Harvey Cushing/John Hay Whitney Medical Library
- Collections
- Research Help
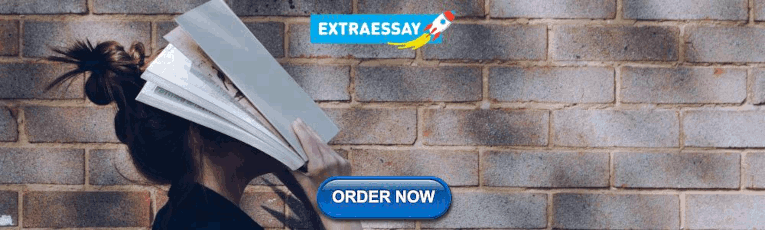
Free Full Text Biomedical Literature: Finding free full text online
- Finding free full text online
- What to do when you can't find free full text
- Before you leave Yale
Find free full text with browser extensions
- Get the Open Access Button This open source project links you to free, legal, full text articles. You can search from their website or through their API, but their browser extension is the most convenient. When you're on the page of a paywalled article, click the extension and it will look for an open access version of the article. And if it can't find one, it makes it easy to request a copy from the author.
- Get Unpaywall The browser extension Unpaywall, for Firefox and Chrome, adds a green tab beside research articles that you can read for free.
Free full text in Google and Google Scholar
Google and Google Scholar both include lots of material and use algorithms that are constantly being adjusted to highlight the "most relevant" results. Expect good recall, low precision, and little quality control.
When you search in Google Scholar, you'll see links labeled [PDF] to the right of search results. This connects you to full text from many resources: PubMed Central, ResearchGate, Academica.edu, or institutional repositories.
Do the same search in Google Scholar and Google, and you'll get different results. You can limit your Google results to PDFs by adding filetype:pdf to your search string; this can help you track down full text of articles, reports, and white papers.
- Google advanced search
- Google Scholar
Free full text in PubMed and PubMed Central
PubMed and PubMed Central are both free databases that you can search with high precision. To check which database you're using, look in the top left corner. Even though they look similar, there's an important difference! PubMed is a database of citations ; PubMed Central is a database of articles .
It's almost always better to look for articles about your topic in PubMed, which is much bigger and updated more quickly. We recommend searching PubMed Central in one particular situation: if you need to search for keywords in the full text of articles.
PubMed has a free full text filter on the results page. Look on the left, under text availability , and click on free full text . The results set for your search will get smaller, but all the remaining articles are easily available in free full text. Just click on the article title to get to the article page, then look for the full text link on the right.That full text link will bring you to the article's full text, maybe in PubMed Central or maybe somewhere else.
- PubMed Central
Other resources for free full text
- HINARI Are you affiliated with an educational or health care institution in a low-income country? Talk to your organization about registering for HINARI to get free access to literature from many scholarly publishers.
- Open Access Dissertations and Theses Use OATD to search almost four million theses and dissertations -- all open access. If you've been using Proquest Dissertations and Theses as a Yale affiliate, try this out.
- Open Science Framework Preprints "Preprints" are typically complete and public versions of articles before they have gone through peer review. Biomedical funders including the NIH and the Wellcome Trust have encouraged researchers to cite preprints as interim research products.
- Sci-Hub: an ethical conundrum You've probably heard about Sci-Hub, an online collection of article PDFs. Before you decide to use Sci-Hub, you should read about the ethical conflicts of their approach; you can start with the coverage in Science, linked above. Librarians don't endorse copyright infringement -- that's why we have recommended all these other pathways to free full text.
You found it, but should you use it? Critical appraisal
- Evidence-Based Medicine Worksheets Use these handy worksheets from Dana Biomedical Library at Dartmouth to appraise randomized control trials, systematic reviews and meta-analyses, practice guidelines, studies of diagnostic tests, prognosis studies, etiology studies, and qualitative studies. Don't outsource your critical thinking to journal editors and peer reviewers!
- Next: What to do when you can't find free full text >>
- Last Updated: May 17, 2021 10:42 AM
- URL: https://guides.library.yale.edu/freefulltext
Open Access Health Resources: Journals
- Clinical/Medicine
- Public Health
- Open Textbook Library
- FAQs about OAR
Links to free journals
- BioMedCentral Online publisher of free peer-reviewed scientific articles in all areas of medical research and biology.
- Directory of Open Access Journals Categorized, searchable links to free, full text, quality controlled scientific and scholarly journals.
- Free Medical Journals List of free medical journals arranged by subject area and searchable by keywords. List maintained by the Geneva Foundation for Medical Education and Research.
- Free Online Public Health Journals Organized and maintained by the University of Massachusetts Medical School.
- J-STAGE / Journal@rchive Japan Science and Technology Information Aggregator, Electronic is online repository of freely accessible journal articles.
- Public Library of Science Nonprofit organization of scientists and physicians committed to making the world's scientific and medical literature a freely available public resource. Publishes six open access journals.
- PubMed Central U.S. National Institutes of Health (NIH) free digital archive of biomedical and life sciences journal literature.
- Scientific Electronic Library Online - SciELO Electronic virtual library covering a collection of Spanish scientific journals selected following preestablished quality criteria.
Programs Providing Access to Developing Countries
- HINARI - World Health Organization program set up in conjunction with major publishers to enable developing countries to gain access to biomedical and health literature. - Provides access to more than 6,400 journal and textbook titles to health institutions in 108 countries, areas and territories.
- AGORA - Access to Global Online Research in Agriculture (AGORA) provides free or low cost access to major scientific journals in agriculture and related biological, environmental and social sciences to public institutions in developing countries. - Led by the Food and Agriculture Organization of the United Nations.
- OARE - Online Access to Research in the Environment (OARE) enables developing countries to gain access to one of the world's largest collections of environmental science research. - Coordinated by the United Nations Environment Programme (UNEP), Yale University, and science and technology publishers.
Subject Guide

- << Previous: Public Health
- Next: Open Textbook Library >>

- Last Updated: Nov 21, 2023 12:14 PM
- URL: https://guides.himmelfarb.gwu.edu/free

- Himmelfarb Intranet
- Privacy Notice
- Terms of Use
- GW is committed to digital accessibility. If you experience a barrier that affects your ability to access content on this page, let us know via the Accessibility Feedback Form .
- Himmelfarb Health Sciences Library
- 2300 Eye St., NW, Washington, DC 20037
- Phone: (202) 994-2850
- [email protected]
- https://himmelfarb.gwu.edu

Library Tutorials
- Finding Full Text Journal Articles
- Register for a MyAccess Account
- Annotation Tool
- Register for a Personal Account
- Activate Self-Managed Remote Access
- App Instructions - Computer, Tablet, or Mobile Device
- Register for an Account
- Tips & Tricks
- How do I...
- Search Strategies
- Full Text Browser Extensions
Full Text Browser extensions are great tools to help you find full text journal articles! Click on the link below to learn more about two options supported by the Medical Library, LibKey Nomad and EndNote Click.
PDF vs. HTML Full Text
Pdf vs. html full text access .

In many cases, you will have the option of how you access the full text of an article found in a library database. Links to both HTML and PDF full text often appear under the article metadata.
What are the differences and does it matter what I use?
A PDF article typically displays the same way it would in a print journal, including pagination, graphics, photographs, illustrations, charts and graphs, etc. It also prints well.
An article appearing in HTML format also will duplicate the same content as the original journal article, but it displays as a normal web page. There may be issues with pagination, as well as with viewing graphics, photographs, illustrations, charts and graphs, etc. Text may display in an odd manner. It may not print as well as a PDF, but may load faster if accessing on a mobile device.
Ideally, choose PDFs if you have the option for the best experience in reading a journal article.
How to find full text journal articles.
There are a variety of ways to find full text journal articles through the Medical Library. This page provides information about two options to find full text:
- Finding full text journal articles through OneSearch
- Finding full text journal articles using the link resolver (Find Full Text @ BU)
You can learn more about these methods by scrolling down, or by watching the video below (5 minutes 22 seconds).
Additionally, this page provides information about related issues:
- Full text browser extensions
- PDF vs. HTML full text
- Using interlibrary loan
Finding Full Text Journal Articles Through the Library
Finding Full Text Through OneSearch
Direct access to full text journal articles in onesearch.
If you are looking for a specific article that you already have the citation for, search OneSearch for the article. You can type in the title and typically find it that way. In some cases you may need to use the filters and/or advanced search options to find the article by entering other information, such as publication year or author information.
When searching for an article in OneSearch , if the library has electronic access to the article, you can often access it from the results page. Simply click on "Read Article" or "Download PDF" (in blue) to access.

Alternatively, if you click on an article title, or the green "Full text available," you will be provided with link(s) to all resources that provide access to your desired article, including various databases and publisher sites. In some cases, instead of "Full text available" you may see a green "Online access" link.

Full Text via the Link Resolver
If you do not have a specific article that you are looking for via citation, you will want to search by keywords in OneSearch or a library database. Within databases, you will sometimes find direct links to the article as PDFs or in HTML format. But other times, you may see a blue box with the text: "Find Full Text @ BU."
What does the blue "Find Full Text @ BU" image mean?

Clicking on that image will either:
- Connect you directly to the article's full text if possible.
- Connect you to the article's result page in OneSearch, where you can access the article, or if not available, connect to interlibrary loan, where you can request the article for free.
Interlibrary Loan
No full-text access for an article you need? Don't pay for it, but use interlibrary loan (ILL)!
ILL is a free service providing full-text articles and book loans for resources that are not available from Belmont. Click on the link below for more information about this service.
- Interlibrary Loan Information for Belmont University faculty, staff, and students about interlibrary loan
- << Previous: Home
- Next: AccessMedicine >>
- Last Updated: Apr 18, 2024 2:22 PM
- URL: https://medlib.belmont.edu/tutorials

An official website of the United States government
The .gov means it’s official. Federal government websites often end in .gov or .mil. Before sharing sensitive information, make sure you’re on a federal government site.
The site is secure. The https:// ensures that you are connecting to the official website and that any information you provide is encrypted and transmitted securely.
- Publications
- Account settings
PubMed User Guide
Last update: April 16, 2024
Follow PubMed New and Noteworthy for brief announcements highlighting recent enhancements and changes to PubMed.
- How can I get the full text article ? What if the link to the full text is not working ?
- How do I search by author ?
- How do I search by journal name ?
- How do I find a specific citation ? I have some information such as the author, journal name, and publication year.
- I retrieved too many citations. How can I focus my search ?
- I retrieved too few citations. How can I expand my search ?
- How do I find consumer health information about a disease or condition?
- How do I find systematic reviews ?
- Are there tools to help with clinical searches or finding medical genetics information?
- I’m not finding what I need. How does a PubMed search work ?
- Can you explain what is shown on the search results ?
- How do I display an abstract ?
- How can I save my results ?
- Can I receive email updates when new results are available for my search ?
- How do I report an error or duplicate citation in PubMed?
- How can I cite an article or export citations to my citation management software program ?
- How do I get a link to bookmark or share my PubMed search ?
- How can I download PubMed?
- Is there a guide to NLM resources for MEDLINE/PubMed ?
- Where can I find further assistance and training ?
Search PubMed
How do i search pubmed, i retrieved too many citations. how can i focus my search, i retrieved too few citations. how can i expand my search, find a specific citation, searching by author, searching by journal, searching by date, searching for a phrase, truncating search terms, combining search terms with boolean operators (and, or, not), using search field tags, proximity searching.
- Identify the key concepts for your search.
- Enter the terms (or key concepts) in the search box.
- Press the Enter key or click Search.
For many searches, it is not necessary to use special tags or syntax. PubMed uses multiple tools to help you find relevant results:
- Best Match sort order uses a state-of-the-art machine learning algorithm to place the most relevant citations at the top of your results.
- An autocomplete feature displays suggestions as you type your search terms. This feature is based on PubMed query log analysis described in " Finding Query Suggestions for PubMed ."
- A spell checking feature suggests alternative spellings for search terms that may include misspellings.
- A citation sensor displays suggested results for searches that include terms characteristic of citation searching, e.g., author names, journal titles, publication dates, and article titles.
To limit the number of search results:
- Replace general search terms with more specific ones (e.g., low back pain instead of back pain).
- Include additional terms in your query.
- Use the sidebar filters to restrict results by publication date, full text availability, article type, and more.
- On the abstract page for a citation, see the Similar Articles section for a pre-calculated set of additional PubMed citations closely related to that article.
- Remove extraneous or specific terms from the search box.
- Try using alternative terms to describe the concepts you are searching.
Paste the article title into the search box, or enter citation details such as the author, journal name and the year the article was published in the search box and the PubMed citation sensor will automatically analyze your query for citation information to return the correct citation. The citation sensor incorporates a fuzzy matching algorithm and will retrieve the best match even if a search includes an incorrect term. You do not need to use field tags or Boolean operators.
Enter the author’s last name and initials without punctuation in the search box, and click Search.
If you only know the author’s last name, use the author search field tag [au], e.g., brody[au].
Names entered using either the lastname+initials format (e.g., smith ja) or the full name format (john a smith) and no search tag are searched as authors as well as collaborators, if they exist in PubMed.
- Enter a full author name in natural or inverted order, e.g., julia s wong or wong julia s.
- Prior to 2002, full author names were not included on PubMed citations, so full author name searches will only retrieve citations from 2002 forward, when the full author name was published in the article.
- A comma following the last name for searching is optional. For some names, however, it is necessary to distinguish which name is the last name by using the comma following the last name, e.g., james, ryan.
- Omit periods after initials and put all suffixes at the end, e.g., vollmer charles jr
Initials and suffixes are not required. If you include a middle initial or suffix, you will only retrieve citations for articles that were published using the middle initial or suffix.
More information about author searching:
- To search by author using the search builder, click Advanced search and then select Author from the All Fields menu. The author search box includes an autocomplete feature.
- You may click an author link on the abstract display to execute a search for the author in PubMed. Results will display using a ranking algorithm if the author name is computationally similar for additional PubMed citations.
- If an author name includes only stopwords , use the author search field tag [au] to search in combination with other terms, e.g., just by[au] seizure.
- Author names are automatically truncated to account for varying initials and designations such as Jr. To turn off the truncation, use double quotes around the author's name with the author search field tag [au], e.g., "smith j"[au].
- Use the search field tag [1au] to search for the first personal author or [lastau] to search for the last personal author name in a citation.
For additional information on author names in PubMed, please see the journal article, " Author Name Disambiguation for PubMed ."
Enter one of the following in the search box:
- full journal title (e.g., molecular biology of the cell)
- title abbreviation (e.g., mol biol cell)
- ISSN number, a standardized international code (e.g., 1059-1524)
More information about journal searching:
- To search by journal using the search builder, click Advanced search and then select Journal from the All Fields menu. The journal search box includes an autocomplete feature.
- Click Journals in NCBI Databases on the PubMed homepage.
- Enter the journal name and click Search.
- Use the journal search field tag [ta] to limit your search to the journal only, e.g., gene therapy[ta], scanning[ta]
- Searching with the full journal title or abbreviation is recommended for complete retrieval of indexed items; older citations may not have an ISSN.
- If a journal title or abbreviation includes a special character (e.g., parentheses, brackets, &), enter the title or abbreviation without the special characters. For example, to search by the journal abbreviation j hand surg [am], enter j hand surg am.
- Searching for a journal will automatically map to the official journal title and the title associated with an alternative title, if one exists. To turn off this automatic mapping enter the journal in double quotes and tag with [ta], e.g., "science"[ta].
A list of journals included in PubMed is available by FTP.
Using the results timeline
Using the search builder, searching by a single date in the search box, searching for a date range in the search box, searching for a relative date range.
Click and drag the sliders on the Results By Year timeline to change the date range for your search.
Note: The Results By Year timeline counts all publication dates for a citation as supplied by the publisher, e.g., print and electronic publication dates. These dates may span more than one year; for example, an article that was published online in November 2018 and published in a print issue in January 2019. This means the sum of results represented in the timeline may differ from the search results count.
- Click Advanced search and use the search builder.
- Select a date field from the All Fields menu, e.g., Date – Publication, and enter a single date or a date range in the fill-in-the-blank boxes. Month and day are optional. If you want to search for a date range up to the current date, do not edit the ‘Present’ date box.
- Add the date from the builder to the query box.
- Once you have finished adding terms to the query box, click Search (or Add to History) to run the search.
Enter dates using the format yyyy/mm/dd [date field]. The month and day are optional.
Use a Boolean operator when combining a date with other search terms.
Use the Boolean operator AND to limit your search to a specific publication date.
The available date fields are:
- Date of Publication [dp] - Date searching includes both print and electronic dates of publication. Searching for a single date does not include items when the electronic date of publication is after the print date.
- Electronic Date of Publication (if applicable) [epdat]
- Print Date of Publication (if applicable) [ppdat]
- Entry Date [edat] - Date used for PubMed processing, such as “Most Recent” sort order.
- MeSH Date [mhda] - The date the citation was indexed with MeSH terms.
- Create Date [crdt] - The date the PubMed record was first created.
Enter date ranges using a colon (:) between each date followed by a [date field].
Use a Boolean operator when combining a date range with other search terms.
Use the Boolean operator AND to limit your search to a date range.
Comprehensive searches for a full year should be entered as 2000:2000[dp] rather than 2000[dp] to retrieve citations with a different print and electronic year of publication.
Date range searching includes both print and electronic dates of publication.
Use the following format to search for a relative date range:
- term="last X days" [date field]
- term="last X months" [date field]
- term="last X years" [date field]
where X is the number of days, months or years immediately preceding today’s date and [date field] is the date field tag: [dp], [edat] or [crdt].
The relative date range search for publication dates will also include citations with publication dates after today's date; therefore, citations with publication dates in the future will be included in the results.
You can use filters to narrow your search results by article type , text availability , publication date , species , article language , sex , age , and other .
To apply a filter:
- Run a search in PubMed.
- Click the filter you would like to activate from the sidebar. A check mark will appear next to the activated filter(s).
- Subsequent searches will be filtered until the selected filters are turned off, or until your browser data is cleared.
The most popular filters are included on the sidebar by default. To display additional filters on the sidebar:
- Click the "Additional filters" button.
- A pop-up menu will appear showing the available filters for each category: article type, species, article language, sex, age, and other.
- Choose a category from the list of options on the left side of the menu: Article Type, Species, etc.
- Within each category, select the filters you would like to add to the sidebar.
- Click Show. This will close the pop-up menu and display your selections on the sidebar with the other filters.
- If you would like to cancel your selections, click Cancel or click on the X in the upper right corner to close the pop-up and return to your search results.
- To apply the filter(s) to your search, click the filter(s) on the sidebar.
More information about filters:
- When filters are selected a "Filters applied" message will display on the results page.
- Click an applied filter to turn it off.
- To turn off all applied filters, click the "Clear all" link or the "Reset all filters" button.
- Citations may be excluded for some filter selections because they have not yet completed the MEDLINE indexing process.
- You can activate additional filters with My NCBI filters .
- See Filter search strategies for the equivalent PubMed query for each filter.
Article type
Select article types to narrow your results based on the type of material the article represents, such as: Clinical Trial or Review.
You can add more article types to the sidebar using the Additional Filters button. The complete list of publication types found in PubMed is available.
These filters may exclude some citations that have not yet completed the MEDLINE indexing process because they rely on the Publication Type [pt] data for the citation; publication type data may be supplied by the publisher or assigned during the MEDLINE indexing process. However, the Systematic Review article type filter uses a search strategy to capture non-MEDLINE citations and citations that have not yet completed MEDLINE indexing in addition to citations assigned the systematic review publication type.
Systematic reviews
To search for systematic reviews in PubMed, use the Systematic Review article type filter on the sidebar, or enter your search terms followed by AND systematic[sb] in the search box. For example, lyme disease AND systematic[sb].
The Systematic Review filter uses a search strategy in addition to the Systematic Review publication type [pt] to find systematic reviews in PubMed. To limit your search to only those citations with the Systematic Review publication type, use the publication type search tag[pt], i.e., systematic review[pt]; however, this may exclude some relevant citations that have not yet completed the MEDLINE indexing process.
Text availability
To filter your results to only citations that include a link to full text, a link to free full text, or an abstract, click the appropriate selections.
Alternatively, you may search for citations with links to full text, free full text or include an abstract using the values: full text[sb], free full text[sb], or 'hasabstract'. No search field tag is required for hasabstract. You may also search for all MEDLINE citations with a structured abstract with ‘hasstructuredabstract’.
Note: Most citations in PubMed to articles published before 1975 do not include abstracts.
Publication date
To filter your results by Publication Date, click 1 year, 5 years, 10 years, or enter a custom range. These filters include both electronic and print publication dates.
Species selections restrict your results to human or animal studies.
You can add species filters to the sidebar using the Additional Filters button.
These filters may exclude some citations because they have not yet completed the MEDLINE indexing process.
Article language
Language filters restrict your search to articles published in the selected language(s). You can add language filters to the sidebar using the Additional Filters button.
By default, PubMed displays English language titles and abstracts when provided by the publisher. Check the Abstract display for links to view the abstract in other languages (when available).
Sex restricts your search results to a specific sex for an animal or human study.
You can add sex filters to the sidebar using the Additional Filters button.
This filter may exclude some citations because they have not yet completed the MEDLINE indexing process.
Age filters restrict results to a specific age group for a human study.
You can add age filters to the sidebar using the Additional Filters button.
Age filters include:
- Child: birth-18 years
- Newborn: birth-1 month
- Infant: birth-23 months
- Infant: 1-23 months
- Preschool Child: 2-5 years
- Child: 6-12 years
- Adolescent: 13-18 years
- Adult: 19+ years
- Young Adult: 19-24 years
- Adult: 19-44 years
- Middle Aged + Aged: 45+ years
- Middle Aged: 45-64 years
- Aged: 65+ years
- 80 and over: 80+ years
Other filters & more subsets
Exclude preprints.
The Exclude preprints filter can be added to the sidebar using the Additional Filters button. Alternatively, you can exclude preprints from your search results by including NOT preprint[pt] at the end of your query.
See Preprints for more information about preprint citations in PubMed.
MEDLINE Subset
The MEDLINE filter can be added to the sidebar using the Additional Filters button. To use this filter in a query, add medline[sb] to your search. The MEDLINE filter limits results to citations that are indexed for MEDLINE .
PubMed Central Subset
To restrict retrieval to citations that have a free full text article available in PubMed Central (PMC), search "pubmed pmc"[sb].
Use the PMID/PMCID/NIHMSID Converter to convert IDs for publications referenced in PubMed and PMC. To retrieve citations that include an NIHMS ID use the query, hasnihmsid.
Citation Status Subsets
The citation status indicates the internal processing stage of an article in the PubMed database (see PubMed Citation Status Subsets ).
To search for a particular citation status, enter one of the search terms below followed by the [sb] search tag:
- pubmednotmedline
To search for the total number of PubMed citations, enter all[sb] in the search box.
Ahead of Print Citations
Publishers may submit citations for articles that appear on the web prior to their publication in final or print format. To search for these ahead-of-print citations, enter pubstatusaheadofprint.
Many phrases are recognized by the subject translation table used in PubMed's Automatic Term Mapping (ATM) . For example, if you enter fever of unknown origin, PubMed recognizes this phrase as a MeSH Term.
You can bypass ATM and search for a specific phrase using the following formats:
- If you use quotes and the phrase is not found in the phrase index , the quotes are ignored and the terms are processed using automatic term mapping. The message "Quoted phrase not found in phrase index" will display at the top of your search results.
- If you use a search tag and the phrase is not found in the phrase index , the phrase will be broken into separate terms. For example, "psittacine flight" is not in the phrase index, so a search for psittacine flight[tw] is broken up and translated as: ((("psittaciformes"[MeSH Terms] OR "psittaciformes"[All Fields]) OR "psittacine"[All Fields]) OR "psittacines"[All Fields]) AND "flight"[Text Word]
- If you use a hyphen and the phrase is not found in the phrase index , the search will not return any results for that phrase.
- Hyphenated phrases matching a MeSH term or entry term will include those terms in the search translation. If you want to prevent such mapping, put the hyphenated phrase inside double quotes: "heart-attack"
When you enter search terms as a phrase, PubMed will not perform automatic term mapping that includes the MeSH term and any specific terms indented under that term in the MeSH hierarchy. For example, "health planning" will include citations that are indexed to the MeSH term, Health Planning, but will not include the more specific terms, e.g., Health Care Rationing, Health Care Reform, Health Plan Implementation, that are included in the automatic MeSH mapping.
Phrase index
PubMed uses a phrase index to provide phrase searching. To browse the phrase index, use the Show Index feature included in the Advanced Search builder: select a search field, enter the beginning of a phrase, and then click Show Index.
Quoted phrase not found
Phrases may appear in a PubMed record but not be in the phrase index. To search for a phrase that is not found in the phrase index, use a proximity search with a distance of 0 (e.g., "cognitive impairment in multiple sclerosis"[tiab:~0] ); this will search for the quoted terms appearing next to each other, in any order.
Automated processes regularly add new phrases to the index based on standard criteria such as phrase frequency and length. If you would like to request a phrase be added to the phrase index, please write to the NLM Help Desk .
To search for all terms that begin with a word, enter the word followed by an asterisk (*): the wildcard character.
To search for a phrase including a truncated term, use the following formats:
- Enclose the phrase in double quotes: "breast feed*"
- Use a search tag: breast feed*[tiab]
- Use a hyphen: breast-feed*
At least four characters must be provided in the truncated term.
The truncated term must be the last word in the phrase.
Truncation turns off automatic term mapping and the process that includes the MeSH term and any specific terms indented under that term in the MeSH hierarchy. For example, heart attack* will not map to the MeSH term Myocardial Infarction or include any of the more specific terms, e.g., Myocardial Stunning; Shock, Cardiogenic.
PubMed applies an AND operator between concepts, e.g., "vitamin c common cold" is translated as vitamin c AND common cold. Enter Boolean operators in uppercase characters to combine or exclude search terms:
- AND retrieves results that include all the search terms.
- OR retrieves results that include at least one of the search terms.
- NOT excludes the retrieval of terms from your search.
PubMed processes searches in a left-to-right sequence. Use parentheses to "nest" concepts that should be processed as a unit and then incorporated into the overall search.
- PubMed uses automatic term mapping to identify concepts. For example, for the search air bladder fistula, PubMed will search "air bladder" as a phrase. If you do not want this automatic phrase parsing, enter each term separated by the Boolean operator AND, e.g., air AND bladder AND fistula.
- Search Details show how a search was translated.
You can search for a term in a specific field by including a search field tag after the term; for example, UCLA[ad] will search for the term “UCLA” in the affiliation field only.
More information about using search field tags:
- The search field tag must be enclosed in square brackets.
- Case and spacing do not matter: crabs [mh] = Crabs[mh].
- Search field tags turn off Automatic Term Mapping (ATM) , limiting your search to the specified term only.
- Using a search field tag after multiple terms will attempt to search those terms as a phrase : kidney allograft[tiab].
- To search multiple terms in the same field, each term must be tagged individually: covid-19[ti] vaccine[ti] children[ti].
- The Advanced Search builder can help you search for terms in specific fields and build large, complex search strings.
Search field tags
Affiliation [ad], all fields [all], article identifier [aid], author [au], author identifier [auid], book [book], comment correction type, completion date [dcom], conflict of interest statement [cois], corporate author [cn], create date [crdt], ec/rn number [rn], editor [ed], entry date [edat], filter [filter] [sb], first author name [1au], full author name [fau], full investigator name [fir], grants and funding [gr], investigator [ir], isbn [isbn], journal [ta], language [la], last author name [lastau], location id [lid], mesh date [mhda], mesh major topic [majr], mesh subheadings [sh], mesh terms [mh], modification date [lr], nlm unique id [jid], other term [ot], pagination [pg], personal name as subject [ps], pharmacological action [pa], place of publication [pl], pmcid and mid, pmid [pmid], publication date [dp], publication type [pt], publisher [pubn], secondary source id [si], subset [sb], supplementary concept [nm], text words [tw], title/abstract [tiab], transliterated title [tt], volume [vi].
You can use proximity searching to search for multiple terms appearing in any order within a specified distance of one another in the [Title], [Title/Abstract], or [Affiliation] fields.
To create a proximity search in PubMed, enter your terms using the following format:
"search terms"[field:~N]
- There is no limit to the number of words you can search together in proximity; however, the more terms you enter, the more restrictive your search becomes. Using the Boolean operator AND to combine terms may be more appropriate than combining many terms into one proximity search.
- Proximity searching is only available in the Title , Title/Abstract , and Affiliation search fields.
- You can use the full search field tags [Title], [Title/Abstract], and [Affiliation], or the abbreviated versions [ti], [tiab], and [ad].
- What N value to use will depend on your search. Try changing the N value and comparing the results to find what works best for your search.
- A higher N creates a broader, more comprehensive search; this will typically retrieve more results overall, but some of these results may be less relevant. Using the Boolean operator AND to combine terms may be more appropriate than proximity searching with a large N value.
- A lower N creates a narrower, more precise search; this will typically retrieve fewer results that are highly relevant, but may exclude other relevant results.
- If N=0, the quoted terms will appear next to each other--with no other words in between.
- For the affiliation field only, an N value of 1,000 or less will search for the double quoted terms together within the same affiliation, rather than spread across all affiliations on the record. See Affiliation [ad] for an example proximity search in the affiliation field and more information about searching for affiliations.
More information about proximity searching:
- Results will include your quoted terms in any order. If you would like to search for an exact phrase with terms appearing in a specific order, use a phrase search instead.
- Automatic Term Mapping is not applied to the quoted terms.
- Proximity searching is not compatible with truncation (*). If the double quoted terms in a proximity search include a wildcard (*), the proximity operator will be ignored.
- You can combine proximity searches with other terms using Boolean operators; for example, "hip pain"[Title:~4] AND stretching
- Booleans and stopwords included in quoted terms for proximity search are searched like regular keywords.
Search PubMed for citations with the terms "rationing" and "healthcare" appearing within 2 words of each other--in any order--in the Title field:
Search results may include: rationing healthcare, healthcare rationing, rationing of healthcare, rationing in healthcare, rationing universal healthcare, rationing strategies in healthcare, rationing limited healthcare… and more.
Search PubMed for citations with the terms "patient," "physician," and "relationship" appearing next to each other—in any order—in the Title/Abstract fields:
Since N=0, the quoted terms must appear next to each other with no other words in between them, although they can still appear in any order.
You can build queries that combine proximity searches with other terms using Boolean operators (AND, OR, NOT):
Display, Sort, and Navigate
Understanding your search results, display an abstract, changing the display format of search results.
- Showing more results
Sorting your results
Finding the full text article, similar articles, grants and funding, navigating searches with more than 10,000 results, discovering related data in ncbi databases, find related resources using linkout, reporting broken or problem links.
Citations are initially displayed 10 items per page and sorted by Best Match.
By default, PubMed search results are displayed in a summary format and include snippets from the citation abstract. Snippets and highlighted terms are selected based on relatedness to your query.
To see the abstract for an individual citation, click the title of the citation to go to its abstract page.
Journal names are shown using the journal title abbreviation. When viewing citations in Abstract format, you can mouseover a journal’s title abbreviation to display the full journal name.
