supported by the U.S. Department of Energy Office of Science, Office of Basic Energy Sciences Department under Contract No. DE-AC02-05CH11231. We thank Bryce Meredig for discussions regarding the Citrination search platform.
Citing articles via
Submit your article.
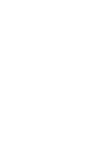
Sign up for alerts
- Online ISSN 2166-532X
- For Researchers
- For Librarians
- For Advertisers
- Our Publishing Partners
- Physics Today
- Conference Proceedings
- Special Topics
pubs.aip.org
- Privacy Policy
- Terms of Use
Connect with AIP Publishing
This feature is available to subscribers only.
Sign In or Create an Account
- REALTOR® Store

- Fostering Consumer-Friendly Real Estate Marketplaces Local broker marketplaces ensure equity and transparency. Close
- Social Media
- Sales Tips & Techniques
- MLS & Online Listings
- Starting Your Career
- Being a Broker
- Being an Agent
- Condominiums
- Smart Growth
- Vacation, Resort, & 2nd Homes
- FHA Programs
- Home Inspections
- Arbitration & Dispute Resolution
- Fair Housing
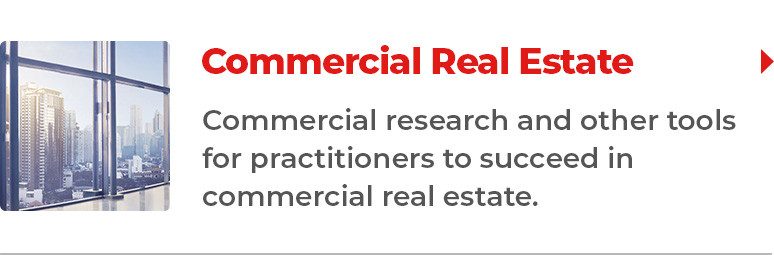
- All Membership Benefits
- NAR REALTOR Benefits® Bringing you savings and unique offers on products and services just for REALTORS®. Close
- Directories Complete listing of state and local associations, MLSs, members, and more. Close
- Dues Information & Payment
- Become a Member As a member, you are the voice for NAR – it is your association and it exists to help you succeed. Close
- Logos and Trademark Rules Only members of NAR can call themselves a REALTOR®. Learn how to properly use the logo and terms. Close
- Your Membership Account Review your membership preferences and Code of Ethics training status. Close
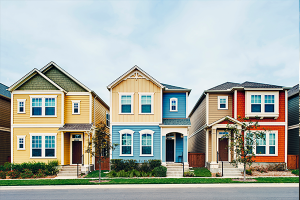
- Highlights & News Get the latest top line research, news, and popular reports. Close
- Housing Statistics National, regional, and metro-market level housing statistics where data is available. Close
- Research Reports Research on a wide range of topics of interest to real estate practitioners. Close
- Presentation Slides Access recent presentations from NAR economists and researchers. Close
- State & Metro Area Data Affordability, economic, and buyer & seller profile data for areas in which you live and work. Close
- Commercial Research Analysis of commercial market sectors and commercial-focused issues and trends. Close
- Statistical News Release Schedule
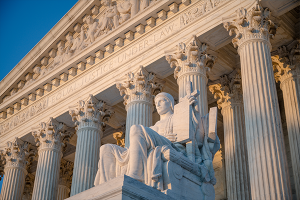
- Advocacy Issues & News
- Federal Advocacy From its building located steps away from the U.S. Capitol, NAR advocates for you. Close
- REALTORS® Political Action Committee (RPAC) Promoting the election of pro-REALTOR® candidates across the United States. Close
- State & Local Advocacy Resources to foster and harness the grassroots strength of the REALTOR® Party. Close
- REALTOR® Party A powerful alliance working to protect and promote homeownership and property investment. Close
- Get Involved Now more than ever, it is critical for REALTORS® across America to come together and speak with one voice. Close