Click the title of the citation to go to its abstract page, or change the search results display to Abstract format using the Display options button in the upper right corner of the search results page.
PubMed may include non-English abstracts if supplied by the publisher. The abstract text defaults to English when a citation has an accompanying non-English abstract. Links to display the additional language(s) are available on the Abstract display. To retrieve citations with non-English abstracts, use the query hasnonenglishabstract.
Results are displayed in the summary format by default, except a single citation result will go directly to the abstract page. You can change the results format using the Display options button:
- Click the Display options button in the upper right corner of the search results page
- Select the display format you would like to use
- Results will be displayed in the new format
Selecting one or more items and changing the display format will display only the selected result(s) in the new format.
By default, the summary format includes snippets from the citation abstract. You can turn off snippets under Display options by deselecting Abstract snippets.
The results page indicates the total number of items retrieved.
Ten items are displayed per page by default. You can change the number of items displayed per page using the Display options button:
- Select the number of items to display per page: 10, 20, 50, 100, or 200
- Your selection will be active for subsequent searches until your browser cookies are cleared.
Click "Show more" to display the next page of results, or click "Jump to page" to navigate directly to a specific page of results.
The default sort order in PubMed is Best Match. You can use the "Sort by" drop-down menu at the top of the search results page to change the sort order.
If you change the sort order, your new selection will be active for subsequent searches until your browser cookies are cleared.
Sort orders
You can sort your search results by:
- Best Match: The Best Match sort order is based on an algorithm that analyzes each PubMed citation found with your search terms. For each search query, "weight" is calculated for citations depending on how many search terms are found and in which fields they are found. In addition, recently-published articles are given a somewhat higher weight for sorting. The top articles returned by the weighted term frequency algorithm above are then re-ranked for better relevance by a new machine-learning algorithm. Please see the Algorithm for finding best matching citations in PubMed for more information.
- Most Recent: Citations sorted by Most Recent are displayed in reverse date added order: last in, first out. The Most Recent date is the date a record was initially added to PubMed, not the publication date. The secondary sort is PMID.
- Publication Date: Citations sorted by Publication Date are displayed in reverse chronological order: newest to oldest. Citations with more than one publication date, such as electronic and print, are sorted by their earliest publication date. Publication dates without a month are set to January, multiple months (e.g., Oct-Dec) are set to the first month, and dates without a day are set to the first day of the month. Dates with a season are set as: winter = January, spring = April, summer = July and fall = October.
- First Author: Citations are sorted alphabetically by first author name. The secondary sort order within a group with the same first author is PMID.
- Journal: Citations are sorted alphabetically by journal name. The secondary sort order within a group with the same journal name is PMID.
Reverse sort order
- When sorting by Most Recent, Publication Date, First Author, or Journal, you can reverse the sort order by clicking the up/down arrow next to the selected sort option to toggle between ascending or descending order.
- The reverse sort option will not display when Best Match sort order is selected.
Computed author sort
Clicking an author name link on the abstract display runs a search for the author in PubMed. If an author name is computationally similar with an author name for additional PubMed citations, the results will display those citations first, in ranked order, followed by the non-similar citations. Author name disambiguation details are available in Liu W and Wilbur WJ .
PubMed records contain citation information (e.g., title, authors, journal, publication date) and abstracts of published articles and books. PubMed search results do not include the full text of the journal article, but the abstract view in PubMed includes links to the full text from other sources when available, such as the publisher’s website or the PubMed Central (PMC) database. The full text journal site may require a fee or subscription, however online journals sometimes provide free access. Access may also be available through your organization, or local medical library.
You may be able to obtain free copies of full text articles in these ways:
Free full text filter
On the filter sidebar, click "Free full text" to narrow results to resources that are available for free on the web, including PubMed Central, Bookshelf, and publishers' websites. Alternately, include free full text[Filter] in your query.
PubMed Central
When full text is available in PubMed Central (PMC) , the "Free in PMC" icon will appear on the citation's abstract display under Full Text Links. Click the icon to view the article in PMC.
PubMed Central (PMC) is the U.S. National Institutes of Health (NIH) free digital archive of biomedical and life sciences journal literature.
From the publisher
Journal publishers or related organizations may provide access to articles for free, for free after registering as an individual or guest, or for a fee. When provided by the publisher or other organization, icons linking to these sources can be found on the citation's abstract display under the "Full Text Links" and/or "LinkOut" sections. Icons will often indicate free full text when the article is available for free.
Note: When you click a full text icon or link in PubMed, you leave PubMed and are directed to the full text at an external provider's site. NCBI does not hold the copyright to this material, and cannot give permission for its use. Users should review all copyright restrictions set forth by the full text provider before reproducing, redistributing, or making commercial use of material accessed through LinkOut.
Please see the Copyright and Disclaimers page for additional information.
If you are affiliated with a hospital, university, or other institution
Your local medical library is your best option. If you see icons for your library on the abstract view this indicates that your library provides a link to the article, has the journal in its collection, or may otherwise obtain the article for you through interlibrary loan. If your library does not have access to the article you need, ask a librarian about ordering the article from another institution.
Local library
Some local libraries have copies of medical journals or can get a copy of an article for you. Ask your local librarian about inter-library loan options and fees.
PubMed abstracts include figures when the full text article is available in PubMed Central (PMC) . Click the thumbnail to view a larger version of the image, caption, and link to the figure and copyright information in PMC.
The abstract page for a citation includes links to PubMed citations for similar articles. The "See all similar articles" link will retrieve a pre-calculated set of PubMed citations that are closely related to the selected article:
- Similar articles are displayed in ranked order from most to least relevant, with the "linked from" citation displayed first.
- Similar articles are generated by comparing words from the title, abstract, and MeSH terms using a word-weighted algorithm.
- Filters are not activated for similar articles.
- You can refine the list of similar articles using your search History , where the similar articles retrieval is represented as a list of PMIDs. Use this search number in a search. Refining the list removes the ranked order and may remove citations that are most relevant.
See Computation of similar articles for more information.
PubMed abstracts include links to other resources citing the current item. "Cited by" is generated using data submitted by publishers and from NCBI resources, when available. "Cited by" may not be a complete list of works citing a particular item.
PubMed abstracts include references when available. Reference lists are available for citations to full text articles included in the open access subset of PMC and for citations where the publisher supplied references in the citation data sent to PubMed.
PubMed displays grant numbers, contract numbers, and intramural research identifiers that have been associated with a publication by:
- Publishers when depositing data in PubMed and PubMed Central;
- depositing a manuscript through the NIH Manuscript Submission (NIHMS) or Europe PMC Plus system; or
- when adding a publication to My Bibliography ; and/or
- NLM text mining and indexing processes.
A grant award or contract may be acknowledged in an article and, therefore, displayed in PubMed, for various reasons, including support for activities that contributed directly to the publication as well as support for the generation of an underlying dataset or another shared resource. Additionally, some articles may not explicitly acknowledge intramural research support, yet the authors may be affiliated with a funding agency and may have associated their intramural support with a PubMed record at the time of manuscript deposit to PMC.
Funding information in PubMed is collected in or converted to a standardized format when possible to enable broad discovery and impact monitoring. For example, if a publication acknowledges support from NIH grant number 1R01 GM987654-01-A1 or GM987654 or ROI GM987654 in a publication, in PubMed the funding information would be normalized to R01 GM987654, consistent with NIH requirements for proper grant number format. Funding associations made in a manuscript submission, grant reporting, or indexing system use standardized project identifiers provided to NLM by the organization administering the funding. To learn about searching funding information, see the search field section on Grants and funding [gr] .
The scope of funding information included in PubMed has expanded over time to support the public access policies of NIH and other funding organizations . Since 1981, NLM has included grant or contract numbers or both that designate financial support by any agency of the United States Public Health Service (PHS), including NIH. Until 2000, only up to three grant numbers were included. Beginning in March 2006, funding information was expanded in PubMed to include grant, contract, and intramural funding assertions made in NIHMS and My Bibliography to support the NIH Public Access Policy. Publishers have been able to supply funding information directly to PubMed since January 2017. For more information on the history of funding information in PubMed, see the Grant Number section of MEDLINE/PubMed Data Element (Field) Descriptions .
Reporting funding information errors
Some publications may be inadvertently linked to the wrong funding information. For example, the association of a publication to NIH-funded extramural research requires that the author(s) acknowledge NIH support in the article and that the acknowledgement be in a form that can be readily associated with a specific grant or contract. Variations in the format used to cite NIH funding may lead to either an inability to make an association or erroneous matches of publications to grants and contracts.
If you identify an error in funding information associated with a PubMed record, please contact the NLM help desk . NLM will not remove funding associations that reflect the acknowledged funding in the article without a published correction to ensure alignment with the scientific record. If an award association was provided by the author, principal investigator, or project director in My Bibliography or the NIHMS for formal NIH progress and public access compliance reporting, removing the association requires the principal investigator be notified and confirm the lack of direct support.
PubMed can display up to 10,000 results. The following options can help you navigate searches with more than 10,000 results:
- Reverse the sort order to see the last results first.
- Divide the result set into smaller chunks using the results timeline or custom date range filter .
- Adjust your search to retrieve fewer results.
- For programmatic use and bulk downloads, PubMed data is available via FTP .
When available, links to other related NCBI databases are included on a citation's Abstract page under the Related information section. The complete list of database options is provided in Entrez Link Descriptions .
MEDLINE indexed citations include additional supplemental information on the Abstract page such as MeSH terms, publication types, and substances with links to search for these data in PubMed and the MeSH Database.
To simultaneously search all NCBI databases, use the NCBI Search page .
Most PubMed records include LinkOut resources to a variety of websites including publishers, aggregators, libraries, biological databases, and sequence centers. LinkOut resources link to providers’ sites to obtain the full text of articles or related information, e.g., consumer health. There may be a charge to access the text or information from a provider's site.
To view LinkOut resources, navigate to the LinkOut section at the end of an individual citation's abstract page.
To find citations with links to free full text articles, apply the "Free full text" filter to your search results.
To find citations with links to full text articles, enter search terms followed by AND full text[sb].
More information about Links:
- LinkOut resource categories such as "free full text" have been selected by the LinkOut provider.
- The current list of LinkOut providers is available.
- A publisher's icon link may display on the abstract format if they have electronically provided their citation data to NCBI. Links are only available for publishers that are participating in LinkOut; publishers are responsible for providing working links.
LinkOut links are supplied by the LinkOut providers. Publishers who electronically supply their data to PubMed may include an icon that links to a site providing the full text. Corrections and changes to links are made by the providers and are their responsibility.
To report problem links or inquire about online journal subscriptions, contact the provider directly. Contact information is typically available at a provider's web site.
Cite, Save, and Share
Save citations temporarily using the clipboard, save citations indefinitely using my ncbi collections, save citations as a text file, cite an article, export citations into citation management software, email citations, create an email alert for a search, create an rss feed for a search, print your search results, get a permalink to bookmark or share your search, download pubmed data.
The Clipboard provides a place to collect up to 500 items from one or more searches. Items saved to the Clipboard are stored in your browser cookies and will expire after 8 hours of inactivity. If you would like to save items for longer than 8 hours or to view on another device, please use Send to: Collections .
To add items to the Clipboard:
- Use the check boxes to select items from your search results. To save all results (up to a maximum of 500), do not tick any check boxes.
- Use the Send to button and choose Clipboard.
- If no items were selected, a drop-down menu of options will display where you may add selected items, all results on the page, or all results (up to a maximum limit of 500 citations) to the Clipboard.
- An individual item can also be added to the Clipboard from its abstract page.
- To view your selections, click the Clipboard link under the Search bar. This link will only appear after one or more items have been added to the Clipboard; the link is not present when the Clipboard is empty.
To delete items from the Clipboard:
- On the Clipboard page, click "Remove from Clipboard" below each item to delete the item from the Clipboard.
- Select one or more items using the check boxes next to each item, then click "Remove selected items."
- To delete all items from the Clipboard, click "Remove all."
More information about the Clipboard:
- Citations added to the Clipboard are marked with the message "Item in Clipboard" in search results.
- The maximum number of items that can be sent to the Clipboard is 500. If you select Clipboard from send to without selecting citations, PubMed will add all (up to 500 citations) of your search results to the Clipboard.
- The Clipboard will not add a citation that is currently in the Clipboard; it will not create duplicate entries.
- Your web browser must accept cookies to use the Clipboard.
- Citations in the Clipboard are represented by the search number #0, which may be used in Boolean search statements. For example, to limit the citations you have collected in the Clipboard to English language articles, use the following search: #0 AND english [la]. This does not affect or replace the Clipboard contents.
Search results can be saved in My NCBI using the Collections feature. There is no limit to the number of collections you may store in My NCBI. In addition, collections can be made public to share with others.
To save results to a new collection:
- Sign into My NCBI. Run a search in PubMed.
- Use the check boxes to select items from your search results or Clipboard. To save all results (up to a maximum of 1,000), do not tick any check boxes.
- Use the Send to button and choose Collections.
- If no items were selected, a drop-down menu of options will display where you may add selected items, all results on the page, or all results (up to a maximum limit of 1,000 citations) to a Collection.
- An individual item can also be added to a Collection from its abstract page.
- Choose Create a new collection.
- Name your collection using a short, meaningful title. The name must be unique and less than 100 characters. Identical names for different Collections are not allowed.
- Click Add to finish.
As you continue to build collections, you may want to add new items to an existing collection. To add search results to an existing collection:
- Follow steps 1 - 4 above. Add to an existing collection will be the default selection.
- Use the pull-down menu to choose a collection.
For more information on viewing, sorting, editing, merging, sharing, and deleting collections, see Collections in My NCBI Help.
Use the Save button to download citations to a text file.
- Use the check boxes to select citations from your search results or Clipboard. You may move to other pages to continue your selections. If you do not make any selections, you can choose to save “All results on this page” or “All results” from the Save menu.
- Selection: The number of selected items will be shown, for example: Selection (87).
- All results on this page
- All results (up to a maximum of 10,000 citations)
- Format: Summary (text), PubMed , PMID list, Abstract (text), or CSV
- Click Create file.
- Your web browser will prompt you to save the file on your computer.
More information about saving citations to a file:
- Saving a large set of results may take several minutes.
- To save citations in HTML format, use the "Save" or "Save as" function of your browser and change the file extension to html. When saving as HTML, only those citations displayed on the page will be saved; therefore, consider showing more results .
The Cite button makes it easy to retrieve styled citations that you can copy and paste into a document, or download an .nbib file to use with your reference manager software.
Using the Cite button for an item will open a pop-up window where you can copy the citation formatted in four popular styles: AMA (American Medical Association), MLA (Modern Language Association), APA (American Psychological Association), or NLM (National Library of Medicine). You can also download the citation as an .nbib file, which most bibliographic reference management software can import.
Note: In all citation styles, there are certain capitalization rules that machines cannot handle. For example, there is no way to identify proper nouns, acronyms, abbreviations, etc., that is 100% accurate and complies with all rules at all times. Capitalization of article titles and other citation elements should be checked for compliance with a particular reference style when required.
To export multiple citations: follow the instructions for saving citations as a text file and choose the format Summary (text) to save a list of citations in NLM style, or follow the instructions to export citations into your citation management software program .
Use Send to: Citation Manager to export citations as an .nbib file that can be used by many citation management programs:
- Use the check boxes to select citations from your search results or Clipboard. You may move to other pages to continue your selections. Alternately, you can choose to save all results on this page or all results from the Send to: Citation Manager menu.
- Click Send to and choose Citation Manager.
- Confirm the citations you want to export: selection, all results on this page, or all results (up to a maximum of 10,000).
- Import this saved file into your citation management program.
You can also download an .nbib file for individual citations using the Cite button.
Questions regarding citation management software should be directed to the respective companies.
- Use the check boxes to select citations from your search results or Clipboard. You may move to other pages and continue your selections. You may also choose to email all citations shown on the page without making any selections.
- Click the Email button.
- Enter an email address. Select which citations to send and the format.
- Click Send email. The system returns you to your results page and displays a confirmation e-mail sent message.
More information about emailing citations:
- Your citations will be sent from the NCBI automatic mail server with the sender's email address [[email protected]]. Do not reply to this message, as this is not a functioning customer service email address and is not monitored.
- The CAPTCHA image does not display for users who are signed in to My NCBI.
Click "Create alert" under the search bar to create an automatic email update for searches. You must sign in to My NCBI to use this feature. See Saving and Managing Searches for more information.
Click on Create RSS under the search box at the top of the page to create an RSS feed for your search.
- The RSS feed name will default to the search terms. You can edit the RSS feed name as needed.
- Use the pull-down menu to select the number of items displayed. You may manually edit the limit= parameter in the RSS feed link created in Step 4 to display up to a maximum of 1000 items. Please note that increasing this limit will also increase the loading time.
- Click the Create RSS button.
- The RSS Feed Link will appear; click on Copy to copy the link.
- Use this link with your feed reader or other application.
Use the print function of your web browser. To print citations from different searches, save the citations in PubMed’s Clipboard , and then print.
- Changing the display format
To get the URL for an individual citation, copy the permalink for the citation under "Share."
To get the URL for your search results, copy the URL from your web browser's address bar or bookmark the URL using your web browser's bookmark function.
To create a URL manually:
- Use the base URL: https://pubmed.ncbi.nlm.nih.gov/?term=search
- Replace “search” in the base URL with your query terms
- Escape spaces by converting them to plus signs (+); for example, Biochem Soc Trans should be entered as: Biochem+Soc+Trans
The number of characters you can use may be limited by your browser’s maximum URL length (which may be different for each browser).
Search PubMed for articles about antioxidant and chocolate:
Optional search parameters:
- format=summary, abstract, pubmed, pmid
- sort=relevance, date, pubdate, fauth, jour
- sort_order=asc
- size=10, 20, 50, 100, 200
Search PubMed for articles about breast cancer, sorted by ascending publication date (oldest to newest), and display 50 citations per page:
More information about PubMed links:
- Some settings in PubMed rely on cookies and other session data that may not be present in the URL. For example, searches that were created using a search number in Advanced History (e.g., #1 OR #2 AND human[mh]) cannot be saved using the URL because the search will be lost when your History expires.
- Users intending to send frequent queries or retrieve large numbers of records from the NCBI databases should use E-Utilities . Users must comply with the usage guidelines and requirements to prevent overloading NCBI systems.
- The NCBI Disclaimer and Copyright notice must be evident to users. Users are advised to consult legal counsel to ensure compliance with intellectual property laws. NLM cannot provide advice about copyright issues.
Once a year, NLM releases a complete (baseline) set of PubMed citation records in XML format for download from our FTP servers. Incremental update files are released daily and include new, revised, and deleted citations. The PubMed DTD states any changes to the structure and allowed elements from year to year.
Note: Binary mode must be used when downloading data from our FTP servers.
- Documentation: PubMed XML Elements and Attributes
- Terms and Conditions
- PubMed Baseline
- PubMed Update Files
For more information, please see Download PubMed Data .
Advanced Search
Searching in a specific field, browsing the index of terms, previewing the number of search results, combining searches using history, viewing the search details.
Tools included on the Advanced Search page help users to: search for terms in a specific field, combine searches and build large, complex search strings, see how each query was translated by PubMed, and compare number of results for different queries.
Use the Advanced Search Builder to search for terms in a specific field, such as author or journal. For some fields, an autocomplete feature will provide suggestions as you type.
- From the "All Fields" drop-down menu, select the field you would like to search.
- Add terms from the builder to the query box to construct your search. The default Boolean operator is AND; if desired, choose OR or NOT from the pull-down menu.
You may also search a specific field -- and bypass Automatic Term Mapping -- by adding a search field tag to a term.
The Advanced Search Builder includes the Show Index feature, which provides an alphabetical display of terms appearing in selected PubMed search fields. You can browse by all fields or within specific fields such as MeSH Terms.
- Click Advanced to navigate to the Advanced Search page, and use the Builder to select a search field from the All Fields menu. Note: Show Index is not available for every search field. The Show Index link will only display for fields that are compatible with this feature.
- Enter a term in the search box, then click Show Index.
- The index displays an alphabetic list of search terms and the approximate number of citations for each term (the actual citation count is returned when the search is executed).
- Scroll until you find a term you want to include in your search, and then highlight it to add it to the search box.
- Multiple terms may be selected from the list and added to the search box.
- Add terms from the builder to the query box to construct your search.
More information about using the index:
- PubMed processes all Boolean operators left to right.
- The builder will automatically OR (and add parentheses) for multiple terms selected from the index.
- A slash will display after a space. For example, the MeSH Term and Subheading "zika virus/analysis" will display after "zika virus infection/virology." Enter MeSH terms followed by a slash to go directly to the display for the MeSH/Subheading combination counts in the index.
- Show Index is not available for date fields.
Your PubMed search history appears on the Advanced Search page under History. This feature requires your web browser to accept cookies.
Descriptions of each column in the History table appear below:
- Search numbers may be used in place of the search string itself when combining queries (e.g., #1 OR #2).
- A repeated query will move to the top of History but will retain its original numbering.
- History is limited to the last 100 searches. Once the maximum number is reached, PubMed will remove the oldest search from history and add the most current search.
- Actions: Add, delete, or save a query. Adding queries from History places the search string into the Query box to be used in the next search. Deleting a query removes it from History.
- Query: This column shows previous search strings as entered by the user.
- Details: PubMed may modify or add search terms to a search to optimize retrieval, e.g., using automatic term mapping. Click the chevron icon " > " to expand search details and see how the search was translated.
- Results: The total number of citations retrieved for that query. Click the number to run the search and see the results in PubMed.
- Time: Timestamp of when the search was conducted.
- Please note, Microsoft Excel is typically unable to display or print more than a maximum of 1024 characters in a cell; therefore, you may want to open the CSV file with a text editor to display your complete searches.
- Delete: Click "Delete" to remove all queries from History; otherwise, History expires after 8 hours of inactivity.
- Click Advanced to navigate to the Advanced Search page.
- Use the builder to add search terms to the query box, or type your search directly into the query box.
- Use the split button to toggle the button function from "Search" to "Add to History".
- Click Add to History. This will run the search without leaving the Advanced Search page.
- See your query including the number of results in the History table.
Searches can be combined or used in later searches using your search History.
- In the History table, click the More Actions icon " ... " next to your query.
- From the available options, select "Add query" to copy the query to the Query box.
- After you've added content to the Query box, options to use the Boolean operators AND, OR, or NOT will appear when adding more queries to the Query box.
- Edit your query in the Query box if you would like to make any changes before running the search.
- Click Search (or Add to History).
More information about combining searches from your History:
- Citations in the Clipboard are represented by the search number #0, which may be used in searches. For example, to limit the citations you have collected in the clipboard to English language citations, use the following search: #0 AND english [la]. This does not change or replace the Clipboard contents.
PubMed may modify or add additional search terms to your search to optimize retrieval, such as: MeSH terms, British/American spellings, singular/plural word forms, and other synonyms.
- Search Details are included on the Advanced Search page under History.
- Click the chevron icon " > " next to a query in History to expand the Search Details.
- When expanded, the details below a query in the History table show the search strategy used to run the search.
More information about search details:
- Translations show individual term mappings using PubMed's search rules and syntax. Query terms without translations will not be listed in this section; for example, exact phrases bypass Automatic Term Mapping (ATM) .
- Warnings are displayed for the original query with potential errors in bold and red type, such as syntax errors, terms not found, or invalid tags. Warnings also appear as a highlighted message in PubMed when the search is run or added to History.
Other services
Clinical queries, single citation matcher, search pubmed using the mesh database, search for journal information in the nlm catalog, using the e-utilities api tools, citation matcher api, batch citation matcher, consumer health.
PubMed Clinical Queries provides specialized searches for:
- COVID-19 Articles
Clinical Study Categories
- Medical Genetics
Search for COVID-19 articles
The COVID-19 article filters limit retrieval to citations about the 2019 novel coronavirus. Results are displayed in a column filtered by research topic categories. See COVID-19 article filters for the filter search strategies; these may evolve over time.
To find citations using the COVID-19 article filters:
- Click Clinical Queries from the PubMed homepage
- Enter your search terms in the search box
- Click Search
- Select a Category: General, Mechanism, Transmission, Diagnosis, Treatment, Prevention, Case Report, Forecasting, or Long COVID
- Preview results in the COVID-19 Articles column
- To view the results in PubMed, click the "See all" link below the results preview
To use the COVID-19 article filters in a query, add the filter name to your search with the search field tag [Filter], e.g., LitCPrevention[Filter]. The available filters are:
- LitCGeneral
- LitCMechanism
- LitCTransmission
- LitCDiagnosis
- LitCTreatment
- LitCPrevention
- LitCCaseReport
- LitCForecasting
- LitCLongCOVID
Search PubMed for Remdesivir with the COVID-19 General filter:
Search by clinical study category
Clinical Study Categories use a specialized search method with built-in search filters that limit retrieval to citations reporting research conducted with specific methodologies, including those that report applied clinical research. See Clinical Study Categories filters for the filter search strategies.
To find citations using the Clinical Study Categories:
- Select a Category: Therapy, Diagnosis, Etiology, Prognosis, or Clinical Prediction Guides
- Select a Scope: Narrow (specific search) or Broad (sensitive search)
- Preview results in the Clinical Study Categories column
Medical genetics searches
The Medical Genetics filters limit retrieval to citations related to various topics in medical genetics. See Medical genetics search filters for the filter search strategies.
To use a Medical Genetics filter, add the filter name to your search with the search field tag [Filter], e.g., Genetic Testing[Filter]. The available filters are:
- Differential Diagnosis
- Clinical Description
- Genetic Counseling
- Molecular Genetics
- Genetic Testing
Search PubMed for sickle cell anemia using the Genetic Counseling filter:
The Single Citation Matcher has a fill-in-the-blank form for searching for a citation when you have some bibliographic information, such as journal name, volume, or page number.
- Click Single Citation Matcher on the PubMed homepage.
- Enter the citation information.
More information about using the Single Citation Matcher:
- The journal box includes an autocomplete feature that suggests titles as you enter a title abbreviation or full title. Titles displayed by the autocomplete menu are in ranked order based on the number of citations in PubMed.
- After selecting a journal with special characters (e.g., ampersand, colon) when using the Back button to return to the Single Citation Matcher you must clear and reenter the title.
- The author box also includes an autocomplete feature that suggests author names in ranked order based on the number of citations. Full author names may be searched for citations published from 2002 forward if the full author name is available in the article.
- Click either the 'Only as first author' or ‘Only as last author’ check box to limit an author name to the first or last author.
MeSH (Medical Subject Headings) is the NLM controlled vocabulary thesaurus used for indexing PubMed citations.
Use the MeSH database to find MeSH terms, including Subheadings, Publication Types, Supplementary Concepts and Pharmacological Actions, and then build a PubMed search. The MeSH database can be searched by MeSH term, MeSH Entry Term, Subheading, Publication Type, Supplementary Concept, or MeSH Scope Note.
More information about the MeSH database:
- An autocomplete feature is available from the search box.
- Search results are displayed in relevance-ranked order, therefore, when a user’s search exactly matches a MeSH Term, that Term is displayed first.
- Click the MeSH term from the Summary display or choose Full from the display format menu to view additional information and search specifications, such as Subheadings, restrict to Major MeSH Topic, or exclude terms below the term in the MeSH hierarchy.
- Year Introduced is the year the term was added to MeSH. If more than one year is shown, the term was available for indexing back to the earliest year noted. Articles are indexed using the vocabulary in place at the time of indexing, therefore, the year introduced for a term and the date of publication of a citation indexed with that term may not agree.
Launch PubMed searches from the MeSH database
To build a PubMed search from MeSH:
- Run a search in the MeSH database .
- Select terms using the check boxes.
- Click "Add to search builder" in the PubMed search builder portlet.
- You may continue searching and including additional terms to the PubMed search builder using the "Add to search builder" and Boolean pull-down menu.
- When you are finished, click "Search PubMed."
The NLM Catalog includes information about the journals in PubMed and the other NCBI databases.
Click Journals in NCBI Databases on the homepage of NLM Catalog or the Journals link on the PubMed homepage to limit your NLM Catalog results to the subset of journals that are referenced in NCBI database records.
See the NLM Catalog help for additional information.
Other journal resources include:
- PubMed journals with links to full text
- List of all journals included in PubMed via FTP
- List of Serials Indexed for Online Users
E-utilities are tools that provide access to data outside of the regular NCBI web search interface. This may be helpful for retrieving search results for use in another environment. If you are interested in large-scale data mining on PubMed data, you may download the data for free from our FTP server . Please see the terms and conditions for data users.
Fielded search
Heuristic search, auto search, rate control.
The PubMed Citation Matcher API finds PubMed identifiers (PMIDs) for citation data in structured or raw form. The interface supports three retrieval methods:
- field - runs a fielded search using core bibliographic information, such as journal, date, or volume.
- heuristic - collects all input elements into a single string and returns the closest matching documents.
- auto - combines the two above methods and switches to heuristic mode if the fielded search has not yielded a result. This is the default method.
More information about the Citation Matcher API:
- The API supports both GET and POST requests.
- Data is exchanged in JSON.
- Input data should be UTF-8 encoded.
- The API returns a maximum of 20 PMIDs; queries returning more than 20 PMIDs are treated as bad requests.
The API root is:
method=field runs a fielded search using core bibliographic information, such as journal, date, or volume. This functionality is similar to E-utilities ESearch ; users should select the API that best suits their needs.
For a structured search, the following fields can be used:
- journal - the name of the journal
- pdat - the publication date, in the format YYYY/MM/DD
- volume - the volume of the publication
- issue - the volume of the publication
- authors - one or more author names, in the format "Surname Initial" (Doe J). Optionally, the position may be specified as first, last, or auto.
Example fielded search:
GET request URL:
POST request data:
method=heuristic collects all input elements into a single string and returns the closest matching documents. It is sufficient to supply a raw citation string, such as: "The role of drag in insect hovering. J. Exp. Biol. 2004;207:4147–4155."
Example heuristic search:
method=auto first runs a fielded search , and if no results are found, it combines the fields and runs a heuristic search . This is the default method.
Example auto search:
First, a fielded search is run but no results are found due to the specified author not appearing on the citation:
Then it concatenates the fields and runs a heuristic search, which returns the closest matching document(s):
When using the PubMed Citation Matcher API programmatically, we request that you limit your application's rate to 3 requests / sec and do not make concurrent requests to this service, even at off-peak times. Additionally, requests must contain the name of the calling project in the User-Agent HTTP header value; e.g. Hydra/1.3.15 .
Use the Batch Citation Matcher to retrieve PMIDs for multiple citations. The Batch Citation Matcher requires that you enter the bibliographic information (journal, volume, page, etc.) in a specific format.
To retrieve PubMed PMIDs:
- Create citation strings for the items you would like to retrieve using the following format: journal_title|year|volume|first_page|author_name|your_key| Fields must be separated by a vertical bar with a final bar at the end of the string.
- Enter your email address. Email messages may take several minutes to process and be sent to your email address.
- Upload your citation strings as a text file (.txt) or enter each citation string on a separate line in the text box. If citation strings are entered in the text box and a file is uploaded, the results will be an aggregate of both.
- Click search.
If a match is not found the citation string will display one of the following:
- your_key|NOT_FOUND;INVALID_JOURNAL - The journal name is not valid. See the journal lists or the NLM Catalog to find the correct journal abbreviation.
- NOT_FOUND - The journal name is valid, but the citation string did not find a match.
- AMBIGUOUS - The information provided matches more than one citation. Citation information with 3 or fewer matches include the PMIDs, and more than 3 matches include the total PMID match count. Use the Single Citation Matcher or ESearch to retrieve all citations for searched fields.
- Text (.txt) format must be used when uploading a file.
- You may receive multiple emails for searches containing more than 2,000 citation strings.
- Enter author names without punctuation as smith jc. Initials are optional.
- Your key is any string you choose to tag the citation, it is returned unaltered.
- The journal title field may include the full journal title or the NLM title abbreviation.
- Each citation field is searched starting with the journal title until a unique match is found.
- The journal title is a required field however you may omit other fields. If you omit fields you must retain the vertical bars in the citation string. For example, if you omit the volume number 88 from the first example below it should be entered as: proc natl acad sci u s a|1991||3248|mann bj|P32022-1|
Example input:
- proc natl acad sci u s a|1991|88|3248|mann bj|P32022-1|
- proc natl acad sci u s a|1992|89|3271|gould se|P26261-1|
- proc natl acad sci u s a|1970|89|3271|smith|P26261-1|
- res microbiol|1992|143|467|ivey dm|P25966-1|
- science|1987|235|182|palmenberg ac|P12296-2|
- eschatology|1993|12|22|public jq|C12233-2|
- virology|1993|193|492|hardy me|Q02945-1|
- virus genes|1992|6|393||P27423-1|
- yeast|1992|8|253|sasnauskas k|P24813-1|
Example output:
- proc natl acad sci u s a|1991|88|3248|mann bj|P32022-1|2014248
- proc natl acad sci u s a|1992|89|3271|gould se|P26261-1|1565618
- proc natl acad sci u s a|1970|89|3271|smith|P26261-1|NOT_FOUND
- res microbiol|1992|143|467|ivey dm|P25966-1|1448623
- science|1987|235|182|palmenberg ac|P12296-2|3026048
- C12233-2|NOT_FOUND;INVALID_JOURNAL
- virology|1993|193|492|hardy me|Q02945-1|8382410
- virus genes|1992|6|393||P27423-1|1335631
- yeast|1992|8|253|sasnauskas k|P24813-1|1514324
The National Library of Medicine cannot provide specific medical advice. NLM urges you to consult a qualified health care professional for answers to your medical questions. NLM does not have pamphlets or other materials to mail.
MedlinePlus and MedlinePlus en español are specifically designed for consumers, containing hundreds of topic pages including NIH-written descriptive information, videos, health check tools, drug, herb and supplement info, links to Fact Sheets from other NIH Institutes, the CDC, etc., and more.
Further assistance and training
How pubmed works: automatic term mapping (atm), algorithm for finding best matching citations in pubmed, pubmed coverage, pubmed format, pubmed data field descriptions, nlm author indexing policy, error messages, mesh subheadings, pubmed character conversions, publication types, status subsets, filter search strategies, clinical queries filters, computation of similar articles, journal lists, contact customer support.
- E-mail the PubMed Help Desk
- Call the NLM Customer service desk: 1-888-FIND-NLM (1-888-346-3656)
Other NLM publications
- PubMed Online Training
- PubMed Trainer's Toolkit
- NLM Technical Bulletin
Untagged terms that are entered in the search box are matched (in this order) against a Subject translation table (including MeSH (Medical Subject Headings) ), a Journals translation table, the Author index, and an Investigator (Collaborator) index.
When a match is found for a term or phrase in a translation table the mapping process is complete and does not continue on to the next translation table.
To see how your terms were translated, check the Search Details available on the Advanced Search page for each query under History. If you want to report a translation that does not seem accurate for your search topic, please e-mail the information to the NLM Help Desk .
1. Subject translation table
The Subject Translation Table contains:
- British and American spellings
- Pairs: singular and plural word forms, synonyms, and other closely related terms
- Drug brand name to generic name translations
- The See-Reference mappings (also known as entry terms) for MeSH terms
- Pharmacologic action terms
- Terms derived from the Unified Medical Language System (UMLS) that have equivalent synonyms or lexical variants in English
- Supplementary concept (substance) names and their synonyms.
If a match is found in this translation table, the term will be searched as MeSH (that includes the MeSH term and any specific terms indented under that term in the MeSH hierarchy), and in all fields.
For example, if you enter child rearing in the search box, PubMed will translate this search to: "child rearing"[MeSH Terms] OR ("child"[All Fields] AND "rearing"[All Fields]) OR "child rearing"[All Fields]
If you enter a MeSH Term that is also a Pharmacologic Action PubMed will search the term as [MeSH Terms], [Pharmacologic Action], and [All Fields].
If you enter an entry term for a MeSH term the translation will also include an all fields search for the MeSH term associated with the entry term. For example, a search for odontalgia will translate to: "toothache"[MeSH Terms] OR "toothache"[All Fields] OR "odontalgia"[All Fields] OR "odontalgias"[All Fields] because Odontalgia is an entry term for the MeSH term toothache.
Substance name mappings do not include a mapping for individual terms in a phrase, e.g., IL-22 will not include IL[All Fields] AND 22[All Fields].
MeSH term mappings that include a standalone number or single character do not include a mapping for individual terms in a phrase, e.g., Protein C will not include Protein[All Fields] or C[All Fields].
2. Journals translation table
The Journals translation table contains the:
- full journal title
- title abbreviation
- ISSN and eISSN number.
These will automatically map to the journal abbreviation that is used to search journals in PubMed and in all fields. For example, a search for endocrine pathology will translate to: "Endocr Pathol"[Journal] OR ("endocrine"[All Fields] AND "pathology"[All Fields]) OR "endocrine pathology"[All Fields]
3. Author index
If the term is not found in the above tables, and is not a single term, PubMed checks the author index for a match. The author index includes author names and initials, as well as full author names for articles published from 2002 forward, if available.
- PubMed automatically truncates a search for an author's name to account for varying initials, e.g., o'brien j retrieves o'brien ja, o'brien jb, o'brien jc jr, as well as o'brien j.
- When combining multiple authors, to avoid a match with full author names, include initials or use the [au] search tag, e.g., ryan[au] james[au]. Author names comprised of only stopwords, e.g., as a, are not searched as authors if they are part of phrase, chemical burn as a danger, unless the search only includes the author name, e.g., as a.
- Initials and suffixes are not required, if you include a middle initial or suffix, you will only retrieve citations for articles that were published using the middle initial or suffix.
- To distinguish author initials that may match a full author name use the [fau] search tag, e.g., peterson do[fau].
4. Investigator (Collaborator) index
If the term is not found in the above tables, except for Author, and is not a single term, the investigator index is consulted for a match. The investigator (collaborator) index includes full names, if available. Enter a full investigator name in natural or inverted order, e.g., harry janes or janes harry.
5. If no match is found?
PubMed breaks apart the phrase and repeats the above automatic term mapping process until a match is found. PubMed ignores stopwords in searches.
If there is no match, the individual terms will be combined (ANDed) together and searched in all fields.
When a search includes terms that were tagged with a search field during the automatic term mapping process and retrieves zero results, the system triggers a subsequent search using "Schema: all ." "Schema: all" modifies the search by removing the automatically added search field tags, and then searches each term in all fields.
The learned ranking algorithm combines over 150 signals that are helpful for finding best matching results. Most of these signals are computed from the query-document term pairs (e.g., number of term matches between the query and the document) while others are either specific to a document (e.g., publication type; publication year) or query (e.g., query length). The new ranking model was built on relevance data extracted from the anonymous and aggregated PubMed search logs over an extended period of time.
For more information about the Best Match algorithm, please see:
- Technical details in the paper Best Match: New relevance search for PubMed by Fiorini N, Canese K, Starchenko G, et al. in PLoS Biol (2018).
- NLM Technical Bulletin article: Updated Algorithm for the PubMed Best Match Sort Order
The PubMed database contains citations and abstracts to biomedical literature, facilitating searching across several NLM literature resources:
PubMed Central (PMC)
Ncbi bookshelf.
For additional information, please see the NLM Fact Sheet: Medline, PubMed, and PMC (PubMed Central): How are they different?
PubMed includes citations to original research articles, literature reviews, case reports, letters, editorials, commentaries, and other selected publications on scientific and medical topics (see: publication types found in PubMed ). Some categories of content are out of scope for PubMed, such as: book reviews, individual conference abstracts, obituaries and in memoriam articles , news and announcements, and brief summaries of research articles. More examples are included in XML Help for PubMed Data Providers: What types of articles are accepted? .
MEDLINE contains citations to journal articles in the life sciences with a concentration on biomedicine. The MEDLINE database contains citations from the late 1940s to the present , with some older material.
New citations from MEDLINE journals are received electronically from publishers and appear in PubMed daily. Most citations progress to in-process, and then to indexed for MEDLINE; however, not all citations will be indexed for MEDLINE. PubMed includes some citations from MEDLINE journals that are not indexed for MEDLINE, such as:
- Citations preceding the date that a journal was selected for MEDLINE indexing.
- Out-of-scope citations (e.g., articles on plate tectonics or astrophysics) from certain MEDLINE journals, primarily general science and chemistry journals, for which the life sciences articles are indexed for MEDLINE.
Citations that have been indexed for MEDLINE and updated with NLM Medical Subject Headings (MeSH) , publication types, GenBank accession numbers, and other indexing data are available daily. To limit your search to MEDLINE citations, add medline[sb] to your search.
Indexing method
The method used to assign Medical Subject Headings (MeSH) has changed over time. For more information, please see Incorporating Values for Indexing Method in MEDLINE/PubMed XML . Use the following searches to find citations indexed with each method:
- Automated - MeSH indexing is provided algorithmically. Search: indexingmethod_automated
- Curated - MeSH indexing is provided algorithmically and a human reviewed (and possibly modified) the algorithm results. Search: indexingmethod_curated
- Fully human indexed – Search: medline[sb] NOT (indexingmethod_curated OR indexingmethod_automated)
PubMed Central (PMC) is a full text archive that includes articles from journals reviewed and selected by NLM for archiving (current and historical), as well as individual articles and preprints collected for archiving in compliance with funder policies. Some PMC content is not cited in PubMed, such as book reviews and conference abstracts (see: PubMed coverage ).
As of June 2020, PubMed Central (PMC) includes preprints that report NIH-funded research results. Citations to these preprints are deposited in PubMed. To learn more, see: NIH Preprint Pilot .
To search for preprints in PubMed, include preprint[filter] in your query.
To exclude preprints from your search results in PubMed, use the Boolean operator NOT.
Bookshelf is a full text archive of books, reports, databases, and other documents related to biomedical, health, and life sciences. PubMed includes citations for books and some individual chapters available on Bookshelf.
The PubMed Format tags table defines the data tags that compose the PubMed format. The tags are presented in alphabetical order. Some of the tags (e.g., CIN) are not mandatory and therefore will not be found in every record. Other tags (e.g., AU, MH, and RN) may occur multiple times in one record. You can download records in PubMed format as a text file (.txt) or as an .nbib file for exporting into citation management software programs .
Not all fields are searchable in PubMed. See Search field tags for the list of searchable fields.
This documentation describes the fields found in PubMed records. If a field is searchable, the search tag appears after the field name in square brackets: Affiliation [ad]. A small number of searchable fields do not correspond to a specific field in the PubMed format .
- See Search field tags for a list of searchable fields.
- See PubMed format for a quick table view of the fields found in PubMed records.
Affiliation may be included for authors, corporate authors and investigators, e.g., cleveland [ad] AND clinic [ad], if submitted by the publisher.
Multiple affiliations were added to citations starting from 2014, previously only the first author’s affiliation was included. PubMed includes the note "Contributed equally" in the affiliation field when this information is supplied by publishers.
Searching for terms in the affiliation field searches in all author affiliations on a citation. For example, a search for Hopkins[ad] AND Bloomberg[ad] can find these terms spread across multiple authors’ affiliations on the same citation.
To search for multiple terms appearing within the same affiliation, use a proximity search . You can also search affiliations using a phrase search ; however, we suggest using a proximity search for more comprehensive results because affiliation data may be provided in a variety of ways for the same institution.
Use proximity searching to find citations with authors from the Johns Hopkins Bloomberg School of Public Health:
This search will find any citation where the words "Hopkins," "Bloomberg," and "Public" appear in the same affiliation, with no more than forty-five words between each term. Search results may include:
- Johns Hopkins Bloomberg School of Public Health
- Johns Hopkins University, Bloomberg School of Public Health
- Bloomberg School of Public Health, Johns Hopkins University
- Bloomberg Johns Hopkins University School of Public Health
- ...and more!
Untagged terms and terms tagged with [all] are processed using Automatic Term Mapping (ATM) . Terms that do not map are searched in all search fields except for Place of Publication, Create Date, Completion Date, Entry Date, MeSH Date, and Modification Date. Terms enclosed in double quotes or truncated will be searched in all fields and not processed using automatic term mapping. PubMed ignores stopwords .
Includes article identifiers submitted by journal publishers such as DOI (digital object identifier).
The format to search for this field is: last name followed by a space and up to the first two initials followed by a space and a suffix abbreviation, if applicable, all without periods or a comma after the last name (e.g., fauci as or o'brien jc jr). Initials and suffixes may be omitted when searching.
PubMed automatically truncates a search for an author's name to account for varying initials, e.g., o'brien j [au] will retrieve o'brien ja, o'brien jb, o'brien jc jr, as well as o'brien j. To turn off automatic truncation, enclose the author's name in double quotes and tag with [au] in brackets, e.g., "o'brien j" [au] to retrieve just o'brien j.
Searching by full author name for articles published from 2002 forward is also possible, if available. See NLM policy on author names .
The author identifier includes a unique identifier associated with an author, corporate or investigator name, if supplied by a publisher. The field includes the organization authority that established the unique identifier, such as, ORCID, ISNI, VIAF, e.g., orcid 0000-0001-5027-4446 [auid].
The book search field includes book citations, e.g., genereviews [book].
Use the following untagged searches to retrieve all book or book chapters, e.g., ataxia AND pmcbookchapter
- books and chapters: pmcbook
- books: pmcbooktitle
- book chapters: pmcbookchapter
The above searches capture book records provided by the NCBI Bookshelf database; they exclude a small number of documents from other providers that appear in both PubMed and Bookshelf. For the most comprehensive search of records appearing in both PubMed and Bookshelf, search "pubmed books"[sb].
The data in these fields are citations to other associated journal publications, e.g., comments or errata. Often these link to the respective citation. Comments/Corrections data can be retrieved by the search term that follows each type:
- Comment in: hascommentin
- Comment on: hascommenton
- Corrected and republished in: hascorrectedrepublishedin
- Corrected and republished from: hascorrectedrepublishedfrom
- Dataset use reported in: hasassociatedpublication
- Dataset described in: hasassociateddataset
- Erratum in: haserratumin
- Erratum for: haserratumfor
- Expression of concern in: hasexpressionofconcernin
- Expression of concern for: hasexpressionofconcernfor
- Original Report in: hasoriginalreportin
- Republished in: hasrepublishedin
- Republished from: hasrepublishedfrom
- Retracted and republished in: hasretractedandrepublishedin
- Retracted and republished from: hasretractedandrepublishedfrom
- Retraction in: hasretractionin
- Retraction of: hasretractionof
- Summary for patients in: hassummaryforpatientsin
- Update in: hasupdatein
- Update of: hasupdateof
Used by NLM for internal processing. Completon Date is not included in All Fields retrieval; the [dcom] search tag is required.
The conflict of interest statement from the published article. Conflict of interest statements are available when supplied by the publisher in the citation data sent to PubMed, or when included in full text articles in PubMed Central (PMC).
To retrieve all citations that contain conflict of interest statements, use the query hascois.
Corporate author identifies the corporate or collective authorship of an article. Corporate names display exactly as they appear in the journal.
Note: Citations indexed pre-2000 and some citations indexed in 2000-2001 retain corporate authors at the end of the title field. For comprehensive searches, consider including terms and/or words searched in the title field [ti].
The date the citation record was first created in PubMed. Create Date can be helpful when checking PubMed for citations added since the last time a query was run. Create Date is not included in All Fields retrieval; the [crdt] search tag is required.
EC/RN numbers are assigned by:
- The Food and Drug Administration (FDA) Substance Registration System for Unique Ingredient Identifiers (UNIIs), e.g., Y92OUS2H9B
- The Enzyme Commission (EC) to designate a particular enzyme, e.g., EC 1.1.1.57
- The Chemical Abstracts Service (CAS) for Registry Numbers, e.g., 2751-14-6
The EC/RN number search field includes both the Registry Number and the Related Registry Number (available in the NLM MeSH Browser).
The editor search field includes the editors for book or chapter citations.
Entry date (EDAT) is used for PubMed processing, such as “Most Recent” sort order (i.e., last in, first out).
EDAT is typically set within 24 hours of the citation’s availability in PubMed. Exceptions: As of December 15, 2008, citations added to PubMed more than twelve months after the date of publication have the EDAT set to the date of publication, except for book citations. Prior to this, the Entry Date was set to the Publication Date on citations published before September 1997. Entry Date is not included in All Fields retrieval; the [edat] search tag is required.
Note: Entry Date was called Entrez Date in the legacy PubMed system (retired in 2020).
Technical tags used by LinkOut, filters include:
- loall[sb] - citations with LinkOut links in PubMed
- free full text[sb] - citations that include a link to a free full text article
- full text[sb] - citations that include a link to a full text article
The first personal author name in a citation.
The full author name for articles published from 2002 forward, if available. Full author searches can be entered in natural or inverted order, e.g., julia s wong or wong julia s.
The index for the article's full investigator or collaborator name, if available. Full investigator searches can be entered in natural or inverted order, e.g., harry janes or janes harry.
The Grants and funding [gr] search field (previously Grant Number) includes grant numbers, contract numbers, or other intramural research identifiers associated with a publication.
The most common type of funding information associated with a publication in PubMed are grant numbers. Data in the Grants and funding search field can consist of up to four parts:
- Number contains the grant, contract, intramural project number (or both) that designates financial support by any agency of the United States Public Health Service (US PHS), any institute of the National Institutes of Health, or other organization.
- Funder code contains the 2-letter grant code or funding organization acronym, for example: CA for National Cancer Institute or DDCF for Doris Duke Charitable Foundation. See Grant Number Information Found in the GR Field in MEDLINE/PubMed (Archived) for the 2-character abbreviations, PHS agency acronyms, and other US and non-US funding organizations.
- Agency includes the acronym or mnemonic in the case of US PHS agencies, or full organization name. As of 2009 this includes the agency's hierarchical structure from lower to higher entity, when known. For example, NCI NIH HHS for National Cancer Institute, National Institutes of Health, U.S. Department of Health and Human Services.
- Country contains the home country of the funding agency, for example: United States.
Each individual part can be searched using [gr], for example: CA101211[gr], CA[gr], NCI[gr], NIH[gr], or United States[gr].
This field can also be searched to find articles with intramural support; e.g., "intramural nih"[gr] finds all journal citations authored by intramural NIH staff.
Completeness of funding information in PubMed will vary by source.
See Grants and funding for more information about data in this field.
Names of principal investigator(s) or collaborators who contributed to the research. Search names following the author field format, for example: soller b[ir].
The ISBN for book or book chapters.
The number of the journal issue in which the article was published.
The journal search field includes the journal title abbreviation, full journal title, or ISSN/eISSN number (e.g., J Biol Chem, Journal of Biological Chemistry, 0021-9258). If a journal title contains special characters, e.g., parentheses, brackets, enter the name without these characters, e.g., enter J Hand Surg [Am] as J Hand Surg Am.
The language search field includes the language in which the article was published. Note that many non-English articles have English language abstracts. You may search using either the language or the first three characters of most languages, e.g., chi [la] retrieves the same results as chinese [la]. The most notable exception is jpn [la] for Japanese.
The last personal author name in a citation.
Location ID includes the DOI or publisher ID that serves the role of pagination to locate an online article.
The date the citation was indexed with MeSH Terms and elevated to MEDLINE for citations with an Entry Date after March 4, 2000. The MeSH Date is initially set to the Entry Date when the citation is added to PubMed. MeSH Date is not included in All Fields retrieval; the [mhda] search tag is required.
Dates must be entered using the format YYYY/MM/DD [mhda], e.g., 2000/03/15 [mhda]. The month and day are optional (e.g., 2000 [mhda] or 2000/03 [mhda]).
To enter a date range, insert a colon (:) between each date, e.g., 1999:2000 [mhda] or 2000/03:2000/04 [mhda].
A MeSH term that is one of the main topics discussed in the article denoted by an asterisk on the MeSH term or MeSH/Subheading combination, e.g., Cytokines/physiology* See MeSH Terms [mh] below.
MeSH Subheadings are used with MeSH terms to help describe more completely a particular aspect of a subject. For example, the drug therapy of asthma is displayed as asthma/drug therapy; see MeSH/Subheading Combinations in MeSH Terms [mh] below.
The MeSH Subheading field allows users to "free float" Subheadings, e.g., hypertension [mh] AND toxicity [sh].
MeSH Subheadings automatically include the more specific Subheading terms under the term in a search. To turn off this automatic feature, use the search syntax [sh:noexp], e.g., therapy [sh:noexp].
In addition, you can enter the two-letter MeSH Subheading abbreviations rather than spelling out the Subheading, e.g., dh [sh] = diet therapy [sh].
The NLM Medical Subject Headings controlled vocabulary of biomedical terms that is used to describe the subject of each journal article in MEDLINE. MeSH is updated annually to reflect changes in medicine and medical terminology. MeSH terms are arranged hierarchically by subject categories with more specific terms arranged beneath broader terms. PubMed allows you to view this hierarchy and select terms for searching in the MeSH Database.
MEDLINE articles are automatically indexed with MeSH terms using a well-refined algorithm. Applying the MeSH vocabulary ensures that articles are uniformly indexed by subject, whatever the author's words. For more information, see Frequently Asked Questions about Indexing for MEDLINE .
More information about MeSH Terms and Major MeSH Topic search fields:
- To search the term only as a MeSH term, it must be tagged using the search field, e.g., [mh] for MeSH Terms or [majr] for MeSH Major Topic. A tagged term is checked against the subject translation table , and then mapped to the appropriate MeSH term(s). To turn off mapping to multiple MeSH terms, enter the tagged MeSH term in double quotes.
- MeSH terms are arranged hierarchically by subject categories with more specific terms arranged beneath broader terms. MeSH terms in PubMed automatically include the more specific MeSH terms in a search. To turn off this automatic feature, use the search syntax [mh:noexp], e.g., neoplasms [mh:noexp].For more detailed information about MeSH vocabulary including the hierarchical structure, please see the MeSH homepage .
- MeSH/Subheading Combinations: To directly attach MeSH Subheadings, use the format MeSH Term/Subheading, e.g., neoplasms/diet therapy. You may also use the two-letter MeSH Subheading abbreviations , e.g., neoplasms/dh. The [mh] tag is not required, however [majr] may be used, e.g., plants/genetics[majr]. Only one Subheading may be directly attached to a MeSH term. For a MeSH/Subheading combination, PubMed always includes the more specific terms arranged beneath broader terms for the MeSH term and also includes the more specific terms arranged beneath broader Subheadings . The broader Subheading, or one of its indentions, will be directly attached to the MeSH term or one of its indentions. For example, hypertension/therapy also retrieves hypertension/diet therapy; hypertension/drug therapy; hypertension, malignant/therapy; hypertension, malignant/drug therapy, and so on, as well as hypertension/therapy.
- To turn off the automatic inclusion of the more specific terms, use the syntax [field:noexp], e.g., hypertension [mh:noexp], or hypertension [majr:noexp], or hypertension/therapy [mh:noexp]. The latter example turns off the more specific terms in both parts, searching for only the one Subheading therapy attached directly to only the one MeSH term hypertension.
- If parentheses are embedded in a MeSH term, replace the parentheses with a space and tag with [mh] e.g., enter the MeSH term Benzo(a)pyrene as benzo a pyrene [mh].
- MeSH terms can be selected for searching in the MeSH database and from the advanced search builder index.
Modification date is a completed citation’s most recent revision date. Modification Date is not included in All Fields retrieval; the [lr] search tag is required.
The NLM ID is the alpha-numeric identifier for the cited journal that was assigned by the NLM Integrated Library System LocatorPlus, e.g., 0375267 [jid].
The author keyword field (OT field) is searchable with the title/abstract [tiab], text word [tw] and other term [ot] search tags. To retrieve all citations that have keywords, use the query haskeyword. Other term data may display an asterisk to indicate a major concept; however, you cannot search other terms with a major concept tag.
The owner search field includes the acronym that identifies the organization that supplied the citation data. Search using owner + the owner acronym, e.g., ownernasa.
Enter only the first page number that the article appears on. The citation will display the full pagination of the article but this field is searchable using only the first page number.
Use this search field tag to limit retrieval to where the name is the subject of the article, e.g., varmus h[ps]. Search for personal names as subject using the author field format, e.g., varmus h[ps].
Substances known to have a particular pharmacologic action. Each pharmacologic action term index is created with the drug/substance terms known to have that effect. This includes both MeSH terms and terms for Supplementary Concept Records.
Indicates the cited journal's country of publication. Geographic place of publication regions are not searchable. In order to retrieve records for all countries in a region (e.g., North America) it is necessary to OR together the countries of interest. Note: This field is not included in all fields or text word retrieval.
Search for PMC or NIH manuscript identifiers using the appropriate prefix followed by the ID number, e.g., PMC2600426. To retrieve all NIH manuscript citations, use the query hasnihmsid.
To search for a PubMed Identifier (PMID), enter the ID with or without the search field tag [pmid]. You can search for several PMIDs by entering each number in the search box separated by a space (e.g., 17170002 16381840); PubMed will OR the PMIDs together.
PMIDs do not change over time or during processing and are never reused.
Publication date is the date that the article was published. The search field tags [dp] and [pdat] may be used interchangeably for publication date searching.
Dates or date ranges must be searched using the format yyyy/mm/dd [dp], e.g., 1998/03/06 [dp]. The month and day are optional (e.g., 1998 [dp] or 1998/03 [dp]).
To enter a date range search, insert a colon (:) between each date, e.g., 1996:1998 [dp] or 1998/01:1998/04 [dp].
Use the following format to search X days, months or years immediately preceding today’s date where X = numeric value:
- "last X days"[dp]
- "last X months"[dp]
- "last X year"[dp]
More information about publication dates:
- The time between an article’s publication and the citation’s availability in PubMed varies depending on when the publisher deposits the citation to PubMed. Because of this, searching with Create Date [crdt] (the date a citation was created in PubMed) is often more comprehensive than Publication Date [dp] when checking PubMed on a regular basis for new citations.
- Journals vary in the way the publication date appears on an issue. Some journals include just the year, whereas others include the year plus month or year plus month plus day. And, some journals use the year and season (e.g., Winter 1997). The publication date in the citation is recorded as it appears in the journal.
- Publication dates without a month are set to January, multiple months (e.g., Oct-Dec) are set to the first month, and dates without a day are set to the first day of the month. Dates with a season are set as: winter = January, spring = April, summer = July and fall = October.
- If an article is published electronically and in print on different dates both dates are searchable and may be included on the citation prefaced with an Epub or Print label. The electronic date will not be searchable if it is later than the print date, except when range searching.
- To search for electronic dates only use the search tag [EPDAT], for print dates only tag with [PPDAT].
- Most journals now publish articles online on a continuous basis, as soon as they are ready for publication (after peer review and editing, etc.) instead of, or in addition to, publishing collections of articles as an "issue" on a periodic basis. When a journal deposits a citation for an "online first" article in PubMed, NLM appends the note "[Online ahead of print]" to the online publication date. The citation is updated, and the ahead of print notation removed, when the article is included in a journal issue. The lag between the "online first" and "issue" publication dates may be days, weeks, months, or more than a year. In many cases, depending on the journal, the online first version is considered to be the version of record. The "[Online ahead of print]" note in PubMed should not be taken to mean that the cited article is not the version of record.
- Bookshelf citation publication dates are generated from the book’s publication date.
Describes the material presented in the article (e.g., Review, Clinical Trial, Retracted Publication, Letter). Citations may include multiple Publication Types. Use the search tag [pt] with any PubMed Publication Type , e.g., review[pt].
Publication Types are arranged hierarchically with more specific terms arranged beneath broader terms, and publication types automatically include the more specific publication types in a search. To turn off this automatic feature, use the search syntax [pt:noexp], e.g., review [pt:noexp].
Includes publisher names for Bookshelf citations.
The SI field identifies secondary source databanks and accession numbers, e.g., GenBank, GEO, PubChem, ClinicalTrials.gov , ISRCTN. The field is composed of the source followed by a slash followed by an accession number and can be searched with one or both components, e.g., genbank [si], AF001892 [si], genbank/AF001892 [si]. To retrieve all citations with an SI value, search hasdatabanklist.
The subset field is a method of restricting retrieval by subject, citation status and journal category, with the search tag [SB]. See also filters and Find related resources using LinkOut.
Includes chemical, protocol, disease or organism terms. Synonyms to the supplementary concepts will automatically map when tagged with [nm]. This field was implemented in mid-1980; however, many chemical names are searchable as MeSH terms before that date.
Includes all words and numbers in the title, abstract, other abstract, MeSH terms, MeSH Subheadings, Publication Types, Substance Names, Personal Name as Subject, Corporate Author, Secondary Source, Comment/Correction Notes, and Other Terms (see Other Term [OT] above) typically non-MeSH subject terms (keywords), including NASA Space Flight Mission, assigned by an organization other than NLM.
Words and numbers included in the title of a citation, as well as the collection title for book citations.
Words and numbers included in a citation's title, collection title, abstract, other abstract and author keywords ( Other Term [ot] field). English language abstracts are taken directly from the published article. If an article does not have a published abstract, NLM does not create one.
Words and numbers in title originally published in a non-English language, in that language. Non-Roman alphabet language titles are transliterated. Transliterated title is not included in Text Word [TW] retrieval.
The number of the journal volume in which an article is published.
NLM author indexing policy is as follows:
- 1966 - 1984: MEDLINE did not limit the number of authors.
- 1984 - 1995: The NLM limited the number of authors to 10, with "et al" as the eleventh occurrence.
- 1996 - 1999: The NLM increased the limit from 10 to 25. If there were more than 25 authors, the first 24 were listed, the last author was used as the 25th, and the twenty-sixth and beyond became "et al."
- 2000 - Present: MEDLINE does not limit the number of authors.
More information:
- Beginning in mid-2005, the policy restrictions on number of author names in past years were lifted so that on an individual basis, a citation may be edited to include all author names in the published article, regardless of the limitation in effect when the citation was created.
- Effective with 1992 date of publication, letters are indexed individually with authors rather than as an anonymous group.
- Until 1990, NLM transliterated up to five authors' Cyrillic or Japanese names to the Roman alphabet.