- All Education & Professional Development
- All NAR & Affiliate Courses Continuing education and specialty knowledge can help boost your salary and client base. Close
- Code of Ethics Training Fulfill your COE training requirement with free courses for new and existing members. Close
- Continuing Education (CE) Meet the continuing education (CE) requirement in state(s) where you hold a license. Close
- Designations & Certifications Acknowledging experience and expertise in various real estate specialties, awarded by NAR and its affiliates. Close
- Library & Archives Offering research services and thousands of print and digital resources. Close
- Commitment to Excellence (C2EX) Empowers REALTORS® to evaluate, enhance and showcase their highest levels of professionalism. Close
- NAR Academy at Columbia College Academic opportunities for certificates, associates, bachelor’s, and master’s degrees. Close
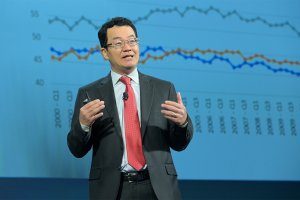
- Latest News
- NAR Newsroom Official news releases from NAR. Close
- REALTOR® Magazine Advancing best practices, bringing insight to trends, and providing timely decision-making tools. Close
- Blogs Commentary from NAR experts on technology, staging, placemaking, and real estate trends. Close
- Newsletters Stay informed on the most important real estate business news and business specialty updates. Close
- NAR NXT, The REALTOR® Experience
- REALTORS® Legislative Meetings
- AE Institute
- Leadership Week
- Sustainability Summit
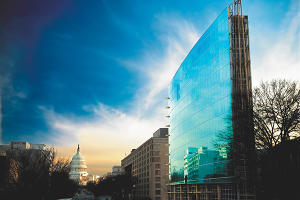
- Mission, Vision, and Diversity & Inclusion
- Code of Ethics
- Leadership & Staff National, state & local leadership, staff directories, leadership opportunities, and more. Close
- Committee & Liaisons
- History Founded as the National Association of Real Estate Exchanges in 1908. Close
- Affiliated Organizations
- Strategic Plan NAR’s operating values, long-term goals, and DEI strategic plan. Close
- Governing Documents Code of Ethics, NAR's Constitution & Bylaws, and model bylaws for state & local associations. Close
- Awards & Grants Member recognition and special funding, including the REALTORS® Relief Foundation. Close
- NAR's Consumer Outreach
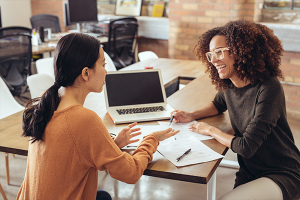
- Find a Member
- Browse All Directories
- Find an Office
- Find an Association
- NAR Group and Team Directory
- Committees and Directors
- Association Executive
- State & Local Volunteer Leader
- Buyer's Rep
- Senior Market
- Short Sales & Foreclosures
- Infographics
- First-Time Buyer
- Window to the Law
- Next Up: Commercial
- New AE Webinar & Video Series
- Drive With NAR
- Real Estate Today
- The Advocacy Scoop
- Center for REALTOR® Development
- Leading with Diversity
- Good Neighbor
- NAR HR Solutions
- Fostering Consumer-Friendly Real Estate Marketplaces Local broker marketplaces ensure equity and transparency.
- Marketing Social Media Sales Tips & Techniques MLS & Online Listings View More
- Being a Real Estate Professional Starting Your Career Being a Broker Being an Agent View More
- Residential Real Estate Condominiums Smart Growth Vacation, Resort, & 2nd Homes FHA Programs View More Home Inspections
- Legal Arbitration & Dispute Resolution Fair Housing Copyright View More
- Commercial Real Estate
- Right Tools, Right Now
- NAR REALTOR Benefits® Bringing you savings and unique offers on products and services just for REALTORS®.
- Directories Complete listing of state and local associations, MLSs, members, and more.
- Become a Member As a member, you are the voice for NAR – it is your association and it exists to help you succeed.
- Logos and Trademark Rules Only members of NAR can call themselves a REALTOR®. Learn how to properly use the logo and terms.
- Your Membership Account Review your membership preferences and Code of Ethics training status.
- Highlights & News Get the latest top line research, news, and popular reports.
- Housing Statistics National, regional, and metro-market level housing statistics where data is available.
- Research Reports Research on a wide range of topics of interest to real estate practitioners.
- Presentation Slides Access recent presentations from NAR economists and researchers.
- State & Metro Area Data Affordability, economic, and buyer & seller profile data for areas in which you live and work.
- Commercial Research Analysis of commercial market sectors and commercial-focused issues and trends.
- Federal Advocacy From its building located steps away from the U.S. Capitol, NAR advocates for you.
- REALTORS® Political Action Committee (RPAC) Promoting the election of pro-REALTOR® candidates across the United States.
- State & Local Advocacy Resources to foster and harness the grassroots strength of the REALTOR® Party.
- REALTOR® Party A powerful alliance working to protect and promote homeownership and property investment.
- Get Involved Now more than ever, it is critical for REALTORS® across America to come together and speak with one voice.
- All NAR & Affiliate Courses Continuing education and specialty knowledge can help boost your salary and client base.
- Code of Ethics Training Fulfill your COE training requirement with free courses for new and existing members.
- Continuing Education (CE) Meet the continuing education (CE) requirement in state(s) where you hold a license.
- Designations & Certifications Acknowledging experience and expertise in various real estate specialties, awarded by NAR and its affiliates.
- Library & Archives Offering research services and thousands of print and digital resources.
- Commitment to Excellence (C2EX) Empowers REALTORS® to evaluate, enhance and showcase their highest levels of professionalism.
- NAR Academy at Columbia College Academic opportunities for certificates, associates, bachelor’s, and master’s degrees.
- NAR Newsroom Official news releases from NAR.
- REALTOR® Magazine Advancing best practices, bringing insight to trends, and providing timely decision-making tools.
- Blogs Commentary from NAR experts on technology, staging, placemaking, and real estate trends.
- Newsletters Stay informed on the most important real estate business news and business specialty updates.
- Leadership & Staff National, state & local leadership, staff directories, leadership opportunities, and more.
- History Founded as the National Association of Real Estate Exchanges in 1908.
- Strategic Plan NAR’s operating values, long-term goals, and DEI strategic plan.
- Governing Documents Code of Ethics, NAR's Constitution & Bylaws, and model bylaws for state & local associations.
- Awards & Grants Member recognition and special funding, including the REALTORS® Relief Foundation.
- Top Directories Find a Member Browse All Directories Find an Office Find an Association NAR Group and Team Directory Committees and Directors
- By Role Broker Association Executive New Member Student Appraiser State & Local Volunteer Leader
- By Specialty Commercial Global Buyer's Rep Senior Market Short Sales & Foreclosures Land Green
- Multimedia Infographics Videos Quizzes
- Video Series First-Time Buyer Level Up Window to the Law Next Up: Commercial New AE Webinar & Video Series
- Podcasts Drive With NAR Real Estate Today The Advocacy Scoop Center for REALTOR® Development
- Programs Fair Housing Safety Leading with Diversity Good Neighbor NAR HR Solutions
Understand Market Behavior
Research and statistics, nar produces and analyzes a wide range of real estate data that can help guide your business and your clients..
- Housing Statistics
- State & Metro Area Data
- Research Reports
- Presentations
Latest Housing Indicators
Median Price
*seasonally adjusted annual rate
Quarterly U.S. Economic Forecast (PDF: 130 KB) Citation guidelines for NAR Research & Statistics
Posts from @NAR_Research
Latest housing statistics and real estate market trends.
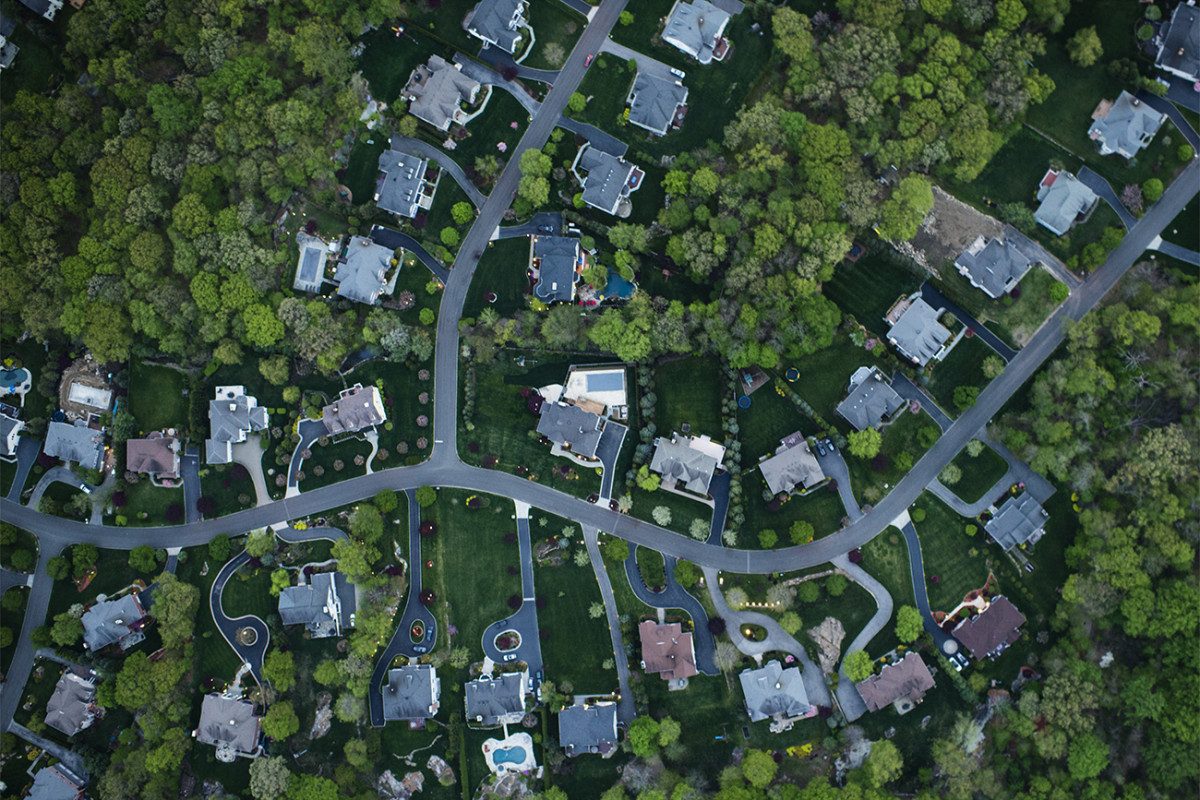
Housing Affordability Index
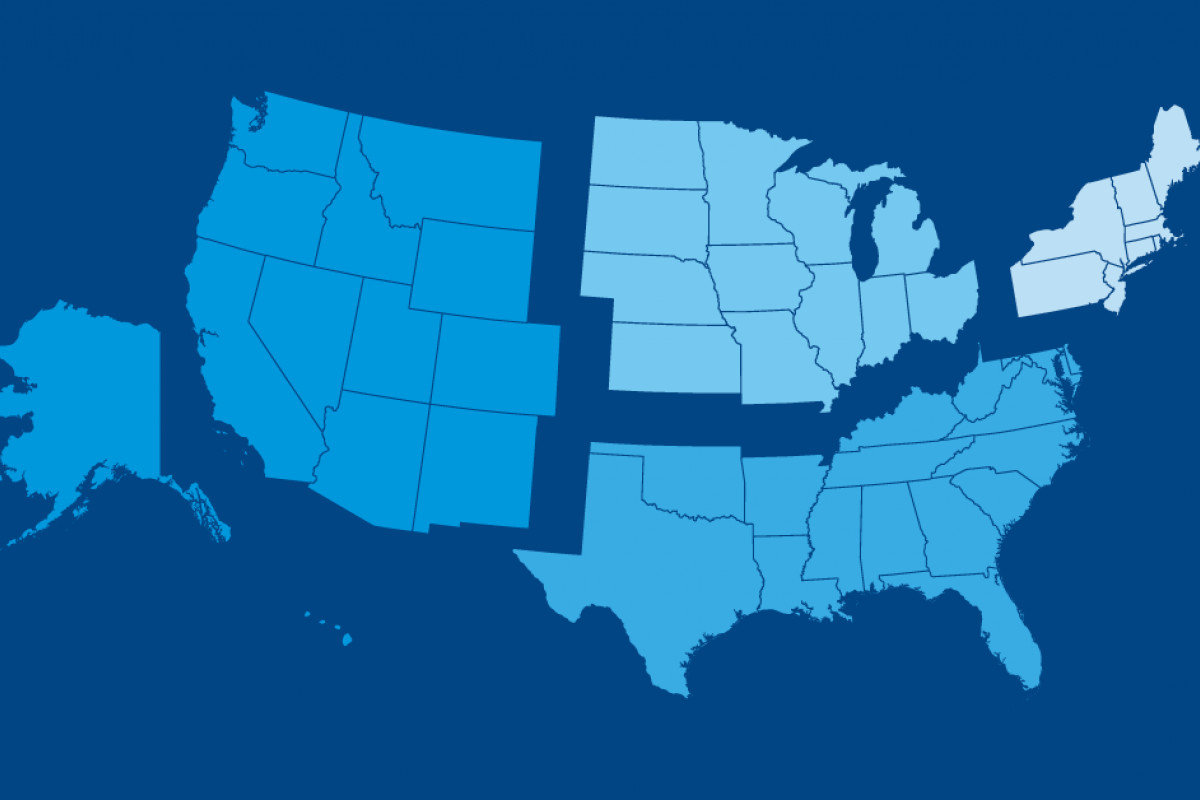
Pending Home Sales Snapshot
- See and share this infographic .
- Get the latest data .
- Read the news release .
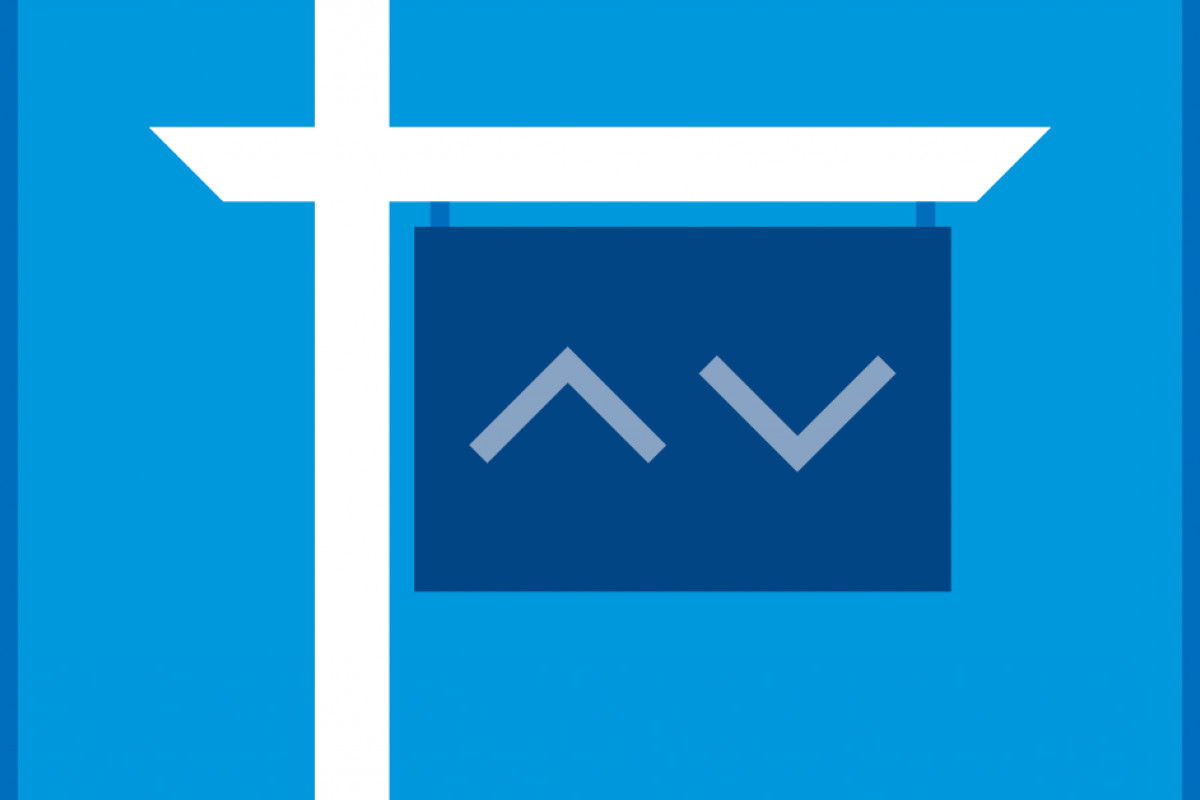
Existing-Home Sales Housing Snapshot
Existing-home sales, pending home sales, housing statistics and real estate market trends.
National, regional, and metro-market level housing statistics where data is available.
Latest State & Metro Area Data

REALTORS® Affordability Distribution Curve and Score

Home Buyers by Metropolitan Statistical Area

County Median Home Prices and Monthly Mortgage Payment
The latest from our research desks.

The Housing Market Dual Impact of Lower Mortgage Rates
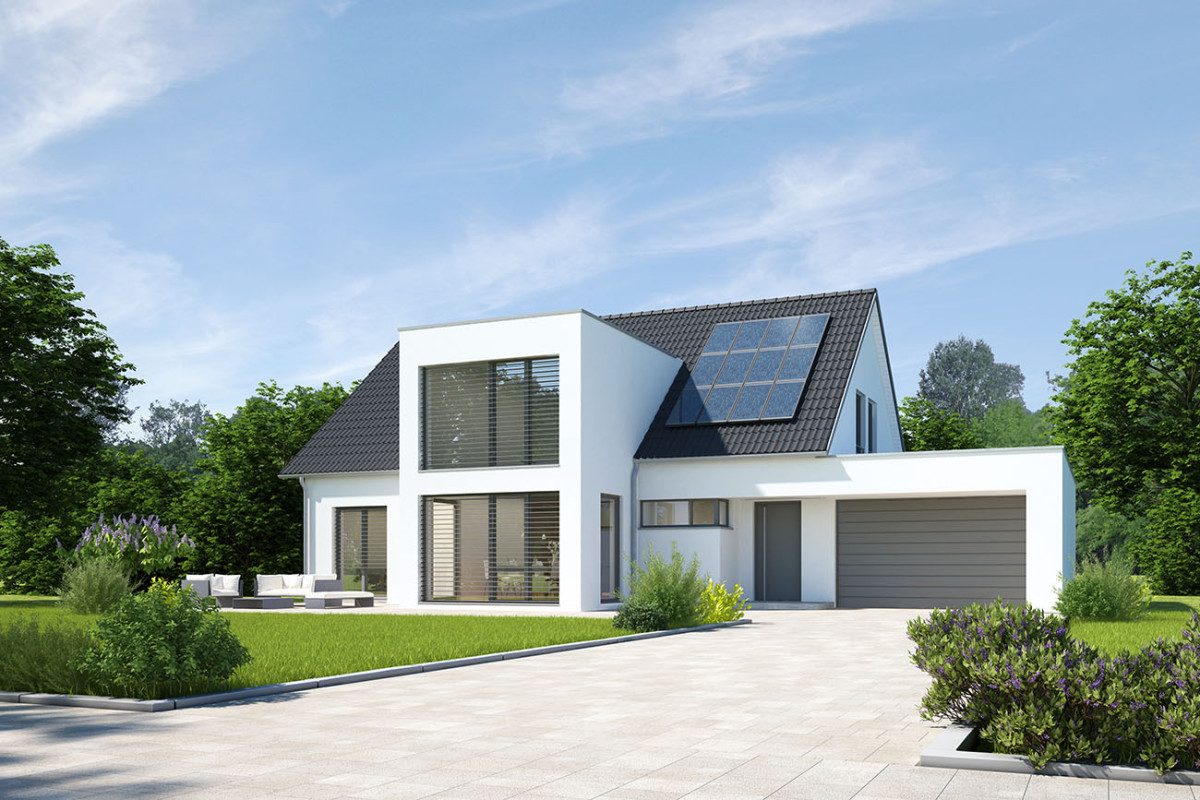
Residential Real Estate Market Snapshot
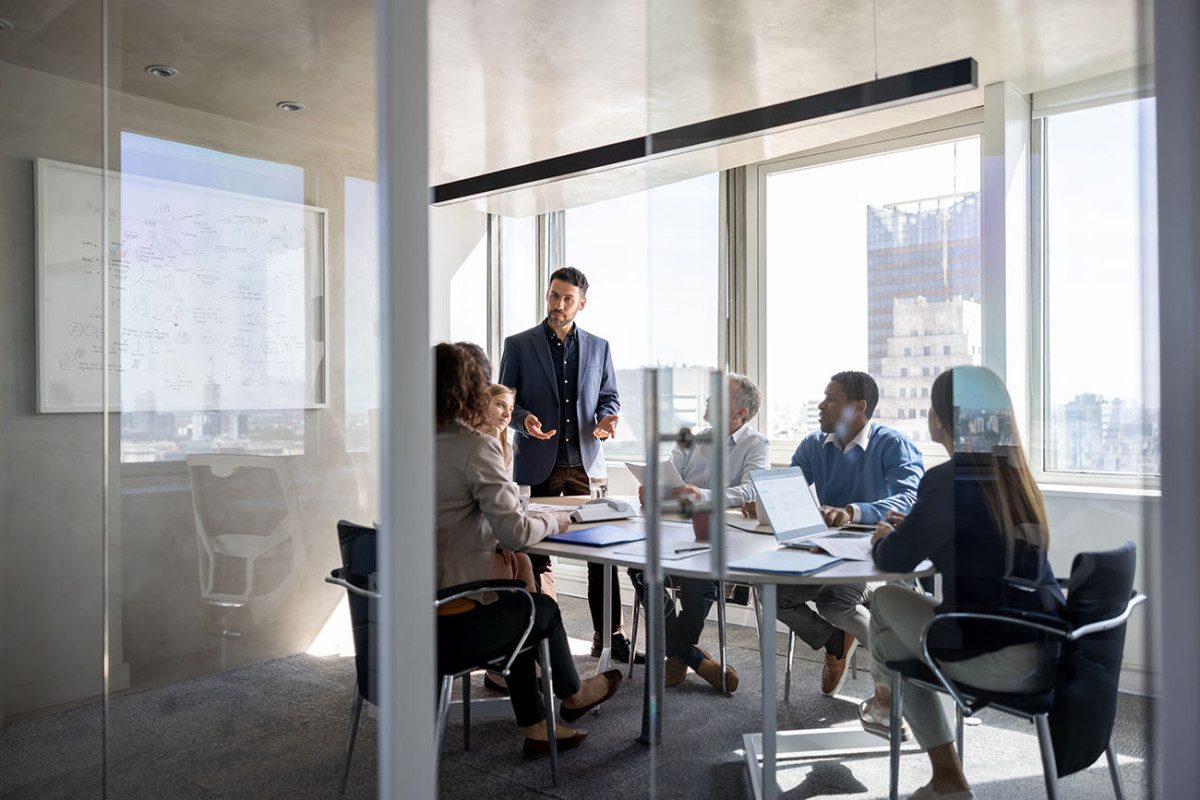
April 2024 Commercial Real Estate Market Insights
View All Reports
Featured Presentations
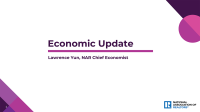
May 2024 Economic Update May 7, 2024: Presented by NAR Chief Economist Lawrence Yun at the Residential Economic Issues & Trends Forum at the 2024 REALTORS® Legislative Meetings