- Between 1990 and 2016, the first ten Cyrillic or Japanese names are transliterated. Chinese ideograms were not transliterated by NLM, but if transliterations of the authors names are available in the journal article or table of contents, they were included in the citation, even if that includes only one author in a multi-author article.
- Beginning in 2016, author names are published in Roman characters in all MEDLINE journals, and NLM no longer transliterates Cyrillic or Japanese names. All author names are included as published.
System error messages
Please provide your IT staff with the technical browser advice for NCBI web pages to ensure your browser, firewall, and servers are enabled for JavaScript, cookies, pop-ups, and HTTP 1.1. Antivirus software may affect page caching which can result in unexpected page expired messages. Also, nlm.nih.gov should be added as a browser exception and be considered a trusted site by your system and network. You may have to delete your browser's cache (temporary files) before trying to access PubMed again.
Typographical errors
Please contact the journal publisher directly to report an error and initiate a correction to PubMed citations for content other than MeSH.
To report a MeSH error in a PubMed citation, please contact the NLM Help Desk and include the PMID number (e.g., PMID: 12345678), and an indication of the incorrect and correct information.
NLM provides data to vendors around the world. Other products and services will not necessarily immediately reflect corrections made to PubMed records. If you search through a vendor's system, please contact your vendor about their maintenance schedules.
A "cookie" is information stored by a web site server on your computer. See the NLM Privacy Policy for additional information.
In the case of PubMed, cookies store information about your interactions that may be needed later to perform a function. To use these interactive features you need to enable cookies on your computer. Consult your browser's help for information on enabling cookies.
If you have problems using cookie-dependent features of PubMed, even after enabling cookies, possible reasons may include:
- Cookies are blocked by your provider or institution. Check with your Internet provider and/or the system administrator at your institution to see if cookies can be accepted. Even if you have them enabled in your web browser, if they are blocked by your provider or institution (e.g., by a firewall, proxy server, etc.), cookie-dependent features of PubMed won't work.
- Your computer's date and time settings are incorrect. Check your computer's time settings to ensure that they are correct.
See the MeSH Subheadings table below and scope notes and allowable categories on the NLM website.
Certain characters have special meaning in searches, others are converted to spaces.
Searches that include the following characters are translated as follows:
- parentheses ( ) - used to create Boolean nesting
- square brackets [ ] - search field tag qualification
- ampersand & - Boolean operator AND
- pipe | - Boolean operator OR
- forward slash / - MeSH/Subheading combinations
- colon : - designates a range operation
- double quotes " - used to force a phrase search
- pound sign # - designates a History search statement when immediately followed by a number, e.g., #1 AND cat
- asterisk * - wildcard symbol for search term truncation, e.g., toxicol*
Characters converted to spaces in search queries:
- exclamation mark !
- pound sign #
- dollar sign $
- percentage sign %
- asterisk * (if it cannot be used in a wildcard search, for example, when a term is too short)
- plus symbol +
- minus symbol -
- semi-colon ;
- angle brackets < >
- equal sign =
- question mark ?
- backslash \
- underscore _
- curly brackets { }
- approximately ~
- single quotes '
Some characters have special meaning in MeSH fields:
- forward slash /
Publication types found in PubMed are listed below. See Publication Type [PT] and MeSH Publication Types with Scope Notes for more information; however, not all MeSH Publication Types are included in PubMed.
- Adaptive Clinical Trial
- Autobiography
- Bibliography
- Case Reports
- Classical Article
- Clinical Conference
- Clinical Study
- Clinical Trial
- Clinical Trial, Phase I
- Clinical Trial, Phase II
- Clinical Trial, Phase III
- Clinical Trial, Phase IV
- Clinical Trial Protocol
- Clinical Trial, Veterinary
- Collected Work
- Comparative Study
- Consensus Development Conference
- Consensus Development Conference, NIH
- Controlled Clinical Trial
- Corrected and Republished Article
- Duplicate Publication
- Electronic Supplementary Materials
- English Abstract
- Equivalence Trial
- Evaluation Study
- Expression of Concern
- Festschrift
- Government Publication
- Historical Article
- Interactive Tutorial
- Introductory Journal Article
- Journal Article (Default value when no more descriptive PT is provided or assigned)
- Legislation
- Meta-Analysis
- Multicenter Study
- Newspaper Article
- Observational Study
- Observational Study, Veterinary
- Patient Education Handout
- Periodical Index
- Personal Narrative
- Practice Guideline
- Pragmatic Clinical Trial
- Published Erratum
- Randomized Controlled Trial
- Randomized Controlled Trial, Veterinary
- Research Support, American Recovery and Reinvestment Act
- Research Support, N.I.H., Extramural
- Research Support, N.I.H., Intramural
- Research Support, Non-U.S. Gov't
- Research Support, U.S. Gov't, Non-P.H.S.
- Research Support, U.S. Gov't, P.H.S.
- Retracted Publication
- Retraction of Publication
- Scientific Integrity Review
- Systematic Review
- Technical Report
- Validation Study
- Video-Audio Media
Article attribute
Most article type filters use the article type name with the publication type [pt] search field tag; for example, "multicenter study"[pt].
The Systematic Review filter uses a search strategy in addition to the publication type [pt].
The Books and Documents filter uses the following query: "pubmed books"[sb].
The article language filters use the language name with the language [la] search field tag; for example, esperanto[la].
See Other filters and more subsets .
COVID-19 article filters
The COVID-19 article filters limit retrieval to citations about the 2019 novel coronavirus; these filters may evolve over time.
The Clinical Study Categories search filters are based on the work of Haynes RB et al.
Clinical Study Categories bibliography
The Clinical Queries search strategies have been updated based on new evidence from Haynes et al. The current strategies have better performance than their predecessors . Details of methods appear in the references below.
Revised December 2011
- Wilczynski NL, McKibbon KA, Haynes RB. Sensitive Clinical Queries retrieved relevant systematic reviews as well as primary studies: an analytic survey. J Clin Epidemiol. 2011 Dec;64(12):1341-9. doi: 10.1016/j.jclinepi.2011.04.007. Epub 2011 Jul 19. PMID: 21775104 .
- Lokker C, Haynes RB, Wilczynski NL, McKibbon KA, Walter SD. Retrieval of diagnostic and treatment studies for clinical use through PubMed and PubMed's Clinical Queries filters. J Am Med Inform Assoc. 2011 Sep-Oct;18(5):652-9. doi: 10.1136/amiajnl-2011-000233. Epub 2011 Jun 15. PMID: 21680559 ; PMCID: PMC3168323 .
- Wilczynski NL, Haynes RB; QI Hedges Team. Optimal search filters for detecting quality improvement studies in Medline. Qual Saf Health Care. 2010 Dec;19(6):e31. doi: 10.1136/qshc.2010.042432. Epub 2010 Jul 29. PMID: 20671080 .
- Kastner M, Wilczynski NL, McKibbon AK, Garg AX, Haynes RB. Diagnostic test systematic reviews: bibliographic search filters ("Clinical Queries") for diagnostic accuracy studies perform well. J Clin Epidemiol. 2009 Sep;62(9):974-81. doi: 10.1016/j.jclinepi.2008.11.006. Epub 2009 Feb 20. PMID: 19230607 ; PMCID: PMC2737707 .
- Wilczynski NL, Haynes RB. Response to Corrao et al.: Improving efficacy of PubMed clinical queries for retrieving scientifically strong studies on treatment. J Am Med Inform Assoc. 2007 Mar-Apr;14(2):247-8. Epub 2007 Jan 9. PMID: 17213490 ; PMCID: PMC2213472 .
- Wilczynski NL, McKibbon KA, Haynes RB. Response to Glanville et al.: How to identify randomized controlled trials in MEDLINE: ten years on. J Med Libr Assoc. 2007 Apr;95(2):117-8; author reply 119-20. PMID: 17443240 ; PMCID: PMC1852612 .
- Wilczynski NL, Morgan D, Haynes RB; Hedges Team. An overview of the design and methods for retrieving high-quality studies for clinical care. BMC Med Inform Decis Mak. 2005 Jun 21;5:20. doi: 10.1186/1472-6947-5-20. PMID: 15969765 ; PMCID: PMC1183213 .
- Haynes RB, McKibbon KA, Wilczynski NL, Walter SD, Werre SR; Hedges Team. Optimal search strategies for retrieving scientifically strong studies of treatment from Medline: analytical survey. BMJ. 2005 May 21;330(7501):1179. doi: 10.1136/bmj.38446.498542.8F. Epub 2005 May 13. PMID: 15894554 ; PMCID: PMC558012 .
- Montori VM, Wilczynski NL, Morgan D, Haynes RB; Hedges Team. Optimal search strategies for retrieving systematic reviews from Medline: analytical survey. BMJ. 2005 Jan 8;330(7482):68. doi: 10.1136/bmj.38336.804167.47. Epub 2004 Dec 24. PMID: 15619601 ; PMCID: PMC543864 .
- Wilczynski NL, Haynes RB, Lavis JN, Ramkissoonsingh R, Arnold-Oatley AE; HSR Hedges team. Optimal search strategies for detecting health services research studies in MEDLINE. CMAJ. 2004 Nov 9;171(10):1179-85. doi: 10.1503/cmaj.1040512. PMID: 15534310 ; PMCID: PMC524948 .
- Wilczynski NL, Haynes RB; Hedges Team. Developing optimal search strategies for detecting clinically sound prognostic studies in MEDLINE: an analytic survey. BMC Med. 2004 Jun 9;2:23. doi: 10.1186/1741-7015-2-23. PMID: 15189561 ; PMCID: PMC441418 .
- Haynes RB, Wilczynski NL. Optimal search strategies for retrieving scientifically strong studies of diagnosis from Medline: analytical survey. BMJ. 2004 May 1;328(7447):1040. doi: 10.1136/bmj.38068.557998.EE. Epub 2004 Apr 8. PMID: 15073027 ; PMCID: PMC403841 .
- Bhandari M, Montori VM, Devereaux PJ, Wilczynski NL, Morgan D, Haynes RB; Hedges Team. Doubling the impact: publication of systematic review articles in orthopaedic journals. J Bone Joint Surg Am. 2004 May;86(5):1012-6. PMID: 15118046 .
- Wong SS, Wilczynski NL, Haynes RB; Hedges Team. Developing optimal search strategies for detecting clinically relevant qualitative studies in MEDLINE. Stud Health Technol Inform. 2004;107(Pt 1):311-6. PMID: 15360825 .
- Montori VM, Wilczynski NL, Morgan D, Haynes RB; Hedges Team. Systematic reviews: a cross-sectional study of location and citation counts. BMC Med. 2003 Nov 24;1:2. doi: 10.1186/1741-7015-1-2. PMID: 14633274 ; PMCID: PMC281591 .
- Wong SS, Wilczynski NL, Haynes RB, Ramkissoonsingh R; Hedges Team. Developing optimal search strategies for detecting sound clinical prediction studies in MEDLINE. AMIA Annu Symp Proc. 2003;2003:728-32. PMID: 14728269 ; PMCID: PMC1479983 .
- Wilczynski NL, Haynes RB; Hedges Team. Developing optimal search strategies for detecting clinically sound causation studies in MEDLINE. AMIA Annu Symp Proc. 2003;2003:719-23. PMID: 14728267 ; PMCID: PMC1480286 .
- Wilczynski NL, McKibbon KA, Haynes RB. Enhancing retrieval of best evidence for health care from bibliographic databases: calibration of the hand search of the literature. Stud Health Technol Inform. 2001;84(Pt 1):390-3. PMID: 11604770 .
- Haynes RB, Wilczynski N, McKibbon KA, Walker CJ, Sinclair JC. Developing optimal search strategies for detecting clinically sound studies in MEDLINE. J Am Med Inform Assoc. 1994 Nov-Dec;1(6):447-58. doi: 10.1136/jamia.1994.95153434. PMID: 7850570 ; PMCID: PMC116228 .
Medical genetics search filters
The medical genetics searches were developed in conjunction with the staff of GeneReviews: Genetic Disease Online Reviews at GeneTests, University of Washington, Seattle.
The neighbors of a document are those documents in the database that are the most similar to it. The similarity between documents is measured by the words they have in common, with some adjustment for document lengths. To carry out such a program, one must first define what a word is. For us, a word is basically an unbroken string of letters and numerals with at least one letter of the alphabet in it. Words end at hyphens, spaces, new lines, and punctuation. The 132 common, but uninformative, words (also known as stopwords) are eliminated from processing at this stage. Next, a limited amount of stemming of words is done, but no thesaurus is used in processing. Words from the abstract of a document are classified as text words. Words from titles are also classified as text words, but words from titles are added in a second time to give them a small advantage in the local weighting scheme. MeSH terms are placed in a third category, and a MeSH term with a subheading qualifier is entered twice, once without the qualifier and once with it. If a MeSH term is starred (indicating a major concept in a document), the star is ignored. These three categories of words (or phrases in the case of MeSH) comprise the representation of a document. No other fields, such as Author or Journal, enter into the calculations.
Having obtained the set of terms that represent each document, the next step is to recognize that not all words are of equal value. Each time a word is used, it is assigned a numerical weight. This numerical weight is based on information that the computer can obtain by automatic processing. Automatic processing is important because the number of different terms that have to be assigned weights is close to two million for this system. The weight or value of a term is dependent on three types of information: 1) the number of different documents in the database that contain the term; 2) the number of times the term occurs in a particular document; and 3) the number of term occurrences in the document. The first of these pieces of information is used to produce a number called the global weight of the term. The global weight is used in weighting the term throughout the database. The second and third pieces of information pertain only to a particular document and are used to produce a number called the local weight of the term in that specific document. When a word occurs in two documents, its weight is computed as the product of the global weight times the two local weights (one pertaining to each of the documents).
The global weight of a term is greater for the less frequent terms. This is reasonable because the presence of a term that occurred in most of the documents would really tell one very little about a document. On the other hand, a term that occurred in only 100 documents of one million would be very helpful in limiting the set of documents of interest. A word that occurred in only 10 documents is likely to be even more informative and will receive an even higher weight.
The local weight of a term is the measure of its importance in a particular document. Generally, the more frequent a term is within a document, the more important it is in representing the content of that document. However, this relationship is saturating, i.e., as the frequency continues to go up, the importance of the word increases less rapidly and finally comes to a finite limit. In addition, we do not want a longer document to be considered more important just because it is longer; therefore, a length correction is applied. This local weight computation is based on the Poisson distribution and the formula can be found in Lin J and Wilbur WJ .
The similarity between two documents is computed by adding up the weights (local wt1 × local wt2 × global wt) of all of the terms the two documents have in common. This provides an indication of how related two documents are. The resultant score is an example of a vector score. Vector scoring was originated by Gerard Salton and has a long history in text retrieval. The interested reader is referred to Salton, Automatic Text Processing, Reading, MA: Addison-Wesley, 1989 for further information on this topic. Our approach differs from other approaches in the way we calculate the local weights for the individual terms. Once the similarity score of a document in relation to each of the other documents in the database has been computed, that document's neighbors are identified as the most similar (highest scoring) documents found. These closely related documents are pre-computed for each document in PubMed so that when you select Similar articles, the system has only to retrieve this list. This enables a fast response time for such queries.
PubMed journals
- Uncompressed
- UNIX Compress
PubMed and NCBI molecular biology database journals
NCBI Literature Resources
MeSH PMC Bookshelf Disclaimer
The PubMed wordmark and PubMed logo are registered trademarks of the U.S. Department of Health and Human Services (HHS). Unauthorized use of these marks is strictly prohibited.

An official website of the United States government
Here’s how you know
Official websites use .gov A .gov website belongs to an official government organization in the United States.
Secure .gov websites use HTTPS A lock ( Lock A locked padlock ) or https:// means you’ve safely connected to the .gov website. Share sensitive information only on official, secure websites.

MEDLINE is the National Library of Medicine's (NLM) premier bibliographic database that contains references to journal articles in life sciences, with a concentration on biomedicine. See the MEDLINE Overview page for more information about MEDLINE.
MEDLINE content is searchable via PubMed and constitutes the primary component of PubMed, a literature database developed and maintained by the NLM National Center for Biotechnology Information (NCBI).
Last Reviewed: February 5, 2024
Free Medical Journals
Direct Link
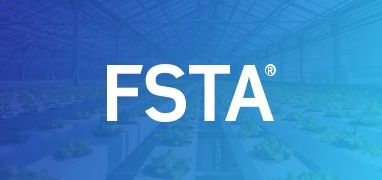
Start your free trial
Arrange a trial for your organisation and discover why FSTA is the leading database for reliable research on the sciences of food and health.
REQUEST A FREE TRIAL
- Research Skills Blog
5 free and legal ways to get the full text of research articles
By Carol Hollier on 07-Apr-2021 13:23:17
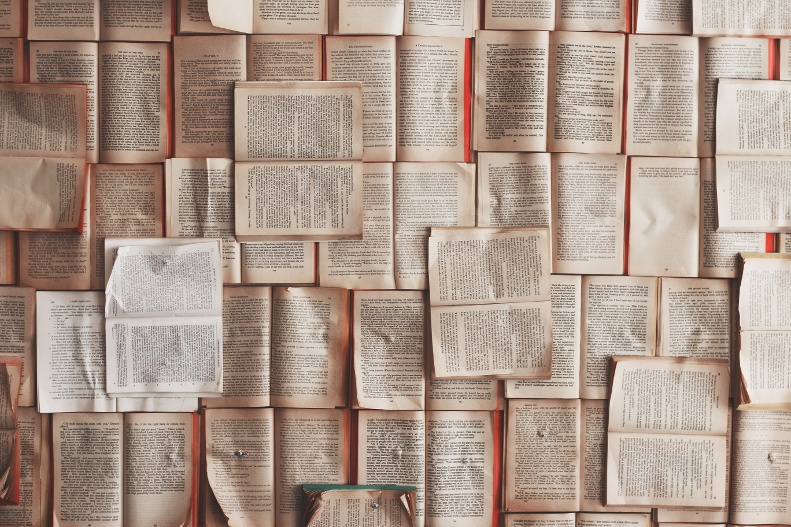
1. Use your library if you have one !
If you are affiliated with a university, you probably have free library access to the full text of millions of research articles. The library will have subscribed to these journals on your behalf. The smartest thing you can do for accessing research articles is familiarize yourself with your own library.
- If you search a database your library will link from the records to the full text if they have it—all you need to do is click through the links.
- When they do not have a copy of an article, a university library can get it for you from another library. This inter-library loan service is usually free to users.
- Your library might use a browser extension like Lean Library or LibKey Nomad to link you to the library subscription or open access full text from wherever you are on the internet.
- Google Scholar lets you configure your account to get links straight to your library’s subscription copy of an article. But remember--side-by-side to library subscriptions for legitimate research, Google Scholar includes links to articles published in predatory and unreliable journals that would be unwise to credit in your own work. Learn more about predatory journals.
If you are not affiliated with a university library, there are still ways you can successfully—and legally—get the full text of research articles.
2. Open Access browser extensions
More and more research is published Open Access as governments around the world are mandating that research paid for by taxpayer money be freely available to those taxpayers.
Browser extensions have been created to make it easy to spot when the full text of an article is free. Some of the best are CORE Discovery , Unpaywall and Open Access Button .
Learn more about difference between discovery and access and why it matters for good research: Where to search - Best Practice for Literature Searching - LibGuides at IFIS
3. Google Scholar
You can search the article title inside quotation marks on Google Scholar to see if a link to a copy of the article appears. If it does, be sure to pay attention to what version of the article you are linking to, to be sure you are getting what you think you’re getting. These links can lead to an article's published version of record, a manuscript version, or to a thesis or conference proceeding with the same title and author as the article you expected to find.
4. Researcher platforms
A Google Scholar search might lead you to a researcher platform like Academia.edu or ResearchGate . There, if you set up an account, you can sometimes download or request a copy of the text. Again, pay attention to which version of the text you get!
5. Write to the author
If you can’t get a copy by other means, you can write to an article’s corresponding author and (politely!) ask them to send you a copy. Their contact information, usually an email address, will be listed in the information you find about the article, either in a database record for the article or on the publishing journal’s page for it. Many authors are happy to share a copy of their work.
Three bonus ways that might work depending on where you live:
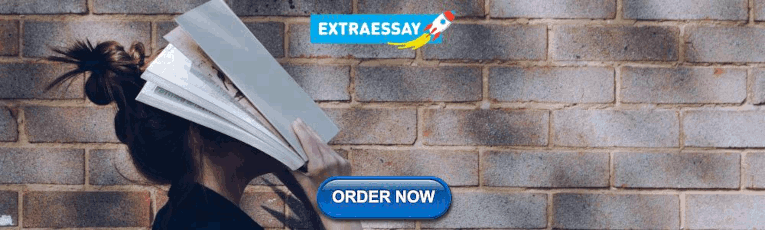
1. A nearby university library might offer access to articles even if you do not work or study there.
Members of the public are sometimes allowed access to university journal subscriptions through visitor access or a walk-in user service. You usually need to use the collections from a dedicated computer terminal located in a library and may need to make an appointment before you go. Do your research before showing up to make sure you bring the correct documents and equipment (like a flash drive) along.
2. Try your public library
In some countries, public libraries partner with publishers to give the public access to research articles. In the UK, for instance, many public libraries participate in the Access to Research scheme, which gives members of the public on-site access to over 30 million academic articles. Contact your local public library to learn what is available to you.
3. Research4Life
In other countries, your institution might have access to a massive collection of research articles and databases through the publisher/library/United Nations agency initiative Research4Life . Check to see if you already have access, and if not, if your institution might be eligible to join. Membership is only available on an organizational or institutional level.
Remember —even though you now have a lot of strategies for finding the full text of articles, research should never be led by the articles you can access most easily.
Good research is driven by first figuring out what articles are most relevant to your question and then getting the full text of what you need. One of the best ways to do this is to use a good discipline-specific database, like FSTA for the sciences of food and health.
Learn more about difference between discovery and access and why it matters for good research:

Photo by Patrick Tomasso on Unsplash
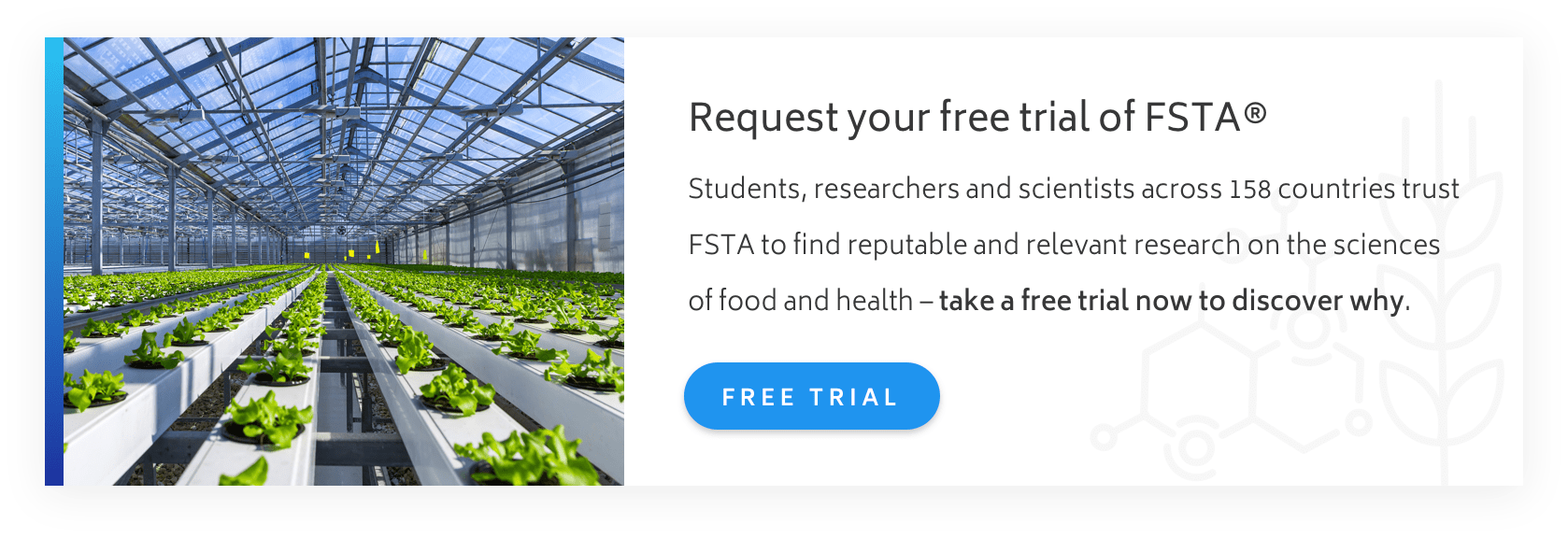
- FSTA - Food Science & Technology Abstracts
- IFIS Collections
- Resources Hub
- Diversity Statement
- Sustainability Commitment
- Company news
- Frequently Asked Questions
- Privacy Policy
- Terms of Use for IFIS Collections
Ground Floor, 115 Wharfedale Road, Winnersh Triangle, Wokingham, Berkshire RG41 5RB
Get in touch with IFIS
© International Food Information Service (IFIS Publishing) operating as IFIS – All Rights Reserved | Charity Reg. No. 1068176 | Limited Company No. 3507902 | Designed by Blend
Featured Clinical Reviews
- Screening for Atrial Fibrillation: US Preventive Services Task Force Recommendation Statement JAMA Recommendation Statement January 25, 2022
- Evaluating the Patient With a Pulmonary Nodule: A Review JAMA Review January 18, 2022
Select Your Interests
Customize your JAMA Network experience by selecting one or more topics from the list below.
- Academic Medicine
- Acid Base, Electrolytes, Fluids
- Allergy and Clinical Immunology
- American Indian or Alaska Natives
- Anesthesiology
- Anticoagulation
- Art and Images in Psychiatry
- Artificial Intelligence
- Assisted Reproduction
- Bleeding and Transfusion
- Caring for the Critically Ill Patient
- Challenges in Clinical Electrocardiography
- Climate and Health
- Climate Change
- Clinical Challenge
- Clinical Decision Support
- Clinical Implications of Basic Neuroscience
- Clinical Pharmacy and Pharmacology
- Complementary and Alternative Medicine
- Consensus Statements
- Coronavirus (COVID-19)
- Critical Care Medicine
- Cultural Competency
- Dental Medicine
- Dermatology
- Diabetes and Endocrinology
- Diagnostic Test Interpretation
- Drug Development
- Electronic Health Records
- Emergency Medicine
- End of Life, Hospice, Palliative Care
- Environmental Health
- Equity, Diversity, and Inclusion
- Facial Plastic Surgery
- Gastroenterology and Hepatology
- Genetics and Genomics
- Genomics and Precision Health
- Global Health
- Guide to Statistics and Methods
- Hair Disorders
- Health Care Delivery Models
- Health Care Economics, Insurance, Payment
- Health Care Quality
- Health Care Reform
- Health Care Safety
- Health Care Workforce
- Health Disparities
- Health Inequities
- Health Policy
- Health Systems Science
- History of Medicine
- Hypertension
- Images in Neurology
- Implementation Science
- Infectious Diseases
- Innovations in Health Care Delivery
- JAMA Infographic
- Law and Medicine
- Leading Change
- Less is More
- LGBTQIA Medicine
- Lifestyle Behaviors
- Medical Coding
- Medical Devices and Equipment
- Medical Education
- Medical Education and Training
- Medical Journals and Publishing
- Mobile Health and Telemedicine
- Narrative Medicine
- Neuroscience and Psychiatry
- Notable Notes
- Nutrition, Obesity, Exercise
- Obstetrics and Gynecology
- Occupational Health
- Ophthalmology
- Orthopedics
- Otolaryngology
- Pain Medicine
- Palliative Care
- Pathology and Laboratory Medicine
- Patient Care
- Patient Information
- Performance Improvement
- Performance Measures
- Perioperative Care and Consultation
- Pharmacoeconomics
- Pharmacoepidemiology
- Pharmacogenetics
- Pharmacy and Clinical Pharmacology
- Physical Medicine and Rehabilitation
- Physical Therapy
- Physician Leadership
- Population Health
- Primary Care
- Professional Well-being
- Professionalism
- Psychiatry and Behavioral Health
- Public Health
- Pulmonary Medicine
- Regulatory Agencies
- Reproductive Health
- Research, Methods, Statistics
- Resuscitation
- Rheumatology
- Risk Management
- Scientific Discovery and the Future of Medicine
- Shared Decision Making and Communication
- Sleep Medicine
- Sports Medicine
- Stem Cell Transplantation
- Substance Use and Addiction Medicine
- Surgical Innovation
- Surgical Pearls
- Teachable Moment
- Technology and Finance
- The Art of JAMA
- The Arts and Medicine
- The Rational Clinical Examination
- Tobacco and e-Cigarettes
- Translational Medicine
- Trauma and Injury
- Treatment Adherence
- Ultrasonography
- Users' Guide to the Medical Literature
- Vaccination
- Venous Thromboembolism
- Veterans Health
- Women's Health
- Workflow and Process
- Wound Care, Infection, Healing
- Download PDF
- Share X Facebook Email LinkedIn
- Permissions
False Health Claims Abound, but Physicians Are Still the Most Trusted Source for Health Information
- 1 Senior Staff Writer, Medical News & Perspectives, JAMA
- 2 Manager and Senior Medical Illustrator, Editorial Graphics, JAMA and JAMA Network
- Editor's Note Introducing JAMA Data Brief Jennifer Abbasi, BA; Karen Bucher, MA, CMI; Andy Rekito, MS; Stephen Schenkel, MD, MPP; Annette Flanagin, RN, MA JAMA
The COVID-19 pandemic spotlighted a growing problem: the pervasiveness of false claims about health. To better understand how people in the US view health inaccuracies and learn about them through media use, KFF (formerly Kaiser Family Foundation), a nonprofit organization focused on health policy, tracked exposure to and beliefs about certain claims. For their Health Misinformation Tracking Pilot Poll , conducted last year, public opinion researchers at KFF asked a nationally representative sample of 2007 Black, Hispanic, and White adults about inaccurate information pertaining to COVID-19, gun violence, and reproductive health. The survey team also asked where people get their news and which sources of health information they trust.
The poll showed that trust in health care professionals crossed party lines: 95% of both Democrats and Republicans reported trusting their personal physicians to provide the right recommendations about health issues. “Doctors are particularly well positioned to be the messengers when it comes to health recommendations that people really trust,” Lunna Lopes, MSc, the lead author of the report and a senior survey analyst at KFF, told JAMA Medical News in an interview.
Most people have a fair amount of trust in the government to provide accurate information about health issues, but trust varies across political party lines. For example, 87% of Democrats reported trust in the Centers for Disease Control and Prevention compared with 49% of Republicans. State and local public health officials were trusted by 74% of Democrats and 58% of Republicans.
Overall, 96% of participants reported that they’ve heard at least 1 of the 10 inaccurate health claims listed on KFF’s survey—that measles-mumps-rubella (MME) vaccines cause autism, for example, or that ivermectin is effective against COVID-19. And while exposure to health misinformation was rampant, the percentage of people who have heard false claims and believe they’re probably or definitely true ranged from 14% to 35%. “A key takeaway that we got from the data is that people are hearing misinformation, but not many are convinced that it’s true,” Lopes said.