Messaging the Data May 7, 2024: Presented by NAR Deputy Chief Economist Jessica Lautz at the Residential Economic Issues & Trends Forum at the 2024 REALTORS® Legislative Meetings

May 2024 Commercial Update May 7, 2024: Presented by NAR Chief Economist Lawrence Yun at the Commercial Economic Issues & Trends Forum at the 2024 REALTORS® Legislative Meetings
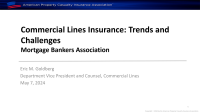
Commercial Lines: Insurance Trends May 7, 2024: Presented by Eric M. Goldberg, Department Vice President and Counsel, Commercial Lines for the American Property Casualty Insurance Association, at the Commercial Economic Issues & Trends Forum at the 2024 REALTORS® Legislative Meetings in Washington, DC.

Exploration of Retail Crime and Commercial Update May 5, 2024: Presented by Oleh Sorokin at the Commercial Real Estate Research Advisory Board at the 2024 REALTORS® Legislative Meetings in Washington, DC.

Commercial and Economic Outlook November 15, 2023: Presented by NAR Chief Economist Lawrence Yun at 2023 NAR NXT: The REALTOR® Experience.
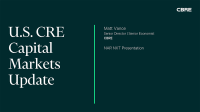
U.S. CRE Capital Market Update November 15, 2023: Presented by Matt Vance at 2023 NAR NXT: The REALTOR® Experience.
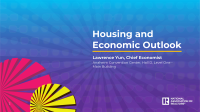
Housing and Economic Outlook November 14, 2023: Presented by NAR Chief Economist Lawrence Yun at 2023 NAR NXT: The REALTOR® Experience.
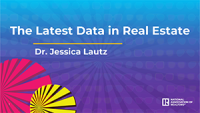
The Latest Data in Real Estate November 13, 2023: Presented by NAR Deputy Chief Economist Dr. Jessica Lautz at 2023 NAR NXT: The REALTOR® Experience.
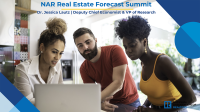
NAR Real Estate Forecast Summit August 2023 August 8, 2023: Presented by NAR Deputy Chief Economist Jessica Lautz at the NAR Real Estate Forecast Summit.
View All Presentations
Latest Research News
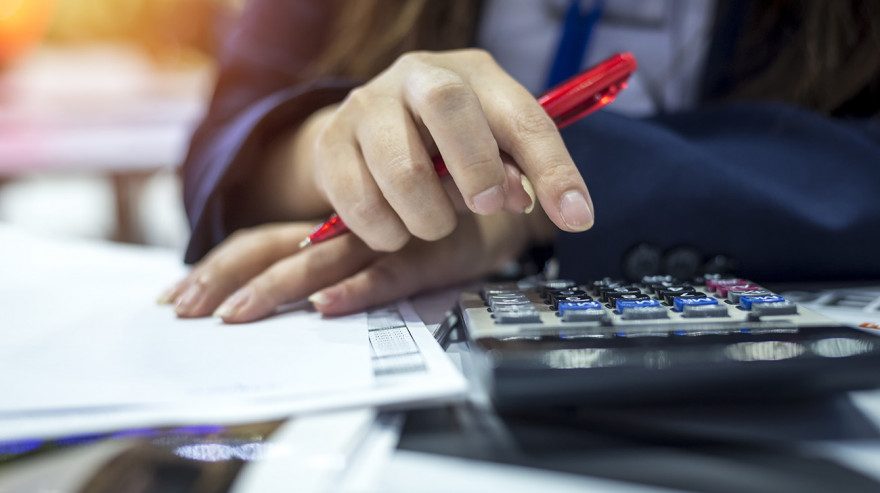
Instant Reaction: Mortgage Rates, May 23, 2024
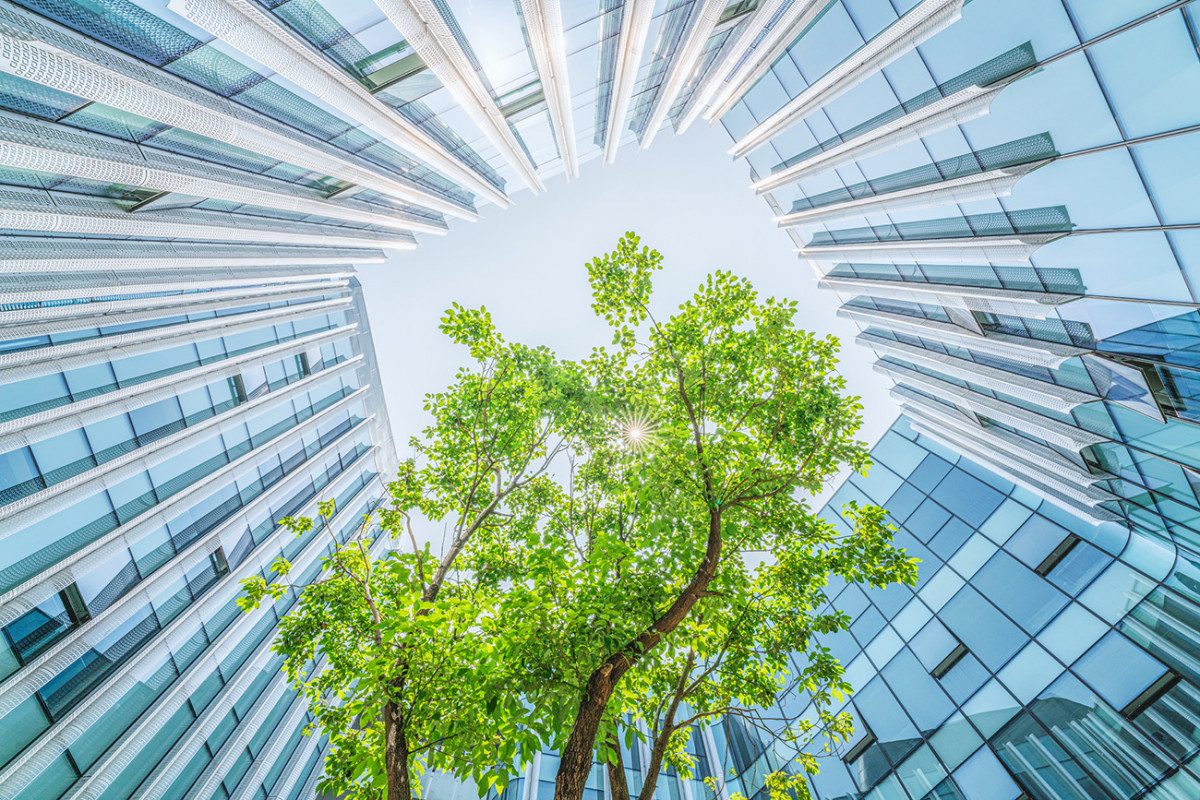
Insights from the 2024 Sustainability Survey
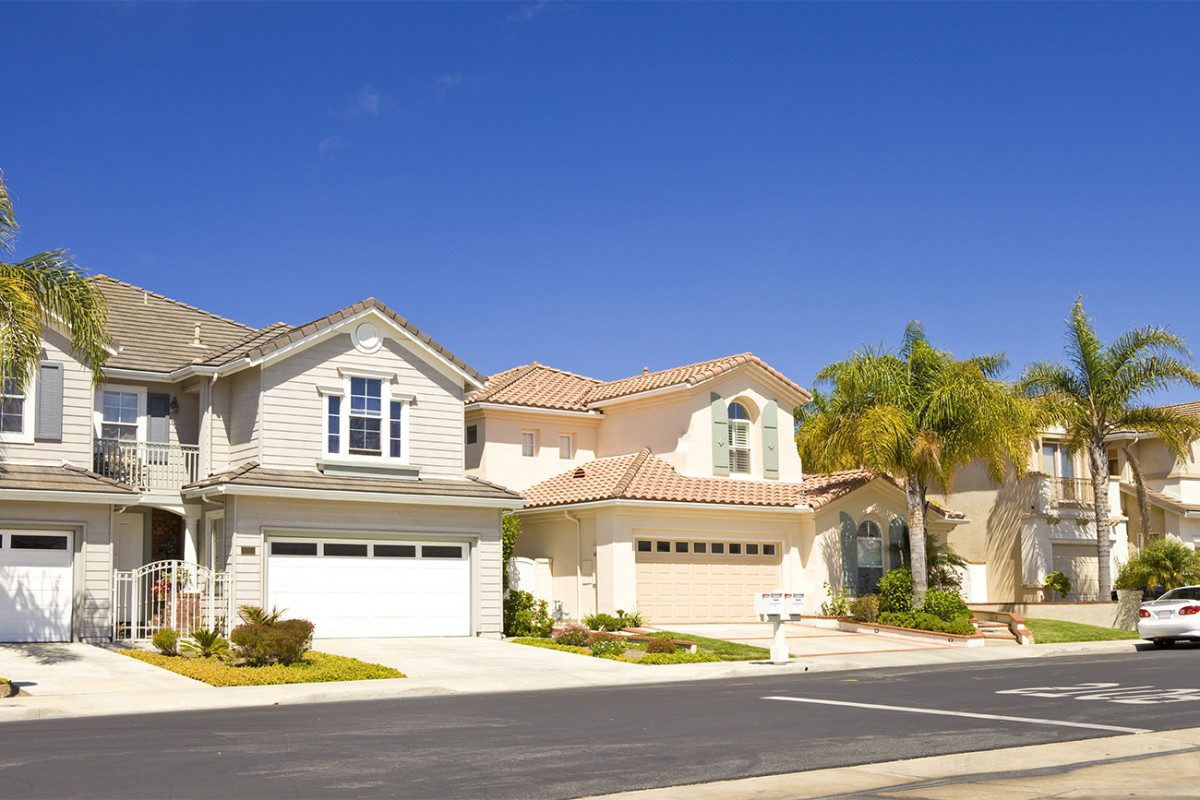
April 2024 Existing-Home Sales Take a Step Back