The results suggest that many people fall into what KFF refers to as the “malleable middle”: people who hear or read incorrect claims but are uncertain whether the information is accurate. “They say the claims are either probably true or probably false, but they’re not convinced in either direction,” Lopes explained. She emphasized that health communication needs to be more customized to reach this group. “They’re in the middle, but that doesn’t mean that they will respond to the same types of messages or interventions.”
Another key finding was that many individuals are concerned about the spread of false health claims and want more done to limit it. People overwhelmingly reported that inaccurate information about health is an important issue. In fact, 86% of respondents said false and inaccurate information in general is a major problem, and 74% reported that false claims about health specifically are a major problem.
Most respondents—regardless of their demographics or partisanship—also believe that not enough is being done to limit false claims about health. Among those polled, 78% and 68%, respectively, reported that Congress and President Joe Biden aren’t doing enough, and about 7 in 10 reported that the US news media and social media platforms need to step up.
But the idea of false claims varies from person to person. “While people agree there’s a problem, there isn’t necessarily agreement on what constitutes health misinformation, so that’s what makes addressing the problem particularly challenging,” Lopes added.
Many people reported high social media use, yet few have trust in health information published on such platforms. YouTube and Facebook were the most popular sites: 65% and 63% of respondents reported visiting the platforms every week, respectively. But trust in these sites was considerably low. Only 8% of respondents said they would have a lot of trust in health information on YouTube, and 5% would trust health information on Facebook. Twitter, now known as X, was used weekly by 21% of respondents, although only 4% would trust health information on the site. People who frequently used social media to search for health information were more likely to believe false claims about health.
When asked about traditional news sources, most respondents said they rely on local television, national networks, and digital aggregators. Overall, 62% reported regularly watching local television news, and 56% often tune into national networks like ABC, CBS, or NBC. Digital aggregators like Apple, Google, or Yahoo news followed closely behind at 55%.
But fewer than 3 in 10 adults reported having a lot of trust in each of the media sources listed in the survey. About a fourth of respondents said they would have a lot of trust in information about health issues reported by local television news or national networks, the most trusted sources.
“You’re going to have at least a little bit of trust in the news source that you’re following, but there is some skepticism there,” Lopes said.
The takeaway, Lopes said, is that although exposure to inaccurate health information remains high, so does public doubt surrounding such claims. “Most adults express uncertainty and may be open to additional information from trusted messengers, especially from their own doctors.”
Published Online: April 26, 2024. doi:10.1001/jama.2024.6837
Conflict of Interest Disclosures: Ms Lopes reported that some support for KFF’s Health Misinformation Tracking Poll Pilot was provided by the Robert Wood Johnson Foundation.
See More About
Suran M , Bucher K. False Health Claims Abound, but Physicians Are Still the Most Trusted Source for Health Information. JAMA. Published online April 26, 2024. doi:10.1001/jama.2024.6837
Manage citations:
© 2024
Artificial Intelligence Resource Center
Cardiology in JAMA : Read the Latest
Browse and subscribe to JAMA Network podcasts!
Others Also Liked
- Register for email alerts with links to free full-text articles
- Access PDFs of free articles
- Manage your interests
- Save searches and receive search alerts
- Open access
- Published: 18 April 2024
Research ethics and artificial intelligence for global health: perspectives from the global forum on bioethics in research
- James Shaw 1 , 13 ,
- Joseph Ali 2 , 3 ,
- Caesar A. Atuire 4 , 5 ,
- Phaik Yeong Cheah 6 ,
- Armando Guio Español 7 ,
- Judy Wawira Gichoya 8 ,
- Adrienne Hunt 9 ,
- Daudi Jjingo 10 ,
- Katherine Littler 9 ,
- Daniela Paolotti 11 &
- Effy Vayena 12
BMC Medical Ethics volume 25 , Article number: 46 ( 2024 ) Cite this article
973 Accesses
6 Altmetric
Metrics details
The ethical governance of Artificial Intelligence (AI) in health care and public health continues to be an urgent issue for attention in policy, research, and practice. In this paper we report on central themes related to challenges and strategies for promoting ethics in research involving AI in global health, arising from the Global Forum on Bioethics in Research (GFBR), held in Cape Town, South Africa in November 2022.
The GFBR is an annual meeting organized by the World Health Organization and supported by the Wellcome Trust, the US National Institutes of Health, the UK Medical Research Council (MRC) and the South African MRC. The forum aims to bring together ethicists, researchers, policymakers, research ethics committee members and other actors to engage with challenges and opportunities specifically related to research ethics. In 2022 the focus of the GFBR was “Ethics of AI in Global Health Research”. The forum consisted of 6 case study presentations, 16 governance presentations, and a series of small group and large group discussions. A total of 87 participants attended the forum from 31 countries around the world, representing disciplines of bioethics, AI, health policy, health professional practice, research funding, and bioinformatics. In this paper, we highlight central insights arising from GFBR 2022.
We describe the significance of four thematic insights arising from the forum: (1) Appropriateness of building AI, (2) Transferability of AI systems, (3) Accountability for AI decision-making and outcomes, and (4) Individual consent. We then describe eight recommendations for governance leaders to enhance the ethical governance of AI in global health research, addressing issues such as AI impact assessments, environmental values, and fair partnerships.
Conclusions
The 2022 Global Forum on Bioethics in Research illustrated several innovations in ethical governance of AI for global health research, as well as several areas in need of urgent attention internationally. This summary is intended to inform international and domestic efforts to strengthen research ethics and support the evolution of governance leadership to meet the demands of AI in global health research.
Peer Review reports
Introduction
The ethical governance of Artificial Intelligence (AI) in health care and public health continues to be an urgent issue for attention in policy, research, and practice [ 1 , 2 , 3 ]. Beyond the growing number of AI applications being implemented in health care, capabilities of AI models such as Large Language Models (LLMs) expand the potential reach and significance of AI technologies across health-related fields [ 4 , 5 ]. Discussion about effective, ethical governance of AI technologies has spanned a range of governance approaches, including government regulation, organizational decision-making, professional self-regulation, and research ethics review [ 6 , 7 , 8 ]. In this paper, we report on central themes related to challenges and strategies for promoting ethics in research involving AI in global health research, arising from the Global Forum on Bioethics in Research (GFBR), held in Cape Town, South Africa in November 2022. Although applications of AI for research, health care, and public health are diverse and advancing rapidly, the insights generated at the forum remain highly relevant from a global health perspective. After summarizing important context for work in this domain, we highlight categories of ethical issues emphasized at the forum for attention from a research ethics perspective internationally. We then outline strategies proposed for research, innovation, and governance to support more ethical AI for global health.
In this paper, we adopt the definition of AI systems provided by the Organization for Economic Cooperation and Development (OECD) as our starting point. Their definition states that an AI system is “a machine-based system that can, for a given set of human-defined objectives, make predictions, recommendations, or decisions influencing real or virtual environments. AI systems are designed to operate with varying levels of autonomy” [ 9 ]. The conceptualization of an algorithm as helping to constitute an AI system, along with hardware, other elements of software, and a particular context of use, illustrates the wide variety of ways in which AI can be applied. We have found it useful to differentiate applications of AI in research as those classified as “AI systems for discovery” and “AI systems for intervention”. An AI system for discovery is one that is intended to generate new knowledge, for example in drug discovery or public health research in which researchers are seeking potential targets for intervention, innovation, or further research. An AI system for intervention is one that directly contributes to enacting an intervention in a particular context, for example informing decision-making at the point of care or assisting with accuracy in a surgical procedure.
The mandate of the GFBR is to take a broad view of what constitutes research and its regulation in global health, with special attention to bioethics in Low- and Middle- Income Countries. AI as a group of technologies demands such a broad view. AI development for health occurs in a variety of environments, including universities and academic health sciences centers where research ethics review remains an important element of the governance of science and innovation internationally [ 10 , 11 ]. In these settings, research ethics committees (RECs; also known by different names such as Institutional Review Boards or IRBs) make decisions about the ethical appropriateness of projects proposed by researchers and other institutional members, ultimately determining whether a given project is allowed to proceed on ethical grounds [ 12 ].
However, research involving AI for health also takes place in large corporations and smaller scale start-ups, which in some jurisdictions fall outside the scope of research ethics regulation. In the domain of AI, the question of what constitutes research also becomes blurred. For example, is the development of an algorithm itself considered a part of the research process? Or only when that algorithm is tested under the formal constraints of a systematic research methodology? In this paper we take an inclusive view, in which AI development is included in the definition of research activity and within scope for our inquiry, regardless of the setting in which it takes place. This broad perspective characterizes the approach to “research ethics” we take in this paper, extending beyond the work of RECs to include the ethical analysis of the wide range of activities that constitute research as the generation of new knowledge and intervention in the world.
Ethical governance of AI in global health
The ethical governance of AI for global health has been widely discussed in recent years. The World Health Organization (WHO) released its guidelines on ethics and governance of AI for health in 2021, endorsing a set of six ethical principles and exploring the relevance of those principles through a variety of use cases. The WHO guidelines also provided an overview of AI governance, defining governance as covering “a range of steering and rule-making functions of governments and other decision-makers, including international health agencies, for the achievement of national health policy objectives conducive to universal health coverage.” (p. 81) The report usefully provided a series of recommendations related to governance of seven domains pertaining to AI for health: data, benefit sharing, the private sector, the public sector, regulation, policy observatories/model legislation, and global governance. The report acknowledges that much work is yet to be done to advance international cooperation on AI governance, especially related to prioritizing voices from Low- and Middle-Income Countries (LMICs) in global dialogue.
One important point emphasized in the WHO report that reinforces the broader literature on global governance of AI is the distribution of responsibility across a wide range of actors in the AI ecosystem. This is especially important to highlight when focused on research for global health, which is specifically about work that transcends national borders. Alami et al. (2020) discussed the unique risks raised by AI research in global health, ranging from the unavailability of data in many LMICs required to train locally relevant AI models to the capacity of health systems to absorb new AI technologies that demand the use of resources from elsewhere in the system. These observations illustrate the need to identify the unique issues posed by AI research for global health specifically, and the strategies that can be employed by all those implicated in AI governance to promote ethically responsible use of AI in global health research.
RECs and the regulation of research involving AI
RECs represent an important element of the governance of AI for global health research, and thus warrant further commentary as background to our paper. Despite the importance of RECs, foundational questions have been raised about their capabilities to accurately understand and address ethical issues raised by studies involving AI. Rahimzadeh et al. (2023) outlined how RECs in the United States are under-prepared to align with recent federal policy requiring that RECs review data sharing and management plans with attention to the unique ethical issues raised in AI research for health [ 13 ]. Similar research in South Africa identified variability in understanding of existing regulations and ethical issues associated with health-related big data sharing and management among research ethics committee members [ 14 , 15 ]. The effort to address harms accruing to groups or communities as opposed to individuals whose data are included in AI research has also been identified as a unique challenge for RECs [ 16 , 17 ]. Doerr and Meeder (2022) suggested that current regulatory frameworks for research ethics might actually prevent RECs from adequately addressing such issues, as they are deemed out of scope of REC review [ 16 ]. Furthermore, research in the United Kingdom and Canada has suggested that researchers using AI methods for health tend to distinguish between ethical issues and social impact of their research, adopting an overly narrow view of what constitutes ethical issues in their work [ 18 ].
The challenges for RECs in adequately addressing ethical issues in AI research for health care and public health exceed a straightforward survey of ethical considerations. As Ferretti et al. (2021) contend, some capabilities of RECs adequately cover certain issues in AI-based health research, such as the common occurrence of conflicts of interest where researchers who accept funds from commercial technology providers are implicitly incentivized to produce results that align with commercial interests [ 12 ]. However, some features of REC review require reform to adequately meet ethical needs. Ferretti et al. outlined weaknesses of RECs that are longstanding and those that are novel to AI-related projects, proposing a series of directions for development that are regulatory, procedural, and complementary to REC functionality. The work required on a global scale to update the REC function in response to the demands of research involving AI is substantial.
These issues take greater urgency in the context of global health [ 19 ]. Teixeira da Silva (2022) described the global practice of “ethics dumping”, where researchers from high income countries bring ethically contentious practices to RECs in low-income countries as a strategy to gain approval and move projects forward [ 20 ]. Although not yet systematically documented in AI research for health, risk of ethics dumping in AI research is high. Evidence is already emerging of practices of “health data colonialism”, in which AI researchers and developers from large organizations in high-income countries acquire data to build algorithms in LMICs to avoid stricter regulations [ 21 ]. This specific practice is part of a larger collection of practices that characterize health data colonialism, involving the broader exploitation of data and the populations they represent primarily for commercial gain [ 21 , 22 ]. As an additional complication, AI algorithms trained on data from high-income contexts are unlikely to apply in straightforward ways to LMIC settings [ 21 , 23 ]. In the context of global health, there is widespread acknowledgement about the need to not only enhance the knowledge base of REC members about AI-based methods internationally, but to acknowledge the broader shifts required to encourage their capabilities to more fully address these and other ethical issues associated with AI research for health [ 8 ].
Although RECs are an important part of the story of the ethical governance of AI for global health research, they are not the only part. The responsibilities of supra-national entities such as the World Health Organization, national governments, organizational leaders, commercial AI technology providers, health care professionals, and other groups continue to be worked out internationally. In this context of ongoing work, examining issues that demand attention and strategies to address them remains an urgent and valuable task.
The GFBR is an annual meeting organized by the World Health Organization and supported by the Wellcome Trust, the US National Institutes of Health, the UK Medical Research Council (MRC) and the South African MRC. The forum aims to bring together ethicists, researchers, policymakers, REC members and other actors to engage with challenges and opportunities specifically related to research ethics. Each year the GFBR meeting includes a series of case studies and keynotes presented in plenary format to an audience of approximately 100 people who have applied and been competitively selected to attend, along with small-group breakout discussions to advance thinking on related issues. The specific topic of the forum changes each year, with past topics including ethical issues in research with people living with mental health conditions (2021), genome editing (2019), and biobanking/data sharing (2018). The forum is intended to remain grounded in the practical challenges of engaging in research ethics, with special interest in low resource settings from a global health perspective. A post-meeting fellowship scheme is open to all LMIC participants, providing a unique opportunity to apply for funding to further explore and address the ethical challenges that are identified during the meeting.
In 2022, the focus of the GFBR was “Ethics of AI in Global Health Research”. The forum consisted of 6 case study presentations (both short and long form) reporting on specific initiatives related to research ethics and AI for health, and 16 governance presentations (both short and long form) reporting on actual approaches to governing AI in different country settings. A keynote presentation from Professor Effy Vayena addressed the topic of the broader context for AI ethics in a rapidly evolving field. A total of 87 participants attended the forum from 31 countries around the world, representing disciplines of bioethics, AI, health policy, health professional practice, research funding, and bioinformatics. The 2-day forum addressed a wide range of themes. The conference report provides a detailed overview of each of the specific topics addressed while a policy paper outlines the cross-cutting themes (both documents are available at the GFBR website: https://www.gfbr.global/past-meetings/16th-forum-cape-town-south-africa-29-30-november-2022/ ). As opposed to providing a detailed summary in this paper, we aim to briefly highlight central issues raised, solutions proposed, and the challenges facing the research ethics community in the years to come.
In this way, our primary aim in this paper is to present a synthesis of the challenges and opportunities raised at the GFBR meeting and in the planning process, followed by our reflections as a group of authors on their significance for governance leaders in the coming years. We acknowledge that the views represented at the meeting and in our results are a partial representation of the universe of views on this topic; however, the GFBR leadership invested a great deal of resources in convening a deeply diverse and thoughtful group of researchers and practitioners working on themes of bioethics related to AI for global health including those based in LMICs. We contend that it remains rare to convene such a strong group for an extended time and believe that many of the challenges and opportunities raised demand attention for more ethical futures of AI for health. Nonetheless, our results are primarily descriptive and are thus not explicitly grounded in a normative argument. We make effort in the Discussion section to contextualize our results by describing their significance and connecting them to broader efforts to reform global health research and practice.
Uniquely important ethical issues for AI in global health research
Presentations and group dialogue over the course of the forum raised several issues for consideration, and here we describe four overarching themes for the ethical governance of AI in global health research. Brief descriptions of each issue can be found in Table 1 . Reports referred to throughout the paper are available at the GFBR website provided above.
The first overarching thematic issue relates to the appropriateness of building AI technologies in response to health-related challenges in the first place. Case study presentations referred to initiatives where AI technologies were highly appropriate, such as in ear shape biometric identification to more accurately link electronic health care records to individual patients in Zambia (Alinani Simukanga). Although important ethical issues were raised with respect to privacy, trust, and community engagement in this initiative, the AI-based solution was appropriately matched to the challenge of accurately linking electronic records to specific patient identities. In contrast, forum participants raised questions about the appropriateness of an initiative using AI to improve the quality of handwashing practices in an acute care hospital in India (Niyoshi Shah), which led to gaming the algorithm. Overall, participants acknowledged the dangers of techno-solutionism, in which AI researchers and developers treat AI technologies as the most obvious solutions to problems that in actuality demand much more complex strategies to address [ 24 ]. However, forum participants agreed that RECs in different contexts have differing degrees of power to raise issues of the appropriateness of an AI-based intervention.
The second overarching thematic issue related to whether and how AI-based systems transfer from one national health context to another. One central issue raised by a number of case study presentations related to the challenges of validating an algorithm with data collected in a local environment. For example, one case study presentation described a project that would involve the collection of personally identifiable data for sensitive group identities, such as tribe, clan, or religion, in the jurisdictions involved (South Africa, Nigeria, Tanzania, Uganda and the US; Gakii Masunga). Doing so would enable the team to ensure that those groups were adequately represented in the dataset to ensure the resulting algorithm was not biased against specific community groups when deployed in that context. However, some members of these communities might desire to be represented in the dataset, whereas others might not, illustrating the need to balance autonomy and inclusivity. It was also widely recognized that collecting these data is an immense challenge, particularly when historically oppressive practices have led to a low-trust environment for international organizations and the technologies they produce. It is important to note that in some countries such as South Africa and Rwanda, it is illegal to collect information such as race and tribal identities, re-emphasizing the importance for cultural awareness and avoiding “one size fits all” solutions.
The third overarching thematic issue is related to understanding accountabilities for both the impacts of AI technologies and governance decision-making regarding their use. Where global health research involving AI leads to longer-term harms that might fall outside the usual scope of issues considered by a REC, who is to be held accountable, and how? This question was raised as one that requires much further attention, with law being mixed internationally regarding the mechanisms available to hold researchers, innovators, and their institutions accountable over the longer term. However, it was recognized in breakout group discussion that many jurisdictions are developing strong data protection regimes related specifically to international collaboration for research involving health data. For example, Kenya’s Data Protection Act requires that any internationally funded projects have a local principal investigator who will hold accountability for how data are shared and used [ 25 ]. The issue of research partnerships with commercial entities was raised by many participants in the context of accountability, pointing toward the urgent need for clear principles related to strategies for engagement with commercial technology companies in global health research.
The fourth and final overarching thematic issue raised here is that of consent. The issue of consent was framed by the widely shared recognition that models of individual, explicit consent might not produce a supportive environment for AI innovation that relies on the secondary uses of health-related datasets to build AI algorithms. Given this recognition, approaches such as community oversight of health data uses were suggested as a potential solution. However, the details of implementing such community oversight mechanisms require much further attention, particularly given the unique perspectives on health data in different country settings in global health research. Furthermore, some uses of health data do continue to require consent. One case study of South Africa, Nigeria, Kenya, Ethiopia and Uganda suggested that when health data are shared across borders, individual consent remains necessary when data is transferred from certain countries (Nezerith Cengiz). Broader clarity is necessary to support the ethical governance of health data uses for AI in global health research.
Recommendations for ethical governance of AI in global health research
Dialogue at the forum led to a range of suggestions for promoting ethical conduct of AI research for global health, related to the various roles of actors involved in the governance of AI research broadly defined. The strategies are written for actors we refer to as “governance leaders”, those people distributed throughout the AI for global health research ecosystem who are responsible for ensuring the ethical and socially responsible conduct of global health research involving AI (including researchers themselves). These include RECs, government regulators, health care leaders, health professionals, corporate social accountability officers, and others. Enacting these strategies would bolster the ethical governance of AI for global health more generally, enabling multiple actors to fulfill their roles related to governing research and development activities carried out across multiple organizations, including universities, academic health sciences centers, start-ups, and technology corporations. Specific suggestions are summarized in Table 2 .
First, forum participants suggested that governance leaders including RECs, should remain up to date on recent advances in the regulation of AI for health. Regulation of AI for health advances rapidly and takes on different forms in jurisdictions around the world. RECs play an important role in governance, but only a partial role; it was deemed important for RECs to acknowledge how they fit within a broader governance ecosystem in order to more effectively address the issues within their scope. Not only RECs but organizational leaders responsible for procurement, researchers, and commercial actors should all commit to efforts to remain up to date about the relevant approaches to regulating AI for health care and public health in jurisdictions internationally. In this way, governance can more adequately remain up to date with advances in regulation.
Second, forum participants suggested that governance leaders should focus on ethical governance of health data as a basis for ethical global health AI research. Health data are considered the foundation of AI development, being used to train AI algorithms for various uses [ 26 ]. By focusing on ethical governance of health data generation, sharing, and use, multiple actors will help to build an ethical foundation for AI development among global health researchers.
Third, forum participants believed that governance processes should incorporate AI impact assessments where appropriate. An AI impact assessment is the process of evaluating the potential effects, both positive and negative, of implementing an AI algorithm on individuals, society, and various stakeholders, generally over time frames specified in advance of implementation [ 27 ]. Although not all types of AI research in global health would warrant an AI impact assessment, this is especially relevant for those studies aiming to implement an AI system for intervention into health care or public health. Organizations such as RECs can use AI impact assessments to boost understanding of potential harms at the outset of a research project, encouraging researchers to more deeply consider potential harms in the development of their study.
Fourth, forum participants suggested that governance decisions should incorporate the use of environmental impact assessments, or at least the incorporation of environment values when assessing the potential impact of an AI system. An environmental impact assessment involves evaluating and anticipating the potential environmental effects of a proposed project to inform ethical decision-making that supports sustainability [ 28 ]. Although a relatively new consideration in research ethics conversations [ 29 ], the environmental impact of building technologies is a crucial consideration for the public health commitment to environmental sustainability. Governance leaders can use environmental impact assessments to boost understanding of potential environmental harms linked to AI research projects in global health over both the shorter and longer terms.
Fifth, forum participants suggested that governance leaders should require stronger transparency in the development of AI algorithms in global health research. Transparency was considered essential in the design and development of AI algorithms for global health to ensure ethical and accountable decision-making throughout the process. Furthermore, whether and how researchers have considered the unique contexts into which such algorithms may be deployed can be surfaced through stronger transparency, for example in describing what primary considerations were made at the outset of the project and which stakeholders were consulted along the way. Sharing information about data provenance and methods used in AI development will also enhance the trustworthiness of the AI-based research process.
Sixth, forum participants suggested that governance leaders can encourage or require community engagement at various points throughout an AI project. It was considered that engaging patients and communities is crucial in AI algorithm development to ensure that the technology aligns with community needs and values. However, participants acknowledged that this is not a straightforward process. Effective community engagement requires lengthy commitments to meeting with and hearing from diverse communities in a given setting, and demands a particular set of skills in communication and dialogue that are not possessed by all researchers. Encouraging AI researchers to begin this process early and build long-term partnerships with community members is a promising strategy to deepen community engagement in AI research for global health. One notable recommendation was that research funders have an opportunity to incentivize and enable community engagement with funds dedicated to these activities in AI research in global health.
Seventh, forum participants suggested that governance leaders can encourage researchers to build strong, fair partnerships between institutions and individuals across country settings. In a context of longstanding imbalances in geopolitical and economic power, fair partnerships in global health demand a priori commitments to share benefits related to advances in medical technologies, knowledge, and financial gains. Although enforcement of this point might be beyond the remit of RECs, commentary will encourage researchers to consider stronger, fairer partnerships in global health in the longer term.
Eighth, it became evident that it is necessary to explore new forms of regulatory experimentation given the complexity of regulating a technology of this nature. In addition, the health sector has a series of particularities that make it especially complicated to generate rules that have not been previously tested. Several participants highlighted the desire to promote spaces for experimentation such as regulatory sandboxes or innovation hubs in health. These spaces can have several benefits for addressing issues surrounding the regulation of AI in the health sector, such as: (i) increasing the capacities and knowledge of health authorities about this technology; (ii) identifying the major problems surrounding AI regulation in the health sector; (iii) establishing possibilities for exchange and learning with other authorities; (iv) promoting innovation and entrepreneurship in AI in health; and (vi) identifying the need to regulate AI in this sector and update other existing regulations.
Ninth and finally, forum participants believed that the capabilities of governance leaders need to evolve to better incorporate expertise related to AI in ways that make sense within a given jurisdiction. With respect to RECs, for example, it might not make sense for every REC to recruit a member with expertise in AI methods. Rather, it will make more sense in some jurisdictions to consult with members of the scientific community with expertise in AI when research protocols are submitted that demand such expertise. Furthermore, RECs and other approaches to research governance in jurisdictions around the world will need to evolve in order to adopt the suggestions outlined above, developing processes that apply specifically to the ethical governance of research using AI methods in global health.
Research involving the development and implementation of AI technologies continues to grow in global health, posing important challenges for ethical governance of AI in global health research around the world. In this paper we have summarized insights from the 2022 GFBR, focused specifically on issues in research ethics related to AI for global health research. We summarized four thematic challenges for governance related to AI in global health research and nine suggestions arising from presentations and dialogue at the forum. In this brief discussion section, we present an overarching observation about power imbalances that frames efforts to evolve the role of governance in global health research, and then outline two important opportunity areas as the field develops to meet the challenges of AI in global health research.
Dialogue about power is not unfamiliar in global health, especially given recent contributions exploring what it would mean to de-colonize global health research, funding, and practice [ 30 , 31 ]. Discussions of research ethics applied to AI research in global health contexts are deeply infused with power imbalances. The existing context of global health is one in which high-income countries primarily located in the “Global North” charitably invest in projects taking place primarily in the “Global South” while recouping knowledge, financial, and reputational benefits [ 32 ]. With respect to AI development in particular, recent examples of digital colonialism frame dialogue about global partnerships, raising attention to the role of large commercial entities and global financial capitalism in global health research [ 21 , 22 ]. Furthermore, the power of governance organizations such as RECs to intervene in the process of AI research in global health varies widely around the world, depending on the authorities assigned to them by domestic research governance policies. These observations frame the challenges outlined in our paper, highlighting the difficulties associated with making meaningful change in this field.
Despite these overarching challenges of the global health research context, there are clear strategies for progress in this domain. Firstly, AI innovation is rapidly evolving, which means approaches to the governance of AI for health are rapidly evolving too. Such rapid evolution presents an important opportunity for governance leaders to clarify their vision and influence over AI innovation in global health research, boosting the expertise, structure, and functionality required to meet the demands of research involving AI. Secondly, the research ethics community has strong international ties, linked to a global scholarly community that is committed to sharing insights and best practices around the world. This global community can be leveraged to coordinate efforts to produce advances in the capabilities and authorities of governance leaders to meaningfully govern AI research for global health given the challenges summarized in our paper.
Limitations
Our paper includes two specific limitations that we address explicitly here. First, it is still early in the lifetime of the development of applications of AI for use in global health, and as such, the global community has had limited opportunity to learn from experience. For example, there were many fewer case studies, which detail experiences with the actual implementation of an AI technology, submitted to GFBR 2022 for consideration than was expected. In contrast, there were many more governance reports submitted, which detail the processes and outputs of governance processes that anticipate the development and dissemination of AI technologies. This observation represents both a success and a challenge. It is a success that so many groups are engaging in anticipatory governance of AI technologies, exploring evidence of their likely impacts and governing technologies in novel and well-designed ways. It is a challenge that there is little experience to build upon of the successful implementation of AI technologies in ways that have limited harms while promoting innovation. Further experience with AI technologies in global health will contribute to revising and enhancing the challenges and recommendations we have outlined in our paper.
Second, global trends in the politics and economics of AI technologies are evolving rapidly. Although some nations are advancing detailed policy approaches to regulating AI more generally, including for uses in health care and public health, the impacts of corporate investments in AI and political responses related to governance remain to be seen. The excitement around large language models (LLMs) and large multimodal models (LMMs) has drawn deeper attention to the challenges of regulating AI in any general sense, opening dialogue about health sector-specific regulations. The direction of this global dialogue, strongly linked to high-profile corporate actors and multi-national governance institutions, will strongly influence the development of boundaries around what is possible for the ethical governance of AI for global health. We have written this paper at a point when these developments are proceeding rapidly, and as such, we acknowledge that our recommendations will need updating as the broader field evolves.
Ultimately, coordination and collaboration between many stakeholders in the research ethics ecosystem will be necessary to strengthen the ethical governance of AI in global health research. The 2022 GFBR illustrated several innovations in ethical governance of AI for global health research, as well as several areas in need of urgent attention internationally. This summary is intended to inform international and domestic efforts to strengthen research ethics and support the evolution of governance leadership to meet the demands of AI in global health research.
Data availability
All data and materials analyzed to produce this paper are available on the GFBR website: https://www.gfbr.global/past-meetings/16th-forum-cape-town-south-africa-29-30-november-2022/ .
Clark P, Kim J, Aphinyanaphongs Y, Marketing, Food US. Drug Administration Clearance of Artificial Intelligence and Machine Learning Enabled Software in and as Medical devices: a systematic review. JAMA Netw Open. 2023;6(7):e2321792–2321792.
Article Google Scholar
Potnis KC, Ross JS, Aneja S, Gross CP, Richman IB. Artificial intelligence in breast cancer screening: evaluation of FDA device regulation and future recommendations. JAMA Intern Med. 2022;182(12):1306–12.
Siala H, Wang Y. SHIFTing artificial intelligence to be responsible in healthcare: a systematic review. Soc Sci Med. 2022;296:114782.
Yang X, Chen A, PourNejatian N, Shin HC, Smith KE, Parisien C, et al. A large language model for electronic health records. NPJ Digit Med. 2022;5(1):194.
Meskó B, Topol EJ. The imperative for regulatory oversight of large language models (or generative AI) in healthcare. NPJ Digit Med. 2023;6(1):120.
Jobin A, Ienca M, Vayena E. The global landscape of AI ethics guidelines. Nat Mach Intell. 2019;1(9):389–99.
Minssen T, Vayena E, Cohen IG. The challenges for Regulating Medical Use of ChatGPT and other large Language models. JAMA. 2023.
Ho CWL, Malpani R. Scaling up the research ethics framework for healthcare machine learning as global health ethics and governance. Am J Bioeth. 2022;22(5):36–8.
Yeung K. Recommendation of the council on artificial intelligence (OECD). Int Leg Mater. 2020;59(1):27–34.
Maddox TM, Rumsfeld JS, Payne PR. Questions for artificial intelligence in health care. JAMA. 2019;321(1):31–2.
Dzau VJ, Balatbat CA, Ellaissi WF. Revisiting academic health sciences systems a decade later: discovery to health to population to society. Lancet. 2021;398(10318):2300–4.
Ferretti A, Ienca M, Sheehan M, Blasimme A, Dove ES, Farsides B, et al. Ethics review of big data research: what should stay and what should be reformed? BMC Med Ethics. 2021;22(1):1–13.
Rahimzadeh V, Serpico K, Gelinas L. Institutional review boards need new skills to review data sharing and management plans. Nat Med. 2023;1–3.
Kling S, Singh S, Burgess TL, Nair G. The role of an ethics advisory committee in data science research in sub-saharan Africa. South Afr J Sci. 2023;119(5–6):1–3.
Google Scholar
Cengiz N, Kabanda SM, Esterhuizen TM, Moodley K. Exploring perspectives of research ethics committee members on the governance of big data in sub-saharan Africa. South Afr J Sci. 2023;119(5–6):1–9.
Doerr M, Meeder S. Big health data research and group harm: the scope of IRB review. Ethics Hum Res. 2022;44(4):34–8.
Ballantyne A, Stewart C. Big data and public-private partnerships in healthcare and research: the application of an ethics framework for big data in health and research. Asian Bioeth Rev. 2019;11(3):315–26.
Samuel G, Chubb J, Derrick G. Boundaries between research ethics and ethical research use in artificial intelligence health research. J Empir Res Hum Res Ethics. 2021;16(3):325–37.
Murphy K, Di Ruggiero E, Upshur R, Willison DJ, Malhotra N, Cai JC, et al. Artificial intelligence for good health: a scoping review of the ethics literature. BMC Med Ethics. 2021;22(1):1–17.
Teixeira da Silva JA. Handling ethics dumping and neo-colonial research: from the laboratory to the academic literature. J Bioethical Inq. 2022;19(3):433–43.
Ferryman K. The dangers of data colonialism in precision public health. Glob Policy. 2021;12:90–2.
Couldry N, Mejias UA. Data colonialism: rethinking big data’s relation to the contemporary subject. Telev New Media. 2019;20(4):336–49.
Organization WH. Ethics and governance of artificial intelligence for health: WHO guidance. 2021.
Metcalf J, Moss E. Owning ethics: corporate logics, silicon valley, and the institutionalization of ethics. Soc Res Int Q. 2019;86(2):449–76.
Data Protection Act - OFFICE OF THE DATA PROTECTION COMMISSIONER KENYA [Internet]. 2021 [cited 2023 Sep 30]. https://www.odpc.go.ke/dpa-act/ .
Sharon T, Lucivero F. Introduction to the special theme: the expansion of the health data ecosystem–rethinking data ethics and governance. Big Data & Society. Volume 6. London, England: SAGE Publications Sage UK; 2019. p. 2053951719852969.
Reisman D, Schultz J, Crawford K, Whittaker M. Algorithmic impact assessments: a practical Framework for Public Agency. AI Now. 2018.
Morgan RK. Environmental impact assessment: the state of the art. Impact Assess Proj Apprais. 2012;30(1):5–14.
Samuel G, Richie C. Reimagining research ethics to include environmental sustainability: a principled approach, including a case study of data-driven health research. J Med Ethics. 2023;49(6):428–33.
Kwete X, Tang K, Chen L, Ren R, Chen Q, Wu Z, et al. Decolonizing global health: what should be the target of this movement and where does it lead us? Glob Health Res Policy. 2022;7(1):3.
Abimbola S, Asthana S, Montenegro C, Guinto RR, Jumbam DT, Louskieter L, et al. Addressing power asymmetries in global health: imperatives in the wake of the COVID-19 pandemic. PLoS Med. 2021;18(4):e1003604.
Benatar S. Politics, power, poverty and global health: systems and frames. Int J Health Policy Manag. 2016;5(10):599.
Download references
Acknowledgements
We would like to acknowledge the outstanding contributions of the attendees of GFBR 2022 in Cape Town, South Africa. This paper is authored by members of the GFBR 2022 Planning Committee. We would like to acknowledge additional members Tamra Lysaght, National University of Singapore, and Niresh Bhagwandin, South African Medical Research Council, for their input during the planning stages and as reviewers of the applications to attend the Forum.
This work was supported by Wellcome [222525/Z/21/Z], the US National Institutes of Health, the UK Medical Research Council (part of UK Research and Innovation), and the South African Medical Research Council through funding to the Global Forum on Bioethics in Research.
Author information
Authors and affiliations.
Department of Physical Therapy, Temerty Faculty of Medicine, University of Toronto, Toronto, Canada
Berman Institute of Bioethics, Johns Hopkins University, Baltimore, MD, USA
Bloomberg School of Public Health, Johns Hopkins University, Baltimore, MD, USA
Department of Philosophy and Classics, University of Ghana, Legon-Accra, Ghana
Caesar A. Atuire
Centre for Tropical Medicine and Global Health, Nuffield Department of Medicine, University of Oxford, Oxford, UK
Mahidol Oxford Tropical Medicine Research Unit, Faculty of Tropical Medicine, Mahidol University, Bangkok, Thailand
Phaik Yeong Cheah
Berkman Klein Center, Harvard University, Bogotá, Colombia
Armando Guio Español
Department of Radiology and Informatics, Emory University School of Medicine, Atlanta, GA, USA
Judy Wawira Gichoya
Health Ethics & Governance Unit, Research for Health Department, Science Division, World Health Organization, Geneva, Switzerland
Adrienne Hunt & Katherine Littler
African Center of Excellence in Bioinformatics and Data Intensive Science, Infectious Diseases Institute, Makerere University, Kampala, Uganda
Daudi Jjingo
ISI Foundation, Turin, Italy
Daniela Paolotti
Department of Health Sciences and Technology, ETH Zurich, Zürich, Switzerland
Effy Vayena
Joint Centre for Bioethics, Dalla Lana School of Public Health, University of Toronto, Toronto, Canada
You can also search for this author in PubMed Google Scholar
Contributions
JS led the writing, contributed to conceptualization and analysis, critically reviewed and provided feedback on drafts of this paper, and provided final approval of the paper. JA contributed to conceptualization and analysis, critically reviewed and provided feedback on drafts of this paper, and provided final approval of the paper. CA contributed to conceptualization and analysis, critically reviewed and provided feedback on drafts of this paper, and provided final approval of the paper. PYC contributed to conceptualization and analysis, critically reviewed and provided feedback on drafts of this paper, and provided final approval of the paper. AE contributed to conceptualization and analysis, critically reviewed and provided feedback on drafts of this paper, and provided final approval of the paper. JWG contributed to conceptualization and analysis, critically reviewed and provided feedback on drafts of this paper, and provided final approval of the paper. AH contributed to conceptualization and analysis, critically reviewed and provided feedback on drafts of this paper, and provided final approval of the paper. DJ contributed to conceptualization and analysis, critically reviewed and provided feedback on drafts of this paper, and provided final approval of the paper. KL contributed to conceptualization and analysis, critically reviewed and provided feedback on drafts of this paper, and provided final approval of the paper. DP contributed to conceptualization and analysis, critically reviewed and provided feedback on drafts of this paper, and provided final approval of the paper. EV contributed to conceptualization and analysis, critically reviewed and provided feedback on drafts of this paper, and provided final approval of the paper.
Corresponding author
Correspondence to James Shaw .
Ethics declarations
Ethics approval and consent to participate.
Not applicable.
Consent for publication
Competing interests.
The authors declare no competing interests.
Additional information
Publisher’s note.
Springer Nature remains neutral with regard to jurisdictional claims in published maps and institutional affiliations.
Rights and permissions
Open Access This article is licensed under a Creative Commons Attribution 4.0 International License, which permits use, sharing, adaptation, distribution and reproduction in any medium or format, as long as you give appropriate credit to the original author(s) and the source, provide a link to the Creative Commons licence, and indicate if changes were made. The images or other third party material in this article are included in the article’s Creative Commons licence, unless indicated otherwise in a credit line to the material. If material is not included in the article’s Creative Commons licence and your intended use is not permitted by statutory regulation or exceeds the permitted use, you will need to obtain permission directly from the copyright holder. To view a copy of this licence, visit http://creativecommons.org/licenses/by/4.0/ . The Creative Commons Public Domain Dedication waiver ( http://creativecommons.org/publicdomain/zero/1.0/ ) applies to the data made available in this article, unless otherwise stated in a credit line to the data.
Reprints and permissions
About this article
Cite this article.
Shaw, J., Ali, J., Atuire, C.A. et al. Research ethics and artificial intelligence for global health: perspectives from the global forum on bioethics in research. BMC Med Ethics 25 , 46 (2024). https://doi.org/10.1186/s12910-024-01044-w
Download citation
Received : 31 October 2023
Accepted : 01 April 2024
Published : 18 April 2024
DOI : https://doi.org/10.1186/s12910-024-01044-w
Share this article
Anyone you share the following link with will be able to read this content:
Sorry, a shareable link is not currently available for this article.
Provided by the Springer Nature SharedIt content-sharing initiative
- Artificial intelligence
- Machine learning
- Research ethics
- Global health
BMC Medical Ethics
ISSN: 1472-6939
- General enquiries: [email protected]
- Open access
- Published: 30 April 2024
The effect of dexmedetomidine on the postoperative recovery of patients with severe traumatic brain injury undergoing craniotomy treatment: a retrospective study
- Zhu Deng 1 na1 ,
- Yong Gu 1 na1 ,
- Le Luo 1 na1 ,
- Lin Deng 2 na1 ,
- Yingwei Li 1 &
- Wanyong Huang 1
European Journal of Medical Research volume 29 , Article number: 256 ( 2024 ) Cite this article
Metrics details
Traumatic brain injury (TBI) has been a worldwide problem for neurosurgeons. Patients with severe TBI may undergo craniotomy. These patients often require sedation after craniotomy. Dexmedetomidine (DEX) has been used in patients receiving anesthesia and in intensive care units. Not much is known about the postoperative effect of DEX in patients with severe TBIs undergoing craniotomy. The purpose of this study was to explore the effects of postoperative DEX administration on severe TBI patients who underwent craniotomy.
Patients who underwent craniectomy for severe TBI at our hospital between January 2019 and February 2022 were included in this study. The patients were admitted to the intensive care unit (ICU) after surgery to receive sedative medication. The patients were then divided into DEX and control groups. We analyzed the sedation, hemodynamics, and other conditions of the patients (hypoxemia, duration of ventilation during endotracheal intubation, whether tracheotomy was performed, and the duration in the ICU) during their ICU stay. Other conditions, such as delirium after the patients were transferred to the general ward, were also analyzed.
A total of 122 patients were included in this study. Among them, 53 patients received DEX, and the remaining 69 did not. The incidence of delirium in the general ward in the DEX group was significantly lower than that in the control group ( P < 0.05). The incidence of bradycardia in the control group was significantly lower than that in the DEX group ( P < 0.05). Other data from the DEX group and the control group (hypotension, hypoxemia, etc.) were not significantly different ( P > 0.05).
The use of DEX in the ICU can effectively reduce the incidence of delirium in patients who return to the general ward after craniotomy. DEX had no adverse effect on the prognosis of patients other than causing bradycardia.
Traumatic brain injury (TBI) is a serious traumatic disease that is a difficult to treat and is considered problem worldwide (especially for critically ill TBI patients) [ 1 ]. Approximately 0.5% of people experience TBI each year due to trauma [ 2 ]. TBI has always been difficult for neurosurgeons due to its high disability and mortality rates. Moreover, TBI not only causes disaster in patients themselves, but also places a heavy burden on families and society [ 2 , 3 ]. According to the mechanism of injury, TBIs can be divided into two categories: primary injury (mainly including epidural hematoma, subdural hematoma, brain tissue contusion and axonal injury) and secondary injury (mainly including brain tissue edema and increased intracranial pressure) [ 4 , 5 ]. According to the severity of the disease, TBI can be divided into mild (score of 13–15), moderate (score of 9–12), and severe (score ≤ 8) according to the Glasgow Coma Scale (GCS) [ 6 , 7 , 8 ].
For patients with TBI, a primary injury such as a subdural hemorrhage results in a series of secondary injuries. The inflammatory response of the nervous system, oxidative stress injury and nerve cell apoptosis are the main causes of these secondary injuries [ 9 , 10 ]. Among these changes, the inflammatory response of the nervous system, caused by trauma, is thought to be the key factor that triggers a series of reactions. Its main response changes to glial activation, peripheral inflammatory cell infiltration and inflammatory mediator release [ 9 ]. For TBI patients, an appropriate inflammatory response plays a positive role in damage repair. However, severe TBI often causes this inflammatory response to be excessive and persistent, resulting in pathophysiological changes that are difficult to control. The series of uncontrollable pathophysiological changes induced by TBI is ultimately responsible for its high disability and mortality rates [ 2 , 3 , 9 ]. Therefore, neurosurgeons have always hoped to reduce the harm caused by TBIs by controlling the excessive inflammatory response to improve neurological function.
Dexmedetomidine (DEX), a highly selective α-2 adrenergic receptor agonist, has been used in patients receiving anesthesia and in intensive care units (ICUs) [ 11 ]. DEX has good performance in terms of preventing anxiety, providing analgesia and providing sedation to patients [ 11 ]. A study of TBI patients revealed that DEX can inhibit an excessive inflammatory response by inhibiting the activation of some inflammasomes, thereby exerting a certain protective effect on the nervous system of TBI patients in the early stage [ 12 ]. In fact, DEX has been used in the management of patients admitted to the ICU for severe TBI. DEX can alleviate agitation, prevent the occurrence of paroxysmal sympathetic hyperactivity, and reduce the occurrence of delirium in patients with severe TBI in the ICU. However, DEX has side effects, such as hemodynamic changes [ 13 , 14 , 15 ].
Although DEX has a clear effect on patients with severe TBI, little is known about the postoperative effect of DEX in patients with severe TBI who underwent craniotomy. Therefore, the purpose of this study was to determine whether DEX is equally safe and effective for treating severe TBI after craniotomy and whether DEX will have a long-term impact on patient prognosis.
Study subjects
Retrospectively and consecutively, the subjects of this study were all severe TBI patients who underwent craniotomy in the Department of Neurosurgery, People's Hospital of Guanghan City, from January 2019 to February 2022. Patients who were older than 80 years and younger than 18 years, followed up for less than half a year, lacked clinical data, died, had a second surgery, or did not receive sedation and patients or family members who declined to participate in this study were excluded. All patients underwent cranial CT on the first day, the third day and 1 week after craniotomy. Patients who experienced postoperative rebleeding or incomplete hematoma removal were excluded (postoperative intracranial hematoma > 5 ml). We also evaluated other relevant organ systems in patients with delirium and agitation. Patients were excluded if metabolic abnormalities and other complications were present. All family members of the patients signed informed consent. The article follows the STROBE statement and was approved by the People's Hospital of Guanghan City Ethics Committee.
Data collection and outcome assessment
The clinical characteristics and demographic data were recorded by the authors of the study after the patients were admitted. The data included sex, age, preoperative GCS score, and preoperative pupil condition (mydriasis). After surgery, patients were treated with sedative drugs in the ICU (dexamethasone (DEX) or not), degree of sedation (Richmond Agitation-Sedation Scores (RASS); patients were then transferred to the ICU immediately after surgery, and scoring began upon admission to the ICU. Hemodynamics during sedation included: heart rate and arterial blood pressure. Blood oxygen saturation during sedation, the duration of sedation, the ventilation time for endotracheal intubation were also recorded. It is unclear whether tracheotomy is acceptable. The duration in the ICU. When vital signs were stable (no vasopressors are needed to maintain blood pressure) and blood oxygen saturation was greater than 90% under ordinary oxygen inhalation (no ventilator is needed to assist breathing), the patient was transferred to the general ward. Delirium was confirmed after the patient was transferred to the general ward. The total length of hospital stay. Condition at discharge: GCS score at discharge. Long-term outcomes were assessed using the Glasgow Outcome Scale (GOS) (Table 1 ). In addition, if the patient's heart rate was lower than 55, it was considered to indicate bradycardia; if the systolic blood pressure was less than 90, it was considered to indicate hypotension; if the blood oxygen saturation was lower than 90, it was considered to indicate hypoxemia. A patient with a RASS score greater than or equal to 2 during sedation was considered to have a poor sedation effect. We assessed patients for delirium risk by using the validation of the delirium rating scale-revised-98 (DRS-R-98). A score of ≥ 1 for the 3 diagnostic items indicated the presence of delirium in a patient. Disturbances due to delirium and agitation due to metabolic conditions were avoided.
Therapy method
All the patients underwent craniotomy at the People's Hospital of Guanghan City by highly experienced and well-trained neurosurgeons. The patients were admitted to the ICU after surgery to receive sedative medication. The sedative drug in the control group was propofol plus midazolam, while the sedative drug in the experimental group was DEX in combination with propofol. In terms of analgesia, our drug of choice is fentanyl. A RASS score ≥ 2 indicated that the sedative effect was poor, and the sedation regimen needed to be adjusted (appropriately increasing the dose of sedative drugs). All patients received essentially the same treatment regimen, with the exception of treatment differences on appeal.
Statistical analyses
All the data we collected were analyzed by R version 4.2.2. All data analyses were performed only between the experimental group (DEX group) and the control group. Continuous variables are expressed as the mean ± standard deviation (for example, age, preoperative GCS score, ventilation time at endotracheal intubation, total length of hospital stay, etc.). We used the numbers and percentages to represent the categorical variables (for example, sex, mydriasis, bradycardia, and delirium after transfer to the general ward). We used the Kolmogorov‒Smirnov test for the normality of continuous variables, and the t test was subsequently used to compare the experimental group and the control group. Additionally, we used Pearson’s χ 2 test or Fisher’s exact test for comparisons between categorical variables. A difference was considered to be statistically significant when P was ≤ 0.05.
Clinical characteristics
A total of 157 patients received craniotomy at People's Hospital of Guanghan City from January 2019 to February 2022. Among those patients, 23 died after surgery, 5 patients were transferred to a high-level hospital for continued treatment, 3 patients abandoned treatment after surgery, and 4 patients were lost to follow-up after discharge. After excluding these patients, we eventually enrolled 122 patients in this study. Of the patients included in the study, 53 received DEX, and the remaining 69 did not.
The demographic and clinical data of the patients are shown in Table 2 . The results showed that there were no significant differences in sex, age, preoperative GCS score, or preoperative pupil condition (mydriasis) between the DEX group and the control group ( P > 0.05).
Sedation with DEX was initiated as soon as the patient was admitted to the ICU, and the duration of use was 44 ± 40 h. The mean rate of administration was 0.53 ± 0.25 μg/kg/h, the mean minimum rate was 0.26 ± 0.11 μg/kg/h, and the mean maximum rate was 0.98 ± 0.21 μg/kg/h. During IUC, 27 patients in the DEX group developed bradycardia. A total of 19 people in the control group developed bradycardia. There was a significant difference between the two groups ( P < 0.05). Other data from the DEX group and the control group collected during the ICU stay (hypotension, hypoxemia, RASS score, ventilation time of endotracheal intubation, whether tracheotomy was performed, and duration in the ICU) were not significantly different ( P > 0.05).
General ward and other data
After the patients were transferred to the general ward, a total of 6 patients in the DEX group developed delirium, and a total of 19 patients in the control group developed delirium. There was a significant difference between the two groups ( P < 0.05). Moreover, the total length of hospital stay, GCS score at discharge and GOS score six months after discharge did not significantly differ between the DEX group and the control group ( P > 0.05). Our subgroup analysis of patients who presented with delirium revealed no significant difference in the preoperative (Tables 3 and 4 ) GCS score or postoperative cranial CT ( P > 0.05).
We conducted a retrospective analysis of 122 patients who underwent craniotomy in the Department of Neurosurgery of our hospital and found that the incidence of delirium in patients who returned to the general ward in the DEX group was significantly lower than that in the control group, but the manifestation of delirium significantly increased the incidence of bradycardia. In addition, DEX did not increase the occurrence of other adverse events. There was no significant difference in length of ICU stay or total length of stay between the two groups. In terms of patient recovery, DEX did not adversely affect patient prognosis.
Many patients with severe TBI are transferred to the ICU for more effective treatment, especially for those with severe TBI who have undergone craniotomy [ 16 ]. Because of the severe nature of TBI itself, the need for craniotomy and other reasons, these patients often exhibit agitation [ 3 , 17 ]. Once a patient has this condition, it usually needs to be controlled with medication. In some cases, restraint bands may even be used to protect patients [ 18 ]. Propofol, midazolam, and other agents are often used to sedate agitated patients during ICU stays [ 19 ]. A descriptive study by Bilodeau et al. revealed a favorable sedative effect of DEX in patients with TBI [ 13 ]. For severe TBI patients undergoing craniotomy, these sedatives can also have a significant effect [ 2 ]. DEX, a new highly selective α-2 adrenergic agonist, has been approved by anesthesiologists and ICU physicians. A small number of studies have shown satisfactory results for sedation with DEX in severe TBI patients who had not undergone craniotomy during an ICU stay [ 20 , 21 ]. As an increasing amount of research has been conducted on DEX in TBI patients, the benefits of DEX on TBI patients have gradually been discovered. A study by Hao et al. revealed DEX to be more effective than propofol at controlling the overstress response after TBI [ 22 ]. In addition, DEX can reduce intestinal tissue damage by improving the inflammatory response after TBI [ 23 ]. The protection of brain function is important for patients with TBI, and Wang et al. reported no adverse effects of DEX use on brain function [ 24 ]. DEX is also gradually being used in the sedation of patients with TBI. Our study revealed that DEX also had a significant sedative effect on severe TBI patients undergoing craniotomy.
Currently, adverse events related to DEX mainly manifest as a result of its effect on hemodynamics. DEX has been found to induce hypotension during the ICU stay [ 25 ]. This was also observed in our study, but DEX did not significantly affect hypotension incidence compared to that in the control group. However, our study revealed that the DEX treatment group had a significantly greater incidence of bradycardia than the control group. DEX has also been used in anesthesiology departments and ICUs in the recent years, but the mechanism of its influence on the hemodynamics of patients is still unclear. Currently, the mechanism of action of DEX, which is recognized by the academic community, is as follows: as an α-2 adrenergic agonist, DEX can activate presynaptic and postsynaptic α-2 receptors, thereby causing contraction of peripheral blood vessels, relaxation of peripheral blood vessels and reflex bradycardia, which can cause increased blood pressure or a decline in blood pressure. Moreover, DEX can significantly reduce the occurrence of stress reactions, which leads to a reduced likelihood of catecholamine secretion due to the stress response; thus, DEX does not cause a hemodynamic effect on catecholamine secretion [ 26 , 27 ].
Delirium, an acute mental state change, is characterized by inattention, arousal disorders and mental disorders [ 28 ]. However, the presence of delirium caused irreversible damage to the brain function of patients, significantly increased the total length of hospital stay, significantly increased the cost of hospitalization, and even seriously affected the prognosis [ 28 , 29 ]. Delirium is highly common in patients with severe TBI and can occur at any time during a patient's hospital stay, and studies suggest that up to 70% of patients present during a hospital stay [ 28 , 30 ]. Surgery is also an important cause of delirium, and the incidence of delirium after emergency surgery is 20–45%. Delirium usually occurs in these patients 2–5 days after surgery, but it is possible throughout the hospital stay [ 31 ]. Because treating delirium in the general ward can be more difficult than treating it in the ICU, our study evaluated the occurrence of delirium in the general ward. Our subjects were patients with severe TBI who had also undergone craniotomy, both of which could have caused a high incidence of delirium during their hospital stay. Our study only measured the incidence of delirium in patients who were transferred back to the general ward after stabilizing. Some of these patients may have developed delirium during their stay in the ICU and may have been treated. Therefore, we do not have a particularly high incidence. In our study, we were surprised to find that the use of DEX during hospitalization in the ICU can effectively reduce the incidence of delirium in patients in general wards.
The main shortcomings in this study are as follows. First, this was a retrospective study, and the retrospective nature of the study itself may have introduced bias. Second, the sample size of this study was relatively small, so there were certain difficulties in performing statistical analysis for those events with a very low incidence (e.g., RASS score ≥ 2), which also leads to an increased risk of bias. We hope to conduct a larger sample size and more in-depth study in the future. In addition, the small sample size prevented us from further subdividing the types of TBI patients, which also resulted in our findings not clarifying whether DEX has the same effect on extradural hematoma and subdural hematoma. However, these findings also point to the next step in our research. Fourth, this study only preliminarily explored patients who underwent craniotomy. There was no further research on the various craniotomy methods used in this study. Finally, this study was aimed at TBI patients, and whether this approach is applicable for non-TBI patients who undergo craniotomy needs to be further studied. In addition, due to the absence of data, our study analyzed only the occurrence of delirium in the general ward and did not evaluate the occurrence of delirium during patients' ICU stay.
In this study, the use of DEX in the ICU effectively reduced the incidence of delirium in patients who returned to the general ward after craniotomy. The sedative effect of DEX was satisfactory. In addition, DEX use in the ICU did not cause adverse events in patients other than an increased incidence of bradycardia.
Availability of data and materials
The data that support the findings of this study are available from the corresponding author upon reasonable request.
Abbreviations
- Dexmedetomidine
- Traumatic brain injury
Glasgow Coma Scale
Intensive care units
Richmond Agitation-Sedation Scores
Glasgow Outcome Scale
Delirium rating scale-revised-98
Godoy DA, Rabinstein AA. How to manage traumatic brain injury without invasive monitoring? Curr Opin Crit Care. 2022;28(2):111–22. https://doi.org/10.1097/mcc.0000000000000914 .
Article PubMed Google Scholar
Capizzi A, Woo J, Verduzco-Gutierrez M. Traumatic brain injury: an overview of epidemiology, pathophysiology, and medical management. Med Clin North Am. 2020;104(2):213–38. https://doi.org/10.1016/j.mcna.2019.11.001 .
Khellaf A, Khan DZ, Helmy A. Recent advances in traumatic brain injury. J Neurol. 2019;266(11):2878–89. https://doi.org/10.1007/s00415-019-09541-4 .
Article PubMed PubMed Central Google Scholar
Stocchetti N, Carbonara M, Citerio G, Ercole A, Skrifvars MB, Smielewski P, et al. Severe traumatic brain injury: targeted management in the intensive care unit. Lancet Neurol. 2017;16(6):452–64. https://doi.org/10.1016/s1474-4422(17)30118-7 .
Wang KK, Yang Z, Zhu T, Shi Y, Rubenstein R, Tyndall JA, et al. An update on diagnostic and prognostic biomarkers for traumatic brain injury. Expert Rev Mol Diagn. 2018;18(2):165–80. https://doi.org/10.1080/14737159.2018.1428089 .
Article CAS PubMed PubMed Central Google Scholar
Najem D, Rennie K, Ribecco-Lutkiewicz M, Ly D, Haukenfrers J, Liu Q, et al. Traumatic brain injury: classification, models, and markers. Biochem Cell Biol. 2018;96(4):391–406. https://doi.org/10.1139/bcb-2016-0160 .
Article CAS PubMed Google Scholar
Ladak AA, Enam SA, Ibrahim MT. A review of the molecular mechanisms of traumatic brain injury. World Neurosurg. 2019;131:126–32. https://doi.org/10.1016/j.wneu.2019.07.039 .
Teasdale G, Jennett B. Assessment of coma and impaired consciousness. A practical scale. Lancet (London, England). 1974;2(7872):81–4. https://doi.org/10.1016/s0140-6736(74)91639-0 .
Shi H, Wang HL, Pu HJ, Shi YJ, Zhang J, Zhang WT, et al. Ethyl pyruvate protects against blood-brain barrier damage and improves long-term neurological outcomes in a rat model of traumatic brain injury. CNS Neurosci Ther. 2015;21(4):374–84. https://doi.org/10.1111/cns.12366 .
Wang C, Chang YX, Chen X, Bai L, Wang H, Pan YC, et al. A calixarene assembly strategy of combined anti-neuroinflammation and drug delivery functions for traumatic brain injury Therapy. Molecules. 2022. https://doi.org/10.3390/molecules27092967 .
Keating GM. Dexmedetomidine: a review of its use for sedation in the intensive care setting. Drugs. 2015;75(10):1119–30. https://doi.org/10.1007/s40265-015-0419-5 .
Wang D, Xu X, Wu YG, Lyu L, Zhou ZW, Zhang JN. Dexmedetomidine attenuates traumatic brain injury: action pathway and mechanisms. Neural Regen Res. 2018;13(5):819–26. https://doi.org/10.4103/1673-5374.232529 .
Bilodeau V, Saavedra-Mitjans M, Frenette AJ, Burry L, Albert M, Bernard F, et al. Safety of dexmedetomidine for the control of agitation in critically ill traumatic brain injury patients: a descriptive study. J Clin Pharm Ther. 2021;46(4):1020–6. https://doi.org/10.1111/jcpt.13389 .
Tang Q, Wu X, Weng W, Li H, Feng J, Mao Q, et al. The preventive effect of dexmedetomidine on paroxysmal sympathetic hyperactivity in severe traumatic brain injury patients who have undergone surgery: a retrospective study. PeerJ. 2017;5: e2986. https://doi.org/10.7717/peerj.2986 .
Reade MC, Eastwood GM, Bellomo R, Bailey M, Bersten A, Cheung B, et al. Effect of dexmedetomidine added to standard care on ventilator-free time in patients with agitated delirium: a randomized clinical trial. JAMA. 2016;315(14):1460–8. https://doi.org/10.1001/jama.2016.2707 .
Stocchetti N, Zanier ER. Chronic impact of traumatic brain injury on outcome and quality of life: a narrative review. Crit Care (London, England). 2016;20(1):148. https://doi.org/10.1186/s13054-016-1318-1 .
Article Google Scholar
Poulsen I, Langhorn L, Egerod I, Aadal L. Sleep and agitation during subacute traumatic brain injury rehabilitation: A scoping review. Aust Crit Care. 2021;34(1):76–82. https://doi.org/10.1016/j.aucc.2020.05.006 .
Singh R, Venkateshwara G, Nair KP, Khan M, Saad R. Agitation after traumatic brain injury and predictors of outcome. Brain Inj. 2014;28(3):336–40. https://doi.org/10.3109/02699052.2013.873142 .
Jakob SM, Ruokonen E, Grounds RM, Sarapohja T, Garratt C, Pocock SJ, et al. Dexmedetomidine vs midazolam or propofol for sedation during prolonged mechanical ventilation: two randomized controlled trials. JAMA. 2012;307(11):1151–60. https://doi.org/10.1001/jama.2012.304 .
Pajoumand M, Kufera JA, Bonds BW, Devabhakthuni S, Boswell S, Hesselton K, et al. Dexmedetomidine as an adjunct for sedation in patients with traumatic brain injury. J Trauma Acute Care Surg. 2016;81(2):345–51. https://doi.org/10.1097/ta.0000000000001069 .
Humble SS, Wilson LD, Leath TC, Marshall MD, Sun DZ, Pandharipande PP, et al. ICU sedation with dexmedetomidine after severe traumatic brain injury. Brain Inj. 2016;30(10):1266–70. https://doi.org/10.1080/02699052.2016.1187289 .