April 2024 Foot Traffic

REALTORS® Confidence Index
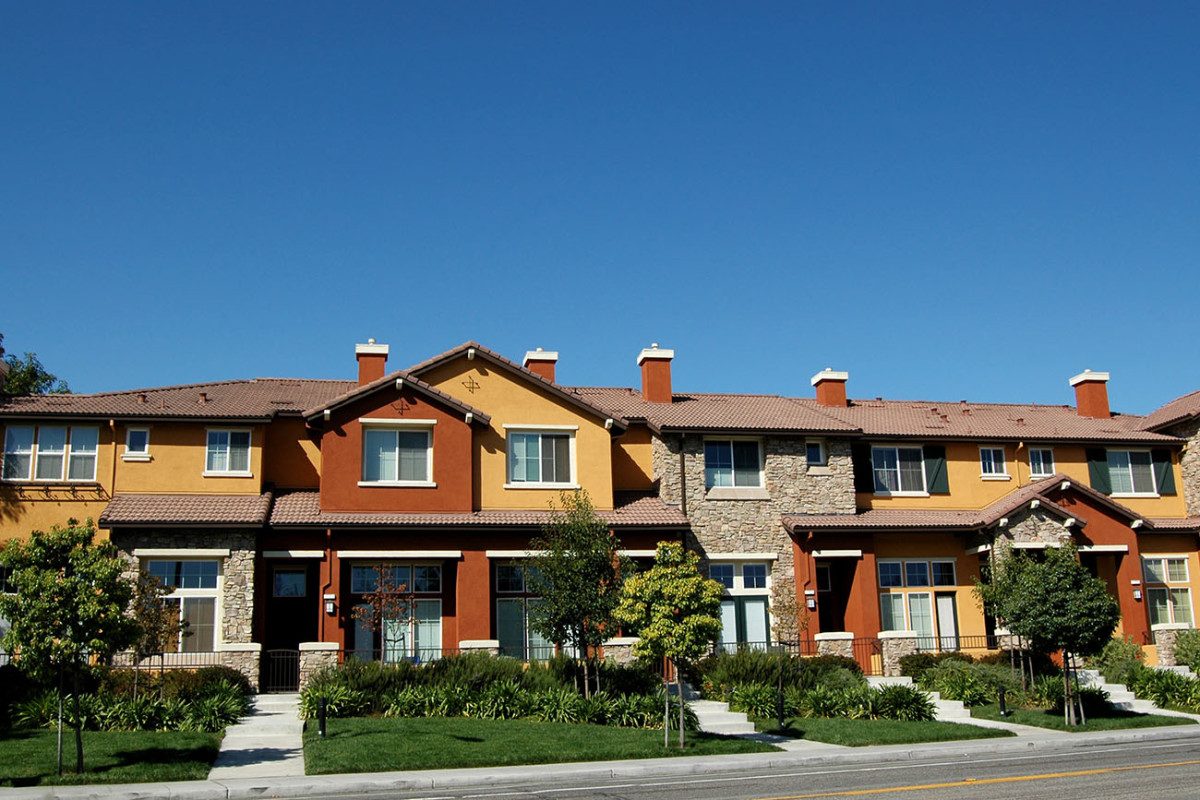
Existing-Home Sales Retreated 1.9% in April
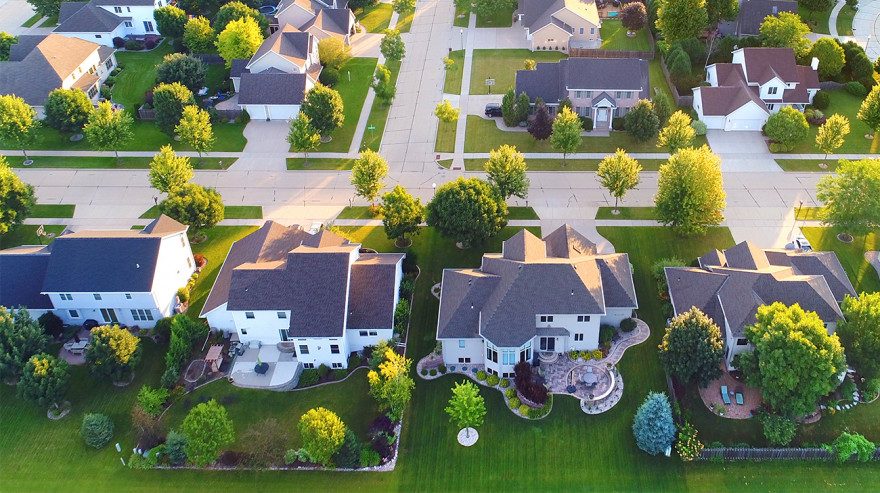
The Most Popular and Fastest Growing Metro Areas in '23
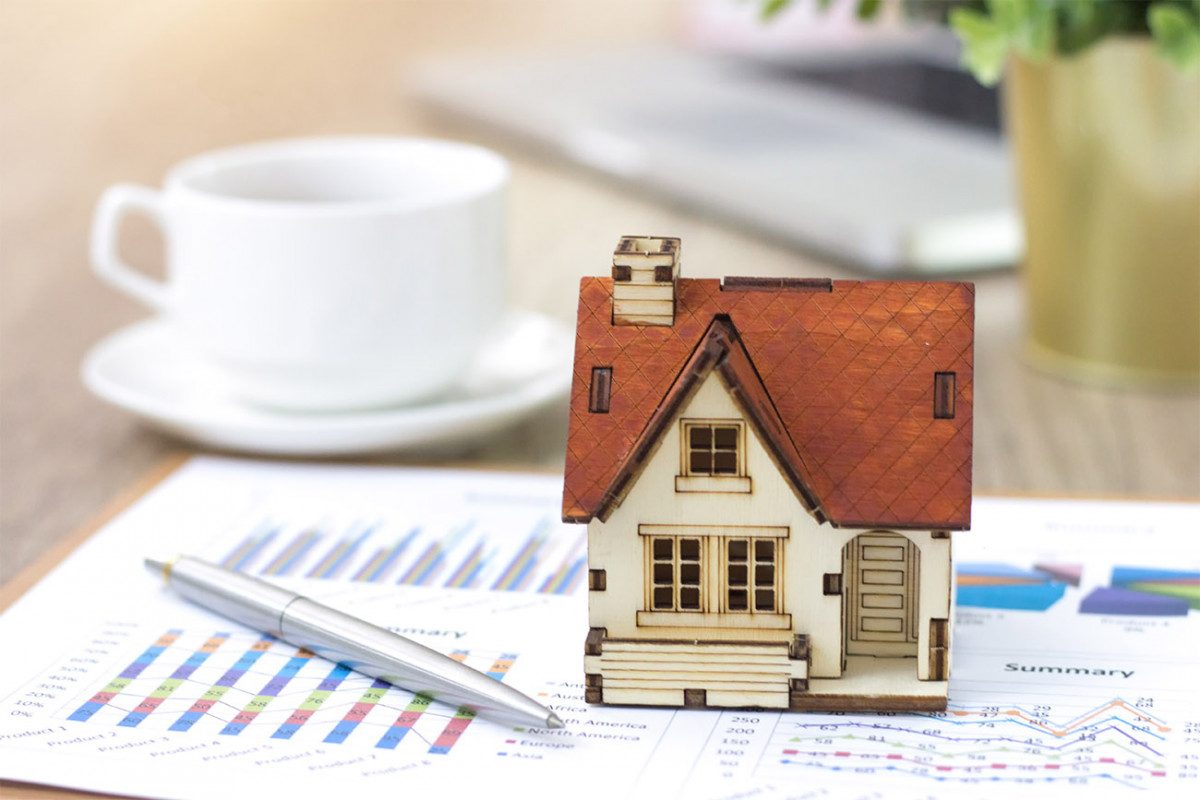
Instant Reaction: Mortgage Rates, May 16, 2024
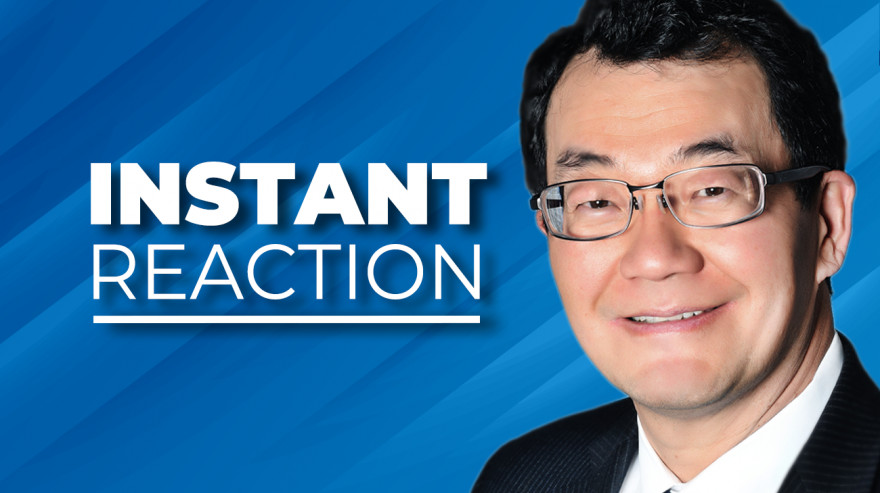
Instant Reaction: Housing Starts, May 16, 2024
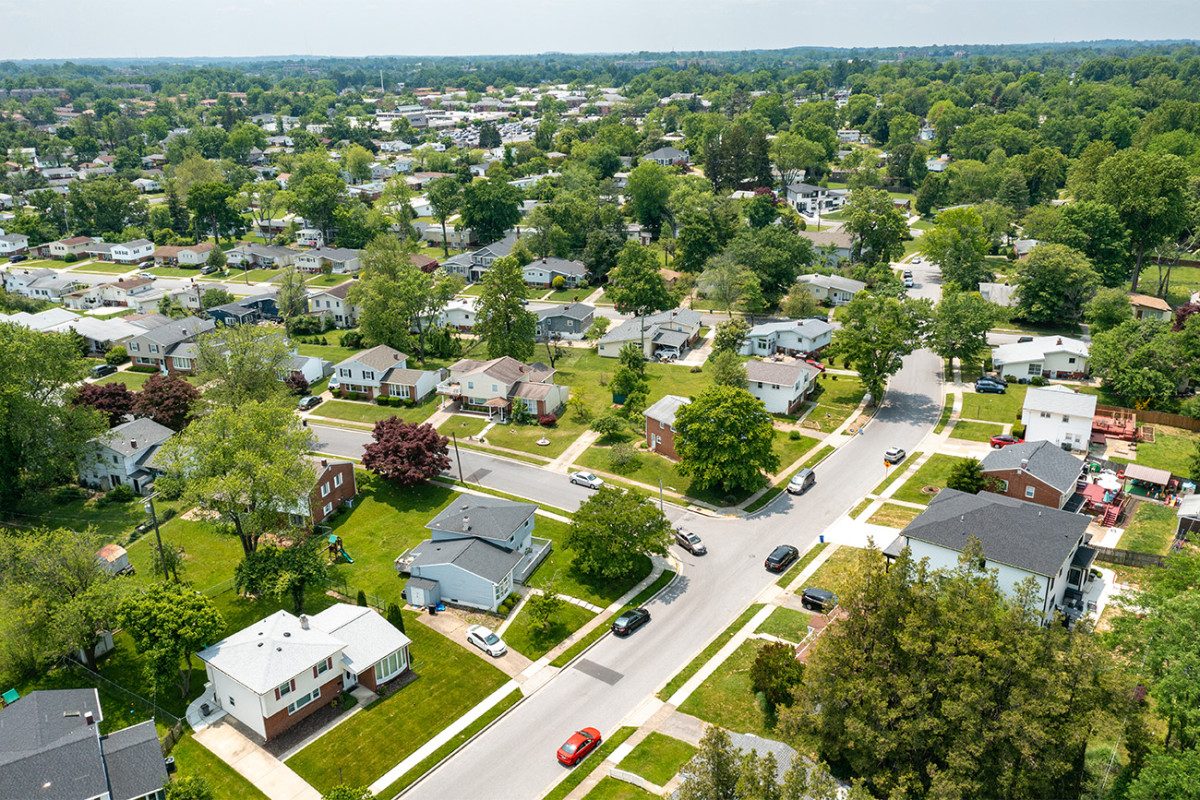
Q1 Single-family Home Prices Up in 92.3% of Metro Areas
Follow nar research.

By subscribing to Navigate With NAR, managed by SmartBrief , you are agreeing to receive one daily email with news, offers, and information from the National Association of REALTORS® and SmartBrief. Visit our Privacy Policy . Easy unsubscribe links are provided in every email.
Internet Explorer Alert
It appears you are using Internet Explorer as your web browser. Please note, Internet Explorer is no longer up-to-date and can cause problems in how this website functions This site functions best using the latest versions of any of the following browsers: Edge, Firefox, Chrome, Opera, or Safari . You can find the latest versions of these browsers at https://browsehappy.com
- Publications
- HealthyChildren.org
Shopping cart
Order Subtotal
Your cart is empty.
Looks like you haven't added anything to your cart.
- Career Resources
- Philanthropy
- About the AAP
- Infant Feeding for Persons Living With and at Risk for HIV in the United States: Clinical Report
- Research on HIV transmission through breastfeeding spurs update to AAP guidance
- Breastfeeding for People with HIV: AAP Policy Explained
- American Academy of Pediatrics Shifts Position on Breastfeeding for People with Human Immunodeficiency Virus
- News Releases
- Policy Collections
- The State of Children in 2020
- Healthy Children
- Secure Families
- Strong Communities
- A Leading Nation for Youth
- Transition Plan: Advancing Child Health in the Biden-Harris Administration
- Health Care Access & Coverage
- Immigrant Child Health
- Gun Violence Prevention
- Tobacco & E-Cigarettes
- Child Nutrition
- Assault Weapons Bans
- Childhood Immunizations
- E-Cigarette and Tobacco Products
- Children’s Health Care Coverage Fact Sheets
- Opioid Fact Sheets
- Advocacy Training Modules
- Subspecialty Advocacy Report
- AAP Washington Office Internship
- Online Courses
- Live and Virtual Activities
- National Conference and Exhibition
- Prep®- Pediatric Review and Education Programs
- Journals and Publications
- NRP LMS Login
- Patient Care
- Practice Management
- AAP Committees
- AAP Councils
- AAP Sections
- Volunteer Network
- Join a Chapter
- Chapter Websites
- Chapter Executive Directors
- District Map
- Create Account
- News from the AAP
- Latest Studies in Pediatrics
- Pediatrics OnCall Podcast
- AAP Voices Blog
- Campaigns and Toolkits
- Spokesperson Resources
- Join the AAP
- Exclusive for Members
- Membership FAQs
- AAP Membership Directory
- Member Advantage Programs
- Red Book Member Benefit
- My Membership
- Join a Council
- Join a Section
- National Election Center
- Medical Students
- Pediatric Residents
- Fellowship Trainees
- Planning Your Career
- Conducting Your Job Search
- Making Career Transitions
- COVID-19 State-Level Data Reports
- Children and COVID-19 Vaccination Trends
- Practice Research in the Office Setting (PROS)
- Pediatrician Life and Career Experience Study (PLACES)
- Periodic Survey
- Annual Survey of Graduating Residents
- Child Population Characteristics Trends
- Child Health Trends
- Child Health Care Trends
- Friends of Children Fund
- Tomorrow’s Children Endowment
- Disaster Recovery Fund
- Monthly Giving Plans
- Honor a Person You Care About
- Donor-Advised Funds
- AAP in Your Will
- Become a Corporate Partner
- Employment Opportunities
- Equity and Inclusion Efforts
- RFP Opportunities
- Board of Directors
- Senior Leadership Team
- Constitution & By-Laws
- Strategic Plan
AAP News Research Updates
The following are AAP Research articles published in AAP News in the last 5 years:
Survey highlights pediatricians’ international backgrounds March 2024
AAP grants provide research opportunities for residents February 2024
Racial, ethnic disparities remain among U.S. children living in poverty December 2023
Hispanic children twice as likely to be uninsured as children of other races/ethnicities November 2023
AAP study: Most early career pediatricians own home despite high educational debt September 2023
Pediatric COVID-19 hospitalizations at lowest recorded level August 2023
Pediatricians report 18.5% of patient families have limited proficiency with English July 2023
Pediatricians value injury prevention counseling but face barriers June 2023
Annual survey shows most pediatric residents land job they want May 2023
AAP study shows telehealth use common in pediatric care April 2023
Nearly 6 million children gained Medicaid/CHIP coverage during pandemic March 2023
Grants aim to kick-start pediatric residents' research careers January 2023
COVID-19 hospital admissions rising among children, especially those under 5 December 2022
AAP membership doubles since 1987; women make up majority December 2022
AAP study shows texting parents can improve timely uptake of 2nd flu shot November 2022
AAP studies examine prevalence of burnout, strategies to reduce occurrence October 2022
Survey shows most pediatricians find work rewarding but some are frustrated September 2022
AAP Surveys Show 14% of Pediatricians, 69% of Residents Treated Firearm Injuries August 2022
AAP Survey Highlights Diverse Backgrounds of Graduating Pediatric Residents July 2022
Hispanic, Black youths represent disproportionate percent of COVID hospitalizations May 2022
AAP study: Pediatricians satisfied with career despite pandemic challenges April 2022
Residency graduates surveyed on pandemic’s effect on training, job search March 2022
Deadline to apply for Resident Research Grants is Feb. 28 January 2022 AAP study: Most early career pediatricians would choose same subspecialty December 2021
US child population decreasing, becoming more diverse November 2021
Survey: Pediatricians anticipate COVID-19 vaccine hesitancy among parents October 2021
Just over half of US children insured by public programs September 2021
Communication training linked to higher HPV vaccination rates August 2021
US births continue declining, a trend that started in 2008 July 2021
1 year of data shows how pediatric COVID-19 cases ebbed and flowed June 2021
Analyses of pediatric COVID-19 cases in 2 states highlight disparities May 2021
Early career pediatricians satisfied with work despite challenges April 2021
Annual survey shows graduating residents have high debt load, high career satisfaction March 2021
High rates of teen vaping reported in early 2020 February 2021
Survey: Pediatricians reeling from pandemic's sustained impact January 2021
AAP grants provide research opportunities for residents January 2021
Percent of children without health insurance rising; Hispanics fare worst December 2020
Study looks at persistence of pediatric hypertension during childhood November 2020
Intervention reduces unnecessary outpatient antibiotic prescribing for children October 2020
56% of pediatricians say they can complete recommended screenings September 2020
Who are America's children? AAP analyzes federal data August 2020
Survey: Pandemic disrupting practices, finances of early, midcareer pediatricians June 2020
Graduating pediatric residents report factors influencing subspecialty choice May 2020
Percent of pediatricians facing malpractice claims drops March 2020
Have passport, will travel: Global health piques more pediatricians' interest March 2020
Deadline to apply for Resident Research Grant is Feb. 28 January 2019
Majority of early and midcareer pediatricians bring work home December 2019
Survey: Suicide, suicidal ideation encountered often in pediatric practice November 2019 Studies find gender disparities in pediatrician pay, household duties October 2019 Minority of pediatricians routinely screen for social needs September 2019
100th Periodic Survey compares pediatricians' concerns in 1987 with 2018 August 2019
Nearly 70% of pediatric residents report caring for children with gun injuries July 2019
Residents satisfied with training but may struggle with work-life balance May 2019
Study compared experiences of U.S., international medical school graduates April 2019
Survey: EHRs a mainstay of practice, but pediatric functionality lacking March 2019
Grant aims to kick-start pediatric residents' research careers January 2019
Study: Young Pediatricians satisfied with careers December 2018
Child poverty rates improve but disparities persist November 2018
Study: pediatricians surveyed on acute care provided outside medical home September 2018
Study examines factors associated with resident choice to enter hospitalist workforce July 2018 Nearly all pediatric residents find job after graduation May 2018
What do early career pediatricians find stressful? April 2018
Survey: Graduating residents' debt levels off, salaries rise modestly March 2018 More pediatricians counseling on sun safety; few discuss indoor tanning February 2018
Deadline to apply for Resident Research Grants is Feb. 28th January 2018
Study looks at trends in breastfeeding attitudes, counseling practices November 2017
Survey: Most pediatricians take family history, fewer order genetic tests October 2017
Percent of AAP members who are women increases from 28%-63% over 30 years September 2017
Survey: Many pediatricians don't follow lipid recommendations August 2017
Study: Primary care pediatricians working fewer hours July 2017
Study analyzes ADHD diagnosis, stimulant use after guideline released June 2017
Nearly all residents satisfied with training; few satisfied with time devoted to practice management May 2017
Study: Most young pediatricians own home despite high debt April 2017
Most pediatricians advise families to quit smoking, but few assist with cessation March 2017
Study: Only 23% of youths with hypertension receive diagnosis February 2017 AAP grants provide research opportunities for residents January 2017
Last Updated
American Academy of Pediatrics
- About the New York Fed
- Bank Leadership
- Diversity and Inclusion
- Communities We Serve
- Board of Directors
- Disclosures
- Ethics and Conflicts of Interest
- Annual Financial Statements
- News & Events
- Advisory Groups
- Vendor Information
- Holiday Schedule
At the New York Fed, our mission is to make the U.S. economy stronger and the financial system more stable for all segments of society. We do this by executing monetary policy, providing financial services, supervising banks and conducting research and providing expertise on issues that impact the nation and communities we serve.
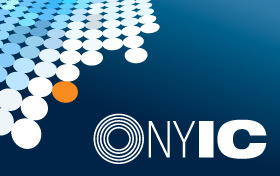
The New York Innovation Center bridges the worlds of finance, technology, and innovation and generates insights into high-value central bank-related opportunities.
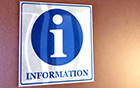
Do you have a request for information and records? Learn how to submit it.
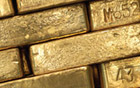
Learn about the history of the New York Fed and central banking in the United States through articles, speeches, photos and video.
- Markets & Policy Implementation
- Reference Rates
- Effective Federal Funds Rate
- Overnight Bank Funding Rate
- Secured Overnight Financing Rate
- SOFR Averages & Index
- Broad General Collateral Rate
- Tri-Party General Collateral Rate
- Desk Operations
- Treasury Securities
- Agency Mortgage-Backed Securities
- Reverse Repos
- Securities Lending
- Central Bank Liquidity Swaps
- System Open Market Account Holdings
- Primary Dealer Statistics
- Historical Transaction Data
- Monetary Policy Implementation
- Agency Commercial Mortgage-Backed Securities
- Agency Debt Securities
- Repos & Reverse Repos
- Discount Window
- Treasury Debt Auctions & Buybacks as Fiscal Agent
- INTERNATIONAL MARKET OPERATIONS
- Foreign Exchange
- Foreign Reserves Management
- Central Bank Swap Arrangements
- Statements & Operating Policies
- Survey of Primary Dealers
- Survey of Market Participants
- Annual Reports
- Primary Dealers
- Standing Repo Facility Counterparties
- Reverse Repo Counterparties
- Foreign Exchange Counterparties
- Foreign Reserves Management Counterparties
- Operational Readiness
- Central Bank & International Account Services
- Programs Archive
- Economic Research
- Consumer Expectations & Behavior
- Survey of Consumer Expectations
- Household Debt & Credit Report
- Home Price Changes
- Growth & Inflation
- Equitable Growth Indicators
- Multivariate Core Trend Inflation
- New York Fed DSGE Model
- New York Fed Staff Nowcast
- R-star: Natural Rate of Interest
- Labor Market
- Labor Market for Recent College Graduates
- Financial Stability
- Corporate Bond Market Distress Index
- Outlook-at-Risk
- Treasury Term Premia
- Yield Curve as a Leading Indicator
- Banking Research Data Sets
- Quarterly Trends for Consolidated U.S. Banking Organizations
- Empire State Manufacturing Survey
- Business Leaders Survey
- Supplemental Survey Report
- Regional Employment Trends
- Early Benchmarked Employment Data
- INTERNATIONAL ECONOMY
- Global Supply Chain Pressure Index
- Staff Economists
- Visiting Scholars
- Resident Scholars
- PUBLICATIONS
- Liberty Street Economics
- Staff Reports
- Economic Policy Review
- RESEARCH CENTERS
- Applied Macroeconomics & Econometrics Center (AMEC)
- Center for Microeconomic Data (CMD)
- Economic Indicators Calendar
- Financial Institution Supervision
- Regulations
- Reporting Forms
- Correspondence
- Bank Applications
- Community Reinvestment Act Exams
- Frauds and Scams
As part of our core mission, we supervise and regulate financial institutions in the Second District. Our primary objective is to maintain a safe and competitive U.S. and global banking system.
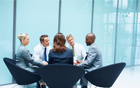
The Governance & Culture Reform hub is designed to foster discussion about corporate governance and the reform of culture and behavior in the financial services industry.
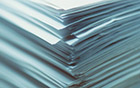
Need to file a report with the New York Fed? Here are all of the forms, instructions and other information related to regulatory and statistical reporting in one spot.
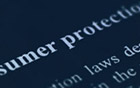
The New York Fed works to protect consumers as well as provides information and resources on how to avoid and report specific scams.
- Financial Services & Infrastructure
- Services For Financial Institutions
- Payment Services
- Payment System Oversight
- International Services, Seminars & Training
- Tri-Party Repo Infrastructure Reform
- Managing Foreign Exchange
- Money Market Funds
- Over-The-Counter Derivatives
The Federal Reserve Bank of New York works to promote sound and well-functioning financial systems and markets through its provision of industry and payment services, advancement of infrastructure reform in key markets and training and educational support to international institutions.
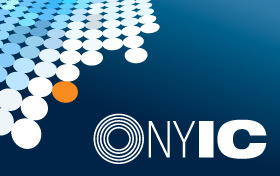
The New York Fed offers the Central Banking Seminar and several specialized courses for central bankers and financial supervisors.
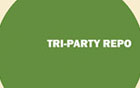
The New York Fed has been working with tri-party repo market participants to make changes to improve the resiliency of the market to financial stress.
- Community Development & Education
- Household Financial Well-being
- Fed Communities
- Fed Listens
- Fed Small Business
- Workforce Development
- Other Community Development Work
- High School Fed Challenge
- College Fed Challenge
- Teacher Professional Development
- Classroom Visits
- Museum & Learning Center Visits
- Educational Comic Books
- Economist Spotlight Series
- Lesson Plans and Resources
- Economic Education Calendar
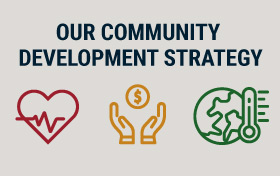
We are connecting emerging solutions with funding in three areas—health, household financial stability, and climate—to improve life for underserved communities. Learn more by reading our strategy.
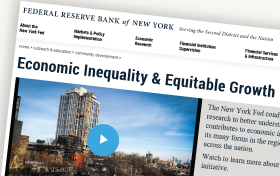
The Economic Inequality & Equitable Growth hub is a collection of research, analysis and convenings to help better understand economic inequality.
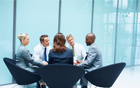
- Request a Speaker
- International Seminars & Training
- Governance & Culture Reform
- Data Visualization
- Economic Research Tracker
- Markets Data APIs
- Terms of Use
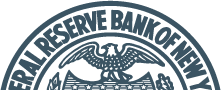