Hao J, Luo JS, Weng Q, He Y, Liu J, Yang MH, et al. Effects of dexmedetomidine on sedation and β-endorphin in traumatic brain injury: a comparative study with propofol. Zhonghua Wei Zhong Bing Ji Jiu Yi Xue. 2013;25(6):373–6. https://doi.org/10.3760/cma.j.issn.2095-4352.2013.06.014 .
Karaca O, Doğan G. The effects of dexmedetomidine in increased intestinal permeability after traumatic brain injury: an experimental study. TJTES. 2020;26(1):15–20. https://doi.org/10.14744/tjtes.2019.49768 .
Wang X, Ji J, Fen L, Wang A. Effects of dexmedetomidine on cerebral blood flow in critically ill patients with or without traumatic brain injury: a prospective controlled trial. Brain Inj. 2013;27(13–14):1617–22. https://doi.org/10.3109/02699052.2013.831130 .
Afonso J, Reis F. Dexmedetomidine: current role in anesthesia and intensive care. Rev Bras Anestesiol. 2012;62(1):118–33. https://doi.org/10.1016/s0034-7094(12)70110-1 .
Weerink MAS, Struys M, Hannivoort LN, Barends CRM, Absalom AR, Colin P. Clinical pharmacokinetics and pharmacodynamics of dexmedetomidine. Clin Pharmacokinet. 2017;56(8):893–913. https://doi.org/10.1007/s40262-017-0507-7 .
Colin PJ, Hannivoort LN, Eleveld DJ, Reyntjens K, Absalom AR, Vereecke HEM, et al. Dexmedetomidine pharmacodynamics in healthy volunteers: 2. Haemodynamic profile. Br J Anaesthesia. 2017;119(2):211–20. https://doi.org/10.1093/bja/aex086 .
Article CAS Google Scholar
Ely EW, Shintani A, Truman B, Speroff T, Gordon SM, Harrell FE Jr, et al. Delirium as a predictor of mortality in mechanically ventilated patients in the intensive care unit. JAMA. 2004;291(14):1753–62. https://doi.org/10.1001/jama.291.14.1753 .
Steimer M, Kaiser S, Ulbrich F, Kalbhenn J, Bürkle H, Schallner N. Expression of HO1 and PER2 can predict the incidence of delirium in trauma patients with concomitant brain injury. Sci Rep. 2021;11(1):15388. https://doi.org/10.1038/s41598-021-94773-6 .
Maneewong J, Maneeton B, Maneeton N, Vaniyapong T, Traisathit P, Sricharoen N, et al. Delirium after a traumatic brain injury: predictors and symptom patterns. Neuropsychiatr Dis Treat. 2017;13:459–65. https://doi.org/10.2147/ndt.s128138 .
Jin Z, Hu J, Ma D. Postoperative delirium: perioperative assessment, risk reduction, and management. Br J Anaesth. 2020;125(4):492–504. https://doi.org/10.1016/j.bja.2020.06.063 .
Download references
Acknowledgements
Not applicable.
No funding was received for this research.
Author information
Zhu Deng, Yong Gu, Le Luo and Lin Deng Joint first authors.
Authors and Affiliations
Department of Neurosurgery, People’s Hospital of Guanghan City, No.9, Section3, Xi’an Road, Guanghan, Sichuan, People’s Republic of China
Zhu Deng, Yong Gu, Le Luo, Yingwei Li & Wanyong Huang
Department of Intensive Care Unit, People’s Hospital of Guanghan City, No.9, Section3, Xi’an Road, Guanghan, Sichuan, People’s Republic of China
You can also search for this author in PubMed Google Scholar
Contributions
Zhu Deng and Yong Gu were responsible for the design. Le Luo and Lin Deng was responsible of search. Yingwei Li, Yong Gu and Wanyong Huang were involving in data screening and data extraction. Zhu Deng was responsible in writing of the manuscript. All authors read and approve the final version of the manuscript.
Corresponding author
Correspondence to Wanyong Huang .
Ethics declarations
Ethics approval and consent to participate.
This article follows the STROBE statement and has been approved by the People's Hospital of Guanghan City Ethics Committee.
Consent for publication
Not Applicable.
Competing interests
The author affirms that there is no conflict of interest in this study.
Additional information
Publisher's note.
Springer Nature remains neutral with regard to jurisdictional claims in published maps and institutional affiliations.
Rights and permissions
Open Access This article is licensed under a Creative Commons Attribution 4.0 International License, which permits use, sharing, adaptation, distribution and reproduction in any medium or format, as long as you give appropriate credit to the original author(s) and the source, provide a link to the Creative Commons licence, and indicate if changes were made. The images or other third party material in this article are included in the article's Creative Commons licence, unless indicated otherwise in a credit line to the material. If material is not included in the article's Creative Commons licence and your intended use is not permitted by statutory regulation or exceeds the permitted use, you will need to obtain permission directly from the copyright holder. To view a copy of this licence, visit http://creativecommons.org/licenses/by/4.0/ . The Creative Commons Public Domain Dedication waiver ( http://creativecommons.org/publicdomain/zero/1.0/ ) applies to the data made available in this article, unless otherwise stated in a credit line to the data.
Reprints and permissions
About this article
Cite this article.
Deng, Z., Gu, Y., Luo, L. et al. The effect of dexmedetomidine on the postoperative recovery of patients with severe traumatic brain injury undergoing craniotomy treatment: a retrospective study. Eur J Med Res 29 , 256 (2024). https://doi.org/10.1186/s40001-024-01861-0
Download citation
Received : 21 December 2022
Accepted : 24 April 2024
Published : 30 April 2024
DOI : https://doi.org/10.1186/s40001-024-01861-0
Share this article
Anyone you share the following link with will be able to read this content:
Sorry, a shareable link is not currently available for this article.
Provided by the Springer Nature SharedIt content-sharing initiative
European Journal of Medical Research
ISSN: 2047-783X
- Submission enquiries: [email protected]
- General enquiries: [email protected]
- Open access
- Published: 24 April 2024
Multimorbidity in middle-aged women and COVID-19: binary data clustering for unsupervised binning of rare multimorbidity features and predictive modeling
- Dayana Benny 1 , 2 ,
- Mario Giacobini 3 ,
- Giuseppe Costa 1 , 4 ,
- Roberto Gnavi 4 &
- Fulvio Ricceri 1
BMC Medical Research Methodology volume 24 , Article number: 95 ( 2024 ) Cite this article
215 Accesses
3 Altmetric
Metrics details
Multimorbidity is typically associated with deficient health-related quality of life in mid-life, and the likelihood of developing multimorbidity in women is elevated. We address the issue of data sparsity in non-prevalent features by clustering the binary data of various rare medical conditions in a cohort of middle-aged women. This study aims to enhance understanding of how multimorbidity affects COVID-19 severity by clustering rare medical conditions and combining them with prevalent features for predictive modeling. The insights gained can guide the development of targeted interventions and improved management strategies for individuals with multiple health conditions.
The study focuses on a cohort of 4477 female patients, (aged 45-60) in Piedmont, Italy, and utilizes their multimorbidity data prior to the COVID-19 pandemic from their medical history from 2015 to 2019. The COVID-19 severity is determined by the hospitalization status of the patients from February to May 2020. Each patient profile in the dataset is depicted as a binary vector, where each feature denotes the presence or absence of a specific multimorbidity condition. By clustering the sparse medical data, newly engineered features are generated as a bin of features, and they are combined with the prevalent features for COVID-19 severity predictive modeling.
From sparse data consisting of 174 input features, we have created a low-dimensional feature matrix of 17 features. Machine Learning algorithms are applied to the reduced sparsity-free data to predict the Covid-19 hospital admission outcome. The performance obtained for the corresponding models are as follows: Logistic Regression (accuracy 0.72, AUC 0.77, F1-score 0.69), Linear Discriminant Analysis (accuracy 0.7, AUC 0.77, F1-score 0.67), and Ada Boost (accuracy 0.7, AUC 0.77, F1-score 0.68).
Mapping higher-dimensional data to a low-dimensional space can result in information loss, but reducing sparsity can be beneficial for Machine Learning modeling due to improved predictive ability. In this study, we addressed the issue of data sparsity in electronic health records and created a model that incorporates both prevalent and rare medical conditions, leading to more accurate and effective predictive modeling. The identification of complex associations between multimorbidity and the severity of COVID-19 highlights potential areas of focus for future research, including long COVID and intervention efforts.
Peer Review reports
Multimorbidity, which refers to the presence of multiple diseases and medical conditions in one individual, is consistently linked to a lower health-related quality of life in mid-life [ 1 , 2 ]. Additionally, there is evidence suggesting that women have a higher likelihood of developing multimorbidity compared to their male counterparts [ 3 ]. Moreover, having multiple health problems at the same time has been found to make healthcare more expensive and create difficulties for healthcare systems in terms of resource allocation and providing appropriate care [ 4 ].
Moreover, multimorbidity can worsen the effects of long COVID in several ways [ 5 , 6 ], when multimorbidity is present, additional symptoms related to other chronic conditions can compound the overall symptom burden, making it more challenging for individuals with long COVID to manage and recover from their illness. Research studies have indicated that individuals with multimorbidity have been adopting various precautionary behaviors during the pandemic [ 7 , 8 ]. This is reflected in the restrictive guidelines recommended by authorities to control transmission [ 9 ]. Furthermore, studies have found that females are more likely to adopt protective measures compared to males [ 8 ]. The difference in precautionary behaviors based on gender underlines the importance of considering various demographic factors in the development of public health interventions during a pandemic.
This study specifically focuses on clustering binary data related to various medical conditions in middle-aged women. Cluster analysis is a valuable statistical technique for grouping objects based on their similarity in terms of indicator variables or features, and can be applied to identify clinically significant multimorbid groupings of medical conditions [ 10 ]. By using cluster analysis, researchers can learn important information about how different medical conditions are related and occur together. This helps them understand the complex connections between diseases and to develop personalized ways of treatment. It is also evident from the existing studies that clustering methodology can be applied to identify patient subgroups with similar disease profiles or symptom patterns [ 11 ]. Furthermore, it also can be utilized for identifying patient subgroups with distinct healthcare utilization trends and identifying risk factors associated with adverse outcomes [ 12 ]. In a multimorbidity study [ 13 ], the authors utilized K-means non-hierarchical cluster analysis to identify patterns of multimorbidity. Similarly, another study [ 14 ] focused on stratifying a population of high-risk multimorbid patients by using cluster analysis for risk stratification and identifying distinct characteristics of each cluster. These findings emphasize the significance of healthcare reform in addressing the unique needs of different patient clusters. By tailoring interventions and care strategies based on these identified clusters, healthcare providers can effectively address the diverse challenges associated with multimorbidity. Self-Organizing Feature Maps (SOFMs) have been widely employed in various clustering applications, including tasks like handwritten digit recognition [ 15 ]. In another study [ 16 ], the authors employed SOFMs to identify clusters of patients based on their healthcare data. However, SOFMs are not commonly used for clustering multimorbidity patterns, as these patterns typically involve clinical and demographic data rather than image data. Instead, other clustering approaches such as k-means, hierarchical clustering, and latent class analysis are more commonly employed for multimorbidity clustering.
However, in our study, we focus solely on clustering rare features, which are medical conditions that are not commonly observed in patient data. The methodology section explains the procedure employed in this study, detailing the process of grouping multimorbidity features into bins using a matrix based on cluster structures. This process involves two levels of clustering: the feature level and the data level, without making assumptions about the number of feature clusters. Once the features associated with each cluster are identified, they are mapped to corresponding bins. The unsupervised bins are then merged with prevalent features to create a new engineered feature matrix. The performance of models using clustered data is compared to models without clustered data, and the importance of the features is investigated, leading to the interpretation of the models.
The study focuses on a cohort of females in Piedmont, Italy. The study examines their medical history to analyze their multiple health conditions prior to the Covid-19 infection. The multiple health conditions are derived from the data of prescribed medications and diagnosed diseases. Prescribed medications are considered multimorbidity features in this study. Moreover, polypharmacy often goes hand in hand with multimorbidity, as individuals with multiple chronic conditions may require a complex medication regimen to manage their health. The severity of Covid-19 in these patients is determined based on whether they were hospitalized due to Covid-19 or not. Each patient’s information in the dataset is represented as a binary vector, indicating the presence or absence of various health conditions. Since many health conditions are very rare and present in some patients only, the data is sparse. By grouping and analyzing this sparse medical data, new composite features are created from rare features and combined with the existing common multimorbidity features including age to develop a predictive model for Covid-19 severity.
Study design and study population
This study is designed as a retrospective cohort study as it involves the retrospective analysis of data to examine the association between multimorbidity and COVID-19 outcomes over time. The historical data on prescriptions and hospital history from 2015 to 2019, which can be considered as exposure variables (multimorbidity) over a period of time. This study is evaluating the outcome of interest, which is the COVID-19 hospitalization status, during the period from February 2020 to May 2020.
Out of the 1,918,549 individuals in Piedmont, aged between 45 and 74 years, 85,348 underwent at least one swab during the observation period from February 2020 to May 2020 [ 17 ]. Of the 12,793 individuals who tested positive, 6832 females were there.
The study focused on a specific subset of the population, namely female patients aged 45-59 residing in Piedmont, Northern Italy. Inclusion criteria comprised individuals who tested positive for COVID-19 during the observation period from February 2020 to May 2020. Exclusion criteria included males, individuals outside the age range of 45-59, those residing outside Piedmont, and individuals who did not test positive for COVID-19 during the specified time frame. The corresponding exclusion and inclusion criteria resulted in 4,477 observations of female patients, and only 13.8% of them were hospitalized due to COVID-19 during the observation period.
Dataset and features
The data used in this study were collected from the Piedmont Longitudinal Study (PLS), utilizing administrative databases that involve linking anonymous records at the individual level [ 17 ]. This study investigates the multimorbidity profile of 4,477 female patients aged 45 to 59 years. We classify individuals aged 45 to 59 years as belonging to the middle-age category, aligning with prior research in this context [ 17 ].
In the dataset. there are 195 input features and 1 outcome variable where data comprises of 4,477 patient records (4477,196) where 3,861 individuals are not hospitalized and 616 individuals are hospitalized due to COVID-19. Since this is unbalanced data, the data is randomly undersampled. The under sampled dataset comprised of 1,232 patients’ records (4477,196). After resampling, zero columns are eliminated, which corresponded to records where all variables had a value of 0 in the resampled dataset. Subsequently, a comparison is conducted on all remaining fields in the resampled data with the original data to assess the similarity of proportions.
It is crucial to examine whether the resampling has been correctly accomplished as it is an important step in training this data using Machine Learning. So, the exclusion of features with significant differences is performed for retaining only those features for which there is no statistical evidence of a significant difference in proportions between the original and resampled datasets. The statistical procedure used to compare the proportion of each feature in the resampled data with that in the original data is based on a one-proportion z-test. By performing this method on each feature individually and eliminating the features in the resampled data that exhibit a significant difference in proportion compared to the original data, a new resampled dataset is created (1232,175) that is statistically similar to the original data. The resulting statistically similar dataset comprised of 1,232 patients records with 175 variables including the outcome variable.
The features used in this study are the prescriptions and diseases diagnosed for each patient in the cohort, along with the age variable. The age variable is represented as a binary variable, where 1 represents patients aged over 53 and 0 represents patients aged 53 or under. Specifically, the threshold of 53 was chosen to reflect the median within the age range, providing a meaningful criterion for distinguishing between patients above and below this central value in our analysis. All features are represented as binary variables, where 1 indicates the presence of the condition and 0 indicates the absence of the condition in the patient’s medical history from 2015 to 2019, prior to the COVID-19 pandemic. The data used in this study is labeled and belongs to the binary class of non-hospitalized and hospitalized patients (0 and 1, respectively).
Unsupervised feature binning
To group the multimorbidity features into various bins, a matrix is reconstructed based on the cluster structures. The clustering process involves two levels: feature level and data level, as shown in Fig. 1 .
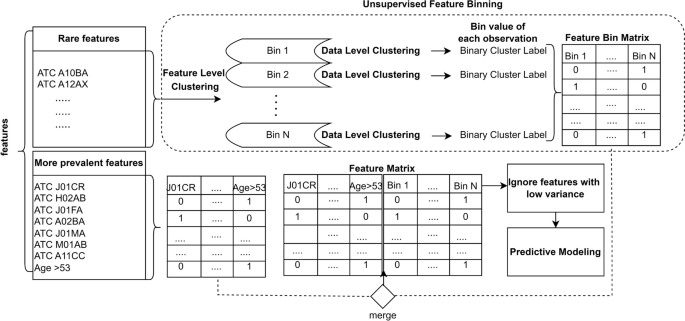
Feature level is performed to assign features into different clusters which are the Bins and data level clustering is performed where patients’ records are grouped into clusters based on the features within each Bin before predictive modeling
Binary Matrix Decomposition (BMD) offers a powerful approach for unsupervised feature reduction in binary data settings [ 18 ]. BMD seeks to factorize given binary data matrix into a reduced feature space that represent data points (U) and another reduced feature space that represent features (V). By projecting the original data points onto the reduced space determined by U, BMD achieves dimensionality reduction [ 19 ]. The latent factors in U capture underlying patterns or hidden structure in the data, providing a compressed representation suitable for various tasks like clustering. Thus, by decomposing the data into lower-dimensional binary matrices, BMD identifies latent features and reduces dimensionality while retaining essential information.
At the feature level clustering, a general model for clustering binary data that use the Binary Matrix Decomposition algorithm [ 20 ] is used to assign features into different clusters without bootstrapping on labeled train data. This method uses a Binary Matrix representation where rows represent the patients’s records, and columns represent features. BMD algorithm decompose the binary matrix to yield a probability matrix indicating the likelihood of features being part of specific clusters. Features are assigned to clusters iteratively based on whether the corresponding probability exceeds a threshold, refining the clustering model through repeated optimization until convergence, providing a systematic way to organize and interpret binary data features into meaningful clusters.
The clustering method does not make any assumptions about the number of feature clusters. After identifying the features associated with each cluster, each feature is mapped to its corresponding bin. Features that are not considered rare (i.e., present in at least 20% of the data) are not mapped to any bin and are used as they are. Only the rare features are mapped to their corresponding cluster, forming the Cluster Map.
Using the Cluster Map, the features within each cluster are represented as a Feature Bin Matrix (FBM). The training FBM consists of the features in the corresponding cluster, along with the feature values for all patients in the training dataset (without the class label). The unsupervised learning [ 21 ] is performed on the training FBM using the same BMD algorithm, iteratively for each cluster in the Cluster Map. The resulting values for each cluster are obtained. The trained model is then used to predict the cluster labels for the test FBM.
The unsupervised bins engineered from the FBMs are merged with the prevalent features (with the features excluded from the Cluster Map) to form a new engineered Feature Matrix (FM). This process is carried out separately for the training and test sets, resulting in the train FM and test FM, respectively. During the data level clustering, both datasets are handled separately without the class label to prevent data leaks.
In the data-level clustering step, we categorize patients’ records into two distinct clusters based on the features within each bin. Each patient’s record is assigned to one of the two clusters, ensuring a comprehensive grouping based on the relevant features within the given bin. For instance, consider a scenario where a cluster comprises n features in a bin. During data-level clustering, each patient’s record (row) in that bin is assigned a specific value. Consequently, this assigned value represents the contribution of that patient to the n features in that bin. After completing the data-level clustering for all patients, each bin accumulates values for every patient. Then that bin act as a new engineered feature where the data level clustering provided values for that feature. The entire procedure is illustrated in Fig. 2 .
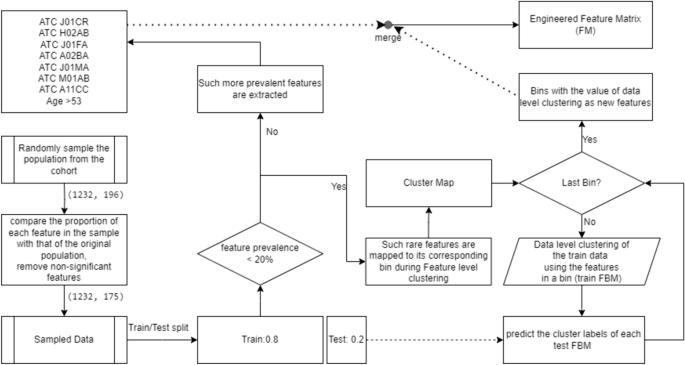
Unsupervised feature binning of rare features and generation of the Feature Matrix using new engineered features and other features: First of all data pertaining to prevalent features are sliced out. On the remaining data which contain the non-prevalent features, the clustering is applied. The process involves both feature-level clustering, where features are grouped into clusters using the BMD algorithm, and data-level clustering, where patients’ records are grouped into clusters. These tasks are interconnected as features within each cluster are used to create FBMs. Subsequently, data-level clustering is performed on these FBMs to assign patients’ records into clusters. Thus value obtained from data level clustering act as new features to replace original sparse data. The ultimate objective is to construct an engineered FM by combining these new bins with prevalent features, ensuring that both prevalent and combinations of non-prevalent features are considered for predictive modeling
- Predictive modeling
To assess the performance of different Machine Learning algorithms in predicting hospital admission due to Covid-19, we utilized the train and test FM datasets. Since the data is labeled, we employed a supervised learning approach on this engineered dataset. The trained binary classification model was then applied to the holdout data to classify patients into one of two classes: whether or not hospital admission is required, taking into account their multimorbidity history.
Following the creation of the train and test FM datasets with the newly engineered features, we analyzed the variance of each feature. We trained the train FM using various Machine Learning algorithms available in the Pycaret package [ 22 ], employing 5-fold cross-validation.
Due to the sparsity of the data and the skewed distribution of value levels (0 or 1), certain levels may dominate others, resulting in insufficient variation to generate informative features. Therefore, during the Machine Learning-based predictive modeling, such non-informative features can be disregarded. The criteria for ignoring low-variance features [ 23 ] are as follows:
The best-performing model is selected by examining the mean area under the curve (AUC) score of each Machine Learning model. Later, the best model is evaluated using the test FM and the performance scores are reported.
After applying feature-level clustering to the training data, a Cluster Map is generated. In this Cluster Map, rare features are clustered and assigned to their respective bins, resulting in 13 feature clusters. The bin values for each observation are calculated by determining the cluster label of the corresponding features in that bin. Table 1 illustrates the resulting 11 bins after excluding features with low variance.
Consequently, from an initial set of 174 input features, we have created a low-dimensional feature matrix consisting of 17 features. Even though mapping data with a higher dimension to a space of low dimension leads to some information loss [ 24 ], the predictive ability of the new data without sparsity can be an advantage for Machine Learning modeling.
Model selection
To select the best model from various Machine Learning algorithms, we compared the AUC score of each Machine Learning model after executing a 5-fold cross-validation.
Using all features
During cross-validation using the train data with all 174 features, the best performance was obtained by LR (accuracy 0.72, AUC 0.76, F1-score 0.69), CatBoost Classifier (accuracy 0.72, AUC 0.76, F1-score 0.68), and Gradient Boosting Classifier (accuracy 0.72, AUC 0.76, F1-score 0.67).
Using the features which are reduced by clustering technique and ignoring the features with low variance
During cross-validation using the train data with only 17 features, the best performance was obtained by LR (accuracy 0.7, AUC 0.74, F1-score 0.68), LDA (accuracy 0.7, AUC 0.74, F1-score 0.66) and Ada Boost Classifier (accuracy 0.7, AUC 0.73, F1-score 0.67). The 5-fold cross-validation scores of each Machine Learning model are tabulated in Table 2 .
Model performance evaluation
After analyzing the cross-validation results, the top three models are selected based on their performance. To assess the predictive ability of these Machine Learning algorithms on the reduced data without sparsity, we applied them to predict the outcome of Covid-19 hospital admission using the test Feature Matrix (FM).
The performance metrics of the selected models on the test FM (holdout data) are as follows: LR (accuracy 0.72, AUC 0.77, F1-score 0.69), LDA (accuracy 0.7, AUC 0.77, F1-score 0.67) and Ada Boost (accuracy 0.7, AUC 0.77, F1-score 0.68). For a comprehensive overview, please refer to Table 3 for the complete set of results.
Feature importance
Feature importance refers to the scores assigned to input features, which indicate their relative significance in making predictions. These scores provide insights into the importance of each feature in the data and the model. Feature importance helps not only in explaining the influential features but also in understanding the data and model better.
Feature importance score from the model coefficients
In linear algorithms such as LR and LDA, the predictions are calculated as a weighted sum of the observations, with the coefficients determined by the algorithm. In this context, negative coefficients indicate that as the value of a feature increases, the severity due to Covid-19 is predicted to decrease, suggesting no hospital admission. The features with negative coefficients in both LR and LDA algorithms are bin 2, bin 3, bin 4, bin 7, bin 10, J01CR, J01FA, and Age >53. On the other hand, features with positive coefficients have a positive association with the severity outcome. A higher negative coefficient indicates a stronger negative association between the input feature and the severity outcome. For example, if the value of a cluster or feature is 1, it suggests that most patients belonging to that cluster or feature category have a lower chance of severe Covid-19 outcomes, and vice versa. Conversely, in the case of a positive coefficient, if the cluster or feature value is 1, it indicates an increased likelihood of severe Covid-19 outcomes, and vice versa.
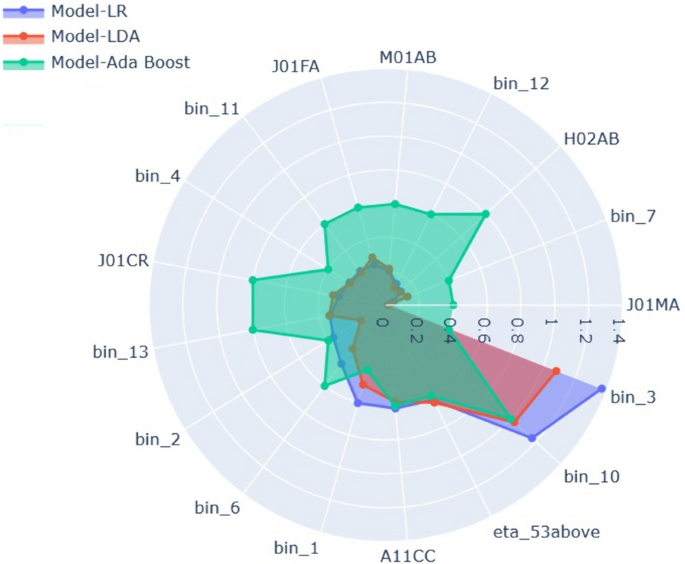
Feature importance scores from LR, LDA, and Ada Boost Models
The impurity-based feature importance
In the Ada Boost algorithm, the feature scores are determined using the Gini importance [ 25 ]. This score is calculated for each decision tree based on how much a single feature split improves the model’s performance, and it is normalized by the number of observations accounted for by that feature.
To analyze the feature importance of all three models (LR, LDA, and Ada Boost), we aggregated them and visualized the results in Fig. 3 . In the case of linear models (LR and LDA), the feature importance is represented by the absolute values of the coefficients. For the Ada Boost Classifier, the feature importance values are scaled and presented in the visualization.
Interpretation of the model
We used SHAP (SHapley Additive exPlanations) [ 26 ] to interpret the most impactful features that our models utilize in determining the status of the hospitalization. The SHAP heatmap for the linear models depicted in Fig. 4 is based on the 20% test samples (X-axis). The sorted global feature importance is represented by the Y-axis and the bar plot (right-hand side). The magnitude of SHAP values of each observation (each patient) is represented by colors. The blue color for a feature denotes, in that patient profile, that particular feature has a value of 0 and this feature contributed to or impacted the prediction of the severity either positively or negatively. The topmost graph, f(x) represents the model predictions of each patient’s multimorbidity profile.
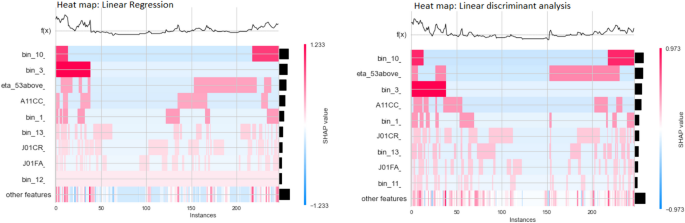
Heatmap matrix and global importance of features
In the LR heatmap of SHAP values, while examining the f(x), the 0th patient observation number possesses a higher prediction. So, it is predicted that the patient is admitted to the hospital, and the features in cluster “bin 10” contribute more positively to the Covid-19 severity of that particular patient. Similarly, we can interpret the results of other patients for all the features using this visualization.
According to literature, women appear to be relatively less susceptible to SARS-CoV-2 infection than men [ 27 ]. But epidemiological data reveals no visible sex-based discrepancy in disease severity, suggesting that the progression of the virus is comparably favorable in both women and men, and there is a similarity in the age at which the rate of SARS-CoV-2 infection peaks for both male and female [ 28 , 29 ]. However, the specific comorbidities that increase the risk of severe COVID-19 outcomes can differ significantly between men and women [ 30 ]. This underscores the need for a refined understanding of gender-specific factors influencing susceptibility and outcomes in the context of the COVID-19 pandemic. While existing literature provides valuable insights, there is a distinct lack of in-depth investigation specifically focusing on women [ 28 ]. To comprehensively address this gap in knowledge, it is imperative to advocate for targeted research works dedicated to understanding the unique aspects of women’s vulnerability or protection against COVID-19.
In our study, we utilized a combination of clustering and Machine Learning approaches to assess the severity of COVID-19 in women in midlife. Clustering less prevalent features into various Bins enhanced the interpretability of our data. By strategically grouping less common features into Bins and integrating them with prevalent ones, we aimed to capture a comprehensive picture of multimorbidity among women in midlife. Constructing clusters of multimorbidity and interpreting the outcomes at the patient level also allows us to identify, for future patients, which cluster value of a Bin contribute to whether a group of patients will be hospitalized or not due to COVID-19. Furthermore, in this study, identifying the most predictive feature or a Bin that includes less prevalent features helps in revealing the underlying combination of multimorbidity that predicts the severity of COVID-19 among women in midlife.
Examining Fig. 3 for the top-performing Machine Learning models in this study reveals that the age variable (age > 53) and the feature as a Anatomical Therapeutic Chemical (ATC) code, ATC A11C (Vitamin D and analogues) play crucial roles as predictors for COVID-19 hospitalization outcomes in middle-aged women. Existing literature provides support for the notion that individuals with a vitamin D deficiency are more susceptible to developing severe or critical cases of COVID-19 compared to those with sufficient vitamin D levels [ 31 ]. The other important features are the engineered features Bin 10 and Bin 3.
We found that Bin 10, which includes only one ATC code, ATC J01DD, consistently stands out as important across all major Machine Learning models. A retrospective drug-utilization study in Campania, southwestern Italy, found a higher prevalence of this third-generation cephalosporins (ATC J01DD) in COVID-19 positive adults under 40 and above 80, compared to the general population [ 32 ]. Additionally, a Hungarian study on hospital antibiotic consumption revealed a noteworthy 63.7% increase in the utilization of ATC J01DD from 2010 to 2019, with a substantial 70.46% surge during the pandemic years from 2019 to 2020 [ 33 ].