An official website of the United States government
Here’s how you know
Official websites use .gov A .gov website belongs to an official government organization in the United States.
Secure .gov websites use HTTPS A lock ( Lock A locked padlock ) or https:// means you’ve safely connected to the .gov website. Share sensitive information only on official, secure websites.
Fact Sheet: USDA, HHS Announce New Actions to Reduce Impact and Spread of H5N1
Contact: [email protected] | [email protected]
On March 25, 2024, immediately following the first detection of H5N1 in dairy cattle in the Texas panhandle region, USDA and HHS began their work to understand the origin of the emergence and its potential impact in bovines and humans. USDA experts also took swift action to trace animal movements, began sampling to assess the disease prevalence in herds, and initiated a variety of testing activities to confirm the safety of the meat and milk supplies alongside federal partners. On April 1, 2024, Texas reported the first and only confirmed human H5N1 infection associated with this outbreak, after confirmation by CDC. On April 24, 2024, USDA issued a Federal Order, that took effect on April 29, to limit the movement of lactating dairy cattle and to collect and aggregate H5N1 test results to better understand the nature of the outbreak.
Since the detection of H5N1 in dairy cattle, the Federal response has leveraged the latest available scientific data, field epidemiology, and risk assessments to mitigate risks to workers and the general public, to ensure the safety of America’s food supply and to mitigate risk to livestock, owners, and producers. Today, USDA is taking a series of additional steps to help achieve these goals and reduce the impact of H5N1 on affected premises and producers, and HHS is announcing new actions through the CDC and FDA to increase testing and laboratory screening and testing capacity, genomic sequencing, and other interventions to protect the health and safety of dairy and other potentially impacted food items.
Today, USDA is announcing assistance for producers with H5N1 affected premises to improve on-site biosecurity in order to reduce the spread. In addition, USDA is taking steps to make available financial tools for lost milk production in herds affected by H5N1. Building on the Federal Order addressing pre-movement testing, these steps will further equip producers with tools they can use to keep their affected herds and workers healthy and reduce risk of the virus spreading to additional herds.
Protect against the potential for spread between human and animals. Provide financial support (up to $2,000 per affected premises per month) for producers who supply PPE to employees and/or provide outerwear uniform laundering, for producers of affected herds who facilitate the participation of their workers in USDA/CDC workplace and farmworker study.
Complementary to USDA’s new financial support for producers, workers who participate in the study are also eligible for financial incentives to compensate them for their time, regardless of whether the study is led by federal, state, or local public health professionals.
Support producers in biosecurity planning and implementation . Provide support (up to $1,500 per affected premises) to develop biosecurity plans based on existing secure milk supply plans. This includes recommended enhanced biosecurity for individuals that frequently move between dairy farms – milk haulers, veterinarians, feed trucks, AI technicians, etc. In addition, USDA will provide a $100 payment to producers who purchase and use an in-line sampler for their milk system.
Provide funding for heat treatment to dispose of milk in a bio secure fashion. This will provide producers a safe option for disposal of milk. Heat treatment performed in accordance with standards set by FDA is the only currently available method considered to effectively inactivate the virus in milk. If a producer establishes a system to heat treat all waste milk before disposal, USDA will pay the producer up to $2,000 per affected premises per month.
Reimburse producers for veterinarian costs associated with confirmed positive H5N1 premises. This provides support to producers to cover veterinary costs necessarily incurred for treating cattle infected with H5N1, as well as fees for veterinarians to collect samples for testing. This can include veterinary fees and/or specific supplies needed for treatment and sample collection. Veterinary costs are eligible to be covered from the initial date of positive confirmation at NVSL for that farm, up to $10,000 per affected premises.
Offset shipping costs for influenza A testing at laboratories in the National Animal Health Laboratory Network (NAHLN). USDA will pay for the cost of shipping samples to NAHLN labs for testing. USDA will pay actual shipping costs, not to exceed $50 per shipment for up to 2 shipments per month for each affected premises. Testing at NAHLN laboratories for samples associated with this event (e.g., pre-movement, testing of sick/suspect animals, samples from concerned producers) is already being conducted at no-cost to the producer.
Taken together, these tools represent a value of up to $28,000 per premises to support increased biosecurity activities over the next 120 days.
Compensate producers for loss of milk production. USDA is taking steps to make funding available from the Emergency Assistance for Livestock, Honey Bees, and Farm-raised Fish Program (ELAP) to compensate eligible producers with positive herds who experience loss of milk production. While dairy cows that have been infected with H5N1 generally recover well, and there is little mortality associated with the disease, it does dramatically limit milk production, causing economic losses for producers with affected premises. USDA can support farmers with the ELAP program to offset some of these losses. This compensation program is distinct from the strategy to contain the spread.
Work with states to limit movement of lactating cattle . Additionally, USDA will work with and support the actions of States with affected herds as they consider movement restrictions within their borders to further limit the spread of H5N1 between herds to reduce further spread of this virus.
USDA will make $98 million in existing funds available to APHIS to fund these initiatives. If needed, USDA has the authority, with Congressional notification, to make additional funds available.
These additional measures build on a suite of actions USDA has taken to date. This includes implementation of the Federal Order to limit spread of the disease, coordinating with federal partners to share expertise and lab capacity, doubling down on our work with producers to practice good biosecurity measures, continuing to conduct investigations to determine how the virus is spread within and between farms, and analyzing and sharing sequences alongside validated epidemiological information.
The U.S. government is addressing this situation with urgency and through a whole-of-government approach. USDA is working closely with federal partners at FDA, which has the primary responsibility for the safety of milk and dairy products, by assisting with conducting lab testing at USDA labs. USDA is also working closely with federal partners at CDC, which has the primary responsibility for public health, by encouraging producer and industry cooperation with public health officials to get vital information necessary to assess the level of risk to human health.
Additional details on how producers can access and apply for the financial tools is forthcoming.
Today, HHS announced new funding investments through CDC and FDA totaling $101 million to mitigate the risk of H5N1 and continue its work to test, prevent, and treat H5N1. Although the CDC’s assessment of the risk of avian influenza infection for the general public continues to remain low at this time, these investments reflect the Department’s commitment to prioritizing the health and safety of the American public.
Public and animal health experts and agencies have been preparing for avian influenza outbreak for 20 years. Our primary responsibility at HHS is to protect public health and the safety of the food supply, which is why we continue to approach the outbreak with urgency. We stood up a response team which includes four HHS agencies – CDC, FDA, NIH and ASPR – which are working closely with USDA to:
- Ensure we keep communities healthy, safe, and informed;
- Ensure that our Nation’s food supply remains safe;
- Safeguard American agriculture and the livelihood and well-being of American farmers and farmworkers; and
- Monitor any and all trends to mitigate risk and prevent the spread of H5N1 among both people and animals.
Some examples of this work include:
- CDC monitoring of the virus to detect any changes that may increase risk to people, and updated avian flu guidance for workers to ensure people who work with dairy cows and those who work in slaughterhouses have the guides and information they need in both English and Spanish.
- CDC's ongoing discussions with multiple states about field investigations and incentives for workers who participate in these on-site studies. CDC has also asked health departments to distribute existing PPE stocks to farm workers, prioritizing those who work with infected cows. To help states comply with CDC recommendations, ASPR has PPE in the Strategic National Stockpile (SNS) available for states to request if needed.
- FDA’s close coordination with USDA to conduct H5N1 retail milk and dairy sample testing from across the country to ensure the safety of the commercial pasteurized milk supply. NIAID – a part of NIH - is also providing scientific support to this entire effort through six U. S. based Centers for Excellence for Influenza Research and Response, known as CEIRRs.
Today, in light of HHS’ ongoing commitment to ensure the safety of the American people and food supply, HHS announced additional resources to further these efforts through CDC and FDA:
CDC announced it has identified an additional $93 million to support its current response efforts for avian influenza. Building on bipartisan investments in public health, this funding will allow CDC to capitalize on the influenza foundation that has been laid over the last two decades, specifically where CDC has worked domestically and globally to prevent, detect, and respond to avian influenza.
These investments will allow CDC to bolster testing and laboratory capacity, surveillance, genomic sequencing, support jurisdictions and partner efforts to reach high risk populations and initiate a new wastewater surveillance pilot.
- Develop and optimize assays that can be used to sequence virus independent of virus identification.
- Assess circulating H5N1 viruses for any concerning viral changes, including increased transmissibility or severity in humans or decreasing efficacy of diagnostics or antivirals.
- Support the ability of STLT Public Health Labs throughout the country to surge their testing abilities, including support for the additional costs of shipping human avian influenza specimens, which are select agents.
- Through the International Reagent Resource (IRR), support manufacture, storage, and distribution of roughly one thousand additional influenza diagnostic test kits (equaling nearly around one million additional tests) for virologic surveillance. The IRR would also provide influenza reagents for research and development activities on a global scale. This is in addition to current influenza testing capacity at CDC and in STLT public health and DOD labs, which is approximately 490,000 H5-specific tests.
- Address the manufacturer issue detected with current avian flu test kits.
- Initiate avian flu testing in one commercial laboratory.
- Scale up existing efforts to monitor people who are exposed to infected birds and poultry to accommodate workers at likely many more poultry facilities, as well as potentially workers at other agricultural facilities and other people (e.g., hunters) who may be exposed to species that pose a threat.
- Scale up contact tracing efforts and data reporting to accommodate monitoring of contacts of additional sporadic cases.
- Support the collection and characterization of additional clinical specimens through established surveillance systems from regions with large numbers of exposed persons to enhance the ability to detect any unrecognized cases in the community if they occur.
- Expand respiratory virus surveillance to capture more samples from persons with acute respiratory illness in different care settings.
- Support continuation and possible expansion of existing respiratory surveillance platforms and vaccine effectiveness platforms.
- Provide bioinformatics and data analytics support for genomic sequencing at CDC that supports surveillance needs for enhanced monitoring.
- Expand sequencing capacity for HPAI in state-level National Influenza Reference Centers (NIRCs), Influenza Sequencing Center (ISC), and Pathogen Genomic Centers of Excellence.
- Analyze circulating H5N1 viruses to determine whether current Candidate Vaccine Viruses (CVVs) would be effective and develop new ones if necessary.
- Support partner efforts to reach high risk populations.
- Initiate wastewater pilot to evaluate the use case for HPAI in up to 10 livestock-adjacent sites in partnership with state and local public health agencies and utility partners.
- Implement a study to evaluate the use of Influenza A sequencing in wastewater samples for highly pathogenic avian influenza typing. Initiate laboratory evaluation for HA typing and examine animal-specific markers in community wastewater to assess wildlife and livestock contribution and inform interpretation of wastewater data for action.
Additionally, the FDA is announcing an additional $8 million is being made available to support its ongoing response activities to ensure the safety of the commercial milk supply. This funding will support the agency’s ability to validate pasteurization criteria, conduct surveillance at different points in the milk production system, bolster laboratory capacity and provide needed resources to train staff on biosecurity procedures. Additionally, these funds will help support H5N1 activities in partnership with state co-regulatory partners, who administer state programs as part of the federal/state milk safety system. It may also allow the FDA to partner with universities on critical research questions.
Additional Information:
To learn more about USDA’s response to H5N1 in dairy cattle, visit https://www.aphis.usda.gov/livestock-poultry-disease/avian/avian-influenza/hpai-detections/livestock .
To learn more about CDC’s response to H5N1, visit https://www.cdc.gov/flu/avianflu/mammals.htm .
To learn more about FDA’s response to H5N1, visit https://www.fda.gov/food/alerts-advisories-safety-information/updates-highly-pathogenic-avian-influenza-hpai
Sign Up for Email Updates
Receive the latest updates from the Secretary, Blogs, and News Releases
Subscribe to RSS
Receive latest updates