In Bin 3, under International Classification of Diseases, Ninth Revision (ICD-9), code ICD 574 corresponds to Cholelithiasis, a condition characterized by gallstone formation in the gallbladder. Research indicates a significant link between obesity and symptomatic gallstones, suggesting that even moderate overweight increases the risk in middle-aged women [ 34 ]. Individuals with gallstones often exhibit impaired gallbladder motility, potentially associated with additional gastrointestinal abnormalities [ 35 ]. Bin 3 also includes ATC A03FA (Propulsives) for stimulating gastrointestinal motility, ATC A05AA for medications related to bile acids and derivatives for managing certain liver diseases, ATC B03BA (Vitamin B12), ATC A12BA (Potassium), and ATC A12AA (Calcium). Calcium supplements for preventing mineral and bone disorders in chronic kidney disease (CKD) have been both praised and criticized [ 36 ].
Acute cholecystitis (AC) is a prevalent gastrointestinal ailment. The primary cause of AC is gallstone-related, but it may also be linked to diabetes, immunosuppression, CKD, and viral illnesses [ 37 ]. CKD significantly increases the risk of experiencing severe complications from COVID-19 [ 38 ]. A UK Biobank Community Cohort study revealed that in women both CKD and asthma posed a substantial risk for COVID-19 hospitalization, whereas in men, these conditions did not carry a similarly significant risk [ 30 ]. Additionally, females with asthma had a higher adjusted risk of hospitalization and death from COVID-19 compared to males with asthma, even after considering other factors [ 39 ]. Bin 3 also includes ATC D05AX (Corticosteroids in combination with vitamin D analogues for psoriasis treatment). Psoriasis may be associated with an increased risk of asthma, and childhood asthma is linked to a significantly higher risk of psoriasis [ 40 ].
Other features in Bin 3 include ICD 727 (Other disorders of synovium, tendon, and bursa) and ICD 338 (Pain, not elsewhere classified), encompassing chronic postoperative pain. A study on pain management during COVID-19 indicates that pain prevalence is 1.5-2 times more common in women than in men, with a higher ratio for specific conditions like fibromyalgia, which predominantly affects middle-aged women [ 41 ].
Research on the COVID-19 pandemic and cholecystitis suggests that the pandemic influenced healthcare-seeking behaviors for individuals with less severe health conditions [ 42 ]. A population-based cross-sectional study found that healthcare avoidance during the pandemic exhibited a robust correlation with being female, perceiving one’s health as fragile, and experiencing elevated levels of depression and anxiety [ 43 ].
Other medications in Bin 3 include Analgesics, Antiepileptics, and those related to the Cardiovascular system (C03, C07, C09, C10). In a study analyzing prescription data from June 2016 to March 2021, women exhibited a greater prevalence of antiseizure medication prescriptions compared to men, totaling around 1.3 million prescriptions [ 44 ]. Additionally, ATC C10AB, referring to fibrates, a type of medication used to lower cholesterol and triglycerides, may contribute to mitigating the inflammatory and thrombotic outcomes associated with SARSCoV-2 infection [ 45 ].
Strengths and limitations
Training Machine Learning models in a reduced feature space would be beneficial, can be supported by our current results. Our intention in applying unsupervised methodology for feature reduction was rooted in the belief that a simplified feature space could lead to more interpretable models and potentially improved generalization performance. However, we recognize that the results obtained for Machine Learning models, which demonstrated superior performance with the complete set of features, appear slightly lower when trained on the reduced feature set.
Despite a slightly lower AUC, achieving competitive predictive performance with less number of features raises the question of the computational cost-effectiveness of using a reduced feature set. If computational resources are a critical consideration, our findings suggest that the reduced feature set could offer a pragmatic solution, providing a reasonable trade-off between predictive accuracy and computational requirements.
In the context of model interpretation, it is noteworthy to mention that, while writing this manuscript, SHAP support for AdaBoostClassifier is in the process of being integrated into the official SHAP library. A relevant pull request is under review on the SHAP GitHub repository [ 46 ]. We are closely monitoring the progress, and once integrated, we plan to incorporate SHAP plots for AdaBoost into our future analyses.
The process of the unsupervised binning of the rare features can be divided into three phases: (1) extracting more prevalent features, (2) feature level clustering of the rare features to create bins, and (3) data level clustering of the features in a bin. The dimensionally reduced data with newly engineered features are used for the predictive modeling. The removal of data sparsity by this unsupervised binning of the rare features offered a low dimensional feature matrix for the predictive modeling. We have compared the predictive ability of the new sparsity-free feature matrix and the original sparse data and found that with a very low number of features itself, the model achieves nearly equal prediction performance. We have also checked the predictive utility of the new feature matrix by interpreting the feature importance and impact of the new features in the Machine Learning model.
The use of the method to address data sparsity in medical data and improve the understanding of the factors associated with the impact of infectious diseases on health outcomes in a population with multimorbidity is significant. By clustering sparse medical data and creating new features, the method could provide a more detailed understanding of multimorbidity patterns and the associations between different diseases. Improving the understanding of the factors associated with the severity of COVID-19 in this population could have important implications for public health policies, as middle-aged women with multimorbidity may be particularly vulnerable to the disease. The method has the potential to lead to better healthcare outcomes and inform public health policies related to COVID-19.
In our future works, we aim to enhance our work by integrating patient stratification based on their healthcare requirements, which entails the clustering of patient data to identify groups with similar healthcare utilization patterns. This approach will aid in identifying patient subgroups with distinct clinical profiles, which can help in designing targeted interventions and personalized care. Also, more research is needed to understand the direct impact of COVID-19 on midlife women’s help-seeking behaviors related to menopause specifically [ 47 ]. As a future work we are also planning to use Machine Learning to identify patterns in healthcare seeking behaviors before and after COVID-19 diagnosis in midlife women.
Availability of data and materials
The datasets are not publicly available. The requirement for informed consent is deemed unnecessary according to national regulation “Presidential Decree N. 20 of the Official Gazette of the Italian Republic N. 122 of May 26, 2022, “National Statistical Program” [ 48 ]. TLS\P (Turin\Piedmont Longitudinal Study) is a specific project of the Italian National Statistical Program (PSN) that is proposed by the National Statistical System (SISTAN), a network of public and private entities that provides the country and international bodies with official statistical information1, and is yearly approved by law by the Italian Parliament. In particular, since 2003 a specific form (PIE-00001 “Monitoring of socio-economic differences in mortality and morbidity through longitudinal studies”) is included in the PSN currently in effect for the three-year period 2020-2022 [ 49 ] and recently renewed for the period 2023-2025. Due to the restrictions imposed by ethical committees, the raw data cannot be made publicly or freely available to ensure the privacy and protection of individual-level data. However, researchers can request access to aggregated data by contacting the corresponding author through a reasonable inquiry.
Harrison C, Fortin M, van den Akker M, Mair F, Calderon-Larranaga A, Boland F, et al. Comorbidity versus multimorbidity: why it matters. J Multimorb Comorb. 2021;11:2633556521993993. https://doi.org/10.1177/2633556521993993 .
Kanesarajah J, Waller M, Whitty JA, Mishra GD. Multimorbidity and quality of life at mid-life: a systematic review of general population studies. Maturitas. 2018;109:53–62.
Article PubMed Google Scholar
Alimohammadian M, Majidi A, Yaseri M, Ahmadi B, Islami F, Derakhshan M, et al. Multimorbidity as an important issue among women: results of a gender difference investigation in a large population-based cross-sectional study in West Asia. BMJ Open. 2017;7(5):e013548.
Article PubMed PubMed Central Google Scholar
McPhail SM. Multimorbidity in chronic disease: impact on health care resources and costs. Risk Manag Healthc Policy. 2016;9:143–56. https://doi.org/10.2147/RMHP.S97248 .
Turner S, Khan MA, Putrino D, Woodcock A, Kell DB, Pretorius E. Long COVID: pathophysiological factors and abnormalities of coagulation. Trends Endocrinol Metab. 2023;34(6):321–44.
Article CAS PubMed PubMed Central Google Scholar
Russell CD, Lone NI, Baillie JK. Comorbidities, multimorbidity and COVID-19. Nat Med. 2023;29(2):334–43.
Article CAS PubMed Google Scholar
Jose R, Narendran M, Bindu A, Beevi N, Manju L, Benny P. Public perception and preparedness for the pandemic COVID 19: a health belief model approach. Clin Epidemiol Glob Health. 2021;9:41–6.
Delerue Matos A, Fonseca de Paiva A, Cunha C, Voss G. Precautionary behaviours of individuals with multimorbidity during the COVID-19 pandemic. Eur J Ageing. 2022;19(4):827–35. https://doi.org/10.1007/s10433-021-00632-8 .
Benny D, Castro S, Mujahid O, Abrosimova OL. Contact tracing for COVID-19 in Ukraine: insights from a case study in the region of Chernivtsi. Reg Acad U N. 2021;8(1):1–43. https://hdl.handle.net/2318/1887191 .
Cornell JE, Pugh JA, Williams JW Jr, Kazis L, Lee AF, Parchman ML, et al. Multimorbidity clusters: clustering binary data from multimorbidity clusters: clustering binary data from a large administrative medical database. Appl Multivar Res. 2008;12(3):163–82.
Article Google Scholar
Ghebre MA, Bafadhel M, Desai D, Cohen SE, Newbold P, Rapley L, et al. Biological clustering supports both “Dutch’’ and “British’’ hypotheses of asthma and chronic obstructive pulmonary disease. J Allergy Clin Immunol. 2015;135(1):63–72.
Ng SHX, Rahman N, Ang IYH, Sridharan S, Ramachandran S, Wang DD, et al. Characterization of high healthcare utilizer groups using administrative data from an electronic medical record database. BMC Health Serv Res. 2019;19(1):1–14.
Violán C, Roso-Llorach A, Foguet-Boreu Q, Guisado-Clavero M, Pons-Vigués M, Pujol-Ribera E, et al. Multimorbidity patterns with K-means nonhierarchical cluster analysis. BMC Fam Pract. 2018;19:1–11.
Bretos-Azcona PE, Sánchez-Iriso E, Cabasés Hita JM. Tailoring integrated care services for high-risk patients with multiple chronic conditions: a risk stratification approach using cluster analysis. BMC Health Serv Res. 2020;20:1–9.
Benny D, Soumya KR, Rao KN. New Dynamic Self-organizing Feature maps for the classification of Extracted Feature vectors of characters. In: 2015 International Conference on Robotics, Automation, Control and Embedded Systems (RACE). IEEE; 2015. p. 1–3. https://doi.org/10.1109/RACE.2015.7097273 .
Khalilia MA, Popescu M, Keller J, Patient stratification based on activity of daily living score using relational self-organizing maps. In: 2014 IEEE Symposium on Computational Intelligence in Healthcare and e-health (CICARE). IEEE; 2014. p. 112–6.
Catalano A, Dansero L, Gilcrease W, Macciotta A, Saugo C, Manfredi L, et al. Multimorbidity and SARS-CoV-2-Related Outcomes: Analysis of a Cohort of Italian Patients. JMIR Public Health Surveill. 2023;9(1):e41404.
Zhang Z, Li T, Ding C, Zhang X. Binary matrix factorization with applications. In: Seventh IEEE international conference on data mining (ICDM 2007). IEEE; 2007. p. 391–400.
Belohlavek R, Vychodil V. Discovery of optimal factors in binary data via a novel method of matrix decomposition. J Comput Syst Sci. 2010;76(1):3–20.
Li T. A general model for clustering binary data. In: Proceedings of the eleventh ACM SIGKDD international conference on Knowledge discovery in data mining (KDD '05). New York: Association for Computing Machinery; 2005. p. 188–197. https://doi.org/10.1145/1081870.1081894 .
Benny D, Soumya KR. New local adaptive thresholding and dynamic self-organizing feature map techniques for handwritten character recognizer. In: 2015 International Conference on Circuits, Power and Computing Technologies [ICCPCT-2015]. IEEE; 2015. p. 1–4. https://doi.org/10.1109/ICCPCT.2015.7159293 .
Ali M. (2022, April). PyCaret: An open source, low-code machine learning library in Python [PyCaret version 2.3.10]. https://pycaret.org/ .
Pycaret. Feature Selection - Docs. Pycaret. https://pycaret.gitbook.io/docs/get-started/preprocessing/feature-selection . Accessed 30 Jan 2023.
Jia W, Sun M, Lian J, Hou S. Feature dimensionality reduction: a review. Complex Intell Syst. 2022;8(3):2663–93.
Patil S, Patil A, Phalle VM. Life prediction of bearing by using adaboost regressor. In: Proceedings of International Conference on Tribology [TRIBOINDIA-2018], Mumbai, India. https://doi.org/10.2139/ssrn.3398399 . Available at: SSRN 3398399.
Lundberg SM, Lee SI. A unified approach to interpreting model predictions. Adv Neural Inf Process Syst. 2017;30. p. 4765–74. https://papers.nips.cc/paper/7062-a-unified-approach-to-interpreting-model-predictions.pdf .
Pivonello R, Auriemma RS, Pivonello C, Isidori AM, Corona G, Colao A, et al. Sex disparities in COVID-19 severity and outcome: are men weaker or women stronger? Neuroendocrinology. 2021;111(11):1066–85.
Stillwell RC. Exclusion of women from COVID-19 studies harms women’s health and slows our response to pandemics. Biol Sex Differ. 2022;13(1):27.
Griffith DM, Sharma G, Holliday CS, Enyia OK, Valliere M, Semlow AR, et al. Men and COVID-19: a biopsychosocial approach to understanding sex differences in mortality and recommendations for practice and policy interventions. Prev Chronic Dis. 2020;17:E63.
Atkins JL, Masoli JA, Delgado J, Pilling LC, Kuo CL, Kuchel GA, et al. Preexisting comorbidities predicting COVID-19 and mortality in the UK biobank community cohort. J Gerontol Ser A. 2020;75(11):2224–30.
Article CAS Google Scholar
Dror AA, Morozov N, Daoud A, Namir Y, Yakir O, Shachar Y, et al. Pre-infection 25-hydroxyvitamin D3 levels and association with severity of COVID-19 illness. PLoS ONE. 2022;17(2):e0263069.
Orlando V, Coscioni E, Guarino I, Mucherino S, Perrella A, Trama U, et al. Drug-utilisation profiles and COVID-19. Sci Rep. 2021;11(1):8913.
Ruzsa R, Benkő R, Hambalek H, Papfalvi E, Csupor D, Nacsa R, et al. Hospital Antibiotic Consumption before and during the COVID-19 Pandemic in Hungary. Antibiotics. 2024;13(1):102.
Maclure KM, Hayes K, Colditz GA, Stampfer MJ, Speizer FE, Willett WC. Weight, diet, and the risk of symptomatic gallstones in middle-aged women. N Engl J Med. 1989;321(9):563–9.
Portincasa P, Di Ciaula A, Palmieri V, Velardi A, vanBerge Henegouwen G, Palasciano G. Impaired gallbladder and gastric motility and pathological gastro-oesophageal reflux in gallstone patients. Eur J Clin Investig. 1997;27(8):653–61.
Evenepoel P, Viaene L, Meijers B. Calcium balance in chronic kidney disease: walking the tightrope. Kidney Int. 2012;81(11):1057–9.
Derebey M, Yüksek Ma, Karabacak U, Mutlu V, Mizan SR, Tarim IA, et al. Effects of COVID-19 pandemic on management of acute cholecystitis: a single tertiary center’s experience. J Exp Clin Med. 2022;39(1):164–8.
Hilbrands Luuk B, Jager Kitty J, Noordzij Marlies Vart Priya Gansevoort Ron T, EWGDRFCFHMH. Chronic kidney disease is a key risk factor for severe COVID-19: a call to action by the ERA-EDTA. Nephrol Dial Transplant. 2021;36(1):87–94. http://orcid.org/0000/0002-4935-9765
Dolby T, Nafilyan V, Morgan A, Kallis C, Sheikh A, Quint JK. Relationship between asthma and severe COVID-19: a national cohort study. Thorax. 2023;78(2):120–7. https://doi.org/10.1136/thoraxjnl-2021-218629 .
Egeberg A, Khalid U, Gislason GH, Mallbris L, Skov L, Hansen PR. Risk of psoriasis in patients with childhood asthma: a Danish nationwide cohort study. Br J Dermatol. 2015;173(1):159–64.
El-Tallawy SN, Nalamasu R, Pergolizzi JV, Gharibo C. Pain management during the COVID-19 pandemic. Pain Ther. 2020;9:453–66.
Vallès KF, Neufeld MY, Caron E, Sanchez SE, Brahmbhatt TS. COVID-19 pandemic and the cholecystitis experience at a major urban safety-net hospital. J Surg Res. 2021;264:117–23.
Steenblock C, Schwarz PE, Perakakis N, Brajshori N, Beqiri P, Bornstein SR. The interface of COVID-19, diabetes, and depression. Discov Ment Health. 2022;2(1):5.
Lavu A, Janzen D, Aboulatta L, Peymani P, Haidar L, Desrochers B, et al. Prescription trends of antiseizure medications before and during the COVID-19 pandemic. Front Neurol. 2023;14:1135962.
Alkhayyat SS, Al-Kuraishy HM, Al-Gareeb AI, El-Bouseary MM, AboKamer AM, Batiha GES, et al. Fenofibrate for COVID-19 and related complications as an approach to improve treatment outcomes: the missed key for Holy Grail. Inflamm Res. 2022;71(10–11):1159–67.
SHAP GitHub Repository. https://github.com/shap/shap/pull/3319 . Accessed 13 Feb 2024.
Lau BHP, Tang CSK, Holroyd E, Wong WCW. Challenges and Implications for Menopausal Health and Help-Seeking Behaviors in Midlife Women From the United States and China in Light of the COVID-19 Pandemic: Web-Based Panel Surveys. JMIR Public Health Surveill. 2024;10:e46538.
Mint S, Institute P. The Official Gazette of the Italian Republic: Approval of the National Statistical Program 2020-2022. Decree of the President of the Republic 9 March 2022. 2022. https://www.gazzettaufficiale.it/eli/gu/2022/05/26/122/so/20/sg/pdf . Accessed 27 May 2023.
Established Legislative Decree no. 322/1989 concerning National Statistical System organization the Sistan includes: the National Institute of Statistics (ISTAT); public bodies and statistical information bodies (INEA, ISFOL); the statistical offices of the State administrations and other public bodies, of the Government Offices of the Government, of the Regions and Autonomous Provinces, of the Provinces, of the Chambers of Commerce (CCIAA), of the Municipalities, single or associated, and the statistics offices of other public and private institutions that perform public interest functions. https://www.sistan.it/index.php?id=422 . Accessed 6 June 2023.
Download references
Acknowledgements
We would like to extend our heartfelt gratitude to all individuals in the Regional Public Health Observatory (SEPI), Grugliasco, the university-managed structure of the ASL TO3, who dedicated their support and assistance throughout this project. Their efforts in conducting epidemiological studies, research, and health promotion for the Region, health authorities, and local authorities of Piedmont have been instrumental. In particular, we would like to express our special appreciation to Alberto Catalano and Nicolas Zengarini for their unwavering support.
The authors declare that they did not receive any funds or grants for conducting this research or writing this manuscript.
Author information
Authors and affiliations.
Centre for Biostatistics, Epidemiology, and Public Health, Department of Clinical and Biological Sciences, University of Turin, Orbassano, Turin, 10043, Piedmont, Italy
Dayana Benny, Giuseppe Costa & Fulvio Ricceri
Modeling and Data Science, Department of Mathematics, University of Turin, Via Carlo Alberto 10, Turin, 10123, Piedmont, Italy
Dayana Benny
Data Analysis and Modeling Unit, Department of Veterinary Sciences, University of Turin, Turin, Italy
Mario Giacobini
Unit of Epidemiology, Regional Health Service, Local Health Unit Torino 3, Grugliasco, Turin, Italy
Giuseppe Costa & Roberto Gnavi
You can also search for this author in PubMed Google Scholar
Contributions
All authors were involved in the project conceptualization. D.B. formulated the methods, analyzed the data, and wrote the first draft which was subsequently revised by all authors in a significant manner. The submitted version has received approval from all authors. D.B. and F.R. were responsible for data curation and statistical analysis. M.G. assisted with the Machine Learning research. G.C. supervised the research work with F.R. and M.G. Additionally, F.R., M.G., G.C., and R.G. contributed to formulating the research question, interpreting results as well as reviewing and editing the manuscript.
Corresponding author
Correspondence to Dayana Benny .
Ethics declarations
Ethics approval and consent to participate.
For this study, there is no need to obtain ethical approval or permits from the ethics committee. This research is part of the TLS\P (Turin\Piedmont Longitudinal Study), which is included in the National Statistical Program (Programma Statistico Nazionale, PSN), an initiative promoted by the National Institute of Statistics (Istituto Nazionale di Statistica, ISTAT) in Italy. It establishes guidelines for the production, collection, processing, and dissemination of official statistics in the country. For the authors to access the data of the PLS within the institution that is in charge of PLS, there is no need for informed consent according to the Presidential Decree published on the N. 20 of the Official Gazette of the Italian Republic N. 122 of May 26, 2022, “National Statistical Program”, that has approved the PSN [ 48 ]. So this study does not require informed consent from an ethical committee. All analyses were conducted in accordance with the World Medical Association’s Declaration of Helsinki. To safeguard privacy, the data used for analysis was de-identified.
Consent for publication
Not applicable.
Competing interests
The authors declare no competing interests.
Additional information
Publisher’s note.
Springer Nature remains neutral with regard to jurisdictional claims in published maps and institutional affiliations.
Rights and permissions
Open Access This article is licensed under a Creative Commons Attribution 4.0 International License, which permits use, sharing, adaptation, distribution and reproduction in any medium or format, as long as you give appropriate credit to the original author(s) and the source, provide a link to the Creative Commons licence, and indicate if changes were made. The images or other third party material in this article are included in the article's Creative Commons licence, unless indicated otherwise in a credit line to the material. If material is not included in the article's Creative Commons licence and your intended use is not permitted by statutory regulation or exceeds the permitted use, you will need to obtain permission directly from the copyright holder. To view a copy of this licence, visit http://creativecommons.org/licenses/by/4.0/ . The Creative Commons Public Domain Dedication waiver ( http://creativecommons.org/publicdomain/zero/1.0/ ) applies to the data made available in this article, unless otherwise stated in a credit line to the data.
Reprints and permissions
About this article
Cite this article.
Benny, D., Giacobini, M., Costa, G. et al. Multimorbidity in middle-aged women and COVID-19: binary data clustering for unsupervised binning of rare multimorbidity features and predictive modeling. BMC Med Res Methodol 24 , 95 (2024). https://doi.org/10.1186/s12874-024-02200-x
Download citation
Received : 27 May 2023
Accepted : 07 March 2024
Published : 24 April 2024
DOI : https://doi.org/10.1186/s12874-024-02200-x
Share this article
Anyone you share the following link with will be able to read this content:
Sorry, a shareable link is not currently available for this article.
Provided by the Springer Nature SharedIt content-sharing initiative
- Sparse binary data
- Binary classification
- Multimorbidity
- Machine learning
- Public health
- Unsupervised binning
BMC Medical Research Methodology
ISSN: 1471-2288
- General enquiries: [email protected]
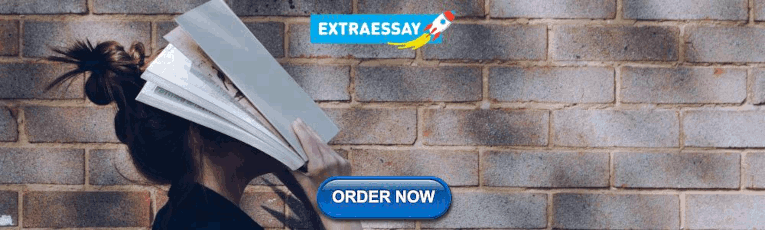
IMAGES
VIDEO
COMMENTS
PubMed Central ® (PMC) is a free full-text archive of biomedical and life sciences journal literature at the U.S. National Institutes of Health's National Library of Medicine (NIH/NLM) About PMC Discover a digital archive of scholarly articles, spanning centuries of scientific research. User Guide ...
PubMed is a comprehensive database of biomedical literature from various sources, including MEDLINE, life science journals, and online books. You can search for citations, access full text content, and explore topics related to health, medicine, and biology. PubMed also provides advanced search options and tools for researchers and clinicians.
The New England Journal of Medicine (NEJM) is a weekly general medical journal that publishes new medical research and review articles, and editorial opinion on a wide variety of topics of ...
Journal of the Endocrine Society, June 10, 2022. Effictiveness of Pfizer COVID-19 Vaccine Among Ages 5-17. Morbidity & Mortality Weekly Report, June 1, 2022. Breast Milk as Route of Tick-Borne ...
PubMed Central® (PMC) is a free full-text archive of biomedical and life sciences journal literature at the U.S. National Institutes of Health's National Library of Medicine (NIH/NLM). Get Started. PMC Overview ... 2881 Full Participation Journals. 292 NIH Portfolio Journals. 0 Selective Deposit Journals. Public Access. Funders and PMC; How ...
The results set for your search will get smaller, but all the remaining articles are easily available in free full text. Just click on the article title to get to the article page, then look for the full text link on the right.That full text link will bring you to the article's full text, maybe in PubMed Central or maybe somewhere else.
Free Articles via PubMed. Search citations to biomedical and life sciences articles dating from the 1950s to the present at PubMed. Free full-text articles are available for selected PubMed citations. See a description of PubMed icons that indicate free text is available. See these instructions to restrict your PubMed search results to free ...
PubMed Overview. PubMed is a free resource supporting the search and retrieval of biomedical and life sciences literature with the aim of improving health-both globally and personally. The PubMed database contains more than 37 million citations and abstracts of biomedical literature. It does not include full text journal articles; however ...
3.3 million articles on ScienceDirect are open access. Articles published open access are peer-reviewed and made freely available for everyone to read, download and reuse in line with the user license displayed on the article. ScienceDirect is the world's leading source for scientific, technical, and medical research.
Online publisher of free peer-reviewed scientific articles in all areas of medical research and biology. ... searchable links to free, full text, quality controlled scientific and scholarly journals. Free Medical Journals. List of free medical journals arranged by subject area and searchable by keywords. List maintained by the Geneva Foundation ...
There are a variety of ways to find full text journal articles through the Medical Library. This page provides information about two options to find full text: Finding full text journal articles through OneSearch; Finding full text journal articles using the link resolver (Find Full Text @ BU)
MEDLINE Overview. MEDLINE is the National Library of Medicine's (NLM) premier bibliographic database that contains more than 31 million references to journal articles in life sciences with a concentration on biomedicine. MEDLINE is a primary component of PubMed, a literature database developed and maintained by the NLM National Center for ...
An open database of 50,001,907 free scholarly articles. We harvest Open Access content from over 50,000 publishers and repositories, and make it easy to find, track, and use. Get the extension "Unpaywall is transforming Open Science" —Nature feature ... Research. Products & integrations
Directory of Open Access Journals (DOAJ) is a directory of over 9,000 high quality, open access, peer-reviewed journals providing over 3 million free full-text articles. F1000 Research F1000Research is a pay-to-publish collection of articles and other research outputs with no editorial input or bias.
Some local libraries have copies of medical journals or can get a copy of an article for you. Ask your local librarian about inter-library loan options and fees. ... To find citations with links to free full text articles, apply the "Free full text" filter to your search results. ... PubMed includes citations to original research articles ...
JAMA Diagnostic Test Interpretation. online firsthas active quiz. Read More. View. Explore the latest in medicine including the JNC8 blood pressure guideline, sepsis and ARDS definitions, autism science, cancer screening guidelines, and.
About the directory. DOAJ is a unique and extensive index of diverse open access journals from around the world, driven by a growing community, and is committed to ensuring quality content is freely available online for everyone. DOAJ is committed to keeping its services free of charge, including being indexed, and its data freely available.
MEDLINE is the National Library of Medicine's (NLM) premier bibliographic database that contains references to journal articles in life sciences, with a concentration on biomedicine. See the MEDLINE Overview page for more information about MEDLINE.. MEDLINE content is searchable via PubMed and constitutes the primary component of PubMed, a literature database developed and maintained by the ...
PubMed is a free resource that is developed and maintained by the National Center for Biotechnology Information (), at the U.S. National Library of Medicine (), located at the National Institutes of Health ().Publishers of journals can submit their citations to NCBI and then provide access to the full-text of articles at journal web sites using LinkOut.
Free full-text articles in medical journals. Direct Link. Health Sciences , Medicine.
The previous analysis of disease-free survival occurred after a median follow-up of 24.1 months (estimated percentage of participants who were alive and free from recurrence at 24 months, 77.3% in ...
2. Open Access browser extensions. More and more research is published Open Access as governments around the world are mandating that research paid for by taxpayer money be freely available to those taxpayers. Browser extensions have been created to make it easy to spot when the full text of an article is free.
Biodegradable polymers have been extensively researched in the field of biomedicine. Polylactic-co-glycolic acid (PLGA), a biodegradable polymer material, has been widely used in drug delivery systems and has shown great potential in various medical fields, including vaccines, tissue engineering such as bone regeneration and wound healing, and 3D printing. Cancer, a group of diseases with high ...
One of the important cause responsible for the relatively less number of research publication is unavailability of free full-text articles. Research works, published by most of the journals, are paid. Many of the undergraduates or postgraduate students may not be able to purchase these high-cost journals or articles. ... Free Medical Journals ...
This Medical News article discusses a KFF poll about the public's exposure to and beliefs about inaccurate health information, as well as media use and ... Research, Methods, Statistics; Resuscitation; Rheumatology; ... Register for email alerts with links to free full-text articles; Access PDFs of free articles; Manage your interests;
While medical treatment options are possible for many types of tumors today, surgery remains the primary treatment option for pituitary tumors [].The multidisciplinary evaluation of patients who will undergo pituitary tumor surgery by endocrinologists, neurosurgeons, ear-nose-throat surgeons, neuroradiologists, neuro-ophthalmologists, endocrine pathologists, and anesthesiologists is ...
The ethical governance of Artificial Intelligence (AI) in health care and public health continues to be an urgent issue for attention in policy, research, and practice [1,2,3].Beyond the growing number of AI applications being implemented in health care, capabilities of AI models such as Large Language Models (LLMs) expand the potential reach and significance of AI technologies across health ...
Traumatic brain injury (TBI) has been a worldwide problem for neurosurgeons. Patients with severe TBI may undergo craniotomy. These patients often require sedation after craniotomy. Dexmedetomidine (DEX) has been used in patients receiving anesthesia and in intensive care units. Not much is known about the postoperative effect of DEX in patients with severe TBIs undergoing craniotomy.
Multimorbidity is typically associated with deficient health-related quality of life in mid-life, and the likelihood of developing multimorbidity in women is elevated. We address the issue of data sparsity in non-prevalent features by clustering the binary data of various rare medical conditions in a cohort of middle-aged women. This study aims to enhance understanding of how multimorbidity ...