Related News Releases
Hhs secretary xavier becerra issues statement on the national institutes of health request for information, biden-harris administration reports significant progress toward protecting children from lead poisoning, readout of hhs secretary xavier becerra’s remarks at the sickle cell disease trailblazers event, related blog posts.
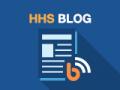
IDEES energy system database gets updated to improve analyses of policy assessments
A new version of a JRC-developed database simplifies data collection and data integration into modelling tools, allowing for an in-depth analysis of climate, energy and transport policy.
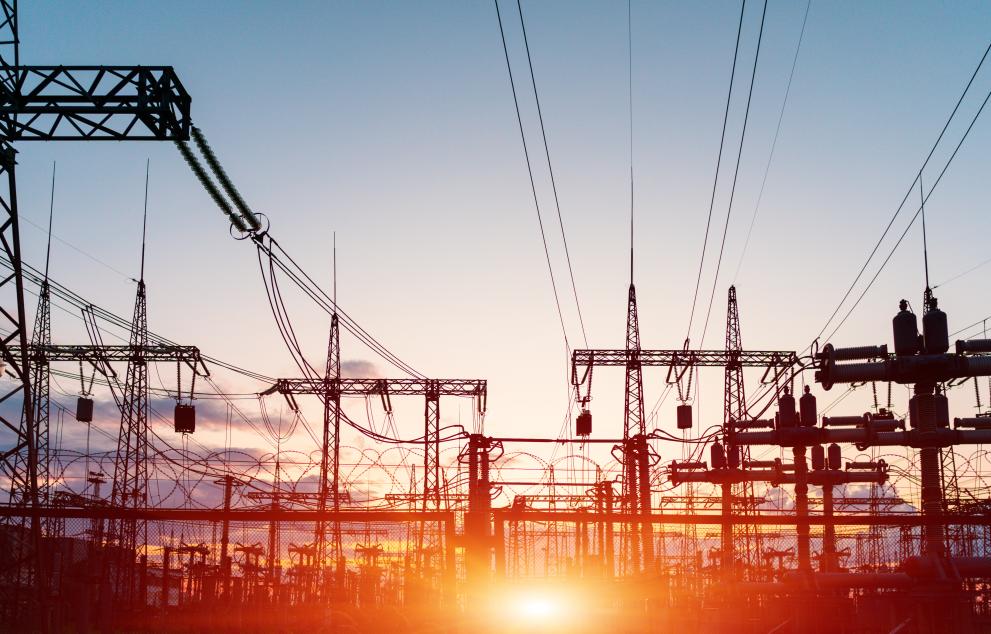
To better understand the current state of the energy system and support decision-making for climate, energy and transport policy, researchers and policy analysts require a wide range of data.
For instance, they need to know how much energy different transport technologies consume in different Member States, and determine the potential for improvement in the future, based on the advancements made in these technologies over the past few decades.
Facilitating this data collection and offering a solid starting point for researchers and analysts, the JRC has published an updated version of its open-access Integrated Database of the European Energy System (JRC-IDEES) , which consolidates a wealth of information, providing a granular breakdown of energy consumption and emissions.
This comprehensive approach, which was also employed to support the European Commission's recommendations on climate action by 2040 , offers valuable insights into the dynamics shaping the European energy landscape, facilitating the assessment of past policies, technological advancements, structural shifts, and macroeconomic factors.
Harmonised approach
First released in 2018, JRC-IDEES harmonises existing statistics with extensive technical assumptions to describe the recent history of all key sectors of the energy system: industry, the building sector, transport, and power generation.
For each Member State, it breaks the energy use and emissions of each of these sectors down to the level of specific processes or technologies. This level of detail enables a granular analysis of recent changes in the energy system, for instance to assess past policies, technology dynamics, structural changes, and macro-economic factors.
Since its initial release, JRC-IDEES has played an important role in EU research and policy analysis, serving as the primary data source for the JRC's Policy Oriented Tool for Energy & Climate Change Impact Assessment ( POTEnCIA model ).
New features
The latest update expands the time coverage of the database from 2000 to 2021 and incorporates new statistical sources as well as feedback from the user community. One key improvement is making the dataset easier to use within automated data workflows, so that researchers can better integrate JRC-IDEES into their analyses.
The data is freely accessible under the Creative Commons BY 4.0 license, ensuring that it can be used by a wide range of stakeholders.
A technical report summarises the statistics and assumptions used to compile the database
Related links
Integrated Database of the European Energy System (JRC-IDEES) (dataset)
JRC-IDEES-2021: the Integrated Database of the European Energy System – Data update and technical documentation
- Climate neutrality
More news on a similar topic
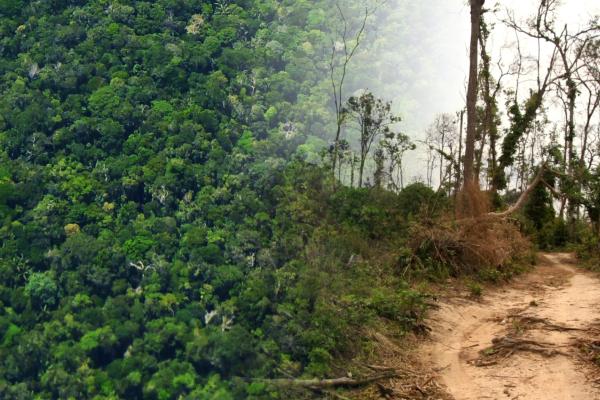
- General publications
- 28 February 2024
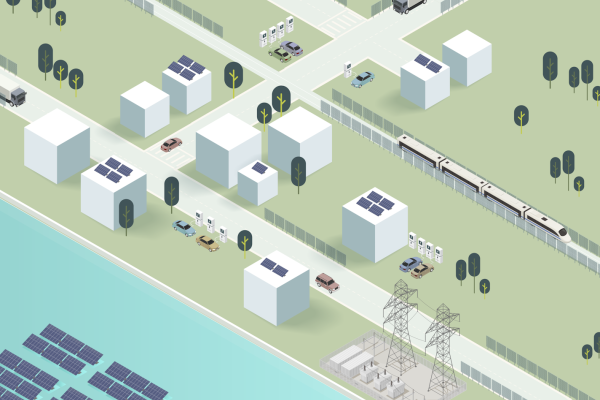
- News announcement
- 14 February 2024
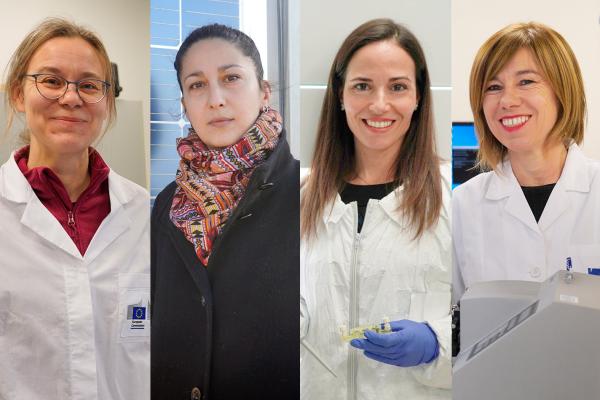
- 9 February 2024
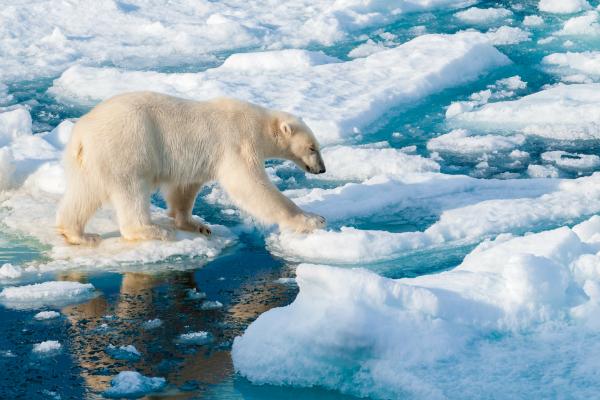
Share this page
An official website of the United States government
Here's how you know
Official websites use .gov A .gov website belongs to an official government organization in the United States.
Secure .gov websites use HTTPS A lock ( ) or https:// means you've safely connected to the .gov website. Share sensitive information only on official, secure websites.
CMS Newsroom
Search cms.gov.
- Physician Fee Schedule
- Local Coverage Determination
- Medically Unlikely Edits
Important Research Data Request & Access Policy Changes
Update (04/15/2024): CMS continues to receive helpful feedback in response to the updated Request for Information (RFI) (PDF) posted on March 1, 2024. Based on responses we have received to date, we have decided to delay implementation of policy changes, originally planned for August 19, 2024, to not begin before 2025. The additional time will allow CMS to carefully consider and be responsive to comments and concerns in developing the policy changes. CMS also will consider improvements to the research request process and Virtual Research Data Center (VRDC) user experience in conjunction with needed policy updates to strengthen data security. We want to remind and encourage all researchers to send your responses to the questions in the RFI to [email protected] by May 15, 2024.
We plan to share a revised implementation schedule for the policy changes in future guidance, as well as additional details on any adjustments to the policy changes based on researchers’ feedback.
The Centers for Medicare & Medicaid Services (CMS) is announcing two changes to the current research data request and access policies. To review the current CMS Research Identifiable File (RIF) policies please click here (PDF) .
Data Dissemination Policy Change for Researchers
BACKGROUND : CMS currently offers researchers two options for accessing CMS Research Identifiable File (RIF) data: (1) researchers can request that physical data extracts are shipped to their institution; and (2) researchers can access the data they need in the Chronic Conditions Warehouse Virtual Research Data Center (CCW VRDC), a secure CMS research environment. Due to growing data security concerns and an increase in data breaches across the healthcare ecosystem, CMS is making changes to the policies around how CMS data is accessed for research.
NEW POLICY : All researchers requesting RIFs must access data within CMS’s CCW VRDC environment and comply with CMS CCW VRDC policies (e.g., only aggregate/statistical information that complies with the CMS cell suppression policy , and is de-identified according to the HIPAA Privacy Rule, may be downloaded from the CCW VRDC). CMS is discontinuing the delivery of physical data extracts in support of external research projects. Only federal and state agencies may request an exception to this policy. CMS will communicate directly with eligible agencies on the process for requesting a policy exception.
IMPLEMENTATION : CMS will implement this policy change in two phases. We plan to share an implementation schedule for this policy change in future guidance, as well as additional details on any adjustments to this policy change based on researchers’ feedback. This policy change will not begin before 2025.
Proposed Phase 1 : Phase 1 policy changes include the following:
- CMS will no longer allow the physical dissemination of RIF data for new research studies . All new RIF Data Use Agreement (DUA) requests will be required to access RIF data within the CCW VRDC.
- Research studies with a RIF DUA approved prior to phase 1 implementation, may continue to receive physical data extracts, but researchers cannot request to expand the study to include new types of data. Researchers may only request additional years of physical data previously approved for the study.
- CMS will no longer offer physical shipment of preliminary Medicare Advantage Encounter or preliminary Transformed Medicaid Statistical Information System (T-MSIS) Analytic Files (TAF) data for any research DUAs. Researchers who wish to access these preliminary files must use the CCW VRDC.
Proposed Phase 2 : During phase 2, all RIF DUAs approved for physical RIF data extracts prior to the implementation of phase 1, must be closed or transitioned into the CCW VRDC.
Request for Information : CMS has posted an informal Request for Information (RFI) to aid CMS in planning for the implementation of these new policies. The RFI will help CMS better understand the potential issues researchers may encounter when transitioning their projects into the CCW VRDC or with the change in physical data pricing (see below). CMS will use insights gained from the RFI feedback to finalize the implementation plans related to these new policies.
Feedback on the RFI will be accepted until May 15, 2024 . Please click here (PDF) to open the RFI. Responses to the RFI must be sent via email to [email protected] .
To learn more about the VRDC, please review the About the VRDC and Requesting Access page on ccwdata.org.
Pricing Changes for Physical Delivery of Research Files :
BACKGROUND : CMS updates the fees for research data requests periodically to account for changes in the costs CMS incurs supporting researcher access to CMS data. In 2021, CMS updated CCW VRDC pricing with the transition to the CCW VRDC Cloud Environment. However, CMS did not update the fees for physical delivery of research files at that time. CMS now needs to update physical data pricing to account for changes to CMS’s costs and to align the physical data pricing approach with the CCW VRDC pricing approach.
NEW POLICY : CMS is implementing a new pricing structure for physical data requests, which will include an annual Project Fee in addition to the current File Dissemination Fees . Fees for access to CMS data are designed to account for the ongoing costs CMS incurs. Costs associated with maintaining the RIF DUA, conducting data privacy and security reviews, and providing Research Data Assistance Center (ResDAC) help desk support are built into a new Project Fee. Costs for building and shipping physical data files are used to calculate the File Dissemination Fees , which are set for each data file.
IMPLEMENTATION : We plan to share an implementation schedule for the pricing changes in future guidance, as well as additional details on any adjustments to the pricing changes based on researchers’ feedback. Pricing changes will not begin before 2025 and may include the following:
- Re searchers with previously approved DUAs that have not yet transitioned to the CCW VRDC will be required to pay a Project Fee annually upon their DUA renewal date.
- Researchers will continue to pay File Dissemination Fees when physical data files are being sent.
- For more information on the new pricing structure please click here .
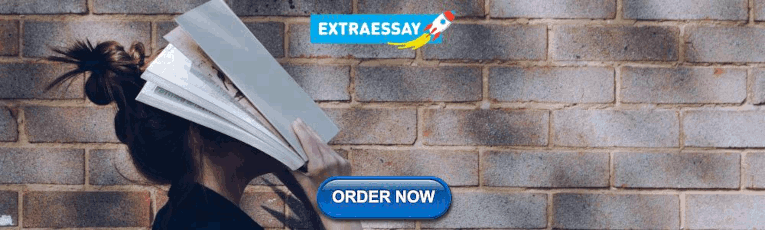
COMMENTS
At Our World in Data, we investigated the strengths and shortcomings of the available data on literacy. Based on this work, our team brought together the long-run data shown in the chart by combining several different sources, including the World Bank, the CIA Factbook, and a range of research publications. Explore and learn more about this data.
5. Data, analytics, and AI leaders are becoming less independent. This past year, we began to notice that increasing numbers of organizations were cutting back on the proliferation of technology and data "chiefs," including chief data and analytics officers (and sometimes chief AI officers).
Read the latest stories published by Towards Data Science. Your home for data science. A Medium publication sharing concepts, ideas and codes.
Neglecting sex and gender in research is a public-health risk The data are clear: taking sex and gender into account in research and using that knowledge to change health care could benefit ...
Research data comprises research observations or findings, such as facts, images, measurements, records and files in various formats, and can be stored in databases. Data publication and archiving ...
New findings provide update on researchers' attitudes towards open data. London, 13 October 2022 . ... Whether it's the broad support of researchers for making research data openly available as common practice or the changing attitudes to open data mandates, we must learn from and deliver concrete steps forward to address what the community ...
The Johns Hopkins Coronavirus Resource Center established a new standard for infectious disease tracking by publicly providing pandemic data in near real time. It began Jan. 22, 2020 as the COVID-19 Dashboard, operated by the Center for Systems Science and Engineering and the Applied Physics Laboratory. But the map of red dots quickly evolved ...
Learn how NIH is supporting research in COVID-19 testing, treatments, and vaccines. Skip ... Information about research and updates on Long COVID. EXPLORE LONG COVID RESOURCES ... Find COVID-19 datasets, data tools, and publications to use in research. ...
more top society/education stories. Breaking science news and articles on global warming, extrasolar planets, stem cells, bird flu, autism, nanotechnology, dinosaurs, evolution -- the latest ...
Respiratory Virus Data Channel Weekly Snapshot. Provides a summary of the key viral respiratory illness findings for COVID-19, influenza, and RSV from the past week and access to additional information and figures. ... Provides an update on how COVID-19, influenza, and RSV may be spreading nationally and in your state. Activity Level. Illness ...
Systematic reviews synthesise relevant research around a particular question. Preparing a systematic review is time and resource consuming, and provides a snapshot of knowledge at the time of incorporation of data from studies identified during the latest search. Newly identified studies can change the conclusion of a review.
Wharton Research Data Services - The Global Standard for Business Research. From the classroom to the boardroom, WRDS is more than just a data platform — data validation, flexible delivery options, simultaneous access to multiple data sources, and dedicated client support provided by doctoral-level professionals.
Between review updates, trends for sharing data and code increased strongly: in the base-review, data and code were available for 13 and 19% respectively, these numbers increased to 78 and 87% within the 23 new publications. ... Within the LSR update we identified research trends such as the emergence of relation-extraction methods, the current ...
One person dies every 33 seconds from cardiovascular disease. 1. About 695,000 people died from heart disease in 2021—that's 1 in every 5 deaths. 1 2. Heart disease costs about $239.9 billion each year from 2018 to 2019. 3 This includes the cost of health care services, medicines, and lost productivity due to death. View Larger.
November 1 deadline update Data Analytics and Research November 1, 2023. Common App state reports Data Analytics and Research. Common App research briefs. Exploring the complexities of detailed parental education First-generation status in context • Part 3 Data Analytics and Research
Learn about the data, systems, and research behind the programs that provide health coverage to more than 100 million people. Data & Research Topics CMS information technology Computer data & systems Files for order Monitoring programs Research Statistics, trends & reports Archives
These inclusion data allow us to report the breakdown of participants in NIH-funded clinical research, so that the public has a better understanding of who is enrolled in our supported studies. For instance, in fiscal year 2023: Women represented 57 percent of participants in all NIH-supported clinical research (55 percent for U.S. participants).
Straightforward to support data updates and multiple data versions. Possible to expose only certain portions of the underlying database and control user access. Flexible to changes in the underlying storage technology; changes to the backend, or several data providers with different backends entirely, can maintain the same API to the end user
Research and development. OHSU data scientist Peter Graven, Ph.D., has provided weekly updates of projections for hospitalizations statewide, which will become biweekly as the wave of infections generated by the omicron variant recedes.Beginning early in the pandemic, Graven modeled the projected unchecked spread of the virus and began sharing those projections with state and local ...
Q1 Single-family Home Prices Up in 92.3% of Metro Areas. May 10, 2024. In Q1 2024, national median home prices rose 5.0% year over year to $389,400, with 28.5% of metro areas seeing double-digit price increases.
The following are AAP Research articles published in AAP News in the last 5 years: Survey highlights pediatricians' international backgrounds March 2024. AAP grants provide research opportunities for residents February 2024. Racial, ethnic disparities remain among U.S. children living in poverty December 2023.
Research Update. This online quarterly spotlights recent published work from the Research and Statistics Group in a newsletter format. Research Update presents summaries of key studies, a complete list of new titles in the Group's research series and Liberty Street Economics blog, announcements of upcoming conferences, and feature articles ...
To keep up with the growing demand for computational power, hyperscale data centers are projected to increase their rack density at a compound annual growth rate (CAGR) of 7.8%. By 2027, average rack density is set to reach 50kW per rack, surpassing the current average of 36kW. Source: JLL Research, 2024. Meanwhile, the data center industry ...
Since the detection of H5N1 in dairy cattle, the Federal response has leveraged the latest available scientific data, field epidemiology, and risk assessments to mitigate risks to workers and the general public, to ensure the safety of America's food supply and to mitigate risk to livestock, owners, and producers.
The latest update expands the time coverage of the database from 2000 to 2021 and incorporates new statistical sources as well as feedback from the user community. One key improvement is making the dataset easier to use within automated data workflows, so that researchers can better integrate JRC-IDEES into their analyses.
BACKGROUND: CMS updates the fees for research data requests periodically to account for changes in the costs CMS incurs supporting researcher access to CMS data. In 2021, CMS updated CCW VRDC pricing with the transition to the CCW VRDC Cloud Environment. However, CMS did not update the fees for physical delivery of research files at that time.
Learn the fundamentals of using X data for academic research with tailored get-started guides. Or, take your current use of the API further with tutorials, code samples, and tools. Curated datasets. Free, no-code datasets are intended to make it easier for academics to study topics that are of frequent interest to the research community.
At the start of the March Core and Spam updates, Google claimed it intended to reduce unhelpful content in search by 40%. After the conclusion of the March Core Update on April 19 (which Google announced seven days after the fact), Google then clarified that it had actually reduced unhelpful content by 45%. One of the main drivers of this drop ...
Ocean water is pushing miles beneath Antarctica's "Doomsday Glacier," making it more vulnerable to melting than previously thought, according to new research which used radar data from space ...